Call our 24 hours, seven days a week helpline at 800.272.3900

- Professionals
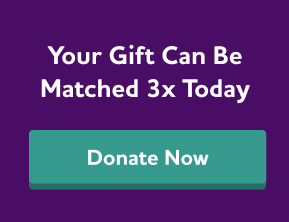
- Younger/Early-Onset Alzheimer's
- Is Alzheimer's Genetic?
- Women and Alzheimer's
- Creutzfeldt-Jakob Disease
- Dementia with Lewy Bodies
- Down Syndrome & Alzheimer's
- Frontotemporal Dementia
- Huntington's Disease
- Mixed Dementia
- Normal Pressure Hydrocephalus
- Posterior Cortical Atrophy
- Parkinson's Disease Dementia
- Vascular Dementia
- Korsakoff Syndrome
- Traumatic Brain Injury (TBI)
- Know the 10 Signs
- Difference Between Alzheimer's & Dementia
- 10 Steps to Approach Memory Concerns in Others
- Medical Tests for Diagnosing Alzheimer's
- Why Get Checked?
- Visiting Your Doctor
- Life After Diagnosis
- Stages of Alzheimer's
- Earlier Diagnosis
- Part the Cloud
- Research Momentum
- Our Commitment to Research
- TrialMatch: Find a Clinical Trial
- What Are Clinical Trials?
- How Clinical Trials Work
- When Clinical Trials End
- Why Participate?
- Talk to Your Doctor
- Clinical Trials: Myths vs. Facts
- Can Alzheimer's Disease Be Prevented?
- Brain Donation
- Navigating Treatment Options
- Lecanemab Approved for Treatment of Early Alzheimer's Disease
- Aducanumab Discontinued as Alzheimer's Treatment
- Medicare Treatment Coverage
- Donanemab for Treatment of Early Alzheimer's Disease — News Pending FDA Review
- Questions for Your Doctor
- Medications for Memory, Cognition and Dementia-Related Behaviors
- Treatments for Behavior
- Treatments for Sleep Changes
- Alternative Treatments
- Facts and Figures
- Assessing Symptoms and Seeking Help
- Now is the Best Time to Talk about Alzheimer's Together
- Get Educated
- Just Diagnosed
- Sharing Your Diagnosis
- Changes in Relationships
- If You Live Alone
- Treatments and Research
- Legal Planning
- Financial Planning
- Building a Care Team
- End-of-Life Planning
- Programs and Support
- Overcoming Stigma
- Younger-Onset Alzheimer's
- Taking Care of Yourself
- Reducing Stress
- Tips for Daily Life
- Helping Family and Friends
- Leaving Your Legacy
- Live Well Online Resources
- Make a Difference
- Daily Care Plan
- Communication and Alzheimer's
- Food and Eating
- Art and Music
- Incontinence
- Dressing and Grooming
- Dental Care
- Working With the Doctor
- Medication Safety
- Accepting the Diagnosis
- Early-Stage Caregiving
- Middle-Stage Caregiving
- Late-Stage Caregiving
- Aggression and Anger
- Anxiety and Agitation
- Hallucinations
- Memory Loss and Confusion
- Sleep Issues and Sundowning
- Suspicions and Delusions
- In-Home Care
- Adult Day Centers
- Long-Term Care
- Respite Care
- Hospice Care
- Choosing Care Providers
- Finding a Memory Care-Certified Nursing Home or Assisted Living Community
- Changing Care Providers
- Working with Care Providers
- Creating Your Care Team
- Long-Distance Caregiving
- Community Resource Finder
- Be a Healthy Caregiver
- Caregiver Stress
- Caregiver Stress Check
- Caregiver Depression
- Changes to Your Relationship
- Grief and Loss as Alzheimer's Progresses
- Home Safety
- Dementia and Driving
- Technology 101
- Preparing for Emergencies
- Managing Money Online Program
- Planning for Care Costs
- Paying for Care
- Health Care Appeals for People with Alzheimer's and Other Dementias
- Social Security Disability
- Medicare Part D Benefits
- Tax Deductions and Credits
- Planning Ahead for Legal Matters
- Legal Documents
- ALZ Talks Virtual Events
- ALZNavigator™
- Veterans and Dementia
- The Knight Family Dementia Care Coordination Initiative
- Online Tools
- Asian Americans and Pacific Islanders and Alzheimer's
- Native Americans and Alzheimer's
- Black Americans and Alzheimer's
- Hispanic Americans and Alzheimer's
- LGBTQ+ Community Resources for Dementia
- Educational Programs and Dementia Care Resources
- Brain Facts
- 50 Activities
- For Parents and Teachers
- Resolving Family Conflicts
- Holiday Gift Guide for Caregivers and People Living with Dementia
- Trajectory Report
- Resource Lists
- Search Databases
- Publications
- Favorite Links
- 10 Healthy Habits for Your Brain
- Stay Physically Active
- Adopt a Healthy Diet
- Stay Mentally and Socially Active
- Online Community
- Support Groups
- Find Your Local Chapter
- Any Given Moment
- New IDEAS Study
- Bruce T. Lamb, Ph.D., Chair
- Christopher van Dyck, M.D.
- Cynthia Lemere, Ph.D.
- David Knopman, M.D.
- Lee A. Jennings, M.D. MSHS
- Karen Bell, M.D.
- Lea Grinberg, M.D., Ph.D.
- Malú Tansey, Ph.D.
- Mary Sano, Ph.D.
- Oscar Lopez, M.D.
- Suzanne Craft, Ph.D.
- RFI Amyloid PET Depletion Following Treatment
- About Our Grants
- Andrew Kiselica, Ph.D., ABPP-CN
- Arjun Masurkar, M.D., Ph.D.
- Benjamin Combs, Ph.D.
- Charles DeCarli, M.D.
- Damian Holsinger, Ph.D.
- David Soleimani-Meigooni, Ph.D.
- Donna M. Wilcock, Ph.D.
- Elizabeth Head, M.A, Ph.D.
- Fan Fan, M.D.
- Fayron Epps, Ph.D., R.N.
- Ganesh Babulal, Ph.D., OTD
- Hui Zheng, Ph.D.
- Jason D. Flatt, Ph.D., MPH
- Jennifer Manly, Ph.D.
- Joanna Jankowsky, Ph.D.
- Luis Medina, Ph.D.
- Marcello D’Amelio, Ph.D.
- Marcia N. Gordon, Ph.D.
- Margaret Pericak-Vance, Ph.D.
- María Llorens-Martín, Ph.D.
- Nancy Hodgson, Ph.D.
- Shana D. Stites, Psy.D., M.A., M.S.
- Walter Swardfager, Ph.D.
- ALZ WW-FNFP Grant
- Capacity Building in International Dementia Research Program
- ISTAART IGPCC
- Alzheimer’s Disease Strategic Fund: Endolysosomal Activity in Alzheimer’s (E2A) Grant Program
- Imaging Research in Alzheimer’s and Other Neurodegenerative Diseases
- Zenith Fellow Awards
- National Academy of Neuropsychology & Alzheimer’s Association Funding Opportunity
- Part the Cloud-Gates Partnership Grant Program: Bioenergetics and Inflammation
- Pilot Awards for Global Brain Health Leaders (Invitation Only)
- Robert W. Katzman, M.D., Clinical Research Training Scholarship
- Funded Studies
- How to Apply
- Portfolio Summaries
- Supporting Research in Health Disparities, Policy and Ethics in Alzheimer’s Disease and Dementia Research (HPE-ADRD)
- Diagnostic Criteria & Guidelines
- Annual Conference: AAIC
- Professional Society: ISTAART
- Alzheimer's & Dementia
- Alzheimer's & Dementia: DADM
- Alzheimer's & Dementia: TRCI
- International Network to Study SARS-CoV-2 Impact on Behavior and Cognition
- Alzheimer’s Association Business Consortium (AABC)
- Global Biomarker Standardization Consortium (GBSC)
- Global Alzheimer’s Association Interactive Network
- International Alzheimer's Disease Research Portfolio
- Alzheimer’s Disease Neuroimaging Initiative Private Partner Scientific Board (ADNI-PPSB)
- Research Roundtable
- About WW-ADNI
- North American ADNI
- European ADNI
- Australia ADNI
- Taiwan ADNI
- Argentina ADNI
- WW-ADNI Meetings
- Submit Study
- RFI Inclusive Language Guide
- Scientific Conferences
- AUC for Amyloid and Tau PET Imaging
- Make a Donation
- Walk to End Alzheimer's
- The Longest Day
- RivALZ to End ALZ
- Ride to End ALZ
- Tribute Pages
- Gift Options to Meet Your Goals
- Founders Society
- Fred Bernhardt
- Anjanette Kichline
- Lori A. Jacobson
- Pam and Bill Russell
- Gina Adelman
- Franz and Christa Boetsch
- Adrienne Edelstein
- For Professional Advisors
- Free Planning Guides
- Contact the Planned Giving Staff
- Workplace Giving
- Do Good to End ALZ
- Donate a Vehicle
- Donate Stock
- Donate Cryptocurrency
- Donate Gold & Sterling Silver
- Donor-Advised Funds
- Use of Funds
- Giving Societies
- Why We Advocate
- Ambassador Program
- About the Alzheimer’s Impact Movement
- Research Funding
- Improving Care
- Support for People Living With Dementia
- Public Policy Victories
- Planned Giving
- Community Educator
- Community Representative
- Support Group Facilitator or Mentor
- Faith Outreach Representative
- Early Stage Social Engagement Leaders
- Data Entry Volunteer
- Tech Support Volunteer
- Other Local Opportunities
- Visit the Program Volunteer Community to Learn More
- Become a Corporate Partner
- A Family Affair
- A Message from Elizabeth
- The Belin Family
- The Eliashar Family
- The Fremont Family
- The Freund Family
- Jeff and Randi Gillman
- Harold Matzner
- The Mendelson Family
- Patty and Arthur Newman
- The Ozer Family
- Salon Series
- No Shave November
- Other Philanthropic Activities
- Still Alice
- The Judy Fund E-blast Archive
- The Judy Fund in the News
- The Judy Fund Newsletter Archives
- Sigma Kappa Foundation
- Alpha Delta Kappa
- Parrot Heads in Paradise
- Tau Kappa Epsilon (TKE)
- Sigma Alpha Mu
- Alois Society Member Levels and Benefits
- Alois Society Member Resources
- Zenith Society
- Founder's Society
- Joel Berman
- JR and Emily Paterakis
- Legal Industry Leadership Council
- Accounting Industry Leadership Council
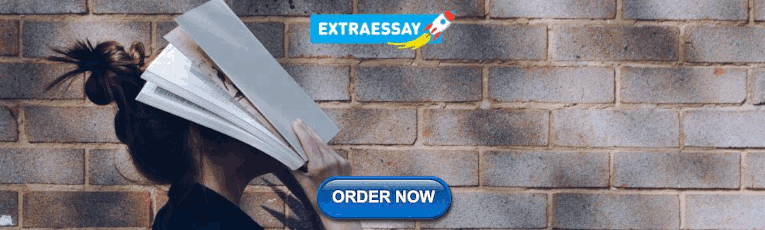
Find Local Resources
Let us connect you to professionals and support options near you. Please select an option below:
Use Current Location Use Map Selector
Search Alzheimer’s Association
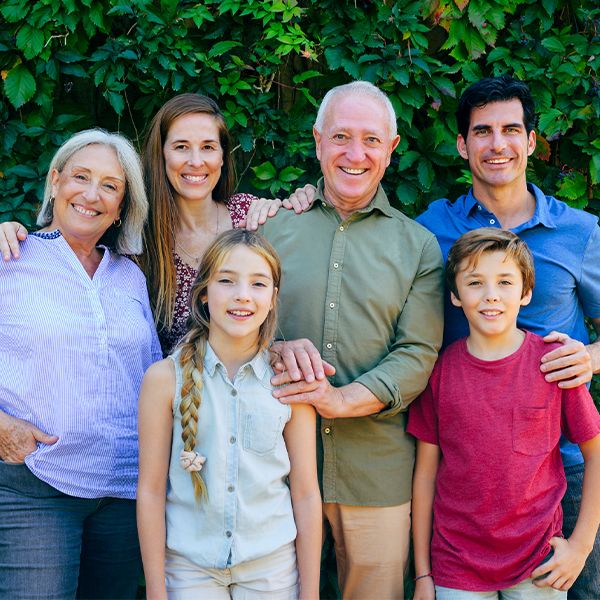
Make 3x the Impact to Help End Alzheimer’s
- Models of Care Case Studies
The Alzheimer’s Association is committed to connecting clinicians to effective, evidence-based models of care that can be replicated in community settings. Two of these models — the UCLA Alzheimer’s and Dementia Care program and the Age-Friendly Health Systems initiative — are detailed below.
UCLA Alzheimer's and Dementia Care program Age-Friendly Health Systems initiative UCLA Alzheimer’s and Dementia Care program
A dementia-specific model of care that significantly improved the experience for caregivers and people living with the disease.
About the program
The Alzheimer’s Association has partnered with UCLA to replicate the UCLA Alzheimer’s and Dementia Care (ADC) program through a grant from the John A. Hartford Foundation. The program follows a co-management model within the UCLA health system and partners with community-based organizations (CBOs) to provide comprehensive, coordinated, individualized care for people living with Alzheimer’s disease and other dementias.
The goals of the program are to:
- Maximize function, independence and dignity for people living with dementia.
- Minimize caregiver strain and burnout.
- Reduce unnecessary costs through improved care.
To qualify for the program, participants must have a diagnosis of dementia and live outside of a nursing home. The mean age of the first program participants was 82 years old. Almost all of the caregivers were the children (59%) or spouses (41%) of individuals living with Alzheimer’s or other dementias.
Comprehensive care
The ADC program utilizes a co-management model in which a nurse practitioner Dementia Care Specialist (DCS) partners with the participant’s primary care doctor to develop and implement a personalized care plan. The DCS provides support via four key components:
- Conducting in-person needs assessments of individuals living with Alzheimer’s and their caregivers.
- Creating and implementing individualized dementia care plans.
- Monitoring and revising care plans, as needed.
- Providing access 24/7, 365 days a year for assistance and advice to help avoid Emergency Department (ED) visits and hospitalizations.
Community resources
The ADC program also connects caregivers with resources provided by CBOs, including:
- Adult day care.
- Counseling.
- Case management.
- Legal and financial advice.
- Workforce development focused on training families and caregivers.
Program effectiveness
At one year, the quality of care provided by the program as measured by nationally accepted quality measures for dementia was exceedingly high — 92% compared to a benchmark of 38%. As a result, the improvements experienced by both caregivers and patients were significant:
- Ninety-four percent of caregivers felt that their role was supported.
- Ninety-two percent would recommend the program to others.
- Confidence in handling problems and complications of Alzheimer’s and other dementias improved by 79%.
- Caregiver distress related to behavioral symptoms, depression scores and strain improved by 31%, 24% and 15%, respectively.
- Despite disease progression, behavioral symptoms like agitation, irritability, apathy and nighttime behaviors in people living with dementia improved by 22%.
- Depressive symptoms experienced by individuals living with the disease were reduced by 34%.
Cost benefits of the program
An external evaluator compared utilization and cost outcomes and determined that over the course of 3 1/2 years, participants in UCLA’s program had lower total Medicare costs of care ($2,404 per year) relative to those receiving usual care.
In addition to cost savings for individuals and their families, the ADC program reports several financial benefits for health systems, including:
- Hospitalizations: 12% reduction
- ED visits: 20% reduction
- ICU stays: 21% reduction
- Hospital days: 26% reduction
- Hospice in last six months: 60% increase
- Nursing home placement: 40% reduction
UCLA finds that a care program following the ADC model may be able to pay for itself depending on local labor costs, comprehensiveness of billing and local overhead applied to clinical revenue.
To learn more or to contact UCLA about training and replication of the program, visit the UCLA Alzheimer’s and Dementia Care Program website.
Age-Friendly Health Systems initiative
A model of care that incorporates person-centered dementia care into a broader framework for the care of older adults.
About the initiative
Age-Friendly Health Systems is an initiative of The John A. Hartford Foundation and the Institute for Healthcare Improvement (IHI) in partnership with the American Hospital Association (AHA) and the Catholic Health Association of the United States (CHA). Together in 2017, they set a bold vision to build a social movement so all care with older adults is age-friendly care, that:
- Follows an essential set of evidence-based practices.
- Causes no harm.
- Aligns with “What Matters” to the older adult and their family caregivers.
The Age-Friendly Health Systems initiative defines “What Matters” as knowing and aligning care with each older adult’s specific health outcome goals and care preferences including, but not limited to, end-of-life care, and across settings of care.
- Health outcome goals relate to the values and activities that matter most to an individual, help motivate the individual to sustain and improve health, and could be impacted by a decline in health — for example, babysitting a grandchild, walking with friends in the morning, or volunteering in the community. When identified in a specific, actionable, and reliable manner, patients’ health outcome goals can guide decision making.
- Care preferences include the health care activities (e.g., medications, self-management tasks, health care visits, testing, and procedures) that patients are willing and able (or not willing or able) to do or receive.
The 4Ms framework of an Age-Friendly Health System
The 4Ms are not a program, but a framework to guide how care is provided to older adults through every interaction with a health system’s care and services. The 4Ms — What Matters, Medication, Mentation, and Mobility — make the complex care of older adults more manageable because they:
- Identify the core issues that should drive all care and decision making with the care of older adults.
- Organize care and focus on the older adult’s wellness and strengths rather than solely on disease.
- Are relevant regardless of an older adult’s individual disease(s).
- Apply regardless of the number of functional problems an older adult may have, or that person’s cultural, ethnic or religious background.
The 4Ms framework is most effective when all 4Ms are implemented together and are practiced reliably (i.e., for all older adults, in all settings and across settings, in every interaction).
The intention is to incorporate the 4Ms into existing care — rather than layering them on top —to organize the efficient delivery of effective care. This is achieved primarily through redeploying existing health system resources. Many health systems have found they already provide care aligned with one or more of the 4Ms for many of their older adult patients. Much of the effort, then, is to incorporate the other elements and organize care so all 4Ms guide every encounter with an older adult and their family caregivers.
Cost benefits of the initiative
The business case for becoming an Age-Friendly Health System focuses on its financial returns and is stronger when:
- The financial benefits are captured by the health system that is making the investment.
- Utilization and associated expenses of “usual” care are especially burdensome.
- The health system is effective in mitigating those costs.
- The added expense of becoming age-friendly is lower.
See the IHI report, The Business Case for Becoming an Age-Friendly Health System , for guidance on how to make the business case for your health system.
To learn more or to contact IHI about joining the initiative, visit the IHI Age-Friendly Health Systems website.
Health Systems and Medical Professionals Menu
- Alzheimer’s Association Innovation Roundtable (AAIR)
- Prescribing Lecanemab
- Billing Codes for Alzheimer’s and Related Dementia
- Cognitive Screening and Assessment
- Dementia Care Navigation Guiding Principles
- Dementia Care Navigation Roundtable
- Differential Diagnosis of Alzheimer's Disease
- Differential Diagnosis of Creutzfeldt-Jakob Disease
- Differential Diagnosis of Lewy Body Dementia
- Differential Diagnosis of Frontotemporal Dementia
- Differential Diagnosis of Huntington's Disease
- Differential Diagnosis of Korsakoff Syndrome
- Differential Diagnosis of Mixed Dementia
- Differential Diagnosis of Normal Pressure Hydrocephalus
- Differential Diagnosis of Parkinson's Disease Dementia
- Differential Diagnosis of Vascular Dementia
- Disclosure of Diagnosis
- Advanced Imaging and Biomarkers
- Dementia Care Delivery and Accreditation
- Care Planning Visit
- Advanced Care Planning
- Alzheimer's Network (ALZ-NET)
- Guidelines Index
- Clinical Trials Recruiting
- Downloadable Resources for Patients and Caregivers
- I Have Alzheimer's
- Clinical Trials
- Instructional Videos
- Cognitive Assessment Tools
- ISTAART Membership
- Alzheimer's & Dementia Journal
- Continuing Education on Alzheimer's and Dementia
- The Alzheimer’s and Dementia Care ECHO® Program for Health Systems and Medical Professionals
- Risk Reduction
Log in using your username and password
- Search More Search for this keyword Advanced search
- Latest content
- Global health
- BMJ Journals More You are viewing from: Google Indexer
You are here
- Volume 13, Issue 3
- Early-onset dementia: diagnostic challenges
- Article Text
- Article info
- Citation Tools
- Rapid Responses
- Article metrics

- Bushra Elhusein ,
- Omer Bakri Mahgoub and
- Abdalla Khairi
- Psychiatry, Department of Medical Education , Hamad Medical Corporation , Doha , Ad Dawhah , Qatar
- Correspondence to Dr Omer Bakri Mahgoub; Omeralshekh{at}hotmail.com
A 56-year-old man was brought to our hospital by his family, seeking medical treatment for the patient’s long-standing progressive word-finding difficulties, forgetfulness, agitation and social withdrawal. After multiple previous physician consultations, the patient was mistakenly diagnosed with epilepsy and prescribed multiple anticonvulsants, to which his above mentioned symptoms were unresponsive. His condition progressed over the next 10 years, resulting in severe cognitive impairments and a complete dysfunctionality. An electroencephalogram (EEG) assessment revealed persistent spike and wave activity in the left temporal lobe. Brain MRI revealed multiple small bright T2 and fluid attenuated inversion recovery (FLAIR) foci within the white matter of both cerebral hemispheres surrounding the ventricular system, as well as some widening of extra-axial cerebrospinal fluid spaces. The patient was finally diagnosed with early-onset dementia and temporal lobe epileptiform abnormalities. This case emphasises the need for diagnostic consideration of dementia in cognitively impaired patients, even when they are not of an advanced age.
- epilepsy and seizures
- memory disorders (psychiatry)
This is an open access article distributed in accordance with the Creative Commons Attribution Non Commercial (CC BY-NC 4.0) license, which permits others to distribute, remix, adapt, build upon this work non-commercially, and license their derivative works on different terms, provided the original work is properly cited and the use is non-commercial. See: http://creativecommons.org/licenses/by-nc/4.0/ .
https://doi.org/10.1136/bcr-2019-233460
Statistics from Altmetric.com
Request permissions.
If you wish to reuse any or all of this article please use the link below which will take you to the Copyright Clearance Center’s RightsLink service. You will be able to get a quick price and instant permission to reuse the content in many different ways.
According to the DSM-5 (Diagnostic and Statistical Manual of Mental Disorders, Fifth edition), dementia (major neurocognitive disorder) is defined as significant acquired cognitive impairment in one or more cognitive domains (eg, learning and memory, language, executive function, complex attention, perceptual-motor function, social cognition) that represents a significant decline from previous baseline and interferes with independence in daily activities. Early-onset dementia usually refers to cases of dementia in adults occurring before the age of 65 years.
Dementia in young individuals can be a diagnostic challenge given its often non-specific symptoms and findings. As a consequence, patients with early-onset dementia are more often misdiagnosed than those with late-onset dementia. 1 Although there is a set of known clinical criteria for early-onset dementia, misdiagnoses can occur even in tertiary facilities. 2 The present case exemplifies these challenges. The patient presented here first experienced symptoms in his early 40s and, given his young age and unspecific clinical findings, received a seizure disorder diagnosis. Given this misdiagnosis, his treatment was delayed, and his condition further deteriorated.
Case presentation
A 56-year-old man with no medical comorbidities who used to work as an electrician was initially brought by his family to our psychiatry hospital-walk in clinic seeking help for memory loss over the past 10 years. During this time, the patient’s family endorsed mood swings, social isolation, an unsteady gait, and word-finding difficulties.
Several months after symptom onset, the patient’s family noticed that his memory loss became more prominent and that he was unable to follow passed event timelines, for instance arguing that long-ago events had happened only days prior. The patient also forgot the names of close relatives, maintained poor sleep (less than 3 hours per night) and altered his normal daily routines (eg, religious rituals). The family also endorsed increased agitation and anxiety at this time. The patient’s functional skills began to gradually decline and he lost the skills required to do his job, which ultimately resulting in his being laid off. Furthermore, the patient’s family reported that he lost his way home on two occasions and was ultimately brought home after 1 day by strangers both times. Finally, the patient’s family reported one incident approximately 5 years prior to the current presentation, the incident happened during a family gathering when the patient lost consciousness for a few minutes. The patient was later taken to a neurologist in his home country.
An MRI and EEG were performed on the patient’s first being examined by a neurologist. The MRI revealed some brain atrophy and the EEG was normal. The patient was started taking carbamazepine but according to his family his condition continued to progress. Medications, including quetiapine, which was later added to the patient’s regimen to treat his sleep problems, were not efficacious.
A mental status examination revealed average grooming and hygiene, poor eye-to-eye contact, psychomotor retardation, a calm and quiet demeanour, irrelevant yet coherent responses to questions and blunted affect. The patient denied experiencing any hallucinations or other perceptual abnormalities and no overt delusions or obsessions were noted during his examination.
A cognitive assessment with the mini-mental state examination revealed a score of zero, as the patient was unable to complete any of the required tasks. While the patient registered three items correctly, he recalled none. He was unable to do the serial sevens or threes measures of attention and was unable to read or write.
The patient’s medical history indicated significant iron deficiency anaemia, which required hospitalisation at the age of 18 years. He had no recorded history of hypertension, diabetes mellitus, dyslipidaemia, stroke, head trauma or any other chronic illnesses. The patient was not on any regular medications before the treatment described above, though he was a smoker since the age of 18 years (quit 2 years prior to the current presentation) and denied any alcohol consumption or illicit drug use.
Finally, the patient’s family, childhood and developmental history were unremarkable. He was educated through secondary school but did not complete his education for financial reasons. He completed professional electrician training and worked as an electrician for more than 20 years. He is married with four children. A physical examination revealed no further abnormalities.
Investigations
A complete blood count and urea, electrolyte and liver function tests were all within normal limits. The patient had normal levels of vitamin B 12 and folate and was negative for HIV and venereal disease (as per the Venereal Disease Research Laboratory test). Inflammatory markers were within normal limits. The patient’s family refused a cerebrospinal spinal fluid analysis.
An EEG assessment revealed persistent left-sided spike and wave activity over the temporal lobe region with an amplitude of 50–80 μV, suggesting left temporal lobe epileptiform discharge.
A head MRI revealed multiple small and bright T2 and fluid attenuated inversion recovery (FLAIR) foci within the white matter of both cerebral hemispheres and surrounding the ventricular system, most notably in the frontal and occipital horns, with no corresponding areas of restricted diffusion on diffusion-weighted image sequences. This likely represented deep white matter ischaemic-related change.
Widening of the extra-axial CSF space, prominent sulci, and deepened gyri implicated both cerebral hemispheres and temporal lobes degenerative changes ( figure 1 ).
- Download figure
- Open in new tab
- Download powerpoint
MRI of head.
Differential diagnosis
The differential diagnoses in this case include Alzheimer’s disease, vascular dementia, Lewy body dementia, multiple system atrophy, Parkinson’s disease, Huntington’s disease and normal pressure hydrocephalus.
Initially, 10 years prior to his latest presentation to our hospital, the patient was diagnosed with a seizure disorder. Accordingly, he started taking carbamazepine (200 mg once daily) and then donepezil 5 years later (5 mg later increased to 10 mg per day with carbamazepine). He did not show any improvement in his symptoms and a marked exacerbation of his forgetfulness, decreased functionality and increased agitation. He was assessed in an outpatient setting at our psychiatric facility and diagnosed with early-onset dementia after an initial assessment. He was subsequently started taking memantine (10 mg two times per day) and quetiapine (50 mg before bed) for the symptomatic treatment of insomnia. The patient’s carbamazepine was discontinued given no evidence for clinical seizures.
Outcome and follow-up
One month after the initiation of his treatment, the patient's family reported improvement in his sleep quality and agitation, but not in his cognitive function. The patient’s family travelled with him back to his home country and he became unavailable for follow-up.
In the present case, we discuss a 56-year-old male patient who sought medical help abroad for progressive word-finding difficulties, forgetfulness, agitation and social withdrawal. His history indicated chronicity, as he endorsed these symptoms for a period of more than 10 years. In general medical practices, the suspicion index for dementia at a young age is low and such cases usually represent a diagnostic challenge given the low presence of key manifestations, unspecific symptoms and the younger age at presentation. Collectively, these factors can result in a delayed diagnosis relative to older dementia patients. 3
The challenges of early-onset dementia cases may relate to its aetiology. Here, a family history of similar conditions was denied, pointing against a genetic contribution to the disease aetiology. Furthermore, genetic testing was not done in this case for financial reasons. A history of metabolic disturbances, head trauma, alcoholism, drug misuse or vitamin deficiencies, all important aetiological factors in early-onset cognitive impairments, was excluded by interviewing the patient’s family.
A head MRI revealed multiple small bright T2 and FLAIR foci within the white matter of both cerebral hemispheres and surrounding the ventricular system, most notably in the frontal and occipital horns. There were no corresponding areas of restricted diffusion for the diffusion-weighted image sequences, likely representing deep white matter ischaemic-related change. Unlike more acute lesions following stroke or multiple sclerosis, which may cause sudden sensorimotor deficits, white matter lesions due to ageing manifest in more subtle and gradual ways and are often cognitive in nature. 4 The MRIs for people older than our patient often exhibit similar white matter lesions surrounding the ventricular system. Critically, this patient’s gradually worsening cognitive symptoms may be, in part, explained by some particularly-located white matter lesions, for example, in the deep forebrain disrupting cholinergic projection fibres at their proximal origin. 4 It is therefore plausible that frontal deep white matter lesions may result not only in cholinergic but also variable monoaminergic (eg, dopamine, serotonin and norepinephrine) neurotransmitters. 4 The involvement of these monoaminergic neuromodulators may also explain some of the non-cognitive symptoms that this patient experienced, such as mood swings, agitation, anxiety and sleep disturbances.
Starting with some difficulties in naming things and people, this patient’s disease process involved progressive cognitive deterioration, a key characteristic of dementia. As the patient also experienced seizures, he was initially diagnosed with epilepsy, which was not confirmed via an initially normal EEG. An initial MRI, which was reported by the family, also indicated brain atrophy and lesions. Despite this, dementia was not considered. However, a follow-up EEG at our hospital revealed persistent spike and wave activity on the left side over the temporal lobe region with an amplitude of 50–80 μV suggesting left temporal epileptiform activity. Widening of the axial CSF spaces, prominent sulci, and deepening of the gyri in both cerebral hemispheres as well as in the temporal lobes bilaterally was further evident on follow-up imaging. Collectively, the patient’s imaging and EEG findings pointed towards both subcortical and cortical brain pathology and potentially epilepsy. Accordingly, mixed type dementia (cortical and subcortical) and co-morbid temporal lobe epilepsy were diagnosed.
Two observations are of note in the present case which indicate the primacy of dementia over epilepsy. First, antiepileptic medication was of no benefit to this patient in his dementia symptoms, likely due to the progressive underlying pathology and resultant dementia. Second, age-related regression measures of memory in patients with epilepsy often show that amnesia/dementia is not a direct consequence of progressive epilepsy. 5
Given the history, physical examination findings and brain imaging findings in this case, we reached a correct aetiological diagnosis of early-onset progressive cognitive deterioration. The clinical diagnosis of mixed cortical and subcortical dementia comorbid with temporal lobe epilepsy remains as a valid diagnosis. Reporting more cases with a similar presentation may ultimately result in case series and the identification of a specific pattern or clinical profile, which will lead to a better understanding of the aetiology and thus management of early-onset dementia cases.
Patient’s perspective
The patient’s wife described what she and her husband faced this way: a few years after the start of my husbands’ symptoms he became dependent and required help carrying out daily tasks. This had a negative impact on our family, both socially and financially. Dementia is a life-changing experience.
Learning points
To highlight the importance of healthcare professionals considering early-onset dementia for relevant patients.
Increased public awareness of this condition is needed.
It is critical that early-onset dementia diagnoses are made as expediently as possible to initiate a comprehensive management plan early in disease course.
- McMurtray A ,
- Christine D , et al
- Elhusein B ,
- Khairi A , et al
- Bohnen NI ,
- Müller MLTM
- Helmstaedter C ,
Contributors All the authors were involved in the patients' care. BE was the primary author and the team leader, and was the clinical fellow who was assigned to the patients and saw the patients regularly in clinics. OBM is psychiatry resident who did the interview and cognitive assessment of the patient. AK is a consultant geriatric psychiatrist who helped the team to establish the diagnosis in dementia and contributed to the case discussion. All the authors met regularly to discuss and write the case report.
Funding The authors have not declared a specific grant for this research from any funding agency in the public, commercial or not-for-profit sectors.
Competing interests None declared.
Patient consent for publication Next of kin consent obtained.
Provenance and peer review Not commissioned; externally peer reviewed.
Read the full text or download the PDF:
Thank you for visiting nature.com. You are using a browser version with limited support for CSS. To obtain the best experience, we recommend you use a more up to date browser (or turn off compatibility mode in Internet Explorer). In the meantime, to ensure continued support, we are displaying the site without styles and JavaScript.
- View all journals
- My Account Login
- Explore content
- About the journal
- Publish with us
- Sign up for alerts
- Open access
- Published: 06 June 2024
Early detection of dementia with default-mode network effective connectivity
- Sam Ereira ORCID: orcid.org/0000-0003-0574-3498 1 , 2 ,
- Sheena Waters ORCID: orcid.org/0000-0001-7241-2272 1 ,
- Adeel Razi ORCID: orcid.org/0000-0002-0779-9439 3 , 4 , 5 &
- Charles R. Marshall ORCID: orcid.org/0000-0002-8227-2354 1 , 6
Nature Mental Health ( 2024 ) Cite this article
2683 Accesses
705 Altmetric
Metrics details
- Alzheimer's disease
- Cognitive ageing
- Predictive markers
Altered functional connectivity precedes structural brain changes and symptoms in dementia. Alzheimer’s disease is the largest contributor to dementia at the population level, and disrupts functional connectivity in the brain’s default-mode network (DMN). We investigated whether a neurobiological model of DMN effective connectivity could predict a future dementia diagnosis at the single-participant level. We applied spectral dynamic causal modeling to resting-state functional magnetic resonance imaging data in a nested case–control group from the UK Biobank, including 81 undiagnosed individuals who developed dementia up to nine years after imaging, and 1,030 matched controls. Dysconnectivity predicted both future dementia incidence (AUC = 0.82) and time to diagnosis ( R = 0.53), outperforming models based on brain structure and functional connectivity. We also evaluated associations between DMN dysconnectivity and major risk factors for dementia, revealing strong relationships with polygenic risk for Alzheimer’s disease and social isolation. Neurobiological models of effective connectivity may facilitate early detection of dementia at population level, supporting rational deployment of targeted dementia-prevention strategies.
Similar content being viewed by others

Age and hippocampal volume predict distinct parts of default mode network activity
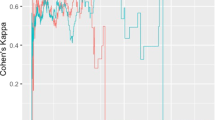
A Novel Joint Brain Network Analysis Using Longitudinal Alzheimer’s Disease Data
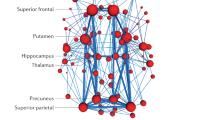
The human connectome in Alzheimer disease — relationship to biomarkers and genetics
There is currently intense interest in identifying strategies to reduce the growing population burden of dementia 1 . Clinical syndromes of dementia are caused by multiple neuropathologies that typically co-occur within individuals 2 . Alzheimer’s disease (AD) pathology is the most important contributor to dementia at the population level, and is associated with distinct patterns of pathological protein deposition and altered neural function that precede the development of structural brain changes and clinical symptoms by a period of years 3 . The ability to reliably detect early changes in neural function associated with AD would provide a platform for the development of individualized dementia-prevention strategies.
Resting-state functional magnetic resonance imaging (rs-fMRI) is increasingly used as a tool for characterizing connectomic biomarkers in AD 4 . It measures endogenous fluctuations in blood-oxygen-level-dependent (BOLD) signal across the brain—which in turn reflect regional neural activation—while a participant lies in an MRI scanner at rest. A map of functional connectivity can be estimated by computing correlations between BOLD time-series from different brain regions 5 . When rs-fMRI is applied to people with AD, or its precursor, mild cognitive impairment (MCI), there are substantial changes in functional connectivity at the group level, when contrasted with healthy controls 6 , 7 , 8 , 9 . Similar changes have been identified in individuals who do not yet have MCI or AD but are considered high risk due to genetic polymorphisms 10 , 11 , 12 , mutations for autosomal dominant AD 13 , a family history of AD 14 or a high burden of pathogenic amyloid and tau proteins 15 , 16 , 17 , 18 , 19 , 20 . Altered functional connectivity—measured with rs-fMRI—is therefore widely considered a potential preclinical biomarker of AD 21 . However, it has not previously been shown to allow single-participant level identification of future dementia risk in a population-based cohort.
The brain regions most commonly implicated in altered functional connectivity in AD are those within the default-mode network (DMN), which is hypothesized to be selectively vulnerable to AD neuropathology 22 . The DMN is typically described as having a core set of brain regions, which includes the medial prefrontal cortex (mPFC), posterior cingulate cortex or precuneus, and bilateral inferior parietal cortices, as well as a set of supplementary brain regions such as the medial temporal lobes and temporal poles 23 . The DMN was initially described as a network of regions that co-activate during a task-negative state in functional imaging studies 24 . In other words, these brain regions seem to be more active when a participant is at rest. However, research shows that the DMN is implicated in several high-level cognitive processes such as social cognition and mental time-travel 23 , 25 , resulting in a contemporary view that the DMN furnishes an individual with their narrative sense of self 26 .
Although findings of altered functional connectivity in the DMN have led to claims that dementia is a syndrome of dysconnectivity, the exact connectivity changes observed are inconsistent across studies 4 , 27 , and are occasionally undetectable 28 . This is perhaps due to methodological limitations associated with defining connectivity on the basis of time-series correlations that overlook biophysical constraints and the established neurobiology of neural circuit function. An alternative approach in connectomics is to fit a neurobiologically informed circuit model to the functional neuroimaging data to characterize the excitatory and inhibitory connections between different brain regions, that is, effective connectivity 29 . Moving beyond correlations in brain activity, effective connectivity describes the causal influence of one brain region over another, by modeling the underlying neural signals that generated the observed data.
Correlations in BOLD activity among brain regions in a network can be explained by an enormous number of possible underlying neural circuitries. With dynamic causal modeling (DCM), multiple putative circuit models of effective connectivity can be compared with each other using model comparison procedures, and the best explanation for the observed data is identified. Thus, effective connectivity provides a more nuanced description of neural connectivity and is likely to detect features that would otherwise be missed when mapping functional connectivity derived from observed BOLD signal. These connectomic subtleties, effective connectivity parameters, are likely to afford discriminative and predictive clinical value, for individualized precision medicine, over and above the correlations measured in functional connectivity 30 .
In neurodegeneration and ageing, neural connections and neurovascular coupling are both impacted 31 , and here DCM becomes particularly useful. As functional connectivity is a multiplexed signal of neural, haemodynamics and noise components, any observed changes in functional connectivity do not differentiate between changes in neural circuitry, haemodynamics or both. DCM, on the other hand, models the neural, haemodynamics and noise components of BOLD separately. Effective connectivity mapping with DCM has been used to successfully discriminate between people with semantic dementia and healthy controls 32 , and also to predict which people with Parkinson’s disease are likely to experience hallucinations 33 . A small number of studies have estimated effective connectivity differences between people with AD or MCI, and healthy controls 34 , 35 , 36 , 37 , 38 , 39 , and have also detected differences in small samples of undiagnosed individuals at high-risk for AD 40 , 41 . However, to the best of our knowledge, there has been no work on the potential for effective connectivity to make predictions about dementia incidence at single-participant level.
Here we investigated whether effective connectivity changes in the DMN can be used to make early predictions about dementia incidence and prognosis in a population cohort. To that end we constructed a nested case-control study using the UK Biobank (UKB) cohort, among which a sample have developed incident dementia in the years since neuroimaging data acquisition. To ensure that the analysis was ecological and reflected the range of dementia pathologies within the population, we used all-cause dementia outcomes rather than restricting the analysis to those with Alzheimer’s disease. We analyzed rs-fMRI data from individuals who developed dementia and a large sample of matched controls. We applied spectral DCM 30 —a technique that fits generative neural and haemodynamic models to the cross-spectra of BOLD time-series from rs-fMRI data—to estimate effective connectivity. We predicted that there would be detectable differences in DMN effective connectivity years before people were diagnosed with dementia, and that these differences would be large enough to make meaningful out-of-sample predictions about future dementia incidence. We also predicted that these early patterns of dysconnectivity would be associated with exposure to known risk factors, particularly polygenic risk for AD as the key driver of AD pathological change, and social isolation due to the role of the DMN in social cognition 23 .
After exclusions for image quality and excessive in-scanner head motion (see Methods ), our final usable sample included 103 dementia cases (22 individuals with prevalent dementia and 81 who later developed incident dementia) and 1,030 matched controls (see Fig. 1 ). The 81 incident cases had a median time to diagnosis of 3.7 years (range = 0.4–8.5). The total sample had a mean age of 70.4 at the time of MRI data acquisition. Cases and controls were matched on age, sex, ethnicity, handedness and geographical location of the testing center (see Extended Data Table 1 for sample characteristics). Cases performed worse than controls in four cognitive tests, which were analyzed as part of this study (see Methods and Supplementary Table 3 ). Although most of our case-sample were prediagnostic, their lower cognitive test scores might reflect objective evidence of cognitive decline. Alternatively, these results might reflect a reduced cognitive reserve in this sample.
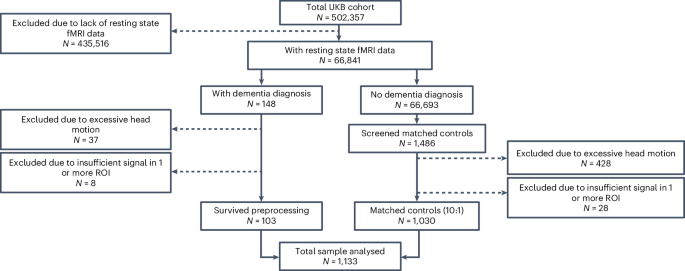
Dementia cases and matched controls were selected from the UKB cohort. Once the final usable sample of dementia cases was determined ( N = 103), matched controls were iteratively selected and screened to ensure they satisfied fMRI preprocessing criteria, until there were ten suitable matched controls for each individual case ( N = 1,030).
The analysis pipeline is illustrated in Fig. 2 . For each participant, BOLD time-series were extracted from ten pre-defined regions-of-interest (ROIs), which together defined our DMN. The network included four mid-line ROIs: the precuneus (PRC), anterior medial prefrontal cortex (amPFC), dorsomedial prefrontal cortex (dmPFC) and ventromedial prefrontal cortex (vmPFC); one ROI in each medial temporal lobe, in the left and right parahippocampal formations (lPHF/rPHF); and four lateral ROIs, the right intraparietal cortex (rIPC), left intraparietal cortex (lIPC), right lateral temporal cortex (rLTC) and left lateral temporal cortex (lLTC). See Methods for further details on these ROIs.
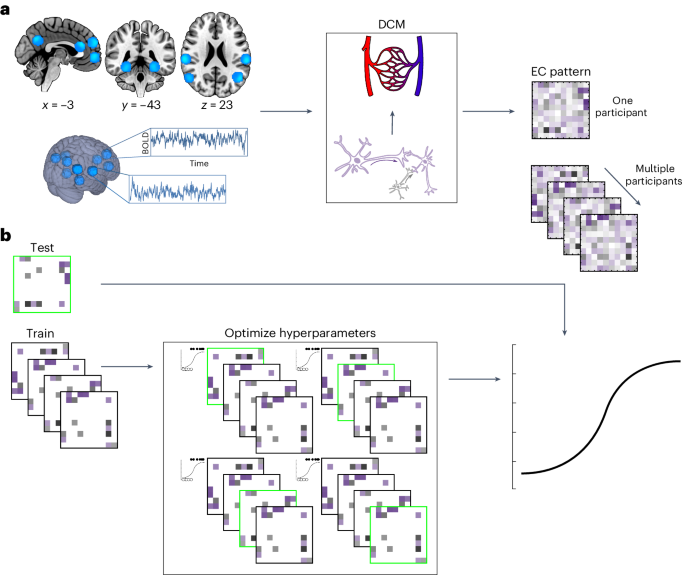
a , Ten ROIs were used to define the default-mode network. There are four mid-line ROIs shown in the sagittal section (top left), two medial temporal ROIs shown in the coronal section (top middle), and four lateral temporal and parietal ROIs shown in the axial section (top right). For each participant, voxels were only selected within the sphere if supra-threshold activation was detected. Lighter shades of blue indicate voxels that were selected more frequently across participants. BOLD time-series were extracted from each of the ten ROIs. A spectral DCM was fitted to these BOLD time-series data. The DCM optimizes effective connectivity parameters to find the best explanation for the observed BOLD time-series in terms of excitatory (purple) and inhibitory (gray) neural connections, as well as altered blood flow that would be expected to result from this neural activity. Each participant’s effective connectivity (EC) pattern is estimated separately and is represented as a 10 × 10 EC matrix, where each cell in the matrix shows the magnitude and valence (excitatory or inhibitory) of a connection between a pair of ROIs. b , Bayesian model reduction is applied to the EC matrix to eliminate unnecessary parameters and find the most parsimonious model to explain the observed data, at the group level. The resulting sparse EC patterns are used to train regularized logistic regression models to predict dementia incidence using nested cross-validation. A single participant (or random subgroup) is left out of the analysis as a test set, highlighted in green. All remaining participants constitute a training set. The hyperparameters of the regression model are optimized on this training set with new nested test and train sets within the outer training set. Once optimal hyperparameters are selected, the optimized model is trained on the full outer training set and tested on the original left-out sample. This procedure is iterated such that every participant (or subgroup) is used once as a test set.
A fully connected DCM was fitted to the cross-spectra of these time-series data (spectral DCM) to estimate the effective connectivity between each and every pair of ROIs in the ten-node network (Fig. 2a ).
Effective connectivity predicts who will get dementia
Bayesian model reduction and averaging were applied (see Methods ) to estimate the simplest effective connectivity map to explain group-level differences between dementia cases and controls (Fig. 3 ) while controlling for age, sex and in-scanner head motion. There was very strong evidence (posterior probability > 0.99) for 15 connectivity parameters that differed between cases and controls. The three largest connectivity changes seen in the dementia cases were: increased inhibition from the vmPFC to lPHF, increased inhibition from lIPC to the lPHF, and attenuated inhibition from the rPHF to the dmPFC.
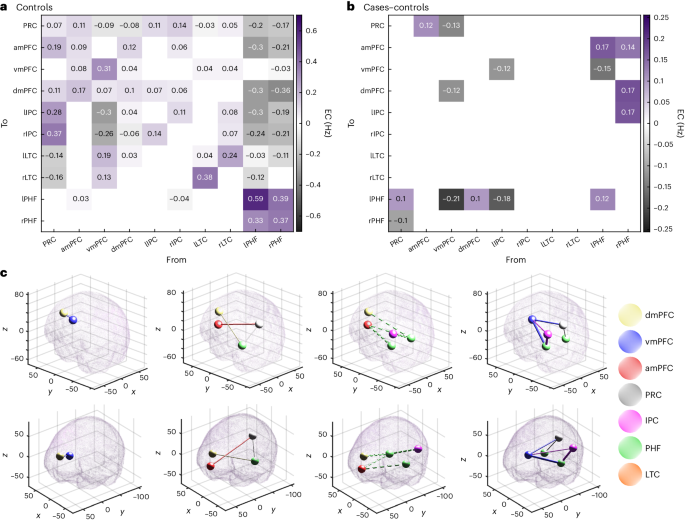
a , Bayesian model average of effective connectivity in healthy controls. Each cell shows the effective connectivity (EC), in hertz, between a pair of regions. Gray and purple colours indicate inhibitory and excitatory connections, respectively. Cells along the diagonal represent auto-inhibitory connections, as unitless scaling parameters. Only parameters with an at least 99% posterior probability of being non-zero (amounting to a very strong evidence) are shown. b , Bayesian model average of the difference in effective connectivity between cases and controls. Gray indicates a change towards increased inhibition (reduced excitation), whereas purple indicates a change towards increased excitation (reduced inhibition). Only parameters with an at least 99% posterior probability of being non-zero are shown. c , Effective connectivity differences between cases and controls visualized in Montreal Neurological Institute (MNI) space. Each tube represents a connection change. Solid tubes represent connections that are strengthened in cases compared with the controls; dashed tubes represent connections that are attenuated in cases compared with the controls; the thickness of the tube represents the magnitude of the connection change; and the color of the tube represents the brain region from where the connection originates. The top row and bottom rows show the same data but from two different angles. The four columns display the following, respectively: attenuated excitatory connections, strengthened excitatory connections, attenuated inhibitory connections, strengthened inhibitory connections.
These 15 connectivity parameters (Fig. 3b ) were used to train an elastic-net logistic regression model to predict future dementia diagnosis in stratified K -fold cross-validation. The model was trained on the entire dataset, including prevalent cases who were already diagnosed with dementia, but performance was evaluated exclusively on classification between prediagnostic cases and their matched controls. Using receiver-operating characteristic (ROC) analysis, we found the model to have excellent discriminative performance (Fig. 4a ) with an area under the curve (AUC) of 0.824 (range = 0.79–0.843). See Methods for further details on how the AUC was computed. As a sensitivity analysis, a classifier was also trained on the full model of 100 effective connectivity parameters. This yielded a marginally reduced AUC of 0.816 (range = 0.807–0.842).
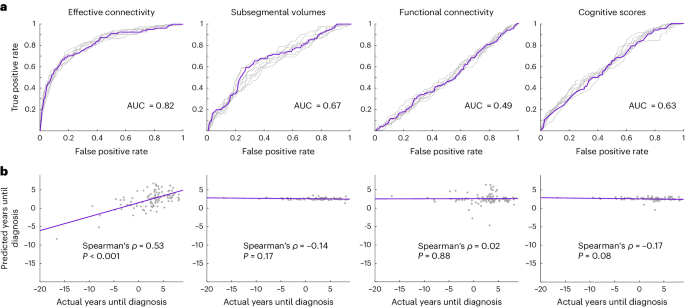
a , ROC curves for regularized logistic regression models trained on effective connectivity parameters, gray matter volumes, functional connectivity or cognitive scores to classify undiagnosed dementia cases from controls. A ROC curve is generated by taking the mean curve across all test-folds of cross-validation. In each plot there are nine ROC curves because nine iterations of stratified K -fold cross-validation were performed. The purple curves indicate the iteration that generated the median AUC across iterations. This median AUC is indicated at the bottom right of the plot. b , Scatter-plots showing the performances of regularized linear regression models trained on the same data types as in a , to predict the time until dementia diagnosis across 102 dementia cases (81 undiagnosed and 21 with a pre-existing diagnosis). All statistical tests are two-sided, and P = 2 × 10 −8 for the effective connectivity prognosticator (left-most scatter-plot).
Effective connectivity predicts time until dementia diagnosis
To assess the potential role of DMN effective connectivity in prognostication, we ran an analysis that only used the case cohort. We used Bayesian model reduction and averaging (see Methods ) to estimate the simplest effective connectivity map to explain the inter-individual variation associated with the time until dementia diagnosis while controlling for age, sex and in-scanner head motion. The time until diagnosis was negatively valued for participants who already had a diagnosis of dementia at the time of data acquisition. There was a very strong evidence (posterior probability > 0.99) for 37 connectivity parameters that were associated with the time until diagnosis (Fig. 5 ), including the three connections, described above, that showed the largest difference between cases and controls (Fig. 3 ).
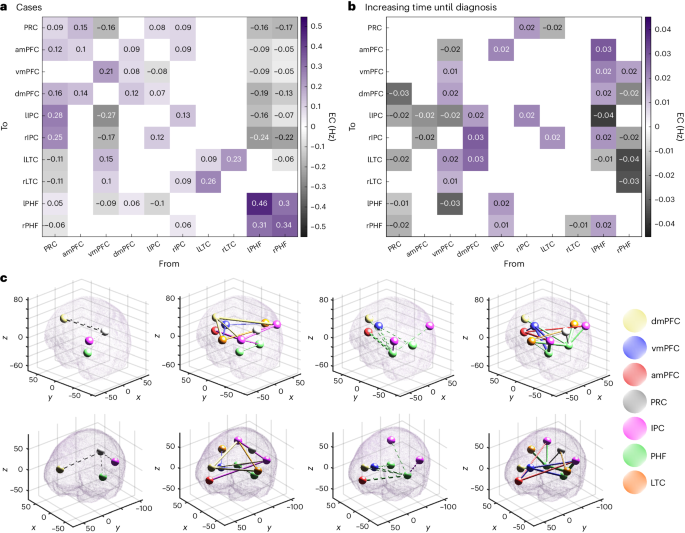
a , Bayesian model average of effective connectivity in cases. Each cell shows the effective connectivity (EC), in hertz, between a pair of regions. Gray and purple colours indicate inhibitory and excitatory connections, respectively. Cells along the diagonal represent auto-inhibitory connections, as unitless scaling parameters. Only parameters with an at least 99% posterior probability of being non-zero are shown. b , Bayesian model average of the changes in effective connectivity, among cases, associated with a longer time until diagnosis. Gray indicates a change towards increased inhibition (reduced excitation), whereas purple indicates a change towards increased excitation (reduced inhibition). Only parameters with an at least 99% posterior probability of being non-zero are shown. c , Effective connectivity changes. Visualization follows the same format as Fig. 2c , but with connectivity changes associated with time until diagnosis, rather than differences between cases and controls.
These 37 connectivity parameters were used to train an elastic-net regularized linear regression model to predict time until diagnosis in K -fold cross-validation. There was a positive correlation between actual time until diagnosis and predicted time until diagnosis (Spearman’s ρ = 0.53, P = 2 × 10 −8 ). As a sensitivity analysis, a linear regression model was also trained on the full model of 100 effective connectivity parameters. This still yielded a positive correlation between the actual and predicted times until diagnosis, but the effect was reduced (Spearman’s ρ = 0.36, P = 1.9 × 10 −4 ).
Comparisons with alternative metrics
To see how effective connectivity compares as a diagnostic and prognostic tool with other MRI-based markers, we trained predictive models using exactly the same methods as above, but using volumetric data and functional connectivity data instead of effective connectivity parameters.
We used 40 hippocampal and parahippocampal subsegmental volumetric features for the volumetric-based models (see Methods ). Interestingly, we found that dementia-related change in effective connectivity was negatively associated ( R 2 = 0.012, P = 0.0002) with the mean volume across these subsegmental regions (see Methods ). In an exploratory post-hoc analysis, we found that the three subsegmental volumes with the strongest negative association with effective connectivity change were: the left head of hippocampal CA3 ( R 2 = 0.014, P = 6.73 × 10 −5 ), left body of subiculum ( R 2 = 0.013, P = 1.03 × 10 −4 ) and left anterior parahippocampal gyrus ( R 2 = 0.012, P = 2.12 × 10 −4 ). These findings suggest that the effective connectivity changes that we observed probably reflect an AD-like pathology in which the earliest volume loss is in the medial temporal lobes, particularly the entorhinal cortex. Despite the fact that effective connectivity change and volume loss may reflect the same pathological process, the elastic-net logistic regression classifier trained on volumetric data (Fig. 4a ) yielded only moderate diagnostic value with an AUC of 0.671 (range = 0.62–0.69). The elastic-net linear regression prognosticator (Fig. 4b ) performed at chance level (Spearman’s ρ = −0.14, P = 0.17).
For the functional connectivity analysis, we computed Fisher z -transformed Pearson coefficients between every pair of ROIs in the same network that we used for the effective connectivity analysis. This yielded 45 functional connectivity values (see Methods ). The elastic-net logistic regression classifier (Fig. 4a ) performed at chance level with an AUC of 0.491 (range = 0.478–0.517); the elastic-net linear regression prognosticator (Fig. 4b ) also performed at chance level (Spearman’s ρ = 0.02, P = 0.88).
Given the considerable differences in cognitive task data between cases and controls, we also tested whether cognitive data alone could predict both a future dementia diagnosis and the time until diagnosis. The elastic-net logistic regression classifier (Fig. 4a ) yielded moderate performance with an AUC of 0.628 (range = 0.606–0.641). The elastic-net linear regression prognosticator (Fig. 4b ) performed at chance level (Spearman’s ρ = −0.17, P = 0.08).
Comparing all four data types, effective connectivity parameters yielded the best classification performance for predicting future dementia diagnosis, and was the only data-type that yielded better-than-chance prognostication (prediction of time until dementia diagnosis).
Associations between risk factors and effective connectivity
Finally, we conducted an exploratory analysis to investigate whether the effective connectivity changes might represent the effects of major risk factors for dementia. We first defined an effective connectivity index for each participant, which was simply the probability of dementia, outputted by a case-control classifier trained on effective connectivity parameters with leave-one-out cross-validation (see Methods ). This value summarizes the extent to which an individual’s DMN effective connectivity pattern conforms to a dementia-like phenotype rather than a control-like phenotype, where a value of 1 indicates a dementia-like pattern and a value of 0 indicates a control-like pattern.
For each individual, we then extracted data from UKB describing the modifiable risk factors identified in the 2020 Lancet commission on dementia 1 (see Methods ), as well as each participant’s AD polygenic risk score (PRS). For each risk factor, we ran a separate weighted linear regression model, across the entire cohort of cases and controls ( N = 1,133), to measure the association between effective connectivity index and that specific risk factor, controlling for age, sex and social deprivation (Townsend) score (Fig. 6a and Supplementary Table 1 ). After correcting for multiple comparisons, the AD PRS was strongly associated with effective connectivity index ( β = 0.053, P = 3.7 × 10 −12 , P FWE-corrected = 4.4 × 10 −11 ), and this association was much stronger than any association between effective connectivity index and a modifiable risk factor (Fig. 6a ). This corroborates the earlier finding of a negative association between hippocampal and parahippocampal volume and effective connectivity change, and suggests that these EC changes probably represent Alzheimer’s pathology rather than a more general reflection of brain health.
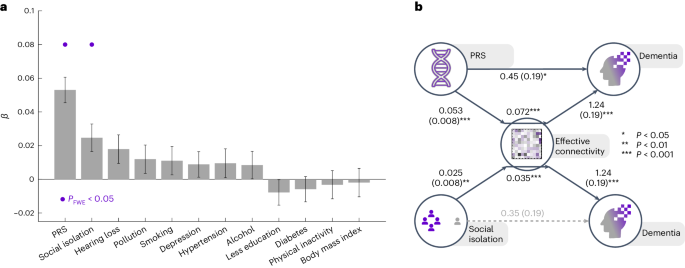
a , Standardized regression coefficients ( β ) from 12 different linear regression models, testing for associations between PRS and 11 modifiable risk factors for dementia and DMN EC index. Each bar reflects the estimated coefficient from a regression model across all N = 1,133 participants, where age, sex and social deprivation (Townsend) score were included as covariates of no-interest. Error bars show standard error of the regression coefficients. Any regression coefficients that are statistically significant after correcting for multiple comparisons are labeled with a purple dot; P FWE-corrected = 4.4 × 10 −11 for PRS, P FWE-corrected = 0.028 for social isolation. All statistical tests are two-sided. See Supplementary Table 1 for more detailed results. b , Results of two mediation models indicating that there are significant indirect paths whereby EC index mediates the association between social isolation and dementia and partially mediates the association between polygenic risk score and dementia. Each path is labeled with regression coefficient and standard error in brackets. All statistical tests are two-sided.
We constructed a mediation model (Fig. 6b ) to see whether effective connectivity index mediated any of the relationship between PRS and dementia incidence. By including the effective connectivity index as a mediator, the direct path coefficient from PRS to dementia incidence was reduced from 0.5 ( P = 0.0007) to 0.45 ( P = 0.017). There was a significant indirect mediated path ( β = 0.07, P < 0.001), which explained away 10% of the association between PRS and dementia incidence. These results indicate that DMN effective connectivity partially mediates the role of genetic risk in dementia pathogenesis.
For the modifiable risk factors, social isolation was the only variable that showed a significant association with effective connectivity index ( β = 0.025, P = 0.003, P FWE-corrected = 0.028). This association demonstrated that individuals with more self-reported social isolation were more likely to have a ‘dementia-like’ pattern of DMN effective connectivity. To see whether social isolation was simply an early sign of cognitive impairment, we constructed a composite score of cognitive ability (see Methods ) and tested whether this was correlated with social isolation. These variables were not correlated (Spearman’s ρ = 0.05, P = 0.11), consistent with social isolation being a cause rather than a consequence of the dementia process.
We constructed a mediation model (Fig. 6b ) to test whether effective connectivity index might mediate the known association between social isolation and dementia incidence. After accommodating for a hypothesized mediating effect of effective connectivity index, we detected a significant indirect path from social isolation to dementia, mediated by effective connectivity index ( P < 0.001). Furthermore, an association between social isolation and dementia incidence ( P = 0.037) was rendered non-significant ( P = 0.07), after accounting for this mediator. To further test the biological plausibility of this model, we repeated this mediation analysis, excluding the 22 prevalent cases and their 220 matched controls. This yielded comparable results, with a significant indirect mediation path ( P < 0.001), and a direct path from social isolation to dementia ( P = 0.043) that was rendered non-significant by including the mediator ( P = 0.09). These results show that prediagnostic effective connectivity changes, in the DMN, mediate an association between premorbid social isolation and subsequent dementia incidence. Taken together, prediagnostic DMN dysconnectivity appears to be a consequence of both genetic and environmental risk factors.
Our findings show that a neurobiologically informed model of DMN effective connectivity can enable accurate predictions about whether and when an individual will develop dementia. The performance of our effective connectivity-based classifier exceeded that of classifiers based on volumetric and functional connectivity data, both in our analyses, and also when comparing it with past works using structural MRI data as a unimodal predictor of future conversion to dementia 42 .
From a clinical perspective, this suggests that rs-fMRI could become a tool for identifying a neural network signature of dementia risk early in the pathological course of the disease. This type of non-invasive early detection of dementia is an increasingly valuable goal, particularly with the arrival of disease-modifying drugs. Recent clinical trials have shown promise for amyloid-β-targeting monoclonal antibodies, which are modifying the disease trajectory in AD for the first time 43 , 44 , supposedly with greater therapeutic potential when started earlier in the disease process. Early detection of dementia risk is also important in the context of targeted risk reduction strategies irrespective of underlying pathology 1 . Whereas pathology-specific biomarkers can guide disease-modifying molecular therapies, non-specific biomarkers for all-cause dementia, such as those developed in this study, will be useful for identifying who is most likely to benefit from lifestyle changes and public health interventions, and when these interventions are likely to have the biggest impact.
Recent research on early detection of dementia tended to prioritize biomarkers that directly reflect pathogenic protein deposition in AD, such as cerebrospinal fluid analysis for amyloid beta and tau proteins. However, these markers have limited predictive ability among healthy population cohorts because a majority of individuals remain asymptomatic during follow up (for example, >90% of those with amyloid beta positivity remain asymptomatic over five years 45 ). Plasma levels of phosphorylated tau are highly predictive of AD neuropathology 46 and can also accurately predict conversion from MCI to AD when combined with cognitive and genetic data 47 . It is likely that rational use of anti-amyloid therapies among asymptomatic individuals would be enhanced by the addition of a proximity marker based on early neural dysfunction, and our results suggest that effective connectivity could be an ideal candidate for this, especially because they demonstrate that effective connectivity can be used to make predictions, not only about who will develop dementia, but also the time until future diagnosis. These predictions were more accurate than previous prognostic models trained on structural MRI data and functional connectivity features 48 .
We acknowledge that fMRI has its limitations as a diagnostic and prognostic tool. It is expensive and the signal can be degraded in the presence of excessive head motion. This is reflected in the high exclusion rate in our own analysis. We used strict exclusion thresholds so that the models were trained on a high-quality dataset, but future work will need to assess the tolerance that these methods have for lower-quality data.
A further limitation of this study is uncertainty around how generalizable these effective connectivity-based models will be. First, our models rely on a feature selection step (Bayesian model reduction) that occurs prior to cross-validation. In theory, this might inflate model performance metrics; however, our sensitivity analysis showed that model performance was comparable when this feature selection step was omitted. Furthermore, by running Bayesian model reduction on the entire dataset, we anticipate that this simplified model is more likely to generalize to external datasets than models using the entire effective connectivity matrix. It will be crucial to validate these preliminary results on an external sample. A second point revolves around who exactly these models should be validated on. Our sample dataset is from the UKB. This cohort—comprising approximately half a million UK-based participants—is healthier than the general population 49 and less socio-economically deprived than non-participants 50 , with a disproportionately high number of white participants. The subsample of this cohort who underwent brain imaging is younger, and has better psychological and physical health compared to the average UKB participant 51 . The generalizability of these results to a more representative sample needs to be assessed.
Another important caveat of using a UKB dataset for this study is that our labeling of cases and controls relies on clinician coding rather than standardized diagnostic criteria. Although a clinician-coded diagnosis is likely to be more clinically relevant, it may mean that symptoms of the disease were already present in the prediagnostic phase, limiting our ability to assess this as a true preclinical biomarker. Indeed, our analysis of cognitive test data revealed that the prediagnostic cases were cognitively impaired with respect to controls. Without more longitudinal cognitive data, it is not clear whether this cognitive impairment reflects cognitive decline from a pathological dementia process, or simply the baseline characteristics of this sample (we note that rates of secondary education attendance were significantly lower in the case-sample; see Extended Data Table 1 ). The median time to diagnosis in our prediagnostic sample was 3.7 years, and it is likely that some of these participants already had MCI. Another avenue of future research will be assessing effective connectivity-based biomarkers at even earlier stages in the pathological process, before any cognitive decline is expected to occur.
Our use of a population cohort of all-cause dementia, rather than a well phenotyped AD-specific cohort, is both a strength and a limitation of this work. Dementia is typically due to mixed pathologies, and syndromic diagnoses in life are frequently found to be incorrect at post mortem 52 . From a pragmatic population health standpoint, the ability to accurately predict all-cause dementia is therefore desirable, and makes it likely that the results of this study would be generalizable to real-world settings. We found associations between DMN effective connectivity and AD polygenic risk score, as well as between DMN effective connectivity and hippocampal and parahippocampal volumes. Although these findings suggest that these effective connectivity changes at least partially represent pathological changes specific to AD, our ability to make pathology-specific inferences is limited. Stronger evidence for a specific relationship with AD pathology could be obtained through future work incorporating biomarkers of AD proteinopathies. Indeed, in previous works, classifiers have made improved predictions on preclinical cohorts when multimodal data were used, for instance, by combining structural MRI, genetic data, cerebrospinal fluid assays and cognitive assessments 53 , 54 . We anticipate that, when combined with other data modalities such as amyloid beta and tau markers, effective connectivity would be likely to yield improved predictive performance.
In an exploratory analysis of modifiable risk factors, we found that social isolation had a unique and strong association with the effective connectivity changes in the dementia cohort. This finding has important implications for our understanding of why DMN dysconnectivity is so frequently observed in clinical and preclinical dementia 21 . There is a substantial overlap between the DMN and what is typically described as a social cognition network 23 , 55 . The mPFC, temporal poles, precuneus and temporo-parietal junction consistently activate during cognitive tasks in which participants are required to think about another person’s intentions or beliefs (that is, engage in Theory of Mind) 55 , 56 , 57 . There is emerging evidence that this network of brain regions is highly sensitive to one’s social environment. Social isolation has been shown to cause hypomyelination in rats 58 , 59 , which can be reversed through re-socialization 58 , 60 . In humans, childhood development of social cognitive skills is associated with white matter tract maturation in the mPFC and temporo-parietal junction 61 . In adulthood, myelin density in the mPFC is associated with one’s ability to flexibly switch between one’s own point of view and another person’s point of view 62 . Social isolation is a well-established risk factor for dementia 1 , 63 , 64 , 65 , 66 . Psychosocial interventions such as cognitive stimulation therapy can improve symptom burden 67 and may also reverse some of the changes in DMN functional connectivity that are seen in AD 68 . These interventions are thought to weaken the link between underlying dementia pathology and cognitive decline, by promoting compensatory brain changes and expanding cognitive reserve 69 .
From a neurobiological perspective, DMN dysconnectivity is thought to be a consequence of activity-dependent tau spread, from the medial temporal lobes to densely connected cortical hubs 8 , 10 . Here, we found that changes in DMN effective connectivity mediated an association between social isolation and dementia incidence. This finding is consistent with a theory that social isolation triggers the DMN dysconnectivity observed in dementia. However, an important limitation of our study is that we are unable to determine which DMN effective connectivity changes are pathological and which are compensatory.
We identified multiple changes to both inhibitory and excitatory connections. Some of these connections were strengthened in dementia cases whilst others were attenuated. Examining the three largest connection differences between cases and controls, we saw a strengthening of inhibitory tone from both prefrontal and intraparietal cortices to the medial temporal lobe, and a weakening of inhibitory tone from the medial temporal lobe to the prefrontal cortex. Electrophysiological studies in people with AD show cortical hyperexcitability 70 , 71 , while in vivo mouse research has shown that tau silences neurons, and actually reduces excitability despite the hyperexcitability caused by amyloid beta (ref. 72 ). Of the three largest connection changes we observed, the only weakened connection was an inhibitory connection from the parahippocampal formation to the prefrontal cortex. This could be due the neuron-silencing effect of tau accumulation in the medial temporal lobe in the early stages of the disease. We speculate that the increased inhibitory tone from frontal and parietal DMN hubs to the medial temporal lobe may reflect a homeostatic compensation to maintain excitation–inhibition balance within the network. An important avenue for future research will be to collect longitudinal imaging data along with data on subjective and objective cognitive impairment to understand the clinical significance of different connections within the DMN dysconnectivity pattern. DMN dysconnectivity is also likely to be better understood in the context of wider cortical dynamics that involve other long-range networks. The salience network and frontoparietal control network are both altered in those at high risk of AD 73 and these changes are associated with future cognitive decline 74 . It may be useful to include these networks in the development of future effective connectivity-based predictive models.
In summary, we found that effective connectivity in the DMN can be used as a non-invasive population-based prediagnostic biomarker for predicting future dementia incidence. This biomarker, using rs-fMRI data, is superior to using structural MRI data. The connectivity changes in the DMN are strongly associated with AD polygenic risk and social isolation, a risk factor that might accelerate the effects of pathological protein in the DMN.
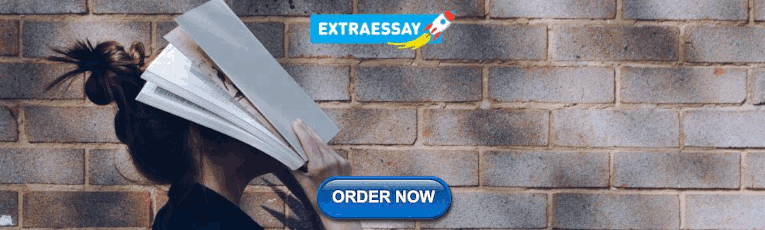
Study design
This was a nested case-control study designed to assess whether DMN effective connectivity can be used predict two outcomes of interest. The first outcome is a future diagnosis of dementia. The second outcome is time until dementia diagnosis. Potential confounders, which we identified and tried to control for in our analyses, included age, sex, ethnicity, handedness, in-scanner head motion, geographical location of data acquisition and social deprivation (Townsend index). All statistical tests reported are two-sided.
UKB sample selection
The UKB is a longitudinal cohort study that is regularly updated with healthcare outcomes from national UK primary and secondary healthcare databases. We identified all UKB participants who have ever had a dementia diagnosis on their health record, as of the UKB data update in May 2023, and who also had rs-fMRI data available on the UKB. Our sample size was therefore determined by data availability. Selection bias was mitigated in this study by identifying every single participant with a dementia diagnosis. This yielded an initial sample of 148 dementia cases. For each of these dementia cases, we identified ten control participants from the UKB who did not have a dementia diagnosis on their health record, and matched them with the dementia case in terms of age, sex, handedness, ethnicity and the geographical location of the MRI scanning center. After excluding participants who failed the preprocessing stage (for example, excessive head motion) and replacing failed controls with new matched controls we were left with a final usable sample of 103 cases and 1,030 matched controls. Of these 103 cases, 81 did not have a dementia diagnosis at the time of MRI data acquisition, whereas 22 already had prevalent dementia. In total, 1,486 control participants were screened through data preprocessing before the target number of 1,030 was achieved.
Participant sex identification was acquired from a central registry (the National Health Service) at the time of recruitment to the UKB, but in some cases was updated through participant self-report. Participant ethnicity was defined through self-report at the time of recruitment to the UKB. Participants were asked to report their ethnicity as ‘white’, ‘mixed’, ‘Asian or Asian British’, ‘Black or Black British’, ‘Chinese’, ‘other ethnic group’, ‘do not know’ or ‘prefer not to answer’.
MRI data acquisition
Magnetic resonance imaging data were acquired between 2006 and 2010 as part of the UKB prospective cohort study, across multiple sites in the United Kingdom (Manchester, Newcastle and Reading). The scanner was a Siemens Skyra 3 T with a Siemens 32-channel RF receive head coil. Each participant underwent a 35 min scanning session, during which the following data were acquired: a T1-weighted structural image, rs-fMRI time-series, a T2-weighted FLAIR structural image, a diffusion MRI structural image, a susceptibility-weighted image and task-based fMRI time-series data. We only used the T1 image and the rs-fMRI data for our analyses.
The T1-weighted image was acquired in a 5 min 3D MPRAGE sequence with a resolution of 1 mm isotropic. The rs-fMRI data were acquired using a 6 min GE-EPI sequence with ×8 multislice acceleration. Resolution, 2.4 mm isotropic; repetition time (TR), 0.735 s; echo time (TE), 39 ms; flip angle, 52°. Data were acquired under the same protocols for cases and controls.
MRI data preprocessing
Preprocessing was performed on raw UKB imaging data in SPM12 using batch scripts in MATLAB R2023a. First, the T1-weighted structural image was segmented into tissue subtypes, skull-stripped and then warped into Montreal Neurological Institute (MNI) space. The rs-fMRI data were spatially realigned to the single-band reference scan that was acquired in addition to the multi-band EPI sequence. Volumes were then co-registered to the skull-stripped T1 image, normalized to MNI space and spatially smoothed using a 6 mm isotropic Gaussian kernel.
In-scanner head motion was estimated for each participant by computing framewise displacement for each participant using the three translational and three rotational motion parameters (assuming rotation around the surface of a sphere with radius 50 mm). Participants were excluded from further analysis if their maximum framewise displacement exceeded 2.4 mm. This threshold was chosen because it was the voxel resolution of the dataset.
Time-series extraction
A DMN was constructed by pre-defining ten ROIs on the basis of pre-existing literature 7 , 75 . This number of ROIs was chosen to compromise between anatomical detail and feasible computation time when fitting dynamic causal models. The ten-node network comprised a core DMN of the anterior medial prefrontal cortex (amPFC), the precuneus, and the left and right intraparietal cortex (IPC). These four ROIs were centered around the following co-ordinates, respectively, on the basis of a previous study on DMN effective connectivity by Almgren and colleagues 75 : ( x = 2, y = 56, z = –4), ( x = 2, y = –58, z = 30), ( x = –44, y = –60, z = 24), ( x = 54, y = –62, z = 28). We included the following additional ROIs in our DMN network, using co-ordinates from a past study on DMN connectivity in amnestic cognitive impairment by Dunn and colleagues 7 : ventromedial prefrontal cortex (vmPFC), dorsomedial prefrontal cortex (dmPFC), left and right lateral temporal cortex (LTC) and left and right parahippocampal formation (PHF), centered on the following co-ordinates, respectively: ( x = 0, y = 26, z = 18), ( x = 0, y = 52, z = 26), ( x = –60, y = –24, z = 18), ( x = 60 , y = –24, z = 18), ( x = –28, y = –40, z = –12), ( x = 28, y = –40, z = –12).
The signal from each ROI was estimated by fitting a general linear model containing a discrete cosine basis set with frequency range 0.0078–0.1 Hz, as well as the following nuisance regressors: six head motion regressors, a regressor for cerebrospinal fluid signal (a principal eigenvariate sphere radius of 5 mm centered in the third ventricle at ( x = 0, y = –40, z = –5)), a regressor for white matter signal (a principal eigenvariate sphere radius of 6 mm centered in the brainstem at ( x = 0, y = –24, z = –33)). Global signal regression was not performed as there is evidence it does not substantially impact results in small network analyses 75 . An F-contrast was specified across all components of the discrete cosine basis set, yielding a BOLD time-series of low-amplitude fluctuations in each voxel within a 10 mm radius sphere centered on each of the ten ROI co-ordinates listed above.
For each ROI, a new 8 mm sphere was then centered on the peak intensity voxel. A summary signal for the ROI was computed as the principal eigenvariate of all supra-threshold voxels (uncorrected α = 0.05) that lay in the conjunction space of the first 10 mm sphere and the second 8 mm sphere. These were voxels with evidence for low frequency BOLD fluctuations. Note that the principal eigenvariate across voxels is used, rather than the mean, so that negative and positive signals do not negate each other and that the extreme values don’t bias the mean estimate. If any of the ten ROIs yielded no supra-threshold voxels then the participant was excluded from further analysis.
Estimating effective connectivity
Effective connectivity was estimated using spectral DCM using the DCM12 toolbox in SPM12. Spectral DCM fits a biophysical state-space model to the observed cross-spectra of BOLD signals, to estimate underlying neuronal states 30 and the rate of change in neural activity in each region (in hertz) as a function of activity in other regions (that is effective connectivity). For each participant we fitted a fully connected DCM with a connectivity parameter for every possible pair of the ten ROIs, including auto-inhibitory self-connections. This model thus comprised 100 connectivity parameters. The DCM software 76 , 77 uses the variational Laplace algorithm to invert the model and estimate these connectivity parameters by minimizing negative free energy. We used the software’s default priors. Each participant’s DCM fit was screened for convergence by ensuring it met the following criteria: explained variance of BOLD signal greater than 10%, at least one connection (excluding self-connections) with an absolute connection strength of greater than 1/8 Hz, and at least one effectively estimated parameter (based on the Kullback–Leibler divergence of posterior from prior). All participants who had a DCM fitted met these criteria for model convergence.
We fit a parametric empirical Bayes (PEB) model 78 to the full set of participant-specific DCMs to estimate an average connectivity matrix across participants and estimate the difference in connectivity between cases and controls. The PEB technique enables us to estimate group-level connectivity strengths by fitting a hierarchical model to the estimated connectivity parameters of each individual and the precisions of those parameters. We specified a between-participants design matrix that contained five columns: a column of ones, to model the average connectivity strengths across all participants; a column of ones and zeros, to model the differences in connectivity between cases and controls; and three columns to model covariates of no-interest (age, sex and mean framewise displacement to model any effects attributable to head motion). The last three columns were mean-centered. Instead of estimating a full covariance matrix across connectivity parameters, a single precision component was shared across connectivity parameters, to permit model estimation within a reasonable amount of time. The resulting PEB model comprised 500 connectivity parameters, that is, a 10 × 10 connectivity matrix for each of the five columns of the between-participants design matrix.
Finally, we used exploratory Bayesian model reduction and Bayesian model comparison to find the best (and simplest) model to explain the data. In this procedure an automatic greedy search over reduced models iteratively discards parameters that don’t contribute to model evidence. A Bayesian model average of parameters is then calculated over the 256 models from the final iteration of the greedy search (default settings of DCM software).
The details of the biophysical model used in DCM, model inversion at a participant and group level and Bayesian model reduction have already been extensively documented 76 , 77 , 79 and will not be reproduced here.
Case-control classifier
Of the group-level parameters that model differences in effective connectivity between cases and controls, we selected all parameters with an at least 99% posterior probability of being non-zero. This way we identified a set of statistically plausible connections to use as data features for our classifier.
We trained an elastic-net regularized logistic regression model on these features to classify cases from controls using the glmnet toolbox for MATLAB. To accommodate for the 10:1 imbalance in class size, observation weights were applied so that cases were weighted ten times more than controls. A nested stratified k -fold cross-validation (CV) scheme was applied for tuning two hyperparameters: elastic mixing parameter \(\alpha\) and regularization penalty \(\lambda\) .
The dataset was partitioned into K = 10 subsets. The first subset contained the 22 participants with prevalent dementia at the time of data acquisition as well as their 220 matched controls; the remaining nine contained a random sampling of the rest of the dataset, with the requirement that each subset contained ten controls per case.
For each outer fold of CV, one subset was held out as a test set while the remaining nine subsets constituted a train-set. Note that the first subset was never used as a test set and only nine folds of outer cross-validation were actually performed. Therefore, data from the 22 participants who had a prevalent diagnosis of dementia at the time of data acquisition were only used to train the model, whereas the performance of the model exclusively refers to its ability to predict a future dementia diagnosis in those who did not yet have a diagnosis at the time of data acquisition.
For each of these nine outer folds of CV, the train-set was randomly partitioned into K = 5 inner subsets, again with the requirement that each inner subset contained ten controls per case. Thus, five folds of inner CV were performed. Each of these five inner folds was repeated for a different value of \(\alpha\) , ranging from zero to one in increments of 0.1. Glmnet automatically uses a range of 100 \(\lambda\) values every time a model is estimated. A different ROC curve was generated for each possible combination of hyperparameters, and for each inner fold of CV. The AUC was averaged across the five inner folds. The combination of hyperparameters that generated the maximum average AUC were then used for a model trained on the entire train-set and applied to the originally held out test set. At the end of the procedure, nine AUC curves were generated, one for each outer fold of CV. The mean AUC from these nine ROC curves was used as the final AUC.
As K -fold CV is sensitive to the way that the data is partitioned, the entire procedure described above was performed nine times, with a different random partitioning of data each time. The median AUC from these nine iterations is reported as the main result with the minimum and maximum AUC reported in brackets. The ROC curves from all nine iterations are plotted in Fig. 4 .
The above analysis was also performed using nested leave-one-out CV instead of K -fold CV, to generate a robust and unique participant-specific probability of dementia. We call this participant-specific value ‘effective connectivity (EC) index’ and it was used for subsequent analyses on individual differences (see the ‘Volumetric data analysis’ and ‘Modifiable risk factors analysis’ sections below).
Prognosticator
We trained a prognosticator model to test whether effective connectivity features could also be used to predict when these individuals got their dementia diagnosis. A group-level effective connectivity matrix was computed, using the PEB framework with Bayesian model reduction, as described above, but this time only the dementia cases were included in the analysis. The second column in the between-participants design matrix was not a column of ones and zeros to represent cases and controls, but rather a continuous variable that was computed as date of MRI acquisition subtracted from the date of dementia diagnosis (that is, how long, in years, until a dementia diagnosis). The value was negative if the participant already had a dementia diagnosis at the time of data acquisition. One participant, with prevalent dementia at the time of data collection, was excluded from this analysis as there was no reliable date of their past dementia diagnosis. Of the group-level parameters that model differences in effective connectivity as a function of the time until diagnosis, we selected all parameters with a posterior probability of at least 99% of being non-zero.
We then trained an elastic-net regularized linear regression model using the same K -fold cross-validation scheme as described above for the classifier. However, in this analysis, we wanted to assess the ability of the prognosticator to predict both positive and negative time until dementia diagnosis. The model was therefore tested on the cases with prevalent dementia at the time of data acquisition, and therefore all K = 10 subsets were used as test sets and ten folds of outer CV were performed. Hyperparameters \(\alpha\) and \(\lambda\) were tuned by minimizing the squared error between predictions and true values. Performance was evaluated as the Spearman correlation coefficient between final model predictions are true values. As above, the entire procedure was iterated nine times, the final reported result was the median Spearman coefficient across the nine iterations.
Volumetric data analysis
We repeated the above analyses to see how useful effective connectivity parameters were at making predictions about dementia compared to other MRI-based features, but this time using volumetric data features from structural MRI instead. We used pre-existing volume data from UKB’s imaging-derived phenotype database 80 . Specifically, we used the following 18 hippocampal subsegmental volumes (segmented using FreeSurfer): body of CA1, head of CA1, body of CA3, head of CA3, body of CA4, head of CA4, molecular layer of hippocampal body, molecular layer of hippocampal head, parasubiculum, presubiculum body, presubiculum head, subiculum body, subiculum head, whole hippocampal tail, whole hippocampal body, whole hippocampal head, whole hippocampus and hippocampal fissure. We also used two additional gray matter volumes from UKB’s imaging-derived phenotype database, segmented using FMRIB’s automated segmentation tool (FAST): anterior division of parahippocampal gyrus and posterior division of parahippocampal gyrus. Volumes were used from both the left and right hemispheres, and thus a total of 40 features were used.
Each feature was normalized by total intracranial volume. We then trained regularized logistic regression and linear regression models on this volumetric data using exactly the same cross-validation procedures that we used for the effective connectivity data features, as described above.
We also tested for an association between effective connectivity index and volumetric data. We took the mean across all 40 subsegmental volumes and fit a weighted linear regression model using fitglm in MATLAB to see whether average volume was associated with effective connectivity index. Individuals with dementia were upweighted and control participants were downweighed such that cases and controls made equal contributions to the regression model. We then ran 40 separate post-hoc regressions where the predictor variable was each individual subsegmental volume. Only the regression models that yielded the three highest R 2 values are reported.
Functional connectivity analysis
We estimated functional connectivity matrices for each participant to compare predictions based on effective connectivity to an alternative rs-fMRI metric. For this analysis we used the same BOLD time-series that were used for the DCM analysis. For each possible pair of ROIs, a Fisher z -transformed Pearson correlation coefficient was computed between the BOLD time-series from these two ROIs. This generated a 10 × 10 functional connectivity matrix for each participant. As this matrix is symmetrical, duplicate elements were removed and the diagonal elements (self-connections) were also removed. This resulted in 45 functional connectivity values. We then trained regularized logistic regression and linear regression models on these functional connectivity values using exactly the same cross-validation procedures that we used for the effective connectivity data features, as described above.
Cognitive data analysis
To assess the cognitive profile of the cases and controls in this study, we utilized UKB data from touchscreen cognitive function tests. Multiple cognitive tests were performed but only four tests were deemed to have sufficient data for analysis. For the other cognitive tests, at least 30% of our analyzed participants had missing data. The four cognitive tests that we used for analysis were assessments of visual declarative memory, processing speed, verbal and numerical reasoning and prospective memory. Missing data were imputed with the median across all participants. Details of the cognitive tests and performance of cases and controls in each of the four tests can be seen in Supplementary Table 3 .
We found significant differences between cases and controls in reaction time, fluid intelligence and prospective memory, with controls performing better in all three tasks. To assess how well these cognitive data could predict future dementia diagnosis and time until dementia diagnosis, we trained regularized logistic regression and linear regression models on these cognitive outcome measures using exactly the same cross-validation procedures that we used for the effective connectivity data features, as described above.
We also constructed a composite score of cognitive ability by running a principal components analysis on the four test scores. We took individual scores for the first principal component, which loaded negatively on number of errors in the pairs matching test and reaction time, and loaded positively on scores in the fluid intelligence and prospective memory task (that is, a higher score on this principal component indicated better performance across the four tasks).
Modifiable risk factors analysis
We investigated which modifiable risk factors were associated with dementia-related changes in DMN effective connectivity using multiple multivariable linear regression models. We constructed a variable for each of the 12 modifiable risk factors identified in the 2020 Lancet commission on dementia 1 . History of hypertension, diabetes, smoking, depression, physical inactivity, traumatic brain injury and hearing loss, absence of secondary education, and residence in a highly polluted neighborhood (top decile) were coded as binary variables. Body mass index, weekly alcohol consumption and social isolation were coded as continuous numerical variables. The social isolation variable was constructed with data from three questions, which participants answered as part of the touchscreen session at baseline data collection. These three questions assessed: (1) weekly attendance at social leisure activities (binary); (2) an estimated number of visits from friends or family within a year (continuous numerical); and (3) an estimated number of times the participant felt able to confide in someone close to them within a year (continuous numerical). We ran a principal components analysis on these three variables and took individual scores for the first principal component, which loaded negatively on all three variables (that is, a higher score on this principal component indicated greater social isolation). Traumatic brain injury was excluded from the subsequent regression analyses as there were only nine positive cases across the entire sample. This left 11 modifiable risk factors for analysis. For all variables, missing data were imputed with the median across all participants (see Supplementary Table 2 for numbers of missing data points). Data acquisition and processing were identical for cases and controls. Supplementary Table 4 shows details of the raw UKB variables used to derive the variables in this analysis.
For each of the 11 modifiable risk factors, as well as AD PRS, a weighted linear regression model was estimated using fitglm in MATLAB, where the risk factor of interest was the predictor variable, and effective connectivity index was the response variable. The effective connectivity index is simply the probability of dementia outputted from the case-control classifier trained with leave-one-out cross-validation. A higher value here indicates that the participant’s overall effective connectivity pattern conforms more to a dementia-like phenotype than a control-like phenotype. Age, sex and Townsend social deprivation score were included as covariates of no-interest in each of the 12 linear regression models. Participants with dementia were upweighted and control participants were downweighed in the linear regression models, such that cases and controls made equal contributions to the regression models. A P -value was estimated for each of the 11 modifiable risk factors and for PRS, which was corrected for multiple comparisons using the Holm–Bonferroni method, to maintain a family wise error rate of 0.05.
A mediation analysis was performed, with social isolation as a predictor, effective connectivity index as a mediator and dementia incidence as a response variable (dummy-coded binary variable). Each regression model estimated in the mediation analysis included age, sex and Townsend social deprivation score as covariates of no-interest, and used weighted observations such that cases and controls contributed equally to the model. A P -value was estimated for the significance of the indirect path coefficient by generating a permutation-based null distribution. For each permutation, the dementia incidence variable was randomly shuffled and an indirect path coefficient was estimated. This was repeated 1,000 times to generate a null distribution of indirect path coefficients with which to evaluate the true indirect path coefficient magnitude.
Alzheimer’s disease polygenic risk score
The AD PRS was downloaded from the UKB standard PRS set 81 . The database comprises PRSs for 28 diseases and 25 traits for every UKB participant. Polygenic risk scores were derived from meta-analysis of multiple external genome-wide association study sources. Detailed methods for how PRSs were generated have already been extensively documented 81 and will not be reproduced here.
Ethics and inclusion statement
This research included local researchers throughout the research process. Roles and responsibilities were agreed amongst collaborators ahead of the research. This research involved no health, safety, security or other risk to participants or researchers.
Data access and ethics
This research was conducted using the UKB Resource under application no. 78867 (PI: C. Marshall). Informed written consent was obtained from all participants on enrollment in UKB and they were informed that they are free to withdraw their consent at any time, at which time their data would be censored and excluded from future analysis. Participants were offered compensation for reasonable travel expenses. The UKB has approval from the North West Multicentre Research Ethics Committee as a Research Tissue Bank (REC reference: 21/NW/0157).
Reporting summary
Further information on research design is available in the Nature Portfolio Reporting Summary linked to this article.
Data availability
Processed group-level DCM results are available at https://github.com/Wolfson-PNU-QMUL/UKB_DCM_dementia . Supplementary Table 4 contains UKB field names for UKB data variables analyzed in this study.
Code availability
MATLAB analysis code is available at https://github.com/Wolfson-PNU-QMUL/UKB_DCM_dementia .
Livingston, G. et al. Dementia prevention, intervention, and care: 2020 report of the Lancet Commission. Lancet 396 , 413–446 (2020).
Article PubMed PubMed Central Google Scholar
Boyle, P. A. et al. Attributable risk of Alzheimer’s dementia attributed to age-related neuropathologies. Ann. Neurol. 85 , 114–124 (2019).
Article PubMed Google Scholar
Jack, C. R. Jr. et al. Tracking pathophysiological processes in Alzheimer’s disease: an updated hypothetical model of dynamic biomarkers. Lancet Neurol. 12 , 207–216 (2013).
Ibrahim, B. et al. Diagnostic power of resting-state fMRI for detection of network connectivity in Alzheimer’s disease and mild cognitive impairment: a systematic review. Hum. Brain Mapp. 42 , 2941–2968 (2021).
van den Heuvel, M. P. & Hulshoff Pol, H. E. Exploring the brain network: a review on resting-state fMRI functional connectivity. Eur. Neuropsychopharmacol. 20 , 519–534 (2010).
Berron, D., van Westen, D., Ossenkoppele, R., Strandberg, O. & Hansson, O. Medial temporal lobe connectivity and its associations with cognition in early Alzheimer’s disease. Brain 143 , 1233–1248 (2020).
Dunn, C. J. et al. Deficits in episodic memory retrieval reveal impaired default mode network connectivity in amnestic mild cognitive impairment. Neuroimage Clin. 4 , 473–480 (2014).
Putcha, D. et al. Tau and the fractionated default mode network in atypical Alzheimer’s disease. Brain Commun. 4 , fcac055 (2022).
Badhwar, A. et al. Resting-state network dysfunction in Alzheimer’s disease: a systematic review and meta-analysis. Alzheimers Dement. 8 , 7373–8585 (2017).
Franzmeier, N. et al. The BDNFVal66Met SNP modulates the association between beta-amyloid and hippocampal disconnection in Alzheimer’s disease. Mol. Psychiatry 26 , 614–628 (2021).
Sheline, Y. I. et al. APOE4 allele disrupts resting state fMRI connectivity in the absence of amyloid plaques or decreased CSF Aβ42. J. Neurosci. 30 , 17035–17040 (2010).
Westlye, E. T., Lundervold, A., Rootwelt, H., Lundervold, A. J. & Westlye, L. T. Increased hippocampal default mode synchronization during rest in middle-aged and elderly APOE epsilon4 carriers: relationships with memory performance. J. Neurosci. 31 , 7775–7783 (2011).
Chhatwal, J. P. et al. Impaired default network functional connectivity in autosomal dominant Alzheimer disease. Neurology 81 , 736–744 (2013).
Wang, L. et al. Alzheimer disease family history impacts resting state functional connectivity. Ann. Neurol. 72 , 571–577 (2012).
Drzezga, A. et al. Neuronal dysfunction and disconnection of cortical hubs in non-demented subjects with elevated amyloid burden. Brain 134 , 1635–1646 (2011).
Hedden, T. et al. Disruption of functional connectivity in clinically normal older adults harboring amyloid burden. J. Neurosci. 29 , 12686–12694 (2009).
Ingala, S. et al. Amyloid-driven disruption of default mode network connectivity in cognitively healthy individuals. Brain Commun. 3 , fcab201 (2021).
Lim, H. K. et al. Regional amyloid burden and intrinsic connectivity networks in cognitively normal elderly subjects. Brain 137 , 3327–3338 (2014).
Sheline, Y. I. et al. Amyloid plaques disrupt resting state default mode network connectivity in cognitively normal elderly. Biol. Psychiatry 67 , 584–587 (2010).
Van Hooren, R. W. E., Riphagen, J. M., Jacobs, H. I. L. & Alzheimer’s disease neuroimaging, I. Inter-network connectivity and amyloid-beta linked to cognitive decline in preclinical Alzheimer’s disease: a longitudinal cohort study. Alzheimers Res. Ther. 10 , 88 (2018).
Sheline, Y. I. & Raichle, M. E. Resting state functional connectivity in preclinical Alzheimer’s disease. Biol. Psychiatry 74 , 340–347 (2013).
Warren, J. D. et al. Molecular nexopathies: a new paradigm of neurodegenerative disease. Trends Neurosci. 36 , 561–569 (2013).
Mars, R. B. et al. On the relationship between the ‘default mode network’ and the ‘social brain. Front. Hum. Neurosci. 6 , 189 (2012).
Shulman, G. L. et al. Common blood flow changes across visual tasks: II. Decreases in cerebral cortex. J. Cogn. Neurosci 9 , 648–663 (1997).
Yeshurun, Y., Nguyen, M. & Hasson, U. The default mode network: where the idiosyncratic self meets the shared social world. Nat. Rev. Neurosci. 22 , 181–192 (2021).
Menon, V. 20 years of the default mode network: a review and synthesis. Neuron 111 , 2469–2487 (2023).
Eyler, L. T. et al. Resting state abnormalities of the default mode network in mild cognitive impairment: a systematic review and meta-analysis. J. Alzheimers Dis. 70 , 107–120 (2019).
Ibnidris, A. et al. Investigating the association between polygenic risk scores for Alzheimer’s disease with cognitive performance and intrinsic functional connectivity in healthy adults. Front. Aging Neurosci. 14 , 837284 (2022).
Stephan, K. E. & Friston, K. J. Analyzing effective connectivity with functional magnetic resonance imaging. Wiley Interdiscip. Rev. Cogn. Sci. 1 , 446–459 (2010).
Friston, K. J., Kahan, J., Biswal, B. & Razi, A. A DCM for resting state fMRI. Neuroimage 94 , 396–407 (2014).
Tsvetanov, K. A. et al. Activity and connectivity differences underlying inhibitory control across the adult life span. J. Neurosci. 38 , 7887–7900 (2018).
Benhamou, E. et al. The neurophysiological architecture of semantic dementia: spectral dynamic causal modelling of a neurodegenerative proteinopathy. Sci. Rep. 10 , 16321 (2020).
Thomas, G. E. C. et al. Changes in both top-down and bottom-up effective connectivity drive visual hallucinations in Parkinson’s disease. Brain Commun. 5 , fcac329 (2023).
Wu, X. et al. Altered default mode network connectivity in Alzheimer’s disease–a resting functional MRI and Bayesian network study. Hum. Brain Mapp. 32 , 1868–1881 (2011).
Li, R. et al. Bayesian network analysis reveals alterations to default mode network connectivity in individuals at risk for Alzheimer’s disease. PLoS ONE 8 , e82104 (2013).
Nie, Y. et al. Spectral dynamic causal modelling of resting-state fMRI: an exploratory study relating effective brain connectivity in the default mode network to genetics. Stat. Appl. Genet. Mol. Biol. https://doi.org/10.1515/sagmb-2019-0058 (2020).
Scherr, M. et al. Effective connectivity in the default mode network is distinctively disrupted in Alzheimer’s disease-A simultaneous resting-state FDG-PET/fMRI study. Hum. Brain Mapp. 42 , 4134–4143 (2021).
Huang, J., Jung, J. Y. & Nam, C. S. Estimating effective connectivity in Alzheimera’s disease progression: a dynamic causal modeling study. Front. Hum. Neurosci. 16 , 1060936 (2022).
Mohammadian, F. et al. Effective connectivity evaluation of resting-state brain networks in Alzheimera’s disease, amnestic mild cognitive impairment, and normal aging: an exploratory study. Brain Sci. 13 , 265 (2023).
Luo, X. et al. Altered effective connectivity anchored in the posterior cingulate cortex and the medial prefrontal cortex in cognitively intact elderly APOE epsilon4 carriers: a preliminary study. Brain Imaging Behav. 13 , 270–282 (2019).
Penny, W., Iglesias-Fuster, J., Quiroz, Y. T., Lopera, F. J. & Bobes, M. A. Dynamic causal modeling of preclinical autosomal-dominant Alzheimer’s disease. J. Alzheimers Dis. 65 , 697–711 (2018).
Lambert, C. et al. Identifying preclinical vascular dementia in symptomatic small vessel disease using MRI. Neuroimage Clin. 19 , 925–938 (2018).
Sims, J. R. et al. Donanemab in early symptomatic Alzheimer disease: The TRAILBLAZER-ALZ 2 randomized clinical trial. JAMA 330 , 512–527 (2023).
van Dyck, C. H. et al. Lecanemab in early Alzheimer’s disease. N. Engl. J. Med. 388 , 9–21 (2023).
Roberts, R. O. et al. Prevalence and outcomes of amyloid positivity among persons without dementia in a longitudinal, population-based setting. JAMA Neurol. 75 , 970–979 (2018).
Ashton, N. J. et al. Diagnostic accuracy of a plasma phosphorylated tau 217 immunoassay for Alzheimer disease pathology. JAMA Neurol. 81 , 255–263 (2024).
Palmqvist, S. et al. Prediction of future Alzheimer’s disease dementia using plasma phospho-tau combined with other accessible measures. Nat. Med. 27 , 1034–1042 (2021).
Vogel, J. W. et al. Brain properties predict proximity to symptom onset in sporadic Alzheimer’s disease. Brain 141 , 1871–1883 (2018).
Stamatakis, E. et al. Is Cohort representativeness passe? Poststratified associations of lifestyle risk factors with mortality in the UK Biobank. Epidemiology 32 , 179–188 (2021).
Fry, A. et al. Comparison of sociodemographic and health-related characteristics of UK Biobank participants with those of the general population. Am. J. Epidemiol. 186 , 1026–1034 (2017).
Lyall, D. M. et al. Quantifying bias in psychological and physical health in the UK Biobank imaging sub-sample. Brain Commun. 4 , fcac119 (2022).
Selvackadunco, S. et al. Comparison of clinical and neuropathological diagnoses of neurodegenerative diseases in two centres from the Brains for Dementia Research (BDR) cohort. J. Neural Transm. 126 , 327–337 (2019).
Payton, N. M. et al. Combining cognitive, genetic, and structural neuroimaging markers to identify individuals with increased dementia risk. J. Alzheimers Dis. 64 , 533–542 (2018).
Mirabnahrazam, G. et al. Predicting time-to-conversion for dementia of Alzheimer’s type using multi-modal deep survival analysis. Neurobiol. Aging 121 , 139–156 (2023).
Li, W., Mai, X. & Liu, C. The default mode network and social understanding of others: what do brain connectivity studies tell us. Front. Hum. Neurosci. 8 , 74 (2014).
Arioli, M., Cattaneo, Z., Ricciardi, E. & Canessa, N. Overlapping and specific neural correlates for empathizing, affective mentalizing, and cognitive mentalizing: a coordinate-based meta-analytic study. Hum. Brain Mapp. 42 , 4777–4804 (2021).
Vaccaro, A. G. & Fleming, S. M. Thinking about thinking: a coordinate-based meta-analysis of neuroimaging studies of metacognitive judgements. Brain Neurosci. Adv. 2 , 2398212818810591 (2018).
Liu, J. et al. Impaired adult myelination in the prefrontal cortex of socially isolated mice. Nat. Neurosci. 15 , 1621–1623 (2012).
Makinodan, M., Rosen, K. M., Ito, S. & Corfas, G. A critical period for social experience-dependent oligodendrocyte maturation and myelination. Science 337 , 1357–1360 (2012).
Makinodan, M. et al. Effects of the mode of re-socialization after juvenile social isolation on medial prefrontal cortex myelination and function. Sci. Rep. 7 , 5481 (2017).
Grosse Wiesmann, C., Schreiber, J., Singer, T., Steinbeis, N. & Friederici, A. D. White matter maturation is associated with the emergence of Theory of Mind in early childhood. Nat. Commun. 8 , 14692 (2017).
Ereira, S. et al. Social training reconfigures prediction errors to shape self–other boundaries. Nat. Commun. 11 , 3030 (2020).
Marioni, R. E. et al. Social activity, cognitive decline and dementia risk: a 20-year prospective cohort study. BMC Public Health 15 , 1089 (2015).
Salinas, J. et al. Associations between social relationship measures, serum brain-derived neurotrophic factor, and risk of stroke and dementia. Alzheimers Dement. 3 , 229–237 (2017).
Article Google Scholar
Shen, C. et al. Associations of social isolation and loneliness with later dementia. Neurology 99 , e164–e175 (2022).
Smith, L. et al. Social participation and mild cognitive impairment in low- and middle-income countries. Prev. Med. 164 , 107230 (2022).
Duan, Y. et al. Psychosocial interventions for Alzheimer’s disease cognitive symptoms: a Bayesian network meta-analysis. BMC Geriatr. 18 , 175 (2018).
Liu, T., Spector, A., Mograbi, D. C., Cheung, G. & Wong, G. H. Y. Changes in default mode network connectivity in resting-state fMRI in people with mild dementia receiving cognitive stimulation therapy. Brain Sci. 11 , 1137 (2021).
Yuill, N. & Hollis, V. A systematic review of cognitive stimulation therapy for older adults with mild to moderate dementia: an occupational therapy perspective. Occup. Ther. Int. 18 , 163–186 (2011).
Vossel, K. A. et al. Seizures and epileptiform activity in the early stages of Alzheimer disease. JAMA Neurol. 70 , 1158–1166 (2013).
van Nifterick, A. M. et al. Resting-state oscillations reveal disturbed excitation-inhibition ratio in Alzheimer’s disease patients. Sci. Rep. 13 , 7419 (2023).
Busche, M. A. et al. Tau impairs neural circuits, dominating amyloid-beta effects, in Alzheimer models in vivo. Nat. Neurosci. 22 , 57–64 (2019).
Wu, X. et al. A triple network connectivity study of large-scale brain systems in cognitively normal APOE4 carriers. Front. Aging Neurosci. 8 , 231 (2016).
Buckley, R. F. et al. Functional network integrity presages cognitive decline in preclinical Alzheimer disease. Neurology 89 , 29–37 (2017).
Almgren, H. et al. Variability and reliability of effective connectivity within the core default mode network: a multi-site longitudinal spectral DCM study. Neuroimage 183 , 757–768 (2018).
Zeidman, P. et al. A guide to group effective connectivity analysis, part 1: first level analysis with DCM for fMRI. Neuroimage 200 , 174–190 (2019).
Zeidman, P. et al. A guide to group effective connectivity analysis, part 2: second level analysis with PEB. Neuroimage 200 , 12–25 (2019).
Friston, K. J. et al. Bayesian model reduction and empirical Bayes for group (DCM) studies. Neuroimage 128 , 413–431 (2016).
Novelli, L., Friston, K. & Razi, A. Spectral dynamic causal modelling: a didactic introduction and its relationship with functional connectivity. Netw. Neurosci. 8 , 178–202 (2023).
Alfaro-Almagro, F. et al. Image processing and Quality Control for the first 10,000 brain imaging datasets from UK Biobank. Neuroimage 166 , 400–424 (2018).
Thompson, D. J. et al. UK Biobank release and systematic evaluation of optimised polygenic risk scores for 53 diseases and quantitative traits. Preprint at medRxiv https://doi.org/10.1101/2022.06.16.22276246 (2022).
Download references
Acknowledgements
We thank all staff at the Centre for Preventive Neurology for helpful comments on the presentation of these results at a laboratory meeting. This research made use of Queen Mary’s Apocrita high-performance computing facility, supported by QMUL Research-IT. We also acknowledge the assistance of the ITS Research team at Queen Mary’s. UKB data access was funded by a grant from the Tom and Sheila Springer Charity. S.E. received funding from the NHSE as part of the Specialized Foundation Programme (SFP). The funders had no role in study design, data collection and analysis, decision to publish or preparation of the manuscript.
Author information
Authors and affiliations.
Centre for Preventive Neurology, Queen Mary University of London, London, UK
Sam Ereira, Sheena Waters & Charles R. Marshall
Max Planck UCL Centre for Computational Psychiatry and Ageing Research, University College London, London, UK
Turner Institute for Brain and Mental Health, School of Psychological Sciences, Monash University, Clayton, Victoria, Australia
Wellcome Centre for Human Neuroimaging, Institute of Neurology, University College London, London, UK
CIFAR Azrieli Global Scholars Program, CIFAR, Toronto, Ontario, Canada
Department of Neurology, Royal London Hospital, London, UK
Charles R. Marshall
You can also search for this author in PubMed Google Scholar
Contributions
C.R.M. conceived the project and edited the paper. S.E. designed the analysis pipeline, analyzed the data and wrote the initial draft of the paper. S.W. conducted the case-control matching, acquired the UKB data and provided comments on the initial draft of the paper. A.R. advised on the DCM analyses and provided comments on the initial draft of the paper.
Corresponding author
Correspondence to Charles R. Marshall .
Ethics declarations
Competing interests.
The authors declare no competing interests.
Peer review
Peer review information.
Nature Mental Health thanks Michel Grothe, Timothy Rittman and the other, anonymous reviewer(s) for their contribution to the peer review of this work.
Additional information
Publisher’s note Springer Nature remains neutral with regard to jurisdictional claims in published maps and institutional affiliations.
Supplementary Information
Supplementary information.
Supplementary Tables 1–4.
Reporting summary.
Rights and permissions.
Open Access This article is licensed under a Creative Commons Attribution 4.0 International License, which permits use, sharing, adaptation, distribution and reproduction in any medium or format, as long as you give appropriate credit to the original author(s) and the source, provide a link to the Creative Commons licence, and indicate if changes were made. The images or other third party material in this article are included in the article’s Creative Commons licence, unless indicated otherwise in a credit line to the material. If material is not included in the article’s Creative Commons licence and your intended use is not permitted by statutory regulation or exceeds the permitted use, you will need to obtain permission directly from the copyright holder. To view a copy of this licence, visit http://creativecommons.org/licenses/by/4.0/ .
Reprints and permissions
About this article
Cite this article.
Ereira, S., Waters, S., Razi, A. et al. Early detection of dementia with default-mode network effective connectivity. Nat. Mental Health (2024). https://doi.org/10.1038/s44220-024-00259-5
Download citation
Received : 26 October 2023
Accepted : 25 April 2024
Published : 06 June 2024
DOI : https://doi.org/10.1038/s44220-024-00259-5
Share this article
Anyone you share the following link with will be able to read this content:
Sorry, a shareable link is not currently available for this article.
Provided by the Springer Nature SharedIt content-sharing initiative
Quick links
- Explore articles by subject
- Guide to authors
- Editorial policies
Sign up for the Nature Briefing newsletter — what matters in science, free to your inbox daily.


An official website of the United States government
The .gov means it’s official. Federal government websites often end in .gov or .mil. Before sharing sensitive information, make sure you’re on a federal government site.
The site is secure. The https:// ensures that you are connecting to the official website and that any information you provide is encrypted and transmitted securely.
- Publications
- Account settings
Preview improvements coming to the PMC website in October 2024. Learn More or Try it out now .
- Advanced Search
- Journal List
- HHS Author Manuscripts

Case Study 3: A 58-Year-Old Woman Referred for Evaluation of Suspected Alzheimer Dementia
Case presentation.
A 58-year-old left-handed woman with 12 years of education was referred for further evaluation and management of progressive cognitive dysfunction. A diagnosis of Alzheimer disease (AD) was suspected by the referring neurologist on the basis of an MRI demonstrating mild temporal atrophy, EEG demonstrating bitemporal slowing, positive cerebrospinal fluid (CSF) AD biomarkers, and apolipoprotein E ( APOE ) ε4, ε4 genotype. The patient had a 4-year history of progressive cognitive symptoms. Early symptoms included difficulties recalling recent events and information, sustaining attention, and learning new tasks. She was repeatedly disoriented to the time of day; for example, she would get dressed for the day in the middle of the night. She had difficulties finding words and expressing her thoughts, with a decline in the richness of her vocabulary.
An initial clinical interview revealed that the patient had become increasingly withdrawn from family and friends. Over the year prior to evaluation at our center, she developed an array of neuropsychiatric symptoms, including depression, anxiety, and recurrent, well-formed visual hallucinations of unfamiliar people in her house. She did not feel threatened or bothered by these people and retained insight that others could not see them.
The patient had last worked as an administrative assistant approximately 3 years prior to evaluation at our center and was unable to obtain new work because of her cognitive symptoms. More recently, her husband assumed responsibility for over-seeing administration of her medications and for shopping and most meal planning and preparation. She continued to drive intermittently and to prepare breakfast independently. She remained independent in basic activities of daily living, including showering, dressing, eating, toileting, and mobility.
The patient’s mother and father lived into their 70s and 80s, respectively, and died from cancer, without any history of progressive cognitive impairment or dementia. A maternal aunt who died in her 70s had progressive cognitive impairment starting in her late 50s.
- Questions: What are the diagnostic considerations based on the history? Is this presentation suggestive of AD? Would additional history be helpful?
Insidious onset and gradual progression of cognitive symptoms over the course of 4 years raises concern for a neurodegenerative disorder. In this type of scenario, it is useful to derive a three-tiered diagnostic formulation comprising neurodegenerative clinical syndrome, severity, and suspected underlying neuropathology ( 1 , 2 ). Setting aside the reports of the neuroimaging, CSF, and genetic results, aspects of the patient’s history suggesting changes in episodic memory, attention, executive function, and word retrieval could suggest a multidomain, amnesic syndrome as frequently occurs in the context of AD neuropathology. However, recurrent, well-formed visual hallucinations are very uncommon with isolated AD neuropathology and suggestive of contributions from Lewy body disease (LBD) neuropathology, as occurs in association with syndromic dementia with Lewy bodies (DLB), Parkinson disease (PD), or PD with dementia (PDD) ( 3 ). It would be useful to know whether this patient has additional history indicating other core clinical features of a DLB syndrome, including fluctuating cognition with pronounced variations in attention and alertness; REM sleep behavior disorder (RBD), suggested by dream enactment behaviors; and motor symptoms potentially reflecting parkinsonism ( 4 , 5 ). Instruments such as the Mayo Fluctuations Scale and the Queen Square Visual Hallucination Inventory provide useful questions for assessing the range of fluctuation phenomena and minor and major visual hallucinations and illusions possible in DLB ( 6 , 7 ) ( Figure 1 ). Additional supportive clinical features would include various other symptoms in the domains of motor function, sleep, neuropsychiatric function, sensory processing, and autonomic function ( 8 – 11 ).

Clinical features of mild cognitive impairment and dementia with Lewy bodies a
a Asterisks denote essential (***), core (**), and supportive (*) clinical features per consensus diagnostic criteria ( 4 , 5 ). †, item from the Mayo Fluctuations Scale ( 6 ); ‡, item from the Queen Square Visual Hallucination Inventory ( 7 ); MCI, mild cognitive impairment; OSA, obstructive sleep apnea; PLMS, periodic limb movements of sleep; REM, rapid eye movement; RLS, restless legs syndrome.
Additional history revealed that the patient’s husband observed her to have periods of being in a “trance-like” state, as well as drowsiness and an increased tendency to sleep in the daytime. She was noted to have recurrent episodes of “acting out her nightmares,” at times kicking and screaming, dating back to the onset of her cognitive symptoms. Her walking had slowed, and her voice had become softer. She was slower and less coordinated when using her hands, her handwriting became smaller, and she developed intermittent tremors of her hands and arms when using them. She experienced constipation with increasing frequency and severity.
- Question: What are the aims of the cognitive and neurological examinations in this context?
In the context of a history highly suggestive of DLB, one can increase diagnostic confidence by establishing a suggestive neuropsychological profile or by confirming features of parkinsonism on examination. DLB is frequently associated with early impairments in attention, executive function, and visuospatial processing ( 12 – 14 ). To assess parkinsonism, it is useful to gain familiarity with elements of the motor examination section of the Movement Disorders Society–sponsored revision of the Unified Parkinson’s Disease Rating Scale, including assessment of speech, facial expression, rigidity, finger tapping, hand movements (opening and closing), hand pronation-supination, toe tapping, leg agility (foot stomping), arising from chair, gait, posture, postural stability, global spontaneity of movement, and presence or absence of postural, kinetic, or rest tremors of the hands ( 15 ). PD syndrome is more likely to be associated with typical parkinsonism, i.e., early asymmetrical “pill-rolling” resting tremor, limb bradykinesia and rigidity that tend to be responsive to levodopa, and minimal to no early postural instability. Although typical parkinsonism can occur in DLB, atypical features occur more frequently, including the absence of resting tremor, the presence of postural-kinetic or mixed tremor, less prominent and more symmetrical early bradykinesia and rigidity, more prominent early postural instability, and reduced responsiveness to levodopa ( 16 – 18 ). Despite these distinctions, it is noteworthy that all symptomatic features of DLB can occur in PDD and vice versa, with the arbitrary distinguishing feature between the syndromes being whether cognitive dysfunction develops prior to or concurrently with parkinsonism (as in DLB and as was observed in this patient) or following parkinsonism (as in PDD) ( 4 ). Considering PD, PDD, and DLB as syndromes under the umbrella term “Lewy body disorders” allows for variability in presentations along a spectrum, from individuals with predominantly motor symptoms to those with predominantly cognitive symptoms ( 19 ). These disorders all share a common neuropathological substrate that is indistinguishable at the microscopic level but appears to differ in terms of topographical distribution and spreading patterns ( 20 ).
This patient’s mental status examination revealed grossly apparent psychomotor slowing. She scored a 24/30 on the Mini-Mental State Examination, missing 2 points on orientation to year and day of the week, 2 points on the three-item delayed word recall test (obtaining both with a category cue), and 1 point for poor pentagon copy ( 21 ). She was given a subset of the Hooper Visual Organization Test, on which she correctly identified seven of 13 objects represented in line drawings as puzzle pieces (suggesting a moderate level of visuospatial impairment) ( 22 ).
On elemental neurological examination, the patient had hypomimia, saccadic intrusions during smooth-pursuit eye movements, and slow, hypophonic speech. Strength was full in the proximal and distal muscles of the arms and legs. There was a postural-kinetic tremor of both hands, mild left-greater-than-right bradykinesia apparent on finger tapping and hand movements, and mild left-greater-than-right cogwheel rigidity in the arms. She rose from a chair easily, without the use of her arms. Her gait was mildly slow, with a narrow base and left-greater-than-right reduced arm swing. There was no retropulsion on pull testing.
Taken together, these examination results provided additional support for a DLB syndrome. The cognitive examination, though limited and nonspecific, provided some evidence of slow processing speed, impaired memory (at least at the level of retrieval), and visuospatial dysfunction. The motor examination provided unequivocal evidence of parkinsonism, some features that were typical, such as asymmetrical limb bradykinesia and cogwheel rigidity, and other features that were atypical, such as postural-kinetic tremor of the hands.
- Question: What initial tests and studies are indicated?
Structural neuroimaging of the brain, preferably with MRI, is a recommended component in the initial evaluation of suspected dementia ( 23 , 24 ). When specific neurodegenerative causes of dementia are under consideration, imaging serves at least two purposes. First, it helps to assess for evidence of alternative (nondegenerative) conditions that might account for or contribute to symptoms. Second, it helps to assess for atrophy in a topographical distribution suggestive of a neurodegenerative syndrome and/or neuropathology, which can be useful in cases with possible underlying AD or frontotemporal lobar degeneration (FTLD) neuropathological changes ( 25 ). In a case such as this one, with features suggesting a DLB syndrome, imaging can be useful to assess for alternative causes of parkinsonism, such as vascular disease. While relative preservation of medial temporal lobe (MTL) regions on structural neuroimaging represents a supportive biomarker for DLB, evidence of MTL atrophy does not preclude a diagnosis of DLB, particularly considering that a DLB clinical syndrome may be associated with mixed LBD and AD neuropathological changes ( 4 , 26 ).
In cases of suspected DLB with profound fluctuations in attention, focal dyscognitive seizures are included in the differential diagnosis. Here, an EEG can be useful to distinguish between epileptiform activity (which is consistent with seizures) and prominent posterior slow-wave activity with periodic fluctuations in the pre-alpha/theta range (which is another supportive biomarker for DLB) ( 4 ).
Otherwise, a standard laboratory evaluation including comprehensive metabolic profile (CMP), vitamin B12 level, TSH, and complete blood counts (CBC) would add value in screening for potential contributing factors.
This patient’s MRI, completed approximately 9 months prior to referral, demonstrated a mild degree of T2 hyper-intense signal changes in the periventricular, subcortical and juxtacortical white matter. There was no diffusion restriction, evidence of atrophy, or abnormal enhancement ( Figure 2 ). A routine EEG demonstrated “mild intermittent bitemporal irregular slowing with no focal or generalized epileptiform features.” There were no pertinent lab result abnormalities.
- Question: Are additional tests and studies indicated?
Given the presence of all four core clinical features, multiple supportive clinical features (constipation, anxiety, and depression), and a supportive biomarker (relative preservation of MTL structures on MRI) for DLB in this case, there was no strong rationale to obtain additional data to confirm the diagnosis. In cases in which a diagnosis of DLB is less clear, consideration may be given to obtaining what have been designated indicative biomarkers, including dopamine transporter SPECT or PET imaging for evidence of reduced uptake in the basal ganglia, 123 iodine-metaiodobenzylguanidine (MIBG) myocardial scintigraphy for evidence of low uptake, or polysomnography for confirmation of REM sleep without atonia ( 4 ).

MRI of the patient’s brain obtained 9 months prior to referral and approximately 3 years after the onset of symptoms
At the time of the patient’s initial presentation, when Lewy body features were less apparent, the referring neurologist had a higher level of suspicion for an amnesic syndrome with underlying AD neuropathology than for a DLB syndrome with underlying LBD or mixed LBD-AD neuropathology. Concern for AD in a patient with mild cognitive impairment or dementia at a young age at onset (<65 years) represents an appropriate indication for obtaining CSF beta-amyloid-42 (Aβ42), total tau, and phosphorylated tau levels, as occurred in this case ( 27 ). Molecular biomarkers of AD neuropathology can also provide value in etiologically mixed presentations in which AD might contribute to an illness in which other factors are also suspected, e.g., LBD, vascular neuropathology, or both ( 28 ). Indeed, CSF evidence of underlying AD in patients with DLB has been associated with more rapid cognitive decline ( 29 ).
CSF results were suggestive of AD, because Aβ42 was low at 437.2 pg/ml, total tau was elevated at 639.7 pg/ml, the Aβ42-to-total tau index was low at 0.44, and phosphorylated tau was elevated at 83 pg/ml.
- Question: Is genetic testing indicated?
Experts do not recommend routine clinical genetic testing for patients with DLB or patients with AD who lack a family history suggesting autosomal-dominant inheritance ( 4 , 30 ). In cases in which a familial autosomal-dominant neurodegenerative disorder is suggested by the clinical presentation and family history, consideration may be given for referral to a genetics counselor and potential testing for mutations in selected genes. Families with an autosomal-dominant disorder typically contain at least three affected individuals in two or more generations, with two of the individuals being first-degree relatives of the third individual, and the disorder usually involves an early age at onset. Although the presence of the APOE ε4 allele is a risk factor for both AD and DLB, its presence is neither sensitive to nor specific for either condition, and APOE genotyping is not recommended as part of a diagnostic evaluation ( 30 , 31 ). Although the utility of APOE genotyping for purposes other than diagnosis, such as clinical prognostication, has not been studied as extensively, the presence of an APOE ε4 allele in patients with DLB has been associated with greater severity of LBD neuropathology, independent of AD neuropathology, and shorter mean time between onset of cognitive symptoms and death ( 32 , 33 ).
Prior to referral, this patient tested negative for mutations in PSEN1 , PSEN2 , and APP . She was found to be a homozygous carrier of the APOE ε4 allele.
- Question: What would be an appropriate diagnostic formulation?
As reviewed above, ample evidence supported a syndromic diagnosis of DLB. The patient’s loss of independence in selected instrumental activities of daily living supported staging at a level of mild dementia. Regarding suspected neuropathology, several factors supported a prediction of mixed LBD and AD neuropathology. A clinical diagnosis of DLB has greater than 95% specificity for pathological confirmation of diffuse neocortical Lewy bodies ( 34 ). Clinical and neuropathological correlation studies likewise suggest that 60%–70% of cases with a clinical diagnosis of DLB have intermediate- or high-level AD copathology ( 26 ).
- Question: What are reasonable therapeutic considerations?
Because many different types of symptoms can arise in the context of DLB, it is helpful to take a systematic approach to therapeutic planning by reviewing the nature and severity of disease impact on cognition, neuropsychiatric health (e.g., mood, anxiety, and psychosis), sleep, motor function, and autonomic function. Considering the impact of symptoms within each of these domains on the patient’s quality of life and ability to carry out intended activities helps to identify and prioritize targets for pharmacological intervention ( 35 – 50 ) ( Table 1 ). Additional research is needed to establish stronger evidence for many symptomatic treatments ( 35 , 45 , 51 ). Importantly, nontrivial symptomatic benefits can frequently be obtained from discontinuing and avoiding non-essential medications with anticholinergic or dopamine receptor–blocking properties. Neuroleptic medications in particular should be avoided, given their propensity to precipitate severe, potentially life-threatening reactions ( 52 ).
Symptomatic pharmacotherapeutic considerations in dementia with Lewy bodies (DLB) a
At the time of referral, the symptoms with the greatest impact on this patient’s level of function and quality of life were those involving her cognitive function, mood, anxiety, and sleep. Although meaningful in terms of diagnosis, the patient’s formed visual hallucinations and motor symptoms were not causing significant distress at presentation and therefore did not warrant pharmacological treatment. Evidence from randomized controlled trials and meta-analyses supports the efficacy of cholinesterase inhibitors for cognitive and potentially for neuropsychiatric symptoms (including anxiety, delusions, and hallucinations) in DLB patients ( 53 , 54 ). Rivastigmine and donepezil have been studied more extensively than galantamine, and the results with rivastigmine and donepezil have been comparable ( 35 , 36 ). Either would be a reasonable choice for this patient, with monitoring for sleep-related and gastrointestinal side effects ( Table 1 ).
No systematic studies of antidepressants or anxiolytics have been conducted for treatment of depression or anxiety among patients with DLB ( 55 ). Selected selective serotonin reuptake inhibitors and serotonin-norepinephrine reuptake inhibitors, such as sertraline, escitalopram, and venlafaxine, may provide benefit, although they should be used carefully (i.e., “start low and go slow”) given their potential to cause or exacerbate gastrointestinal and sleep-related problems in this population. Patients with treatment-refractory depression may benefit from repetitive transcranial magnetic stimulation or electroconvulsive therapy ( 56 , 57 ).
In cases of RBD involving frequent, disruptive, or injurious behaviors, melatonin is often well tolerated and effective in reducing dream enactment behaviors ( 58 ). Standard practice is to start at 3 mg or 5 mg and to titrate weekly in increments of 3 mg or 5 mg as needed, up to 15 mg to 18 mg nightly ( 59 ). Clonazepam may be used as a second-line treatment in severe cases; however, the potential to exacerbate cognitive dysfunction and obstructive sleep apnea should be noted.
This patient was started on transdermal rivastigmine, titrated from 4.6 mg daily to 9.5 mg daily after 1 month, and there were notable reductions in her visual hallucinations and fluctuations in attention and alertness. She did not tolerate a further increase in dose to 13.3 mg daily on account of insomnia. She derived a moderate benefit from melatonin 6 mg nightly, with respect to reducing dream enactment behaviors. Sertraline, started approximately 6 months later, was effective in reducing her anxiety at a dose of 50 mg daily. Insomnia, initially nonresponsive to medications, including trazodone and mirtazapine, improved later with an increase in the dose of melatonin to 15 mg nightly.
The patient’s clinical status deteriorated steadily despite these early symptomatic improvements. Two years after her initial behavioral neurology evaluation and 6 years after onset of symptoms, she required full-time supervision and assistance to pick out clothes and dress, and she appeared frequently frustrated and agitated. Her husband derived benefit from visits with a social worker in the behavioral neurology and neuropsychiatry unit, who provided disease-specific counseling and education, assistance with completion of medical and financial legal documents, and recommendations on care arrangements and reviewed behavioral and environment-based strategies for managing neuropsychiatric symptoms. In-home services and support from additional family members allowed her husband to continue to work on a part-time basis.
In subsequent months, the patient’s course was complicated by intermittent problems, such as dehydration, constipation, and emotional lability, and by chronic problems, including the loss of communicative abilities and mobility. She was transitioned to a memory care unit and later to skilled nursing. She died at age 63, 5 years after the initial behavioral neurology presentation and 9 years after the onset of symptoms.
NEUROPATHOLOGY
The weight of the patient’s brain was 1,160 g, which is at the lower end of the normal range for an adult female. On gross examination of the right cerebral hemisphere (the left hemisphere was banked for future research), there was mild atrophy of the anterior temporal lobe and superior temporal gyrus and no significant atrophy of the frontal, parietal, and occipital lobes. Cut sections of the midbrain revealed pallor of the substantia nigra. Similar gross depigmentation was noted in the region of the locus coeruleus in the pons.
Histologically, microscopy with hematoxylin and eosin staining confirmed a loss of pigmented neurons in the substantia nigra, as well as abundant extraneuronal pigment. There were frequent small, round eosinophilic inclusions in the neurons of the substantia nigra, representing Lewy bodies ( Figure 3 ). These were also identified with synuclein immunohistochemistry (IHC) as higher-intensity brown areas, with horseradish peroxidase chromagen–reflecting, synuclein-positive intraneuronal inclusions, which are distinct from the smaller punctate pigmented endogenous neuromelanin granules. Numerous synuclein-positive neurites were also apparent. Moderate or frequent Lewy bodies were likewise seen in the medulla, locus coeruleus, nucleus basalis of Meynert, amygdala, hippocampus, and anterior cingulate cortex.

Histopathological findings for a patient with mixed Lewy body dementia and Alzheimer disease neuropathology a
a Microscopic views of the midbrain substantia nigra with hematoxylin and eosin (panel A) and synuclein immunohistochemistry (panel B) demonstrate Lewy bodies (arrows) and extraneuronal pigment (arrow-head). Tau immunohistochemistry on Ammon’s horn of the hippocampus indicates the presence of neurofibrillary tangles (panel C). β-amyloid immunohistochemistry of the frontal cortex indicates the presence of β-amyloid plaques (arrow) and cerebral amyloid angiopathy (arrowhead) (panel D).
LBD can be classified into one of three neuropathological types—brainstem predominant, limbic (transitional), or diffuse neocortical—by semiquantitative grading of Lewy body severity in selected regions in the brainstem, basal forebrain and limbic systems, and neocortex ( 60 ). In this case, the presence of moderate numbers of synuclein-positive inclusions in the frontal, temporal, and parietal cortices reflected relatively severe disease and merited the designation of diffuse neocortical LBD.
In the hippocampus, mild loss of pyramidal neurons was noted in the CA1 and CA2 regions. Higher-power microscopic examination of neurons demonstrated vacuolated cytoplasm with small eosinophilic granules, a finding that is termed granulovacuolar neurodegeneration. β-amyloid IHC revealed moderate to frequent plaques in the amygdala and hippocampus, as well as in the anterior cingulate, frontal, temporal, parietal, and occipital cortices. Tau IHC revealed frequent tau-positive neurofibrillary tangles (NFTs) and neurites within the hippocampus and amygdala; less frequent NFTs in the anterior cingulate, frontal, temporal, and parietal cortices; and rare NFTs in the occipital cortex. Taken together, these findings were consistent with stage IV neurofibrillary pathology according to the Braak and Braak ( 61 ) scheme and an intermediate level of AD neuropathological change per the National Institute on Aging–Alzheimer’s Association guidelines for the neuropathological assessment of AD ( 62 ).
β-amyloid deposition in the walls of scattered cerebral blood vessels in the hippocampus and temporal neocortex led to the additional neuropathological diagnosis of cerebral amyloid angiopathy of mild to moderate severity.
This case highlights the complexity of DLB as a multisystem disorder affecting cognition, neuropsychiatric function, sleep, motor function, and autonomic function. Symptoms in these different domains are variably present at varying levels of severity across individuals and at different time points along the disease course, which may lead to confusion in distinguishing DLB from PD in some cases (i.e., in patients who have prominent early parkinsonism) and from amnesic mild cognitive impairment or dementia due to suspected AD in others (i.e., in patients who do not have early parkinsonism). Recognizing prominent early impairments in attention and in executive and visuospatial function and proactively reviewing for fluctuations in arousal and attention, formed and minor visual hallucinations, dream enactment, and autonomic dysfunction promotes early consideration of DLB in cases without salient parkinsonism at presentation. It is useful for cognitive neurologists and neuropsychiatrists to develop a time-efficient examination of motor systems for elements of parkinsonism, to identify and characterize typical versus atypical features, and to grade severity. A systematic approach to reviewing symptom severity and the influence of symptoms on daily life across the domains potentially affected by DLB can help the clinician identify and prioritize potential targets for therapeutic intervention. Neuropsychiatric symptoms are very common, independent of motor symptoms, and tied to alterations in monoaminergic neurotransmitters ( 63 ). Published case series have suggested high rates of delusions (>75%), anxiety (65%–70%), depression (60%–65%), and apathy (55%–60%), with some symptoms tending to worsen with disease progression (delusions, hallucinations, and anxiety) and others tending to remain stable (depression) ( 8 , 52 ).
Neuropathologically, this case highlights the principle that the presence of multiple underlying neuropathologies is more the rule than the exception and that pathologies can have additive effects contributing to the severity and rate of progression of cognitive dysfunction ( 64 ). Patients with mixed LBD-AD neuropathology are more likely to have parkinsonism, visual hallucinations, RBD, and cognitive fluctuations, as well as faster rates of cognitive and functional decline than those with pure AD neuropathology ( 65 – 67 ). Patients with mixed LBD-AD neuropathology are more likely to have memory loss and less likely to have autonomic dysfunction than those with pure LBD ( 65 ). Important topics for ongoing research include understanding what factors contribute to the high coprevalence of AD and diffuse neocortical LBD pathology and understanding more fully how APOE ε4 might influence both.
Acknowledgments
This work was supported in part by NIH grants K08 AG065502 and T32 HL007627 (to Dr. Miller).
The authors report no financial relationships with commercial interests.
The authors have confirmed that details of the case have been disguised to protect patient privacy.
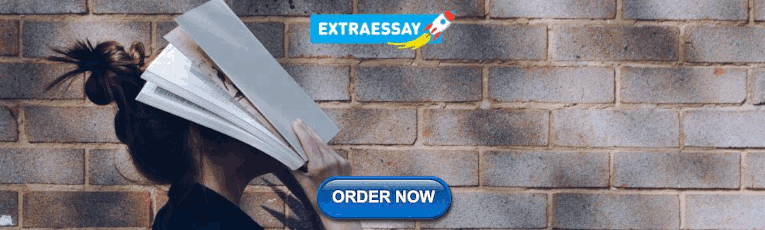
IMAGES
VIDEO
COMMENTS
Study with Quizlet and memorize flashcards containing terms like Betty is upset about visiting the HPP and insists her memory problems are nothing to worry about.. Betty states, "This is all really silly. I don't need to be here."Which response by the PN is the best?, Which information about Betty, obtained by the PN, is a risk factor(s) for Alzheimer's disease? (Select all that apply.), The ...
Prenatal Education 1 Healthy Pregnancy Checklist. Week 4 Sherpath Menstruation and Menopause. Week 4 Sherpath First Stage of Labor. Week 3 Sherpath Interpretation of Fetal and Uterine Monitoring. Week 3 Sherpath Fetal and Uterine Monitoring. collapse disease (advanced stages) case study thr imin meet the client section section section section ...
Case Study 1: A 55-Year-Old Woman With Progressive Cognitive, Perceptual, and Motor Impairments. Scott M. McGinnis, M.D., ... Onset of symptoms before age 65 should prompt consideration of syndromes in the spectrum of frontotemporal dementia (FTD) and atypical (nonamnesic) presentations of Alzheimer's disease (AD) (1, 2). This patient's ...
The Alzheimer's Association is committed to connecting clinicians to effective, evidence-based models of care that can be replicated in community settings. Two of these models — the UCLA Alzheimer's and Dementia Care program and the Age-Friendly Health Systems initiative — are detailed below. A dementia-specific model of care that ...
Early onset Alzheimer disease (EOAD) is a neurodegenerative dementing disorder that is relatively rare (<1% of all Alzheimer cases). Various genetic mutations of the presenilin 1 (PSEN1) and presenilin 2 (PSEN2) as well as the amyloid precursor protein (APP) gene have been implicated.Mutations of PSEN1 and PSEN2 alter γ-secretase enzyme that cleaves APP resulting in increase in the relative ...
hesi Alzheimer's Disease case study with rationales collapse disease (advanced stages) case study 1hr 1min meet the client section section section section ... s disease, several imaging tests provide data that show changes consistent with AD. Other problems that cause dementia may also be found via neuroimaging tests. Halter, M. (2018 ...
Students can complete a case study in small groups then discuss as a class. Have each group report to the class what they found to be the most interesting or valuable. Consider posing "What if" questions to expand on the case outcome. Have students write an SBAR of the case study and share with their peer(s).
HESI Case Studies: Complete PN Collection (2 Year Version) - Next Generation Version, 1st Edition. by HESI. HESI Case Studies. ISBN: 9781455741403. ... $0.00 with a valid access code. register. This product requires an instructor adoption and is not available for independent study. Related Products. Authors. HESI. $67.00. Or $0.00 with a valid ...
Dementia in young individuals can be a diagnostic challenge given its often non-specific symptoms and findings. As a consequence, patients with early-onset dementia are more often misdiagnosed than those with late-onset dementia. 1 Although there is a set of known clinical criteria for early-onset dementia, misdiagnoses can occur even in ...
One study adopted 18F-florbetaben PET imaging in Down syndrome patients, suggesting potential role of amyloid imaging in identifying population at risk of dementia. 17 Similar study was conducted on patients with Down syndrome, but with 18F-florbetapir tracer. 18 An attempt to differentiate Down Syndrome pathology from AD has also been made ...
2. Institution. Chamberlain College Nursing. Course. i Human Janet Riley, Alzheimer's Dementia CaSE. Get higher grades by finding the best i Human Janet Riley, Alzheimer's Dementia CaSE notes available, written by your fellow students at Chamberlain College Nursing.
An NIH-funded case study published recently in the journal Nature Medicine falls into this far-reaching category [1]. The report highlights the world's second person known to have an extreme resilience to a rare genetic form of early onset Alzheimer's disease. These latest findings in a single man follow a 2019 report of a woman with ...
HESI Comprehensive Review for the NCLEX-PN ® Examination, 7th Edition provides a complete, all-in-one review of the information that you need to know. Written in an easy-to-read outline format, this study guide reviews content by concepts and clinical areas. Along the way, valuable tips from HESI ® help you apply principles of clinical decision-making and clinical judgment.
Distribution of Out-of-Class Time Out-of-Class Assignments Time (hours) Reading (chapters from Basic Geriatric Nursing and other references) 38 Focused Competency Development Activities: 30 HESI PN Case Study (5 @ 2 hours) = 10 NCLEX Questions (200) = 20 Community Resources Activity (2 hour per page @ 2 pgs.) 4 Discussion Questions (3 @ 2 hours ...
Study design. This was a nested case-control study designed to assess whether DMN effective connectivity can be used predict two outcomes of interest. The first outcome is a future diagnosis of ...
CASE PRESENTATION. A 58-year-old left-handed woman with 12 years of education was referred for further evaluation and management of progressive cognitive dysfunction. A diagnosis of Alzheimer disease (AD) was suspected by the referring neurologist on the basis of an MRI demonstrating mild temporal atrophy, EEG demonstrating bitemporal slowing ...
Skip to main content