Developing a Data Strategy
An effective data strategy for a online subscriptions business that wasn’t getting value from the data they held.
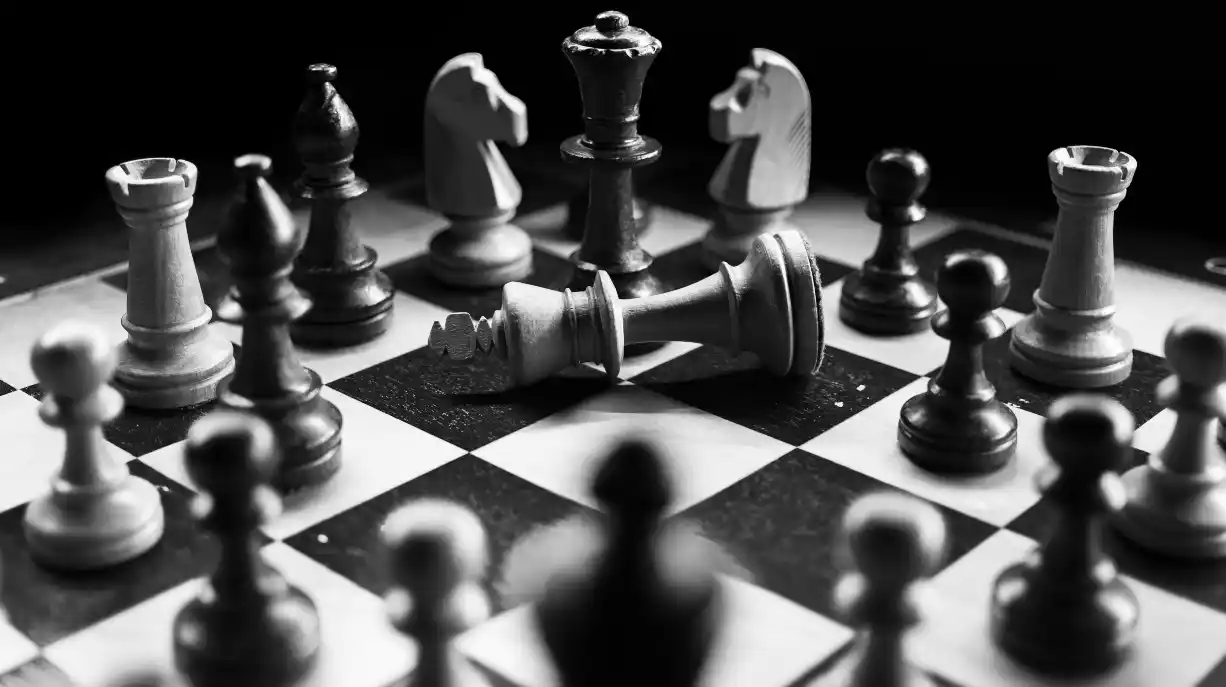
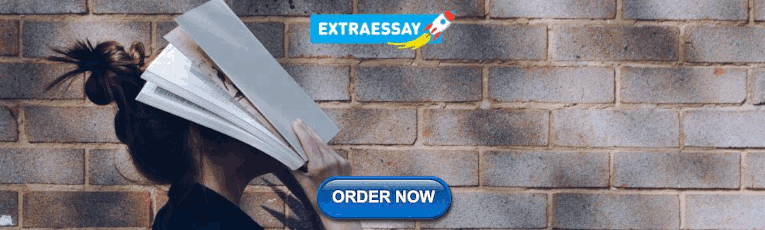
The Opportunity
Our client held large amounts of operational and behavioural data about their product and how their users interacted with it, but were primarily using it for regular and ad-hoc reporting and basic analysis.
They had an extensive team of analysts but they were fully utilised on BAU and short-term, unplanned work.
Our client needed us to take a holistic view of all the data held, tools and technologies in use and how the team was structured to meet the needs of a demanding internal customer base.
What we did
✔︎ Audited and mapped the existing data infrastructure
✔︎ Process mapped all the existing demands on the team
✔︎ Worked with senior functional leaders to develop a data strategy that could support and deliver the wider company strategy
✔︎ Built a mile stoned transition plan to enable the company to move safely from the as-is structure to the new architecture
✔︎ Designed development plans for existing team members
✔︎ Suggested several POCs that drove incremental value using advanced machine learning using their existing data
The Results
The client has a clear data strategy to support the long term growth plans of the business.
BAU work has been significantly reduced, by replacing legacy processes and repetitive tasks with smart automation.
The utilisation of existing data resource has improved significantly, through our delivery of onsite training and implementation of modern tools, driving engagement in the team and providing the business with a strategic advantage over the competition.
Less time spent on BAU activities
How we did it
Our framework can be separated into three sections:
Audit: Understanding current data management, storage, analysis, compliance and responsibilities across the organisation
Strategy & Structure: Independent of the current structure, design a long term approach to data that implements the overall company strategy through a staged delivery plan.
Transformation: Build the delivery plan for how existing tools, roles and responsibilities can be transitioned, and how new tools and processes should be implemented
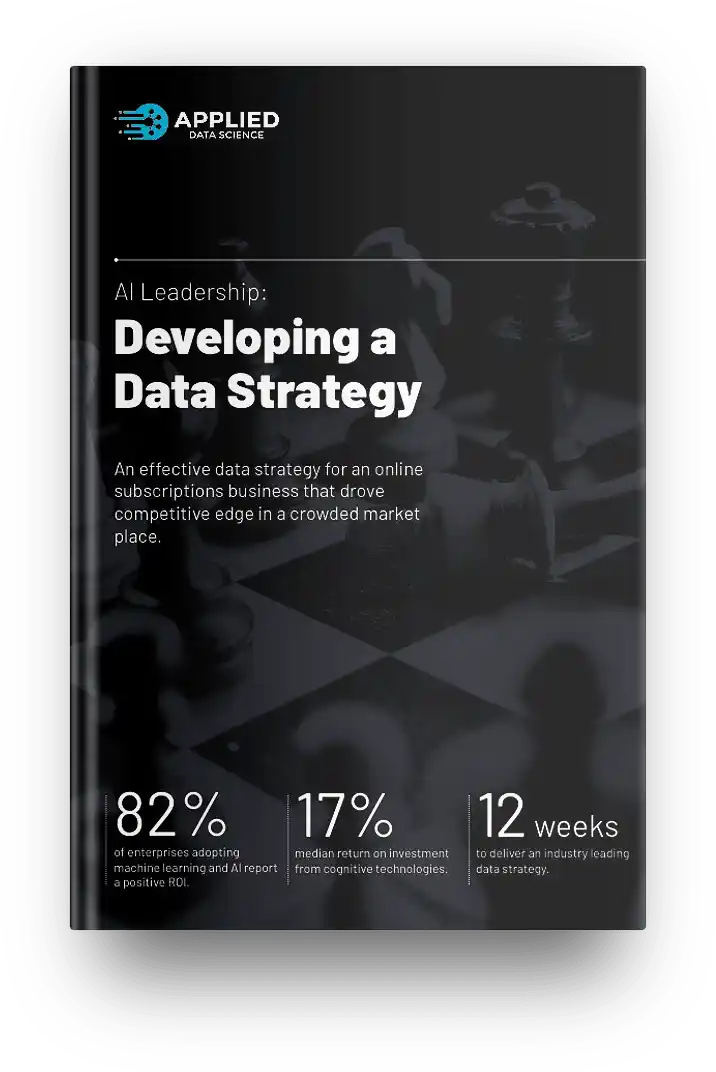
Download our case study
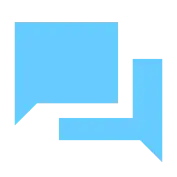
Start a conversation
Take the first step by speaking with one of our data experts today.
Sales Propensity Modelling
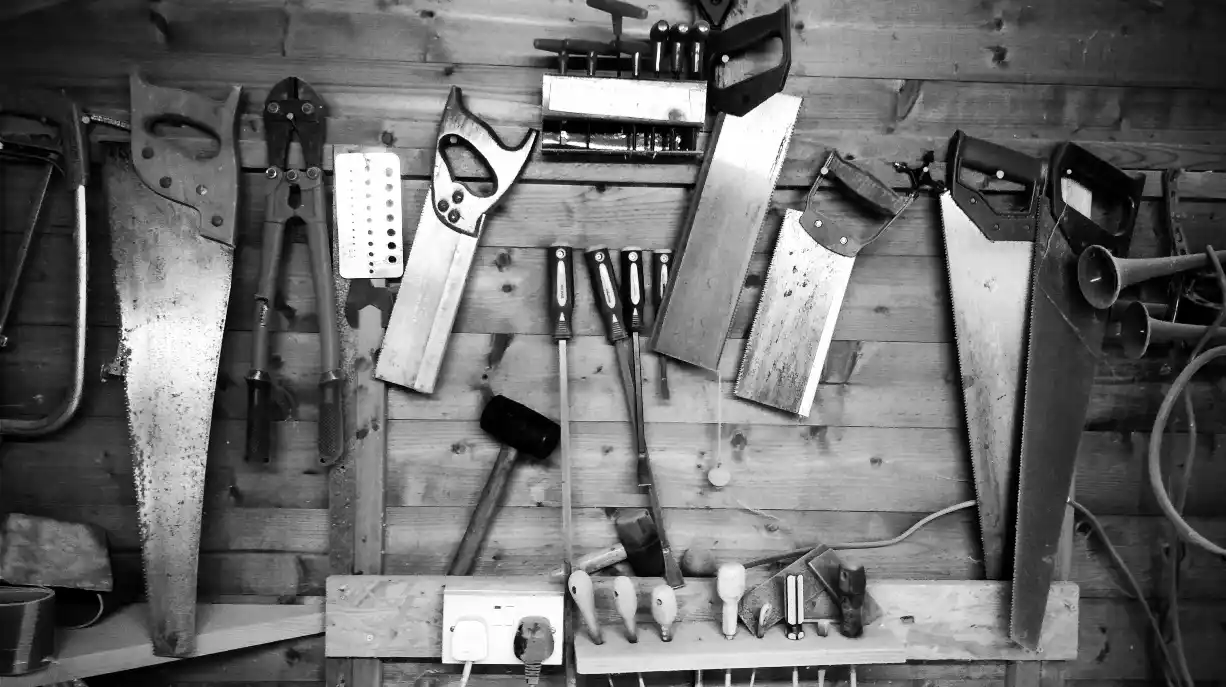
Data Topics
- Data Architecture
- Data Literacy
- Data Science
- Data Strategy
- Data Modeling
- Governance & Quality
- Education Resources For Use & Management of Data
Case Study: Executing an Effective Data Strategy
Few tasks are more logistically and technologically daunting than providing air, land, and sea transportation for the U.S. military across the entire world. Yet that is precisely the mission of the United States Transportation Command, or USTRANSCOM. According to a Congressional Research Service report, on any given day USTRANSCOM conducts over 240 air missions, sends […]
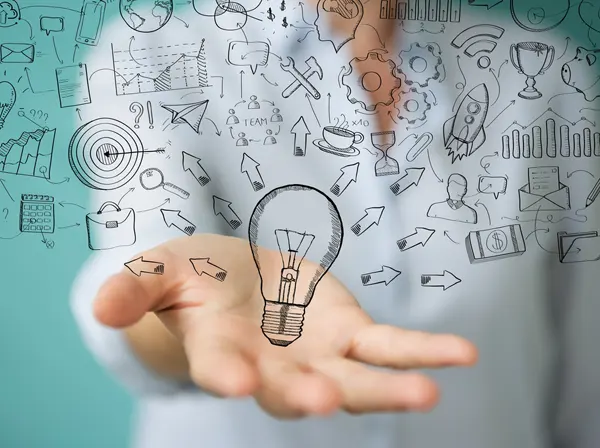
Few tasks are more logistically and technologically daunting than providing air, land, and sea transportation for the U.S. military across the entire world. Yet that is precisely the mission of the United States Transportation Command, or USTRANSCOM. According to a Congressional Research Service report , on any given day USTRANSCOM conducts over 240 air missions, sends 1,500 ground shipments, and has 20 ships underway, as well as aiding in humanitarian relief efforts and transporting patients who need aeromedical evacuation.

Asking the Right Data Strategy Questions
First and foremost, the Data Strategy nests under the company’s broader organizational vision. The organization’s vision is the basis of the strategy, the strategy guides the creation of goals, and goals are achieved through objectives.
The organization must ask itself: Is the current status quo good enough to survive? Do the data and digital strategies align with the organization’s goals ? And do you hope to gain or maintain a strategic advantage over your peers? Having an effective Data Strategy allows the government to make better strategic and tactical decisions. “Especially in our military and government world, we need to make decisions quickly, and they need to be accurate,” said McLean.
Having an effective Data Strategy also helps organizations reap the benefits of data in the first place: better-informed decision-making, understanding customers and trends, providing better products, improving internal operations, and creating additional revenue.
“In the government, we don’t make money. But we sure as heck really want to look at how we can become more efficient, to find ways to do things faster, better, cheaper,” said McLean.
In the particular case of USTRANSCOM, there were a variety of specific reasons that a Data Strategy was necessary, including the need to:
- Advance decision-making
- Mature as a data-driven organization
- Offload common tasks
- Provide information at the speed of operation needs
- Use personnel where they’re needed most
- Understand the vast amount of data that the organization uses and produces every day
Developing the Data Strategy
For McLean, it became clear that developing a Data Strategy was not going to happen over a weekend, especially within the context of a government agency with longstanding bureaucratic norms and entrenched ways of doing things. It would be a multi-year endeavor, requiring organization, patience, and support. The first step was to create a vision: What was the realm of possibility for the organization? What did it want to do?
“You need to ensure that your organization can achieve it. But don’t be so short-sighted that it’s too easy. Make it a challenge, make it difficult,” said McLean.
If the vision is the “to-be” state, the next step is to define the “as-is” state. In the case of USTRANSCOM, there was a great deal of legacy infrastructure that worked well at the time of development – before cloud computing was a widespread option – but now led to unhelpful silos that threw up barriers to enterprise interoperability.
Key exercises during this period included a gap analysis that looked at what the organization needed to accomplish to go from the “as-is” state to the “to-be” state, a consideration of organization priorities, and a SWOT analysis considering strengths, weaknesses, opportunities, and threats.
A major challenge at this point is to bring about cultural change. “You’re potentially uprooting the very processes and legacy knowledge and skills that brought people to their career pinnacle,” said McLean.
People will be highly motivated to defend legacy programs that have worked in the past. The value proposition must be equally defensible, with clear benefits outlined, in order to overcome that resistance. On the other side of the coin, overselling the vision can feed into the hype cycle, where inflated expectations deflate into disillusionment. So, the vision has to be broken down into clear, digestible chunks, to give people some early clear “wins” in what will inevitably be a multi-year process.
Properly selling your vision is key, and that means engaging at all levels of the organization: the C-suite, middle management, and the grassroots level of personnel who do the frontline work. There has to be a sense of transparency and partnership, so that the program isn’t seen as something merely for the data people, but rather something that will make everyone in the company function better. There has to some marketing of the vision to create buy-in at all levels. “People support what they help create,” said McLean.
However, one advantage the organization did have was strong support for a Data Strategy from the commander of USTRANSCOM. There was a standing monthly meeting with the commander to keep him updated on how they were moving forward with strategy and what their achievements were, and this created strong forward momentum and buy-in from management.
Executing the Data Strategy
After the strategy is developed, it’s time to make it a reality. The first step, McLean said, is establishing a foundation by investing in people, technology, and processes. USTRANSCOM established a new chief data officer (CDO) position and a new Data Management team, added people to the Data Architecture team, and hired new data scientists and data engineers.
As training materials, the organization used DATAVERSITY courses, sent personnel to conferences, and brought in noted speakers. For technology investment, the organization bought into Plateau as an integrator and installed IBM’s Cloud Pak for data.
In terms of processes, the team first defined terms in a common lexicon to create a business glossary , which was sent out for peer review, and created a DataOps team. They identified data sources, tables, and elements, then created data profiles and meta-tagged data for quality standards.
Next, they ingested the data, making system connections and security adjustments and optimizing the portfolio. After that came data enrichment and quality review, and that led finally to the creation of data visualizations, expanded analytics, and data services.
The key was to approach the execution from both the “top down” and “bottom up.” Approaching from the top down meant finding ways to immediately show value through tangible results, such as by reducing IT portfolio costs. That reduction in costs led to greater funding to do even more for the organization. The bottom-up approach was to start populating the environment with trusted, valuable data. Although that approach is not glamorous and results are not so immediately tangible, it’s still crucial.
Since starting the process of executing a Data Strategy, USTRANSCOM has embarked on a number of ambitious data projects. The organization currently has 10 active data analytics use cases in motion, and they are working on building a data environment – one project alone has 1,425 tables of reference data that need to be moved out of silos. The current goal of the Data Science team is to build out reusable analytics to be used for future endeavors.
While there have already been notable achievements, there’s still a long way to go. Thinking in the long-term is, ultimately, one of the biggest factors in successfully executing a Data Strategy.
“The real takeaway here is it’s always a multi-year plan,” said McLean. “There’s nothing that you’re going to solve in the first year or two. These are things that you have to be committed to as an organization.”
Want to learn more about DATAVERSITY’s upcoming events? Check out our current lineup of online and face-to-face conferences here .

Here is the video of the Enterprise Data World Presentation:
Image used under license from Shutterstock.com
Leave a Reply Cancel reply
You must be logged in to post a comment.

Data-Driven Decision-Making Case Studies: Insights from Real-World Examples
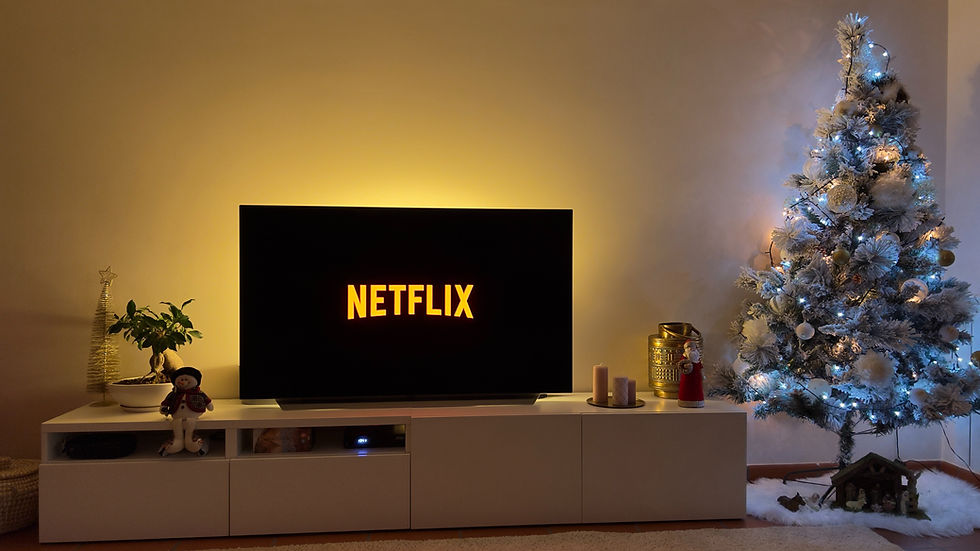
Data has become crucial for making informed decisions in today's fast-paced and ever-changing business environment. Companies use data to gain valuable insights, improve processes, and foster innovation. By studying successful examples of data-driven decision-making, we can gain valuable insights and comprehend the impact of data-driven strategies on business outcomes.
Define Data-Driven Decision Making (DDDM)
Are you tired of making business decisions based on gut instincts and guesswork? It's time to adopt Data-Driven Decision Making (DDDM). DDDM is a strategic approach that leverages collected data to inform and guide your business decisions. You can gain insights by identifying patterns and making informed choices using relevant and accurate data. "This can enhance the precision and efficiency of your decision-making procedure." allowing you to optimize outcomes, mitigate risks, and adapt more dynamically to changing circumstances in today's data-rich environment. Switch to DDDM and give your business the competitive edge it needs!
Importance of DDDM in Modern Businesses
In today's fast-paced and competitive business world, making informed and accurate decisions is more critical than ever. Data-Driven Decision Making (DDDM) is a powerful tool to help modern businesses achieve this goal. By using data and insights to inform business decisions rather than relying on guesswork, companies "Businesses that strategically position themselves to gain a competitive advantage are more likely to achieve success." With DDDM, businesses can make data-backed decisions, leading to better outcomes and tremendous success. So, if you want to stay ahead of your competition and make helpful decisions that drive success, embracing DDDM is the way to go!
Brief Overview of the Success Stories to be Discussed
Discover the success stories showcasing how businesses leverage advanced technologies to drive growth and profitability. Join me for an engaging and thought-provoking session where we will delve into the intricacies of these fascinating case studies. Your active participation will help us uncover valuable insights and unlock new perspectives that can benefit your work. "Make the most of this valuable opportunity to enhance your knowledge and skills!"
1. Netflix's Personalized Recommendations
2. Amazon's Supply Chain Optimization
3. Starbucks Location Analytics
4. American Express Fraud Detection
5. Zara's Fast Fashion Foresight.
You can benefit greatly from this unique opportunity to learn from some of the most innovative companies in the industry. Ensure you take advantage of this chance to expand your knowledge and skills!
Case Study 1: Netflix's Personalized Recommendation
Overview of netflix's challenges in content delivery.
Netflix faced challenges delivering content due to the diverse viewer preferences and vast content library. However, the company has been working hard to address these challenges and ensure users can discover content that aligns with their tastes. By doing so, Netflix aims to improve user satisfaction and retention rates.
How Netflix Used Viewer Data to Tailor Recommendations
By leveraging extensive viewer data, Netflix confidently tackled the challenge of recommending relevant content to its users. The platform thoroughly analyzed user behavior, viewing history, and preferences to create highly sophisticated algorithms. These algorithms were based on machine learning and could personalize content recommendations for each user. This approach significantly increased the likelihood of viewers engaging with content that resonated with their interests.
The Impact on Customer Retention and Satisfaction
The personalized content recommendations profoundly affected customer retention and satisfaction rates. Netflix enhanced the value of its service by providing users with content that closely matched their preferences. This created a stronger bond between users and the platform, leading to longer subscription durations and increased satisfaction.
Lessons Learned and Key Takeaways
Data is a Strategic Asset: Netflix's strategic use of data has wholly revolutionized content delivery. By utilizing excellent viewer data, they have successfully met the needs and preferences of each viewer in an incredibly effective manner.
Personalization Enhances Customer Experience: Personalized recommendations are essential to enhancing the overall customer experience. They can increase engagement, satisfaction, loyalty, and retention. Make no mistake - if you want to take your business to new heights, personalized recommendations are a must!
Continuous Adaptation is Crucial: It is crucial to adapt to achieve success, as Netflix continuously demonstrates. With the ever-evolving preferences of viewers, it is imperative to perform ongoing analysis and make necessary adjustments to algorithms to ensure that recommendations stay consistently relevant.
Balancing Privacy and Personalization: When utilizing viewer data, it is crucial to hit the right balance between personalization and privacy. Netflix has accomplished this by delivering highly personalized recommendations without compromising user privacy.
Netflix's approach to content delivery serves as an inspiration for the transformative power of data-driven decision-making. The personalized recommendations derived from customer data have proven to be a game changer regarding customer retention and satisfaction. The significance of adaptability, strategic use of data, and the balance between personalization and privacy, as highlighted by Netflix's success, can serve as a guide for other businesses looking to impact their customers positively.
Case study 2: amazon's supply chain optimization.
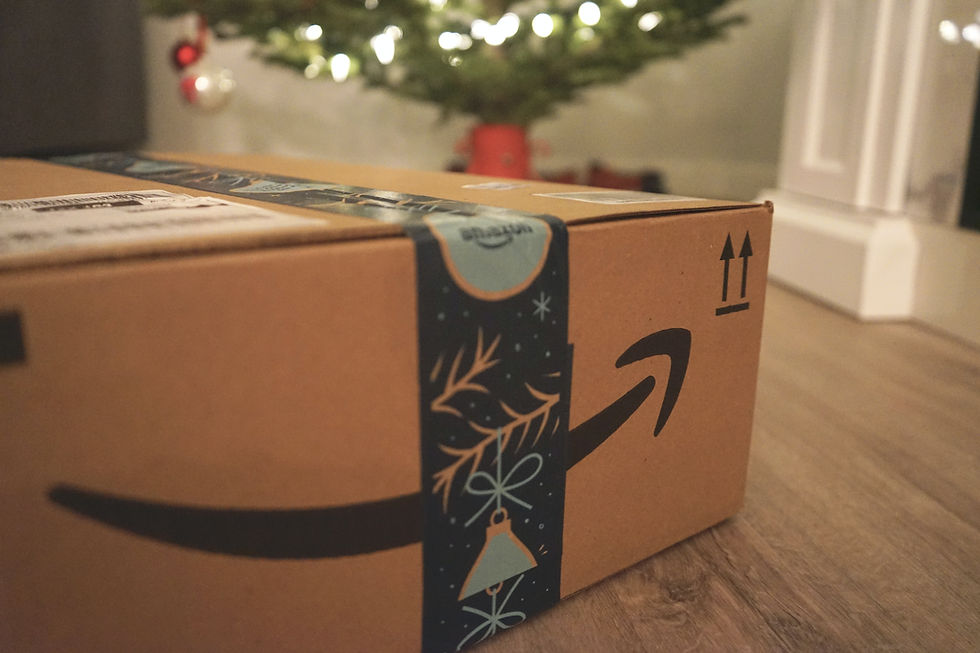
Understanding Amazon's Complex Supply Chain
Amazon has a complex supply chain involves various stages, from sourcing the products to delivering them to customers. The company manages a vast network of fulfillment centers, distribution hubs, and transportation systems. The complexity arises due to the need to manage different types of products, fluctuating demand, and the commitment to fast and efficient delivery.
Implementation of Predictive Analytics for Inventory Management
Using predictive analytics, Amazon has optimized inventory management by accurately forecasting future demand by analyzing historical data, current market trends, and seasonality. This has helped them prevent stockouts and overstock situations, improving their overall business efficiency and customer satisfaction.
Results Achieved in Cost Savings and Delivery Times
Anticipating the future, the implementation of predictive analytics is expected to yield significant results. Amazon will likely achieve cost savings by minimizing excess inventory and improving warehouse efficiency. Additionally, streamlined inventory management contributes to faster order fulfillment, which reduces delivery times and enhances the customer experience. We can expect a boost in efficiency and a better customer experience shortly.
Insights Gained and How Businesses Can Apply Similar Strategies
Data-Driven Decision-Making: In today's business landscape, data-driven decision-making has become the cornerstone of success. If you want your business to thrive, you must leverage advanced analytics to gain actionable insights into your supply chains. This will enable you to make proactive and strategic decisions to enhance your projects. Don't fall behind the competition - take charge and start leveraging the power of data-driven decision-making now.
Dynamic Inventory Optimization: Incorporating a dynamic approach to inventory management that relies on predictive analytics is "Businesses must prioritize their competitive edge and remain ahead of the industry. This is crucial to ensure success and longevity.". It helps them to quickly adjust to changing market conditions and meet the ever-evolving demands of consumers. This not only optimizes the utilization of resources but also reduces wastage, making it a sound strategy crucial for any business that wishes to survive in today's competitive market landscape.
Focus on Customer-Centric Logistics: To improve customer satisfaction, businesses can focus on optimizing logistics and reducing delivery times. Amazon's customer-centric approach demonstrates the importance of fast and reliable delivery. Companies can boost customer loyalty and drive growth by enhancing the customer experience.
Investment in Technology: To stay ahead in supply chain optimization, businesses must adopt cutting-edge technologies like AI and machine learning. Amazon's supply chain success is a testament to the power of continuous investment in technology. So, if you want to thrive in today's competitive market, it's high time you leverage these technologies to your advantage.
Amazon's journey toward optimizing its supply chain through predictive analytics has tremendously impacted cost savings and delivery times. Other businesses can achieve similar results by utilizing data-driven decision-making, implementing dynamic inventory management, prioritizing customer-centric logistics, and investing in advanced technologies.
Case study 3: starbucks location analytics.

The Problem with Traditional Site Selection
The traditional approach to retail site selection, which relied on broad demographic data and market trends, must be improved in identifying optimal locations. Adopting a more precise approach that considers specific local factors influencing consumer behavior and store performance is imperative to ensure success.
How Starbucks Leveraged Geographic Information Systems (GIS)
Starbucks has transformed its approach to selecting store locations by leveraging Geographic Information Systems (GIS). This innovative technology has enabled Starbucks to systematically evaluate and visualize location-specific data, such as foot traffic patterns, nearby businesses, demographics, and local economic factors. By conducting this comprehensive analysis, Starbucks can gain a more nuanced understanding of potential store locations and make informed decisions.
Outcomes in Terms of New Store Performance and Sales
Starbucks, the renowned coffeehouse chain, has achieved notable success in its site selection strategy by implementing a Geographic Information System (GIS). GIS technology has enabled Starbucks to strategically place its new stores in locations that cater to the preferences and traffic patterns of the local population. As a result, the company has witnessed a significant improvement in the performance of its new stores, surpassing the sales of those selected through traditional methods. The successful implementation of GIS in site selection has contributed to optimizing location decisions, leading to a more efficient and effective expansion strategy for Starbucks.
Broader Implications for Retail Location Decision-Making
Precision in Site Selection: Geographic Information System (GIS) technology has opened new doors for retailers to make informed decisions. By leveraging GIS, businesses can analyze and interpret specific geographic data to optimize their site selection process. This helps them understand local nuances and customer behavior more precisely, allowing them to make data-driven decisions that lead to better business outcomes.
Adaptability to Local Factors: To establish a closer relationship with their customers, retailers must consider various local factors such as competition, demographics, and cultural preferences. By doing so, they can customize their offerings and marketing strategies to fit the local communities' specific needs and preferences. This approach can lead to better customer engagement and loyalty and, ultimately, higher sales for the retailer.
Cost Efficiency: Regarding retail businesses, selecting the right location for a store is crucial for success. Optimal site selection can significantly reduce the risk of underperforming stores and minimize the financial impact of poor location decisions. Retail businesses can enhance their overall cost efficiency and profitability by doing so. This is why it is essential for companies to carefully analyze and consider factors before making any site selection decisions.
Strategic Expansion: Geographic Information System (GIS) provides retailers with a powerful tool to make informed decisions about expanding their business. By leveraging location-based data, retailers can discover new markets and potential locations for growth. This data-driven approach helps retailers create a more sustainable and prosperous expansion plan, resulting in long-term prosperity for the business.
Enhanced Customer Experience: Retailers can improve the shopping experience for their customers by strategically selecting store locations that cater to their preferences and habits. Retailers can attract more foot traffic and enhance customer satisfaction by offering conveniently located stores. In this case, it can increase sales and customer loyalty.
To make it more understandable, Starbucks has been using fantastic GIS technology to help them pick the best locations for their stores. It's like a digital map that allows them to look at much different information, like how many people live nearby, how much traffic there is, and what other businesses are in the area. By using this technology, Starbucks can make better choices about where to put their stores and how they can be successful. Other businesses can also use GIS to make better decisions about where to open new stores and how to compete in the changing world of retail.
Case study 4: american express fraud detection.

Rise in Credit Card Fraud and the Challenge for Card Issuers
As more and more people turn to digital transactions and online commerce, it's essential to be aware of the increased incidence of credit card fraud. Protect yourself and others from financial crimes by staying informed and proactively safeguarding your financial information. Thus, posing a significant challenge for card issuers. Fraudsters are constantly devising new and innovative tactics to steal sensitive information and exploit vulnerabilities in payment systems. This makes it imperative for financial institutions to stay ahead of the game in detecting and preventing fraudulent activities. Today, with advanced technologies like machine learning and artificial intelligence, card issuers can analyze big data that can identify patterns and anomalies and indicate fraudulent behavior. By adopting a proactive approach to fraud detection and prevention, financial institutions can safeguard their customers' personal information and financial assets, thus building trust and loyalty among their clients.
American Express's Use of Machine Learning for Early Detection
American Express always prioritizes the security of its customers' financial transactions. The company has employed advanced machine-learning algorithms to analyze vast amounts of real-time transaction data to achieve this. These algorithms can identify patterns, anomalies, and behavioral indicators typically associated with fraudulent activities. By continuously learning from new data, the system adapts to evolving fraud tactics and enhances its ability to detect irregularities early on. This advanced technology is a critical component of American Express's fraud prevention strategy, and it helps the company safeguard its customers against potential financial losses.
Effectiveness in Preventing Fraud and Protecting Customers
American Express utilizes an advanced fraud detection system powered by machine learning that has demonstrated exceptional efficacy in preventing fraudulent activities and safeguarding customers. By detecting fraudulent transactions early, the company can promptly take necessary measures, such as notifying customers of suspicious activities or blocking them altogether, thus reinforcing trust in the company's commitment to security and ensuring customer satisfaction.
What Companies Can Learn About Proactive Data Monitoring
Invest in Advanced Analytics: Advanced analytics, such as machine learning, helps companies proactively monitor data for potential fraud indicators and unusual patterns. It identifies issues before they become significant problems, saving the company millions. It also identifies new business opportunities, market trends, and operational inefficiencies, enhancing customer satisfaction and the bottom line.
Real-Time Analysis: Real-time data analysis is a powerful tool for detecting and responding to suspicious activities. By monitoring data in real-time, organizations can quickly "Identify possible threats and take prompt action to reduce their impact." we can overcome any challenges that come our way and pave the path to success. This approach reduces the window of vulnerability and enhances the effectiveness of fraud prevention measures. Therefore, real-time data analysis can help organizations prevent fraudsters from exploiting them. It's important to stay vigilant and proactive in protecting yourself and your finances from potential threats. Please take action now and don't give them the chance to cause any harm. Remember, it's better to be safe than sorry. their interests.
Continuous Learning Systems: Adopting systems that can learn and adapt to new fraud patterns is highly recommended. This approach ensures that the monitoring mechanisms remain up-to-date and effective despite the constantly evolving threats. Embracing such systems can protect businesses. The objective is to safeguard individuals and organizations against financial losses and reputational harm resulting from fraudulent activities.
Customer Communication: Implementing solid and effective communication methods is essential to inform customers of any potential fraud promptly. Through transparent communication, customers can be informed of the situation and take immediate action, building trust between them and the organization.
Collaboration with Industry Partners: Collaborating with industry partners and sharing insights on emerging fraud trends is essential. By working together, we can enhance our ability to combat fraud and protect the entire ecosystem. We can stay informed and better equipped to prevent fraudulent activities through a collective effort.
Balancing Security and User Experience: It's crucial to balance strong security measures with a seamless user experience for online platform security. While taking all necessary steps to prevent fraud and unauthorized access to your system is critical, ensuring that your legitimate customers don't face any inconvenience or dissatisfaction due to stringent security protocols is equally essential. Therefore, adopting a multi-layered approach to security is recommended to shield your system from potential threats without making the user experience cumbersome or frustrating. This may involve utilizing two-factor and risk-based authentication and real-time fraud detection. Furthermore, educating users on secure online practices and equipping them with the necessary tools and resources to protect their personal information and transactions is essential.
American Express has implemented machine learning techniques to detect and prevent fraud at an early stage. This is an excellent example for businesses seeking to improve their proactive data monitoring capabilities. By adopting advanced analytical tools, real-time analysis, continuous learning systems, and effective communication, companies can establish a solid and proactive strategy to combat emerging threats in the digital realm.
Case study 5: zara's fast fashion foresight.
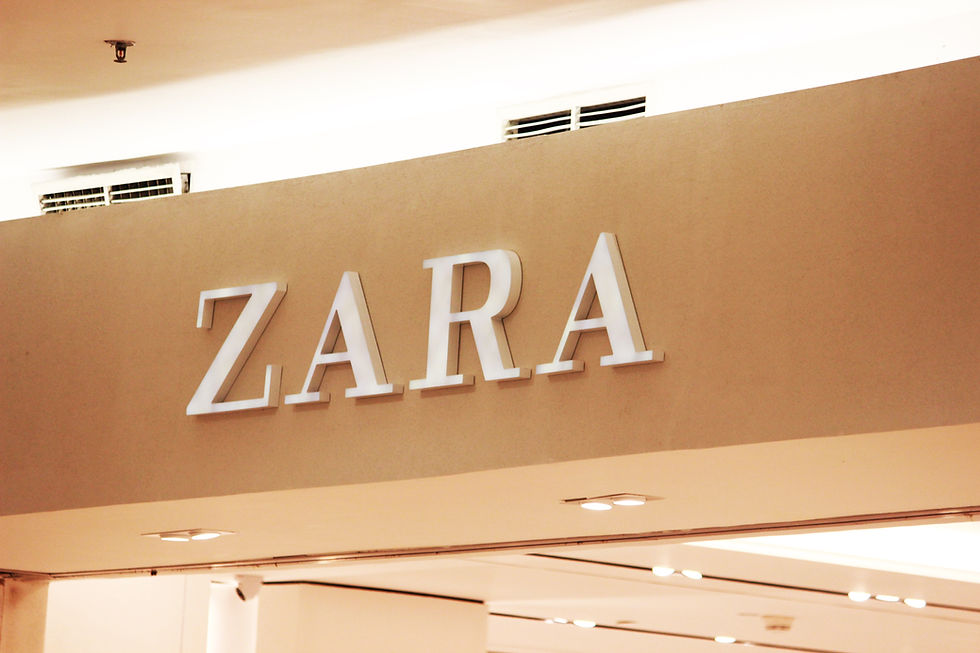
Fast Fashion Industry Challenges in Demand Forecasting
The fast fashion industry, known for producing trendy and affordable clothing rapidly, faces significant challenges in accurately predicting consumer demand. One of the main reasons for this is the constantly changing nature of fashion trends. What is popular today may be out of fashion tomorrow, making it difficult for companies to plan their production processes effectively.
Additionally, fast fashion products have short life cycles, meaning they are only in style for a limited time. As a result, companies need to be able to respond to market shifts and adjust their production accordingly quickly. This can be challenging, as traditional forecasting methods rely on historical data, which may need to be more relevant in a fast-changing market.
To overcome these challenges, the fast fashion industry needs innovative and agile forecasting methods to keep up with the dynamic nature of consumer preferences. This may involve leveraging data analytics and machine learning algorithms to identify emerging trends and predict future demand. Companies can enhance efficiency, reduce waste, and provide excellent customer value.
Zara's Integration of Real-Time Sales Data into Production Decisions
Zara, one of the world's leading fashion retailers, has redefined the fashion industry by leveraging real-time sales data. Zara has integrated real-time sales data into its production decisions, allowing the company to stay ahead of the competition. Zara's vertically integrated supply chain and responsive production model enable it to capture up-to-the-minute sales data from its stores worldwide. This data is then fed back to the design and production teams, who use it to rapidly adjust inventory levels and introduce new designs based on current demand trends. Using real-time sales data, Zara can create a customer-centric approach, ensuring its customers always have access to the latest and most stylish designs.
Benefits Seen in Reduced Waste and Increased Sales
Zara has adopted a real-time analytics approach that has proven to be highly beneficial. The company's production is now closely aligned with actual customer demand, which results in a significant reduction in overstock and markdowns. This approach has minimized the environmental impact of excessive inventory. In addition, the quick response to emerging trends and consumer preferences has led to an increase in full-price sales, boosting revenue and profitability for Zara.
Strategies for Incorporating Real-Time Analytics into Product Development
Connected Supply Chain: Establishing a connected and transparent supply chain that enables seamless real-time data flow from sales channels to production and design teams is imperative. This will ensure that all the teams are on the same page and can make quick, informed decisions based on accurate, up-to-date information. Failure to do so can result in costly delays, inefficiencies, and missed opportunities. So, let's prioritize this and set up a robust supply chain that works for us!
Agile Production Processes: To maintain a competitive edge, it is crucial to adopt constructive and flexible production processes that can quickly respond to changes in demand. This involves embracing shorter production cycles and smaller batch sizes, which makes us more efficient and proactive in meeting customer needs.
Advanced Data Analytics: To optimize your sales strategies, you can use advanced data analytics tools to process and analyze real-time sales data efficiently. You can accurately forecast demand and make data-driven decisions by implementing predictive modeling and machine learning algorithms.
Cross-Functional Collaboration: Promoting collaboration among different organizational departments is crucial to ensure the sales, marketing, design, and production teams have a unified interpretation of real-time data. This way, they can collectively make informed decisions that are in the company's best interest. The organization can improve its efficiency, productivity, and profitability by promoting open communication and collaboration between departments.
Customer Feedback Integration: One way to enhance the accuracy of real-time analytics is to consider customer feedback and preferences. Social media listening and direct customer interactions can provide valuable insights into emerging trends and demands.
Technology Integration: As we look towards the future, it's becoming increasingly clear that investing in technologies that facilitate real-time data collection and processing will be crucial. With the rise of automation and the growing need for instant information, businesses that have point-of-sale systems, inventory management software, and communication tools that streamline information flow will be better equipped to thrive in the fast-paced and ever-changing world of tomorrow. So, it's never too soon to start thinking about how you can integrate these technologies into your business strategy.
Zara's outstanding achievement in the fast fashion industry is a remarkable example of how incorporating real-time sales data into production decisions can lead to immense success. By reducing waste, swiftly responding to market trends, and utilizing advanced analytics, Zara has set a benchmark for other companies in integrating real-time insights into their product development strategies. This approach enhances efficiency and competitiveness in the highly dynamic and ever-evolving fashion industry.
In the constantly shifting business realm, the adage' knowledge is power' has never been more accurate, particularly about tangible, data-derived knowledge. data-driven decision making (dddm) presents an approach where critical business decisions are made not on intuition or experience alone, but on deep dives into data analysis. empirical evidence garnered through this method provides actionable insights leading to strategic, evidence-based decisions..
The stories of Netflix, Amazon, Starbucks, American Express, and Zara demonstrate the immense potential of Data-Driven Decision Making (DDDM). By analyzing vast data points and leveraging advanced analytics, these companies transformed their businesses and achieved unparalleled success.
For instance, Netflix utilized DDDM to create tailor-made recommendations, engaging existing users and attracting new ones. Amazon used data analytics to optimize its supply chain, lowering costs and accelerating shipping times. Starbucks leveraged location analytics to predict the profitability of new store locations with impressive accuracy. American Express used machine learning algorithms to identify frauds faster than ever, saving millions in potential losses. Lastly, Zara demonstrated agility in the competitive fast fashion market by adapting its production and supply chain to meet real-time demand.
As seen in these success stories, data-driven decision-making can powerfully impact business, from customer engagement to trend forecasting. They underscore the importance of meticulous data analysis in navigating the present and forecasting an ever-changing future, paving the way for unparalleled business success. Companies can draw inspiration from these cases and embark on their DDDM journey to achieve similar outcomes.
Recent Posts
Maximizing the potential of WFM in the Gig Economy: Achieving the perfect balance between Flexibility and Stability through Case Studies.
WFM in the Gig Economy: Balancing Flexibility and Stability
Data-Driven Decision Making: Utilizing Analytics in Workforce Management
Comentarios

An official website of the United States government
Here’s how you know
The .gov means it’s official. Federal government websites often end in .gov or .mil. Before sharing sensitive information, make sure you’re on a federal government site.
The site is secure. The https:// ensures that you are connecting to the official website and that any information you provide is encrypted and transmitted securely.
Case studies & examples
Agencies mobilize to improve emergency response in puerto rico through better data.
Federal agencies' response efforts to Hurricanes Irma and Maria in Puerto Rico was hampered by imperfect address data for the island. In the aftermath, emergency responders gathered together to enhance the utility of Puerto Rico address data and share best practices for using what information is currently available.
Federal Data Strategy
BUILDER: A Science-Based Approach to Infrastructure Management
The Department of Energy’s National Nuclear Security Administration (NNSA) adopted a data-driven, risk-informed strategy to better assess risks, prioritize investments, and cost effectively modernize its aging nuclear infrastructure. NNSA’s new strategy, and lessons learned during its implementation, will help inform other federal data practitioners’ efforts to maintain facility-level information while enabling accurate and timely enterprise-wide infrastructure analysis.
Department of Energy
data management , data analysis , process redesign , Federal Data Strategy
Business case for open data
Six reasons why making your agency's data open and accessible is a good business decision.
CDO Council Federal HR Dashboarding Report - 2021
The CDO Council worked with the US Department of Agriculture, the Department of the Treasury, the United States Agency for International Development, and the Department of Transportation to develop a Diversity Profile Dashboard and to explore the value of shared HR decision support across agencies. The pilot was a success, and identified potential impact of a standardized suite of HR dashboards, in addition to demonstrating the value of collaborative analytics between agencies.
Federal Chief Data Officer's Council
data practices , data sharing , data access
CDOC Data Inventory Report
The Chief Data Officers Council Data Inventory Working Group developed this paper to highlight the value proposition for data inventories and describe challenges agencies may face when implementing and managing comprehensive data inventories. It identifies opportunities agencies can take to overcome some of these challenges and includes a set of recommendations directed at Agencies, OMB, and the CDO Council (CDOC).
data practices , metadata , data inventory
DSWG Recommendations and Findings
The Chief Data Officer Council (CDOC) established a Data Sharing Working Group (DSWG) to help the council understand the varied data-sharing needs and challenges of all agencies across the Federal Government. The DSWG reviewed data-sharing across federal agencies and developed a set of recommendations for improving the methods to access and share data within and between agencies. This report presents the findings of the DSWG’s review and provides recommendations to the CDOC Executive Committee.
data practices , data agreements , data sharing , data access
Data Skills Training Program Implementation Toolkit
The Data Skills Training Program Implementation Toolkit is designed to provide both small and large agencies with information to develop their own data skills training programs. The information provided will serve as a roadmap to the design, implementation, and administration of federal data skills training programs as agencies address their Federal Data Strategy’s Agency Action 4 gap-closing strategy training component.
data sharing , Federal Data Strategy
Data Standdown: Interrupting process to fix information
Although not a true pause in operations, ONR’s data standdown made data quality and data consolidation the top priority for the entire organization. It aimed to establish an automated and repeatable solution to enable a more holistic view of ONR investments and activities, and to increase transparency and effectiveness throughout its mission support functions. In addition, it demonstrated that getting top-level buy-in from management to prioritize data can truly advance a more data-driven culture.
Office of Naval Research
data governance , data cleaning , process redesign , Federal Data Strategy
Data.gov Metadata Management Services Product-Preliminary Plan
Status summary and preliminary business plan for a potential metadata management product under development by the Data.gov Program Management Office
data management , Federal Data Strategy , metadata , open data
PDF (7 pages)
Department of Transportation Case Study: Enterprise Data Inventory
In response to the Open Government Directive, DOT developed a strategic action plan to inventory and release high-value information through the Data.gov portal. The Department sustained efforts in building its data inventory, responding to the President’s memorandum on regulatory compliance with a comprehensive plan that was recognized as a model for other agencies to follow.
Department of Transportation
data inventory , open data
Department of Transportation Model Data Inventory Approach
This document from the Department of Transportation provides a model plan for conducting data inventory efforts required under OMB Memorandum M-13-13.
data inventory
PDF (5 pages)
FEMA Case Study: Disaster Assistance Program Coordination
In 2008, the Disaster Assistance Improvement Program (DAIP), an E-Government initiative led by FEMA with support from 16 U.S. Government partners, launched DisasterAssistance.gov to simplify the process for disaster survivors to identify and apply for disaster assistance. DAIP utilized existing partner technologies and implemented a services oriented architecture (SOA) that integrated the content management system and rules engine supporting Department of Labor’s Benefits.gov applications with FEMA’s Individual Assistance Center application. The FEMA SOA serves as the backbone for data sharing interfaces with three of DAIP’s federal partners and transfers application data to reduce duplicate data entry by disaster survivors.
Federal Emergency Management Agency
data sharing
Federal CDO Data Skills Training Program Case Studies
This series was developed by the Chief Data Officer Council’s Data Skills & Workforce Development Working Group to provide support to agencies in implementing the Federal Data Strategy’s Agency Action 4 gap-closing strategy training component in FY21.
FederalRegister.gov API Case Study
This case study describes the tenets behind an API that provides access to all data found on FederalRegister.gov, including all Federal Register documents from 1994 to the present.
National Archives and Records Administration
PDF (3 pages)
Fuels Knowledge Graph Project
The Fuels Knowledge Graph Project (FKGP), funded through the Federal Chief Data Officers (CDO) Council, explored the use of knowledge graphs to achieve more consistent and reliable fuel management performance measures. The team hypothesized that better performance measures and an interoperable semantic framework could enhance the ability to understand wildfires and, ultimately, improve outcomes. To develop a more systematic and robust characterization of program outcomes, the FKGP team compiled, reviewed, and analyzed multiple agency glossaries and data sources. The team examined the relationships between them, while documenting the data management necessary for a successful fuels management program.
metadata , data sharing , data access
Government Data Hubs
A list of Federal agency open data hubs, including USDA, HHS, NASA, and many others.
Helping Baltimore Volunteers Find Where to Help
Bloomberg Government analysts put together a prototype through the Census Bureau’s Opportunity Project to better assess where volunteers should direct litter-clearing efforts. Using Census Bureau and Forest Service information, the team brought a data-driven approach to their work. Their experience reveals how individuals with data expertise can identify a real-world problem that data can help solve, navigate across agencies to find and obtain the most useful data, and work within resource constraints to provide a tool to help address the problem.
Census Bureau
geospatial , data sharing , Federal Data Strategy
How USDA Linked Federal and Commercial Data to Shed Light on the Nutritional Value of Retail Food Sales
Purchase-to-Plate Crosswalk (PPC) links the more than 359,000 food products in a comercial company database to several thousand foods in a series of USDA nutrition databases. By linking existing data resources, USDA was able to enrich and expand the analysis capabilities of both datasets. Since there were no common identifiers between the two data structures, the team used probabilistic and semantic methods to reduce the manual effort required to link the data.
Department of Agriculture
data sharing , process redesign , Federal Data Strategy
How to Blend Your Data: BEA and BLS Harness Big Data to Gain New Insights about Foreign Direct Investment in the U.S.
A recent collaboration between the Bureau of Economic Analysis (BEA) and the Bureau of Labor Statistics (BLS) helps shed light on the segment of the American workforce employed by foreign multinational companies. This case study shows the opportunities of cross-agency data collaboration, as well as some of the challenges of using big data and administrative data in the federal government.
Bureau of Economic Analysis / Bureau of Labor Statistics
data sharing , workforce development , process redesign , Federal Data Strategy
Implementing Federal-Wide Comment Analysis Tools
The CDO Council Comment Analysis pilot has shown that recent advances in Natural Language Processing (NLP) can effectively aid the regulatory comment analysis process. The proof-ofconcept is a standardized toolset intended to support agencies and staff in reviewing and responding to the millions of public comments received each year across government.
Improving Data Access and Data Management: Artificial Intelligence-Generated Metadata Tags at NASA
NASA’s data scientists and research content managers recently built an automated tagging system using machine learning and natural language processing. This system serves as an example of how other agencies can use their own unstructured data to improve information accessibility and promote data reuse.
National Aeronautics and Space Administration
metadata , data management , data sharing , process redesign , Federal Data Strategy
Investing in Learning with the Data Stewardship Tactical Working Group at DHS
The Department of Homeland Security (DHS) experience forming the Data Stewardship Tactical Working Group (DSTWG) provides meaningful insights for those who want to address data-related challenges collaboratively and successfully in their own agencies.
Department of Homeland Security
data governance , data management , Federal Data Strategy
Leveraging AI for Business Process Automation at NIH
The National Institute of General Medical Sciences (NIGMS), one of the twenty-seven institutes and centers at the NIH, recently deployed Natural Language Processing (NLP) and Machine Learning (ML) to automate the process by which it receives and internally refers grant applications. This new approach ensures efficient and consistent grant application referral, and liberates Program Managers from the labor-intensive and monotonous referral process.
National Institutes of Health
standards , data cleaning , process redesign , AI
FDS Proof Point
National Broadband Map: A Case Study on Open Innovation for National Policy
The National Broadband Map is a tool that provide consumers nationwide reliable information on broadband internet connections. This case study describes how crowd-sourcing, open source software, and public engagement informs the development of a tool that promotes government transparency.
Federal Communications Commission
National Renewable Energy Laboratory API Case Study
This case study describes the launch of the National Renewable Energy Laboratory (NREL) Developer Network in October 2011. The main goal was to build an overarching platform to make it easier for the public to use NREL APIs and for NREL to produce APIs.
National Renewable Energy Laboratory
Open Energy Data at DOE
This case study details the development of the renewable energy applications built on the Open Energy Information (OpenEI) platform, sponsored by the Department of Energy (DOE) and implemented by the National Renewable Energy Laboratory (NREL).
open data , data sharing , Federal Data Strategy
Pairing Government Data with Private-Sector Ingenuity to Take on Unwanted Calls
The Federal Trade Commission (FTC) releases data from millions of consumer complaints about unwanted calls to help fuel a myriad of private-sector solutions to tackle the problem. The FTC’s work serves as an example of how agencies can work with the private sector to encourage the innovative use of government data toward solutions that benefit the public.
Federal Trade Commission
data cleaning , Federal Data Strategy , open data , data sharing
Profile in Data Sharing - National Electronic Interstate Compact Enterprise
The Federal CDO Council’s Data Sharing Working Group highlights successful data sharing activities to recognize mature data sharing practices as well as to incentivize and inspire others to take part in similar collaborations. This Profile in Data Sharing focuses on how the federal government and states support children who are being placed for adoption or foster care across state lines. It greatly reduces the work and time required for states to exchange paperwork and information needed to process the placements. Additionally, NEICE allows child welfare workers to communicate and provide timely updates to courts, relevant private service providers, and families.
Profile in Data Sharing - National Health Service Corps Loan Repayment Programs
The Federal CDO Council’s Data Sharing Working Group highlights successful data sharing activities to recognize mature data sharing practices as well as to incentivize and inspire others to take part in similar collaborations. This Profile in Data Sharing focuses on how the Health Resources and Services Administration collaborates with the Department of Education to make it easier to apply to serve medically underserved communities - reducing applicant burden and improving processing efficiency.
Profile in Data Sharing - Roadside Inspection Data
The Federal CDO Council’s Data Sharing Working Group highlights successful data sharing activities to recognize mature data sharing practices as well as to incentivize and inspire others to take part in similar collaborations. This Profile in Data Sharing focuses on how the Department of Transportation collaborates with the Customs and Border Patrol and state partners to prescreen commercial motor vehicles entering the US and to focus inspections on unsafe carriers and drivers.
Profiles in Data Sharing - U.S. Citizenship and Immigration Service
The Federal CDO Council’s Data Sharing Working Group highlights successful data sharing activities to recognize mature data sharing practices as well as to incentivize and inspire others to take part in similar collaborations. This Profile in Data Sharing focuses on how the U.S. Citizenship and Immigration Service (USCIS) collaborated with the Centers for Disease Control to notify state, local, tribal, and territorial public health authorities so they can connect with individuals in their communities about their potential exposure.
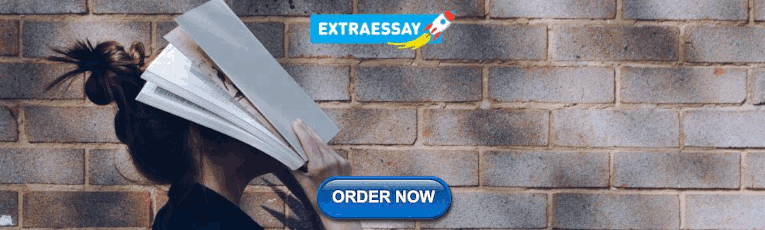
SBA’s Approach to Identifying Data, Using a Learning Agenda, and Leveraging Partnerships to Build its Evidence Base
Through its Enterprise Learning Agenda, Small Business Administration’s (SBA) staff identify essential research questions, a plan to answer them, and how data held outside the agency can help provide further insights. Other agencies can learn from the innovative ways SBA identifies data to answer agency strategic questions and adopt those aspects that work for their own needs.
Small Business Administration
process redesign , Federal Data Strategy
Supercharging Data through Validation as a Service
USDA's Food and Nutrition Service restructured its approach to data validation at the state level using an open-source, API-based validation service managed at the federal level.
data cleaning , data validation , API , data sharing , process redesign , Federal Data Strategy
The Census Bureau Uses Its Own Data to Increase Response Rates, Helps Communities and Other Stakeholders Do the Same
The Census Bureau team produced a new interactive mapping tool in early 2018 called the Response Outreach Area Mapper (ROAM), an application that resulted in wider use of authoritative Census Bureau data, not only to improve the Census Bureau’s own operational efficiency, but also for use by tribal, state, and local governments, national and local partners, and other community groups. Other agency data practitioners can learn from the Census Bureau team’s experience communicating technical needs to non-technical executives, building analysis tools with widely-used software, and integrating efforts with stakeholders and users.
open data , data sharing , data management , data analysis , Federal Data Strategy
The Mapping Medicare Disparities Tool
The Centers for Medicare & Medicaid Services’ Office of Minority Health (CMS OMH) Mapping Medicare Disparities Tool harnessed the power of millions of data records while protecting the privacy of individuals, creating an easy-to-use tool to better understand health disparities.
Centers for Medicare & Medicaid Services
geospatial , Federal Data Strategy , open data
The Veterans Legacy Memorial
The Veterans Legacy Memorial (VLM) is a digital platform to help families, survivors, and fellow veterans to take a leading role in honoring their beloved veteran. Built on millions of existing National Cemetery Administration (NCA) records in a 25-year-old database, VLM is a powerful example of an agency harnessing the potential of a legacy system to provide a modernized service that better serves the public.
Veterans Administration
data sharing , data visualization , Federal Data Strategy
Transitioning to a Data Driven Culture at CMS
This case study describes how CMS announced the creation of the Office of Information Products and Data Analytics (OIPDA) to take the lead in making data use and dissemination a core function of the agency.
data management , data sharing , data analysis , data analytics
PDF (10 pages)
U.S. Department of Labor Case Study: Software Development Kits
The U.S. Department of Labor sought to go beyond merely making data available to developers and take ease of use of the data to the next level by giving developers tools that would make using DOL’s data easier. DOL created software development kits (SDKs), which are downloadable code packages that developers can drop into their apps, making access to DOL’s data easy for even the most novice developer. These SDKs have even been published as open source projects with the aim of speeding up their conversion to SDKs that will eventually support all federal APIs.
Department of Labor
open data , API
U.S. Geological Survey and U.S. Census Bureau collaborate on national roads and boundaries data
It is a well-kept secret that the U.S. Geological Survey and the U.S. Census Bureau were the original two federal agencies to build the first national digital database of roads and boundaries in the United States. The agencies joined forces to develop homegrown computer software and state of the art technologies to convert existing USGS topographic maps of the nation to the points, lines, and polygons that fueled early GIS. Today, the USGS and Census Bureau have a longstanding goal to leverage and use roads and authoritative boundary datasets.
U.S. Geological Survey and U.S. Census Bureau
data management , data sharing , data standards , data validation , data visualization , Federal Data Strategy , geospatial , open data , quality
USA.gov Uses Human-Centered Design to Roll Out AI Chatbot
To improve customer service and give better answers to users of the USA.gov website, the Technology Transformation and Services team at General Services Administration (GSA) created a chatbot using artificial intelligence (AI) and automation.
General Services Administration
AI , Federal Data Strategy
resources.data.gov
An official website of the Office of Management and Budget, the General Services Administration, and the Office of Government Information Services.
This section contains explanations of common terms referenced on resources.data.gov.

The Business of Data
Case Studies
Starfish family services.

Environment Agency

This case study will highlight how to implement effective data standards in a large and complex organisation through a business led, collaborative approach. Download the case study here
Clients We’ve Worked With
The following is a sampling of the clients we’ve worked with.
- Member Login
- Terms & Conditions
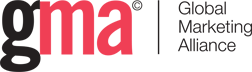
More results...
- Programmatic Advertising
- Content Marketing
- Marketing Automation
- Internet of Things
- Location marketing
- Artificial Intelligence
- Machine Learning
- Data Strategy
- Web Analytics
- Data Management
- Business Analytics
- ePrivacy Regulation
- Data Protection
- Data Governance & Security
- Latin America
- Asia-Pacific
- Eastern Europe
- In the News
- Case Studies
- Market Data
- Best practice
- Next Practice
- Most Viewed
How to build a data strategy: a step-by-step guide
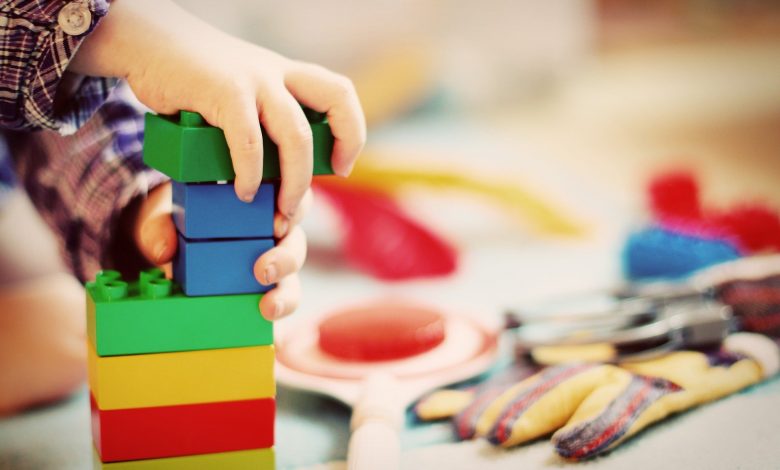
With the colossal amount of data we all have access to, understanding how to build a data strategy has never been more important. The IDC predicts that the amount of data in the world will grow from 33 zettabytes in 2019 to 175ZB by 2025
According to my calculations… that’s a whopping growth rate of 61 percent each year!
So how do those poor CMOs, CDOs and business leaders work out how to maximise the rapidly expanding potential that data offers? The answer, surprise-surprise, lies in building an effective data strategy.
Whether you’re keen to develop a data strategy or improve your existing one, this practical guide will take you on the path to success by:
- Developing your understanding of data strategy and its importance
- Providing actionable tips on how to get started
- Showcasing data strategy models and real-life case studies
We’ll begin by exploring why building a data strategy is so important…
Why you REALLY need a data strategy
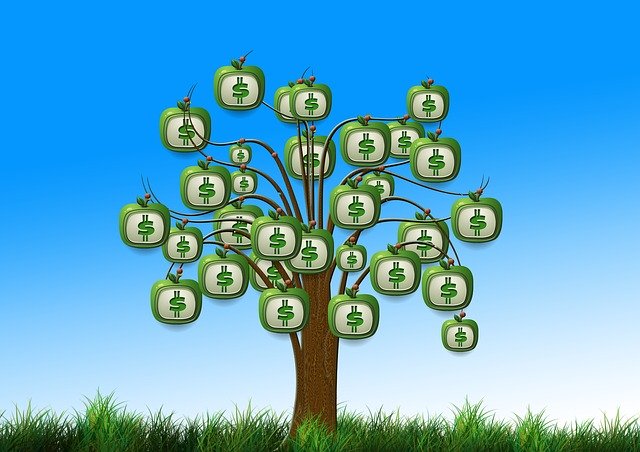
However, a 2019 study by Domo revealed that 83 percent of marketing leaders are struggling to stay on top of everything amidst new technologies and techniques. Essentially, they are running “data blind”.
Consequently, few organisations are coming close to deriving the full value out of their data. And there are two main reasons: poor strategy or a complete absence of strategy.
The issue of data overwhelm has resulted in two common ‘dangerous’ approaches from business leaders:
1. The eager beaver: the business leader who invests in new technologies and fresh analytics without understanding how it fits in with the wider business strategy and how they will be used day-to-day.
2. The overwhelmed ostrich: the business leader who can’t bear the thought of trying to handle the complexity of the data challenge and thinks it better to make-do with outdated ways of working. (Because at least they know what they’re doing!).
Hopefully, you’re looking for a different approach. One in which you grasp the opportunity that data offers by creating an actionable data strategy.
A well thought-through data strategy improves your ability to:
- Use data in your businesses
- Make data-driven/evidence-based business decisions
- Understand what your customers think and feel
- Uncover the latest trends relevant to your business
- Deliver smarter products and services
- Improve internal operations
- Monetise data
- Stay compliant with data protection regulations
And that’s just the start.
If you can piece together the disparate parts of the data jigsaw, you can gain a competitive advantage regardless of the size of your business. According to the Insights2020 study , 67% of execs at over-performing firms were skilled at linking disparate data sources; 61% of over-performers have Insights involved in all key areas of planning; and 71% of over-performing firms combine analytical and creative thinking.
“It’s not a coincidence that many of the companies who are leaders in the market also have a good handle on their data, and how to best leverage it as a strategic differentiator.” Donna Burbank, MD, Global Data Strategy.
Donna also makes it clear that it’s not just the big players who are benefiting from data strategies but also small-to-medium-sized businesses who are realising the value of using data more strategically.
While data may be considered the new oil , it’s only valuable if you have a reliable engine. And – to extend the metaphor – the right tyres, aerodynamic set-up and other constituent parts that combine to make the thing go in the right direction without breaking down. The data strategy, then, ensures all the composite parts work well and work well together.
A more formal definition of data strategy, is provided by PwC:
“….The basic goal of data strategy is to create and maintain an enterprise-wide strategy that ensures the adequate protection, quality, value and utilization of corporate data assets…”
Key takeaway: Data has a key role to play in the success or otherwise of your business. Having a strategy to ensure you gain maximum value from data is essential. Set aside time to learn how to build a data strategy and start putting it into practice.
Further reading:
- PwC: defining data strategy and its importance
- Dataversity: data strategy trends in 2018
- Forbes: Why every company needs a data strategy for 2019
- The GMA: Data strategy planning: roll with the punches and see what your data can do for you.
How to get started with building your data strategy
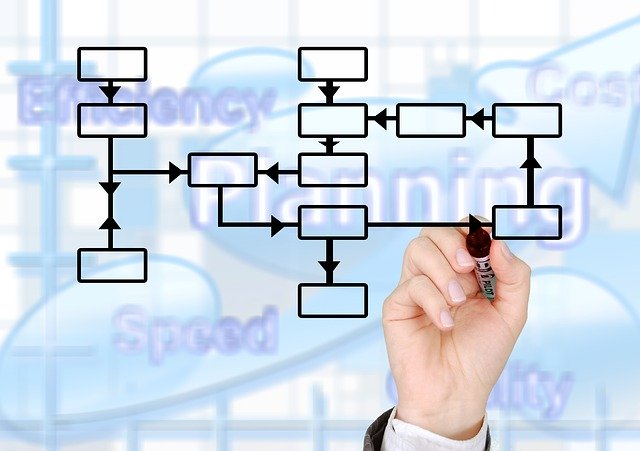
“The emphasis should be on clarity, not volume,” he said. “Too often I see key decision makers in organisations trying to figure out how to make use of all the information they can gather. To make matters worse, they are persuaded by vendors who promise even more data insights which they insist are a “must-have” for their organisation.”
“The end result is an organisation entangled in its own data.”
The key question they need to ask then is this:
“What problem am I trying to solve?”
The objective of the data strategy should draw on two vital resources:
1. Your business strategy. 2. Your people
Drawing on your business strategy Go through your business plan and consider how data can help you meet your corporate goals. On every page and through every section, identify the important role data has to play:
- What is the problem I need to solve?
- What kind of data would help?
- Where will I source it from?
- How will I store and safeguard it?
- How will I analyse it?
- Who will be responsible?
- How will it be shared across the team?
- How will it be implemented into the team’s working processes?
Relating data to your business strategy provides much needed focus and goes a long way to curing data overwhelm.
Drawing on your people
Every person in your organisation will likely have an idea of how data could help in their day-to-day roles. Perhaps there’s a specific data set that would help a content writer to better understand their audience, or a customer insight that would help a sales agent accelerate the route to sale.
Virtually every person in the organisation uses data or could be using data. If you haven’t already, it’s time to kickstart discussions with people in your team.
Key action point: Open up your business plan, talk to your people and work out how data can help grow your business and improve operational efficiency across the whole organisation. What problems are you trying to solve with your data?
- How to build a business strategy that grows your business – Cognetik
- Have one hour? Create a strategic plan on page – Forbes
- How to Build a Data Democratization and Data Governance Strategy – Heap
- Data may be the new oil, but it’s only valuable if you have a reliable engine – GMA
#Bonus tip: common mistakes to avoid
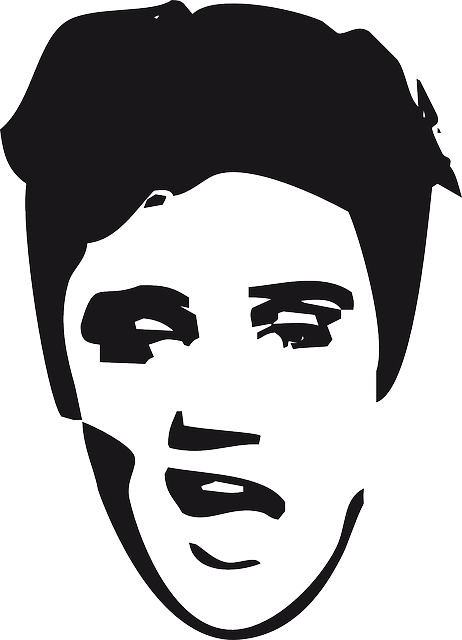
“Give humans a vague stimulus and we’ll find faces, butterflies, and a reason to allocate budget to our favorite project or launch an AI system.”
In other words, we are liable to read too much into data.
To help protect against this, here are three behaviours to avoid:
1. DO NOT collect data just because you can: There must be a clear plan and strategy in place for all the data you collect 2. DO NOT lose sight of the big picture: Big Data makes it easy to lose sight of what matters. Play it SMART instead (Specific, Measurable, Achievable, Relevant, Time-bound). 3. DO NOT present data without context: It confuses everyone. Instead present data in a way that everyone can understand, so that it relates to the wider business context. Ask yourself: how does the data relate to longer-term business goals and KPIs?
How to gain support for your data strategy
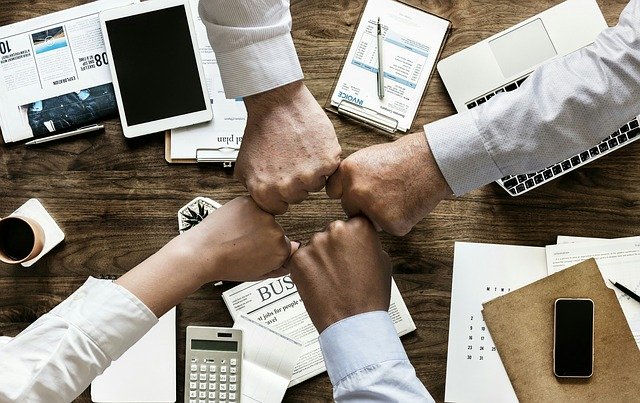
1. Transparency: share data across the business
David Siegel, CEO of Investopedia took what might be considered by some a radical step. He gave all employees at the organisation access to data detailing progress across the company. Reports were distributed to everyone, everyone was invited to weekly metrics meetings, and any employee could join any email list.
It’s worth considering that information was once a rare commodity – or at least much harder to come by than it is now. Exclusive access to information gave an individual power and so they clung to it and shared it sparingly.
But in today’s information and data rich world, it’s counterproductive. Hogging insight is bad for business. A sharing culture is much healthier. With insight shared, everyone’s wiser, everyone’s empowered to make suggestions or respond to what the data is telling them.
2. Readability: present data that anyone can understand
Data access is one thing. Understanding what it means is another. So ensuring that your data strategy makes data both accessible and understandable is of prime importance.
In 2009, Google’s Chief Economist Dr. Hal R.Varian said:
“The ability to take data—to be able to understand it, to process it, to extract value from it, to visualize it, to communicate it—that’s going to be a hugely important skill in the next decades.”
There are many ways of communicating data, such as data visualisations, infographics and dashboards. But visualisation isn’t everything: it also needs to tell a story. Any visualisation should clearly convey what the data means and why it is important.
3. Trackability: track data that monitors business performance
As a business leader or chief marketer, you need to be able to track business performance over time across key metrics. These metrics may include areas like sales revenues and customer retention rates.
Identifying the most important business performance metrics is vitally important. It ensures you are focused on what matters most and not getting lost in the minutiae of metrics. It helps you track your overall business performance and where you stand in meeting key business targets.
If you’re failing to meet your business performance goals, then it’s time to investigate the reasons why.
Which leads us to:
4. Actionability: source data that pinpoints where to take action
Your team should have access to data insight that is not only intelligible but also actionable. In other words, it must highlight a specific problem (or opportunity) that needs addressing. Adviso provides the following example of actionable insight:
“The 12% drop in our conversion rate coincides with the introduction of our new payment gateways in the checkout flow.”
It’s specific and pinpoints a clear area that needs to be addressed urgently, i.e. the payment gateway.
Key action: Put the processes in place to ensure that data insights are shared across the business. To get started, identify insights you currently have access to that other people in the organisation could benefit from.
Useful resources:
- Complete Transparency is key to success – Business Insider
- Data Storytelling: The Essential Data Science Skill Everyone Needs – Forbes
- How to turn your data into actionable insights – Adviso
- The Difference Between Reporting and Analysis and Why it Matters – The Data Hero Blog
How to build a data strategy: case studies and templates
Data strategies vary greatly depending on the size, nature and complexity of the business. However, the ambition and data literacy of decision-makers also plays a major role.
So what do data strategies look like in the real world? To help, we’re going to share some data strategy templates and case studies.
It’s reiterating noting that each template and strategy begins by aligning the data strategy with the business strategy and identifying the problem that needs solving. The strategy then moves onto ‘implementation processes’: these are the processes by which the objectives are putting into practice. They include: data governance procedures, technology & infrastructure, training & skills and change management.
Data Enterprise Ltd. provides this useful infographic for building an enterprise data strategy :
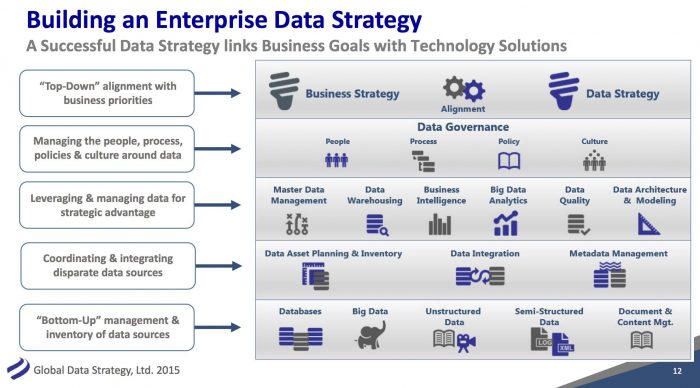
Alternatively, this data strategy roadmap from Roadmunk illustrates how to build a data strategy in stages:
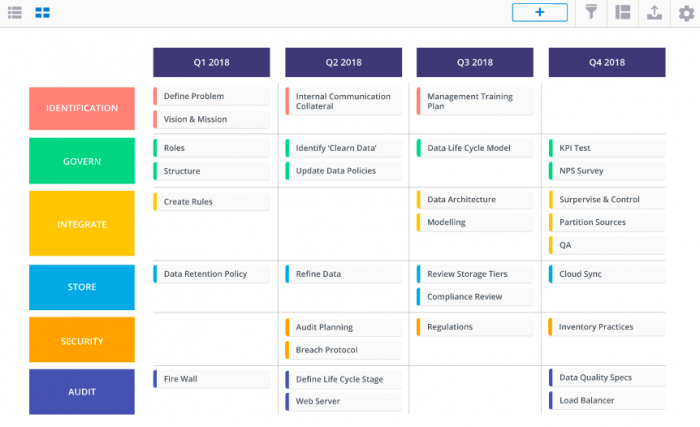
Global Data Strategy Ltd. provides four separate data strategy case studies , ranging from a consumer energy company to a professional development organisation. Each demonstrate how a data strategy was aligned to a business strategy, and the specific frameworks required to effectively utilise and govern data for their particular needs.
Key action: Start building a data strategy for your business that will meet your organisation’s most pressing needs.
- How To Develop A Data Strategy (template included) – Bernard Marr
- Data strategy roadmap: templates and examples – Roadmunk
- What’s your data strategy? – Harvard Business Review
- The Executive’s Guide to Assessing and Improving Your Data Strategy – Concepta
It’s time to see clearly
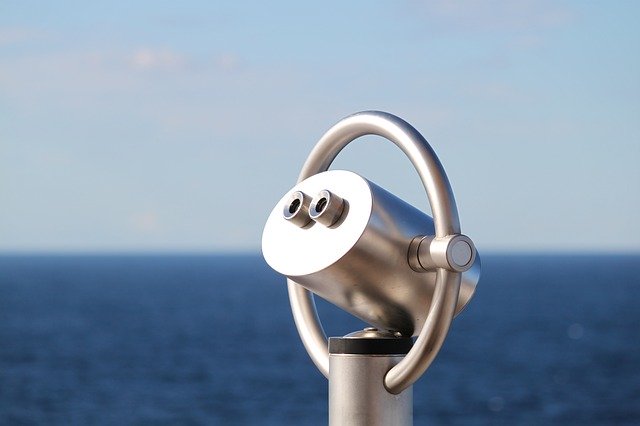
This point was perhaps best summed up by Samir Sharma, CEO at Datazuum at a GMA Data Briefing in 2019 :
Leaders must understand what data can do and how it can be harnessed. The result will be improved decision-making and the ability to foster a company culture where the true value of data is grasped by all.
Are there any data strategy tips I’ve missed? Feel free to add them in the comments below.
Title image by Esi Grünhagen from Pixabay
Please register below to unlock this article.
An email will be sent to you with your membership details.
Basic Information
Account information.
Share a little biographical information to fill out your profile. This may be shown publicly.
Terms and Conditions
Terms and conditions – website usage
Welcome to the G lobal Marketing Alliance (GMA) . The GMA website is a product of the Global Marketing Alliance Ltd. If you continue to browse and use this website, you are agreeing to comply with the following terms and conditions of use, which together with our privacy policy, govern GMA’s dealings with you in relation to this website. If you disagree with any part of these terms and conditions, please do not use our website.
GMA may amend these Terms and Conditions at any time by posting the amended Terms and Conditions on the GMA site.
The term GMA or ‘us’ or ‘we’ refers to the owner of the website whose registered office is at 7a Queen Street, Godalming, Surrey, GU7 1BA, UK. The term ‘you’ refers to the user or viewer of our website or to those who become members of GMA.
The use of this website is subject to the following terms of use:
- The content of the pages of this website is for your general information and use only. It is subject to change without notice.
- The information provided and the opinions expressed in this website represent the views of the authors and contributors.
- Neither we nor any third parties provide any warranty or guarantee as to the accuracy, timeliness, performance, completeness or suitability of the information and materials found or offered on this website for any particular purpose. We are not liable for any inaccuracies or errors.
- Your use of any information or materials on this website is entirely at your own risk, it is your responsibility to check that the information available meets your requirements.
- This website contains material which is owned by or licensed to us. This material includes, but is not limited to, the design, layout, look, appearance and graphics. Reproduction is prohibited other than in accordance with the copyright notice, which forms part of these terms and conditions.
- Information on this site may be contributed by our members or other contributors (including in blogs). We do not control the information they provide and are not responsible for the views expressed
- If you believe that your intellectual property rights have been infringed on this site please notify us. We accept no liability for infringements as a result of user generated content but we will take reasonable action to remove such content where possible.
- Unauthorised use of this website may give rise to a claim for damages and/or be a criminal offence.
- Every effort is made to keep the website up and running smoothly. However, we take no responsibility for, and will not be liable for, the website being temporarily unavailable due to technical issues beyond our control.
- We reserve the right to refuse, suspend or cancel your access to the site at any time and without notice.
- If you are acting as an employee, you warrant that you are authorised to enter into legally binding contracts on behalf of your employer. You further warrant that your employer agrees to be bound by these Terms and Conditions.
- Purchases of training products or delegate places at events advertised on this site are subject to the terms and conditions of the providers.
- Your use of this website and any dispute arising out of such use of the website is subject to the laws of England, Northern Ireland, Scotland and Wales.
Copyright notice
This website and its content are copyright of Global Marketing Alliance Ltd and our contributors. All rights reserved.
Any redistribution or reproduction of part or all of the contents in any form is prohibited other than the following:
- you may print extracts for your personal and non-commercial use only
- you may refer to and quote from the content for review or reference purposes within the limits of “fair dealing”, but only if you acknowledge the website as the source of the material
- You may not, except with our express written permission, distribute or commercially exploit the content. Nor may you transmit it or store it in any other website or other form of electronic retrieval system.
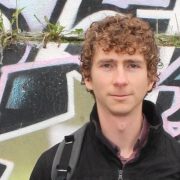
Editor and freelance writer wading his way through the world of data. One step at a time. Interested in data driven marketing insights supported by... data.
Leave your thoughts
Related reading.
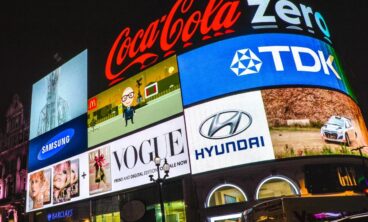
Building brand awareness: Companies reveal their strategies
New research from LinkedIn reveals that at any one time of B B buyers are not in the market for the thing you are selling so while all brands want instant results building awareness will always be key Whether you ...
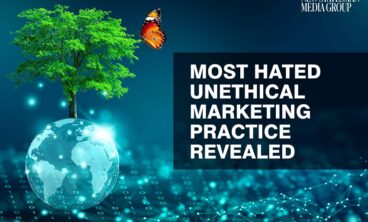
Worst examples of unethical marketing revealed in new survey
There is a litany of poor marketing and advertising practices remembered in a new survey from the New Statesman Media Group New Statesman Press Gazette sustainable luxury lifestyle and ESG publishers From Fyre Festival to Kendall Jenner and Pepsi to ...
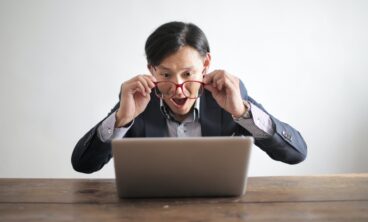
"I've never seen anything like this before in my life!"
Drayton Bird recently received an email that left him astounded. It was the work of a mad man! Yet its level of crazy was matched by its brilliance. On your marks... Get set... Go!
Keep up to date with global best practice in data driven marketing
- Email This field is for validation purposes and should be left unchanged.
- Privacy Policy

Home » Case Study – Methods, Examples and Guide
Case Study – Methods, Examples and Guide
Table of Contents

A case study is a research method that involves an in-depth examination and analysis of a particular phenomenon or case, such as an individual, organization, community, event, or situation.
It is a qualitative research approach that aims to provide a detailed and comprehensive understanding of the case being studied. Case studies typically involve multiple sources of data, including interviews, observations, documents, and artifacts, which are analyzed using various techniques, such as content analysis, thematic analysis, and grounded theory. The findings of a case study are often used to develop theories, inform policy or practice, or generate new research questions.
Types of Case Study
Types and Methods of Case Study are as follows:
Single-Case Study
A single-case study is an in-depth analysis of a single case. This type of case study is useful when the researcher wants to understand a specific phenomenon in detail.
For Example , A researcher might conduct a single-case study on a particular individual to understand their experiences with a particular health condition or a specific organization to explore their management practices. The researcher collects data from multiple sources, such as interviews, observations, and documents, and uses various techniques to analyze the data, such as content analysis or thematic analysis. The findings of a single-case study are often used to generate new research questions, develop theories, or inform policy or practice.
Multiple-Case Study
A multiple-case study involves the analysis of several cases that are similar in nature. This type of case study is useful when the researcher wants to identify similarities and differences between the cases.
For Example, a researcher might conduct a multiple-case study on several companies to explore the factors that contribute to their success or failure. The researcher collects data from each case, compares and contrasts the findings, and uses various techniques to analyze the data, such as comparative analysis or pattern-matching. The findings of a multiple-case study can be used to develop theories, inform policy or practice, or generate new research questions.
Exploratory Case Study
An exploratory case study is used to explore a new or understudied phenomenon. This type of case study is useful when the researcher wants to generate hypotheses or theories about the phenomenon.
For Example, a researcher might conduct an exploratory case study on a new technology to understand its potential impact on society. The researcher collects data from multiple sources, such as interviews, observations, and documents, and uses various techniques to analyze the data, such as grounded theory or content analysis. The findings of an exploratory case study can be used to generate new research questions, develop theories, or inform policy or practice.
Descriptive Case Study
A descriptive case study is used to describe a particular phenomenon in detail. This type of case study is useful when the researcher wants to provide a comprehensive account of the phenomenon.
For Example, a researcher might conduct a descriptive case study on a particular community to understand its social and economic characteristics. The researcher collects data from multiple sources, such as interviews, observations, and documents, and uses various techniques to analyze the data, such as content analysis or thematic analysis. The findings of a descriptive case study can be used to inform policy or practice or generate new research questions.
Instrumental Case Study
An instrumental case study is used to understand a particular phenomenon that is instrumental in achieving a particular goal. This type of case study is useful when the researcher wants to understand the role of the phenomenon in achieving the goal.
For Example, a researcher might conduct an instrumental case study on a particular policy to understand its impact on achieving a particular goal, such as reducing poverty. The researcher collects data from multiple sources, such as interviews, observations, and documents, and uses various techniques to analyze the data, such as content analysis or thematic analysis. The findings of an instrumental case study can be used to inform policy or practice or generate new research questions.
Case Study Data Collection Methods
Here are some common data collection methods for case studies:
Interviews involve asking questions to individuals who have knowledge or experience relevant to the case study. Interviews can be structured (where the same questions are asked to all participants) or unstructured (where the interviewer follows up on the responses with further questions). Interviews can be conducted in person, over the phone, or through video conferencing.
Observations
Observations involve watching and recording the behavior and activities of individuals or groups relevant to the case study. Observations can be participant (where the researcher actively participates in the activities) or non-participant (where the researcher observes from a distance). Observations can be recorded using notes, audio or video recordings, or photographs.
Documents can be used as a source of information for case studies. Documents can include reports, memos, emails, letters, and other written materials related to the case study. Documents can be collected from the case study participants or from public sources.
Surveys involve asking a set of questions to a sample of individuals relevant to the case study. Surveys can be administered in person, over the phone, through mail or email, or online. Surveys can be used to gather information on attitudes, opinions, or behaviors related to the case study.
Artifacts are physical objects relevant to the case study. Artifacts can include tools, equipment, products, or other objects that provide insights into the case study phenomenon.
How to conduct Case Study Research
Conducting a case study research involves several steps that need to be followed to ensure the quality and rigor of the study. Here are the steps to conduct case study research:
- Define the research questions: The first step in conducting a case study research is to define the research questions. The research questions should be specific, measurable, and relevant to the case study phenomenon under investigation.
- Select the case: The next step is to select the case or cases to be studied. The case should be relevant to the research questions and should provide rich and diverse data that can be used to answer the research questions.
- Collect data: Data can be collected using various methods, such as interviews, observations, documents, surveys, and artifacts. The data collection method should be selected based on the research questions and the nature of the case study phenomenon.
- Analyze the data: The data collected from the case study should be analyzed using various techniques, such as content analysis, thematic analysis, or grounded theory. The analysis should be guided by the research questions and should aim to provide insights and conclusions relevant to the research questions.
- Draw conclusions: The conclusions drawn from the case study should be based on the data analysis and should be relevant to the research questions. The conclusions should be supported by evidence and should be clearly stated.
- Validate the findings: The findings of the case study should be validated by reviewing the data and the analysis with participants or other experts in the field. This helps to ensure the validity and reliability of the findings.
- Write the report: The final step is to write the report of the case study research. The report should provide a clear description of the case study phenomenon, the research questions, the data collection methods, the data analysis, the findings, and the conclusions. The report should be written in a clear and concise manner and should follow the guidelines for academic writing.
Examples of Case Study
Here are some examples of case study research:
- The Hawthorne Studies : Conducted between 1924 and 1932, the Hawthorne Studies were a series of case studies conducted by Elton Mayo and his colleagues to examine the impact of work environment on employee productivity. The studies were conducted at the Hawthorne Works plant of the Western Electric Company in Chicago and included interviews, observations, and experiments.
- The Stanford Prison Experiment: Conducted in 1971, the Stanford Prison Experiment was a case study conducted by Philip Zimbardo to examine the psychological effects of power and authority. The study involved simulating a prison environment and assigning participants to the role of guards or prisoners. The study was controversial due to the ethical issues it raised.
- The Challenger Disaster: The Challenger Disaster was a case study conducted to examine the causes of the Space Shuttle Challenger explosion in 1986. The study included interviews, observations, and analysis of data to identify the technical, organizational, and cultural factors that contributed to the disaster.
- The Enron Scandal: The Enron Scandal was a case study conducted to examine the causes of the Enron Corporation’s bankruptcy in 2001. The study included interviews, analysis of financial data, and review of documents to identify the accounting practices, corporate culture, and ethical issues that led to the company’s downfall.
- The Fukushima Nuclear Disaster : The Fukushima Nuclear Disaster was a case study conducted to examine the causes of the nuclear accident that occurred at the Fukushima Daiichi Nuclear Power Plant in Japan in 2011. The study included interviews, analysis of data, and review of documents to identify the technical, organizational, and cultural factors that contributed to the disaster.
Application of Case Study
Case studies have a wide range of applications across various fields and industries. Here are some examples:
Business and Management
Case studies are widely used in business and management to examine real-life situations and develop problem-solving skills. Case studies can help students and professionals to develop a deep understanding of business concepts, theories, and best practices.
Case studies are used in healthcare to examine patient care, treatment options, and outcomes. Case studies can help healthcare professionals to develop critical thinking skills, diagnose complex medical conditions, and develop effective treatment plans.
Case studies are used in education to examine teaching and learning practices. Case studies can help educators to develop effective teaching strategies, evaluate student progress, and identify areas for improvement.
Social Sciences
Case studies are widely used in social sciences to examine human behavior, social phenomena, and cultural practices. Case studies can help researchers to develop theories, test hypotheses, and gain insights into complex social issues.
Law and Ethics
Case studies are used in law and ethics to examine legal and ethical dilemmas. Case studies can help lawyers, policymakers, and ethical professionals to develop critical thinking skills, analyze complex cases, and make informed decisions.
Purpose of Case Study
The purpose of a case study is to provide a detailed analysis of a specific phenomenon, issue, or problem in its real-life context. A case study is a qualitative research method that involves the in-depth exploration and analysis of a particular case, which can be an individual, group, organization, event, or community.
The primary purpose of a case study is to generate a comprehensive and nuanced understanding of the case, including its history, context, and dynamics. Case studies can help researchers to identify and examine the underlying factors, processes, and mechanisms that contribute to the case and its outcomes. This can help to develop a more accurate and detailed understanding of the case, which can inform future research, practice, or policy.
Case studies can also serve other purposes, including:
- Illustrating a theory or concept: Case studies can be used to illustrate and explain theoretical concepts and frameworks, providing concrete examples of how they can be applied in real-life situations.
- Developing hypotheses: Case studies can help to generate hypotheses about the causal relationships between different factors and outcomes, which can be tested through further research.
- Providing insight into complex issues: Case studies can provide insights into complex and multifaceted issues, which may be difficult to understand through other research methods.
- Informing practice or policy: Case studies can be used to inform practice or policy by identifying best practices, lessons learned, or areas for improvement.
Advantages of Case Study Research
There are several advantages of case study research, including:
- In-depth exploration: Case study research allows for a detailed exploration and analysis of a specific phenomenon, issue, or problem in its real-life context. This can provide a comprehensive understanding of the case and its dynamics, which may not be possible through other research methods.
- Rich data: Case study research can generate rich and detailed data, including qualitative data such as interviews, observations, and documents. This can provide a nuanced understanding of the case and its complexity.
- Holistic perspective: Case study research allows for a holistic perspective of the case, taking into account the various factors, processes, and mechanisms that contribute to the case and its outcomes. This can help to develop a more accurate and comprehensive understanding of the case.
- Theory development: Case study research can help to develop and refine theories and concepts by providing empirical evidence and concrete examples of how they can be applied in real-life situations.
- Practical application: Case study research can inform practice or policy by identifying best practices, lessons learned, or areas for improvement.
- Contextualization: Case study research takes into account the specific context in which the case is situated, which can help to understand how the case is influenced by the social, cultural, and historical factors of its environment.
Limitations of Case Study Research
There are several limitations of case study research, including:
- Limited generalizability : Case studies are typically focused on a single case or a small number of cases, which limits the generalizability of the findings. The unique characteristics of the case may not be applicable to other contexts or populations, which may limit the external validity of the research.
- Biased sampling: Case studies may rely on purposive or convenience sampling, which can introduce bias into the sample selection process. This may limit the representativeness of the sample and the generalizability of the findings.
- Subjectivity: Case studies rely on the interpretation of the researcher, which can introduce subjectivity into the analysis. The researcher’s own biases, assumptions, and perspectives may influence the findings, which may limit the objectivity of the research.
- Limited control: Case studies are typically conducted in naturalistic settings, which limits the control that the researcher has over the environment and the variables being studied. This may limit the ability to establish causal relationships between variables.
- Time-consuming: Case studies can be time-consuming to conduct, as they typically involve a detailed exploration and analysis of a specific case. This may limit the feasibility of conducting multiple case studies or conducting case studies in a timely manner.
- Resource-intensive: Case studies may require significant resources, including time, funding, and expertise. This may limit the ability of researchers to conduct case studies in resource-constrained settings.
About the author
Muhammad Hassan
Researcher, Academic Writer, Web developer
You may also like

Correlational Research – Methods, Types and...

Basic Research – Types, Methods and Examples

One-to-One Interview – Methods and Guide

Mixed Methods Research – Types & Analysis

Transformative Design – Methods, Types, Guide

Quasi-Experimental Research Design – Types...
What the Case Study Method Really Teaches
by Nitin Nohria

Summary .
During my decade as dean of Harvard Business School, I spent hundreds of hours talking with our alumni. To enliven these conversations, I relied on a favorite question: “What was the most important thing you learned from your time in our MBA program?”
Partner Center
New Atlan Named a Leader in The Forrester Wave™: Enterprise Data Catalogs, Q3 2024. Read Full Report Learn More
The Forrester Wave™: Enterprise Data Catalogs for DataOps, Q2 2022
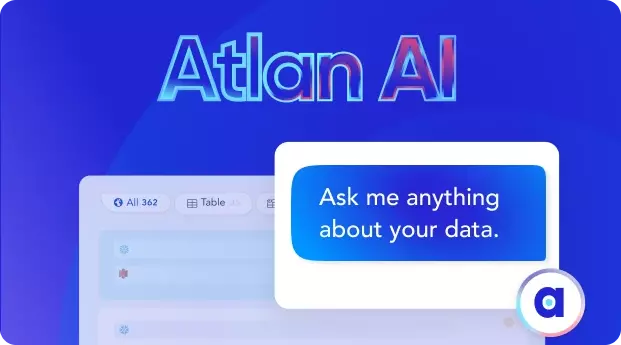
- Document hundreds of tables on autopilot
- Explore data with natural language
- Ask any question about your data stack to your personal AI copilot.
Start integrating with Atlan on the go
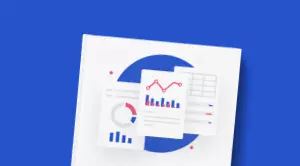
The role of active metadata in the modern data stack
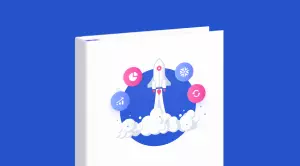
A deep dive into the 10 data trends you should know
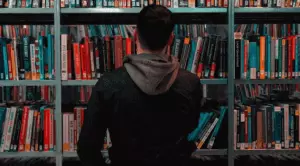
May 24, 2022
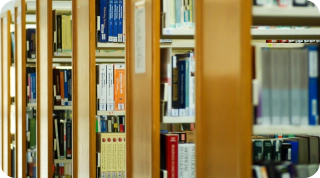
May 10, 2023
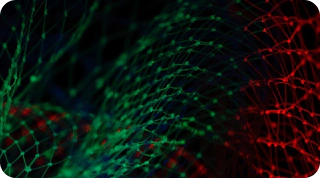
Feb 02, 2022
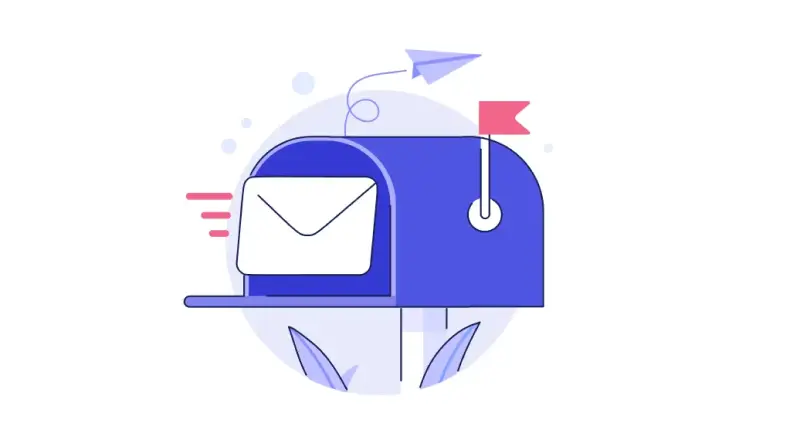
Join over 5k data leaders from companies like Amazon, Apple, and Spotify who subscribe to our weekly newsletter.
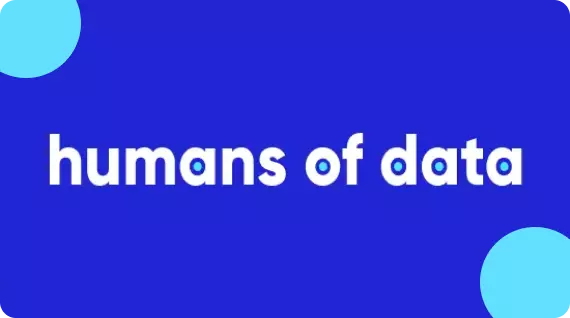
Best practices for building a collaborative data culture
5 Data Governance Examples: Case Studies from Airbnb, Uber, and More
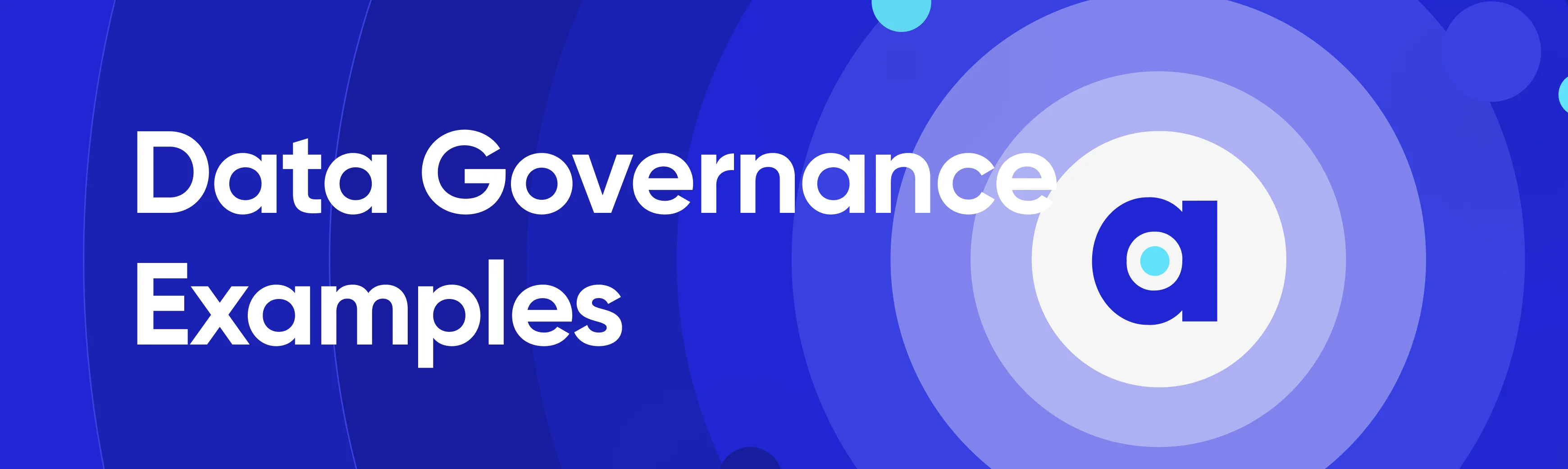
Share this article
Data governance goes beyond policies. It elevates trust in your data and empowers you to make better decisions, improve operational efficiency and save costs.
But what does successful data governance look like in action?
Let’s dive into five real-world data governance examples from top organizations, such as Airbnb, GE Aviation, Wells Fargo, CSE Insurance, and Uber.
Table of contents #
- Data governance examples: 5 case studies
- Data governance examples: 5 key takeaways
- Related reads
Data governance examples: 5 case studies to visualize the impact of data governance #
Let’s take a closer look at five data governance examples , each showcasing how data governance can drive success and contribute toward your business outcomes:
- How Airbnb used data literacy to promote data-driven decision-making
- How GE Aviation balanced data governance with data enablement
- How Wells Fargo built a single source of truth to ensure proper governance
- How CSE Insurance transformed its data culture for better data management
- How Uber set up a flexible approach to data governance
Let’s explore each case study further.
How Airbnb used data literacy to promote data-driven decision-making #
At Airbnb, effective data governance encompasses data management and enhancing data literacy.
How are data literacy and data governance connected?
Data literacy is a crucial component of data governance as it supports the ability to read, understand, create, and communicate data.
Organizations that do not fully understand their data, lose control of it throughout its lifecycle. Moreover, when people are fluent in the generation, use, and application of data, teams can make data-informed decisions.
Data literacy also makes them use data responsibly, which is essential for the success of any data governance program.
How did Airbnb promote data literacy?
To ensure data literacy, Airbnb has launched an in-house educational initiative called “ Data University .”
The program is designed to boost data literacy across all departments, equipping employees to understand, interpret, and use data effectively in their roles.
Data University is Airbnb’s dynamic data education program , with the vision to empower every employee to make data-informed decisions .
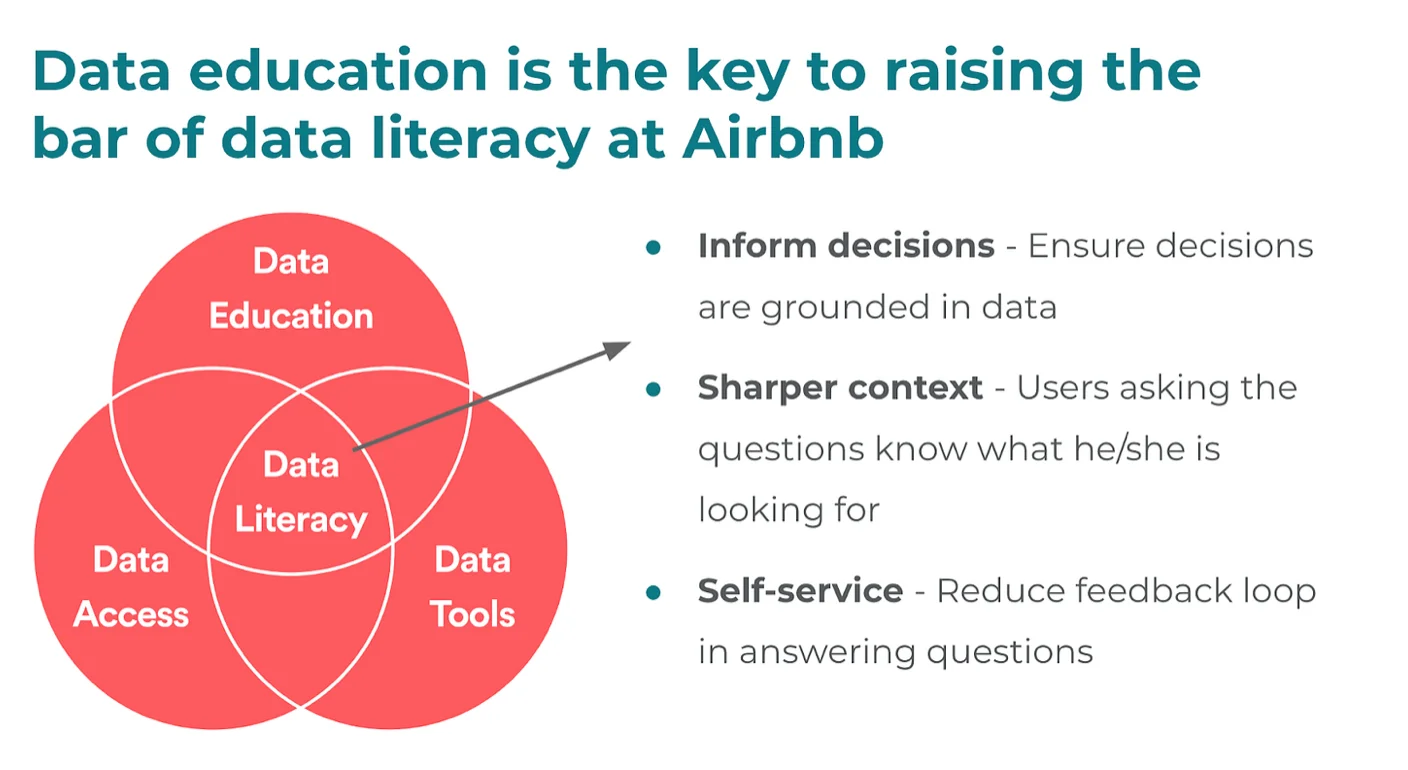
Data literacy at Airbnb helped democratize data. - Source: Medium.
The success of this initiative is reflected in the fact that 45% of Airbnb is now a WAU (weekly active user) of their internal data platform .
As a result, Airbnb could democratize data and scale decision-making, while ensuring responsible use of their data.
Also, read → How to measure data literacy
How GE Aviation balanced data governance with data enablement #
GE Aviation embarked on a mission to centralize its scattered data sources, making it more accessible and reliable for all users within the organization. They called the initiative Self-Service Data (SSD).
“ Self-service initiatives often fail in large enterprises for a variety of reasons. The issues boil down to a larger problem: self-service gets treated as a one-time project that gets launched, then forgotten.”
That’s why GE Aviation got started by setting up the SSD data team responsible for user enablement, tooling, data product (i.e., dashboards) deployment, and exploring new opportunities to improve processes.
Simultaneously, GE Aviation also set up a Database Admin team responsible for data governance, proper use of data, and supporting users whenever required. They ensured that all data products are well-documented, have project owners, and describe the workflow of approvers in detail.
What is the process for deploying data products at GE Aviation?
There’s a 4-step process to deploying data products (i.e., dashboards):
- Any user can look up and use the datasets for which they have access permissions. The datasets being used for setting up the data product must be tagged appropriately.
- After adhering to the compliance rules, users can create projects in a design environment to explore that data and test various data pipelines.
- Once the user pushes their project to production, a series of checks occur — verifying naming conventions, checking the schemas being used, ensuring proper data distribution, verifying dataset size, etc. The Database Admin and Self-Service Data teams run these checks manually.
- If the data product passes all checks, it is automatically pushed to the production environment.
According to Jonathan Tudor , the former Director of Data Platforms and Governance at GE Aviation:
“ It’s incredibly important to create and communicate common definitions and processes so that you and your user base are speaking the same language and to ensure that you use automation to enforce those definitions and processes. Otherwise, you’ll have chaos instead of outcomes .”
The approach accelerated decision-making processes and instilled a sense of data ownership among employees. This has led to improvements in safety and operational efficiency.
Read more → Data governance framework examples, templates, standards, and best practices
How Wells Fargo built a single source of truth to ensure proper governance #
Wells Fargo’s data governance strategy highlights the importance of creating a single source of truth to enhance data accuracy and reliability.
Their approach was to centralize data from multiple sources to create a unified, trustworthy source that reduced discrepancies and improved consistency.
This streamlined data management and allowed for more accurate reporting and analysis, thereby enhancing decision-making across the organization.
Here’s how Prahalad Thota , the former Senior Vice President, Head of Enterprise Analytics & Data Science at Wells Fargo, describes their efforts:
“ We established a team that brings data together and organizes it into our Enterprise Data League. The team focuses on establishing the right governance, in terms of storage and management, for the data. The team also focuses on leveraging data for driving cutting-edge use cases .”
Wells Fargo’s data governance strategy also emphasized the importance of data visualization (using Tableau) to make data more accessible to non-technical stakeholders and improve overall data literacy.
As a result, Wells Fargo was able to ensure data consistency, accuracy, and visualization to reduce risks and foster data-driven decision-making.
Read more → How do large organizations avoid data silos
How CSE Insurance transformed its data culture for better data management #
CSE Insurance offers property and casualty insurance across the United States. Like many other insurance companies, they faced challenges in governing and managing their data.
The data was siloed across various sources, making it difficult for teams to find, access, and use the information they needed.
Moreover, basic definitions and metrics weren’t consistent, and migrating their documentation required starting everything from scratch.
To remedy these issues, CSE Insurance set up a single source of truth powered by data governance policies and procedures .
They also set up a small group of data champions to kick off their documentation initiative using a single platform to document data. The platform also helped all users get complete context with shareable data profiles and collaborate effectively.
As a result, CSE Insurance was able to transform its data culture and manage data more efficiently.
Also, read → Modern data culture
How Uber set up a flexible approach to data governance #
Uber operates in 70+ countries, each with its own data governance framework to comply with local and regional laws.
To ensure compliance across all regions, Uber uses a core platform to take care of data privacy and security centrally. Uber collects data globally but adapts its governance policies depending on the origins of each dataset by using customizations and plugins.
Here’s how Manikandan Thangarathnam , the Senior Director of Mobility and Platforms at Uber, puts it:
“ For example, a city or a country may have a rule by which they do not want to expose the driver’s name to the rider. So we have the capability to switch on or off depending on the city ; we do not have to create a different application for that. That is the amount of engineering and flexibility that we have built into our system .”
The company has invested in training its people and honing their skills to ensure the success of its data governance programs across all regions.
Data governance examples: 5 key takeaways #
Having explored real-world data governance examples, let’s highlight some of the vital lessons from these stories:
- Establish a clear data governance structure with well-defined roles and responsibilities , as demonstrated by GE Aviation’s SSD and Data Admin teams
- Set up a single source of truth , as CSE Insurance and Wells Fargo did, to eliminate data silos and make your data governance efforts more streamlined
- Treat data as a product and assign owners responsible for ensuring its adherence to your rules, policies, and standards
- Adopt a flexible , rather than a ‘one-size-fits-all’, approach to data governance
- Invest in enhancing data literacy so that your employees can understand, interpret, and use data effectively and responsibly, just like Airbnb did with its in-house educational initiative
Read more → Data governance best practices
Bottomline #
It’s crucial to remember that effective data governance goes beyond compliance and risk management — it serves as a strategic catalyst for cultivating trust and generating value from data.
Drawing insights from the successful data governance practices of established organizations helps you learn how to balance compliance with data enablement and democratization.
Such insights help you overcome common obstacles and develop a data governance program that drives business growth, besides helping you navigate the regulatory landscape.
Data governance examples: Related reads #
- What is Data Governance? Its Importance, Principles & How to Get Started?
- Data Governance in Manufacturing : Steps, Challenges, and Practical Examples
- Data Governance in Retail : Best Practices, Challenges, and Viable Solutions
- Data Governance in Insurance : Why is it Important and How it Drives Positive Business Outcomes
- Key Objectives of Data Governance : How Should You Think About Them?
- Data Governance Framework — Examples, Templates, Standards, Best Practices & How to Create One?
- Data Governance and Compliance : Act of Checks & Balances
- How to implement data governance? Steps, Prerequisites, Essential Factors & Business Case
- How to Improve Data Governance? Steps, Tips & Template
- 7 Steps to Simplify Data Governance for Your Entire Organization
Qualitative case study data analysis: an example from practice
Affiliation.
- 1 School of Nursing and Midwifery, National University of Ireland, Galway, Republic of Ireland.
- PMID: 25976531
- DOI: 10.7748/nr.22.5.8.e1307
Aim: To illustrate an approach to data analysis in qualitative case study methodology.
Background: There is often little detail in case study research about how data were analysed. However, it is important that comprehensive analysis procedures are used because there are often large sets of data from multiple sources of evidence. Furthermore, the ability to describe in detail how the analysis was conducted ensures rigour in reporting qualitative research.
Data sources: The research example used is a multiple case study that explored the role of the clinical skills laboratory in preparing students for the real world of practice. Data analysis was conducted using a framework guided by the four stages of analysis outlined by Morse ( 1994 ): comprehending, synthesising, theorising and recontextualising. The specific strategies for analysis in these stages centred on the work of Miles and Huberman ( 1994 ), which has been successfully used in case study research. The data were managed using NVivo software.
Review methods: Literature examining qualitative data analysis was reviewed and strategies illustrated by the case study example provided. Discussion Each stage of the analysis framework is described with illustration from the research example for the purpose of highlighting the benefits of a systematic approach to handling large data sets from multiple sources.
Conclusion: By providing an example of how each stage of the analysis was conducted, it is hoped that researchers will be able to consider the benefits of such an approach to their own case study analysis.
Implications for research/practice: This paper illustrates specific strategies that can be employed when conducting data analysis in case study research and other qualitative research designs.
Keywords: Case study data analysis; case study research methodology; clinical skills research; qualitative case study methodology; qualitative data analysis; qualitative research.
- Case-Control Studies*
- Data Interpretation, Statistical*
- Nursing Research / methods*
- Qualitative Research*
- Research Design
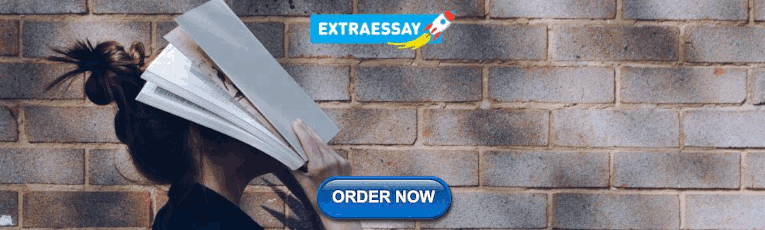
IMAGES
VIDEO
COMMENTS
Strategy & Structure: Independent of the current structure, design a long term approach to data that implements the overall company strategy through a staged delivery plan. Transformation: Build the delivery plan for how existing tools, roles and responsibilities can be transitioned, and how new tools and processes should be implemented
Developing the Data Strategy. For McLean, it became clear that developing a Data Strategy was not going to happen over a weekend, especially within the context of a government agency with longstanding bureaucratic norms and entrenched ways of doing things. It would be a multi-year endeavor, requiring organization, patience, and support.
Read more on Data management or related topics Competitive strategy and IT management A version of this article appeared in the May-June 2017 issue of Harvard Business Review. LD
Discover real-world case studies of Data-Driven Decision-Making (DDDM) and how companies like Netflix, Amazon, and Starbucks leveraged data for success. ... Data is a Strategic Asset: Netflix's strategic use of data has wholly revolutionized content delivery. By utilizing excellent viewer data, they have successfully met the needs and ...
Federal CDO Data Skills Training Program Case Studies. This series was developed by the Chief Data Officer Council's Data Skills & Workforce Development Working Group to provide support to agencies in implementing the Federal Data Strategy's Agency Action 4 gap-closing strategy training component in FY21.
Case studies are a preferred strategy when the researcher has little control over events and when the focus is on contemporary phenomenon within some real-life context ... The authors interpreted the raw data for case studies with the help of a four-step interpretation process (PESI). Raw empirical material, in the form of texts from interviews ...
John Ladley is an experienced practitioner who helps organizations define and transition to new business and data capabilities. His work and books enable alignment of business and data strategy ...
It is unique given one characteristic: case studies draw from more than one data source. In this post find definitions and a collection of multidisciplinary examples. ... Methodological Uncertainty and Multi-Strategy Analysis: Case Study of the Long-Term Effects of Government Sponsored Youth Training on Occupational Mobility.
A case study is one of the most commonly used methodologies of social research. This article attempts to look into the various dimensions of a case study research strategy, the different epistemological strands which determine the particular case study type and approach adopted in the field, discusses the factors which can enhance the effectiveness of a case study research, and the debate ...
A case study is a detailed study of a specific subject, such as a person, group, place, event, organization, or phenomenon. Case studies are commonly used in social, educational, clinical, and business research. A case study research design usually involves qualitative methods, but quantitative methods are sometimes also used.
Collaboration and consensus-building played a critical part in the success of this initiative, and data models were a helpful tool for building consensus in the production of data standards and shared reference data. This case study will highlight how to implement effective data standards in a large and complex organisation through a business ...
Global Data Strategy Ltd. provides four separate data strategy case studies, ranging from a consumer energy company to a professional development organisation. Each demonstrate how a data strategy was aligned to a business strategy, and the specific frameworks required to effectively utilise and govern data for their particular needs.
Case studies can help educators to develop effective teaching strategies, evaluate student progress, and identify areas for improvement. ... Rich data: Case study research can generate rich and detailed data, including qualitative data such as interviews, observations, and documents. This can provide a nuanced understanding of the case and its ...
136 CASE STUDY RESEARCH data, and rival explanations. All four strategies underlie the analytic techniques to be described below. Without such strategies (or alternatives to them), case study analysis will proceed with difficulty. The remainder of this chapter covers the specific analytic techniques, to be
It's been 100 years since Harvard Business School began using the case study method. Beyond teaching specific subject matter, the case study method excels in instilling meta-skills in students.
Data governance examples: 5 case studies to visualize the impact of data governance #. Let's take a closer look at five data governance examples, each showcasing how data governance can drive success and contribute toward your business outcomes:. How Airbnb used data literacy to promote data-driven decision-making; How GE Aviation balanced data governance with data enablement
Qualitative case study methodology provides tools for researchers to study complex phenomena within their contexts. When the approach is applied correctly, it becomes a valuable method for health ...
Data Quality and Accessibility: The quality and readiness of the necessary data for a project is also a significant factor. Projects might be prioritized if the required data is readily available and high-quality. Senior executives and product leaders at Spotify guide the overall strategy for data projects.
The specific strategies for analysis in these stages centred on the work of Miles and Huberman ( 1994 ), which has been successfully used in case study research. The data were managed using NVivo software. Review methods: Literature examining qualitative data analysis was reviewed and strategies illustrated by the case study example provided ...
It is a good mix of contemporary as well as classic case studies on big data strategy that have withstood the test of time. For instance, the Netflix case study has been a global bestseller right ...