Click through the PLOS taxonomy to find articles in your field.
For more information about PLOS Subject Areas, click here .
Loading metrics
Open Access
Peer-reviewed
Research Article
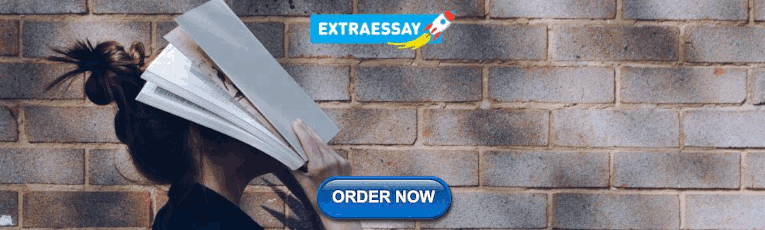
Long-term memory effects on working memory updating development
Roles Conceptualization, Data curation, Formal analysis, Investigation, Methodology, Project administration, Software, Validation, Visualization, Writing – original draft
* E-mail: [email protected]
Affiliation University of Urbino, Urbino, Italy

Roles Conceptualization, Funding acquisition, Methodology, Project administration, Resources, Supervision, Validation, Writing – review & editing
Affiliation University of Pavia, Pavia, Italy
- Caterina Artuso,
- Paola Palladino
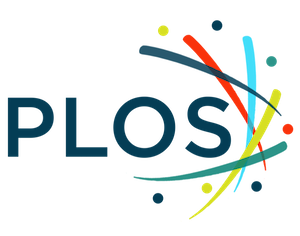
- Published: May 31, 2019
- https://doi.org/10.1371/journal.pone.0217697
- Reader Comments
Long-term memory (LTM) associations appear as important to cognition as single memory contents. Previous studies on updating development have focused on cognitive processes and components, whereas our investigation examines how contents, associated with different LTM strength (strong or weak), might be differentially updated at different ages. To this end, we manipulated association strength of information given at encoding, in order to focus on updating pre-existing LTM associations; specifically, associations for letters. In particular, we controlled for letters usage frequency at the sub-lexical level. We used a task where we dissociated inhibition online (i.e., RTs for updating and controlling inhibition from the same set) and offline (i.e., RTs for controlling inhibition from previously updated sets). Mixed-effect analyses were conducted and showed a substantial behavioural cost when strong associations had to be dismantled online (i.e., longer RTs), compared to weak ones; here, in primary school age children. Interestingly, this effect was independent of age; in fact, children from 7–8 to 9–10 years were comparably sensitive to the strength of LTM associations in updating. However, older children were more effective in offline inhibitory control.
Citation: Artuso C, Palladino P (2019) Long-term memory effects on working memory updating development. PLoS ONE 14(5): e0217697. https://doi.org/10.1371/journal.pone.0217697
Editor: Burcu Arslan, Educational Testing Service, UNITED STATES
Received: November 26, 2018; Accepted: May 16, 2019; Published: May 31, 2019
Copyright: © 2019 Artuso, Palladino. This is an open access article distributed under the terms of the Creative Commons Attribution License , which permits unrestricted use, distribution, and reproduction in any medium, provided the original author and source are credited.
Data Availability: All relevant data are within the paper and its Supporting Information files.
Funding: This work was supported by Blue Sky Research (BRS) 2017 Established Investigator awarded to PP. The funder played no role in the study design, data collection and analysis, decision to publish, or preparation of the manuscript.
Competing interests: The authors have declared that no competing interests exist.
Introduction
Working memory (WM) is a capacity limited system, able to maintain actively sets of representations useful in complex cognitive skills such as reading [ 1 , 2 ] or text comprehension [ 3 , 4 ]. WM performance improves substantially over childhood with linear increases [ 5 , 6 ]. These developmental improvements may be driven by increases in storage capacity [ 7 ], rehearsal strategies [ 8 ], or also updating processes [ 9 ].
In fact, given capacity limits and the continuous flow of information to be processed, it is important to explore a mechanism that potentially allows WM content to be updated constantly via maintenance of relevant information and inhibition of irrelevant information. Updating investigation is usually applied to memory contents [ 10 ]. However, usually, updating tasks are based on binding and unbinding processes between memory contents (e.g., [ 11 ]). Binding updating (but not content updating) is a more sensitive measure in accounting for performance in accuracy-based updating tasks [ 12 ]. In addition, the role of associative contextual bindings in episodic memory retrieval was also supported [ 13 ]. Overall, it appears that the monitoring of associative bindings between contents is a specific challenge for the updating process (see also [ 14 , 15 ]).
In the current paper, we aimed to study how updating of long-term memory (LTM) bindings (or LTM associations) develops in primary school children (in particular from third to fifth grade). First, we briefly review development of updating components and the role of LTM representations in WM tasks through childhood; in particular, lexical-semantic and sub-lexical representations. Next, we will focus on sub-lexical LTM representations and how these are updated specifically, introducing the aims of the current study.
Updating processes, components and development
Development of the WM updating function is a recent research topic that has arisen from adult studies and modelling research. In a recent developmental study, an accuracy-based updating task modelled after the one developed by [ 4 ] was administered to children [ 9 ]; here, they were able to differentiate between inhibition (i.e., ability to suppress same-lists intrusions) and proactive interference (PI) control (i.e., ability to suppress previous-lists intrusions). They showed that memory performance improves with age, together with development of inhibitory process efficiency. However, the most interesting finding here, is that these two components are relatively dissociable. The inhibition of information explained a considerable amount of variance, but of a similar percentage magnitude at ages 7, 11 and 15 years (42%, 49% and 46%, respectively); thus, its developmental contribution is less pronounced. On the other hand, the PI control component explained smaller amounts of variance across all ages, but especially at 7 years (25%), at 11 years (17%) and at 15 years (13%; [ 9 ]); thus its developmental role appeared more pronounced.
This two-component model of updating development is consistent with other models that emphasize additional features of updating and/or investigate alternative mechanisms [ 16 ]; here, the authors decomposed the updating process, individuating at least three components: retrieval (i.e., searching for a specific representation among many competing elements maintained in the region of direct access; see also [ 17 ]); transformation (i.e., modifying a representation maintained in WM); and the most distinctive component, item-removal (i.e., replacement of previously relevant content -now irrelevant- with new relevant information; [ 16 , 18 ]).
Within this theoretical framework, age-related differences through development, from 8 years to adulthood were found [ 19 ]. They found that only the retrieval component has age-related effects, with clear development from 8 years; no differences were observed for transformation or item-removal, despite their crucial role in updating.
LTM associations and WM development
The role of LTM associations in WM performance has been previously explored in order to understand how enduring properties of verbal material affects ongoing performance, mainly through simple WM tasks involving recall (e.g., [ 20 , 21 ]). The impact of informational organization in LTM on WM performance can be observed at different processing levels, e.g., lexical, sub-lexical and semantic.
In general, it has been shown that LTM associations interact with recall, facilitating the process; the more strongly items are associated in LTM, the more WM performance will benefit. That said, few studies have investigated the influence of lexical/semantic LTM representations on verbal WM performance in children, although previous research seems to suggest that effects are similar in children and adults (e.g., [ 22 , 23 , 24 ]).
Semantically-related information enhanced WM performance more than descriptive or unrelated information [ 22 ]. Similar lexico-semantic effects to adults across development were reported [ 23 ]. In an immediate serial recall task with words, they found replication of effects observed in adults, (e.g., lexicality, word frequency and imageability) from 6 to 22 years. These were accounted for by similar redintegration processes that would operate effectively on high frequency words because their phonological representations are more easily accessed by partial information. Accordingly, item frequency effects on recall are observed with the relevant item only, and occur at the time the individual item is retrieved/recalled (see also [ 20 , 21 , 25 ]).
How LTM lexical/semantic knowledge (such as lexicality and language familiarity effects) impacts on WM performance was examined by [ 24 ]. They compared children aged 5 and 9 years in tasks of immediate serial recall, finding evidence of the semantic-similarity effect in 5 year-olds. In fact, the specific organization of semantic LTM was found to enhance recall performance.
Overall, these studies have focused on WM recall tasks (i.e., entailing temporary maintenance of information in WM; [ 2 ]) and suggest that the more associated the information is, the better memory performance will be. In addition, studies suggest that developmental changes of the LTM system happens between the age of 5 and 11 years [ 24 ]; thus, interactions between LMT and WM recall are linked to developmental changes in WM capacity and efficiency [ 6 ]. In contrast, here, we focused on the interaction between LTM and updating; here, a complex WM function comprising not only temporary maintenance of information, but also inhibition and item-removal [ 9 , 16 , 18 ].
How LTM associations are updated
To the best of our knowledge, few studies have investigated the updating of LTM associations between verbal materials [ 14 , 26 ]. Indeed, updating can be distinguished from recall, as it allows memory focus to remain attuned to the most relevant information in any specific moment.
In an initial study, the strength of association between LTM stimuli was manipulated [ 26 ]; and how strength might modulate the updating process itself. Following the literature on the beneficial effects of highly-associated LTM information (e.g., [ 20 , 25 ]), Artuso and Palladino [ 26 ] investigated whether strong or weak associations were updated differently. Strength was represented by the frequency of sub-lexical associations between consonants. Association strength was manipulated at encoding, in order to observe how strong and weak associations were updated subsequently. Overall, it was shown that the stronger the LTM association, the longer latencies (i.e., to substitute information and to control for irrelevant information) were required. Therefore, a processing cost was found for updating; this is in direct opposition to recall, which is boosted by association strength [ 14 ].
In a further study, the association strength was manipulated at both encoding and updating, and added two conditions (i.e., strong associations that were updated to strong, and weak associations updated to strong), in order to gain a more complete view of accumulation and disruption of specific associations [ 14 ]. Here, the data supported the view that as pre-existing associations became stronger, they became harder to dismantle (i.e., longer RTs). In addition, when a strong association had to be recreated, this was usually enhanced (i.e., with shorter RTs from weak to strong association). The result was observed for both processing speed (inhibition process) and interference control (i.e., of a previously activated strong association). In particular, it was shown that inhibitory control requested was greater for items strongly associated, indicating, in turn, the long lasting of the pre-existing LTM association. Those experiments demonstrated clearly that associations from LTM modulate the updating process. In fact, these results suggested that, on the one hand, strong associations are dismantled and updated with greater difficulty (i.e., they require longer RTs), and on the other, that strong associations are activated more easily (i.e., requiring shorter RTs). This evidence supported the idea that the nature of updating rests in the interplay between dismantling and reconstructing bindings via different memory systems such as WM [ 11 , 24 ] and episodic LTM [ 13 ].
In the numerical domain, it was found that numerical similarity produces facilitation during updating of information. When the numbers involved in updating were near as far as concern numerical distance, or similar through sharing a digit, substitution occurred more quickly [ 27 , 28 ]. There, it was proposed that updating is a function of the overlapping features [ 29 ] between numbers to update and those stored in LTM; the greater the amount of overlap, the quicker the update will be, as both numbers share many (already activated) features. In summary, if, as well as inhibition [ 9 ], item-removal in LTM association is a distinctive updating component [ 16 ], it is important to investigate how the strength of this inter- item association retained in LTM affects WM processing (e.g., updating, [ 14 ]).
The current study
As previously described, studies on updating development have focused on processes and components [ 9 , 19 ], whereas our aim is to examine the associative effects of updating through development. In particular, given that LTM inter-item associations seem as important as single contents [ 14 ], we aimed to investigate whether associated information modulates updating performance in development.
Hence, we manipulated LTM associations for letters as they represent initial elements of learning and therefore, should be highly familiar to children, in addition to their established use in many studies on their role across cognition. In particular, we controlled for their frequency of use at the sub-lexical level. Broad evidence has shown recall accuracy is greater for words containing high frequency phoneme combinations in English (“phonotactic effect”, see [ 25 ]). Performance would likely benefit from use of stored phonotactic representations for familiar words to fill in incomplete traces prior to output. In contrast, for unfamiliar words, no stored representations are available to reconstruct partial traces, and this will lead to diminished accuracy at recall. In addition, recall is better for high phonotactic frequency of the language in WM. As fully described in [ 25 ] the “phonotactic effect” elicits better recall for ‘consonant-vowel-consonant’ non-words containing ‘consonant-vowel’ and ‘vowel-consonant’ combinations, common in the language’s native phonology, than for non-words containing low probability ‘consonant-vowel’ combinations. Such effect would reflect the influence of phonotactic knowledge about properties of that language [ 25 ].
With this aim, we administered an updating task previously used with both children [ 30 ] and adults [ 12 , 31 ], focused on active item-removal of information shown to be the most distinctive component of updating [ 14 , 16 ]; but see also [ 19 ]. In particular, this task allows collection of both online response times (RTs) during updating (i.e., dismantling of an item-set) and offline accuracy/RTs after updating of a memory set, in order to ensure updating effectiveness and inhibition of irrelevant information [ 31 ].
Therefore, we believe this task could include at least two different types of inhibition, that is online (i.e., inhibition within the same set) as well as offline (i.e., inhibition of the previously updated set of information). Thus, the specific object of our investigation is how information, associated with different strength in LTM, i.e., strongly or weakly, might be differently updated at various ages. To this end, we manipulated association strength of the information at encoding (but not updating), in order to focus on the specific function of dismantling pre-existing LTM associations rather than reconstruction of new associations. We hypothesize that, in line with adult studies (e.g., [ 23 ]), we should observe similar effects with children, as soon as LTM representations are strengthened and consolidated (i.e., with a behavioural cost for updating strongly associated information). In particular, we should observe an increase in online updating RTs when inhibiting and dismantling a strong pre-existing association (once encoded), and a decrease when dismantling a weak pre-existing association (once encoded). Accordingly, offline, we predict greater difficulty in inhibiting items from strong LTM associations, relative to weak ones).
Participants
The initial sample was of 90 children. At the end of the testing phase, we were informed from teachers that one child had received a diagnosis of learning disorder. We therefore decided to not include his data in the final sample. Thus, a sample of 89 children took part in the study. They did not present any specific learning, neurological or psychiatric disorder. Children were divided into two groups: 44 younger children (aged 7–8 years) and 45 older children (aged 9–10 years). These specific ages were chosen as they represent the most crucial steps for children to become more and more skilled in reading and writing, and access to meaning of written texts is more automatized. In addition, and in line with previous studies suggesting the relevance of the specific age range 5–11 years (e.g., [ 6 , 24 ]), we chose two central and crucial steps that are coherent with previous research and allow comparison. All children were Italian native speaker. See breakdown of participants’ characteristics in Table 1 .
- PPT PowerPoint slide
- PNG larger image
- TIFF original image
Descriptive statistics (mean, standard deviations for accuracy rate and score range) for the Italian vocabulary and nonverbal reasoning test. SDs are in brackets.
https://doi.org/10.1371/journal.pone.0217697.t001
Children came from a public school located in Northern Italy, within an urban environment and mixed socio-economic background. All children had normal or corrected-to-normal vision. The study was conducted in accordance with the Ethical Standards laid down in the 1964 Declaration of Helsinki and the standard ethical procedures recommended by the Italian Psychological Association (AIP). The study was reviewed and approved by the IRB (ethical committee) of the University of Pavia/IUSS before the study began. Written informed parental consent (as well as oral informed child assent) was obtained prior to participating, according to the ethical norms in our university.
Children were administered two tasks to assess general cognitive abilities (see following method sections for full description). Descriptive statistics for the two general cognitive abilities administered to the two age groups are displayed in Table 1 . Analyses on the accuracy scores (independent sample t-tests) showed age-related differences in the vocabulary test, t( 87) = 2.09, p = .04, with older children better scoring than younger children, but no differences in the visuospatial reasoning test, t( 88) = 1.02, p = .31.
Materials and procedures
In order to verify that children’s general cognitive performance adhered to the average for their age, they were presented with two measures: a standardized Italian vocabulary test and a nonverbal reasoning test. In particular, the vocabulary can be taken as an index of crystallized intelligence, whereas the nonverbal reasoning test is held to measure fluid intelligence.
In addition, a computerized letter updating task was administered. The vocabulary test and the nonverbal reasoning test were administered in a classroom-based group session. The updating task was administered individually at school, in a quiet room. The group session lasted on average 15 minutes, and the updating task lasted about 20–25 minutes. The two sessions were non-consecutive, in order to avoid possible fatigue effects.
Italian vocabulary and nonverbal reasoning
The vocabulary and nonverbal reasoning subtests, taken from the Primary Mental Aptitude Battery [ 32 ] were presented to the whole class group during a school day; both have a four alternative multiple-choice structure. The vocabulary subtest has 30 items and the nonverbal reasoning subtest, 25 items. Participants had time constraints for both subtests; specifically, 5 minutes for the vocabulary and 6 minutes for the nonverbal reasoning.
Letter updating task
The task we used in the current paper was described in detail previously, in [ 14 ]. As in [ 14 ] the stimuli were sub-lexical units between two consonants of the Latin alphabet. The association was based on LTM consonant representation; that is, on the basis of their combined frequency in the spoken Italian language. We evaluated this from the lexicon of frequency of Italian spoken language [ 33 ], a corpus of about 490,000 lemmas collected in four main Italian cities, emerging from different subgroups of discourse. High and low frequency lemmas were selected. Low frequency ranged from 0 to 2 (i.e., lemmas with less than 3 occurrences in the corpus). High frequency lemmas had at least 3 occurrences in the corpus.
Next, we inferred strong and weak sub-lexical associations between consonants, based on the lemmas’ frequency. That said, we should note there is no specific frequency information for consonant couples, only for lemmas of the corpus. So, for example, from the lemma “ ardere” which is low frequency, we inferred the low frequency sub-lexical association “ rd ”. In addition, low frequency associations, typically, were in the middle of the lemma, whereas high frequency lemmas were at the beginning of the lemma. In addition, we checked the corpus to find occurrences of low frequency sub-lexical associations in different lemmas, in order to preclude their presence in high frequency lemmas. We included in the low frequency sub-lexical associations those one occurring in low frequency lemmas only.
We employed the following set of consonants: B C D F G H L N P R S T. Strong associations were: T-R, S-P, P-R, N-T, B-R, C-H, G-R, F-R. Weak ones were: F-L, S-N, G-H, P-S, G-L, R-D, N-D, L-T. Strong and weak associations between consonants were controlled in order to avoid obviously familiar or meaningful couplets. Each association was legal, and thus possible at the sub-lexical level of the Italian language [ 14 ].
As described in [ 14 ] and in order to avoid ceiling (i.e., with two items) or floor effects (i.e., with four items), we used memory sets composed of three letters (i.e., triplets), which have been established as being within average memory span [ 34 ]. Some letters were overrepresented relative to others, but we controlled for this bias by randomizing these across association strengths. Further, the position of the sub-lexical unit within the triplet (i.e., in positions 1/2 or 2/3 ) was randomized between trials. We did not control for potential position effects, as it was shown in a previous experiment that position did not interact with either updating or strength (see [ 14 ], Experiment 2)
The third letter of each triplet was another consonant, which was always unrelated to the other two. Specifically, the link between the sub-lexical unit and the third letter was always linguistically impossible in Italian (e.g., see example from Fig 1 where C-H is a strong association, and the link between H and B (H-B) is impossible in Italian). This was done in order to avoid any LTM (strong or weak) or some other meaningful way association between these letters.
After encoding the first triplet ( CHB ), participants had to maintain it actively in memory (pre-updating maintenance process: + + +). Next, they were instructed to update part of the association, that is, to remove the item C and substitute the G . Thus, the triplet they were now maintaining was GHB . Lastly, they had to maintain the recently updated triplet (post-updating maintenance process). At recognition, a single red probe was displayed: here, participants had to recognize if the probed item belonged to the most recent studied/updated item or not. In the example, a target probe was presented ( B ), to which they had to give a positive answer.
https://doi.org/10.1371/journal.pone.0217697.g001
Design and analyses
A three factor mixed design was implemented: Strength and Phase were within-participants factors, and Age group between-participants. The variable Strength had two levels: strong-to-weak and weak-to-weak. ‘Strong-to-weak’ represented associations between letters where the association was strong at encoding, but modified with a weak one upon updating (e.g., from C-H to G-H). Weak-to-weak represented associations between letters occurred where the association was weak at encoding and updated with another weak association (e.g., from P-S to P-R). For each trial, we considered two main phases of encoding (i.e., studying/encoding the initial triplet), and updating (i.e., partial into the triplet). Although the trial was constituted of four phases, only encoding and updating phases (i.e., phases that produce effects on RTs, see [ 31 ]) were entered into the analysis.
In addition, to make the task less predictable and ensure participants were engaged, we included several control trials (approximately 20% of the total number). Here, no updating occurred, and maintenance alone was required throughout the trials. These data were not included in further analyses, but were checked to ensure that all updating trials had longer RTs than controls ( p < .05 for each comparison; control vs. strong-to-weak, and weak-to-weak; [ 14 ]).
Procedure was described in detail previously [ 14 , 26 ]. The task was administered on a standard PC and consisted of four phase subject-paced trials, where participants pressed the spacebar to start each trial, and after each phase, in order to proceed with the task.
In each phase, triplets were always displayed in the centre of the screen. Each trial started with an encoding phase (Phase 1; see an example with letters in Fig 1 , where a strong-to-weak association is represented), where participants had to memorize the first triplet of consonants (e.g., C-H-B). A pre-updating maintenance phase followed (Phase 2), where three pluses were displayed; this indicated that the previously encoded triplet had to be actively maintained. Then, at updating (Phase 3), participants had to substitute the no-longer-relevant information (here, C) with newly relevant information (here, G). Concurrently, they needed to maintain previously relevant detail (here, H-B), thus, updating the triplet (i.e., from C-H-B to G-H-B). Finally, a post-updating maintenance (Phase 4) ended the sequence, to control for recency biases. See also Fig 1 .
Only one letter of the triplet had to be updated; this letter could be presented in any position of the triplet (i.e., left letter, right letter, or center). Position was balanced across trials, and only new consonants were presented across each phase. When a consonant did not change, a plus symbol was presented, in order to encourage active maintenance of previously encoded/memorized information.
At the end of each trial (Phase 5), participants were presented with a probe recognition task: a single red consonant was displayed in the centre of the screen. Here, they had to indicate whether this belonged to the most-recently studied triplet or not. They responded by pressing one of two keys on the keyboard; one (M for Yes ) for target probes requiring a positive answer (i.e., belonging to the final triplet of the trial); another one (Z for No ) for probes requiring a negative answer (i.e., not previously presented in the trial. For these, we included both lures i.e., (probes encoded in the trial, then substituted at updating step) and negative probes (i.e., probes not presented in that trial), mixed within the trial. Half the probes were targets (50%); the other half was equally shared between lures (25%) and negative probes (25%).
Afterwards, each participant was presented with a practice block of eight trials to familiarize themselves with the task. One hundred and twenty trials were then presented shared equally in four blocks. We recorded subject-paced RT at each of the four phases, in addition to probe recognition accuracy at Phase 5.
Results and discussion
Updating task: accuracy and data treatment.
Participants performed accurately on an average of 92.80% of trials. As expected, participants were very good in completing the task and very few errors were produced. Accuracy was analysed to verify adequate performance, but the main focus of the analysis was on RT. We ran a mixed 2 x 3 ANOVA, with Strength (weak-to-weak, strong-to-weak) as within-participants factor and Age Group (younger children, older children) as between-participants factor on mean accuracy rates of target, lures and negative responses. A main effect of Age Group reached significance, F (1, 87) = 8.38, p = .005. Accuracy rate was significantly lower in younger children (116/120 correct trials) than in older children (118/120 correct trials). Only subject-paced RTs for trials that ended with correct probe recognition were analysed. Trials with RTs of less than 150 ms, or exceeding a participant’s mean RT for each condition by more than three intra-individual standard deviations, were considered outliers, and therefore excluded from further analyses (3.92%).
In addition, updating measures (in particular, indexes of RT at the updating step), were highly inter-correlated, suggesting good reliability of the task. In particular, RTs for weak-to-weak associations were strongly correlated, r (89) = .84, p < .001, to RTs for strong-to-weak.
Overview of the statistical analyses
We used a mixed-effects model approach to test our hypotheses; the most important advantage of such models is that they allow simultaneous consideration of all factors that may contribute to understanding the structure of the data [ 35 ]. Raw RTS were logarithmically transformed to normalize them. These factors comprise not only the standard fixed-effects factors controlled by the experimenter (in our case, age group and strength) but also random-effects factors; that is, factors whose levels are drawn at random from a population (in our case, children). To test the effect of age group (younger children, older children) and strength (strong-to-weak, weak-to-weak) on the variables of online RT, and offline RT three mixed-models were used: one for online RT (with encoding and updating phases as additional factors), another one for RT of correctly detected target probes, and a third for RT of correctly rejected lures. See specific details in the subsections below.
All analyses were performed using the R software [ 36 ]; for generalized mixed-effect models, the R package lme4 was used [ 37 ]; and the lmer test package was used to obtain Type III ANOVA Tables. Results for each dependent variable are presented below. For planned comparisons, Tukey correction was used to control the Type I error rate.
Online RT analyses
A linear mixed-effects model was constructed with 3-way interactions between Age Group (younger children, older children), Strength (strong-to-weak, weak-to-weak), and Phase (encode, update). The model revealed a significant effect of Age Group, F (1, 87) = 8.11, p = .006. Overall, older children ( M = 2709.26 ms, SD = 67 ms) were faster than younger children ( M = 2960.22 ms, SD = 66 ms). Moreover, Strength affected the online processing, F (1, 261) = 5.71, p = .01; strong-to-weak associations ( M = 2898.36 ms, SD = 65 ms) were hardly updated than weak-to-weak ones ( M = 2768.30 ms, SD = 68 ms).
In addition, the Phase by Strength interaction reached significance, F (1, 261) = 7.18, p = .008. Post-hoc comparisons showed no differences at encode across associations, t( 261) = -0.21, p = .83; in contrast, at updating, strong-to-weak associations showed longer RTs compared to weak-to-weak associations, t( 261) = 3.59, p = .004, as shown in Fig 2 . No other interaction reached significance.
Plot dots represent mean predicted RTs (ms) and bars represent 95% CIs.
https://doi.org/10.1371/journal.pone.0217697.g002
Offline RT analyses: Target probes
A linear mixed-effects model was constructed with 2-way interactions between Age Group (younger children, older children) and Strength (strong-to-weak, weak-to-weak). The model revealed a significant effect of Strength, F (1, 87.353) = 11.13, p = .001. Indeed, we found significantly longer RTs for correct recognition of a target probe from strong-to-weak associations ( M = 2058.33 ms, SD = 58 ms), compared to weak-to-weak associations ( M = 1867.85 ms, SD = 43 ms). No other effect reached significance.
Offline RT analyses: Lures
First, we conducted a control analysis with Strength (weak-to-weak, strong-to-weak), and Probe (lure, negative) as within-participant factors and Age Group (younger children, older children) as between-participant factor, for lures vs. negative probe RTs. Importantly here, we found a main effect of Probe, F (1, 87) = 8.61, p = .004, showing longer RTs to recognize and respond to lures ( M = 2395.68 ms, SD = 52 ms) than to negative probes ( M = 2208.06 ms, SD = 44 ms).
In addition, to test our hypotheses more specifically, a linear mixed-effects model was constructed with 2-way interactions between Age Group (younger children, older children) and Strength (strong-to-weak, weak-to-weak) and was run on lures only, as these represent a measure of the ability to inhibit irrelevant information once completed the updating task. The model revealed a significant effect of Age Group, F (1, 85.250) = 16.92, p < .001. In addition, we found a main effect of Strength, F (1, 87.394) = 45.75, p < .001.
The two-way Strength by Age Group interaction reached significance, F (1, 87.394) = 25.57, p < .001. Subsequently, post-hoc comparisons showed that rejection of a lure from a strong-to-weak association needed longer RTs (compared to weak-to-weak association), but only for older children, t (87.39) = 8.41, p < .001. Rejection of a lure from a strong-to-weak association did not differ from a weak-to-weak condition in younger children, t (87.39) = 1.20, p = .23, as shown in Fig 3 .
Plot dots represent mean predicted RTs (ms) at lure rejection and bars represent 95% CIs.
https://doi.org/10.1371/journal.pone.0217697.g003
We believe our task is mainly based on phonological/orthographic knowledge and less on lexico-semantic knowledge (see also [ 30 ]). In fact, in order to engage with the task rapidly and effectively, the child should have developed an automatic access to orthographic/letter form representation. Therefore, we do not predict any specific vocabulary-related effect. However, in order to control for the role of vocabulary in the process examined, we ran the same mixed-effect models, covarying for vocabulary. Overall, the results did not change, showing the same effects and significance levels for both target probes (main effect of Strength, p = .002) and lures (Age group, Strength, and two-ways interaction, all ps < .001).
Conclusions
In this study, our aim was to investigate how LTM associations affect updating development. Updating is a complex activity that involves inhibition at different levels such as from the same lists set, or from previous lists [ 9 ], with the distinguishing component of the item-removal process [ 16 , 18 ]. More specifically here, we analysed how the strength of LTM association between items affects updating from a developmental perspective.
Typically, the literature on adults shows enhanced recall for strongly associated items; the stronger the pre-existing association in LTM, the better the performance in WM. For updating, a somewhat different process is indicated (i.e. not only maintenance of information in the short term, but also removal of irrelevant information). In this case, the opposite was shown: the stronger the pre-existing association, the harder it is to dismantle it [ 26 ].
In addition, the first notable difference between updating and recall (i.e., slowing of RTs in the former) could be related to the number of cognitive operations required in the task. Indeed, recall involves maintenance of information only; whereas updating entails a further item-removal component. Therefore, it is reasonable to assume that an additional operation (i.e., item-removal) will add a cost in terms of longer processing latencies. However, results comparing updating performance compared to recall have demonstrated the reverse effect; that is a cost rather than a benefit. This difference is likely to be due to the nature of updating, an essential process in adaptation of WM content to new elements. In other words, updating involves integration of new elements, as well as new bindings between elements (after disrupting previous ones), thus inhibiting and removing/substituting irrelevant information [ 11 , 16 ].
A recent model of updating [ 9 ] showed that updating develops via two main components of inhibition, one more related to control of inhibition from same lists; another one of inhibition from previous lists. The former, shows fewer developmental differences, the latter (also called PI control in [ 9 ]) shows greater age-related differences. In our view, the task used here with children is suitable for consideration of both components in terms of processing speed (an index useful in studying development via more subtle and fine-grained measurement). In fact, in the current task, each participant needs to maintain information and inhibit it, when no longer relevant, by substituting with new information during the tasks (same list inhibition component). Further, to ensure effective updating, s/he has to control for interference from previously studied items which are no longer relevant (i.e., inhibition from previously studied items set).
In particular, in accordance with [ 9 ] model, we found different outcomes consistent with the measures considered. Accordingly, the online RT showed a global age-related effect (older children faster than younger children), but not specific for strength with which letter were associated (in fact, no interaction). This finding could be accounted for, if we consider the development of self-monitoring (i.e., the ability to control one own’s behaviour) in children. That is, monitoring skills develop between 7 to 10 years, and subtle but important improvements are found over the primary school years [ 38 ]. Our self-paced task, where the child had to press the spacebar when s/he thinks to have memorized/updated a given mental set, requires a self-judgment of performance from the child him/herself. In particular, it has been shown that children (from 8 years of age) are more accurate in judgment of learning when given after a delay of about 2 minutes, than immediately after study [ 39 ]. Thus our task (which requires self-monitoring of learning during the study/updating phases, and immediately after, in order to press the spacebar) might not enhance an appropriate child self-regulation. For this reason, we believe we did not find age-related effects relative to strength for self-paced RTs and thus, failed to replicate the effects found with adults [ 14 , 26 ].
Conversely, for offline inhibitory control (i.e., lure recognition), we found more pronounced developmental effects, with significant differences; older children took more time to reject strong lures than weak, whereas no difference was observed for younger children ( Fig 3 ). Therefore, we found that online inhibition component was less affected by developmental change: younger children are able to perform updating tasks successfully. The real challenge in updating (i.e., due to control for previously relevant information) elicits significantly better performance from 10 years onwards. Here, in fact there is no need for self-regulation (i.e., as in the probe recognition task) as the task is not self-paced. The modulation of association strength development in older children (but not in younger) could be well accounted by the development of both lexico-orthographic knowledge and executive mechanisms that can work simultaneously [ 5 , 6 ].
This finding supports claims that the ability to inhibit irrelevant information is a fundamental mechanism that underlines many other developmental changes [ 40 , 41 , 42 ]. In particular, decreased susceptibility to interference is observable as age increases; 7/8 years olds children were shown to be more susceptible to interference than 9/10 years old [ 40 ], as we found in our study. However, we believe the novelty of the current study lies in the specificity of the experimental manipulation. Notably, these results indicated that, from 10 years onward, children found highly familiar stimuli (such as letters) more intrusive and difficult to control when strongly associated. Therefore, although we find that older children are less susceptible to interference, it seems that they are more sensitive to strong and weakly associated stimuli, similarly to performance in adults [ 14 , 26 ].
Future studies should further investigate any additional benefits/costs in updating strong and weak LTM associations, by also manipulating the strength of the item-association at updating [ 14 ]. Through this further manipulation, a more fine-grained examination of the dismantling and recreation of associations during updating would be enabled, including analysis of the relative ease/difficulty of the process. In addition, it could be useful to administer the task to children with specific learning disorders in order to show possible modulation of WM performance by LTM knowledge. Specifically, the task could then be useful to implement ad hoc measures to train children to remediate identified weaknesses, both in educational and clinical settings. We might also speculate that, as we found that strong LTM associations are more difficult to modify, this could in turn indicate the importance of correct support for the child, so that s/he will not act to strengthen incorrect sub-lexical/phonotactic associations. Indeed, it is likely that the more those incorrect associations are reinforced, the harder will be to modify/update them.
In conclusion, the present study demonstrated how WM updating is affected by LTM strength of association in a developmental sample. A significant cost of dismantling and updating strong associations was shown, and this effect was independent from age; all children from 7 to 10 years were comparably sensitive to association strength. In addition, results allowed us to differentiate age-related effects for interference control in updating of strong LTM associations; older children (but not younger) were more susceptible to interference from strongly-associated information.
Supporting information
S1 data. dataset online rts..
https://doi.org/10.1371/journal.pone.0217697.s001
S2 Data. Dataset recognition probe RTs.
https://doi.org/10.1371/journal.pone.0217697.s002
Acknowledgments
We wish to thank all children and schools participating in the study. We also thank Beatrice Colombani for her help with data collection.
- View Article
- Google Scholar
- PubMed/NCBI
- 32. Thurstone L. L. & Thurstone T. G. (1963). Italian edition 1981. PMA : Batteria delle attitudini mentali primarie , 7–11 anni [PMA : Primary mental abilities , 7–11 years ]. Edizioni Giunti OS: Firenze.
- 33. De Mauro T., Mancini F., Vedovelli M. & Voghera M. (1993). Lessico di frequenza dell'italiano parlato. [Frequency Lexicon of Spoken Italian Language] Milano: Etas Libri.
- 36. R Development Core Team. (2010). R: A language and environment for statistical computing. Vienna, Austria: R foundation for statistical computing.
Examining the relationship between working memory consolidation and long-term consolidation
- Theoretical/Review
- Published: 31 March 2022
- Volume 29 , pages 1625–1648, ( 2022 )
Cite this article
- Kelly Cotton ORCID: orcid.org/0000-0001-9014-9762 1 &
- Timothy J. Ricker 2
4840 Accesses
8 Citations
3 Altmetric
Explore all metrics
An emerging area of research is focused on the relationship between working memory and long-term memory and the likely overlap between these processes. Of particular interest is how some information first maintained in working memory is retained for longer periods and eventually preserved in long-term memory. The process of stabilizing transient memory representations for lasting retention is referred to as consolidation in both the working memory and long-term memory literature, although these have historically been viewed as independent constructs. The present review aims to investigate the relationship between working memory consolidation and long-term memory consolidation, which both have rich, but distinct, histories. This review provides an overview of the proposed models and neural mechanisms of both types of consolidation, as well as clinical findings related to consolidation and potential approaches for the manipulation of consolidation. Finally, two hypotheses are proposed to explain the relationship between working memory consolidation and long-term memory consolidation.
Similar content being viewed by others
Consolidation and restoration of memory traces in working memory.
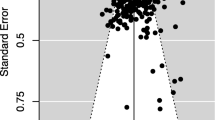
The effect of working memory maintenance on long-term memory
Beyond Long-Term Declarative Memory: Evaluating Hippocampal Contributions to Unconscious Memory Expression, Perception, and Short-Term Retention
Avoid common mistakes on your manuscript.
Early models of memory viewed the acquisition of memories as a progression through a series of stages varying in duration from short-term to long-term (Atkinson & Shiffrin, 1968 ; Waugh & Norman, 1965 ). Later models focusing on the interaction between short-term and long-term memory updated this relationship to include a more explicit working memory system used in ongoing cognition and which interfaces with long-term memory (Baddeley, 2000 ; Baddeley & Hitch, 1974 ). Other models revised the entire system as a single process model, relying on varying levels of activation to determine what is in the focus of attention and “in working memory” and what remains in long-term memory (Cowan, 1988 ; Oberauer, 2002 ).
Extensive research has investigated the processes comprising these memory systems, although often separately for working and long-term memory systems. For example, encoding in working memory may mean something different than encoding in long-term memory, the latter often encompassing all initial memory processing, including multiple stages of working memory processing. Similarly, the concept of storage is often conceptualized very differently across memory types (e.g., Cowan, 1984 ). Still, there appear to be underlying principles which are shared across working and long-term memory. For example, memories must be strengthened to withstand interference and time. Consolidation, or the process of strengthening fragile memory representations to allow accurate retrieval, has developed in separate streams of research in the differing traditions, leading to three types of memory consolidation: working memory consolidation (also called short-term consolidation; Jolicøeur & Dell’Acqua, 1998 ), cellular (synaptic) consolidation (for review, see Kandel et al., 2014 ), and systems consolidation (for review, see Dudai et al., 2015 ). While working memory consolidation is thought to occur over milliseconds to seconds (Nieuwenstein & Wyble, 2014 ; Vogel et al., 2006 ), cellular and systems consolidation may take up to minutes or years, respectively (Dudai et al., 2015 ; McGaugh, 1966 ). Recent work has found improved long-term memory for an item held in working memory when more free time is given after initial item presentation (Hartshorne & Makovski, 2019 ; Jarjat et al., 2018 , 2020 ; Souza & Oberauer, 2017 ). At least one study has directly linked this to increased working memory consolidation (Cotton & Ricker, 2021 ). Identifying shared mechanisms and understanding the relationship between working memory consolidation and long-term memory consolidation is an important step in refining models of human memory and may aid in the development of treatments to improve overall memory function.
In both working memory and long-term memory, consolidation broadly refers to the postacquisition process of stabilizing transient traces into durable memory representations that would otherwise be forgotten (Chun & Potter, 1995 ; Dudai, 2004 ; Jolicøeur & Dell’Acqua, 1998 ; Ricker et al., 2018 ; Squire & Alvarez, 1995 ). The underlying concept shared in working memory consolidation and long-term memory consolidation is that an initially fragile memory trace must be strengthened to withstand interference and time and allow later retrieval. Though they share a general purpose, working memory consolidation and long-term memory consolidation have divergent histories and distinct, though perhaps overlapping, mechanisms.
Both working memory consolidation and long-term consolidation have mechanisms that appear similar at face value. The question remains unanswered whether there is a single consolidation process or two consolidation processes that are initiated simultaneously. Working memory consolidation may be an early step (perhaps even long-term memory encoding) in a single consolidation process that eventually leads to systems consolidation. Conversely, these may be separate processes that occur in parallel, at least in the early stages. This could occur in two ways, as illustrated in Fig. 1 : first, a short-term consolidation process creates a stable working memory representation, which may be further strengthened during the long-term memory consolidation process or else forgotten. Second, a working memory representation is created via working memory consolidation and a long-term memory representation is created via a separate long-term memory consolidation process. These representations are used for separate purposes and likely do not overlap with one another.
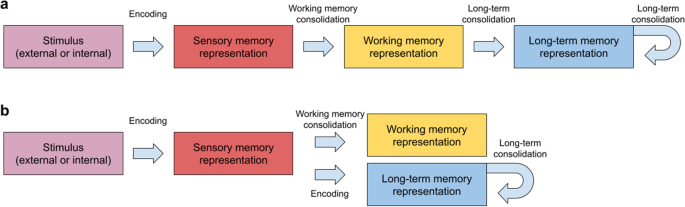
Diagrams of proposed relationships between working memory consolidation and long-term memory consolidation. a The serial relationship hypothesis. b The parallel relationship hypothesis
This review will examine the similarities and differences between working memory consolidation and long-term memory consolidation, with the ultimate goal of understanding how they relate to each other. We will first discuss terminology, conceptual models, and neural substrates of both types of consolidation. Next, we will detail potential shared underlying mechanisms and attempt to describe the relationship between consolidation processes. Finally, we will identify crucial areas of future research focusing on memory consolidation.
Comparing encoding, consolidation, and maintenance
When broadly discussing memory, terms such as encoding, retention, maintenance, storage, and consolidation are often used interchangeably. While this confusion is prevalent even within specific types of memory (Camos et al., 2018 ; Ricker, 2015 ), the resulting confusion is particularly relevant when comparing the working memory and long-term memory literature. It is important to clarify how we differentiate between these concepts and discuss their relationship with each other.
Encoding refers to the initial creation of a memory representation. In the working memory literature, the presentation of stimulus automatically activates perceptual and related long-term representations, creating a transient sensory memory trace (Cowan, 1984 ; Sperling, 1960 ). This sensory memory will quickly be lost unless it is encoded into a working memory representation, a subset of ongoing activated long-term memories. This working memory representation can then be consolidated, maintained, or manipulated as long as attention is directed towards it (Craik et al., 1996 ; Woodman & Vogel, 2008 ). Encoding into working memory is distinct from consolidation, as consolidation begins once the memory has been encoded and can occur even after encoding is no longer possible (Nieuwenstein & Wyble, 2014 ; Ricker, 2015 ; Ricker & Cowan, 2014 ). One method to interrupt encoding is with a perceptual mask, or an irrelevant stimulus immediately after presentation of the target stimulus (Phillips, 1974 ). In contrast, consolidation is not interrupted by a mask. Ricker and Cowan ( 2014 ) demonstrated this distinction in a working memory change detection task by varying the time available for encoding and consolidation across different item presentation methods. In this study, neither equal memory-item presentation times nor the mask-onset delay (i.e., encoding time) equalized forgetting rates across simultaneous and sequential presentation conditions. Instead, the critical factor was the total time from the start of item presentation until the retention interval began. When this amount of time was held constant, both sequential and simultaneous presentation methods allowed for equal consolidation time and subsequently similar rates of forgetting. Furthermore, while masking is traditionally thought to end the encoding process, Nieuwenstein and Wyble ( 2014 ) found evidence to suggest that masking does not end consolidation. Across several experiments, the authors found that a secondary decision-making task retroactively interfered with the primary working memory task at short delays (i.e., 250 – 1000 ms) after a perceptual mask. This interference effect decreased as the delay increased (i.e., beyond 1,000 ms), suggesting that consolidation continues several hundred milliseconds after a perceptual mask.
In contrast to the relatively short time required for working memory encoding, long-term memory encoding often encompasses the entirety of working memory processing, collapsing both creation and maintenance of working memory representation into one encoding process. For example, in Atkinson and Shiffrin’s ( 1968 ) model, a presented stimulus is first registered in the sensory store, then the short-term store, until finally the representation is transferred (as in, selected information is copied from one store to another while still remaining in the first store) to long-term storage. According to this model, information in the short-term store may undergo repeated rehearsal (i.e., maintenance rehearsal) to extend its time in the short-term store or coding processes (i.e., elaborative rehearsal) and as a result is encoded into the long-term store. Although not addressed by the authors, it is possible that this model also allows for other, more recently proposed working memory processes (e.g., other maintenance mechanisms addressed below or consolidation) to initiate or occur as part of the long-term encoding process. Popov and Reder ( 2020 ) directly addresses the impact of working memory processing on long-term encoding. According to this model, the strength of a long-term memory trace is dependent on the efficacy of the encoding process. Long-term encoding, in turn, is dependent on the working memory resources that are available during initial processing. These resources are depleted by different working memory processes, including strengthening and maintenance of the memory trace, and must slowly recover before being used again to process additional stimuli. Long-term encoding may include deeper encoding processes, such as elaboration, which generally result in a stronger long-term (Bartsch & Oberauer, 2021 ; Bartsch et al., 2018 , 2019 ; Bradshaw & Anderson, 1982 ; Craik & Tulving, 1975 ), but not working memory representation (Bartsch & Oberauer, 2021 ; Bartsch et al., 2018 , 2019 ), suggesting that long-term encoding may encompass both working memory processing as well as deeper elaboration. As in working memory, consolidation is distinct from encoding in long-term memory. The memory representation resulting from long-term encoding consists of the physical connections within the brain, which are then strengthened during long-term consolidation.
In working memory and long-term memory, memory retention may refer to active maintenance or passive storage. In working memory, retention via active maintenance requires attention and may refer to a number of processes, including attentional refreshing (Camos et al., 2018 ; Raye et al., 2007 ), rehearsal (Baddeley et al., 1975 ; Lucidi et al., 2016 ), and removal of interference (Ecker et al., 2014a , b ; Oberauer et al., 2012 ). These processes are distinct from consolidation (Bayliss et al., 2015 ; Woodman & Vogel, 2005 ) but are important in preserving a memory representation for use in working memory tasks. Bayliss et al. ( 2015 ) distinguished consolidation from both rehearsal and attentional refreshing. In Experiments 1 and 2, participants completed a working memory task in which the memory items were presented sequentially, with a secondary task interspersed between each memory item. After each item presentation, a variable delay period preceded the secondary task, which also varied in difficulty or pace. Using this paradigm, the authors were able to dissociate the effects of consolidation (the delay period) from the effect of attentional refreshing (the opportunities to attentionally refresh modulated by the difficulty of the secondary task) on working memory performance. Both consolidation time and attentional refreshing opportunities affected performance, but these manipulations did not interact, suggesting that these are separate processes. The processes are also conceptually distinct, in that while both occur with some delay after initial encoding and extend the accessibility of the memory trace, attentional refreshing maintains this accessibility only while the refreshing process is ongoing. Consolidation, on the other hand, extends the accessibility of a representation for some time even after the consolidation process itself has ended. The findings of the first two experiments of Bayliss et al. ( 2015 ) were replicated in a third experiment, with the addition of an articulatory suppression task during the delay interval to prevent articulatory rehearsal. As in the earlier experiments, increased consolidation time improved memory performance, even when participants were engaging in articulatory suppression, suggesting the benefit of time after item presentation is uniquely due to improved consolidation and not more verbal rehearsal. While outside the scope of the present paper, other research has questioned the importance of verbal rehearsal as a maintenance mechanism (Ricker et al., 2020 ; Souza & Oberauer, 2018 , 2020 ), providing further support for a consolidation or consolidation + maintenance account of successful memory performance. Retention functions differently in long-term memory, as it occurs over much longer periods of time compared with working memory, making sustained attention impossible. Instead, long-term memory retention refers to generally more passive storage. In both working memory and long-term memory, consolidation is necessary for retention processes.
Working memory consolidation
Several different task paradigms have been used to identify the effects of consolidation on working memory performance, illustrated in Fig. 2 . While these tasks may appear different, they all share a basic structure: presentation of a memory item followed by some variable delay and a second item, which may be another memory item, a distractor, or a secondary task. A key marker of a consolidation effect during these tasks is a one-way effect in which the time given to process the first item only affects performance for the next item. Various paradigms have demonstrated evidence of a consolidation effect. Early investigations by researchers on attention and visual processing using rapid serial visual presentation (RSVP) paradigms found that an item presented quickly after target item is unlikely to be identified, a phenomenon now referred to as the attentional blink (Broadbent & Broadbent, 1987 ; Chun & Potter, 1995 ; Raymond et al., 1992 ; Shapiro et al., 1997 ). Chun and Potter ( 1995 ) proposed a two-stage model of the attentional blink, discussed in detail below, which posits the second stage involves consolidating the item into working memory. These findings were subsequently corroborated with electrical recordings demonstrating that presentation of the first target item modulated event-related potential components related to the consolidation of the second item (Luck et al., 1996 ; Vogel & Luck, 2002 ; Vogel et al., 1998 ). A short-term consolidation process directly involved in working memory processing was identified by Jolicøeur and Dell’Acqua ( 1998 ) in a series of experiments, discussed in the next section. Together, these findings suggest the existence of some central processing occurring after presentation of an item, which temporarily inhibits processing of subsequently presented items. This process was found to be distinct from initial encoding (for review, see Ricker, 2015 ) and maintenance (Woodman & Vogel, 2005 ), suggesting a third process (i.e., consolidation).
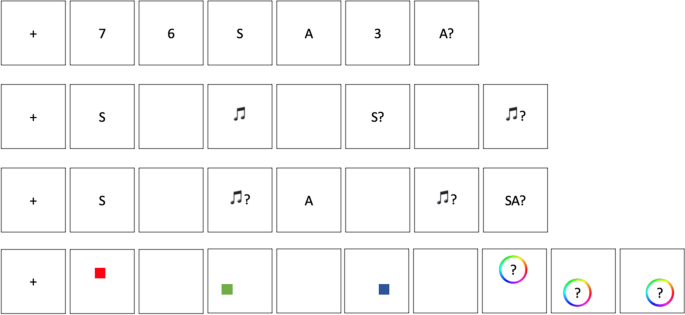
Examples of experimental paradigms typically used to study working memory consolidation. In the first row, a series of stimuli are rapidly presented and the two target items (S and A) are presented in succession. Memory for the second target item is then tested. In the second row, the target item (S) is presented, followed by a delay. A secondary target item (a tone) is then presented and memory for the first item is tested before a response to the second item is given. In the third row, each target item (S and A) is followed by a delay and then a secondary task (tone identification). After all target items have been presented, memory for each target item is tested. In the fourth row, each target item (colored square) is followed by a delay before presentation of the next target item. After all items have been presented, memory for each color is tested
Models of working memory consolidation
Early investigations into working memory consolidation focused on the attentional bottleneck that occurs when processing multiple items. Jolicøeur and Dell’Acqua ( 1998 ) examined memory performance in a dual-task procedure by varying the stimulus onset-time asynchrony (SOA) between memory stimuli presentation and a secondary two-alternative forced-choice task presentation. Across a series of experiments in which participants had to remember items while performing an auditory identification task, the authors found that at short SOAs (less than about 1,800 ms, though the exact time depends on the particular task demands), participants were slower to respond to the secondary task than at longer SOAs (i.e., they took more time to identify whether a tone was high or low). As the SOAs increased, reaction times to the second task decreased. The authors suggested that the secondary task performance impairments were due to an attentional resource bottleneck caused by consolidation of the initial memory item into working memory. In other words, the consolidation of the first item required both central attentional resources and time, preventing processing of other items (i.e., responding to tone in the secondary task) until the first item is fully consolidated. In trials in which participants saw but did not have to remember the items, there was no delay in processing the secondary task, suggesting that the resource-demanding working memory consolidation did not occur. Subsequent recall of the memory items was poor in those trials, underscoring the necessity of working memory consolidation in creating lasting memories. Later research suggested that this working memory consolidation process takes no more than one or two seconds (Nieuwenstein et al., 2009 ; Ricker & Hardman, 2017 ; Stevanovski & Jolicøeur, 2007 ; Wyble et al., 2011 ).
According to this strict bottleneck model, due to the limits on attentional resources, consolidation will prevent subsequent items from being processed until it has completed (Jolicøeur & Dell’Acqua, 1998 ). Consolidation must continue until it is completed and cannot be stopped. More flexible models have since been proposed which suggest that individuals may flexibly allocate attentional resources, depending on task instructions (Lehle & Hübner, 2008 ; Tombu & Jolicøeur, 2003 ). A recent study by Vergauwe et al. ( 2019 ) support the flexible bottleneck model. The results of this study suggested that in some contexts, such as when there is an expectation of relatively short amount of processing time, participants engage primarily in consolidation, while in other contexts, such as when there is an expectation of more time is available, participants rely on attentional refreshing. Rather than a fixed postencoding process, consolidation is one strategy that may be flexibly adopted, depending on the task. However, once consolidation is chosen, it acts as a bottleneck and prevents initiation of another process (i.e., attentional refreshing) until completion.
While Jolicøeur and Dell’Acqua ( 1998 ) was an earlier adopter of the term consolidation to refer to postencoding processing in working memory, a similar concept can be found in research on the attentional blink. The attentional blink effect often lasts for about 500 ms, such that any items presented within 500 ms of a target item are unlikely to be processed (Broadbent & Broadbent, 1987 ; Raymond et al., 1992 ), but sometimes lasts for a few hundred milliseconds longer (Lagroix et al., 2012 ). Chun and Potter’s ( 1995 ) two-stage model for the attentional blink posits that all items are first processed in Stage 1 (rapid detection) and then, when given enough time, items will be processed in Stage 2 (capacity-limited processing). While Stage 2 processing of an initial target item is occurring, subsequent items will only be processed at a Stage 1 level, where they will be prone to rapid forgetting if not processed further. Stage 2, capacity-limited processing, is necessary for items to withstand interference and be retrieved later. As some researchers have proposed that the attentional blink occurs because consolidation of the first target item into working memory prevents processing of items that fall within the consolidation period (Arnell, 2006 ; Ricker et al., 2018 ), Stage 1 could represent working memory encoding, while Stage 2 could represent working memory consolidation.
The episodic Simultaneous Type/Serial Token (eSTST) model of working memory consolidation builds on models of the attentional blink. This model was initially developed to describe the role of temporal attention in object identification and working memory encoding, with a particular focus on the findings of the attentional blink research (Bowman & Wyble, 2007 ; Nieuwenstein & Wyble, 2014 ). According to the eSTST model, after a target item appears, a two-stage process begins. In the first stage, visual processing (including feature extraction and categorization, resulting in type representations) occurs in parallel, allowing multiple items to be processed simultaneously. The representations formed during Stage 1 processing are similar to iconic memory (Coltheart, 1980 ) and will rapidly decay without more processing. A salience filter, defined as all mechanisms involved in top-down selection, is employed to determine, based on expectations or goals, which items are relevant and should be further processed in the second stage. During Stage 2, task-relevant items (types) are associated with episodic contexts (tokens). This type-token binding process results in a “pointer” tying a token (which is maintained in working memory) to its related type (which no longer needs to be processed). During Stage 2 processing attention is suppressed and cannot be used to process other items, resulting in the attentional blink. Completion of type-token binding frees up attentional resources to be used to process other items. This mirrors earlier bottleneck approaches, which suggested that working memory consolidation was the result of limitations on attentional resources. However, the eSTST model places a higher emphasis on the initial parallel processing of multiple items, though only relevant items will be consolidated. The final component of the eSTST model is the binding pool, or the limited capacity resource used to bind types to token (similar to the distributed representations created in long-term memory, discussed below). While the initial model was formulated primarily to account for the attentional blink, the eSTST model was later extended to also explain working memory consolidation (Nieuwenstein & Wyble, 2014 ).
A recent model has taken the basic two-stage processing model and adapted it to a precision-based working memory consolidation model (Ye et al., 2017 , 2020 ). This model involves creation of a low-precision representation of all memory items in the first stage, which is then further refined during the second stage. This is a divergence from both bottleneck models, which focus on an all-or-none approach (the first item is fully consolidated before moving on), and attentional blink models, which suggest that items not processed in the second stage will be forgotten completely. In the multistage model proposed by Ye et al. ( 2017 ), the first stage is involuntary and, like the eSTST model, driven by a sensory representation and the stimuli’s physical properties. Given sufficient consolidation time, the voluntary second stage may occur, during which attention may be flexibly controlled and directed to further increasing the precision of a memory representation. In either case, the memory item may be recalled later, although more time will produce a more precise memory representation.
Models of working memory consolidation typically focus on the availability of cognitive resources while engaging with a task, leading potentially to a bottleneck or an attentional blink, and impacting memory for a second item that appears during consolidation of a first item. These models often view consolidation as an all-or-none process, although a few argue for a graded increase in memory precision. Across all models, more time given to fully complete a consolidation process leads to improved memory, whether by increasing the number of items consolidated or improving the precision of the memory representations.
Neural mechanisms underlying working memory consolidation
The neural underpinnings of working memory consolidation are difficult to study, and few studies have directly explored them. While there is some recent direct evidence in the occipital lobe, other regions in the frontal and parietal cortices are likely involved. Activity that previously has been associated with working memory maintenance or storage in these regions may instead reflect working memory consolidation. The following section outlines regions that have been directly implicated in consolidation as well as regions in which activity may be reinterpreted in a consolidation context.
A number of studies have found that activity in the occipital cortex during visual working memory tasks is related to representations stored in working memory (Emrich et al., 2013 ; Ester et al., 2009 ; S. A. Harrison & Tong, 2009 ; Serences et al., 2009 ; Tallon-Baudry et al., 1998 ; Tallon-Baudry et al., 1999 ; Vogel & Machizawa, 2004 ). Recent studies have directly manipulated this activity to investigate this region’s role in working memory consolidation. Makovski and Lavidor ( 2014 ) used transcranial direct current stimulation (tDCS) of the occipital cortex during a change detection task and found that tDCS stimulation led to better memory performance, in the short (200 ms), but not the long (500 ms) encoding condition compared with no-stimulation trials. Although the authors interpret these findings as evidence of occipital cortex involvement in working memory consolidation and not maintenance due to the different results between encoding conditions, the estimates of time course of consolidation vary greatly and are often considered longer than 500 ms (e.g., Nieuwenstein et al., 2009 ; Ricker & Hardman, 2017 ; Stevanovski & Jolicøeur, 2007 ; Wyble et al., 2011 ). Further research is needed to clarify the exact timing and role of the occipital cortex in working memory consolidation. The occipital region was also investigated using transcranial magnetic stimulation (TMS) by van Lamsweerde and Johnson ( 2017 ). This study found that TMS applied to the occipital cortex immediately poststimulus onset resulted in a decrease in the number of items maintained in working memory as well as an effect on the quality of the memory representations. Other studies using TMS have similarly found effects on working memory consolidation (for a review, see van de Ven & Sack, 2013 ). Together, these results suggest that the occipital cortex plays a role in visual working memory consolidation. Other sensory cortices likely play similar roles in their respective sensory modalities, though this question has not yet been answered.
Although not directly measuring working memory consolidation specifically, research investigating postencoding processing has found evidence of neural activity that may reflect consolidation activity. Persistent neural activity in the prefrontal cortex during the delay period in a working memory task has been linked to memory processing in nonhuman primates (Fuster, 1973 ; Fuster & Alexander, 1971 ; Kubota & Niki, 1971 ) and humans (Courtney et al., 1997 ; Zarahn et al., 1997 ; for review, see D’Esposito & Postle, 2015 ). More specifically, neurons in the dorsolateral prefrontal region seem to be active during working memory delay periods. This activity is often associated with working memory maintenance but may also or instead reflect working memory consolidation. Research has found that this delay-period activity changes with the length of the delay period, suggesting that it is not solely representative of a response selection, but rather some type of memory-related activity (Goldman-Rakic, 1991 ). Similarly, if activity only represented nonmnemonic processing, then activity should be similar for both correct and incorrect responses. In reality, it seems the stimulus-relevant neurons fail to increase their firing rate during the delay period preceding an incorrect response (Funahashi et al., 1989 ; Goldman-Rakic, 1991 ), indicating the possibility that the presence or magnitude of the delay-period activity of the relevant neurons directly corresponds with the efficacy of the consolidation process, though this was not tested directly.
Activity in frontal regions has also been linked with working memory in humans (Deiber et al., 2007 ; Ester et al., 2015 ; Johnson et al., 2005 ; Lefebvre et al., 2013 ; Majerus et al., 2010 ; Raye et al., 2002 ; Stern et al., 2001 ; Tallon-Baudry et al., 1998 , 1999 ). In particular, the magnitude of delay-period activity in frontal regions has been shown to correspond with the memory accuracy (Curtis et al., 2004 ). This delay-period activity has been suggested to represent working memory maintenance mechanisms, such as attentional refreshing, but it may also reflect consolidation activity. Given the length of delay periods in some of these studies exceed even the longest estimates for the time course of working memory consolidation (for example, Curtis et al., 2004 , uses delays of nearly 10 s), it is likely that consolidation is one of several processes occurring during this period. Further research explicitly designed to dissociate between working memory maintenance and consolidation is needed to distinguish the unique neural signatures of these processes.
Parietal regions have also been implicated in working memory processing (e.g., Berryhill et al., 2010 ; Deiber et al., 2007 ; Ester et al., 2015 ; Hahn et al., 2018 ; Majerus et al., 2010 ; Sreenivasan & D’Esposito, 2019 ; Todd & Marois, 2004 ; Vogel & Machizawa, 2004 ) and may be involved in working memory consolidation. Hahn et al. ( 2018 ) found that number of items held in working memory predicted activation in the posterior parietal cortex. In this study, participants underwent functional magnetic resonance imaging (fMRI) scanning while completing a change detection task. During the change detection task, participants were presented with an encoding array of colored squares for 200 ms. The items were then removed, and the participants were presented with a blank screen for varying delays (ranging from 1,100 to 4,400 ms). After the delay, one of the colored squares from the encoding array appeared in its original location, and the participants indicated whether the color of that square had changed. The number of items maintained in working memory (calculated as K ; Cowan, 2001 ) correlated with more activation in the bilateral posterior parietal cortex (as well as the bilateral middle occipital gyrus), in line with previous studies (Todd & Marois, 2004 , 2005 ). Given the temporal resolution of fMRI, it is reasonable to suggest that at least some of the early activity during this delay period, like previously discussed in frontal regions, corresponded to working memory consolidation. However, the temporal resolution of these methods did not allow for such a distinction between consolidation and other working memory processing, though future research should explore the possibility of clarifying the precise nature of this postencoding neural activity in parietal regions.
Hahn et al. ( 2018 ) also investigated the brain regions associated with working memory storage in individuals with schizophrenia and found that these individuals stored fewer items in working memory and demonstrated less activation in the left posterior parietal cortex. These results, along with findings (discussed in detail below) that individuals with schizophrenia exhibit slowed working memory consolidation (Fuller et al., 2005 , 2009 ) and that faster working memory consolidation leads to a large number of items stored in working memory (Xie & Zhang, 2017 ), suggest that the posterior parietal cortex may be a central component in working memory consolidation. Dysfunction of this region may cause a slowing of consolidation and subsequent decrease in working memory capacity.
In summary, the neural mechanisms involved in working memory and particularly working memory consolidation are spread throughout the cortex. There is substantial evidence suggesting that the primary regions involved include the dorsolateral prefrontal cortex, posterior parietal cortex, and sensory areas, such as the occipital cortex for visual working memory. While these regions may all be broadly involved in working memory consolidation, their exact role in the process may vary. For example, the prefrontal regions may correspond to the control of consolidation, including its initiation, as well as the control and initiation of other maintenance processes. Given the time course of the delay-period activity exceeds typical estimates of working memory consolidation time, the prefrontal cortex is likely to be involved in the coordination of multiple processes. The occipital lobe may correspond with the selection of what needs to be consolidated into visual working memory, as evidence by the relatively early activity in this region corresponding with working memory consolidation processing. Parietal regions may serve as the location of the cortical representations to be consolidated or a pointer system indicating the location of the cortical representation. Other regions, such as the hippocampus and surrounding regions, may also be involved in some aspects of working memory functioning (Borders et al., 2021 ; Mitchell et al., 2000 ; Nee & Jonides, 2011 ; Newmark et al., 2013 ; Piekema et al., 2006 ; Ranganath & D’Esposito, 2001 ; Rose et al., 2012 ; Stern et al., 2001 ), and its potential role in working memory consolidation is discussed below. These proposals are speculative as this question has not been tested thoroughly, but future research may precisely delineate each region’s role in working memory consolidation.
Long-term memory consolidation
An early conceptualization of long-term memory consolidation was introduced by Müller and Pilzecker ( 1900 ), in which they proposed that a single occurrence of learning does not instantly result in a permanent memory and stabilization of the memory takes some amount of time after learning (Lechner et al., 1999 ; McGaugh, 1999 ). Across 40 experiments, Müller and Pilzecker attempted to outline the process of memory formation and retrieval. Although not directly influenced by Müller and Pilzecker, the theories of learning proposed by Hebb ( 1949 ) are related to the earlier consolidation theories. Hebb’s synaptic plasticity hypothesis proposes that memories are represented in the brain as changes in synaptic connectivity strength. When two neurons fire together repeatedly, they are more likely to fire together again in the future (Hebb, 1949 ). While this idea has since been expanded upon (for review, see Bailey et al., 2015 ), the basic underlying principle of activity-related changes in synaptic plasticity underlying long-term memory formation and storage remains (Martin et al., 2000 ; but see Arshavsky, 2006 ).
Consolidation was again popularized in research focusing on the pattern of deficits of individuals with memory impairments. For example, Patient H.M., after undergoing a temporal lobectomy, subsequently suffered from both anterograde and graded retrograde amnesia: he was unable to form lasting new memories and could only retrieve memories from long before his surgery (Scoville & Milner, 1957 ). These findings suggested that the hippocampus and medial temporal lobe are involved in the acquisition of new memories but were not the final resting place of all memory, again illustrating a role of a longer stabilization process.
Unlike the experimental paradigms to investigate working memory consolidation shown in Fig. 2 , long-term consolidation is typically investigated by examining the quality or existence of a memory after a length of time from tens of minutes to several weeks or more. Common manipulations include varying the length of time between encoding and retrieval, presenting interfering information during the delay, or re-presenting the studied information in order to “reactivate,” illustrated in Fig. 3 . Many studies use some combination of these paradigms. For example, Müller and Pilzecker ( 1900 ) asked participants to both learn a list of syllable pairs (List A) before learning a second list (List X) or learn a list of syllables pairs (List B) before a blank retention interval. When List X was presented after 17 s, memory for List A was much poorer than for List B. Memory for List A was not affected when List X was presented after 6 min (Lechner et al., 1999 ), demonstrating a benefit to increased and uninterrupted consolidation time. A similar method was employed by Takashima et al. ( 2009 ). In this study, participants first learned on series of face-location pairs 24 h before testing and then a second series of pairs 15 min before test. In this way, memory for more remote events, which had theoretically undergone more consolidation, could be distinguished from more recent events. Similar methods have been employed with amnesic patients with hippocampal or medial temporal lobe lesions (for review, see Squire et al., 2015 ). Long-term consolidation has also been investigated via measuring electrical activity in the brain during sleep, during which the brain is thought to “replay” or reactivate certain patterns of neural activity in order to consolidate memories (Carr et al., 2011 ; Louie & Wilson, 2001 ; Schapiro et al., 2018 ; for review, see Zhang et al., 2017 ). For example, Jiang et al. ( 2017 ) measured participant’s brain activity while awake and identified patterns of activity that corresponded with certain “events” that occurred during waking periods. These patterns of activity were also identified during subsequent sleeping periods. This memory replay has been suggested to correspond with long-term sleep-dependent consolidation and has been experimentally manipulated via odor cueing (for review, see Shanahan & Gottfried, 2017 ), but is outside the scope of the present review.
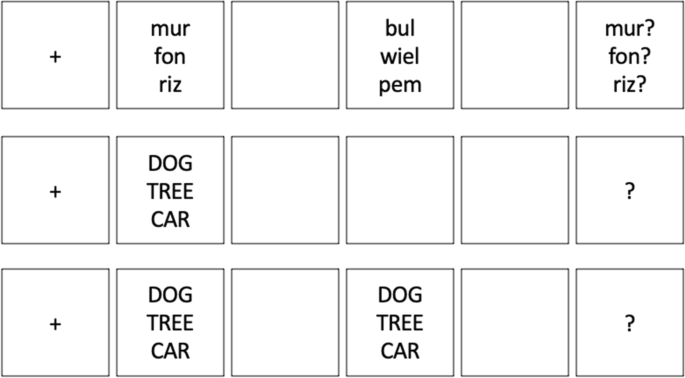
Examples of experimental paradigms typically used to study long-term consolidation. In the first row, a list of nonsense syllables is first learned, followed by a delay period. Another list of nonsense syllables is presented and later memory for the first list is tested. In the second row, a list of words is learned, followed by a long (often overnight) delay before test. In the third row, a list of words is first learned and later the same list is presented again before the final test
Models of long-term memory consolidation
According to many current models of long-term memory consolidation, the first step is a cellular consolidation process which begins immediately postlearning and continues for a few hours (for reviews, see Genzel & Wixted, 2017 ; Kandel et al., 2014 ). Cellular consolidation involves a cascade of molecular processes that increase the synaptic efficiency of a neuron, leading to easier reactivation later.
The second step in the two-stage models is systems consolidation, which may refer to the process of reorganizing the memory and distributing it across multiple brain regions, or the schematization of the memory. An early and prominent model of systems consolidation is Standard Consolidation Theory, which posits that hippocampal involvement in memory creation and storage is relatively limited to early processing (McClelland et al., 1995 ; Squire, 1992 , 2004 ). Initially, the memory is encoded in networks in both the hippocampus and the neocortex. During subsequent rest, the hippocampal network is reactivated, triggering activity of the neocortical network. This repeated coordinated reactivation strengthens the neocortical network, until it is stabilized enough to no longer require hippocampal reactivation (Frankland & Bontempi, 2005 ). This view is primarily supported by research involving amnesic patients, particularly in individuals with temporally graded retrograde amnesia who experience the loss of recent memories while more remote memories remain (Winocur & Moscovitch, 2011 ). According to the Standard Consolidation Theory, hippocampal damage interferes with the stabilization of recent memories undergoing consolidation, resulting in loss of memory for the period immediately surround the damaging incident. In contrast, remote memories are more likely to have undergone substantially more or even completed consolidation and thus be represented in the neocortex and retrieved without involvement of the hippocampus. Similar to the eSTST model of working memory consolidation described earlier, a model of consolidation proposed by Alvarez and Squire ( 1994 ) suggests that consolidation reflects the binding of features that comprise a memory representation, in this case stored across different brain regions. The medial temporal lobe rapidly learns and later simultaneously activates the connected cortical regions, which learn much slower. Eventually, these areas become more strongly connected and form a robust memory representation.
While Standard Consolidation Theory posits the reduction of hippocampal involvement as memories become more remote, other theories have suggested that the hippocampus continues to play a crucial role in memory storage and retrieval. Reviews of the literature concerning individuals with hippocampal damage and retrograde amnesia suggest that some individuals exhibited nongraded retrograde amnesia. In other words, although classic studies involving Patient H.M. demonstrated that his memory loss included primarily recent events, some patients with similar lesions experience memory loss even for very remote memories (Winocur & Moscovitch, 2011 ). These findings suggest that the hippocampus may play a larger role than a simple relay station to neocortical representation.
Multiple Trace Theory was among the first to suggest that while interactions between the hippocampus and neocortex leads to stabilization of generalized semantic memories, more detailed episodic memories are dependent upon the hippocampus (Nadel & Moscovitch, 1997 ; Nadel et al., 2000 ). This hypothesis is supported by findings that the hippocampus is active during retrieval of even very remote memories (Harand et al., 2012 ; Ryan et al., 2001 ). According to the Multiple Trace Theory, during encoding two memory traces are created, a hippocampal trace and cortical trace. The cortical trace contains the generalized features of the memory, while the hippocampal trace maintains the contextual details of the memory. Each time a memory is reactivated, a new copy of the cortical trace is created. Older memories that have been reactivated more have a greater number of copies distributed throughout the cortex, allowing them to be more easily accessed, and less likely to be affected by partial hippocampal lesions (Cheng, 2017 ; Nadel & Moscovitch, 1997 ; Nadel et al., 2000 ). Trace Transformation Theory similarly focuses on the memory trace and its representation within the hippocampus and cortex (Winocur & Moscovitch, 2011 ). According to this theory, while initially dependent on the hippocampus for retrieval, some memories are transformed from the contextually rich hippocampal memory traces to more generalized memory traces that rely on cortical regions. These two memory traces may coexist and interact with each other, depending on a variety of factors.
More recent models for consolidation-like processing build on the Multiple Trace and Trace Transformation theories. Yonelinas et al. ( 2019 ) recently proposed the Contextual Binding Theory as an alternative to Standard Consolidation Theories. According to this model, the role of the hippocampus in memory formation is to bind together contextual information from various regions, including the amygdala and perirhinal and parahippocampal cortices. One main contribution of this theory lies in the idea that while some aspects of an event’s context change quickly (e.g., beginning a new task or moving to a new room), others change much more gradually (e.g., a participant’s mood). Interference from information with a similar context leads to forgetting and reducing this contextual interference (i.e., during sleep) leads to less overall forgetting. Much like the Multiple Trace and Trace Transformation Theories, the Contextual Binding Theory relies on the continued involvement of the hippocampus in memory storage beyond initial encoding, particularly for highly detailed memories. In contrast, the neocortex is primarily responsible for less contextually detailed memories. Other recent models have also emphasized the importance of context in memory creation (e.g., Gisquet-Verrier et al., 2015 ).
Neural mechanisms underlying long-term memory consolidation
Cellular consolidation in long-term memory begins when some stimulation triggers a release of neurotransmitters from a presynaptic neuron. This leads to a cascade of neurochemical reactions, including increasing the number of neurotransmitter receptors on the neuron, ultimately making it more easily activated in the future (for a thorough overview of the molecular mechanisms involved, see Sekeres et al., 2017 ). Further stimulation of this neuron will lead to increased learning. When two neurons are activated simultaneously, this process allows the synapses between these neurons to strengthen. With enough simultaneous firing, an association between these items (and the subsequent memory) is created (Hebb, 1949 ). During later systems consolidation, these synaptic connections are further reinforced, or pruned if deemed unimportant.
Systems consolidation involves several regions across the brain. One crucial region is the medial temporal lobe (Scoville & Milner, 1957 ; Squire et al., 2015 ). As previously outlined, the major models of consolidation agree that the hippocampus in particular plays a crucial role in long-term memory consolidation (McClelland et al., 1995 ; Nadel & Moscovitch, 1997 ; Squire, 2004 ; Wiltgen et al., 2004 ; Winocur & Moscovitch, 2011 ; Yonelinas et al., 2019 ). Distributed representations of elements of an acquired memory held in cortical regions are temporarily bound together by the hippocampus, which are then reactivated during off-line periods, such as sleep or wakeful rest (A. K. Lee & Wilson, 2002 ; Louie & Wilson, 2001 ; Schapiro et al., 2018 ; Tambini & Davachi, 2013 ; Zhang et al., 2017 ). This reactivation involves a reinstatement of the patterns of neural activity that occurred during the original learning event, which strengthens the connections between the memory elements and stabilizes the memory.
Prefrontal regions have also been implicated in long-term systems consolidation, though exact nature of their involvement varies across models. As previously discussed, according to Standard Consolidation Theory, as memories become more remote, regions in the prefrontal cortex are more active during subsequent retrieval (in particular, medial prefrontal regions, including the prelimbic and anterior cingulate cortex; e.g., Bontempi et al., 1999 ; Takehara et al., 2003 ). Other models also involve the prefrontal regions, though focus less on a recent versus remote distinction and more on the level of contextual detail of a memory. There is also a direct pathway from the hippocampus to prefrontal cortex, though it is unidirectional, and the hippocampus does not receive projections from the prefrontal cortex (Laroche et al., 2000 ).
Most relevant to the current question are the regions involved in the immediate post-long-term encoding period. As discussed earlier, it is likely that long-term encoding encompasses several working memory functions, including working memory consolidation, so this period is particularly important to understanding the relationship between working memory consolidation and long-term consolidation. Cohen et al. ( 2015 ) reviewed several studies that investigated how the seconds immediately following encoding predict subsequent long-term memory. Several studies found that later memory was predicted by the immediate postencoding activity in the hippocampus (Ben-Yakov & Dudai, 2011 ; Evensmoen et al., 2015 ), entorhinal cortex (Evensmoen et al., 2015 ), striatum (Ben-Yakov & Dudai, 2011 ), cerebellum (Ben-Yakov & Dudai, 2011 ), and posterior parietal cortex (Elman et al., 2013 ). Other research has also found that the posterior parietal cortex is active during initial long-term memory encoding (Brodt et al., 2016 , 2018 ; Himmer et al., 2019 ).
In summary, the main regions historically considered to be involved in long-term memory consolidation are medial temporal and prefrontal regions, but more targeted research focusing on the immediate postencoding period suggests a role of other regions, in particular the posterior parietal cortex. The exact roles of these regions in long-term memory consolidation are debated, but it is likely that the hippocampus and parietal regions are involved in initial encoding and early consolidation of memory representations, while prefrontal regions become more involved later in the consolidation process.
Differences in consolidation
It is well-understood that not only do general memory abilities vary across different populations (e.g., schizophrenia, J. Lee & Park, 2005 ; attention-deficit/hyperactivity disorder, Martinussen et al., 2005 ; Alzheimer’s disease, Morris & Kopelman, 1986 ), they also differ within a person depending on a variety of factors, such as emotional state or stress (Figueira et al., 2017 ; Leight & Ellis, 1981 ; Long et al., 2020 ; Shields et al., 2017 ). Often it is unclear which underlying mnemonic process is affected, and impairments in retention may reflect disruptions of earlier processes. For example, it was evident that Patient H.M. did not have trouble with processes such as memory encoding or very long-term retrieval, leading to the development of systems consolidation theories previously discussed. Other memory impairments may similarly be explained as deficits in working memory consolidation or long-term memory consolidation ability (e.g., long-term memory consolidation in epilepsy, Blake et al., 2000 ; working memory consolidation in schizophrenia, Fuller et al., 2005 ). As you will see, conditions associated with impaired consolidation tend to have impairments in both working memory and long-term memory, suggesting a connection between the two processes.
Early research found marked impairments on working memory performance in schizophrenia (Knight et al., 1985 ), and more recent research has specifically attributed these deficits to working memory consolidation impairments (Fuller et al., 2005 , 2009 ). Individuals with schizophrenia appear to exhibit longer attentional blinks, suggesting that they take longer to consolidate the first item in a sequence (Cheung et al., 2002 ; C. R. Li et al., 2002 ). Fuller et al. ( 2005 ) found that individuals with schizophrenia were much slower at consolidating information into working memory compared with healthy controls, requiring twice as much time to fully consolidate the information. Similarly, individuals with schizophrenia also exhibit long-term memory consolidation impairments, likely related to sleep disruptions (Genzel et al., 2015 ; Manoach & Stickgold, 2009 , 2019 ; Wamsley et al., 2012 ). Baran et al. ( 2018 ) found that patients with schizophrenia showed reduced consolidation of verbal memory, but not visuospatial procedural memory or statistical learning, compared with healthy controls. These results support a potential relationship between working memory consolidation and long-term memory consolidation, though this has not been explicitly tested. As working memory consolidation has been shown to influence later long-term memory performance (Cotton & Ricker, 2021 ), slowed or ineffective working memory consolidation may impact later long-term memory consolidation by failing to create a durable representation while awake, leaving sleep-related processes nothing to consolidate. According to this view, deficits in long-term memory performance are not solely due to long-term memory processing impairments, but instead are impacted much earlier, specifically during working memory consolidation.
Another population which has demonstrated impairments in memory consolidation is individuals with multiple sclerosis (MS; Sandry et al., 2019 ). Common cognitive symptoms include impaired working memory, processing speed, and long-term memory (Chiaravalloti & DeLuca, 2008 ). A recent meta-analysis confirmed that memory acquisition (which includes consolidation) in particular underlies the general memory impairments (Johnen et al., 2017 ). One study investigated the role of acquisition and found that individuals with MS required more trials to reach a learning threshold (DeLuca et al., 1998 ). The authors suggested that each additional trial provided an opportunity to improve the quality of the memory representation. Although not identified by the authors, one possible mechanism to improve quality of the memory representations may be working memory consolidation, by increasing the precision of the representation or the likelihood that the episode resulted in the creation of a memory representation. In line with this hypothesis, Kavcic and Scheid ( 2011 ) found that individuals with MS experienced a longer attentional blink, suggesting they require more time to fully consolidate information. Furthermore, Sandry et al. ( 2019 ) differentiated two separate components of acquisition and found that patients with MS had both poor encoding (number of items initially stored) and consolidation (number of items retained between immediate test and delayed test). Other studies have investigated impairments of long-term memory consolidation of motor memory in individuals with MS and found that while individuals with MS do exhibit deficits in sleep-related consolidation (Nguemeni et al., 2021 ), those impairments may specifically be related to corticosteroid treatment (Dresler et al., 2010 ). While there is limited and mixed research on consolidation in individuals with MS, it appears that like schizophrenia, the initial memory acquisition phase may suffer from inefficient working memory consolidation, leading to poor representations to consolidate during off-line periods.
Stress and anxiety have been shown to impair both working memory (e.g., Diamond et al., 1996 ; Luethi et al., 2009 ; Moran, 2016 ) and long-term memory (Chamberlain et al., 2006 ; Meir Drexler & Wolf, 2017 ), although the specific effects on consolidation are unclear, as other studies have found no effect (e.g., Lewis et al., 2008 ; Luethi et al., 2009 ; Vedhara et al., 2000 ) or even a benefit (e.g., Lai et al., 2014 ; Lewis et al., 2008 ; Luethi et al., 2009 ; Vedhara et al., 2000 ). Stress-related hormones, epinephrine and norepinephrine, have been found to relate directly to long-term memory consolidation (Murchison et al., 2004 ; Wiltgen et al., 2004 ), and psychological stress has been found to impair memory performance via interaction with hippocampal function and long-term potentiation (Diamond et al., 1996 ), a potential mechanism for working memory consolidation (Ricker, 2015 ). Additionally, acute pretest stress has been found to attenuate the attentional blink effect (Schwabe & Wolf, 2010 ), though persistent stress, such as an anxious mood state, enhances the attentional blink effect (Jefferies et al., 2008 ). While these findings may provide a reasonable expectation for the effects of stress on working memory consolidation, it has not been directly tested. Further, the effects of stress on consolidation vary depending on the timing of the stress (for review, see Meir Drexler & Wolf, 2017 ). When stress occurs at the beginning of long-term memory consolidation processing (perhaps overlapping with working memory consolidation), it generally enhances overall memory performance (Buchanan & Lovallo, 2001 ; Cahill et al., 2003 ; Joëls et al., 2006 ; Preuß & Wolf, 2009 ; Smeets et al., 2008 ). When stress occurs after initial long-term memory consolidation and before retrieval, it impairs memory performance ( de Quervain et al., 2000 , 2009 ; Kuhlmann et al., 2005 ; Wolf, 2009 ) and is related to reduced activity in the medial temporal lobe, in particular the hippocampus (de Quervain et al., 2003 ; Oei et al., 2007 ). At least one study has found that inhibiting stress-related hormone activity during initial encoding impairs delayed, but not immediate memory (Lupien et al., 2002 ). The amount of stress may also play a role in the effects on memory performance, as small amounts may enhance consolidation while large amounts may impair consolidation (for review, see Joëls et al., 2006 ). Together, these findings suggest a complex relationship between stress, working memory consolidation, and long-term consolidation that future research should explore.
There are several different factors that may result in differences in general consolidation ability. As outlined above, these may be related to different disease states, for example multiple sclerosis and schizophrenia, which both exhibit evidence of working memory and long-term memory impairments. In these diseases, what has traditionally been considered an impairment of long-term memory may instead be the result of earlier working memory consolidation deficits. Consolidation ability may also change in relation to an individual’s emotional state, as stress and anxiety have been shown to interact, both positively and negatively, with working memory consolidation and long-term memory consolidation. A better understanding of these differences may lead to an improved understanding of the underlying processes, as well as avenues for future treatments to improve cognitive symptoms of these and other diseases.
Altering consolidation
If there are population and individual differences in memory consolidation and there is evidence that both types of consolidation affect general memory performance, modulating consolidation is a promising avenue for future research and clinical treatment. A wide variety of experimental manipulations and biological mechanisms have been shown to alter both working memory consolidation (e.g., expectations, Chen et al., 2019 ; task goals, Jolicøeur & Dell’Acqua, 1998 ; or masking, Ricker & Sandry, 2018 ) and long-term memory consolidation (e.g., pharmacological agents, Barsegyan et al., 2010 ; sleep deprivation, Graves et al., 2003 ; electroconvulsive shock, McGaugh, 1966 ). With the understanding that neither working memory nor long-term memory consolidation is a fixed cognitive ability, instead changing in efficiency under a variety of conditions, systematically altering consolidation may be possible and would likely have an impact on memory performance. Some possible methods to alter consolidation are discussed below.
Previous studies have investigated the efficacy of brain training games on cognitive function, with generally mixed results (for reviews, see Kueider et al., 2012 ; Melby-Lervag & Hulme, 2013 ; Rund & Borg, 1999 ; Tetlow & Edwards, 2017 ). While some research has found benefits to global cognitive functions after extensive cognitive training (e.g., Jaeggi et al., 2008 ), several other studies have failed to find evidence of global transfer from the trained skills to general cognitive function (e.g., T. L. Harrison et al., 2013 ). Many of these studies have primarily focused on executive function and general memory ability, without a specific focus on memory consolidation. Previous research has investigated the effect of cognitive training and video games on reducing the attentional blink (Choi et al., 2012 ; Garner et al., 2014 ; R. W. Li et al., 2015 ; but see Tang et al., 2014 ), which may result from working memory consolidation processing. However, these results have not been extended to performance on long-term memory tasks.
A training program that improves the quality or speed of consolidation into working memory through repeated practice may result in improved long-term consolidation and performance. For example, as previously discussed, people may choose to flexibly adopt working memory consolidation as a strategy given certain task conditions (Vergauwe et al., 2019 ). Training participants to opt for consolidation over working memory maintenance may improve their working memory consolidation ability more generally and potentially exhibit long-term memory effects. Given the previously discussed findings that certain patient populations exhibit impairments specifically in consolidation (e.g., working memory consolidation in schizophrenia and long-term memory consolidation in amnesia), a more tailored approach to cognitive training that focuses on improving memory consolidation and focuses on specific populations may be more successful than other cognitive training programs.
Noninvasive brain stimulation presents another possible method to impact working memory consolidation and long-term memory consolidation (for reviews, see Brunoni & Vanderhasselt, 2014 ; Coffman et al., 2014 ; Tremblay et al., 2014 ). Brain stimulation has shown to affect working memory (Andrews et al., 2011 ; Berryhill et al., 2010 ; Fregni et al., 2005 ; Hoy et al., 2013 ; Makovski & Lavidor, 2014 ; Marshall et al., 2005 ; Ohn et al., 2008 ; van Lamsweerde & Johnson, 2017 ; Zaehle et al., 2011 ), although these findings have not always been replicated (Sloan et al., 2020 ; Teo et al., 2011 ). Many of these studies have primarily investigated working memory performance broadly, and so the effects of brain stimulation may be on any working memory component process (i.e., encoding, consolidation, maintenance, or retrieval). At least two studies have specifically interpreted their effects as related to working memory consolidation. As outlined in detail earlier, Makovski and Lavidor ( 2014 ) found that tDCS stimulation led to better working memory performance and van Lamsweerde and Johnson ( 2017 ) found that TMS applied immediately post-stimulus onset resulted in a decrease in the number of items and quality of representations maintained in working memory. While these findings are promising for altering working memory consolidation, more research is necessary to better understand the implications of brain stimuliation for working memory performance.
The effects of brain stimulation on long-term memory consolidation have also been mixed. Several studies have found a benefit to memory performance after tDCS (Flöel et al., 2012 ; Javadi & Walsh, 2012 ; Javadi et al., 2012 ; Manenti et al., 2013 ; Zhao & Woodman, 2020 ). Other studies have failed to find an effect on memory performance in healthy (Chua & Ahmed, 2016 ) and patient populations (e.g., in MS, Rumpf et al., 2018 ). Some research has found benefits of tDCS on sleep-dependent long-term memory consolidation (Marshall et al., 2004 , 2006 ), though other studies have failed to find an effect (Bueno-Lopez et al., 2019 ; Eggert et al., 2013 ; Paßmann et al., 2016 ). This pattern of results may reflect a general long-term memory enhancement from tDCS when applied before or during a learning session (though an effect of tDCS during retrieval was found in Manenti et al., 2013 ), perhaps resulting from enhanced working memory consolidation. When applied post-learning, as in Rumpf et al. ( 2018 ) or Javadi et al. ( 2012 ), working memory consolidation is not enhanced, leading to little effect on long-term memory consolidation. Past research has not specifically examined this question but dissociating between these processes in conjunction with brain stimulation would greatly benefit our understanding both of underlying neural mechanisms as well as each type of consolidation’s role in long-term memory formation.
While the overall benefits of improving memory consolidation are evident, there may be beneficial applications of inhibiting or disrupting memory consolidation, such as in psychiatric treatment for post-traumatic stress disorder (PTSD). Noradrenergic agents (i.e., beta blockers) have been shown to affect working memory and long-term memory (for review, see Chamberlain et al., 2006 ). In particular, propranolol has been shown to impair working memory performance (Frcka & Lader, 1988 ; U. Müller et al., 2005 ) and long-term memory (Cahill et al., 1994 ; Reist et al., 2001 ; van Stegeren et al., 1998 , 2005 ), although at least one study failed to find this effect (O’Carroll et al., 1999 ). Propranolol seems to affect long-term memory performance only pre-encoding, with no effect found with postencoding administration (van Stegeren et al., 2002 ). Propranolol has been explored as a treatment for PTSD with mixed results, when administered shortly after the trauma (Nugent et al., 2010 ; Vaiva et al., 2003 ) or after memory reactivation (Brunet et al., 2008 , 2014 ; McGhee et al., 2009 ; Poundja et al., 2012 ). Given the conflicting findings of these studies, it is difficult to determine the effect of propranolol as a treatment for PTSD. While it has been shown to affect working memory performance generally and seems to specifically impair encoding of long-term memories, the effects of propranolol on working memory consolidation have not been explicitly studied.
Another current PTSD treatment method directly related to memory processing is eye-movement desensitization and reprocessing (EMDR) therapy (e.g., Resick et al., 2008 ). In EMDR therapy, the patient first attempts to retrieve a traumatic memory, before using back-and-forth eye movements to overload working memory capacity and prevent reconsolidation of the memory (Maxfield et al., 2008 ; van den Hout & Engelhard, 2012 ). This memory may then be seen as less vivid and emotional (Andrade et al., 1997 ). Other forms of working memory overload have been shown to have a similar impact on memory reconsolidation (for review, see van den Hout & Engelhard, 2012 ). For example, in one study, participants retrieved negative and positive memories either alone, with horizontal eye movements, or while playing a computer game (Engelhard et al., 2010 ). Both experimental conditions resulted in larger decreases in the emotionality (but not vividness) of negative memories compared with the control condition. For positive memories, the eye movement condition resulted in decreases in emotionality, but not vividness, while the reverse was true for the computer game condition. These findings suggest that the reconsolidation process involves working memory consolidation and impairing it may result in an altered long-term memory. This remains an open question, but a better understanding of the role consolidation plays in memory function may lead to better clinical treatment of trauma-related disorders.
Past research has illustrated several ways that working memory consolidation, long-term memory consolidation, or both may be manipulated. However, the effectiveness of these manipulations is often mixed, and there are few methods that have been explored in both types of consolidation. The ideas described here provide many opportunities for areas of future research to explore, with the aim of better understanding the mechanisms underlying consolidation, as well as potential applications for improved clinical treatments.
The relationship between working memory and long-term memory consolidation
There is extensive evidence suggesting that working memory and long-term memory overlap. Models such as those of Cowan ( 1988 ) and Oberauer ( 2002 ) rely on conceptualizing working memory as an activated state of long-term memory and neuroimaging evidence has supported this claim (D’Esposito & Postle, 2015 ; Jonides et al., 2008 ; Lewis-Peacock & Postle, 2008 ; Ranganath & D’Esposito, 2001 , 2005 ). An open question, and the goal of this review, is to what extent does this relationship extend to the consolidation process. Several computational models incorporate a dual-learning process with a rapid learning network representing a process similar to working memory consolidation and a slower learning mechanism representing a process similar to consolidation into long-term memory (Alvarez & Squire, 1994 ; McClelland et al., 1995 ; Oberauer et al., 2013 ). Both working memory and long-term memory rely on stable memory representations, which are achieved through historically distinct consolidation processes. However, there are two potential ways these consolidation processes may operate in relation with each other. First, a short-term consolidation process may create a stable working memory representation which may be further strengthened during the long-term memory consolidation process or else forgotten. These may be two separate consolidation processes or a single continuous process, but the result is a serial relationship between working memory consolidation and long-term memory consolidation. Second, a working memory representation may be created via working memory consolidation and a long-term memory representation may be created in parallel via a separate long-term memory consolidation process. These representations are used for separate purposes and likely do not overlap with one another. The evidence previously discussed provides different levels of support for each of these hypotheses. A summary of the distinctions between these two hypotheses are presented in Table 1 .
The first hypothesis presents a serial relationship between working memory consolidation and long-term consolidation. According to this hypothesis, a stimulus perception results in an initial sensory memory representation. This sensory memory is then consolidated in working memory, resulting in a more durable working memory representation. Inhibiting working memory consolidation before this stage begins or completes would disrupt long-term consolidation. This working memory representation may be used in ongoing cognition, then either forgotten or further consolidated if long-term memory consolidation has been initiated. The long-term representation created via initial cellular consolidation may then undergo more extensive systems consolidation, resulting in a lasting memory trace. Later reactivation of the memory trace would lead to initiation of working memory consolidation of the new episode and repetition of the whole consolidation process. For example, in the case of episodic memories, reactivation of the memory representation occurs when one thinks back to the episode, either spontaneously or due to targeted reactivation. Bringing the episodic memory to the focus of attention initiates the working memory-long-term memory consolidation process of this “new” episode. With frequent reactivations and consolidation opportunities, these memories may become more generalized learning.
There are various pieces of evidence and potential gaps, both described in this paper, in support of this hypothesis. First, a clear effect of working memory consolidation on subsequent long-term memory consolidation would need to be established. This would entail evidence of poor long-term memory when working memory consolidation does not occur as well as improved long-term memory with increased working memory consolidation. Some research has explored this in healthy populations (e.g., Cotton & Ricker, 2021 ; Jarjat et al., 2018 , 2020 ; Souza & Oberauer, 2017 ), however clinical research in MS, schizophrenia, and PTSD provides further support. All three groups exhibit impairments in both working memory consolidation (e.g., a longer attentional blink, Cheung et al., 2002 ; Kavcic & Scheid, 2011 ; or slowed working memory consolidation, Fuller et al., 2005 ) and long-term memory (Baran et al., 2018 ; Kiy et al., 2011 ; Manoach & Stickgold, 2009 ; Nguemeni et al., 2021 ; Sandry et al., 2019 ), as discussed in earlier sections, suggesting a strong relationship between the two processes.
EMDR therapy demonstrates the effect of overloading working memory processing, and perhaps prevention of working memory consolidation, on long-term memory consolidation of traumatic memories (Maxfield et al., 2008 ; van den Hout & Engelhard, 2012 ). The use of pharmacological agents, such as beta blockers, have been shown to impair long-term memory consolidation, but only when introduced pre-encoding, not postencoding (Cahill et al., 1994 ; Reist et al., 2001 ; van Stegeren et al., 1998 , 2002 , 2005 ). Together with evidence that beta blockers also impair working memory (Frcka & Lader, 1988 ; U. Müller et al., 2005 ), this suggests that the initial working memory consolidation is critical for later long-term memory consolidation. This may be similar to the role of brain stimulation, which is mostly effective when applied before learning, but not after (e.g., Flöel et al., 2012 ; Javadi & Walsh, 2012 ; Javadi et al., 2012 ; Manenti et al., 2013 ; Zhao & Woodman, 2020 ).
The second piece of support for this hypothesis can be found in the regions involved in each consolidation process. To support the serial relationship hypothesis, the regions involved in working memory consolidation should overlap with the regions involved in early long-term memory consolidation, or there should be connections between them. The regions involved in working memory consolidation primarily include the dorsolateral prefrontal cortex, posterior parietal cortex, and sensory cortices (e.g., occipital lobe in visual working memory), as discussed previously. Conversely, the regions involved in long-term memory consolidation primarily include areas of medial temporal lobe, in particular the hippocampus, and the prefrontal cortex. While the role of the hippocampus has not been explicitly explored in working memory consolidation, there is evidence that hippocampal activity is influenced by attentional processes during initial memory processing (Aly & Turk-Browne, 2015 , 2016 ) and that activity in the hippocampus and surrounding areas during working memory maintenance affects subsequent long-term memory (Ranganath et al., 2005 ; Schon et al., 2004 ; for review, see Leszczynski, 2011 ). In MS, hippocampal damage is associated with poor memory and consolidation (Kiy et al., 2011 ; Rocca et al., 2018 ), potentially illustrating an overlap between consolidation processes in the hippocampus. Regions of the prefrontal cortex have been implicated in both types of consolidation and activity in dorsolateral prefrontal cortex during working memory maintenance (Ranganath et al., 2005 ) and manipulation (Blumenfeld & Ranganath, 2006 ) has been associated with long-term memory. As discussed previously, Cohen et al. ( 2015 ) identified immediate postencoding activity in the posterior parietal cortex which correlated with long-term memory performance, suggesting a potential link between posterior parietal activity during working memory consolidation and subsequent long-term memory. This is consistent with research suggesting that the parietal region is involved in representing memory features in both working memory and long-term memory (Brodt et al., 2016 ; Ester et al., 2009 ). In a broader view of overlapping physiology, electroencephalography alpha, gamma, and theta oscillations during working memory processing and long-term encoding have been linked to successful long-term memory (Khader et al., 2010 ; Sederberg et al., 2003 ). Together, these results suggest several regions that may be involved in the overlap between working memory consolidation and long-term consolidation.
The third piece of evidence that would support the serial relationship hypothesis is non-overlapping time courses of the consolidation processes. In other words, working memory consolidation would need to initiate and terminate before long-term memory consolidation begins. There is extensive evidence that working memory consolidation takes at most a few seconds (Nieuwenstein et al., 2009 ; Ricker & Hardman, 2017 ; Stevanovski & Jolicøeur, 2007 ; Vogel et al., 2006 ; Wyble et al., 2011 ), while even early long-term memory consolidation takes minutes (Dudai, 2004 ). While there is seemingly plentiful evidence to support the serial relationship hypothesis, many of these interpretations have not been directly tested.
The second hypothesis proposes a parallel relationship between working memory consolidation and long-term memory consolidation. According to this hypothesis, a stimulus undergoes initial encoding that results in a sensory memory representation. Both working memory consolidation and long-term memory consolidation are then initiated simultaneously. This results in multiple representations (one in working memory and one in long-term memory) that serve different purposes. The working memory representation may be used in ongoing cognition and eventually forgotten. Meanwhile, long-term memory consolidation processes are ongoing, ultimately resulting in a stable long-term memory that may be different from the working memory representation, based on subsequent processing. As long-term memory consolidation continues, the representation may lose contextual detail and become more generalized, resulting in a qualitatively different representation than what was initially encoded.
As with the serial relationship hypothesis, this review has described both supporting evidence and open questions for this parallel relationship hypothesis. First, the parallel processes would likely need to occur in separate regions and with little connection between the regions. As mentioned previously, working memory consolidation may primarily occur in areas such as the dorsolateral prefrontal cortex, posterior parietal cortex, and occipital lobe, while initial long-term memory consolidation primarily depends on medial temporal regions. One of the areas most likely to link both types of consolidation, the hippocampus, has not been directly tested in working memory consolidation. These findings potentially suggest that these processes occur in distinct regions.
Second, the parallel relationship hypothesis posits at least two distinct representations stabilized via separate consolidation processes and that manipulating one type of consolidation has no effect on the other type of consolidation. A targeted enhancement or inhibition of working memory consolidation should have no impact on the long-term memory representation and improvement, or suppression of only long-term memory consolidation should not impact working memory performance. For example, removal of the hippocampus led to impaired long-term memory consolidation in patients like H.M. (Scoville & Milner, 1957 ), though his working memory performance remained relatively intact (Milner et al., 1968 ; Squire & Wixted, 2011 ). Additional evidence can be found in the various brain training programs which may improve working memory consolidation (evidenced by reducing the attentional blink, e.g., Choi et al., 2012 ; Garner et al., 2014 ; R. W. Li et al., 2015 ; but see Tang et al., 2014 ), but seemingly have little transfer to general cognitive abilities, including long-term memory (e.g., T. L. Harrison et al., 2013 ). Similarly, brain stimulation has been shown to improve working memory (and specifically working memory consolidation, e.g., Makovski & Lavidor, 2014 ; van Lamsweerde & Johnson, 2017 ), with mixed effects on long-term memory (for review, see Coffman et al., 2014 ). While these studies did not directly test the existence of distinct memory representations in working memory and long-term memory, they do suggest that manipulation of one consolidation process may not influence the other.
There is substantial and conflicting evidence in support of both the serial relationship hypothesis and the parallel relationship hypothesis, and it remains unclear exactly how the different consolidation processes relate. While there is little research directly investigating both working memory consolidation and long-term consolidation within the same paradigm, future research should focus on this interrelationship. For example, a paradigm may first manipulate time allowed for consolidation into working memory and test retrieval the following day, allowing for sufficient long-term consolidation to occur. If working memory consolidation is critical for the initiation of long-term consolidation, then later memory will be worse for items that were not able to be consolidated fully. Other paradigms may opt to investigate how the different factors that affect working memory consolidation addressed in this review (e.g., stress) affect performance on both working memory and long-term memory tasks. By focusing on the many open questions presented in this review, future research will clarify this relationship and provide a better understanding of memory consolidation.
The goal of this review was to outline working memory consolidation and long-term memory consolidation, in order to determine how these processes relate to each other. Clarifying this relationship would both strengthen our understanding of the human memory system and provide potential clinical applications. Identifying how working memory consolidation and long-term memory consolidation may or may not interact with each other has important implications on clinical treatment for patients with memory impairments, such as those with schizophrenia, amnesia, multiple sclerosis, and post-traumatic stress disorder. Such treatments may include enhancing consolidation abilities through cognitive training or electrical stimulation or inhibiting consolidation pharmacologically or with EMDR therapy.
In summary, working memory consolidation and long-term memory consolidation have historically been investigated as distinct processes in separate fields of research; however, these may be related, if not overlapping, constructs. Unfortunately, little research has explicitly investigated this question, leading to two equally possible conceptualizations of the relationship. Future research is needed that specifically tests each of these hypotheses and further delineates the underlying relationship between working memory consolidation and long-term memory consolidation. As the recent interest in the potential overlaps between working memory and long-term memory expand, there is a growing need for a more refined and specific terminology for the several conceptually related processes including encoding, consolidation, refreshing, and other maintenance processes. The present review provides a framework for considering the separation and relationships between these processes.
Alvarez, P., & Squire, L. R. (1994). Memory consolidation and the medial temporal lobe: A simple network model. Proceedings of the National Academy of Sciences , 91 (15), 7041–7045. 10/bh9hkr
Aly, M., & Turk-Browne, N. B. (2015). Attention stabilizes representations in the human hippocampus. Cerebral Cortex, 26 (4), 783–796 . 10/gdz7v6
Aly, M., & Turk-Browne, N. B. (2016). Attention promotes episodic encoding by stabilizing hippocampal representations. Proceedings of the National Academy of Sciences , 113 (4), E420–E429. 10/f77rhz
Andrade, J., Kavanagh, D., & Baddeley, A. (1997). Eye-movements and visual imagery: A working memory approach to the treatment of post-traumatic stress disorder. British Journal of Clinical Psychology , 36 (2), 209–223. 10/fh2tdh
Andrews, S. C., Hoy, K. E., Enticott, P. G., Daskalakis, Z. J., & Fitzgerald, P. B. (2011). Improving working memory: The effect of combining cognitive activity and anodal transcranial direct current stimulation to the left dorsolateral prefrontal cortex. Brain Stimulation , 4 (2), 84–89. 10/bcvcct
Arnell, K. M. (2006). Visual, auditory, and cross-modality dual-task costs: Electrophysiological evidence for an amodal bottleneck on working memory consolidation. Perception & Psychophysics , 68 (3), 447–457. 10/dppsvq
Arshavsky, Y. I. (2006). “The seven sins” of the Hebbian synapse: Can the hypothesis of synaptic plasticity explain long-term memory consolidation? Progress in Neurobiology , 80 (3), 99–113. 10/b7j97d
Atkinson, R. C., & Shiffrin, R. M. (1968). Human memory: A proposed system and its control processes. In K. Spence & J. T. Spence (Eds.), Psychology of learning and motivation (Vol. 2, pp. 89–195). Elsevier.
Baddeley, A. (2000). The episodic buffer: A new component of working memory? Trends in Cognitive Sciences, 4 (11), 417–423. https://doi.org/10.1016/S1364-6613(00)01538-2
Baddeley, A., & Hitch, G. (1974). Working memory. In Psychology of learning and motivation (vol. 8, pp. 47–89). Elsevier.
Baddeley, A. D., Thomson, N., & Buchanan, M. (1975). Word length and the structure of short-term memory. Journal of Verbal Learning and Verbal Behavior , 14 (6), 575–589. 10/b97v4v
Bailey, C. H., Kandel, E. R., & Harris, K. M. (2015). Structural Components of Synaptic Plasticity and Memory Consolidation. Cold Spring Harbor Perspectives in Biology , 7 (7), a021758. 10/ggrbm2
Baran, B., Correll, D., Vuper, T. C., Morgan, A., Durrant, S. J., Manoach, D. S., & Stickgold, R. (2018). Spared and impaired sleep-dependent memory consolidation in schizophrenia. Schizophrenia Research , 199 , 83–89. 10/gfcqcd
Barsegyan, A., Mackenzie, S. M., Kurose, B. D., McGaugh, J. L., & Roozendaal, B. (2010). Glucocorticoids in the prefrontal cortex enhance memory consolidation and impair working memory by a common neural mechanism. Proceedings of the National Academy of Sciences, 107 (38), 16655–16660. https://doi.org/10.1073/pnas.1011975107
Article Google Scholar
Bartsch, L. M., Loaiza, V. M., Jäncke, L., Oberauer, K., & Lewis-Peacock, J. A. (2019). Dissociating refreshing and elaboration and their impacts on memory. NeuroImage , 199 , 585–597. 10/gf7tn5
Bartsch, L. M., & Oberauer, K. (2021). The effects of elaboration on working memory and long-term memory across age. Journal of Memory and Language , 118 , 104215. 10/gjrq8r
Bartsch, L. M., Singmann, H., & Oberauer, K. (2018). The effects of refreshing and elaboration on working memory performance, and their contributions to long-term memory formation. Memory & Cognition , 46 (5), 796–808. 10/gd3vsh
Bayliss, D. M., Bogdanovs, J., & Jarrold, C. (2015). Consolidating working memory: Distinguishing the effects of consolidation, rehearsal and attentional refreshing in a working memory span task. Journal of Memory and Language, 81 , 34–50. https://doi.org/10.1016/j.jml.2014.12.004
Ben-Yakov, A., & Dudai, Y. (2011). Constructing realistic engrams: Poststimulus activity of hippocampus and dorsal striatum predicts subsequent episodic memory. Journal of Neuroscience , 31 (24), 9032–9042. 10/ddwb63
Berryhill, M. E., Wencil, E. B., Branch Coslett, H., & Olson, I. R. (2010). A selective working memory impairment after transcranial direct current stimulation to the right parietal lobe. Neuroscience Letters , 479 (3), 312–316. 10/fm6hbj
Blake, R. V., Wroe, S. J., Breen, E. K., & McCarthy, R. A. (2000). Accelerated forgetting in patients with epilepsy: Evidence for an impairment in memory consolidation. Brain , 123 (3), 472–483. 10/b23zhw
Blumenfeld, R. S., & Ranganath, C. (2006). Dorsolateral prefrontal cortex promotes long-term memory formation through its role in working memory organization. Journal of Neuroscience , 26 (3), 916–925. 10/bbrzp9
Bontempi, B., Laurent-Demir, C., Destrade, C., & Jaffard, R. (1999). Time-dependent reorganization of brain circuitry underlying long-term memory storage. Nature , 400 (6745), 671–675. 10/bv4gm3
Borders, A. A., Ranganath, C., & Yonelinas, A. P. (2021). The hippocampus supports high-precision binding in visual working memory. Hippocampus, 32 (3), 217–230. 10/gnw7hf
Bowman, H., & Wyble, B. (2007). The simultaneous type, serial token model of temporal attention and working memory. Psychological Review , 114 (1), 38. 10/fchwp5
Bradshaw, G. L., & Anderson, J. R. (1982). Elaborative encoding as an explanation of levels of processing. Journal of Verbal Learning and Verbal Behavior , 21 (2), 165–174. 10/b76z29
Broadbent, D. E., & Broadbent, M. H. P. (1987). From detection to identification: Response to multiple targets in rapid serial visual presentation. Perception & Psychophysics , 42 (2), 105–113. 10/ff45pz
Brodt, S., Gais, S., Beck, J., Erb, M., Scheffler, K., & Schönauer, M. (2018). Fast track to the neocortex: A memory engram in the posterior parietal cortex. Science . https://www.science.org/doi/abs/10.1126/science.aau2528
Brodt, S., Pöhlchen, D., Flanagin, V. L., Glasauer, S., Gais, S., & Schönauer, M. (2016). Rapid and independent memory formation in the parietal cortex. Proceedings of the National Academy of Sciences , 113 (46), 13251–13256. 10/f9f6js
Brunet, A., Orr, S. P., Tremblay, J., Robertson, K., Nader, K., & Pitman, R. K. (2008). Effect of post-retrieval propranolol on psychophysiologic responding during subsequent script-driven traumatic imagery in post-traumatic stress disorder. Journal of Psychiatric Research , 42 (6), 503–506. 10/d2ktjd
Brunet, A., Thomas, É., Saumier, D., Ashbaugh, A. R., Azzoug, A., Pitman, R. K., Orr, S. P., & Tremblay, J. (2014). Trauma Reactivation Plus Propranolol is Associated with Durably Low Physiological Responding during Subsequent Script-Driven Traumatic Imagery. The Canadian Journal of Psychiatry, 59 (4), 228–232. https://doi.org/10.1177/070674371405900408
Article PubMed Google Scholar
Brunoni, A. R., & Vanderhasselt, M.-A. (2014). Working memory improvement with non-invasive brain stimulation of the dorsolateral prefrontal cortex: A systematic review and meta-analysis. Brain and Cognition, 86 , 1–9. https://doi.org/10.1016/j.bandc.2014.01.008
Buchanan, T. W., & Lovallo, W. R. (2001). Enhanced memory for emotional material following stress-level cortisol treatment in humans. Psychoneuroendocrinology , 26 (3), 307–317. 10/fd8dph
Bueno-Lopez, A., Eggert, T., Dorn, H., & Danker-Hopfe, H. (2019). Slow oscillatory transcranial direct current stimulation (so-tDCS) during slow wave sleep has no effects on declarative memory in healthy young subjects. Brain Stimulation , 12 (4), 948–958. 10/gf95gd
Cahill, L., Gorski, L., & Le, K. (2003). Enhanced human memory consolidation with post-learning stress: Interaction with the degree of arousal at encoding. Learning & Memory , 10 (4), 270–274. 10/d4gnpr
Cahill, L., Prins, B., Weber, M., & McGaugh, J. L. (1994). β-Adrenergic activation and memory for emotional events. Nature , 371 (6499), 702–704. 10/ckqp8x
Camos, V., Johnson, M., Loaiza, V., Portrat, S., Souza, A., & Vergauwe, E. (2018). What is attentional refreshing in working memory? Annals of the New York Academy of Sciences , 1424 (1), 19–32. 10/gd59v4
Carr, M. F., Jadhav, S. P., & Frank, L. M. (2011). Hippocampal replay in the awake state: A potential substrate for memory consolidation and retrieval. Nature Neuroscience , 14 (2), 147–153. 10/dbhjzd
Chamberlain, S. R., Müller, U., Blackwell, A. D., Robbins, T. W., & Sahakian, B. J. (2006). Noradrenergic modulation of working memory and emotional memory in humans. Psychopharmacology , 188 (4), 397–407. 10/dv2d7v
Chen, H., Yan, N., Zhu, P., Wyble, B., Eitam, B., & Shen, M. (2019). Expecting the unexpected: Violation of expectation shifts strategies toward information exploration. Journal of Experimental Psychology. Human Perception and Performance , 45 (4), 513–522. 10/gf4ncp
Cheng, S. (2017). Consolidation of episodic memory: An epiphenomenon of semantic learning. In N. Axmacher & B. Rasch (Eds.), Cognitive neuroscience of memory consolidation (pp. 57–72). Springer.
Chapter Google Scholar
Cheung, V., Chen, E. Y. H., Chen, R. Y. L., Woo, M. F., & Yee, B. (2002). A comparison between schizophrenia patients and healthy controls on the expression of attentional blink in a rapid serial visual presentation (RSVP) paradigm. Schizophrenia Bulletin , 28 (3), 443–458. 10/fzpw7q
Chiaravalloti, N. D., & DeLuca, J. (2008). Cognitive impairment in multiple sclerosis. The Lancet Neurology , 7 (12), 1139–1151. 10/bb5ps3
Choi, H., Chang, L.-H., Shibata, K., Sasaki, Y., & Watanabe, T. (2012). Resetting capacity limitations revealed by long-lasting elimination of attentional blink through training. Proceedings of the National Academy of Sciences , 109 (30), 12242–12247. 10/f36gb2
Chua, E. F., & Ahmed, R. (2016). Electrical stimulation of the dorsolateral prefrontal cortex improves memory monitoring. Neuropsychologia , 85 , 74–79. 10/f8pb87
Chun, M. M., & Potter, M. C. (1995). A two-stage model for multiple target detection in rapid serial visual presentation. Journal of Experimental Psychology: Human Perception and Performance , 21 (1), 109. 10/dpvqvw
Coffman, B. A., Clark, V. P., & Parasuraman, R. (2014). Battery powered thought: Enhancement of attention, learning, and memory in healthy adults using transcranial direct current stimulation. NeuroImage , 85 , 895–908. 10/f5mk96
Cohen, N., Pell, L., Edelson, M. G., Ben-Yakov, A., Pine, A., & Dudai, Y. (2015). Peri-encoding predictors of memory encoding and consolidation. Neuroscience & Biobehavioral Reviews , 50 , 128–142. 10/f67qcx
Coltheart, M. (1980). Iconic memory and visible persistence. Perception & Psychophysics , 27 (3), 183–228. 10/ddjrs9
Cotton, K., & Ricker, T. J. (2021). Working memory consolidation improves long-term memory recognition. Journal of Experimental Psychology: Learning, Memory, and Cognition , 47 (2), 208–219. 10/gg89v8
Courtney, S. M., Ungerleider, L. G., Keil, K., & Haxby, J. V. (1997). Transient and sustained activity in a distributed neural system for human working memory. Nature , 386 (6625), 608–611. 10/dprpwp
Cowan, N. (1984). On short and long auditory stores. Psychological Bulletin , 96 (2), 341. 10/d3gmgw
Cowan, N. (1988). Evolving conceptions of memory storage, selective attention, and their mutual constraints within the human information-processing system. Psychological Bulletin , 104 (2), 163. 10/dxmphk
Cowan, N. (2001). The magical number 4 in short-term memory: A reconsideration of mental storage capacity. The Behavioral and Brain Sciences , 24 (1), 87–114; discussion 114–85. 10/ddq83h
Craik, F. I. M., Govoni, R., Naveh-Benjamin, M., & Anderson, N. D. (1996). The effects of divided attention on encoding and retrieval processes in human memory. Journal of Experimental Psychology: General , 125 (2), 159. 10/dcfddg
Craik, F. I. M., & Tulving, E. (1975). Depth of processing and the retention of words in episodic memory. Journal of Experimental Psychology: General , 104 (3), 268–294. Scopus. 10/c89jv7
Curtis, C. E., Rao, V. Y., & D’Esposito, M. (2004). Maintenance of spatial and motor codes during oculomotor delayed response tasks. Journal of Neuroscience , 24 (16), 3944–3952. 10/fs74wg
de Quervain, D. J.-F., Aerni, A., Schelling, G., & Roozendaal, B. (2009). Glucocorticoids and the regulation of memory in health and disease. Frontiers in Neuroendocrinology , 30 (3), 358–370. 10/b223qb
de Quervain, D. J.-F. Henke, K., Aerni, A., Treyer, V., McGaugh, J. L., Berthold, T., Nitsch, R. M., Buck, A., Roozendaal, B., & Hock, C. (2003). Glucocorticoid-induced impairment of declarative memory retrieval is associated with reduced blood flow in the medial temporal lobe. European Journal of Neuroscience , 17 (6), 1296–1302. 10/drqmzr
de Quervain, D. J.-F., Roozendaal, B., Nitsch, R. M., McGaugh, J. L., & Hock, C. (2000). Acute cortisone administration impairs retrieval of long-term declarative memory in humans. Nature Neuroscience , 3 (4), 313–314. 10/fn48kt
Deiber, M.-P., Missonnier, P., Bertrand, O., Gold, G., Fazio-Costa, L., Ibanez, V., & Giannakopoulos, P. (2007). Distinction between perceptual and attentional processing in working memory tasks: A study of phase-locked and induced oscillatory brain dynamics. Journal of Cognitive Neuroscience , 19 (1), 158–172. 10/brz4dw
DeLuca, J., Gaudino, E. A., Diamond, B. J., Christodoulou, C., & Engel, R. A. (1998). Acquisition and Storage Deficits in Multiple Sclerosis. Journal of Clinical and Experimental Neuropsychology , 20 (3), 376–390. 10/c3vvbr
D’Esposito, M., & Postle, B. R. (2015). The cognitive neuroscience of working memory. Annual Review of Psychology , 66 . 10/gc96sj
Diamond, D. M., Fleshner, M., Ingersoll, N., & Rose, G. (1996). Psychological stress impairs spatial working memory: Relevance to electrophysiological studies of hippocampal function. Behavioral Neuroscience , 110 (4), 661–672. 10/fb8mdw
Dresler, M., Genzel, L., Kluge, M., Schüssler, P., Weber, F., Rosenhagen, M., & Steiger, A. (2010). Off-line memory consolidation impairments in multiple sclerosis patients receiving high-dose corticosteroid treatment mirror consolidation impairments in depression. Psychoneuroendocrinology , 35 (8), 1194–1202. 10/cv9ksd
Dudai, Y. (2004). The Neurobiology of Consolidations, Or, How Stable is the Engram? Annual Review of Psychology , 55 (1), 51–86. 10/dkv4fk
Dudai, Y., Karni, A., & Born, J. (2015). The Consolidation and Transformation of Memory. Neuron , 88 (1), 20–32. 10/gfc669
Ecker, U. K. H., Lewandowsky, S., & Oberauer, K. (2014a). Removal of information from working memory: A specific updating process. Journal of Memory and Language , 74 , 77–90. 10/ggrw2k
Ecker, U. K. H., Oberauer, K., & Lewandowsky, S. (2014b). Working memory updating involves item-specific removal. Journal of Memory and Language , 74 , 1–15. 10/gd3vs9
Eggert, T., Dorn, H., Sauter, C., Nitsche, M. A., Bajbouj, M., & Danker-Hopfe, H. (2013). No effects of slow oscillatory transcranial direct current stimulation (tDCS) on sleep-dependent memory consolidation in healthy elderly subjects. Brain Stimulation , 6 (6), 938–945. 10/f2ns2c
Elman, J. A., Rosner, Z. A., Cohn-Sheehy, B. I., Cerreta, A. G., & Shimamura, A. P. (2013). Dynamic changes in parietal activation during encoding: Implications for human learning and memory. NeuroImage , 82 , 44–52. 10/f498bf
Emrich, S. M., Riggall, A. C., LaRocque, J. J., & Postle, B. R. (2013). Distributed patterns of activity in sensory cortex reflect the precision of multiple items maintained in visual short-term memory. Journal of Neuroscience , 33 (15), 6516–6523. 10/f4trdr
Engelhard, I. M., van Uijen, S. L., & van den Hout, M. A. (2010). The impact of taxing working memory on negative and positive memories. European Journal of Psychotraumatology, 1 (1), 5623. https://doi.org/10.3402/ejpt.v1i0.5623
Ester, E. F., Serences, J. T., & Awh, E. (2009). Spatially global representations in human primary visual cortex during working memory maintenance. Journal of Neuroscience , 29 (48), 15258–15265. 10/dgp983
Ester, E. F., Sprague, T. C., & Serences, J. T. (2015). Parietal and frontal cortex encode stimulus-specific mnemonic representations during visual working memory. Neuron , 87 (4), 893–905. 10/f7qh69
Evensmoen, H. R., Ladstein, J., Hansen, T. I., Møller, J. A., Witter, M. P., Nadel, L., & Håberg, A. K. (2015). From details to large scale: The representation of environmental positions follows a granularity gradient along the human hippocampal and entorhinal anterior–posterior axis. Hippocampus , 25 (1), 119–135. 10/f6s55x
Figueira, J. S. B., Oliveira, L., Pereira, M. G., Pacheco, L. B., Lobo, I., Motta-Ribeiro, G. C., & David, I. A. (2017). An unpleasant emotional state reduces working memory capacity: Electrophysiological evidence. Social Cognitive and Affective Neuroscience , 12 (6), 984–992. 10/f9zrwj
Flöel, A., Suttorp, W., Kohl, O., Kürten, J., Lohmann, H., Breitenstein, C., & Knecht, S. (2012). Non-invasive brain stimulation improves object-location learning in the elderly. Neurobiology of Aging , 33 (8), 1682–1689. 10/djwdxw
Frankland, P. W., & Bontempi, B. (2005). The organization of recent and remote memories. Nature Reviews Neuroscience , 6 (2), 119–130. 10/dzhpr2
Frcka, G., & Lader, M. (1988). Psychotropic effects of repeated doses of enalapril, propranolol and atenolol in normal subjects. British Journal of Clinical Pharmacology , 25 (1), 67–73. 10/gh23x5
Fregni, F., Boggio, P. S., Nitsche, M., Bermpohl, F., Antal, A., Feredoes, E., Marcolin, M. A., Rigonatti, S. P., Silva, M. T. A., Paulus, W., & Pascual-Leone, A. (2005). Anodal transcranial direct current stimulation of prefrontal cortex enhances working memory. Experimental Brain Research , 166 (1), 23–30. 10/c478n2
Fuller, R. L., Luck, S. J., Braun, E. L., Robinson, B. M., McMahon, R. P., & Gold, J. M. (2009). Impaired visual working memory consolidation in schizophrenia. Neuropsychology , 23 (1), 71–80. 10/bktxrh
Fuller, R. L., Luck, S. J., McMahon, R. P., & Gold, J. M. (2005). Working memory consolidation is abnormally slow in schizophrenia. Journal of Abnormal Psychology, 114 (2), 279. https://doi.org/10.1037/0021-843X.114.2.279
Funahashi, S., Bruce, C. J., & Goldman-Rakic, P. S. (1989). Mnemonic coding of visual space in the monkey’s dorsolateral prefrontal cortex. Journal of Neurophysiology , 61 (2), 331–349. 10/ggcg98
Fuster, J. M. (1973). Unit activity in prefrontal cortex during delayed-response performance: Neuronal correlates of transient memory. Journal of Neurophysiology , 36 (1), 61–78. 10/ggbv6s
Fuster, J. M., & Alexander, G. E. (1971). Neuron activity related to short-term memory. Science , 173 (3997), 652–654. 10/b324t9
Garner, K. G., Tombu, M. N., & Dux, P. E. (2014). The influence of training on the attentional blink and psychological refractory period. Attention, Perception, & Psychophysics , 76 (4), 979–999. 10/f6d4vg
Genzel, L., Dresler, M., Cornu, M., Jäger, E., Konrad, B., Adamczyk, M., Friess, E., Steiger, A., Czisch, M., & Goya-Maldonado, R. (2015). Medial prefrontal-hippocampal connectivity and motor memory consolidation in depression and schizophrenia. Biological Psychiatry , 77 (2), 177–186. 10/f6s67p
Genzel, L., & Wixted, J. T. (2017). Cellular and systems consolidation of declarative memory. In N. Axmacher & B. Rasch (Eds.), Cognitive neuroscience of memory consolidation (pp. 3–16). Springer.
Gisquet-Verrier, P., Lynch, J. F., Cutolo, P., Toledano, D., Ulmen, A., Jasnow, A. M., & Riccio, D. C. (2015). Integration of new information with active memory accounts for retrograde amnesia: A challenge to the consolidation/reconsolidation hypothesis? Journal of Neuroscience , 35 (33), 11623–11633. 10/f7tfqt
Goldman-Rakic, P. S. (1991). Cellular and circuit basis of working memory in prefrontal cortex of nonhuman primates. Progress in Brain Research, 85 , 325–336.
Graves, L. A., Heller, E. A., Pack, A. I., & Abel, T. (2003). Sleep deprivation selectively impairs memory consolidation for contextual fear conditioning. Learning & Memory , 10 (3), 168–176. 10/bnhcpq
Hahn, B., Robinson, B. M., Leonard, C. J., Luck, S. J., & Gold, J. M. (2018). Posterior parietal cortex dysfunction is central to working memory storage and broad cognitive deficits in schizophrenia. Journal of Neuroscience , 38 (39), 8378–8387. 10/gfcqpb
Harand, C., Bertran, F., La Joie, R., Landeau, B., Mézenge, F., Desgranges, B., Peigneux, P., Eustache, F., & Rauchs, G. (2012). The hippocampus remains activated over the long term for the retrieval of truly episodic memories. PLOS ONE , 7 (8), e43495. 10/f36t35
Harrison, S. A., & Tong, F. (2009). Decoding reveals the contents of visual working memory in early visual areas. Nature , 458 (7238), 632–635. 10/fk2ccg
Harrison, T. L., Shipstead, Z., Hicks, K. L., Hambrick, D. Z., Redick, T. S., & Engle, R. W. (2013). Working Memory Training May Increase Working Memory Capacity but Not Fluid Intelligence. Psychological Science , 24 (12), 2409–2419. 10/f5krbf
Hartshorne, J. K., & Makovski, T. (2019). The effect of working memory maintenance on long-term memory. Memory & Cognition , 47 (4), 749–763. 10/gf2tz2
Hebb, D. O. (1949). The organization of behavior; a neuropsychological theory (pp. xix, 335). Wiley.
Himmer, L., Schönauer, M., Heib, D. P. J., Schabus, M., & Gais, S. (2019). Rehearsal initiates systems memory consolidation, sleep makes it last. Science Advances , 5 (4), eaav1695. 10/f9j5
Hoy, K. E., Emonson, M. R. L., Arnold, S. L., Thomson, R. H., Daskalakis, Z. J., & Fitzgerald, P. B. (2013). Testing the limits: Investigating the effect of tDCS dose on working memory enhancement in healthy controls. Neuropsychologia , 51 (9), 1777–1784. 10/f46n2n
Jaeggi, S. M., Buschkuehl, M., Jonides, J., & Perrig, W. J. (2008). Improving fluid intelligence with training on working memory. Proceedings of the National Academy of Sciences , 105 (19), 6829–6833. 10/cx2vf8
Jarjat, G., Hoareau, V., Plancher, G., Hot, P., Lemaire, B., & Portrat, S. (2018). What makes working memory traces stable over time? Annals of the New York Academy of Sciences , 1424 (1), 149–160. 10/gd59vr
Jarjat, G., Plancher, G., & Portrat, S. (2020). Core mechanisms underlying the long-term stability of working memory traces still work in aging. LAnnee Psychologique , 120 (2), 203–229. 10/gn2x7w
Javadi, A. H., Cheng, P., & Walsh, V. (2012). Short duration transcranial direct current stimulation (tDCS) modulates verbal memory. Brain Stimulation , 5 (4), 468–474. 10/bqcsrv
Javadi, A. H., & Walsh, V. (2012). Transcranial direct current stimulation (tDCS) of the left dorsolateral prefrontal cortex modulates declarative memory. Brain Stimulation , 5 (3), 231–241. 10/dpj2kp
Jefferies, L. N., Smilek, D., Eich, E., & Enns, J. T. (2008). Emotional valence and arousal interact in attentional control. Psychological Science , 19 (3), 290–295. 10/d92wc6
Jiang, X., Shamie, I., K. Doyle, W., Friedman, D., Dugan, P., Devinsky, O., Eskandar, E., Cash, S. S., Thesen, T., & Halgren, E. (2017). Replay of large-scale spatio-temporal patterns from waking during subsequent NREM sleep in human cortex. Scientific Reports , 7 (1), 1–17. 10/ggt62w
Joëls, M., Pu, Z., Wiegert, O., Oitzl, M. S., & Krugers, H. J. (2006). Learning under stress: How does it work? Trends in Cognitive Sciences , 10 (4), 152–158. 10/cjnrh6
Johnen, A., Landmeyer, N. C., Bürkner, P.-C., Wiendl, H., Meuth, S. G., & Holling, H. (2017). Distinct cognitive impairments in different disease courses of multiple sclerosis—A systematic review and meta-analysis. Neuroscience & Biobehavioral Reviews , 83 , 568–578. 10/gct78j
Johnson, M. K., Raye, C. L., Mitchell, K. J., Greene, E. J., Cunningham, W. A., & Sanislow, C. A. (2005). Using fMRI to investigate a component process of reflection: Prefrontal correlates of refreshing a just-activated representation. Cognitive, Affective, & Behavioral Neuroscience , 5 (3), 339–361. 10/ch6d7z
Jolicøeur, P., & Dell’Acqua, R. (1998). The demonstration of short-term consolidation. Cognitive Psychology, 36 (2), 138–202. https://doi.org/10.1006/cogp.1998.0684
Jonides, J., Lewis, R. L., Nee, D. E., Lustig, C. A., Berman, M. G., & Moore, K. S. (2008). The mind and brain of short-term memory. Annual Review of Psychology, 59 (1), 193–224. https://doi.org/10.1146/annurev.psych.59.103006.093615
Article PubMed PubMed Central Google Scholar
Kandel, E. R., Dudai, Y., & Mayford, M. R. (2014). The molecular and systems biology of memory. Cell , 157 (1), 163–186. 10/f5xmt3
Kavcic, V., & Scheid, E. (2011). Attentional blink in patients with multiple sclerosis. Neuropsychologia , 49 (3), 454–460. 10/dd6c83
Khader, P. H., Jost, K., Ranganath, C., & Rösler, F. (2010). Theta and alpha oscillations during working-memory maintenance predict successful long-term memory encoding. Neuroscience Letters , 468 (3), 339–343. 10/b2x4tv
Kiy, G., Lehmann, P., Hahn, H. K., Eling, P., Kastrup, A., & Hildebrandt, H. (2011). Decreased hippocampal volume, indirectly measured, is associated with depressive symptoms and consolidation deficits in multiple sclerosis. Multiple Sclerosis Journal , 17 (9), 1088–1097. 10/bzc6bx
Knight, R. A., Elliott, D. S., & Freedman, E. G. (1985). Short-term visual memory in schizophrenics. Journal of Abnormal Psychology , 94 (4), 427. 10/d69473
Kubota, K., & Niki, H. (1971). Prefrontal cortical unit activity and delayed alternation performance in monkeys. Journal of Neurophysiology , 34 (3), 337–347. 10/gg9jb9
Kueider, A. M., Parisi, J. M., Gross, A. L., & Rebok, G. W. (2012). Computerized Cognitive Training with Older Adults: A Systematic Review. PLOS ONE , 7 (7), e40588. 10/9ph
Kuhlmann, S., Piel, M., & Wolf, O. T. (2005). Impaired Memory Retrieval after Psychosocial Stress in Healthy Young Men. Journal of Neuroscience , 25 (11), 2977–2982. 10/d3gqdc
Lagroix, H. E., Spalek, T. M., Wyble, B., Jannati, A., & Di Lollo, V. (2012). The root cause of the attentional blink: First-target processing or disruption of input control? Attention, Perception, & Psychophysics , 74 (8), 1606–1622. 10/f4gq57
Lai, V., Theppitak, C., Makizuka, T., Higuchi, Y., Movahed, M., Kumudini, G., Izumi, H., & Kumashiro, M. (2014). A normal intensity level of psycho-physiological stress can benefit working memory performance at high load. International Journal of Industrial Ergonomics , 44 (3), 362–367. 10/gh3nw7
Laroche, S., Davis, S., & Jay, T. M. (2000). Plasticity at hippocampal to prefrontal cortex synapses: Dual roles in working memory and consolidation. Hippocampus , 10 (4), 438–446. 10/d5mt3z
Lechner, H. A., Squire, L. R., & Byrne, J. H. (1999). 100 Years of Consolidation—Remembering Müller and Pilzecker. Learning & Memory, 6 (2), 77–87. https://doi.org/10.1101/lm.6.2.77
Lee, A. K., & Wilson, M. A. (2002). Memory of sequential experience in the hippocampus during slow wave sleep. Neuron , 36 (6), 1183–1194. 10/cmf2h4
Lee, J., & Park, S. (2005). Working Memory Impairments in Schizophrenia: A Meta-Analysis. Journal of Abnormal Psychology , 114 (4), 599–611. 10/bfqtxz
Lefebvre, C., Vachon, F., Grimault, S., Thibault, J., Guimond, S., Peretz, I., Zatorre, R. J., & Jolicøeur, P. (2013). Distinct electrophysiological indices of maintenance in auditory and visual short-term memory. Neuropsychologia , 51 (13), 2939–2952. 10/f5mngh
Lehle, C., & Hübner, R. (2008). Strategic capacity sharing between two tasks: Evidence from tasks with the same and with different task sets. Psychological Research PRPF , 73 (5), 707. 10/ddgxz8
Leight, K. A., & Ellis, H. C. (1981). Emotional mood states, strategies, and state-dependency in memory. Journal of Verbal Learning and Verbal Behavior , 20 (3), 251–266. 10/cs778d
Leszczynski, M. (2011). How does hippocampus contribute to working memory processing? Frontiers in Human Neuroscience , 5 , 168. 10/cpkq99
Lewis, R. S., Nikolova, A., Chang, D. J., & Weekes, N. Y. (2008). Examination stress and components of working memory. Stress , 11 (2), 108–114. 10/dr84g7
Lewis-Peacock, J. A., & Postle, B. R. (2008). Temporary Activation of Long-Term Memory Supports Working Memory. Journal of Neuroscience , 28 (35), 8765–8771. 10/c856nj
Li, C. R., Lin, W., Yang, Y., Huang, C., Chen, T., & Chen, Y. (2002). Impairment of temporal attention in patients with schizophrenia. NeuroReport , 13 (11), 1427–1430. 10/ct7vj9
Li, R. W., Ngo, C. V., & Levi, D. M. (2015). Relieving the Attentional Blink in the Amblyopic Brain with Video Games. Scientific Reports , 5 (1), 8483. 10/gh29gc
Long, F., Ye, C., Li, Z., Tian, Y., & Liu, Q. (2020). Negative emotional state modulates visual working memory in the late consolidation phase. Cognition and Emotion , 34 (8), 1646–1663. 10/ghp84s
Louie, K., & Wilson, M. A. (2001). Temporally structured replay of awake hippocampal ensemble activity during rapid eye movement sleep. Neuron , 29 (1), 145–156. 10/b8psgr
Lucidi, A., Langerock, N., Hoareau, V., Lemaire, B., Camos, V., & Barrouillet, P. (2016). Working memory still needs verbal rehearsal. Memory & Cognition, 44 (2), 197–206. https://doi.org/10.3758/s13421-015-0561-z
Luck, S. J., Vogel, E. K., & Shapiro, K. L. (1996). Word meanings can be accessed but not reported during the attentional blink. Nature , 383 (6601), 616. 10/bxgghv
Luethi, M., Meier, B., & Sandi, C. (2009). Stress effects on working memory, explicit memory, and implicit memory for neutral and emotional stimuli in healthy men. Frontiers in Behavioral Neuroscience , 2 . https://doi.org/10.3389/neuro.08.005.2008
Lupien, S. J., Wilkinson, C. W., Brière, S., Ménard, C., Ng Ying Kin, N. M. K., & Nair, N. P. V. (2002). The modulatory effects of corticosteroids on cognition: Studies in young human populations. Psychoneuroendocrinology , 27 (3), 401–416. 10/dgjs4p
Majerus, S., D’Argembeau, A., Martinez Perez, T., Belayachi, S., Van der Linden, M., Collette, F., Salmon, E., Seurinck, R., Fias, W., & Maquet, P. (2010). The commonality of neural networks for verbal and visual short-term memory. Journal of Cognitive Neuroscience , 22 (11), 2570–2593. 10/b7dxz8
Makovski, T., & Lavidor, M. (2014). Stimulating occipital cortex enhances visual working memory consolidation. Behavioural Brain Research , 275 , 84–87. 10/f6n4cb
Manenti, R., Brambilla, M., Petesi, M., Ferrari, C., & Cotelli, M. (2013). Enhancing verbal episodic memory in older and young subjects after non-invasive brain stimulation. Frontiers in Aging Neuroscience , 5 . https://doi.org/10.3389/fnagi.2013.00049
Manoach, D. S., & Stickgold, R. (2009). Does abnormal sleep impair memory consolidation in schizophrenia? Frontiers in Human Neuroscience , 3 . 10/dqskbx
Manoach, D. S., & Stickgold, R. (2019). Abnormal Sleep Spindles, Memory Consolidation, and Schizophrenia. Annual Review of Clinical Psychology , 15 (1), 451–479. 10/ggrvfr
Marshall, L., Helgadóttir, H., Mölle, M., & Born, J. (2006). Boosting slow oscillations during sleep potentiates memory. Nature , 444 (7119), 610–613. 10/fkkwrc
Marshall, L., Mölle, M., Hallschmid, M., & Born, J. (2004). Transcranial Direct Current Stimulation during Sleep Improves Declarative Memory. Journal of Neuroscience , 24 (44), 9985–9992. 10/c46232
Marshall, L., Mölle, M., Siebner, H. R., & Born, J. (2005). Bifrontal transcranial direct current stimulation slows reaction time in a working memory task. BMC Neuroscience , 6 (1), 23. 10/ctfdf4
Martin, S. J., Grimwood, P. D., & Morris, R. G. M. (2000). Synaptic plasticity and memory: An evaluation of the hypothesis. Annual Review of Neuroscience, 23 (1), 649–711. https://doi.org/10.1146/annurev.neuro.23.1.649
Martinussen, R., Hayden, J., Hogg-johnson, S., & Tannock, R. (2005). A meta-analysis of working memory impairments in children with attention-deficit/hyperactivity disorder. Journal of the American Academy of Child & Adolescent Psychiatry, 44 (4), 377–384. https://doi.org/10.1097/01.chi.0000153228.72591.73
Maxfield, L., Melnyk, W. T., & Hayman, G. C. (2008). A working memory explanation for the effects of eye movements in EMDR. Journal of EMDR Practice and Research , 2 (4), 247–261. 10/fqrvd2
McClelland, J. L., McNaughton, B. L., & O’Reilly, R. C. (1995). Why there are complementary learning systems in the hippocampus and neocortex: Insights from the successes and failures of connectionist models of learning and memory. Psychological Review , 102 (3), 419–457. 10/dvtrsx
McGaugh, J. L. (1966). Time-dependent processes in memory storage. Science , 153 (3742), 1351–1358. 10/cvj882
McGaugh, J. L. (1999). The perseveration-consolidation hypothesis: Mueller and Pilzecker, 1900. Brain Research Bulletin , 50 (5–6), 445–446. 10/ckdrr5
McGhee, L. L., Maani, C. V., Garza, T. H., DeSocio, P. A., Gaylord, K. M., & Black, I. H. (2009). The effect of propranolol on posttraumatic stress disorder in burned service members. Journal of Burn Care & Research, 30 (1), 92–97. https://doi.org/10.1097/BCR.0b013e3181921f51
Meir Drexler, S., & Wolf, O. T. (2017). Stress and Memory Consolidation. In N. Axmacher & B. Rasch (Eds.), Cognitive neuroscience of memory consolidation (pp. 285–300). Springer International Publishing. https://doi.org/10.1007/978-3-319-45066-7_17
Melby-Lervag, M., & Hulme, C. (2013). Is working memory training effective? A Meta-Analytic Review. Developmental Psychology, 49 (2), 270.
PubMed Google Scholar
Milner, B., Corkin, S., & Teuber, H.-L. (1968). Further analysis of the hippocampal amnesic syndrome: 14-year follow-up study of H.M. Neuropsychologia , 6 (3), 215–234. 10/dp9jzh
Mitchell, K. J., Johnson, M. K., Raye, C. L., & D’Esposito, M. (2000). FMRI evidence of age-related hippocampal dysfunction in feature binding in working memory. Cognitive Brain Research , 10 (1/2), 197–206. 10/cj5n2t
Moran, T. P. (2016). Anxiety and working memory capacity: A meta-analysis and narrative review. Psychological Bulletin , 142 (8), 831. 10/f8xcjw
Morris, R. G., & Kopelman, M. D. (1986). The memory deficits in alzheimer-type dementia: A review. The Quarterly Journal of Experimental Psychology Section A , 38 (4), 575–602. 10/b37s5v
Müller, G. E., & Pilzecker, A. (1900). Experimentelle beiträge zur lehre vom gedächtniss (Vol. 1). JA Barth.
Müller, U., Mottweiler, E., & Bublak, P. (2005). Noradrenergic blockade and numeric working memory in humans. Journal of Psychopharmacology , 19 (1), 21–28. 10/ddgf6w
Murchison, C. F., Zhang, X.-Y., Zhang, W.-P., Ouyang, M., Lee, A., & Thomas, S. A. (2004). A distinct role for norepinephrine in memory retrieval. Cell , 117 (1), 131–143. 10/d6p5d6
Nadel, L., & Moscovitch, M. (1997). Memory consolidation, retrograde amnesia and the hippocampal complex. Current Opinion in Neurobiology , 7 (2), 217–227. 10/bw275g
Nadel, L., Samsonovich, A., Ryan, L., & Moscovitch, M. (2000). Multiple trace theory of human memory: Computational, neuroimaging, and neuropsychological results. Hippocampus , 10 (4), 352–368. 10/b8krgt
Nee, D. E., & Jonides, J. (2011). Dissociable contributions of prefrontal cortex and the hippocampus to short-term memory: Evidence for a 3-state model of memory. NeuroImage , 54 (2), 1540–1548. 10/cmzg6x
Newmark, R. E., Schon, K., Ross, R. S., & Stern, C. E. (2013). Contributions of the hippocampal subfields and entorhinal cortex to disambiguation during working memory. Hippocampus , 23 (6), 467–475. 10/f4x76z
Nguemeni, C., Nakchbandi, L., Homola, G., & Zeller, D. (2021). Impaired consolidation of visuomotor adaptation in patients with multiple sclerosis. European Journal of Neurology, 28 (3), 884–892. https://doi.org/10.1111/ene.14599
Nieuwenstein, M. R., Potter, M. C., & Theeuwes, J. (2009). Unmasking the attentional blink. Journal of Experimental Psychology: Human Perception and Performance, 35 (1), 159–169. https://doi.org/10.1037/0096-1523.35.1.159
Nieuwenstein, M. R., & Wyble, B. (2014). Beyond a mask and against the bottleneck: Retroactive dual-task interference during working memory consolidation of a masked visual target. Journal of Experimental Psychology: General , 143 (3), 1409. 10/f56df3
Nugent, N. R., Christopher, N. C., Crow, J. P., Browne, L., Ostrowski, S., & Delahanty, D. L. (2010). The efficacy of early propranolol administration at reducing PTSD symptoms in pediatric injury patients: A pilot study. Journal of Traumatic Stress , 23 (2), 282–287. 10/frrmrs
Oberauer, K. (2002). Access to information in working memory: Exploring the focus of attention. Journal of Experimental Psychology: Learning, Memory, and Cognition , 28 (3), 411. 10/bpqg2q
Oberauer, K., Lewandowsky, S., Farrell, S., Jarrold, C., & Greaves, M. (2012). Modeling working memory: An interference model of complex span. Psychonomic Bulletin & Review , 19 (5), 779–819. 10/f38mf6
Oberauer, K., Souza, A. S., Druey, M. D., & Gade, M. (2013). Analogous mechanisms of selection and updating in declarative and procedural working memory: Experiments and a computational model. Cognitive Psychology , 66 (2), 157–211. 10/f4qq6b
O’Carroll, R. E., Drysdale, E., Cahill, L., Shajahan, P., & Ebmeier, K. P. (1999). Memory for emotional material: A comparison of central versus peripheral beta blockade. Journal of Psychopharmacology , 13 (1), 32–39. 10/ch4g99
Oei, N. Y. L., Elzinga, B. M., Wolf, O. T., de Ruiter, M. B., Damoiseaux, J. S., Kuijer, J. P. A., Veltman, D. J., Scheltens, P., & Rombouts, S. A. R. B. (2007). Glucocorticoids decrease hippocampal and prefrontal activation during declarative memory retrieval in young men. Brain Imaging and Behavior , 1 (1), 31–41. 10/dzktbk
Ohn, S. H., Park, C.-I., Yoo, W.-K., Ko, M.-H., Choi, K. P., Kim, G.-M., Lee, Y. T., & Kim, Y.-H. (2008). Time-dependent effect of transcranial direct current stimulation on the enhancement of working memory. Neuroreport , 19 (1), 43–47. 10/d89rtf
Paßmann, S., Külzow, N., Ladenbauer, J., Antonenko, D., Grittner, U., Tamm, S., & Flöel, A. (2016). Boosting slow oscillatory activity using tdcs during early nocturnal slow wave sleep does not improve memory consolidation in healthy older adults. Brain Stimulation , 9 (5), 730–739. 10/f8z42r
Phillips, W. A. (1974). On the distinction between sensory storage and short-term visual memory. Perception & Psychophysics , 16 (2), 283–290. 10/bzgrwm
Piekema, C., Kessels, R. P. C., Mars, R. B., Petersson, K. M., & Fernández, G. (2006). The right hippocampus participates in short-term memory maintenance of object–location associations. NeuroImage, 33 (1), 374–382. https://doi.org/10.1016/j.neuroimage.2006.06.035
Popov, V., & Reder, L. M. (2020). Frequency effects on memory: A resource-limited theory. Psychological Review , 127 (1), 1–46. 10/gg2wrx
Poundja, J., Sanche, S., Tremblay, J., & Brunet, A. (2012). Trauma reactivation under the influence of propranolol: An examination of clinical predictors. European Journal of Psychotraumatology , 3 (1), 15470. 10/gh23zb
Preuß, D., & Wolf, O. T. (2009). Post-learning psychosocial stress enhances consolidation of neutral stimuli. Neurobiology of Learning and Memory , 92 (3), 318–326. 10/dgbfs6
Ranganath, C., Cohen, M. X., & Brozinsky, C. J. (2005). Working memory maintenance contributes to long-term memory formation: Neural and behavioral evidence. Journal of Cognitive Neuroscience , 17 (7), 994–1010. 10/c4hrm3
Ranganath, C., & D’Esposito, M. (2001). Medial temporal lobe activity associated with active maintenance of novel information. Neuron , 31 (5), 865–873. 10/fjq6rm
Ranganath, C., & D’Esposito, M. (2005). Directing the mind’s eye: Prefrontal, inferior and medial temporal mechanisms for visual working memory. Current Opinion in Neurobiology , 15 (2), 175–182. 10/c33cfb
Raye, C. L., Johnson, M. K., Mitchell, K. J., Greene, E. J., & Johnson, M. R. (2007). Refreshing: A minimal executive function. Cortex , 43 , 135–145. 10/cfck36
Raye, C. L., Johnson, M. K., Mitchell, K. J., Reeder, J. A., & Greene, E. J. (2002). Neuroimaging a single thought: Dorsolateral PFC activity associated with refreshing just-activated information. NeuroImage , 15 (2), 447–453. 10/b9sxgb
Raymond, J. E., Shapiro, K. L., & Arnell, K. M. (1992). Temporary suppression of visual processing in an RSVP task: An attentional blink? Journal of Experimental Psychology: Human Perception and Performance , 18 (3), 849. 10/bbztdn
Reist, C., Duffy, J. G., Fujimoto, K., & Cahill, L. (2001). β-Adrenergic blockade and emotional memory in PTSD. International Journal of Neuropsychopharmacology , 4 (4), 377–383. 10/d9xbmn
Resick, P. A., Monson, C. M., & Rizvi, S. L. (2008). Posttraumatic stress disorder. In D. J. Miklowitz, L. W. Craighead, & W. E. Craighead (Eds.), Psychopathology: History, diagnosis, and empirical foundations (pp. 234–278). John Wiley & Sons Inc.
Google Scholar
Ricker, T. J. (2015). The role of short-term consolidation in memory persistence. AIMS Neuroscience , 2 (4), 259–279. 10/gd4qqs
Ricker, T. J., & Cowan, N. (2014). Differences between presentation methods in working memory procedures: A matter of working memory consolidation. Journal of Experimental Psychology: Learning, Memory, and Cognition , 40 (2), 417. 10/gd4qqr
Ricker, T. J., & Hardman, K. O. (2017). The nature of short-term consolidation in visual working memory. Journal of Experimental Psychology: General , 146 (11), 1551. 10/gchkd8
Ricker, T. J., Nieuwenstein, M. R., Bayliss, D. M., & Barrouillet, P. (2018). Working memory consolidation: Insights from studies on attention and working memory. Annals of the New York Academy of Sciences . 10/gd4qqp
Ricker, T. J., & Sandry, J. (2018). The relationship between masking and short-term consolidation during recall from visual working memory. Annals of the New York Academy of Sciences . 10/gd59vx
Ricker, T. J., Sandry, J., Vergauwe, E., & Cowan, N. (2020). Do familiar memory items decay? Journal of Experimental Psychology: Learning, Memory, and Cognition , 46 (1), 60–76. 10/ggtf36
Rocca, M. A., Barkhof, F., De Luca, J., Frisén, J., Geurts, J. J. G., Hulst, H. E., Sastre-Garriga, J., Filippi, M., Barkhof, F., Ciccarelli, O., De Stefano, N., Enzinger, C., Filippi, M., Frederiksen, J. L., Gasperini, C., Kappos, L., Palace, J., Rocca, M. A., Rovira, A., … Yousry, T. A. (2018). The hippocampus in multiple sclerosis. The Lancet Neurology , 17 (10), 918–926. 10/gfcsz3
Rose, N. S., Olsen, R. K., Craik, F. I. M., & Rosenbaum, R. S. (2012). Working memory and amnesia: The role of stimulus novelty. Neuropsychologia , 50 (1), 11–18. 10/dj8p84
Rumpf, J.-J., Dietrich, S., Stoppe, M., Fricke, C., Weise, D., Then Bergh, F., & Classen, J. (2018). Compromised tDCS-induced facilitation of motor consolidation in patients with multiple sclerosis. Journal of Neurology , 265 (10), 2302–2311. 10/gfdx7z
Rund, B. R., & Borg, N. E. (1999). Cognitive deficits and cognitive training in schizophrenic patients: A review. Acta Psychiatrica Scandinavica , 100 (2), 85–95. 10/c57mf2
Ryan, L., Nadel, L., Keil, K., Putnam, K., Schnyer, D., Trouard, T., & Moscovitch, M. (2001). Hippocampal complex and retrieval of recent and very remote autobiographical memories: Evidence from functional magnetic resonance imaging in neurologically intact people. Hippocampus , 11 (6), 707–714. 10/fwkwf2
Sandry, J., Zuppichini, M., Rothberg, J., Valdespino-Hayden, Z., & DeLuca, J. (2019). Poor encoding and weak early consolidation underlie memory acquisition deficits in multiple sclerosis: Retroactive interference, processing speed, or working memory? Archives of Clinical Neuropsychology , 34 (2), 162–182. 10/ghdcgp
Schapiro, A. C., McDevitt, E. A., Rogers, T. T., Mednick, S. C., & Norman, K. A. (2018). Human hippocampal replay during rest prioritizes weakly learned information and predicts memory performance. Nature Communications , 9 (1), 3920. 10/gd8gfp
Schon, K., Hasselmo, M. E., LoPresti, M. L., Tricarico, M. D., & Stern, C. E. (2004). Persistence of parahippocampal representation in the absence of stimulus input enhances long-term encoding: A functional magnetic resonance imaging study of subsequent memory after a delayed match-to-sample task. Journal of Neuroscience , 24 (49), 11088–11097. 10/fhxk9t
Schwabe, L., & Wolf, O. T. (2010). Emotional modulation of the attentional blink: Is there an effect of stress? Emotion , 10 (2), 283–288. 10/dhm7q5
Scoville, W. B., & Milner, B. (1957). Loss of recent memory after bilateral hippocampal lesions. Journal of Neurology, Neurosurgery, and Psychiatry , 20 (1), 11. 10/cfp6bs
Sederberg, P. B., Kahana, M. J., Howard, M. W., Donner, E. J., & Madsen, J. R. (2003). Theta and gamma oscillations during encoding predict subsequent recall. Journal of Neuroscience , 23 (34), 10809–10814. 10/gfxfsd
Sekeres, M. J., Moscovitch, M., & Winocur, G. (2017). Mechanisms of memory consolidation and transformation. In N. Axmacher & B. Rasch (Eds.), Cognitive neuroscience of memory consolidation (pp. 17–44). Springer International Publishing. https://doi.org/10.1007/978-3-319-45066-7_2
Serences, J. T., Ester, E. F., Vogel, E. K., & Awh, E. (2009). Stimulus-specific delay activity in human primary visual cortex. Psychological Science , 20 (2), 207–214. 10/fp7qzc
Shanahan, L. K., & Gottfried, J. A. (2017). Scents and reminiscence: Olfactory influences on memory consolidation in the sleeping human brain. In Cognitive Neuroscience of Memory Consolidation (pp. 335–346). Springer.
Shapiro, K. L., Raymond, J. E., & Arnell, K. M. (1997). The attentional blink. Trends in Cognitive Sciences , 1 (8), 291–296. 10/bcrhnp
Shields, G. S., Sazma, M. A., McCullough, A. M., & Yonelinas, A. P. (2017). The effects of acute stress on episodic memory: A meta-analysis and integrative review. Psychological Bulletin , 143 (6), 636–675. 10/f98kxb
Sloan, N. P., Byrne, L. K., Enticott, P. G., & Lum, J. A. G. (2020). Non-invasive brain stimulation does not improve working memory in schizophrenia: A meta-analysis of randomised controlled trials. Neuropsychology Review . 10/ghbk2w
Smeets, T., Otgaar, H., Candel, I., & Wolf, O. T. (2008). True or false? Memory is differentially affected by stress-induced cortisol elevations and sympathetic activity at consolidation and retrieval. Psychoneuroendocrinology , 33 (10), 1378–1386. 10/dbh47g
Souza, A. S., & Oberauer, K. (2017). Time to process information in working memory improves episodic memory. Journal of Memory and Language , 96 , 155–167. 10/gftmq7
Souza, A. S., & Oberauer, K. (2018). Does articulatory rehearsal help immediate serial recall? Cognitive Psychology , 107 , 1–21. 10/gfbzqk
Souza, A. S., & Oberauer, K. (2020). No evidence that articulatory rehearsal improves complex span performance. Journal of Cognition , 3 (1). 10/ghg7vq
Sperling, G. (1960). The information available in brief visual presentations. Psychological Monographs: General and Applied , 74 (11), 1. 10/bmhp9n
Squire, L. R. (1992). Memory and the hippocampus: A synthesis from findings with rats, monkeys, and humans. Psychological Review , 99 (2), 195. 10/bb57s3
Squire, L. R. (2004). Memory systems of the brain: A brief history and current perspective. Neurobiology of Learning and Memory, 82 (3), 171–177. https://doi.org/10.1016/j.nlm.2004.06.005
Squire, L. R., & Alvarez, P. (1995). Retrograde amnesia and memory consolidation: A neurobiological perspective. Current Opinion in Neurobiology , 5 (2), 169–177. 10/bkhzqn
Squire, L. R., Genzel, L., Wixted, J. T., & Morris, R. G. (2015). Memory consolidation. Cold Spring Harbor Perspectives in Biology , 7 (8), a021766. 10/gg4g4b
Squire, L. R., & Wixted, J. T. (2011). The cognitive neuroscience of human memory since HM. In S. E. Hyman, T. M. Jessell, C. J. Shatz, C. F. Stevens, & H. Y. Zoghbi (Eds.), Annual Review of Neuroscience (Vol. 34, pp. 259–288). Annual Reviews. https://doi.org/10.1146/annurev-neuro-061010-113720
Sreenivasan, K. K., & D’Esposito, M. (2019). The what, where and how of delay activity. Nature Reviews Neuroscience , 20 (8), 466–481. 10/gf2tz4
Stern, C. E., Sherman, S. J., Kirchhoff, B. A., & Hasselmo, M. E. (2001). Medial temporal and prefrontal contributions to working memory tasks with novel and familiar stimuli. Hippocampus , 11 (4), 337–346. 10/cmz6jr
Stevanovski, B., & Jolicøeur, P. (2007). Visual short-term memory: Central capacity limitations in short-term consolidation. Visual Cognition , 15 (5), 532–563. 10/cqd8mg
Takashima, A., Nieuwenhuis, I. L., Jensen, O., Talamini, L. M., Rijpkema, M., & Fernández, G. (2009). Shift from hippocampal to neocortical centered retrieval network with consolidation. Journal of Neuroscience , 29 (32), 10087–10093. 10/fjn2qc
Takehara, K., Kawahara, S., & Kirino, Y. (2003). Time-dependent reorganization of the brain components underlying memory retention in trace eyeblink conditioning. Journal of Neuroscience, 23 (30), 9897–9905. https://doi.org/10.1523/JNEUROSCI.23-30-09897.2003
Tallon-Baudry, C., Bertrand, O., Peronnet, F., & Pernier, J. (1998). Induced γ-band activity during the delay of a visual short-term memory task in humans. Journal of Neuroscience , 18 (11), 4244–4254. 10/gg2hzt
Tallon-Baudry, C., Kreiter, A., & Bertrand, O. (1999). Sustained and transient oscillatory responses in the gamma and beta bands in a visual short-term memory task in humans. Visual Neuroscience , 16 (3), 449–459. 10/ddxrvb
Tambini, A., & Davachi, L. (2013). Persistence of hippocampal multivoxel patterns into postencoding rest is related to memory. Proceedings of the National Academy of Sciences , 110 (48), 19591–19596. 10/f5kjxv
Tang, M. F., Badcock, D. R., & Visser, T. A. W. (2014). Training and the attentional blink: Limits overcome or expectations raised? Psychonomic Bulletin & Review , 21 (2), 406–411. 10/f5xx4x
Teo, F., Hoy, K. E., Daskalakis, Z. J., & Fitzgerald, P. B. (2011). Investigating the role of current strength in tDCS modulation of working memory performance in healthy controls. Frontiers in Psychiatry , 2 . https://doi.org/10.3389/fpsyt.2011.00045
Tetlow, A. M., & Edwards, J. D. (2017). Systematic literature review and meta-analysis of commercially available computerized cognitive training among older adults. Journal of Cognitive Enhancement , 1 (4), 559–575. 10/gf9jg2
Todd, J. J., & Marois, R. (2004). Capacity limit of visual short-term memory in human posterior parietal cortex. Nature , 428 (6984), 751–754. 10/bkdmnf
Todd, J. J., & Marois, R. (2005). Posterior parietal cortex activity predicts individual differences in visual short-term memory capacity. Cognitive, Affective, & Behavioral Neuroscience , 5 (2), 144–155. 10/fnqn2g
Tombu, M., & Jolicøeur, P. (2003). A central capacity sharing model of dual-task performance. Journal of Experimental Psychology: Human Perception and Performance , 29 (1), 3. 10/fjb9h3
Tremblay, S., Lepage, J.-F., Latulipe-Loiselle, A., Fregni, F., Pascual-Leone, A., & Théoret, H. (2014). The uncertain outcome of prefrontal tDCS. Brain Stimulation , 7 (6), 773–783. 10/f2wmfq
Vaiva, G., Ducrocq, F., Jezequel, K., Averland, B., Lestavel, P., Brunet, A., & Marmar, C. R. (2003). Immediate treatment with propranolol decreases posttraumatic stress disorder two months after trauma. Biological Psychiatry, 54 (9), 947–949. https://doi.org/10.1016/S0006-3223(03)00412-8
van de Ven, V., & Sack, A. T. (2013). Transcranial magnetic stimulation of visual cortex in memory: Cortical state, interference and reactivation of visual content in memory. Behavioural Brain Research , 236 , 67–77. 10/gbcv53
van den Hout, M. A., & Engelhard, I. M. (2012). How does EMDR work? Journal of Experimental Psychopathology , 3 (5), 724–738. 10/ggkg9g
van Lamsweerde, A. E., & Johnson, J. S. (2017). Assessing the effect of early visual cortex transcranial magnetic stimulation on working memory consolidation. Journal of Cognitive Neuroscience , 29 (7), 1226–1238. 10/gh4jwx
van Stegeren, A. H., Everaerd, W., Cahill, L., McGaugh, J. L., & Gooren, L. J. G. (1998). Memory for emotional events: Differential effects of centrally versus peripherally acting β-blocking agents. Psychopharmacology (berl), 138 (3), 305–310. https://doi.org/10.1007/s002130050675
van Stegeren, A. H., Everaerd, W., & Gooren, L. J. (2002). The effect of beta-adrenergic blockade after encoding on memory of an emotional event. Psychopharmacology , 163 (2), 202–212. 10/cv6zz6
van Stegeren, A. H., Goekoop, R., Everaerd, W., Scheltens, P., Barkhof, F., Kuijer, J. P. A., & Rombouts, S. A. R. B. (2005). Noradrenaline mediates amygdala activation in men and women during encoding of emotional material. NeuroImage , 24 (3), 898–909. 10/b9f42p
Vedhara, K., Hyde, J., Gilchrist, I. D., Tytherleigh, M., & Plummer, S. (2000). Acute stress, memory, attention and cortisol. Psychoneuroendocrinology , 25 (6), 535–549. 10/djqxbd
Vergauwe, E., Ricker, T. J., Langerock, N., & Cowan, N. (2019). What do people typically do between list items? The nature of attention-based mnemonic activities depends on task context. Journal of Experimental Psychology. Learning, Memory, and Cognition . 10/gd4qqv
Vogel, E. K., & Luck, S. J. (2002). Delayed working memory consolidation during the attentional blink. Psychonomic Bulletin & Review, 9 (4), 739–743.
Vogel, E. K., Luck, S. J., & Shapiro, K. L. (1998). Electrophysiological evidence for a postperceptual locus of suppression during the attentional blink. Journal of Experimental Psychology: Human Perception and Performance , 24 (6), 1656. 10/cg6rjz
Vogel, E. K., & Machizawa, M. G. (2004). Neural activity predicts individual differences in visual working memory capacity. Nature , 428 (6984), 748. 10/dpb3j5
Vogel, E. K., Woodman, G. F., & Luck, S. J. (2006). The time course of consolidation in visual working memory. Journal of Experimental Psychology: Human Perception and Performance , 32 (6), 1436. 10/c2vrp7
Wamsley, E. J., Tucker, M. A., Shinn, A. K., Ono, K. E., McKinley, S. K., Ely, A. V., Goff, D. C., Stickgold, R., & Manoach, D. S. (2012). Reduced sleep spindles and spindle coherence in schizophrenia: Mechanisms of impaired memory consolidation? Biological Psychiatry , 71 (2), 154–161. 10/fs4t57
Waugh, N. C., & Norman, D. A. (1965). Primary memory. Psychological Review , 72 (2), 89–104. 10/d42xrj
Wiltgen, B. J., Brown, R. A., Talton, L. E., & Silva, A. J. (2004). New circuits for old memories: The role of the neocortex in consolidation. Neuron , 44 (1), 101–108. 10/c9rccd
Winocur, G., & Moscovitch, M. (2011). Memory transformation and systems consolidation. Journal of the International Neuropsychological Society, 17 (05), 766–780. https://doi.org/10.1017/S1355617711000683
Wolf, O. T. (2009). Stress and memory in humans: Twelve years of progress? Brain Research , 1293 , 142–154. 10/ddncj8
Woodman, G. F., & Vogel, E. K. (2005). Fractionating Working Memory: Consolidation and Maintenance Are Independent Processes. Psychological Science , 16 (2), 106–113. 10/d7f3x7
Woodman, G. F., & Vogel, E. K. (2008). Selective storage and maintenance of an object’s features in visual working memory. Psychonomic Bulletin & Review , 15 (1), 223–229. 10/bwznzq
Wyble, B., Potter, M. C., Bowman, H., & Nieuwenstein, M. (2011). Attentional episodes in visual perception. Journal of Experimental Psychology: General , 140 (3), 488. 10/cnjrvc
Xie, W., & Zhang, W. (2017). Familiarity speeds up visual short-term memory consolidation. Journal of Experimental Psychology: Human Perception and Performance , 43 (6), 1207–1221. 10/gbh2n6
Ye, C., Hu, Z., Li, H., Ristaniemi, T., Liu, Q., & Liu, T. (2017). A two-phase model of resource allocation in visual working memory. Journal of Experimental Psychology: Learning, Memory, and Cognition , 43 (10), 1557. 10/gcx8n5
Ye, C., Liang, T., Zhang, Y., Xu, Q., Zhu, Y., & Liu, Q. (2020). The two-stage process in visual working memory consolidation. Scientific Reports , 10 (1), 13564. 10/gg72fm
Yonelinas, A. P., Ranganath, C., Ekstrom, A. D., & Wiltgen, B. J. (2019). A contextual binding theory of episodic memory: Systems consolidation reconsidered. Nature Reviews Neuroscience , 20 (6), 364–375. 10/gfw2kq
Zaehle, T., Sandmann, P., Thorne, J. D., Jäncke, L., & Herrmann, C. S. (2011). Transcranial direct current stimulation of the prefrontal cortex modulates working memory performance: Combined behavioural and electrophysiological evidence. BMC Neuroscience , 12 (1), 1–11. 10/b75vr3
Zarahn, E., Aguirre, G., & D’Esposito, M. (1997). A trial-based experimental design for fMRI. NeuroImage, 6 (2), 122–138. https://doi.org/10.1006/nimg.1997.0279
Zhang, H., Deuker, L., & Axmacher, N. (2017). Replay in humans—First evidence and open questions. In N. Axmacher & B. Rasch (Eds.), Cognitive neuroscience of memory consolidation (pp. 251–263). Springer.
Zhao, C., & Woodman, G. F. (2020). Converging evidence that neural plasticity underlies transcranial direct-current stimulation. Journal of Cognitive Neuroscience , 1–12. Advance online publication. 10/ghh7bf
Download references
Author information
Authors and affiliations.
Department of Psychology, The Graduate Center, City University of New York, 365 5th Avenue, New York, NY, 10016, USA
Kelly Cotton
Department of Psychology, University of South Dakota, Vermillion, SD, USA
Timothy J. Ricker
You can also search for this author in PubMed Google Scholar
Corresponding author
Correspondence to Kelly Cotton .
Additional information
Publisher's note.
Springer Nature remains neutral with regard to jurisdictional claims in published maps and institutional affiliations.
Rights and permissions
Reprints and permissions
About this article
Cotton, K., Ricker, T.J. Examining the relationship between working memory consolidation and long-term consolidation. Psychon Bull Rev 29 , 1625–1648 (2022). https://doi.org/10.3758/s13423-022-02084-2
Download citation
Accepted : 04 March 2022
Published : 31 March 2022
Issue Date : October 2022
DOI : https://doi.org/10.3758/s13423-022-02084-2
Share this article
Anyone you share the following link with will be able to read this content:
Sorry, a shareable link is not currently available for this article.
Provided by the Springer Nature SharedIt content-sharing initiative
- Working memory
- Short-term memory
- Long-term memory
- Consolidation
- Find a journal
- Publish with us
- Track your research
Information
- Author Services
Initiatives
You are accessing a machine-readable page. In order to be human-readable, please install an RSS reader.
All articles published by MDPI are made immediately available worldwide under an open access license. No special permission is required to reuse all or part of the article published by MDPI, including figures and tables. For articles published under an open access Creative Common CC BY license, any part of the article may be reused without permission provided that the original article is clearly cited. For more information, please refer to https://www.mdpi.com/openaccess .
Feature papers represent the most advanced research with significant potential for high impact in the field. A Feature Paper should be a substantial original Article that involves several techniques or approaches, provides an outlook for future research directions and describes possible research applications.
Feature papers are submitted upon individual invitation or recommendation by the scientific editors and must receive positive feedback from the reviewers.
Editor’s Choice articles are based on recommendations by the scientific editors of MDPI journals from around the world. Editors select a small number of articles recently published in the journal that they believe will be particularly interesting to readers, or important in the respective research area. The aim is to provide a snapshot of some of the most exciting work published in the various research areas of the journal.
Original Submission Date Received: .
- Active Journals
- Find a Journal
- Proceedings Series
- For Authors
- For Reviewers
- For Editors
- For Librarians
- For Publishers
- For Societies
- For Conference Organizers
- Open Access Policy
- Institutional Open Access Program
- Special Issues Guidelines
- Editorial Process
- Research and Publication Ethics
- Article Processing Charges
- Testimonials
- Preprints.org
- SciProfiles
- Encyclopedia
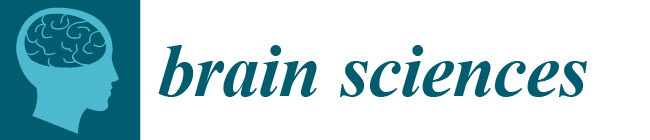
Article Menu
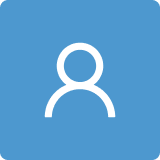
- Subscribe SciFeed
- Recommended Articles
- PubMed/Medline
- Google Scholar
- on Google Scholar
- Table of Contents
Find support for a specific problem in the support section of our website.
Please let us know what you think of our products and services.
Visit our dedicated information section to learn more about MDPI.
JSmol Viewer
The relationship between short- and long-term memory is preserved across the age range, 1. introduction, 2. experiment 1, 2.1. methods, 2.1.1. participants, 2.1.2. stimuli, 2.1.3. tasks and procedures, encoding and short-term memory task, explicit long-term memory retrieval, 2.1.4. data analysis, 2.2. results, 2.2.1. participants, 2.2.2. identification performance, 2.2.3. localisation error, 2.2.4. relationship between short- and long-term memories, 3. experiment 2, 3.1. methods, 3.1.1. participants, 3.1.2. stimuli, 3.1.3. tasks and procedures, short-term memory/encoding task, 3.1.4. data analysis, 3.2. results, 3.2.1. identification performance, 3.2.2. localisation error, 4. discussion, supplementary materials, author contributions, institutional review board statement, informed consent statement, data availability statement, acknowledgments, conflicts of interest.
- Atkinson, R.C.; Shiffrin, R.M. Human Memory: A Proposed System and Its Control Processes. In Psychology of Learning and Motivation ; Academic Press: New York, NY, US, 1968; Volume 2, pp. 89–195. ISBN 978-0-12-543302-0. [ Google Scholar ]
- Shiffrin, R.M.; Atkinson, R.C. Storage and Retrieval Processes in Long-Term Memory. Psychol. Rev. 1969 , 76 , 179–193. [ Google Scholar ] [ CrossRef ] [ Green Version ]
- Baddeley, A. The Episodic Buffer: A New Component of Working Memory? Trends Cogn. Sci. 2000 , 4 , 417–423. [ Google Scholar ] [ CrossRef ] [ PubMed ]
- Hebb, D.O. Distinctive Features of Learning in the Higher Animal ; Blackwell: Oxford, UK, 1961. [ Google Scholar ]
- Oberauer, K.; Lewandowsky, S.; Awh, E.; Brown, G.D.A.; Conway, A.; Cowan, N.; Donkin, C.; Farrell, S.; Hitch, G.J.; Hurlstone, M.J.; et al. Benchmarks for Models of Short-Term and Working Memory. Psychol. Bull. 2018 , 144 , 885–958. [ Google Scholar ] [ CrossRef ] [ PubMed ]
- Baddeley, A. Working Memory: Theories, Models, and Controversies. Annu. Rev. Psychol. 2012 , 63 , 1–29. [ Google Scholar ] [ CrossRef ] [ Green Version ]
- Brady, T.F.; Konkle, T.; Gill, J.; Oliva, A.; Alvarez, G.A. Visual Long-Term Memory Has the Same Limit on Fidelity as Visual Working Memory. Psychol. Sci. 2013 , 24 , 981–990. [ Google Scholar ] [ CrossRef ] [ Green Version ]
- Brown, G.D.A.; Neath, I.; Chater, N. A Temporal Ratio Model of Memory. Psychol. Rev. 2007 , 114 , 539–576. [ Google Scholar ] [ CrossRef ] [ Green Version ]
- Cowan, N. Memory Theories From A to Z. Contemp. Psychol. A J. Rev. 1995 , 40 , 552–555. [ Google Scholar ] [ CrossRef ]
- Forsberg, A.; Guitard, D.; Cowan, N. Working Memory Limits Severely Constrain Long-Term Retention. Psychon. Bull. Rev. 2021 , 28 , 537–547. [ Google Scholar ] [ CrossRef ]
- Fukuda, K.; Vogel, E.K. Visual Short-Term Memory Capacity Predicts the “Bandwidth” of Visual Long-Term Memory Encoding. Mem. Cogn. 2019 , 47 , 1481–1497. [ Google Scholar ] [ CrossRef ] [ Green Version ]
- Khader, P.; Ranganath, C.; Seemüller, A.; Rösler, F. Working Memory Maintenance Contributes to Long-Term Memory Formation: Evidence from Slow Event-Related Brain Potentials. Cogn. Affect. Behav. Neurosci. 2007 , 7 , 212–224. [ Google Scholar ] [ CrossRef ]
- Oberauer, K. Access to Information in Working Memory: Exploring the Focus of Attention. J. Exp. Psychol. Learn. Mem. Cogn. 2002 , 28 , 411–421. [ Google Scholar ] [ CrossRef ] [ PubMed ]
- Ranganath, C.; Cohen, M.X.; Brozinsky, C.J. Working Memory Maintenance Contributes to Long-Term Memory Formation: Neural and Behavioral Evidence. J. Cogn. Neurosci. 2005 , 17 , 994–1010. [ Google Scholar ] [ CrossRef ] [ PubMed ]
- Schurgin, M.W.; Wixted, J.T.; Brady, T.F. Psychophysical Scaling Reveals a Unified Theory of Visual Memory Strength. Nat. Hum. Behav. 2020 , 4 , 1156–1172. [ Google Scholar ] [ CrossRef ]
- Gorbach, T.; Pudas, S.; Lundquist, A.; Orädd, G.; Josefsson, M.; Salami, A.; de Luna, X.; Nyberg, L. Longitudinal Association between Hippocampus Atrophy and Episodic-Memory Decline. Neurobiol. Aging 2017 , 51 , 167–176. [ Google Scholar ] [ CrossRef ] [ PubMed ]
- Cam, C.; Henson, R.N.; Campbell, K.L.; Davis, S.W.; Taylor, J.R.; Emery, T.; Erzinclioglu, S.; Kievit, R.A. Multiple Determinants of Lifespan Memory Differences. Sci. Rep. 2016 , 6 , 32527. [ Google Scholar ] [ CrossRef ] [ Green Version ]
- Nilsson, L.-G.; BÄCkman, L.; Erngrund, K.; Nyberg, L.; Adolfsson, R.; Bucht, G.; Karlsson, S.; Widing, M.; Winblad, B. The Betula Prospective Cohort Study: Memory, Health, and Aging. Aging Neuropsychol. Cogn. 1997 , 4 , 1–32. [ Google Scholar ] [ CrossRef ]
- Peich, M.-C.; Husain, M.; Bays, P.M. Age-Related Decline of Precision and Binding in Visual Working Memory. Psychol. Aging 2013 , 28 , 729–743. [ Google Scholar ] [ CrossRef ]
- Pertzov, Y.; Heider, M.; Liang, Y.; Husain, M. Effects of Healthy Ageing on Precision and Binding of Object Location in Visual Short Term Memory. Psychol. Aging 2015 , 30 , 26–35. [ Google Scholar ] [ CrossRef ] [ PubMed ]
- Rönnlund, M.; Nyberg, L.; Bäckman, L.; Nilsson, L.-G. Stability, Growth, and Decline in Adult Life Span Development of Declarative Memory: Cross-Sectional and Longitudinal Data From a Population-Based Study. Psychol. Aging 2005 , 20 , 3–18. [ Google Scholar ] [ CrossRef ]
- Zokaei, N.; Grogan, J.; Fallon, S.J.; Slavkova, E.; Hadida, J.; Manohar, S.; Nobre, A.C.; Husain, M. Short-Term Memory Advantage for Brief Durations in Human APOE Ε4 Carriers. Sci. Rep. 2020 , 10 , 9503. [ Google Scholar ] [ CrossRef ]
- Pudas, S.; Persson, J.; Josefsson, M.; de Luna, X.; Nilsson, L.-G.; Nyberg, L. Brain Characteristics of Individuals Resisting Age-Related Cognitive Decline over Two Decades. J. Neurosci. 2013 , 33 , 8668–8677. [ Google Scholar ] [ CrossRef ] [ PubMed ] [ Green Version ]
- Rogalski, E.J.; Gefen, T.; Shi, J.; Samimi, M.; Bigio, E.; Weintraub, S.; Geula, C.; Mesulam, M.-M. Youthful Memory Capacity in Old Brains: Anatomic and Genetic Clues from the Northwestern SuperAging Project. J. Cogn. Neurosci. 2013 , 25 , 29–36. [ Google Scholar ] [ CrossRef ] [ PubMed ] [ Green Version ]
- Sun, F.W.; Stepanovic, M.R.; Andreano, J.; Barrett, L.F.; Touroutoglou, A.; Dickerson, B.C. Youthful Brains in Older Adults: Preserved Neuroanatomy in the Default Mode and Salience Networks Contributes to Youthful Memory in Superaging. J. Neurosci. 2016 , 36 , 9659–9668. [ Google Scholar ] [ CrossRef ] [ PubMed ] [ Green Version ]
- Milner, B.; Corkin, S.; Teuber, H.-L. Further Analysis of the Hippocampal Amnesic Syndrome: 14-Year Follow-up Study of H.M. Neuropsychologia 1968 , 6 , 215–234. [ Google Scholar ] [ CrossRef ]
- Scoville, W.B.; Milner, B. Loss Of Recent Memory After Bilateral Hippocampal Lesions. J. Neurol. Neurosurg. Psychiatry 1957 , 20 , 11–21. [ Google Scholar ] [ CrossRef ] [ Green Version ]
- Squire, L.R. The Legacy of Patient H.M. for Neuroscience. Neuron 2009 , 61 , 6–9. [ Google Scholar ] [ CrossRef ] [ Green Version ]
- Olson, I.R. Working Memory for Conjunctions Relies on the Medial Temporal Lobe. J. Neurosci. 2006 , 26 , 4596–4601. [ Google Scholar ] [ CrossRef ] [ Green Version ]
- Pertzov, Y.; Miller, T.D.; Gorgoraptis, N.; Caine, D.; Schott, J.M.; Butler, C.; Husain, M. Binding Deficits in Memory Following Medial Temporal Lobe Damage in Patients with Voltage-Gated Potassium Channel Complex Antibody-Associated Limbic Encephalitis. Brain 2013 , 136 , 2474–2485. [ Google Scholar ] [ CrossRef ]
- Watson, P.D.; Voss, J.L.; Warren, D.E.; Tranel, D.; Cohen, N.J. Spatial Reconstruction by Patients with Hippocampal Damage Is Dominated by Relational Memory Errors: Relational Errors in Hippocampal Amnesia. Hippocampus 2013 , 23 , 570–580. [ Google Scholar ] [ CrossRef ] [ PubMed ] [ Green Version ]
- Zokaei, N.; Nour, M.M.; Sillence, A.; Drew, D.; Adcock, J.; Stacey, R.; Voets, N.; Sen, A.; Husain, M. Binding Deficits in Visual Short-Term Memory in Patients with Temporal Lobe Lobectomy. Hippocampus 2019 , 29 , 63–67. [ Google Scholar ] [ CrossRef ]
- Olson, I.R.; Moore, K.S.; Stark, M.; Chatterjee, A. Visual Working Memory Is Impaired When the Medial Temporal Lobe Is Damaged. J. Cogn. Neurosci. 2006 , 18 , 1087–1097. [ Google Scholar ] [ CrossRef ] [ PubMed ]
- Baddeley, A.; Jarrold, C.; Vargha-Khadem, F. Working Memory and the Hippocampus. J. Cogn. Neurosci. 2011 , 23 , 3855–3861. [ Google Scholar ] [ CrossRef ] [ PubMed ]
- Jeneson, A.; Squire, L.R. Working Memory, Long-Term Memory, and Medial Temporal Lobe Function. Learn. Mem. 2012 , 19 , 15–25. [ Google Scholar ] [ CrossRef ] [ PubMed ] [ Green Version ]
- Lugtmeijer, S.; de Haan, E.H.F.; Kessels, R.P.C. A Comparison of Visual Working Memory and Episodic Memory Performance in Younger and Older Adults. Aging Neuropsychol. Cogn. 2019 , 26 , 387–406. [ Google Scholar ] [ CrossRef ] [ Green Version ]
- van Geldorp, B.; Heringa, S.M.; van den Berg, E.; Olde Rikkert, M.G.M.; Biessels, G.J.; Kessels, R.P.C. Working Memory Binding and Episodic Memory Formation in Aging, Mild Cognitive Impairment, and Alzheimer’s Dementia. J. Clin. Exp. Neuropsychol. 2015 , 37 , 538–548. [ Google Scholar ] [ CrossRef ]
- Korkki, S.M.; Richter, F.R.; Jeyarathnarajah, P.; Simons, J.S. Healthy Ageing Reduces the Precision of Episodic Memory Retrieval. Psychol. Aging 2020 , 35 , 124–142. [ Google Scholar ] [ CrossRef ]
- Kiyonaga, A.; Scimeca, J.M.; Bliss, D.P.; Whitney, D. Serial Dependence across Perception, Attention, and Memory. Trends Cogn. Sci. 2017 , 21 , 493–497. [ Google Scholar ] [ CrossRef ]
- Makovski, T.; Jiang, Y.V. Proactive Interference from Items Previously Stored in Visual Working Memory. Mem. Cogn. 2008 , 36 , 43–52. [ Google Scholar ] [ CrossRef ] [ Green Version ]
- Sun, S.Z.; Fidalgo, C.; Barense, M.D.; Lee, A.C.H.; Cant, J.S.; Ferber, S. Erasing and Blurring Memories: The Differential Impact of Interference on Separate Aspects of Forgetting. J. Exp. Psychol. Gen. 2017 , 146 , 1606–1630. [ Google Scholar ] [ CrossRef ]
- Forsberg, A.; Guitard, D.; Greene, N.R.; Naveh-Benjamin, M.; Cowan, N. The Proportion of Working Memory Items Recoverable from Long-Term Memory Remains Fixed despite Adult Aging. Psychol. Aging 2022 , 37 , 777–786. [ Google Scholar ] [ CrossRef ]
- Peterson, D.J.; Schmidt, N.E.; Naveh-Benjamin, M. The Role of Schematic Support in Age-Related Associative Deficits in Short-Term and Long-Term Memory. J. Mem. Lang. 2017 , 92 , 79–97. [ Google Scholar ] [ CrossRef ]
- Zokaei, N.; Čepukaitytė, G.; Board, A.G.; Mackay, C.E.; Husain, M.; Nobre, A.C. Dissociable Effects of the Apolipoprotein-E (APOE) Gene on Short- and Long-Term Memories. Neurobiol. Aging 2019 , 73 , 115–122. [ Google Scholar ] [ CrossRef ] [ PubMed ]
- Bohbot, V.D.; Kalina, M.; Stepankova, K.; Spackova, N.; Petrides, M.; Nadel, L. Spatial Memory Deficits in Patients with Lesions to the Right Hippocampus and to the Right Parahippocampal Cortex. Neuropsychologia 1998 , 36 , 1217–1238. [ Google Scholar ] [ CrossRef ] [ PubMed ]
- Stepankova, K.; Fenton, A.A.; Pastalkova, E.; Kalina, M.; Bohbot, V.D. Object–Location Memory Impairment in Patients with Thermal Lesions to the Right or Left Hippocampus. Neuropsychologia 2004 , 42 , 1017–1028. [ Google Scholar ] [ CrossRef ]
- Brady, T.F.; Konkle, T.; Alvarez, G.A.; Oliva, A. Visual Long-Term Memory Has a Massive Storage Capacity for Object Details. Proc. Natl. Acad. Sci. USA 2008 , 105 , 14325–14329. [ Google Scholar ] [ CrossRef ] [ Green Version ]
- Brodeur, M.B.; Dionne-Dostie, E.; Montreuil, T.; Lepage, M. The Bank of Standardized Stimuli (BOSS), a New Set of 480 Normative Photos of Objects to Be Used as Visual Stimuli in Cognitive Research. PLoS ONE 2010 , 5 , e10773. [ Google Scholar ] [ CrossRef ] [ Green Version ]
- Brunner, E.; Puri, M.L. Nonparametric Methods in Factorial Designs. Stat. Pap. 2001 , 42 , 1–52. [ Google Scholar ] [ CrossRef ]
- Noguchi, K.; Gel, Y.R.; Brunner, E.; Konietschke, F. NparLD: An R Software Package for the Nonparametric Analysis of Longitudinal Data in Factorial Experiments. J. Stat. Soft. 2012 , 50 , 1–23. [ Google Scholar ] [ CrossRef ] [ Green Version ]
- Hsieh, S.; Schubert, S.; Hoon, C.; Mioshi, E.; Hodges, J.R. Validation of the Addenbrooke’s Cognitive Examination III in Frontotemporal Dementia and Alzheimer’s Disease. Dement. Geriatr. Cogn. Disord. 2013 , 36 , 242–250. [ Google Scholar ] [ CrossRef ]
- Li, Q.; Joo, S.J.; Yeatman, J.D.; Reinecke, K. Controlling for Participants’ Viewing Distance in Large-Scale, Psychophysical Online Experiments Using a Virtual Chinrest. Sci. Rep. 2020 , 10 , 904. [ Google Scholar ] [ CrossRef ]
- Kolarik, B.S.; Baer, T.; Shahlaie, K.; Yonelinas, A.P.; Ekstrom, A.D. Close but No Cigar: Spatial Precision Deficits Following Medial Temporal Lobe Lesions Provide Novel Insight into Theoretical Models of Navigation and Memory. Hippocampus 2018 , 28 , 31–41. [ Google Scholar ] [ CrossRef ] [ PubMed ]
- Yonelinas, A.P. The Hippocampus Supports High-Resolution Binding in the Service of Perception, Working Memory and Long-Term Memory. Behav. Brain Res. 2013 , 254 , 34–44. [ Google Scholar ] [ CrossRef ] [ PubMed ] [ Green Version ]
- Chamberlain, J.D.; Gagnon, H.; Lalwani, P.; Cassady, K.E.; Simmonite, M.; Seidler, R.D.; Taylor, S.F.; Weissman, D.H.; Park, D.C.; Polk, T.A. GABA Levels in Ventral Visual Cortex Decline with Age and Are Associated with Neural Distinctiveness. Neurobiol. Aging 2021 , 102 , 170–177. [ Google Scholar ] [ CrossRef ] [ PubMed ]
- Jorge, L.; Canário, N.; Quental, H.; Bernardes, R.; Castelo-Branco, M. Is the Retina a Mirror of the Aging Brain? Aging of Neural Retina Layers and Primary Visual Cortex Across the Lifespan. Front. Aging Neurosci. 2020 , 11 , 360. [ Google Scholar ] [ CrossRef ] [ Green Version ]
- Pitchaimuthu, K.; Wu, Q.; Carter, O.; Nguyen, B.N.; Ahn, S.; Egan, G.F.; McKendrick, A.M. Occipital GABA Levels in Older Adults and Their Relationship to Visual Perceptual Suppression. Sci. Rep. 2017 , 7 , 14231. [ Google Scholar ] [ CrossRef ] [ PubMed ] [ Green Version ]
- Grogan, J.P.; Fallon, S.J.; Zokaei, N.; Husain, M.; Coulthard, E.J.; Manohar, S.G. A New Toolbox to Distinguish the Sources of Spatial Memory Error. J. Vis. 2020 , 20 , 6. [ Google Scholar ] [ CrossRef ]
- Schurgin, M.W. Visual Memory, the Long and the Short of It: A Review of Visual Working Memory and Long-Term Memory. Atten. Percept. Psychophys. 2018 , 80 , 1035–1056. [ Google Scholar ] [ CrossRef ] [ Green Version ]
- Babu, R.J.; Leat, S.J.; Irving, E.L. Effect of Age and Pop out Distracter on Attended Field of View. J. Optom. 2014 , 7 , 229–237. [ Google Scholar ] [ CrossRef ] [ Green Version ]
- Owsley, C. Visual Processing Speed. Vis. Res. 2013 , 90 , 52–56. [ Google Scholar ] [ CrossRef ]
Click here to enlarge figure
Young | Middle-Aged | Older | p | |
---|---|---|---|---|
n | 20 | 20 | 20 | - |
Age | 25.2 (4.9) | 49.6 (7.0) | 70.8 (4.4) | - |
Male/Female | 11/9 | 6/14 | 9/11 | 0.28 |
Years in education | 17.8 (2.4) | 17.5 (3.4) | 16.8 (4.6) | 0.66 |
ACE-III Total | - | 96.5 (2.8) | 96.2 (2.1) | 0.67 |
The statements, opinions and data contained in all publications are solely those of the individual author(s) and contributor(s) and not of MDPI and/or the editor(s). MDPI and/or the editor(s) disclaim responsibility for any injury to people or property resulting from any ideas, methods, instructions or products referred to in the content. |
Share and Cite
Čepukaitytė, G.; Thom, J.L.; Kallmayer, M.; Nobre, A.C.; Zokaei, N. The Relationship between Short- and Long-Term Memory Is Preserved across the Age Range. Brain Sci. 2023 , 13 , 106. https://doi.org/10.3390/brainsci13010106
Čepukaitytė G, Thom JL, Kallmayer M, Nobre AC, Zokaei N. The Relationship between Short- and Long-Term Memory Is Preserved across the Age Range. Brain Sciences . 2023; 13(1):106. https://doi.org/10.3390/brainsci13010106
Čepukaitytė, Giedrė, Jude L. Thom, Melvin Kallmayer, Anna C. Nobre, and Nahid Zokaei. 2023. "The Relationship between Short- and Long-Term Memory Is Preserved across the Age Range" Brain Sciences 13, no. 1: 106. https://doi.org/10.3390/brainsci13010106
Article Metrics
Article access statistics, supplementary material.
ZIP-Document (ZIP, 204 KiB)
Further Information
Mdpi initiatives, follow mdpi.
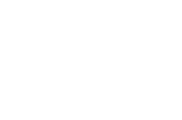
Subscribe to receive issue release notifications and newsletters from MDPI journals

- News Releases
How do our memories last a lifetime? New study offers a biological explanation
Ground-breaking research uncovers “molecular glue” that helps ensure memory formation and stabilization
New York University
Memories are stored by the interaction of two proteins: a structural protein, KIBRA (green), that acts as a persistent synaptic tag, and a synapse-strengthening enzyme, protein kinase Mzeta (red). Drugs that disrupt the memory-perpetuating interaction (other colors) erase pre-established long-term and remote memories.
Credit: Changchi Hsieh, Ph.D.
Whether it’s a first-time visit to a zoo or when we learned to ride a bicycle, we have memories from our childhoods kept well into adult years. But what explains how these memories last nearly an entire lifetime?
A new study in the journal Science Advances , conducted by a team of international researchers, has uncovered a biological explanation for long-term memories. It centers on the discovery of the role of a molecule, KIBRA, that serves as a “glue” to other molecules, thereby solidifying memory formation.
“Previous efforts to understand how molecules store long-term memory focused on the individual actions of single molecules,” explains André Fenton, a professor of neural science at New York University and one of the study’s principal investigators. “Our study shows how they work together to ensure perpetual memory storage.”
“A firmer understanding of how we keep our memories will help guide efforts to illuminate and address memory-related afflictions in the future,” adds Todd Sacktor, a professor at SUNY Downstate Health Sciences University and one of the study’s principal investigators.
It’s been long-established that neurons store information in memory as the pattern of strong synapses and weak synapses, which determines the connectivity and function of neural networks. However, the molecules in synapses are unstable, continually moving around in the neurons, and wearing out and being replaced in hours to days, thereby raising the question: How, then, can memories be stable for years to decades?
In a study using laboratory mice, the scientists focused on the role of KIBRA, or kidney and brain expressed protein, the human genetic variants of which are associated with both good and poor memory. They focused on KIBRA’s interactions with other molecules crucial to memory formation—in this case, protein kinase Mzeta (PKMzeta). This enzyme is the most crucial molecule for strengthening normal mammalian synapses that is known, but it degrades after a few days.
Their experiments reveal that KIBRA is the “missing link” in long-term memories, serving as a “persistent synaptic tag,” or glue, that sticks to strong synapses and to PKMzeta while also avoiding weak synapses.
“During memory formation the synapses involved in the formation are activated—and KIBRA is selectively positioned in these synapses,” explains Sacktor, a professor of physiology, pharmacology, anesthesiology, and neurology at SUNY Downstate. “PKMzeta then attaches to the KIBRA-synaptic-tag and keeps those synapses strong. This allows the synapses to stick to newly made KIBRA, attracting more newly made PKMzeta.”
More specifically, their experiments in the Science Advances paper show that breaking the KIBRA-PKMzeta bond erases old memory. Previous work had shown that randomly increasing PKMzeta in the brain enhances weak or faded memories, which was mysterious because it should have done the opposite by acting at random locations, but the persistent synaptic tagging by KIBRA explains why the additional PKMzeta was memory enhancing, by only acting at the KIBRA tagged sites.
“The persistent synaptic tagging mechanism for the first time explains these results that are clinically relevant to neurological and psychiatric disorders of memory,” observes Fenton, who is also on the faculty at NYU Langone Medical Center’s Neuroscience Institute.
The paper’s authors note that the research affirms a concept introduced in 1984 by Francis Crick. Sacktor and Fenton point out that his proposed hypothesis to explain the brain’s role in memory storage despite constant cellular and molecular changes is a Theseus’s Ship mechanism—borrowed from a philosophical argument stemming from Greek mythology in which new planks replace old ones to maintain Theseus’s Ship for years.
“The persistent synaptic tagging mechanism we found is analogous to how new planks replace old planks to maintain Theseus’s Ship for generations, and allows memories to last for years even as the proteins maintaining the memory are replaced,” says Sacktor. “Francis Crick intuited this Theseus’s Ship mechanism, even predicting the role for a protein kinase. But it took 40 years to discover that the components are KIBRA and PKMzeta and to work out the mechanism of their interaction.”
The study also included researchers from Canada’s McGill University, Germany’s University Hospital of Münster, and University of Texas Medical School at Houston.
This work was supported by grants from the National Institutes of Health (R37 MH057068, R01 MH115304, R01 NS105472, R01 MH132204, R01 NS108190), the Natural Sciences and Engineering Research Council of Canada Discovery (203523), and the Garry and Sarah S. Sklar Fund.
About New York University Founded in 1831, NYU is one of the world’s foremost research universities (with more than $1 billion per year in research expenditures, it is ranked seventh among private research universities) and is a member of the selective Association of American Universities. NYU has degree-granting university campuses in New York, Abu Dhabi, and Shanghai; has 13 other global academic sites, including London, Paris, Florence, Tel Aviv, Buenos Aires, and Accra, and US sites in Washington, DC, Los Angeles, CA, and Tulsa, OK; and both sends more students to study abroad and educates more international students than any other U.S. college or university. Through its numerous schools and colleges, NYU is a leader in conducting research and providing education in the arts and sciences, law, medicine, business, dentistry, engineering, education, nursing, the cinematic and performing arts, music and studio arts, public service, social work, public health, and professional studies, among other areas.
About SUNY Downstate Health Sciences University Downstate Health Sciences University in Brooklyn is one of four academic health centers (AMCs) in the 64-campus State University of New York (SUNY) system and the only SUNY AMC in New York City dedicated to health education, research, and patient care for the borough’s 2.7 million residents. Its flagship hospital, University Hospital at Downstate (UHD), is a teaching hospital that benefits from the expertise of Downstate’s exceptional medical school and world-class research facilities. Beyond its clinical excellence, Downstate houses a range of esteemed educational institutions, including the College of Medicine, College of Nursing, School of Health Professions, School of Graduate Studies, and School of Public Health. Downstate fosters innovation through its multifaceted biotechnology initiatives, including the Biotechnology Incubator and BioBAT, which support both early-stage and more mature biotech companies. Downstate’s research enterprise drives innovation and discovery across a wide array of disciplines. Our investigators are making discoveries that are changing the world and pushing the boundaries of what is possible in biomedicine and healthcare.
Science Advances
10.1126/sciadv.adl0030
Method of Research
Experimental study
Subject of Research
Article title.
KIBRA anchoring the action of PKMζ maintains the persistence of memory
Article Publication Date
26-Jun-2024
Disclaimer: AAAS and EurekAlert! are not responsible for the accuracy of news releases posted to EurekAlert! by contributing institutions or for the use of any information through the EurekAlert system.
Featured Topics
Featured series.
A series of random questions answered by Harvard experts.
Explore the Gazette
Read the latest.

Putting human past on the MAPS
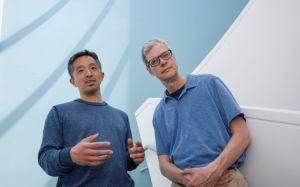
Does AI help humans make better decisions?
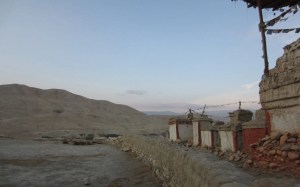
Tracking entwined histories of malaria, humans
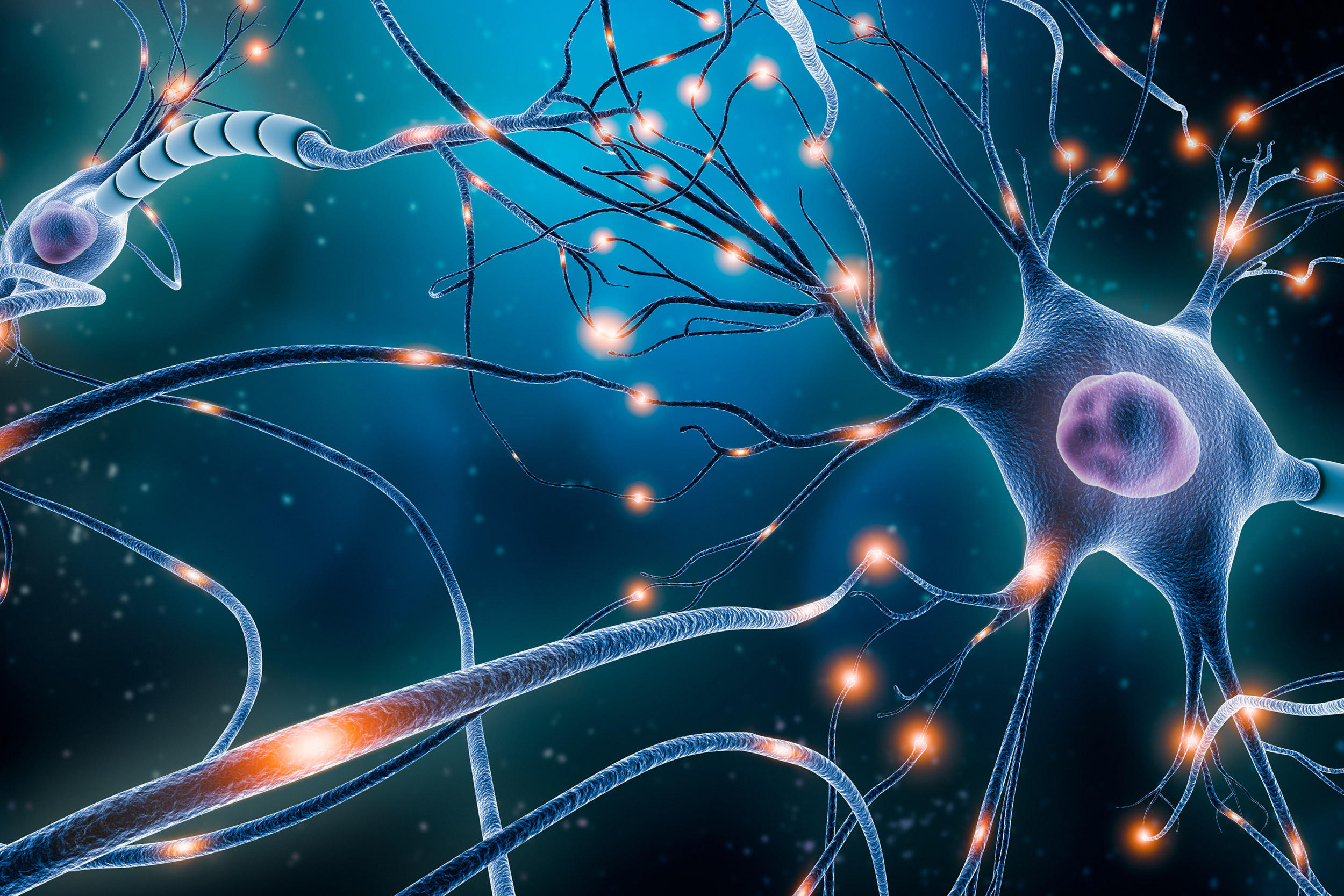
Making memories
Kevin Jiang
HMS Communications
Study sheds light on how neurons form long-term memories
On a late summer day in 1953, a young man who would soon be known as patient H.M. underwent experimental surgery. In an attempt to treat his debilitating seizures, a surgeon removed portions of his brain, including part of a structure called the hippocampus. The seizures stopped.
Unfortunately, for patient H.M., so too did time. When he woke up after surgery, he could no longer form new long-term memories, despite retaining normal cognitive abilities, language and short-term working memory. Patient H.M.’s condition ultimately revealed that the brain’s ability to create long-term memories is a distinct process that depends on the hippocampus.
Scientists had discovered where memories are made. But how they are made remained unknown.
Now, neuroscientists at Harvard Medical School (HMS) have taken a decisive step in the quest to understand the biology of long-term memory and find ways to intervene when memory deficits occur with age or disease.
Reporting in Nature on Dec. 9, they describe a newly identified mechanism that neurons in the adult mouse hippocampus use to regulate signals they receive from other neurons, in a process that appears critical for memory consolidation and recall.
The study was led by Lynn Yap , HMS graduate student in neurobiology, and Michael Greenberg , chair of neurobiology in the Blavatnik Institute at HMS.
“If we can better understand this process, we will have new handles on memory and how to intervene when things go wrong, …” Michael Greenberg, Blavatnik Institute at HMS
“Memory is essential to all aspects of human existence. The question of how we encode memories that last a lifetime is a fundamental one, and our study gets to the very heart of this phenomenon,” said Greenberg, the HMS Nathan Marsh Pusey Professor of Neurobiology and study corresponding author.
The researchers observed that new experiences activate sparse populations of neurons in the hippocampus that express two genes, Fos and Scg2. These genes allow neurons to fine-tune inputs from so-called inhibitory interneurons, cells that dampen neuronal excitation. In this way, small groups of disparate neurons may form persistent networks with coordinated activity in response to an experience.
“This mechanism likely allows neurons to better talk to each other so that the next time a memory needs to be recalled, the neurons fire more synchronously,” Yap said. “We think coincident activation of this Fos-mediated circuit is potentially a necessary feature for memory consolidation, for example, during sleep, and also memory recall in the brain.”
Circuit orchestration
In order to form memories, the brain must somehow wire an experience into neurons so that when these neurons are reactivated, the initial experience can be recalled. In their study, Greenberg, Yap and team set out to explore this process by looking at the gene Fos.
First described in neuronal cells by Greenberg and colleagues in 1986, Fos is expressed within minutes after a neuron is activated. Scientists have taken advantage of this property, using Fos as a marker of recent neuronal activity to identify brain cells that regulate thirst, torpor , and many other behaviors.
Scientists hypothesized that Fos might play a critical role in learning and memory, but for decades, the precise function of the gene has remained a mystery.
To investigate, the researchers exposed mice to new environments and looked at pyramidal neurons, the principal cells of the hippocampus. They found that relatively sparse populations of neurons expressed Fos after exposure to a new experience. Next, they prevented these neurons from expressing Fos, using a virus-based tool delivered to a specific area of the hippocampus, which left other cells unaffected.
Mice that had Fos blocked in this manner showed significant memory deficits when assessed in a maze that required them to recall spatial details, indicating that the gene plays a critical role in memory formation.
The researchers studied the differences between neurons that expressed Fos and those that did not. Using optogenetics to turn inputs from different nearby neurons on or off, they discovered that the activity of Fos-expressing neurons was most strongly affected by two types of interneurons.
Neurons expressing Fos were found to receive increased activity-dampening, or inhibitory, signals from one distinct type of interneuron and decreased inhibitory signals from another type. These signaling patterns disappeared in neurons with blocked Fos expression.
“What’s critical about these interneurons is that they can regulate when and how much individual Fos-activated neurons fire, and also when they fire relative to other neurons in the circuit,” Yap said. “We think that at long last we have a handle on how Fos may in fact support memory processes, specifically by orchestrating this type of circuit plasticity in the hippocampus.”
Imagine the day
The researchers further probed the function of Fos, which codes for a transcription factor protein that regulates other genes. They used single-cell sequencing and additional genomic screens to identify genes activated by Fos and found that one gene in particular, Scg2, played a critical role in regulating inhibitory signals.
In mice with experimentally silenced Scg2, Fos-activated neurons in the hippocampus displayed a defect in signaling from both types of interneurons. These mice also had defects in theta and gamma rhythms, brain properties thought to be critical features of learning and memory.
Previous studies had shown that Scg2 codes for a neuropeptide protein that can be cleaved into four distinct forms, which are then secreted. In the current study, Yap and colleagues discovered that neurons appear to use these neuropeptides to fine-tune inputs they receive from interneurons.
Together, the team’s experiments suggest that after a new experience, a small group of neurons simultaneously express Fos, activating Scg2 and its derived neuropeptides, in order to establish a coordinated network with its activity regulated by interneurons.
“When neurons are activated in the hippocampus after a new experience, they aren’t necessarily linked together in any particular way in advance,” Greenberg said. “But interneurons have very broad axonal arbors, meaning they can connect with and signal to many cells at once. This may be how a sparse group of neurons can be linked together to ultimately encode a memory.”
The study findings represent a possible molecular- and circuit-level mechanism for long-term memory. They shed new light on the fundamental biology of memory formation and have broad implications for diseases of memory dysfunction.
The researchers note, however, that while the results are an important step in our understanding of the inner workings of memory, numerous unanswered questions about the newly identified mechanisms remain.
“We’re not quite at the answer yet, but we can now see many of the next steps that need to be taken,” Greenberg said. “If we can better understand this process, we will have new handles on memory and how to intervene when things go wrong, whether in age-related memory loss or neurodegenerative disorders such as Alzheimer’s disease.”
The findings also represent the culmination of decades of research, even as they open new avenues of study that will likely take decades more to explore, Greenberg added.
“I arrived at Harvard in 1986, just as my paper describing the discovery that neuronal activity can turn on genes was published,” he said. “Since that time, I’ve been imagining the day when we would figure out how genes like Fos might contribute to long-term memory.”
Additional authors include Noah Pettit, Christopher Davis, M. Aurel Nagy, David Harmin, Emily Golden, Onur Dagliyan, Cindy Lin, Stephanie Rudolph, Nikhil Sharma, Eric Griffith, and Christopher Harvey.
The study was supported by the National Institutes of Health (grants R01NS028829, R01NS115965, R01NS089521, T32NS007473 and F32NS112455), a Stuart H.Q. and Victoria Quan fellowship, a Harvard Department of Neurobiology graduate fellowship, an Aramont Fund.
Share this article
You might like.

Harvard digital atlas plots patterns from history ancient and modern

One judge’s track record — with and without algorithm — surprises researchers

New study of ancient genomes tracks disease over 5,500 years, factors in spread, including trade, warfare, colonialism, and slavery
When should Harvard speak out?
Institutional Voice Working Group provides a roadmap in new report
Had a bad experience meditating? You're not alone.
Altered states of consciousness through yoga, mindfulness more common than thought and mostly beneficial, study finds — though clinicians ill-equipped to help those who struggle
College sees strong yield for students accepted to Class of 2028
Financial aid was a critical factor, dean says
ORIGINAL RESEARCH article
Long-term visual memory and its role in learning suppression.
- 1 Department of Neurosurgery, Harvard Medical School, Massachusetts General Hospital, Boston, MA, United States
- 2 Department of Neurobiology, Harvard University, Cambridge, MA, United States
- 3 Harvard-MIT Health Sciences and Technology, Boston, MA, United States
- 4 Program in Neuroscience, Harvard Medical School, Harvard University, Boston, MA, United States
Long-term memory is a core aspect of human learning that permits a wide range of skills and behaviors often important for survival. While this core ability has been broadly observed for procedural and declarative memory, whether similar mechanisms subserve basic sensory or perceptual processes remains unclear. Here, we use a visual learning paradigm to show that training humans to search for common visual features in the environment leads to a persistent improvement in performance over consecutive days but, surprisingly, suppresses the subsequent ability to learn similar visual features. This suppression is reversed if the memory is prevented from consolidating, while still permitting the ability to learn multiple visual features simultaneously. These findings reveal a memory mechanism that may enable salient sensory patterns to persist in memory over prolonged durations, but which also functions to prevent false-positive detection by proactively suppressing new learning.
Introduction
Learning is an essential aspect of human behavior ( Squire, 2004 ). At its core, learning allows us to improve our performance in activities, such as playing the piano, over time but whether similar mechanisms of memory underlie other basic sensory processes remain unclear. It is well known that memory can take either implicit forms, which underlie procedural skills such as riding a bicycle, or explicit forms, which underlie the remembrance of factual content, such as being able to recall the year of one’s wedding anniversary ( Schacter et al., 1993 ; Rugg et al., 1998 ). Visual search falls within the realm of implicit memory as it concerns a sensory process rather than a fact-based learning structure ( Chun and Jiang, 1998 ). The extension of memory to the visual system is far less understood compared with other sensory or motor processes ( Kristjánsson, 2000 ). Visual search is a task in which a participant must distinguish the identity and/or position of a “target” visual item from an array of “distractor” visual items ( Sireteanu and Rettenbach, 1995 ). It is thought to be a primitive skill that allows us to rapidly identify visual features in our environment – from predators hiding in the bushes to a particular item of clothing ( Yang and Zelinsky, 2009 ).
The process of visual search requires the coordination of multiple elements and processes including oculomotor control, covert visual attention, temporal integration of visual information, and memory of scene configuration ( Eckstein, 2011 ). The components of visual search that are expected to be affected by training include oculomotor fixations and contralateral delay activity, an electrophysiological characteristic of visual working memory ( Peterson et al., 2001 , 2007 ; Emrich et al., 2009 ). Attentional systems are also known to play an important role in influencing search patterns during visual search ( Jiang, 2018 ), while statistical learning is thought to influence how attention is distributed during visual search experiments ( Failing and Theeuwes, 2018 ; Ferrante et al., 2018 ).
With repeated practice, this ability to identify specific contextual features can also improve over the course of a single training session ( Eckstein, 2011 ). For example, it has been shown that repeated visual search can lead to improvement in performance over seconds to minutes ( Võ and Wolfe, 2012 ) and that this increase in performance can be influenced by the presence or absence distractor visual items ( Schneider and Fisk, 1982 ; Boettcher et al., 2013 ). It has also been found that repeated search for similarly positioned items can persist after training ( Geyer et al., 2013 ), although questions remain to what extent this effect is dependent on memory for where visual items are presented in space as opposed to the extent that it is a memory for the visual items themselves ( Jiang and Wagner, 2004 ; Travis et al., 2013 ).
These observations demonstrate that repeated search for specific visual features can improve with training, and also raise the intriguing possibility that this improvement could be further subserved by a form of long-term memory. In particular, if visual search is indeed modulated by memory processes, then we should expect that repeated training could lead to long-term improvement over consecutive days or perhaps be susceptible to phenomena observed in other forms of long-term memory such as consolidation, interference, and selectivity ( Brady et al., 2008 ; Cunningham et al., 2015 ).
Materials and Methods
Here, we devised a visual search paradigm that required participants to search for particular items from other similar items presented on a screen (representative arrays shown in Figures 1A,B ). In order to distinguish between simple procedural improvements in response (due to task familiarity) and to evaluate the selectivity of visual long-term memory, two orthogonal categories of items were presented; (i) summer vs. winter clothing and (ii) upper vs. lower body clothing. Clothing items were chosen because of their validation in prior visual search experiments ( Wolfe et al., 2000 , 2007 ; Nako et al., 2014 ). The participants were always asked to identify the summer clothing item, as variations were made in whether summer/winter or upper/lower body clothing were presented and at what times over the course of the study they were given (Experiments 1–3).
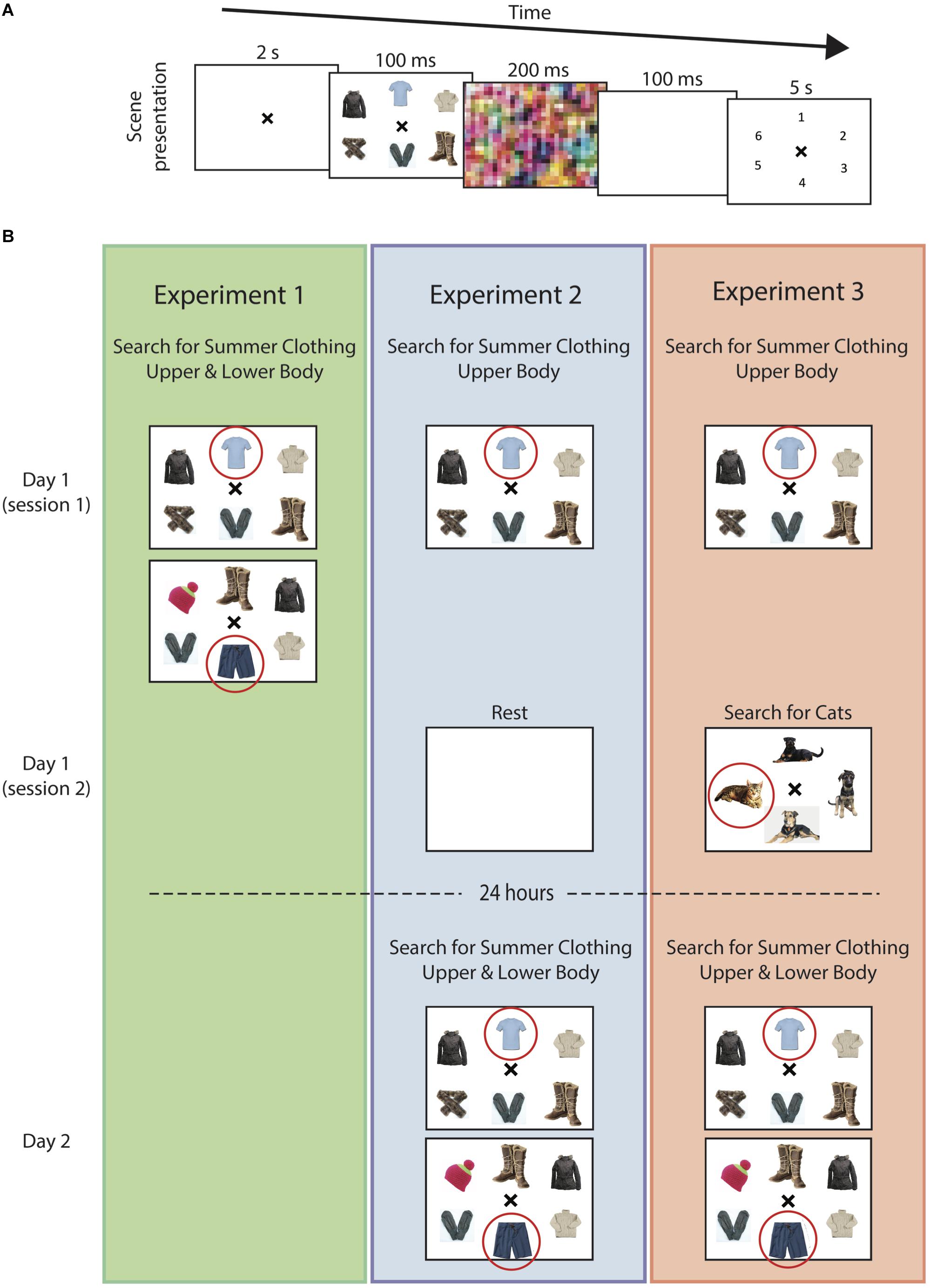
FIGURE 1. Visual search task. (A) In all experiments, subjects were shown an array of winter clothing items (distractors) with, in most cases, a single summer clothing item (target). Each array was followed by a visual screen. Subjects were then asked to select the location corresponding to the target search item. (B) In Experiment 1 ( left ), subjects searched for both upper-body and lower-body clothing items in randomly interspersed trials. In Experiment 2 ( center ), participants searched for upper-body clothing items on Day 1 and following 24 h, searched for upper-body and lower-body clothing items in randomly interspersed trials, similar to the paradigm used in Experiment 1. In Experiment 3 ( right ), participants searched for upper-body items on Day 1, similar to Experiment 2. Immediately following this experiment, subjects then performed an unrelated visual search task (cats vs. dogs). The following day, similar to Experiment 2, participants searched for both upper-body and lower-body clothing items in randomly interspersed trials. The circled item represents the target search summer clothing article (these were not shown during the task). Images were selected via with search engine provided at https://search.creativecommons.org .
Three main experiments were performed. For the first experiment, the participants were shown a slide with either a single upper-body or lower-body summer clothing item (e.g., short sleeve shirts, skirt, bathing suit, flip flop, etc.) that was positioned within an array of winter-clothing (e.g., sweaters, coats, scarves, gloves, etc.). For Experiment 1, the distractor items could come from either sub-category (i.e., a lower-body summer clothing item was surrounded by both upper- and lower-body winter clothing distractor items). The participants were only instructed to find the summer clothing item, without reference to whether the target item was an upper-body or lower-body clothing item. The upper- and lower-body summer clothing slides were randomly interspersed in order to assess baseline levels of task difficulty and learning patterns over the course of simultaneous training. The following two experiments were designed to assess for the presence of long-term visual memory and its selectivity. In Experiment 2, participants on the 1st day were asked to identify an upper-body summer-clothing item out of an array of winter clothing items. The next day, they had to identify either upper-body or lower-body summer clothing, again out of an array of winter clothing (i.e., the same as the first experiment but on Day 2). Experiment 3 was identical to Experiment 2 except that participants were given an interference task immediately following training in which they had to search for a new, unrelated set of items. For Experiments 2 and 3, distractor items on Day 1 were only upper-body clothing items, and upper- and lower-body items for Day 2 (consistent with the target items).
Experiment 1 was designed to evaluate baseline learning performances for the upper-body and lower-body search tasks. In contrast to the following two experiments, here, participants were asked to search for either upper- or lower-body summer clothing items on randomly interspersed trials throughout the experiment (Figure 1B ). This allowed us to assess whether potential differences in learning over time could be due to a simple difference in baseline performance prior to learning or difficulty with learning two, within-category items, simultaneously. The participants performed a total of 88 trials over the course of this experiment.
Experiment 2 was designed to assess whether searching for items within a particular category can lead to sustained improvements in performance after 24 h, without intermediate training, and whether this improvement in performance was selective to the particular items being searched. As described above, the participants were first required to search for upper-body summer clothing from an array of winter clothing on Day 1 (Figure 1 ). To further examine the selectivity of training, on Day 1 the participants were given only an upper-body summer clothing item whereas on Day 2, after 24 h, they were given both upper- and lower-body summer clothing items (identical to Experiment 1). The participants performed a total of 88 such trials over the course of Day 1 and were then required to rest for 15 min after training without mental effort. After 24 h, they returned to complete the second search task.
Experiment 3 was used to examine whether the introduction of an interference task following training on Day 1 could influence the learning-suppression effect observed on Day 2 and further confirm that this effect is memory-selective. Here, the participants performed a similar set of trials as experiment 1, but now had to search for items within a different entry-level, rather than subordinate-level, category after training on Day 1 (Figure 1 ). The participants waited 15 min after finishing the initial visual memory task before starting the interference task.
The task was performed during mid-day so that it would have no interference with participants’ sleep-wake cycle, and participants were asked not to make adjustments to their normal daily routine. A total of 14 individuals participated in these experiments, with each participant only participating in one of the three experiments (ages 19–31, M:F ratio of 9:6). The experiments outlined in this study were found to meet the criteria for exemption by the Harvard Medical School Institutional Review Board. Therefore, an ethics approval was not required per our institution’s guidelines and United States federal regulations. Nevertheless, subjects provided their verbal informed consent prior to the initiation of their participation in this study.
For each experiment, the participants were presented with a screen containing the visual search task and were asked to identify a summer clothing item out of an array of otherwise winter clothing items. Each trial began with a blank screen and a central ‘x’ that provided a cue for the upcoming search task. Following cue presentation, the participants were presented with a random selection of items positioned in an equidistant circular configuration for 100 ms. On most trials, this arrangement constituted 1 article of summer clothing and 4–5 articles of winter clothing randomly positioned in circular configuration. These were then erased, and followed by the presentation of a visual mask. Finally, the participants were then given numbers at the same, previously presented locations, and were asked in which location, if any, they observed a piece of summer clothing (Figure 1A ).
To limit guessing or a stereotypic spatial bias, 18% of trials contained no summer clothing and 31% of trials contained 4 rather than 5 upper-body winter clothing items. The items were randomly selected from a set of 19 target items (i.e., summer clothing) and 19 distractors (i.e., winter clothing), randomly positioned on the screen. If the participants did not see a piece of summer clothing, they were asked to select “none.” Finally, similar to prior reports ( Potter et al., 2010 ), we measured performance in terms of accuracy in order to emphasize learning based on search rather than response reaction times which can be confounded by strategic spatial searching or variations in motoric performance between individuals ( Cunningham and Wolfe, 2014 ).
Statistical Analysis
The general approach taken in the data analysis was to assess differences in performance by using the paired t -test which allowed us to determine individual changes in performance, and by extension, memory, at the group level. Performance accuracy was quantified by tallying the participants responses with the correct target item location and dividing this number of correct responses by the total number of arrays visualized during the task. Experimental epochs were aggregated into time periods, the first half of an experiment and the second half, in order to assess changes in learning over the course of a single day, and to compare these changes on subsequent days. Data analysis was performed using MATLAB.
In Experiment 1, participants demonstrated a significant improvement in performance over the course of training for both the lower-body (43% vs. 68%; paired t -test, df = 3, d = -1.60, t s = -3.20, p = 0.049) and upper-body visual search components (62% vs. 78%; paired t -test, df = 3, d = -1.91, t s = -3.82, p = 0.031; Figure 2A ) when comparing the first- vs. second-half of the task. Taking these items together, the participants demonstrated significant learning over the course of training (52% vs. 75%; paired t -test, df = 3, d = -2.33, t s = -4.68, p = 0.019). There was no significant difference in performance for upper-body clothing and lower-body clothing on the second half of Day 1 (78% vs. 68%; paired t -test, df = 3, d = 0.30, t s = 0.77, p = 0.50).
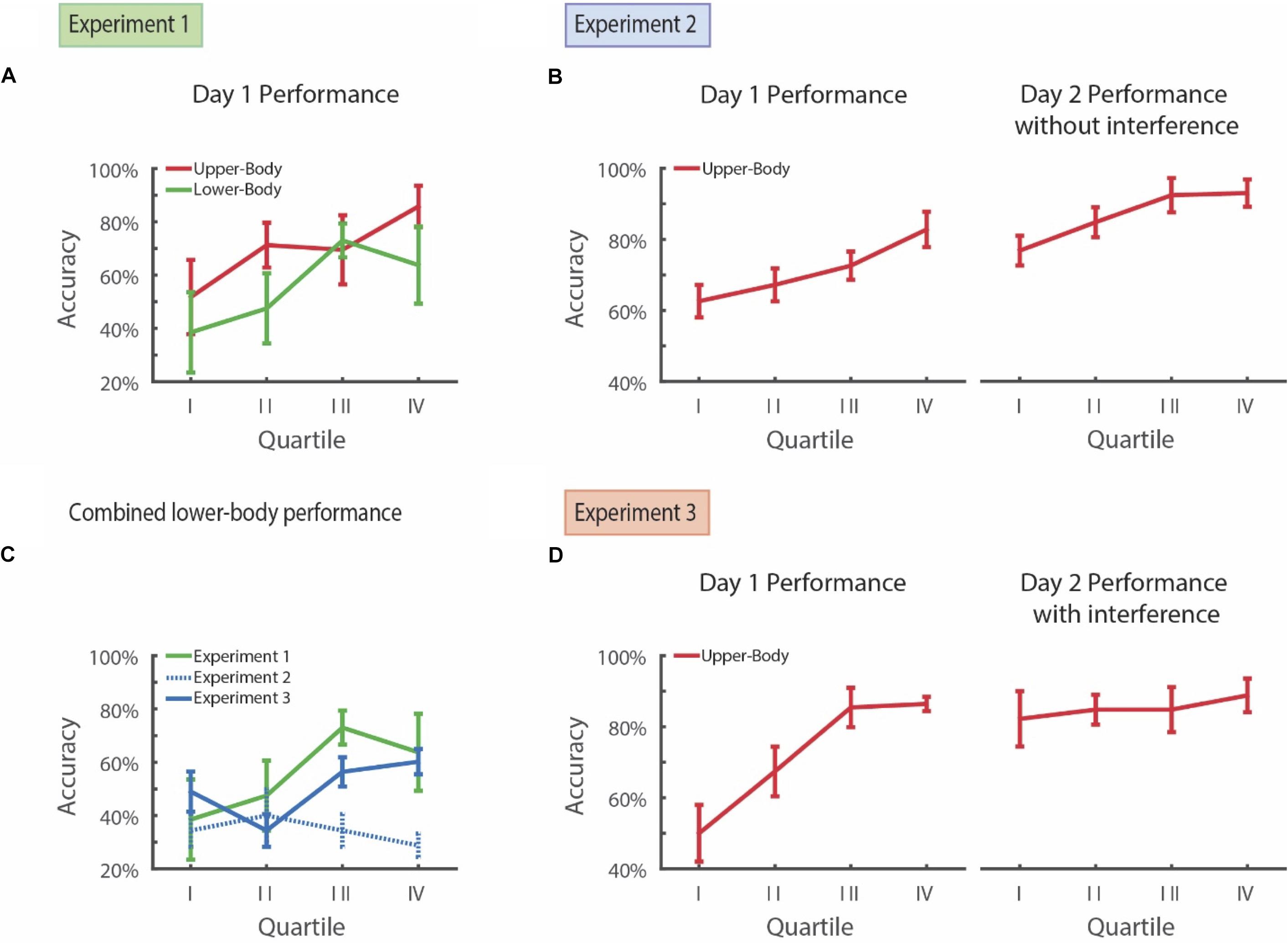
FIGURE 2. Long-term improvement in visual search after training and learning suppression. (A) Subjects performing simultaenous visual search for both upper- and lower-body in Experiment 1 demonstrate improvement in both overall and subcategory learning over the course of a single day. (B) Participants in Experiment 2 show learning over the course of Day 1 on upper-body visual search and this level of accuracy is maintained the following day for the upper-body visual search subcategory. (C) Subjects in Experiment 3, who performed the interference task on the prior day, demonstrated accuracy on the novel lower-body search task similar to subjects who participated in this task on Day 1 of Experiment 1. In contrast, subjects in Experiment 2, who did not perform the interference task on the prior day, showed significantly lower accuracy on the novel lower-body search task. (D) Subjects in Experiment 3 show improvement in upper-body learning during the course of Day 1 and, following an interference task on the 1st day, maintain this level of accuracy on the following day for the upper-body subcategory. Error bars represent ± 1 SEM .
Based on these data, we therefore conclude that (i) the participants were able to improve their performance over the course of training, (ii) they were able learn both the lower-body and upper-body items simultaneously, and (iii) there was relatively little difference in task difficulty between the lower- and upper-body items as evidenced by their final performances.
For Experiment 2, the participants demonstrated improvement in upper-body summer clothing search performance over the course of training on Day 1 (Figure 2B ). Their performance increased from 65% in the first half of the training session to 78% on the second half of the training session (paired t -test, df = 4, d = -1.62, t s = -3.61, p = 0.023). While baseline performance varied somewhat between individuals, ranging from 50 to 75% within the first half of the session, all participants demonstrated an improvement in performance (Supplementary Figure S1 ).
Next, we tested whether improvement in performance persisted after 24 h without training. We first examined the Day 2 performance on the upper-body summer clothing search. Amongst the upper-body summer clothing presentations, the same participants from Day 1 had a similar performance on the first half of Day 2 as they had on the second half of Day 1 (81% vs. 78%; paired t -test, df = 4, d = -0.32, t s = -0.72, p = 0.51). Moreover, their performance on the first half of Day 2 was significantly higher than their performance on the first half of Day 1 (81% vs. 65%; paired t -test, df = 4, d = 1.62, t s = -4.12, p = 0.015). Their performance on Day 2 continued to slightly improve over the course of the session (93% vs. 81%; paired t -test, df = 4, d = 1.63, t s = -3.64, p = 0.022; Figure 2B ). Therefore, training on Day 1 led to a sustained improvement in performance the following day.
Improvement in performance on Day 2 was selective , and did not simply result in an enhanced familiarity with the task or a generalized improvement in visual search. When we examined performance on the lower-body clothing search, which was new to these participants, their performance was 34% on the first half of Day 2. More notably, performance for lower-body clothing on the first half of Day 2 was both lower than first-half performance for upper body clothing on Day 2, at 81% (paired t -test, df = 4, d = -2.94, t s = -6.58, p = 0.0028), as well as lower than the 65% first-half accuracy of upper-body clothing search on Day 1 of Experiment 1 (paired t -test, df = 4, d = 1.6812, t s = -3.7592, p = 0.0198).
Improvement in performance on Day 2 appeared to suppress the ability to learn new lower-body items. Unlike the observed improvement in performance on Day 1 for the upper-body clothing, the participants were unable to improve their performance for the lower-body clothing over the course of Day 2. Specifically, they demonstrated no difference in performance between the first and second halves of Day 2 (37% vs. 32%; paired t -test, df = 4, d = 0.3987, t s = 0.89, p = 0.42). This difference was especially notable when comparing performances from experiment 1 and 2 (Figure 2C ). Lower-body performance for the second half of Day 2 of Experiment 2 was also significantly lower than lower-body performance for the second half of Day 1 of Experiment 1 (32% vs. 68%; two-sample t -test, df = 4, d = -4.52, t s = 3.59, p = 0.0088). Moreover, as detailed above in Experiment 1, this lower performance for upper-body clothing was not simply due to a potential difficulty difference in searching for both upper- and lower-body clothing items simultaneously.
Taken together, these observations suggest that (i) training on the upper-body summer clothing on Day 1 limited the participant’s ability to improve their performance on lower-body summer clothing on Day 2, (ii) this lack of improvement was not due to difficulty in searching for upper- and lower-body items simultaneously, and (iii) prior learning of upper-body clothing did not generalize to improved performance on lower-body clothing as would be expected from task familiarity or simple procedural improvement.
For Experiment 3, similar to before, we find that these participants improved their performance for upper-body clothing items over the course of training on Day 1 (59 to 88%; paired t -test, df = 4, d = -2.96, t s = -6.63, p = 0.0027; Figure 2D ). Here, however, immediately following training (i.e., after trial 88), they were then asked to search for pictures of cats (e.g., Tobe cat, Siamese cat, etc.) out of an array of dogs (as with the main task, some of trials contained no cats and about a third contained 4 rather than 5 dogs). Introduction of the interference task after searching for upper-body clothing on Day 1 prevented suppression of lower-body learning on Day 2. As in Experiment 1, overall upper-body search performance on Day 2 was significantly higher than lower-body search accuracy (84% vs. 50%; paired t -test, df = 4, d = 4.89, t s = -13.08, p = 0.00020). However, unlike Experiment 1, the participants in Experiment 3 demonstrated a significant improvement in performance on lower-body search over the course of the Day 2. Overall, their accuracy increased from 42 to 58% from the first half to the second half (paired t -test, df = 4, d = -1.51, t s = -3.38, p = 0.028). Lower-body performance for the second half of Day 2 of Experiment 3 was also significantly higher than that of lower-body performance for Experiment 2 (58% vs. 32%; two sample t -test, df = 4, d = 2.87, t s = 4.55, p = 0.0019). Therefore, interference on Day 1 following upper-body clothing training appeared to limit the suppressive effect of prior training on learning.
Improvement in performance for lower-body clothing in Experiment 3 was not due to a concurrent suppression of performance for upper-body clothing. Specifically, upper-body performance on the first half of Day 2 was 84% and was significantly higher than the 59% performance on the first half of Day 1 prior to interference (paired t -test, df = 4, d = 1.37, t s = -3.06, p = 0.038). Therefore, introduction of an interference task following training (i) did not lead to reduced performance for upper-body clothing the following day, but (ii) prevented the suppression of performance improvement for lower-body clothing.
Finally, we directly compared experiments 2 and 3 to evaluate the selectivity of interference on learning. We find that, when comparing the learning rates for Day 2 (difference between first and second half performance) of Experiment 2 to Experiment 3, participants who were given the interference task had a higher learning rate for the unfamiliar lower-body search task, compared to those who did not receive the interference task (two sample t -test, df = 4, d = -1.77, t s = -2.78, p = 0.024; see Figure 3 ). This suggested that both learning rate as well as absolute performance for those who received interference was higher for the novel items. By contrast, we did not observe this improvement for the group that did not receive interference, suggesting that the suppression from the previous task prevented learning from taking place. When comparing the performance solely of participants who did not receive interference (Experiment 2), they demonstrated significantly higher learning on the familiar upper-body task on day 2 compared with the unfamiliar lower-body task (two sample t -test, df = 4, d = 1.57, t s = 2.48, p = 0.038). Furthermore, these differences do not appear to be a result of differences in overall learning rates between the two groups, but rather specific to the individual upper- and lower-body clothing conditions (Supplementary Figure S2 ). We therefore conclude that the interference task prevented performance on the familiar items from suppressing improvement in performance for the novel items (see further below).
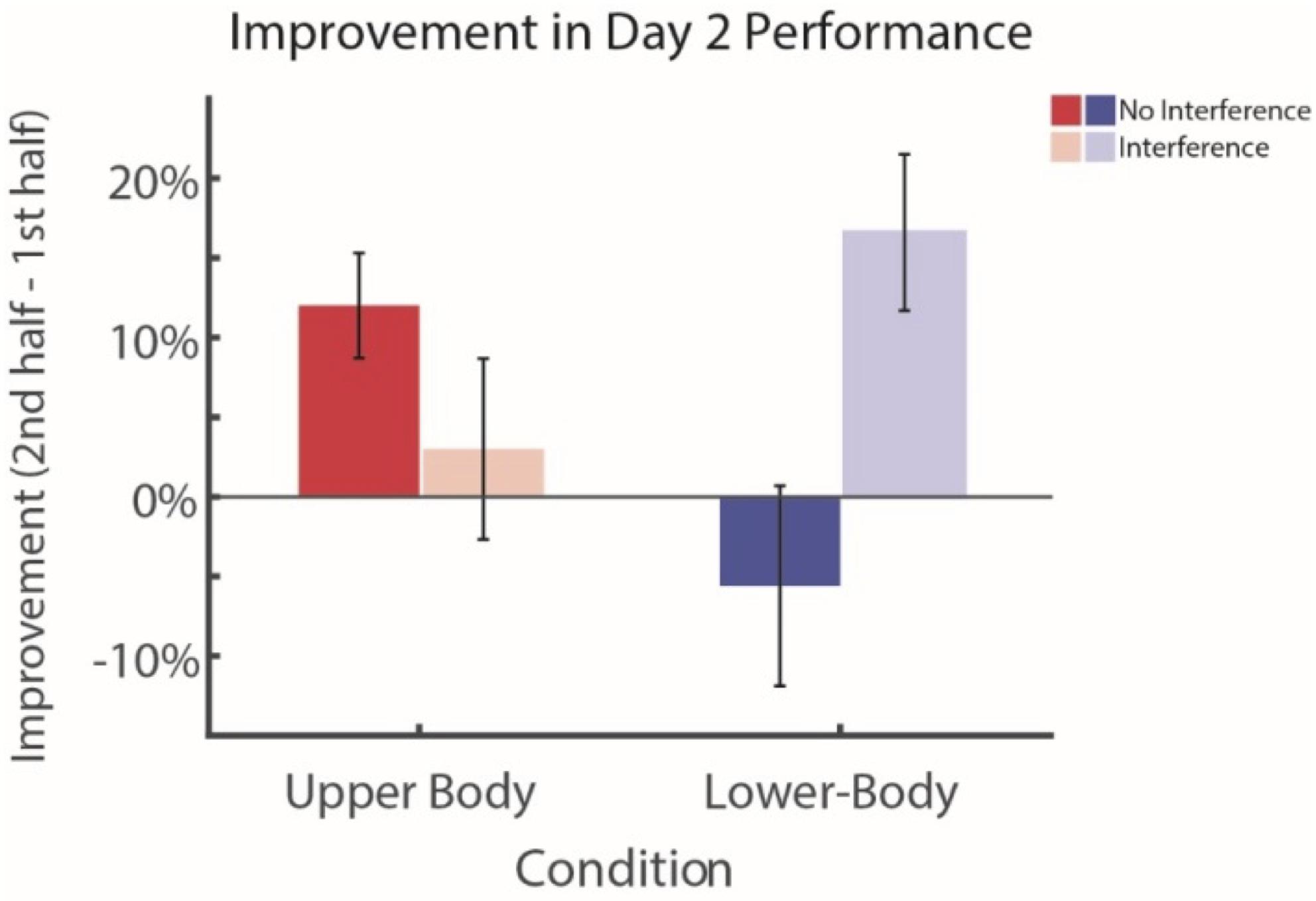
FIGURE 3. Effects of interference in long-term visual search performance and learning. Subjects who perform the interference task (Experiment 3) demonstrate significant improvement during the course of Day 2 for the novel lower-body visual search task. In contrast, subjects who do not perform the interference task (Experiment 2) demonstrate a significant decrease in learning on the novel lower-body search task, both when compared to their own improvement on the familiar upper-body search as well as in comparison to the learning of the interference group during lower-body visual search. Error bars represent ± 1 SEM .
Humans and many animals have the ability to search for and rapidly identify complex sensory features in our environment such as the presence of predators or food items ( Alexander and Zelinsky, 2011 ; Cunningham and Wolfe, 2014 ). This skill requires a rapid interplay between perceptual, attentional, executive function, and oculomotor systems ( Eckstein, 2011 ), but there is mixed evidence regarding the extent to which memory processes subserve our visual search capabilities ( Brady et al., 2011 ).
One model proposes that memory is not an integral element of visual search, either within a single trial ( Horowitz and Wolfe, 1998 ), where performance benefits if non-target distractors can be ignored, or across trials, where implicit learning patterns could theoretically produce more efficient search ( Wolfe et al., 2000 ). Moreover, there is some evidence that even in instances where short-term memory does develop during visual search, it does not provide an advantage over search that is based on simple visual feature analysis ( Oliva et al., 2004 ). This line of research proposes an “amnesic” visual system that is “eternally present,” allowing individuals to purely focus their attention on specific visual features rather than relying on past memories to promote search ability ( Horowitz and Wolfe, 1998 ).
Other models, on the other hand, have suggested a putative role for memory in the development of visual search. For example, some studies have shown that short-term memory can guide intra-trial search strategy ( Gibson et al., 2000 ; Kristjánsson, 2000 ; Peterson et al., 2007 ; Höfler et al., 2015 ), and is affected by recent and delayed implicit priming effects ( Kruijne and Meeter, 2016 ). Other studies have also shown that visual search produces short-term improvement in performance ( Võ and Wolfe, 2012 ), and that visual search is susceptible to memory interference effects in the short-term ( Schneider and Fisk, 1982 ; Boettcher et al., 2013 ) as well as associative links between both visually and semantically similar objects ( Moores et al., 2003 ; Belke et al., 2008 ). Taken together, it seems likely that visual search is indeed influenced by short-term memory processes, but whether and by what processes such improvements may be represented over longer timescales has remained largely unknown.
Here, we find that searching for items of a particular category can improve performance in the long term. Specifically, we find that participants trained to search for a particular category of items can demonstrate a persistent improvement in performance without intermediate training over consecutive days. From that perspective, visual search training appears to produce a similar effect to that observed in other memory phenomena, such as item recognition and associative memory ( Poldrack et al., 2001 ; Fell and Axmacher, 2011 ), whereby repeated training can produce a long lasting change in performance over two or more consecutive days.
However, we also find here that prior learning led to a suppression of performance when searching for novel subordinate-level items within the same category as well as to an inability to improve performance over time. These effects were not due to increased difficult or distraction as there was no suppression of performance when the items were initially learned concurrently. Moreover, introduction of an interference task following training did not lead to reduced performance for the initially trained item but rather prevented the suppression of performance improvement for the new items (i.e., it prevented learning suppression). While it could be argued that our study merely demonstrates new vs. familiar learning as opposed to true visual categorical learning, given that the distractors were context specific, it is unlikely that participants were simply gaining the ability to recognize individual visual search items.
Our interpretation of these findings is that searching for particular visual features within the environment can lead to long-term retention of the same visual features, but also suppresses the ability to form additional similar memory traces. These findings share some parallel with models of pattern suppression that may be category-modulated and potentially reversible with non-target category interference ( Wimber et al., 2009 , 2015 ; Maxcey and Woodman, 2014 ). However, they also demonstrate that prior learning prevents additional learning of unfamiliar categories. These findings are in contrast to prior observations that learning of categorical features can sometimes lead to improved categorization accuracy ( Scott et al., 2008 ). Lastly, to further support this distinction between short- and long-term memory components of visual memory, interference after training on the original visual feature did not suppress performance when searching for that same feature the following day but prevented the suppression of additional visual features. Taken together, these data suggest a neural mechanism that may allow attended visual patterns to persist in memory over prolonged durations but which suppresses the ability to learn similar visual patterns once consolidation occurs. The prospective benefit of such a system could be to enhance specificity to trained visual features within the environment by suppressing the ability to learn similar but distinct visual features and, therefore, the possibility of ‘false-positive’ detection. These observations provide an important addition to our understanding of long-term memory processes ( Frankland and Bontempi, 2005 ; Nader and Hardt, 2009 ).
Data Availability Statement
The raw data supporting the conclusions of this manuscript will be made available by the authors, without undue reservation, to any qualified researcher.
Author Contributions
GF, LJ, and ZW were involved in the conception and design of the study and conducted data analysis and interpretation. LJ and GF performed data collection. GF and ZW were involved in drafting the manuscript.
GF was supported by the Howard Hughes Medical Institute Medical Fellows Program.
Conflict of Interest Statement
The authors declare that the research was conducted in the absence of any commercial or financial relationships that could be construed as a potential conflict of interest.
Supplementary Material
The Supplementary Material for this article can be found online at: https://www.frontiersin.org/articles/10.3389/fpsyg.2018.01896/full#supplementary-material
Alexander, R. G., and Zelinsky, G. J. (2011). Visual similarity effects in categorical search. J. Vis. 11, 9. doi: 10.1167/11.8.9
PubMed Abstract | CrossRef Full Text | Google Scholar
Belke, E., Humphreys, G. W., Watson, D. G., Meyer, A. S., and Telling, A. L. (2008). Top-down effects of semantic knowledge in visual search are modulated by cognitive but not perceptual load. Percept. Psychophys. 70, 1444–1458. doi: 10.3758/PP.70.8.1444
Boettcher, S. E. P., Drew, T., and Wolfe, J. M. (2013). Hybrid search in context: how to search for vegetables in the produce section and cereal in the cereal aisle. Vis. Cogn. 21, 678–682. doi: 10.1080/13506285.2013.844959
Brady, T. F., Konkle, T., and Alvarez, G. A. (2011). A review of visual memory capacity: beyond individual items and toward structured representations. J. Vis. 11, 4. doi: 10.1167/11.5.4
Brady, T. F., Konkle, T., Alvarez, G. A., and Oliva, A. (2008). Visual long-term memory has a massive storage capacity for object details. Proc. Natl. Acad. Sci. U.S.A. 105, 14325–14329. doi: 10.1073/pnas.0803390105
Chun, M. M., and Jiang, Y. (1998). Contextual cueing: implicit learning and memory of visual context guides spatial attention. Cogn. Psychol. 36, 28–71. doi: 10.1006/cogp.1998.0681
Cunningham, C. A., and Wolfe, J. M. (2014). The role of object categories in hybrid visual and memory search. J. Exp. Psychol. Gen. 143, 1585–1599. doi: 10.1037/a0036313
Cunningham, C. A., Yassa, M. A., and Egeth, H. E. (2015). Massive memory revisited: limitations on storage capacity for object details in visual long-term memory. Learn. Mem. 22, 563–566. doi: 10.1101/lm.039404.115
Eckstein, M. P. (2011). Visual search: a retrospective. J. Vis. 11, 14. doi: 10.1167/11.5.14
Emrich, S. M., Al-Aidroos, N., Pratt, J., and Ferber, S. (2009). Visual search elicits the electrophysiological marker of visual working memory. PLoS One 4:e8042. doi: 10.1371/journal.pone.0008042
Failing, M., and Theeuwes, J. (2018). Selection history: how reward modulates selectivity of visual attention. Psychon. Bull. Rev. 25, 514–538. doi: 10.3758/s13423-017-1380-y
Fell, J., and Axmacher, N. (2011). The role of phase synchronization in memory processes. Nat. Rev. Neurosci. 12, 105–118. doi: 10.1038/nrn2979
Ferrante, O., Patacca, A., Di Caro, V., Della Libera, C., Santandrea, E., and Chelazzi, L. (2018). Altering spatial priority maps via statistical learning of target selection and distractor filtering. Cortex 102, 67–95. doi: 10.1016/j.cortex.2017.09.027
Frankland, P. W., and Bontempi, B. (2005). The organization of recent and remote memories. Nat. Rev. Neurosci. 6, 119–130. doi: 10.1038/nrn1607
Geyer, T., Mueller, H. J., Assumpcao, L., and Gais, S. (2013). Sleep-effects on implicit and explicit memory in repeated visual search. PLoS One 8:e69953. doi: 10.1371/journal.pone.0069953
Gibson, B. S., Li, L., Skow, E., Brown, K., and Cooke, L. (2000). Searching for one versus two identical targets: when visual search has a memory. Psychol. Sci. 11, 324–327.
PubMed Abstract | Google Scholar
Höfler, M., Gilchrist, I. D., and Körner, C. (2015). Guidance toward and away from distractors in repeated visual search. J. Vis. 15, 12. doi: 10.1167/15.5.12
Horowitz, T. S., and Wolfe, J. M. (1998). Visual search has no memory. Nature 394, 575–577. doi: 10.1038/29068
Jiang, Y., and Wagner, L. C. (2004). What is learned in spatial contextual cuing–configuration or individual locations? Percept. Psychophys. 66, 454–463.
Google Scholar
Jiang, Y. V. (2018). Habitual versus goal-driven attention. Cortex 102, 107–120. doi: 10.1016/j.cortex.2017.06.018
Kristjánsson, A. (2000). In search of remembrance: evidence for memory in visual search. Psychol. Sci. 11, 328–332.
Kruijne, W., and Meeter, M. (2016). Implicit short- and long-term memory direct our gaze in visual search. Atten. Percept. Psychophys. 78, 761–773. doi: 10.3758/s13414-015-1021-3
Maxcey, A. M., and Woodman, G. F. (2014). Forgetting induced by recognition of visual images. Vis. Cogn. 22, 789–808. doi: 10.1080/13506285.2014.917134
Moores, E., Laiti, L., and Chelazzi, L. (2003). Associative knowledge controls deployment of visual selective attention. Nat. Neurosci. 6, 182–189. doi: 10.1038/nn996
Nader, K., and Hardt, O. A. (2009). single standard for memory: the case for reconsolidation. Nat. Rev. Neurosci. 10, 224–234. doi: 10.1038/nrn2590
Nako, R., Wu, R., and Eimer, M. (2014). Rapid guidance of visual search by object categories. J. Exp. Psychol. Hum. Percept. Perform. 40, 50–60. doi: 10.1037/a0033228
Oliva, A., Wolfe, J. M., and Arsenio, H. C. (2004). Panoramic search: the interaction of memory and vision in search through a familiar scene. J. Exp. Psychol. Hum. Percept. Perform. 30, 1132–1146. doi: 10.1037/0096-1523.30.6.1132
Peterson, M. S., Beck, M. R., and Vomela, M. (2007). Visual search is guided by prospective and retrospective memory. Percept. Psychophys. 69, 123–135.
Peterson, M. S., Kramer, A. F., Wang, R. F., Irwin, D. E., and McCarley, J. S. (2001). Visual search has memory. Psychol. Sci. 12, 287–292.
Poldrack, R. A., Clark, J., Paré-Blagoev, E. J., Shohamy, D., Creso Moyano, J., Myers, C., et al. (2001). Interactive memory systems in the human brain. Nature 414, 546–550. doi: 10.1038/35107080
Potter, M. C., Wyble, B., Pandav, R., and Olejarczyk, J. (2010). Picture detection in rapid serial visual presentation: features or identity? J. Exp. Psychol. Hum. Percept. Perform. 36, 1486–1494. doi: 10.1037/a0018730
Rugg, M. D., Mark, R. E., Walla, P., Schloerscheidt, A. M., Birch, C. S., and Allan, K. (1998). Dissociation of the neural correlates of implicit and explicit memory. Nature 392, 595–598. doi: 10.1038/33396
Schacter, D. L., Chiu, C. Y. P., and Ochsner, K. N. (1993). Implicit memory: a selective review. Annu. Rev. Neurosci. 16, 159–182. doi: 10.1146/annurev.ne.16.030193.001111
CrossRef Full Text | Google Scholar
Schneider, W., and Fisk, A. D. (1982). Degree of consistent training: improvements in search performance and automatic process development. Percept. Psychophys. 31, 160–168.
Scott, L. S., Tanaka, J. W., Sheinberg, D. L., and Curran, T. (2008). The role of category learning in the acquisition and retention of perceptual expertise: a behavioral and neurophysiological study. Brain Res. 1210, 204–215. doi: 10.1016/j.brainres.2008.02.054
Sireteanu, R., and Rettenbach, R. (1995). Perceptual learning in visual search: fast, enduring, but non-specific. Vis. Res. 35, 2037–2043. doi: 10.1016/0042-6989(94)00295-W
Squire, L. R. (2004). Memory systems of the brain: a brief history and current perspective. Neurobiol. Learn. Mem. 82, 171–177. doi: 10.1016/j.nlm.2004.06.005
Travis, S. L., Mattingley, J. B., and Dux, P. E. (2013). On the role of working memory in spatial contextual cueing. J. Exp. Psychol. Learn. Mem. Cogn. 39, 208–219. doi: 10.1037/a0028644
Võ, M. L.-H., and Wolfe, J. M. (2012). When does repeated search in scenes involve memory? Looking AT versus looking FOR objects in scenes. J. Exp. Psychol. Hum. Percept. Perform. 38, 23–41. doi: 10.1037/a0024147
Wimber, M., Alink, A., Charest, I., Kriegeskorte, N., and Anderson, M. C. (2015). Retrieval induces adaptive forgetting of competing memories via cortical pattern suppression. Nat. Neurosci. 18, 582–589. doi: 10.1038/nn.3973
Wimber, M., Rutschmann, R. M., Greenlee, M. W., and Bäuml, K.-H. (2009). Retrieval from episodic memory: neural mechanisms of interference resolution. J. Cogn. Neurosci. 21, 538–549. doi: 10.1162/jocn.2009.21043
Wolfe, J. M., Horowitz, T. S., Van Wert, M. J., Kenner, N. M., Place, S. S., and Kibbi, N. (2007). Low target prevalence is a stubborn source of errors in visual search tasks. J. Exp. Psychol. Gen. 136, 623–638. doi: 10.1037/0096-3445.136.4.623
Wolfe, J. M., Klempen, N., and Dahlen, K. (2000). Postattentive vision. J. Exp. Psychol. 26, 693–716. doi: 10.1037/0096-1523.26.2.693
Yang, H., and Zelinsky, G. J. (2009). Visual search is guided to categorically-defined targets. Vis. Res. 49, 2095–2103. doi: 10.1016/j.visres.2009.05.017
Keywords : long-term memory, visual memory, learning suppression, visual search, memory consolidation
Citation: Friedman GN, Johnson L and Williams ZM (2018) Long-Term Visual Memory and Its Role in Learning Suppression. Front. Psychol. 9:1896. doi: 10.3389/fpsyg.2018.01896
Received: 26 January 2018; Accepted: 18 September 2018; Published: 12 October 2018.
Reviewed by:
Copyright © 2018 Friedman, Johnson and Williams. This is an open-access article distributed under the terms of the Creative Commons Attribution License (CC BY) . The use, distribution or reproduction in other forums is permitted, provided the original author(s) and the copyright owner(s) are credited and that the original publication in this journal is cited, in accordance with accepted academic practice. No use, distribution or reproduction is permitted which does not comply with these terms.
*Correspondence: Gabriel N. Friedman, [email protected]
† These authors have contributed equally to this work
Disclaimer: All claims expressed in this article are solely those of the authors and do not necessarily represent those of their affiliated organizations, or those of the publisher, the editors and the reviewers. Any product that may be evaluated in this article or claim that may be made by its manufacturer is not guaranteed or endorsed by the publisher.
Help | Advanced Search
Computer Science > Computation and Language
Title: memorybank: enhancing large language models with long-term memory.
Abstract: Revolutionary advancements in Large Language Models have drastically reshaped our interactions with artificial intelligence systems. Despite this, a notable hindrance remains-the deficiency of a long-term memory mechanism within these models. This shortfall becomes increasingly evident in situations demanding sustained interaction, such as personal companion systems and psychological counseling. Therefore, we propose MemoryBank, a novel memory mechanism tailored for LLMs. MemoryBank enables the models to summon relevant memories, continually evolve through continuous memory updates, comprehend, and adapt to a user personality by synthesizing information from past interactions. To mimic anthropomorphic behaviors and selectively preserve memory, MemoryBank incorporates a memory updating mechanism, inspired by the Ebbinghaus Forgetting Curve theory, which permits the AI to forget and reinforce memory based on time elapsed and the relative significance of the memory, thereby offering a human-like memory mechanism. MemoryBank is versatile in accommodating both closed-source models like ChatGPT and open-source models like ChatGLM. We exemplify application of MemoryBank through the creation of an LLM-based chatbot named SiliconFriend in a long-term AI Companion scenario. Further tuned with psychological dialogs, SiliconFriend displays heightened empathy in its interactions. Experiment involves both qualitative analysis with real-world user dialogs and quantitative analysis with simulated dialogs. In the latter, ChatGPT acts as users with diverse characteristics and generates long-term dialog contexts covering a wide array of topics. The results of our analysis reveal that SiliconFriend, equipped with MemoryBank, exhibits a strong capability for long-term companionship as it can provide emphatic response, recall relevant memories and understand user personality.
Comments: | 10 pages |
Subjects: | Computation and Language (cs.CL); Artificial Intelligence (cs.AI) |
Cite as: | [cs.CL] |
(or [cs.CL] for this version) | |
Focus to learn more arXiv-issued DOI via DataCite |
Submission history
Access paper:.
- Other Formats
References & Citations
- Google Scholar
- Semantic Scholar
BibTeX formatted citation

Bibliographic and Citation Tools
Code, data and media associated with this article, recommenders and search tools.
- Institution
arXivLabs: experimental projects with community collaborators
arXivLabs is a framework that allows collaborators to develop and share new arXiv features directly on our website.
Both individuals and organizations that work with arXivLabs have embraced and accepted our values of openness, community, excellence, and user data privacy. arXiv is committed to these values and only works with partners that adhere to them.
Have an idea for a project that will add value for arXiv's community? Learn more about arXivLabs .
Opinion: I have recurring memory lapses. Is it dementia?

- Copy Link URL Copied!
Ever since I had a stroke five years ago, I have worried about dementia. I remember a task, then immediately forget about it. Water is poured, then left. Rooms are entered, then I can’t remember why. Pills are taken. Or are they?
In a “ 60 Minutes ” segment a while back, a researcher explained the science of losing your mind. She had been studying high-functioning nonagenarians in California for years. The brain scans in her “ 90+ Study ” might show evidence of trouble — bright swaths of red and yellow that indicate plaque and tangle-like tissues, well-known harbingers of dementia — and yet the participants may never show symptoms of decline. A neuro mystery.
I’m 62, not 92. My brain had been through its own disasters. Was something worse coming?
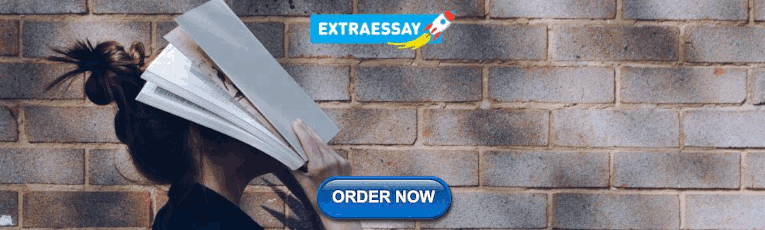
Opinion: What a doctor sees when Joe Biden hesitates
The president’s enemies and the uninformed combine his recall issues with stereotypes to create a false narrative of intellectual impairment.
March 7, 2024
Actually, my stroke wasn’t a disabling catastrophe. Initially I couldn’t talk, but a miracle drug flushed out the blockage almost immediately and my brain began to recover in just days. I got my voice back within a week and I began putting sentences and paragraphs back together shortly after that. My doctors were pretty sure I wouldn’t be able to resume writing professionally, but I did. I even recorded a portion of the audio version of my memoir a year after the stroke, which, given what I’d been through, was perhaps more meaningful to me than writing it. It seemed I was out of the woods.
But there were still these memory glitches, almost daily. How could I write a publishable essay but not remember that I had, seconds earlier, taken the half-and-half out for my coffee? I began to discount, then hide the mistakes from my wife, including the times I left the burner on or the car running.
I decided to take a cognitive test, worrying about the possibility of progression. I knew some of what to expect. In 2014 I had successful spinal cancer surgery, and afterward my doctors wanted me to answer the test questions. I protested then because I viewed it as an indignity: I’ve just been through cancer surgery and you want me to do what? Eventually I gave in.
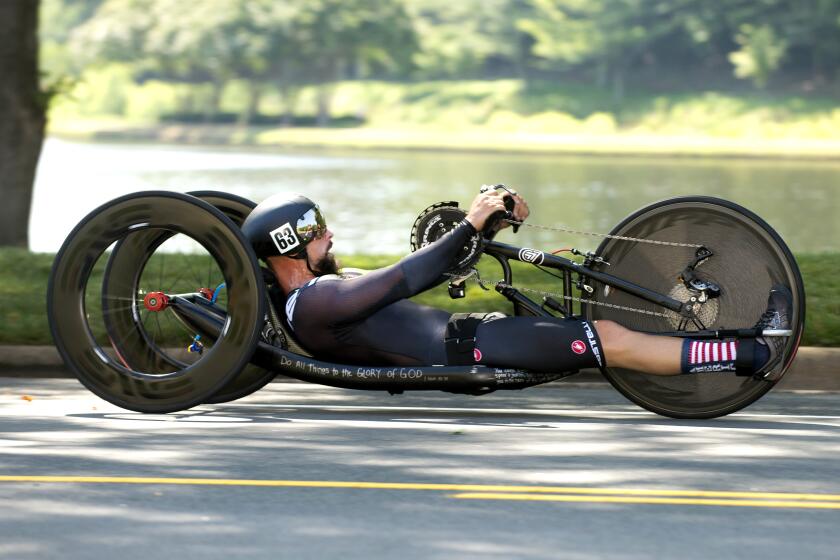
Op-Ed: As I watch the Paralympics for pro tips, I realize I am one of them: A proud para-athlete
My legs no longer worked well but my arms did. My doctor said there were two ways to get better: heal and adapt. She suggested I get a hand-bike.
Sept. 2, 2021
Now I wanted the test, even though the results might mean I would lose my driver’s license, or worse. The therapist assured me they would be kept private, shared only with my doctors. Still, I hesitated, canceling once.
The three-hour test wasn’t fun. Participants in the “90+ Study” took a similar test annually as part of their protocol, and I saw myself in the “60 Minutes” footage of a failing and frustrated 90-year-old. It was humbling. He wasn’t missing broad areas of recall, but more nuanced things: He couldn’t repeat back three words he had been told 40 seconds earlier, and he failed to recall number sequences. Researchers gave him a second shot at some of the questions, as the person administering my test did with me. No, nothing, I had to tell them. No, I can’t remember.
The report was 14 pages long. When it showed up in my inbox, I ignored it for several days. I wondered if I really wanted to know what they found. What if keeping my lapses in the background — a kind of strategic denial, I know — was critical to my high-functioning self?
It was scary, but I finally read the exhaustive report. Dozens of cognitive tasks were graded on a scale from “exceptional low” to “above average.” My scores weren’t great. I was found wanting in “executive function,” a term that refers to the planning and executing of jobs that the brain routinely does. The clinical fellow in psychology who gave me the test made practical suggestions for daily living (such as putting up Post-it notes with reminders) and recommended follow-up appointments with a speech and language therapist and my neurologist.
Maybe this isn’t surprising — I read the report and then I forgot about it. And I didn’t follow up. That was three years ago.
In a few days, my first nonfiction book in 10 years will be published. I started researching it within a few months of seeing my cognitive test report. It includes source notes and a lengthy bibliography of the books I read for research. Because of several family emergencies, I got a late start writing but I met the book deadline, finishing the bulk of it in a three-month window.
And as I prepare for publication, I know I will have moments where I walk into a room and wonder, what am I doing here?
Todd Balf’s new book, “ Three Kings: Race, Class and the Barrier-breaking Rivals Who Launched the Modern Olympic Age, ” will be published July 2.
More to Read
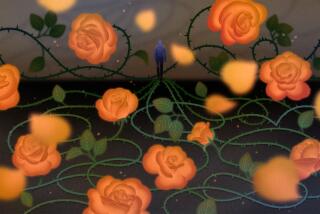
Want to ease the pain of loss? Create a grief ritual
June 26, 2024
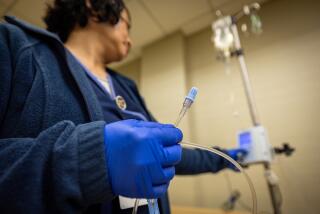
Alzheimer’s drug’s potentially fatal side effect, ARIA, obscured by ‘soothing acronym,’ doctors say
June 17, 2024
Column: Suggesting that Biden has dementia? ‘If ... shame still exists, I’d call it shameful’
June 13, 2024
A cure for the common opinion
Get thought-provoking perspectives with our weekly newsletter.
You may occasionally receive promotional content from the Los Angeles Times.
More From the Los Angeles Times
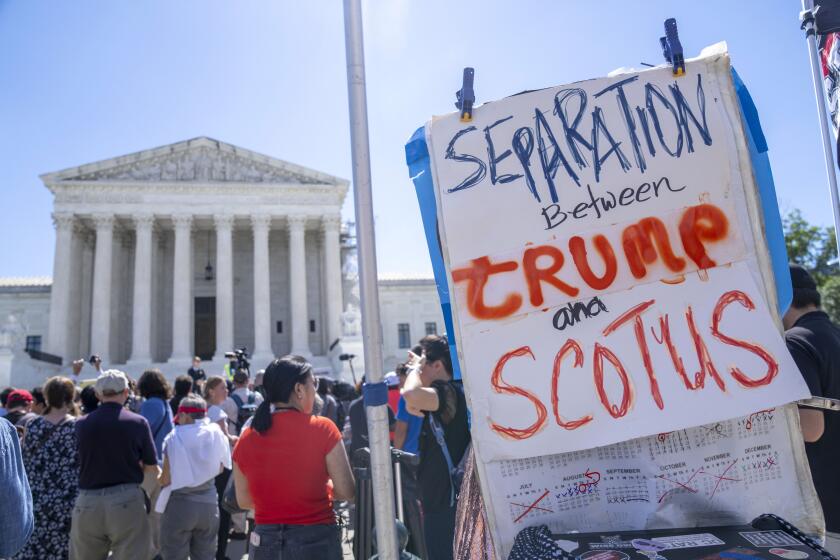
Litman: After the Supreme Court’s immunity ruling, can Donald Trump still be tried for Jan. 6?
July 1, 2024
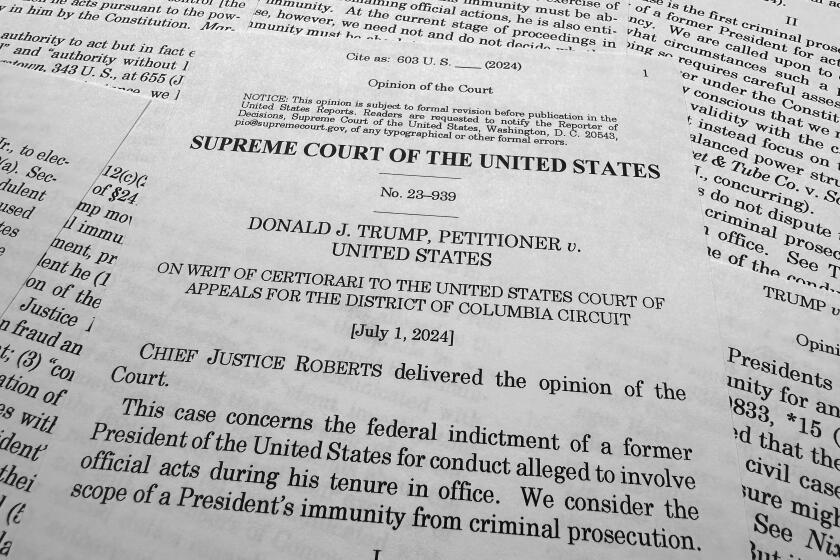
Opinion: We should all dissent from the Supreme Court’s immunity decision, and not respectfully
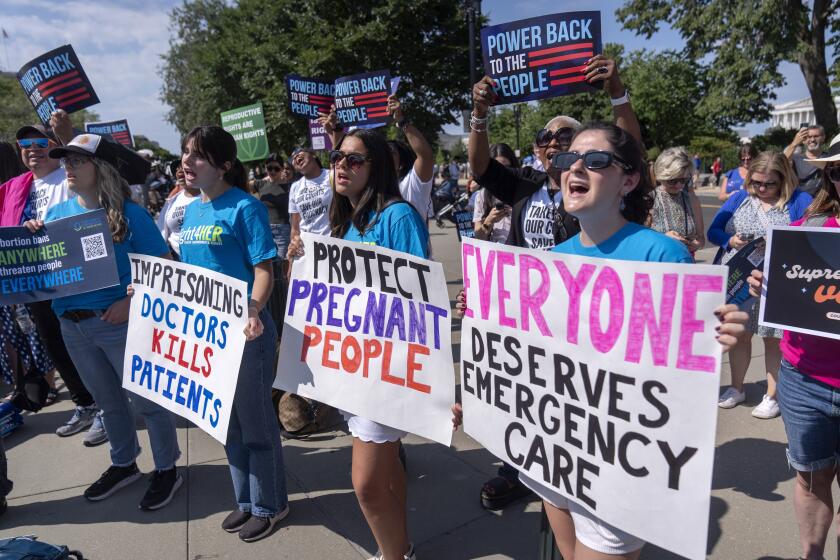
Opinion: If you were relieved by the Supreme Court’s abortion rulings this term, think again
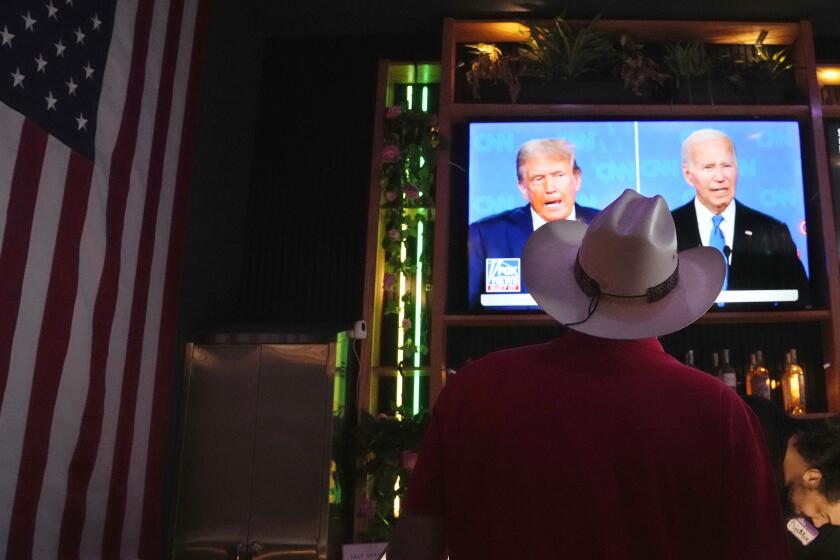
Calmes: The Biden debate and the Supreme Court — the full catastrophe
June 30, 2024
Thank you for visiting nature.com. You are using a browser version with limited support for CSS. To obtain the best experience, we recommend you use a more up to date browser (or turn off compatibility mode in Internet Explorer). In the meantime, to ensure continued support, we are displaying the site without styles and JavaScript.
- View all journals
- Explore content
- About the journal
- Publish with us
- Sign up for alerts
- Open access
- Published: 01 July 2024
Complex artificial intelligence models for energy sustainability in educational buildings
- Rasikh Tariq ORCID: orcid.org/0000-0002-3310-432X 1 ,
- Awsan Mohammed ORCID: orcid.org/0000-0001-6500-5994 2 , 3 ,
- Adel Alshibani ORCID: orcid.org/0000-0001-7809-106X 2 , 4 &
- Maria Soledad Ramírez-Montoya ORCID: orcid.org/0000-0002-1274-706X 1 , 5
Scientific Reports volume 14 , Article number: 15020 ( 2024 ) Cite this article
Metrics details
- Energy science and technology
- Mathematics and computing
Energy consumption of constructed educational facilities significantly impacts economic, social and environment sustainable development. It contributes to approximately 37% of the carbon dioxide emissions associated with energy use and procedures. This paper aims to introduce a study that investigates several artificial intelligence-based models to predict the energy consumption of the most important educational buildings; schools. These models include decision trees, K-nearest neighbors, gradient boosting, and long-term memory networks. The research also investigates the relationship between the input parameters and the yearly energy usage of educational buildings. It has been discovered that the school sizes and AC capacities are the most impact variable associated with higher energy consumption. While 'Type of School' is less direct or weaker correlation with 'Annual Consumption'. The four developed models were evaluated and compared in training and testing stages. The Decision Tree model demonstrates strong performance on the training data with an average prediction error of about 3.58%. The K-Nearest Neighbors model has significantly higher errors, with RMSE on training data as high as 38,429.4, which may be indicative of overfitting. In contrast, Gradient Boosting can almost perfectly predict the variations within the training dataset. The performance metrics suggest that some models manage this variability better than others, with Gradient Boosting and LSTM standing out in terms of their ability to handle diverse data ranges, from the minimum consumption of approximately 99,274.95 to the maximum of 683,191.8. This research underscores the importance of sustainable educational buildings not only as physical learning spaces but also as dynamic environments that contribute to informal educational processes. Sustainable buildings serve as real-world examples of environmental stewardship, teaching students about energy efficiency and sustainability through their design and operation. By incorporating advanced AI-driven tools to optimize energy consumption, educational facilities can become interactive learning hubs that encourage students to engage with concepts of sustainability in their everyday surroundings.
Similar content being viewed by others
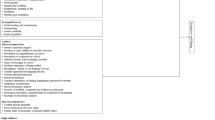
Identifying the top predictors of student well-being across cultures using machine learning and conventional statistics
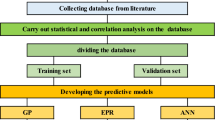
Estimating the energy consumption for residential buildings in semiarid and arid desert climate using artificial intelligence
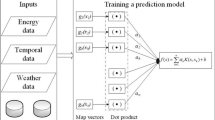
Proposing a hybrid metaheuristic optimization algorithm and machine learning model for energy use forecast in non-residential buildings
Introduction.
Energy consumption of constructed facility has a significant impact on the environment. The world is currently experiencing significant fluctuations in the energy landscape, which has far-reaching implications for many aspects of life on a daily basis. Furthermore, global energy demand has risen by 0.9% to 120 millions tons of oil equivalent (Mtoe) 1 . The International Energy Agency anticipates that by 2021, the buildings and construction industry will account for more than one-third of global energy demand. Furthermore, this sector is projected to contribute to approximately 37% of the carbon dioxide emissions associated with energy use and procedures 2 . The impact of this dioxide emissions on the environments, social, and have recently increased, emphasizing the growing importance of sustainable development. Meanwhile, there is a growing demand for building energy services. Therefore, there is a critical need to develop effective strategies for energy planning and prediction. This not only ensures the future progress of the built environment, but also enhances energy efficiency, conserves resources, and promotes sustainable development 3 , 4 . Efficient strategies involve accurately forecasting building energy usage to effectively oversee and conserve energy within buildings.
Educational institutions, including schools, represent a major part of built environment facility that play a crucial role in shaping the future of society through knowledge dissemination and fostering intellectual growth. However, schools represent significant energy consumers, with diverse facilities requiring heating, cooling, lighting, and other services. The efficient management of energy resources in educational buildings is not only essential for cost savings but also aligns with broader sustainability goals aimed at reducing carbon emissions and mitigating climate change impacts 5 . The energy usage in school buildings is influenced by various factors, including their location, size, number of occupants, age, and extent of air conditioning. Accurately predicting energy usage is essential for the effective functioning of contemporary electrical grids 6 , 7 . Numerous research studies have emphasized the importance of precise electricity forecasting. Accurate and reliable estimates of electricity consumption are critical for planning future electricity generation systems to meet the growing demand for electrical energy 8 , 9 . Understanding the variations in building energy usage allows to development of focused and efficient energy conservation strategies 10 . Comprehensive knowledge of building energy consumption prediction is essential for developing innovative approaches such as demand-side management plans 11 , intelligent control systems 12 , and fault detection and diagnosis methods 13 . These methods use predictive analysis to improve energy efficiency, reduce waste, and ensure that building systems run smoothly. These systems encourage both energy conservation and building infrastructure efficiency by determining potential energy-saving possibilities and resolving inefficiencies in operation 14 . Studies have shown that even slight improvements in estimating building energy usage can result in significant energy savings 15 . Managers of buildings and users may adopt better decisions and take proactive steps to increase energy efficiency by precisely predicting energy use patterns. This could involve changing HVAC configurations, improving the schedule of lighting, installing energy-efficient devices, and applying behavioral adjustments to help achieve energy-saving targets 16 .
However, accurate prediction of energy consumption is difficult due to a variety of unpredictable situations or noisy data disorders, and the methods used frequently produce inaccurate projections. Thus, this field requires additional work and attention, particularly from governmental agencies. Consequently, this paper investigates number of artificial intelligence-based models to estimate the energy consumption of school buildings. These models include decision trees, K-nearest neighbors, gradient boosting, and long-term memory networks. The investigated algorithms are developed and validated using real data collected for educational buildings; school. In addition, different statistical tests are conducted to ensure the quality of data. The models are expected to contribute to effective resource management, environmental sustainability, educational opportunities, operational efficiency, and regulatory compliance. Educational institutions can create greener schools while saving money by accurately estimating energy needs and implementing consumption-reduction strategies.
Literature review
Sustainable school buildings contributing towards educational innovation.
In the context of complexity, it is important to look for innovative options that support institutions to become more sustainable in terms of energy. Purnell et al. 17 , implemented plans involving the academic and social community, where they learned about innovation, leadership, coal mining, greenhouse problems, energy audits, alternative energy and promotion of energy efficiency practices in the school and community. Designing the construction or restructuring of school buildings is vital to improve energy consumption 18 . New solutions are needed, hence Zeiler and De Waard 19 presented alternatives for sustainable schools through ‘Plus Energy Schools’, the authors based on a study comparing passive house schools, near-zero energy schools and additional energy schools, where they evaluated the indoor air quality and comfort of some of the schools measured, with a view to contribute to the challenging design towards more sustainable schools. Improving school infrastructure requires attention to the principles of sustainable development.
The designs of school institutions require new ecological, sustainable and healthy practices that accompany educational innovation practices. Educational innovation integrates inputs of processes, products, services or knowledge with a view to improving complex environments 20 and in institutions, the use of new technologies, evolving sustainable design practices and innovative ‘green’ building materials should be promoted for each new project to be reflected in constantly improving energy efficiency results 21 . In parallel to sustainable care, it is relevant that institutions are energy neutral, energy positive and provide comfort to stakeholders (students, teachers, managers, parents) in learning environments 22 . Zhang et al. 23 provided an energy prototype with sustainable strategies and energy-saving technologies, which can reduce energy consumption in school facilities as well as improve the indoor environment. Sustainable design and construction strategies that combine high levels of energy efficiency, performance standards and indoor environmental quality should integrate experimentation and contribute to formulating innovative sustainable building strategies 24 . Innovation and sustainability are substantial elements for educational institutions in the context of constant change.
Complex artificial intelligence modelling through machine learning methods in educational buildings
In Artificial intelligence has been of value in modelling solutions in various sectors. For example, in river studies, Msaddek et al. 25 employed artificial intelligence to reduce potential uncertainties using the Unsupervised Multi-Frameworks Technique and Fuzzy Membership Framework and supervised learning based on the Multi-Model Approach and Gene Expression Programming. Similarly, Tao et al. 26 applied artificial intelligence models for suspended river sediment prediction to provide an updated description of the most recent and relevant AI-based applications for modelling sediment transport in watershed systems. A combination of water management combined with socio-technical systems is presented by Baki et al. 27 , assessing the dynamic nature of socio-economic variables in order to test the effectiveness of different policies, such as awareness campaigns, and dynamically simulate the subsequent response of the urban water system over time. In the financial sector, Méndez-Suárez et al. 28 integrated artificial intelligence for automated financial advice in the copper market with promising results, both in terms of statistics and trading metrics. Modelling through machine learning provides opportunities for various sectors, including the education sector.
Educational buildings also benefit from complex artificial intelligence modelling. Reddy et al. 29 conducted a plug load study in educational buildings using machine learning algorithms to monitor their energy consumption where they applied machine learning techniques. López-Pérez & Flores-Prieto 30 studied energy savings in an air-conditioned educational building in the tropical climate of Aw in relation to annual cooling load and degree-days, with adequate comfort levels following the adaptive thermal comfort approach. Comfort temperature modelling was performed using fuzzy logic, artificial neural networks, adaptive neuro-fuzzy inference system and a local linear model. Hosseini et al. 31 modelled, analysed and optimised an integrated energy system for the provision of triple loads of an educational building using artificial intelligence, showing that the dynamic load pattern provides the highest energy efficiency and the lowest total cost rate. Lee & Zhang 32 provide a new artificial intelligence of things (AIoT)-based framework for predicting multidimensional indoor environment quality (IEQ) conditions that provides information on IEQ conditions and their potential impacts on student well-being, thus facilitating the future development of climate-adaptive, data-driven, human-centric educational facilities. Artificial intelligence modelling through machine learning methods in educational buildings has a high potential to improve educational environments, their sustainability and energy efficiency.
Summary of literature review and research contribution
Since 2002, government agencies, including the European Union, have assessed building energy efficiency to forecast energy consumption 33 . The fundamental purpose of energy prediction is identifying and forecasting potential enhancements to optimize energy consumption within a building. There are several energy prediction methodologies available, each with its own set of benefits and drawbacks 34 . Previous studies provided a review of energy prediction methodologies used to forecast building energy performance 35 , 36 , 37 . Chae et al. 38 used an Artificial Neural Network (ANN) model with a Bayesian regularization technique to predict the short term energy usage of building. The authors analyzed several design criteria to determine the most effective structure for the prediction model. Biswas et al. 39 built and verified an ANN model to meet the non linear difficulty of acquiring data on energy use while providing reliable computation of large dynamical datasets. Deb et al. 40 also introduced an ANN for estimating the consumption of energy from both night and day cooling demands in educational facilities. The authors separated the energy usage data into various kinds of inputs.
Yan et al. 41 proposed a hybrid model based on a neural network to forecaste energy consumption in individual households. This model was created primarily to address issues related to irregular human behavior and univariate datasets found in single household energy consumption prediction. Zhong et al. 42 developed a support vector machine to address issues with the accuracy of forecasting related to the significant non-linearity between both outcomes and inputs in energy usage prediction models. Wang 43 and Tabrizchi 44 also used a self-adaptive multiverse optimization to enhance the accuracy of prediction and tune the features of the support vector machine. Meanwhile, Iwafune et al. 45 used regression models to forecast the electricity usage in 50 households. Albuquerque et al. 46 employed standardized machine-learning algorithms to forecast Brazil's consumption of electricity. The findings showed that random forests provided forecasts. In addition, Dong et al. 47 introduced a technique for forecasting energy consumption specific to building operations across multiple timeframes, employing pattern classification and integrated learning. They utilized random forests to assess the significance of input features in the model and conducted correlation analysis to explore the connection between the input parameters and output. The results showed that the integrated consumption of energy forecasting approach with pattern categorization outperformed the unclassified integrated energy consumption prediction model.
Cao et al. 48 presented an integrated model for predicting energy consumption that uses spatial features taken from time-series information to estimate short-term energy usage in higher education organizations. They used the collaborative game theory SHAP approach to evaluate the influence of features on model performance, using ablation analysis to identify the optimum amount of features needed. Faiq et al. 49 utilized LSTM to estimate energy usage in institutional buildings. The authors evaluated the model's performance against SVM and Gaussian process models. Álvarez et al. 50 studied 453 homes in Spain to estimate their U-opaque rating. Several ANN structures were developed and evaluated on actual recorded data, with a Pearson correlation factor of 0.967. Beccali et al. 51 proposed using artificial neural networks to anticipate building energy efficiency in buildings in Italy. Ahmad et al. 52 investigated the performance of random forest and ANN models for predicting HVAC power usage in hostels. The findings indicated that ANN outperformed random forest slightly. Martellotta et al. 53 employed to estimate heat energy usage, training the network on EnergyPlus-generated simulated data. Williams and Gomez 54 studied 426,305 single-family dwellings to anticipate monthly energy usage using building features and monthly weather data. Sun and Han 55 provided an ANN model that took into account a variety of input factors such as orientation, window size, windows per bay, number of floors, and floor height. Similarly, Wong et al. 56 utilized ANN to forecast the overall energy usage, taking into account nine weather-related input factors, four-building envelope variables, and one-day category.
Moreover, Catalina et al. 57 constructed regression models to forecast the monthly heat demand of homes in France. The study incorporated factors such as the building's structural U-value, window-to-wall ratios, and the construction shape factor. Al-Rashed and Asif 58 investigated a variety of variables that impact the use of energy in residential buildings, including building envelopes, weather patterns, cooking appliances, dwelling types, and air conditioning systems. the findings indicated the use of double-glazing installations and air conditioning with mini-split systems. However, the authors point out a limitation: the inability to evaluate architectural design in order to reduce energy costs during the early design phase. Abdel-Aal et al. 59 developed a model to predict electric power energy consumption in Saudi Arabia's Eastern Province that takes into account conditions as well as economic and population aspects. Nasr et al. 60 proposed an ANN forecasting approach for estimating electric energy consumption in Lebanon using weather variables and time series data. Meng et al. 61 used a hybrid approach to forecast the increasing trend for electrical consumption of energy. However, one disadvantage of this methodology is that it fails to include architectural aspects and design integration when estimating monthly electric energy demand. Karatasou et al. 62 used statistical analysis tools to investigate potential improvements in ANNs accuracy for forecasting the consumption of building energy. Mena-Yedra et al. 63 developed a short term ANN approach for estimating energy load at an industrial buildings, resulting in timely solutions.
In addition to the studies mentioned above, Somu et al. 64 presented an integrated approach for forecasting residential energy consumption that combines long short-term memory and neural network. The findings demonstrated promising results in predicting previously difficult energy consumption patterns. Fath U Min Ullah et al. 65 employed an ANN algorithm to anticipate household electricity demand in Korea, demonstrating the efficacy of the prediction technique. Meanwhile, Liu et al. 66 also used an artificial neural network to predict electricity consumption in China, demonstrating the model's outstanding precision. Khalil et al. 67 developed an ANN approach to anticipate a building's cooling and heating requirements. The model addressed several variables including wall thickness, and orientation, glazing density distribution, glazing, roofing surface, surface area, relative compactness, and overall height. The dataset used for model construction was obtained from existing publications. Furthermore, Rahman et al. 68 developed a recurrent neural network model to forecast medium- to long-term power demand patterns in both residential and commercial buildings, recognizing their significant effect on total electrical usage in the United States. Tartibu and Kabengele 69 proposed an innovative way to estimate South Africa's prospective consumption of energy using an ANN. The proposed technique for estimating power consumption has been verified and evaluated using data from the Council for Scientific and Industrial Research spanning 2014 to 2050. The findings demonstrated that the ANN was capable of accurately predicting energy demand. Fayaz et al. 70 presented a technique for evaluating short-term home energy use. This system had four separate layers: data gathering, preparation, forecasting, and validation. The research employed real data from four multi-story buildings in Seoul, South Korea, to demonstrate the efficiency of the proposed approach. Alshibani 7 identified the factors impacting the schools’ energy consumption in hot and humid climate weather conditions. Also investigated the influence of the identified factors on the energy consumption of school facilities. Mohammed et al. 71 proposed a regression model for predicting the energy consumption of schools in Saudi Arabia. The results revealed the regression models are proposing tools for estimating energy consumption.
The literature review indicated that there is a need to develop efficient models for predicting the energy consumption of educational buildings. Therefore, this paper proposes effective artificial intelligence approaches to estimate the energy consumption of educational buildings. Specifically, this paper proposes machine learning algorithms based on decision trees, K-nearest neighbors, gradient boosting, and long-term memory networks for estimating the energy consumption of school buildings. Real data are collected to develop and evaluate the proposed model. In addition, the variables that influence the energy consumption of educational buildings are determined based on the literature and experts. The relationship between the input factors and the energy consumption is investigated using the Pearson correlation test. The collected data are filtered and prepared. Moreover, the hyperparameters of the proposed models are tuned optimally.
Material and method
This paper proposes machine learning algorithms for estimating the energy consumption of educational buildings. These models include decision trees, K-Nearest neighbor, gradient boosting, and long-term memory networks. The development process begins with identifying the factors influencing energy consumption, which are identified based on both literature reviews and experts. The actual data on energy consumption and relevant input parameters are then gathered and separated into distinct subsets for training, validation, and testing. The collected data are filtered and analyzed to develop robust machine-learning models.
In this paper, descriptive statistics are conducted to provide concise summaries that facilitate understanding and interpretation of the collected data. A Scatter matrix also is constructed to investigate relationships among multiple variables within a dataset and to offer a comprehensive overview of how variables interact. The correlation between the input variables and the target, which is the annual energy consumption of educational buildings, is investigated. Correlation statistics are thoroughly applied to all input variables to identify redundant variables and explore the relationship between the factors and energy consumption. Moreover, parallel coordinate plots are developed to provide a comprehensive visual representation of data while also providing insights into the dataset's underlying structure. The second phase of the research methodology is to select and train the machine learning algorithm that will estimate the energy usage of educational buildings on a yearly bases. The optimal machine learning structure is selected so that the Coefficient of Determination (COD), Mean Absolute Percentage Error (MAPE), Mean Absolute Error (MAE), and Root Mean Square Error (RMSE) are minimized. The model's performance is then evaluated using the testing dataset. Figure 1 depicts the research methodology for the proposed model.
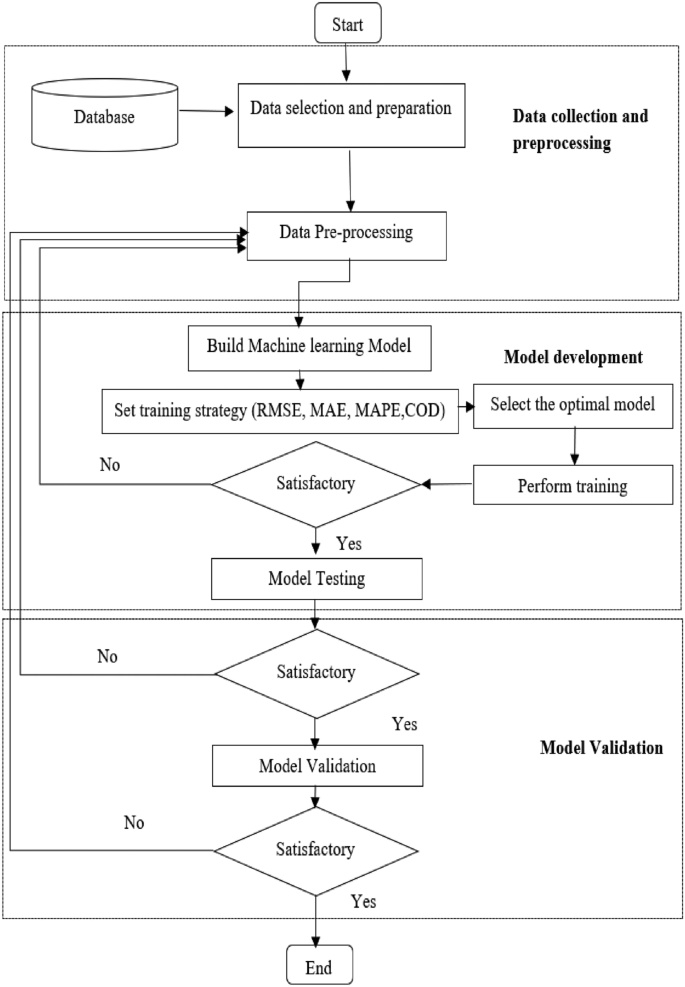
Overall flow diagram of the research method.
Data collection
Direct data collection from educational buildings has replaced paper records as the most efficient way to monitor energy usage. This method seeks to shorten the amount of time needed to collect data. The data gathered covered a wide range of factors that affect energy use and was used as input for a machine-learning model. one parameter was the energy consumption, which was expressed in kWh annually and designated as the output. The dataset was established by filtering real energy consumption data from 352 educational facilities in order to eliminate any outliers. Eleven input variables and one output variable were selected from this refined dataset. In particular, some variables—like different kinds of lamps—were left out of the inputs since they had less of an effect on consumption than other variables. Table 1 illustrates the primary variables impacting the consumption of energy of educational buildings, which were employed as inputs for the machine learning models.
Data pre-processing
Data pre-processing plays a crucial role in the development of machine learning models. It involves a set of steps designed to prepare raw data for analysis. In this paper, descriptive statistics are used to better understand the interpretation of data science algorithms. The average, standard deviation, maximum, minimum, and quartile values for each parameter are calculated. In addition, different visualization tools to understand the behavior of the data are used. Data visualization is an effective tool for interpreting and communicating insights derived from complex datasets. By converting raw data into graphical representations, we can reveal the patterns, trends, and relationships that would otherwise be hidden. Through visualization, we can overcome the limitations of tabular data and gain a thorough understanding of its inherent structure and characteristics. This deeper understanding enables us to draw meaningful conclusions and make informed decisions. In this research, we also use the scatter matrix to perform a detailed pairwise comparison of variables, revealing their interrelationships and individual distributions. Moreover, we use a parallel coordinate plot to visualize multivariate data, allowing us to discern intricate patterns and correlations among the dataset's various dimensions, with a particular focus on annual energy consumption in schools. These visualization techniques not only illuminate complex data landscapes but also help to identify key insights that are critical for informed decision-making and strategic planning. Furthermore, the relationship between the input factors and between the inputs and output is investigated. Pearson's coefficient of correlation is used to improve understanding of the relationship between the input variables and the target, which is the annual energy consumption of educational buildings.
Complex artificial intelligence models through machine and deep learning
In this paper, various machine-learning models are proposed to estimate the energy consumption of educational buildings. These techniques include decision trees, K-nearest neighbors, gradient boosting, and long short-term memory. A brief description of each algorithm is provided in the subsequent subsections:
Decision Tree: A decision tree algorithm is a machine learning technique that partitions input data recursively based on feature values, resulting in the prediction of a target variable. Starting with the entire dataset at the root node, the algorithm selects the best attribute to split the data into subsets, to maximize information gain, minimize impurity, or reduce variance, depending on the task. This process continues iteratively, resulting in a tree-like structure with internal nodes representing decision points based on feature values and leaf nodes representing predicted outcomes. Overfitting can be prevented by using a variety of stopping criteria, such as maximum depth or minimum samples per leaf. Pruning techniques can help refine the tree by removing unnecessary branches. Prediction involves traversing the tree from the root to a leaf node based on the attribute values of a new instance, and the majority class or average value in the leaf node is assigned as the prediction 72 .
K-Nearest Neighbor: The k-nearest neighbors is a useful and simple technique for prediction and classification. It works by identifying the k closest data points (neighbors) in the feature space to a given query instance and then making predictions based on their labels or values. In classification, the predicted class is usually determined by a majority vote of the k nearest neighbors, whereas in regression, the predicted value is frequently the average of the k nearest neighbors' values. The number of neighbors to consider (k) is an important hyperparameter that can have a significant impact on the algorithm's performance. A lower value of k results in more flexible decision boundaries but may increase sensitivity to noise, whereas a higher value of k smooths out the decision boundaries but may oversimplify the model. The k-NN algorithm is a popular choice for a variety of machine learning tasks due to its simplicity and effectiveness, particularly when the underlying data distribution is unknown or interpretability is required 73 .
Gradient Boosting: Gradient boosting is an effective machine-learning technique for both regression and classification tasks. It constructs a predictive model sequentially using an ensemble of weak learners, typically decision trees. The algorithm works by fitting a series of trees to the previous trees' residuals (or gradients), with each new tree attempting to correct the errors made by the predecessors. Gradient boosting, an iterative process, builds a strong learner by combining the predictions of multiple weak learners, frequently achieving high predictive accuracy. Gradient boosting's key components include the selection of a loss function, the learning rate, which controls the contribution of each tree to the ensemble, and the maximum depth or complexity of the individual trees 74 .
Long Short-Term Memory: Long Short-Term Memory (LSTM) is a recurrent neural network structure that aims to overcome the limitations of traditional RNNs in recording extensive dependents within data that is ordered. LSTMs use a gating mechanism consisting of input, forget, and output gates to control information flow throughout the network, in contrast to traditional RNNs, which have difficulties with the vanishing gradient problem when learning from remote dependencies. With the use of this gating mechanism, long sequences of data can be retained with greater relevance while lowering the likelihood of signal degradation. This allows LSTMs to selectively update and discard information over time. Furthermore, LSTMs include a cell state that acts as a conveyor belt, carrying information across time steps and facilitating the flow of gradients during training. As a result, LSTMs have become a key component in sequence modeling tasks, outperforming traditional RNN architectures 75 .
Performance evaluation of machine learning models
Different assessment measures are utilized to assess the accuracy of the proposed machine learning algorithms. These measures include Root Mean Square Error (RMSE), Mean Absolute Error (MAE), Mean Absolute Percentage Error (MAPE), and Coefficient of Determination (COD).
1. Mean Absolute Error 76 : It measures the mean absolute difference between the values that a model predicts and the values that are actually in a dataset. It is given by the following formula:
where the yi is the actual value of the energy consumption at point , n represents the number of educational buildings, and \(\widehat{{y}_{i}}\) is predicted value of the energy consumption at point.
2. Root Mean Square Error 77 : It calculates the square root of the average squared variances between the actual and anticipated values in a dataset. Mathematically, RMSE is calculated as:
3. Mean Absolute Percentage Error 78 : It calculates the mean absolute percentage difference between a dataset's actual values and its predicted values. Mathematically, MAPE is calculated as:
4. Coefficient of Determination 79 : It demonstrates the percentage of a machine learning model's dependent variable's variance that can be predicted based on the independent variables. In other words, COD quantifies the goodness of fit of the model to the actual data. It is given by the following formula:
Results and discussion
Descriptive statistics of the data variables.
The descriptive statistics is fundamental to understand the interpretation of data science algorithms, which is depicted in Table 2 . The study encompassed data collection from 352 schools, presenting a comprehensive analysis of various structural and operational characteristics. The mean number of floors was approximately 3.26, with the built area averaging at 2,596.59 square meters and the roof area at 4,165.63 square meters. School types varied with a mean value suggesting a mix of primary and secondary education facilities. On average, schools had 381.10 students and 28.18 staff members. The average age of the school buildings was noted to be approximately 32.82 years, reflecting a moderate level of infrastructural maturity. Classrooms averaged at 14.26 per school, indicating moderate to large school sizes. Air-conditioned areas averaged 817.71 square meters, with the AC capacity averaging at 160.12 tons of refrigeration. The mean annual energy consumption of the schools was a substantial 410,968 kWh/year. The data displayed a standard deviation in total built area and roof area of 1902.62 and 649.17 square meters, respectively, indicating significant variability among the sizes of the schools surveyed. The standard deviation in the number of students and staff was 217.57 and 9.35, respectively, which illustrates a diverse range of school populations and staffing levels. The variability in air-conditioned area and AC capacity (std of 354.60 m 2 and 55.82 tons of refrigeration) reflects different approaches to climate control.
Data visualization and interpretation
The data visualization is supported through the usage of a scatter matrix which is displayed in Fig. 2 . The scatter matrix provided allows for a comprehensive pairwise comparison of the variables in relation to one another, including their individual distributions. This form of analysis is instrumental in discerning the nature of relationships across multiple dimensions of the dataset, especially focusing on how they may correlate with annual energy consumption in schools. Analyzing the histograms on the diagonal, a distribution of each variable can be noted. For instance, 'City' appears to have a uniform distribution, whereas 'Annual Consumption' seems to be skewed, with a high occurrence of lower values and fewer instances of high energy consumption, suggesting a concentration of schools with lower energy use. The scatter plots off the diagonal offer insights into bivariate relationships. For example, 'Total Built Area' vs. 'Total Roof Area' displays a linear trend, indicating a strong positive correlation, as expected since larger built areas typically result in larger roof areas. Similarly, 'Number of Students' vs. 'Number of Classrooms' shows a clear positive trend, highlighting that schools with more students tend to have more classrooms. Considering 'Annual Consumption', the plots against variables like 'Total Built Area', 'Total Roof Area', and 'AC Capacity' reveal positive correlations, as indicated by the concentration of points along an upward trajectory. This supports the hypothesis that larger school sizes and greater AC capacities are associated with higher energy consumption. Conversely, variables such as 'Type of School' show a more dispersed scatter with 'Annual Consumption', suggesting a less direct or weaker correlation. This implies that the type of school may not be a strong determinant of its energy consumption levels. The 'Number of Floors' does not show a distinct trend in relation to 'Annual Consumption', which might indicate that the number of floors in a school is not a straightforward predictor of its energy use, possibly due to variations in building design and usage patterns. It is also interesting to note the patterns in 'Number of Students', 'Number of Staff', and 'Number of Classrooms', which when plotted against 'Annual Consumption', show a more cloud-like distribution with a slight positive correlation. This suggests that while larger numbers in these categories can lead to increased energy use, the relationship is not as pronounced or linear as with physical building characteristics. Finally, the relationship between 'Age of Building' and 'Annual Consumption' does not present a clear pattern, indicating that the age may not have a straightforward impact on energy usage. This could be due to a variety of factors, such as renovations, maintenance practices, or the installation of energy-efficient systems in older buildings. In summary, the scatter matrix provides a rich visual framework for understanding the complex interrelationships between school characteristics and energy consumption. It highlights significant variables that warrant closer examination and could inform strategies for energy efficiency, facility design, and management within the educational sector.
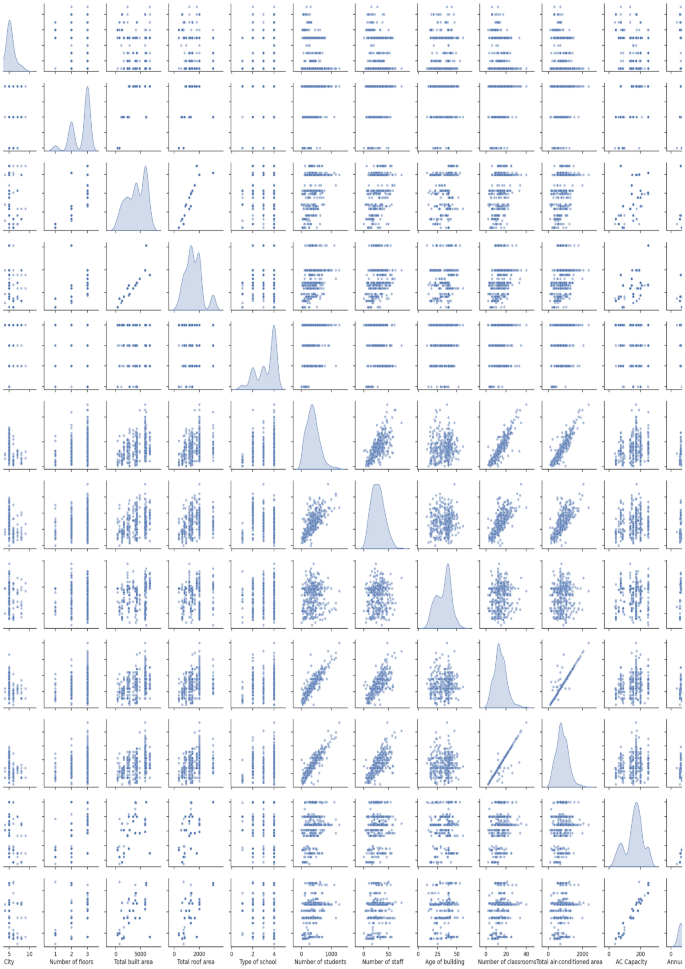
Scatter matrix of the variables. A full resolution figure can be reviewed on the link: https://doi.org/10.6084/m9.figshare.26024836 .
The visualization of data is higher order dimensions is quite tricky, therefore a parallel coordinate plot of all the variables is displayed in Fig. 3 , whereas, the line colors on it are only tracing the variability in the annual energy consumption. Therefore, the parallel coordinate plot provides a visual representation of multivariate data, allowing us to discern patterns and relationships between the various dimensions of the dataset, particularly in relation to annual energy consumption in schools. From the visualization, it is evident that certain variables exhibit a greater range of values and are more densely interwoven with annual consumption, which is color-coded from purple to yellow, indicating low to high energy usage, respectively. Notably, the lines connecting 'Total Roof Area', 'Number of Students', 'Number of Classrooms', and 'AC Capacity' to 'Annual Consumption' display a gradient trend, transitioning from cooler to warmer colors as the values increase. This suggests a positive correlation where higher values in these variables tend to be associated with greater energy consumption. The variables 'City' and 'Number of Floors', while also connected to varying degrees of energy consumption, show less of a clear gradient, indicating that the relationship with energy use may be influenced by other factors or is less directly correlated. Furthermore, the 'Type of School' and 'Age of Building' appear to have a more diffuse distribution of colors across their range, implying a weaker or less direct relationship with energy consumption. These lines do not follow a clear pattern, suggesting that the type of school and the age of the building are not as strongly predictive of energy usage as the size or occupancy-related variables. It is also noteworthy that 'Total Built Area', much like 'Total Roof Area', displays a concentration of warmer colors at higher values, supporting the idea that larger physical dimensions are indicative of increased energy needs. The densely packed lines connecting 'Number of Classrooms' and 'Total Air-Conditioned Area' with higher annual consumption rates further underscore the significant impact of space usage on energy consumption. The correlation appears stronger as the air-conditioned space is a direct consumer of energy, primarily through cooling systems. In contrast, the visual representation does not suggest a strong direct relationship between 'Number of Staff' and energy consumption, as evidenced by the more uniformly distributed color range across its values. Lastly, 'AC Capacity' shows a very strong association with energy consumption, indicated by a predominance of yellow lines as AC capacity increases, reinforcing the assumption that air conditioning is a substantial component of a school's energy profile. Overall, the parallel coordinate plot underlines the multidimensional nature of energy consumption in educational facilities. It highlights the significance of spatial parameters and student density as strong indicators of energy use, while also illustrating the intricate relationships and potential confounding factors that must be considered in the pursuit of energy efficiency and sustainability in school design and operation.
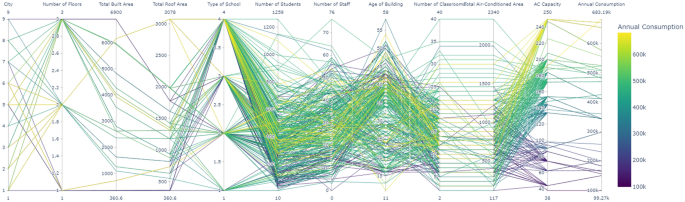
Parallel coordinate plot of the variables. A full resolution figure can be reviewed on the link: https://doi.org/10.6084/m9.figshare.26025634 .
The coefficient of correlation based upon the mathematics of Pearson can improve the understanding between the correlation of the input variables and the target which is annual energy consumption of the educational buildings, which is depicted in Fig. 4 . Upon analyzing the Pearson Coefficient of Correlation between various school characteristics and their annual energy consumption, several interesting relationships emerge. The city shows a moderate negative correlation (− 0.3256) with energy consumption, indicating that certain urban factors might be leading to more efficient energy use or that different locales may have varying energy requirements. In contrast, the number of floors within a school displays a positive correlation (0.3218) with energy consumption. This could be due to the additional energy required for heating or cooling larger vertical spaces and the use of elevators. The total built area and roof area of schools show positive correlations of 0.4678 and 0.6511 with annual energy consumption, respectively, which are among the strongest observed in the dataset. These correlations suggest that as the physical footprint of a school increases, so does its energy demand, likely due to the larger volumes of space requiring climate control. Interestingly, the type of school has an almost negligible positive correlation (0.0119) with energy consumption. The student and staff numbers show positive correlations with energy consumption, 0.3088 and 0.1589 respectively, though the strength of these relationships is varied. The number of students has a stronger correlation, which could be due to the fact that more students likely require more resources, including lighting, heating, cooling, and technology, all of which contribute to energy use. The number of staff has a weaker positive correlation, indicating that staff numbers alone are not as significant a determinant of energy consumption as student numbers. Age of the building shows a very weak positive correlation (0.0217) with energy consumption, suggesting that older buildings may not necessarily consume more energy than newer ones, possibly due to factors such as construction materials, design, or retrofits. The number of classrooms and the total air-conditioned area both show weak to moderate positive correlations (0.2495 and 0.2301, respectively) with energy consumption. More classrooms could imply more space to heat, cool, and light, whereas more air-conditioned space directly relates to higher energy use due to the demands of cooling systems. Finally, the AC capacity shows a very strong positive correlation (0.9703) with annual energy consumption. This is to be expected, as air conditioning is a major energy consumer within buildings, especially in climates that require it year-round or for large parts of the year. In summary, the size of the school (in terms of both physical dimensions and occupancy) and the capacity of air conditioning systems are prominent factors in energy consumption. The relationship between these variables and energy use is multifaceted, with implications for energy management, sustainability initiatives, and the design of educational facilities. While certain correlations are stronger than others, each variable contributes to the overall energy footprint of a school, and understanding these relationships is key for developing strategies to reduce energy consumption and promote efficiency.
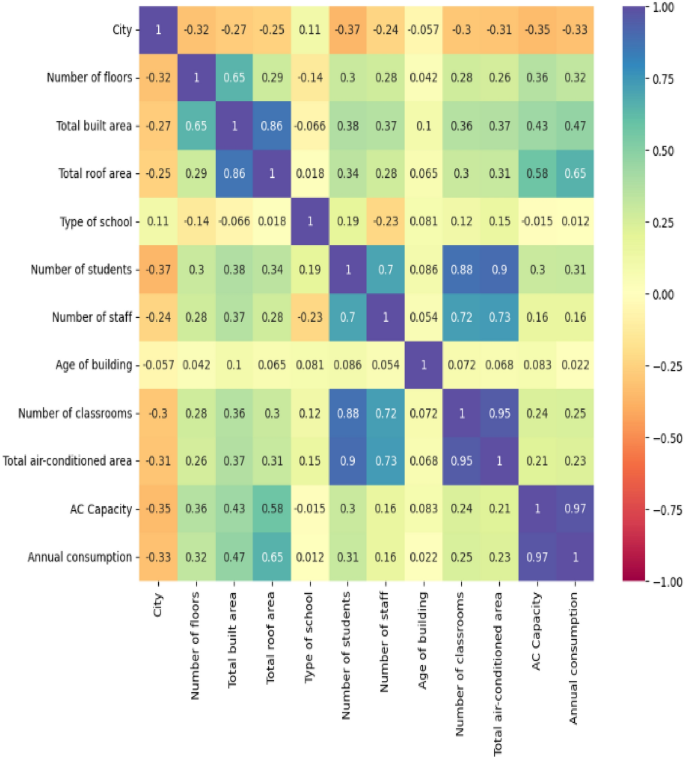
Heat map based upon Pearson coefficient of correlation.
Artificial intelligence models to predict the energy consumption in educational buildings
Various artificial intelligence methods from the machine learning block like decision tree, K-Nearest Neighbors, Gradient Boosting, and one deep learning method which is Long-Short-Term Memory was implemented, and the construction of the hyper-parameters are developed as follows:
Decision tree: In this study, a Decision Tree Regressor algorithm was developed to model and predict annual energy consumption in schools, considering various structural and operational characteristics as predictors. The model was constructed using a robust machine learning pipeline employing Scikit-learn, a popular Python library for data science and machine learning. The predictive features (X) incorporated in the model were: 'Number of floors', 'Total built area', 'Total roof area', 'Number of students', 'Number of staff', 'Age of building', 'Number of classrooms', 'Total air-conditioned area', and 'AC Capacity', with the target variable (y) being 'Annual consumption'. The dataset was divided into a training set (80%) and a testing set (20%), ensuring the model's ability to generalize to new, unseen data. To optimize the model's performance, a grid search with five-fold cross-validation was executed over a predefined range of hyperparameters. The parameters included various depths, minimum samples for splitting and at leaf nodes, maximum features at each split, and the maximum number of leaf nodes. The grid search aimed to find the optimal combination of parameters that minimized the negative mean squared error (MSE). The best hyperparameters for the Decision Tree were determined to be a maximum depth of 5, utilizing all features at each split ('auto'), a limit of 10 maximum leaf nodes, a minimum of 10 samples at each leaf node, and a minimum of 20 samples required to split a node. This configuration indicates a model complex enough to capture underlying patterns, yet restrained to prevent overfitting.
K-Nearest Neighbor: A K-Nearest Neighbors (KNN) Regressor algorithm was implemented to forecast the annual energy consumption in educational institutions, leveraging a variety of structural and operational features as predictive variables. The model was constructed using Scikit-learn, an esteemed Python library renowned for its proficiency in machine learning and data science endeavors. The feature set incorporated into the model constituted all available variables except for the 'Annual consumption', which served as the target variable (y). The dataset was prudently partitioned into training and testing subsets, with an 80–20 split, reinforcing the model's robustness in generalizing to unseen data points. To ascertain the most efficacious configuration of hyperparameters, an exhaustive grid search, coupled with five-fold cross-validation, was executed. This entailed a methodical exploration of different numbers of neighbors, weight schemes, algorithms, and distance metrics. The grid search's chief objective was to pinpoint the hyperparameter combination that would minimize the negative mean squared error, thereby enhancing the model's predictive accuracy. The implementation of the K-Nearest Neighbors (KNN) algorithm involved an extensive hyperparameter tuning process using GridSearchCV, a method that systematically works through multiple combinations of parameter tunes, cross-validating as it goes to determine which tune gives the best performance. The algorithm evaluated a total of 64 candidate models across 5 different folds of the dataset, resulting in 320 individual fits. Subsequent predictions on both the training and testing data were then generated using this refined model.
Gradient Boosting: Gradient Boosting Regressor model was implemented to forecast annual energy consumption in schools based on a set of selected features. Employing Scikit-learn's comprehensive toolkit, the integrated features that represent both physical characteristics and demographic factors of schools, such as 'Number of floors', 'Total built area', 'Total roof area', 'Number of students', 'Number of staff', 'Age of building', 'Number of classrooms', 'Total air-conditioned area', and 'AC Capacity', with the target variable being the 'Annual consumption'. A systematic division of the dataset facilitated the creation of distinct training and testing cohorts, comprising 80% and 20% of the data, respectively. This was followed by standardization of the features to normalize the data, enabling more effective learning by the model. The model's calibration was meticulously conducted through a GridSearchCV process that spanned a diverse array of hyperparameters, including the number of estimators, learning rate, subsample rate, and tree depth, across fivefold cross-validation to enhance the reliability of the results. The objective was to discover the optimal settings that minimize the negative mean squared error, a metric that captures the average squared difference between the estimated values and the actual value. The search across the hyperparameter space evaluated 54 unique candidates in 270 fitting iterations, rigorously assessing each combination to ensure the selection of the most predictive model configuration.
Long-Short Term Memory (LSTM) : LSTM neural network model was developed to predict the annual energy consumption of schools. This model represents a sophisticated form of recurrent neural network capable of learning order dependence in sequence prediction problems. Normalization of the data was paramount, achieved through the application of MinMaxScaler , which scaled the feature set and target variables to a bounded interval of [0, 1]. This scaling facilitated the neural network's convergence during training by providing numerical stability and improved efficiency. The dataset was then divided into a training subset accounting for 80% of the data and a testing subset forming the remaining 20%, with the intention of validating the model's predictive performance on unseen data. The LSTM model's architecture was constructed with an input layer designed to accept the reshaped feature set, followed by an LSTM layer with 50 units and 'relu' activation, and culminating in a Dense output layer for prediction. The model's learning process involved an 'adam' optimizer and mean squared error loss function across 50 epochs, with a batch size of 32, underscoring the iterative refinement of the model's weights.
Once the computer program for the corresponding machine learning algorithm is developed, afterwards, it is evaluated graphically and quantitatively. The graphical evaluation can be noted in Fig. 5 which shows the regression for all of the techniques. Figure 5 comprises four panels labeled (a), (b), (c), and (d), each representing a scatter plot comparing actual versus predicted annual energy consumption using different predictive models: Decision Tree, K-Nearest Neighbors (KNN), Gradient Boosting, and Long Short-Term Memory (LSTM) neural network, respectively. The actual annual consumption is plotted along the x-axis, while the predicted values are on the y-axis for each model. The presence of a trend line in each graph provides a reference for perfect prediction, where the predicted values would ideally match the actual consumption exactly. The points are color-coded, with blue dots representing training data and red crosses denoting testing data. This color scheme allows for immediate visual discrimination between the model's performance on the data it was trained on and its predictive power on new, unseen data. In the similar context, Fig. 5 e explains another way of presenting LSTM data points.
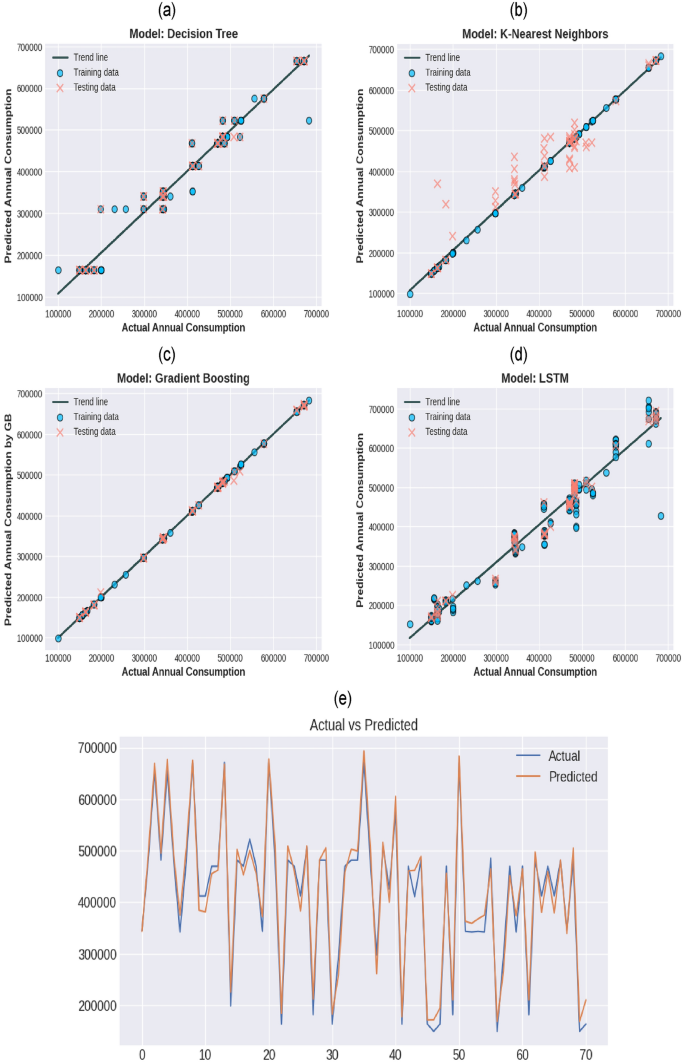
Regression fit of ( a ) Decision trees, ( b ) K-nearest neighbor, ( c ) gradient boosting, ( d ) LSTM. ( e ) LSTM representation in time series.
The Decision Tree model displays a reasonable spread of training data around the trend line but shows some variance in the predictions of testing data, as seen by the red crosses that stray further from the trend line. This might suggest a degree of overfitting to the training data, or it could simply reflect the model's inherent variance when dealing with more complex patterns not captured during training. In contrast, the KNN model exhibits a more consistent pattern of prediction across both training and testing datasets, with the majority of points closely aligned with the trend line. However, there are noticeable outliers in the testing data, which may imply that while the model generalizes well in most cases, it may be sensitive to certain data points or to noise within the dataset. The Gradient Boosting scatter plot demonstrates an impressive adherence of the data points to the trend line, with both training and testing data showing tight clustering around this line. This indicates that the model not only learned the training data effectively but also generalized well to the testing data, showcasing the strength of Gradient Boosting in capturing complex relationships within the data. Lastly, the LSTM neural network, known for its ability to capture sequential and temporal relationships, shows a distinctive pattern. Training data is well-fitted, and testing data, while slightly more scattered, still follows the trend line reasonably well. Notably, the LSTM shows more variation in the higher range of actual consumption values, which could indicate the model's different handling of more extreme values or its response to the nuances of the sequence-based data. When comparing the four models, Gradient Boosting (Panel c) seems to outperform the others in terms of the tightness of data points around the trend line, suggesting a high accuracy and good generalization. The KNN and LSTM model also generalizes well but with some noted exceptions.
Each model's unique characteristics influence its performance, with tree-based models like Decision Trees and Gradient Boosting often handling non-linear relationships effectively, while KNN relies on localized data patterns, and LSTM models excel in capturing temporal dynamics. The selection of the best model would therefore depend on the specific characteristics of the dataset and the performance metric of interest, whether it be accuracy, robustness, or the ability to generalize from limited data.
Our analysis compares four machine learning techniques across two phases: training and testing. Table 3 provides performance metrics such as Root Mean Square Error (RMSE), Mean Absolute Error (MAE), Mean Absolute Percentage Error (MAPE), and Coefficient of Determination (COD). The Decision Tree model exhibits strong performance on the training data, with a relatively low RMSE of 20,716.25 and MAE of 10,764.99, indicating good fit. The MAPE value of 3.580435 shows that, on average, the prediction error is about 3.58% of the actual value, which is respectable in practical applications. The high COD of 0.978928 for training and 0.980852 for testing indicates that the model explains a large portion of the variance in the data, confirming its effectiveness. The K-Nearest Neighbors model has significantly higher errors, with RMSE on training data as high as 38,429.4, which may be indicative of overfitting, considering the perfect COD of 0.934134. The testing phase does not fare much better, with a further increased RMSE of 40,603.04. This could be due to the model's sensitivity to the local structure of the training data, which doesn't generalize well. In contrast, Gradient Boosting outperforms other models during the training phase with a remarkably low RMSE of 374.7943 and an almost perfect COD, signifying that the model can almost perfectly predict the variations within the training dataset. However, the model's ability to generalize is not as clear without the testing phase COD, even though the testing RMSE increases to 3559.443. The LSTM network, designed to capture long-term dependencies and temporal dynamics in sequential data, shows good performance on both training and testing data. The RMSE and MAE values are moderate, with the model achieving a higher COD value on the testing set (0.975606) than on the training set (0.956432), which suggests it generalizes well and confirms its suitability for time-series forecasting like annual consumption. Our dataset's characteristics, with a substantial standard deviation, imply significant variability in annual consumption, which is a common challenge in time-series prediction. The performance metrics suggest that some models manage this variability better than others, with Gradient Boosting and LSTM standing out in terms of their ability to handle diverse data ranges, from the minimum consumption of approximately 99,274.95 to the maximum of 683,191.8. This evaluation demonstrates the importance of choosing the right model for time-series forecasting. While Decision Trees and KNN provided baseline performances, the Gradient Boosting and LSTM models showed advanced capabilities, which could be attributed to their sophisticated handling of non-linear relationships and temporal dependencies in the data.
Educational facilities, including schools, represent a major piece of infrastructure that significantly impacts economic, social and environment sustainable development. They contribute to approximately 37% of the carbon dioxide emissions associated with energy use and procedures. This paper presents an investigation study for prediction energy consumption of school buildings utilizing machine learning technologies. The methodology followed to meet the objectives of the study consists of four main steps. The first step begins with identifying the variable impacting consumption of energy in school buildings based on both review of the literature and meeting the experts. In the second step, the actual data on energy consumption was collected, filtered, and analyzed to develop robust machine-learning models. The third step comprises of separating the collected data into distinct subsets for training, validation, and testing. The fourth step involves the development and the investigation of several artificial intelligence-based models for energy consumption of educational buildings including decision trees, K-nearest neighbors, gradient boosting, and long-term memory networks.
The study revealed that the decision tree-based prediction model illustrates strong performance with an average prediction error of about 3.58%. The K-Nearest Neighbors model has significantly higher errors, with RMSE on training data as high as 38,429.4. Conversely, Gradient Boosting perfectly predicts energy consumption of school buildings. The performance metrics suggest that some models manage this variability better than others. Gradient boosting and LSTM stand out in terms of their ability to handle diverse data ranges, from the minimum consumption of approximately 99,274.95 to the maximum of 683,191.8. Furthermore, the relationship between the input factors and the annual consumption of energy of educational buildings illustrates that school sizes and AC capacities are the most impacted variable associated with higher energy consumption. While 'Type of School' is less direct or weaker correlation with 'Annual Consumption'.
It is essential to note that this study was carried out based on typical school facilities in hot climate. Thus, the results of this research may not be suitable to other school types and/or climates. Moreover, extended study can be conducted in the future to include different school types, structure system, and different climate.
Future research should focus on enhancing the role of educational buildings as interactive learning environments that promote sustainability principles. By using advanced AI-driven tools to optimize energy consumption, future studies could develop and implement smart, adaptive systems that not only manage energy use more efficiently but also serve as educational tools themselves. These systems could provide real-time data and simulations that allow students to observe, interact with, and learn from the building’s energy dynamics. This approach would not only improve the sustainability of educational facilities but also actively engage students with practical lessons on energy efficiency and environmental concepts, thereby embedding these critical concepts into their daily experiences and learning environments, as a part of lifelong learning.
Data availability
Data will be made available on request. Please request Professor Rasikh Tariq for the data simulation and Professor Awsan Mohammed for the experimentation.
Alfaoyzan, F. A. & Almasri, R. A. Benchmarking of energy consumption in higher education buildings in Saudi Arabia to be sustainable: Sulaiman Al-Rajhi University case. Energies 16 (3), 1204 (2023).
Article CAS Google Scholar
IEA. Buildings. Paris. License: IEA. https://www.iea.org/reports/buildings . CC BY 4.0 (2022).
Zhao, H. X. & Magoules, F. A review on the prediction of building energy consumption. Renew. Sustain. Energy Rev. 16 (6), 3586–3592 (2012).
Article Google Scholar
Gassar, A. A. A. & Cha, S. H. Energy prediction techniques for large-scale buildings towards a sustainable built environment: A review. Energy Build. 224 , 110238 (2020).
Asimakopoulos, D. N. & Doulamis, A. D. Predictive analytics for energy consumption in educational buildings: A review of modeling techniques. Sustain. Cities Soc. 50 , 101656 (2019).
Google Scholar
Debnath, K. B. & Mourshed, M. Forecasting methods in energy planning models. Renew. Sustain. Energy Rev. 88 , 297–325 (2018).
Alshibani, A. Prediction of the energy consumption of school buildings. Appl. Sci. 10 , 5885 (2020).
Kim, S.-G., Jung, J.-Y. & Sim, M. K. A two-step approach to solar power generation prediction based on weather data using machine learning. Sustainability 11 , 1501 (2019).
Blumsack, S. & Fernandez, A. Ready or not, here comes the smart grid!. Energy 37 , 61–68 (2012).
Zhong, H., Wang, J., Jia, H., Mu, Y. & Lv, S. Vector field-based support vector regression for building energy consumption prediction. Appl. Energy 242 , 403–414 (2019).
Article ADS Google Scholar
Zhao, Y., Zhang, C., Zhang, Y., Wang, Z. & Li, J. A review of data mining technologies in building energy systems: Load prediction, pattern identification, fault detection and diagnosis. Energy Built. Environ. 1 , 149–164 (2020).
Wang, J., Hou, J., Chen, J., Fu, Q. & Huang, G. Data mining approach for improving the optimal control of HVAC systems: An event-driven strategy. J. Build Eng. 39 , 102246 (2021).
Darwazeh, D., Duquette, J., Gunay, B., Wilton, I. & Shillinglaw, S. Review of peak load management strategies in commercial buildings. Sustain. Cities Soc. 77 , 103493 (2022).
Jin, W. et al. A novel building energy consumption prediction method using deep reinforcement learning with consideration of fluctuation points. J. Build. Eng. 63 , 105458. https://doi.org/10.1016/j.jobe.2022.105458 (2023).
Gellert, A., Fiore, U., Florea, A., Chis, R. & Palmieri, F. Forecasting electricity consumption and production in smart homes through statistical methods. Sustain. Cities Soc. 76 , 103426 (2022).
Zhang, W., Chen, Q., Yan, J., Zhang, S. & Xu, J. A novel asynchronous deep reinforcement learning model with adaptive early forecasting method and reward incentive mechanism for short-term load forecasting. Energy 236 , 121492. https://doi.org/10.1016/j.energy.2021.121492 (2021).
Purnell, K., Sinclair, M. & Gralton, A. Sustainable schools: Making energy efficiency a lifestyle priority. Aust. J. Environ. Educ. 20 (2), 81–91. https://doi.org/10.1017/S0814062600002226 (2004).
Rogora, A., & Dessì, V. Recent Examples of Low Energy and Sustainable Schools in Italy. In 22nd International Conference, PLEA 2005: Passive and Low Energy Architecture - Environmental Sustainability: The Challenge of Awareness in Developing Societies, Proceedings , 1, 275–280. https://re.public.polimi.it/bitstream/11311/693887/1/plea%202005-%20esempi%20di%20scuole.pdf (2005).
Zeiler W. & De Waard M. Some dutch examples of sustainable school concepts towards plus energy schools. In 28th Conference PLEA, Opportunities, Limits & Needs Towards an environmentally responsible architecture . Lima: Pontificia Universidad Católica del Perú (2012).
Ramírez-Montoya, M.S., Basabe, E., Carlos Arroyo, M., Patiño Zúñiga, I.A., & Portuguez Castro, M. Modelo abierto de pensamiento complejo para el futuro de la educación. Octaedro. https://hdl.handle.net/11285/652033 (2024).
Passa, J. & Rompf, D. Energy efficient sustainable schools in Canada South. J. Green Build. 2 (2), 14–30. https://doi.org/10.3992/jgb.2.2.14 (2007).
Golshan, M., Thoen, H. & Zeiler, W. Dutch sustainable schools towards energy positive. J. Build. Eng. 19 , 161–171. https://doi.org/10.1016/j.jobe.2018.05.002 (2018).
Zhang, Q., Koh, B. B., & Ahn, Y. H. Energy saving technologies and sustainable strategies of sustainable school buildings: A case study of isaac dickson elementary school. Int. J. Sustain. Build. Technol. Urban Dev. 11 (2), 94–111. https://doi.org/10.22712/susb.20200008 (2020).
Boeri, A. & Longo, D. Environmental quality and energy efficiency: sustainable school buildings design strategies. Int. J. Sustain. Dev. Plan. 8 (2), 140–157. https://doi.org/10.2495/SDP-V8-N2-140-157 (2013).
Msaddek, M. H., Moumni, Y., Ayari, A., El May, M. & Chenini, I. Artificial intelligence modelling framework for mapping groundwater vulnerability of fractured aquifer. Geocarto Int. 37 (25), 10480–10510. https://doi.org/10.1080/10106049.2022.2037729 (2022).
Tao, H. et al. Artificial intelligence models for suspended river sediment prediction: state-of-the art, modeling framework appraisal, and proposed future research directions. Eng. Appl. Comput. Fluid Mech. 15 (1), 1585–1612. https://doi.org/10.1080/19942060.2021.1984992 (2021).
Baki, S., Koutiva, I. & Makropoulos, C. A hybrid artificial intelligence modelling framework for the simulation of the complete, socio-technical, urban water system. Eng. Appl. Comput. Fluid Mech. 15 (1), 1585–1612. https://doi.org/10.1080/19942060.2021.1984992 (2012).
Méndez-Suárez, M., García-Fernández, F. & Gallardo, F. Artificial intelligence modelling framework for financial automated advising in the copper market. J. Open Innov. Technol. Mark. Complex. 5 (4), 81. https://doi.org/10.3390/joitmc5040081 (2019).
Reddy, R. S., Keesara, N., Pudi, V., & Garg, V. Plug load identification in educational buildings using machine learning algorithms. In Proceedings of BS2015: 14th conference of international building performance simulation association, Hyderabad, India , pp. 1940–1946 (2015).
López-Pérez, L. A. & Flores-Prieto, J. J. Adaptive thermal comfort approach to save energy in tropical climate educational building by artificial intelligence. Energy 263 , 125706. https://doi.org/10.1016/j.energy.2022.125706 (2023).
Hosseini, P., Nikbakht Naserabad, S., Keshavarzzadeh, A. H. & Ansari, N. Artificial intelligence-based tri-objective optimization of different demand load patterns on the optimal sizing of a smart educational buildings. Int. J. Energy Res. 46 (15), 21373–21396. https://doi.org/10.1002/er.8095 (2022).
Lee, M. J. & Zhang, R. Human-centric artificial intelligence of things-based indoor environment quality modeling framework for supporting student well-being in educational facilities. J. Comput. Civ. Eng. 38 (2), 04024002. https://doi.org/10.1061/JCCEE5.CPENG-5632 (2024).
Directive 2002/91/EC of the European parliament and of the council of 16 December 2002 on the energy performance of buildings. Off J Eur Union 65e71. https://doi.org/10.1039/ap9842100196 (2002).
Foucquier, A., Robert, S., Suard, F., Stephan, L. & Jay, A. State of the art in building modelling and energy performances prediction: A review. Renew. Sustain. Energy Rev. 23 , 272–288 (2013).
Foucquier, S., Robert, F., Suard, L. & Stephan, A. Jay, State of the art in building modelling and energy performances prediction: A review. Renew. Sustain. Energy Rev. 23 , 272–288 (2013).
Runge, J. & Zmeureanu, R. A review of deep learning techniques for forecasting energy use in buildings. Energies 14 , 1 (2021).
Fathi, S., Srinivasan, R., Fenner, A. & Fathi, S. Machine learning applications in urban building energy performance forecasting: A systematic review. Renew. Sustain. Energy Rev. 133 , 110287 (2020).
Chae, Y. T. et al. An artificial neural network model for forecasting sub-hourly electricity usage in commercial buildings[J]. Energy Build 111 , 184–194 (2016).
Biswas, M., Robinson, M. D. & Fumo, N. Prediction of residential building energy consumption: A neural network approach [J]. Energy 117 , 84–92 (2016).
Deb, C. et al. Forecasting diurnal cooling energy load for institutional buildings using artificial neural networks [J]. Energy Build 121 , 284–297 (2016).
Yan, K. et al. A hybrid LSTM neural network for energy consumption forecasting of individual households[J]. IEEE Access 7 , 157633–157642 (2019).
Zhong, H. et al. Vector field-based support vector regression for building energy consumption prediction[J]. Appl. Energy 242 , 403–414 (2019).
Wang, X. et al. Estimates of energy consumption in China using a self-adaptive multi-verse optimizer-based support vector machine with rolling cross-validation[J]. Energy 152 , 539–548 (2018).
Tabrizchi, H., Javidi, M. M. & Amirzadeh, V. Estimates of residential building energy consumption using a multi-verse optimizer-based support vector machine with k-fold cross-validation[J]. Evol. Syst. 12 , 755–767 (2019).
Iwafune, Y., Yagita, Y., & Ikegami, T., et al. Short-term forecasting of residential building load for distributed energy management[C]. In 2014 IEEE international energy conference (ENERGYCON). IEEE ; pp. 1197–204 (2014).
Albuquerque, P. C., Cajueiro, D. O. & Rossi, M. D. C. Machine learning models for forecasting power electricity consumption using a high dimensional dataset[J]. Expert. Syst. Appl. 187 , 115917 (2022).
Dong, Z. et al. Hourly energy consumption prediction of an office building based on ensemble learning and energy consumption pattern classification [J]. Energy Build 241 , 110929 (2021).
Cao, W. et al. Short-term energy consumption prediction method for educational buildings based on model integration. Energy 283 , 128580 (2023).
Faiq, M. et al. Prediction of energy consumption in campus buildings using long short-term memory. Alex. Eng. J. 67 , 65–76 (2023).
Álvarez, J.A., et al. Modeling of energy efficiency for residential buildings using artificial neuronal networks. Adv. Civ. Eng. (2018).
Beccali, M. et al. Artificial neural network decision support tool for assessment of the energy performance and the refurbishment actions for the non-residential building stock in southern Italy. Energy. 137 , 1201–1218 (2017).
Ahmad, M. W., Mourshed, M. & Rezgui, Y. Trees vs neurons: Comparison between random forest and ANN forhigh-resolution prediction of building energy consumption. Energy Build. 147 , 77–89 (2017).
Martellotta, F. et al. On the use of artificial neural networks to model household energy consumptions. Energy Proc. 126 , 250–257 (2017).
Williams, K. T. & Gomez, J. D. Predicting future monthly residential energy consumption using building characteristics and climate data: A statistical learning approach. Energy Build. 128 , 1–11 (2016).
Sun, C. & Han, Y. Constructing heating energy consumption forecast ANN model for office building in severe cold zone. Architectural 538 (10), 154–158 (2013).
Wong, S., Wan, K. K. & Lam, T. N. Artificial neural networks for energy analysis of office buildings with daylighting. Appl. Energy 87 (2), 551–557 (2010).
Catalina, T., Virgone, J. & Blanco, E. Development and validation of regression models to predict monthly heating demand for residential buildings. Energy Build. 40 (10), 1825–1832 (2008).
Alrashed, F. & Asif, M. Trends in residential energy consumption in Saudi Arabia with particular reference to the Eastern Province. J. Sustain. Dev. Energy Water Environ. Syst. 2 (4), 376–387 (2014).
Abdel-Aal, R. E., Al-Garni, A. Z. & Al-Nassar, Y. N. Modelling and forecasting monthly electric energy consumption in eastern Saudi Arabia using abductive networks. Energy 22 (9), 911–921 (1997).
Nasr, G. E., Badr, E. A. & Younes, M. R. Neural networks in forecasting electrical energy consumption: Univariate and multivariate approaches. Int. J. Energy Res. 26 (1), 67–78 (2002).
Meng, M., Shang, W. & Niu, D. Monthly electric energy consumption forecasting using multiwindow moving average and hybrid growth models. J. Appl. Math. 2014 (1), 243171 (2014).
Karatasou, S., Santamouris, M. & Geros, V. Modeling and predicting building’s energy use with artificial neural networks: Methods and results. Energy Build. 38 (8), 949–958 (2006).
Mena-Yedra, R., Rodriguez, F., Castilla, M. M., & Arahal, M. R. A Neural Network Model for Energy Consumption Prediction of CIESOL Bioclimatic Building. In International Joint Conference SOCO (2013).
Somu, N., Gauthama Raman, M. R. & Ramamritham, K. A hybrid model for building energy consumption forecasting using long short term memory networks. Appl. Energy 261 , 114131 (2020).
Ullah, F., & Min, U. Short-term prediction of residential power energy consumption via CNN and multilayer bi-directional LSTM networks. IEEE Access (2019).
Liu, B. & Chuanchuan, Fu. Arlene Bielefield and Yan Quan Liu “Forecasting of Chinese primary energy consumption in 2021 with GRU artificial neural network”. Energies 10 (10), 1453 (2017).
Khalil, A. J., Barhoom, A. M., Abu-Nasser, B. S., Musleh, M. M., & Abu-Naser, S. S. Energy efficiency predicting using artificial neural network. 3 (9), 1–1 (2019).
Rahman, A., Srikumar, V. & Smith, A. D. Predicting electricity consumption for commercial and residential buildings using deep recurrent neural networks. Appl. Energy 212 , 372–385 (2018).
Tartibu, L. K., & Kabengele, K. T. Forecasting net energy consumption of South Africa using artificial neural network. In: 2018 International Conference on the Industrial and Commercial Use of Energy (ICUE). IEEE, pp. 1–7 (2018).
Fayaz, M., Shah, H., Aseere, A. M., Mashwani, W. K. & Shah, A. S. A framework for prediction of household energy consumption using feed forward back propagation neural network. Technologies 7 (2), 30 (2019).
Mohammed, A., Alshibani, A., Alshamrani, O. & Hassanain, M. A regression-based model for estimating the energy consumption of school facilities in Saudi Arabia. Energy Build. 237 , 110809 (2021).
Breiman, L. Classification and regression trees (Routledge, 2017).
Book Google Scholar
Peterson, L. E. K-nearest neighbor. Scholarpedia 4 (2), 1883 (2009).
Chen, T., & Guestrin, C. Xgboost: A scalable tree boosting system. In Proceedings of the 22nd acm sigkdd international conference on knowledge discovery and data mining (pp. 785–794) (2016).
Graves, A., & Graves, A. Long short-term memory. Supervised sequence labelling with recurrent neural networks, 37–45 (2012).
Uzuner, S., & Çekmecelioğlu, D. Comparison of artificial neural networks (ANN) and adaptive neuro-fuzzy inference system (ANFIS) models in simulating polygalacturonase production (2016).
Sada, S. O., & Ikpeseni, S. C. Evaluation of ANN and ANFIS modeling ability in the prediction of AISI 1050 steel machining performance. Heliyon 7 (2) (2021).
Zhang, G., Patuwo, B. E. & Hu, M. Y. Forecasting with artificial neural networks: The state of the art. Int. J. Forecast. 14 , 35–62 (1998).
Chong, D. J. S., Chan, Y. J., Arumugasamy, S. K., Yazdi, S. K. & Lim, J. W. Optimisation and performance evaluation of response surface methodology (RSM), artificial neural network (ANN) and adaptive neuro-fuzzy inference system (ANFIS) in the prediction of biogas production from palm oil mill effluent (POME). Energy 266 , 126449 (2023).
Download references
Acknowledgements
The authors would like to thank Tecnológico de Monterrey for the financial support provided through the ‘Challenge-Based Research Funding Program 2023’, Project ID #IJXT070-23EG99001, titled ‘Complex Thinking Education for All (CTE4A): A Digital Hub and School for Lifelong Learners’. The authors would like to acknowledge the financial and the technical support of Writing Lab, Institute for the Future of Education, Tecnologico de Monterrey, Mexico, in the production of this work.
Author information
Authors and affiliations.
Institute for the Future of Education, Tecnologico de Monterrey, Ave. Eugenio Garza Sada 2501, 64849, Monterrey, NL, Mexico
Rasikh Tariq & Maria Soledad Ramírez-Montoya
Architectural Engineering and Construction Management Department, King Fahd University of Petroleum and Minerals, 31261, Dhahran, Saudi Arabia
Awsan Mohammed & Adel Alshibani
Interdisciplinary Research Center for Smart Mobility and Logistics, King Fahd University of Petroleum and Minerals, 31261, Dhahran, Saudi Arabia
Awsan Mohammed
Interdisciplinary Research Center of Construction and Building Materials, King Fahd University of Petroleum and Minerals, 34463, Dhahran, Saudi Arabia
Adel Alshibani
EGADE Business School, Tecnologico de Monterrey, 64849, Monterrey, NL, Mexico
Maria Soledad Ramírez-Montoya
You can also search for this author in PubMed Google Scholar
Contributions
Awsan Mohammed and Adel Alshibani gathered the experimental data, wrote the method, abstract, Introduction, and Current-State-of-the-Art. Rasikh Tariq analyzed the experimental data, applied machine learning algorithms and wrote the results section. Maria Soledad Ramírez-Montoya developed the complex modeling framework through AI, wrote the theoretical framework, and reviewed the manuscript.
Corresponding authors
Correspondence to Rasikh Tariq or Awsan Mohammed .
Ethics declarations
Competing interests.
The authors declare no competing interests.
Additional information
Publisher's note.
Springer Nature remains neutral with regard to jurisdictional claims in published maps and institutional affiliations.
Rights and permissions
Open Access This article is licensed under a Creative Commons Attribution 4.0 International License, which permits use, sharing, adaptation, distribution and reproduction in any medium or format, as long as you give appropriate credit to the original author(s) and the source, provide a link to the Creative Commons licence, and indicate if changes were made. The images or other third party material in this article are included in the article's Creative Commons licence, unless indicated otherwise in a credit line to the material. If material is not included in the article's Creative Commons licence and your intended use is not permitted by statutory regulation or exceeds the permitted use, you will need to obtain permission directly from the copyright holder. To view a copy of this licence, visit http://creativecommons.org/licenses/by/4.0/ .
Reprints and permissions
About this article
Cite this article.
Tariq, R., Mohammed, A., Alshibani, A. et al. Complex artificial intelligence models for energy sustainability in educational buildings. Sci Rep 14 , 15020 (2024). https://doi.org/10.1038/s41598-024-65727-5
Download citation
Received : 07 May 2024
Accepted : 24 June 2024
Published : 01 July 2024
DOI : https://doi.org/10.1038/s41598-024-65727-5
Share this article
Anyone you share the following link with will be able to read this content:
Sorry, a shareable link is not currently available for this article.
Provided by the Springer Nature SharedIt content-sharing initiative
- Higher education
- Educational innovation
- Educational buildings
- Energy consumption
- Machine learning
By submitting a comment you agree to abide by our Terms and Community Guidelines . If you find something abusive or that does not comply with our terms or guidelines please flag it as inappropriate.
Quick links
- Explore articles by subject
- Guide to authors
- Editorial policies
Sign up for the Nature Briefing: AI and Robotics newsletter — what matters in AI and robotics research, free to your inbox weekly.


An official website of the United States government
The .gov means it’s official. Federal government websites often end in .gov or .mil. Before sharing sensitive information, make sure you’re on a federal government site.
The site is secure. The https:// ensures that you are connecting to the official website and that any information you provide is encrypted and transmitted securely.
- Publications
- Account settings
Preview improvements coming to the PMC website in October 2024. Learn More or Try it out now .
- Advanced Search
- Journal List
- HHS Author Manuscripts

The Mind and Brain of Short-Term Memory
The past 10 years have brought near-revolutionary changes in psychological theories about short-term memory, with similarly great advances in the neurosciences. Here, we critically examine the major psychological theories (the “mind”) of short-term memory and how they relate to evidence about underlying brain mechanisms. We focus on three features that must be addressed by any satisfactory theory of short-term memory. First, we examine the evidence for the architecture of short-term memory, with special attention to questions of capacity and how—or whether—short-term memory can be separated from long-term memory. Second, we ask how the components of that architecture enact processes of encoding, maintenance, and retrieval. Third, we describe the debate over the reason about forgetting from short-term memory, whether interference or decay is the cause. We close with a conceptual model tracing the representation of a single item through a short-term memory task, describing the biological mechanisms that might support psychological processes on a moment-by-moment basis as an item is encoded, maintained over a delay with some forgetting, and ultimately retrieved.
INTRODUCTION
Mentally add 324 and 468. Follow the instructions to complete any form for your federal income taxes. Read and comprehend this sentence.
What are the features of the memory system that allows us to complete these and other complex tasks? Consider the opening example. First, you must create a temporary representation in memory for the two numbers. This representation needs to survive for several seconds to complete the task. You must then allocate your attention to different portions of the representation so that you can apply the rules of arithmetic required by the task. By one strategy, you need to focus attention on the “tens” digits (“2” and “6”) and mitigate interference from the other digits (e.g., “3” and “4”) and from partial results of previous operations (e.g., the “12” that results from adding “4” and “8”). While attending to local portions of the problem, you must also keep accessible the parts of the problem that are not in the current focus of attention (e.g., that you now have the units digit “2” as a portion of the final answer). These tasks implicate a short-term memory (STM). In fact, there is hardly a task that can be completed without the involvement of STM, making it a critical component of cognition.
Our review relates the psychological phenomena of STM to their underlying neural mechanisms. The review is motivated by three questions that any adequate account of STM must address:
1. What is its structure?
A proper theory must describe an architecture for short-term storage. Candidate components of this architecture include storage buffers, a moving and varying focus of attention, or traces with differing levels of activation. In all cases, it is essential to provide a mechanism that allows a representation to exist beyond the sensory stimulation that caused it or the process that retrieved the representation from long-term memory (LTM). This architecture should be clear about its psychological constructs. Furthermore, being clear about the neural mechanisms that implement those constructs will aid in development of psychological theory, as we illustrate below.
2. What processes operate on the stored information?
A proper theory must articulate the processes that create and operate on representations. Candidate processes include encoding and maintenance operations, rehearsal, shifts of attention from one part of the representation to another, and retrieval mechanisms. Some of these processes are often classified as executive functions.
3. What causes forgetting?
A complete theory of STM must account for the facts of forgetting. Traditionally, the two leading contending accounts of forgetting have relied on the concepts of decay and interference. We review the behavioral and neurophysiological evidence that has traditionally been brought to the table to distinguish decay and interference accounts, and we suggest a possible mechanism for short-term forgetting.
Most models of STM fall between two extremes: Multistore models view STM and LTM as architecturally separate systems that rely on distinct representations. By contrast, according to unitary-store models, STM and LTM rely largely on the same representations, but differ by ( a ) the level of activation of these representations and ( b ) some of the processes that normally act upon them. We focus on the distinctions drawn by these theories as we examine the evidence concerning the three questions that motivate our review. In this discussion, we assume that a representation in memory consists of a bundle of features that define a memorandum, including the context in which that memorandum was encountered.
WHAT IS THE STRUCTURE OF SHORT-TERM MEMORY?
Multistore models that differentiate short- and long-term memory.
In his Principles of Psychology , William James (1890) articulated the view that short-term (“primary”) memory is qualitatively different from long-term (“secondary”) memory (see also Hebb 1949 ). The most influential successor to this view is the model of STM developed by Baddeley and colleagues (e.g., Baddeley 1986 , 1992 ; Baddeley & Hitch 1974 ; Repov & Baddeley 2006 ). For the years 1980 to 2006, of the 16,154 papers that cited “working memory” in their titles or abstracts, fully 7339 included citations to Alan Baddeley.
According to Baddeley’s model, there are separate buffers for different forms of information. These buffers, in turn, are separate from LTM. A verbal buffer, the phonological loop, is assumed to hold information that can be rehearsed verbally (e.g., letters, digits). A visuospatial sketchpad is assumed to maintain visual information and can be further fractionated into visual/object and spatial stores ( Repov & Baddeley 2006 , Smith et al. 1995 ). An episodic buffer that draws on the other buffers and LTM has been added to account for the retention of multimodal information ( Baddeley 2000 ). In addition to the storage buffers described above, a central executive is proposed to organize the interplay between the various buffers and LTM and is implicated in controlled processing.
In short, the multistore model includes several distinctions: ( a ) STM is distinct from LTM, ( b ) STM can be stratified into different informational buffers based on information type, and ( c ) storage and executive processes are distinguishable. Evidence in support of these claims has relied on behavioral interference studies, neuropsychological studies, and neuroimaging data.
Evidence for the distinction between short- and long-term memory
Studies of brain-injured patients who show a deficit in STM but not LTM or vice versa lead to the implication that STM and LTM are separate systems. 1 Patients with parietal and temporal lobe damage show impaired short-term phonological capabilities but intact LTM( Shallice & Warrington 1970 , Vallar & Papagno 2002 ). Conversely, it is often claimed that patients with medial temporal lobe (MTL) damage demonstrate impaired LTM but preserved STM (e.g., Baddeley & Warrington 1970 , Scoville & Milner 1957 ; we reinterpret these effects below).
Neuroimaging data from healthy subjects have yielded mixed results, however. A meta-analysis comparing regions activated during verbal LTM and STM tasks indicated a great deal of overlap in neural activation for the tasks in the frontal and parietal lobes ( Cabeza et al. 2002 , Cabeza & Nyberg 2000 ). Three studies that directly compared LTM and STM in the same subjects did reveal some regions selective for each memory system ( Braver et al. 2001 , Cabeza et al. 2002 , Talmi et al. 2005 ). Yet, of these studies, only one found that the MTL was uniquely activated for LTM ( Talmi et al. 2005 ). What might account for the discrepancy between the neuropsychological and neuroimaging data?
One possibility is that neuroimaging tasks of STM often use longer retention intervals than those employed for neuropsychological tasks, making the STM tasks more similar to LTM tasks. In fact, several studies have shown that the MTL is important when retention intervals are longer than a few seconds ( Buffalo et al. 1998 , Cabeza et al. 2002 , Holdstock et al. 1995 , Owen et al. 1995 ). Of the studies that compared STM and LTM in the same subjects, only Talmi et al. (2005) used an STM retention interval shorter than five seconds. This study did find, in fact, that the MTL was uniquely recruited at longer retention intervals, providing support for the earlier neuropsychological work dissociating long- and short-term memory. As we elaborate below, however, there are other possible interpretations, especially with regard to the MTL’s role in memory.
Evidence for separate buffers in short-term memory
The idea that STM can be parceled into information-specific buffers first received support from a series of studies of selective interference (e.g., Brooks 1968 , den Heyer & Barrett 1971 ). These studies relied on the logic that if two tasks use the same processing mechanisms, they should show interfering effects on one another if performed concurrently. This work showed a double dissociation: Verbal tasks interfered with verbal STM but not visual STM, and visual tasks interfered with visual STM but not verbal STM, lending support to the idea of separable memory systems (for reviews, see Baddeley 1986 and Baddeley & Hitch 1974 ).
The advent of neuroimaging has allowed researchers to investigate the neural correlates of the reputed separability of STM buffers. Verbal STM has been shown to rely primarily on left inferior frontal and left parietal cortices, spatial STM on right posterior dorsal frontal and right parietal cortices, and object/visual STM on left inferior frontal, left parietal, and left inferior temporal cortices (e.g., Awh et al. 1996 , Jonides et al. 1993 , Smith & Jonides 1997 ; see review by Wager & Smith 2003 ). Verbal STM shows a marked left hemisphere preference, whereas spatial and object STM can be distinguished mainly by a dorsal versus ventral separation in posterior cortices (consistent with Ungerleider & Haxby 1994 ; see Baddeley 2003 for an account of the function of these regions in the service of STM).
The more recently postulated episodic buffer arose from the need to account for interactions between STM buffers and LTM. For example, the number of words recalled in an STM experiment can be greatly increased if the words form a sentence ( Baddeley et al. 1987 ). This “chunking” together of words to increase short-term capacity relies on additional information from LTM that can be used to integrate the words ( Baddeley 2000 ). Thus, there must be some representational space that allows for the integration of information stored in the phonological loop and LTM. This ability to integrate information from STM and LTM is relatively preserved even when one of these memory systems is damaged ( Baddeley & Wilson 2002 , Baddeley et al. 1987 ). These data provide support for an episodic buffer that is separable from other short-term buffers and from LTM ( Baddeley 2000 , Baddeley & Wilson 2002 ). Although neural evidence about the possible localization of this buffer is thin, there is some suggestion that dorsolateral prefrontal cortex plays a role ( Prabhakaran et al. 2000 , Zhang et al. 2004 ).
Evidence for separate storage and executive processes
Baddeley’s multistore model assumes that a collection of processes act upon the information stored in the various buffers. Jointly termed the “central executive,” these processes are assumed to be separate from the storage buffers and have been associated with the frontal lobes.
Both lesion and neuroimaging data support the distinction between storage and executive processes. For example, patients with frontal damage have intact STM under conditions of low distraction ( D’Esposito & Postle 1999 , 2000 ; Malmo 1942 ). However, when distraction is inserted during a delay interval, thereby requiring the need for executive processes to overcome interference, patients with frontal damage show significant memory deficits ( D’Esposito & Postle 1999 , 2000 ). By contrast, patients with left temporo-parietal damage show deficits in phonological storage, regardless of the effects of interference ( Vallar & Baddeley 1984 , Vallar & Papagno 2002 ).
Consistent with these patterns, a meta-analysis of 60 functional neuroimaging studies indicated that increased demand for executive processing recruits dorsolateral frontal cortex and posterior parietal cortex ( Wager & Smith 2003 ). By contrast, storage processes recruit predominately posterior areas in primary and secondary association cortex. These results corroborate the evidence from lesion studies and support the distinction between storage and executive processing.
Unitary-Store Models that Combine Short-Term and Long-Term Memory
The multistore models reviewed above combine assumptions about the distinction between short-term and long-term systems, the decomposition of short-term memory into information-specific buffers, and the separation of systems of storage from executive functions. We now consider unitary models that reject the first assumption concerning distinct systems.
Contesting the idea of separate long-term and short-term systems
The key data supporting separable short-term and long-term systems come from neuropsychology. To review, the critical contrast is between patients who show severely impaired LTM with apparently normal STM (e.g., Cave & Squire 1992 , Scoville & Milner 1957 ) and those who show impaired STM with apparently normal LTM (e.g., Shallice & Warrington 1970 ). However, questions have been raised about whether these neuropsychological studies do, in fact, support the claim that STM and LTM are separable. A central question is the role of the medial temporal lobe. It is well established that the MTL is critical for long-term declarative memory formation and retrieval ( Gabrieli et al. 1997 , Squire 1992 ). However, is the MTL also engaged by STM tasks? Much research with amnesic patients showing preserved STM would suggest not, but Ranganath & Blumenfeld (2005) have summarized evidence showing that MTL is engaged in short-term tasks (see also Ranganath & D’Esposito 2005 and Nichols et al. 2006 ).
In particular, there is growing evidence that a critical function of the MTL is to establish representations that involve novel relations. These relations may be among features or items, or between items and their context. By this view, episodic memory is a special case of such relations (e.g., relating a list of words to the experimental context in which the list was recently presented), and the special role of the MTL concerns its binding capabilities, not the timescale on which it operates. STM that is apparently preserved in amnesic patients may thus reflect a preserved ability to maintain and retrieve information that does not require novel relations or binding, in keeping with their preserved retrieval of remote memories consolidated before the amnesia-inducing lesion.
If this view is correct, then amnesic patients should show deficits in situations that require STM for novel relations, which they do (Hannula et al. 2005, Olson et al. 2006b ). They also show STM deficits for novel materials (e.g., Buffalo et al. 1998 , Holdstock et al. 1995 , Olson et al. 1995, 2006a ). As mentioned above, electrophysiological and neuroimaging studies support the claim that the MTL is active in support of short-term memories (e.g., Miyashita & Chang 1968 , Ranganath & D’Esposito 2001 ). Taken together, the MTL appears to operate in both STM and LTM to create novel representations, including novel bindings of items to context.
Additional evidence for the STM-LTM distinction comes from patients with perisylvian cortical lesions who are often claimed to have selective deficits in STM (e.g., Hanley et al. 1991 , Warrington & Shallice 1969 ). However, these deficits may be substantially perceptual. For example, patients with left perisylvian damage that results in STM deficits also have deficits in phonological processing in general, which suggests a deficit that extends beyond STM per se (e.g., Martin 1993 ).
The architecture of unitary-store models
Our review leads to the conclusion that short- and long-term memory are not architecturally separable systems—at least not in the strong sense of distinct underlying neural systems. Instead, the evidence points to a model in which short-term memories consist of temporary activations of long-term representations. Such unitary models of memory have a long history in cognitive psychology, with early theoretical unification achieved via interference theory ( Postman 1961 , Underwood & Schultz 1960). Empirical support came from demonstrations that memories in both the short and long term suffered from proactive interference (e.g., Keppel & Underwood 1962 ).
Perhaps the first formal proposal that short-term memory consists of activated long-term representations was by Atkinson & Shiffrin (1971 , but also see Hebb 1949) . The idea fell somewhat out of favor during the hegemony of the Baddeley multistore model, although it was given its first detailed computational treatment by Anderson (1983) . It has recently been revived and greatly developed by Cowan (1988 , 1995 , 2000) , McElree (2001) , Oberauer (2002) , Verhaeghen et al. (2004) , Anderson et al. (2004) , and others. The key assumption is the construct of a very limited focus of attention, although as we elaborate below, there are disagreements regarding the scope of the focus.
One shared assumption of these models is that STM consists of temporary activations of LTM representations or of representations of items that were recently perceived. The models differ from one to another regarding specifics, but Cowan’s model (e.g., Cowan 2000 ) is representative. According to this model, there is only one set of representations of familiar material—the representations in LTM. These representations can vary in strength of activation, where that strength varies as a function of such variables as recency and frequency of occurrence. Representations that have increased strength of activation are more available for retrieval in STM experiments, but they must be retrieved nonetheless to participate in cognitive action. In addition, these representations are subject to forgetting over time. A special but limited set of these representations, however, can be within the focus of attention, where being within the focus makes these representations immediately available for cognitive processing. According to this and similar models, then, STM is functionally seen as consisting of LTM representations that are either in the focus of attention or at a heightened level of activation.
These unitary-store models suggest a different interpretation of frontal cortical involvement in STM from multistore models. Early work showing the importance of frontal cortex for STM, particularly that of Fuster and Goldman-Rakic and colleagues, was first seen as support for multistore models (e.g., Funahashi et al. 1989 , Fuster 1973 , Jacobsen 1936 , Wilson et al. 1993 ). For example, single-unit activity in dorsolateral prefrontal cortex regions (principal sulcus, inferior convexity) that was selectively responsive to memoranda during the delay interval was interpreted as evidence that these regions were the storage sites for STM. However, the sustained activation of frontal cortex during the delay period does not necessarily mean that this region is a site of STM storage. Many other regions of neo-cortex also show activation that outlasts the physical presence of a stimulus and provides a possible neural basis for STM representations (see Postle 2006 ). Furthermore, increasing evidence suggests that frontal activations reflect the operation of executive processes [including those needed to keep the representations in the focus of attention; see reviews by Postle (2006) , Ranganath & D’Esposito (2005) , Reuter-Lorenz & Jonides (2007) , and Ruchkin et al. (2003) ]. Modeling work and lesion data provide further support for the idea that the representations used in both STM and LTM are stored in those regions of cortex that are involved in initial perception and encoding, and that frontal activations reflect processes involved in selecting this information for the focus of attention and keeping it there ( Damasio 1989 , McClelland et al. 1995 ).
The principle of posterior storage also allows some degree of reconciliation between multi- and unitary-store models. Posterior regions are clearly differentiated by information type (e.g., auditory, visual, spatial), which could support the information-specific buffers postulated by multistore models. Unitary-store models focus on central capacity limits, irrespective of modality, but they do allow for separate resources ( Cowan 2000 ) or feature components ( Lange & Oberauer 2005 , Oberauer & Kliegl 2006 ) that occur at lower levels of perception and representation. Multi- and unitary-store models thus both converge on the idea of modality-specific representations (or components of those representations) supported by distinct posterior neural systems.
Controversies over Capacity
Regardless of whether one subscribes to multi- or unitary-store models, the issue of how much information is stored in STM has long been a prominent one ( Miller 1956 ). Multistore models explain capacity estimates largely as interplay between the speed with which information can be rehearsed and the speed with which information is forgotten ( Baddeley 1986 , 1992 ; Repov & Baddeley 2006 ). Several studies have measured this limit by demonstrating that approximately two seconds worth of verbal information can be re-circulated successfully (e.g., Baddeley et al. 1975 ).
Unitary-store models describe capacity as limited by the number of items that can be activated in LTM, which can be thought of as the bandwidth of attention. However, these models differ on what that number or bandwidth might be. Cowan (2000) suggested a limit of approximately four items, based on performance discontinuities such as errorless performance in immediate recall when the number of items is less than four, and sharp increases in errors for larger numbers. (By this view, the classic “seven plus or minus two” is an overestimate because it is based on studies that allowed participants to engage in processes of rehearsal and chunking, and reflected contributions of both the focus and LTM; see also Waugh & Norman 1965 .) At the other extreme are experimental paradigms suggesting that the focus of attention consists of a single item ( Garavan 1998 , McElree 2001 , Verhaeghen & Basak 2007 ). We briefly consider some of the central issues behind current controversies concerning capacity estimates.
Behavioral and neural evidence for the magic number 4
Cowan (2000) has reviewed an impressive array of studies leading to his conclusion that the capacity limit is four items, plus or minus one (see his Table 1). Early behavioral evidence came from studies showing sharp drop-offs in performance at three or four items on short-term retrieval tasks (e.g., Sperling 1960 ). These experiments were vulnerable to the criticism that this limit might reflect output interference occurring during retrieval rather than an actual limit on capacity. However, additional evidence comes from change-detection and other tasks that do not require the serial recall of individual items. For example, Luck & Vogel (1997) presented subjects with 1 to 12 colored squares in an array. After a blank interval of nearly a second, another array of squares was presented, in which one square may have changed color. Subjects were to respond whether the arrays were identical. These experiments and others that avoid the confound of output-interference (e.g., Pashler 1988 ) likewise have yielded capacity estimates of approximately four items.
Electrophysiological and neuroimaging studies also support the idea of a four-item capacity limit. The first such report was by Vogel & Machizawa (2004) , who recorded event-related potentials (ERPs) from subjects as they performed a visual change-detection task. ERP recording shortly after the onset of the retention interval in this task indicated a negative-going wave over parietal and occipital sites that persisted for the duration of the retention interval and was sensitive to the number of items held in memory. Importantly, this signal plateaued when array size reached between three and four items. The amplitude of this activity was strongly correlated with estimates of each subject’s memory capacity and was less pronounced on incorrect than correct trials, indicating that it was causally related to performance. Subsequent functional magnetic resonance imaging (fMRI) studies have observed similar load- and accuracy-dependent activations, especially in intraparietal and intraoccipital sulci ( Todd & Marois 2004 , 2005 ). These regions have been implicated by others (e.g., Yantis & Serences 2003 ) in the control of attentional allocation, so it seems plausible that one rate-limiting step in STM capacity has to do with the allocation of attention ( Cowan 2000 ; McElree 1998 , 2001 ; Oberauer 2002 ).
Evidence for more severe limits on focus capacity
Another set of researchers agree there is a fixed capacity, but by measuring a combination of response time and accuracy, they contend that the focus of attention is limited to just one item (e.g., Garavan 1998 , McElree 2001 , Verhaeghen & Basak 2007 ). For example, Garavan (1998) required subjects to keep two running counts in STM, one for triangles and one for squares—as shape stimuli appeared one after another in random order. Subjects controlled their own presentation rate, which allowed Garavan to measure the time spent processing each figure before moving on. He found that responses to a figure of one category (e.g., a triangle) that followed a figure from the other category (e.g., a square) were fully 500 milliseconds longer than responses to the second of two figures from the same category (e.g., a triangle followed by another triangle). These findings suggested that attention can be focused on only one internal counter in STM at a time. Switching attention from one counter to another incurred a substantial cost in time. Using a speed-accuracy tradeoff procedure, McElree (1998) came to the same conclusion that the focus of attention contained just one item. He found that the retrieval speed for the last item in a list was substantially faster than for any other item in the list, and that other items were retrieved at comparable rates to each other even though the accuracy of retrieval for these other items varied.
Oberauer (2002) suggested a compromise solution to the “one versus four” debate. In his model, up to four items can be directly accessible, but only one of these items can be in the focus of attention. This model is similar to that of Cowan (2000) , but adds the assumption that an important method of accessing short-term memories is to focus attention on one item, depending on task demands. Thus, in tasks that serially demand attention on several items (such as those of Garavan 1998 or McElree 2001 ), the mechanism that accomplishes this involves changes in the focus of attention among temporarily activated representations in LTM.
Alternatives to capacity limits based on number of items
Attempting to answer the question of how many items may be held in the focus implicitly assumes that items are the appropriate unit for expressing capacity limits. Some reject this basic assumption. For example, Wilken & Ma (2004) demonstrated that a signal-detection account of STM, in which STM capacity is primarily constrained by noise, better fit behavioral data than an item-based fixed-capacity model. Recent data from change-detection tasks suggest that object complexity ( Eng et al. 2005 ) and similarity ( Awh et al. 2007 ) play an important role in determining capacity. Xu & Chun (2006) offer neuroimaging evidence that may reconcile the item-based and complexity accounts: In a change-detection task, they found that activation of inferior intra-parietal sulcus tracked a capacity limit of four, but nearby regions were sensitive to the complexity of the memoranda, as were the behavioral results.
Other researchers disagree with fixed item-based limits because they have demonstrated that the limit is mutable. Practice may improve subjects’ ability to use processes such as chunking to allow greater functional capacities ( McElree 1998 , Verhaeghen et al. 2004 ; but see Oberauer 2006 ). However, this type of flexibility appears to alter the amount of information that can be compacted into a single representation rather than the total number of representations that can be held in STM ( Miller 1956 ). The data of Verhaegen et al. (2004; see Figure 5 of that paper) suggest that the latter number still approximates four, consistent with Cowan’s claims.
Building on these findings, we suggest a new view of capacity. The fundamental idea that attention can be allocated to one piece of information in memory is correct, but the definition of what that one piece is needs to be clarified. It cannot be that just one item is in the focus of attention because if that were so, hardly any computation would be possible. How could one add 3+4, for example, if at any one time, attention could be allocated only to the “3” or the “4” or the “+” operation? We propose that attention focuses on what is bound together into a single “functional context,” whether that context is defined by time, space, some other stimulus characteristic such as semantic or visual similarity or momentary task relevance. By this account, attention can be placed on the whole problem “3+4,” allowing relevant computations to be made. Complexity comes into play by limiting the number of subcomponents that can be bound into one functional context.
What are we to conclude from the data concerning the structure of STM? We favor the implication that the representational bases for perception, STM, and LTM are identical. That is, the same neural representations initially activated during the encoding of a piece of information show sustained activation during STM (or retrieval from LTM into STM; Wheeler et al. 2000 ) and are the repository of long-term representations. Because regions of neocortex represent different sorts of information (e.g., verbal, spatial), it is reasonable to expect that STM will have an organization by type of material as well. Functionally, memory in the short term seems to consist of items in the focus of attention along with recently attended representations in LTM. These items in the focus of attention number no more than four, and they may be limited to just a single representation (consisting of items bound within a functional context).
We turn below to processes that operate on these representations.
WHAT PROCESSES OPERATE ON THE STORED INFORMATION?
Theoretical debate about the nature of STM has been dominated by discussion of structure and capacity, but the issue of process is also important. Verbal rehearsal is perhaps most intuitively associated with STM and plays a key role in the classic model ( Baddeley 1986 ). However, as we discuss below, rehearsal most likely reflects a complex strategy rather than a primitive STM process. Modern approaches offer a large set of candidate processes, including encoding and maintenance ( Ranganath et al. 2004 ), attention shifts ( Cowan 2000 ), spatial rehearsal ( Awh & Jonides 2001 ), updating (Oberauer 2005), overwriting ( Neath & Nairne 1995 ), cue-based parallel retrieval ( McElree 2001 ), and interference-resolution ( Jonides & Nee 2006 ).
Rather than navigating this complex and growing list, we take as our cornerstone the concept of a limited focus of attention. The central point of agreement for the unitary-store models discussed above is that there is a distinguishable focus of attention in which representations are directly accessible and available for cognitive action. Therefore, it is critical that all models must identify the processes that govern the transition of memory representations into and out of this focused state.
The Three Core Processes of Short-Term Memory: Encoding, Maintenance, and Retrieval
If one adopts the view that a limited focus of attention is a key feature of short-term storage, then understanding processing related to this limited focus amounts to understanding three basic types of cognitive events 2 : ( a ) encoding processes that govern the transformation from perceptual representations into the cognitive/attentional focus, ( b ) maintenance processes that keep information in the focus (and protect it from interference or decay), and ( c ) retrieval processes that bring information from the past back into the cognitive focus (possibly reactivating perceptual representations).
Encoding of items into the focus
Encoding processes are the traditional domain of theories of perception and are not treated explicitly in any of the current major accounts of STM. Here we outline three implicit assumptions about encoding processes made in most accounts of STM, and we assess their empirical and theoretical support.
First, the cognitive focus is assumed to have immediate access to perceptual processing— that is, the focus may include contents from the immediate present as well as contents retrieved from the immediate past. In Cowan’s (2000) review of evidence in favor of the number four in capacity estimates, several of the experimental paradigms involve focused representations of objects in the immediate perceptual present or objects presented less than a second ago. These include visual tracking experiments ( Pylyshyn et al. 1994 ), enumeration ( Trick & Pylyshyn 1993 ), and whole report of spatial arrays and spatiotemporal arrays ( Darwin et al. 1972 , Sperling 1960 ). Similarly, in McElree’s (2006) and Garavan’s (1998) experiments, each incoming item in the stream of material (words or letters or objects) is assumed to be represented momentarily in the focus.
Second, all of the current theories assume that perceptual encoding into the focus of attention results in a displacement of other items from the focus. For example, in McElree’s single-item focus model, each incoming item not only has its turn in the focus, but it also replaces the previous item. On the one hand, the work reviewed above regarding performance discontinuities after the putative limit of STM capacity has been reached appears to support the idea of whole-item displacement. On the other hand, as also described above, this limit may be susceptible to factors such as practice and stimulus complexity. An alternative to whole-item displacement as the basis for interference is a graded similarity-based interference, in which new items entering the focus may partially overwrite features of the old items or compete with old items to include those featural components in their representations as a function of their similarity. At some level, graded interference is clearly at work in STM, as Nairne (2002) and others have demonstrated (we review this evidence in more detail below). But the issue at hand is whether the focus is subject to such graded interference, and if such interference is the process by which encoding (or retrieving) items into the focus displaces prior items. Although there does not appear to be evidence that bears directly on this issue (the required experiments would involve manipulations of similarity in just the kinds of paradigms that Cowan, McElree, Oberauer, and others have used to provide evidence for the limited focus), the performance discontinuities strongly suggest that something like displacement is at work.
Third, all of the accounts assume that perceptual encoding does not have obligatory access to the focus. Instead, encoding into the focus is modulated by attention. This follows rather directly from the assumptions about the severe limits on focus capacity: There must be some controlled way of directing which aspects of the perceptual present, as well as the cognitive past, enter into the focused state. Stated negatively, there must be some way of preventing aspects of the perceptual present from automatically entering into the focused state. Postle (2006) recently found that increased activity in dorsolateral prefrontal cortex during the presentation of distraction during a retention interval was accompanied by a selective decrease in inferior temporal cortical activity. This pattern suggests that prefrontal regions selectively modulated posterior perceptual areas to prevent incoming sensory input from disrupting the trace of the task-relevant memorandum.
In summary, current approaches to STM have an obligation to account for how controlled processes bring relevant aspects of perception into cognitive focus and leave others out. It is by no means certain that existing STM models and existing models of perceptual attention are entirely compatible on this issue, and this is a matter of continued lively debate ( Milner 2001 , Schubert & Frensch 2001 , Woodman et al. 2001 ).
Maintenance of items in the focus
Once an item is in the focus of attention, what keeps it there? If the item is in the perceptual present, the answer is clear: attention-modulated, perceptual encoding. The more pressing question is: What keeps something in the cognitive focus when it is not currently perceived? For many neuroscientists, this is the central question of STM—how information is held in mind for the purpose of future action after the perceptual input is gone. There is now considerable evidence from primate models and from imaging studies on humans for a process of active maintenance that keeps representations alive and protects them from irrelevant incoming stimuli or intruding thoughts (e.g., Postle 2006 ).
We argue that this process of maintenance is not the same as rehearsal. Indeed, the number of items that can be maintained without rehearsal forms the basis of Cowan’s (2000) model. Under this view, rehearsal is not a basic process but rather is a strategy for accomplishing the functional demands for sustaining memories in the short term—a strategy composed of a series of retrievals and re-encodings. We consider rehearsal in more detail below, but we consider here the behavioral and neuroimaging evidence for maintenance processes.
There is now considerable evidence from both primate models and human electroencephalography and fMRI studies for a set of prefrontal-posterior circuits underlying active maintenance. Perhaps the most striking is the classic evidence from single-cell recordings showing that some neurons in prefrontal cortex fire selectively during the delay period in delayed-match-to-sample tasks (e.g., Funahashi et al. 1989 , Fuster 1973 ). As mentioned above, early interpretations of these frontal activations linked them directly to STM representations ( Goldman-Rakic 1987 ), but more recent theories suggest they are part of a frontal-posterior STM circuit that maintains representations in posterior areas ( Pasternak & Greenlee 2005 , Ranganath 2006 , Ruchkin et al. 2003 ). Furthermore, as described above, maintenance operations may modulate perceptual encoding to prevent incoming perceptual stimuli from disrupting the focused representation in posterior cortex ( Postle 2006 ). Several computational neural-network models of circuits for maintenance hypothesize that prefrontal cortical circuits support attractors, self-sustaining patterns observed in certain classes of recurrent networks ( Hopfield 1982 , Rougier et al. 2005 , Polk et al. 2002 ). A major challenge is to develop computational models that are able to engage in active maintenance of representations in posterior cortex while simultaneously processing, to some degree, incoming perceptual material (see Renart et al. 1999 for a related attempt).
Retrieval of items into the focus
Many of the major existing STM architectures are silent on the issue of retrieval. However, all models that assume a limited focus also assume that there is some means by which items outside that focus (either in a dormant long-term store or in some highly activated portion of LTM) are brought into the focus by switching the attentional focus onto those items. Following Sternberg (1966) , McElree (2006) , and others, we label this process “retrieval.” Despite this label, it is important to keep in mind that the associated spatial metaphor of an item moving from one location to another is misleading given our assumption about the common neural representations underlying STM and LTM.
There is now considerable evidence, mostly from mathematical models of behavioral data, that STM retrieval of item information is a rapid, parallel, content-addressable process. The current emphasis on parallel search processes is quite different from the earliest models of STM retrieval, which postulated a serial scanning process (i.e., Sternberg 1966 ; see McElree 2006 for a recent review and critique). Serial-scanning models fell out of favor because of empirical and modeling work showing that parallel processes provide a better account of the reaction time distributions in STM tasks (e.g., Hockley 1984 ). For example, McElree has created a variation on the Sternberg recognition probe task that provides direct support for parallel, rather than serial, retrieval. In the standard version of the task, participants are presented with a memory set consisting of a rapid sequence of verbal items (e.g., letters or digits), followed by a probe item. The task is to identify whether the probe was a member of the memory set. McElree & Dosher’s (1989) innovation was to manipulate the deadline for responding. The time course of retrieval (accuracy as a function of response deadline) can be separately plotted for each position within the presentation sequence, allowing independent assessments of accessibility (how fast an item can be retrieved) and availability (asymptotic accuracy) as a function of set size and serial position. Many experiments yield a uniform rate of access for all items except for the most recent item, which is accessed more quickly. The uniformity of access rate is evidence for parallel access, and the distinction between the most recent item and the other items is evidence for a distinguished focus of attention.
Neural Mechanisms of Short- and Long-Term Memory Retrieval
The cue-based retrieval processes described above for STM are very similar to those posited for LTM (e.g., Anderson et al. 2004 , Gillund & Shiffrin 1984 , Murdock 1982 ). As a result, retrieval failures resulting from similarity-based interference and cue overlap are ubiquitous in both STM and LTM. Both classic studies of recall from STM (e.g., Keppel & Underwood 1962 ) and more recent studies of interference in probe-recognition tasks (e.g., Jonides & Nee 2006 , McElree & Dosher 1989 , Monsell 1978 ) support the idea that interference plays a major role in forgetting over short retention intervals as well as long ones (see below). These common effects would not be expected if STM retrieval were a different process restricted to operate over a limited buffer, but they are consistent with the notion that short-term and long-term retrieval are mediated by the same cue-based mechanisms.
The heavy overlap in the neural substrates for short-term and long-term retrieval provides additional support for the idea that retrieval processes are largely the same over different retention intervals. A network of medial temporal regions, lateral prefrontal regions, and anterior prefrontal regions has been extensively studied and shown to be active in long-term retrieval tasks (e.g., Buckner et al. 1998 , Cabeza & Nyberg 2000 , Fletcher & Henson 2001 ). We reviewed above the evidence for MTL involvement in both short- and long-term memory tasks that require novel representations (see section titled “Contesting the Idea of Separate Long-Term and Short-Term Systems”). Here, we examine whether the role of frontal cortex is the same for both short- and long-term retrieval.
The conclusion derived from neuroimaging studies of various different STM procedures is that this frontal role is the same in short-term and long-term retrieval. For example, several event-related fMRI studies of the retrieval stage of the probe-recognition task found increased activation in lateral prefrontal cortex similar to the activations seen in studies of LTM retrieval (e.g., D’Esposito et al. 1999 , D’Esposito & Postle 2000 , Manoach et al. 2003 ). Badre & Wagner (2005) also found anterior prefrontal activations that overlapped with regions implicated in episodic recollection. The relatively long retention intervals often used in event-related fMRI studies leaves them open to the criticism that by the time of the probe, the focus of attention has shifted elsewhere, causing the need to retrieve information from LTM (more on this discussion below). However, a meta-analysis of studies that involved bringing very recently presented items to the focus of attention likewise found specific involvement of lateral and anterior prefrontal cortex ( Johnson et al. 2005 ). These regions appear to be involved in retrieval, regardless of timescale.
The same conclusion may be drawn from recent imaging studies that have directly compared long- and short-term retrieval tasks using within-subjects designs ( Cabeza et al. 2002 , Ranganath et al. 2003 , Talmi et al. 2005 ). Ranganath et al. (2003) found the same bilateral ventrolateral and dorsolateral prefrontal regions engaged in both short- and long-term tasks. In some cases, STM and LTM tasks involve the same regions but differ in the relative amount of activation shown within those regions. For example, Cabeza et al. (2002) reported similar engagement of medial temporal regions in both types of task, but greater anterior and ventrolateral activation in the long-term episodic tasks. Talmi et al. (2005) reported greater activation in both medial temporal and lateral frontal cortices for recognition probes of items presented early in a 12-item list (presumably necessitating retrieval from LTM) versus items presented later in the list (presumably necessitating retrieval from STM). One possible reason for this discrepancy is that recognition for late-list items did not require retrieval because these items were still in the focus of attention. This account is plausible since late-list items were drawn either from the last-presented or second-to-last presented item and preceded the probe by less than two seconds.
In summary, the bulk of the neuroimaging evidence points to the conclusion that the activation of frontal and medial temporal regions depends on whether the information is currently in or out of focus, not whether the task nominally tests STM or LTM. Similar reactivation processes occur during retrieval from LTM and from STM when the active maintenance has been interrupted (see Sakai 2003 for a more extensive review).
The Relationship of Short-Term Memory Processes to Rehearsal
Notably, our account of core STM processes excludes rehearsal. How does rehearsal fit in? We argue that rehearsal is simply a controlled sequence of retrievals and re-encodings of items into the focus of attention ( Baddeley 1986 , Cowan 1995 ). The theoretical force of this assumption can be appreciated by examining the predictions it makes when coupled with our other assumptions about the structures and processes of the underlying STM architecture. Below we outline these predictions and the behavioral, developmental, neuroimaging, and computational work that support this view.
Rehearsal as retrieval into the focus
When coupled with the idea of a single-item focus, the assumption that rehearsal is a sequence of retrievals into the focus of attention makes a very clear prediction: A just-rehearsed item should display the same retrieval dynamics as a just-perceived item. McElree (2006) directly tested this prediction using a version of his response-deadline recognition task, in which subjects were given a retention interval between presentation of the list and the probe rather than presented with the probe immediately after the list. Subjects were explicitly instructed to rehearse the list during this interval and were trained to do so at a particular rate. By controlling the rate, it was possible to know when each item was rehearsed and hence re-established in the focus. The results were compelling: When an item was predicted to be in focus because it had just been rehearsed, it showed the same fast retrieval dynamics as an item that had just been perceived. In short, the speed-accuracy tradeoff functions showed the familiar in-focus/out-of-focus dichotomy of the standard paradigm, but the dichotomy was established for internally controlled rehearsal as well as externally controlled perception.
Rehearsal as strategic retrieval
Rehearsal is often implicitly assumed as a component of active maintenance, but formal theoretical considerations of STM typically take the opposite view. For example, Cowan (2000) provides evidence that although first-grade children do not use verbal rehearsal strategies, they nevertheless have measurable focus capacities. In fact, Cowan (2000) uses this evidence to argue that the performance of very young children is revealing of the fundamental capacity limits of the focus of attention because it is not confounded with rehearsal.
If rehearsal is the controlled composition of more primitive STM processes, then rehearsal should activate the same brain circuits as the primitive processes, possibly along with additional (frontal) circuits associated with their control. In other words, there should be overlap of rehearsal with brain areas sub-serving retrieval and initial perceptual encoding. Likewise, there should be control areas distinct from those of the primitive processes.
Both predictions receive support from neuroimaging studies. The first prediction is broadly confirmed: There is now considerable evidence for the reactivation of areas associated with initial perceptual encoding in tasks that require rehearsal (see Jonides et al. 2005 for a recent review; note also that evidence exists for reactivation in LTM retrieval: Wheeler 2000 , 2006 ).
The second prediction—that rehearsal engages additional control areas beyond those participating in maintenance, encoding, and retrieval—receives support from two effects. One is that verbal rehearsal engages a set of frontal structures associated with articulation and its planning: supplementary motor, premotor, inferior frontal, and posterior parietal areas (e.g., Chein & Fiez 2001, Jonides et al. 1998 , Smith & Jonides 1999 ). The other is that spatial rehearsal engages attentionally mediated occipital regions, suggesting rehearsal processes that include retrieval of spatial information ( Awh et al. 1998 , 1999 , 2001 ).
Computational modeling relevant to strategic retrieval
Finally, prominent symbolic and connectionist computational models of verbal STM tasks are based on architectures that do not include rehearsal as a primitive process, but rather assume it as a strategic composition of other processes operating over a limited focus. The Burgess & Hitch (2005 , 2006) connectionist model, the Executive-Process/Interactive Control (EPIC) symbolic model ( Meyer and Kieras 1997 ), and the Atomic Components of Thought (ACT-R) hybrid model ( Anderson & Matessa 1997 ) all assume that rehearsal in verbal STM consists of a controlled sequence of retrievals of items into a focused state. They all assume different underlying mechanisms for the focus (the Burgess & Hitch model has a winner-take-all network; ACT-R has an architectural buffer with a capacity of one chunk; EPIC has a special auditory store), but all assume strategic use of this focus to accomplish rehearsal. These models jointly represent the most successful attempts to account for a range of detailed empirical phenomena traditionally associated with rehearsal, especially in verbal serial recall tasks. Their success therefore provides further support for the plausibility of a compositional view of rehearsal.
WHY DO WE FORGET?
Forgetting in STM is a vexing problem: What accounts for failures to retrieve something encoded just seconds ago? There are two major explanations for forgetting, often placed in opposition: time-based decay and similarity-based interference. Below, we describe some of the major findings in the literature related to each of these explanations, and we suggest that they may ultimately result from the same underlying principles.
Decay Theories: Intuitive but Problematic
The central claim of decay theory is that as time passes, information in memory erodes, and so it is less available for later retrieval. This explanation has strong intuitive appeal. However, over the years there have been sharp critiques of decay, questioning whether it plays any role at all (for recent examples, see Lewandowsky et al. 2004 and the review in this journal by Nairne 2002 ).
Decay explanations are controversial for two reasons: First, experiments attempting to demonstrate decay can seldom eliminate alternative explanations. For example, Keppel & Underwood (1962) demonstrated that forgetting in the classic Brown-Peterson paradigm (designed to measure time-based decay) was due largely, if not exclusively, to proactive interference from prior trials. Second, without an explanation of how decay occurs, it is difficult to see decay theories as more than a restatement of the problem. Some functional arguments have been made for the usefulness of the notion of memory decay—that decaying activations adaptively mirror the likelihood that items will need to be retrieved ( Anderson & Schooler 1991 ), or that decay is functionally necessary to reduce interference ( Altmann & Gray 2002 ). Nevertheless, McGeoch’s famous (1932) criticism of decay theories still holds merit: Rust does not occur because of time itself, but rather from oxidation processes that occur with time. Decay theories must explain the processes by which decay could occur, i.e., they must identify the oxidation process in STM.
Retention-interval confounds: controlling for rehearsal and retroactive interference
The main problem in testing decay theories is controlling for what occurs during the retention interval. Many experiments include an attention-demanding task to prevent participants from using rehearsal that would presumably circumvent decay. However, a careful analysis of these studies by Roediger et al. (1977) makes one wonder whether the use of a secondary task is appropriate to prevent rehearsal at all. They compared conditions in which a retention interval was filled by nothing, by a relatively easy task, or by a relatively difficult one. Both conditions with a filled interval led to worse memory performance, but the difficulty of the intervening task had no effect. Roediger et al. (1977) concluded that the primary memory task and the interpolated task, although demanding, used different processing pools of resources, and hence the interpolated tasks may not have been effective in preventing rehearsal. So, they argued, this sort of secondary-task technique may not prevent rehearsal and may not allow for a convincing test of a decay hypothesis.
Another problem with tasks that fill the retention interval is that they require subjects to use STM (consider counting backward, as in the Brown-Peterson paradigm). This could lead to active displacement of items from the focus according to views (e.g., McElree 2001 ) that posit such displacement as a mechanism of STM forgetting, or increase the noise according to interference-based explanations (see discussion below in What Happens Neurally During the Delay?). By either account, the problem with retention-interval tasks is that they are questionable ways to prevent rehearsal of the to-be-remembered information, and they introduce new, distracting information that may engage STM. This double-edged sword makes it difficult to tie retention-interval manipulations directly to decay.
Attempts to address the confounding factors
A potential way out of the rehearsal conundrum is to use stimuli that are not easily converted to verbal codes and that therefore may be difficult to rehearse. For example, Harris (1952) used tones that differed so subtly in pitch that they would be difficult to name by subjects without perfect pitch. On each trial, participants were first presented with a to-be-remembered tone, followed by a retention interval of 0.1 to 25 seconds, and finally a probe tone. The accuracy of deciding whether the initial and probe tones were the same declined with longer retention intervals, consistent with the predictions of decay theory.
Using another technique, McKone (1995 , 1998) reduced the probability of rehearsal or other explicit-memory strategies by using an implicit task. Words and nonwords were repeated in a lexical-decision task, with the measure of memory being faster performance on repeated trials than on novel ones (priming). To disentangle the effects of decay and interference, McKone varied the time between repetitions (the decay-related variable) while holding the number of items between repetitions (the interference-related variable) constant, and vice versa. She found that greater time between repetitions reduced priming even after accounting for the effects of intervening items, consistent with decay theory. However, interference and decay effects seemed to interact and to be especially important for nonwords.
Procedures such as those used by Harris (1952) and McKone (1995 , 1998) do not have the problems associated with retention-interval tasks. They are, however, potentially vulnerable to the criticism of Keppel & Underwood (1962) regarding interference from prior trials within the task, although McKone’s experiments address this issue to some degree. Another potential problem is that these participants’ brains and minds are not inactive during the retention interval ( Raichle et al. 2001 ). There is increasing evidence that the processes ongoing during nominal “resting states” are related to memory, including STM ( Hampson et al. 2006 ). Spontaneous retrieval by participants during the retention interval could interfere with memory for the experimental items. So, although experiments that reduce the influence of rehearsal provide some of the best evidence of decay, they are not definitive.
What happens neurally during the delay?
Neural findings of delay-period activity have also been used to support the idea of decay. For example, at the single-cell level, Fuster (1995) found that in monkeys performing a delayed-response task, delay-period activity in inferotemporal cortex steadily declined over 18 seconds (see also Pasternak & Greenlee 2005 ). At a molar level, human neuroimaging studies often show delay-period activity in prefrontal and posterior regions, and this activity is often thought to support maintenance or storage (see review by Smith & Jonides 1999 ). As reviewed above, it is likely that the posterior regions support storage and that frontal regions support processes related to interference-resolution, control, attention, response preparation, motivation, and reward.
Consistent with the suggestive primate data, Jha & McCarthy (2000) found a general decline in activation in posterior regions over a delay period, which suggests some neural evidence for decay. However, this decline in activation was not obviously related to performance, which suggests two (not mutually exclusive) possibilities: ( a ) the decline in activation was not representative of decay, so it did not correlate with performance, or ( b ) these regions might not have been storage regions (but see Todd & Marois 2004 and Xu & Chun 2006 for evidence more supportive of load sensitivity in posterior regions).
The idea that neural activity decays also faces a serious challenge in the classic results of Malmo (1942) , who found that a monkey with frontal lesions was able to perform a delayed response task extremely well (97% correct) if visual stimulation and motor movement (and therefore associated interference) were restricted during a 10-second delay. By contrast, in unrestricted conditions, performance was as low as 25% correct (see also Postle & D’Esposito 1999 ). In summary, evidence for time-based declines in neural activity that would naturally be thought to be part of a decay process is at best mixed.
Is there a mechanism for decay?
Although there are data supporting the existence of decay, much of these data are subject to alternative, interference-based explanations. However, as Crowder (1976) noted, “Good ideas die hard.” At least a few key empirical results ( Harris 1952 ; McKone 1995 , 1998) do seem to implicate some kind of time-dependent decay. If one assumes that decay happens, how might it occur?
One possibility—perhaps most compatible with results like those of Malmo (1942) —is that what changes over time is not the integrity of the representation itself, but the likelihood that attention will be attracted away from it. As more time passes, the likelihood increases that attention will be attracted away from the target and toward external stimuli or other memories, and it will be more difficult to return to the target representation. This explanation seems compatible with the focus-of-attention views of STM that we have reviewed. By this explanation, capacity limits are a function of attention limits rather than a special property of STM per se.
Another explanation, perhaps complementary to the first, relies on stochastic variability in the neuronal firing patterns that make up the target representation. The temporal synchronization of neuronal activity is an important part of the representation (e.g., Deiber et al. 2007 , Jensen 2006 , Lisman & Idiart 1995 ). As time passes, variability in the firing rates of individual neurons may cause them to fall increasingly out of synchrony unless they are reset (e.g., by rehearsal). As the neurons fall out of synchrony, by this hypothesis, the firing pattern that makes up the representation becomes increasingly difficult to discriminate from surrounding noise [see Lustig et al. (2005) for an example that integrates neural findings with computational ( Frank et al. 2001 ) and behaviorally based ( Brown et al. 2000 ) models of STM].
Interference Theories: Comprehensive but Complex
Interference effects play several roles in memory theory: First, they are the dominant explanation of forgetting. Second, some have suggested that STM capacity and its variation among individuals are largely determined by the ability to overcome interference (e.g., Hasher & Zacks 1988 , Unsworth & Engle 2007 ). Finally, differential interference effects in STM and LTM have been used to justify the idea that they are separate systems, and common interference effects have been used to justify the idea that they are a unitary system.
Interference theory has the opposite problem of decay: It is comprehensive but complex ( Crowder 1976 ). The basic principles are straightforward. Items in memory compete, with the amount of interference determined by the similarity, number, and strength of the competitors. The complexity stems from the fact that interference may occur at multiple stages (encoding, retrieval, and possibly storage) and at multiple levels (the representation itself or its association with a cue or a response). Interference from the past (proactive interference; PI) may affect both the encoding and the retrieval of new items, and it often increases over time. By contrast, interference from new items onto older memories (retroactive interference; RI) frequently decreases over time and may not be as reliant on similarity (see discussion by Wixted 2004 ).
Below, we review some of the major findings with regard to interference in STM, including a discussion of its weaknesses in explaining short-term forgetting. We then present a conceptual model of STM that attempts to address these weaknesses and the questions regarding structure, process, and forgetting raised throughout this review.
Interference Effects in Short-Term Memory
Selection-based interference effects.
The Brown-Peterson task, originally conceived to test decay theory, became a workhorse for testing similarity-based interference as well. In the “release-from-PI” version ( Wickens 1970 ), short lists of categorized words are used as memoranda. Participants learn one three-item list on each trial, perform some other task during the retention interval, and then attempt to recall the list. For the first three trials, all lists consist of words from the same category (e.g., flowers). The typical PI effects occur: Recall declines over subsequent trials. The critical manipulation occurs at the final list. If it is from a different category (e.g., sports), recall is much higher than if it is from the same category as preceding trials. In some cases, performance on this set-shift or release from-PI trial is nearly as high as on the very first trial.
The release-from-PI effect was originally interpreted as an encoding effect. Even very subtle shifts (e.g., from “flowers” to “wild-flowers”) produce the effect if participants are warned about the shift before the words are presented (see Wickens 1970 for an explanation). However, Gardiner et al. (1972) showed that release also occurs if the shift-cue is presented only at the time of the retrieval test—i.e., after the list has been encoded. They suggested that cues at retrieval could reduce PI by differentiating items from the most recent list, thus aiding their selection.
Selection processes remain an important topic in interference research. Functional neuroimaging studies consistently identify a region in left inferior frontal gyrus (LIFG) as active during interference resolution, at least for verbal materials (see a review by Jonides & Nee 2006 ). This region appears to be generally important for selection among competing alternatives, e.g., in semantic memory as well as in STM ( Thompson-Schill et al. 1997 ). In STM, LIFG is most prominent during the test phase of interference trials, and its activation during this phase often correlates with behavioral measures of interference resolution ( D’Esposito et al. 1999 , Jonides et al. 1998 , Reuter-Lorenz et al. 2000 , Thompson-Schill et al. 2002 ). These findings attest to the importance of processes for resolving retrieval interference. The commonality of the neural substrate for interference resolution across short-term and long-term tasks provides yet further support for the hypothesis of shared retrieval processes for the two types of memory.
Interference effects occur at multiple levels, and it is important to distinguish between interference at the level of representations and interference at the level of responses. The LIFG effects described above appear to be familiarity based and to occur at the level of representations. Items on a current trial must be distinguished and selected from among items on previous trials that are familiar because of prior exposure but are currently incorrect. A separate contribution occurs at the level of responses: An item associated with a positive response on a prior trial may now be associated with a negative response, or vice versa. This response-based conflict can be separated from the familiarity-based conflict, and its resolution appears to rely more on the anterior cingulate ( Nelson et al. 2003 ).
Other mechanisms for interference effects?
Despite the early work of Keppel & Underwood (1962) , most studies examining encoding in STM have focused on RI: how new information disrupts previous memories. Early theorists described this disruption in terms of displacement of entire items from STM, perhaps by disrupting consolidation (e.g., Waugh & Norman 1965 ). However, rapid serial visual presentation studies suggest that this type of consolidation is complete within a very short time—approximately 500 milliseconds, and in some situations as short as 50 milliseconds ( Vogel et al. 2006 ).
What about interference effects beyond this time window? As reviewed above, most current focus-based models implicitly assume something like whole-item displacement is at work, but these models may need to be elaborated to account for retroactive similarity-based interference, such as the phonological interference effects reviewed by Nairne (2002) . The models of Nairne (2002) and Oberauer (2006) suggest a direction for such an elaboration. Rather than a competition at the item level for a single-focus resource, these models posit a lower-level similarity-based competition for “feature units.” By this idea, items in STM are represented as bundles of features (e.g., color, shape, spatial location, temporal location). Representations of these features in turn are distributed over multiple units. The more two items overlap, the more they compete for these feature units, resulting in greater interference. This proposed mechanism fits well with the idea that working memory reflects the heightened activation of representations that are distributed throughout sensory, semantic, and motor cortex ( Postle 2006 ), and that similarity-based interference constrains the capacity due to focusing (see above; Awh et al. 2007 ). Hence, rather than whole-item displacement, specific feature competition may underlie the majority of encoding-stage RI.
Interference-based decay?
Above, we proposed a mechanism for decay based on the idea that stochastic variability causes the neurons making up a representation to fall out of synchrony (become less coherent in their firing patterns). Using the terminology of Nairne (2002) and Oberauer (2006) , the feature units become less tightly bound. Importantly, feature units that are not part of a representation also show some random activity due to their own stochastic variability, creating a noise distribution. Over time, there is an increasing likelihood that the feature units making up the to-be-remembered item’s representation will overlap with those of the noise distribution, making them increasingly difficult to distinguish. This increasing overlap with the noise distribution and loss of feature binding could lead to the smooth forgetting functions often interpreted as evidence for decay.
Such a mechanism for decay has interesting implications. It may explain why PI effects interact with retention interval. Prior trials with similar items would structure the noise distribution so that it is no longer random but rather is biased to share components with the representation of the to-be remembered item (target). Representations of prior, now-irrelevant items might compete with the current target’s representation for control of shared feature units, increasing the likelihood (rate) at which these units fall out of synchrony.
Prior similar items may also dampen the fidelity of the target representation to begin with, weakening their initial binding and thus causing these items to fall out of synchrony more quickly. In addition, poorly learned items might have fewer differentiating feature units, and these units may be less tightly bound and therefore more vulnerable to falling out of synchrony. This could explain why Keppel & Underwood (1962) found that poorly learned items resulted in retention interval effects even on the first trial. It may also underlie the greater decay effects that McKone (1995 , 1998) found for nonwords than for words, if one assumes that non-words have fewer meaning-based units and connections.
A SUMMARY OF PRINCIPLES AND AN ILLUSTRATION OF SHORT-TERM MEMORY AT WORK
Here we summarize the principles of STM that seem best supported by the behavioral and neural evidence. Building on these principles, we offer a hypothetical sketch of the processes and neural structures that are engaged by a canonical STM task, the probe recognition task with distracting material.
Principles of Short-Term Memory
We have motivated our review by questions of structure, process, and forgetting. Rather than organize our summary this way, we wish to return here to the title of our review and consider what psychological and neural mechanisms seem best defended by empirical work. In that we have provided details about each of these issues in our main discussion, we summarize them here as bullet points. Taken together, they provide answers to our questions about structure, process, and forgetting.
The mind of short-term memory
Representations in memory are composed of bundles of features for stored information, including features representing the context in which that information was encountered.
- ■ Representations in memory vary in activation, with a dormant state characterizing long-term memories, and varying states of activation due to recent perceptions or retrievals of those representations.
- ■ There is a focus of attention in which a bound collection of information may be held in a state that makes it immediately available for cognitive action. Attention may be focused on only a single chunk of information at a time, where a chunk is defined as a set of items that are bound by a common functional context.
- ■ Items may enter the focus of attention via perceptual encoding or via cue-based retrieval from LTM.
- ■ Items are maintained in the focus via a controlled process of maintenance, with rehearsal being a case of controlled sequential allocation of attentional focus.
- ■ Forgetting occurs when items leave the focus of attention and must compete with other items to regain the focus (interference), or when the fidelity of the representation declines over time due to stochastic processes (decay).
The brain of short-term memory
Items in the focus of attention are represented by patterns of heightened, synchronized firing of neurons in primary and secondary association cortex.
- ■ The sensorimotor features of items in the focus of attention or those in a heightened state of activation are the same as those activated by perception or action. Information within a representation is associated with the cortical region that houses it (e.g., verbal, spatial, motor). In short, item representations are stored where they are processed.
- ■ Medial temporal structures are important for binding items to their context for both the short- and long-term and for retrieving items whose context is no longer in the focus of attention or not yet fully consolidated in the neocortex.
- ■ The capacity to focus attention is constrained by parietal and frontal mechanisms that modulate processing as well as by increased noise in the neural patterns arising from similarity-based interference or from stochastic variability in firing.
- ■ Frontal structures support controlled processes of retrieval and interference resolution.
- ■ Placing an item into the focus of attention from LTM involves reactivating the representation that is encoded in patterns of neural connection weights.
- ■ Decay arises from the inherent variability of the neural firing of feature bundles that build a representation: The likelihood that the firing of multiple features will fall out of synchrony increases with time due to stochastic variability.
A Sketch of Short-Term Memory at Work
The theoretical principles outlined above summarize our knowledge of the psychological and neural bases of STM, but further insight can be gained by attempting to see how these mechanisms might work together, moment-by-moment, to accomplish the demands of simple tasks. We believe that working through an illustration will not only help to clarify the nature of the proposed mechanisms, but it may also lead to a picture of STM that is more detailed in its bridging of neural process and psychological function.
Toward these ends, we present here a specific implementation of the principles that allows us to give a description of the mechanisms that might be engaged at each point in a simple visual STM task. This exercise leads us to a view of STM that is heavily grounded in concepts of neural activation and plasticity. More specifically, we complement the assumptions about cognitive and brain function above with simple hypotheses about the relative supporting roles of neuronal firing and plasticity (described below). Although somewhat speculative in nature, this description is consistent with the summary principles, and it grounds the approach more completely in a plausible neural model. In particular, it has the virtue of providing an unbroken chain of biological mechanisms that supports the encoding of short-term memories over time.
Figure 1 traces the representation of one item in memory over the course of a few seconds in our hypothetical task. The cognitive events are demarcated at the top of the figure, and the task events at the bottom. In the hypothetical task, the subject must keep track of three visual items (such as novel shapes). The first item is presented for 700 milliseconds, followed by a delay of 2 seconds. The second stimulus then appears, followed by a delay of a few seconds, then the third stimulus, and another delay. Finally, the probe appears, and contact must be made with the memory for the first item. The assumption is that subjects will engage in a strategy of actively maintaining each item during the delay periods.

The processing and neural representation of one item in memory over the course of a few seconds in a hypothetical short-term memory task, assuming a simple single-item focus architecture. The cognitive events are demarcated at the top; the task events, at the bottom. The colored layers depict the extent to which different brain areas contribute to the representation of the item over time, at distinct functional stages of short-term memory processing. The colored layers also distinguish two basic types of neural representation: Solid layers depict memory supported by a coherent pattern of active neural firing, and hashed layers depict memory supported by changes in synaptic patterns. The example task requires processing and remembering three visual items; the figure traces the representation of the first item only. In this task, the three items are sequentially presented, and each is followed by a delay period. After the delay following the third item, a probe appears that requires retrieval of the first item. See the text for details corresponding to the numbered steps in the figure.
Before walking through the timeline in Figure 1 , let us take a high-level view. At any given time point, a vertical slice through the figure is intended to convey two key aspects of the neural basis of the memory. The first is the extent to which multiple cortical areas contribute to the representation of the item, as indicated by the colored layers corresponding to different cortical areas. The dynamic nature of the relative sizes of the layers captures several of our theoretical assumptions concerning the evolving contribution of those different areas at different functional stages of STM. The second key aspect is the distinction between memory supported by a coherent pattern of active neural firing (captured in solid layers) and memory supported by synaptic plasticity (captured in the hashed layers) ( Fuster 2003 , Grossberg 2003 , Rolls 2000 ). The simple hypothesis represented here is that perceptual encoding and active-focus maintenance are supported by neuronal firing, and memory of items outside the focus is supported by short-term synaptic plasticity ( Zucker & Regehr 2002 ). 3
We now follow the time course of the neural representation of the first item (in the order indicated by the numbers in the figure). ( 1 ) The stimulus is presented and rapidly triggers a coherent pattern of activity in posterior perceptual regions, representing both low-level visual features of the item content and its abstract identification in higher-level regions. ( 2 ) There is also a rapid onset of the representation of item-context binding (temporal context in our example) supported by the medial-temporal lobes (see section titled “Contesting the Idea of Separate Long-Term and Short-Term Systems”) ( Ranganath & Blumenfeld 2005 ). ( 3 ) Over the first few hundred milliseconds, this pattern increases in quality, yielding speed-accuracy tradeoffs in perceptual identification. ( 4 ) Concurrent with the active firing driven by the stimulus, very short-term synaptic plasticity across cortical areas begins to encode the item’s features and its binding to context. Zucker & Regehr (2002) identify at least three distinct plasticity mechanisms that begin to operate on this time scale (tens of milliseconds) and that together are sufficient to produce memories lasting several seconds. (For the use of this mechanism in a prominent neural network model of STM, see Burgess & Hitch 1999 , 2005 , 2006 .) ( 5 ) At the offset of the stimulus, the active firing pattern decays very rapidly (consistent with identified mechanisms of rapid decay in short-term potentiation; Zucker & Regehr 2002 ), but ( 6 ) active maintenance, mediated by increased activity in frontal and parietal systems, maintains the firing pattern during the delay period (see sections titled “The Architecture of Unitary-Store Models” and “Maintenance of Items in the Focus”) ( Pasternak & Greenlee 2005 , Ranganath 2006 , Ruchkin et al. 2003 ). This active delay firing includes sustained contribution of MTL to item-context binding (see section titled “Contesting the Idea of Separate Long-Term and Short-Term Systems”). Significant reduction in coherence of the firing pattern may occur as a result of stochastic drift as outlined above (in sections titled “What Happens Neurally During the Delay?” and “Interference-Based Decay?”), possibly leading to a kind of short-term decay during maintenance (see section titled “What Happens Neurally During the Delay?”) ( Fuster 1995 , Pasternak & Greenlee 2005 ). ( 7 ) The active maintenance involves the reuse of posterior perceptual regions in the service of the task demands on STM. This reuse includes even early perceptual areas, but we show here a drop in the contribution of primary perceptual regions to maintenance in order to indicate a relatively greater effect of top-down control on the later high-level regions ( Postle 2006 , Ranganath 2006 ). ( 8 ) During this delay period of active maintenance, short-term potentiation continues to lay down a trace of the item and its binding to context via connection weights both within and across cortical regions. The overall efficacy of this memory encoding is the result of the interaction of the possibly decaying active firing pattern with the multiple plasticity mechanisms and their individual facilitation and depression profiles ( Zucker & Regehr 2002 ).
( 9 ) At the end of the delay period and the onset of the second stimulus, the focus rapidly shifts to the new stimulus, and the active firing of the neural pattern of the target stimulus ceases. ( 10 ) The memory of the item is now carried completely by the changed synaptic weights, but this change is partially disrupted by the incoming item and its engagement of a similar set of neural activity patterns. Cognitively, this disruption yields similarity-based retroactive interference (see “Other Mechanisms for Interference Effects?”) ( Nairne 2002 ). ( 11 ) Even in the absence of interference, a variety of biochemical processes give rise to the decay of short-term neural change and therefore the gradual loss of the memory trace over time. This pattern of interference and decay continues during processing of both the second and third stimulus. The probe triggers a rapid memory retrieval of the target item ( 12 ), mediated in part by strategic frontal control (see “Neural Mechanisms of Short- and Long-Term Memory Retrieval”) ( Cabeza et al. 2002 , Ranganath et al. 2004 ). This rapid retrieval corresponds to the reinstantiation of the target item’s firing pattern in both posterior perceptual areas ( 13 ) and medial-temporal regions, the latter supporting the contextual binding. A plausible neural mechanism for the recovery of this activity pattern at retrieval is the emergent pattern-completion property of attractor networks ( Hopfield 1982 ). Attractor networks depend on memories encoded in a pattern of connection weights, whose formation and dynamics we have sketched above in terms of short-term synaptic plasticity. Such networks also naturally give rise to the kind of similarity-based proactive interference clearly evident in STM retrieval (see “Selection-Based Interference Effects”) ( Jonides & Nee 2006 , Keppel & Underwood 1962 ).
We have intentionally left underspecified a precise quantitative interpretation of the y -axis in Figure 1 . Psychologically, it perhaps corresponds to a combination of availability (largely driven by the dichotomous nature of the focus state) and accessibility (driven by a combination of both firing and plasticity). Neurally, it perhaps corresponds to some measure of both firing amplitude and coherence and potential firing amplitude and coherence.
We are clearly a long way from generating something like the plot in Figure 1 from neuroimaging data on actual tasks—though plots of event-related potentials in STM tasks give us an idea of what these data may look like ( Ruchkin et al. 2003 ). There no doubt is more missing from Figure 1 than is included (e.g., the role of subcortical structures such as the basal ganglia in the frontal/parietal mediated control, or the reciprocal cortical-thalamic circuits that shape the nature of the neocortical patterns).We nevertheless believe that the time course sketched in Figure 1 is useful for making clear many of the central properties that characterize the psychological and neural theory of human STM outlined above: ( a ) STM engages essentially all cortical areas—including medial temporal lobes—and does so from the earliest moments, though it engages these areas differentially at different functional stages. ( b ) STM reuses the same posterior cortical areas and representations that subserve perception, and active maintenance of these representations depends on these posterior areas receiving input from frontal-parietal circuits. ( c ) Focused items are distinguished both functionally and neurally by active firing patterns, and nonfocused memories depend on synaptic potentiation and thereby suffer from decay and retroactive interference. ( d ) Nonfocused memories are reinstantiated into active firing states via an associative retrieval process subject to proactive interference from similarly encoded patterns.
Postscript: Revisiting Complex Cognition
A major goal of this review has been to bring together psychological theorizing (the mind) and neuroscientific evidence (the brain) of STM. However, any celebration of this union is premature until we address this question: Can our account explain how the mind and brain accomplish the everyday tasks (e.g., completing a tax form) that opened this review? The recognition probe task used in our example and the other procedures discussed throughout the main text are considerably simpler than those everyday tasks. Is it plausible to believe that the system outlined here, particularly in light of its severely limited capacity, could support human cognition in the wild?
It is sobering to note that Broadbent (1993) and Newell (1973 , 1990) asked this question nearly two decades ago, and at that time they were considering models of STM with even larger capacities than the one advocated here. Even so, both observed that none of the extant computational models of complex cognitive tasks (e.g., the Newell & Simon 1972 models of problem solving) used contemporary psychological theories of STM. Instead, the complex-cognition models assumed much larger (in some cases, effectively unlimited) working memories. The functional viability of the STM theories of that time was thus never clearly demonstrated. Since then, estimates of STM capacity have only grown smaller, so the question, it would seem, has grown correspondingly more pressing.
Fortunately, cognitive modeling and cognitive theory have also developed over that time, and in ways that would have pleased both Broadbent and Newell. Importantly, many computational cognitive architectures now make assumptions about STM capacity that are congruent with the STM models discussed in this review. The most prominent example is ACT-R, a descendent of the early Newell production-system models. ACT-R continues to serve as the basis of computational models of problem solving (e.g., Anderson & Douglass 2001 ), sentence processing ( Lewis & Vasishth 2005 , Lewis et al. 2006 ), and complex interactive tasks ( Anderson et al. 2004 ). However, the current version of ACT-R has a focus-based structure with an effective capacity limit of four or fewer items ( Anderson et al. 2004 ).
Another important theoretical development is the long-term working memory approach of Ericsson & Kintsch (1995) . This approach describes how LTM, using the kind of fast-encoding and cue-based associative retrieval processes assumed here, can support a variety of complex cognitive tasks ranging from discourse comprehension to specialized expert performance. In both the modern approaches to computational architecture and long-term working memory, the power of cognition resides not in capacious short-term buffers but rather in the effective use of an associative LTM. A sharply limited focus of attention does not, after all, seem to pose insurmountable functional problems.
In summary, this review describes the still-developing convergence of computational models of complex cognition, neural network models of simple memory tasks, modern psychological studies of STM, and neural studies of memory in both humans and primates. The points of contact among these different methods of studying STM have multiplied over the past several years. As we have pointed out, significant and exciting challenges in furthering this integration lie ahead.
1 Another line of neural evidence about the separability of short- and long-term memory comes from electrophysiological studies of animals engaged in short-term memory tasks. We review this evidence and its interpretation in The Architecture of Unitary-Store Models section.
2 This carving up of STM processes is also consistent with recent approaches to individual differences in working memory, which characterize individual variation not in terms of variation in buffer capacity, but rather in variation in maintenance and retrieval processes ( Unsworth & Engle 2007 ).
3 The alternative to this strong claim is that memory items outside the focus might also be supported by residual active firing. The empirical results reviewed above indicating load-dependent posterior activation might lend support to this alternative if one assumes that the memory load in those experiments was not entirely held in the focus, and that these activations exclusively index firing associated with the memory load itself.
LITERATURE CITED
- Altmann EM, Gray WD. Forgetting to remember: the functional relationship of decay and interference. Psychol. Sci. 2002; 13 (1):27–33. [ PubMed ] [ Google Scholar ]
- Anderson JR. Retrieval of information from long-term memory. Science. 1983; 220 (4592):25–30. [ PubMed ] [ Google Scholar ]
- Anderson JR, Bothell D, Byrne MD, Douglass S, Lebiere C, Qin Y. An integrated theory of mind. Psychol. Rev. 2004; 111 :1036–1060. [ PubMed ] [ Google Scholar ]
- Anderson JR, Douglass S. Tower of Hanoi: evidence for the cost of goal retrieval. J. Exp. Psychol.: Learn. Mem. Cogn. 2001; 27 :1331–1346. [ PubMed ] [ Google Scholar ]
- Anderson JR, Matessa M. A production system theory of serial memory. Psychol. Rev. 1997; 104 (4):728–748. [ Google Scholar ]
- Anderson JR, Schooler LJ. Reflections of the environment in memory. Psychol. Sci. 1991; 2 (6):396–408. [ Google Scholar ]
- Atkinson RC, Shiffrin RM. The control of short-term memory. Sci. Am. 1971; 224 :82–90. [ PubMed ] [ Google Scholar ]
- Awh E, Barton B, Vogel EK. Visual working memory represents a fixed number of items regardless of complexity. Psychol. Sci. 2007; 18 (7):622–628. [ PubMed ] [ Google Scholar ]
- Awh E, Jonides J. Overlapping mechanisms of attention and spatial working memory. Trends Cogn. Sci. 2001; 5 (3):119–126. [ PubMed ] [ Google Scholar ]
- Awh E, Jonides J, Reuter-Lorenz PA. Rehearsal in spatial working memory. J. Exp. Psychol.: Hum. Percept. Perform. 1998; 24 :780–790. [ PubMed ] [ Google Scholar ]
- Awh E, Jonides J, Smith EE, Buxton RB, Frank LR, et al. Rehearsal in spatial working memory: evidence from neuroimaging. Psychol. Sci. 1999; 10 (5):433–437. [ Google Scholar ]
- Awh E, Jonides J, Smith EE, Schumacher EH, Koeppe RA, Katz S. Dissociation of storage and rehearsal in verbal working memory: evidence from PET. Psychol. Sci. 1996; 7 :25–31. [ Google Scholar ]
- Baddeley AD. Working Memory. Oxford: Clarendon; 1986. [ Google Scholar ]
- Baddeley AD. Working memory. Science. 1992; 225 :556–559. [ PubMed ] [ Google Scholar ]
- Baddeley AD. The episodic buffer: a new component of working memory? Trends Cogn. Sci. 2000; 4 (11):417–423. [ PubMed ] [ Google Scholar ]
- Baddeley AD. Working memory: looking back and looking forward. Nat. Rev. Neurosci. 2003; 4 (10):829–839. [ PubMed ] [ Google Scholar ]
- Baddeley AD, Hitch G. Working memory. In: Bower GA, editor. Recent Advances in Learning and Motivation. Vol. 8. New York: Academic; 1974. pp. 47–90. [ Google Scholar ]
- Baddeley AD, Thomson N, Buchanan M. Word length and structure of short-term memory. J. Verbal Learn. Verbal Behav. 1975; 14 (6):575–589. [ Google Scholar ]
- Baddeley AD, Vallar G, Wilson BA. Sentence comprehension and phonological working memory: some neuropsychological evidence. In: Coltheart M, editor. Attention and Performance XII: The Psychology of Reading. London: Erlbaum; 1987. pp. 509–529. [ Google Scholar ]
- Baddeley AD, Warrington EK. Amnesia and the distinction between long- and short-term memory. J. Verbal Learn. Verbal Behav. 1970; 9 :176–189. [ Google Scholar ]
- Baddeley AD, Wilson BA. Prose recall and amnesia: implications for the structure of working memory. Neuropsychologia. 2002; 40 :1737–1743. [ PubMed ] [ Google Scholar ]
- Badre D, Wagner AD. Frontal lobe mechanisms that resolve proactive interference. Cereb. Cortex. 2005; 15 :2003–2012. [ PubMed ] [ Google Scholar ]
- Braver TS, Barch DM, Kelley WM, Buckner RL, Cohen NJ, et al. Direct comparison of prefrontal cortex regions engaged by working and long-term memory tasks. Neuroimage. 2001; 14 :48–59. [ PubMed ] [ Google Scholar ]
- Broadbent D. Comparison with human experiments. In: Broadbent D, editor. The Simulation of Human Intelligence. Oxford: Blackwell Sci; 1993. pp. 198–217. [ Google Scholar ]
- Brooks LR. Spatial and verbal components of the act of recall. Can. J. Psychol. 1968; 22 :349–368. [ Google Scholar ]
- Brown GDA, Preece T, Hulme C. Oscillator-based memory for serial order. Psychol. Rev. 2000; 107 (1):127–181. [ PubMed ] [ Google Scholar ]
- Buckner RL, Koutstaal W, Schacter DL, Wagner AD, Rosen BR. Functional-anatomic study of episodic retrieval using fMRI: I. Retrieval effort versus retrieval success. NeuroImage. 1998; 7 (3):151–162. [ PubMed ] [ Google Scholar ]
- Buffalo EA, Reber PJ, Squire LR. The human perirhinal cortex and recognition memory. Hippocampus. 1998; 8 :330–339. [ PubMed ] [ Google Scholar ]
- Burgess N, Hitch GJ. Memory for serial order: a network model of the phonological loop and its timing. Psychol. Rev. 1999; 106 (3):551–581. [ Google Scholar ]
- Burgess N, Hitch GJ. Computational models of working memory: putting long-term memory into context. Trends Cogn. Sci. 2005; 9 :535–541. [ PubMed ] [ Google Scholar ]
- Burgess N, Hitch GJ. A revised model of short-term memory and long-term learning of verbal sequences. J. Mem. Lang. 2006; 55 :627–652. [ Google Scholar ]
- Cabeza R, Dolcos F, Graham R, Nyberg L. Similarities and differences in the neural correlates of episodic memory retrieval and working memory. Neuroimage. 2002; 16 :317–330. [ PubMed ] [ Google Scholar ]
- Cabeza R, Nyberg L. Imaging cognition II: an empirical review of 275 PET and fMRI studies. J. Cogn. Neurosci. 2000; 9 :254–265. [ PubMed ] [ Google Scholar ]
- Cave CB, Squire LR. Intact verbal and nonverbal short-term memory following damage to the human hippocampus. Hippocampus. 1992; 2 :151–163. [ PubMed ] [ Google Scholar ]
- Cowan N. Evolving conceptions of memory storage, selective attention, and their mutual constraints within the human information processing system. Psychol. Bull. 1988; 104 :163–191. [ PubMed ] [ Google Scholar ]
- Cowan N. Attention and Memory: An Integrated Framework. New York: Oxford Univ. Press; 1995. [ Google Scholar ]
- Cowan N. The magical number 4 in short-term memory: a reconsideration of mental storage capacity. Behav. Brain Sci. 2000; 24 :87–185. [ PubMed ] [ Google Scholar ]
- Crowder R. Principles of Learning and Memory. Hillsdale, NJ: Erlbaum; 1976. [ Google Scholar ]
- Damasio AR. Time-locked multiregional retroactivation: a system-level proposal for the neuronal substrates of recall and recognition. Cognition. 1989; 33 :25–62. [ PubMed ] [ Google Scholar ]
- Darwin CJ, Turvey MT, Crowder RG. Auditory analogue of Sperling partial report procedure—evidence for brief auditory storage. Cogn. Psychol. 1972; 3 (2):255–267. [ Google Scholar ]
- Deiber MP, Missonnier P, Bertrand O, Gold G, Fazio-Costa L, et al. Distinction between perceptual and attentional processing in working memory tasks: a study of phase-locked and induced oscillatory brain dynamics. J. Cogn. Neurosci. 2007; 19 (1):158–172. [ PubMed ] [ Google Scholar ]
- den Heyer K, Barrett B. Selective loss of visual and verbal information in STM by means of visual and verbal interpolated tasks. Psychon. Sci. 1971; 25 :100–102. [ Google Scholar ]
- D’Esposito M, Postle BR. The dependence of span and delayed-response performance on prefrontal cortex. Neuropsychologia. 1999; 37 (11):1303–1315. [ PubMed ] [ Google Scholar ]
- D’Esposito M, Postle BR. Neural correlates of processes contributing to working memory function: evidence from neuropsychological and pharmacological studies. In: Monsell S, Driver J, editors. Control of Cognitive Processes. Cambridge, MA: MIT Press; 2000. pp. 580–602. [ Google Scholar ]
- D’Esposito M, Postle BR, Jonides J, Smith EE, Lease J. The neural substrate and temporal dynamics of interference effects in working memory as revealed by event-related fMRI. Proc. Natl. Acad. Sci. USA. 1999; 96 :7514–7519. [ PMC free article ] [ PubMed ] [ Google Scholar ]
- Eng HY, Chen DY, Jiang YH. Visual working memory for simple and complex visual stimuli. Psychon. Bull. Rev. 2005; 12 :1127–1133. [ PubMed ] [ Google Scholar ]
- Ericsson KA, Kintsch W. Long-term working memory. Psychol. Rev. 1995; 102 :211–245. [ PubMed ] [ Google Scholar ]
- Fletcher PC, Henson RNA. Frontal lobes and human memory—insights from functional neuroimaging. Brain. 2001; 124 :849–881. [ PubMed ] [ Google Scholar ]
- Frank MJ, Loughry B, O’Reilly RC. Interactions between the frontal cortex and basal ganglia in working memory: a computational model. Cogn. Affect. Behav. Neurosci. 2001; 1 :137–160. [ PubMed ] [ Google Scholar ]
- Funahashi S, Bruce CJ, Goldman-Rakic PS. Mnemonic coding of visual space in the monkey’s dorsolateral prefrontal cortex. J. Neurophysiol. 1989; 61 :331–349. [ PubMed ] [ Google Scholar ]
- Fuster JK. Thoughts from the long-term memory chair. Behav. Brain Sci. 2003; 26 :734–735. [ Google Scholar ]
- Fuster JM. Unit activity in prefrontal cortex during delayed response performance: neuronal correlates of transient memory. J. Neurophysiol. 1973; 36 :61–78. [ PubMed ] [ Google Scholar ]
- Fuster JM. Memory in the Cerebral Cortex. Cambridge, MA: MIT Press; 1995. [ Google Scholar ]
- Gabrieli JDE, Brewer JB, Desmond JE, Glover GH. Separate neural bases of two fundamental memory processes in the human medial temporal lobe. Science. 1997; 276 :264–266. [ PubMed ] [ Google Scholar ]
- Garavan H. Serial attention within working memory. Mem. Cogn. 1998; 26 :263–276. [ PubMed ] [ Google Scholar ]
- Gardiner JM, Craik FIM, Birtwist J. Retrieval cues and release from proactive inhibition. J. Verbal Learn. Verbal Behav. 1972; 11 (6):778–783. [ Google Scholar ]
- Gillund G, Shiffrin RM. A retrieval model for both recognition and recall. Psychol. Rev. 1984; 91 (1):1–67. [ PubMed ] [ Google Scholar ]
- Goldman-Rakic PS. Circuitry of primate pre-frontal cortex and regulation of behavior by representational memory. In: Plum F, editor. Handbook of Physiology: The Nervous System. Vol. 5. Bethesda, MD: 1987. pp. 373–417. Am. Physiol. Soc. [ Google Scholar ]
- Grossberg S. From working memory to long-term memory and back: linked but distinct. Behav. Brain Sci. 2003; 26 :737–738. [ Google Scholar ]
- Hampson M, Driesen NR, Skudlarski P, Gore JC, Constable RT. Brain connectivity related to working memory performance. J. Neurosci. 2006; 26 (51):13338–13343. [ PMC free article ] [ PubMed ] [ Google Scholar ]
- Hanley JR, Young AW, Pearson NA. Impairment of the visuo-spatial sketch pad. Q. J. Exp. Psychol. Hum. Exp. Psychol. 1991; 43 :101–125. [ PubMed ] [ Google Scholar ]
- Hannula DE, Tranel D, Cohen NJ. The long and the short of it: relational memory impairments in amnesia, even at short lags. J. Neurosci. 2006; 26 (32):8352–8359. [ PMC free article ] [ PubMed ] [ Google Scholar ]
- Harris JD. The decline of pitch discrimination with time. J. Exp. Psychol. 1952; 43 (2):96–99. [ PubMed ] [ Google Scholar ]
- Hasher L, Zacks RT. Working memory, comprehension, and aging: a review and a new view. In: Bower GH, editor. The Psychology of Learning and Motivation. Vol. 22. New York: Academic; 1988. pp. 193–225. [ Google Scholar ]
- Hebb DO. The Organization of Behavior. New York: Wiley; 1949. [ Google Scholar ]
- Hockley WE. Analysis of response-time distributions in the study of cognitive-processes. J. Exp. Psychol.: Learn. Mem. Cogn. 1984; 10 (4):598–615. [ Google Scholar ]
- Holdstock JS, Shaw C, Aggleton JP. The performance of amnesic subjects on tests of delayed matching-to-sample and delayed matching-to-position. Neuropsychologia. 1995; 33 :1583–1596. [ PubMed ] [ Google Scholar ]
- Hopfield JJ. Neural networks and physical systems with emergent collective computational abilities. Proc. Natl. Acad. Sci. USA. 1982; 79 (8):2554–2558. [ PMC free article ] [ PubMed ] [ Google Scholar ]
- Jacobsen CF. The functions of the frontal association areas in monkeys. Comp. Psychol. Monogr. 1936; 13 :1–60. [ Google Scholar ]
- James W. Principles of Psychology. New York: Henry Holt; 1890. [ Google Scholar ]
- Jensen O. Maintenance of multiple working memory items by temporal segmentation. Neuroscience. 2006; 139 :237–249. [ PubMed ] [ Google Scholar ]
- Jha AP, McCarthy G. The influence of memory load upon delay-interval activity in a working-memory task: an event-related functional MRI study. J. Cogn. Neurosci. 2000; 12 :90–105. [ PubMed ] [ Google Scholar ]
- Johnson MK, Raye CL, Mitchell KJ, Greene EJ, Cunningham WA, Sanislow CA. Using fMRI to investigate a component process of reflection: prefrontal correlates of refreshing a just-activated representation. Cogn. Affect. Behav. Neurosci. 2005; 5 :339–361. [ PubMed ] [ Google Scholar ]
- Jonides J, Lacey SC, Nee DE. Processes of working memory in mind and brain. Curr. Dir. Psychol. Sci. 2005; 14 :2–5. [ Google Scholar ]
- Jonides J, Nee DE. Brain mechanisms of proactive interference in working memory. Neuroscience. 2006; 139 :181–193. [ PubMed ] [ Google Scholar ]
- Jonides J, Smith EE, Koeppe RA, Awh E, Minoshima S, Mintun MA. Spatial working memory in humans as revealed by PET. Nature. 1993; 363 :623–625. [ PubMed ] [ Google Scholar ]
- Jonides J, Smith EE, Marshuetz C, Koeppe RA, Reuter-Lorenz PA. Inhibition in verbal working memory revealed by brain activation. Proc. Natl. Acad. Sci. USA. 1998; 95 :8410–8413. [ PMC free article ] [ PubMed ] [ Google Scholar ]
- Keppel G, Underwood BJ. Proactive-inhibition in short-term retention of single items. J. Verbal Learn. Verbal Behav. 1962; 1 :153–161. [ Google Scholar ]
- Lange EB, Oberauer K. Overwriting of phonemic features in serial recall. Memory. 2005; 13 :333–339. [ PubMed ] [ Google Scholar ]
- Lewandowsky S, Duncan M, Brown GDA. Time does not cause forgetting in short-term serial recall. Psychon. Bull. Rev. 2004; 11 :771–790. [ PubMed ] [ Google Scholar ]
- Lewis RL, Vasishth S. An activation-based theory of sentence processing as skilled memory retrieval. Cogn. Sci. 2005; 29 :375–419. [ PubMed ] [ Google Scholar ]
- Lewis RL, Vasishth S, Van Dyke J. Computational principles of working memory in sentence comprehension. Trends Cogn. Sci. 2006; 10 :447–454. [ PMC free article ] [ PubMed ] [ Google Scholar ]
- Lisman JE, Idiart MAP. Storage of 7+/−2 short-term memories in oscillatory subcycles. Science. 1995; 267 :1512–1515. [ PubMed ] [ Google Scholar ]
- Luck SJ, Vogel EK. The capacity of visual working memory for features and conjunctions. Nature. 1997; 390 :279–281. [ PubMed ] [ Google Scholar ]
- Lustig C, Matell MS, Meck WH. Not “just” a coincidence: frontal-striatal interactions in working memory and interval timing. Memory. 2005; 13 :441–448. [ PubMed ] [ Google Scholar ]
- Malmo RB. Interference factors in delayed response in monkeys after removal of frontal lobes. J. Neurophysiol. 1942; 5 :295–308. [ Google Scholar ]
- Manoach DS, Greve DN, Lindgren KA, Dale AM. Identifying regional activity associated with temporally separated components of working memory using event-related functional MRI. NeuroImage. 2003; 20 (3):1670–1684. [ PubMed ] [ Google Scholar ]
- Martin RC. Short-term memory and sentence processing: evidence from neuropsychology. Mem. Cogn. 1993; 21 :176–183. [ PubMed ] [ Google Scholar ]
- McClelland JL, McNaughton BL, O’Reilly RC. Why there are complementary learning systems in the hippocampus and neocortex: insights from the successes and failures of connectionist models of learning and memory. Psychol. Rev. 1995; 102 :419–457. [ PubMed ] [ Google Scholar ]
- McElree B. Attended and nonattended states in working memory: accessing categorized structures. J. Mem. Lang. 1998; 38 :225–252. [ Google Scholar ]
- McElree B. Working memory and focal attention. J. Exp. Psychol.: Learn. Mem. Cogn. 2001; 27 :817–835. [ PMC free article ] [ PubMed ] [ Google Scholar ]
- McElree B. Accessing recent events. Psychol. Learn. Motiv. 2006; 46 :155–200. [ Google Scholar ]
- McElree B, Dosher BA. Serial position and set size in short-term memory: time course of recognition. J. Exp. Psychol.: Gen. 1989; 118 :346–373. [ Google Scholar ]
- McGeoch J. Forgetting and the law of disuse. Psychol. Rev. 1932; 39 :352–370. [ Google Scholar ]
- McKone E. Short-term implicit memory for words and non-words. J. Exp. Psychol.: Learn. Mem. Cogn. 1995; 21 (5):1108–1126. [ Google Scholar ]
- McKone E. The decay of short-term implicit memory: unpacking lag. Mem. Cogn. 1998; 26 (6):1173–1186. [ PubMed ] [ Google Scholar ]
- Meyer DE, Kieras DE. A computational theory of executive cognitive processes and multiple-task performance: 1. Basic mechanisms. Psychol. Rev. 1997; 104 (1):3–65. [ PubMed ] [ Google Scholar ]
- Miller GA. The magical number seven, plus or minus two: some limits on our capacity for processing information. Psychol. Rev. 1956; 63 :81–97. [ PubMed ] [ Google Scholar ]
- Milner PM. Magical attention. Behav. Brain Sci. 2001; 24 (1):131. [ Google Scholar ]
- Miyashita Y, Chang HS. Neuronal correlate of pictorial short-term memory in the primate temporal cortex. Nature. 1968; 331 :68–70. [ PubMed ] [ Google Scholar ]
- Monsell S. Recency, immediate recognition memory, and reaction-time. Cogn. Psychol. 1978; 10 (4):465–501. [ Google Scholar ]
- Murdock BB. A theory for the storage and retrieval of item and associative information. Psychol. Rev. 1982; 89 (6):609–626. [ PubMed ] [ Google Scholar ]
- Nairne JS. Remembering over the short-term: the case against the standard model. Annu. Rev. Psychol. 2002; 53 :53–81. [ PubMed ] [ Google Scholar ]
- Neath I, Nairne JS. Word-length effects in immediate memory: overwriting trace decay theory. Psychon. Bull. Rev. 1995; 2 :429–441. [ PubMed ] [ Google Scholar ]
- Nelson JK, Reuter-Lorenz PA, Sylvester CYC, Jonides J, Smith EE. Dissociable neural mechanisms underlying response-based and familiarity-based conflict in working memory. Proc. Natl. Acad. Sci. USA. 2003; 100 :11171–11175. [ PMC free article ] [ PubMed ] [ Google Scholar ]
- Newell A. You can’t play 20 questions with nature and win: projective comments on the papers of this symposium. In: Chase WG, editor. Visual Information Processing; Academic; New York. 1973. pp. 283–310. [ Google Scholar ]
- Newell A. Unified Theories of Cognition. Cambridge, MA: Harvard Univ. Press; 1990. [ Google Scholar ]
- Newell A, Simon H. Human Problem Solving. Englewood Cliffs, NJ: Prentice-Hall; 1972. [ Google Scholar ]
- Nichols EA, Kao Y-C, Verfaellie M, Gabrieli JDE. Working memory and long-term memory for faces: evidence from fMRI and global amnesia for involvement of the medial temporal lobes. Hippocampus. 2006; 16 :604–616. [ PMC free article ] [ PubMed ] [ Google Scholar ]
- Oberauer K. Access to information in working memory: exploring the focus of attention. J. Exp. Psychol.: Learn. Mem. Cogn. 2002; 28 :411–421. [ PubMed ] [ Google Scholar ]
- Oberauer K. Is the focus of attention in working memory expanded through practice? J. Exp. Psychol.: Learn. Mem. Cogn. 2006; 32 :197–214. [ PubMed ] [ Google Scholar ]
- Oberauer K, Kliegl R. A formal model of capacity limits in working memory. J. Mem. Lang. 2006; 55 :601–626. [ Google Scholar ]
- Olson IR, Moore KS, Stark M, Chatterjee A. Visual working memory is impaired when the medial temporal lobe is damaged. J. Cogn. Neurosci. 2006a; 18 :1087–1097. [ PubMed ] [ Google Scholar ]
- Olson IR, Page K, Moore KS, Chatterjee A, Verfaellie M. Working memory for conjunctions relies on the medial temporal lobe. J. Neurosci. 2006b; 26 :4596–4601. [ PMC free article ] [ PubMed ] [ Google Scholar ]
- Owen AM, Sahakian BJ, Semple J, Polkey CE, Robbins TW. Visuo-spatial short-term recognition memory and learning after temporal lobe excisions, frontal lobe excisions or amygdala-hippocampectomy in man. Neuropsychologia. 1995; 33 :1–24. [ PubMed ] [ Google Scholar ]
- Pashler H. Familiarity and visual change detection. Percept. Psychophys. 1988; 44 :369–378. [ PubMed ] [ Google Scholar ]
- Pasternak T, Greenlee MW. Working memory in primate sensory systems. Nat. Rev. Neurosci. 2005; 6 :97–107. [ PubMed ] [ Google Scholar ]
- Polk TA, Simen P, Lewis RL, Freedman E. A computational approach to control in complex cognition. Cogn. Brain Res. 2002; 15 (1):71–83. [ PubMed ] [ Google Scholar ]
- Postle BR. Working memory as an emergent property of the mind and brain. Neuroscience. 2006; 139 :23–38. [ PMC free article ] [ PubMed ] [ Google Scholar ]
- Postle BR, D’Esposito M. “What”—then—“where” in visual working memory: an event-related, fMRI study. J. Cogn. Neurosci. 1999; 11 (6):585–597. [ PubMed ] [ Google Scholar ]
- Postman L. Extra-experimental interference and retention of words. J. Exp. Psychol. 1961; 61 (2):97–110. [ PubMed ] [ Google Scholar ]
- Prabhakaran V, Narayanan ZZ, Gabrieli JDE. Integration of diverse information in working memory within the frontal lobe. Nat. Neurosci. 2000; 3 :85–90. [ PubMed ] [ Google Scholar ]
- Pylyshyn ZW. Some primitive mechanisms of spatial attention. Cognition. 1994; 50 :363–384. [ PubMed ] [ Google Scholar ]
- Pylyshyn ZW, Burkell J, Fisher B, Sears C, Schmidt W, Trick L. Multiple parallel access in visual-attention. Can. J. Exp. Psychol. Rev. Can. Psychol. Exp. 1994; 48 (2):260–283. [ PubMed ] [ Google Scholar ]
- Raichle ME, MacLeod AM, Snyder AZ, Powers WJ, Gusnard DA, Shulman GL. A default mode of brain function. Proc. Natl. Acad. Sci. USA. 2001; 98 :676–682. [ PMC free article ] [ PubMed ] [ Google Scholar ]
- Ranganath C. Working memory for visual objects: complementary roles of inferior temporal, medial temporal, and prefrontal cortex. Neuroscience. 2006; 139 :277–289. [ PubMed ] [ Google Scholar ]
- Ranganath C, Blumenfeld RS. Doubts about double dissociations between short- and long-term memory. Trends Cogn. Sci. 2005; 9 :374–380. [ PubMed ] [ Google Scholar ]
- Ranganath C, DeGutis J, D’Esposito M. Category-specific modulation of inferior temporal activity during working memory encoding and maintenance. Cogn. Brain Res. 2004; 20 :37–45. [ PubMed ] [ Google Scholar ]
- Ranganath C, D’Esposito M. Medial temporal lobe activity associated with active maintenance of novel information. Neuron. 2001; 31 :865–873. [ PubMed ] [ Google Scholar ]
- Ranganath C, D’Esposito M. Directing the mind’s eye: prefrontal, inferior and medial temporal mechanisms for visual working memory. Curr. Opin. Neurobiol. 2005; 15 :175–182. [ PubMed ] [ Google Scholar ]
- Ranganath C, Johnson MK, D’Esposito M. Prefrontal activity associated with working memory and episodic long-term memory. Neuropsychologia. 2003; 41 (3):378–389. [ PubMed ] [ Google Scholar ]
- Renart A, Parga N, Rolls ET. Backward projections in the cerebral cortex: implications for memory storage. Neural Comput. 1999; 11 (6):1349–1388. [ PubMed ] [ Google Scholar ]
- Repov G, Baddeley AD. The multi-component model of working memory: explorations in experimental cognitive psychology. Neuroscience. 2006; 139 :5–21. [ PubMed ] [ Google Scholar ]
- Reuter-Lorenz PA, Jonides J. The executive is central to working memory: insights from age performance and task variations. In: Conway AR, Jarrold C, Kane MJ, Miyake A, Towse JN, editors. Variations in Working Memory. London/New York: Oxford Univ. Press: 2007. pp. 250–270. [ Google Scholar ]
- Reuter-Lorenz PA, Jonides J, Smith EE, Hartley A, Miller A, et al. Age differences in the frontal lateralization of verbal and spatial working memory revealed by PET. J. Cogn. Neurosci. 2000; 12 :174–187. [ PubMed ] [ Google Scholar ]
- Roediger HL, Knight JL, Kantowitz BH. Inferring decay in short-term-memory—the issue of capacity. Mem. Cogn. 1977; 5 (2):167–176. [ PubMed ] [ Google Scholar ]
- Rolls ET. Memory systems in the brain. Annu. Rev. Psychol. 2000; 51 :599–630. [ PubMed ] [ Google Scholar ]
- Rougier NP, Noelle DC, Braver TS, Cohen JD, O’Reilly RC. Prefrontal cortex and flexible cognitive control: rules without symbols. Proc. Natl. Acad. Sci. USA. 2005; 102 (20):7338–7343. [ PMC free article ] [ PubMed ] [ Google Scholar ]
- Ruchkin DS, Grafman J, Cameron K, Berndt RS. Working memory retention systems: a state of activated long-term memory. Behav. Brain Sci. 2003; 26 :709–777. [ PubMed ] [ Google Scholar ]
- Sakai K. Reactivation of memory: role of medial temporal lobe and prefrontal cortex. Rev. Neurosci. 2003; 14 (3):241–252. [ PubMed ] [ Google Scholar ]
- Schubert T, Frensch PA. How unitary is the capacity-limited attentional focus? Behav. Brain Sci. 2001; 24 (1):146. [ Google Scholar ]
- Scoville WB, Milner B. Loss of recent memory after bilateral hippocampal lesions. J. Neurol. Neurosurg. Psychiatry. 1957; 20 :11–21. [ PMC free article ] [ PubMed ] [ Google Scholar ]
- Shallice T, Warrington EK. Independent functioning of verbal memory stores: a neuropsychological study. Q. J. Exp. Psychol. 1970; 22 :261–273. [ PubMed ] [ Google Scholar ]
- Smith EE, Jonides J. Working memory: a view from neuroimaging. Cogn. Psychol. 1997; 33 :5–42. [ PubMed ] [ Google Scholar ]
- Smith EE, Jonides J. Neuroscience—storage and executive processes in the frontal lobes. Science. 1999; 283 :1657–1661. [ PubMed ] [ Google Scholar ]
- Smith EE, Jonides J, Koeppe RA, Awh E, Schumacher EH, Minoshima S. Spatial vs object working-memory: PET investigations. J. Cogn. Neurosci. 1995; 7 :337–356. [ PubMed ] [ Google Scholar ]
- Sperling G. The information available in brief visual presentations. Psychol. Monogr. 1960; 74 Whole No. 498. [ Google Scholar ]
- Squire L. Memory and the hippocampus: a synthesis from findings with rats, monkeys, and humans. Psychol. Rev. 1992; 99 :195–231. [ PubMed ] [ Google Scholar ]
- Sternberg S. High-speed scanning in human memory. Science. 1966; 153 :652–654. [ PubMed ] [ Google Scholar ]
- Talmi D, Grady CL, Goshen-Gottstein Y, Moscovitch M. Neuroimaging the serial position curve. Psychol. Sci. 2005; 16 :716–723. [ PubMed ] [ Google Scholar ]
- Thompson-Schill SL, D’Esposito M, Aguirre GK, Farah MJ. Role of left inferior prefrontal cortex in retrieval of semantic knowledge: a reevaluation. Proc. Natl. Acad. Sci. USA. 1997; 94 :14792–14797. [ PMC free article ] [ PubMed ] [ Google Scholar ]
- Thompson-Schill SL, Jonides J, Marshuetz C, Smith EE, D’Esposito M, et al. Effects of frontal lobe damage on interference effects in working memory. J. Cogn. Affect. Behav. Neurosci. 2002; 2 :109–120. [ PubMed ] [ Google Scholar ]
- Todd JJ, Marois R. Capacity limit of visual short-term memory in human posterior parietal cortex. Nature. 2004; 428 (6984):751–754. [ PubMed ] [ Google Scholar ]
- Todd JJ, Marois R. Posterior parietal cortex activity predicts individual differences in visual short-term memory capacity. Cogn. Affect. Behav. Neurosci. 2005; 5 :144–155. [ PubMed ] [ Google Scholar ]
- Trick LM, Pylyshyn ZW. What enumeration studies can show us about spatial attention—evidence for limited capacity preattentive processing. J. Exp. Psychol.: Hum. Percept. Perform. 1993; 19 (2):331–351. [ PubMed ] [ Google Scholar ]
- Ungerleider LG, Haxby JV. “What” and “where” in the human brain. Curr. Opin. Neurobiol. 1994; 4 :157–165. [ PubMed ] [ Google Scholar ]
- Unsworth N, Engle RW. The nature of individual differences in working memory capacity: active maintenance in primary memory and controlled search from secondary memory. Psychol. Rev. 2007; 114 :104–132. [ PubMed ] [ Google Scholar ]
- Vallar G, Baddeley AD. Fractionation of working memory: neuropsychological evidence for a phonological short-term store. J. Verbal Learn. Verbal Behav. 1984; 23 :151–161. [ Google Scholar ]
- Vallar G, Papagno C. Neuropsychological impairments of verbal short-term memory. In: Baddeley AD, Kopelman MD, Wilson BA, editors. The Handbook of Memory Disorders. 2nd ed. Chichester, UK: Wiley; 2002. pp. 249–270. [ Google Scholar ]
- Verhaeghen P, Basak C. Aging and switching of the focus of attention in working memory: results from a modified N-Back task. Q. J. Exp. Psychol. A. 2007 In press. [ PubMed ] [ Google Scholar ]
- Verhaeghen P, Cerella J, Basak C. A working memory workout: how to expand the focus of serial attention from one to four items in 10 hours or less. J. Exp. Psychol.: Learn. Mem. Cogn. 2004; 30 :1322–1337. [ PubMed ] [ Google Scholar ]
- Vogel EK, Machizawa MG. Neural activity predicts individual differences in visual working memory capacity. Nature. 2004; 426 :748–751. [ PubMed ] [ Google Scholar ]
- Vogel EK, Woodman GF, Luck SJ. The time course of consolidation in visual working memory. J. Exp. Psychol.: Hum. Percept. Perform. 2006; 32 :1436–1451. [ PubMed ] [ Google Scholar ]
- Wager TD, Smith EE. Neuroimaging studies of working memory: a meta-analysis. Neuroimage. 2003; 3 :255–274. [ PubMed ] [ Google Scholar ]
- Warrington EK, Shallice T. The selective impairment of auditory verbal short-term memory. Brain. 1969; 92 :885–896. [ PubMed ] [ Google Scholar ]
- Waugh NC, Norman DA. Primary memory. Psychol. Rev. 1965; 72 :89–104. [ PubMed ] [ Google Scholar ]
- Wheeler ME, Peterson SE, Buckner RL. Memory’s echo: vivid remembering reactivates sensory-specific cortex. Proc. Natl. Acad. Sci. USA. 2000; 97 (20):11125–11129. [ PMC free article ] [ PubMed ] [ Google Scholar ]
- Wheeler ME, Shulman GL, Buckner RL, Miezin FM, Velanova K, Petersen SE. Evidence for separate perceptual reactivation and search processes during remembering. Cereb. Cortex. 2006; 16 (7):949–959. [ PubMed ] [ Google Scholar ]
- Wickens DD. Encoding categories of words—empirical approach to meaning. Psychol. Rev. 1970; 77 :1–15. [ Google Scholar ]
- Wilken P, Ma WJ. A detection theory account of change detection. J. Vis. 2004; 4 :1120–1135. [ PubMed ] [ Google Scholar ]
- Wilson FAW, O’Scalaidhe SP, Goldman-Rakic PS. Dissociation of object and spatial processing domains in primate prefrontal cortex. Science. 1993; 260 :1955–1958. [ PubMed ] [ Google Scholar ]
- Wixted JT. The psychology and neuroscience of forgetting. Annu. Rev. Psychol. 2004; 55 :235–269. [ PubMed ] [ Google Scholar ]
- Woodman GF, Vogel EK, Luck SJ. Attention is not unitary. Behav. Brain Sci. 2001; 24 (1):153. [ Google Scholar ]
- Xu YD, Chun MM. Dissociable neural mechanisms supporting visual short-term memory for objects. Nature. 2006; 440 :91–95. [ PubMed ] [ Google Scholar ]
- Yantis S, Serences JT. Cortical mechanisms of space-based and object-based attentional control. Curr. Opin. Neurobiol. 2003; 13 :187–193. [ PubMed ] [ Google Scholar ]
- Zhang D, Zhang X, Sun X, Li Z, Wang Z, et al. Cross-modal temporal order memory for auditory digits and visual locations: an fMRI study. Hum. Brain Mapp. 2004; 22 :280–289. [ PMC free article ] [ PubMed ] [ Google Scholar ]
- Zucker RS, Regehr WG. Short-term synaptic plasticity. Annu. Rev. Physiol. 2002; 64 :355–405. [ PubMed ] [ Google Scholar ]
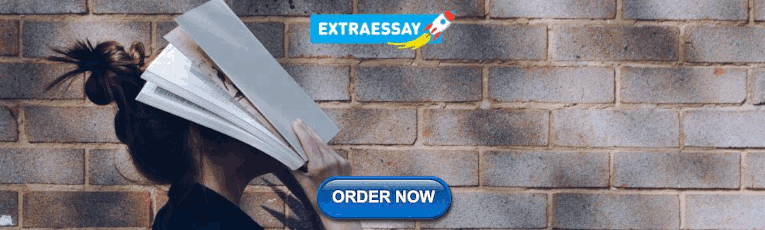
IMAGES
VIDEO
COMMENTS
Although research has suggested that episodic buffer is localized to the hippocampus (Berlingeri et al., 2008) or the inferior lateral parietal cortex, it is thought to be not dependent on a single anatomical structure but instead can be influenced by the subsystems of working memory, long term memory, and even through perception (Vilberg and ...
Long-term memory (LTM) associations appear as important to cognition as single memory contents. Previous studies on updating development have focused on cognitive processes and components, whereas our investigation examines how contents, associated with different LTM strength (strong or weak), might be differentially updated at different ages. To this end, we manipulated association strength ...
RSS Feed. Long-term memory is information encoded in the brain on the time-scale of years. It consists of explicit (declarative) memories that are consciously reportable and depend heavily on the ...
An Embedded-Processes Model of Working Memory. Notwithstanding the widespread use of the multicomponent working memory model, Cowan (1999, 2005) proposed the embedded-processes model that highlights the roles of long-term memory and attention in facilitating working memory functioning.Arguing that the Baddeley and Hitch (1974) model simplified perceptual processing of information presentation ...
The reviewed studies indicate that stress does impair retrieval, particularly when induced with the TSST, in the afternoon, up to 45 minutes before the onset of the final memory test, in healthy young men. These results may inform future research on the impact of stress-induced cortisol surges on memory retrieval.
At the molecular level, long term memories are formed by a process called cellular consolidation, which induces long lasting changes in synaptic contacts between memory ensemble neurons (Fig. 1a ...
a system with an unlimited capacity that lasts for years. Short term memory. Also known as working memory, it is considered to. be the recording of conscious thought in humans. It. refers to the ...
An emerging area of research is focused on the relationship between working memory and long-term memory and the likely overlap between these processes. Of particular interest is how some information first maintained in working memory is retained for longer periods and eventually preserved in long-term memory. The process of stabilizing transient memory representations for lasting retention is ...
Previous research has characterized a capacity-limited working memory (WM) store for brief maintenance of information and an unlimited long-term memory (LTM) store for sustained maintenance of ...
Both short- and long-term memories decline with healthy ageing. The aims of the current study were twofold: firstly, to build on previous studies and investigate the presence of a relationship between short- and long-term memories and, secondly, to examine cross-sectionally whether there are changes in this relationship with age. In two experiments, participants across the age range were ...
A new study by a team of international researchers has uncovered a biological explanation for long-term memories. It centers on the discovery of the role of a molecule, KIBRA, that serves as a ...
A new study in the journal Science Advances, conducted by a team of international researchers, has uncovered a biological explanation for long-term memories.It centers on the discovery of the role ...
Study sheds light on how neurons form long-term memories. On a late summer day in 1953, a young man who would soon be known as patient H.M. underwent experimental surgery. In an attempt to treat his debilitating seizures, a surgeon removed portions of his brain, including part of a structure called the hippocampus. The seizures stopped.
In a second discussion paper (Slotnick, this issue), a selective review was conducted to identify whether any fMRI studies have provided evidence that the hippocampus is associated with working memory. Because long-term memory processes can operate during the study phase, early delay phase (due to extended encoding), or test phase, it was ...
Long-term memory is a core aspect of human learning that permits a wide range of skills and behaviors often important for survival. While this core ability has been broadly observed for procedural and declarative memory, whether similar mechanisms subserve basic sensory or perceptual processes remains unclear.
1. Introduction. Implicit memory does not involve explicit or conscious recollection and is inferred from behavioral performance (Graf and Schacter, 1985, Roediger, 2012, Schacter, 1987).Mitchell (2006) published a report demonstrating that implicit memory could be preserved on the very long-term and over a much longer time period than the several months that had been previously investigated ...
A flavor of the claims made in some neuroimaging papers can be found in the following quotations: "This result implies that activated long-term memory provides a representational basis for semantic verbal short-term memory, and hence supports theories that postulate that short- and long-term stores are not separate" (Cameron, Haarmann ...
INTRODUCTION. The relationship between the properties of a training session and the strength and duration of the resultant memory trace has been a focus of psychological and neurobiological research since the late 1800s (Ebbinghaus 1913).Exposure to a few training stimuli can lead to long-lasting memories in organisms with even the simplest of nervous systems such as nematodes (Rankin et al ...
View PDF Abstract: Revolutionary advancements in Large Language Models have drastically reshaped our interactions with artificial intelligence systems. Despite this, a notable hindrance remains-the deficiency of a long-term memory mechanism within these models. This shortfall becomes increasingly evident in situations demanding sustained interaction, such as personal companion systems and ...
The majority of research on visual memory has taken a compartmentalized approach, focusing exclusively on memory over shorter or longer durations, that is, visual working memory (VWM) or visual ...
Originally based entirely on introspection (e.g., James, 1890 ), the idea that there are separate long- and short-term. memory (LTM and STM, respectively) systems subsequently be-. came a core ...
Psychologists have found that memory includes three important categories: sensory, short-term, and long-term. Each of these kinds of memory have different attributes, for example, sensory memory is not consciously controlled, short-term memory can only hold limited information, and long-term memory can store an indefinite amount of information.
We briefly review Hochreiter's (1991) analysis of this problem, then address it by introducing a novel, efficient, gradient-based method called long short-term memory (LSTM). Truncating the ...
Ever since I had a stroke five years ago, I have worried about dementia. I remember a task, then immediately forget about it. Water is poured, then left. Rooms are entered, then I can't remember ...
Specifically, this paper proposes machine learning algorithms based on decision trees, K-nearest neighbors, gradient boosting, and long-term memory networks for estimating the energy consumption ...
This review, in addition, identifies declarative memory as a system that appears to function relatively well in SLI. Declarative memory is a powerful and flexible long-term memory system that can have a compensatory role in language learning (Ullman & Pierpont, 2005). This information provides an evidenced-based rationale for existing clinical ...
Traditional machine learning techniques are employed by researchers for stock price prediction, but due to shallow architecture, adequate accuracy is not achieved. In this paper, recently introduced bidirectional encoder representations from transformers (BERT) and long short-term memory (LSTM) hybrid model is utilised for stock price prediction.
First, we examine the evidence for the architecture of short-term memory, with special attention to questions of capacity and how—or whether—short-term memory can be separated from long-term memory. Second, we ask how the components of that architecture enact processes of encoding, maintenance, and retrieval. Third, we describe the debate ...