Thank you for visiting nature.com. You are using a browser version with limited support for CSS. To obtain the best experience, we recommend you use a more up to date browser (or turn off compatibility mode in Internet Explorer). In the meantime, to ensure continued support, we are displaying the site without styles and JavaScript.
- View all journals
- My Account Login
- Explore content
- About the journal
- Publish with us
- Sign up for alerts
- Open access
- Published: 16 September 2022
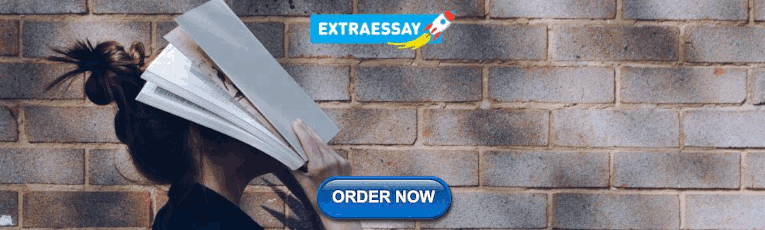
Human and economic impacts of natural disasters: can we trust the global data?
- Rebecca Louise Jones ORCID: orcid.org/0000-0002-2099-6461 1 , 2 ,
- Debarati Guha-Sapir 3 &
- Sandy Tubeuf ORCID: orcid.org/0000-0001-9001-1157 1 , 2
Scientific Data volume 9 , Article number: 572 ( 2022 ) Cite this article
11k Accesses
30 Citations
31 Altmetric
Metrics details
- Natural hazards
Reliable and complete data held in disaster databases are imperative to inform effective disaster preparedness and mitigation policies. Nonetheless, disaster databases are highly prone to missingness. In this article, we conduct a missing data diagnosis of the widely-cited, global disaster database, the Emergency Events Database (EM-DAT) to identify the extent and potential determinants of missing data within EM-DAT. In addition, through a review of prominent empirical literature, we contextualise how missing data within EM-DAT has been handled previously. A large proportion of missing data was identified for disasters attributed to natural hazards occurring between 1990 and 2020, particularly on the economic losses. The year the disaster occurred, income-classification of the affected country and disaster type were all significant predictors of missingness for key human and economic loss variables. Accordingly, data are unlikely to be missing completely at random. Advanced statistical methods to handle missing data are thus warranted when analysing disaster data to minimise the risk of biasing statistical inferences and to ensure global disaster data can be trusted.
Similar content being viewed by others
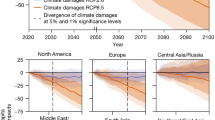
The economic commitment of climate change
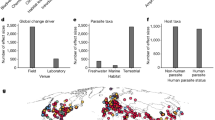
A meta-analysis on global change drivers and the risk of infectious disease
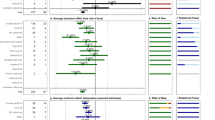
Systematic review and meta-analysis of ex-post evaluations on the effectiveness of carbon pricing
Introduction.
As the global effects of climate change are felt more intensively, so too are the human and economic consequences of catastrophic disaster events. In 2020 alone, disaster events attributed to natural hazards affected approximately 100 million people, accounted for an estimated 190 billion US$ of global economic losses and resulted in 15,082 deaths 1 , 2 . In light of COP-26 and recent topical events including, but certainly not limited to, the Haitian Earthquake (2021), Central European Floods (2020) and Australian Bushfires (2020), a renewed urgency has been granted to the research of, preparedness to and mitigation of disasters attributed to natural hazards.
Comprehensive historical data held in disaster databases are central for numerous purposes across both the public and private domain to inform emergency disaster relief management; configure catastrophe risk assessment models; and conduct cost-benefit analyses of disaster risk reduction policies 3 . However, inconsistencies in the reporting of disaster events and methodological difficulties quantifying their impacts, mean that disaster databases are prone to gaps in data availability 3 , 4 . As a result, the scope and reliability of statistical inferences which can be made from disaster data are reduced 5 . Systematic reporting of disaster events is required to minimise missing data. However, to achieve this is a major challenge. Instead, the use of valid and often complex statistical methods to account for missing data are required 6 , 7 .
Missing data is a common issue across all research areas. Standard practices are adopted in randomised clinical trials to combat patient attrition 8 , 9 and in survey-based observational studies to handle unit- and item-non response 10 , 11 . However, within the disaster literature, there is little insight as to how missing data should be handled.
To date, there are six disaster databases which have global coverage: the Emergency Events Database (EM-DAT); NatCatSERVICE; Sigma; GLIDE; GFDRR; and BD CATNAT Global 12 . This analysis utilises EM-DAT data alone, as it is the only publicly available, global disaster database and is widely cited; an initial search of the terms: ‘EM-DAT’, ‘CRED’, ‘Emergency Events Database’ and ‘International Disaster Database’ in Google Scholar returned 21,000 search results spanning numerous disciplines. EM-DAT was founded in 1988 by the Université catholique de Louvain (Belgium) with support from the United States Agency for International Development (USAID), the World Health Organisation (WHO) and the Belgium Government 13 . Data are collated from sources including the United Nations, reinsurance firms, research institutions and the press. As well as reporting the occurrence of major disastrous events attributed to natural, technological and complex hazards, its meta-data captures the human and economic impacts to assist national and international humanitarian action.
In this study, we conduct a missing data diagnosis to assess the extent of missing data in EM-DAT and to identify observable factors associated with missingness. We restrict our analysis to disaster events attributed to natural hazards occurring between the years 1990 and 2020. In addition, through a review of highly-cited empirical literature utilising EM-DAT data, we illustrate how missing data has previously been dealt with. We conclude by providing a discussion on the potential methods to handle missing data within disaster databases.
The state of missing data in EM-DAT
The advent of digital technologies in academia and research from the 1980s initiated a proliferation in the collection of data and subsequently, the adoption of data governance and data quality tools to ensure its reliability 14 . In the disaster space, technological advances in disaster surveillance and a progressive global agenda towards standardising disaster reporting, embedded in the 2015–2030 Sendai framework 15 , favour a climate for complete data reporting. Despite this, there was a high proportion of missing data in EM-DAT for disaster events attributed to natural hazards occurring between 1990 and 2020 (Fig. 1 ). This was particularly evident for the reporting of economic losses: data were missing for 96.2% of disaster events on reconstruction costs, for 88.1% on insured damages and 41.5% on total estimated damages. In the three months following a disaster event, when EM-DAT collates the majority of its data, precise information on reconstruction costs and insured damages is likely to be sparse. Reporting of human losses were more complete, with proportions of missing data ranging from 1.3% to 22.3%. Given that the volume of external disaster aid hinges predominantly on death tolls and injury counts, this finding is unsurprising. Of note, there were substantial inconsistencies in the reporting of both human and economic losses, where in both cases, aggregate variables (total deaths and total estimated damages) were better informed. In particular, missing data on total deaths were negligible (1.3%). Hence, in this case, there is little risk of missing data biasing statistical inferences.
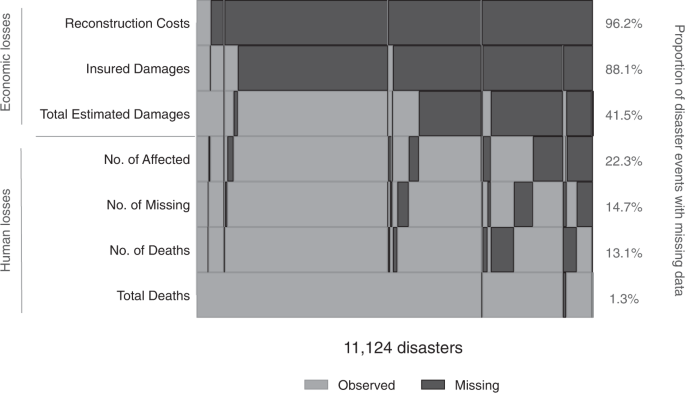
Missing data patterns and proportions of missing data for key human and economic loss variables in EM-DAT across all disaster events attributed to natural hazards and occurring between 1990 and 2020. Black shading denotes missing data for one or more disaster events; grey shading denotes observed data. Disaster events are ordered along the x-axis by the STATA default: from the least to the most missing across the variables analysed. The proportion of missing data for each variable, given as a percentage of the total data, is shown to the right-hand side of the figure. Variables are presented in descending order of missingness. Human loss variables include No. of Affected, defined as the number of people requiring immediate assistance during a period of emergency; No. of Missing, defined as the number of people whose whereabouts is unknown and who are presumed dead; No. of Deaths, defined as the number of people who lost their lives as a result of the disaster event; and Total Deaths, defined as the sum of No. of Affected and No. of Deaths. Economic loss variables include Reconstruction Costs, defined as the costs incurred due to the replacement of lost assets and the implementation of disaster mitigation measures; Insured Damages, defined as economic losses born by the insurance sector; and Total Estimated Damages, defined as a value of all economic losses directly or indirectly related to the disaster event.
It is important to distinguish not only the extent, but the pattern of missing data to inform the complexity of missingness 16 . For a small proportion of disaster events, data were missing across all variables analysed, denoted in Fig. 1 by the black, continuous vertical band along the right-hand side. More commonly, data were observed for at least one human loss variable and one economic loss variable. For human losses, missing information may be informed through the interdependence of human loss variables; EM-DAT defines total deaths as the sum of the number of people affected and the number of deaths 17 . Whereas for economic losses, there is no such interdependence across economic loss variables. EM-DAT defines total estimated damages as a value of all the economic losses directly or indirectly related to the disaster event, which does not necessarily include reconstruction costs. Missing data occurred sporadically throughout the dataset for each human loss variable, demonstrating an intermittent missing data pattern. However, across the economic loss variables, missing data patterns were less sporadic. For instance, data on reconstruction costs and insured damages were largely missing throughout, even when data on total estimated damages were informed.
Explaining missing data
Logistic regression analysis was used to identify whether the probability of data to be missing was dependent on observed data within EM-DAT 5 , 18 . Accordingly, it informs the nature of the missing data mechanism, specifically whether the mechanism of missing data deviates from Missing Completely At Random (MCAR). Data which are MCAR, that is, their probability to be missing is independent of observed and unobserved data, can be excluded from analyses with little risk of introducing bias. Conversely, if the missing data mechanism deviates from MCAR, the deletion of missing values may bias statistical inferences. In this case, more advanced methods are necessary to account for data gaps 6 . Here, we restricted our logistic regression analysis to model the probability of missingness within the variables: total estimated damages, the number of people affected, the number of people missing and the number of deaths (Supplementary Table 1 ). Methods to account for missing data are viable only for partially incomplete data 5 . In the case of reconstruction costs and insured damages, where data were almost entirely incomplete in our dataset (96.2% and 88.1% missing respectively) little can be done during data analysis to account for missingness. Hence, these variables were not investigated in the logistic regression analysis. The opposite was true for total deaths; the proportion of missing data on total deaths was negligible (1.3%). This precludes logistic regression analysis due to insufficient variation in the outcome variable, the probability to be missing. As a result, the variable total deaths was not investigated in the logistic regression analysis.
The observable data partially explained the probability of total estimated damages to be missing, revealed by a sizeable pseudo-R 2 value (pseudo-R 2 = 0.416) (Supplementary Table 1 ). Missing data on the number of people affected and the number of deaths were explained less by the observed data (pseudo-R 2 = 0.206 and pseudo-R 2 = 0.188 respectively) (Supplementary Table 2 ). This agrees with the intermittent missing data pattern identified for these variables (Fig. 1 ). The observed data explained much of the probability to be missing for the variable, the number of people missing (pseudo-R 2 = 0.621) (Supplementary Table 2 ). However, a large number of disaster events (n = 6,218) were omitted from the analysis by STATA, by default, due to occurring in years which perfectly predicted the probability to be missing. As a consequence, the precision of the parameter estimates is reduced, which requires the results to be interpreted with caution.
A number of observable factors in EM-DAT were statistically significantly (p < 0.05) associated to the probability of total estimated damages to be missing: the year the disaster occured, the income-group classification of the affected country, disaster severity and disaster type (Supplementary Table 1 ). Disaster events occurring after the year 2002 and in lower-income countries were positive predictors of the probability of data on total estimated damages to be missing. In addition, disaster severity, estimated by the natural logarithm of total deaths, and disaster types having prolonged effects including droughts, epidemics and extreme temperature events were also positive predictors of missingness.
The observed predictors of missingness differed for human loss variables. In contrast to missingness within the variable total estimated damages, the probability of data to be missing for the variables: the number of people affected and the number of deaths were statistically significantly (p < 0.01) lower for disasters which occurred in lower-income countries relative to high-income countries. Lower-income countries are the predominant recipients of international disaster aid. This result suggests that disaster aid incentivises more complete reporting of human losses. The influence of disaster type on the probability to be missing was largely heterogeneous across each variable analysed. In contrast, associations between the year the disaster occurred and the probability of data to be missing were similar across human and economic loss variables, with a higher probability of missingness for disaster events occurring after 2002.
Shortfalls of the current literature
The absence of published, standard procedures to account for missing data in disaster databases has set a precedent for a general lack of consideration of the issue in the empirical literature. We exemplify this by reviewing the top-20 most cited empirical studies utilising EM-DAT as a primary or secondary data source (Supplementary Table 3 ).
Eight studies neglected to mention missing data. Of the studies that did, missing data were often considered more broadly under the remit of data availability. With the exception of Brooks, Adger and Kelly 19 who assessed missing data in-depth in an accompanying paper, mention of missing data or data availability more generally, was limited to one or two sentences.
All disaster events meeting at least one of the following inclusion criteria are included in EM-DAT: i) ten or more people reported dead; ii) 100 or more people affected, injured or homeless; iii) a declaration of a state of emergency by the affected country, or an appeal for international assistance. Accordingly, for disaster events which meet these criteria, data on disaster occurrences are entirely observed in EM-DAT. As such, the extent to which missing data need be considered is dictated by the type of EM-DAT data utilised in empirical studies, namely whether data are utilised on disaster occurrence alone, or on the consequences.
Statistical methods utilised during data analysis typically presume complete information for all variables specified in the model. Therefore, assumptions on the mechanism of missing data are made, whether explicitly or not. Neither the publication year, nor the number of times a paper had been cited affected the approach taken to handle missing data. Of the studies reviewed, half attempted to account for missing data. However, the approaches employed were mostly ad-hoc with no statistical grounding. In six studies, authors restricted their analysis to variables or subsets of data with improved data availability. In addition to restricting the scope of their analysis, Brooks, Adger and Kelly 19 imputed zero values in place of missing values. However, this approach may heavily skew the distribution of data. Dilley et al . 20 aggregated mortality estimates over all disaster events attributed to a natural hazard between 1981 and 2000. This method intends to reduce the relative impact of missing data. Nevertheless, it also compromises the precision of analyses. Barredo 21 and Doocy et al . 22 supplemented EM-DAT data with data from alternative sources in an attempt to generate a more complete dataset. However, merging of datasets can be time-consuming and can itself introduce ‘file matching’ biases if datasets are heterogeneous in their structure or content 16 . Yang 23 utilised mean imputation whereby missing values are imputed with a single, unconditional mean of the observed values. Nevertheless, this method is generally not recommended, as the uncertainty in the predicted values due to missing data are not adequately reflected 24 .
Handling missing data in disaster databases
Identifying a suitable approach to handle missing data will depend on the characteristics of the dataset, the variables of interest and the intended data analysis. Regardless, the choice of missing data method should be grounded on plausible assumptions about the missing data mechanisms as informed through a diagnosis of the missing data. In Supplementary Table 4 , we describe conventional and advanced missing data methods which are relevant to handling missing data within disaster databases. An exhaustive review of the various missing data methods is beyond the scope of this paper and can be found elsewhere, see Allison 24 , Graham 25 and Graham, Patricio and Allison 26 .
Two broad approaches to handle missing data exist: deletion and imputation. Deletion methods, which are so-called ‘quick-fix’ methods, include column deletion, Complete Case Analysis (CCA) and Available Case Analysis (ACA). However, these methods can exclude a large proportion of the original dataset when a high proportion of missing data exists. In addition, deletion methods generally rely on missing data to be MCAR. Hence, when the mechanism of missingness deviates from this assumption, these methods can do more harm than good, biasing statistical inferences reported by studies 7 . Imputation-based methods are of two main varieties: single imputation and multiple imputation. Single imputation methods, such as mean imputation and regression-based imputation replace missing values with a single, predicted value estimated from the observed data. In contrast, multiple imputation generates a range of predicted values through multiply imputed datasets and thus, adequately reflects the uncertainty in the predicted values due to missing data 26 . Other advanced missing data methods include inverse probability weighting, maximum likelihood and Bayesian simulation. In addition, missing data methods can be combined. For instance, Bayesian techniques can be incorporated into the first step of multiple imputation.
Neglecting the issue of missing data exposes studies which inform regional, national and international disaster policies to biases and compromises their effectiveness. The complete, peer-reviewed reporting of disaster events through strengthened field-level data quality remains the eventual goal. In the meantime, given the data available, we argue that to achieve reliable evidence-based disaster policy, it is crucial that missing data is appropriately diagnosed and accounted for in the empirical literature.
This analysis is one of the first to reveal deviations from the assumption of MCAR for missing data on key human and economic loss variables in EM-DAT. Consequently, methods to handle missing data which are MAR should be considered. Deviations from the assumption of MAR, namely that missing data are Missing Not At Random (MNAR) and are thus dependent on unobserved data, cannot be explicitly tested. Instead, sensitivity analyses should be performed to test the robustness of parameter estimates to different assumptions about the mechanisms of missing data. Advanced missing data methods such as multiple imputation, maximum-likelihood and Bayesian simulation can be adapted to account for missing data which are MNAR.
In addition, this analysis highlights key limitations of EM-DAT data. Despite technological advances in disaster surveillance and general progress in data collection, an increase in the proportions of missing data since 2002 suggest shortfalls in current data quality procedures. More-so, the large disparities in the reporting of human losses compared to economic losses points to a wider issue in the types of data collected. Count data in physical units (number of deaths, number of people affected etc.) is principally used to portray human losses, whereas monetary estimates (reconstruction costs, insured damages etc.) are used to denote economic losses. Direct inference of monetary estimates is not straightforward. A two-stage valuation approach may therefore be more suitable and limit the extent of missing data on economic losses. In this case, count data, for instance the number of buildings damaged, the number of days without electricity, would primarily be used to evaluate economic losses in disaster databases. Standard economic valuation methods could then be applied, where necessary, to transform these values into monetary estimates.
By examining the global disaster database EM-DAT, this paper highlights shortfalls in the quality and use of disaster data which are rarely considered. Limitations in the quality of disaster databases arising from data collection procedures have been explored in previous literature 3 , 4 , 27 , 28 . In particular, limitations in the availability of data on low-intensity disaster events due to the inclusion criteria specified by CRED are well known. Instead, this paper contributes a different perspective to the existing literature; given the data collected, to what extent is there an issue of missing data and how should it be handled to ensure global disaster data can be trusted? In this respect, we aim to prompt the reader to apply a critical eye when assessing global disaster data, rather than implicitly trust its reliability.
The extent, complexity and mechanisms of missing data will vary according to the data analysed. Because of this, we are unable to prescribe a single approach to handle missing data within disaster databases. Instead, we advocate for analysts to consider missing data on an individual study basis. Although this article is a step in the right direction, a considerable research effort is still required to inform how missing data within disaster databases should be handled. In particular, the mechanisms of missing data in EM-DAT, and other disaster databases, should be more clearly identified. This would allow data limitations to be better informed and the performance of statistical methods, grounded on plausible assumptions about the mechanisms of missing data, to be tested.
Missing data diagnosis
As per procedures initially employed by Faria et al . 18 and Gabrio et al . 29 , but adapted to the context of disaster databases, this missing data diagnosis comprised three steps; i) a description of the proportions of missing data; ii) an analysis of the patterns of missing data; and iii) a logistic regression analysis to assess associations between the probability to be missing and observed values in the dataset. The dataset was restricted to disasters attributed to natural hazards and occurring between the years 1990 and 2020. Data analysis was carried out in STATA (version 16.1) and graphics were produced using the scheme ‘cleanplots’ 30 . The code used for the data analysis can be found in the supplementary material ( Supplementary Material ).
The proportion of missing data was computed across all variables using the command ‘mdesc’ 31 . Missing data patterns were produced using the command ‘misspattern, novarsort’ 32 . Due to computational limits attributed to a large number of observations for each variable, the option ‘noidsort’ could not be applied. Accordingly, observations were arranged by the default: from the least to the most missing across the variables of interest. Missing data patterns were produced for all human loss variables in the dataset (number of people affected, number of people missing, number of deaths and total deaths) and select economic loss variables (reconstruction costs, insured damages and total estimated damages). While individual direct, indirect, sectoral and infrastructural losses were included in the dataset, these were omitted from visualisations of missing data patterns as they were rarely informed.
Statistical analyses, including the t-test, Pearson’s chi-squared test, Little’s MCRA test and binary probability models, are used to test the assumption that missing data is MAR. That is, missing data can be explained by observed values in the dataset 18 . Logistic regression analysis was used in this study to model the probability of missingness for a given disaster event i , conditional on a vector of observable predictors of missingness ( X i ) (Eq. 1 ). The outcome variable ( y i ) was therefore a dichotomous missing data indicator taking value 1 if data were missing, or 0 if data were informed. Missing data indicators were generated for the variables: total estimated damages, number of people affected, number of people missing and number of deaths.
with ε it denoting the error term and F (·) denoting the cumulative distribution function (cdf) which takes a standard logistic distribution (Eq. 2 ) in logistic regression analysis.
Observed predictors of missingness ( X i ) included disaster type, a categorical variable denoting the type of natural disaster, omitting the disaster type flood as the reference to control for multi-collinearity; income-classification of the affected country, omitting high-income as the reference category; and the year the disaster occurred, between 1990 and 2020, included in the model as a categorical variable with the year 1990 omitted as a reference. Reference categories were selected due to being the most frequently observed in the dataset. The year the disaster occurred was included in the model as fixed effects to assess both the trend in missing data over time and the individual threshold effects across each year. Disaster severity, estimated by the logarithm of total deaths, was also included in the model specification when the probability of total estimated damages to be missing was assessed. Otherwise, disaster severity was excluded from the model due to being highly correlated with the variables: number of people affected, number of people missing and number of deaths. Total deaths was log transformed to account for the left-skewed, binomial distribution, arising from the high proportion (60%) of zero reported deaths in the dataset. Observed predictors of missingness were selected based on a step-wise approach.
Logistic regression analysis was estimated by maximum likelihood estimation (Eq. 3 ) using the command ‘logistic’ for all disaster events having complete information on the predictors of missingness. Marginal effects were computed at the mean using the command ‘mfx’ to enable interpretation of the magnitude of effect in the probability scale.
The robustness of our results was assessed using a probit model and a conditional (fixed-effect) logit model. The latter was used to test for potential ‘incidental parameter’ bias arising from the inclusion of individual fixed effects.
Review of the literature
Disaster databases are highly prone to missingness. Despite this, little insight exists as to how missing data should be handled in this context. To inform previous approaches to handling missing data in disaster databases, empirical studies utilising EM-DAT as a primary or secondary data source were reviewed. As this review was conducted for illustrative purposes only, we sought only prominent, highly-cited studies since these typically shape the standards for subsequent research. Studies were identified through electronic database searches of Web of Science, Scopus, PubMed and Google Scholar on 01/06/2022 using the key search terms: ‘EM-DAT’, ‘Emergency Events Database’, ‘International Disaster Database’ and ‘CRED’. Only papers considered to be empirical in nature and published between 1990 and 2022 were considered. No language restrictions were applied. Of the initial 2,127 search results, 421 papers were deemed to meet the inclusion criteria. Of these, the top-20 most cited papers were identified. For each paper, data were extracted on the use of EM-DAT data, the consideration given to missing data and the approach taken to handle missing data 33 , 34 , 35 , 36 , 37 , 38 , 39 , 40 , 41 , 42 , 43 , 44 , 45 , 46 , 47 .
Data availability
The data utilised in this analysis were acquired from the Emergency Events Database (EM-DAT). Data from EM-DAT is publicly and freely available to download from: https://public.emdat.be/ . Restrictions apply to the dataset used in this analysis, which included additional data from that freely available. This was acquired by the authors on a direct request to the Centre for Research on the Epidemiology of Disasters (CRED). Data is however available from the corresponding author upon reasonable request and with the permission of CRED, which can be obtained via written request to Ms. Regina Below, the EM-DAT database manager, at [email protected].
Code availability
The code used to generate the results of this analysis are available as supplementary material. Data analysis was carried out in STATA (version 16.1).
International Federation of Red Cross and Red Crescent Societies, National Disaster Reduction Centre of China & Academy of Disaster Reduction and Emergency Management. 2020 Global Natural Disaster Assessment Report . https://www.preventionweb.net/publication/2020-global-natural-disaster-assessment-report (2021).
Bevere, L. Natural catastrophes in 2020. Swiss RE sigma https://www.swissre.com/institute/research/sigma-research/sigma-2021-01.html (2021).
Wirtz, A., Kron, W., Löw, P. & Steuer, M. The need for data: natural disasters and the challenges of database management. Nat. Hazards. 70 , 135–157 (2012).
Article Google Scholar
Guha-Sapir, D. & Below, R. Quality and accuracy of disaster data: A comparative analyse of 3 global data sets. CRED Work. Pap . 1–18 (2002).
Rubin, D. B. Inference and Missing Data. Oxford Univ. Press 63 , 581–592 (1976).
ADS MathSciNet MATH Google Scholar
Harrell, F. E. Regression Modeling Strategies 2nd edn (Springer International Publishing, 2015).
Schafer, J. L. & Graham, J. W. Missing Data: Our View of the State of the Art. Psychol. Methods. 7 , 147–177 (2002).
Schulz, K. F., Altman, D. G. & Moher, D. CONSORT 2010 statement: updated guidelines for reporting parallel group randomised trials. PLoS Med. 7 , 1–7 (2010).
Little, R. J. et al . The Prevention and Treatment of Missing Data in Clinical Trials. N. Engl. J. Med. 367 , 1355–1360 (2012).
Article CAS Google Scholar
Young, R. & Johnson, D. R. Handling Missing Values in Longitudinal Panel Data With Multiple Imputation. J. Marriage Fam. 77 , 277 (2015).
Vandenbroucke, J. P. et al . Strengthening the Reporting of Observational Studies in Epidemiology (STROBE): Explanation and Elaboration. PLOS Med. 4 , 297 (2007).
Mazhin, S. et al . Worldwide disaster loss and damage databases: A systematic review. J. Educ. Health Promot. 10 , 329 (2021).
Article PubMed PubMed Central Google Scholar
Cummings, W. S. In Economic Effects of Natural Disasters (ed. Chaiechi, T.) Ch.1 (Academic Press, 2020).
Foote, K. A Brief History of Data Quality. DATAVERSITY https://www.dataversity.net/a-brief-history-of-data-quality/# (2021).
United Nations Office for Disaster Risk Reduction (UNDRR). Sendai Framework for Disaster Risk Reduction 2015–2030. United Nations https://www.undrr.org/publication/sendai-framework-disaster-risk-reduction-2015-2030 (2015).
Little, R. J. A. & Rubin, D. B. Statistical analysis with missing data (Wiley, 2002).
Centre for Research on the Epidemiology of Disasters (CRED). EM-DAT Glossary https://www.emdat.be/Glossary (2021).
Faria, R., Gomes, M., Epstein, D. & White, I. R. A Guide to Handling Missing Data in Cost-Effectiveness Analysis Conducted Within Randomised Controlled Trials. Pharmacoeconomics. 32 , 1157–1170 (2014).
Brooks, N., Adger, W. N. & Kelly, P. M. The determinants of vulnerability and adaptive capacity at the national level and the implications for adaptation. Glob. Environ. Chang. 15 , 151–163 (2005).
Dilley, M., Chen, R. S., Deichmann, U., Lerner-Lam, A. L. & Arnold, M. Natural Disaster Hotspots: A Global Risk Analysis. World Bank https://openknowledge.worldbank.org/handle/10986/7376 (2005).
Barredo, J. I. Major flood disasters in Europe: 1950-2005. Nat. Hazards. 42 , 125–148 (2007).
Doocy, S., Daniels, A., Murray, S. & Kirsch, T. D. The Human Impact of Floods: a Historical Review of Events 1980-2009 and Systematic Literature Review. PLoS Curr . 5 (2013).
Yang, D. Coping with disaster: The impact of hurricanes on international financial flows, 1970–2002. B.E. J. Econ. Anal. Policy . 8 (2008).
Allison, P. D. In The Sage handbook of quantitative methods in pyschology (ed. Millsap, R. E.) Ch. 4 (Springer Publications Ltd., 2009).
Graham, J. W. Missing Data Analysis: Making It Work in the Real World. Annu. Rev. Psychol. 60 , 549–576 (2009).
Graham, J. W., Cumsille, P. E. & Shevock, A. E. In Handbook of Psychology Vol. 2 (ed. Weiner) Ch. 4 (Wiley, 2012).
Osuteye, E., Johnson, C. & Brown, D. The data gap: An analysis of data availability on disaster losses in sub-Saharan African cities. Int. J. Disaster Risk Reduct. 26 , 24–33 (2017).
Lin, Y. C., Khan, F., Jenkins, S. F. & Lallemant, D. Filling the Disaster Data Gap: Lessons from Cataloging Singapore’s Past Disasters. Int. J. Disaster Risk Sci. 12 , 188–204 (2021).
Gabrio, A., Mason, A. J. & Baio, G. Handling Missing Data in Within-Trial Cost-Effectiveness Analysis: A Review with Future Recommendations. Pharmacoeconomics. 1 , 79–97 (2017).
Mize, T. D. Source code for: Cleanplots: Stata graphics scheme. Trenton Mize https://www.trentonmize.com/software/cleanplots (2018).
Medeiros, R. A., Blanchette, D., Medeiros, R. A. & Blanchette, D. Source code for: MDESC: Stata module to tabulate prevalence of missing values. Statistical Software Components https://econpapers.repec.org/software/bocbocode/s457318.htm (2011).
White, I. Network meta-analysis. Stata J. 15 , 951–985 (2015).
Lesk, C., Rowhani, P. & Ramankutty, N. Influence of extreme weather disasters on global crop production. Nature. 529 , 84–87 (2016).
Article ADS CAS Google Scholar
Kahn, M. E. The Death Toll from Natural Disasters: The Role of Income, Geography, and Institutions. Rev. Econ. Stat. 87 , 271–284 (2005).
Noy, I. The macroeconomic consequences of disasters. J. Dev. Econ. 88 , 221–231 (2009).
Alcántara-Ayala, I. Geomorphology, natural hazards, vulnerability and prevention of natural disasters in developing countries. Geomorphology. 47 , 107–124 (2002).
Article ADS Google Scholar
Toya, H. & Skidmore, M. Economic development and the impacts of natural disasters. Econ. Lett. 94 , 20–25 (2007).
Jonkman, S. N. Global Perspectives on Loss of Human Life Caused by Floods. Nat. Hazards. 34 , 151–175 (2005).
Strömberg, D. Natural Disasters, Economic Development, and Humanitarian Aid. J. Econ. Perspect. 21 , 5–222 (2007).
Kovács, G. & Spens, K. Identifying challenges in humanitarian logistics. Int. J. Phys. Distrib. Logist. Manag. 39 , 506–528 (2009).
Barredo, J. I. Normalised flood losses in Europe: 1970-2006. Nat. Hazards Earth Syst. Sci. 9 , 97–104 (2009).
Nadim, F., Kjekstad, O., Peduzzi, P., Herold, C. & Jaedicke, C. Global landslide and avalanche hotspots. Landslides. 3 , 159–173 (2006).
Kellenberg, D. K. & Mobarak, A. M. Does rising income increase or decrease damage risk from natural disasters? J. Urban Econ. 63 , 788–802 (2008).
Deressa, T., Hassan, R. M. & Ringler, C. Measuring Ethiopian farmers’ vulnerability to climate change across regional states . IFPRI Discussion Paper 806 (International Food Policy Research Institute, 2008).
Raschky, P. A. Institutions and the losses from natural disasters. Nat. Hazards Earth Syst. Sci. 8 , 627–634 (2008).
Mohapatra, S., Joseph, G. & Ratha, D. Remittances and natural disasters: Ex-post response and contribution to ex-ante preparedness. Environ. Dev. Sustain. 14 , 365–387 (2012).
Kvaløy, B., Finseraas, H. & Listhaug, O. The publics’ concern for global warming: A cross-national study of 47 countries. J. Peace Res. 49 , 11–22 (2012).
Download references
Acknowledgements
The authors would like to thank the Université catholique de Louvain for their support on this research paper and the University of York who permitted R.L.J. to undertake part of this research as part of her Master’s dissertation. We would also like to thank the reviewers and editors at Scientific Data, who helped to shape the final version of this paper.
Author information
Authors and affiliations.
Institut de Recherche Santé Société (IRSS), Faculté de Santé Publique, Université catholique de Louvain, 1200 Woluwe-Saint-Lambert, Brussels, Belgium
Rebecca Louise Jones & Sandy Tubeuf
Institut de Recherches Économiques et Sociales (IRES), Université catholique de Louvain, 1348 Louvain-la-Neuve, Belgium
John Hopkins Bloomberg School of Public Health, Baltimore, Maryland, 21205, USA
Debarati Guha-Sapir
You can also search for this author in PubMed Google Scholar
Contributions
The study was conceptualised by S.T. and D.G.S. Data analysis was performed by R.L.J. under the supervision of S.T. All authors contributed to the writing and revision of the manuscript.
Corresponding author
Correspondence to Sandy Tubeuf .
Ethics declarations
Competing interests.
DGS founded the Emergency Events Database (EM-DAT) and contributed her expert knowledge on EM-DAT procedures and mechanics. RLJ and ST declare no competing interests.
Additional information
Publisher’s note Springer Nature remains neutral with regard to jurisdictional claims in published maps and institutional affiliations.
Supplementary information
Supplementary material table of contents, 41597_2022_1667_moesm2_esm.pdf.
Results of logistic regression analysis to test associations between the probability of data to be missing on total estimated damages and observable data in the Emergency Events Database (EM-DAT)
41597_2022_1667_MOESM3_ESM.pdf
Results of logistic regression analysis to test associations between the probability of data to be missing on select human loss variables and observable data in the Emergency Events Database (EM-DAT)
Consideration of missing data in pivotal empirical studies utilising the Emergency Events Database (EM-DAT)
Reference list, glossary of conventional and advanced missing data methods, rights and permissions.
Open Access This article is licensed under a Creative Commons Attribution 4.0 International License, which permits use, sharing, adaptation, distribution and reproduction in any medium or format, as long as you give appropriate credit to the original author(s) and the source, provide a link to the Creative Commons license, and indicate if changes were made. The images or other third party material in this article are included in the article’s Creative Commons license, unless indicated otherwise in a credit line to the material. If material is not included in the article’s Creative Commons license and your intended use is not permitted by statutory regulation or exceeds the permitted use, you will need to obtain permission directly from the copyright holder. To view a copy of this license, visit http://creativecommons.org/licenses/by/4.0/ .
Reprints and permissions
About this article
Cite this article.
Jones, R.L., Guha-Sapir, D. & Tubeuf, S. Human and economic impacts of natural disasters: can we trust the global data?. Sci Data 9 , 572 (2022). https://doi.org/10.1038/s41597-022-01667-x
Download citation
Received : 08 April 2022
Accepted : 26 August 2022
Published : 16 September 2022
DOI : https://doi.org/10.1038/s41597-022-01667-x
Share this article
Anyone you share the following link with will be able to read this content:
Sorry, a shareable link is not currently available for this article.
Provided by the Springer Nature SharedIt content-sharing initiative
This article is cited by
Exploring em-dat for depicting spatiotemporal trends of drought and wildfires and their connections with anthropogenic pressure.
- Michael Nones
- Hossein Hamidifar
- Seyed Mohammad Bagher Shahabi-Haghighi
Natural Hazards (2024)
Floods have become less deadly: an analysis of global flood fatalities 1975–2022
- S. N. Jonkman
- L. M. Bouwer
Evolutionary characteristics and influencing factors of wheat production risk in Gansu Province of China under the background of climate change
Theoretical and Applied Climatology (2024)
Towards an understanding of landslide risk assessment and its economic losses: a scientometric analysis
- Nini Johana Marín-Rodríguez
- Johnny Vega
- Sergio Botero
Landslides (2024)
The wider the gap between rich and poor the higher the flood mortality
- Sara Lindersson
- Elena Raffetti
- Giuliano Di Baldassarre
Nature Sustainability (2023)
Quick links
- Explore articles by subject
- Guide to authors
- Editorial policies
Sign up for the Nature Briefing newsletter — what matters in science, free to your inbox daily.

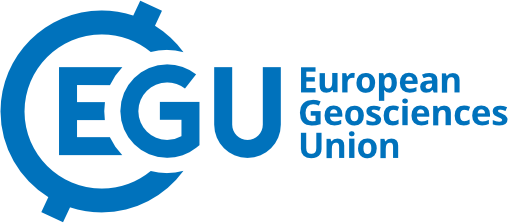
Article
- Volume 20, issue 4
- NHESS, 20, 1069–1096, 2020
- Peer review
- Related articles
- Global- and continental-scale risk assessment for natural...
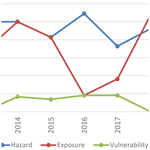
Review article: Natural hazard risk assessments at the global scale
Philip j. ward, veit blauhut, nadia bloemendaal, james e. daniell, marleen c. de ruiter, melanie j. duncan, robert emberson, susanna f. jenkins, dalia kirschbaum, michael kunz, susanna mohr, graeme a. riddell, andreas schäfer, thomas stanley, ted i. e. veldkamp, hessel c. winsemius.
Since 1990, natural hazards have led to over 1.6 million fatalities globally, and economic losses are estimated at an average of around USD 260–310 billion per year. The scientific and policy communities recognise the need to reduce these risks. As a result, the last decade has seen a rapid development of global models for assessing risk from natural hazards at the global scale. In this paper, we review the scientific literature on natural hazard risk assessments at the global scale, and we specifically examine whether and how they have examined future projections of hazard, exposure, and/or vulnerability. In doing so, we examine similarities and differences between the approaches taken across the different hazards, and we identify potential ways in which different hazard communities can learn from each other. For example, there are a number of global risk studies focusing on hydrological, climatological, and meteorological hazards that have included future projections and disaster risk reduction measures (in the case of floods), whereas fewer exist in the peer-reviewed literature for global studies related to geological hazards. On the other hand, studies of earthquake and tsunami risk are now using stochastic modelling approaches to allow for a fully probabilistic assessment of risk, which could benefit the modelling of risk from other hazards. Finally, we discuss opportunities for learning from methods and approaches being developed and applied to assess natural hazard risks at more continental or regional scales. Through this paper, we hope to encourage further dialogue on knowledge sharing between disciplines and communities working on different hazards and risk and at different spatial scales.
- Article (PDF, 1268 KB)
- Supplement (246 KB)
- Included in Encyclopedia of Geosciences
- Article (1268 KB)

Ward, P. J., Blauhut, V., Bloemendaal, N., Daniell, J. E., de Ruiter, M. C., Duncan, M. J., Emberson, R., Jenkins, S. F., Kirschbaum, D., Kunz, M., Mohr, S., Muis, S., Riddell, G. A., Schäfer, A., Stanley, T., Veldkamp, T. I. E., and Winsemius, H. C.: Review article: Natural hazard risk assessments at the global scale, Nat. Hazards Earth Syst. Sci., 20, 1069–1096, https://doi.org/10.5194/nhess-20-1069-2020, 2020.
The risk caused by natural hazards is extremely high and increasing. Since 1990, reported disasters have led to over 1.6 million fatalities globally, and economic losses are estimated at an average of around USD 260–310 billion per year (UNDRR, 2015a). The need to reduce the risk associated with natural hazards is recognised by the international community and is at the heart of the Sendai Framework for Disaster Risk Reduction (Sendai Framework; UNDRR, 2015b). The Sendai Framework adopts the conceptualisation of disaster risk as the product of hazard, exposure, and vulnerability. The hazard refers to the hazardous phenomena itself, such as a flood event, including its characteristics and probability of occurrence; exposure refers to the location of economic assets or people in a hazard-prone area; and vulnerability refers to the susceptibility of those assets or people to suffer damage and loss (e.g. due to unsafe housing and living conditions or lack of early warning procedures). Reducing risk is also recognised as a key aspect of sustainable development in the Sustainable Development Goals (SDGs) and the Paris Agreement on climate change.
Managing disaster risk requires an understanding of risk and its drivers, from household to global scales. This includes an understanding of how risk may change in the future and how that risk may be reduced through disaster risk reduction (DRR) efforts. A global-scale understanding of disaster risk is important for identifying regions most at risk, providing science-based information for DRR advocacy, and assessing the potential effectiveness of DRR solutions. Efforts to assess and map natural hazard risk at the global scale have been ongoing since the mid-2000s, starting with the natural disaster hotspots analysis of Dilley et al. (2005). This was followed by the global risk assessments for an increasing number of natural hazards in the biennial Global Assessment Reports (GARs) of the United Nations Office for Disaster Risk Reduction (UNDRR) (UNDRR, 2009, 2011, 2013, 2015a, 2017).
At the same time, there have been increasing efforts in the scientific community to develop global methods to assess the potential risks of natural hazards at the global scale, in order to inform (inter)national decision makers. In 2012, the session “Global and continental scale risk assessment for natural hazards: methods and practice” was established at the General Assembly of the European Geosciences Union, in order to bring together people and institutes working on large-scale risk assessment from different disciplinary communities. The session has been held each year since 2012, leading to the current special issue in Natural Hazards and Earth System Sciences . One of the enduring themes of these sessions has been assessing future natural hazard risk. In Fig. 1, we show the percentage of abstracts accepted to this session each year that explicitly mentions examining future projections of risk based on future scenarios of hazard, exposure, or vulnerability projections. The number of abstracts dealing with future scenarios of hazard and exposure is much higher than those dealing with future scenarios of vulnerability. Over the 8-year period that the session has run, scenarios of future hazards have been the most common aspect amongst those abstracts dealing with future risk projections.
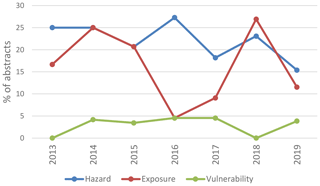
Figure 1 Percentage of abstracts accepted and presented at the EGU session “Global and continental scale risk assessment for natural hazards: methods and practice” that explicitly mention examining future projections of risk based on future scenarios of hazard, exposure, or vulnerability projections.
In this paper, we review the scientific literature on natural hazard risk assessments at the global scale, and we specifically examine whether and how they have examined future projections of hazard, exposure, and/or vulnerability. In doing so, we examine similarities and differences between the approaches taken across the different hazards, thereby identifying potential ways in which different hazard communities can learn from each other whilst acknowledging the challenges faced by the respective hazard communities. First, we review the scientific literature for each natural hazard risk individually. Second, we compare and contrast the state of the art across the different hazard types. Third, we conclude by discussing future research challenges faced by the global risk modelling community and several opportunities for addressing those challenges.
In this section, we review scientific literature on global-scale natural hazard risk assessments. We limit the review to those studies that have used a spatial representation of the risk elements. Therefore, we do not include studies that use global (or continental) damage functions to directly translate from a global stressor (e.g. global temperature change) to a loss or studies that solely use normalisation methods to assess trends in past reported losses. The results are presented for major natural hazards, including those that have been modelled for the UNDRR Global Assessment Reports.
In carrying out the review, we focus on the aspects described in the bullets below. For each of the reviewed studies, the information across these aspects is summarised in Table 1.
Risk elements . We indicate whether hazard, exposure, and vulnerability are explicitly represented in the study. If so, we indicate whether these are represented in a static or dynamic nature over time. By static, we mean that no future projections are included (i.e. only current representation is used), and by dynamic we mean that future projections are included. In the table, no representation is shown in white, static is in orange, and dynamic is in green.
Resolution of risk elements . We indicate the spatial resolution at which each risk element is represented.
Risk indicators . We show the indicators used to express the risk.
Future DRR measures . We indicate whether the study explicitly represents future DRR measures in its modelling framework, and if so we indicate whether these are related to structural, nature-based, or non-structural measures. We also indicate whether the costs of these measures are assessed and whether the impact of human behaviour on their effectiveness is assessed.
Risk analysis . We indicate the type of risk assessment that was carried out. We have classed these as either non-probabilistic (NP) or probabilistic (P). By probabilistic, we mean that expected annual impacts are assessed either by integrating across return periods or based on a probabilistic stochastic event set. We also indicate whether the risk assessment represents hazard using stochastic event sets (S), return period maps (R), maps of yearly (Y) or monthly (M) hazard, past events (PE), or the radius around a specific volcano (V). We also indicate the time horizon reported in the study, the resolution at which the risk analysis is carried out, and the geographical scale to which the results are aggregated.
In the following subsections, each hazard is reported individually.
Table 1 Summary of findings across the reviewed literature and across the reviewed aspects. Explanatory notes: risk elements – white if this risk element is not included, orange if included and static, green if included and dynamic. Types of risk analysis are as follows. NP: non-probabilistic; P: probabilistic; S: stochastic event sets; RP: return period maps; Y: maps of yearly hazard; M: maps of monthly hazard; PE: past events; V: radius around specific volcano; IDF: intensity–damage function.
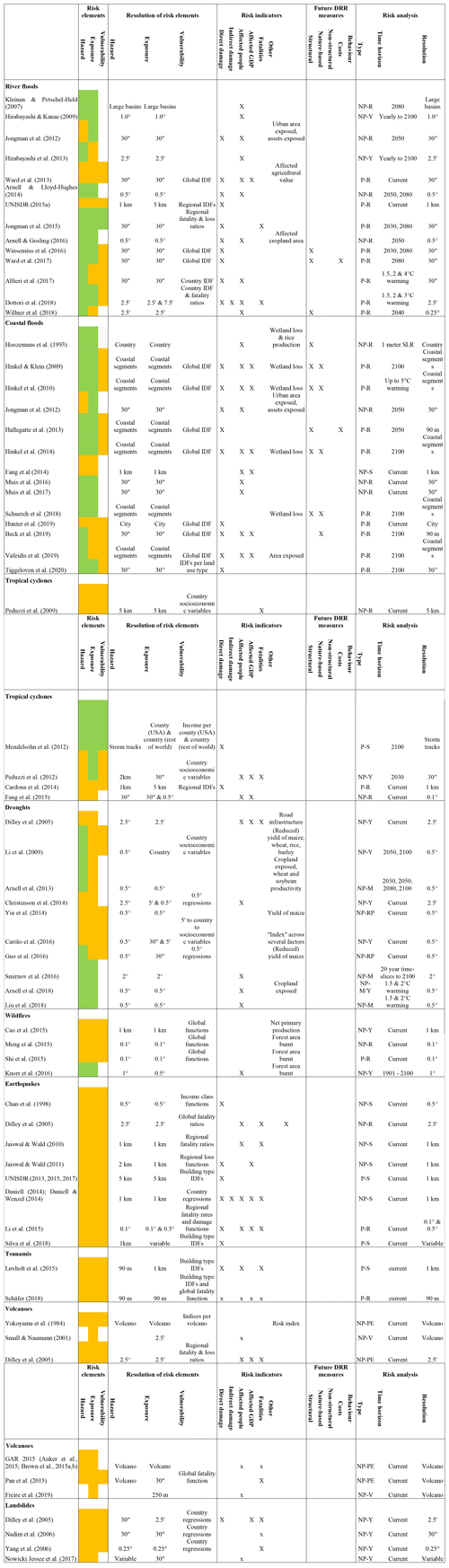
2.1 Floods
2.1.1 river floods.
A relatively large number of studies have been carried out to assess global risk from river floods. A description of each study and the main findings can be found in the Supplement. These studies are summarised in this section, and details of the reviewed aspects for each study are shown in Table 1.
Dilley et al. (2005) was the first study to overlay regions that had been affected by large floods between 1985 and 2003 with population data. Güneralp et al. (2015) used the same data to map potential changes in urban areas in flood-prone regions. However, the flood maps used only provide a general impression of regions affected by floods, but not necessarily actual inundated areas, and are therefore not included in Table 1. The earliest assessments of future river flood risk examine changes in the number of people experiencing discharge flows of different magnitudes, at coarse resolutions ranging from 0.5 ∘ × 0.5 ∘ to “large river basins” (Kleinen and Petschel-Held, 2007; Hirabayashi and Kanae, 2009; Arnell and Lloyd-Hughes, 2014; Arnell and Gosling, 2016). Whilst the numbers differ greatly between studies, they all reveal a large increase throughout the 20th century.
Since then, river flood risk modelling has progressed to examine flood hazard based on modelled inundation maps at resolutions varying from 30 ′′ × 30 ′′ to 2.5 ′ × 2.5 ′ . It should be noted that each of the models described here has its own minimum catchment size (ranging from ∼500 to ∼5000 km 2 ), under which hazard (and therefore risk) are not calculated. Several early studies only examined current risk (Ward et al., 2013; UNDRR, 2015a) or examined either dynamic hazard (Hirabayashi et al., 2013; Alfieri et al., 2017; Willner et al., 2018) or dynamic exposure (Jongman et al., 2012). Several of the most recent studies have used dynamic projections of both hazard and exposure (Winsemius et al., 2016; Ward et al., 2017; Dottori et al., 2018), whilst Jongman et al. (2015) also added dynamic vulnerability.
In terms of hazard modelling, there has been a clear movement from the coarse-resolution maps of extreme discharge to approaches using inundation maps of different return periods, whereby probabilistic risk is also assessed. There has been an overall shift from coarse exposure maps (0.5 ∘ × 0.5 ∘ to large river basins) of population only to higher-resolution maps with population, gross domestic product (GDP), and land use. This has accompanied a transition from addressing affected people only to moving towards a broader range of risk indicators, including direct damage and, in the case of Dottori et al. (2018), also indirect damage. In most studies where vulnerability has been considered, this has been done by using (one or a limited number of) intensity–damage functions (IDFs), namely depth–damage functions, whilst Jongman et al. (2015) and Dottori et al. (2018) have also used vulnerability ratios. The only study to develop dynamic vulnerability scenarios is that of Jongman et al. (2015).
There has also been a clear shift from studies using non-probabilistic approaches focusing on impacts in a given year or time period, or on one or several discrete return periods, to studies using a probabilistic approach. The latter studies have integrated impacts across a range of return periods to estimate risk in terms of expected annual impacts. To date, none of the global-scale studies use probabilistic stochastic event sets.
Planned future DRR measures have only been considered in the studies of Winsemius et al. (2016), Ward et al. (2017), and Willner et al. (2018) and only through structural measures. Of these studies, only Ward et al. (2017) assess the associated costs and benefits. No studies to date have assessed behavioural aspects of future DRR measures. All studies project huge increases in future absolute risk, assuming no future DRR measures, on the order of hundreds to thousands of percent depending on indicator, scenario, time periods, and study. However, the increases are significantly lower when expressed relative to population and/or GDP. Moreover, the more recent papers that include either change in vulnerability or future DRR measures show that much of the projected future risk could be reduced if effective DRR measures are taken, often with the benefits of these exceeding the costs.
2.1.2 Coastal floods
As with river floods, a relatively large number of studies have been carried out to assess global risk from coastal floods. Details of each study can, therefore, be found in the Supplement, and they are summarised in this section and in Table 1.
Pioneering work on global coastal flood risk was performed in the Global Vulnerability Assessment for the Intergovernmental Panel on Climate Change (IPCC) (Hoozemans et al., 1993). This study used empirical approaches to derive extreme sea levels for four different return periods using co-variables such as wind and pressure climatologies and bathymetry. These were combined with socio-economic data and scenarios, as well as cost estimates for increasing protection standards to estimate present-day and future risk and the costs of future DRR measures.
This method was extended in the European DINAS-COAST project, in which the Dynamic and Interactive Vulnerability Assessment (DIVA) model was developed. This model assesses coastal flood risks at a finer spatial resolution than Hoozemans et al. (1993) using coastal segments, whereby each segment represents a different coastal archetype (Vafeidis et al., 2008). In total, 12 148 coastal segments are defined, with the length of each section ranging from less than 1 to 5213 km, depending on physical and socio-economic conditions. For these segments, extreme sea levels were computed following Hoozemans et al. (1993) and combined with biophysical and socio-economic data along these coastal segments to enable assessment of risk in terms of affected coastal area and population, population forced to migrate, and damage based on a single depth–damage function. From the start, the DIVA model has explicitly represented the costs and benefits of future DRR measures, since it was developed to assist climate adaptation studies. DIVA has been used in several studies at a global scale, including those of Hinkel and Klein (2009) and Hinkel et al. (2010, 2014), to assess risks under scenarios of sea level rise, subsidence, and population growth, with several assumptions on future DRR measures. It has also been used by Hallegatte et al. (2013) to assess coastal flood risk and the costs and benefits of structural DRR measures in major coastal cities by 2050. Jongman et al. (2012) used extreme sea level estimates from the DIVA studies to produce a gridded inundation map for a 100-year flood. Using this, they assessed the increase in risk during the 21st century as a result of change in exposure only. More recently, Schuerch et al. (2018) modified DIVA to perform a more comprehensive assessment of coastal wetland responses to climate change globally. Combined, the aforementioned studies show that in general risk will increase by a large amount throughout the 21st century if no future DRR measures take place. The costs of implementing future DRR measures are large but are far smaller than the benefits gained by their risk-reducing effect.
The previous studies all use the extreme sea levels from the original DIVA model. Fang et al. (2014) present the first gridded analysis of current flood risk in terms of affected people and affected GDP. Not only in 2013 were the extreme sea levels in coastal flood risk studies improved through hydrodynamic modelling. Muis et al. (2016) made the dynamic Global Tide and Surge Reanalysis (GTSR) – the first of its kind – using the physically based global-coverage Global Tide and Surge Model (GTSM). GTSR was validated against sea level observations worldwide and compared against the previously used extreme sea levels within DIVA. GTSR represents extreme sea levels much better than the previously used extreme sea levels. Muis et al. (2016, 2017) used these new extreme sea levels to estimate the population exposed to a 100-year-return-period flood, and they found the global numbers to be about 28 % lower than those with the previously used extreme sea levels. Recent work by Hunter et al. (2017) used the approach of Hallegatte et al. (2013) to assess flood risk in global cities, but using extreme sea levels derived from tide gauges. They find that the original extreme sea levels are overestimated compared to observations and that average annual damages are about 30 % lower using observations. Tiggeloven et al. (2020) used an updated version of the GTSR dataset, including observed tropical cyclones, to assess current risk in terms of expected annual damage (EAD) and future risk until 2080 (using projections of change in hazard and exposure). They also assess the benefits and costs of structural flood protection and find various strategies to show high potential of cost-effectively reducing (future) coastal flood risk at the global scale.
Several recent studies have examined new avenues for examining coastal risk. Beck et al. (2018) estimate the global flood protection savings that can be provided by coral reefs. Vafeidis et al. (2019) investigate the uncertainty introduced to global coastal risk modelling by the flood attenuation land inwards. They show that the uncertainties in attenuation are similar in size to the uncertainties in sea level rise, suggesting this is an important future research direction.
From the outset, coastal flood risk assessments have been forward-looking, since the original studies were developed to assist climate adaptation studies. The use of future hazard and exposure scenarios is therefore widespread, as is the assessment of the costs and benefits of DRR measures, both structural and nature-based. Vulnerability has been included in many of the DIVA studies; dynamic vulnerability scenarios have not been developed. Several recent studies have focused more on current risk, using the newer datasets on extreme sea levels. Recently, a number of studies have assessed risks at a 30 ′′ × 30 ′′ gridded resolution, moving towards a higher spatial resolution compared to the studies carried out using the DIVA model. Coastal flood risk studies have either assessed impacts for a single return period or have assessed probabilistic risk by aggregating across several return periods. As with river floods, to date none of the global-scale studies have used probabilistic stochastic event sets.
2.1.3 Pluvial floods
To the best of our knowledge, the scientific literature does not contain any examples of global-scale pluvial flood risk assessments, i.e. flooding caused by intense rainfall that exceeds the capacity of the drainage system. Pluvial flooding is most commonly assessed using flood models for a small area (e.g. city or even part of a city) that generate data on depth and velocity of surface water associated with rainfall events of different intensities. Guerreiro et al. (2017) did develop a modelling approach to assess pluvial flood hazard for 571 cities at the continental scale in Europe. The paper outlines some of the key challenges in such a continental approach, which would be amplified for a potential global-scale application. These include difficulties in obtaining the required hourly rainfall records; low resolution of continental to global digital elevation models (DEMs) compared to those typically used for pluvial flood models; and the lack of data to represent local sewer systems, building shapes, and infiltration in local green spaces. Opportunities to collect such data lie in high-resolution remote sensing and data science (Schumann and Bates, 2018), but also in local crowdsourced methods such as community mapping (Winsemius et al., 2019), which is a promising avenue, particularly in strongly growing urban centres in developing countries. Guerreiro et al. (2017) demonstrate that current modelling capabilities and requisite computing power make large-scale pluvial flood hazard assessment a possibility, if these data challenges can be overcome. Sampson et al. (2015) do include floods in small river channels (with catchment less than 50 km 2 ) driven by intense local precipitation. To do this, they use a “rain-on-grid” method in which flow is generated by simulating rainfall directly on the DEM at a high resolution ( 3 ′′ × 3 ′′ ), using intensity–duration–frequency relationships of extreme rainfall from ∼200 locations around the world. However, they state that it is not known whether this method provides robust estimates of return period rainfall globally, and they also indicate the importance of tackling the aforementioned difficulties. Wing et al. (2018) use this method to assess flood hazard and risk in the conterminous USA.
2.2 Tropical cyclones
Several studies on tropical cyclone (TC) risk have been carried out at the global scale, using various methods (Table 1). Peduzzi et al. (2009) assess current TC risk hotspots, expressed in terms of expected number of fatalities per year per country. Hazard is represented by computing buffers along individual TC tracks between 1980 and 2000, where wind speed exceeds a threshold of 42.5 m s −1 . The tracks are taken from the PreView Global Cyclones Asymmetric Windspeed Profile dataset. The average cyclone frequency per cell is determined by taking the spatial extents of individual cyclones (5 km × 5 km) and averaging the frequency over the entire period. Exposure is represented by population and GDP per capita (5 km × 5 km), taken from GRID and the World Bank, respectively. Vulnerability is represented using a selection of 32 socio-economic and environmental variables. Since the results are only for current conditions, future DRR measures are not accounted for.
Cardona et al. (2014) also assess risk from TCs in the current time period. They express risk in terms of economic damage (average annual loss and probable maximum loss for a fixed return period of 250 years) by combining data on hazard, exposure, and vulnerability within the CAPRA Platform Risk Calculator ( https://ecapra.org/ , last access: 30 November 2019). Hazard is represented by maps of wind speed for different return periods at a horizontal resolution of 1 km × 1 km. These are derived from a stochastic set of wind fields, calculated using a model forced by historical observations of TCs from the IBTrACS v02r01 dataset (Knapp et al., 2010). Exposure is represented using the common dataset of the GAR 2015, in which it is represented as a group of buildings in each point or cell of analysis with a resolution of 5 km × 5 km. Vulnerability is represented by IDFs relating maximum wind velocity sustained for 5 s gusts at 10 m above ground level (Yamin et al., 2014). Since the results are only for current conditions, future DRR measures are not included. In the paper, rankings of national-level risk are shown.
Fang et al. (2015) assess the global population and GDP at risk from TC winds in the current period. To represent hazard, they develop a 6-hourly TC track database up to 2012 using CMA-track (Ying et al., 2014), HURDAT (Landsea and Franklin, 2013), and IBTrACS (Knapp et al., 2010). To convert the gust wind to sustained wind speed, a gust factor model is applied. Next, a Gumbel distribution is fitted to all grid cells ( 30 ′′ × 30 ′′ ) with more than 20 TC events to calculate wind speeds at several return periods. Exposure is represented using gridded population data at a resolution of 30 ′′ × 30 ′′ from LandScan 2010 from the Oak Ridge National Laboratory (ORNL) and is represented using gridded GDP data at a resolution of 0.5 ∘ × 0.5 ∘ from the Greenhouse Gas Initiative (GGI) dataset of the International Institute for Applied Systems Analysis (IIASA) for 2010. Vulnerability is not accounted for, and since the study only examines current risk no future DRR measures are included. They find that China has the highest expected annual affected population and GDP, and the top 10 % of countries are largely in Asia.
Peduzzi et al. (2012) assess risk from TCs over the period 1970–2009, with projections of future risk to 2030 based on projections of increased exposure only. Hazard is represented by maps showing TC frequency and maximum intensity for events between 1970 and 2009 at a horizontal resolution of 2 km × 2 km. The dataset is derived from a TC model of wind speed profiles using a parametric Holland model (Holland, 1980), which is forced using historical observations of TCs from the IBTrACS v02r01 dataset (Knapp et al., 2010). Exposure is represented by gridded maps of population and GDP at a horizontal resolution of 30 ′′ × 30 ′′ . Current population data are taken from LandScan 2008 (LandScan, 2008) and current GDP data are taken from data from the World Bank. They are both extrapolated to each decade from 1970 to 2030, based on UN Environment Programme (UNEP) country data. Vulnerability is represented using different country-level parameters relating to the economy, demography, environment, development, early warnings, governance, health, education, and remoteness. These are used to calculate exposed GDP, affected population, and mortality risk per country for each year between 1970 and 2030. Future DRR measures are not included. The average population exposed to TCs per year is projected to increase by 11.7 % by 2030, with about 90 % of this increase in Asia. In relative terms, the largest increase in risk is in Africa.
Mendelsohn et al. (2012) assess TC risk using future scenarios of hazard, exposure, and vulnerability. Risk is expressed in terms of direct damage, and the damage pertains to storm surge, wind, and freshwater flooding, without distinguishing between the three sources. Hazard is represented by TC landfall locations and intensity from a synthetic dataset of TC tracks, simulated using the model of Emanuel et al. (2008). The TC model is seeded with climate data from four GCMs: CNRM-CM3, ECHAM5, GFDL CM2.0, and MIROC 3.2, for both the current period (1981–2000) and future period (2081–2100) under the SRES A1b emissions scenario; sea level rise is not accounted for. The damage per storm is calculated using a statistical damage function per county in the USA or per country for the rest of the world. The damage function uses population density to represent exposure and income as an indicator of vulnerability. Current exposure and income data are used per county for the USA and per country for other regions. Future projections of population per country are taken from the World Bank (2010), and future projections of GDP per country are based on long-term growth rates for three income groups. Probabilistic risk is expressed as direct damage per year, based on the damages from the stochastic storm tracks. Future DRR measures are not included. The main findings are an increase in global damage of ∼115 % by 2100 due to changes in population and income, with approximately a further doubling due to climate change.
In summary, several studies have examined the risk from TCs at the global scale. Most have only considered current conditions, except Peduzzi et al. (2009) (change in exposure) and Mendelsohn et al. (2012) (changes in hazard, exposure, vulnerability). A defining aspect of a TC hazard is that it is composed of wind, precipitation, and storm surge, and the impacts result from a combination of these. However, the current studies to date do not explicitly model all of these aspects. At present there are no methods available to parametrically model 2-D precipitation fields from TCs in the same way that wind fields are parametrically modelled using the Holland Model (Holland, 1980). A large range of different approaches have been used for defining and modelling both the hazard and risk. Mendelsohn et al. (2012) and Cardona et al. (2014) express risk in probabilistic terms. Mendelsohn et al. (2012) is the only study to use a synthetic TC dataset, whilst the other studies use historical TC events to construct the hazard.
2.3 Hazards associated with severe convective storms
Perils associated with severe convective storms (SCSs), such as large hail, heavy rainfall, strong wind gusts, or tornadoes, are among the most important perils in several regions of the world (Christian et al., 2003; Virts et al., 2013; Cecil et al., 2014). Of all SCS-related hazards, hail causes the largest economic damage (Kunz and Geissbuehler, 2017). However, the modelling of SCS risk is still in its infancy (Allen et al., 2016; Martius et al., 2018). As a result, there are currently no global risk models available for these SCS events or their sub-perils (hail, wind gusts, tornadoes, heavy rain, lightning), nor are efforts being made in this direction.
However, as insurance companies have to either provide the solvency capital for rare events (e.g. 200-year-return-period event according to the EU Solvency II directive in Europe) or have to reinsure their risk, there is a growing and large demand to better estimate the risk related to the different SCS sub-perils in a scientific manner (Allen et al., 2020). The few existing models owned by the insurance market quantify the risk mainly on a regional (e.g. Schmidberger, 2018), national, or continental level (e.g. Punge et al., 2014). These models are not freely available and the literature is scarce. Initial loss estimates in the insurance industry were based on mathematical analyses of the company's own damage data and portfolio. As more and more data on SCS events and their sub-perils have become available during the last decade, an increasing number of damage and risk models for SCS have been developed either by insurance companies or by companies developing catastrophe models (CAT models). The CAT models consider basic hazard characteristics (e.g. length, width, angle of the footprints of sub-perils) and intensity metrics (e.g. hailstone size, hail kinetic energy, precipitation amount, maximum wind speed) of an underlying event set and quantify the damage for a certain portfolio (exposure data) via fragility curves, a kind of IDF. Due to the restricted record length of SCS hazard event sets, stochastic simulations based on, for example, statistical distributions of the relevant input parameters in combination with Markov or Poisson processes are performed (e.g. Punge et al., 2014; Holmes, 2015; Ritz, 2017; Schmidberger, 2018; Schmidberger et al., 2018). In some cases, the CAT models also consider atmospheric conditions relevant for SCS development. None of the insurance models consider projected changes in the frequency and severity of SCS due to climate change.
2.4 Droughts
A relatively large number of studies have been carried out to assess global risk from droughts. Therefore, these are summarised in this section and in Table 1 and further elaborated upon in the Supplement. Here, we focus specifically on drought risk, rather than water scarcity. Drought itself is a difficult concept to define, and as a result more than 150 indices have been developed for its identification over the past decades. Commonly, drought hazard is defined as a relative concept, mostly as a deficit to normal, e.g. when such drought indices fall below or exceed a given threshold. Nevertheless, since drought is a complex hazard which propagates from a rainfall deficit (meteorological drought) to soil moisture drought to hydrological drought, a variety of corresponding impacts may result with regard to the different types of drought, as well as underlying socio-economic and ecological conditions. Hence, since a universal definition of drought seems impracticable, a paradigm shift towards a definition of drought by its impacts has been recommended (Lloyd-Hughes, 2014).
The multifaceted aspects of drought are reflected in the large range of risk indicators used in the studies described below as well as the very diverse range of approaches and datasets used to represent hazard, exposure, and vulnerability. The studies described below neither explicitly include future DRR measures nor assess risk in a probabilistic sense.
Dilley et al. (2005) are some of the first to conduct a global-scale assessment of drought risk, overlaying layers of hazard (Weighted Anomaly of Standardized Precipitation, WASP), and exposure (population, GDP, road density) information at a relatively coarse resolution of 2.5 ∘ × 2.5 ∘ for the current time period. They assess risk in terms of affected GDP, population, roads, and infrastructure. Christenson et al. (2014) build on the drought risk assessment of Dilley et al. (2005) by making a further distinction between the type of population exposed (i.e. urban or rural). Neither of the aforementioned studies assess vulnerability. The studies of Yin et al. (2014) and Carrão et al. (2016) do include vulnerability (as well as hazard and exposure) and also calculate risk at a higher spatial resolution of 0.5 ∘ × 0.5 ∘ . Yin et al. (2014) express risk in terms of maize yield, and they represent vulnerability by fitted logistic regressions between historical maize crop loss estimates and simulations of drought stress. Drought hazard is represented as the normalised cumulative water stress index during the growing season, and exposure is represented by a map of fields and maize yield. Carrão et al. (2016) assess risk using a drought index integrating several factors. Vulnerability is represented in the form of proxies of economic, social, and infrastructural vulnerability, at resolutions from 5 ′ × 5 ′ to the country scale. Hazard is represented by WASP, whereby a drought is identified when the monthly precipitation deficit is less than or equal to 50 % of its long-term median value for 3 or more consecutive months. Exposure is represented by gridded maps of agricultural land, population, and livestock. On the basis of their results, Carrão et al. (2016) state that a reduction in drought risk could be rapidly achieved by improved irrigation and water harvesting in regions where infrastructural vulnerability is high.
Several studies assess future drought risk, as a result of either hazard or hazard and exposure. The forward-looking studies below all use a horizontal resolution of 0.5 ∘ × 0.5 ∘ , except for that of Smirnov et al. (2016), where a resolution of 2 ∘ × 2 ∘ is used. The majority of these studies do not include vulnerability (Arnell et al., 2013, 2018; Smirnov et al., 2016; Liu et al., 2018), whilst Li et al. (2009) and Guo et al. (2016) do include vulnerability, but only under current conditions.
Li et al. (2009), Arnell et al. (2013), and Guo et al. (2016) perform future simulations by projecting changes in hazard only for different time slices up to the end of the 21st century, as a result of climate change. As with the current drought risk studies, the risk, hazard, exposure, and vulnerability are represented using very diverse metrics. All assess risk in terms of agricultural impacts, although the metric used is different in all cases. Hazard is represented by the Palmer Drought Severity Index (PDSI), Standardized Precipitation Index (SPI), namely SPI-12, and normalised cumulative water stress index, whereby different thresholds are used to identify hazardous drought conditions. Representation of exposure is diverse but shows some similarities across the studies, represented by data on crop yields, cropland/field areas, or a combination of both. Vulnerability is included in very different ways: the proportion of area equipped for irrigation per country in Li et al. (2009) and proxies of economic, social, and infrastructural vulnerability in Guo et al. (2016).
Smirnov et al. (2016), Arnell et al. (2018), and Liu et al. (2018) add a layer of complexity in their future drought risk assessments by including projections of change in exposure as well as hazard, although vulnerability is not included in these studies. Risk is expressed in terms of the population affected in all of the studies, as well as cropland area affected by Arnell et al. (2018). Again, the indicators used to represent hazard are very diverse: Standardized Precipitation Evapotranspiration Index (SPEI), namely SPEI-24; Standardised Runoff Index (SRI); and PDSI respectively. Since they all assess the population affected by drought, they all use gridded population projections for the current and future time period, with Arnell et al. (2018) additionally using crop data.
As a result of the wide range of risk indicators and approaches used, estimates of global drought risk vary significantly from study to study. Nevertheless, all studies find a robust increase in future drought risk due to changes in both climate and socio-economic conditions.
2.5 Wildfire
Wildfire is increasingly understood as being an inherently socio-natural phenomenon with feedbacks between society, vegetation, fire weather, and climate (Riley et al., 2019). However, global wildfire risk is a particularly understudied area of disaster risk assessment. This may be due to a focus on global burnt area products to provide input to global climate modelling as a significant source of emissions (Giglio et al., 2009; GCOS, 2011; Chuvieco et al., 2016) rather than a disaster risk emphasis. It is also due to the large degree of complexity with interactions between natural and human processes driving occurrence and intensity of wildfires as well as exposure and vulnerability to them.
In the studies reviewed below, DRR measures are not explicitly accounted for in the modelling frameworks. This is largely due to uncertainty in human actions – continued ability to manage fuel and suppress fires under different climatic conditions and increased sprawl into wildland–urban interface (WUI) areas – and due to regime changes in weather and vegetation making previously non-hazardous vegetation areas susceptible to fire, especially with increased logging and fragmentation, particularly in tropical areas (Laurance and Williamson, 2001; Flannigan et al., 2009; Corlett, 2011; Jolly et al., 2015). Significant steps forward could be made by examining interactions between vegetation, weather, climate, and human activities that cause wildfires.
Meng et al. (2015) map forest wildfire risk globally under current conditions only. Risk is expressed in terms of forest area burnt for different return periods at a resolution of 0.1 ∘ × 0.1 ∘ but is not integrated into estimates of probabilistic risk. Hazard is represented as annual forest wildfire occurrence based on historical data from MODIS satellite imagery (0.1 ∘ × 0.1 ∘ ). The short historical time series of wildfire occurrence (12 years) required the use of fuzzy mathematics to allow for the calculation of different return periods of forest wildfire (Huang, 1997, 2012). Exposure is represented by the area of forest (representing economic value), using land cover data at 0.1 ∘ × 0.1 ∘ . Vulnerability is modelled as a function derived from fire occurrence versus burnt area, whereby a cell with high vulnerability indicates that a small number of fires can cause a large amount of burnt area, with the inverse being true for low vulnerability. The results show the highest risk in central Africa, central South America, northwestern Southeast Asia, middle-eastern Siberia, and the northern regions of North America. High risk can also be seen in eastern Australia, Laos, Cambodia, Thailand, Bangladesh, eastern Russia, and the borders of Zambia, Angola, and the Democratic Republic of Congo. Risk results from Meng et al. (2015) are used by Shi and Kasperson (2015) as part of a multi-hazard global risk assessment. In this study, impacts are integrated across return periods to estimate probabilistic risk. However, the results are aggregated across 11 different hazards, so the risk attributed to wildland fire cannot be identified.
Cao et al. (2015) performed a similar study to Meng et al. (2015), but they present results for grassland wildfire risk at a resolution of 1 km × 1 km. The analysis is carried out for current conditions, expressing risk as average impacts per year over 2000–2010. Hazard is calculated based on probability of ignition, slope, and vegetation properties (calculated from MODIS data) (1 km × 1 km). A logistic regression model is developed using these properties and historical burnt area records to model the probability of grassland burning. Exposure is based on the assumption that the primary impact of grassland fires is on the stock industry, which is dependent on available biomass from grassland. Therefore global net primary product (NPP) (1 km × 1 km) is used as the proxy for exposed value for grassland wildfire. Vulnerability is the probability of fire spread or propagation and contributes to the calculation of probability of grassland burning. The key results show that the areas of highest risk are in Australia, Brazil, Mozambique, Madagascar, the United States of America, Russia, Kazakhstan, China, Tanzania, Canada, Angola, South Africa, Venezuela, Argentina, Nigeria, Sudan, and Colombia. Again, the results are used in Shi and Kasperson (2015) as part of a multi-hazard global risk assessment.
Knorr et al. (2016) present wildfire risk for 1901 to 2005 and then to 2100 using Representative Concentration Pathways (RCPs) and Shared Socioeconomic Pathways (SSPs) to project hazard and exposure. Risk is expressed in terms of affected people and burnt area per year at a resolution of 1 ∘ × 1 ∘ . Hazard is modelled by coupling a semi-empirical fire model, SIMFIRE (Knorr et al., 2014), with a global dynamic land ecosystem and biogeochemical model LPJ-GUESS (Ahlström et al., 2012). This produces hazard metrics of fractional burnt area per year. The coupling with LPJ-GUESS allows vegetation and weather factors to update, with SIMFIRE providing annual updates on fire frequency per grid cell. The hazard modelling is driven using RCP4.5 and RCP8.5 data for monthly mean precipitation, temperature, and radiation from CMIP5. Exposure is represented by population under SSP2, SSP3, and SSP5, although this does not include changing rates of population splits between urban and rural areas, which is an important factor for future interactions with wildfire. Vulnerability and DRR measures are not explicitly considered, although population density is a factor within SIMFIRE, accounting for the idea that generally increasing population will suppress wildfires. Under the future scenarios, there is a significant increase in the number of people in fire-prone areas between 1971–2000 and 2071–2100: between 23 % and 56 % for RCP4.5 and between 25 % and 73 % for RCP8.5, averaged across SSPs.
2.6 Earthquakes
A relatively large number of studies have been carried out to assess global risk from earthquakes. Therefore, these are summarised in this section and in Table 1 and elaborated on in the Supplement. Several global assessments have used index-based methods or overlays of a single hazard map with exposure data (e.g. population, GDP) to assess global exposure to earthquake hazard, and they are not explicitly discussed in this review. All of the studies reviewed in Table 1 explicitly include hazard, exposure, and vulnerability, although none of them carry out future projections of risk.
One of the first global earthquake risk models to go beyond this approach is that of Chan et al. (1998), who examine direct damage via macroeconomic indicators to derive global seismic loss at the relatively coarse scale of 0.5 ∘ × 0.5 ∘ . The study of Dilley et al. (2005) also uses hazard data based on past events (based on the Richter scale), but in addition it uses 50-year-return-period hazard maps from the Global Seismic Hazard Assessment Program (GSHAP). The resolution of the risk analysis is higher, at 2.5 ′ × 2.5 ′ , and a wider range of impact indicators are used (affected population, affected GDP, affected road and rail infrastructure, and fatalities). Global studies at a higher resolution of 1 km × 1 km were performed by Jaiswal and Wald (2010, 2011). These two studies essentially use the same approach, but the former assesses risk in terms of affected people and fatalities, whilst the latter also assesses risk in terms of direct economic damage and affected GDP. Hazard is represented by ShakeMaps of intensity from past events at a resolution of 1 km × 1 km.
Daniell (2014) and Daniell and Wenzel (2014) also assess global earthquake risk at 1 km × 1 km, whereby risk is expressed in terms of direct and indirect damage, fatalities, affected people, and affected GDP, at a resolution of 1 km × 1 km. In this case, hazard is represented by the spectral acceleration and/or macroseismic intensity (MMI) at each point, which is then rasterised on a 1 km × 1 km grid for each event. None of the aforementioned studies assess risk probabilistically, instead calculating impacts for past events or for a given return period.
Li et al. (2015) describe a global earthquake risk model using a probabilistic approach, in which the impacts are integrated over several exceedance probabilities. Hazard is represented by peak ground acceleration (PGA) at 0.1 ∘ × 0.1 ∘ with conversion to macroseismic intensity. Risk is expressed in terms of direct damage, fatalities, affected people, and affected GDP, at a resolution of 0.5 ∘ × 0.5 ∘ for mortality and 0.1 ∘ × 0.1 ∘ for economic–social wealth.
From the GAR2013 onwards (UNDRR, 2013, 2015a, 2017), a probabilistic approach using stochastic hazard modelling has been used in the GARs. Risk, in terms of direct damages, is calculated stochastically at a country resolution and expressed at the national scale in terms of probable maximum loss and annual average losses. Hazard is represented by spectral accelerations at a resolution of 5 km × 5 km, using a stochastic event set of earthquakes around the world. The Global Earthquake Model (GEM) (Silva et al., 2018) also uses stochastic event sets to produce probabilistic risk estimates in terms of economic damage to buildings (1 km × 1 km).
Exposure data used in global earthquake models have generally increased in resolution from ∼0.5 ∘ to ∼1 km, usually using datasets such as gridded population and GDP. A defining feature of more recent global studies has been the use of capital stock data (e.g. Daniell, 2014; Daniell and Wenzel, 2014; Silva et al., 2018). Vulnerability is represented in various ways, ranging from empirical loss and fatality functions based on reported losses and fatalities to empirical fatality and loss ratios and IDFs based on different building types.
2.7 Tsunamis
As with other natural hazards, tsunami risk can be broken down into hazard, vulnerability, and exposure. However, limited empirical data on vulnerability and the computational burden of tsunami wave propagation simulations mean that only a limited number of studies have so far been developed, and most of them have either limited scope or resolution. Early risk assessments at the local scale from Berryman (2005) and Grezio et al. (2012) use methods to estimate the inundation at low resolution. The highest spatial resolution simulations have been carried out by Wiebe and Cox (2014) for parts of Oregon, Jelínek et al. (2012) for Cádiz in Spain, and De Risi and Goda (2017) for the Miyagi prefecture in Japan. Some models, such those of Tinti et al. (2008), or Okumura et al. (2017), have assessed risk in terms of fatalities.
Løvholt et al. (2015) assess global risk in terms of direct damage, affected people, and fatalities at a resolution of 1 km × 1 km. Risk is calculated probabilistically using stochastic event sets and a probable maximum loss curve at 100, 500, and 1500 years, as well as average annual losses. Risk is only assessed for current conditions, and therefore no DRR measures are included. Hazard is represented in terms of inundation depth at a resolution of 90 m × 90 m. This is simulated using a 25 km propagation model, followed by a 2-D model at a resolution of 1 ′ × 1 ′ and is then further downscaled using a 90 m DEM. Exposure is represented by capital stock and population at 1 km × 1 km, taken from the coastal database of De Bono and Chatenoux (2015) used as part of GAR2015. Vulnerability is represented by IDFs for different building types derived from expert workshops (Maqsood et al., 2014) for the Asia Pacific. The Suppasri et al. (2013) functions are used for the rest of the world. They find that Japan and the Philippines, among other island nations, have the highest relative risk.
Schäfer (2018) introduce a generic tsunami risk assessment framework that could be applied for global risk modelling, and they apply it to various regions around the world. Risk is expressed in terms of direct damage, affected GDP, affected population, and fatalities for current conditions not accounting for DRR measures. It is calculated at 90 m × 90 m resolution for various return periods, with expected annual impacts being assessed by integrating across different exceedance probabilities. Hazard is represented by maps of inundation depths (90 m × 90 m), based on numerical simulations using shallow water wave equations and machine learning. Exposure is derived using a population-based capital stock model (90 m × 90 m) built from the Global Human Settlement Layer (Pesaresi et al., 2016). Vulnerability is represented by depth–damage IDFs that are resolved for three building classes (light, moderate, and massive buildings) and a fatality function considering both water depth and arrival time. The study is tested in various regions, including Japan, Chile, and the Caribbean, and derives average annual losses and probable maximum loss curves.
2.8 Volcanoes
Volcanoes can produce a variety of hazards, including pyroclastic density currents (pyroclastic flows, surges, and blasts), tsunamis, lahars, tephra (including volcanic ash and ballistics), debris avalanches (sector collapse), gases and aerosols, lava flows and domes, and lightning. Not all volcanic hazards are produced by every volcano or eruption, and some can occur without an eruption, for instance volcanic gas. Whilst many hazards might be triggered by the volcano directly, the occurrence or distribution of others can be influenced by hydrometeorological factors, for instance, rainfall-triggered lahars and landslides or the influence of wind on the distribution of volcanic ash. Comprehensive assessments of volcanic risk at the global scale do not exist. Quantitative risk assessments at the local scale (where they exist) rarely include the effect of DRR measures, many of which are not measurable in a quantitative sense (e.g. engagement between scientists and civil protection, policymaking, preparedness, and planning). The field of volcanic hazard and risk assessment can therefore appear less well developed compared to other natural hazard fields of study, with the effect that assessments combining multiple natural hazards typically underestimate the threat from volcanic activity. There are at least three key factors that limit our ability to assess volcanic risk at the global scale: the multi-hazard, time-varying, and complex nature of volcanic events; a large discrepancy in the quality and quantity of data required to inform global volcanic hazard assessments between regions; and limitations of data to inform global volcanic risk assessment, especially because large, damaging eruptions impacting populated areas are relatively infrequent and impacted zones can be dangerous and sometimes inaccessible for long periods. International collaborations have now been established to facilitate the production of systematic evidence, data, and analysis of volcanic hazards and risk from local to global scales (e.g. Loughlin et al., 2015; Newhall et al., 2017; Bonadonna et al., 2018).
Past approaches to assessing global volcano risk, described below, have mainly aimed to identify those volcanoes or cells that pose the greatest relative danger, in order to inform subsequent in-depth investigations using local data and knowledge. The first assessment of the hazard threat posed by volcanoes globally is Yokoyama et al. (1984). They use an index-based approach to identify “high-risk” volcanoes. Binary indices are used to score 10 hazard and five exposure components, describing the frequency of recent explosive activity and hazards, and the size of the population within a certain radius of the volcano, respectively. Two quasi-vulnerability binary scores are used: one for if the volcano had produced historical fatalities and one for if evacuations had resulted from historical eruptions. Scores for each volcano are summed, with high-risk volcanoes defined as those with a score ≥10 . This approach results in notable volcanoes that have produced some of the worst volcanic catastrophes of the 20th century being considered not high risk.
Small and Naumann (2001) and Freire et al. (2019) rank volcanoes globally according to the population exposed within radii of 200 and 10–100 km from a volcano, respectively. Hence, hazard and vulnerability are not included. Exposure is represented by gridded population data at a resolution of 2.5 ′ × 2.5 ′ (Small and Naumann, 2001) and 250 m (Freire et al., 2019). For the GAR2015, an index-based approach to assessing volcanic hazard and risk is also used, combining data and the approaches of Auker et al. (2015) and Brown et al. (2015a, b). Hazard is represented by Auker et al. (2015) using an index method to represent the hazard level of each volcano according to the frequency and intensity of past eruptions, while accounting for record incompleteness. Exposure is represented using the method of Brown et al. (2015a), an index of population exposure weighted to historical data of fatalities (Auker et al., 2013) with distance, and vulnerability is expressed in terms of affected population and fatalities for past events. Combining the hazard index with fatality-weighted population counts gives estimates of individual volcanic threat. Not all volcanic hazards are considered in the weighting; the weights are sourced from volcanic flow fatality data only, as pyroclastic density currents and lahars are the source of the majority of direct historical fatalities (Auker et al., 2013). Brown et al. (2015b) further aggregate the volcanoes to the national scale to provide country-level estimates of volcanic threat. Whilst this approach represents our most recent attempt at considering volcanic hazard and risk at the global scale, it still assumes concentric radii around each volcano.
Grid-based global assessments of volcanic risk have been carried out by Dilley et al. (2005) and Pan et al. (2015). Dilley et al. (2005) include volcanoes as part of their multi-hazard hotspot analysis, whereby risk is expressed in terms of affected GDP and population and fatalities ( 2.5 ′ × 2.5 ′ ). Hazard is expressed in terms of the count of volcanic activity, which is gridded to 2.5 ∘ × 2.5 ∘ , between 79 and 2000 CE (i.e. no consideration of the size of eruptions or their spatial extent). Exposure is represented by gridded population from the Gridded Population of the World 3 (GPWv3) dataset from CIESIN; GDP per capita at the national scale from the World Bank; and transportation lengths from the VMAP datasets, all at 2.5 ′ × 2.5 ′ . Vulnerability is represented by two quasi-vulnerability values, for mortality and economic loss rates globally as a result of volcanic activity between 1981 and 2000 based on the EM-DAT database, aggregated to country and regional levels. A major limitation of this approach, as recognised by the authors themselves, is that the hazard records are too short to capture the larger, typically more damaging events and are biased towards those volcanoes for which we have good and recent records of past activity. Also, no attempt was made to account for far-reaching volcanic hazards like ash. Pan et al. (2015) built on this approach to assess fatalities, by extending the length of the fatality database used to 1600 CE. They also add relationships between eruption Volcanic Explosivity Index (VEI) and frequency and hazard extent, although concentric circles are still assumed. Hazard is defined here as the frequency of each VEI eruption, using the method of Jenkins et al. (2012). Exposure is represented using gridded population data ( 30 ′′ × 30 ′′ ) of 2010 from the Oak Ridge National Laboratory (ORNL) (Bright et al., 2011). Vulnerability is represented by fatality curves fitted to the historical average fatality of each VEI provided by the National Oceanic and Atmospheric Administration (NOAA).
2.9 Landslides
Assessing the risk associated with landslides at a global scale is challenging for several reasons. Firstly, the spatial extent of individual landslides is typically small, limiting the effectiveness of routinely monitoring these events at a global scale. Further, the diversity of parameters influencing the hazard susceptibility (e.g. elevation, lithology), preconditioning (e.g. soil moisture, seismicity), and triggering (e.g. extreme rainfall, earthquakes) make it difficult to physically model these processes using uniform approaches. While the spatial extent of landslide source regions is generally small, the downstream hazards such as debris flows – which are often associated with the greatest damage (Badoux et al., 2014) – can be more widely distributed, meaning risk assessments must consider locations both close to and far from the source areas.
Efforts have been made to catalogue landslides and their impacts, based on a range of data sources including media reports, government statistics and other written sources, remote sensing, and citizen science (e.g. Guzzetti et al., 1994; Kirschbaum et al., 2010; Petley, 2012; Tanyas et al., 2017; Froude and Petley, 2018; Juang et al., 2019). In addition, several studies have tried to model global landslide hazard (e.g. Stanley and Kirschbaum, 2017; Kirschbaum and Stanley, 2018). In the following paragraphs, those studies that have explicitly assessed global risk are described and summarised in Table 1.
Nadim et al. (2004, and updated in 2006) are among the first to assess risk associated with landslides at the global scale. Risk is expressed in terms of the number of fatalities per year at a resolution of 30 ′′ × 30 ′′ , over the period 1980–2000, associated with landslides and avalanches. Hazard is estimated using a range of global datasets based on both susceptibility (factors such as slope, lithology, and soil moisture) and triggering factors (rainfall and seismicity). A similar approach is used to define the hazard associated with avalanches. Exposure is represented by the Global Population of the World v4 (GPWv4) dataset ( 30 ′′ × 30 ′′ ) (CIESIN, 2016). Vulnerability is estimated using empirical data on loss of life from landslides in a number of countries, using the EM-DAT database. Future projections and therefore future DRR measures are not included in the study. They find that hotspots for landslide fatalities are the Himalayas, Taiwan, the Philippines, Central America, northwestern South America, the Caucasus, Indonesia, Italy, and Japan, with smaller proportional impacts in other countries and regions.
Dilley et al. (2005) estimate the risk associated with landslides, expressed in terms of direct damage and affected GDP and population at 2.5 ′ × 2.5 ′ , for current conditions. Hazard is represented using the data of Nadim et al. (2004) at 30 ′′ × 30 ′′ . Exposure is represented by data at 2.5 ′ × 2.5 ′ constructed from Gridded Population of the World (GPW) population data, road density data from VMAP datasets developed by the United States National Imagery and Mapping Agency, and gridded economic and agricultural activity from the World Bank and based on Sachs et al. (2001). Vulnerability is represented by empirical loss rates based on the EM-DAT database. Future projections and therefore future DRR measures are not included in the study. Their primary output is in map form, with the most elevated impacts found in many of the same locations as in the study of Nadim et al. (2004, 2006), although they find higher impacts in terms of total GDP in China.
Yang et al. (2015) assess risk in terms of fatalities for the current period at a resolution of 0.25 ∘ × 0.25 ∘ . Hazard is represented based on the method of Nadim et al. (2006), using TRMM satellite rainfall data to estimate the number of landslide events and filling in gaps in the data using information diffusion theory. Exposure is represented by population data from LandScan, resampled to 0.25 ∘ × 0.25 ∘ . Vulnerability is based on empirical mortality rates per country calibrated using a dataset of global landslides causing fatalities from Kirschbaum et al. (2010). Future projections and therefore future DRR measures are not included in the study. They find similar patterns to Dilley et al. (2005) and Nadim et al. (2006), but they additionally find many areas that have elevated risk of mortality compared to those prior studies, including large parts of China and sub-Saharan Africa.
Nowicki Jessee et al. (2018) present a global earthquake-induced landslide hazard model, which is implemented within the USGS Ground Failure hazard and risk model. Risk is expressed in terms of exposed population in near-real time for each earthquake event that triggers landsliding. Hazard is calculated by leveraging the earthquake-triggered database from Tanyas et al. (2017), based on various sources of information describing factors controlling susceptibility (such as slope and lithology) and earthquake parameters, such as shaking intensity, to estimate the relative density of landsliding within an area impacted by a major earthquake. The model landslide density estimates are not dependent on resolution. Exposure is represented by LandScan population maps, with a resolution of 30 ′′ × 30 ′′ (Bright et al., 2017). No vulnerability data are incorporated. Future projections and therefore future DRR measures are not included in the study. For each earthquake, an estimate of population exposed to landsliding and liquefaction is presented.
3.1 (Dynamics of) risk elements
As our review focuses on global-scale natural hazard risk assessments, we have not included studies that only examine the hazard. All but two of the studies have an explicit representation of hazard intensity and/or probability and of exposure. The only exceptions are the volcano studies of Small and Naumann (2001) and Freire et al. (2019), in which the population living within a set radius of volcanoes is estimated, without an explicit representation of the hazard. About two-thirds of the reviewed studies include a specific representation of vulnerability. Across the various hazards, there is no clear difference in the proportion of studies including vulnerability as we move towards the most recent publications. It is noteworthy that all of the earthquake and tsunami studies reviewed include a specific representation of vulnerability. For pluvial flooding and SCS, there are currently no global-scale risk models, with the local scale of the hazard and impact of these events making their large-scale modelling difficult.
In terms of the inclusion of dynamic risk drivers, there is a clear difference between studies focusing on hydrological, climatological, and meteorological hazards and those focusing on geological hazards. For geological hazards, none of the reviewed studies include future projections, whilst for hydrological, climatological, and meteorological hazards, around two-thirds of the studies include projections of at least one of the risk drivers. The difference between the studies of hydrological, climatological, and meteorological hazards, compared to those of geological hazards in terms of projections, may be due to the climate-change-related focus of many studies in the former group. This provides a policy context for carrying out forward-looking hazard projections to examine the influence of climate change on risk. In total, there are 32 reviewed studies that include future projections: 19 include projections of hazard and exposure, eight include projections of hazard only, and three include projections of exposure only. The remaining two studies include projections of vulnerability (as well as hazard and exposure): one study for river flooding and one for TCs. Time horizons used for the forward-looking studies tend to be towards the middle and late 21st century. For river flooding and drought, several recent studies have examined future warming levels, rather than future time slices. It is interesting to note that projections of the other risk drivers have not yet been examined for the geological hazards at the global scale, despite their importance for other policy contexts, such as the Sendai Framework and SDGs, although definitions used for monitoring of these frameworks explicitly state disaster risk assessment as “evaluating existing conditions of exposure and vulnerability” without considering future change (UNGA, 2016). The global-scale geological risk studies could apply some of the forward-looking models of exposure in their analysis to estimate future risk, which is of importance for designing prospective risk management strategies and measures.
3.2 Resolution and type of input data
The list of studies in Table 1 shows a general tendency towards higher-resolution risk analyses as we move towards more recent studies, though this is not the case for all studies. Many of the early studies are at resolutions from several degrees to 0.5 ∘ , and for many hazards there has been a progression towards higher resolutions of 30 ′′ or 1 km, and even up to 90 m per point value in some cases. We do see differences between the various hazards. Most of the early coastal flood risk studies examine risk using the coastal segments from the DIVA database, although recent studies have also moved towards 30 ′′ or 1 km. For drought, the resolution tends to be much lower, from around 0.5 to 2.0 ∘ in general. This is in line with the difference in resolution at which drought impacts are felt (Stahl and Hisdal, 2004), compared to many of the other hazards for which the direct impacts are felt more locally. For a similar reason, TC studies tend to show a much higher resolution in their analysis, which reflects the fact that TC impacts for an individual event are felt over smaller areas. Most global volcano risk studies to date examine risk at the resolution of an individual volcano, rather than on a raster grid. Several recent studies on earthquakes (Silva et al., 2018) and earthquake-induced landslides (Nowicki Jessee et al., 2017) use model frameworks that can use variable scales.
In most cases, the resolution of the risk analysis follows the resolution of the hazard and exposure datasets used as input. In most cases these have the same resolution, and where this is not the case the tendency is to either resample the lower-resolution datasets to the higher resolution or vice versa. Therefore, the resolution of the input hazard and exposure databases tends to show the same overall patterns as those discussed above for the risk calculations. The most commonly used datasets used to represent exposure are gridded datasets of population and GDP. Direct economic damage is further assessed using land use data, whilst in recent studies related to earthquakes and tsunamis the use of capital stock estimates (based on building typologies) has become more common. Given the importance of agricultural impacts for drought, exposure is also represented using datasets such as gridded agricultural area, cropland area, planting area, and so forth. For wildfires, areas of forest and grassland are also used. Nevertheless, Kreibich et al. (2019) highlighted that, especially for drought, data on losses and impacts that are directly attributed to the hazard of drought are lacking.
Methods and datasets used to represent vulnerability are highly diverse. Within the flooding community, the most common approach is to use intensity–damage functions (IDFs). For flooding, the IDF takes the form of a depth–damage function. In most studies, one global IDF is used (especially for coastal flooding), whilst for river flooding several studies have also used regional or country-level IDFs. Jongman et al. (2015) also use regional ratios of affected GDP to report losses and affected population to report fatalities; the latter is also used by Dottori et al. (2018). Global IDFs are also used in studies that examine wildfires. Some earthquake and tsunami studies have also used IDFs. They either use IDFs per income class or region (i.e. not a global function), or in more recent years there has been a tendency to use IDFs related to building types. Another approach is to use empirically derived regressions between reported impacts and a given level of hazard and exposure to derive empirical regression equations. In volcanology the limited amount of impact data has meant that only a few vulnerability and fragility functions for physical vulnerability have been developed (Blong, 2003; Jenkins et al., 2014; Maqsood et al., 2014; Wilson et al., 2014). Global TC and volcano studies tend to use various socio-economic variables at the country (or state) level as a proxy of vulnerability. Therefore, the spatial representation of vulnerability is much coarser and does not use gridded datasets in the same way as hazard or exposure.
It is clear that the different hazard communities use some common datasets to represent exposure, for example relating to population and GDP. However, there are also differences and opportunities for interaction, sharing, and knowledge exchange. For example, the use of building stock data based on building typologies in earthquake and tsunami studies has provided opportunities for improving the assessment of other hazards (e.g. volcanic tephra). The same can be said for the other hazards, where advances in IDFs related to building type offer opportunities outside the seismic community. On the other hand, approaches that have been developed to project future exposure in the flood risk community could be harnessed by the other hazard communities.
3.3 Risk indicators
The most commonly used risk indicator is the number of affected people, which is used in 59 % of the reviewed studies. A large number of studies also use some indicator of direct economic damage (44 %), with fatalities (26 %) and affected GDP (24 %) having also been used in many studies. Fatalities have been much less commonly assessed in flood and drought risk studies than in studies of the other hazards, offering potential for cross hazard knowledge exchange on methods for fatality assessment.
3.4 Future DRR measures
To date, future DRR measures have only been explicitly included in several studies, all of which are related to flooding. Global-scale assessments in coastal flooding have been forward looking from the outset, since the original studies were developed to assist climate adaptation studies. As such, they also include a wider range of DRR measures (both structural and nature-based) than the few river flood studies that have explicitly included DRR measures in recent years. The costs of DRR measures have only been explicitly assessed in a couple of studies, for coastal or river flood risk. Hence, in this regard the global flood modelling community has a lot of knowledge and examples that can be used to begin to include DRR measures in global-scale assessments of other hazards. To date, no global studies have assessed the influence that human behaviour and perception can have on the effectiveness of DRR measures.
3.5 Type of analysis
In Table 1, we have classed studies as either non-probabilistic (NP) or probabilistic (P), whereby probabilistic refers to studies that assess expected annual impacts by integrating across return periods based on a probabilistic stochastic event set. For droughts, volcanoes, and landslides, studies to date have used non-probabilistic approaches only. Studies on floods and earthquakes have seen a move towards more probabilistic studies in more recent years, and the two studies reviewed for tsunamis also use a probabilistic approach. For wildfires and TCs, both approaches are used, with too few studies to be able to see any particular change in focus through time. A major difference between the studies of earthquakes and tsunamis, in comparison to the other hazards, is the extensive use of stochastic event sets in the former. Stochastic modelling could also be beneficial for the assessment of several other hazards, as discussed in Sect. 4.
Our review shows that the field of global natural hazard risk modelling has developed rapidly over the last decade, and advances continue to be made at a rapid pace. We show that there are differences between the modelling and assessment methods used in the different hazard communities, and we show possibilities for learning between hazards. There are also opportunities for learning from methods and approaches being developed and applied to assess natural hazard risks at continental or regional scales. As Sect. 2 demonstrates, rather than simply make direct comparisons, it is essential to contextualise the reasons for advances in global risk assessments for certain hazards compared with others, which include funding, policy drivers (e.g. climate change), different levels of complexity (e.g. multi-hazard environments), and the relative frequency of occurrence of certain hazard-related disasters. We have identified within the literature opportunities for addressing some of the key challenges. Given the constraints of space, this is not intended to be an exhaustive list and is more intended to encourage further dialogue on knowledge sharing between scientists' disciplines and communities working on different hazards and risk and at different spatial scales. An overall challenge for global risk modellers is the lack of high-quality impact data for model validation. Efforts are constantly ongoing to improve the collection of impact data used in databases such as EM-DAT (CRED, 2020), NatCatSERVICE (Munich Re, 2020), DesInventar (UNDRR, 2020), and CATDAT (Daniell, 2020), but issues relating to incompleteness, fragmentation, bias, and differences in reporting conventions remain a challenge (e.g. Kron et al., 2012; CRED and UNISDR, 2018).
4.1 Hazard
Continual improvements in hazard modelling are required, both to correctly represent processes and to increase resolution. The availability of higher-resolution input datasets with increased accuracy is helping in this regard and is a common theme across hazard studies. In some cases, global hazard models are available but have not yet been used in global risk assessments. For example, Jenkins et al. (2015) provide a global volcanic hazard assessment (10 km × 10 km) that accounts for ash fall affected by local wind conditions. While the geographic extent of major disasters may be large, the specific hotspots for hazard may be much more localised. As such, there are ample opportunities to model the downscaled impact of hazards. For example, global models at present do not capture the downstream impacts of landslide material (e.g. Nowicki Jesse et al., 2017), even though in many settings debris flows and sediment-related damage can be the costliest type of hazard (Badoux et al., 2014).
Within the global earthquake and tsunami risk modelling community, we see many examples of the use of stochastic event sets. Similar approaches could be developed for assessing risk of other hazards. For example, for TCs, several models have been developed to generate synthetic TCs, such as the Synthetic Tropical Cyclone Generation Model (STORM; Bloemendaal et al., 2020), the MIT model (Emanuel and Nolan, 2004), or the Columbia Hazard (CHAZ) model (Lee et al., 2018). Such TC events could then be used to force global storm surge models, thereby also benefitting global coastal flood risk assessment. Methods for generating large, synthetic event sets could also be especially useful for those events with high spatial and temporal resolution that currently miss global approaches, such as pluvial flooding and SCS-related perils.
There is also a tendency to focus on one parameter of the hazard, whilst a hazard's impact is often related to several parameters. For example, in flood risk analysis, global hazard studies focus on the flood depth, whilst risk is also related to other parameters such as flood duration, velocity, and the rate at which floodwaters rise (Ward et al., 2016). Similar issues exist for TCs, where there has been a lot of recent attention on their possible slowing down and stalling (Kossin, 2018; Wang et al., 2018; Hall and Kossin, 2019). For example, Hurricane Dorian in 2019 stalled over the Bahamas for 36 h, pounding large parts of the archipelago with 297 km h −1 winds and 7 m storm surges.
For the water-related hazards, one avenue towards improved global hazard modelling is the improvement in hydrodynamic modelling of floods. For example, Sampson et al. (2015) present a fully hydrodynamic modelling approach for the globe, which could address some of the stated problems. The approach has been further developed by Wing et al. (2017) for the conterminous USA and further been applied for current and future flood risk assessment in the USA at the continental scale (Wing et al., 2018). For coastal flooding, the fully hydrodynamic model GTSM is now being used to simulate water levels due to surge and tide up to the coastline, but then simple planar models are used to translate these water levels into inundation maps on land. Vafeidis et al. (2019) have shown the importance of accounting for hydrodynamic processes by developing an approach to assess the impacts of water-level attenuation due to different land cover on flood hazard. This approach can be used as a first step towards improving global coastal flood risk assessment. Vousdoukas et al. (2016) use the hydrodynamic LISFLOOD-FP model to assess coastal flood hazard at the European scale, and this has been applied for European-scale coastal flood hazard by Koks et al. (2019). Hydrodynamic inundation modelling is also being applied by Schäfer (2018) for the modelling of tsunami events. For the case of drought, studies typically focus only on a single type of drought or drought index. To comprehensively understand drought events and corresponding risks, an all-angle view is needed. Furthermore, for the global scale, remote sensing products that capture hazard and impact at the same time (NDVI, fAPAR) should be applied more.
4.2 Exposure
Similarly, continual developments are being made in the improvement of global exposure databases. As stated in the review, building typologies and/or investment data have been used to develop global databases of capital stock, which are now routinely used in global earthquake and tsunami modelling (Gunasekera et al., 2015). These data, where accessible, could also be applied to other hazard types in most cases, through communication and collaboration across hazard communities. Efforts are also ongoing to develop exposure maps based on building material types within the flood risk community. For example, Englhardt et al. (2019) have developed an approach for mapping exposure in urban and rural areas in Ethiopia, based on data on buildings and their materials. This method is currently being tested for several other countries in Africa. Pittore et al. (2017) discuss the challenges in designing a global and spatial-temporal dynamic exposure database, focusing on building stock. Other data sources, such as OpenStreetMap, also offer the opportunity to use building-level information to improve global risk modelling. For the USA, Wing et al. (2018) have used the FEMA National Structure Inventory. This dataset is interesting in that it is also accompanied by projected distributions under several future SSPs, whilst currently forward-looking projections of exposure at the global level are limited to GDP, population, and land use. Recent studies have also shown the importance of examining temporal variations in exposure (e.g. between day and night and between seasons) (e.g. Freire et al., 2015) and between different income groups (e.g. Winsemius et al., 2018; Hallegatte et al., 2016). The importance of capital stock models such as those which encompass buildings, infrastructure, cross-sector applications, and contents is shown in the GRADE process, given that in most cases the building stock is only one portion of the capital stock at risk (Gunasekera et al., 2018).
4.3 Vulnerability
As evidenced from the review, much attention is required to improve the representation of vulnerability in global risk models. Currently there are a limited number of vulnerability and fragility functions for some hazards (see Murnane et al., 2019), compounded by the limited amount of impact data available to inform them (e.g. volcanic eruptions). However, a limited number of socio-economic vulnerabilities in volcanic environments are being considered in – for instance – global datasets (e.g. global fatalities; Brown et al., 2017) and there are some studies accounting for the indirect impacts of eruptions (loss of livelihood, displacement, and resettlement) at the volcano scale (e.g. Barclay et al., 2019). In time and with more resources and studies, such efforts may be scalable and used to inform future global risk studies.
The highly temporal and spatial dynamics of vulnerability and the resulting non-linearity of risk have been underscored by UNDRR's Global Platform for Disaster Risk Reduction. While recent studies at regional and local scales have begun to account for these aspects in changing hazard (e.g. Mora et al., 2018) and exposure conditions (e.g. Cammerer et al., 2013), only a few studies account for the dynamics of vulnerability (e.g. Kreibich et al., 2019) across multiple hazards. Specifically, there is an increased recognition of the need to assess how socio-economic processes can influence spatio-temporal changes in vulnerability (Cutter et al., 2015). For example, there can be a temporary rise in risk perception after a natural hazard, resulting in an increase in DRR activities. Conversely, the absence of a natural hazard over a prolonged period can create a (false) sense of safety, which can increase vulnerability (Di Baldassarre et al., 2015). An improved understanding of these dynamics of socio-economic vulnerability can significantly improve the ability of risk managers to more efficiently implement DRR measures (Hallegatte et al., 2017). Recent studies have attempted to assess some of these aspects, for example by developing indicators of socio-economic resilience for over 90 countries (Hallegatte et al., 2016; Wens et al., 2019), examining spatial differences in risk in different poverty groups (Hallegatte et al., 2016; Winsemius et al., 2018) or modelling dynamic feedbacks between levees and risk perception (Di Baldassarre et al., 2018; Haer et al., 2019). De Ruiter et al. (2020a) discuss how the impacts of consecutive disasters can be distinctly different from single hazards due to changes in socio-economic vulnerability. Cutter and Finch (2008) have assessed future spatial and temporal patterns of social vulnerability at a national scale based on historical events. For future projections, climate change is widely recognised as an important driver of the increased frequency and intensity of weather-related hazards but does not explain the (projected) changes in damages caused by geophysical hazards such as earthquakes. An improved understanding of future vulnerability can significantly improve the ability of risk managers to more efficiently implement DRR measures. Recent studies at the continental or global scale show that vulnerability to natural-hazard-related disasters is decreasing in some areas because people adapt over time and reduce vulnerability (e.g. Ciscar et al., 2019; Jongman et al., 2015). In other areas, future vulnerability is expected to increase, for example due to a limited availability of resources to adapt (Winsemius et al., 2018) or due to the impacts of successive disasters that push communities into poverty (Mirza, 2003). Moreover, international organisations on the ground are calling for an even higher level of granularity of these exposure, vulnerability, and risk estimates in order to correctly target those individuals who are in most need of disaster relief aid. For this to be achieved, it is required not only to combine estimates of natural hazard with higher-resolution vulnerability and exposure information, but also to increase the level of detail of the latter for different groups, for example with regards to gender, income, livelihood, and access to healthcare.
Another remaining scientific challenge is the harmonisation of indicators used to assess damages across a wide range of different hazard types, in order to enable the collection of loss data that are comparable across hazards. This would allow for a better comparison of the dynamics of vulnerability between different hazards. Currently, the impact data that are collected by countries, first responders, and researchers from different fields remain very heterogeneous (Cutter et al., 2015; AghaKouchak et al., 2018), and the data are often collected at different times after a disaster.
4.4 DRR measures
The number of global risk studies that explicitly include DRR measures is extremely limited and limited to flood risk studies, especially coastal flooding. Even then, most of these studies have assessed structural measures and do not explicitly examine the costs. Global volcanic fatality data demonstrate the impact of DRR measures; for instance, in spite of population growth, the number of fatalities per eruption has declined dramatically in the last few decades, suggesting that mortality has been reduced as a consequence of improved volcano monitoring, increasing awareness, early warning, communication, and preparedness around specific volcanoes (Auker et al., 2013; Brown et al., 2017). Much can be learnt from studies at local to regional scales, and it is certainly beyond the scope of this paper to provide a review of the many studies addressing DRR at this scale. A specific aspect that has not been covered in any of the global risk studies reviewed is the influence that human behaviour and perception can have on the effectiveness of DRR measures, through various feedbacks. A classic example in hydrology is the levee effect (White, 1945), in which increased levels of flood protection from levees and dikes can also lead to increased exposure and/or vulnerability in areas protected by dikes. Similarly, for wildfires feedbacks exist between the physical risk and human actions to attempt to manage fuel and suppress fires. A promising way to address these feedbacks is through agent-based models that attempt to represent the behaviour of agents (e.g. individuals, businesses, governments) through a set of decision rules (e.g. Aerts et al., 2018). An application of the agent-based model (ABM) approach has recently been used in natural hazard risk modelling at the continental scale (Haer et al., 2019), paving the way towards exploring the use of these methods at a global scale. Another aspect that is often overlooked, especially on a global scale, is the interactions between different DRR measures that are aimed at specific hazards (Zaghi et al., 2016; Scolobig et al., 2017). DRR measures aimed at decreasing the risk of one hazard can increase the risk of another, so-called asynergies of DRR measures (De Ruiter et al., 2020b). For example, building on stilts is a commonly used measure to decrease a building's flood vulnerability, but it can simultaneously increase a building's earthquake vulnerability (Wood and Good, 2004). Accounting for such asynergies between DRR measures in a risk analysis is crucial, for example when developing tools that enable policymakers to assess the effectiveness of DRR measures. A first attempt to quantify these asymmetries at a large spatial scale has recently been carried out by De Ruiter et al. (2020b), for measures to reduce flood and earthquake risk. The expansion of these approaches to the global scale would be a large step forward for global risk modelling.
Moreover, assessing the impact of complex multi-hazard damages that result from hazard chains (e.g. an earthquake followed by a flood) has only been performed at local scales, for example by using a probabilistic approach to calculate the probabilities of different final damage states (e.g. Korswagen et al., 2019). These complex hazard chains require the design of structures and DRR measures that are able to address the combined damages of different hazard chains (Korswagen et al., 2019).
Studies that consider the dynamics of how drivers impact risk into the future also enable the assessment of prospective DRR actions across exposure and vulnerability components. By modelling exposure profiles dynamically, risk reduction actions that consider where development occurs and how this can be changed to reduce future losses can be assessed. This would support demonstrating the effectiveness of land use planning and risk-sensitive developments as a DRR action. Similarly, for vulnerability, incorporating assessment of future vulnerability and the inclusion of improved building standards allows for demonstrating the benefits of more resilient construction. If changes in exposure and vulnerability are excluded from disaster risk modelling, then the assessment of prospective DRR measures is extremely challenging. Examples of this globally however are limited. Regional and city-level studies showing elements of these benefits have been demonstrated for multiple risks using coupled hazard models with cellular-automata land use models and capital stock models (Lallemant, 2015; Riddell et al., 2019). Global dynamic models of urban and land use change do however exist, and efforts could be made to effectively couple these with global hazard and risk models (Hasegawa et al., 2017; Van Asselen and Verburg, 2013).
4.5 Multi-hazard and multi-risk
There is a rapidly growing policy and scientific recognition and dialogue on the need for multi-hazard (both the multiple hazards and the simultaneous, cascading, or cumulative occurrence of these; UNDRR, 2017) risk assessments, as exemplified by high-level discussions at the UNDRR Global Platform 2019 and the aims of the Sendai Framework. These call upon the science community for an increased understanding of the risk of consecutive and cascading disasters (UNDRR, 2019). So far, the vast majority of global risk assessment studies have examined risk from a single hazard type and indeed have examined a single parameter of the hazard (Sect. 4.1). However, many environments are multi-hazardous and many hazards can trigger secondary or cascading hazards. For example, volcanoes can produce a wide variety of primary and secondary hazards that can occur simultaneously or sequentially and that differ widely in their spatial extent, duration, dynamic characteristics, and associated impacts. Capturing all these hazards, and their impacts, within the one assessment is very challenging, and typically the future hazard or risk is considered separately for each type of hazard (e.g. Sandri et al., 2014) or by assuming a given eruption scenario (e.g. Lindsay and Robertson, 2018). Methods for volcanic multi-hazard assessment across multiple scenarios, where the range of potential future volcanic hazards is shown on the one map, have been developed but as yet only been applied at the single volcano scale (e.g. Neri et al., 2013).
Interactions among different primary hazards can also influence the overall risk (Gill and Malamud, 2014; Korswagen et al., 2019). For example, within the flooding community, there has recently been much attention for so-called compound floods, whereby the interaction of coastal, river, and pluvial floods can influence the overall hazard and risk (Zscheischler et al., 2018). Methods are being developed at the global scale to assess both the statistical dependence among these hazards (e.g. Ward et al., 2018; Bevacqua et al., 2019; Couasnon et al., 2020) and their physical impacts in terms of hazard (Ikeuchi et al., 2017), with the step towards risk being the next logical one.
Understanding inter-hazard linkages is also important for properly calibrating estimates of risk in the aftermath of major disasters. Earthquakes in particular can affect the long-term propensity of a given landscape to fail via landsliding (Marc et al., 2015), while the prior saturation state of a landscape – due to flooding or human input of irrigation water – can increase landslide susceptibility during an earthquake itself (Bradley et al., 2019; Watkinson and Hall, 2019). These interactions remain poorly constrained but can influence the long-term recovery from major hazards.
Several studies have identified the current shortcomings in future exposure and vulnerability projections for multi-hazard risk assessments (e.g. Gallina et al., 2016). In spite of the clear need to adopt a multi-hazard risk approach to global risk assessments, the very nature of the endeavour (accounting for multiple, interrelated hazards and their dynamic influence on vulnerability and exposure) has arguably restricted progress towards truly comprehensive analyses of global multi-hazard risk. There is a need to address the previously mentioned challenges with critical work at multiple scales (local to global) towards comprehensive global multi-hazard risk assessments. Collaboration between the international hazard science and risk research communities is key to progress.
4.6 Use of citizen science and crowdsourced data
All elements of risk may be better quantified or qualified using citizen science and crowdsourced data, which are utilised across a number of natural hazards (Hicks et al., 2019). De Bruijn et al. (2018) already showed that passively shared information through social media platforms can provide a plethora of qualitative and sometimes quantitative information of flood hazards, as well as impacts in near-real time. Also, active sharing mechanisms are becoming available. For example, citizen scientists report information on landslide hazard that is otherwise difficult to obtain. At the global scale, volunteers can contribute information through Landslide Reporter ( https://landslides.nasa.gov/reporter , last access: 30 November 2019). Numerous local, regional, or national crowdsourcing projects have also been undertaken (Juang et al., 2019). Information on landslide timing is often missing from existing landslide inventories, because neither remote sensing nor geologic fieldwork determines this feature (Kocaman and Gokceoglu, 2018). However, precise knowledge of landslide timing is crucial for research into the triggering mechanisms of landslides. Citizen scientists could remedy this in cases where they have first-hand knowledge of recent events. Citizen science has been applied in volcanic environments, from observations of ash fall (Wallace et al., 2015) to community-based monitoring (Stone et al., 2014), and has a well-established application in earthquakes (e.g. USGS “Did You Feel It?”).
An exciting avenue in citizen science lies in more active mechanisms to report on hazards and their impacts (damages, households affected, people in need of help, and so on). In particular, where such reports concern citizens' own property or surroundings, they are likely to be more motivated and more accurate in their reporting. By having an active reporting mechanism, this can lead to a more structured approach to monitor and keep track of past hazards and their impacts. Opportunities lie in particular in reporting local flash floods, local landslides, and local drought conditions such as small reservoir states for rainfed farming and grass states for pastoralists. These natural hazard conditions concern time and space scales that are too small to capture with other means but may occur frequently and in many locations. Structured and organised data collection by citizens is taking place more and more. Large-scale community mapping projects are initiated that rapidly increase the availability of well-organised taxonomic information on buildings and infrastructure (e.g. Soden and Palen, 2014; Iliffe et al., 2017). These may serve as exposure and vulnerability databases for multiple natural hazards, as well as drainage information, which can be used to establish flood hazard models (Winsemius et al., 2019). The tools and data platforms to collect, store, and share such data in any resource setting are broadly available and include Open Data Kit (Brunette et al., 2013) and OpenStreetMap (Haklay and Weber, 2008). These data may be used for instance to train machine-learning algorithms that estimate exposure and vulnerability characteristics based on remote sensing or to keep risk models in rapidly changing environments, such as growing urban centres, up to date (Winsemius et al., 2019). These opportunities have been explored only to a limited extent, and we foresee a growth in demand of these research directions.
4.7 Concluding remarks
As shown by this review, efforts to assess and map natural hazard risk at the global scale have increased considerably in the last decade, in an attempt to contribute to the Sendai Framework's first Priority for Action of Understanding Disaster Risk. This paper presents a first attempt to review global risk studies across different hazards, thereby examining similarities and differences between the approaches taken within and across the different hazards and identifying potential ways in which different communities can continue to exchange knowledge and approaches, for example through UNDRR's Global Risk Assessment Framework and Global Platforms or the EGU session on “Global and continental scale risk assessment for natural hazards: methods and practice”. We also indicate several opportunities for addressing some of the pressing challenges in global risk modelling. We hope that this review paper can serve to encourage further dialogue on knowledge sharing between scientists and communities working on different hazards and at different spatial scales that has been facilitated by the session “Global and continental scale risk assessment for natural hazards: methods and practice” at the EGU General Assembly since 2012.
No data sets were used in this article.
The supplement related to this article is available online at: https://doi.org/10.5194/nhess-20-1069-2020-supplement .
All authors contributed to reviewing the literature and writing the paper. PJW furthermore coordinated the review and writing process.
The authors declare that they have no conflict of interest.
This article is part of the special issue “Global- and continental-scale risk assessment for natural hazards: methods and practice”. It is a result of the European Geosciences Union General Assembly 2018, Vienna, Austria, 8–13 April 2018.
Melanie J. Duncan publishes with the permission of the executive director of the British Geological Survey (UKRI).
Philip J. Ward, Marleen de Ruiter, and Nadia Bloemendaal received funding from the Dutch Research Council (NWO) in the form of a VIDI grant (grant no. 016.161.324) and VICI grant (grant no. 453.13.006). Veit Blauhut is funded by the DRIeR project funded under the framework of the Water Research Network by the Ministry of Science, Research, and Arts of the German Federal State of Baden Württemberg (AZ. 7532.21/2.1.6). Robert Emberson is supported by an appointment to the NASA Postdoctoral Program at the Goddard Space Flight Center.
This paper was edited by Bruno Merz and reviewed by Francesco Dottori and Brian Walsh.
Aerts, J. C. J. H., Botzen, W. J., Clarke, K. C., Cutter, S. L., Hall, J. W., Merz, B., Michel-Kerjan, E., Mysiak, J., Surminski, S., and Kunreuther, H.: Integrating human behaviour dynamics into flood disaster risk assessment, Nat. Clim. Change, 8, 193–199, https://doi.org/10.1038/s41558-018-0085-1 , 2018.
AghaKouchak, A., Huning, L. S., Chiang, F., Sadegh, M., Vahedifard, F., Mazdiyasni, O., Moftakhari, H., and Mallakpour, I.: How do natural hazards cascade to cause disasters?, Nature, 561, 458–460, https://doi.org/10.1038/d41586-018-06783-6 , 2018.
Ahlström, A., Schurgers, G., Arneth, A., and Smith, B.: Robustness and uncertainty in terrestrial ecosystem carbon response to CMIP5 climate change projections, Environ. Res. Lett., 7, 044008, https://doi.org/10.1088/1748-9326/7/4/044008 , 2012.
Alfieri, L., Bisselink, B., Dottori, F., Naumann, G., de Roo, A., Salamon, P., Wyser, K., and Feyen, L.: Global projections of river flood risk in a warmer world, Earth's Future, 5, 171–182, https://doi.org/10.1002/2016EF000485 , 2017.
Allen, J. T., Tippett, M. K., Sobel, A. H., and Lepore, C.: Understanding the drivers of variability in severe convection: Bringing together the scientific and insurance communities, B. Am. Meteorol. Soc., 97, ES221–ES223, https://doi.org/10.1175/BAMS-D-16-0208.1 , 2016.
Allen, J. T., Giammanco, I. M., Kumjian, M. R., Punge, H. J., Zhang, Q., Groenemeijer, P., Kunz, M., and Ortega, K.: Understanding hail in the earth system, Rev. Geophys., 58, e2019RG000665, https://doi.org/10.1029/2019RG000665 , 2020.
Arnell, N. W. and Gosling, S. N.: The impacts of climate change on river flood risk at the global scale, Climatic Change, 134, 387–401, https://doi.org/10.1007/s10584-014-1084-5 , 2016.
Arnell, N. W. and Lloyd-Hughes, B.: The global-scale impacts of climate change on water resources and flooding under new climate and socio-economic scenarios, Climatic Change, 122, 127–140, https://doi.org/10.1007/s10584-013-0948-4 , 2014.
Arnell, N. W., Lowe, J. A., Brown, S., Gosling, S. N., Gottschalk, P., Hinkel, J., Lloyd-Hughes, B., Nicholls, R. J., Osborn, T. J., Osborne, T. M., Rose, G. A., Smith, P., and Warren, R. F.: A global assessment of the effects of climate policy on the impacts of climate change, Nat. Clim. Change, 3, 512–519, 2013.
Arnell, N. W., Lowe, J. A., Lloyd-Hughes, B., and Osborn, T. J.: The impacts avoided with a 1.5 ∘ C climate target: a global and regional assessment, Climatic Change, 147, 61–76, https://doi.org/10.1007/s10584-017-2115-9 , 2018.
Auker, M. R., Sparks, R. S. J., Siebert, L., Crosweller, H. S., and Ewert, J.: A statistical analysis of the global historical volcanic fatalities record, J. Appl. Volcanol., 2, 1–24, 2013.
Auker, M. R., Sparks, R. S. J., Jenkins, S. F., Aspinall, W. P., Brown, S. K., Deligne, N. I., Jolly, G., Loughlin, S. C., Marzocchi, W., Newhall, C. G., and Palma, J. L.: Development of a new global Volcanic Hazard Index (VHI), in: Global Volcanic Hazards and Risk, edited by: Loughlin, S. C., Sparks, R. S. J., Brown, S. K., Jenkins, S. F., and Vye-Brown, C., Cambridge University Press, Cambridge, 2015.
Badoux, A., Andres, N., and Turowski, J. M.: Damage costs due to bedload transport processes in Switzerland, Nat. Hazards Earth Syst. Sci., 14, 279–294, https://doi.org/10.5194/nhess-14-279-2014 , 2014.
Barclay, J., Few, R., Armijos, M. T., Phillips, J. C., Pyle, D. M., Hicks, A., Brown, S. K., and Robertson, R. E. A.: Livelihoods, wellbeing and the risk to life during volcanic eruptions, Front. Earth Sci., 7, 205, https://doi.org/10.3389/feart.2019.00205 , 2019.
Beck, M. W., Losada, I. J., Menéndez, P., Reguero, B. G., Díaz-Simal, P., and Fernández, F: The global flood protection savings provided by coral reefs, Na. Commun., 9, 2186, https://doi.org/10.1038/s41467-018-04568-z , 2018.
Berryman, K.: Review of tsunami hazard and risk in New Zealand, Report no. CR2005-104, Institute of Geological & Nuclear Sciences, Lower Hutt, 2005.
Bevacqua, E., Maraun, D., Vousdoukas, M. I., Voukouvalas, E., Vrac, M., Mentaschi, L., and Widmann, M.: Higher probability of compound flooding from precipitation and storm surge in Europe under anthropogenic climate change, Sci. Adv., 5, eaaw5531, https://doi.org/10.1126/sciadv.aaw5531 , 2019.
Bloemendaal, N., Haigh, I. D., De Moel, H., Muis, S., Haarsma, R. J., and Aerts, J. C. J. H.: Generation of a global synthetic tropical cyclone hazard dataset using STORM, Sci. Data, 7, 40, https://doi.org/10.1038/s41597-020-0381-2 , 2020.
Blong, R.: A new damage index, Nat. Hazards, 30, 1–23, https://doi.org/10.1023/A:1025018822429 , 2003.
Bonadonna, C., Biass, S., Calder, E. S., Frischknecht C., Gregg, C. E., Jenkins, S. F., Loughlin, S. C., Menoni, S., Takarada, S., and Wilson, T., 2018, in: 1st IAVCEI/GVM Workshop: From Volcanic Hazard to Risk Assessment, 27–29 June 2018, Geneva, available at: https://vhub.org/resources/4498 (last access: 30 November 2019), 2018.
Bradley, K, Mallick, R., Andikagumi, H., Hubbard, J., Meilianda, E., Switzer, A., Du, N., Brocard, G., Alfian, D., Benazir, B., Feng, G., Yun, S.-H., Majewski, J., Wei, S., and Hill, E. M.: Earthquake-triggered 2018 Palu Valley landslides enabled by wet rice cultivation, Nat. Geosci., 12, 935–939, https://doi.org/10.1038/s41561-019-0444-1 , 2019.
Bright, E. A., Coleman, P. R., Rose, A. N., and Urban, M. L.: LandScan 2010, Oak Ridge National Laboratory, Oak Ridge, TN, 2011.
Bright, E. A., Rose, A. N., Urban, M. L., and McKee, J. J.: LandScan 2016 High-Resolution Global Population Data Set, Oak Ridge National Laboratory, Oak Ridge, TN, 2017.
Brown, S. K., Auker, M. R., and Sparks, R. S. J.: Populations around Holocene volcanoes and development of a Population Exposure Index, in: Global Volcanic Hazards and Risk, edited by: Loughlin, S. C., Sparks, R. S. J., Brown, S. K., Jenkins, S. F., and Vye-Brown, C., Cambridge University Press, Cambridge, 2015a.
Brown, S. K., Sparks, R., and Jenkins, S.: Global distribution of volcanic threat, in: Global Volcanic Hazards and Risk, Cambridge University Press, Cambridge, 2015b.
Brown, S. K., Jenkins, S. F., Sparks, R. S. J., Odbert, H., and Auker, M. R.: Volcanic fatalities database: analysis of volcanic threat with distance and victim classification, J. Appl. Volcanol., 6, 15, https://doi.org/10.1186/s13617-017-0067-4 , 2017.
Brunette, W., Sundt, M., Dell, N., Chaudhri, R., Breit, N., and Borriello, G.: Open Data Kit 2.0: Expanding and refining information services for developing regions, in: Proceedings of the 14th Workshop on Mobile Computing Systems and Applications, New York, https://doi.org/10.1145/2444776.2444790 , 2013.
Cammerer, H., Thieken, A. H., and Verburg, P. H.: Spatio-temporal dynamics in the flood exposure due to land use changes in the Alpine Lech Valley in Tyrol (Austria), Nat. Hazards, 68, 1243–1270, https://doi.org/10.1007/s11069-012-0280-8 , 2013.
Cao, X., Meng, Y., and Chen, J.: Mapping Grassland Wildfire Risk of the World, in: World Atlas of Natural Disaster Risk, IHDP/Future Earth-Integrated Risk Governance Project Series, edited by: Shi, P. and Kasperson, R., Springer, Berlin, Heidelberg, 2015.
Cardona, O. D., Ordaz, M. G., Mora, M. G., Salgado-Gálvez, M. A., Bernal, G. A., and Zuloaga-Romero, D.: Global risk assessment: A fully probabilistic seismic and tropical cyclone wind risk assessment, Int. J. Disast. Risk Reduct., 10, 461–476, https://doi.org/10.1016/j.ijdrr.2014.05.006 , 2014.
Carrão, H., Naumann, G., and Barbosa, P.: Mapping global patterns of drought risk: An empirical framework based on sub-national estimates of hazard, exposure and vulnerability, Global Environ. Change, 39, 108–124, https://doi.org/10.1016/j.gloenvcha.2016.04.012 , 2016.
Cecil, D. J., Buechler, D. E., and Blakeslee, R. J.: Gridded lightning climatology from TRMM-LIS and OTD: Dataset description, Atmos. Res., 135, 404–414, https://doi.org/10.1016/j.atmosres.2012.06.028 , 2014.
Chan, L.S., Chen, Y., Chen, Q., Chen, L., Liu, J., Dong, W., and Shah, H.: Assessment of global seismic loss based on macroeconomic indicators, Nat. Hazards, 17, 269–283, https://doi.org/10.1023/A:1008060510137 , 1998.
Christenson, E., Elliott, M., Banerjee, O., Hamrick, L., and Bartram, J.: Climate-related hazards: a method for global assessment of urban and rural population exposure to cyclones, droughts, and floods, Int. J. Environ. Res. Publ. Health, 11, 2169–2192, https://doi.org/10.3390/ijerph110202169 , 2014.
Christian, H. J., Blakeslee, R. J., Boccippio, D. J., Boeck, W. L., Buechler, D. E., Driscoll, K. T., Goodman, S. J., Hall, J. M., Koshak, W. J., Mach, D. M., and Stewart, M. F.: Global frequency and distribution of lightning as observed from space by the Optical Transient Detector, J. Geophys. Res., 108, 4005, https://doi.org/10.1029/2002JD002347 , 2003.
Chuvieco, E., Yue, C., Heil, A., Mouillot, F., Alonso-Canas, I., Padilla, M., Pereira, J. M., Oom, D., and Tansey, K.: A new global burned area product for climate assessment of fire impacts, Global Ecol. Biogeogr., 25, 619–629, https://doi.org/10.1111/geb.12440 , 2016.
CIESIN: Columbia University Center for International Earth Science Information Network Gridded Population of the World, Version 4 (GPWv4): Population Count, CIESIN, Columbia University, Palisades, New York, https://doi.org/10.7927/H4X63JVC , 2016.
Ciscar, J.-C., Rising, J., Kopp, R. E., and Feyen, L.: Assessing future climate change impacts in the EU and the USA: insights and lessons from two continental-scale projects, Environ. Res. Lett., 14, 084010, https://doi.org/10.1088/1748-9326/ab281e , 2019.
Corlett, R. T.: Impacts of warming on tropical lowland rainforests, Trends Ecol. Evol., 26, 606–613, https://doi.org/10.1016/j.tree.2011.06.015 , 2011.
Couasnon, A., Eilander, D., Muis, S., Veldkamp, T. I. E., Haigh, I. D., Wahl, T., Winsemius, H. C., Ward, P. J.: Measuring compound flood potential from river discharge and storm surge extremes at the global scale and its implications for flood hazard, Nat. Hazards Earth Syst. Sci., 20, 489–504, https://doi.org/10.5194/nhess-20-489-2020 , 2020.
CRED: EM-DAT. The Emergency Events Database, Université catholique de Louvain (UCL) – CRED, Brussels, available at: https://www.emdat.be/ (last access: 30 November 2019), 2020.
CRED and UNISDR: Economic losses, poverty and disasters 1998–2017, CRED, Brussels and UNISDR, Geneva, available at: https://www.unisdr.org/2016/iddr/IDDR2018_Economic Losses.pdf (last access: 30 November 2019), 2018.
Cutter, S. L. and Finch, C.: Temporal and spatial changes in social vulnerability to natural hazards, P. Natl. Acad. Sci. USA, 105, 2301–2306, https://doi.org/10.1073/pnas.0710375105 , 2008.
Cutter, S. L., Ismail-Zadeh, A., Alcántara-Ayala, I., Altan, O., Baker, D. N., Briceño, S., Gupta, H., Holloway, A., Johnston, D., McBean, G. A., Ogawa, Y., Paton, D., Porio, E., Silbereisen, R. K., Takeuchi, K., Valsecchi, G. B., Vogel, V., and Wu, G.: Global risks: Pool knowledge to stem losses from disasters, Nature, 522, 277–279, https://doi.org/10.1038/522277a , 2015.
Daniell, J. E.: The development of socio-economic fragility functions for use in worldwide rapid earthquake loss estimation procedures, Doctoral Thesis, Karlsruhe Institute of Technology, Karlsruhe, 2014.
Daniell, J. E.: The CATDAT Integrated Natural Catastrophes Database, Karlsruhe, available at: http://www.risklayer.com/en/service/catdat/ (last access: 30 November 2019), 2020.
Daniell, J. E. and Wenzel, F.: The production and implementation of socioeconomic fragility functions for use in rapid worldwide earthquake loss estimation, Paper No. 490, in: 15th ECEE, Istanbul, 2014.
De Bono, A. and Chatenoux, B.: A Global Exposure Model for GAR 2015 Background Paper prepared for the 2015 Global Assessment Report on Disaster Risk Reduction, UNDRR, Geneva, 2015.
De Bruijn, J. A., de Moel, H., Jongman, B., Wagemaker, J., and Aerts, J. C. J. H.: TAGGS: Grouping Tweets to Improve Global Geoparsing for Disaster Response, J. Geovisual. Spat. Anal., 2, 1–14, https://doi.org/10.1007/s41651-017-0010-6 , 2018.
De Risi, R. and Goda, K.: Simulation-based probabilistic tsunami hazard analysis: empirical and robust hazard predictions, Pure Appl. Geophys., 174, 3083–3106, 2017.
De Ruiter, M. C., Couasnon, A., Van den Homberg, M. J. C., Daniell, J. E., Gill, J. C., and Ward, P. J.: Why we can no longer ignore consecutive disasters, Earths Future, 8, e2019EF001425, https://doi.org/10.1029/2019EF001425 , 2020a.
De Ruiter, M. C., De Bruijn, J. A., Englhardt, J., Daniell, J. W., Ward, P. J., and De Moel, H.: The asynergies of disaster risk reduction measures: comparing floods and earthquakes, in review, 2020b.
Di Baldassarre, G., Viglione, A., Carr, G., Kuil, L., Yan, K., Brandimarte, L., and Blöschl, G.: Debates-Perspectives on socio-hydrology: Capturing feedbacks between physical and social processes, Water Resour. Res., 51, 4770–4781, https://doi.org/10.1002/2014WR016416 , 2015.
Di Baldassarre, G., Kreibich, H., Vorogushyn, S., Aerts, J., Arnbjerg-Nielsen, K., Barendrecht, M., Bates, P., Borga, M., Botzen, W., Bubeck, P., De Marchi, B., Carmen Llasat, M., Mazzoleni, M., Molinari, D., Mondino, E., Mård, J., Petrucci, O., Scolobig, A., Viglione, A., and Ward, P. J.: An interdisciplinary research agenda to explore the unintended consequences o f structural flood protection, Hydrol. Earth Syst. Sci., 22, 5629–5637, https://doi.org/10.5194/hess-22-5629-2018 , 2018.
Dilley, M., Chen, R. S., Deichmann, U., Lerner-Lam, A., Arnold, M., Agwe, J., Buys, P., Kjekstad, O., Lyon, B., and Yetman, G.: Natural Disaster Hotspots. A Global Risk Analysis, The World Bank, Washington, D.C., 2005.
Dottori, F., Szewczyk, W., Ciscar, J. C., Zhao, F., Alfieri, L., Hirabayashi, Y., Bianchi, A., Mongelli, I., Frieler, K., Betts, R. A., and Feyen, L.: Increased human and economic losses from river flooding with anthropogenic warming, Nat. Clim. Change, 8, 781–786, https://doi.org/10.1038/s41558-018-0257-z , 2018.
Emanuel, K. and Nolan, D. S.: Tropical cyclone activity and the global climate system, in: 26th Conference on Hurricanes and Tropical Meteorology, 2–7 May 2004, Miami, USA, 240–241, 2004.
Emanuel, K., Sundararajan, R., and William, J.: Hurricanes and global warming: Results from downscaling IPCC AR4 simulations, B. Am. Meterol. Soc., 89, 347–367, https://doi.org/10.1175/BAMS-89-3-347 , 2008.
Englhardt, J., De Moel, H., Huyck, C. K., De Ruiter, M. C., and Aerts, J. C. J. H.: Enhancement of large-scale flood risk assessments using building-material-based vulnerability curves for an object-based approach in urban and rural areas, Nat. Hazards Earth Syst. Sci., 19, 1703–1722, https://doi.org/10.5194/nhess-19-1703-2019 , 2019.
Fang, J., Sun, S., and Shi, P.: Assessment and mapping of potential storm surge impacts on global population and economy, Int. J. Disast. Risk Sci., 5, 323–331, https://doi.org/10.1007/s13753-014-0035-0 , 2014.
Fang W., Tan, C., Lin, W., Wu, X., Ye, Y., Cao, S., Mo, W., Li, Y., Li, Y., Wu, Y., Lin, G., and Yang, Y.: Mapping Tropical Cyclone Wind Risk of the World, in: World Atlas of Natural Disaster Risk, IHDP/Future Earth-Integrated Risk Governance Project Series, edited by: Shi, P. and Kasperson, R., Springer, Berlin, Heidelberg, 2015.
Flannigan, M. D., Krawchuk, M. A., De Groot, W. J., Wotton, B. M., and Gowman, L. M.: Implications of changing climate for global wildland fire, Int. J. Wildland Fire, 18, 483–507, https://doi.org/10.1071/WF08187 , 2009.
Freire, S., Kemper, T., Pesaresi, M., Florczyk, A., and Syrris, V.: Combining GHSL and GPW to improve global population mapping, in: 2015 IEEE International Geoscience & Remote Sensing Symposium Proceedings, 26–31 July 2015, Milan, Italy, 2541–2543, 2015.
Freire, S., Florczyk, A. J., Pesaresi, M., and Sliuzas, R.: An Improved Global Analysis of Population Distribution in Proximity to Active Volcanoes, 1975–2015, ISPRS Int. J. Geo.-Inf., 8, 341, https://doi.org/10.3390/ijgi8080341 , 2019.
Froude, M. J. and Petley, D. N.: Global fatal landslide occurrence from 2004 to 2016, Nat. Hazards Earth Syst. Sci., 18, 2161–2181, https://doi.org/10.5194/nhess-18-2161-2018 , 2018.
Gallina, V., Torresan, S., Critto, A., Sperotto, A., Glade, T., and Marcomini, A.: A review of multi-risk methodologies for natural hazards: Consequences and challenges for a climate change impact assessment, J. Environ. Manage., 168, 123–132, https://doi.org/10.1016/j.jenvman.2015.11.011 , 2016.
GCOS: Systematic observation requirements for satellite-based products for climate, 2011 update, edn. WMO GCOS Rep. 154, WMO, Geneva, 2011.
Giglio, L., Loboda, T., Roy, D. P., Quayle, B., and Justice, C. O.: An active-fire based burned area mapping algorithm for the MODIS sensor, Remote Sens. Environ., 113, 408–420, https://doi.org/10.1016/j.rse.2008.10.006 , 2009.
Gill, J. C. and Malamud, B. D.: Reviewing and visualizing the interactions of natural hazards, Rev. Geophys., 52, 680–722, https://doi.org/10.1002/2013RG000445 , 2014.
Grezio, A., Gasparini, P., Marzocchi, W., Patera, A., and Tinti, S.: Tsunami risk assessments in messina, Sicily – Italy, Nat. Hazards Earth Syst. Sci., 12, 151–163, https://doi.org/10.5194/nhess-12-151-2012 , 2012.
Guerreiro, S. B., Glenis, V., Dawson, R. J., and Kilsby, C.: Pluvial flooding in European cities – A continental approach to urban flood modelling, Water, 9, 296, https://doi.org/10.3390/w9040296 , 2017.
Gunasekera, R., Ishizawa, O., Aubrecht, C., Blankespoor, B., Murray, S., Pomonis, A., and Daniell, J.: Developing an adaptive global exposure model to support the generation of country disaster risk profiles, Earth-Sci. Rev., 150, 594–608, https://doi.org/10.1016/j.earscirev.2015.08.012 , 2015.
Gunasekera, R., Daniell, J. E., Pomonis, A., Arias, R. A. D., Ishizawa, O., and Stone, H.: Methodology Note: The Global RApid post-disaster Damage Estimation (GRADE) approach, World Bank and GFDRR Technical Report, World Bank and GFDRR, Washington, D.C., 2018.
Güneralp, B., Güneralp, I., and Liu, Y.: Changing global patterns of urban exposure to flood and drought hazards, Global Environ. Change, 31, 217–225, https://doi.org/10.1016/j.gloenvcha.2015.01.002 , 2015.
Guo, H., Zhang, X., Lian, F., Gao, Y., Lin, D., and Wang, J.: Drought risk assessment based on vulnerability surfaces: a case study of maize, Sustainability, 8, 813, https://doi.org/10.3390/su8080813 , 2016.
Guzzetti, F., Cardinali, M., and Reichenbach, P.: The AVI project: a bibliographical and archive inventory of landslides and floods, Environ. Manage., 18, 623–633, 1994.
Haer, T., Botzen, W. J. W., and Aerts, J. C. J. H.: Advancing disaster policies by integrating dynamic adaptive behaviour in risk assessments using an agent-based modelling approach, Environ. Res. Lett., 14, 044022, https://doi.org/10.1088/1748-9326/ab0770 , 2019.
Haklay, M. and Weber, P.: OpenStreetMap: user-generated street maps, IEEE Pervasive Comput., 7, 12–18, https://doi.org/10.1109/MPRV.2008.80 , 2008.
Hall, T. M. and Kossin, J. P.:. Hurricane stalling along the North American coast and implications for rainfall, Clim. Atmos. Sci., 2, 17, https://doi.org/10.1038/s41612-019-0074-8 , 2019.
Hallegatte, S., Green, C., Nicholls, R. J., and Corfee-Morlot, J.: Future flood losses in major coastal cities, Nat. Clim. Change, 3, 802–806, https://doi.org/10.1038/nclimate1979 , 2013.
Hallegatte, S., Bangalore, M., Bonzanigo, L., Fay, M., Kane, T., Narloch, U., Rozenberg, J., Treguer, D., and Vogt-Schilb, A.: Shock Waves: Managing the Impacts of Climate Change on Poverty, World Bank, Washington, D.C., 2016.
Hallegatte, S., Vogt-Schilb, A., Bangalore, M., and Rozenberg, J.: Unbreakable: Building the Resilience of the Poor in the Face of Natural Disasters, World Bank, Washington, D.C., 2017.
Hasegawa, T., Fujimori, S., Ito, A., Takahashi, K., and Masui, T.: Global land-use allocation model linked to a n integrated assessment model, Sci. Total Environ., 580, 787–796, https://doi.org/10.1016/j.scitotenv.2016.12.025 , 2017.
Hicks, A., Barclay, J., Chilvers, J., Armijos, M. T., Oven, K., Simmons, P., and Haklay, M.: Global mapping of citizen science projects for Disaster Risk Reduction, Front. Earth Sci., 7, 1–18, https://doi.org/10.3389/feart.2019.00226 , 2019.
Hinkel, J. and Klein, R. J. T.: Integrating knowledge to assess coastal vulnerability to sea-level rise: The development of the DIVA tool, Global Environ. Change, 19, 384–395, https://doi.org/10.1016/j.gloenvcha.2009.03.002 , 2009.
Hinkel, J., Nicholls, R. J., Vafeidis, A. T., Tol, R. S. J., and Avagianou, T.: Assessing risk of and adaptation to sea-level rise in the European Union: an application of DIVA, Mitig. Adapt. Strateg. Glob. Change, 15, 703–719, https://doi.org/10.1007/s11027-010-9237-y , 2010.
Hinkel, J., Lincke, D., Vafeidis, A. T., Perrette, M., Nicholls, R. J., Tol, R. S. J., Marzeion, B., Fettweis, X., Ionescu, C., and Levermann, A.: Coastal flood damage and adaptation costs under 21st century sea-level rise, P. Natl. Acad. Sci. USA, 111, 3292–3297, https://doi.org/10.1073/pnas.1222469111 , 2014.
Hirabayashi, Y. and Kanae, S.: First estimate of the future global population at risk of flooding, Hydrolog. Res. Lett., 3, 6–9, https://doi.org/10.3178/HRL.3.6 , 2009.
Hirabayashi, Y., Mahendran, R., Koirala, S., Konoshima, L., Yamazaki, D., Watanabe, S., Kim, H., and Kanae, S.: Global flood risk under climate change, Nat. Clim. Change, 3, 816–821, https://doi.org/10.1038/nclimate1911 , 2013.
Holland, G. J.: An analytic model of the wind and pressure profiles in hurricanes, Mon. Weather Rev., 108, 1212–1218, https://doi.org/10.1175/1520-0493(1980)108<1212:AAMOTW>2.0.CO;2 , 1980.
Holmes, J. D.: Wind loading of structures, CRC Press, Boca Raton, 2015.
Hoozemans, F. M. J., Marchand, M., and Pennekamp, H. A.: Sea Level Rise: A Global Vulnerability Assessment (GVA) – vulnerability assessment for population, coastal wetlands and rice production on a global scale, Delft Hydraulics and Rijkswaterstaat, Delft and The Hague, 1993.
Huang, C.: Principle of information diffusion, Fuzzy Set. Syst., 91, 69–90, https://doi.org/10.1016/S0165-0114(96)00257-6 , 1997.
Huang, C. F.: Risk analysis and management of natural disasters, Science Press, Beijing, 2012.
Hunter, J. R., Woodworth, P. L., Wahl, T., and Nicholls, R. J.: Using global tide gauge data to validate and improve the representation of extreme sea levels in flood impact studies, Global Planet. Change, 156, 34–45, https://doi.org/10.1016/j.gloplacha.2017.06.007 , 2017.
Ikeuchi, H., Hirabayashi, Y., Yamazaki, D., Muis, S., Ward, P. J., Winsemius, H. C., Verlaan, M., and Kanae, S.: Compound simulation of fluvial floods and storm surges in a global coupled river-coast flood model: Model development and its application to 2007 Cyclone Sidr in Bangladesh, J. Adv. Model. Earth Syst., 9, 1847–1862, https://doi.org/10.1002/2017MS000943 , 2017.
Iliffe, M., Goulding, J., and Winsemius, H. C.: Towards a conceptual framework for participatory mapping in developing countries, GISRUK 2017, Manchester, 2017.
Jaiswal, K. and Wald, D.: An empirical model for global earthquake fatality estimation, Earthq. Spectra, 26, 1017–1037, https://doi.org/10.1193/1.3480331 , 2010.
Jaiswal, K. S. and Wald, D. J.: Rapid estimation of the economic consequences of global earthquakes, US Geological Survey Open-File Report 2011-1116, USGS, Denver, 2011.
Jelínek, R., Krausmann, E., Gonzalez, M., Álvarez-Gómez, J., Birkmann, J., and Welle, T.: Approaches for tsunami risk assessment and application to the city of Cádiz, Spain, Nat. Hazards, 60, 273–293, https://doi.org/10.1007/s11069-011-0009-0 , 2012.
Jenkins, S., Magill, C., McAneney, J., and Blong, R.: Regional ash fall hazard I: A probabilistic assessment methodology, Bull. Volcanol., 74, 1699–1712, https://doi.org/10.1007/s00445-012-0627-8 , 2012.
Jenkins, S. F., Spence, R. J. S., Fonseca, J. F. B. D., Solidum, R. U., and Wilson, T. M.: Volcanic risk assessment: Quantifying physical vulnerability in the built environment, J. Volcanol. Geoth. Res., 276, 105–120, https://doi.org/10.1016/j.jvolgeores.2014.03.002 , 2014.
Jenkins, S. F., Wilson, T. M., Magill, C. R., Miller, V., Stewart, C., Blong, R., Marzocchi, W., Boulton, M., Bonadonna, C. and Costa, A.: Volcanic ash fall hazard and risk, n: Global Volcanic Hazards and Risk, edited by: Loughlin, S. C., Sparks, R. S. J., Brown, S. K., Jenkins, S. F., and Vye-Brown, C., Cambridge University Press, Cambridge, 2015.
Jolly, W. M., Cochrane, M. A., Freeborn, P. H., Holden, Z. A., Brown, T. J., Williamson, G. J., and Bowman, D. M. J. S.: Climate-induced variations in global wildfire danger from 1979 to 2013, Nat. Commun., 6, 7537, https://doi.org/10.1038/ncomms8537 , 2015.
Jongman, B., Ward, P. J., and Aerts, J. C. J. H.: Global exposure to river and coastal flooding: long term trends and changes, Global Environ. Change, 22, 823–835, https://doi.org/10.1016/j.gloenvcha.2012.07.004 , 2012.
Jongman, B., Winsemius, H. C., Aerts, J. C. J. H., Coughlan de Perez, E., Van Aalst, M. K., Kron, W., and Ward, P. J.: Declining vulnerability to river floods and the global benefits of adaptation, P. Natl. Acad. Sci. USA, 112, E227—E2280, https://doi.org/10.1073/pnas.1414439112 , 2015.
Juang, C. S., Kirschbaum, D. B., and Stanley, T.: Using citizen science to expand the global map of landslides: Introducing the Cooperative Open Online Landslide Repository (COOLR), PLOS One, 14, e0218657, https://doi.org/10.1371/journal.pone.0218657 , 2019.
Kirschbaum, D. and Stanley, T.: Satellite-Based Assessment of Rainfall-Triggered Landslide Hazard for Situational Awareness, Earth's Future, 6, 505–523, https://doi.org/10.1002/2017EF000715 , 2018.
Kirschbaum, D. B., Adler, R., Hong, Y., Hill, S., and Lerner-Lam, A.: A global landslide catalog for hazard applications: method, results, and limitations, Nat. Hazards, 52, 561–575, https://doi.org/10.1007/s11069-009-9401-4 , 2010.
Kleinen, T. and Petschel-Held, G.: Integrated assessment of changes in flooding probabilities due to climate change, Climatic Change, 81, 283–312, https://doi.org/10.1007/s10584-006-9159-6 , 2007.
Knapp, K. R., Kruk, M. C., Levinson, D. H., Diamond, H. J., and Neumann, C. J.: The International Best Track Archive for Climate Stewardship (IBTrACS): Unifying tropical cyclone data, B. Am. Meteorol. Soc., 91, 363–376, https://doi.org/10.1175/2009BAMS2755.1 , 2010.
Knorr, W., Kaminski, T., Arneth, A., and Weber, U.: Impact of human population density on fire frequency at the global scale, Biogeosciences, 11, 1085–1102, https://doi.org/10.5194/bg-11-1085-2014 , 2014.
Knorr, W., Arneth, A., and Jiang, L.: Demographic controls of future global fire risk, Nat. Clim. Change, 6, 781–785, 2016.
Kocaman, S. and Gokceoglu, C.: Possible contributions of citizen science for landslide hazard assessment, Int. Arch. Photogramm., 42, 295–300, https://doi.org/10.5194/isprs-archives-XLII-3-W4-295-2018 , 2018.
Koks, E. E., Rozenberg, J., Zorn, C., Tariverdi, M., Vousdoukas, M., Fraser, S. A., Hall, J. W., and Hallegatte, S.: A global multi-hazard risk analysis of road and railway infrastructure assets, Nat. Commun., 10, 2677, https://doi.org/10.1038/s41467-019-10442-3 , 2019.
Korswagen, P. A., Jonkman, S. N., and Terwel, K. C.: Probabilistic assessment of structural damage from coupled multi-hazards, Struct. Saf., 76, 135–148, https://doi.org/10.1016/j.strusafe.2018.08.001 , 2019.
Kossin, J. P.: A global slowdown of tropical-cyclone translation speed, Nature, 558, 104–107, https://doi.org/10.1038/s41586-018-0158-3 , 2018.
Kreibich, H., Blauhut, V., Aerts, J. C. J. H., Bouwer, L. M., Van Lanen, H. A., Mejia, A., Mens, M., and Van Loon, A. F.: How to improve attribution of changes in drought and flood impacts, Hydrolog. Sci. J., 64, 1–18, 2019.
Kron, W., Steuer, M., Löw, P., and Wirtz, A.: How to deal properly with a natural catastrophe database – analysis of flood losses, Nat. Hazards Earth Syst. Sci., 12, 535–550, https://doi.org/10.5194/nhess-12-535-2012 , 2012.
Kunz, M. and Geissbuehler, P.: Severe convective storm, in: Natural Catastrophe Risk Management and Modelling: A Practitioner's Guide, edited by: Mitchell-Wallace, K., Jones, M., Hillier, J., and Foote, M., John Wiley & Sons Ltd., Chicherster, 2017.
Lallemant, D.: Modeling the future disaster risk of cities to envision paths towards their future resilience, Stanford University, Stanford, 2015.
LandScan: LandScan™ Global, Oak Ridge National Laboratory, Oak Ridge, available at: http://www.ornl.gov/sci/landscan/ (last access: 30 November 2019), 2008.
Landsea, C. W. and Franklin, J. L.: Atlantic hurricane database uncertainty and presentation of a new database format, Mon. Weather Rev., 141, 3576–3592, https://doi.org/10.1175/MWR-D-12-00254.1 , 2013.
Laurance, W. F. and Williamson, G. B.: Positive feedbacks among forest fragmentation, drought, and climate change in the Amazon, Conserv. Biol., 15, 1529–1535, https://doi.org/10.1046/j.1523-1739.2001.01093.x , 2001.
Lee, C.-Y., Tippett, M. K., Sobel, A. H., and Camargo, S. J.: An Environmentally Forced Tropical Cyclone Hazard Model, J. Adv. Model. Earth Syst., 10, 223–241, https://doi.org/10.1002/2017MS001186 , 2018.
Li, M., Zou, Z., Xu, G., and Shi, P.: Mapping earthquake risk of the world, in: World Atlas of Natural Disaster Risk, IHDP/Future Earth-Integrated Risk Governance Project Series, edited by: Shi, P. and Kasperson, R., Springer, Berlin, Heidelberg, 2015.
Li, Y., Ye, W., Wang, M., and Yan, X.: Climate change and drought: a risk assessment of crop-yield impacts, Clim. Res., 39, 31–46, https://doi.org/10.3354/cr00797 , 2009.
Lindsay, J. M. and Robertson, R. E. A.: Integrating Volcanic Hazard Data in a Systematic Approach to Develop Volcanic Hazard Maps in the Lesser Antilles, Front. Earth Sci., 6, 42, https://doi.org/10.3389/feart.2018.00042 , 2018.
Liu, W., Sun F., Ho Lim, W., Zhang, J., Wang, H., Shiogama, H., and Zhang, Y.: Global drought and severe drought-affected populations in 1.5 and 2.0 ∘ C warmer worlds, Earth Syst. Dynam., 9, 267–283, https://doi.org/10.5194/esd-9-267-2018 , 2018.
Lloyd-Hughes, B.: The impracticality of a universal drought definition, Theor. Appl. Climatol., 117, 607–611, https://doi.org/10.1007/s00704-013-1025-7 , 2014.
Loughlin, S. C., Sparks, R. S. J., Brown, S. K., Jenkins, S. F., and Vye-Brown, C.: Global Volcanic Hazards and Risk, Cambridge University Press, Cambridge, 2015.
Løvholt, F., Griffin, J., and Salgado-Gálvez, M.: Tsunami Hazard and Risk Assessment on the Global Scale, in: Encyclopedia of Complexity and Systems Science, edited by: Meyers, R., Springer, Berlin, Heidelberg, 2015.
Maqsood, T., Wehner, M., Ryu, H., Edwards, M., Dale, K., and Miller, V.: GAR15 Vulnerability Functions. Reporting on the UNISDR/GA SE Asian Regional Workshop on Structural Vulnerability Models for the GAR Global Risk Assessment, Record 2014/38, 11–14 November 2013, Geoscience Australia, Canberra, Australia, https://doi.org/10.11636/Record.2014.038 , 2014.
Marc, O., Hovius, N., Meunier, P., Uchida, T., and Hayashi, S.: Transient Changes of Landslide Rates after Earthquakes, Geology, 43, 883–886, https://doi.org/10.1130/G36961.1 , 2015.
Martius, O., Hering, A., Kunz, M., Manzato, A., Mohr, S., Nisi, L., and Trefald, S.: Challenges and recent advances in hail research, B. Am. Meteorol. Soc., 99, ES51–ES54, https://doi.org/10.1175/BAMS-D-17-0207.1 , 2018.
Mendelsohn, R., Emanuel, K., Chonabayashi, S., and Bakkensen, L.: The impact of climate change on global tropical cyclone damage, Nat. Clim. Change, 2, 205–209, https://doi.org/10.1038/NCLIMATE1357 , 2012.
Meng, Y., Deng, Y., and Shi, P.: Mapping forest wildfire risk of the world, in: World Atlas of Natural Disaster Risk. IHDP/Future Earth-Integrated Risk Governance Project Series, edited by: Shi, P. and Kasperson, R., Springer, Berlin, Heidelberg, 2015.
Mirza, M. M. Q.: Climate change and extreme weather events: can developing countries adapt?, Clim. Policy, 3, 233–248, 2003.
Mora, C., Spirandelli, D., Franklin, E. C., Lynham, J., Kantar, M. B., Miles, W., Smith, C. Z., Freel, K., Moy, J., Louis, L. V., Barba, E. W., Bettinger, K., Frazier, A. G., Colburn IX, J. F., Hanasaki, N., Hawkins, E., Hirabayashi, Y., Knorr, W., Little, C. M., Emanuel, K., Sheffield, J., Patz, J. A., and Hunter, C. L.: Broad threat to humanity from cumulative climate hazards intensified by greenhouse gas emissions, Nat. Clim. Change, 8, 1062–1071, 2018.
Muis, S., Verlaan, M., Winsemius, H. C., Aerts, J. C. J. H., and Ward, P. J.: A global reanalysis of storm surges and extreme sea levels, Nat. Commun., 7, 11969, https://doi.org/10.1038/ncomms11969 , 2016.
Muis, S., Verlaan, M., Nicholls, R. J., Brown, S., Hinkel, J., Lincke, D., Vafeidis, T., Scussolini, P., Winsemius, H. C., and Ward, P. J.: A comparison of two global datasets of extreme sea levels and resulting flood exposure, Earth's Future, 5, 379–392, https://doi.org/10.1002/2016EF000430 , 2017.
Munich Re: NatCatSERVICE, Munich Re, Munich, available at: https://natcatservice.munichre.com/ (last access: 30 November 2019), 2020.
Murnane, R., Allegri, G., Bushi, A., Dabbeek, J., de Moel, H., Duncan, M., Fraser, S., Galasso, C., Giovando, C., Henshaw, P., Horsburgh, K., Huyck, C., Jenkins, S., Johnson, C., Kamihanda, G., Kijazi, J., Kikwasi, W., Kombe, W., Loughlin, S., Løvholt, F., Masanja, A., Mbongoni, G., Minas, S., Msabi, M., Msechu, M., Mtongori, H., Nadim, F., O'Hara, M., Pagani, M., Phillips, E., Rossetto, T., Rudari, R., Sangana, P., Silva, V., Twigg, J., Uhinga, G. Verrucci, E.: Data schemas for multiple hazards, exposure and vulnerability, Disast. Prevent. Manage., 28, 752–763, https://doi.org/10.1108/DPM-09-2019-0293 , 2019.
Nadim, F., Kjekstad, O., Peduzzi, P., Herold, C., and Jaedicke, C.: Global landslide and avalanche risk hotspots, The World Bank, Washington, D.C., 2004.
Nadim, F., Kjekstad, O., Peduzzi, P., Herold, C., and Jaedicke, C.: Global landslide and avalanche hotspots, Landslides, 3, 159–173, https://doi.org/10.1007/s10346-006-0036-1 , 2006.
Neri, M., Le Cozannet, G., Thierry, P., Bignami, C., and Ruch, J.: A method for multi-hazard mapping in poorly known volcanic areas: an example from Kanlaon (Philippines), Nat. Hazard. Earth Syst. Sci., 13, 1929–1943, https://doi.org/10.5194/nhess-13-1929-2013 , 2013.
Newhall, C., Costa, F., Ratdomopurbo, A., Venezky, D., Widiwijayanti, C., Win, N. T. Z., Tan, K., and Fajiculay, E.: WOVOdat – an online, growing library of worldwide volcanic unrest, J. Volcanol. Geoth. Res., 345, 184–199, https://doi.org/10.1016/j.jvolgeores.2017.08.003 , 2017.
Nowicki Jessee, M. A. N., Hamburger, M. W., Allstadt, K., Wald, D. J., Robeson, S. M., Tanyas, H., Hearne, M., and Thompson, E. M.: A Global Empirical Model for Near-Real-Time Assessment of Seismically Induced Landslides, J. Geophys. Res.-Earth, 123, 1835–1859, https://doi.org/10.1029/2017JF004494 , 2018.
Okumura, N., Jonkman, S. N., Esteban, M., Hoand, B., and Shibayama, T.: A method for tsunami risk assessment: a case study for Kamakura, Japan, Nat. Hazards, 88, 1451, https://doi.org/10.1007/s11069-017-2928-x , 2017.
Pan, H., Shi, P., Ye, T., Xu, W., and Wang, J.: Mapping the expected annual fatality risk of volcano on a global scale, Int. J. Disast. Risk Reduct., 13, 52–60, https://doi.org/10.1016/j.ijdrr.2015.03.004 , 2015.
Peduzzi, P., Dao, H., Herold, C., and Mouton, F.: Assessing global exposure and vulnerability towards natural hazards: the Disaster Risk Index, Nat. Hazards Earth Syst. Sci., 9, 1149–1159, https://doi.org/10.5194/nhess-9-1149-2009 , 2009.
Peduzzi, P., Chatenoux, B., Dao, H., De Bono, A., Herold, C., Kossin, J., Mouton, F., and Nordbeck, O.: Global trends in tropical cyclone risk, Nat. Clim. Change, 2, 289–294, https://doi.org/10.1038/NCLIMATE1410 , 2012.
Pesaresi, M., Ehrlich, D., Ferri, S., Florczyk, A. J., Freire, S., Halkia, M., Julea, A., Kemper, T., Soille, P., and Syrris, V.: Operating procedure for the production of the Global Human Settlement Layer from Landsat data of the epochs 1975, 1990, 2000, and 2014, JRC Technical Report EUR 27741 EN, European Commission Joint Research Centre, Ispra, Italy, https://doi.org/10.2788/253582 , 2016.
Petley, D.: Global patterns of loss of life from landslides, Geology, 40, 927–930, https://doi.org/10.1130/G33217.1 , 2012.
Pittore, M., Wieland, M., and Fleming, K.: Perspectives on global dynamic exposure modelling for geo-risk assessment, Nat. Hazards, 86, 7–30, https://doi.org/10.1007/s11069-016-2437-3 , 2017.
Punge, H., Werner, A., Bedka, K., and Kunz, M.: A new physically based stochastic event catalogue for hail in Europe, Nat. Hazards, 73, 1625–1645, https://doi.org/10.1007/s11069-014-1161-0 , 2014.
Riddell, G. A., Van Delden, H., Maier, H. R., and Zecchin, A. C.: Exploratory scenario analysis for disaster risk reduction: Considering alternative pathways in disaster risk assessment, Int. J. Disast. Risk Reduct., 39, 101230, https://doi.org/10.1016/j.ijdrr.2019.101230 , 2019.
Riley, K. L., Williams, A. P., Urbanski, S. P., Calkin, D. E., Short, K. C., and O'Connor, C. D.: Will Landscape Fire Increase in the Future? A Systems Approach to Climate, Fire, Fuel, and Human Drivers, Curr. Pollut. Rep., 5, 9–24, https://doi.org/10.1007/s40726-019-0103-6 , 2019.
Ritz, S.: Incorporating distributions of insurance loss data into a stochastic hail loss model, in: 2nd European Hail Workshop, 19–21 April 2017, Bern, 2017.
Sachs, J. D., Mellinger, A. D., and Gallup, J. L.: The Geography of Poverty and Wealth, Scient. Am., 284, 70–75, https://doi.org/10.1038/scientificamerican0301-70 , 2001.
Sampson, C. C., Smith, A. M., Bates, P. D., Neal, J. C., Alfieri, A., and Freer, J. E.: A high-resolution global flood hazard model, Water Resour. Res., 51, 7358–7381, https://doi.org/10.1002/2015WR016954 , 2015.
Sandri, L., Thouret, J.-C., Constantinescu, R., Biass, S., and Tonini, R.: Long-term multi-hazard assessment for El Misti volcano (Peru), Bull. Volcanol., 76, 1–26, https://doi.org/10.1007/s00445-013-0771-9 , 2014.
Schäfer, A. M.: Development of a Global Tsunami Risk Model, PhD thesis, Karlsruhe Institute of Technology, Karlsruhe, 2018.
Schmidberger, M.: Hagelgefährdung und Hagelrisiko in Deutschland basierend auf einer Kombination von Radardaten und Versicherungsdaten, in: Vol. 78, Wiss. Berichte d. Instituts für Meteorologie und Klimaforschung des Karlsruher Instituts für Technologie, KIT Scientific Publishing, Karlsruhe, 2018.
Schmidberger, M., Daniell, J. E., and Kunz, M.: Hail hazard and hail risk modeling for Germany based on a combination of radar and insurance data, in: 1st North American Workshop on Hail & Hailstorms, 14–16 August 2018, Boulder, Colorado, 2018.
Schuerch, M., Spencer, T., Temmerman, S., Kirwan, M. L., Wolff, C., Lincke, D., McOwen, C. J., Pickering, M. D., Reef, R., Vafeidis, A. T., Hinkel, J., Nicholls, R. J., and Brown, S.: Future response of global coastal wetlands to sea-level rise, Nature, 561, 231–234, https://doi.org/10.1038/s41586-018-0476-5 , 2018.
Schumann, G. J. P. and Bates, P. D.: The Need for a High-Accuracy, Open-Access Global DEM, Front. Earth Sci., 6, 225, https://doi.org/10.3389/feart.2018.00225 , 2018.
Scolobig, A., Komendantova, N., and Mignan, A.: Mainstreaming multi-risk approaches into policy, Geosciences, 7, 129, https://doi.org/10.3390/geosciences7040129 , 2017.
Shi, P. and Kasperson, R.: World Atlas of Natural Disaster Risk, in: IHDP/Future Earth-Integrated Risk Governance Project Series, Springer, Berlin, Heidelberg, 2015.
Silva, V., Crowley, H., Jaiswal, K., Acevedo, A. B., Pittore, M., and Journey, M.: Developing a global earthquake risk model , in: 16th European Conference on Earthquake Engineering, 18–21 June 2018, Thessaloniki, 2018.
Small, C. and Naumann, T.: The global distribution of human population and recent volcanism, Environ. Hazards, 3, 93–109, https://doi.org/10.1016/S1464-2867(02)00002-5 , 2001.
Smirnov, O., Zhang, M., Xiao, T., Orbell, J., Lobben, A., and Gordon, J.: The relative importance of climate change and population growth for exposure to future extreme droughts, Climatic Change, 138, 41–53, https://doi.org/10.1007/s10584-016-1716-z , 2016.
Soden, R. and Palen, L.: From crowdsourced mapping to community mapping: the post-earthquake work of OpenStreetMap Haiti, in: Proceedings of the 11th International Conference on the Design of Cooperative Systems, 27–30 May 2014, Nice, Springer International Publishing, https://doi.org/10.1007/978-3-319-06498-7__19 , 2014.
Stahl, K. and Hisdal, H.: Hydroclimatology, Dev. Water Sci., 48, 19–51, 2004.
Stanley, T. A. and Kirschbaum, D. B.: A heuristic approach to global landslide susceptibility mapping, Nat. Hazards, 87, 145–164, https://doi.org/10.1007/s11069-017-2757-y , 2017.
Stone, J., Barclay, J., Simmons, P., Cole, P., Loughlin, S. C., Ramón, P., and Mothes, P.: Risk reduction through community-based monitoring: the vigías of Tungurahua, Ecuador, J Appl. Volcanol. 3, 11, https://doi.org/10.1186/s13617-014-0011-9 , 2014.
Suppasri, A., Mas, E., Charvet, I., Gunasekera, G., Imai, K., Fukutani, Y., Abe, Y., and Imamura, F.: Building damage characteristics based on surveyed data and fragility curves of the 2011 great east Japan tsunami, Nat. Hazards, 66, 319–341, https://doi.org/10.1007/s11069-012-0487-8 , 2013.
Tanyas, H., Van Westen, C., Allstadt, K., Nowicki Jessee, A., Görüm, T., Jibson, R. W., Godt, J. W., Sato, H. P., Schmitt, R. G., Marc, O., Hovius, N.: Presentation and Analysis of Earthquake-Induced Landslide Inventories, J. Geophys. Res.-Earth, 122, 1991–2015, https://doi.org/10.1002/2017JF004236 , 2017.
Tiggeloven, T., De Moel, H., Winsemius, H. C., Eilander, D., Erkens, D., Erkens, G., Gebremedhin, E., Diaz Loaiza, A., Kuzma, S., Luo, T., Iceland, C., Bouwman, A., Van Huijstee, J., Ligtvoet, J., and Ward, P. J.: Global-scale benefit–cost analysis of coastal flood adaptation to different flood risk drivers using structural measures, Nat. Hazards Earth Syst. Sci., 20, 1025–1044, https://doi.org/10.5194/nhess-20-1025-2020 , 2020.
Tinti, S., Tonini, R., Pontrelli, P., Pagnoni, G., and Santoro, L.: Tsunami risk assessment in the Messina Straits, Italy, with application to the urban area of Messina, Geophys. Res. Abstr., 10, EGU2008-a-08045, 2008.
UNGA: Report of the open ended intergovernmental expert working group on indicators and terminology relating to disaster risk reduction, United Nations General Assembly A/71/644, New York, 2016.
UNDRR: Global Assessment Report 2009, Risk and Poverty in a Changing Climate, Global Assessment Report on Disaster Risk Reduction, UN Office for Disaster Risk Reduction, Geneva, 2009.
UNDRR: GAR 2011, Global Assessment Report on Disaster Risk Reduction, Revealing Risk, Redefining Development, UN Office for Disaster Risk Reduction, Geneva, 2011.
UNDRR: GAR 2013. From Shared Risk to Shared Value: the Business Case for Disaster Risk Reduction, UN Office for Disaster Risk Reduction, Geneva, 2013.
UNDRR: Global Assessment Report 2015, Making Development Sustainable: The Future of Disaster Risk Management, UN Office for Disaster Risk Reduction, Geneva, 2015a.
UNDRR: Sendai Framework for Disaster Risk Reduction 2015–2030 United Nations Office for Disaster Risk Reduction, Geneva, Switzerland, 2015b.
UNDRR: GAR 2019. Global Assessment Report on Disaster Risk Reduction, UN Office for Disaster Risk Reduction, Geneva, 2017.
UNDRR: DesInventar database, UNDRR, Geneva, available at: https://www.desinventar.net/ (last access: 30 November 2019), 2020.
Vafeidis, A. T., Nicholls, R. J., McFadden, L., Tol, R. S. J., Hinkel, J., Spencer, T., Grashoff, P. S., Boot, G., and Klein, R. J. T.: A new global coastal database for impact and vulnerability analysis to sea-level rise, J. Coast. Res., 24, 917–924, https://doi.org/10.2112/06-0725.1 , 2008.
Vafeidis, A. T., Schuerch, M., Wolff, C., Spencer, T., Merkens, J. L., Hinkel, J., Lincke, D., Brown, S., and Nicholls, R. J.: Water-level attenuation in global-scale assessments of exposure to coastal flooding: a sensitivity analysis, Nat. Hazards Earth Syst. Sci., 19, 973–984, https://doi.org/10.5194/nhess-19-973-2019 , 2019.
Van Asselen, S. and Verburg, P.: Land cover change or land-use intensification: simulating land system change with a global-scale land change model, Global Change Biol., 19, 3648–3667, https://doi.org/10.1111/gcb.12331 , 2013.
Virts, K. S., Wallace, J. M., Hutchins, M. L., and Holzworth, R. H.: Highlights of a new ground-based, hourly global lightning climatology, B. Am. Meteorol. Soc., 94, 1381–1391, https://doi.org/10.1175/BAMS-D-12-00082.1 , 2013.
Vousdoukas, M. I., Voukouvalas, E., Annunziato, A., Giardino, A., and Feyen, L.: Projections of extreme storm surge levels along Europe, Clim. Dynam., 47, 3171–3190, https://doi.org/10.1007/s00382-016-3019-5 , 2016.
Wallace, K., Snedigar, S., and Cameron, C.: `Is Ash Falling?', an online ashfall reporting tool in support of improved ashfall warnings and investigations of ashfall processes, J. Appl. Volcanol., 4, 1–10, 2015.
Wang, S. Y. S., Zhao, L., Yoon, J.-H., Klotzbach, P., and Gillies, R. R.: Quantitative attribution of climate effects on Hurricane Harvey's extreme rainfall in Texas, Environ. Res. Lett., 13, 054014, https://doi.org/10.1088/1748-9326/aabb85 , 2018.
Ward, P. J., Jongman, B., Sperna Weiland, F., Bouwman, A., Van Beek, R., Bierkens, M. F. P., Ligtvoet, W., and Winsemius, H. C.: Assessing flood risk at the global scale: model setup, results, and sensitivity, Environ. Res. Lett., 8, 044019, https://doi.org/10.1088/1748-9326/8/4/044019 , 2013.
Ward, P. J. Kummu, M., and Lall, U.: Flood frequencies and durations and their response to El Niño Southern Oscillation: Global analysis, J. Hydrol., 539, 358–378, https://doi.org/10.1016/j.jhydrol.2016.05.045 , 2016.
Ward, P. J., Jongman, B., Aerts, J. C. J. H., Bates, P. D., Botzen, W. J. W., Diaz Loaiza, A., Hallegatte, S., Kind, J. M., Kwadijk, J., Scussolini, P., and Winsemius, H. C.: A global framework for future costs and benefits of river-floo d protection in urban areas, Nat. Clim. Change, 7, 642–646, https://doi.org/10.1038/NCLIMATE3350 , 2017.
Ward, P. J., Couasnon, A., Eilander, D., Haigh, I. D., Hendry, A., Muis, S., Veldkamp, T. I. E., Winsemius, H. C., and Wahl, T.: Dependence between high sea-level and high river discharge increases flood hazard in global deltas and estuaries, Environ. Res. Lett., 13, 084012, https://doi.org/10.1088/1748-9326/aad400 , 2018.
Watkinson, I. M. and Hall, R.: Impact of communal irrigation on the 2018 Palu earthquake-triggered landslides, Nat. Geosci., 12, 940–945, https://doi.org/10.1038/s41561-019-0448-x , 2019.
Wens, M., Johnson, J. M., Zagaria, C., and Veldkamp, T. I. E.: Integrating human behavior dynamics into drought risk assessment – A sociohydrologic, agent-based approach, WIREs Water, 6, e1345, https://doi.org/10.1002/wat2.1345 , 2019.
White, G. F.: Human adjustment to floods, University of Chicago Press, Chicago, 1945.
Wiebe, D. M. and Cox, D. T.: Application of fragility curves to estimate building damage and economic loss at a community scale: a case study of Seaside, Oregon, Nat. Hazards, 71, 2043–2061, https://doi.org/10.1007/s11069-013-0995-1 , 2014.
Willner, S.N., Levermann, A., Zhao, F., and Frieler, K.: Adaptation required to preserve future high-end river flood risk at present levels, Sci. Adv., 4, eaao1914, https://doi.org/10.1126/sciadv.aao1914 , 2018.
Wilson, G., Wilson, T. M., Deligne, N. I., and Cole, J. W.: Volcanic hazard impacts to critical infrastructure: A review, J. Volcanol. Geoth. Res., 286, 148–182, https://doi.org/10.1016/j.jvolgeores.2014.08.030 , 2014.
Wing, O. E. J., Bates, P. D., Sampson, C. C., Smith, A. M., Johnson, K. A., and Erickson, T. A.: Validation of a 30 m resolution flood hazard model of the conterminous United States, Water Resour. Res., 53, 7968–7986, https://doi.org/10.1002/2017WR020917 , 2017.
Wing, O. E. J., Bates, P. D., Smith, A. M., Sampson, C. C., Johnson, K. A., Fargione, J., and Morefield, P.: Estimates of present and future flood risk in the conterminous United States, Environ. Res. Lett., 13, 034023, https://doi.org/10.1088/1748-9326/aaac65 , 2018.
Winsemius, H. C., Aerts, J. C. J. H., Van Beek, L. P. H., Bierkens, M. F. P., Bouwman, A., Jongman, B., Kwadijk, J., Ligtvoet, W., Lucas, P. L., Van Vuuren, D. P., and Ward, P. J.: Global drivers of future river flood risk, Nat. Clim. Change, 6, 381–385, https://doi.org/10.1038/nclimate2893 , 2016.
Winsemius, H. C., Jongman, B., Veldkamp, T. I. E., Hallegatte, S., Bangalore, M., and Ward, P. J.: Disaster Risk, Climate Change, and Poverty: Assessing the Global Exposure of Poor People to Floods and Droughts, Environ. Dev. Econ., 23, 328–348, https://doi.org/10.1017/S1355770X17000444 , 2018.
Winsemius, H. C., Ward, P. J., Gayton, I., ten Veldhuis, M.-C., Meijer, D. H., and Iliffe, M.: Commentary: The need for a high-accuracy, open-access global DEM, Front. Earth Sci., 7, 33, https://doi.org/10.3389/feart.2019.00033 , 2019.
Wood, N. J. and Good, J. W.: Vulnerability of port and harbor communities to earthquake and tsunami hazards: the use of GIS in community hazard planning, Coast. Manage., 32, 243–269, https://doi.org/10.1080/08920750490448622 , 2004.
World Bank: Natural Hazards, Unnatural Disasters: The Economics of Effective Prevention, World Bank, Washington, D.C., 2010.
Yamin, L. E., Hurtado, A., Barbat, A. H., and Cardona, O. D.: Seismic and wind vulnerability assessment for the GAR-13 global risk assessment, Int. J. Disast. Risk Reduct., 10, 452–460, https://doi.org/10.1016/j.ijdrr.2014.05.007 , 2014.
Yang, W., Shen, L., and Shi, P.: Mapping Landslide Risk of the World, in: World Atlas of Natural Disaster Risk. IHDP/Future Earth-Integrated Risk Governance Project Series, edited by: Shi, P. and Kasperson, R., Springer, Berlin, Heidelberg, 2015.
Yin, Y., Zhang, X., Lin, D., Yu, H., Wang, J., and Shi, P.: GEPIC-V-R model: A GIS-based tool for regional crop drought risk assessment, Agr. Water Assess., 144, 107–119, https://doi.org/10.1016/j.agwat.2014.05.017 , 2014.
Ying, M., Zhangm W., Yu, H., Lu, X., Feng, J., Fan, Y., Zhu, Y., and Chen, D.: An overview of the China Meteorological Administration tropical cyclone database, J. Atmos. Ocean. Tech., 31, 287–301, https://doi.org/10.1175/JTECH-D-12-00119.1 , 2014.
Yokoyama, I., Tilling, R. I., and Scarpa, R.: International mobile early-warning system(s) for volcanic eruptions and related seismic activities, UNEP, Paris, 1984.
Zaghi, A. E., Padgett, J. E., Bruneau, M., and Barbato, M.: Establishing common nomenclature, characterizing the problem, and identifying future opportunities in multihazard design, J. Struct. Eng., 142, H2516001, https://doi.org/10.1061/(ASCE)ST.1943-541X.0001586 , 2016.
Zscheischler, J., Westra, S., Van den Hurk, B. J. J. M., Seneviratne, S. I., Ward, P J., Pitman, A., AghaKouchak, A., Bresch, D. N., Leonard, M., Wahl, T., and Zhang, X.: Future climate risk from compound events, Nat. Clim. Change, 8, 469–477, https://doi.org/10.1038/s41558-018-0156-3 , 2018.
- Introduction
- Review of literature per hazard type
- Comparison of approaches across hazard types
- Future research challenges and opportunities
- Data availability
- Author contributions
- Competing interests
- Special issue statement
- Acknowledgements
- Financial support
- Review statement
- Full-text XML
- Open supplemental data
- Reference Manager
- Simple TEXT file
People also looked at
Methods article, research needs, challenges, and strategic approaches for natural hazards and disaster reconnaissance.
- 1 RAPID Facility, Department of Civil & Environmental Engineering, University of Washington, Seattle, WA, United States
- 2 Evans School of Public Policy & Governance, University of Washington, Seattle, WA, United States
- 3 Department of Human-Centered Design and Engineering, University of Washington, Seattle, WA, United States
- 4 Geomatics Lab, School of Civil and Construction Engineering, Oregon State University, Corvallis, OR, United States
- 5 Department of Civil and Coastal Engineering, University of Florida, Gainesville, FL, United States
- 6 Center for Coastal Studies and Department of Civil & Environmental Engineering, Virginia Tech, Blacksburg, VA, United States
- 7 Applied Physics Laboratory, University of Washington, Seattle, WA, United States
- 8 Formerly, RAPID Facility, Department of Civil & Environmental Engineering, University of Washington, Seattle, WA, United States
Natural hazards and disaster reconnaissance investigations have provided many lessons for the research and practice communities and have greatly improved our scientific understanding of extreme events. Yet, many challenges remain for these communities, including improving our ability to model hazards, make decisions in the face of uncertainty, enhance community resilience, and mitigate risk. State-of-the-art instrumentation and mobile data collection applications have significantly advanced the ability of field investigation teams to capture quickly perishable data in post-disaster settings. The NHERI RAPID Facility convened a community workshop of experts in the professional, government, and academic sectors to determine reconnaissance data needs and opportunities, and to identify the broader challenges facing the reconnaissance community that hinder data collection and use. Participants highlighted that field teams face many practical and operational challenges before and during reconnaissance investigations, including logistics concerns, safety issues, emotional trauma, and after-returning, issues with data processing and analysis. Field teams have executed many effective missions. Among the factors contributing to successful reconnaissance are having local contacts, effective teamwork, and pre-event training. Continued progress in natural hazard reconnaissance requires adaptation of new, strategic approaches that acquire and integrate data over a range of temporal, spatial, and social scales across disciplines.
Introduction
Natural hazards and disaster reconnaissance investigations have led to important discoveries that have greatly improved our scientific understanding of hazards and their physical, social, and environmental consequences. For example, findings from one of the earliest field reconnaissance missions in the United States, the Lawson and Reid (1908) investigation of the 1906 ∼M7.9 San Francisco earthquake, led to the development of the landmark theory of elastic rebound ( Reid, 1910 ), among other significant scientific and engineering advancements ( Ellsworth, 1990 ). More recently, post-event reconnaissance investigations have provided new, fundamental knowledge essential for the development of computational models to simulate the physical and socioeconomic impacts of natural hazards, and for identifying ways that communities can restore their infrastructure, rebuild their built environment, and recover their socioeconomic capital (e.g., Xiao and Van Zandt, 2012 ; Xiao and Peacock, 2014 ; Cong et al., 2018 ; Kang et al., 2018 ; Nejat et al., 2019 ). Far from an uncaring or indifferent data-gathering exercise in the face of tragedy, reconnaissance campaigns are at their core “a humanitarian mission in the broadest sense” ( Kaplan, 2010 ).
Natural hazards, such as wind events (i.e., tornadoes and coastal storms, including wind-generated waves and surges), earthquakes (and secondary effects such as shaking-induced damage to buildings and infrastructure, soil liquefaction and co-seismic landslides, and tsunamis), landslides, and volcanic eruptions, produce an extraordinary volume and quality of data that can inform our preparation and response to future events ( Nature Geoscience, 2017 ). Such data are often highly ephemeral or “perishable” since they may be altered or removed during rescue and recovery activities, or by natural agents such as precipitation or wind following an event. Therefore, reconnaissance data must be collected soon after an event occurs. These data are also unique because they inherently include the real-world complexities (e.g., the interplay between natural, human, and built systems) that allow us to better understand and quantify the socio-technical dimensions related to damage, restoration, and resiliency of the built environment; such data are difficult to duplicate in a traditional laboratory setting. Reconnaissance data, once collected, processed, curated, and archived ( Rathje et al., 2017 ), may be used and reused for a range of purposes, including (i) making discoveries and gaining fresh insights, (ii) testing and verifying models, (iii) reducing uncertainties in probabilistic models, and (iv) inspiring new simulation models, including new data-driven methods (e.g., Loggins et al., 2019 ).
In the past, reconnaissance investigators collected data and documented field observations using conventional recording and measurement tools, such as photography, note-taking, and surveying ( Geotechnical Extreme Events Reconnaissance [GEER], 2014 ). Today, the availability of state-of-the-art instrumentation, mobile data collection technologies (e.g., RApp; Miles and Tanner, 2018 ; Berman et al., in press ), training, and field support services, such as those provided by the Natural Hazards Engineering Research Infrastructure (NHERI) Natural Hazards Reconnaissance Facility (known as the RAPID) ( Wartman et al., 2018 ; Berman et al., in press ), has significantly advanced the ability of field investigation teams to capture perishable data in post-disaster settings.
This article briefly reviews the current state of natural hazards and disaster reconnaissance, including highlights from recent missions, difficulties teams face, and opportunities for progress. It then examines the grand challenges facing the natural hazards community and presents new approaches to meet these challenges through the strategic design, planning, and execution of reconnaissance campaigns. Many of the ideas presented in the article were developed with input from key stakeholders, including participants of a 2-day reconnaissance workshop, previous and current users of RAPID facility instrumentation, and other disciplinary experts in the professional, government, and academic sectors.
Natural Hazards and Disaster Reconnaissance
The history of natural hazard and disaster investigations spans many centuries. Interest in natural hazards, frequently by religious scholars, gathered momentum during the Renaissance and Reformation (14th to 16th centuries) when authorities began systematically cataloging earthquakes and other rare events such as plagues ( Schenk, 2007 ; Tülüveli, 2015 ). Scholars often used these data in an attempt to reconcile extreme events with spiritual beliefs and religious concepts. Lawson and Reid (1908) comprehensive, two-volume report on the San Francisco, California earthquake ( Figure 1 ) is one of the first rigorous scientific field studies of a major natural hazard ( Ellsworth, 1990 ). A decade later, Prince (1920) conducted one of the first social sciences investigations of an extreme event, the Halifax, Nova Scotia, Canada explosion of a munitions ship in the city harbor. Social sciences studies of disasters became more systematic and formalized in the 1940s through the 1960s, largely due to work at the Disaster Research Center (Ohio State University), which was initially supported by the U.S. Office of Civil Defense to inform cold war civil defense efforts (e.g., Knowles, 2012 ). Earthquake Engineering Research Institute [EERI] (1971) conducted one of the first in-depth multidisciplinary investigations of a natural hazard event, the San Fernando, California earthquake.
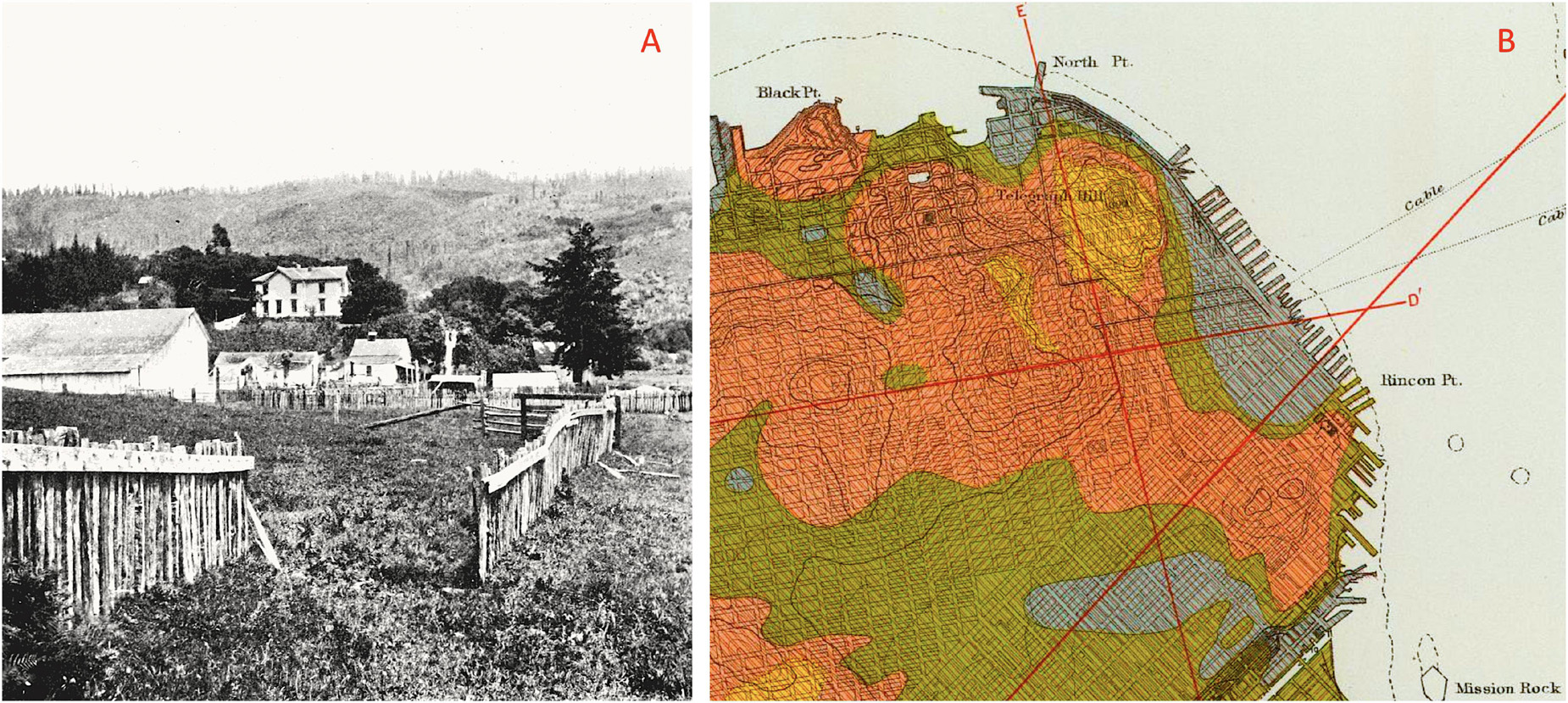
Figure 1. The Lawson and Reid (1908) reconnaissance investigation of the 1906 San Francisco earthquake led to significant scientific and engineering advancements. (A) Reconnaissance photograph showing fence offset by earthquake surface fault rupture near Bolinas, Marin County, CA, United States. This observation led to the development of the theory of elastic rebound ( Reid, 1910 ). (B) Excerpt of “Map of San Francisco showing apparent intensity of the earthquake shock” ( Lawson and Reid, 1908 ) showing area of high intensity shaking revealing the modern engineering concept of non-linear site response and effects (Gray and green tones depict areas of highest local shaking intensity). Both images are reproduced from Lawson and Reid (1908) .
The EERI was one of the first professional organizations to formalize regular reconnaissance investigations of major seismic events by establishing the Learning from Earthquakes (LFE) program in 1973. Largely multidisciplinary in its approach, the LFE program deploys teams of geoscientists, engineers, and social scientists to investigate and observe the damaging effects of significant earthquakes worldwide. Recently, the LFE program has expanded to include a virtual earthquake reconnaissance teams, or “VERT,” that conduct rapid “virtual” (i.e., non-field based) assessments within 48 h of an earthquake ( Fischer and Hakhamaneshi, 2019 ).
With the support of the U.S. National Science Foundation (NSF), the Geotechnical Extreme Events Reconnaissance (GEER) Association was formed in 1999 to conduct reconnaissance investigations of the geotechnical aspects of significant earthquakes in the U.S. and abroad ( Bray et al., 2019 ). In 2011, GEER’s scope was expanded to include the study of the geotechnical aspects of other natural hazard events such as hurricanes, floods, and landslides (e.g., Dashti et al., 2014 ; Wartman et al., 2016 ; Hughes and Morales Vélez, 2017 ; Gallant et al., 2020 ; Montgomery et al., 2020 ). GEER authorizes research missions based upon (i) the opportunity to learn about new scientific hypotheses or engineering models, (ii) the availability of additional field data (e.g., ground motion recordings) to supplementary data gathered in the reconnaissance, and (iii), for international (non-U.S.) events, the potential for a similar event to occur in the future in the U.S ( Geotechnical Extreme Events Reconnaissance [GEER], 2014 ). During the past several years, NSF began supporting other similar “extreme event reconnaissance (or research),” or EER, organizations including StEER (Structural Extreme Events Reconnaissance), OSEEER (Operations and Systems Engineering Extreme Events Research), SSEER (Social Science Extreme Events Research), ISEEER (Interdisciplinary Science and Engineering Extreme Events Research), NEER (Nearshore Extreme Events Reconnaissance), and SUstainable Material Management Extreme Events Reconnaissance (SUMMEER). These EER organizations are coordinated by CONVERGE ( Peek et al., 2020 ), which seeks to advance ethically-grounded ( Gaillard and Peek, 2019 ), scientifically rigorous, disciplinary, and interdisciplinary extreme events research.
There are other natural hazards reconnaissance organizations based at professional societies worldwide. The Earthquake Engineering Field Investigation Team (EEFIT), based in the United Kingdom, supports earthquake reconnaissance missions with the goals of making technical assessments, collecting geological and seismological data, assessing the effectiveness of earthquake protection systems, and investigating disaster management procedures and socioeconomic impacts ( Stone et al., 2017 ). Italy hosts two organizations that have organized earthquake reconnaissance missions and conducted follow-on seismic policy analyses (e.g., Mazzoni et al., 2018 ), the Italian Network of University Laboratories for Earthquake Engineering (ReLUIS), and the European Centre for Training and Research in Earthquake Engineering (Eucentre). Elsewhere, the New Zealand Society for Earthquake Engineering (NZSEE) has supported reconnaissance investigations of earthquakes and major tsunamis worldwide for six decades ( Wood P. R. et al., 2017 ). In Asia, the Asian Technical Committee (ATC3) “Geotechnology for Natural Hazards” has conducted reconnaissance missions following natural hazard events. Other organizations, such as the Nepalese Engineering Society, the Building Research Institute of Japan, among others, also conduct investigations in the region. Similarly, the American Society of Civil Engineers (ASCE) has supported reconnaissance missions in the U.S. and abroad through the primary society (e.g., Silva-Tulla and Nicholson, 2007 ) or its disciplinary institutes (e.g., Wartman et al., 2013 ).
In addition to these organizations, self-organized teams sometimes form in the aftermath of an event, often with a focused hypothesis-driven research question or inquiry, to collect data. Table 1 summarizes the objectives and outcomes of recent reconnaissance investigations of several representative natural hazard events. Figures 2 through 5 present field data collected during several of the missions highlighted in Table 1 .
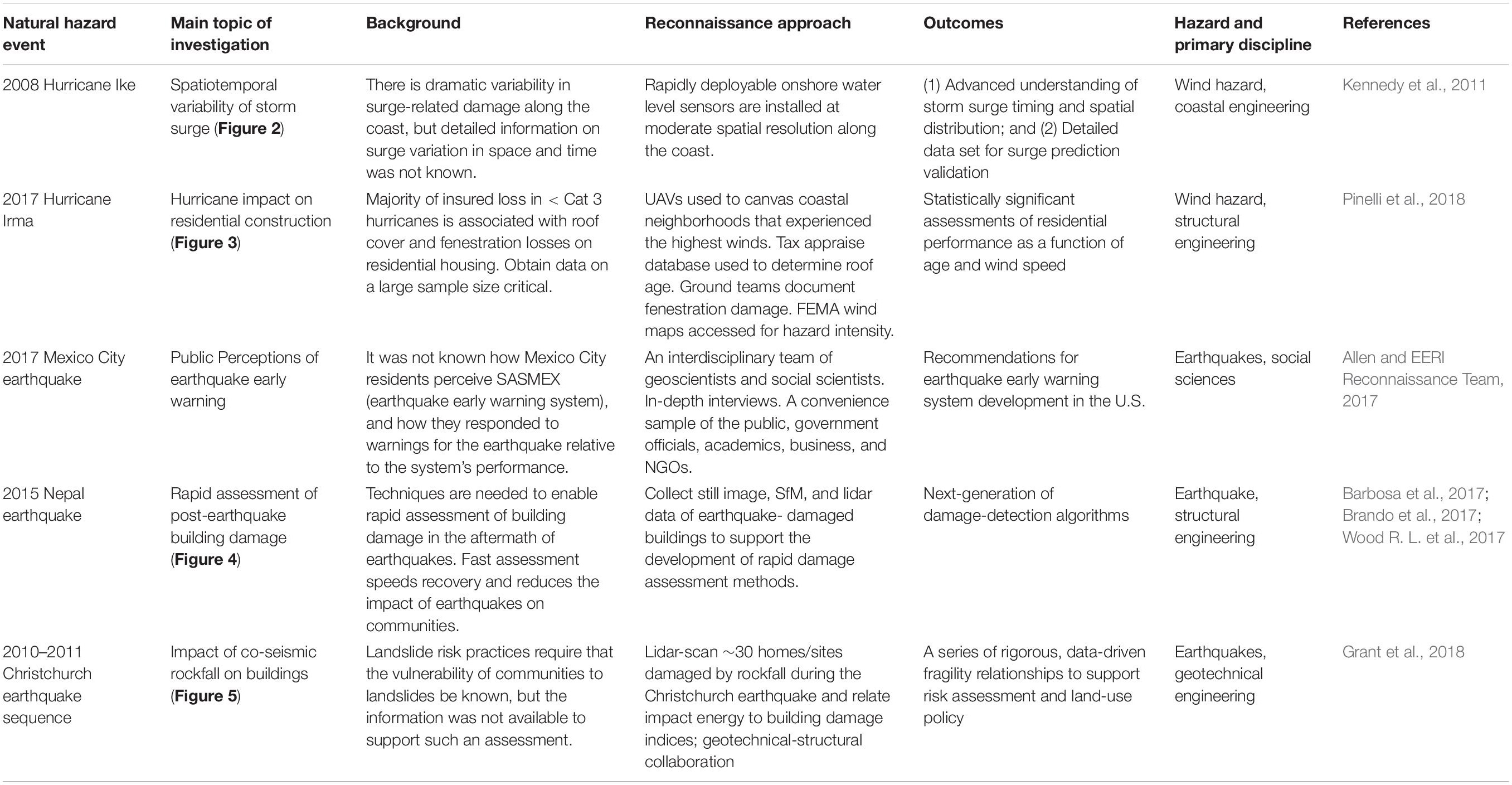
Table 1. Examples of reconnaissance approach, objectives, and outcomes from several recent earthquake and wind hazard missions ( Figure 2 ).
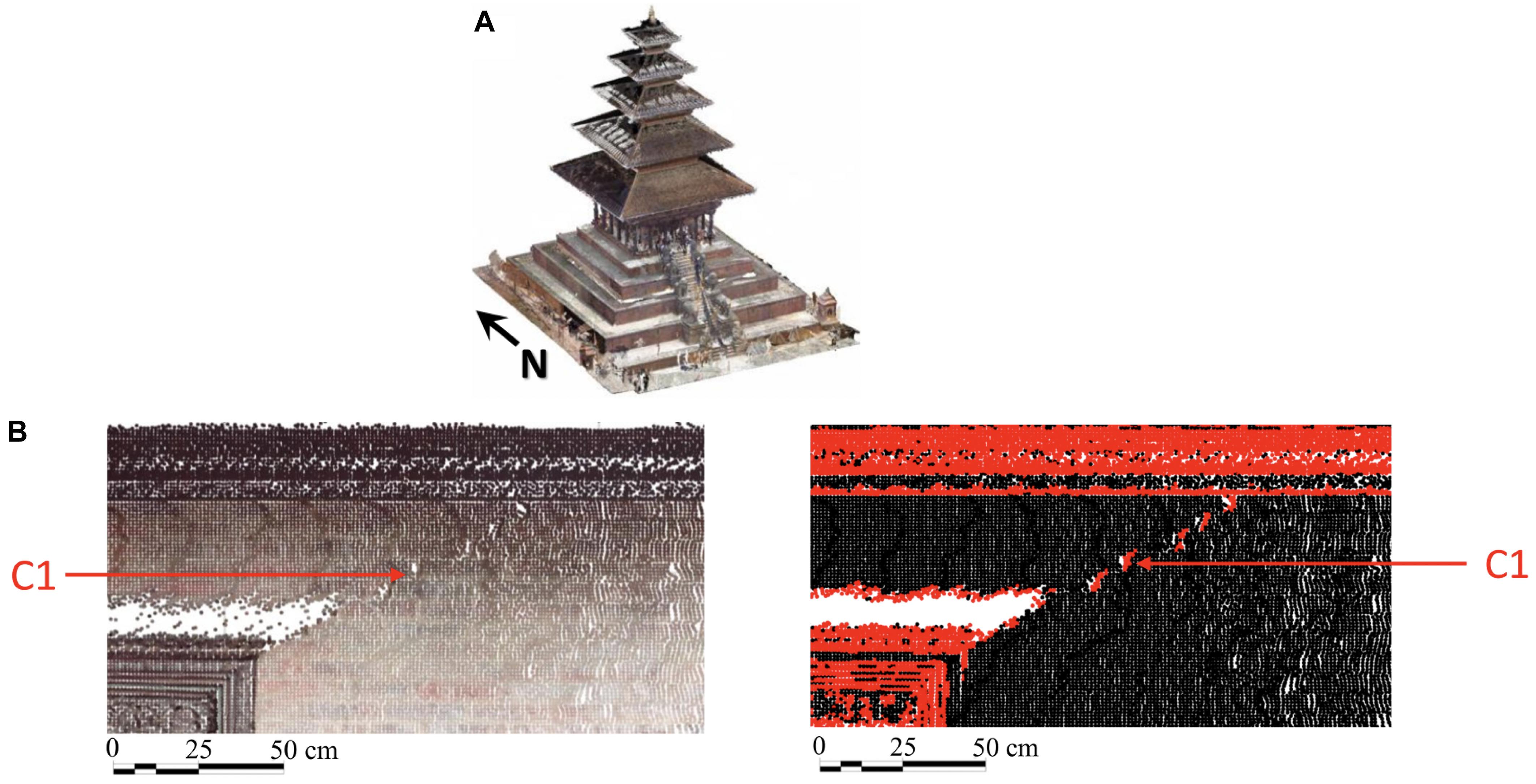
Figure 2. Assessing the performance of buildings using lidar data collected during reconnaissance. (A) Lidar-derived 3D model of Nyatapola Temple following the 2015 Ghorka Nepal Earthquake (B) Earthquake-induced crack (designated as “C1”) seen in a color point cloud (left) and detected defects shown in red (right). Reproduced from Wood P. R. et al. (2017) .
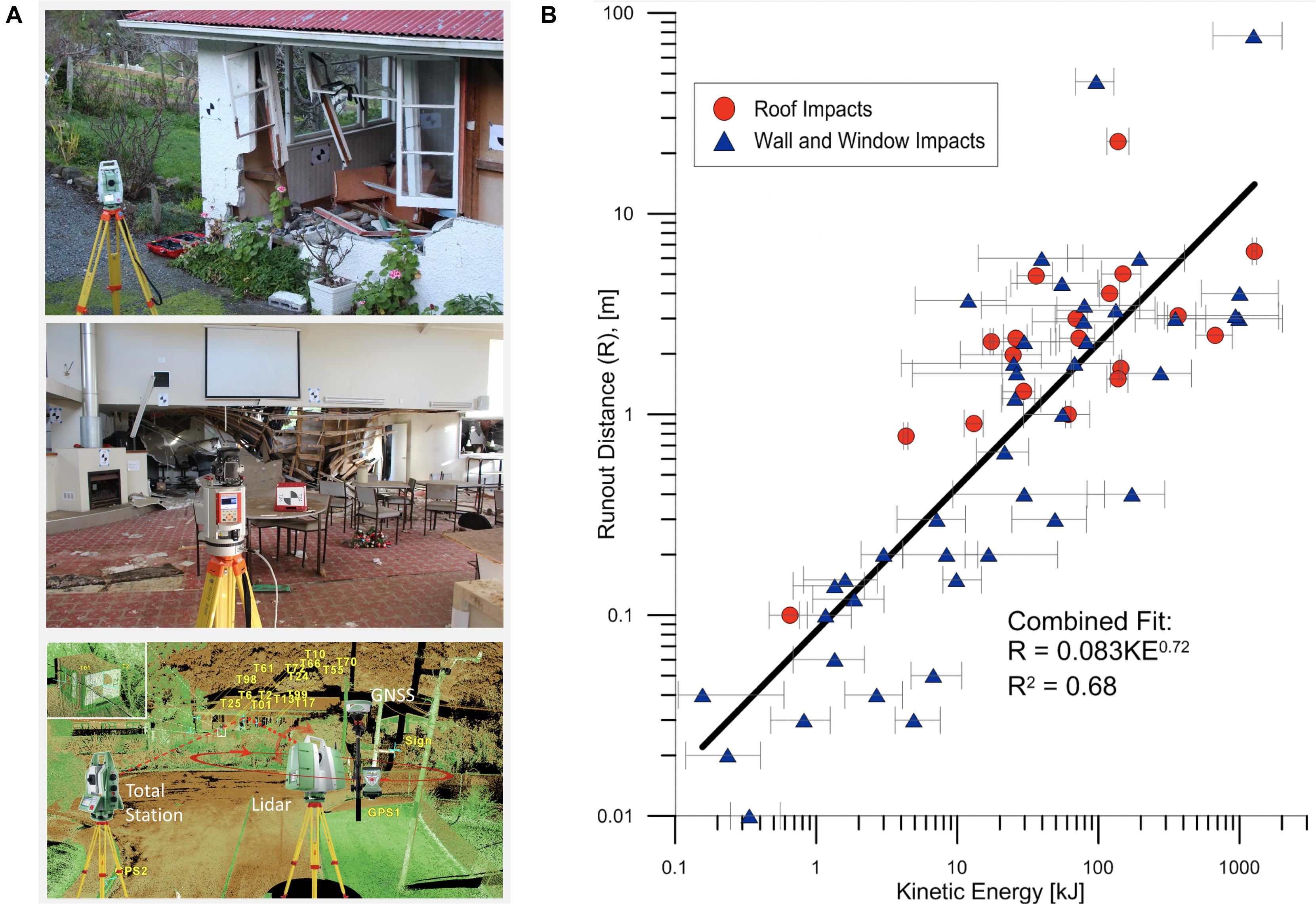
Figure 3. Reconnaissance investigation of the impact of rockfalls on dwellings during the 2011 Christchurch, New Zealand, earthquakes. (A) Lidar data was collected inside and outside buildings, geo-registered, then fusing into a single 3D model. (B) Field data reveals a direct correlation between rockfall impact energy and rock penetration into buildings. Modified from Grant et al. (2018) .
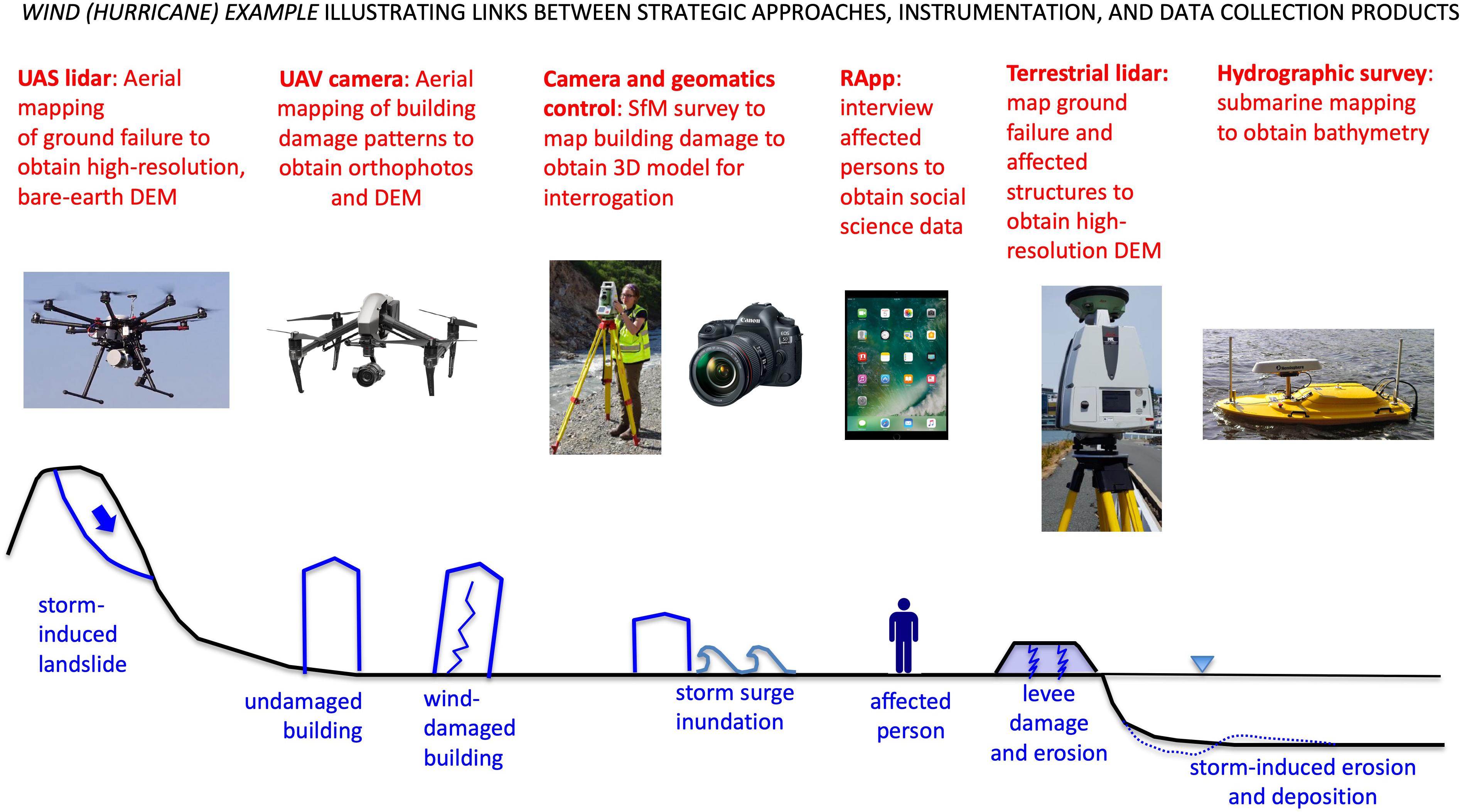
Figure 4. Diagram depicting damage features, secondary effects, and human and societal impacts that commonly result from an extreme wind event. The diagram is similar to Figure 7 , illustrating the commonalities between seismic and wind natural hazard events. Diagrams and inset images are as noted in Figure 7 .
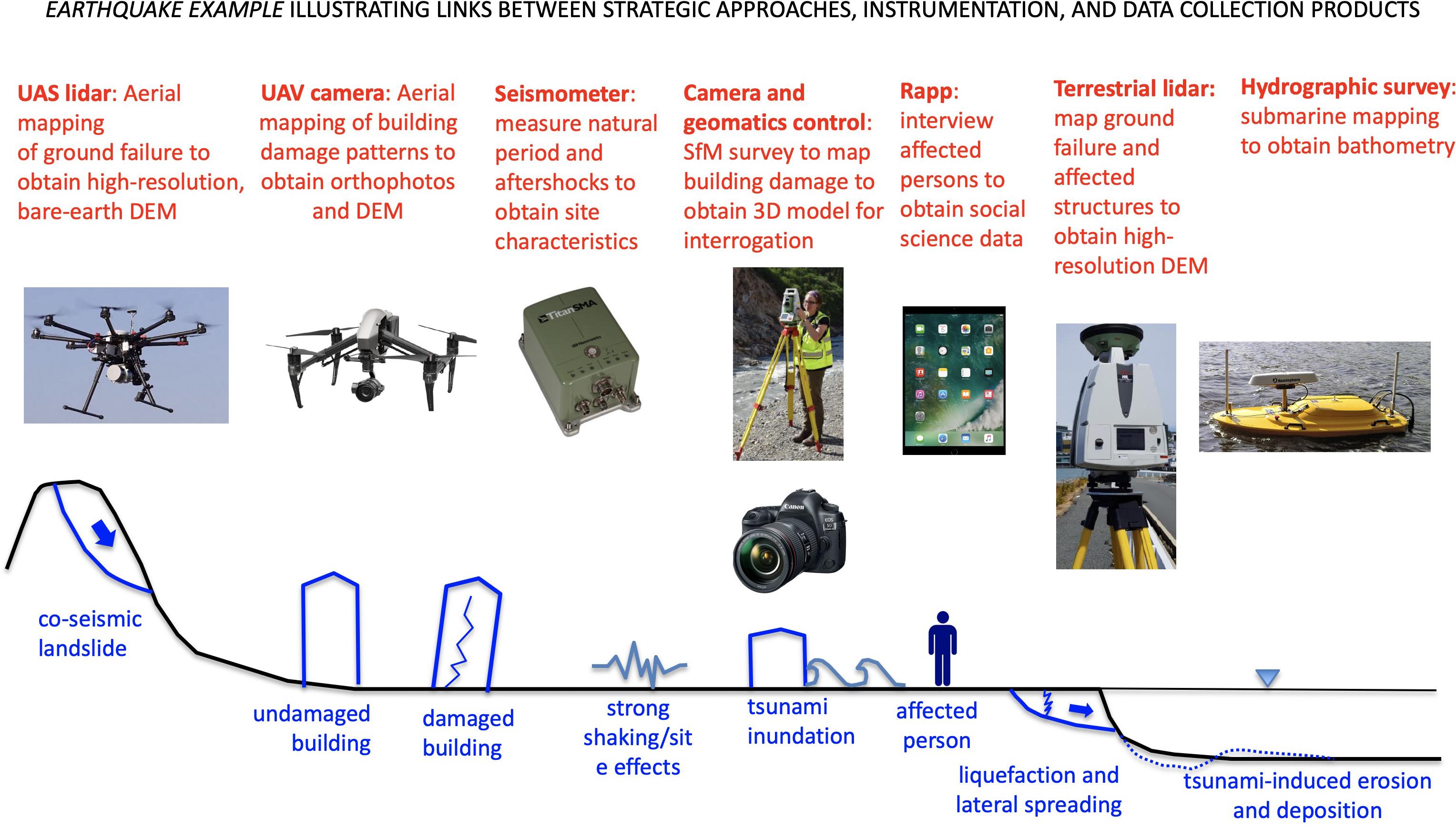
Figure 5. Diagram illustrating damage features, secondary effects, and human and societal impacts that often result from a significant earthquake (blue illustrations and accompanying text). Superimposed above this hypothetical post-event landscape are annotations linking instrumentation (shown with inset photographs) and data collections activities and products (shown in red) to event features.
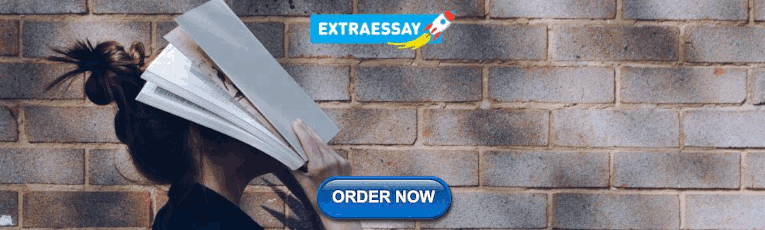
Reconnaissance Instrumentation and Natural Hazard Simulation
By enabling the prompt collection of high-resolution data sets, advanced reconnaissance instrumentation now plays a central role in providing the academic, research, and professional communities with an unprecedented volume of high-quality, open-source, engineering, geophysical, social, and behavioral data. In addition, new software and cyberinfrastructure tools allow complex data sets to be archived, integrated, explored, and visualized ( Rathje et al., 2017 ). These computational resources facilitate collaboration among experts across different fields to support advancements at the intersections of the natural hazards specialty disciplines. A unique aspect of the RAPID Facility is its portfolio of geospatial, image-centric data collection instrumentation. High-resolution georeferenced laser, image, and video data collected from full fields of view (i.e., top to bottom; inside and outside) of infrastructure within affected regions support the development of 3D post-event models ( Berman et al., in press ). Such models can be safely interrogated to extensive detail by geographically distributed research teams—an aspect that allows investigators the time and vision to collaboratively continue to discover new and important aspects of the impact of the surveyed event ( Olsen and Kayen, 2013 ; Olsen et al., 2015 ). These types of terrestrial data sets are increasingly being fused with broader scale satellite imagery to appreciate the regional context for damage at a specific site (e.g., Yamazaki and Matsuoka, 2007 ; Eguchi et al., 2008 ; Rathje and Franke, 2016 ; Gallant et al., 2020 ).
Modeling and simulation lie at the center of the natural hazard community’s broader goal to understand, simulate, and predict the performance of built, natural, and social systems during and after natural hazards events ( Edge et al., 2020 ). Over the past decade, a portfolio of highly sophisticated natural hazards models has significantly improved our ability to simulate the effects of extreme events across a wide range of spatial and temporal scales (e.g., Roelvink et al., 2009 ; Dietrich et al., 2011 ; LeVeque et al., 2011 ; Pita et al., 2013 ; Mandli and Dawson, 2014 ; Yim et al., 2014 ; Baradaranshoraka et al., 2019 ). These natural hazards models have become increasingly data-driven, requiring comprehensive data sets to capture complex, system-level responses. Examples of such models include performance-based earthquake engineering (PBEE) design methods and resilience-based design methods (e.g., FEMA, 2018 ; McAllister et al., 2019 ), which require fragility data to relate structural, non-structural, and infrastructure systems performance to engineering demand parameters, and stochastic wind hazard loss models ( Hamid et al., 2011 ; Pita et al., 2015 ) that require field data to better calibrate and validate the hazard, infrastructure vulnerability, costing components, and economic impacts of preparedness and mitigation policies.
The RAPID Facility’s principal scientific goal is to inform natural hazards computational simulation models, infrastructure performance assessment, and economic impact analysis by supporting the collection, development, and assessment of high-quality disaster data sets ( Figure 6 ). These data sets help advance our fundamental understanding of natural hazards and their impacts. Examples of reconnaissance data collection required to improve the natural hazards modeling and simulation include the following:
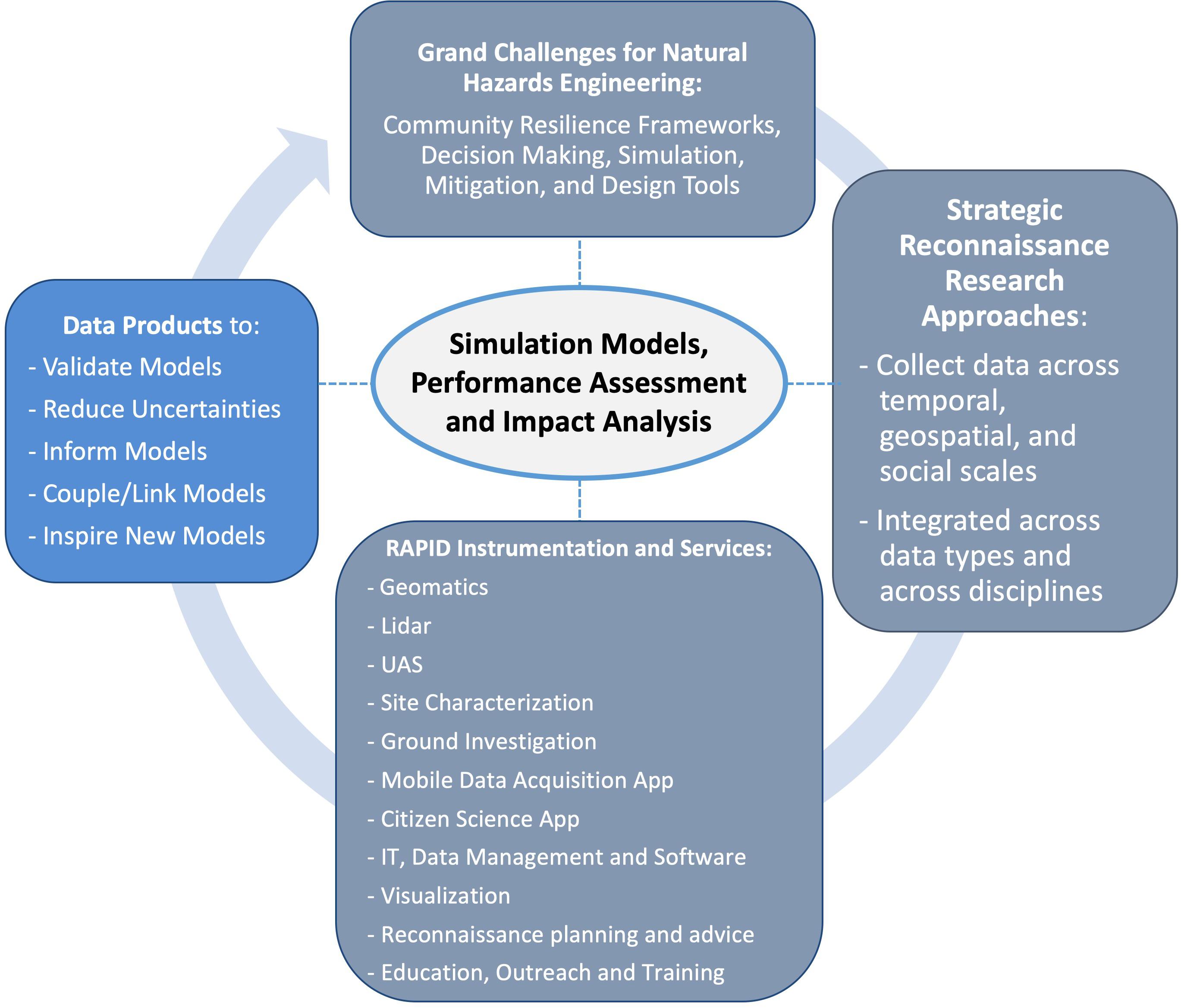
Figure 6. Grand challenges for the natural hazards community require new strategic approaches for reconnaissance data collection utilizing RAPID instrumentation and services. This data collection will produce data products that are needed to meet grand challenges. Central to this cycle is the RAPID Facility’s scientific goal of informing natural hazards computational simulation models, infrastructure performance assessment, and socioeconomic impact analysis by supporting the collection, development, and assessment of high-quality data sets [digital elevation model (DEM)].
1. Lifelines and other elements of the built environments are ultimately socio-technical systems ( Miles et al., 2014 ). That is, there are core social, economic, and behavioral components to the development, operation, and maintenance of all engineered systems. There is a crucial need for research to better unpack and quantify the socio-technical dimensions related to damage, restoration, and reconstruction of elements of the built environment. This research is needed to advance existing socio-technical loss (e.g., Kircher et al., 2006 ) and recovery models ( Miles and Chang, 2011 ), as well as to develop new ones. Most socio-technical modeling efforts to date have focused on modeling losses.
2. Development of high-resolution, geocoded data sets, such as aerial photography, lidar, and ground-based documentation of post-event damage (e.g., Gurley and Masters, 2011 ; Lombardo et al., 2015 ), to reduce uncertainties in stochastic models characterizing the vulnerability of infrastructure to wind and earthquake damage. Modern catastrophe risk models ultimately seek to project damage, loss, and recovery time at the whole-building, infrastructure system, or regional scale; examples modeling tools include FEMA (2018) as well as the community and regional resilience modeling tools such as OpenQuake ( Pagani et al., 2014 ) and those being developed by the Center for Risk-Based Community Resilience Planning ( van de Lindt et al., 2015 ) and the NHERI SimCenter. These tools predict building performance through the aggregation of component failures (e.g., FEMA, 2018 for earthquake hazard and Pita et al., 2015 for wind hazard) or based on building-level models such as those incorporated in FEMA HAZUS-MH ( Kircher et al., 2006 ). These simulation tools include numerous assumptions regarding probabilistic structural component capacities, load paths, the influence of aging, and cascading damage from neighboring structures. Thus, they benefit substantially from refinements to these assumptions informed by detailed geocoded field data stratified by building code and localized hazard intensity.
Provision of appropriate data to test, verify, and calibrate co-seismic landslide displacement models [e.g., the popular and practice-oriented Newmark et al. (1965) sliding model, as well as more advanced coupled (e.g., Rathje and Bray, 2000 ) or finite element formulations]. Specifically, advanced geomatics technologies such as lidar could capture intricate ground deformation patterns and landslide morphological features, eroded quickly after an event. There are relatively few high-quality case histories of co-seismic landslide displacement, which represents a pressing research need in the field of geotechnical earthquake engineering ( Harp et al., 2011 ).
1. Provision of the appropriate data to quantify underlying physical phenomena and to develop, validate, improve, and reduce uncertainty in physics-based, computational modeling of wind, waves, storm surge, tsunami inundation, sediment transport, morphological change, and other related processes representing the inter-related, destructive forcing mechanisms of natural hazards ( Kennedy et al., 2020b and references therein). Specifically, modern reconnaissance instrumentation can capture rare, but critical, perishable data during and following natural hazards, including the quantification of inundation extent, flow speeds, flow depth, wave conditions, wind speeds, soil properties, erosion and accretion, and inundation-related damage to civil infrastructure and the natural environment ( Kennedy et al., 2020a ). These data help improve understanding of, for example, (a) the interplay between the natural landscape (land cover, topographic features), the built environment (critical infrastructure, homes), and hydrodynamics and (b) how and when concurrent multi-hazard components (e.g., wind vs. surge) lead to the functional failure of critical infrastructure—ultimately leading to more resilient communities (e.g., Baradaranshoraka et al., 2017 ).
2. Simulation of structural response to ground shaking is validated mainly through comparison with data from experiments in controlled laboratory environments and with data collected from reconnaissance following earthquakes. The structural models may be focused on component behaviors, building behaviors, or even the behavior of entire classes of buildings through the development of fragility functions. Recent examples of field data informing advances in local structural behavior models include Kanvinde et al. (2015) , who investigated fracture of eccentrically braced frame links during the 2011 Christchurch earthquake and used collected field data helped to validate newly developed fracture models employed in detailed finite element analyses. At the macro-level, fragility functions derived from reconnaissance data on the performance of wood-frame buildings have resulted in large-scale loss estimations for San Francisco arising from the soft-story collapse of wood-frame structures and spurred public policy to encourage retrofit ( FEMA, 2012 ). Such observation-based fragility data are also critical to loss estimation software such as FEMA (2018) , FEMA HAZUS-MH ( Kircher et al., 2006 ) and OpenQuake ( Pagani et al., 2014 ), and the regional loss estimation tools being developed by the Center for Risk-Based Community Resilience Planning and the NHERI SimCenter.
Grand Challenges for the Natural Hazards and Disaster Research Communities
In 2011, the National Research Council convened a community workshop to identify grand challenges for earthquake engineering. These challenges served to guide research after the conclusion of the George E. Brown, Jr. Network for Earthquake Engineering Simulation operations ( National Research Council [NRC], 2011 ). While the title of the workshop highlighted earthquake engineering, the NRC steering committee noted that the identified grand challenges (community resilience, decision making, simulation, mitigation, design tools) were broad and also pertained to other natural and anthropogenic hazards. These grand challenges are adopted here as an overarching framework for identifying reconnaissance research opportunities for natural hazards and disaster research communities.
Community Resilience
To better understand the direct and indirect impacts of natural hazards events, a framework is needed to measure, monitor, and evaluate community-level resilience. The lack of historical data on community impacts and recovery following past disasters presents a significant impediment to meeting this goal ( National Research Council [NRC], 2011 ). Advanced reconnaissance instrumentation helps address this challenge by enabling the systematic collection and archiving integrated, interdisciplinary data pertinent to engineering and the natural and social sciences. This knowledge is necessary to evaluate the utility and validity of the range of community resilience frameworks—a significant gap in the state-of-the-art in disaster science and engineering ( Miles, 2015 ).
Hazard and Impact Simulation and Decision Making
Computational simulation and forecasting of the timing and regional distribution of the hazard itself (e.g., Frankel et al., 2018 ), as well as its physical and social impacts and recovery, are essential for decision making, planning, and mitigation. Such simulations—which span a range of temporal scales, including both short-term (e.g., informing electricity restoration with expected damage patterns) and long-term time frames (e.g., identifying local vulnerabilities for risk reduction policy-making)—present a challenge to the professional community ( National Research Council [NRC], 2011 ). New, high-performance computing and software platforms such as the NHERI DesignSafe-CI and SimCenter ( Blain et al., 2020 ) create the opportunity to make significant progress with this challenge. However, such simulations are highly complex and require extensive hypervariable data sets for model development and testing. Since many of these models are inherently data-driven, they also require high-quality data (e.g., initial and boundary conditions) to provide reliable forecasts.
Renewal and retrofit strategies are essential to mitigate hazards posed to infrastructure systems and communities (e.g., water and wastewater supply and distribution systems, power and energy systems, at-risk buildings, and coastal communities) ( National Research Council [NRC], 2011 ). The development of effective mitigation strategies requires computational models (see above), design methods, and construction standards that, when harmonized, are capable of identifying critical vulnerabilities and quantifying the impacts of risk reduction measures. In addition, post-event data are needed to evaluate loss estimation methodologies, such as HAZUS-MH, investigate the efficacy of mitigation approaches (e.g., Gurley and Masters, 2011 ), and provide feedback on state-mandated insurance incentives for homeowners who employ mitigation. New multiscale data collection tools provide the means to address these needs. For example, terrestrial lidar and building survey equipment could be used to collect data on the seismic performance of retrofitted buildings. Similarly, lidar or structure from motion (SfM)/multi view stereo photogrammetry ( Eltner et al., 2016 ; Özyeşil et al., 2017 ) technology can be used in coastal communities after hurricanes to quantify morphological changes, civil infrastructure damage, and ecological damage in detail and on a large scale. Importantly, all of these data sources can be integrated and overlaid with imagery to develop three-dimensional models of impacted regions or damage-affected infrastructure.
Design Tools
Improved capability to characterize uncertainty in the predictive ability of design tools is essential to exploit newer, more sustainable, and resilient building materials. Improved design tools are also needed to capitalize on innovative structural concepts (e.g., self- centering structural systems with replaceable fuses) ( National Research Council [NRC], 2011 ). Performance-based design provides the framework for addressing this challenge, but such design relies on high-quality performance data to define model relationships (e.g., fragility functions). Advanced instrumentation offers a means to meet this challenge. For example, sensors could be installed on structures and earth systems to monitor response to aftershocks ( Geli et al., 1988 ; Zhou et al., 2013 ), and aerial imagery could be used to validate the performance of wind-resistant roof covers.
In 2017, the Network Coordination Office ( Johnson et al., 2020 ) of NHERI convened a task group to prepare a network-wide science plan to guide future research and to focus investigators on keeping the communities and the built environment safe from natural hazards. The NCO’s NHERI network science plan was first published in July 2017 ( Smith et al., 2017 ) and reflected many of the principals of the National Research Council Grand Challenges report ( National Research Council [NRC], 2011 ). The NHERI network science plan highlights the need to (1) identify and quantify the characteristics of natural hazards that are damaging to civil infrastructure and disruptive to communities, (2) evaluate the physical vulnerability of civil infrastructure and the social vulnerability of populations in communities exposed to natural hazards, and (3) create the technologies and engineering tools to design, construct, retrofit, and operate multi-hazard resilient and sustainable infrastructure. The network issued a revised science plan in January 2020 ( Edge et al., 2020 ) that reflects the potential role of several new, rapidly advancing technologies (e.g., advanced computational methods, information science, bio-inspired design, convergence science) in improving community resilience to natural hazards. The revised science plan identifies three grand challenges for the community. These include (1) identifying and quantifying the characteristics of earthquake, windstorm, and associated hazards that are damaging to civil infrastructure and disruptive to communities, (2) assessing the physical vulnerability of civil infrastructure and the social vulnerability of populations in communities, and (3) creating the technologies and engineering tools to design, construct, retrofit, and operate a multi-hazard resilient and sustainable infrastructure. In addition to the NRC workshop report and the NHERI network science plans, other reports suggest specific research activities and tasks to help meet challenges in the fields of earthquake hazard reduction ( National Earthquake Hazards Reduction Program, 2008 ), resilience ( National Research Council [NRC], 2011 ), windstorm and coastal inundation impact reduction ( Coulbourne et al., 2014 ), and disaster risk reduction ( Aitsi-Selmi et al., 2015 ).
Research Needs, Challenges, and Opportunities for Natural Hazards Reconnaissance
Methodology.
In January 2017, the RAPID Facility convened a 2-day workshop to determine natural hazards and disaster reconnaissance data needs and opportunities and identify the broader challenges facing the reconnaissance community that encumber data collection and use. The workshop attendees—individuals having expertise across a range of natural hazards (e.g., wind events, earthquakes, and their secondary effects) and disciplines (engineering, and the natural and social sciences)—participated in three types of activities (also see Supplementary Material ).
(1) Informational presentations to provide background material to help stimulate later discussion in activity groups
(2) Guided “brainstorming-type” small group activities
(3) Responding to open-ended questions posed on poster boards placed in the break area during the first day of the workshop
During the brainstorming activities, participants were asked to first reflect on questions individually and later to share, discuss, and synthesize their ideas in small, pre-assigned groups. For some disciplinary-focused activities, groups were organized by specialty, while in other activities, groups were intentionally interdisciplinary to allow cross-fertilization of ideas between sciences and engineering domains. The ideas developed during the individual sessions and group discussions were attached to poster boards using sticky notes. Each group reported the general themes to all of the workshop participants. Over 1,600 ideas, comments, and replies recorded on sticky notes during the workshop. After the workshop, each of these notes was assigned a unique identifier code, cataloged, and then read and transcribed to a comprehensive database, which is included as Supplementary Material to this article. The workshop organizers then synthesized and analyzed the database of workshop comments and transcriptions to identify significant themes on needs, challenges, and opportunities for natural hazards and disaster reconnaissance.
Many of the workshop participants were seasoned reconnaissance investigators with the collective experience of dozens of reconnaissance missions following natural hazard events and other disasters—both natural and anthropogenic in origin. The participants responded to questions about the practical and operational challenges they have faced before, during, and after reconnaissance investigations. They also provided feedback about what went well (i.e., their “successes”) during reconnaissance missions. As noted in Table 2 , major challenges before deploying for reconnaissance mainly involve logistics in the compressed time frames intrinsic to extreme event investigations. During field missions, many of the challenges relate to the on-the-ground realities of working in a disaster zone, including safety concerns and emotional trauma. The difficulties after reconnaissance missions primarily pertain to data processing, analysis, and archiving. The participants reported a range of common themes about pre- and during deployment successes ( Table 3 ), including having previously established local contacts in the affected region, teamwork and camaraderie, and prior training on instrumentation reconnaissance methods, and safety. Successes after reconnaissance missions mainly pertain to the production of unique data products, improved fundamental knowledge, and positive impacts on policy and practice.
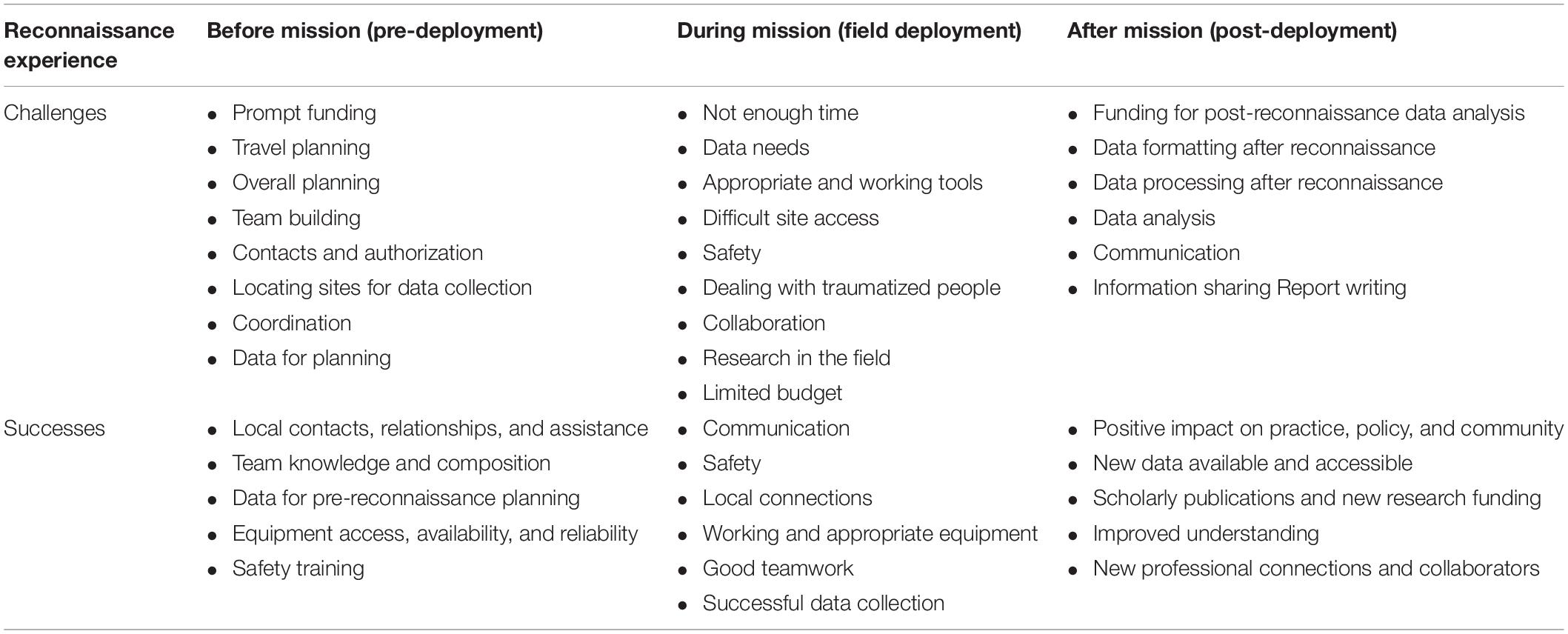
Table 2. Synthesis of key themes in workshop participant responses to questions about challenges and successes before, during, and after reconnaissance missions (see Supplementary Material for complete list).
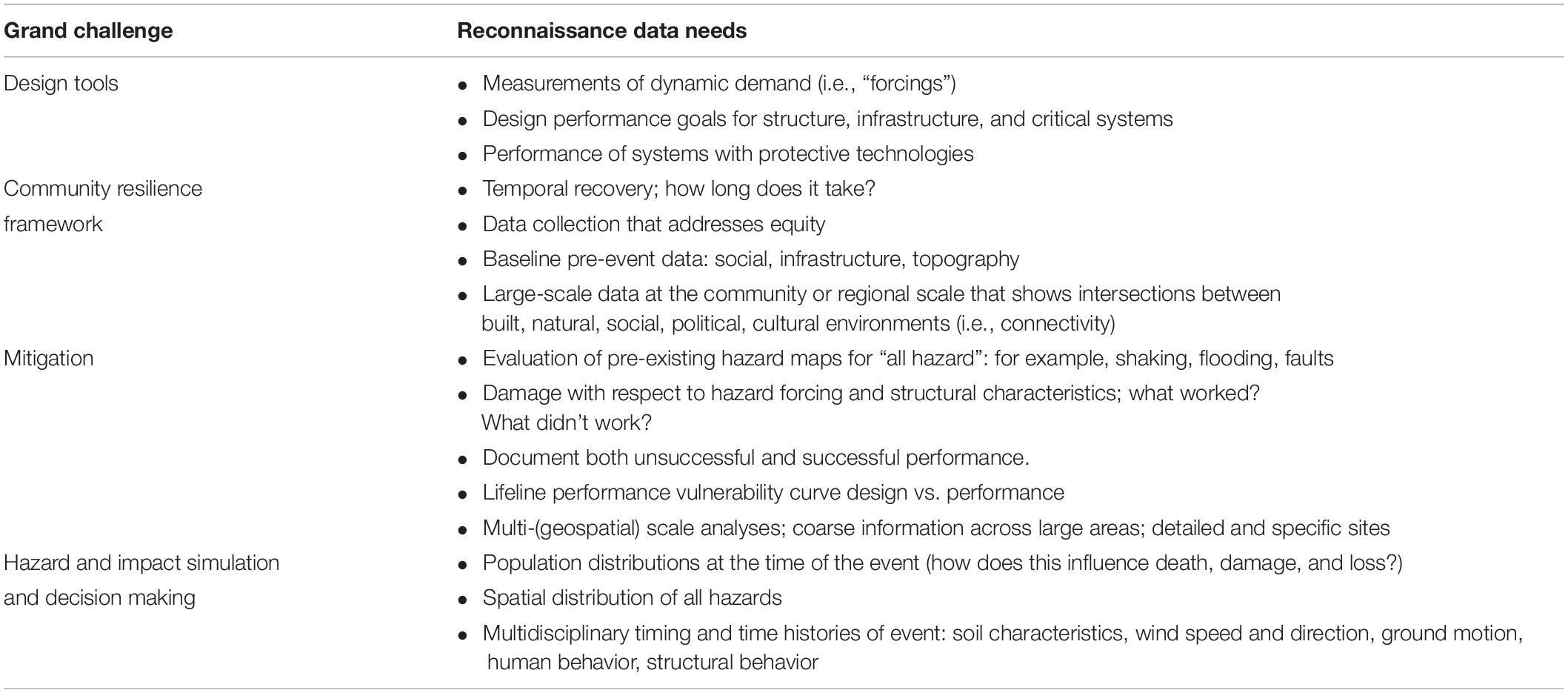
Table 3. Synthesis of High Priority Reconnaissance Data Needs to address Grand Challenges for the natural hazards and disaster research communities (see Supplementary Material for complete list).
The workshop participants were also asked to identify reconnaissance data needed to support the four National Research Council [NRC] (2011) grand challenges (i.e., community resilience framework, hazard and impact simulation and decision making, mitigation, and design tools). As indicated in Table 3 , the responses, which form the basis of our recommended strategic approaches for natural hazards and disaster reconnaissance, are broadly themed on concepts of cross-scale, multidisciplinary data collection.
Strategic Approaches for Natural Hazards and Disaster Reconnaissance
Post-disaster reconnaissance investigations have historically often involved the collection and development of data sets by disciplinary teams following natural hazard events. These data sets have usually been collected over limited geospatial scales (e.g., at the site or neighborhood scales) with little supporting metadata. As a result, such data sets can be challenging, if not impossible, to integrate. Meeting community challenges and accomplishing the scientific goal of improving simulation models requires new strategic approaches for reconnaissance investigations that acquire and integrate data over a range of temporal, spatial, and social scales across disciplines. Figures 7 , 8 illustrate links between the strategic approaches for natural hazard reconnaissance data collection, instrumentation, and resulting data products, for a hypothetical earthquake and wind event, respectively.
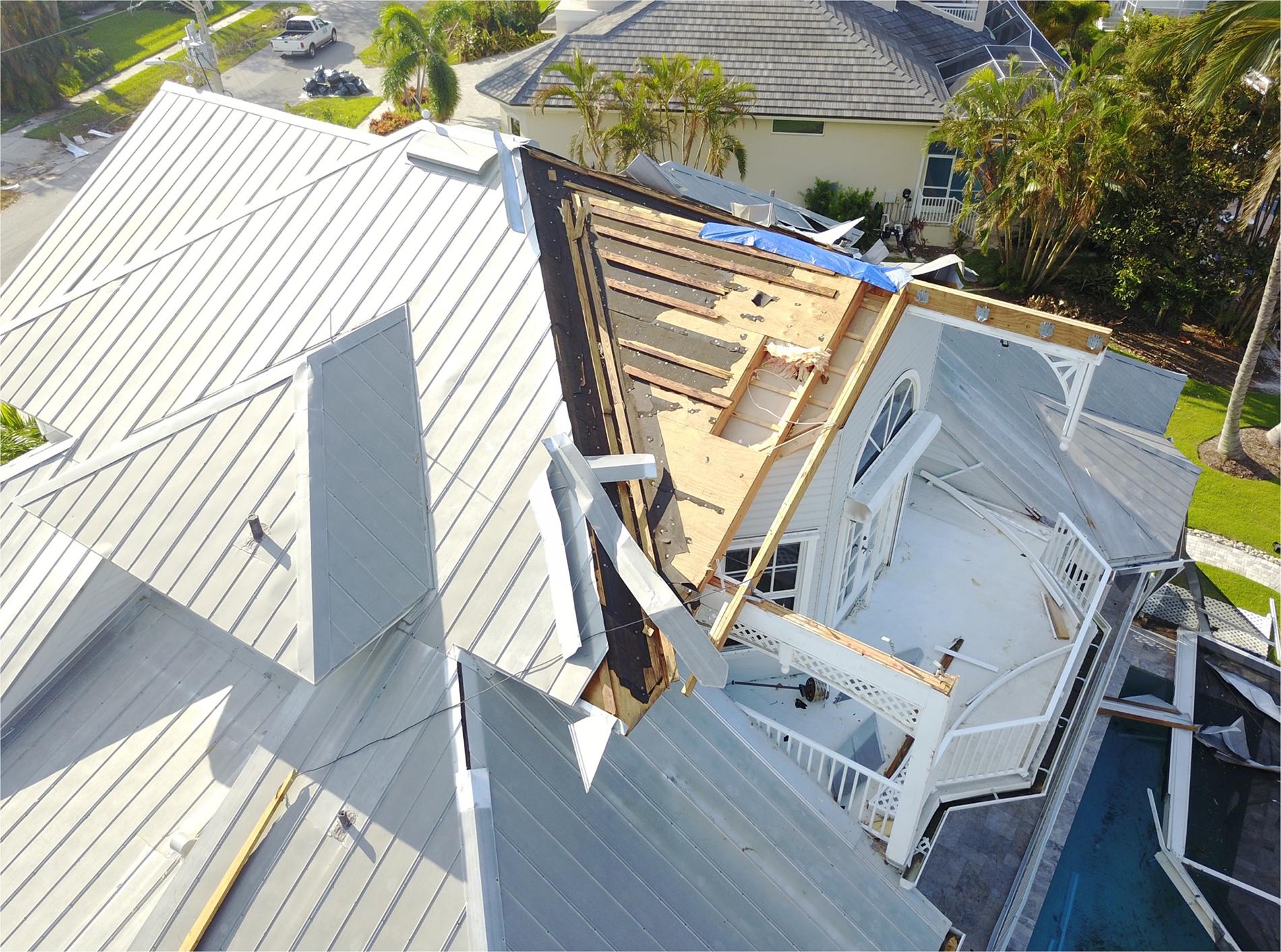
Figure 7. UAVs with high-resolution cameras are well-suited capture perishable data (e.g., roof cover damage, debris field), and provide complementary datasets for ground-based damage surveys. The areal perspective of UAVs reveals structural damage that is hidden from the view of ground-based damage surveyors. Photograph by Kwasi Oerry was acquired under sponsorship from the Florida Building Commission.
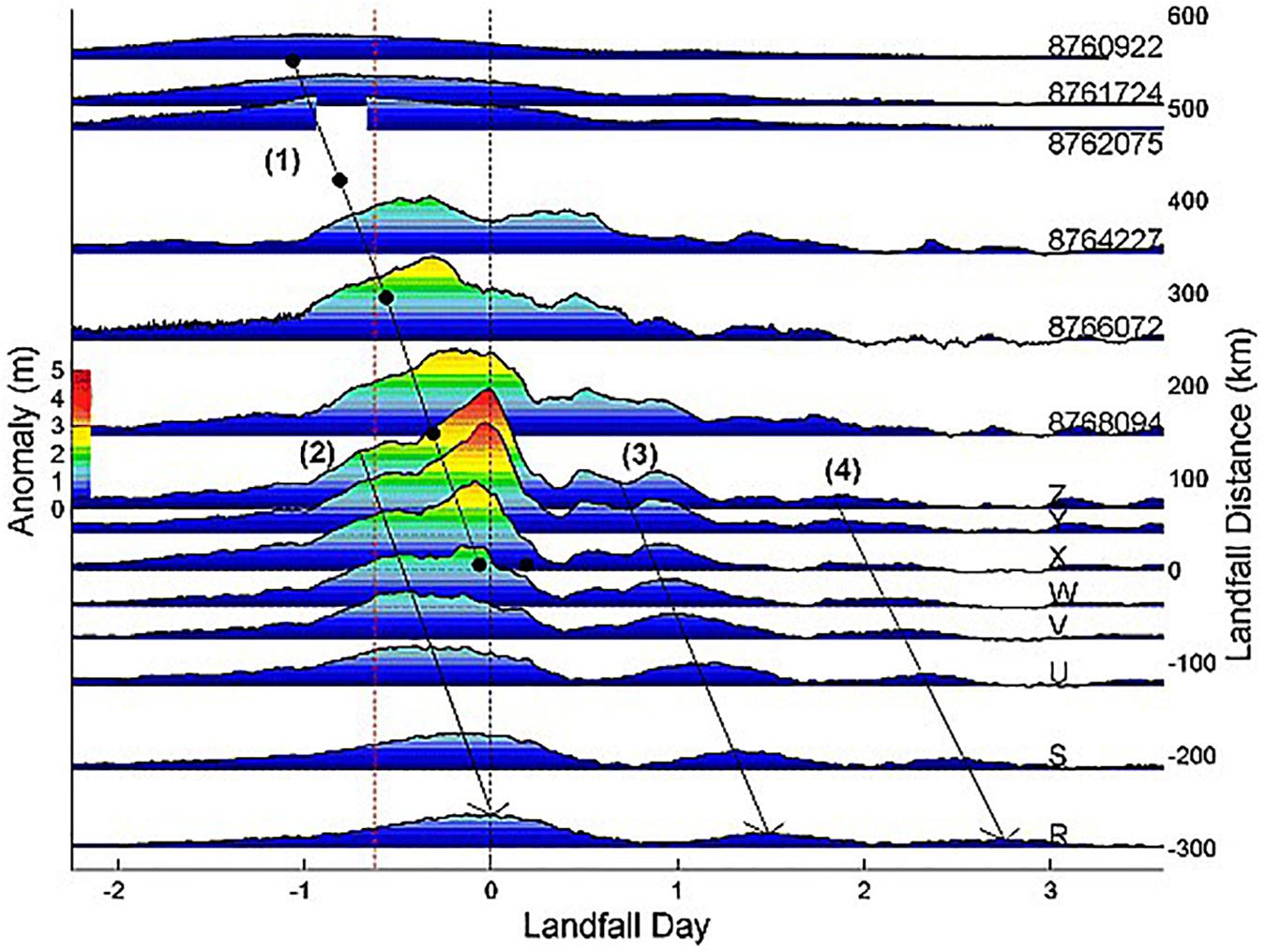
Figure 8. Observed time series of water level anomaly during Hurricane Ike (2008) along the open coasts of Louisiana and Texas (top-to-bottom show easternmost locations to westernmost locations). Data shown include rapidly installed pressure sensors (R-Z) by A. Kennedy (University of Notre Dame) and NOAA stations (8760922, 8761724, 8762075, 8764227, 8766072, 8768094). Line 1 shows the location of Hurricane Ike, while Line 2 shows the propagation of the forerunner wave. Reproduced from Kennedy et al. (2011) with permission of the publisher.
Temporal Scales
Resilience is the central, unifying goal of the natural hazards and disaster research communities (e.g., National Research Council [NRC], 2011 ; National Research Council, 2012 ). The term refers to an impacted community’s ability to resist, absorb, accommodate, adapt to, transform and ultimately recover and move on from the effects of a hazard in a timely and efficient manner ( United Nations, 2017 ). A path toward better upstanding, assessing, and improving resilience involves collecting and analyzing data over time frames representing conditions and states before, during, and after significant natural hazard events. Data on pre-event or “before” conditions are essential for understanding the pre-existing factors that influence, shape, and define a community’s response to a natural hazard event. With its emphasis on post-event response, the collection of pre-event data is largely outside the scope of the traditional reconnaissance community; however, much of this data currently exists or is being collected by governmental agencies and authorities, non- governmental organizations, and the private sector.
Moreover, there exists an opportunity for the natural hazards and disaster communities to lead organized efforts to catalog, organize, and synthesize such data, making it possible to link them with reconnaissance data. Data on the direct impacts of an event (“during event data”) are the traditional focus of reconnaissance investigations. These data provide critical information on the character of the loadings and the consequent physical response of the built environment, the immediate social, economic and public health impacts on communities, and interactions between these. These data also represent the starting point for recovery from natural hazard events. After an event, data collected are critical for understanding the response, recovery, and evolution of communities following events. Collecting data representing during and after events conditions requires both traditional rapid response reconnaissance investigations and follow-up data-gathering efforts. These longer-term data gathering investigations may span periods of weeks, months, or years, depending on the nature of the event and the characteristics of the affected communities.
Geospatial Scales
Natural hazard events often impact areas spanning 100s-to-1000s of square kilometers. Their widespread geographic distribution makes them, by definition, regional-scale events. The resulting damage and impact patterns reflect the fundamental nature of the hazard and the characteristics of the communities and built systems within affected regions. Over the past several decades, the ability to analyze the effects of natural hazards at the site- and building-scales has significantly improved, leading to better modeling tools, new building technologies, and robust building codes. In recent years the natural hazards and disaster communities have shown a growing interest in regional-scale impact modeling. A key advantage of regional-scale models is their ability to forecast the distribution of hazard impacts and thus capture system-level performance and propagation of risk across a region. Such models are particularly important when considering the impact of hazards on geographically distributed critical infrastructure systems.
Improving our understanding of hazard impacts and advancing regional scale modeling requires collection and synthesis of data over spatial scales spanning multiple orders of magnitude (i.e., from the site-specific to the regional scales; ∼m 2 to ∼km 2 ). This necessitates a portfolio of instrumentation that can facilitate the acquisition of fine-grained, high-resolution “site-specific” data and also support the collection in a practical manner of data from a much broader area. This also requires reconnaissance investigations to be conducted at both local and regional scales. Acquiring multiscale data enables the local impacts of a hazard to be understood in the broader context of regional-scale loading patterns and community characteristics. Equally important, this data can support the information necessary to bridge site-specific and regional scale models, which improves the ability to simulate the consequences of an extreme event across a vast region.
Social Scales
Natural hazard events can have immensely varying impacts and consequences at all social scales, from individuals and households to neighborhoods and communities; organizations, businesses, and governments; and up to and including countries, cultures, and global consequences (e.g., Oliver-Smith, 1996 ; Paton and Johnston, 2001 ; Quarantelli, 2003 ; Boon et al., 2012 ). As the Covid-19 pandemic wreaks havoc on individual lives, senior centers, vulnerable communities, nations, and the global economy, inequities and the heterogeneity of hazard effects at different social scales have commanded renewed attention (e.g., Adams-Prassl et al., 2020 ). Differences in natural and built environments contribute to potentially predictable variation in hazard impacts on society and individuals and can interact with societal responses ( Paton and Johnston, 2001 ). Infrastructure damages can hinder immediate and longer-term responses, including emergency responses, evacuation, and sheltering, but also communications and governance. Direct hazard effects on the physical environment, such as flooding, landslides, and fire, are not only potentially deadly to individuals but can also cause longer-term mental harm and disrupt social and economic activities at multiple scales. However, the lack of population-representative fine-scaled data on damages and human exposures for natural hazards and disasters continues to be called out (e.g., Bakkensen and Mendelsohn, 2016 ).
Assessing hazard exposures and consequences across these domains and social scales requires instrumentation and data collection sensitive to and associated with social, built, and natural environmental conditions, as well as temporal and spatial scales. Data collection processes that are multi-scalar and consider the social processes that can make people hard to reach will have a better chance of representing minority populations most likely to be among the most vulnerable to the majority of hazard events ( Shaghaghi et al., 2011 ). Data can be contextualized with the appropriate metadata, but also improved by designing direct data collections—such as observations, interviews, and surveys—to address these contextual factors and link geophysical, engineering, and social data. Social scientists have long acknowledged interactions across social scales (e.g., Bronfenbrenner and Morris, 2006 ). New technologies, analytical approaches, and data sources—such as biophysical and EEG (electroencephalogram) measurement tools (e.g., Bailey et al., 2017 ), crowdsourcing (e.g., Cobb et al., 2014 ), social media (e.g., Chae et al., 2014 ; Spence et al., 2016 ; Wang and Taylor, 2018 ), and satellite observations of night lights and other forms of evidence of human activities and interventions at larger scales (e.g., Ehrlich et al., 2009 ; Ceola et al., 2014 )—can enable researchers to examine these interactions in new ways. They can also support insights into and simulations of how individual responses and behaviors contribute to or are shaped by responses and events at larger social scales.
Multidisciplinary Data Sets
A disaster is a severe disruption of the functioning of a community or a society at any scale due to hazardous events interacting with conditions of exposure, vulnerability, and capacity leading to human, material, economic and environmental losses and/or impacts ( United Nations, 2020 ). A better understanding of the complicated relationship between hazards, the built environment, and communities requires that the physical and socioeconomic factors leading to disasters be untangled. Accomplishing this requires the reconnaissance community to collect and synthesize multidisciplinary data sets. In addition to improving our fundamental understanding of disasters, these data can play a critical role in establishing relationships between hazards and their broad consequences, ultimately leading to an improved ability to model, manage, and mitigate risk to communities.
Natural hazard events provide extraordinary opportunities to improve our fundamental understanding of disasters and their consequences. This understanding is critical for reducing the growing human and capital losses arising from extreme events (e.g., Coronese et al., 2019 ). To minimize losses, the natural hazard and disaster research and practice communities must meet several key challenges related to improving modeling and design making, community resilience, and hazard mitigation (e.g., National Research Council [NRC], 2011 ). Reconnaissance data, which captures real-world complexities of events (e.g., the interplay between natural, human, and built systems), plays an increasingly important role in meeting these challenges. The recent availability of state-of-the-art instrumentation and mobile data collection applications has dramatically improved the quality and increased the quantity of disaster data, paving the way toward a new era of natural hazards reconnaissance. However, to fully realize the potential of these advancements, we must employ new strategic approaches for natural hazards reconnaissance that acquire and integrate data over a range of temporal, spatial, and social scales across disciplines. Specifically, this involves the following.
(1) Data collection over time frames representing conditions and states before, during, and after significant natural hazard events.
(2) The collection and synthesis of data over spatial scales spanning multiple orders of magnitude (i.e., from the site-specific to the regional scales; ∼m 2 to ∼km 2 ).
(3) Data collection is sensitive to and associated with social, built, and natural environmental conditions, and considers the social processes that can make populations hard to reach.
(4) The collection and synthesis of multidisciplinary data sets to establish relationships between hazard events, their antecedents, and their broad consequences, ultimately leading to an improved ability to model, manage, and mitigate disaster risk to communities.
Data Availability Statement
All datasets generated for this study are included in the article/ Supplementary Material .
Author Contributions
JW, JB, AB, SM, MO, KG, JI, LL, TT, and JD developed the RAPID Facility science plan reported in this article. SM and AB designed the community workshop and later worked in close collaboration with JW and JB to interpret the result and develop findings. MG, AL, and JP work with members of the reconnaissance community users to implement the science plan into field missions. All authors contributed to manuscript drafting and revision, and read and approved the submitted version.
The U.S. National Science Foundation supported this work under grant number 1611820. Any opinions, findings, and conclusions or recommendations expressed in this material are those of the authors and do not necessarily reflect the views of the National Science Foundation.
Conflict of Interest
The authors declare that the research was conducted in the absence of any commercial or financial relationships that could be construed as a potential conflict of interest.
Acknowledgments
We thank the many experts (see the list in Supplementary Material ) who participated in the January 2017 reconnaissance workshop in Seattle and engaged in thoughtful discussions that led to many of the ideas expressed in this article. A portion of the content if this manuscript has been published as part of the RAPID Facility Science Plan ( RAPID Facility, 2017 ).
Supplementary Material
The Supplementary Material for this article can be found online at: https://www.frontiersin.org/articles/10.3389/fbuil.2020.573068/full#supplementary-material
Adams-Prassl, A., Boneva, T., Golin, M., and Rauh, C. (2020). Inequality in the Impact of the Coronavirus Shock: Evidence from Real Time Surveys. IZA Discussion Papers, No. 13183. Bonn: Institute of Labor Economics (IZA).
Google Scholar
Aitsi-Selmi, A., Egawa, S., Sasaki, H., Wannous, C., and Murray, V. (2015). The sendai framework for disaster risk reduction: renewing the global commitment to people’s resilience, health, and well-being. Int. J. Disast. Risk Sci. 6, 164–176. doi: 10.1007/s13753-015-0050-9
CrossRef Full Text | Google Scholar
Allen, R. M., and EERI Reconnaissance Team (2017). Quake warnings, seismic culture. Science 358:1111. doi: 10.1126/science.aar4640
PubMed Abstract | CrossRef Full Text | Google Scholar
Bailey, A. W., Johann, J., and Kang, H. (2017). Cognitive and physiological impacts of adventure activities: beyond self-report data. J. Exper. Educ. 40, 153–169. doi: 10.1177/1053825917701250
Bakkensen, L. A., and Mendelsohn, R. O. (2016). Risk and adaptation: evidence from global hurricane damages and fatalities. J. Assoc. Environ. Resour. Econ. 3, 555–587. doi: 10.1086/685908
Baradaranshoraka, M., Pinelli, J.-P., Gurley, K., Peng, X., and Zhao, M. (2017). Hurricane wind versus storm surge damage in the context of a risk prediction model. ASCE J Struct. Eng. 143:04017103. doi: 10.1061/(ASCE)ST.1943-541X.0001824
Baradaranshoraka, M., Pinelli, J.-P., Gurley, K., Zhao, M., Peng, X., and Paleo-Torres, A. (2019). Characterization of coastal flood damage states for residential buildings. ASCE ASME J. Risk Uncertain. Eng. Syst. 5:04019001. doi: 10.1061/AJRUA6.0001006
Barbosa, A. R., Fahnestock, L. A., Fick, D. R., Gautam, D., Soti, R., Wood, R., et al. (2017). Performance of medium-to-high rise reinforced concrete frame buildings with masonry infill in the 2015 Gorkha, Nepal, earthquake. Earthq. Spec. 33, 197–218. doi: 10.1193/051017eqs087m
Berman, J. W., Wartman, J., Olsen, M. J., Irish, J., Miles, S., Tanner, T., et al. (in press). Natural hazards reconnaissance with the NHERI RAPID facility. Front. Built Environ.
Blain, C., Bobet, A., Browning, J., Edge, B., Holmes, W., Johnson, D., et al. (2020). The Network coordination office of NHERI (Natural hazards engineering research infrastructure). Front. Built Environ. 6:108.
Boon, H., Cottrell, A., King, D., Stevenson, R., and Millar, J. (2012). Bronfenbrenner’s bioecological theory for modelling community resilience to natural disasters. Nat. Hazards 60, 381–408. doi: 10.1007/s11069-011-0021-24
Brando, G., Rapone, D., Spacone, E., Matt, S. O., and Olsen, M. J. (2017). Damage reconnaissance of unreinforced masonry bearing wall buildings after the 2015 Gorkha, Nepal, earthquake. Earthq. Spec. 33, 243–273. doi: 10.1193/010817eqs009m
Bray, J. D., Frost, J. D., Rathje, E. M., and Garcia, F. E. (2019). Recent advances in geotechnical post-earthquake reconnaissance. Front. Built Environ. 5:5. doi: 10.3389/fbuil.2019.00005
Bronfenbrenner, U., and Morris, P. (2006). “The bioecological model of human development,” in Handbook of Child Psychology: Theoretical Models of Human Development , 6th Edn, eds R. M. Lerner and W. Damon (Washington, DC: John Wiley & Sons Inc), 793–828.
Ceola, S., Laio, F., and Montanari, A. (2014). Satellite nighttime lights reveal increasing human exposure to floods worldwide. Geophys. Res. Lett. 41, 7184–7190. doi: 10.1002/2014GL061859
Chae, J., Thom, D., Jang, Y., Kim, S., Ertl, T., and Ebert, D. S. (2014). Public behavior response analysis in disaster events utilizing visual analytics of microblog data. Comput. Graph. 38, 51–60. doi: 10.1016/j.cag.2013.10.008
Cobb, C., Mccarthy, T., Perkins, A., Bharadwaj, A., Comis, J., Do, B., et al. (2014). “Designing for the deluge: understanding & supporting the distributed, collaborative work of crisis volunteers,” in Proceedings of the 17th ACM Conference on Computer Supported Cooperative Work & Social Computing , New York, NY.
Cong, Z., Nejat, A., Liang, D., Pei, Y., and Javid, R. J. (2018). Individual relocation decisions after tornadoes: a multi-level analysis. Disasters 42, 233–250. doi: 10.1111/disa.12241
Coronese, M., Lamperti, F., Keller, K., Chiaromonte, F., and Roventini, A. (2019). Evidence for sharp increase in the economic damages of extreme natural disasters. Proc. Natl. Acad. Sci. U.S.A. 116, 21450–21455. doi: 10.1073/pnas.1907826116
Coulbourne, W., Galsworthy, J., Hangan, H., Jones, C., Letchford, C., Smith, T., et al. (2014). Measurement Science R&D Roadmap for wIndstorm and Coastal Inundation Impact Reduction. Grant/Contract Reports (NISTGCR)-14-973-13. Available online at: www.nist.gov/publications/measurement-science-rd-roadmap-windstorm-and-coastal-inundation-impact-reduction (accessed October 9, 2020).
Dashti, S., Palen, L., Heris, M. P., Anderson, K. M., Anderson, T. J., and Anderson, S. (2014). “Supporting disaster reconnaissance with social media data: A design-oriented case study of the 2013 Colorado floods,” in Proceedings of the 11th International Conference on Information Systems for Crisis Response and Management (ISCRAM) , University Park, PA.
Dietrich, J. C., Zijlema, M., Westerink, J. J., Holthuijsen, L. H., Dawson, C., Luettich, R. A., et al. (2011). Modeling hurricane waves and storm surge using integrally-coupled, scalable computations. Coast. Eng. 58, 45–65. doi: 10.1016/j.coastaleng.2010.08.001
Earthquake Engineering Research Institute [EERI] (1971). Earthquake Investigation Committee Los Angeles Earthquake of February 9, 1971., ed. Moran, D., Meehan, J. F., Pinkham, C. W., Brugger, W. A., Allen, C., Duke, C. M., Housner, G. W., Degenkolb, H. J., Crandall, L. Available online at: https://www.eeri.org/1971/02/san-fernando/ (accessed June 12, 2020).
Edge, B., Ramirez, J., Peek, L., Bobet, A., Holmes, W., Robertson, I., et al. (2020). Natural Hazards Engineering Research Infrastructure, 5-Year Science Plan, Multi-Hazard Research To Make a More Resilient World, Second Edition. DesignSafe-CI. Available online at: https://www.designsafe-ci.org/data/browser/public/designsafe.storage.published/PRJ-2731 (accessed September 25, 2020).
Eguchi, R., Huyck, C., Ghosh, S., and Adams, B. (2008). “The application of remote sensing technologies for disaster management,” in Proceedings of the 14th World Conference on Earthquake Engineering , Beijing.
Ehrlich, D., Guo, H. D., Molch, K., Ma, J. W., and Pesaresi, M. (2009). Identifying damage caused by the 2008 Wenchuan earthquake from VHR remote sensing data. Int. J. Digit. Earth 2, 309–326. doi: 10.1080/17538940902767401
Ellsworth, W. L. (1990). “Earthquake history 1769-1989,” in The San Andreas Fault System , ed. R. E. Wallace (California: U. S. Geological Survey), 153–178.
Eltner, A., Kaiser, A., Castillo, C., Rock, G., Neugirg, F., Abellá, L., et al. (2016). Image-based surface reconstruction in geomorphometry – merits, limits and developments. Earth Surf. Dynam. 4, 359–389. doi: 10.5194/esurf-4-359-2016
FEMA (2012). “Seismic evaluation and retrofit of multi-unit wood-frame buildings with weak first stories,” in FEMA P-807 (Washington, DC: Federal Emergency Management Agency).
FEMA (2018). Seismic Performance Assessment of Buildings: FEMA P-58-1. Washington, DC: Federal Emergency Management Agency.
Fischer, E. C., and Hakhamaneshi, M. (2019). The New Paradigm of Post-Disaster Reconnaissance. Geotechnics Of Extreme Events. Available online at: https://www.readgeo.com/geostrata/may_jun_2019/MobilePagedArticle.action?articleId=1489220#articleId1489220 (accessed June 12, 2020).
Frankel, A., Wirth, E., Marafi, N., Vidale, J., and Stephenson, W. (2018). Broadband synthetic seismograms for magnitude 9 Earthquakes on the Cascadia megathrust Based on 3D simulations and stochastic synthetics, Part 1: methodology and overall results. Bull. Seismol. Soc. Am. 108, 2347–2369. doi: 10.1785/0120180034
Gaillard, J. C., and Peek, L. (2019). Disaster-zone research needs a code of conduct. Nature 575, 440–442. doi: 10.1038/d41586-019-03534-z
Gallant, A. P., Montgomery, J., Mason, H. B., Hutabarat, D., Reed, A. N., Wartman, J., et al. (2020). The sibalaya flowslide initiated by the 28 September 2018 MW 7.5 Palu-Donggala, Indonesia earthquake. Landslides 17, 1925–1934. doi: 10.1007/s10346-020-01354-1
Geli, L., Bard, P.-Y., and Jullien, B. (1988). The effect of topography on earthquake ground motion: a review and new results. Bull. Seismol. Soc. Am. 78, 42–63. doi: 10.1016/0148-9062(88)90024-1
Geotechnical Extreme Events Reconnaissance [GEER] (2014). Manual for GEER Reconnaissance Teams, Ver 4., ed.Kayen, R. Available online at: http://www.geerassociation.org/media/files/Important%20Docs/GEER_Recon_Team_Manual_2014_v4.pdf (accessed June 12 2020).
Grant, A., Wartman, J., Massey, C., Olsen, M., O’Banion, M., and Motley, M. (2018). The impact of rockfalls on dwellings during the 2011 Christchurch, New Zealand, earthquakes. Landslides 15, 31–42. doi: 10.1007/s10346-017-0855-852
Gurley, K. R., and Masters, F. J. (2011). Post-2004 hurricane field survey of residential building performance. ASCE Nat. Haz. Rev. 12, 177–183. doi: 10.1061/(ASCE)NH.1527-6996.0000044
Hamid, S. S., Pinelli, J.-P., Chen, S.-C., and Gurley, K. (2011). Catastrophe model-based assessment of hurricane risk and estimates of potential insured losses for the State of Florida. ASCE Nat. Haz. Rev. 12, 171–176. doi: 10.1061/(ASCE)NH.1527-6996.0000050
Harp, E., Keefer, D., Hiroshi, S., and Yagi, H. (2011). Landslide inventories: The essential part of seismic landslide hazard analyses. Eng. Geol. 122, 9–21. doi: 10.1016/j.enggeo.2010.06.013
Hughes, K. S., and Morales Vélez, A. C. (2017). “Characterization of landslide sites in Puerto Rico after Hurricanes Irma and Maria,” in Proceedings of the American Geophysical Union, Fall Meeting 2017, Abstract #NH23E-2859 , Washington, DC.
Johnson, D. R., Blain, C. A., Bobet, A., Browning, J., Edge, B., Holmes, B., et al. (2020). The Network coordination office of NHERI (Natural hazards engineering research infrastructure). Front. Built Environ. 6:108. doi: 10.3389/fbuil.2020.00108
Kang, H., Burton, H. V., and Miao, H. (2018). Replicating the recovery following the 2014 south napa earthquake using stochastic process models. Earthq. Spect. 34, 1247–1266. doi: 10.1193/012917EQS020M
Kanvinde, A. M., Marshall, K. S., Grilli, D. A., and Bomba, G. (2015). Forensic analysis of link fractures in eccentrically braced frames during the February 2011 Christchurch Earthquake: testing and simulation. J. Struct. Eng. 141:5. doi: 10.1061/(ASCE)ST.1943-541X.0001043
Kaplan, K. (2010). Mining Destruction for Data to Help Others. Los Angeles Times, February 1. Available online at: https://web.archive.org/web/20151011135150/http://articles.latimes.com/2010/feb/01/science/la-sci-disaster-research1-2010feb01/2 (accessed September 25, 2020).
Kennedy, A., Copp, A., Florence, M., Gradel, A., Gurley, K., Janssen, M., et al. (2020a). Hurricane Michael (2018) in the area of Mexico Beach, Florida. J. Water Port. C. 146:05020004. doi: 10.1061/(ASCE)WW.1943-5460.0000590
Kennedy, A., Cox, D., Irish, J., Kaihatu, J., Lynett, P., and Tomiczek, T. (2020b). “Envisioning the future coast: coastal engineering research in the coming decades,” in Proceedings of the A report from the Coastal Engineering Research Framework Workshop, November 13–14, 2018 , Arlington, VA.
Kennedy, A. B., Gravois, U., Zachry, B. C., Westerink, J. J., Hope, M. E., Dietrich, J. C., et al. (2011). Origin of the Hurricane Ike forerunner surge. Geophys. Res. Lett. 38:L08608. doi: 10.1029/2011GL047090
Kircher, C. A., Whitman, R. V., and Holmes, W. T. (2006). HAZUS Earthquake Loss Estimation. Methods Nat. Hazards Rev. 7:45. doi: 10.1061/(ASCE)1527-6988
Knowles, S. G. (2012). The Disaster Experts: Mastering Risk in Modern America. Philadelphia: University of Pennsylvania Press.
Lawson, A. C., and Reid, H. F. (1908). The California Earthquake of April 18, 1906. Report of the State Earthquake Investigation Commission. Washington, DC: Carnegie Institution of Washington.
LeVeque, R. J., George, D. L., and Berger, M. J. (2011). Tsunami modelling with adaptively refined finite volume methods. Acta Numer. 20, 211–289. doi: 10.1017/S0962492911000043
Loggins, R., Little, R., Mitchell, J., Sharkey, T., and Wallace, W. (2019). CRISIS: modeling the restoration of interdependent civil and social infrastructure systems following an extreme event. Nat. Hazards Rev. 20:326. doi: 10.1061/(ASCE)NH.1527-6996.0000326
Lombardo, F. T., Roueche, D. B., and Prevatt, D. O. (2015). Comparison of two methods of near-surface wind speed estimation in the 22 May, 2011 Joplin, Missouri Tornado. J. Wind Eng. Indust. Aerodyn. 138, 87–97. doi: 10.1016/j.jweia.2014.12.007
Mandli, K. T., and Dawson, C. N. (2014). Adaptive mesh refinement for storm surge. Ocean Model. 75, 36–50. doi: 10.1016/j.ocemod.2014.01.002
Mazzoni, S., Castori, G., Galasso, C., Calvi, P., Dreyer, R., Fischer, E., et al. (2018). 2016–2017 Central Italy earthquake sequence: seismic retrofit policy and effectiveness. Earthq. Spec. 34, 1671–1691. doi: 10.1193/100717EQS197M
McAllister, T., Clavin, C., Ellingwood, B., van de Lindt, J. W., Mizzen, D., and Lavelle, F. (2019). Data, Information, and Tools Needed for Community Resilience Planning and Decision Making. Gaithersburg, MD: National Institute of Standards and Technology.
Miles, S. B. (2015). Foundations of community disaster resilience: well-being, identity, services, and capitals. Environ. Hazards 14, 103–121. doi: 10.1080/17477891.2014.999018
Miles, S. B., and Chang, S. E. (2011). ResilUS: a community based disaster resilience model. Cartogr. Geogr. Inform. Sci. 38, 36–51. doi: 10.1559/1523040638136
Miles, S. B., Gallagher, H., and Huxford, C. J. (2014). Restoration and impacts from the September 8, 2011, San Diego Power Outage. J. Infrastruct. Syst. 20:176. doi: 10.1061/(ASCE)IS.1943-555X.0000176
Miles, S. B., and Tanner, T. (2018). “Designed a disaster reconnaissance field app with a user-centered approach,” in Proceedings of the 11th U.S. National Conference of Earthquake Engineering , Los Angeles, CA.
Montgomery, J., Candia, G., Lemnitzer, A., and Martinez, A. (2020). The September 19, 2017 Mw 7.1 Puebla-Mexico City earthquake: observed rockfall and landslide activity. Soil Dyn. Earthq. Eng. 130:105972. doi: 10.1016/j.soildyn.2019.105972
National Earthquake Hazards Reduction Program (2008). Strategic Plan for the National Earthquake Hazards Reduction Program, Fiscal Years 2009–2013.
National Research Council [NRC] (2011). Grand Challenges in Earthquake Engineering Research: A. (Community) Workshop Report. Washington DC: The National Academies Press.
National Research Council (2012). Disaster Resilience: A National Imperative. Washington, DC: The National Academies Press. doi: 10.17226/13457
Nature Geoscience (2017). Progress from catastrophe. Nat. Geosci. 10:537. doi: 10.1038/ngeo3004
Nejat, A., Moradi, S., and Ghosh, S. (2019). Anchors of social network awareness index: a key to modeling postdisaster housing recovery. J. Infrastruct. Syst. 25:04019004. doi: 10.1061/(asce)is.1943-555x.0000471
Newmark, N. M. (1965). Effects of earthquakes on dams and embankments. Geotechnique 15, 139–159. doi: 10.1680/geot.1965.15.2.139
Oliver-Smith, A. (1996). Anthropological research on hazards and disasters. Annu. Rev. Anthropol. 25, 303–328. doi: 10.1146/annurev.anthro.25.1.303
Olsen, M., and Kayen, R. (2013). “Post-Earthquake and Tsunami 3D laser scanning forensic investigations,” in Proceedings of the Sixth Congress on Forensic Engineering , San Francisco, CA.
Olsen, M. J., Gillins, D. T., Cubrinovski, M., Bradley, B. A., Price, C., and Chin, C. Y. (2015). “How can geomatics technologies benefit geotechnical studies?,” in Proceedings of the 6th International Conference on Earthquake Geotechnical Engineering , Christchurch.
Özyeşil, O., Voroninski, V., Basri, R., and Singer, A. (2017). A survey of structure from motion. Acta Numer. 26, 305–364. doi: 10.1017/S096249291700006X
Pagani, M., Monelli, D., Weatherill, G., Danciu, L., Crowley, H., Silva, V., et al. (2014). OpenQuake engine: an open hazard (and Risk) software for the global Earthquake model. Seismol. Res. Lett. 85, 692–702. doi: 10.1785/0220130087
Paton, D., and Johnston, D. (2001). Disasters and communities: vulnerability, resilience and preparedness. Disaster Prev. Mgmt. Intern. J. 10, 270–277. doi: 10.1108/EUM0000000005930
Peek, L., Tobin, J., Adams, R., Wu, H., and Mathews, M. (2020). A framework for convergence research in the hazards and DisasterField: the natural hazards engineering research infrastructure CONVERGE facility. Front. Built Environ. 6:110. doi: 10.3389/fbuil.2020.00110
Pinelli, J.-P., David, R., Kijewski-Correa, T., Fernando, P., David, P., Ioannis, Z., et al. (2018). “Overview of damage observed in regional construction during the passage of Hurricane Irma over the State of Florida,” in Proceedings of the Forging Forensic Frontiers, ASCE Forensic Engineering 8th Congress , Austin, TX.
Pita, G., Pinelli, J.-P., Gurley, K., and Mitrani-Reiser, J. (2015). State of the art of Hurricane vulnerability estimation methods: a review. ASCE Nat. Haz. Rev. 16:04014022. doi: 10.1061/(ASCE)NH.1527-6996.0000153
Pita, G. L., Pinelli, J.-P., Gurley, K. R., and Hamid, S. (2013). Hurricane vulnerability modeling: development and future trends. J. Wind Eng. Ind. Aerod. 114, 96–105. doi: 10.1016/j.jweia.2012.12.004
Prince, S. H. (1920). Catastrophe and Social Change Based Upon a Sociological Study of the Halifax Disaster. Chapel Hill: Project Gutenberg.
Quarantelli, E. (2003). A Half Century Of Social Science Disaster Research:Selected Major Findings And Their Applicability. University of Delaware Preliminary Paper No. 336. Newark, DE: Disaster Research Center.
RAPID Facility (2017). Science Plan. Available online at: https://rapid.designsafe-ci.org/media/filer_public/f5/a8/f5a875ed-6061-4795-89ab-eeb8f9c24d67/additional_materials.pdf (accessed June 19, 2020).
Rathje, E., and Franke, K. (2016). Remote sensing for geotechnical earthquake reconnaissance. Soil Dyn. Earthq. Eng. 91, 304–316. doi: 10.1016/j.soildyn.2016.09.016
Rathje, E. M., and Bray, J. D. (2000). Nonlinear coupled seismic sliding analysis of earth structures. J. Geotech. Geoenviron. Eng. 126, 1002–1014. doi: 10.1061/(ASCE)1090-0241(2000)126:11(1002)
Rathje, E. M., Dawson, C., Padgett, J. E., Pinelli, J.-P., Stanzione, D., Adair, A., et al. (2017). DesignSafe: new cyberinfrastructure for natural hazards engineering. Nat. Haz. Rev. 18:06017001. doi: 10.1061/(ASCE)NH.1527-6996.0000246
Reid, H. F. (1910). The Mechanics of the Earthquake, The California Earthquake of April 18, 1906; Report of the State Investigation Commission. Washington, DC: Carnegie Institution of Washington.
Roelvink, D., Reniers, A., van Dongeren, A., van Thiel de Vries, J. A., Mccall, R., and Lescinski, J. (2009). Modelling storm impacts on beaches, dunes and barrier islands. Coast. Eng. 56, 11–12. doi: 10.1016/j.coastaleng.2009.08.006
Schenk, G. J. (2007). Historical disaster research. state of research, concepts, methods and case studies. Histor. Soc. Res. 32:3.
Shaghaghi, A., Bhopal, R., and Sheikh, A. (2011). Approaches to recruiting ‘hard-to-reach’ populations into research: a review of the literature. Health Promot. Perspect. 1, 86–94. doi: 10.5681/hpp.2011.009
Silva-Tulla, F., and Nicholson, P. (2007). Embankments, Dams, and Slopes: Lessons From the New Orleans Levee Failures and Other Current Issues. Reston, VA: American Society of Civil Engineers.
Smith, T., Holmes, W., and Edge, B. (2017). Natural Hazards Engineering Research Infrastructure, Five-Year Science Plan, Multi-Hazard Research to Make a More Resilient World , 1st Edn, Arlington, VA: DesignSafe-CI.
Spence, P., Lachlan, K., and Rainear, A. (2016). Social media and crisis research: data collection and directions. Comput. Hum. Behav. 54, 667–672. doi: 10.1016/j.chb.2015.08.045
Stone, H., D’Ayala, D., and Wilkinson, S. (2017). The Use of Emerging Technology in Post- Disaster Reconnaissance Missions, Earthquake Engineering Field Investigation Team Report. Available online at: https://www.istructe.org/IStructE/media/Public/Resources/report-eefit-grant-emerging-technology-reconnaissance-20170131.pdf (accessed June 5, 2020).
Tülüveli, G. (2015). Historical seismicity in the Middle East: new insights from Ottoman primary sources (sixteenth to mid- eighteenth centuries). J. Seismol. 19, 1003–1008. doi: 10.1007/s10950-015-9499-7
United Nations (2017). United Nations Office for Disaster Reduction, Terminology. Available online at: www.undrr.org/terminology/resilience (accessed October 9, 2020).
United Nations (2020). United Nations Office for Disaster Reduction, Terminology. New York, NY: United Nations.
van de Lindt, J. W., Ellingwood, B., McAllister, T., Gardoni, P., Cox, D., Cutler, H., et al. (2015). “Computational environment for modeling and enhancing community resilience: Introducing the center for risk-based community resilience planning,” in Proceedings of the 2nd International Conference on Performance-based and Life-Cycle Structural Engineering (PLSE 2015) , Gaithersburg, MD.
Wang, Y., and Taylor, J. (2018). Coupling sentiment and human mobility in natural disasters: a Twitter-based study of the 2014 South Napa Earthquake. Nat. Hazards 92, 907–925. doi: 10.1007/s11069-018-3231-1
Wartman, J., Berman, J., Olsen, M. J., Irish, J. L., Miles, S., Gurley, K., et al. (2018). “The NHERI RAPID facility; enabling the next-generation of natural hazards reconnaissance,” in Proceedings of the 11th U.S. National Conference of Earthquake Engineering , Los Angeles, CA.
Wartman, J., Dunham, L., Tiwari, B., and Pradel, D. (2013). Landslides in eastern Honshu induced by the 2011 off the Pacific Coast of Tohoku earthquake. Bull. Seismol. Soc. Am. 103, 1503–1521. doi: 10.1785/0120120128
Wartman, J., Montgomery, D. R., Anderson, S. A., Keaton, J. R., Benoît, J., Dela Chapelle, J., et al. (2016). The 22 March 2014 Oso landslide, Washington, USA. Geomorphology 253, 275–288. doi: 10.1016/j.geomorph.2015.10.022
Wood, P. R., Elwood, K., Brunsdon, D. R., and Horspool, N. A. (2017). “Learning from earthquakes; past, present, future,” in Proceedings of the 2017 NZSEE Annual Technical Conference , Wellington, NZ.
Wood, R. L., Mohammadi, M. E., Barbosa, A. R., Abdulrahman, L., Soti, R., Kawan, C. K., et al. (2017). Damage assessment and modeling of the five-tiered pagoda-style Nyatapola temple. Earthq. Spec. 33, 377–384. doi: 10.1193/121516eqs235m
Xiao, Y., and Peacock, W. G. (2014). Do hazard mitigation and preparedness reduce physical damage to businesses in disasters? Critical role of business disaster planning. ASCE Nat. Haz. Rev. 15:137. doi: 10.1061/(ASCE)NH.1527-6996.0000137
Xiao, Y., and Van Zandt, S. (2012). Building community resiliency: spatial links between household and business post-disaster return. Urban Stud. 49, 2523–2542. doi: 10.1177/0042098011428178
Yamazaki, F., and Matsuoka, M. (2007). Remote sensing technologies in post-disaster damage assessment. J. Earthq. Tsunami 1, 93–210. doi: 10.1142/S1793431107000122
Yim, S. C., Olsen, M. J., Cheung, K. F., and Azadbakht, M. (2014). Tsunami modeling, fluid load simulation, and validation using geospatial field data. J. Struct. Eng. ASCE Special Issue Comp. Sim. Struct. Eng. 140, 1–14. doi: 10.1061/(ASCE)ST.1943-541X.0000940
Zhou, W., Li, H., Mao, C., Mevel, L., and Ou, J. (2013). Seismic damage detection for a masonry building using aftershock monitoring data. Adv. Struct. Eng. 16, 605–618. doi: 10.1260/1369-4332.16.4.605
Keywords : natural hazard, disaster, reconnaissance, instrumentation, simulation, data
Citation: Wartman J, Berman JW, Bostrom A, Miles S, Olsen M, Gurley K, Irish J, Lowes L, Tanner T, Dafni J, Grilliot M, Lyda A and Peltier J (2020) Research Needs, Challenges, and Strategic Approaches for Natural Hazards and Disaster Reconnaissance. Front. Built Environ. 6:573068. doi: 10.3389/fbuil.2020.573068
Received: 16 June 2020; Accepted: 28 September 2020; Published: 10 November 2020.
Reviewed by:
Copyright © 2020 Wartman, Berman, Bostrom, Miles, Olsen, Gurley, Irish, Lowes, Tanner, Dafni, Grilliot, Lyda and Peltier. This is an open-access article distributed under the terms of the Creative Commons Attribution License (CC BY) . The use, distribution or reproduction in other forums is permitted, provided the original author(s) and the copyright owner(s) are credited and that the original publication in this journal is cited, in accordance with accepted academic practice. No use, distribution or reproduction is permitted which does not comply with these terms.
*Correspondence: Joseph Wartman, [email protected]
This article is part of the Research Topic
Natural Hazards Engineering Research Infrastructure (NHERI) 2016-2020: Mitigating the Impact of Natural Hazards on Civil Infrastructure and Communities
Natural Hazards Research
Open access

- Guide for Authors
- Abstracting and Indexing
Editor-in-Chief
Editorial Board
Natural hazards can have catastrophic impacts. Natural Hazards Research promotes communication between the various fields that have an interest in natural disasters and catastrophic events, with the ...
Natural hazards can have catastrophic impacts. Natural Hazards Research promotes communication between the various fields that have an interest in natural disasters and catastrophic events, with the goal of raising awareness and improving detection and response.
The journal covers multiple disciplines in natural and social sciences, emphasizing new theories, techniques, methods and case studies. It also promotes the integration and interaction of multiple scientific disciplines, including geology, geophysics, geochemistry, petrology, geodesy and instrumentation.
The journal welcomes submissions exploring all kinds of hazards: geological, atmospheric, climatological, meteorological, oceanographic, hydrological, and ecological. Papers on space weather and space radiation are also welcome. Topics covered include, but are not limited to:
- The mechanisms and nature of related hazards
- Disaster detection, monitoring, and early warning
- Geospace observation for natural hazards
- Disaster vulnerability, hazard, and risk assessment and management
- Prevention and prediction of disasters
- High-teches for disaster mitigation and reduction
- Natural Hazards and human society
View full aims and scope
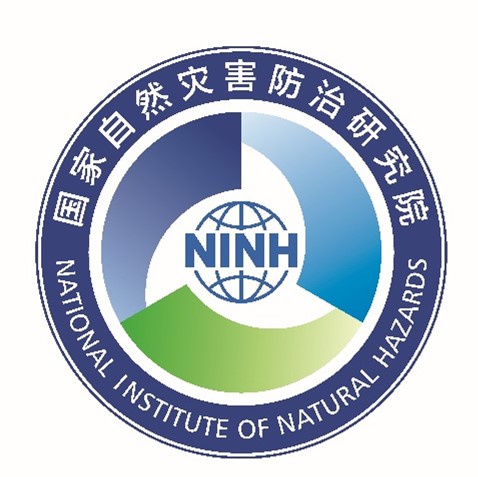
Society affiliation
The journal is owned by the National Institute of Natural Hazards (NINH), China‘s first specialized national research institute for comprehensive prevention and control of natural hazards. NINH focuses on hazards prevention and reduction and rescue. The efforts are underpinned by a philosophy of “inclusiveness, openness, and innovation&...
The journal is owned by the National Institute of Natural Hazards (NINH), China‘s first specialized national research institute for comprehensive prevention and control of natural hazards. NINH focuses on hazards prevention and reduction and rescue. The efforts are underpinned by a philosophy of “inclusiveness, openness, and innovation” and it is executed through a structural configuration characterized by a “small core, big network” driven by innovation-based science and technology.
NINH brings together outstanding researchers and resources from China and beyond to conduct scientific research, innovate technologies, talent development and dissemination of knowledge for natural hazards prevention and control. NINH also strives to be a world-class science innovation platform to support national needs and hazards prevention.
From more information about the society, please visit: http://www.ninhm.ac.cn/index.html
Meanwhile, the journal receives financial support from the Beijing Limited Company of Natural Hazards Prevention and Control Science and Technology Institute (NHPC Sci. & Tech. Co. Ltd.). This company is the sole wholly-owned entity enterprise of NINH. Established in 1989, NHPC Sci. & Tech. Co. Ltd. began with a registered capital of RMB5 million. Leveraging the robust research and development capabilities and technical infrastructure of NINH, the company has consistently been at the forefront of research, development, production, and service of geophysical field observation instruments and software. The company's products find wide-ranging applications in earthquake and geological disaster monitoring, early warning and forecasting, as well as in natural disaster emergency response and management.
View full society affiliation
- Recent Articles
- Most Downloaded Articles
- Most Cited Articles
Cultural Adaptation in the era of Climate Change: An Ethnographic Study on the Resilience of Indigenous People at Chittagong Hill Tracts area in Bangladesh
Joydeb Garai | Hok-Bun Ku
Assessment of gully erosion susceptibility using four data-driven models AHP, FR, RF and XGBoosting machine learning algorithms
Md Hasanuzzaman | Pravat Kumar Shit
The Aspects of Food Security and Subsistence Systems of Climate-Induced Hazards Affected Coastal People in the Era of the COVID-19 Pandemic in Bangladesh
Joydeb Garai
View all ScienceDirect
1. A review of socio-economic vulnerability: The emergence of its theoretical concepts, models and methodologies
Sneha Biswas | Sunil Nautiyal
2. Environmental and socioeconomic impacts of forest fires: A call for multilateral cooperation and management interventions
Chandra Prakash Kala
3. Floods in Pakistan: A state-of-the-art review
Hassam Bin Waseem | Irfan Ahmad Rana
Earthquake-induced landslides susceptibility assessment: A review of the state-of-the-art
Xiaoyi Shao | Chong Xu
Flood susceptibility zonation using advanced ensemble machine learning models within Himalayan foreland basin
Supriya Ghosh | Soumik Saha | Biswajit Bera
Coulomb stress changes associated with the M7.3 Maduo earthquake and implications for seismic hazards
Yujiang Li | Luyuan Huang | Rui Ding | Shuxin Yang | Lei Liu | Shimin Zhang | Haoqing Liu
View all Scopus
First Papers from Natural Hazards Research Are Now Online!
Submit to the new open access journal natural hazards research, call for papers, research progress on prehistoric/historical giant hazards and their social effects in china, special issue on experimental and numerical simulation of civil infrastructure’s behaviour under natural hazards, special issue on recent progress on geological hazards in china's loess plateau and yellow river basin, related journals.

China Geology
ISSN: 2096-5192

Earthquake Research Advances
ISSN: 2772-4670

Water Cycle
ISSN: 2666-4453

Geodesy and Geodynamics
ISSN: 1674-9847
Stay Informed
Register your interest and receive email alerts tailored to your needs. Sign up below.
- Stay up to date KeAi About us
Cookie notice
We use cookies to analyse and improve our service, to improve and personalise content, advertising and your digital experience. We also share information about your use of our site with our social media, advertising and analytics partners.
Your cookie preferences
We use cookies and similar technologies on our website. Cookies are text files containing small amounts of information, which your computer or mobile device downloads when you visit a website. When you return to websites, or visit websites that use the same cookies, they recognise these cookies and therefore your browsing device.
We use different types of cookies for different things, such as:
- Analysing how you use our website
- Giving you a better, more personalised experience
- Recognising when you’ve sign in
We split our cookies into three distinct categories. You can turn Functional and Performance cookies on and off right here. Strictly necessary cookies can’t be turned off.
Strictly necessary cookies
These cookies are necessary for the website to function and cannot be switched off in our systems. They are usually only set in response to actions made by you which amount to a request for services, such as setting your privacy preferences, logging in or filling in forms. You can set your browser to block or alert you about these cookies, but some parts of the site will not then work. These cookies do not store any personally identifiable information.
Performance cookies
These cookies allow us to count visits and traffic sources so we can measure and improve the performance of our site. They help us to know which pages are the most and least popular and see how visitors move around the site.
Functional cookies
These cookies enable the website to provide enhanced functionality and personalisation. They may be set by us or by third party providers whose services we have added to our pages. If you do not allow these cookies then some or all of these services may not function properly.
Targeting cookies
These cookies are set by a range of social media services that we have added to the site to enable you to share our content with your friends and networks. They are capable of tracking your browser across other sites and building up a profile of your interests. This may impact the content and messages you see on other websites you visit. If you do not allow these cookies you may not be able to use or see these sharing tools.
- Natural Hazards Center
- Vision and Mission
- Advisory Board
- How to Contribute
- In the News
- Center Staff
- Directors 1976-Present
- Mary Fran Myers Scholarship
- Disability and Disasters Award
- Student Paper Competition
- Mary Fran Myers Gender and Disaster Award
- Press/Contact Us
- Disaster Research - News You Can Use
- Current Issue
- Issue Index
- Research Counts
- Children and Disasters
- Mass Sheltering
- The Disaster Cycle
- Equity and Inclusion
- Research to Practice Publications
- Publications Index
- Research Briefs
- Community Engagement Briefs
- Director’s Corner
- Director’s Corner Index
- Quick Response Reports
- Mitigation Matters Reports
- Weather Ready Reports
- Public Health Reports
- Legacy Publications
- Natural Hazards Observer
- Natural Hazards Informer
- Monograph Series
- Working Papers
- Our Scholarship
- Books and Monographs
- Journal Articles
- Book Chapters
- Making Mitigation Work
- CONVERGE Training Modules
- Tribal Listening Sessions
- Indigenous Sovereignty and Emergency Response
- NSF Enabling Program
- 2024 Workshop
- 2024 Researchers Meeting
- 2024 Practitioners Meeting
- Save the Dates
- Workshop History
- Past Workshops
- Quick Response Research
- Special Call: Climate and Health
- Public Health Disaster Research
- Weather Ready Research
- Mitigation Matters Research
- Current Projects and Grants
- Completed Projects and Grants
- Dissertations
- General Interest
- Upcoming Conferences
- Webinars and Training
- Documentaries
- Resource Lists
- Publication Outlets
- Book Series
- Award Opportunities
- Research Centers
- Disaster Grads Listserv
For full functionality of this site it is necessary to enable JavaScript.
Here are the instructions how to enable JavaScript in your web browser .
Hazards and Disaster Journals
The Natural Hazards Center has compiled the following list of hazards and disaster, risk, and climate focused journals including the title, link to the journal, and five year impact factor .
*Impact factors measure the average number of yearly citations of articles published in each journal. This score is used to determine the relative importance of a journal within its field, with higher scores indicating a stronger impact. Impact factors were found using Journal Citation Reports . Journals with a "n/a" did not have an impact factor listed. This page was last updated on March 7, 2023.
Questions or Updates?
If you have questions about a listing, or a journal that you recommend be added to this list, please contact the Natural Hazards Center at [email protected] .
Review on the progress and future prospects of geological disasters prediction in the era of artificial intelligence
- Review Article
- Published: 24 May 2024
Cite this article
- Xiang Zhang ORCID: orcid.org/0000-0002-1017-742X 1 , 2 , 3 ,
- Minghui Zhang 1 , 2 , 3 ,
- Xin Liu 1 , 2 , 3 ,
- Berhanu Keno Terfa 4 ,
- Won-Ho Nam 5 ,
- Xihui Gu 6 , 7 , 8 ,
- Xu Zhang 1 , 2 , 3 ,
- Chao Wang 9 ,
- Jian Yang 10 ,
- Peng Wang 11 ,
- Chenghong Hu 12 ,
- Wenkui Wu 1 &
- Nengcheng Chen 1 , 2
70 Accesses
Explore all metrics
Geological disasters such as landslide, debris flow and collapse are major natural disasters faced by both China and the world, which seriously threaten people’s lives, property security and the socio-economic development. Although the method of using the paradigm of traditional mathematical statistics and physical model to predict the low-probability events of geological disasters have been developed for decades, the difficulty of accurate prediction still remains significant, which is recognized as a major and urgent scientific challenge in the field of Earth science. Artificial intelligence is an important driving force for a new round of scientific and technological revolution and industrial transformation. However, how to systematically establish the AI prediction paradigm for low-probability events of geological disasters and deeply coupled with the physical mechanisms of geological disaster evolution and AI learning models still remains as a scientific bottleneck at the intersection of Earth science and information science. In order to clarify the latest research progress of AI prediction of geological disasters such as landslide, collapse and debris flow, this paper first quantifies the current status of global geological disasters and the urgency of prediction, and then summarizes the overall methodology of AI prediction of geological disasters. In particular, prediction feature selection, data set collection and AI prediction models have been detailly reviewed. Moreover, this review discussed the approaches in establishing the physical-informed AI model for higher accurate, robust, and explainable prediction performance. Subsequently, this paper summarizes the recent research achievements of AI prediction for landslide, collapse, and debris flow. Based on these progresses, we also analyzed the existing problems in the field of AI prediction of geological disasters, and indicated the key directions of AI prediction of geological disasters in the future. This review work is believed to be a critical guidance for future intelligent prediction on the severe geological disasters.
This is a preview of subscription content, log in via an institution to check access.
Access this article
Price includes VAT (Russian Federation)
Instant access to the full article PDF.
Rent this article via DeepDyve
Institutional subscriptions
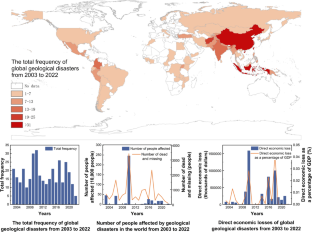
Similar content being viewed by others
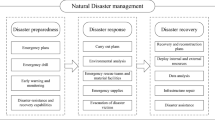
Can we detect trends in natural disaster management with artificial intelligence? A review of modeling practices
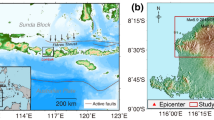
Using short-interval landslide inventories to build short-term and overall spatial prediction models for earthquake-triggered landslides based on machine learning for the 2018 Lombok earthquake sequence
Artificial Intelligence and Spatial Modelling in Natural Hazards and Environmental Applications
Abu El-Magd SA, Ali SA, Pham QB (2021) Spatial modeling and susceptibility zonation of landslides using random forest, naïve bayes and K-nearest neighbor in a complicated terrain. Earth Sci Inform 14(3):1227–1243
Article Google Scholar
Adnan MSG, Rahman MS, Ahmed N, Ahmed B, Rabbi MF, Rahman RM (2020) Improving spatial agreement in machine learning-based landslide susceptibility mapping. Remote Sens 12(20):3347
Akgun A (2012) A comparison of landslide susceptibility maps produced by logistic regression, multi-criteria decision, and likelihood ratio methods: a case study at İzmir, Turkey. Landslides 9(1):93–106
Antoine R, Lopez T, Tanguy M, Lissak C, Gailler L, Labazuy P, Fauchard C (2020) Geoscientists in the sky: unmanned aerial vehicles responding to geohazards. Surv Geophys 41(6):1285–1321
Auflič MJ, Herrera G, Mateos RM, Poyiadji E, Quental L, Severine B, Peternel T, Podolszki L, Calcaterra S, Kociu A, Warmuz B (2023) Landslide monitoring techniques in the Geological Surveys of Europe. Landslides 20(5):951–965
Ayawah PE, Sebbeh-Newton S, Azure JW, Kaba AG, Anani A, Bansah S, Zabidi H (2022) A review and case study of Artificial intelligence and Machine learning methods used for ground condition prediction ahead of tunnel boring Machines. Tunn Undergr Space Technol 125:104497
Baghbani A, Choudhury T, Costa S, Reiner J (2022) Application of artificial intelligence in geotechnical engineering: a state-of-the-art review. Earth Sci Rev 228:103991
Ballabio C, Sterlacchini S (2012) Support Vector machines for landslide susceptibility mapping: the Staffora River Basin case study, Italy. Math Geosci 44(1):47–70. https://doi.org/10.1007/s11004-011-9379-9
Banihabib ME, Jurik L, Kazemi MS, Soltani J, Tanhapour M (2020) A hybrid intelligence model for the prediction of the peak flow of debris floods. Water 12(8):2246
Belli G, Walter F, McArdell B, Gheri D, Marchetti E (2022) Infrasonic and seismic analysis of debris-flow events at Illgraben (Switzerland): relating signal features to flow parameters and to the seismo-acoustic source mechanism. J Geophys Res Earth Surf 127(6):e2021JF006576
Bergstra J, Yamins D, Cox D (2013) Making a science of model search: hyperparameter optimization in hundreds of dimensions for vision architectures. Paper presented at the International conference on machine learning
Bhagya SB, Sumi AS, Balaji S, Danumah JH, Costache R, Rajaneesh A, Gokul A, Chandrasenan CP, Quevedo RP, Johny A, Sajinkumar KS (2023) Landslide susceptibility assessment of a part of the Western Ghats (India) employing the AHP and F-AHP models and comparison with existing susceptibility maps. Land 12(2):468
Brocca L, Zhao W, Lu H (2023) High-resolution observations from space to address new applications in hydrology. Innovation (camb) 4(3):100437. https://doi.org/10.1016/j.xinn.2023.100437
Bui DT, Tsangaratos P, Nguyen V-T, Van Liem N, Trinh PT (2020a) Comparing the prediction performance of a Deep Learning Neural Network model with conventional machine learning models in landslide susceptibility assessment. CATENA 188:104426
Bui X-N, Nguyen H, Choi Y, Nguyen-Thoi T, Zhou J, Dou J (2020b) Prediction of slope failure in open-pit mines using a novel hybrid artificial intelligence model based on decision tree and evolution algorithm. Sci Rep 10(1):9939
Article CAS Google Scholar
Cao J, Zhang Z, Du J, Zhang L, Song Y, Sun G (2020) Multi-geohazards susceptibility mapping based on machine learning—a case study in Jiuzhaigou, China. Nat Hazards 102:851–871
Casagli N, Intrieri E, Tofani V, Gigli G, Raspini F (2023) Landslide detection, monitoring and prediction with remote-sensing techniques. Nat Rev Earth Environ 4(1):51–64
Catani F, Segoni S, Falorni G (2010) An empirical geomorphology‐based approach to the spatial prediction of soil thickness at catchment scale. Water Res Res 46(5)
Chang K-T, Merghadi A, Yunus AP, Pham BT, Dou J (2019) Evaluating scale effects of topographic variables in landslide susceptibility models using GIS-based machine learning techniques. Sci Rep 9(1):12296
Chang Z, Catani F, Huang F, Liu G, Meena SR, Huang J, Zhou C (2023) Landslide susceptibility prediction using slope unit-based machine learning models considering the heterogeneity of conditioning factors. J Rock Mech Geotech Eng 15(5):1127–1143
Chen C-Y (2020) Event-based rainfall warning regression model for landslide and debris flow issuing. Environ Earth Sci 79(6):127. https://doi.org/10.1007/s12665-020-8877-9
Chen W, Zhang S (2021) GIS-based comparative study of Bayes network, Hoeffding tree and logistic model tree for landslide susceptibility modeling. CATENA 203:105344
Chen W, Pourghasemi HR, Kornejady A, Zhang N (2017) Landslide spatial modeling: introducing new ensembles of ANN, MaxEnt, and SVM machine learning techniques. Geoderma 305:314–327. https://doi.org/10.1016/j.geoderma.2017.06.020
Chen H, Qin S, Xue L, Yang B, Zhang K (2018a) A physical model predicting instability of rock slopes with locked segments along a potential slip surface. Eng Geol 242:34–43
Chen W, Peng J, Hong H, Shahabi H, Pradhan B, Liu J, Zhu AX, Pei X, Duan Z (2018b) Landslide susceptibility modelling using GIS-based machine learning techniques for Chongren County, Jiangxi Province, China. Sci Total Environ 626:1121–1135
Chen W, Fan L, Li C, Pham BT (2019) Spatial prediction of landslides using hybrid integration of artificial intelligence algorithms with frequency ratio and index of entropy in Nanzheng County, China. Appl Sci 10(1):29
Chen W, Sun Z, Zhao X, Lei X, Shirzadi A, Shahabi H (2020a) Performance evaluation and comparison of bivariate statistical-based artificial intelligence algorithms for spatial prediction of landslides. ISPRS Int J Geo Inf 9(12):696
Chen Z, He C, Yang W, Guo W, Li Z, Xu G (2020b) Impacts of geological conditions on instability causes and mechanical behavior of large-scale tunnels: a case study from the Sichuan–Tibet highway, China. Bull Eng Geol Env 79:3667–3688
Chen W, Chen X, Peng J, Panahi M, Lee S (2021) Landslide susceptibility modeling based on ANFIS with teaching-learning-based optimization and Satin bowerbird optimizer. Geosci Front 12(1):93–107. https://doi.org/10.1016/j.gsf.2020.07.012
Chen J, Wang Z, Chen W, Wan C, Liu Y, Huang J (2023) The influence of the selection of non-geological disasters sample spatial range on the evaluation of environmental geological disasters susceptibility: a case study of Liulin County. Environ Sci Pollut Res 30(15):44756–44772
Chmiel M, Walter F, Wenner M, Zhang Z, McArdell BW, Hibert C (2021) Machine learning improves debris flow warning. Geophys Res Lett 48(3):e2020GL090874
Conforti M, Pascale S, Robustelli G, Sdao F (2014) Evaluation of prediction capability of the artificial neural networks for mapping landslide susceptibility in the Turbolo River catchment (northern Calabria, Italy). CATENA 113:236–250
Cui P, Ge Y, Li S, Li Z, Xu X, Zhou GG, Chen H, Wang H, Lei Y, Zhou L, Yi S (2022) Scientific challenges in disaster risk reduction for the Sichuan–Tibet Railway. Eng Geol 309:106837
Dai F, Lee CF, Ngai YY (2002) Landslide risk assessment and management: an overview. Eng Geol 64(1):65–87
Daviran M, Shamekhi M, Ghezelbash R, Maghsoudi A (2023) Landslide susceptibility prediction using artificial neural networks, SVMs and random forest: hyperparameters tuning by genetic optimization algorithm. Int J Environ Sci Technol 20(1):259–276
De Luca DL, Versace P (2017) A comprehensive framework for empirical modeling of landslides induced by rainfall: the Generalized FLaIR Model (GFM). Landslides 14:1009–1030
de Oliveira GG, Ruiz LFC, Guasselli LA, Haetinger C (2019) Random forest and artificial neural networks in landslide susceptibility modeling: a case study of the Fão River Basin, Southern Brazil. Nat Hazards 99(2):1049–1073
Demir G (2019) GIS-based landslide susceptibility mapping for a part of the North Anatolian Fault Zone between Reşadiye and Koyulhisar (Turkey). CATENA 183:104211
Depina I, Jain S, Mar Valsson S, Gotovac H (2022) Application of physics-informed neural networks to inverse problems in unsaturated groundwater flow. Georisk: Assess Manag Risk Eng Syst Geohazards 16(1):21–36
Google Scholar
Di Napoli M, Carotenuto F, Cevasco A, Confuorto P, Di Martire D, Firpo M, Pepe G, Raso E, Calcaterra D (2020) Machine learning ensemble modelling as a tool to improve landslide susceptibility mapping reliability. Landslides 17(8):1897–1914
Di B, Zhang H, Liu Y, Li J, Chen N, Stamatopoulos CA, Luo Y, Zhan Y (2019) Assessing susceptibility of debris flow in southwest China using gradient boosting machine. Sci Rep 9(1):1–12
Dikshit A, Pradhan B, Alamri AM (2021) Pathways and challenges of the application of artificial intelligence to geohazards modelling. Gondwana Res 100:290–301
Du H, Song D, Chen Z, Shu H, Guo Z (2020) Prediction model oriented for landslide displacement with step-like curve by applying ensemble empirical mode decomposition and the PSO-ELM method. J Clean Prod 270:122248
Fan W, Wei X-S, Cao Y-B, Zheng B (2017) Landslide susceptibility assessment using the certainty factor and analytic hierarchy process. J Mt Sci 14(5):906–925. https://doi.org/10.1007/s11629-016-4068-2
Fan X, Scaringi G, Korup O, West AJ, van Westen CJ, Tanyas H, Hovius N, Hales TC, Jibson RW, Allstadt KE, Zhang L (2019) Earthquake-induced chains of geologic hazards: patterns, mechanisms, and impacts. Rev Geophys 57(2):421–503
Fan X, Yang F, Siva Subramanian S, Xu Q, Feng Z, Mavrouli O, Peng M, Ouyang C, Jansen JD, Huang R (2020) Prediction of a multi-hazard chain by an integrated numerical simulation approach: the Baige landslide, Jinsha River, China. Landslides 17:147–164
Fathi MH, Khohdel K, Kandi AS, Ashrafifeini Z, Khaliji MA (2015) The combination of spectral and spatial data in zoning of landslide susceptibility (Case study: Sangorchay reservoir). J Biodivers Environ Sci (JBES) 6(2):515–527
Felicísimo ÁM, Cuartero A, Remondo J, Quirós E (2013) Mapping landslide susceptibility with logistic regression, multiple adaptive regression splines, classification and regression trees, and maximum entropy methods: a comparative study. Landslides 10:175–189
Froude MJ, Petley DN (2018) Global fatal landslide occurrence from 2004 to 2016. Nat Hazard 18(8):2161–2181. https://doi.org/10.5194/nhess-18-2161-2018
Ge Q, Sun Q, Zhang N, Hu J (2023) Evaluation of geological hazard susceptibility of Baiyin City based on MT-InSAR deformation measurements. Geomatics and Information Science of Wuhan University
Goetz J, Brenning A, Petschko H, Leopold P (2015) Evaluating machine learning and statistical prediction techniques for landslide susceptibility modeling. Comput Geosci 81:1–11
Gomez H, Kavzoglu T (2005) Assessment of shallow landslide susceptibility using artificial neural networks in Jabonosa River Basin, Venezuela. Eng Geol 78(1–2):11–27
Gong W, Luo Z, Juang CH, Huang H, Zhang J, Wang L (2014) Optimization of site exploration program for improved prediction of tunneling-induced ground settlement in clays. Comput Geotech 56:69–79
Gorsevski PV, Jankowski P, Gessler PE (2006) An heuristic approach for mapping landslide hazard by integrating fuzzy logic with analytic hierarchy process. Control Cybern 35(1):121–146
Grahn T, Jaldell H (2016) Assessment of data availability for the development of landslide fatality curves. Landslides 14(3):1113–1126. https://doi.org/10.1007/s10346-016-0775-6
Groisman PY, Legates DR (1994) The accuracy of United States precipitation data. Bull Am Meteor Soc 75(2):215–228
Guo C, Montgomery DR, Zhang Y, Wang K, Yang Z (2015) Quantitative assessment of landslide susceptibility along the Xianshuihe fault zone, Tibetan Plateau, China. Geomorphology 248:93–110
Guo Z, Chen L, Gui L, Du J, Yin K, Do HM (2020) Landslide displacement prediction based on variational mode decomposition and WA-GWO-BP model. Landslides 17:567–583
Guo C, Xu Q, Dong X, Liu X, She J (2021) Geohazard recognition by airborne LiDAR technology in complex mountain areas. 武汉大学学报● 信息科学版, 46(10):1538–1547
Guzzetti F, Mondini AC, Cardinali M, Fiorucci F, Santangelo M, Chang K-T (2012) Landslide inventory maps: new tools for an old problem. Earth Sci Rev 112(1–2):42–66. https://doi.org/10.1016/j.earscirev.2012.02.001
Han H, Shi B, Zhang L (2021) Prediction of landslide sharp increase displacement by SVM with considering hysteresis of groundwater change. Eng Geol 280:105876
Hansen A, Franks C, Kirk P, Brimicombe A, Tung F (1995) Application of GIS to hazard assessment, with particular reference to landslides in Hong Kong. Geogr Inform Syst Assess Nat Hazards 273–298
Harsa H, Anistia MH, Mulsandi A, Suprihadi B, Kurniawan R, Habibie MN, Hutapea TD, Swarinoto YS, Makmur EES, Fitria W, Sudewi RSS (2023) Machine learning and artificial intelligence models development in rainfall-induced landslide prediction. IAES Int J Artif Intell 12(1):262
He J, Qiu H, Qu F, Hu S, Yang D, Shen Y, Cao M (2021a) Prediction of spatiotemporal stability and rainfall threshold of shallow landslides using the TRIGRS and Scoops3D models. CATENA 197:104999
He L, Coggan J, Francioni M, Eyre M (2021b) Maximizing impacts of remote sensing surveys in slope stability—a novel method to incorporate discontinuities into machine learning landslide prediction. ISPRS Int J Geo Inf 10(4):232
Hewitt K, Clague JJ, Orwin JF (2008) Legacies of catastrophic rock slope failures in mountain landscapes. Earth Sci Rev 87(1–2):1–38
Hirschberg J, Badoux A, McArdell BW, Leonarduzzi E, Molnar P (2021) Evaluating methods for debris-flow prediction based on rainfall in an Alpine catchment. Nat Hazard 21(9):2773–2789
Ho J-Y, Lee KT, Chang T-C, Wang Z-Y, Liao Y-H (2012) Influences of spatial distribution of soil thickness on shallow landslide prediction. Eng Geol 124:38–46
Hong H, Liu J, Zhu A-X (2019a) Landslide susceptibility evaluating using artificial intelligence method in the Youfang district (China). Environ Earth Sci 78:1–20
Hong H, Miao Y, Liu J, Zhu A-X (2019b) Exploring the effects of the design and quantity of absence data on the performance of random forest-based landslide susceptibility mapping. CATENA 176:45–64
Hu J, Li Z, Ding X, Zhu J, Zhang L, Sun Q (2014) Resolving three-dimensional surface displacements from InSAR measurements: a review. Earth Sci Rev 133:1–17
Hu Q, Zhou Y, Wang S, Wang F (2020) Machine learning and fractal theory models for landslide susceptibility mapping: case study from the Jinsha River Basin. Geomorphology 351:106975. https://doi.org/10.1016/j.geomorph.2019.106975
Hu X, Wu S, Zhang G, Zheng W, Liu C, He C, Zhang H (2021) Landslide displacement prediction using kinematics-based random forests method: a case study in Jinping Reservoir Area, China. Eng Geol 283:105975
Huang Y, Zhao L (2018) Review on landslide susceptibility mapping using support vector machines. CATENA 165:520–529. https://doi.org/10.1016/j.catena.2018.03.003
Huang F, Huang J, Jiang S, Zhou C (2017) Landslide displacement prediction based on multivariate chaotic model and extreme learning machine. Eng Geol 218:173–186
Huang F, Cao Z, Guo J, Jiang S-H, Li S, Guo Z (2020) Comparisons of heuristic, general statistical and machine learning models for landslide susceptibility prediction and mapping. CATENA 191:104580
Huang W, Ding M, Li Z, Yu J, Ge D, Liu Q, Yang J (2023) Landslide susceptibility mapping and dynamic response along the Sichuan–Tibet transportation corridor using deep learning algorithms. CATENA 222:106866
Huffman GJ, Adler RF, Arkin P, Chang A, Ferraro R, Gruber A, Schneider U (1997) The global precipitation climatology project (GPCP) combined precipitation dataset. Bull Am Meteorol Soc 78(1):5–20
Hung W-C, Hwang C, Liou J-C, Lin Y-S, Yang H-L (2012) Modeling aquifer-system compaction and predicting land subsidence in central Taiwan. Eng Geol 147:78–90
Iadanza C, Trigila A, Napolitano F (2016) Identification and characterization of rainfall events responsible for triggering of debris flows and shallow landslides. J Hydrol 541:230–245
Intrieri E, Bardi F, Fanti R, Gigli G, Fidolini F, Casagli N, Capparelli G (2017) Big data managing in a landslide early warning system: experience from a ground-based interferometric radar application. Nat Hazards Earth Syst Sci 17(10):1713–1723
Javed S (2019) Landslide hazard mapping of Bagh district in Azad Kashmir. Int J Econ Environ Geol 8(3):47–50
Ji J, Zhou Y, Cheng Q, Jiang S, Liu S (2023) Landslide susceptibility mapping based on deep learning algorithms using information value analysis optimization. Land 12(6):1125
Jiang W, Rao P, Cao R, Tang Z, Chen K (2017) Comparative evaluation of geological disaster susceptibility using multi-regression methods and spatial accuracy validation. J Geog Sci 27:439–462
Jiang H, Li Y, Zhou C, Hong H, Glade T, Yin K (2020) Landslide displacement prediction combining LSTM and SVR algorithms: a case study of Shengjibao Landslide from the Three Gorges Reservoir Area. Appl Sci 10(21):7830
Jiang Z, Wang M, Liu K (2023) Comparisons of convolutional neural network and other machine learning methods in landslide susceptibility assessment: a case study in Pingwu. Remote Sens 15(3):798
Jiang Y, Wang W, Zou L, Cao Y (2024) Regional landslide susceptibility assessment based on improved semi-supervised clustering and deep learning. Acta Geotech 19(1):509–529
Jones DT, Thornton JM (2022) The impact of AlphaFold2 one year on. Nat Methods 19(1):15–20
Kanjanakul C, Chub-uppakarn T, Chalermyanont T (2016) Rainfall thresholds for landslide early warning system in Nakhon Si Thammarat. Arab J Geosci 9:1–11
Kargel JS, Leonard GJ, Shugar DH, Haritashya UK, Bevington A, Fielding EJ, Fujita K, Geertsema M, Miles ES, Steiner J, Anderson E, Bajracharya S, Bawden GW, Breashears DF, Byers A, Collins B, Dhital MR, Donnellan A, Evans TL, Geai ML, Glasscoe MT, Green D, Gurung DR, Heijenk R, Hilborn A, Hudnut K, Huyck C, Immerzeel WW, Jiang Liming R, Jibson A, Kääb NR, Khanal D, Kirschbaum PDA, Kraaijenbrink DL, Shiyin L, Lv Mingyang D, McKinney NK, Nahirnick NZ, Ojha S, Olsenholler J, Painter TH, Pleasants M, Pratima KC, Yuan QI, Raup BH, Regmi D, Rounce DR, Sakai A, Shangguan Donghui JM, Shea AB, Shrestha A, Shukla D, van der Stumm M, Kooij KV, Wang Xin B, Weihs DW, Lizong Wu, Yao Xiaojun MR, Yoder NY (2016) Geomorphic and geologic controls of geohazards induced by Nepal’s 2015 Gorkha earthquake. Science 351(6269):aac8353
Kavzoglu T, Sahin EK, Colkesen I (2013) Landslide susceptibility mapping using GIS-based multi-criteria decision analysis, support vector machines, and logistic regression. Landslides 11(3):425–439. https://doi.org/10.1007/s10346-013-0391-7
Kavzoglu T, Colkesen I, Sahin EK (2019) Machine learning techniques in landslide susceptibility mapping: a survey and a case study. Landslides Theory Pr Modell 50:283–301
Kawabata D, Bandibas J (2009) Landslide susceptibility mapping using geological data, a DEM from ASTER images and an Artificial Neural Network (ANN). Geomorphology 113(1–2):97–109
Kern AN, Addison P, Oommen T, Salazar SE, Coffman RA (2017) Machine learning based predictive modeling of debris flow probability following wildfire in the intermountain Western United States. Math Geosci 49:717–735
Kirschbaum D, Stanley T, Zhou Y (2015) Spatial and temporal analysis of a global landslide catalog. Geomorphology 249:4–15. https://doi.org/10.1016/j.geomorph.2015.03.016
Kornejady A, Ownegh M, Bahremand A (2017) Landslide susceptibility assessment using maximum entropy model with two different data sampling methods. CATENA 152:144–162
Korup O, Stolle A (2014) Landslide prediction from machine learning. Geol Today 30(1):26–33
Lan H, Peng J, Zhu Y, Li L, Pan B, Huang Q, Li J, Zhang Q (2022) Research on geological and surfacial processes and major disaster effects in the Yellow River Basin. Sci China Earth Sci 65(2):234–256
Le Breton M, Bontemps N, Guillemot A, Baillet L, Larose É (2021) Landslide monitoring using seismic ambient noise correlation: challenges and applications. Earth Sci Rev 216:103518. https://doi.org/10.1016/j.earscirev.2021.103518
Lee KT, Ho J-Y (2009) Prediction of landslide occurrence based on slope-instability analysis and hydrological model simulation. J Hydrol 375(3–4):489–497
Lee M-J, Park I, Lee S (2015) Forecasting and validation of landslide susceptibility using an integration of frequency ratio and neuro-fuzzy models: a case study of Seorak mountain area in Korea. Environ Earth Sci 74(1):413–429. https://doi.org/10.1007/s12665-015-4048-9
Lee D-H, Cheon E, Lim H-H, Choi S-K, Kim Y-T, Lee S-R (2021) An artificial neural network model to predict debris-flow volumes caused by extreme rainfall in the central region of South Korea. Eng Geol 281:105979
Lei X, Chen W, Pham BT (2020) Performance evaluation of GIS-based artificial intelligence approaches for landslide susceptibility modeling and spatial patterns analysis. ISPRS Int J Geo Inf 9(7):443
Li L, Lan H (2020) Integration of spatial probability and size in slope-unit-based landslide susceptibility assessment: a case study. Int J Environ Res Public Health 17(21):8055. https://doi.org/10.3390/ijerph17218055
Li H, Zhao J (2018) Evaluation of the newly released worldwide AW3D30 DEM over typical landforms of China using two global DEMs and ICESat/GLAS data. IEEE J Sel Top Appl Earth Obs Remote Sens 11(11):4430–4440
Li C, Tang H, Hu X, Li D, Hu B (2009) Landslide prediction based on wavelet analysis and cusp catastrophe. J Earth Sci 20(6):971
Li C, Tang H, Ge Y, Hu X, Wang L (2014) Application of back-propagation neural network on bank destruction forecasting for accumulative landslides in the three Gorges Reservoir Region, China. Stoch Env Res Risk Assess 28:1465–1477
Li X, Zhang LM, Li J (2016) Using conditioned random field to characterize the variability of geologic profiles. J Geotech Geoenviron Eng 142(4):04015096
Li H, Xu Q, He Y, Deng J (2018) Prediction of landslide displacement with an ensemble-based extreme learning machine and copula models. Landslides 15:2047–2059
Li Y, Sun R, Yin K, Xu Y, Chai B, Xiao L (2019) Forecasting of landslide displacements using a chaos theory based wavelet analysis-Volterra filter model. Sci Rep 9(1):19853. https://doi.org/10.1038/s41598-019-56405-y
Li H, Xu Q, He Y, Fan X, Li S (2020) Modeling and predicting reservoir landslide displacement with deep belief network and EWMA control charts: a case study in Three Gorges Reservoir. Landslides 17(3):693–707
Lian C, Zeng Z, Yao W, Tang H (2014) Extreme learning machine for the displacement prediction of landslide under rainfall and reservoir level. Stoch Env Res Risk Assess 28:1957–1972
Liao K, Zhang W, Zhu H-H, Zhang Q, Shi B, Wang J-T, Xu W-T (2022) Forecasting reservoir-induced landslide deformation using genetic algorithm enhanced multivariate Taylor series Kalman filter. Bull Eng Geol Env 81(3):104
Lin L, Lin Q, Wang Y (2017) Landslide susceptibility mapping on a global scale using the method of logistic regression. Nat Hazard 17(8):1411–1424
Lin Z, Sun X, Ji Y (2022) Landslide displacement prediction based on time series analysis and Double-BiLSTM Model. Int J Environ Res Public Health 19(4):2077
Ling Q, Qu W, Zhang Q, Kong L, Zhang J, Zhu L (2020) Improved Kalman filter method considering multiple factors and its application in landslide prediction. Front Earth Sci 14(3):625–636. https://doi.org/10.1007/s11707-019-0796-2
Ling Q, Zhang Q, Zhang J, Kong L, Zhang W, Zhu L (2021) Prediction of landslide displacement using multi-kernel extreme learning machine and maximum information coefficient based on variational mode decomposition: a case study in Shaanxi, China. Nat Hazards 108(1):925–946. https://doi.org/10.1007/s11069-021-04713-w
Liu M, He Y, Wang J, Lee HP, Liang Y (2015) Hybrid intelligent algorithm and its application in geological hazard risk assessment. Neurocomputing 149:847–853
Liu Y, Xu C, Huang B, Ren X, Liu C, Hu B, Chen Z (2020) Landslide displacement prediction based on multi-source data fusion and sensitivity states. Eng Geol 271:105608
Liu Q, Jian W, Nie W (2021a) Rainstorm-induced landslides early warning system in mountainous cities based on groundwater level change fast prediction. Sustain Cities Soc 69:102817
Liu R, Peng J, Leng Y, Lee S, Panahi M, Chen W, Zhao X (2021b) Hybrids of support vector regression with grey wolf optimizer and firefly algorithm for spatial prediction of landslide susceptibility. Remote Sens 13(24):4966. https://doi.org/10.3390/rs13244966
Liu W, Zhang Y, Liang Y, Sun P, Li Y, Su X, Wang A, Meng X (2022) Landslide risk assessment using a combined approach based on InSAR and random forest. Remote Sens 14(9):2131
Lu Y, Mei G (2022) A deep learning approach for predicting two-dimensional soil consolidation using physics-informed neural networks (PINN). Mathematics 10(16):2949
Luo X, Lin F, Chen Y, Zhu S, Xu Z, Huo Z, Yu M, Peng J (2019) Coupling logistic model tree and random subspace to predict the landslide susceptibility areas with considering the uncertainty of environmental features. Sci Rep 9(1):15369
Ma Z, Mei G (2021a) Deep learning for geological hazards analysis: data, models, applications, and opportunities. Earth Sci Rev 223:103858. https://doi.org/10.1016/j.earscirev.2021.103858
Ma Z, Mei G (2021b) Deep learning for geological hazards analysis: data, models, applications, and opportunities. Earth Sci Rev 223:103858
Ma J, Tang H, Liu X, Hu X, Sun M, Song Y (2017) Establishment of a deformation forecasting model for a step-like landslide based on decision tree C5.0 and two-step cluster algorithms: a case study in the Three Gorges Reservoir area, China. Landslides 14(3):1275–1281. https://doi.org/10.1007/s10346-017-0804-0
Ma Z, Mei G, Piccialli F (2021) Machine learning for landslides prevention: a survey. Neural Comput Appl 33:10881–10907
Merghadi A, Yunus AP, Dou J, Whiteley J, ThaiPham B, Bui DT, Avtar R, Abderrahmane B (2020) Machine learning methods for landslide susceptibility studies: a comparative overview of algorithm performance. Earth Sci Rev 207:103225. https://doi.org/10.1016/j.earscirev.2020.103225
Mirus BB, Jones ES, Baum RL, Godt JW, Slaughter S, Crawford MM, Schmitt RG, Lindsey KO, McCoy KM, Lancaster J, Stanley T, Kirschbaum DB, Burns WJ (2020) Landslides across the USA: occurrence, susceptibility, and data limitations. Landslides 17:2271–2285
Moayedi H, Mehrabi M, Mosallanezhad M, Rashid ASA, Pradhan B (2019) Modification of landslide susceptibility mapping using optimized PSO-ANN technique. Eng Comput 35:967–984
Mukherjee A, Sarkar S, Chakraborty M, Duttagupta S, Bhattacharya A, Saha D, Bhattacharya P, Mitra A, Gupta S (2021) Occurrence, predictors and hazards of elevated groundwater arsenic across India through field observations and regional-scale AI-based modeling. Sci Total Environ 759:143511
Murillo-García FG, Steger S, Alcántara-Ayala I (2019) Landslide susceptibility: a statistically-based assessment on a depositional pyroclastic ramp. J Mt Sci 16(3):561–580
NachtergaeleF, Velthuizen HV, Verelst L, Batjes N, Dijkshoorn K, Engelen VV, Fischer G, Jones A, Petri M, Prieler S, Montanarela L (2010) The harmonized world soil database. Paper presented at the Proceedings of the 19th World Congress of soil science, soil solutions for a changing world, Brisbane, Australia, 1–6 Aug 2010
Nava L, Carraro E, Reyes-Carmona C, Puliero S, Bhuyan K, Rosi A, Monserrat O, Floris M, Meena SR, Galve JP, Catani F (2023) Landslide displacement forecasting using deep learning and monitoring data across selected sites. Landslides 20(10):2111–2129
Ngo PTT, Panahi M, Khosravi K, Ghorbanzadeh O, Kariminejad N, Cerda A, Lee S (2021) Evaluation of deep learning algorithms for national scale landslide susceptibility mapping of Iran. Geosci Front 12(2):505–519
Nichol A (2022) Dalle 2 pre-training mitigations.
Nicu IC (2017) Frequency ratio and GIS-based evaluation of landslide susceptibility applied to cultural heritage assessment. J Cult Herit 28:172–176
Nowicki Jessee M, Hamburger MW, Allstadt K, Wald DJ, Robeson SM, Tanyas H, Hearne M, Thompson EM (2018) A global empirical model for near-real-time assessment of seismically induced landslides. J Geophys Res Earth Surf 123(8):1835–1859
Oh C-H, Choo K-S, Go C-M, Choi J-R, Kim B-S (2021) Forecasting of debris flow using machine learning-based adjusted rainfall information and RAMMS model. Water 13(17):2360
Orland E, Roering JJ, Thomas MA, Mirus BB (2020) Deep learning as a tool to forecast hydrologic response for landslide-prone hillslopes. Geophys Res Lett 47(16):e2020GL088731
Osmanoğlu B, Sunar F, Wdowinski S, Cabral-Cano E (2016) Time series analysis of InSAR data: methods and trends. ISPRS J Photogramm Remote Sens 115:90–102
Panahi M, Gayen A, Pourghasemi HR, Rezaie F, Lee S (2020) Spatial prediction of landslide susceptibility using hybrid support vector regression (SVR) and the adaptive neuro-fuzzy inference system (ANFIS) with various metaheuristic algorithms. Sci Total Environ 741:139937
Peng L, Niu R, Huang B, Wu X, Zhao Y, Ye R (2014) Landslide susceptibility mapping based on rough set theory and support vector machines: a case of the Three Gorges area, China. Geomorphology 204:287–301
Pham BT, Prakash I, Singh SK, Shirzadi A, Shahabi H, Bui DT (2019) Landslide susceptibility modeling using Reduced Error Pruning Trees and different ensemble techniques: hybrid machine learning approaches. CATENA 175:203–218
Pham QB, Chandra Pal S, Chakrabortty R, Saha A, Janizadeh S, Ahmadi K, Khedher KM, Anh DT, Tiefenbacher JP, Bannari A (2022) Predicting landslide susceptibility based on decision tree machine learning models under climate and land use changes. Geocarto Int 37(25):7881–7907
Piciullo L, Calvello M, Cepeda JM (2018) Territorial early warning systems for rainfall-induced landslides. Earth Sci Rev 179:228–247. https://doi.org/10.1016/j.earscirev.2018.02.013
Pourghasemi HR, Moradi HR, Fatemi Aghda SM (2013) Landslide susceptibility mapping by binary logistic regression, analytical hierarchy process, and statistical index models and assessment of their performances. Nat Hazards 69(1):749–779. https://doi.org/10.1007/s11069-013-0728-5
Psomiadis E, Charizopoulos N, Efthimiou N, Soulis KX, Charalampopoulos I (2020a) Earth observation and GIS-based analysis for landslide susceptibility and risk assessment. ISPRS Int J Geo Inf 9(9):552
Psomiadis E, Papazachariou A, Soulis KX, Alexiou D-S, Charalampopoulos I (2020b) Landslide mapping and susceptibility assessment using geospatial analysis and earth observation data. Land 9(5):133
Qi T, Zhao Y, Meng X, Chen G, Dijkstra T (2021) Ai-based susceptibility analysis of shallow landslides induced by heavy rainfall in Tianshui, China. Remote Sens 13(9):1819
Qin S, Jiao JJ, Li Z (2006) Nonlinear evolutionary mechanisms of instability of plane-shear slope: catastrophe, bifurcation, chaos and physical prediction. Rock Mech Rock Eng 39:59–76
Qing F, Zhao Y, Meng X, Su X, Qi T, Yue D (2020) Application of machine learning to debris flow susceptibility mapping along the China–Pakistan Karakoram Highway. Remote Sens 12(18):2933
Qiu H, Cui P, Regmi AD, Hu S, Hao J (2019) Loess slide susceptibility assessment using frequency ratio model and artificial neural network. Q J Eng Geol Hydrogeol 52(1):38–45
Qiu C, Su L, Zou Q, Geng X (2022) A hybrid machine-learning model to map glacier-related debris flow susceptibility along Gyirong Zangbo watershed under the changing climate. Sci Total Environ 818:151752
Rahman MM, Szabó G (2021) Multi-objective urban land use optimization using spatial data: a systematic review. Sustain Cities Soc 74:103214
Rashid B, Iqbal J, Su L-J (2020) Landslide susceptibility analysis of Karakoram highway using analytical hierarchy process and scoops 3D. J Mt Sci 17(7):1596–1612
Ray RL, Jacobs JM, Cosh MH (2010) Landslide susceptibility mapping using downscaled AMSR-E soil moisture: a case study from Cleveland Corral, California. US Remote Sens Environ 114(11):2624–2636
Ray A, Kumar V, Kumar A, Rai R, Khandelwal M, Singh T (2020) Stability prediction of Himalayan residual soil slope using artificial neural network. Nat Hazards 103(3):3523–3540
Reichenbach P, Rossi M, Malamud BD, Mihir M, Guzzetti F (2018) A review of statistically-based landslide susceptibility models. Earth Sci Rev 180:60–91
Ruff M, Czurda K (2008) Landslide susceptibility analysis with a heuristic approach in the Eastern Alps (Vorarlberg, Austria). Geomorphology 94(3–4):314–324
Saad N, Bahari N, Talib N, Zaki NM, Rasam AA (2021) Landslide hazard analysis using Landsat-8 OLI and AHP technique in Tanjung Bungah, Penang. Paper presented at the IOP Conference Series: earth and environmental science
Saha A, Saha S (2022) Integrating the artificial intelligence and hybrid machine learning algorithms for improving the accuracy of spatial prediction of landslide hazards in Kurseong Himalayan Region. Artif Intell Geosci 3:14–27
Saito M (1965) Forecasting the time of occurrence of a slope failure. Paper presented at the Proceedings of 6th international conference soil mechanics and foundation eng
Segoni S, Pappafico G, Luti T, Catani F (2020) Landslide susceptibility assessment in complex geological settings: Sensitivity to geological information and insights on its parameterization. Landslides 17:2443–2453
Selamat SN, Majid NA, Taha MR, Osman A (2022) Landslide susceptibility model using artificial neural network (ANN) approach in Langat river basin, Selangor, Malaysia. Land 11(6):833
Sezer EA, Pradhan B, Gokceoglu C (2011) Manifestation of an adaptive neuro-fuzzy model on landslide susceptibility mapping: Klang valley, Malaysia. Expert Syst Appl 38(7):8208–8219
Sousa JJ, Liu G, Fan J, Perski Z, Steger S, Bai S, Wei L, Salvi S, Wang Q, Tu J, Tong L (2021) Geohazards monitoring and assessment using multi-source earth observation techniques. Remote Sens 13(21):4269
SS VC, Shaji E (2022) Landslide identification using machine learning techniques: review, motivation, and future prospects. Earth Sci Inform 1–28
Stanley T, Kirschbaum DB (2017) A heuristic approach to global landslide susceptibility mapping. Nat Hazards 87:145–164
Sun X, Chen J, Han X, Bao Y, Zhou X, Peng W (2020) Landslide susceptibility mapping along the upper Jinsha River, south-western China: a comparison of hydrological and curvature watershed methods for slope unit classification. Bull Eng Geol Env 79:4657–4670
Sun X, Zeng P, Li T, Wang S, Jimenez R, Feng X, Xu Q (2021) From probabilistic back analyses to probabilistic run-out predictions of landslides: a case study of Heifangtai terrace, Gansu Province, China. Eng Geol 280:105950. https://doi.org/10.1016/j.enggeo.2020.105950
Takahashi T (2009) A review of Japanese debris flow research. Int J Eros Control Eng 2(1):1–14
Tang Y, Feng F, Guo Z, Feng W, Li Z, Wang J, Sun Q, Ma H, Li Y (2020) Integrating principal component analysis with statistically-based models for analysis of causal factors and landslide susceptibility mapping: a comparative study from the loess plateau area in Shanxi (China). J Clean Prod 277:124159
Tehrani FS, Calvello M, Liu Z, Zhang L, Lacasse S (2022) Machine learning and landslide studies: recent advances and applications. Nat Hazards 114(2):1197–1245
Thorp HH (2023) ChatGPT is fun, but not an author. Science 379(6630):313. https://doi.org/10.1126/science.adg7879
Tien Bui D, Nguyen QP, Hoang N-D, Klempe H (2017) A novel fuzzy K-nearest neighbor inference model with differential evolution for spatial prediction of rainfall-induced shallow landslides in a tropical hilly area using GIS. Landslides 14:1–17
Tien Bui D, Shahabi H, Omidvar E, Shirzadi A, Geertsema M, Clague J, Khosravi K, Pradhan B, Pham BT, Chapi K, Barati Z, Lee S (2019) Shallow landslide prediction using a novel hybrid functional machine learning algorithm. Remote Sens 11(8):931. https://doi.org/10.3390/rs11080931
Tomás R, Li Z (2017) Earth observations for geohazards: present and future challenges. Remote Sens 9:194
Ullah I, Aslam B, Shah SHIA, Tariq A, Qin S, Majeed M, Havenith H-B (2022) An integrated approach of machine learning, remote sensing, and GIS data for the landslide susceptibility mapping. Land 11(8):1265
Vali A, Comai S, Matteucci M (2020) Deep learning for land use and land cover classification based on hyperspectral and multispectral earth observation data: a review. Remote Sens 12(15):2495
Vallet A, Varron D, Bertrand C, Fabbri O, Mudry J (2016) A multi-dimensional statistical rainfall threshold for deep landslides based on groundwater recharge and support vector machines. Nat Hazards 84:821–849
Van Dao D, Jaafari A, Bayat M, Mafi-Gholami D, Qi C, Moayedi H, Van Phong T, Ly HB, Le TT, Trinh PT, Luu C (2020) A spatially explicit deep learning neural network model for the prediction of landslide susceptibility. CATENA 188:104451
Van Westen CJ, Castellanos E, Kuriakose SL (2008) Spatial data for landslide susceptibility, hazard, and vulnerability assessment: an overview. Eng Geol 102(3–4):112–131
Vaze J, Teng J, Spencer G (2010) Impact of DEM accuracy and resolution on topographic indices. Environ Model Softw 25(10):1086–1098
Wang Q, Wang D, Huang Y, Wang Z, Zhang L, Guo Q, Chen W, Chen W, Sang M (2015) Landslide susceptibility mapping based on selected optimal combination of landslide predisposing factors in a large catchment. Sustainability 7(12):16653–16669
Wang F-Y, Zhang JJ, Zheng X, Wang X, Yuan Y, Dai X, Zhang J, Yang L (2016) Where does AlphaGo go: from church-turing thesis to AlphaGo thesis and beyond. IEEE/CAA J Autom Sin 3(2):113–120
Wang Y, Tang H, Wen T, Ma J (2019) A hybrid intelligent approach for constructing landslide displacement prediction intervals. Appl Soft Comput 81:105506
Wang X, Zhang C, Wang C, Liu G, Wang H (2021) GIS-based for prediction and prevention of environmental geological disaster susceptibility: From a perspective of sustainable development. Ecotoxicol Environ Saf 226:112881. https://doi.org/10.1016/j.ecoenv.2021.112881
Wang Y, Tang H, Huang J, Wen T, Ma J, Zhang J (2022) A comparative study of different machine learning methods for reservoir landslide displacement prediction. Eng Geol 298:106544. https://doi.org/10.1016/j.enggeo.2022.106544
Wang F, Harindintwali JD, Wei K, Shan Y, Mi Z, Costello MJ, Grunwald S, Feng Z, Wang F, Guo Y, Wu X, Tiedje JM (2023a) Climate change: Strategies for mitigation and adaptation. Innov Geosci 1(1):100015–100061. https://doi.org/10.59717/j.xinn-geo.2023.100015
Wang L, Zuo B, Le Y, Chen Y, Li J (2023b) Penetrating remote sensing: next-generation remote sensing for transparent earth. The Innovation 4(6):100519
Wang Z, Chen J, Chen W, Wan C, Liu Y, Huang J (2023) Susceptibility assessment of environmental geological disasters in Liulin County based on RF: from the perspective of positive and negative sample proportion. Environ Sci Pollut Res 30:122245–122261
Wen T, Tang H, Wang Y, Lin C, Xiong C (2017) Landslide displacement prediction using the GA-LSSVM model and time series analysis: a case study of Three Gorges Reservoir, China. Nat Hazard 17(12):2181–2198. https://doi.org/10.5194/nhess-17-2181-2017
Wickel B, Lehner B, Sindorf N (2007) HydroSHEDS: a global comprehensive hydrographic dataset. Paper presented at the AGU Fall Meeting Abstracts
Wu Y, Shao K, Piccialli F, Mei G (2022) Numerical modeling of the propagation process of landslide surge using physics-informed deep learning. Adv Model Simul Eng Sci 9(1):1–15
Xu S, Niu R (2018) Displacement prediction of Baijiabao landslide based on empirical mode decomposition and long short-term memory neural network in Three Gorges area, China. Comput Geosci 111:87–96
Xu K, Guo Q, Li Z, Xiao J, Qin Y, Chen D, Kong C (2015) Landslide susceptibility evaluation based on BPNN and GIS: a case of Guojiaba in the Three Gorges Reservoir area. Int J Geogr Inf Sci 29(7):1111–1124
Xue Y, Kong F, Li S, Zhang Q, Qiu D, Su M, Li Z (2021) China starts the world’s hardest “Sky-High Road” project: challenges and countermeasures for Sichuan–Tibet railway. Innovation (camb) 2(2):100105. https://doi.org/10.1016/j.xinn.2021.100105
Yalcin A (2008) GIS-based landslide susceptibility mapping using analytical hierarchy process and bivariate statistics in Ardesen (Turkey): comparisons of results and confirmations. CATENA 72(1):1–12
Yang B, Yin K, Lacasse S, Liu Z (2019) Time series analysis and long short-term memory neural network to predict landslide displacement. Landslides 16:677–694
Yang H, Wei F, Ma Z, Guo H, Su P, Zhang S (2020) Rainfall threshold for landslide activity in Dazhou, southwest China. Landslides 17:61–77
Yang C, Liu L-L, Huang F, Huang L, Wang X-M (2023) Machine learning-based landslide susceptibility assessment with optimized ratio of landslide to non-landslide samples. Gondwana Res 123:198–216
Yilmaz I (2010) Comparison of landslide susceptibility mapping methodologies for Koyulhisar, Turkey: conditional probability, logistic regression, artificial neural networks, and support vector machine. Environ Earth Sci 61:821–836
Yin J, Dong J, Hamm NA, Li Z, Wang J, Xing H, Fu P (2021) Integrating remote sensing and geospatial big data for urban land use mapping: a review. Int J Appl Earth Obs Geoinf 103:102514
Yonggang J, Chaoqi Z, Liping L, Dong W (2016) Marine geohazards: review and future perspective. Acta Geologica Sin Engl Ed 90(4):1455–1470
Zezere JL, Pereira S, Melo R, Oliveira SC, Garcia RAC (2017) Mapping landslide susceptibility using data-driven methods. Sci Total Environ 589:250–267. https://doi.org/10.1016/j.scitotenv.2017.02.188
Zhang J, Gurung DR, Liu R, Murthy MSR, Su F (2015) Abe Barek landslide and landslide susceptibility assessment in Badakhshan Province, Afghanistan. Landslides 12:597–609
Zhang P, Wu H-N, Chen R-P, Chan TH (2020) Hybrid meta-heuristic and machine learning algorithms for tunneling-induced settlement prediction: a comparative study. Tunn Undergr Space Technol 99:103383
Zhang J, Tang H, Tannant DD, Lin C, Xia D, Liu X, Zhang Y, Ma J (2021a) Combined forecasting model with CEEMD-LCSS reconstruction and the ABC-SVR method for landslide displacement prediction. J Clean Prod 293:126205
Zhang Y-G, Tang J, Liao R-P, Zhang M-F, Zhang Y, Wang X-M, Su Z-Y (2021b) Application of an enhanced BP neural network model with water cycle algorithm on landslide prediction. Stoch Env Res Risk Assess 35:1273–1291
Zhang W, Gu X, Tang L, Yin Y, Liu D, Zhang Y (2022a) Application of machine learning, deep learning and optimization algorithms in geoengineering and geoscience: comprehensive review and future challenge. Gondwana Res 109:1–17
Zhang Y, Tang J, Cheng Y, Huang L, Guo F, Yin X, Li N (2022b) Prediction of landslide displacement with dynamic features using intelligent approaches. Int J Min Sci Technol 32(3):539–549
Zhang S, Tan S, Zhou J, Sun Y, Ding D, Li J (2023a) Geological disaster susceptibility evaluation of a random-forest-weighted deterministic coefficient model. Sustainability 15(17):12691
Zhang Z, Liu M, Tan YJ, Walter F, He S, Chmiel M, Su J (2024) Landslide hazard cascades can trigger earthquakes. Nat Commun 15(1):2878
Zhang Z, Pan Q, Yang Z, Yang X (2023) Physics-informed deep learning method for predicting tunnelling-induced ground deformations. Acta Geotech 18:4957–4972
Zhao Y, Meng X, Qi T, Chen G, Li Y, Yue D, Qing F (2021) Modeling the spatial distribution of debris flows and analysis of the controlling factors: a machine learning approach. Remote Sens 13(23):4813
Zhao P, Masoumi Z, Kalantari M, Aflaki M, Mansourian A (2022a) A GIS-based landslide susceptibility mapping and variable importance analysis using artificial intelligent training-based methods. Remote Sens 14(1):211
Zhao Y, Meng X, Qi T, Li Y, Chen G, Yue D, Qing F (2022b) AI-based rainfall prediction model for debris flows. Eng Geol 296:106456. https://doi.org/10.1016/j.enggeo.2021.106456
Zhao Y, Meng X, Qi T, Chen G, Li Y, Yue D, Qing F (2023) Estimating the daily rainfall thresholds of regional debris flows in the Bailong River Basin, China. Bull Eng Geol Env 82(2):46
Zhou C, Yin K, Cao Y, Ahmed B, Fu X (2018a) A novel method for landslide displacement prediction by integrating advanced computational intelligence algorithms. Sci Rep 8(1):7287. https://doi.org/10.1038/s41598-018-25567-6
Zhou C, Yin K, Cao Y, Intrieri E, Ahmed B, Catani F (2018b) Displacement prediction of step-like landslide by applying a novel kernel extreme learning machine method. Landslides 15:2211–2225
Zhou X, Wen H, Zhang Y, Xu J, Zhang W (2021) Landslide susceptibility mapping using hybrid random forest with GeoDetector and RFE for factor optimization. Geosci Front 12(5):101211
Zhu X, Xu Q, Tang M, Nie W, Ma S, Xu Z (2017) Comparison of two optimized machine learning models for predicting displacement of rainfall-induced landslide: a case study in Sichuan Province, China. Eng Geol 218:213–222
Zhu C, Xu Y, Tao Z, Zhu H-H, Cao C, He M (2023) Overview of comprehensive risk assessment methods and hazards early warning system for geological hazards in the mountain area. Remote Sens 15:2239
Download references
This work was supported by grants from the National Natural Science Foundation of China (Grant U2340230), Open Fund of Hubei Luojia Laboratory (2201000059), Special Fund of Hubei Luojia Laboratory (220100034), Pre-research Project of SongShan Laboratory (YYYY062022001), Open Fund of State Key Laboratory of Remote Sensing Science (OFSLRSS202114), and KartoBit Research Network (KRN2301GK).
Author information
Authors and affiliations.
National Engineering Research Center of Geographic Information System, School of Geography and Information Engineering, China University of Geosciences, Wuhan, 430074, China
Xiang Zhang, Minghui Zhang, Xin Liu, Xu Zhang, Wenkui Wu & Nengcheng Chen
Hubei Luojia Laboratory, Wuhan, 430079, China
Xiang Zhang, Minghui Zhang, Xin Liu, Xu Zhang & Nengcheng Chen
SongShan Laboratory, Zhengzhou, 450046, China
Xiang Zhang, Minghui Zhang, Xin Liu & Xu Zhang
Institute of Geophysics, Space Science and Astronomy, Addis Ababa University, Addis Ababa, Ethiopia
Berhanu Keno Terfa
School of Social Safety and Systems Engineering, Institute of Agricultural Environmental Science, National Agricultural Water Research Center, Hankyong National University, Anseong, Republic of Korea
Department of Atmospheric Science, School of Environmental Studies, China University of Geosciences, Wuhan, 430074, China
Centre for Severe Weather and Climate and Hydro-geological Hazards, Wuhan, 430074, China
School of Geography and the Environment, University of Oxford, Oxford, OX1 3QY, UK
State Key Laboratory of Information Engineering in Surveying, Mapping, and Remote Sensing (LIESMARS), Wuhan University, Wuhan, 430079, China
School of Geospatial Information, Information Engineering University, Zhengzhou, 450052, China
GAEA Space Time Co., Ltd, Wuhan, 430079, China
AutoNavi Software Co., Ltd, Beijing, 100012, China
Chenghong Hu
You can also search for this author in PubMed Google Scholar
Corresponding author
Correspondence to Xiang Zhang .
Ethics declarations
Conflict of interest.
The authors declare that they have no conflict of interest.
Ethical approval
This work did not involve any live subjects (human or animal).
Additional information
Publisher's note.
Springer Nature remains neutral with regard to jurisdictional claims in published maps and institutional affiliations.
Rights and permissions
Springer Nature or its licensor (e.g. a society or other partner) holds exclusive rights to this article under a publishing agreement with the author(s) or other rightsholder(s); author self-archiving of the accepted manuscript version of this article is solely governed by the terms of such publishing agreement and applicable law.
Reprints and permissions
About this article
Zhang, X., Zhang, M., Liu, X. et al. Review on the progress and future prospects of geological disasters prediction in the era of artificial intelligence. Nat Hazards (2024). https://doi.org/10.1007/s11069-024-06673-3
Download citation
Received : 20 August 2023
Accepted : 07 May 2024
Published : 24 May 2024
DOI : https://doi.org/10.1007/s11069-024-06673-3
Share this article
Anyone you share the following link with will be able to read this content:
Sorry, a shareable link is not currently available for this article.
Provided by the Springer Nature SharedIt content-sharing initiative
- Artificial intelligent
- Find a journal
- Publish with us
- Track your research
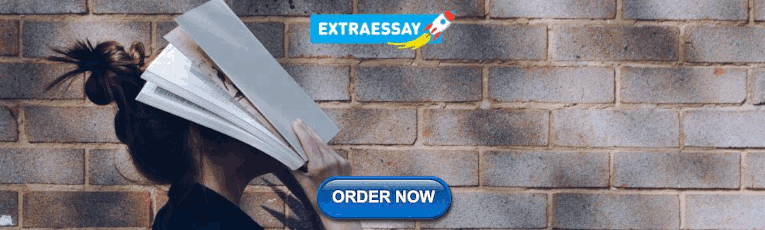
IMAGES
VIDEO
COMMENTS
Natural hazards can have catastrophic impacts. Natural Hazards Research promotes communication between the various fields that have an interest in natural disasters and catastrophic events, with the goal of raising awareness and improving detection and response. The journal covers multiple disciplines in natural and social sciences, emphasizing new theories, techniques, methods and case studies.
Overview. Natural Hazards is devoted to original research work on all aspects of natural hazards, including the forecasting of catastrophic events, risk management, and the nature of precursors of natural and technological hazards. Although hazards can originate in different sources and systems, such as atmospheric, hydrologic, oceanographic ...
The Natural Hazards Review addresses the range of events, processes, and consequences that occur when natural hazards interact with the physical, social, economic, and engineered dimensions of communities and the people who live, work, and play in them. As these conditions interact and change, the impact on human communities increases in size, scale, and scope. Such interactions necessarily ...
The classification of natural hazards discussed in this paper follows a hierarchical structure, drawn from various sources such as INSPIRE (2012), Integrated Research on Disaster Risk (IRDR, 2014), and the United Nations General Assembly (UNGA, 2016).
1 Introduction. Natural hazards, that is, the physical events that cause severe damage or loss (IPCC, 2007), pose an increasing threat to health, safety, property, and critical infrastructure (Cutter & Emrich, 2005; Gall et al., 2011; White et al., 2001).In the United States alone, over 927 billion USD and 32,366 human lives were lost to natural disasters between 1960 and 2015 (CEMHS, 2019).
Aims & Scope. npj Natural Hazards is a fully open access, interdisciplinary journal that publishes high-quality research on the causes and effects of natural hazards, including earthquakes ...
Exploring EM-DAT for depicting spatiotemporal trends of drought and wildfires and their connections with anthropogenic pressure. Michael Nones. Hossein Hamidifar. Seyed Mohammad Bagher Shahabi-Haghighi. Research Letter 25 September 2023 Pages: 957 - 973. Volume 120, issue 1 articles listing for Natural Hazards.
Danilo Couto de Souza. Natália Machado Crespo. Sarah Sparrow. Original Paper Open access 03 May 2024. 1. 2. …. 172. Natural Hazards is devoted to original research work on all aspects of natural hazards, including the forecasting of catastrophic events, risk management, and ...
1 Introduction 1.1 Natural Hazards in a Changing World. Global data bases on natural hazards show an increasing trend in the number of reported events, the number of affected people, and the amount of economic losses in past decades (CRED & UNISDR, 2020).For instance, the direct economic losses as a consequence of natural hazard events have almost doubled in the past four decades, from 1.63 ...
A large proportion of missing data was identified for disasters attributed to natural hazards occurring between 1990 and 2020, particularly on the economic losses. ... IFPRI Discussion Paper 806 ...
Abstract. Since 1990, natural hazards have led to over 1.6 million fatalities globally, and economic losses are estimated at an average of around USD 260-310 billion per year. The scientific and policy communities recognise the need to reduce these risks. As a result, the last decade has seen a rapid development of global models for assessing risk from natural hazards at the global scale.
Since early 2018 the "Natural Hazards" Section of Geosciences journal has aimed to publish pure, experimental, or applied research that is focused on advancing methodologies, technologies, expertise, and capabilities to detect, characterize, monitor, and model natural hazards and assess their associated risks. This stream of geoscientific research has reached a high degree of ...
Natural hazards and disaster reconnaissance investigations have provided many lessons for the research and practice communities and have greatly improved our scientific understanding of extreme events. Yet, many challenges remain for these communities, including improving our ability to model hazards, make decisions in the face of uncertainty, enhance community resilience, and mitigate risk.
The study has concluded that natural hazards (73%) are more predominant in research than man-made hazards (14%). Of the man-made hazards covered, terrorism is the most prevalent (83%).
Natural hazards can have catastrophic impacts. Natural Hazards Research promotes communication between the various fields that have an interest in natural disasters and catastrophic events, with the goal of raising awareness and improving detection and response.. The journal covers multiple disciplines in natural and social sciences, emphasizing new theories, techniques, methods and case studies.
1.2 The Disaster System. In order to deepen understanding of the relationship between hazards and the formation of disasters, the concept of disaster system (DS) has been proposed (Shi 1991).The DS is regarded as the object of disaster risk science research, and has both structure and function (Fig. 2).The structure presents the elements of a DS.
Natural Hazards: 3.102 Natural Hazards and Earth System Sciences: 4.345 Natural Hazards Research: n/a Natural Hazards Review: 3.169 Nature Climate Change: 25.29 npj Natural Hazards: n/a Population and Environment: 3.537 Pracademic Affairs: n/a Prehospital and Disaster Medicine: 2.04 Progress in Disaster Science: n/a
Italy. 1. Introduction. A natural hazard is a geophysical, atmospheric or hydrological event (e.g., earthquake, landslide, tsunami, windstorm, flood or drough t) that has the potential to cause ...
Generic natural hazards, in this context, are those papers where a specific hazard type is not allocated and instead reference is only made to natural disasters in general. For the specific hazard types identified, the most common research hazards are hydrological (20%) and geophysical (20%), followed by meteorological (15%).
In this paper, the state of the art in research on natural hazards is summarized from seven aspects: formation process, mechanism and dynamic of natural hazards, disaster risk assessment, forecast, monitoring and early warning, disaster mitigation, emergency treatment and rescue, risk management and post-disaster reconstruction.
Natural Hazards Research. Volume 2, Issue 3, September 2022, Pages 172-182. ... Meanwhile, we used CiteSpace to visually analyze papers in the Web of Science database with the theme word "landslide susceptibility" from 2008 to 2020. Each paper is summarized in terms of the evaluation scale. Finally, the research difficulties of landslide ...
To assess the economic impact of chronic exposure to natural disasters, it is essential to evaluate the severity and frequency of catastrophic events and align them with the at-risk assets. This assessment holds critical importance as countries must address NatCat risks through policy measures and pose a major challenge to the capacity of the ...
With the increasing occurrence of disasters, how to respond to disasters has attracted a lot of interest. However, a systematic study of emergency response in disasters (ER) has been ignored. Based on the bibliometric analysis and visualization of 3678 journal articles (1970-2019) related to ER from the Web of Science, the current research situation in the field of ER has been studied. The ...
Geological disasters such as landslide, debris flow and collapse are major natural disasters faced by both China and the world, which seriously threaten people's lives, property security and the socio-economic development. Although the method of using the paradigm of traditional mathematical statistics and physical model to predict the low-probability events of geological disasters have been ...