4 Case Study Questions for Interviewing Data Analysts at a Startup
A good data analyst is one who has an absolute passion for data, he/she has a strong understanding of the business/product you are running, and will be always seeking meaningful insights to help the team make better decisions.
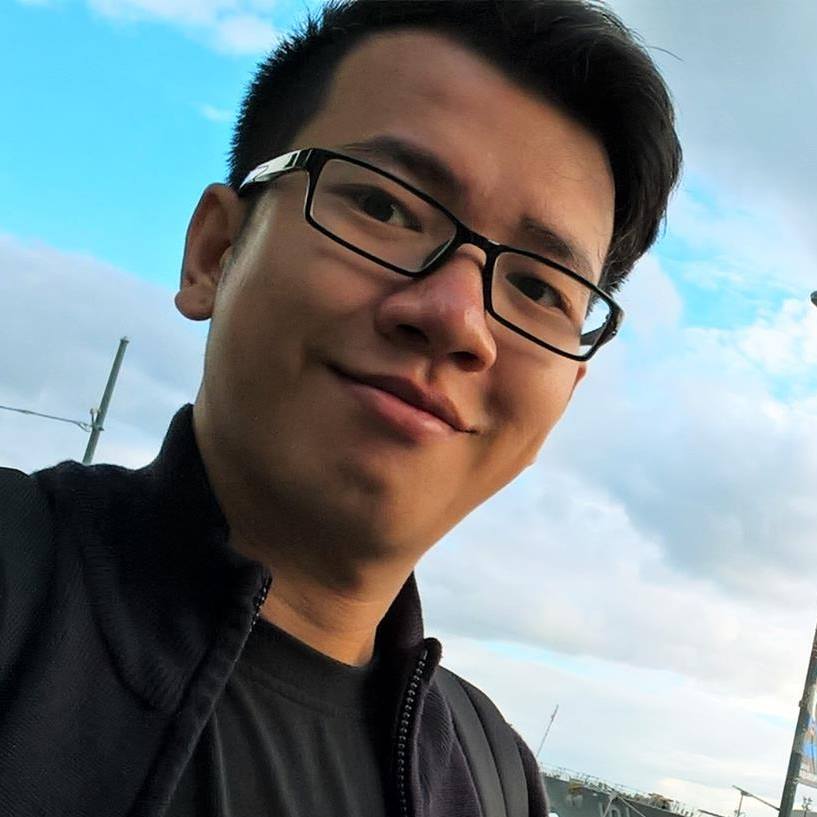
Jan 22, 2019 . 4 min read
- If you're an aspiring data professionals wanting to learn more about how the underlying data world works, check out: The Analytics Setup Guidebook
- Doing a case study as part of analytics interview? Check out: Nailing An Analytics Interview Case Study: 10 Practical Tips
At Holistics, we understand the value of data in making business decisions as a Business Intelligence (BI) platform, and hiring the right data team is one of the key elements to get you there.
To get hired for a tech product startup, we all know just doing reporting alone won't distinguish a potential data analyst, a good data analyst is one who has an absolute passion for data. He/she has a strong understanding of the business/product you are running, and will be always seeking meaningful insights to help the team make better decisions.
That's the reason why we usually look for these characteristics below when interviewing data analyst candidates:
- Ability to adapt to a new domain quickly
- Ability to work independently to investigate and mine for interesting insights
- Product and business growth Mindset Technical skills
In this article, I'll be sharing with you some of our case studies that reveal the potential of data analyst candidates we've hired in the last few months.
For a list of questions to ask, you can refer to this link: How to interview a data analyst candidate
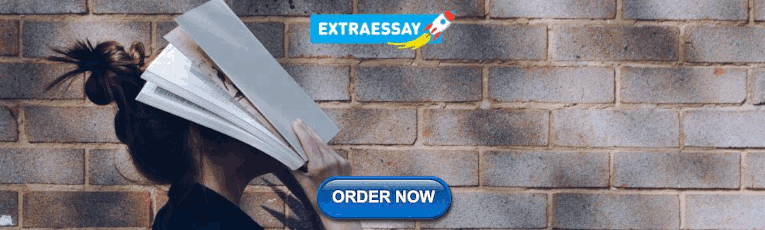
1. Analyze a Dataset
- Give us top 5–10 interesting insights you could find from this dataset
Give them a dataset, and let them use your tool or any tools they are familiar with to analyze it.
Expectations
- Communication: The first thing they should do is ask the interviewers to clarify the dataset and the problems to be solved, instead of just jumping into answering the question right away.
- Strong industry knowledge, or an indication of how quickly they can adapt to a new domain.
- The insights here should not only be about charts, but also the explanation behind what we should investigate more of, or make decisions on.
Let's take a look at some insights from our data analyst's work exploring an e-commerce dataset.
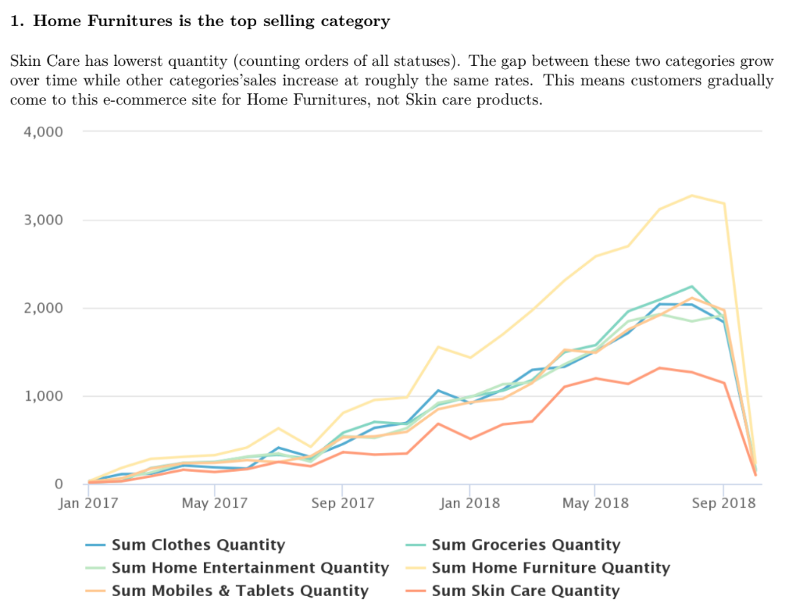
2. Product Mindset
In a product startup, the data analyst must also have the ability to understand the product as well as measure the success of the product.
- How would you improve our feature X (Search/Login/Dashboard…) using data?
- Show effort for independent research, and declaring some assumptions on what makes a feature good/bad.
- Ask/create a user flow for the feature, listing down all the possible steps that users should take to achieve that result. Let them assume they can get all the data they want, and ask what they would measure and how they will make decisions from there.
- Provide data and current insights to understand how often users actually use the feature and assess how they evaluate if it's still worth working on.
3. Business Sense
Data analysts need to be responsible for not only Product, but also Sales, Marketing, Financial analyses and more as well. Hence, they must be able to quickly adapt to any business model or distribution strategy.
- How would you increase our conversion rate?
- How would you know if a customer will upgrade or churn?
- The candidate should ask the interviewer to clarify the information, e.g. How the company defines conversion rate?
- Identify data sources and stages of the funnels, what are the data sources we have and what others we need, how to collect and consolidate the data?
- Ability to extract the data into meaningful insights that can inform business decisions, the insights would differ depending on the business model (B2B, B2C, etc.) e.g. able to list down all the factors that could affect users subscriptions (B2B).
- Able to compare and benchmark performance with industry insights e.g able to tell what is the average conversion rate of e-commerce companies.
4. Metric-driven
- Top 3 metrics to define the success of this product, what, why and how would you choose?
- To answer this question, the candidates need to have basic domain knowledge of the industry or product as well as the understanding of the product's core value propositions.
- A good candidate would also ask for information on company strategy and vision.
- Depending on each product and industry, the key metrics would be different, e.g. Facebook - Daily active users (DAU), Number of users adding 7 friends in the first 10 days; Holistics - Number of reports created and viewed, Number of users invited during the trial period; Uber - Weekly Rides, First ride/passenger …
According to my experience, there are a lot of data analysts who are just familiar with doing reporting from requirements, while talented analysts are eager to understand the data deeply and produce meaningful insights to help their team make better decisions, and they are definitely the players you want to have in your A+ team.
Finding a great data analyst is not easy, technical skill is essential, however, mindset is even more important. Therefore, list down all you need from a data analyst, trust your gut and hiring the right person will be a super advantage for your startup.
What's happening in the BI world?
Join 30k+ people to get insights from BI practitioners around the globe. In your inbox. Every week. Learn more
No spam, ever. We respect your email privacy. Unsubscribe anytime.
10 Real World Data Science Case Studies Projects with Example
Top 10 Data Science Case Studies Projects with Examples and Solutions in Python to inspire your data science learning in 2023.
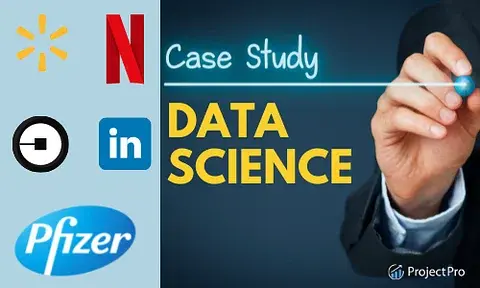
BelData science has been a trending buzzword in recent times. With wide applications in various sectors like healthcare , education, retail, transportation, media, and banking -data science applications are at the core of pretty much every industry out there. The possibilities are endless: analysis of frauds in the finance sector or the personalization of recommendations on eCommerce businesses. We have developed ten exciting data science case studies to explain how data science is leveraged across various industries to make smarter decisions and develop innovative personalized products tailored to specific customers.

Walmart Sales Forecasting Data Science Project
Downloadable solution code | Explanatory videos | Tech Support
Table of Contents
Data science case studies in retail , data science case study examples in entertainment industry , data analytics case study examples in travel industry , case studies for data analytics in social media , real world data science projects in healthcare, data analytics case studies in oil and gas, what is a case study in data science, how do you prepare a data science case study, 10 most interesting data science case studies with examples.
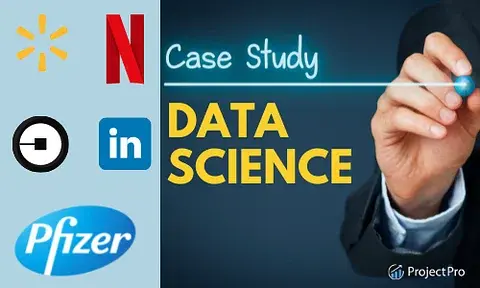
So, without much ado, let's get started with data science business case studies !
With humble beginnings as a simple discount retailer, today, Walmart operates in 10,500 stores and clubs in 24 countries and eCommerce websites, employing around 2.2 million people around the globe. For the fiscal year ended January 31, 2021, Walmart's total revenue was $559 billion showing a growth of $35 billion with the expansion of the eCommerce sector. Walmart is a data-driven company that works on the principle of 'Everyday low cost' for its consumers. To achieve this goal, they heavily depend on the advances of their data science and analytics department for research and development, also known as Walmart Labs. Walmart is home to the world's largest private cloud, which can manage 2.5 petabytes of data every hour! To analyze this humongous amount of data, Walmart has created 'Data Café,' a state-of-the-art analytics hub located within its Bentonville, Arkansas headquarters. The Walmart Labs team heavily invests in building and managing technologies like cloud, data, DevOps , infrastructure, and security.
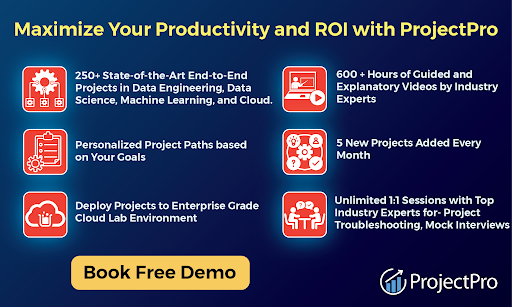
Walmart is experiencing massive digital growth as the world's largest retailer . Walmart has been leveraging Big data and advances in data science to build solutions to enhance, optimize and customize the shopping experience and serve their customers in a better way. At Walmart Labs, data scientists are focused on creating data-driven solutions that power the efficiency and effectiveness of complex supply chain management processes. Here are some of the applications of data science at Walmart:
i) Personalized Customer Shopping Experience
Walmart analyses customer preferences and shopping patterns to optimize the stocking and displaying of merchandise in their stores. Analysis of Big data also helps them understand new item sales, make decisions on discontinuing products, and the performance of brands.
ii) Order Sourcing and On-Time Delivery Promise
Millions of customers view items on Walmart.com, and Walmart provides each customer a real-time estimated delivery date for the items purchased. Walmart runs a backend algorithm that estimates this based on the distance between the customer and the fulfillment center, inventory levels, and shipping methods available. The supply chain management system determines the optimum fulfillment center based on distance and inventory levels for every order. It also has to decide on the shipping method to minimize transportation costs while meeting the promised delivery date.
Here's what valued users are saying about ProjectPro
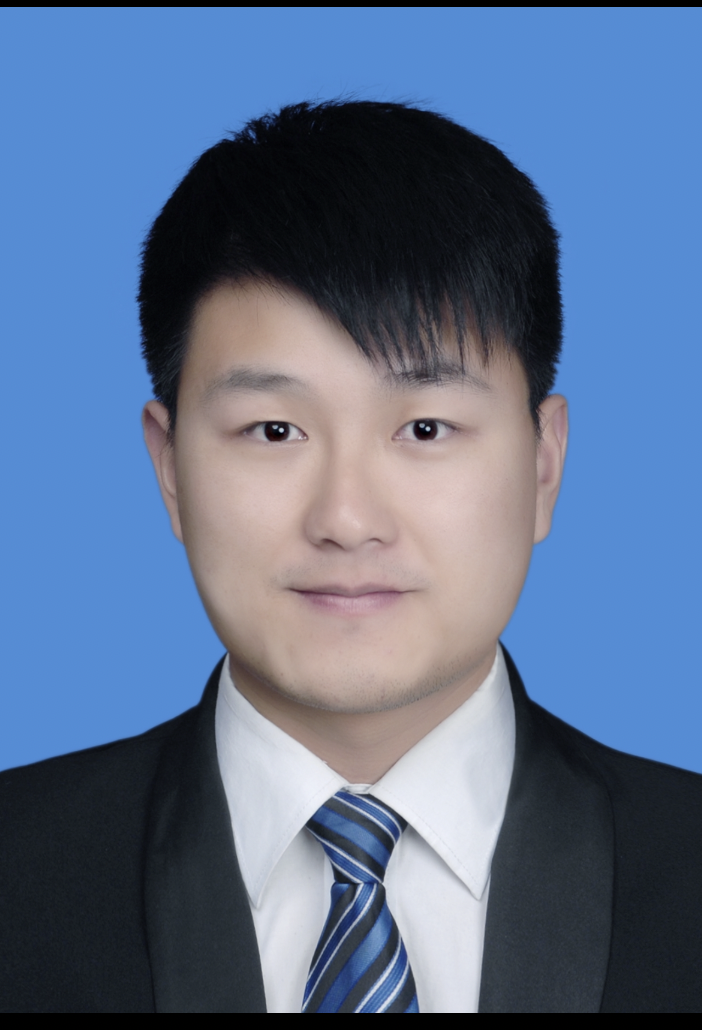
Graduate Research assistance at Stony Brook University
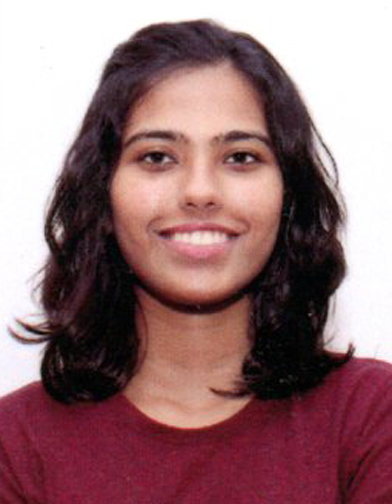
Savvy Sahai
Data Science Intern, Capgemini
Not sure what you are looking for?
iii) Packing Optimization
Also known as Box recommendation is a daily occurrence in the shipping of items in retail and eCommerce business. When items of an order or multiple orders for the same customer are ready for packing, Walmart has developed a recommender system that picks the best-sized box which holds all the ordered items with the least in-box space wastage within a fixed amount of time. This Bin Packing problem is a classic NP-Hard problem familiar to data scientists .
Whenever items of an order or multiple orders placed by the same customer are picked from the shelf and are ready for packing, the box recommendation system determines the best-sized box to hold all the ordered items with a minimum of in-box space wasted. This problem is known as the Bin Packing Problem, another classic NP-Hard problem familiar to data scientists.
Here is a link to a sales prediction data science case study to help you understand the applications of Data Science in the real world. Walmart Sales Forecasting Project uses historical sales data for 45 Walmart stores located in different regions. Each store contains many departments, and you must build a model to project the sales for each department in each store. This data science case study aims to create a predictive model to predict the sales of each product. You can also try your hands-on Inventory Demand Forecasting Data Science Project to develop a machine learning model to forecast inventory demand accurately based on historical sales data.
Get Closer To Your Dream of Becoming a Data Scientist with 70+ Solved End-to-End ML Projects
Amazon is an American multinational technology-based company based in Seattle, USA. It started as an online bookseller, but today it focuses on eCommerce, cloud computing , digital streaming, and artificial intelligence . It hosts an estimate of 1,000,000,000 gigabytes of data across more than 1,400,000 servers. Through its constant innovation in data science and big data Amazon is always ahead in understanding its customers. Here are a few data analytics case study examples at Amazon:
i) Recommendation Systems
Data science models help amazon understand the customers' needs and recommend them to them before the customer searches for a product; this model uses collaborative filtering. Amazon uses 152 million customer purchases data to help users to decide on products to be purchased. The company generates 35% of its annual sales using the Recommendation based systems (RBS) method.
Here is a Recommender System Project to help you build a recommendation system using collaborative filtering.
ii) Retail Price Optimization
Amazon product prices are optimized based on a predictive model that determines the best price so that the users do not refuse to buy it based on price. The model carefully determines the optimal prices considering the customers' likelihood of purchasing the product and thinks the price will affect the customers' future buying patterns. Price for a product is determined according to your activity on the website, competitors' pricing, product availability, item preferences, order history, expected profit margin, and other factors.
Check Out this Retail Price Optimization Project to build a Dynamic Pricing Model.
iii) Fraud Detection
Being a significant eCommerce business, Amazon remains at high risk of retail fraud. As a preemptive measure, the company collects historical and real-time data for every order. It uses Machine learning algorithms to find transactions with a higher probability of being fraudulent. This proactive measure has helped the company restrict clients with an excessive number of returns of products.
You can look at this Credit Card Fraud Detection Project to implement a fraud detection model to classify fraudulent credit card transactions.
New Projects
Let us explore data analytics case study examples in the entertainment indusry.
Ace Your Next Job Interview with Mock Interviews from Experts to Improve Your Skills and Boost Confidence!
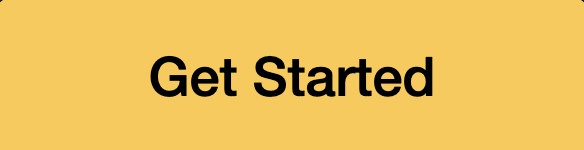
Netflix started as a DVD rental service in 1997 and then has expanded into the streaming business. Headquartered in Los Gatos, California, Netflix is the largest content streaming company in the world. Currently, Netflix has over 208 million paid subscribers worldwide, and with thousands of smart devices which are presently streaming supported, Netflix has around 3 billion hours watched every month. The secret to this massive growth and popularity of Netflix is its advanced use of data analytics and recommendation systems to provide personalized and relevant content recommendations to its users. The data is collected over 100 billion events every day. Here are a few examples of data analysis case studies applied at Netflix :
i) Personalized Recommendation System
Netflix uses over 1300 recommendation clusters based on consumer viewing preferences to provide a personalized experience. Some of the data that Netflix collects from its users include Viewing time, platform searches for keywords, Metadata related to content abandonment, such as content pause time, rewind, rewatched. Using this data, Netflix can predict what a viewer is likely to watch and give a personalized watchlist to a user. Some of the algorithms used by the Netflix recommendation system are Personalized video Ranking, Trending now ranker, and the Continue watching now ranker.
ii) Content Development using Data Analytics
Netflix uses data science to analyze the behavior and patterns of its user to recognize themes and categories that the masses prefer to watch. This data is used to produce shows like The umbrella academy, and Orange Is the New Black, and the Queen's Gambit. These shows seem like a huge risk but are significantly based on data analytics using parameters, which assured Netflix that they would succeed with its audience. Data analytics is helping Netflix come up with content that their viewers want to watch even before they know they want to watch it.
iii) Marketing Analytics for Campaigns
Netflix uses data analytics to find the right time to launch shows and ad campaigns to have maximum impact on the target audience. Marketing analytics helps come up with different trailers and thumbnails for other groups of viewers. For example, the House of Cards Season 5 trailer with a giant American flag was launched during the American presidential elections, as it would resonate well with the audience.
Here is a Customer Segmentation Project using association rule mining to understand the primary grouping of customers based on various parameters.
Get FREE Access to Machine Learning Example Codes for Data Cleaning , Data Munging, and Data Visualization
In a world where Purchasing music is a thing of the past and streaming music is a current trend, Spotify has emerged as one of the most popular streaming platforms. With 320 million monthly users, around 4 billion playlists, and approximately 2 million podcasts, Spotify leads the pack among well-known streaming platforms like Apple Music, Wynk, Songza, amazon music, etc. The success of Spotify has mainly depended on data analytics. By analyzing massive volumes of listener data, Spotify provides real-time and personalized services to its listeners. Most of Spotify's revenue comes from paid premium subscriptions. Here are some of the examples of case study on data analytics used by Spotify to provide enhanced services to its listeners:
i) Personalization of Content using Recommendation Systems
Spotify uses Bart or Bayesian Additive Regression Trees to generate music recommendations to its listeners in real-time. Bart ignores any song a user listens to for less than 30 seconds. The model is retrained every day to provide updated recommendations. A new Patent granted to Spotify for an AI application is used to identify a user's musical tastes based on audio signals, gender, age, accent to make better music recommendations.
Spotify creates daily playlists for its listeners, based on the taste profiles called 'Daily Mixes,' which have songs the user has added to their playlists or created by the artists that the user has included in their playlists. It also includes new artists and songs that the user might be unfamiliar with but might improve the playlist. Similar to it is the weekly 'Release Radar' playlists that have newly released artists' songs that the listener follows or has liked before.
ii) Targetted marketing through Customer Segmentation
With user data for enhancing personalized song recommendations, Spotify uses this massive dataset for targeted ad campaigns and personalized service recommendations for its users. Spotify uses ML models to analyze the listener's behavior and group them based on music preferences, age, gender, ethnicity, etc. These insights help them create ad campaigns for a specific target audience. One of their well-known ad campaigns was the meme-inspired ads for potential target customers, which was a huge success globally.
iii) CNN's for Classification of Songs and Audio Tracks
Spotify builds audio models to evaluate the songs and tracks, which helps develop better playlists and recommendations for its users. These allow Spotify to filter new tracks based on their lyrics and rhythms and recommend them to users like similar tracks ( collaborative filtering). Spotify also uses NLP ( Natural language processing) to scan articles and blogs to analyze the words used to describe songs and artists. These analytical insights can help group and identify similar artists and songs and leverage them to build playlists.
Here is a Music Recommender System Project for you to start learning. We have listed another music recommendations dataset for you to use for your projects: Dataset1 . You can use this dataset of Spotify metadata to classify songs based on artists, mood, liveliness. Plot histograms, heatmaps to get a better understanding of the dataset. Use classification algorithms like logistic regression, SVM, and Principal component analysis to generate valuable insights from the dataset.
Explore Categories
Below you will find case studies for data analytics in the travel and tourism industry.
Airbnb was born in 2007 in San Francisco and has since grown to 4 million Hosts and 5.6 million listings worldwide who have welcomed more than 1 billion guest arrivals in almost every country across the globe. Airbnb is active in every country on the planet except for Iran, Sudan, Syria, and North Korea. That is around 97.95% of the world. Using data as a voice of their customers, Airbnb uses the large volume of customer reviews, host inputs to understand trends across communities, rate user experiences, and uses these analytics to make informed decisions to build a better business model. The data scientists at Airbnb are developing exciting new solutions to boost the business and find the best mapping for its customers and hosts. Airbnb data servers serve approximately 10 million requests a day and process around one million search queries. Data is the voice of customers at AirBnB and offers personalized services by creating a perfect match between the guests and hosts for a supreme customer experience.
i) Recommendation Systems and Search Ranking Algorithms
Airbnb helps people find 'local experiences' in a place with the help of search algorithms that make searches and listings precise. Airbnb uses a 'listing quality score' to find homes based on the proximity to the searched location and uses previous guest reviews. Airbnb uses deep neural networks to build models that take the guest's earlier stays into account and area information to find a perfect match. The search algorithms are optimized based on guest and host preferences, rankings, pricing, and availability to understand users’ needs and provide the best match possible.
ii) Natural Language Processing for Review Analysis
Airbnb characterizes data as the voice of its customers. The customer and host reviews give a direct insight into the experience. The star ratings alone cannot be an excellent way to understand it quantitatively. Hence Airbnb uses natural language processing to understand reviews and the sentiments behind them. The NLP models are developed using Convolutional neural networks .
Practice this Sentiment Analysis Project for analyzing product reviews to understand the basic concepts of natural language processing.
iii) Smart Pricing using Predictive Analytics
The Airbnb hosts community uses the service as a supplementary income. The vacation homes and guest houses rented to customers provide for rising local community earnings as Airbnb guests stay 2.4 times longer and spend approximately 2.3 times the money compared to a hotel guest. The profits are a significant positive impact on the local neighborhood community. Airbnb uses predictive analytics to predict the prices of the listings and help the hosts set a competitive and optimal price. The overall profitability of the Airbnb host depends on factors like the time invested by the host and responsiveness to changing demands for different seasons. The factors that impact the real-time smart pricing are the location of the listing, proximity to transport options, season, and amenities available in the neighborhood of the listing.
Here is a Price Prediction Project to help you understand the concept of predictive analysis which is widely common in case studies for data analytics.
Uber is the biggest global taxi service provider. As of December 2018, Uber has 91 million monthly active consumers and 3.8 million drivers. Uber completes 14 million trips each day. Uber uses data analytics and big data-driven technologies to optimize their business processes and provide enhanced customer service. The Data Science team at uber has been exploring futuristic technologies to provide better service constantly. Machine learning and data analytics help Uber make data-driven decisions that enable benefits like ride-sharing, dynamic price surges, better customer support, and demand forecasting. Here are some of the real world data science projects used by uber:
i) Dynamic Pricing for Price Surges and Demand Forecasting
Uber prices change at peak hours based on demand. Uber uses surge pricing to encourage more cab drivers to sign up with the company, to meet the demand from the passengers. When the prices increase, the driver and the passenger are both informed about the surge in price. Uber uses a predictive model for price surging called the 'Geosurge' ( patented). It is based on the demand for the ride and the location.
ii) One-Click Chat
Uber has developed a Machine learning and natural language processing solution called one-click chat or OCC for coordination between drivers and users. This feature anticipates responses for commonly asked questions, making it easy for the drivers to respond to customer messages. Drivers can reply with the clock of just one button. One-Click chat is developed on Uber's machine learning platform Michelangelo to perform NLP on rider chat messages and generate appropriate responses to them.
iii) Customer Retention
Failure to meet the customer demand for cabs could lead to users opting for other services. Uber uses machine learning models to bridge this demand-supply gap. By using prediction models to predict the demand in any location, uber retains its customers. Uber also uses a tier-based reward system, which segments customers into different levels based on usage. The higher level the user achieves, the better are the perks. Uber also provides personalized destination suggestions based on the history of the user and their frequently traveled destinations.
You can take a look at this Python Chatbot Project and build a simple chatbot application to understand better the techniques used for natural language processing. You can also practice the working of a demand forecasting model with this project using time series analysis. You can look at this project which uses time series forecasting and clustering on a dataset containing geospatial data for forecasting customer demand for ola rides.
Explore More Data Science and Machine Learning Projects for Practice. Fast-Track Your Career Transition with ProjectPro
7) LinkedIn
LinkedIn is the largest professional social networking site with nearly 800 million members in more than 200 countries worldwide. Almost 40% of the users access LinkedIn daily, clocking around 1 billion interactions per month. The data science team at LinkedIn works with this massive pool of data to generate insights to build strategies, apply algorithms and statistical inferences to optimize engineering solutions, and help the company achieve its goals. Here are some of the real world data science projects at LinkedIn:
i) LinkedIn Recruiter Implement Search Algorithms and Recommendation Systems
LinkedIn Recruiter helps recruiters build and manage a talent pool to optimize the chances of hiring candidates successfully. This sophisticated product works on search and recommendation engines. The LinkedIn recruiter handles complex queries and filters on a constantly growing large dataset. The results delivered have to be relevant and specific. The initial search model was based on linear regression but was eventually upgraded to Gradient Boosted decision trees to include non-linear correlations in the dataset. In addition to these models, the LinkedIn recruiter also uses the Generalized Linear Mix model to improve the results of prediction problems to give personalized results.
ii) Recommendation Systems Personalized for News Feed
The LinkedIn news feed is the heart and soul of the professional community. A member's newsfeed is a place to discover conversations among connections, career news, posts, suggestions, photos, and videos. Every time a member visits LinkedIn, machine learning algorithms identify the best exchanges to be displayed on the feed by sorting through posts and ranking the most relevant results on top. The algorithms help LinkedIn understand member preferences and help provide personalized news feeds. The algorithms used include logistic regression, gradient boosted decision trees and neural networks for recommendation systems.
iii) CNN's to Detect Inappropriate Content
To provide a professional space where people can trust and express themselves professionally in a safe community has been a critical goal at LinkedIn. LinkedIn has heavily invested in building solutions to detect fake accounts and abusive behavior on their platform. Any form of spam, harassment, inappropriate content is immediately flagged and taken down. These can range from profanity to advertisements for illegal services. LinkedIn uses a Convolutional neural networks based machine learning model. This classifier trains on a training dataset containing accounts labeled as either "inappropriate" or "appropriate." The inappropriate list consists of accounts having content from "blocklisted" phrases or words and a small portion of manually reviewed accounts reported by the user community.
Here is a Text Classification Project to help you understand NLP basics for text classification. You can find a news recommendation system dataset to help you build a personalized news recommender system. You can also use this dataset to build a classifier using logistic regression, Naive Bayes, or Neural networks to classify toxic comments.
Get confident to build end-to-end projects
Access to a curated library of 250+ end-to-end industry projects with solution code, videos and tech support.
Pfizer is a multinational pharmaceutical company headquartered in New York, USA. One of the largest pharmaceutical companies globally known for developing a wide range of medicines and vaccines in disciplines like immunology, oncology, cardiology, and neurology. Pfizer became a household name in 2010 when it was the first to have a COVID-19 vaccine with FDA. In early November 2021, The CDC has approved the Pfizer vaccine for kids aged 5 to 11. Pfizer has been using machine learning and artificial intelligence to develop drugs and streamline trials, which played a massive role in developing and deploying the COVID-19 vaccine. Here are a few data analytics case studies by Pfizer :
i) Identifying Patients for Clinical Trials
Artificial intelligence and machine learning are used to streamline and optimize clinical trials to increase their efficiency. Natural language processing and exploratory data analysis of patient records can help identify suitable patients for clinical trials. These can help identify patients with distinct symptoms. These can help examine interactions of potential trial members' specific biomarkers, predict drug interactions and side effects which can help avoid complications. Pfizer's AI implementation helped rapidly identify signals within the noise of millions of data points across their 44,000-candidate COVID-19 clinical trial.
ii) Supply Chain and Manufacturing
Data science and machine learning techniques help pharmaceutical companies better forecast demand for vaccines and drugs and distribute them efficiently. Machine learning models can help identify efficient supply systems by automating and optimizing the production steps. These will help supply drugs customized to small pools of patients in specific gene pools. Pfizer uses Machine learning to predict the maintenance cost of equipment used. Predictive maintenance using AI is the next big step for Pharmaceutical companies to reduce costs.
iii) Drug Development
Computer simulations of proteins, and tests of their interactions, and yield analysis help researchers develop and test drugs more efficiently. In 2016 Watson Health and Pfizer announced a collaboration to utilize IBM Watson for Drug Discovery to help accelerate Pfizer's research in immuno-oncology, an approach to cancer treatment that uses the body's immune system to help fight cancer. Deep learning models have been used recently for bioactivity and synthesis prediction for drugs and vaccines in addition to molecular design. Deep learning has been a revolutionary technique for drug discovery as it factors everything from new applications of medications to possible toxic reactions which can save millions in drug trials.
You can create a Machine learning model to predict molecular activity to help design medicine using this dataset . You may build a CNN or a Deep neural network for this data analyst case study project.
Access Data Science and Machine Learning Project Code Examples
9) Shell Data Analyst Case Study Project
Shell is a global group of energy and petrochemical companies with over 80,000 employees in around 70 countries. Shell uses advanced technologies and innovations to help build a sustainable energy future. Shell is going through a significant transition as the world needs more and cleaner energy solutions to be a clean energy company by 2050. It requires substantial changes in the way in which energy is used. Digital technologies, including AI and Machine Learning, play an essential role in this transformation. These include efficient exploration and energy production, more reliable manufacturing, more nimble trading, and a personalized customer experience. Using AI in various phases of the organization will help achieve this goal and stay competitive in the market. Here are a few data analytics case studies in the petrochemical industry:
i) Precision Drilling
Shell is involved in the processing mining oil and gas supply, ranging from mining hydrocarbons to refining the fuel to retailing them to customers. Recently Shell has included reinforcement learning to control the drilling equipment used in mining. Reinforcement learning works on a reward-based system based on the outcome of the AI model. The algorithm is designed to guide the drills as they move through the surface, based on the historical data from drilling records. It includes information such as the size of drill bits, temperatures, pressures, and knowledge of the seismic activity. This model helps the human operator understand the environment better, leading to better and faster results will minor damage to machinery used.
ii) Efficient Charging Terminals
Due to climate changes, governments have encouraged people to switch to electric vehicles to reduce carbon dioxide emissions. However, the lack of public charging terminals has deterred people from switching to electric cars. Shell uses AI to monitor and predict the demand for terminals to provide efficient supply. Multiple vehicles charging from a single terminal may create a considerable grid load, and predictions on demand can help make this process more efficient.
iii) Monitoring Service and Charging Stations
Another Shell initiative trialed in Thailand and Singapore is the use of computer vision cameras, which can think and understand to watch out for potentially hazardous activities like lighting cigarettes in the vicinity of the pumps while refueling. The model is built to process the content of the captured images and label and classify it. The algorithm can then alert the staff and hence reduce the risk of fires. You can further train the model to detect rash driving or thefts in the future.
Here is a project to help you understand multiclass image classification. You can use the Hourly Energy Consumption Dataset to build an energy consumption prediction model. You can use time series with XGBoost to develop your model.
10) Zomato Case Study on Data Analytics
Zomato was founded in 2010 and is currently one of the most well-known food tech companies. Zomato offers services like restaurant discovery, home delivery, online table reservation, online payments for dining, etc. Zomato partners with restaurants to provide tools to acquire more customers while also providing delivery services and easy procurement of ingredients and kitchen supplies. Currently, Zomato has over 2 lakh restaurant partners and around 1 lakh delivery partners. Zomato has closed over ten crore delivery orders as of date. Zomato uses ML and AI to boost their business growth, with the massive amount of data collected over the years from food orders and user consumption patterns. Here are a few examples of data analyst case study project developed by the data scientists at Zomato:
i) Personalized Recommendation System for Homepage
Zomato uses data analytics to create personalized homepages for its users. Zomato uses data science to provide order personalization, like giving recommendations to the customers for specific cuisines, locations, prices, brands, etc. Restaurant recommendations are made based on a customer's past purchases, browsing history, and what other similar customers in the vicinity are ordering. This personalized recommendation system has led to a 15% improvement in order conversions and click-through rates for Zomato.
You can use the Restaurant Recommendation Dataset to build a restaurant recommendation system to predict what restaurants customers are most likely to order from, given the customer location, restaurant information, and customer order history.
ii) Analyzing Customer Sentiment
Zomato uses Natural language processing and Machine learning to understand customer sentiments using social media posts and customer reviews. These help the company gauge the inclination of its customer base towards the brand. Deep learning models analyze the sentiments of various brand mentions on social networking sites like Twitter, Instagram, Linked In, and Facebook. These analytics give insights to the company, which helps build the brand and understand the target audience.
iii) Predicting Food Preparation Time (FPT)
Food delivery time is an essential variable in the estimated delivery time of the order placed by the customer using Zomato. The food preparation time depends on numerous factors like the number of dishes ordered, time of the day, footfall in the restaurant, day of the week, etc. Accurate prediction of the food preparation time can help make a better prediction of the Estimated delivery time, which will help delivery partners less likely to breach it. Zomato uses a Bidirectional LSTM-based deep learning model that considers all these features and provides food preparation time for each order in real-time.
Data scientists are companies' secret weapons when analyzing customer sentiments and behavior and leveraging it to drive conversion, loyalty, and profits. These 10 data science case studies projects with examples and solutions show you how various organizations use data science technologies to succeed and be at the top of their field! To summarize, Data Science has not only accelerated the performance of companies but has also made it possible to manage & sustain their performance with ease.
FAQs on Data Analysis Case Studies
A case study in data science is an in-depth analysis of a real-world problem using data-driven approaches. It involves collecting, cleaning, and analyzing data to extract insights and solve challenges, offering practical insights into how data science techniques can address complex issues across various industries.
To create a data science case study, identify a relevant problem, define objectives, and gather suitable data. Clean and preprocess data, perform exploratory data analysis, and apply appropriate algorithms for analysis. Summarize findings, visualize results, and provide actionable recommendations, showcasing the problem-solving potential of data science techniques.
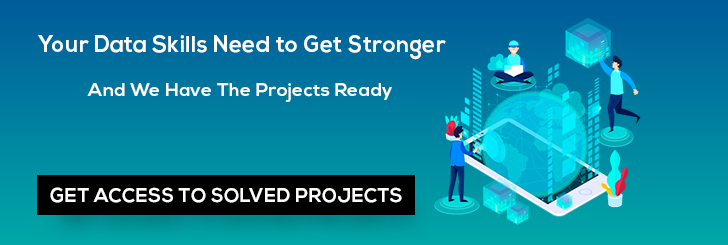
About the Author

ProjectPro is the only online platform designed to help professionals gain practical, hands-on experience in big data, data engineering, data science, and machine learning related technologies. Having over 270+ reusable project templates in data science and big data with step-by-step walkthroughs,

© 2024
© 2024 Iconiq Inc.
Privacy policy
User policy
Write for ProjectPro
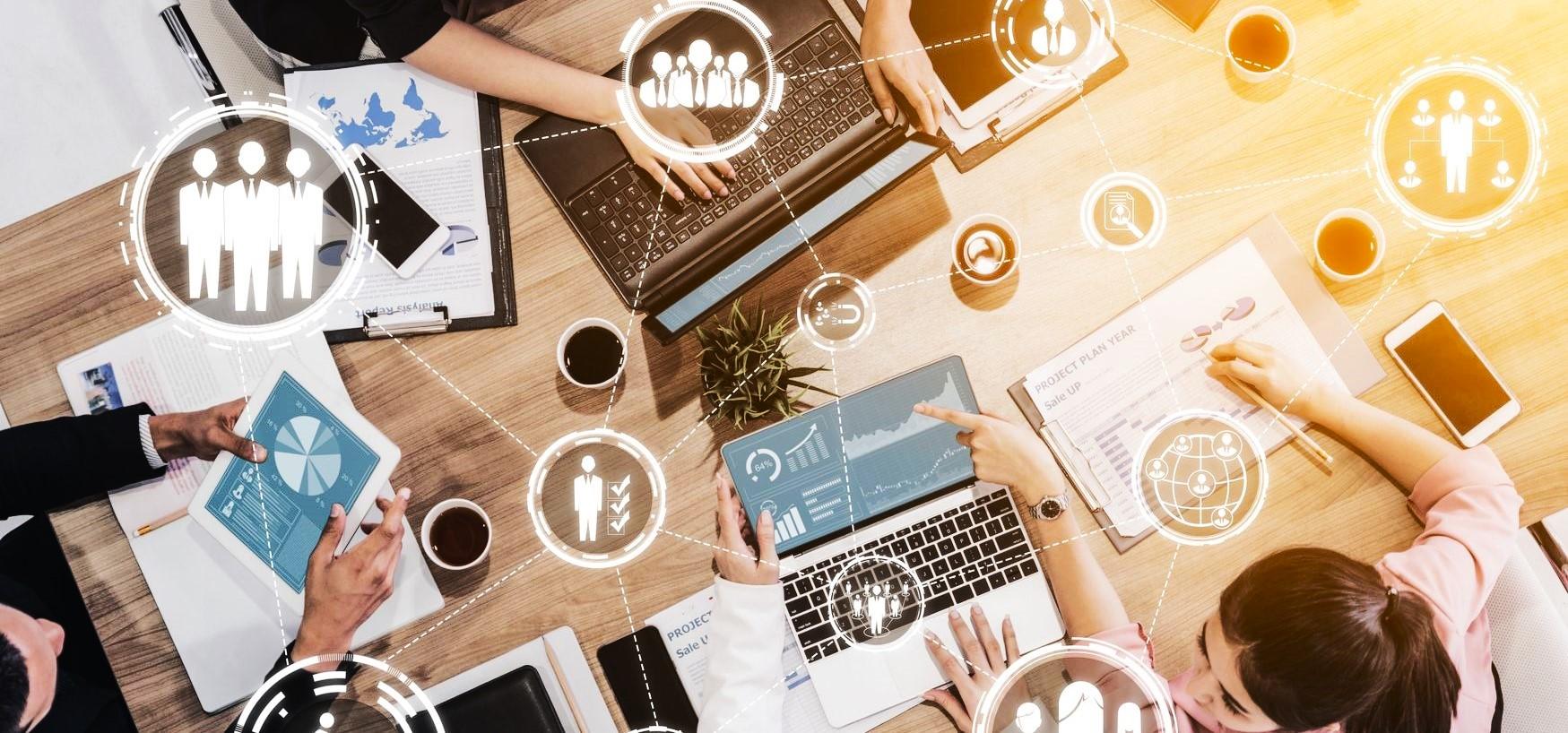
2024 Guide: 20+ Essential Data Science Case Study Interview Questions
Case studies are often the most challenging aspect of data science interview processes. They are crafted to resemble a company’s existing or previous projects, assessing a candidate’s ability to tackle prompts, convey their insights, and navigate obstacles.
To excel in data science case study interviews, practice is crucial. It will enable you to develop strategies for approaching case studies, asking the right questions to your interviewer, and providing responses that showcase your skills while adhering to time constraints.
The best way of doing this is by using a framework for answering case studies. For example, you could use the product metrics framework and the A/B testing framework to answer most case studies that come up in data science interviews.
There are four main types of data science case studies:
- Product Case Studies - This type of case study tackles a specific product or feature offering, often tied to the interviewing company. Interviewers are generally looking for a sense of business sense geared towards product metrics.
- Data Analytics Case Study Questions - Data analytics case studies ask you to propose possible metrics in order to investigate an analytics problem. Additionally, you must write a SQL query to pull your proposed metrics, and then perform analysis using the data you queried, just as you would do in the role.
- Modeling and Machine Learning Case Studies - Modeling case studies are more varied and focus on assessing your intuition for building models around business problems.
- Business Case Questions - Similar to product questions, business cases tackle issues or opportunities specific to the organization that is interviewing you. Often, candidates must assess the best option for a certain business plan being proposed, and formulate a process for solving the specific problem.
How Case Study Interviews Are Conducted
Oftentimes as an interviewee, you want to know the setting and format in which to expect the above questions to be asked. Unfortunately, this is company-specific: Some prefer real-time settings, where candidates actively work through a prompt after receiving it, while others offer some period of days (say, a week) before settling in for a presentation of your findings.
It is therefore important to have a system for answering these questions that will accommodate all possible formats, such that you are prepared for any set of circumstances (we provide such a framework below).
Why Are Case Study Questions Asked?
Case studies assess your thought process in answering data science questions. Specifically, interviewers want to see that you have the ability to think on your feet, and to work through real-world problems that likely do not have a right or wrong answer. Real-world case studies that are affecting businesses are not binary; there is no black-and-white, yes-or-no answer. This is why it is important that you can demonstrate decisiveness in your investigations, as well as show your capacity to consider impacts and topics from a variety of angles. Once you are in the role, you will be dealing directly with the ambiguity at the heart of decision-making.
Perhaps most importantly, case interviews assess your ability to effectively communicate your conclusions. On the job, data scientists exchange information across teams and divisions, so a significant part of the interviewer’s focus will be on how you process and explain your answer.
Quick tip: Because case questions in data science interviews tend to be product- and company-focused, it is extremely beneficial to research current projects and developments across different divisions , as these initiatives might end up as the case study topic.
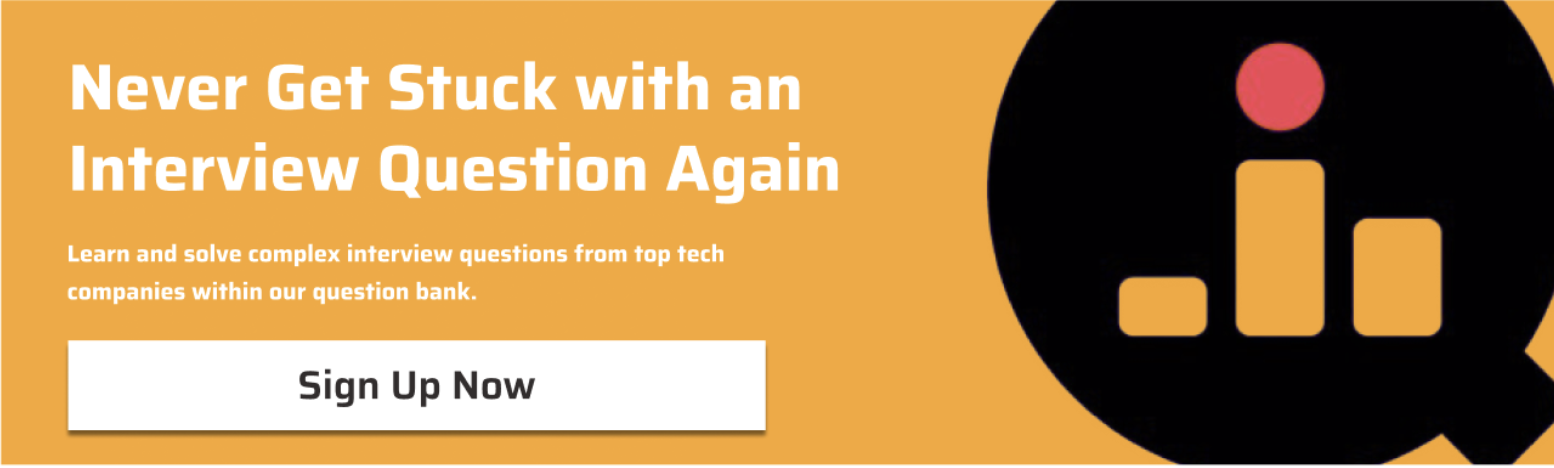
How to Answer Data Science Case Study Questions (The Framework)
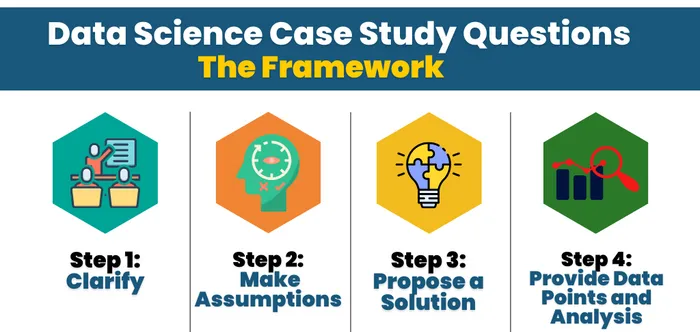
There are four main steps to tackling case questions in Data Science interviews, regardless of the type: clarify, make assumptions, gather context, and provide data points and analysis.
Step 1: Clarify
Clarifying is used to gather more information . More often than not, these case studies are designed to be confusing and vague. There will be unorganized data intentionally supplemented with extraneous or omitted information, so it is the candidate’s responsibility to dig deeper, filter out bad information, and fill gaps. Interviewers will be observing how an applicant asks questions and reach their solution.
For example, with a product question, you might take into consideration:
- What is the product?
- How does the product work?
- How does the product align with the business itself?
Step 2: Make Assumptions
When you have made sure that you have evaluated and understand the dataset, start investigating and discarding possible hypotheses. Developing insights on the product at this stage complements your ability to glean information from the dataset, and the exploration of your ideas is paramount to forming a successful hypothesis. You should be communicating your hypotheses with the interviewer, such that they can provide clarifying remarks on how the business views the product, and to help you discard unworkable lines of inquiry. If we continue to think about a product question, some important questions to evaluate and draw conclusions from include:
- Who uses the product? Why?
- What are the goals of the product?
- How does the product interact with other services or goods the company offers?
The goal of this is to reduce the scope of the problem at hand, and ask the interviewer questions upfront that allow you to tackle the meat of the problem instead of focusing on less consequential edge cases.
Step 3: Propose a Solution
Now that a hypothesis is formed that has incorporated the dataset and an understanding of the business-related context, it is time to apply that knowledge in forming a solution. Remember, the hypothesis is simply a refined version of the problem that uses the data on hand as its basis to being solved. The solution you create can target this narrow problem, and you can have full faith that it is addressing the core of the case study question.
Keep in mind that there isn’t a single expected solution, and as such, there is a certain freedom here to determine the exact path for investigation.
Step 4: Provide Data Points and Analysis
Finally, providing data points and analysis in support of your solution involves choosing and prioritizing a main metric. As with all prior factors, this step must be tied back to the hypothesis and the main goal of the problem. From that foundation, it is important to trace through and analyze different examples– from the main metric–in order to validate the hypothesis.
Quick tip: Every case question tends to have multiple solutions. Therefore, you should absolutely consider and communicate any potential trade-offs of your chosen method. Be sure you are communicating the pros and cons of your approach.
Note: In some special cases, solutions will also be assessed on the ability to convey information in layman’s terms. Regardless of the structure, applicants should always be prepared to solve through the framework outlined above in order to answer the prompt.
The Role of Effective Communication
There have been multiple articles and discussions conducted by interviewers behind the Data Science Case Study portion, and they all boil down success in case studies to one main factor: effective communication.
All the analysis in the world will not help if interviewees cannot verbally work through and highlight their thought process within the case study. Again, interviewers are keyed at this stage of the hiring process to look for well-developed “soft-skills” and problem-solving capabilities. Demonstrating those traits is key to succeeding in this round.
To this end, the best advice possible would be to practice actively going through example case studies, such as those available in the Interview Query questions bank . Exploring different topics with a friend in an interview-like setting with cold recall (no Googling in between!) will be uncomfortable and awkward, but it will also help reveal weaknesses in fleshing out the investigation.
Don’t worry if the first few times are terrible! Developing a rhythm will help with gaining self-confidence as you become better at assessing and learning through these sessions.
Finding the right data science talent for case studies? OutSearch.ai ’s AI-driven platform streamlines this by pinpointing candidates who excel in real-world scenarios. Discover how they can help you match with top problem-solvers.
Product Case Study Questions
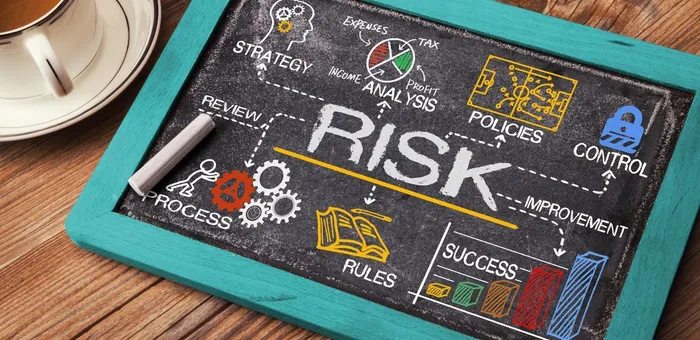
With product data science case questions , the interviewer wants to get an idea of your product sense intuition. Specifically, these questions assess your ability to identify which metrics should be proposed in order to understand a product.
1. How would you measure the success of private stories on Instagram, where only certain close friends can see the story?
Start by answering: What is the goal of the private story feature on Instagram? You can’t evaluate “success” without knowing what the initial objective of the product was, to begin with.
One specific goal of this feature would be to drive engagement. A private story could potentially increase interactions between users, and grow awareness of the feature.
Now, what types of metrics might you propose to assess user engagement? For a high-level overview, we could look at:
- Average stories per user per day
- Average Close Friends stories per user per day
However, we would also want to further bucket our users to see the effect that Close Friends stories have on user engagement. By bucketing users by age, date joined, or another metric, we could see how engagement is affected within certain populations, giving us insight on success that could be lost if looking at the overall population.
2. How would you measure the success of acquiring new users through a 30-day free trial at Netflix?
More context: Netflix is offering a promotion where users can enroll in a 30-day free trial. After 30 days, customers will automatically be charged based on their selected package. How would you measure acquisition success, and what metrics would you propose to measure the success of the free trial?
One way we can frame the concept specifically to this problem is to think about controllable inputs, external drivers, and then the observable output . Start with the major goals of Netflix:
- Acquiring new users to their subscription plan.
- Decreasing churn and increasing retention.
Looking at acquisition output metrics specifically, there are several top-level stats that we can look at, including:
- Conversion rate percentage
- Cost per free trial acquisition
- Daily conversion rate
With these conversion metrics, we would also want to bucket users by cohort. This would help us see the percentage of free users who were acquired, as well as retention by cohort.
3. How would you measure the success of Facebook Groups?
Start by considering the key function of Facebook Groups . You could say that Groups are a way for users to connect with other users through a shared interest or real-life relationship. Therefore, the user’s goal is to experience a sense of community, which will also drive our business goal of increasing user engagement.
What general engagement metrics can we associate with this value? An objective metric like Groups monthly active users would help us see if Facebook Groups user base is increasing or decreasing. Plus, we could monitor metrics like posting, commenting, and sharing rates.
There are other products that Groups impact, however, specifically the Newsfeed. We need to consider Newsfeed quality and examine if updates from Groups clog up the content pipeline and if users prioritize those updates over other Newsfeed items. This evaluation will give us a better sense of if Groups actually contribute to higher engagement levels.
4. How would you analyze the effectiveness of a new LinkedIn chat feature that shows a “green dot” for active users?
Note: Given engineering constraints, the new feature is impossible to A/B test before release. When you approach case study questions, remember always to clarify any vague terms. In this case, “effectiveness” is very vague. To help you define that term, you would want first to consider what the goal is of adding a green dot to LinkedIn chat.
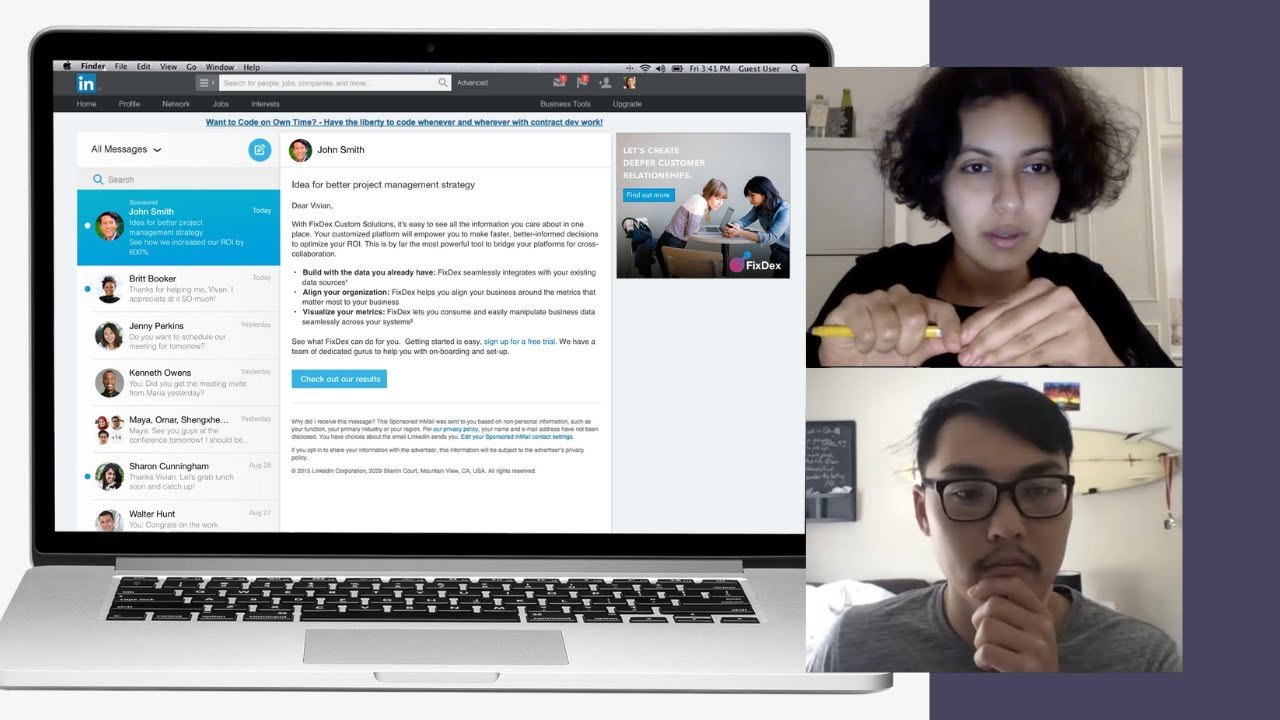
5. How would you diagnose why weekly active users are up 5%, but email notification open rates are down 2%?
What assumptions can you make about the relationship between weekly active users and email open rates? With a case question like this, you would want to first answer that line of inquiry before proceeding.
Hint: Open rate can decrease when its numerator decreases (fewer people open emails) or its denominator increases (more emails are sent overall). Taking these two factors into account, what are some hypotheses we can make about our decrease in the open rate compared to our increase in weekly active users?
Data Analytics Case Study Questions
Data analytics case studies ask you to dive into analytics problems. Typically these questions ask you to examine metrics trade-offs or investigate changes in metrics. In addition to proposing metrics, you also have to write SQL queries to generate the metrics, which is why they are sometimes referred to as SQL case study questions .
6. Using the provided data, generate some specific recommendations on how DoorDash can improve.
In this DoorDash analytics case study take-home question you are provided with the following dataset:
- Customer order time
- Restaurant order time
- Driver arrives at restaurant time
- Order delivered time
- Customer ID
- Amount of discount
- Amount of tip
With a dataset like this, there are numerous recommendations you can make. A good place to start is by thinking about the DoorDash marketplace, which includes drivers, riders and merchants. How could you analyze the data to increase revenue, driver/user retention and engagement in that marketplace?
7. After implementing a notification change, the total number of unsubscribes increases. Write a SQL query to show how unsubscribes are affecting login rates over time.
This is a Twitter data science interview question , and let’s say you implemented this new feature using an A/B test. You are provided with two tables: events (which includes login, nologin and unsubscribe ) and variants (which includes control or variant ).
We are tasked with comparing multiple different variables at play here. There is the new notification system, along with its effect of creating more unsubscribes. We can also see how login rates compare for unsubscribes for each bucket of the A/B test.
Given that we want to measure two different changes, we know we have to use GROUP BY for the two variables: date and bucket variant. What comes next?
8. Write a query to disprove the hypothesis: Data scientists who switch jobs more often end up getting promoted faster.
More context: You are provided with a table of user experiences representing each person’s past work experiences and timelines.
This question requires a bit of creative problem-solving to understand how we can prove or disprove the hypothesis. The hypothesis is that a data scientist that ends up switching jobs more often gets promoted faster.
Therefore, in analyzing this dataset, we can prove this hypothesis by separating the data scientists into specific segments on how often they jump in their careers.
For example, if we looked at the number of job switches for data scientists that have been in their field for five years, we could prove the hypothesis that the number of data science managers increased as the number of career jumps also rose.
- Never switched jobs: 10% are managers
- Switched jobs once: 20% are managers
- Switched jobs twice: 30% are managers
- Switched jobs three times: 40% are managers
9. Write a SQL query to investigate the hypothesis: Click-through rate is dependent on search result rating.
More context: You are given a table with search results on Facebook, which includes query (search term), position (the search position), and rating (human rating from 1 to 5). Each row represents a single search and includes a column has_clicked that represents whether a user clicked or not.
This question requires us to formulaically do two things: create a metric that can analyze a problem that we face and then actually compute that metric.
Think about the data we want to display to prove or disprove the hypothesis. Our output metric is CTR (clickthrough rate). If CTR is high when search result ratings are high and CTR is low when the search result ratings are low, then our hypothesis is proven. However, if the opposite is true, CTR is low when the search result ratings are high, or there is no proven correlation between the two, then our hypothesis is not proven.
With that structure in mind, we can then look at the results split into different search rating buckets. If we measure the CTR for queries that all have results rated at 1 and then measure CTR for queries that have results rated at lower than 2, etc., we can measure to see if the increase in rating is correlated with an increase in CTR.
10. How would you help a supermarket chain determine which product categories should be prioritized in their inventory restructuring efforts?
You’re working as a Data Scientist in a local grocery chain’s data science team. The business team has decided to allocate store floor space by product category (e.g., electronics, sports and travel, food and beverages). Help the team understand which product categories to prioritize as well as answering questions such as how customer demographics affect sales, and how each city’s sales per product category differs.
Check out our Data Analytics Learning Path .
Modeling and Machine Learning Case Questions
Machine learning case questions assess your ability to build models to solve business problems. These questions can range from applying machine learning to solve a specific case scenario to assessing the validity of a hypothetical existing model . The modeling case study requires a candidate to evaluate and explain any certain part of the model building process.
11. Describe how you would build a model to predict Uber ETAs after a rider requests a ride.
Common machine learning case study problems like this are designed to explain how you would build a model. Many times this can be scoped down to specific parts of the model building process. Examining the example above, we could break it up into:
How would you evaluate the predictions of an Uber ETA model?
What features would you use to predict the Uber ETA for ride requests?
Our recommended framework breaks down a modeling and machine learning case study to individual steps in order to tackle each one thoroughly. In each full modeling case study, you will want to go over:
- Data processing
- Feature Selection
- Model Selection
- Cross Validation
- Evaluation Metrics
- Testing and Roll Out
12. How would you build a model that sends bank customers a text message when fraudulent transactions are detected?
Additionally, the customer can approve or deny the transaction via text response.
Let’s start out by understanding what kind of model would need to be built. We know that since we are working with fraud, there has to be a case where either a fraudulent transaction is or is not present .
Hint: This problem is a binary classification problem. Given the problem scenario, what considerations do we have to think about when first building this model? What would the bank fraud data look like?
13. How would you design the inputs and outputs for a model that detects potential bombs at a border crossing?
Additional questions. How would you test the model and measure its accuracy? Remember the equation for precision:
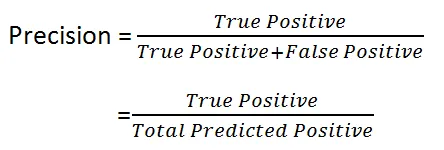
Because we can not have high TrueNegatives, recall should be high when assessing the model.
14. Which model would you choose to predict Airbnb booking prices: Linear regression or random forest regression?
Start by answering this question: What are the main differences between linear regression and random forest?
Random forest regression is based on the ensemble machine learning technique of bagging . The two key concepts of random forests are:
- Random sampling of training observations when building trees.
- Random subsets of features for splitting nodes.
Random forest regressions also discretize continuous variables, since they are based on decision trees and can split categorical and continuous variables.
Linear regression, on the other hand, is the standard regression technique in which relationships are modeled using a linear predictor function, the most common example represented as y = Ax + B.
Let’s see how each model is applicable to Airbnb’s bookings. One thing we need to do in the interview is to understand more context around the problem of predicting bookings. To do so, we need to understand which features are present in our dataset.
We can assume the dataset will have features like:
- Location features.
- Seasonality.
- Number of bedrooms and bathrooms.
- Private room, shared, entire home, etc.
- External demand (conferences, festivals, sporting events).
Which model would be the best fit for this feature set?
15. Using a binary classification model that pre-approves candidates for a loan, how would you give each rejected application a rejection reason?
More context: You do not have access to the feature weights. Start by thinking about the problem like this: How would the problem change if we had ten, one thousand, or ten thousand applicants that had gone through the loan qualification program?
Pretend that we have three people: Alice, Bob, and Candace that have all applied for a loan. Simplifying the financial lending loan model, let us assume the only features are the total number of credit cards , the dollar amount of current debt , and credit age . Here is a scenario:
Alice: 10 credit cards, 5 years of credit age, $\$20K$ in debt
Bob: 10 credit cards, 5 years of credit age, $\$15K$ in debt
Candace: 10 credit cards, 5 years of credit age, $\$10K$ in debt
If Candace is approved, we can logically point to the fact that Candace’s $\$10K$ in debt swung the model to approve her for a loan. How did we reason this out?
If the sample size analyzed was instead thousands of people who had the same number of credit cards and credit age with varying levels of debt, we could figure out the model’s average loan acceptance rate for each numerical amount of current debt. Then we could plot these on a graph to model the y-value (average loan acceptance) versus the x-value (dollar amount of current debt). These graphs are called partial dependence plots.
Business Case Questions
In data science interviews, business case study questions task you with addressing problems as they relate to the business. You might be asked about topics like estimation and calculation, as well as applying problem-solving to a larger case. One tip: Be sure to read up on the company’s products and ventures before your interview to expose yourself to possible topics.
16. How would you estimate the average lifetime value of customers at a business that has existed for just over one year?
More context: You know that the product costs $\$100$ per month, averages 10% in monthly churn, and the average customer stays for 3.5 months.
Remember that lifetime value is defined by the prediction of the net revenue attributed to the entire future relationship with all customers averaged. Therefore, $\$100$ * 3.5 = $\$350$… But is it that simple?
Because this company is so new, our average customer length (3.5 months) is biased from the short possible length of time that anyone could have been a customer (one year maximum). How would you then model out LTV knowing the churn rate and product cost?
17. How would you go about removing duplicate product names (e.g. iPhone X vs. Apple iPhone 10) in a massive database?
See the full solution for this Amazon business case question on YouTube:
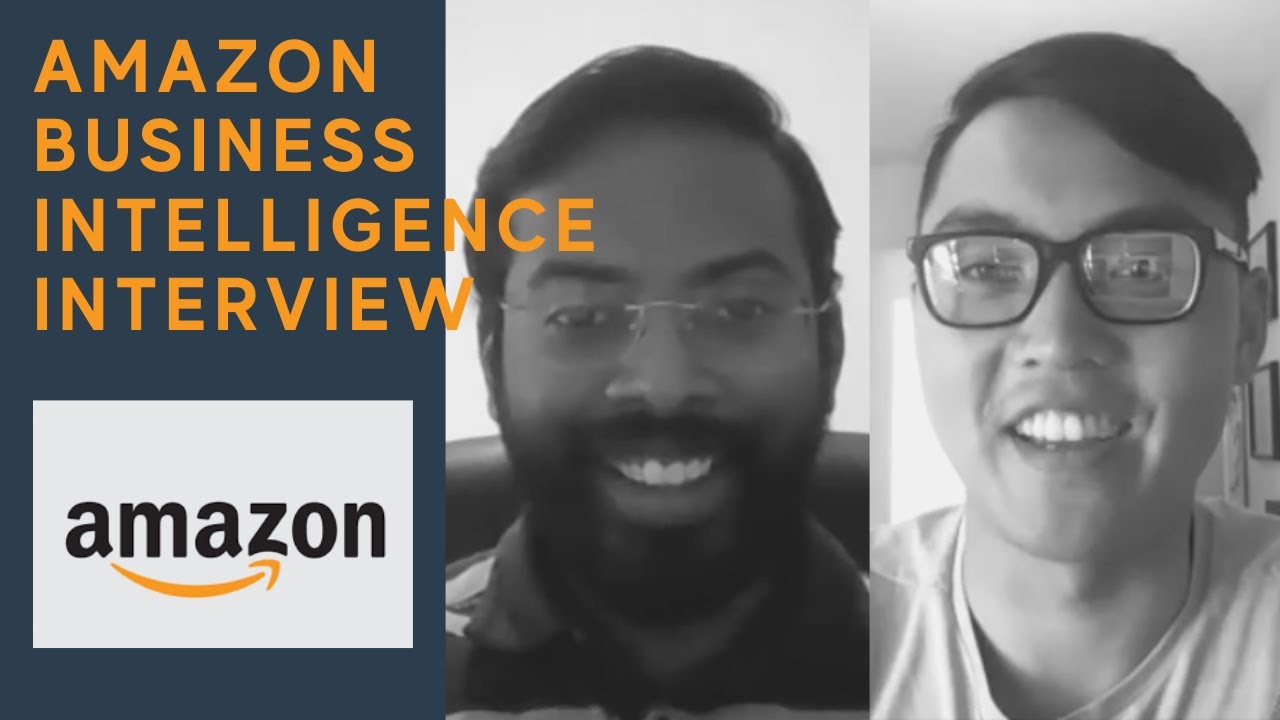
18. What metrics would you monitor to know if a 50% discount promotion is a good idea for a ride-sharing company?
This question has no correct answer and is rather designed to test your reasoning and communication skills related to product/business cases. First, start by stating your assumptions. What are the goals of this promotion? It is likely that the goal of the discount is to grow revenue and increase retention. A few other assumptions you might make include:
- The promotion will be applied uniformly across all users.
- The 50% discount can only be used for a single ride.
How would we be able to evaluate this pricing strategy? An A/B test between the control group (no discount) and test group (discount) would allow us to evaluate Long-term revenue vs average cost of the promotion. Using these two metrics how could we measure if the promotion is a good idea?
19. A bank wants to create a new partner card, e.g. Whole Foods Chase credit card). How would you determine what the next partner card should be?
More context: Say you have access to all customer spending data. With this question, there are several approaches you can take. As your first step, think about the business reason for credit card partnerships: they help increase acquisition and customer retention.
One of the simplest solutions would be to sum all transactions grouped by merchants. This would identify the merchants who see the highest spending amounts. However, the one issue might be that some merchants have a high-spend value but low volume. How could we counteract this potential pitfall? Is the volume of transactions even an important factor in our credit card business? The more questions you ask, the more may spring to mind.
20. How would you assess the value of keeping a TV show on a streaming platform like Netflix?
Say that Netflix is working on a deal to renew the streaming rights for a show like The Office , which has been on Netflix for one year. Your job is to value the benefit of keeping the show on Netflix.
Start by trying to understand the reasons why Netflix would want to renew the show. Netflix mainly has three goals for what their content should help achieve:
- Acquisition: To increase the number of subscribers.
- Retention: To increase the retention of active subscribers and keep them on as paying members.
- Revenue: To increase overall revenue.
One solution to value the benefit would be to estimate a lower and upper bound to understand the percentage of users that would be affected by The Office being removed. You could then run these percentages against your known acquisition and retention rates.
21. How would you determine which products are to be put on sale?
Let’s say you work at Amazon. It’s nearing Black Friday, and you are tasked with determining which products should be put on sale. You have access to historical pricing and purchasing data from items that have been on sale before. How would you determine what products should go on sale to best maximize profit during Black Friday?
To start with this question, aggregate data from previous years for products that have been on sale during Black Friday or similar events. You can then compare elements such as historical sales volume, inventory levels, and profit margins.
Learn More About Feature Changes
This course is designed teach you everything you need to know about feature changes:
More Data Science Interview Resources
Case studies are one of the most common types of data science interview questions . Practice with the data science course from Interview Query, which includes product and machine learning modules.

The New Equation

Executive leadership hub - What’s important to the C-suite?
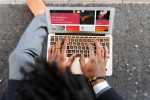
Tech Effect

Shared success benefits
Loading Results
No Match Found
Data analytics case study data files
Inventory analysis case study data files:.
Beginning Inventory
Purchase Prices
Vendor Invoices
Ending Inventory
Inventory Analysis Case Study Instructor files:
Instructor guide
Phase 1 - Data Collection and Preparation
Phase 2 - Data Discovery and Visualization
Phase 3 - Introduction to Statistical Analysis

Stay up to date
Subscribe to our University Relations distribution list
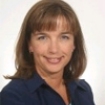
Julie Peters
University Relations leader, PwC US

© 2017 - 2024 PwC. All rights reserved. PwC refers to the PwC network and/or one or more of its member firms, each of which is a separate legal entity. Please see www.pwc.com/structure for further details.
- Data Privacy Framework
- Cookie info
- Terms and conditions
- Site provider
- Your Privacy Choices

Data Analytics Case Study Guide 2024
by Sam McKay, CFA | Data Analytics
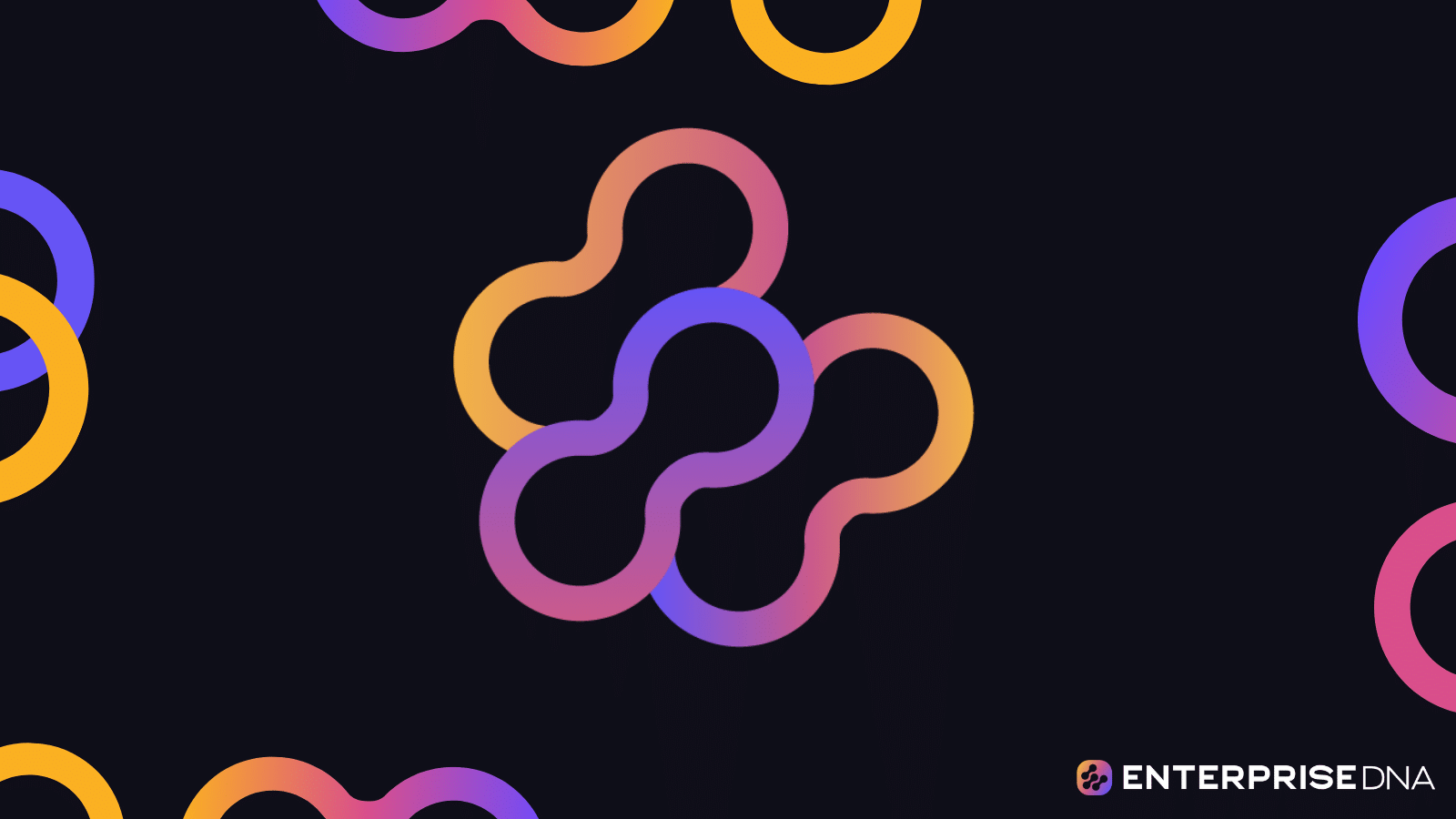
Data analytics case studies reveal how businesses harness data for informed decisions and growth.
For aspiring data professionals, mastering the case study process will enhance your skills and increase your career prospects.
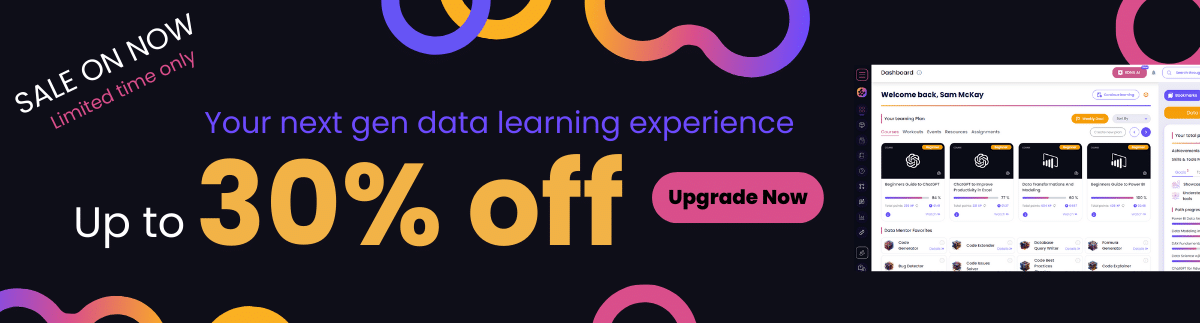
So, how do you approach a case study?
Use these steps to process a data analytics case study:
Understand the Problem: Grasp the core problem or question addressed in the case study.
Collect Relevant Data: Gather data from diverse sources, ensuring accuracy and completeness.
Apply Analytical Techniques: Use appropriate methods aligned with the problem statement.
Visualize Insights: Utilize visual aids to showcase patterns and key findings.
Derive Actionable Insights: Focus on deriving meaningful actions from the analysis.
This article will give you detailed steps to navigate a case study effectively and understand how it works in real-world situations.
By the end of the article, you will be better equipped to approach a data analytics case study, strengthening your analytical prowess and practical application skills.
Let’s dive in!
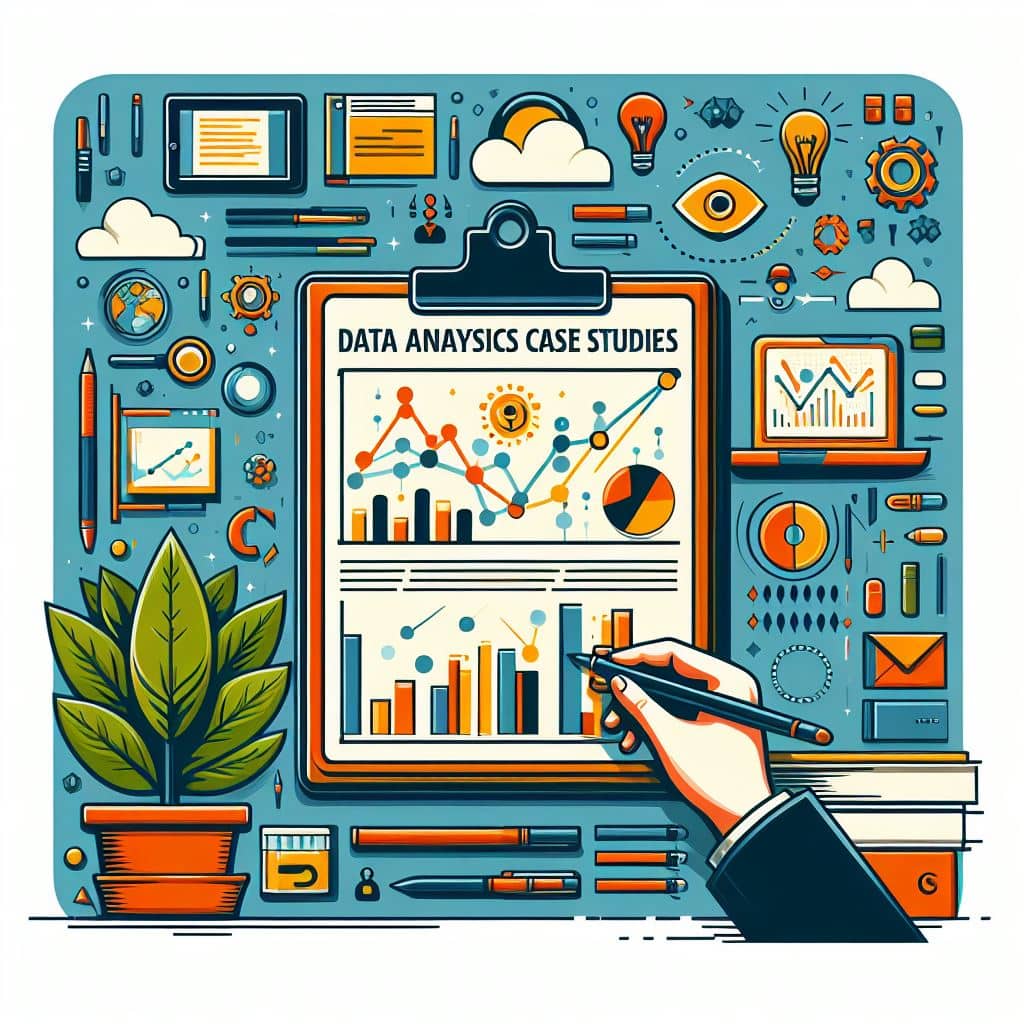
Table of Contents
What is a Data Analytics Case Study?
A data analytics case study is a real or hypothetical scenario where analytics techniques are applied to solve a specific problem or explore a particular question.
It’s a practical approach that uses data analytics methods, assisting in deciphering data for meaningful insights. This structured method helps individuals or organizations make sense of data effectively.
Additionally, it’s a way to learn by doing, where there’s no single right or wrong answer in how you analyze the data.
So, what are the components of a case study?
Key Components of a Data Analytics Case Study
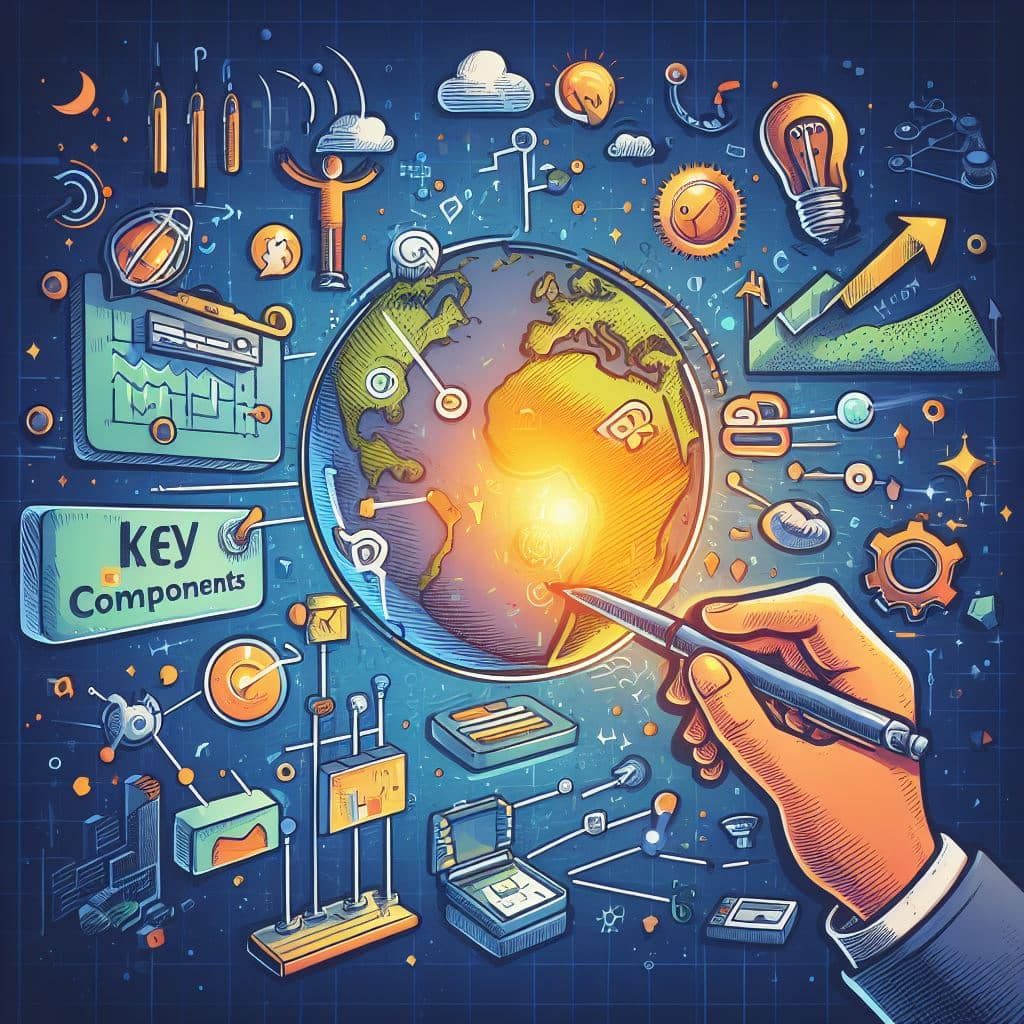
A data analytics case study comprises essential elements that structure the analytical journey:
Problem Context: A case study begins with a defined problem or question. It provides the context for the data analysis , setting the stage for exploration and investigation.
Data Collection and Sources: It involves gathering relevant data from various sources , ensuring data accuracy, completeness, and relevance to the problem at hand.
Analysis Techniques: Case studies employ different analytical methods, such as statistical analysis, machine learning algorithms, or visualization tools, to derive meaningful conclusions from the collected data.
Insights and Recommendations: The ultimate goal is to extract actionable insights from the analyzed data, offering recommendations or solutions that address the initial problem or question.
Now that you have a better understanding of what a data analytics case study is, let’s talk about why we need and use them.
Why Case Studies are Integral to Data Analytics
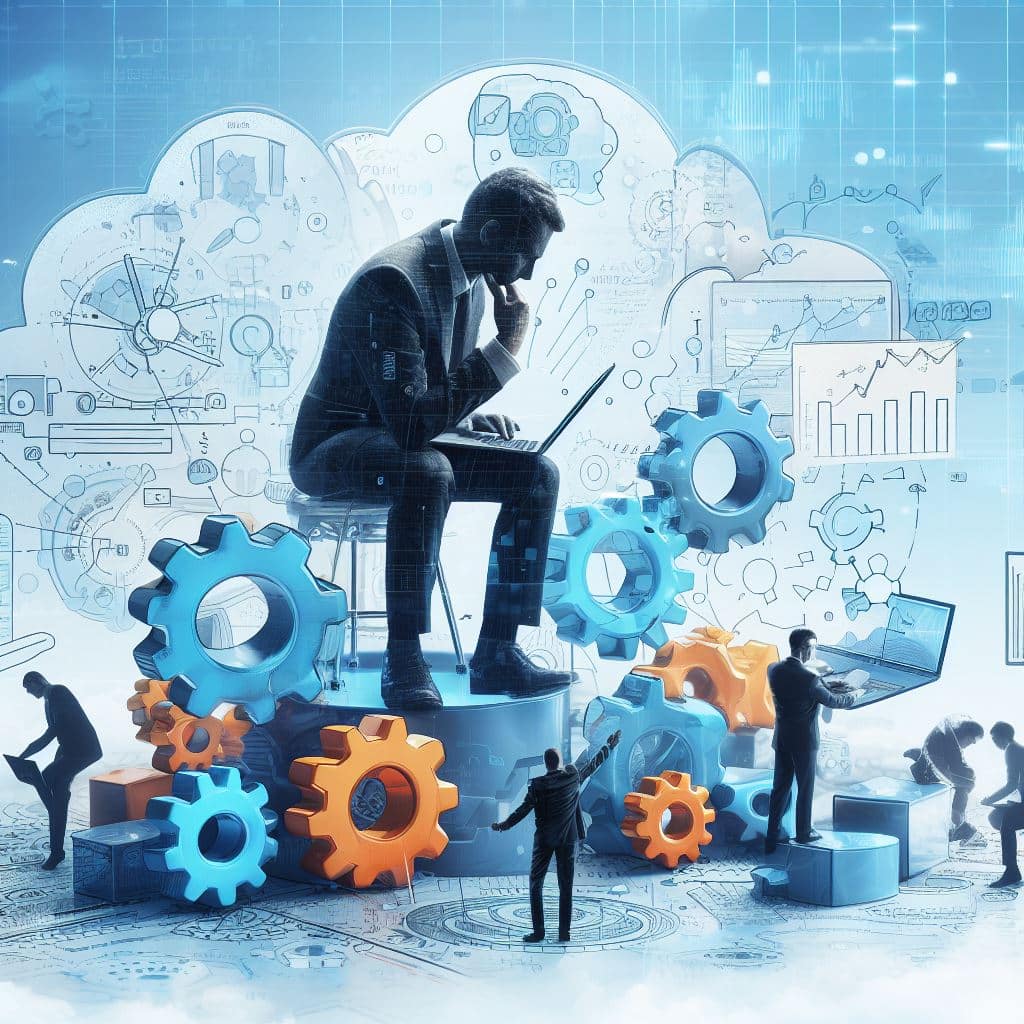
Case studies serve as invaluable tools in the realm of data analytics, offering multifaceted benefits that bolster an analyst’s proficiency and impact:
Real-Life Insights and Skill Enhancement: Examining case studies provides practical, real-life examples that expand knowledge and refine skills. These examples offer insights into diverse scenarios, aiding in a data analyst’s growth and expertise development.
Validation and Refinement of Analyses: Case studies demonstrate the effectiveness of data-driven decisions across industries, providing validation for analytical approaches. They showcase how organizations benefit from data analytics. Also, this helps in refining one’s own methodologies
Showcasing Data Impact on Business Outcomes: These studies show how data analytics directly affects business results, like increasing revenue, reducing costs, or delivering other measurable advantages. Understanding these impacts helps articulate the value of data analytics to stakeholders and decision-makers.
Learning from Successes and Failures: By exploring a case study, analysts glean insights from others’ successes and failures, acquiring new strategies and best practices. This learning experience facilitates professional growth and the adoption of innovative approaches within their own data analytics work.
Including case studies in a data analyst’s toolkit helps gain more knowledge, improve skills, and understand how data analytics affects different industries.
Using these real-life examples boosts confidence and success, guiding analysts to make better and more impactful decisions in their organizations.
But not all case studies are the same.
Let’s talk about the different types.
Types of Data Analytics Case Studies
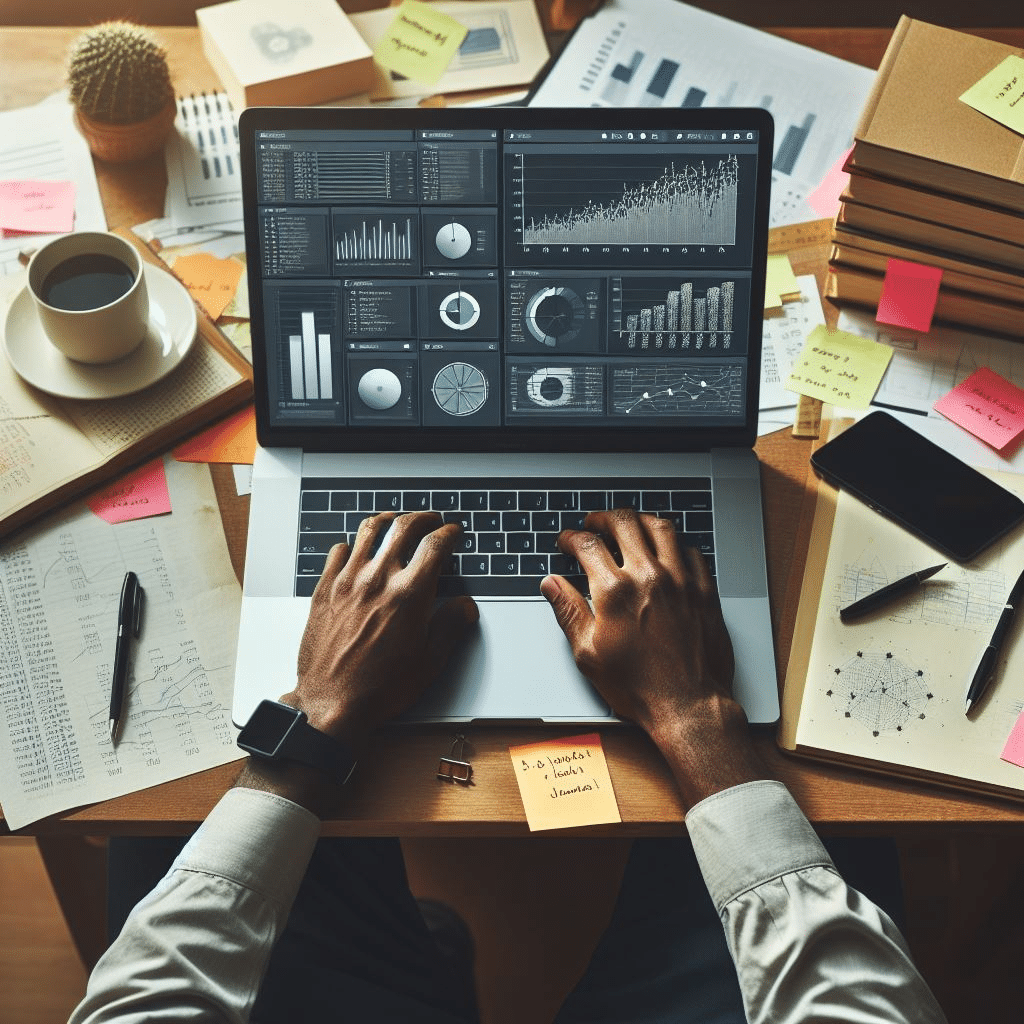
Data analytics encompasses various approaches tailored to different analytical goals:
Exploratory Case Study: These involve delving into new datasets to uncover hidden patterns and relationships, often without a predefined hypothesis. They aim to gain insights and generate hypotheses for further investigation.
Predictive Case Study: These utilize historical data to forecast future trends, behaviors, or outcomes. By applying predictive models, they help anticipate potential scenarios or developments.
Diagnostic Case Study: This type focuses on understanding the root causes or reasons behind specific events or trends observed in the data. It digs deep into the data to provide explanations for occurrences.
Prescriptive Case Study: This case study goes beyond analytics; it provides actionable recommendations or strategies derived from the analyzed data. They guide decision-making processes by suggesting optimal courses of action based on insights gained.
Each type has a specific role in using data to find important insights, helping in decision-making, and solving problems in various situations.
Regardless of the type of case study you encounter, here are some steps to help you process them.
Roadmap to Handling a Data Analysis Case Study
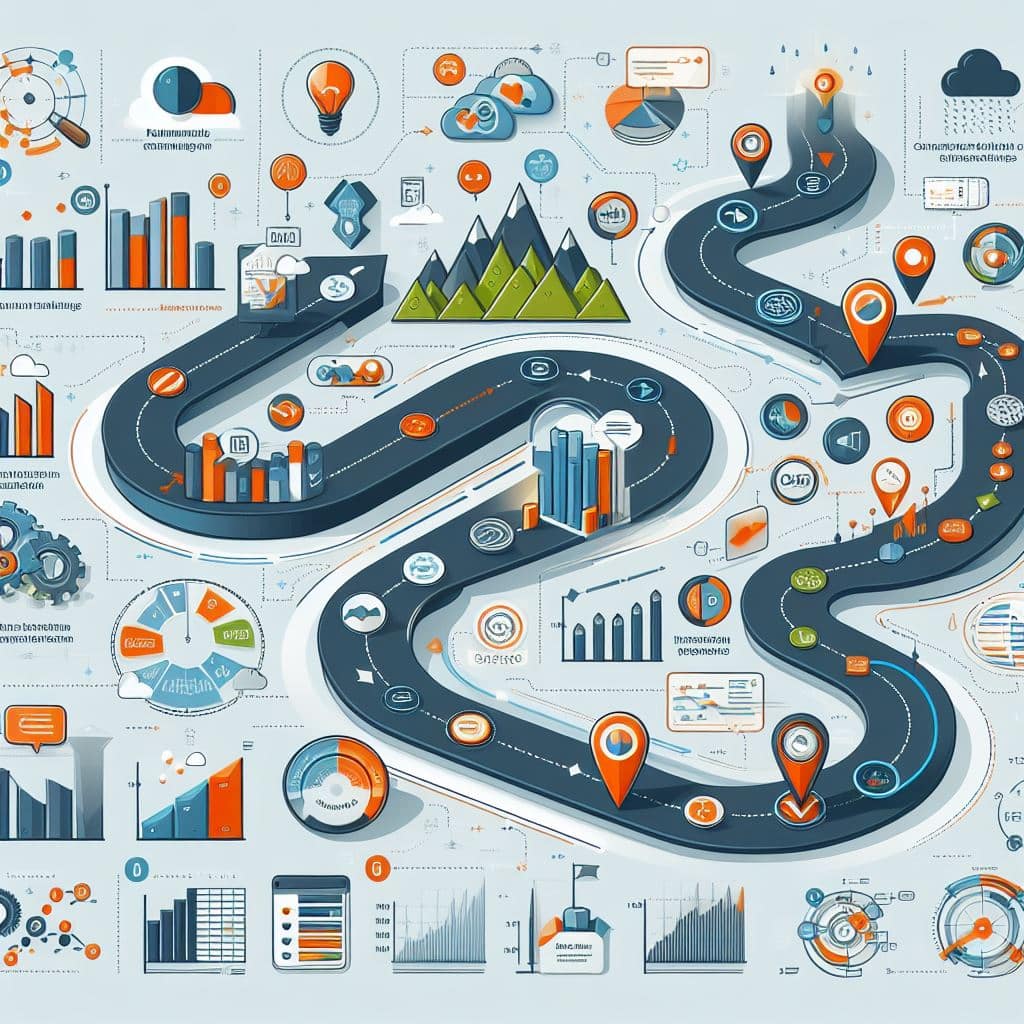
Embarking on a data analytics case study requires a systematic approach, step-by-step, to derive valuable insights effectively.
Here are the steps to help you through the process:
Step 1: Understanding the Case Study Context: Immerse yourself in the intricacies of the case study. Delve into the industry context, understanding its nuances, challenges, and opportunities.
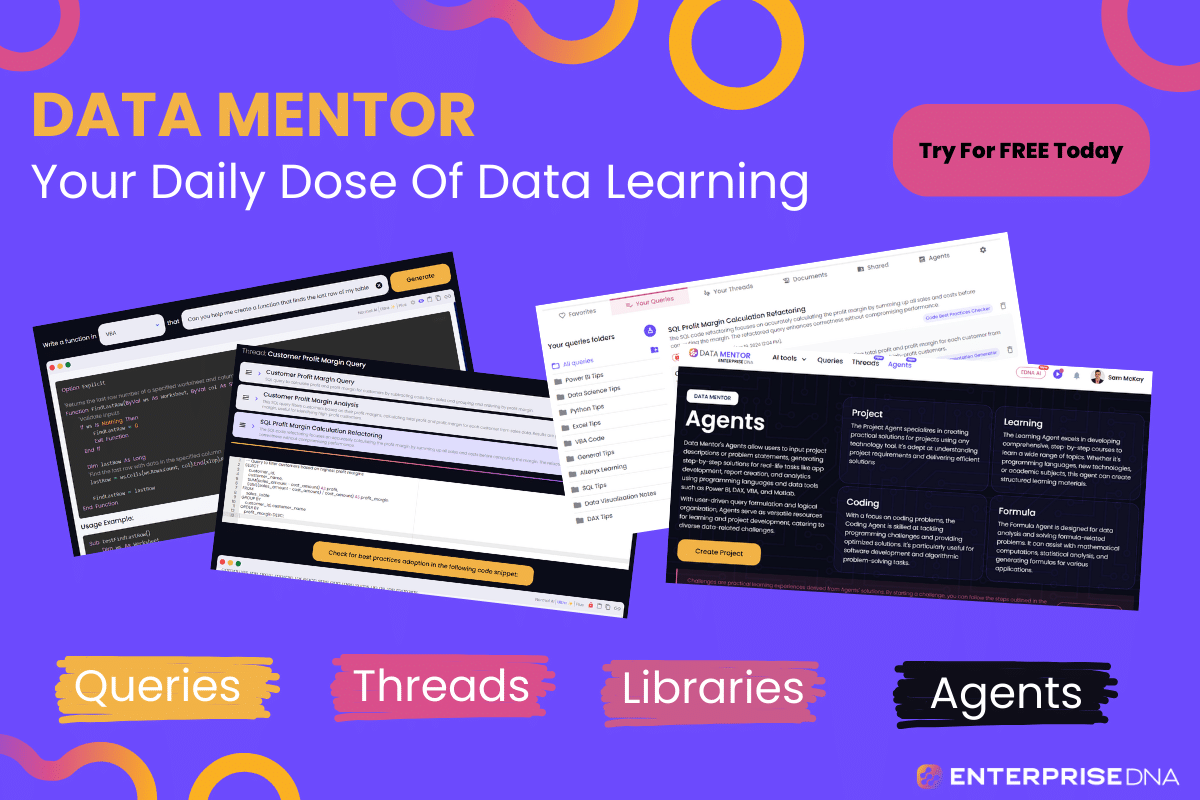
Identify the central problem or question the study aims to address. Clarify the objectives and expected outcomes, ensuring a clear understanding before diving into data analytics.
Step 2: Data Collection and Validation: Gather data from diverse sources relevant to the case study. Prioritize accuracy, completeness, and reliability during data collection. Conduct thorough validation processes to rectify inconsistencies, ensuring high-quality and trustworthy data for subsequent analysis.
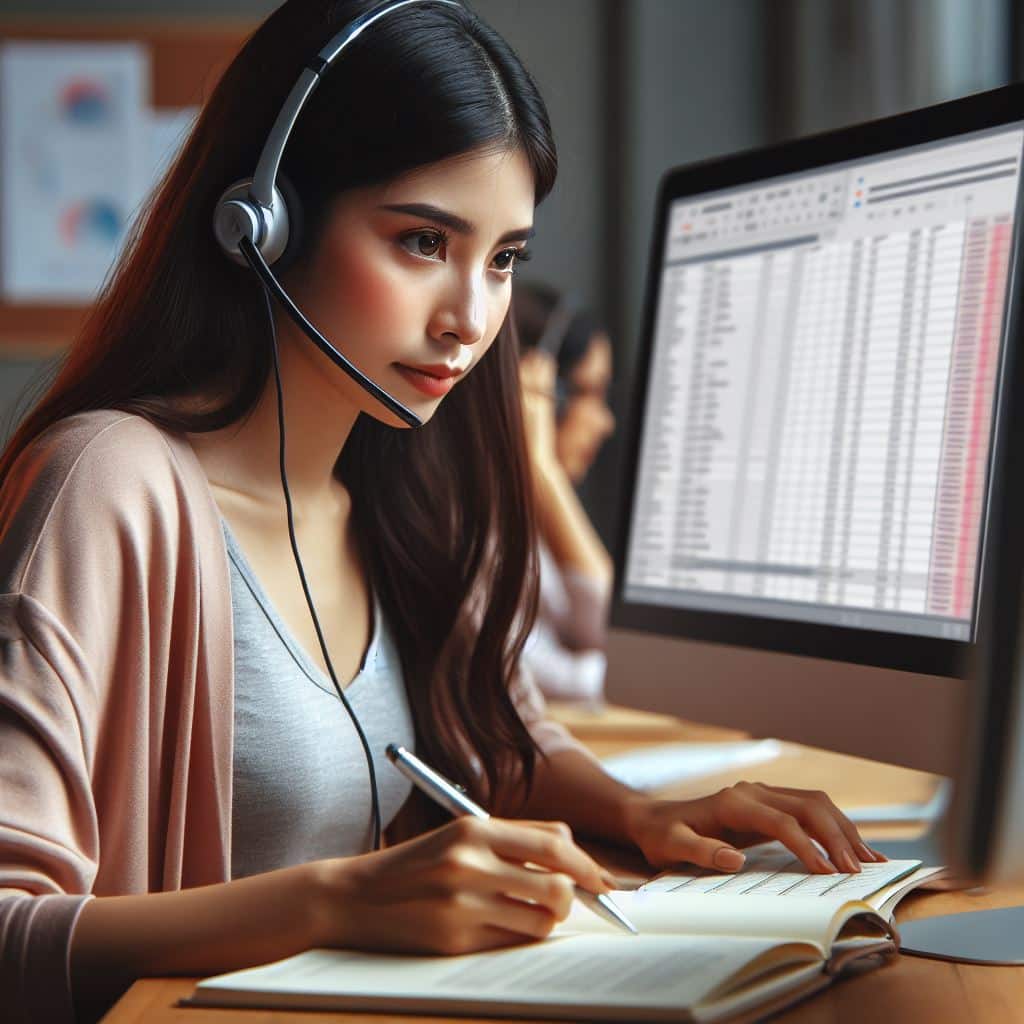
Step 3: Problem Definition and Scope: Define the problem statement precisely. Articulate the objectives and limitations that shape the scope of your analysis. Identify influential variables and constraints, providing a focused framework to guide your exploration.
Step 4: Exploratory Data Analysis (EDA): Leverage exploratory techniques to gain initial insights. Visualize data distributions, patterns, and correlations, fostering a deeper understanding of the dataset. These explorations serve as a foundation for more nuanced analysis.
Step 5: Data Preprocessing and Transformation: Cleanse and preprocess the data to eliminate noise, handle missing values, and ensure consistency. Transform data formats or scales as required, preparing the dataset for further analysis.
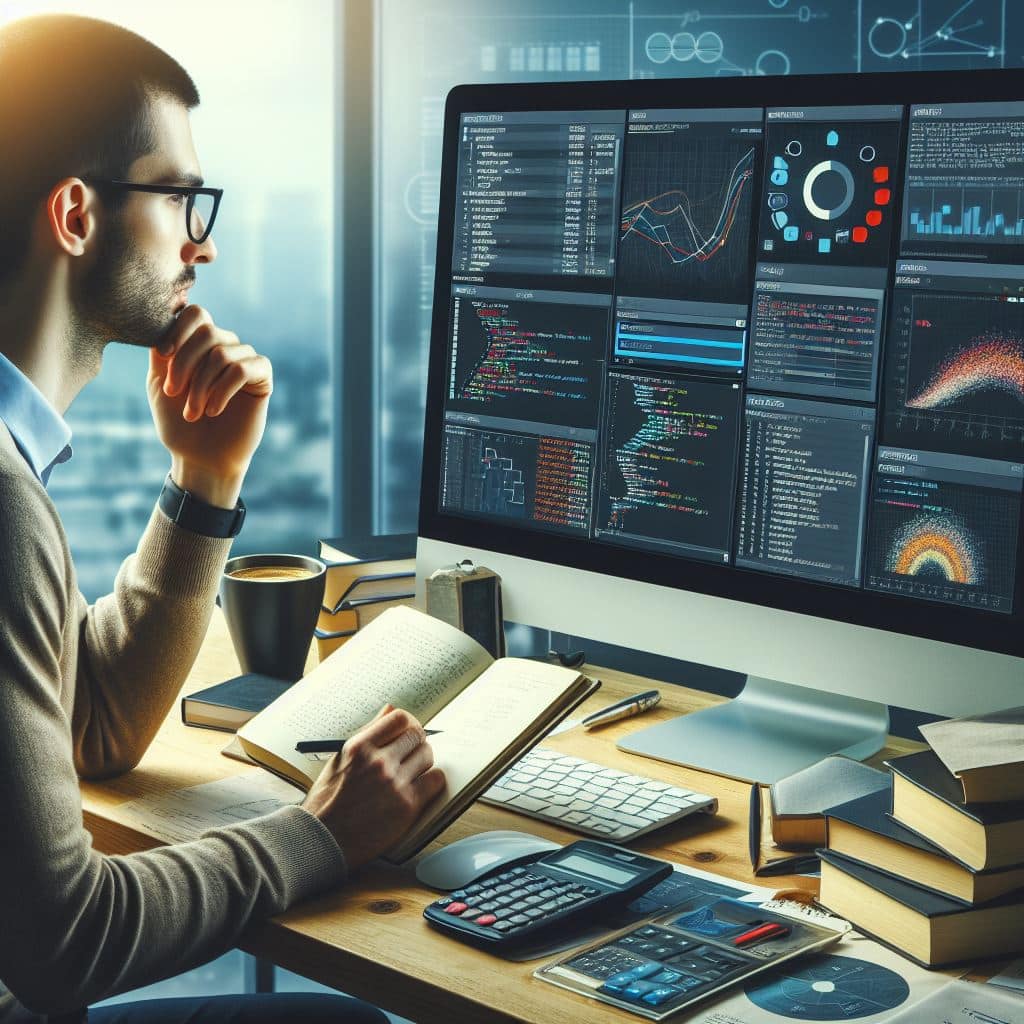
Step 6: Data Modeling and Method Selection: Select analytical models aligning with the case study’s problem, employing statistical techniques, machine learning algorithms, or tailored predictive models.
In this phase, it’s important to develop data modeling skills. This helps create visuals of complex systems using organized data, which helps solve business problems more effectively.
Understand key data modeling concepts, utilize essential tools like SQL for database interaction, and practice building models from real-world scenarios.
Furthermore, strengthen data cleaning skills for accurate datasets, and stay updated with industry trends to ensure relevance.
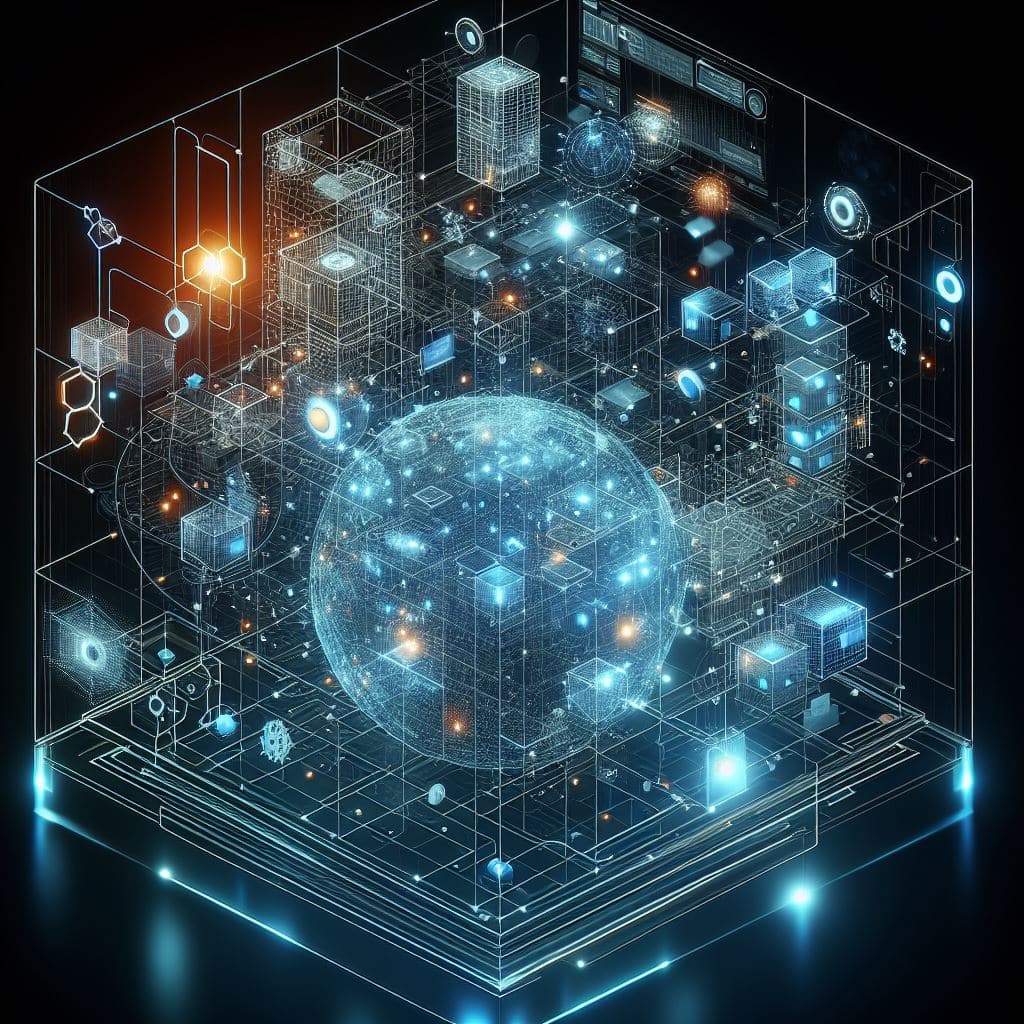
Step 7: Model Evaluation and Refinement: Evaluate the performance of applied models rigorously. Iterate and refine models to enhance accuracy and reliability, ensuring alignment with the objectives and expected outcomes.
Step 8: Deriving Insights and Recommendations: Extract actionable insights from the analyzed data. Develop well-structured recommendations or solutions based on the insights uncovered, addressing the core problem or question effectively.
Step 9: Communicating Results Effectively: Present findings, insights, and recommendations clearly and concisely. Utilize visualizations and storytelling techniques to convey complex information compellingly, ensuring comprehension by stakeholders.
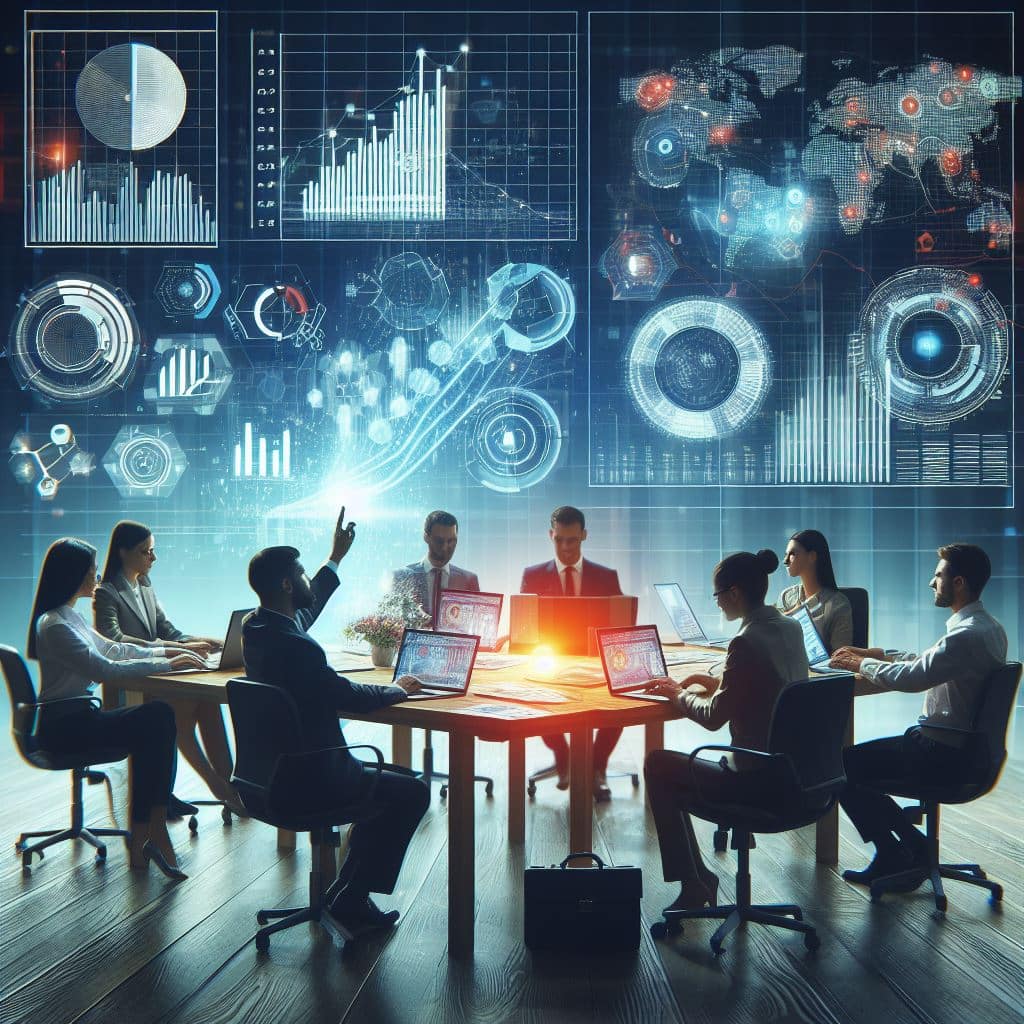
Step 10: Reflection and Iteration: Reflect on the entire analysis process and outcomes. Identify potential improvements and lessons learned. Embrace an iterative approach, refining methodologies for continuous enhancement and future analyses.
This step-by-step roadmap provides a structured framework for thorough and effective handling of a data analytics case study.
Now, after handling data analytics comes a crucial step; presenting the case study.
Presenting Your Data Analytics Case Study
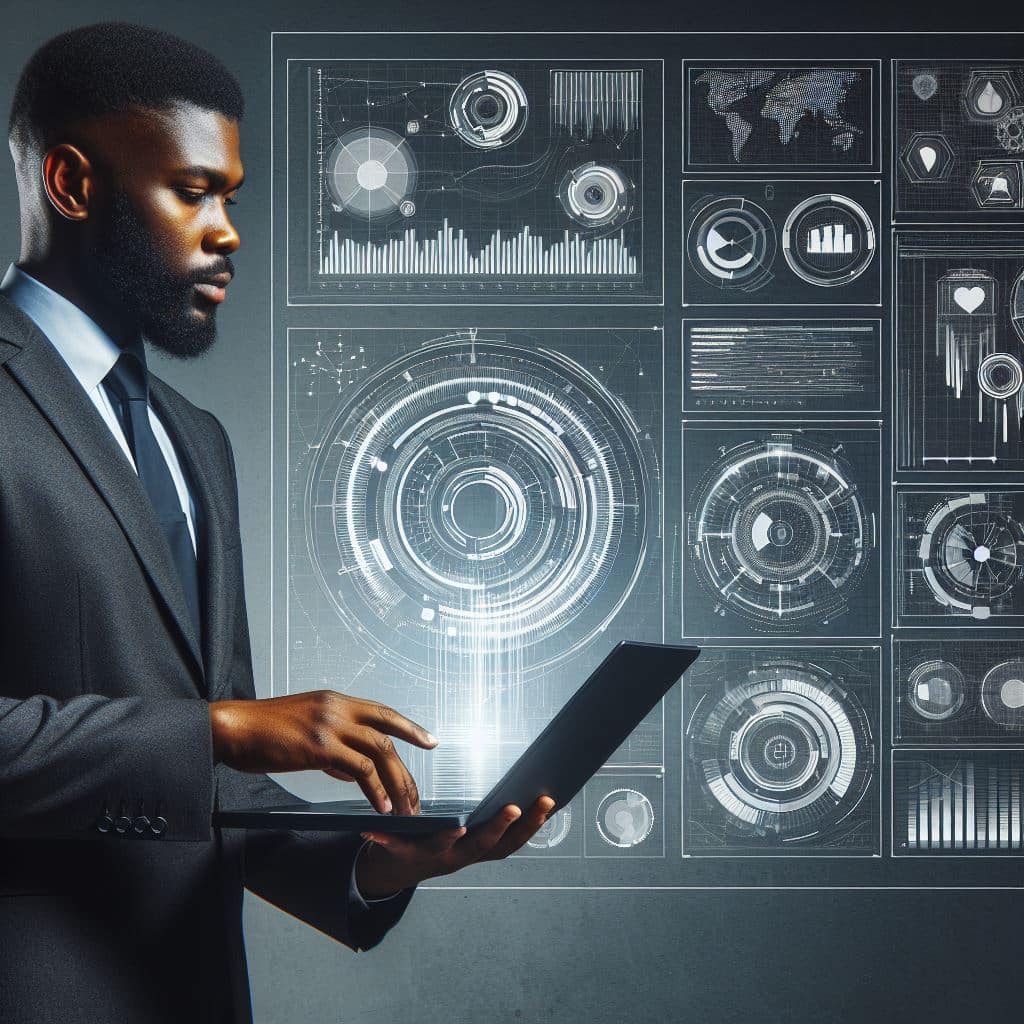
Presenting a data analytics case study is a vital part of the process. When presenting your case study, clarity and organization are paramount.
To achieve this, follow these key steps:
Structuring Your Case Study: Start by outlining relevant and accurate main points. Ensure these points align with the problem addressed and the methodologies used in your analysis.
Crafting a Narrative with Data: Start with a brief overview of the issue, then explain your method and steps, covering data collection, cleaning, stats, and advanced modeling.
Visual Representation for Clarity: Utilize various visual aids—tables, graphs, and charts—to illustrate patterns, trends, and insights. Ensure these visuals are easy to comprehend and seamlessly support your narrative.
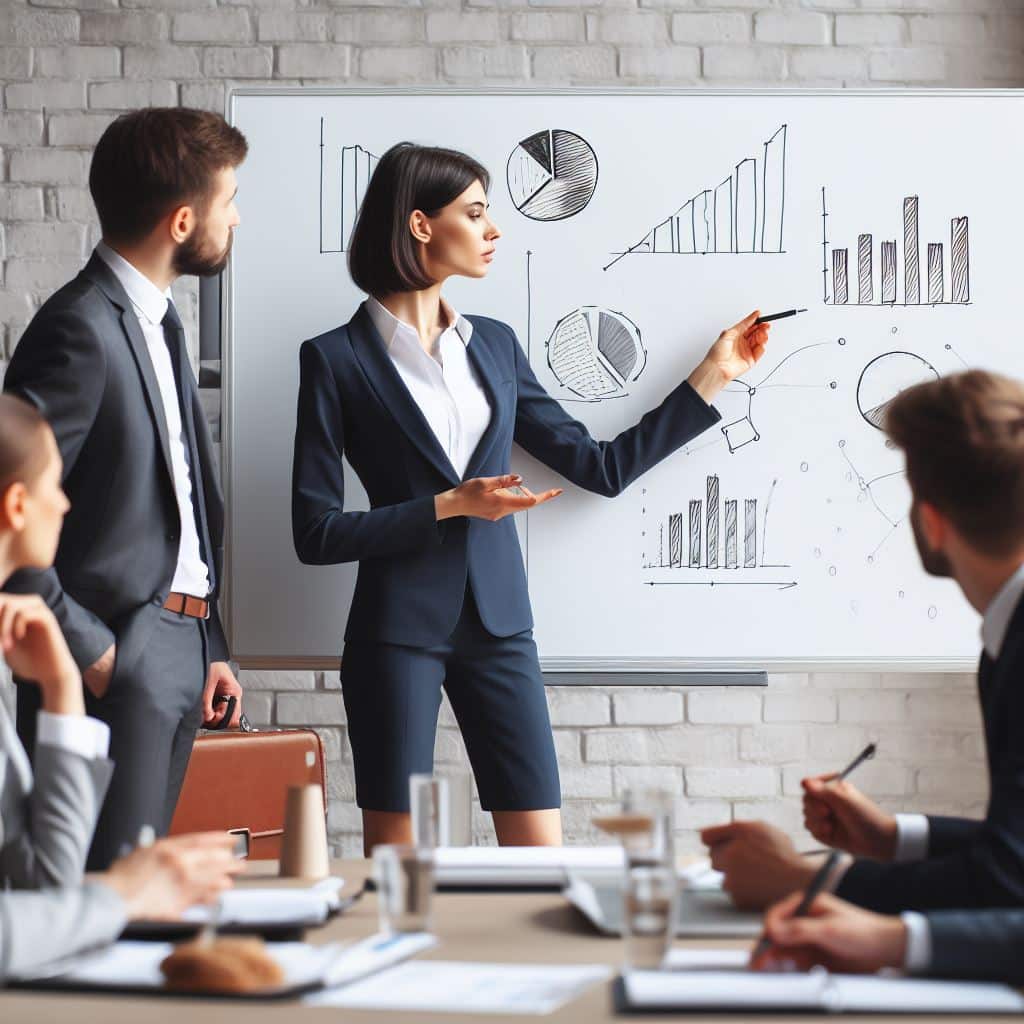
Highlighting Key Information: Use bullet points to emphasize essential information, maintaining clarity and allowing the audience to grasp key takeaways effortlessly. Bold key terms or phrases to draw attention and reinforce important points.
Addressing Audience Queries: Anticipate and be ready to answer audience questions regarding methods, assumptions, and results. Demonstrating a profound understanding of your analysis instills confidence in your work.
Integrity and Confidence in Delivery: Maintain a neutral tone and avoid exaggerated claims about findings. Present your case study with integrity, clarity, and confidence to ensure the audience appreciates and comprehends the significance of your work.
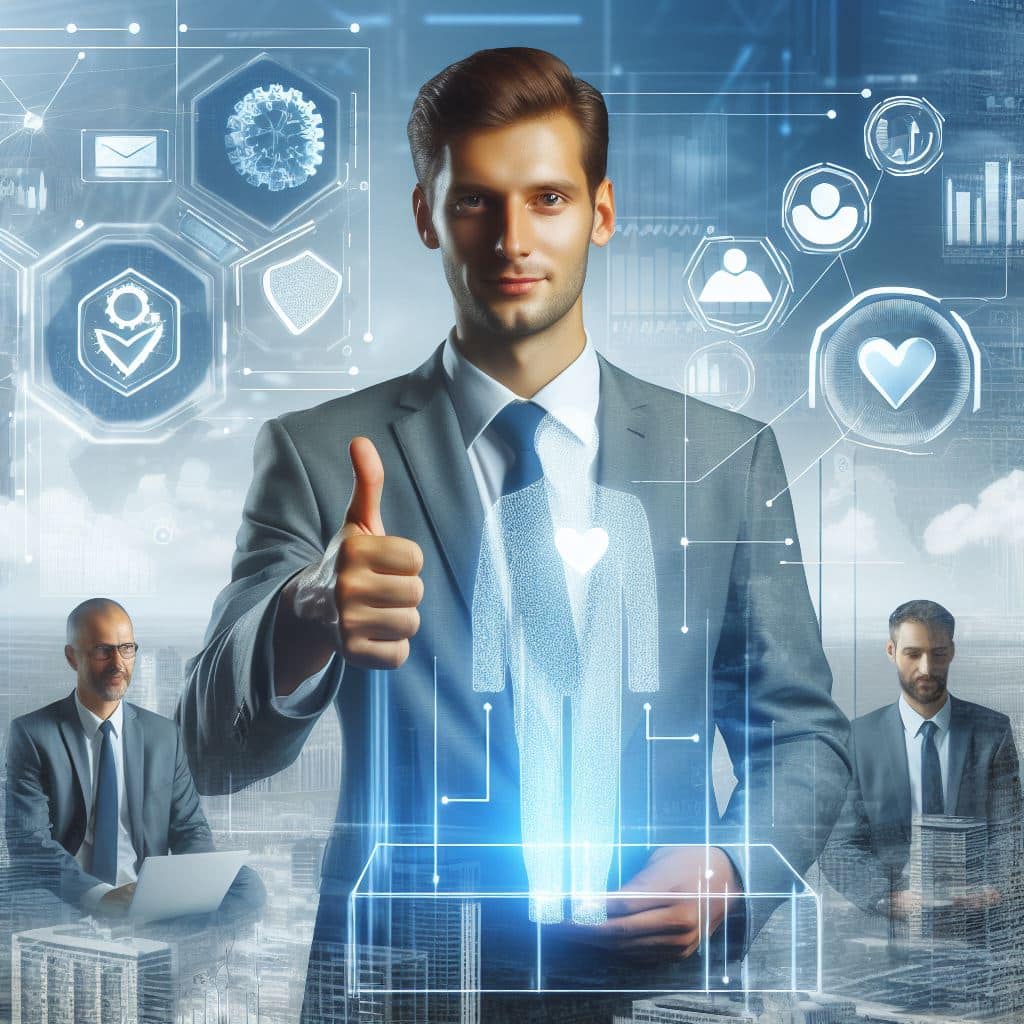
By organizing your presentation well, telling a clear story through your analysis, and using visuals wisely, you can effectively share your data analytics case study.
This method helps people understand better, stay engaged, and draw valuable conclusions from your work.
We hope by now, you are feeling very confident processing a case study. But with any process, there are challenges you may encounter.
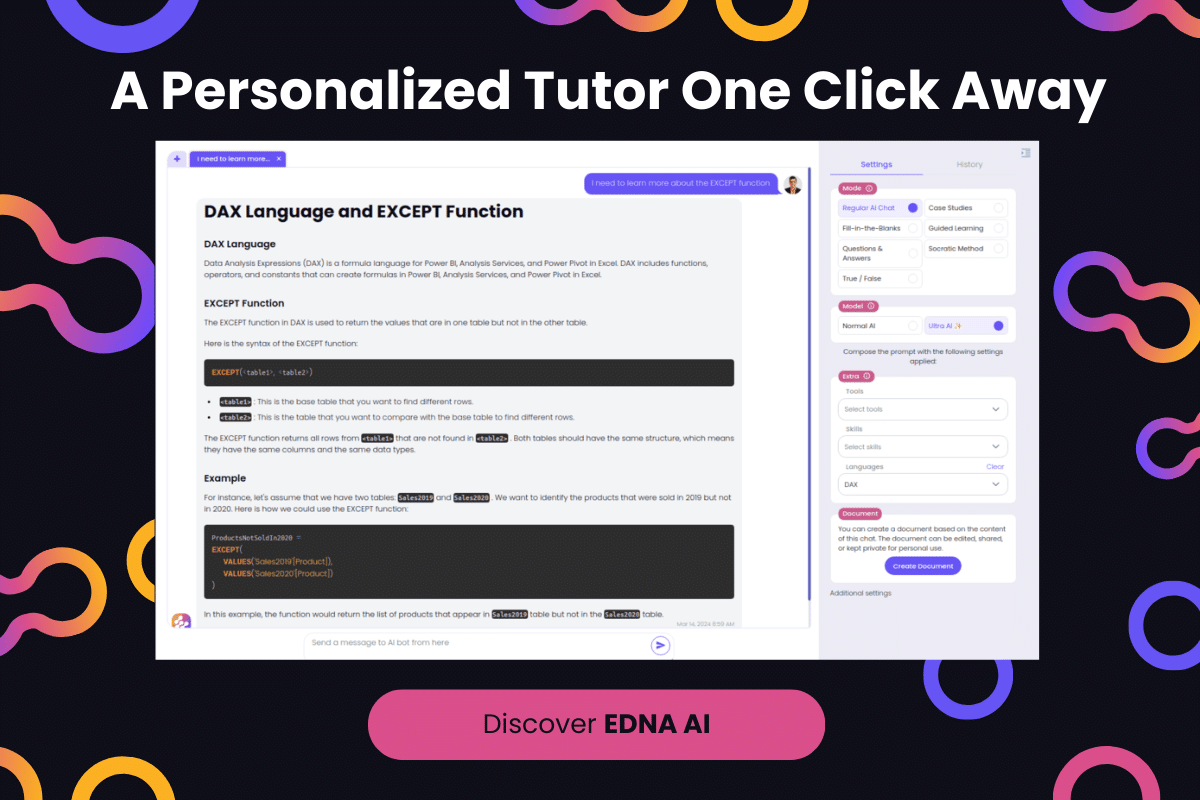
Key Challenges in Data Analytics Case Studies
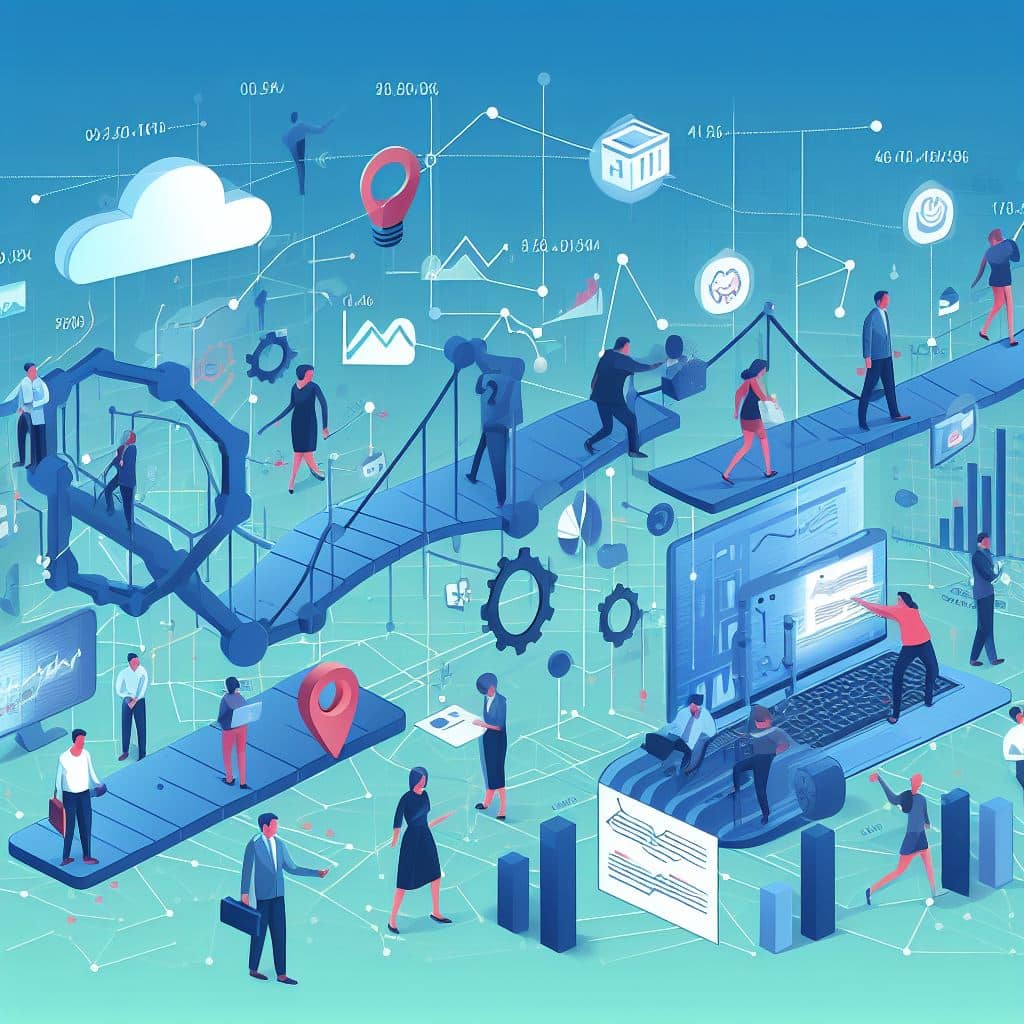
A data analytics case study can present various hurdles that necessitate strategic approaches for successful navigation:
Challenge 1: Data Quality and Consistency
Challenge: Inconsistent or poor-quality data can impede analysis, leading to erroneous insights and flawed conclusions.
Solution: Implement rigorous data validation processes, ensuring accuracy, completeness, and reliability. Employ data cleansing techniques to rectify inconsistencies and enhance overall data quality.
Challenge 2: Complexity and Scale of Data
Challenge: Managing vast volumes of data with diverse formats and complexities poses analytical challenges.
Solution: Utilize scalable data processing frameworks and tools capable of handling diverse data types. Implement efficient data storage and retrieval systems to manage large-scale datasets effectively.
Challenge 3: Interpretation and Contextual Understanding
Challenge: Interpreting data without contextual understanding or domain expertise can lead to misinterpretations.
Solution: Collaborate with domain experts to contextualize data and derive relevant insights. Invest in understanding the nuances of the industry or domain under analysis to ensure accurate interpretations.
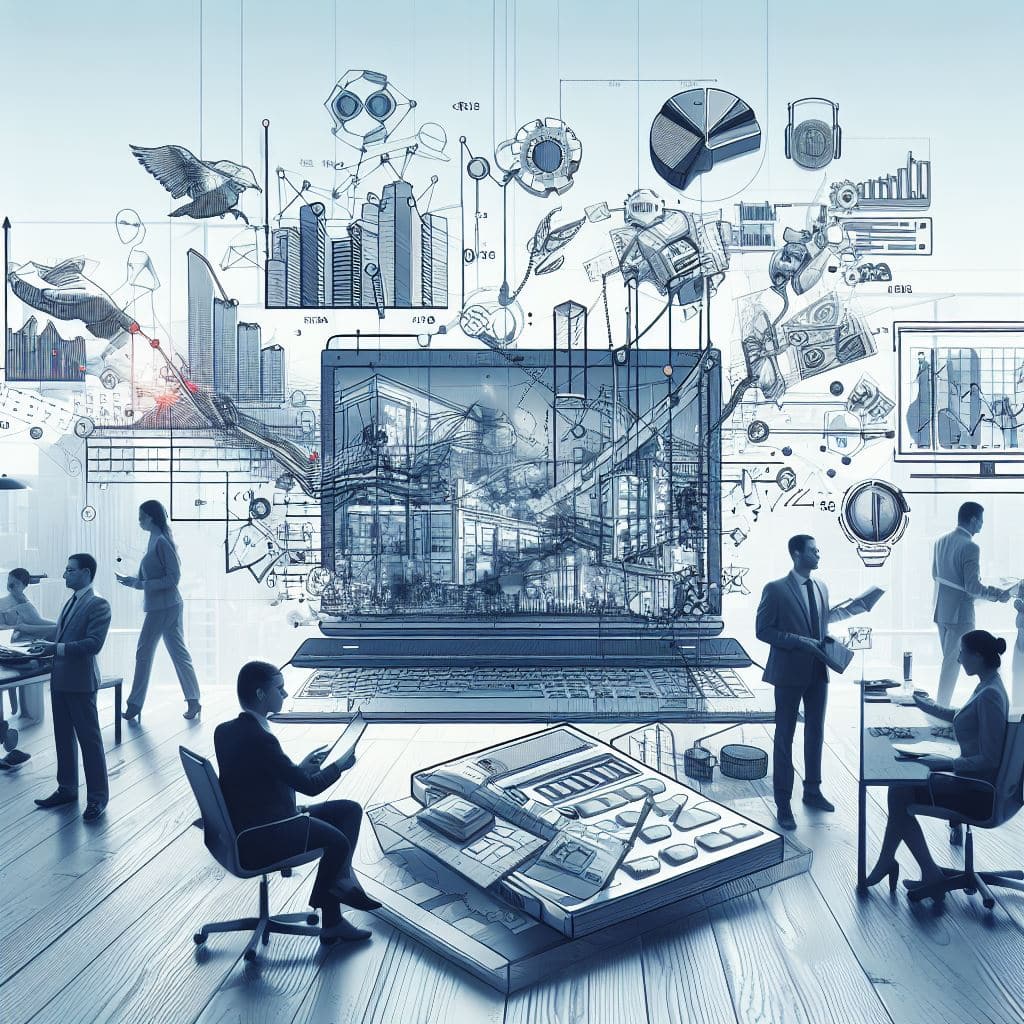
Challenge 4: Privacy and Ethical Concerns
Challenge: Balancing data access for analysis while respecting privacy and ethical boundaries poses a challenge.
Solution: Implement robust data governance frameworks that prioritize data privacy and ethical considerations. Ensure compliance with regulatory standards and ethical guidelines throughout the analysis process.
Challenge 5: Resource Limitations and Time Constraints
Challenge: Limited resources and time constraints hinder comprehensive analysis and exhaustive data exploration.
Solution: Prioritize key objectives and allocate resources efficiently. Employ agile methodologies to iteratively analyze and derive insights, focusing on the most impactful aspects within the given timeframe.
Recognizing these challenges is key; it helps data analysts adopt proactive strategies to mitigate obstacles. This enhances the effectiveness and reliability of insights derived from a data analytics case study.
Now, let’s talk about the best software tools you should use when working with case studies.
Top 5 Software Tools for Case Studies
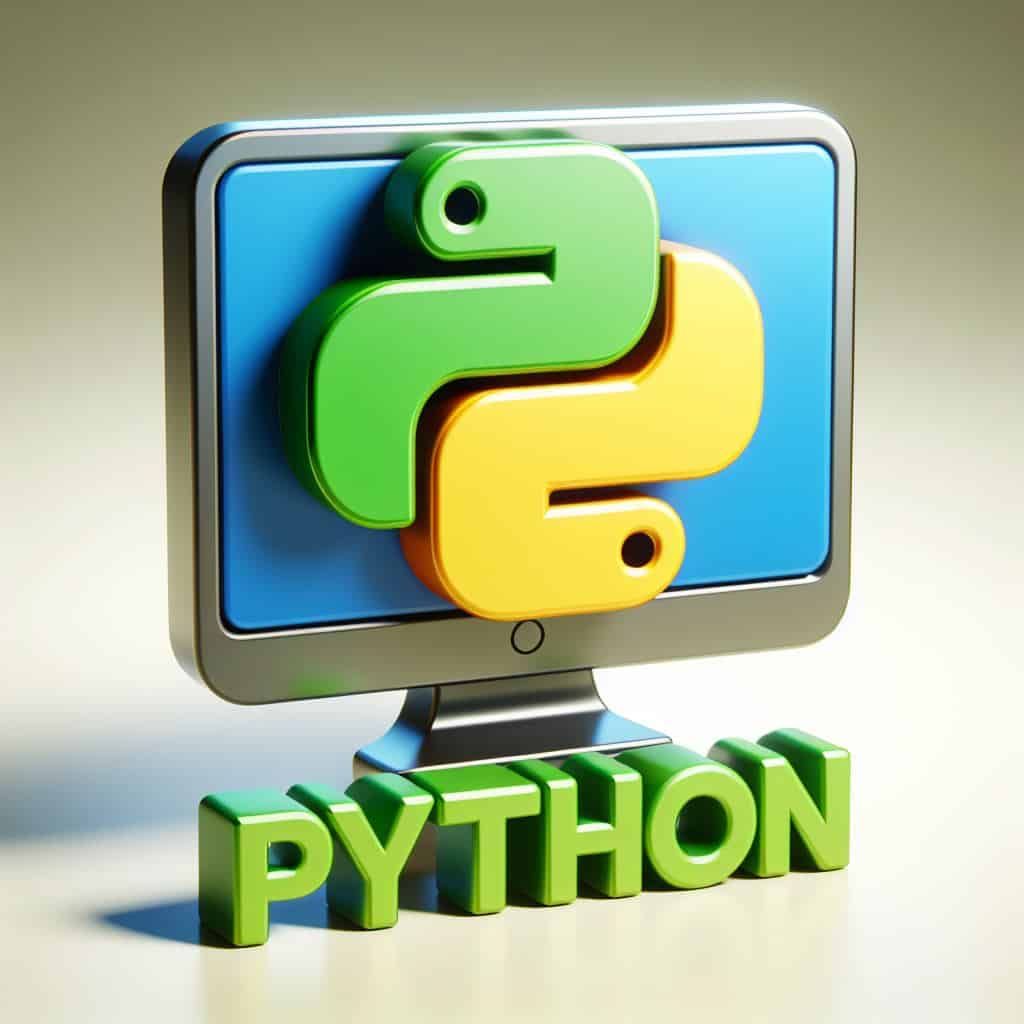
In the realm of case studies within data analytics, leveraging the right software tools is essential.
Here are some top-notch options:
Tableau : Renowned for its data visualization prowess, Tableau transforms raw data into interactive, visually compelling representations, ideal for presenting insights within a case study.
Python and R Libraries: These flexible programming languages provide many tools for handling data, doing statistics, and working with machine learning, meeting various needs in case studies.
Microsoft Excel : A staple tool for data analytics, Excel provides a user-friendly interface for basic analytics, making it useful for initial data exploration in a case study.
SQL Databases : Structured Query Language (SQL) databases assist in managing and querying large datasets, essential for organizing case study data effectively.
Statistical Software (e.g., SPSS , SAS ): Specialized statistical software enables in-depth statistical analysis, aiding in deriving precise insights from case study data.
Choosing the best mix of these tools, tailored to each case study’s needs, greatly boosts analytical abilities and results in data analytics.
Final Thoughts
Case studies in data analytics are helpful guides. They give real-world insights, improve skills, and show how data-driven decisions work.
Using case studies helps analysts learn, be creative, and make essential decisions confidently in their data work.
Check out our latest clip below to further your learning!
Frequently Asked Questions
What are the key steps to analyzing a data analytics case study.
When analyzing a case study, you should follow these steps:
Clarify the problem : Ensure you thoroughly understand the problem statement and the scope of the analysis.
Make assumptions : Define your assumptions to establish a feasible framework for analyzing the case.
Gather context : Acquire relevant information and context to support your analysis.
Analyze the data : Perform calculations, create visualizations, and conduct statistical analysis on the data.
Provide insights : Draw conclusions and develop actionable insights based on your analysis.
How can you effectively interpret results during a data scientist case study job interview?
During your next data science interview, interpret case study results succinctly and clearly. Utilize visual aids and numerical data to bolster your explanations, ensuring comprehension.
Frame the results in an audience-friendly manner, emphasizing relevance. Concentrate on deriving insights and actionable steps from the outcomes.
How do you showcase your data analyst skills in a project?
To demonstrate your skills effectively, consider these essential steps. Begin by selecting a problem that allows you to exhibit your capacity to handle real-world challenges through analysis.
Methodically document each phase, encompassing data cleaning, visualization, statistical analysis, and the interpretation of findings.
Utilize descriptive analysis techniques and effectively communicate your insights using clear visual aids and straightforward language. Ensure your project code is well-structured, with detailed comments and documentation, showcasing your proficiency in handling data in an organized manner.
Lastly, emphasize your expertise in SQL queries, programming languages, and various analytics tools throughout the project. These steps collectively highlight your competence and proficiency as a skilled data analyst, demonstrating your capabilities within the project.
Can you provide an example of a successful data analytics project using key metrics?
A prime illustration is utilizing analytics in healthcare to forecast hospital readmissions. Analysts leverage electronic health records, patient demographics, and clinical data to identify high-risk individuals.
Implementing preventive measures based on these key metrics helps curtail readmission rates, enhancing patient outcomes and cutting healthcare expenses.
This demonstrates how data analytics, driven by metrics, effectively tackles real-world challenges, yielding impactful solutions.
Why would a company invest in data analytics?
Companies invest in data analytics to gain valuable insights, enabling informed decision-making and strategic planning. This investment helps optimize operations, understand customer behavior, and stay competitive in their industry.
Ultimately, leveraging data analytics empowers companies to make smarter, data-driven choices, leading to enhanced efficiency, innovation, and growth.
Related Posts
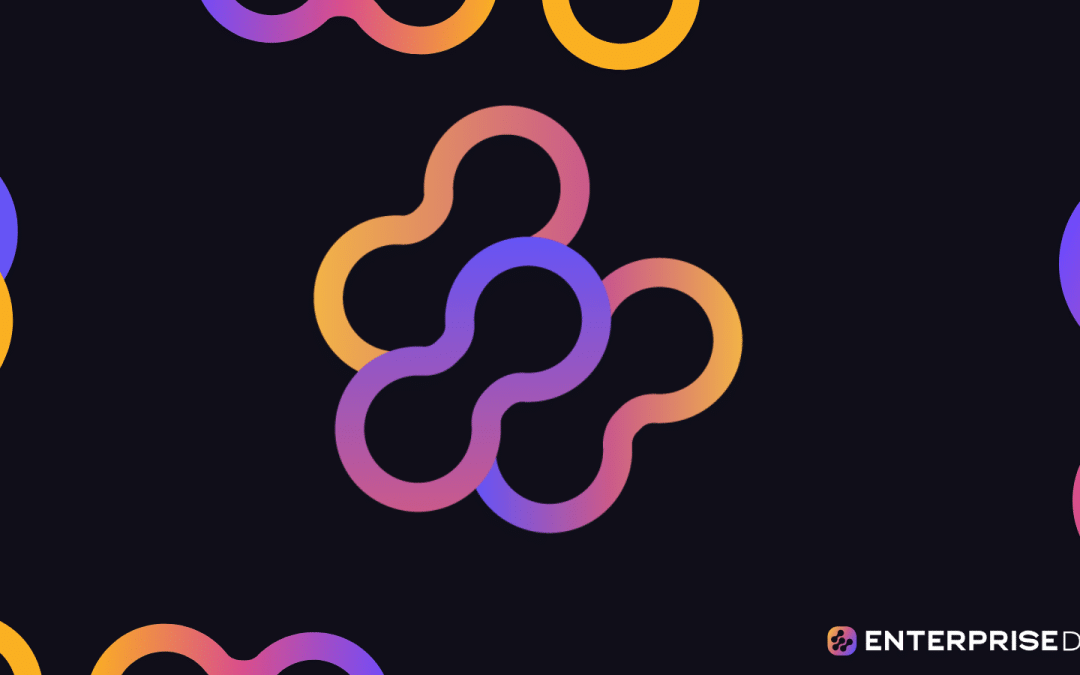
Beginner’s Guide to Choosing the Right Data Visualization
Data Analytics
As a beginner in data visualization, you’ll need to learn the various chart types to effectively...
Ultimate Guide to Mastering Color in Data Visualization
Color plays a vital role in the success of data visualization. When used effectively, it can help guide...
Simple To Use Best Practises For Data Visualization
So you’ve got a bunch of data and you want to make it look pretty. Or maybe you’ve heard about this...
Exploring The Benefits Of Geospatial Data Visualization Techniques
Data visualization has come a long way from simple bar charts and line graphs. As the volume and...
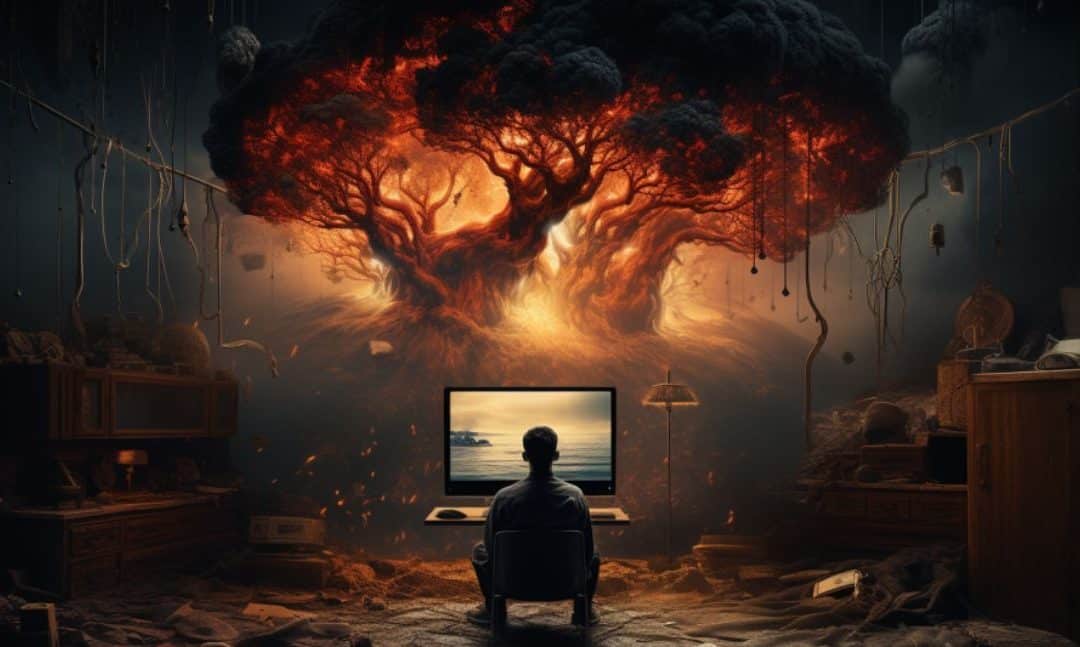
4 Types of Data Analytics: Explained
In a world full of data, data analytics is the heart and soul of an operation. It's what transforms raw...
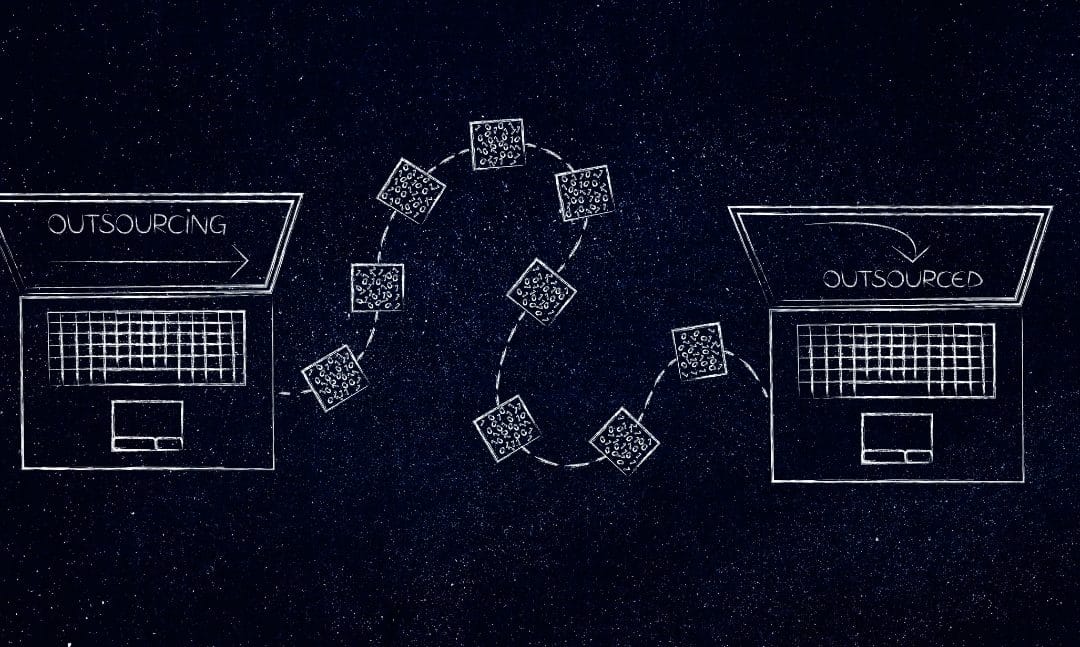
Data Analytics Outsourcing: Pros and Cons Explained
In today's data-driven world, businesses are constantly swimming in a sea of information, seeking the...
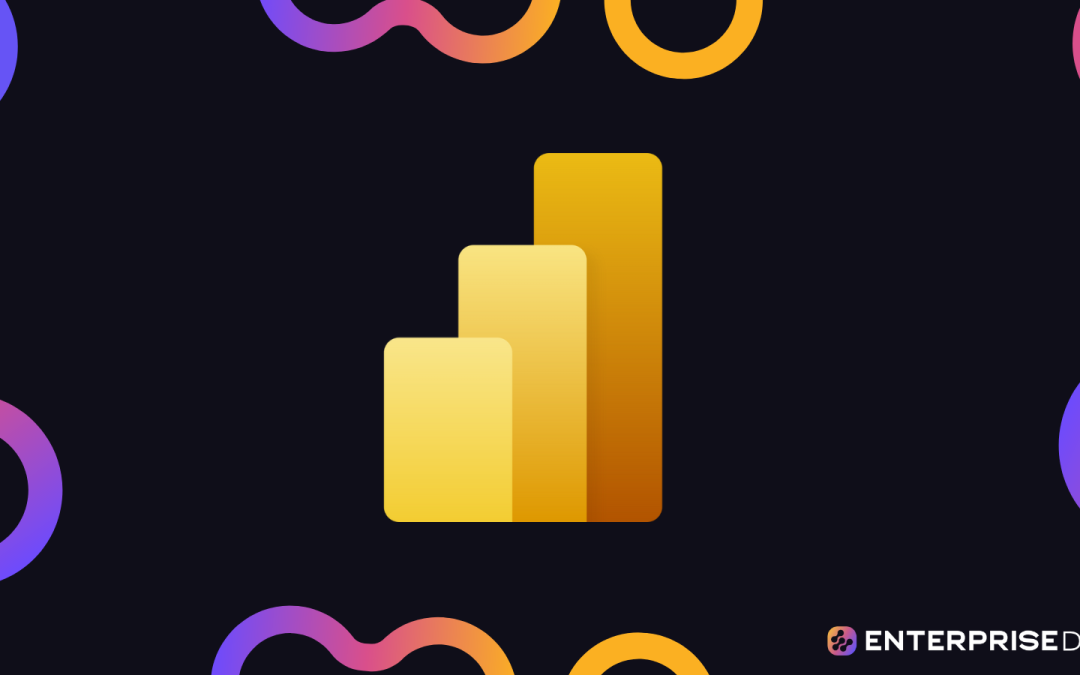
What Does a Data Analyst Do on a Daily Basis?
In the digital age, data plays a significant role in helping organizations make informed decisions and...
Removing A Hidden Date Table In Power BI To Improve Performance
In this tutorial, you’ll learn how to remove a hidden date table in Power BI to reduce RAM usage and...
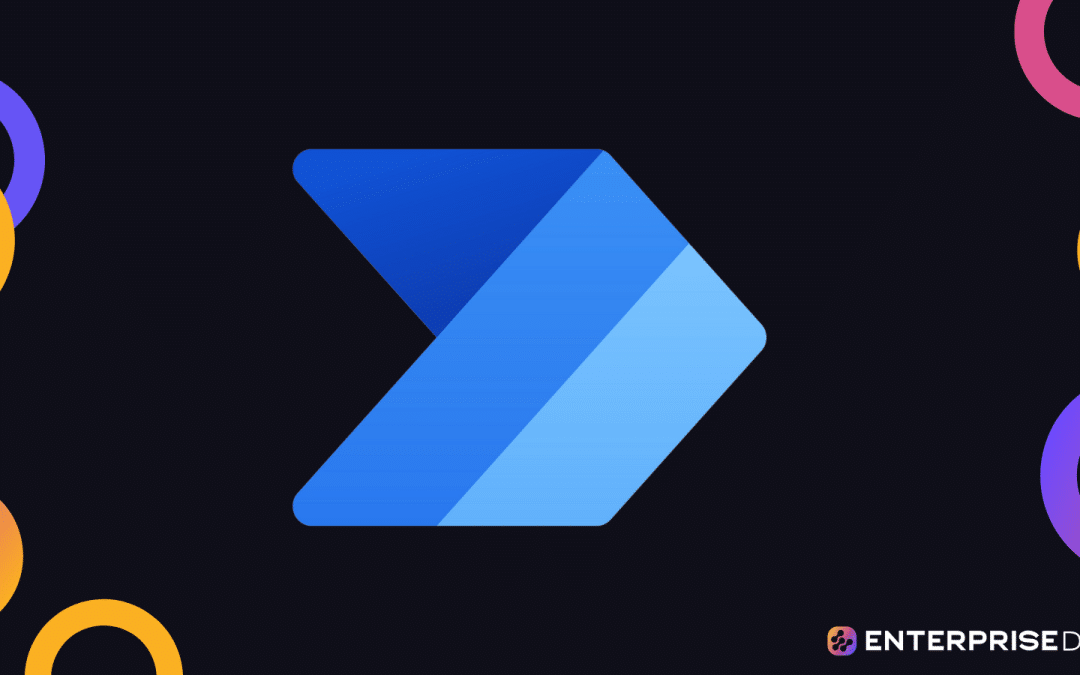
Power Automate Switch Control Action In Workflows
Power Automate
In this tutorial, we’ll talk about Power Automate Switch control, which is under the advanced built-in...
The Analyst Cloud Is Now Completely Free For Employers
We have recently made a decision at Enterprise DNA to make our Analyst Cloud platform now free for...
Report Layouts: Designing With Native Visuals
Designing report layouts in Power BI is a challenge for most people. Some data analysts sometimes...
Using The Offset Function In Extended Date Tables
I'm going to show you how to use the offset function in Power BI. It's one of the most dynamic tools...
Power BI Slicer Search: User Guide With Examples
Ready to get started with the Power BI slicer? This feature will allow you to filter and slice your...
How To Import Datasets Into Report Builder
In this tutorial, you’ll learn about the three different ways you can import datasets into Report...
Using Dynamic Management Views (DMV) In DAX Studio
In this tutorial, you’ll learn about Dynamic Management Views (DMV) in DAX Studio and how to use them...
Power Automate Environment: Setup And Overview
In this tutorial, we’ll learn how to set up an account and explore the features that the Power Automate...
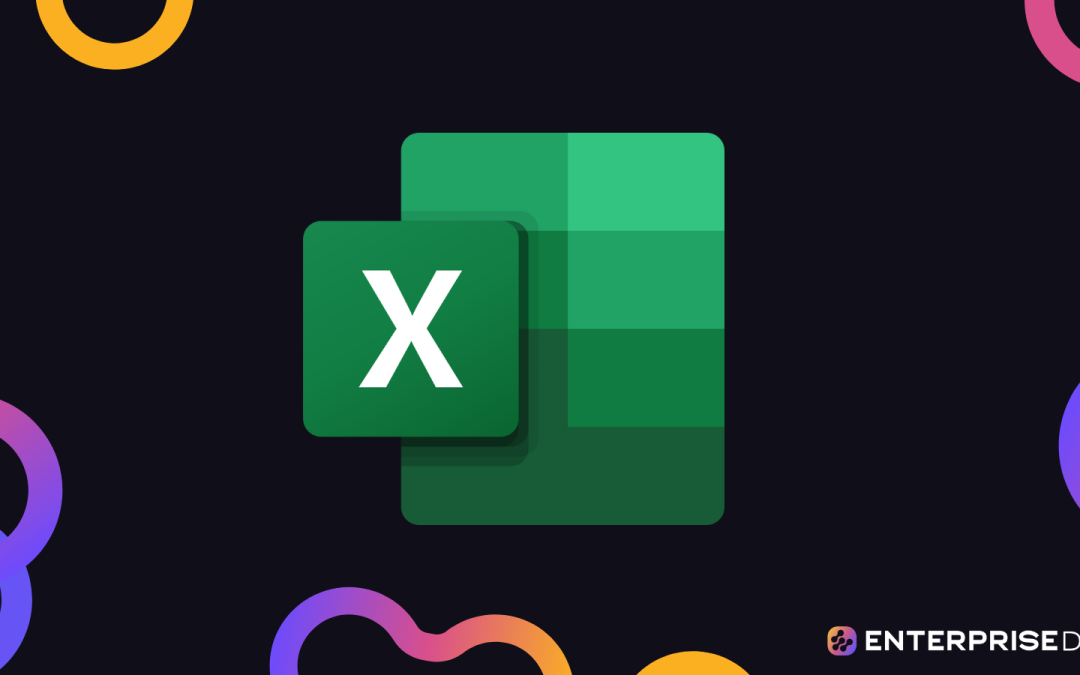
Can You Convert Excel to Word? 5 Easy Ways Explained
Looking to convert Excel to Word? You'll be thrilled to discover that you can, and it's easy to do! The...
Procurement Decisions Model And Analysis In Power BI
In this tutorial, we’re going to dive into some procurement analysis or purchasing decision-making...
Calculate Days Of Zero Stock – Power BI Inventory Management Insights
I'm going to go over a unique piece of analysis here concerning Power BI Inventory Management. You may...
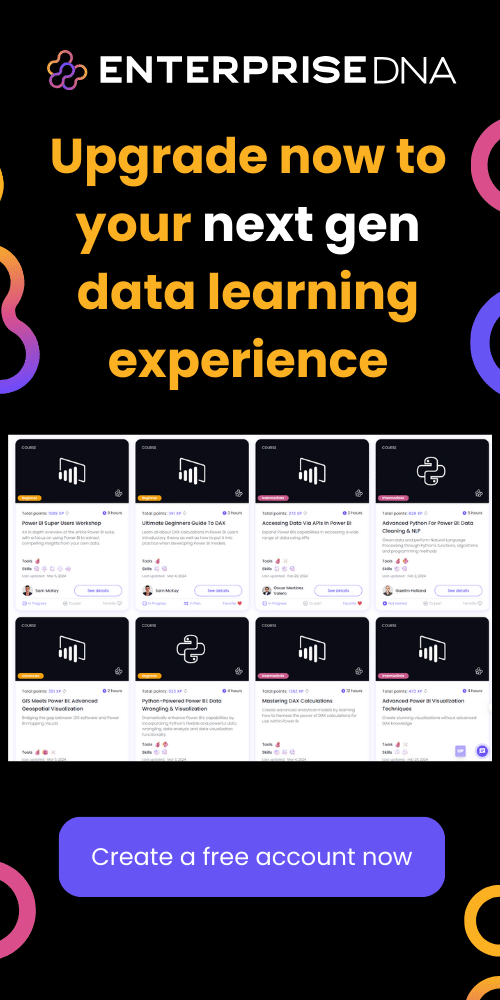
Register Now
Confirm Password *
Terms * By registering, you agree to the terms of service and Privacy Policy .
Lost Password
Lost your password? Please enter your email address. You will receive a link and will create a new password via email.
It will take less than 1 minute to register for lifetime. Bonus Tip - We don't send OTP to your email id Make Sure to use your own email id for free books and giveaways
The Data Monk
Case study interview questions for analytics – day 5, top categories.

Topic – Case Study Interview Questions How to solve case study in analytics interview? Solving a case study in an analytics interview requires a structured and analytical approach. Here are the steps you can follow to effectively solve a case study:
- Understand the Problem : Begin by carefully reading and understanding the case study prompt or problem statement. Pay attention to all the details provided, including any data sets, context, and specific questions to be answered.
- Clarify Questions : If anything is unclear or ambiguous, don’t hesitate to ask for clarification from the interviewer. It’s crucial to have a clear understanding of the problem before proceeding.
- Define Objectives : Clearly define the objectives of the case study. What is the problem you are trying to solve? What are the key questions you need to answer? Having a clear sense of purpose will guide your analysis.
- Gather Data : If the case study provides data, gather and organize it. This may involve cleaning and preprocessing the data, handling missing values, and converting it into a suitable format for analysis.
- Explore Data : Conduct exploratory data analysis (EDA) to gain insights into the data. This includes generating summary statistics, creating visualizations, and identifying patterns or trends. EDA helps you become familiar with the data and can suggest potential directions for analysis.
- Hypothesize and Plan : Based on your understanding of the problem and the data, formulate hypotheses or initial ideas about what might be driving the issues or opportunities in the case study. Develop a plan for your analysis, outlining the steps you will take to test your hypotheses.
- Conduct Analysis : Execute your analysis plan, which may involve statistical tests, machine learning algorithms, regression analysis, or any other relevant techniques. Ensure that your analysis aligns with the objectives of the case study.
- Interpret Results : Once you have conducted the analysis, interpret the results. Are your findings statistically significant? Do they answer the key questions posed in the case study? Use visualizations and clear explanations to support your conclusions.
- Make Recommendations : Based on your analysis and interpretation, provide actionable recommendations or solutions to the problem. Explain the rationale behind your recommendations and consider any potential implications.
- Communicate Effectively : Present your findings and recommendations in a clear and structured manner. Be prepared to explain your thought process and defend your conclusions during the interview. Effective communication is essential in analytics interviews.
- Consider Business Impact : Discuss the potential impact of your recommendations on the business. Think about how your solutions might be implemented and the expected outcomes.
- Ask Questions : At the end of your analysis, you may have an opportunity to ask questions or seek feedback from the interviewer. This shows your engagement and curiosity about the case study.
- Practice, Practice, Practice : Preparing for case studies in advance is crucial. Practice solving similar case studies on your own or with peers to build your problem-solving skills and analytical thinking.
Remember that in analytics interviews, interviewers are not only assessing your technical skills but also your ability to think critically, communicate effectively, and derive meaningful insights from data. Practice and a structured approach will help you excel in these interviews Case Study Interview Questions

Customer Segmentation Case Study
Customer Segmentation: You work for an e-commerce company. How would you use data analytics to segment your customers for targeted marketing campaigns? What variables or features would you consider, and what techniques would you apply to perform this segmentation effectively?
Segmenting customers for targeted marketing campaigns is a crucial task for any e-commerce company. Data analytics plays a pivotal role in this process. Here’s a step-by-step guide on how you can use data analytics to segment your customers effectively:
- Demographic information (age, gender, location)
- Purchase history (frequency, recency, monetary value)
- Website behavior (pages visited, time spent, products viewed)
- Interaction with marketing campaigns (click-through rates, open rates)
- Customer feedback and reviews
- Data Cleaning and Preprocessing : Clean and preprocess the data to ensure accuracy and consistency. Handle missing values, outliers, and inconsistencies in the data. Convert categorical variables into numerical representations using techniques like one-hot encoding or label encoding.
- Feature Engineering : Create new features or variables that could be valuable for segmentation. For example, you might calculate the average order value, customer lifetime value, or purchase frequency.
- RFM (Recency, Frequency, Monetary) scores for purchase behavior
- Demographic variables such as age, gender, and location
- Customer engagement metrics like click-through rates or time spent on the website
- Product category preferences
- K-Means Clustering : Groups customers into clusters based on similarities in selected variables.
- Hierarchical Clustering : Divides customers into a tree-like structure of clusters.
- DBSCAN : Identifies clusters of arbitrary shapes and densities.
- PCA (Principal Component Analysis) : Reduces dimensionality while preserving key information.
- Machine Learning Models : Utilize supervised or unsupervised machine learning algorithms to find patterns in the data.
- Segmentation and Interpretation : Apply the chosen segmentation technique to the data and segment your customer base. Interpret the results to understand the characteristics of each segment. Assign meaningful labels or names to the segments, such as “High-Value Shoppers” or “Casual Shoppers.”
- Validation and Testing : Evaluate the effectiveness of your segmentation by assessing how well it aligns with your business goals. Use metrics such as within-cluster variance, silhouette score, or business KPIs like revenue growth within each segment.
- Targeted Marketing Campaigns : Design marketing campaigns tailored to each customer segment. This could involve personalized product recommendations, email content, advertising channels, and messaging strategies that resonate with the characteristics and preferences of each segment.
- Monitoring and Iteration : Continuously monitor the performance of your marketing campaigns and customer segments. Refine your segments and marketing strategies as you gather more data and insights.
- Privacy and Compliance : Ensure that you handle customer data in compliance with privacy regulations, such as GDPR or CCPA, and prioritize data security throughout the process.
By effectively using data analytics to segment your customers, you can create more targeted and personalized marketing campaigns that are likely to yield better results and improve overall customer satisfaction.
A/B Testing Case Study
A social media platform wants to test a new feature to increase user engagement. Describe the steps you would take to design and analyze an A/B test to determine the impact of the new feature. What metrics would you track, and how would you interpret the results?
Designing and analyzing an A/B test for a new feature on a social media platform involves several critical steps. A well-executed A/B test can provide valuable insights into whether the new feature has a significant impact on user engagement. Here’s a step-by-step guide:
1. Define the Objective: Clearly define the objective of the A/B test. In this case, it’s to determine whether the new feature increases user engagement. Define what you mean by “user engagement” (e.g., increased time spent on the platform, higher interaction with posts, more shares, etc.).
2. Select the Test Group: Randomly select a representative sample of users from your platform. This will be your “test group.” Ensure that the sample size is statistically significant to detect meaningful differences.
3. Create Control and Test Groups: Divide the test group into two subgroups:
- Control Group (A): This group will not have access to the new feature.
- Test Group (B): This group will have access to the new feature.
4. Implement the Test: Implement the new feature for the Test Group while keeping the Control Group’s experience unchanged. Make sure that the user experience for both groups is consistent in all other aspects.
5. Measure Metrics: Define the metrics you will track to measure user engagement. Common metrics for social media platforms might include:
- Time spent on the platform
- Number of posts/comments/likes/shares
- User retention rate
- Click-through rate on recommended content
6. Collect Data: Run the A/B test for a predetermined period (e.g., one week or one month) to collect data on the selected metrics for both the Control and Test Groups.
7. Analyze the Results: Use statistical analysis to compare the metrics between the Control and Test Groups. Common techniques include:
- T-Tests : To compare means of continuous metrics like time spent on the platform.
- Chi-Square Tests : For categorical metrics like the number of shares.
- Cohort Analysis : To examine user behavior over time.
8. Interpret the Results: Interpret the results of the A/B test based on statistical significance and practical significance. Consider the following scenarios:
a. Statistically Significant Positive Results : If the new feature shows a statistically significant increase in user engagement, it may be a strong indicator that the feature positively impacts engagement.
b. Statistically Significant Negative Results : If the new feature shows a statistically significant decrease in user engagement, this suggests that the feature might have a negative impact, and you may need to reevaluate or iterate on the feature.
c. No Statistical Significance : If there’s no statistically significant difference between the Control and Test Groups, it’s inconclusive, and the new feature may not have a significant impact on user engagement.
9. Consider Secondary Metrics and User Feedback: Alongside primary metrics, consider secondary metrics and gather user feedback to gain a more comprehensive understanding of the new feature’s impact.
10. Make Informed Decisions: Based on the results, make informed decisions about whether to roll out the new feature to all users, iterate on the feature, or abandon it.
11. Monitor and Iterate: Continuously monitor user engagement metrics even after implementing the feature to ensure its long-term impact and make further improvements if necessary.
Remember that A/B testing is a powerful tool, but it’s important to ensure that your test design and statistical analysis are sound to draw accurate conclusions about the new feature’s impact on user engagement.
How The Data Monk can help you?
We have created products and services on different platforms to help you in your Analytics journey irrespective of whether you want to switch to a new job or want to move into Analytics. Our services
- YouTube channel covering all the interview-related important topics in SQL, Python, MS Excel, Machine Learning Algorithm, Statistics, and Direct Interview Questions Link – The Data Monk Youtube Channel
- Website – ~2000 completed solved Interview questions in SQL, Python, ML, and Case Study Link – The Data Monk website
- E-book shop – We have 70+ e-books available on our website and 3 bundles covering 2000+ solved interview questions Link – The Data E-shop Page
- Instagram Page – It covers only Most asked Questions and concepts (100+ posts) Link – The Data Monk Instagram page
- Mock Interviews Book a slot on Top Mate
- Career Guidance/Mentorship Book a slot on Top Mate
- Resume-making and review Book a slot on Top Mate
The Data Monk e-books
We know that each domain requires a different type of preparation, so we have divided our books in the same way:
✅ Data Analyst and Product Analyst -> 1100+ Most Asked Interview Questions
✅ Business Analyst -> 1250+ Most Asked Interview Questions
✅ Data Scientist and Machine Learning Engineer -> 23 e-books covering all the ML Algorithms Interview Questions
✅ Full Stack Analytics Professional – 2200 Most Asked Interview Questions
The Data Monk – 30 Days Mentorship program
We are a group of 30+ people with ~8 years of Analytics experience in product-based companies. We take interviews on a daily basis for our organization and we very well know what is asked in the interviews. Other skill enhancer website charge 2lakh+ GST for courses ranging from 10 to 15 months. We only focus on making you a clear interview with ease. We have released our Become a Full Stack Analytics Professional for anyone in 2nd year of graduation to 8-10 YOE. This book contains 23 topics and each topic is divided into 50/100/200/250 questions and answers. Pick the book and read it thrice, learn it, and appear in the interview. We also have a complete Analytics interview package – 2200 questions ebook (Rs.1999) + 23 ebook bundle for Data Science and Analyst role (Rs.1999) – 4 one-hour mock interviews, every Saturday (top mate – Rs.1000 per interview) – 4 career guidance sessions, 30 mins each on every Sunday (top mate – Rs.500 per session) – Resume review and improvement (Top mate – Rs.500 per review)
Total cost – Rs.10500 Discounted price – Rs. 9000 How to avail of this offer? Send a mail to [email protected]

About TheDataMonk Grand Master
Related posts, adobe analytics interview questions – sql, statistics complete tutorial – 7 days analytics course, case study for analytics interviews – 7 days analytics, pandas complete tutorial – 7 days analytics, python complete tutorial – 7 days analytics.
Previous post
Next post
Subscribe to our newsletter
Currently taking bookings for July >>
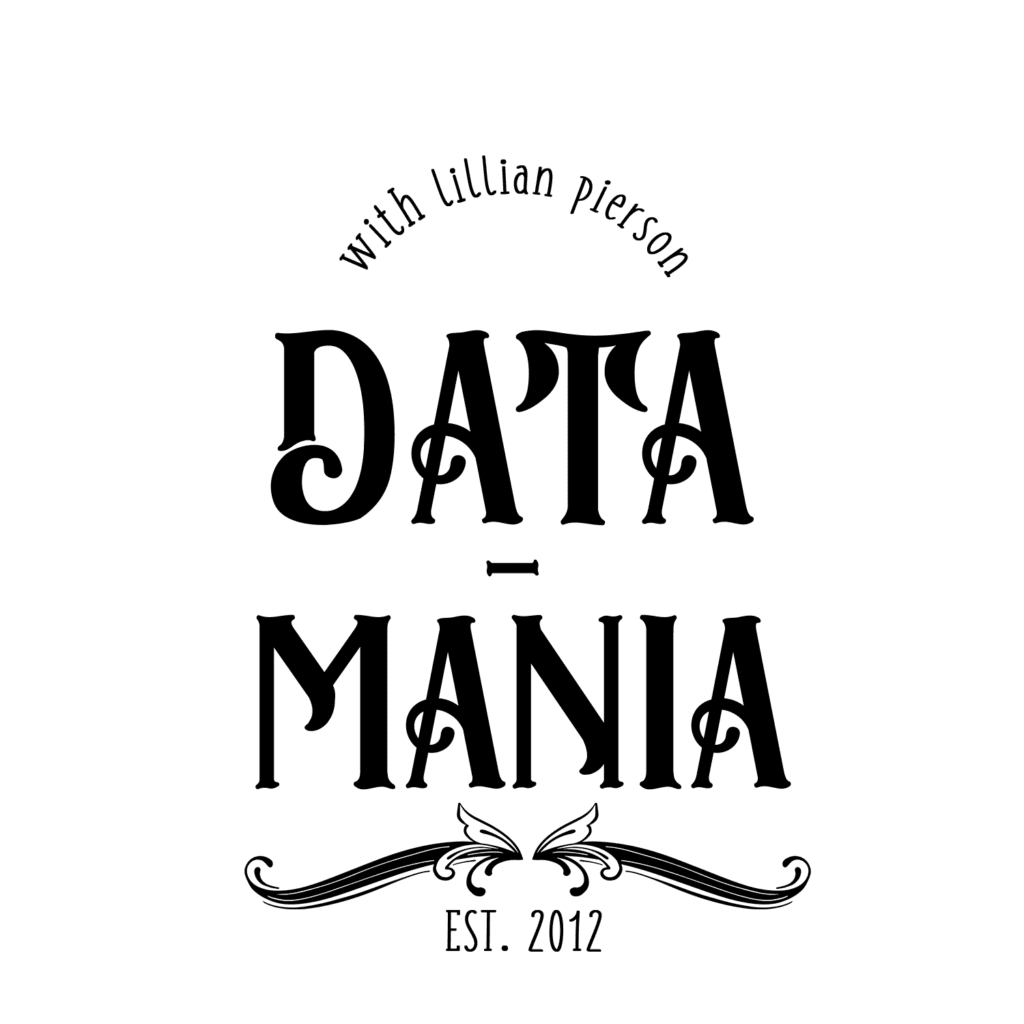
Data Analysis Case Study: Learn From Humana’s Automated Data Analysis Project
Lillian Pierson, P.E.
Playback speed:
Got data? Great! Looking for that perfect data analysis case study to help you get started using it? You’re in the right place.
If you’ve ever struggled to decide what to do next with your data projects, to actually find meaning in the data, or even to decide what kind of data to collect, then KEEP READING…
Deep down, you know what needs to happen. You need to initiate and execute a data strategy that really moves the needle for your organization. One that produces seriously awesome business results.
But how you’re in the right place to find out..
As a data strategist who has worked with 10 percent of Fortune 100 companies, today I’m sharing with you a case study that demonstrates just how real businesses are making real wins with data analysis.
In the post below, we’ll look at:
- A shining data success story;
- What went on ‘under-the-hood’ to support that successful data project; and
- The exact data technologies used by the vendor, to take this project from pure strategy to pure success
If you prefer to watch this information rather than read it, it’s captured in the video below:
Here’s the url too: https://youtu.be/xMwZObIqvLQ
3 Action Items You Need To Take
To actually use the data analysis case study you’re about to get – you need to take 3 main steps. Those are:
- Reflect upon your organization as it is today (I left you some prompts below – to help you get started)
- Review winning data case collections (starting with the one I’m sharing here) and identify 5 that seem the most promising for your organization given it’s current set-up
- Assess your organization AND those 5 winning case collections. Based on that assessment, select the “QUICK WIN” data use case that offers your organization the most bang for it’s buck
Step 1: Reflect Upon Your Organization
Whenever you evaluate data case collections to decide if they’re a good fit for your organization, the first thing you need to do is organize your thoughts with respect to your organization as it is today.
Before moving into the data analysis case study, STOP and ANSWER THE FOLLOWING QUESTIONS – just to remind yourself:
- What is the business vision for our organization?
- What industries do we primarily support?
- What data technologies do we already have up and running, that we could use to generate even more value?
- What team members do we have to support a new data project? And what are their data skillsets like?
- What type of data are we mostly looking to generate value from? Structured? Semi-Structured? Un-structured? Real-time data? Huge data sets? What are our data resources like?
Jot down some notes while you’re here. Then keep them in mind as you read on to find out how one company, Humana, used its data to achieve a 28 percent increase in customer satisfaction. Also include its 63 percent increase in employee engagement! (That’s such a seriously impressive outcome, right?!)
Step 2: Review Data Case Studies
Here we are, already at step 2. It’s time for you to start reviewing data analysis case studies (starting with the one I’m sharing below). I dentify 5 that seem the most promising for your organization given its current set-up.
Humana’s Automated Data Analysis Case Study
The key thing to note here is that the approach to creating a successful data program varies from industry to industry .
Let’s start with one to demonstrate the kind of value you can glean from these kinds of success stories.
Humana has provided health insurance to Americans for over 50 years. It is a service company focused on fulfilling the needs of its customers. A great deal of Humana’s success as a company rides on customer satisfaction, and the frontline of that battle for customers’ hearts and minds is Humana’s customer service center.
Call centers are hard to get right. A lot of emotions can arise during a customer service call, especially one relating to health and health insurance. Sometimes people are frustrated. At times, they’re upset. Also, there are times the customer service representative becomes aggravated, and the overall tone and progression of the phone call goes downhill. This is of course very bad for customer satisfaction.
Humana wanted to use artificial intelligence to improve customer satisfaction (and thus, customer retention rates & profits per customer).
Humana wanted to find a way to use artificial intelligence to monitor their phone calls and help their agents do a better job connecting with their customers in order to improve customer satisfaction (and thus, customer retention rates & profits per customer ).
In light of their business need, Humana worked with a company called Cogito, which specializes in voice analytics technology.
Cogito offers a piece of AI technology called Cogito Dialogue. It’s been trained to identify certain conversational cues as a way of helping call center representatives and supervisors stay actively engaged in a call with a customer.
The AI listens to cues like the customer’s voice pitch.
If it’s rising, or if the call representative and the customer talk over each other, then the dialogue tool will send out electronic alerts to the agent during the call.
Humana fed the dialogue tool customer service data from 10,000 calls and allowed it to analyze cues such as keywords, interruptions, and pauses, and these cues were then linked with specific outcomes. For example, if the representative is receiving a particular type of cues, they are likely to get a specific customer satisfaction result.
The Outcome
Customers were happier, and customer service representatives were more engaged..
This automated solution for data analysis has now been deployed in 200 Humana call centers and the company plans to roll it out to 100 percent of its centers in the future.
The initiative was so successful, Humana has been able to focus on next steps in its data program. The company now plans to begin predicting the type of calls that are likely to go unresolved, so they can send those calls over to management before they become frustrating to the customer and customer service representative alike.
What does this mean for you and your business?
Well, if you’re looking for new ways to generate value by improving the quantity and quality of the decision support that you’re providing to your customer service personnel, then this may be a perfect example of how you can do so.
Humana’s Business Use Cases
Humana’s data analysis case study includes two key business use cases:
- Analyzing customer sentiment; and
- Suggesting actions to customer service representatives.
Analyzing Customer Sentiment
First things first, before you go ahead and collect data, you need to ask yourself who and what is involved in making things happen within the business.
In the case of Humana, the actors were:
- The health insurance system itself
- The customer, and
- The customer service representative
As you can see in the use case diagram above, the relational aspect is pretty simple. You have a customer service representative and a customer. They are both producing audio data, and that audio data is being fed into the system.
Humana focused on collecting the key data points, shown in the image below, from their customer service operations.
By collecting data about speech style, pitch, silence, stress in customers’ voices, length of call, speed of customers’ speech, intonation, articulation, silence, and representatives’ manner of speaking, Humana was able to analyze customer sentiment and introduce techniques for improved customer satisfaction.
Having strategically defined these data points, the Cogito technology was able to generate reports about customer sentiment during the calls.
Suggesting actions to customer service representatives.
The second use case for the Humana data program follows on from the data gathered in the first case.
In Humana’s case, Cogito generated a host of call analyses and reports about key call issues.
In the second business use case, Cogito was able to suggest actions to customer service representatives, in real-time , to make use of incoming data and help improve customer satisfaction on the spot.
The technology Humana used provided suggestions via text message to the customer service representative, offering the following types of feedback:
- The tone of voice is too tense
- The speed of speaking is high
- The customer representative and customer are speaking at the same time
These alerts allowed the Humana customer service representatives to alter their approach immediately , improving the quality of the interaction and, subsequently, the customer satisfaction.
The preconditions for success in this use case were:
- The call-related data must be collected and stored
- The AI models must be in place to generate analysis on the data points that are recorded during the calls
Evidence of success can subsequently be found in a system that offers real-time suggestions for courses of action that the customer service representative can take to improve customer satisfaction.
Thanks to this data-intensive business use case, Humana was able to increase customer satisfaction, improve customer retention rates, and drive profits per customer.
The Technology That Supports This Data Analysis Case Study
I promised to dip into the tech side of things. This is especially for those of you who are interested in the ins and outs of how projects like this one are actually rolled out.
Here’s a little rundown of the main technologies we discovered when we investigated how Cogito runs in support of its clients like Humana.
- For cloud data management Cogito uses AWS, specifically the Athena product
- For on-premise big data management, the company used Apache HDFS – the distributed file system for storing big data
- They utilize MapReduce, for processing their data
- And Cogito also has traditional systems and relational database management systems such as PostgreSQL
- In terms of analytics and data visualization tools, Cogito makes use of Tableau
- And for its machine learning technology, these use cases required people with knowledge in Python, R, and SQL, as well as deep learning (Cogito uses the PyTorch library and the TensorFlow library)
These data science skill sets support the effective computing, deep learning , and natural language processing applications employed by Humana for this use case.
If you’re looking to hire people to help with your own data initiative, then people with those skills listed above, and with experience in these specific technologies, would be a huge help.
Step 3: S elect The “Quick Win” Data Use Case
Still there? Great!
It’s time to close the loop.
Remember those notes you took before you reviewed the study? I want you to STOP here and assess. Does this Humana case study seem applicable and promising as a solution, given your organization’s current set-up…
YES ▶ Excellent!
Earmark it and continue exploring other winning data use cases until you’ve identified 5 that seem like great fits for your businesses needs. Evaluate those against your organization’s needs, and select the very best fit to be your “quick win” data use case. Develop your data strategy around that.
NO , Lillian – It’s not applicable. ▶ No problem.
Discard the information and continue exploring the winning data use cases we’ve categorized for you according to business function and industry. Save time by dialing down into the business function you know your business really needs help with now. Identify 5 winning data use cases that seem like great fits for your businesses needs. Evaluate those against your organization’s needs, and select the very best fit to be your “quick win” data use case. Develop your data strategy around that data use case.
More resources to get ahead...
Get income-generating ideas for data professionals, are you tired of relying on one employer for your income are you dreaming of a side hustle that won’t put you at risk of getting fired or sued well, my friend, you’re in luck..
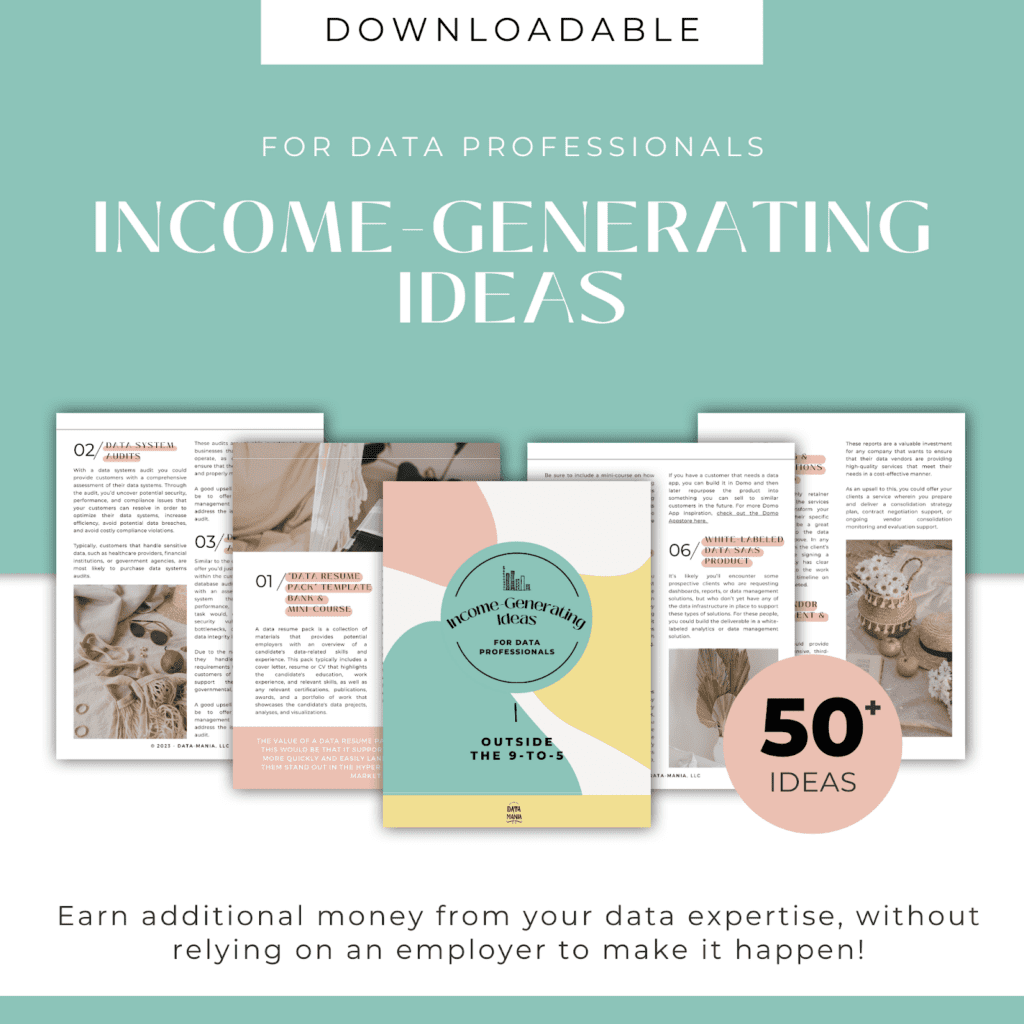
This 48-page listing is here to rescue you from the drudgery of corporate slavery and set you on the path to start earning more money from your existing data expertise. Spend just 1 hour with this pdf and I can guarantee you’ll be bursting at the seams with practical, proven & profitable ideas for new income-streams you can create from your existing expertise. Learn more here!
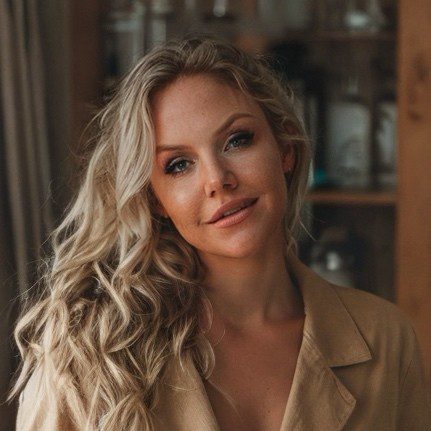
Apply To Work Together
Get featured, join the convergence newsletter.
Our newsletter is exclusively written for operators in the data & AI industry. Hi, I'm Lillian Pierson, Data-Mania's founder. We welcome you to our little corner of the internet. Data-Mania offers fractional CMO and marketing consulting services to deep tech B2B businesses. The Convergence community is sponsored by Data-Mania, as a tribute to the data community from which we sprung. You are welcome anytime.
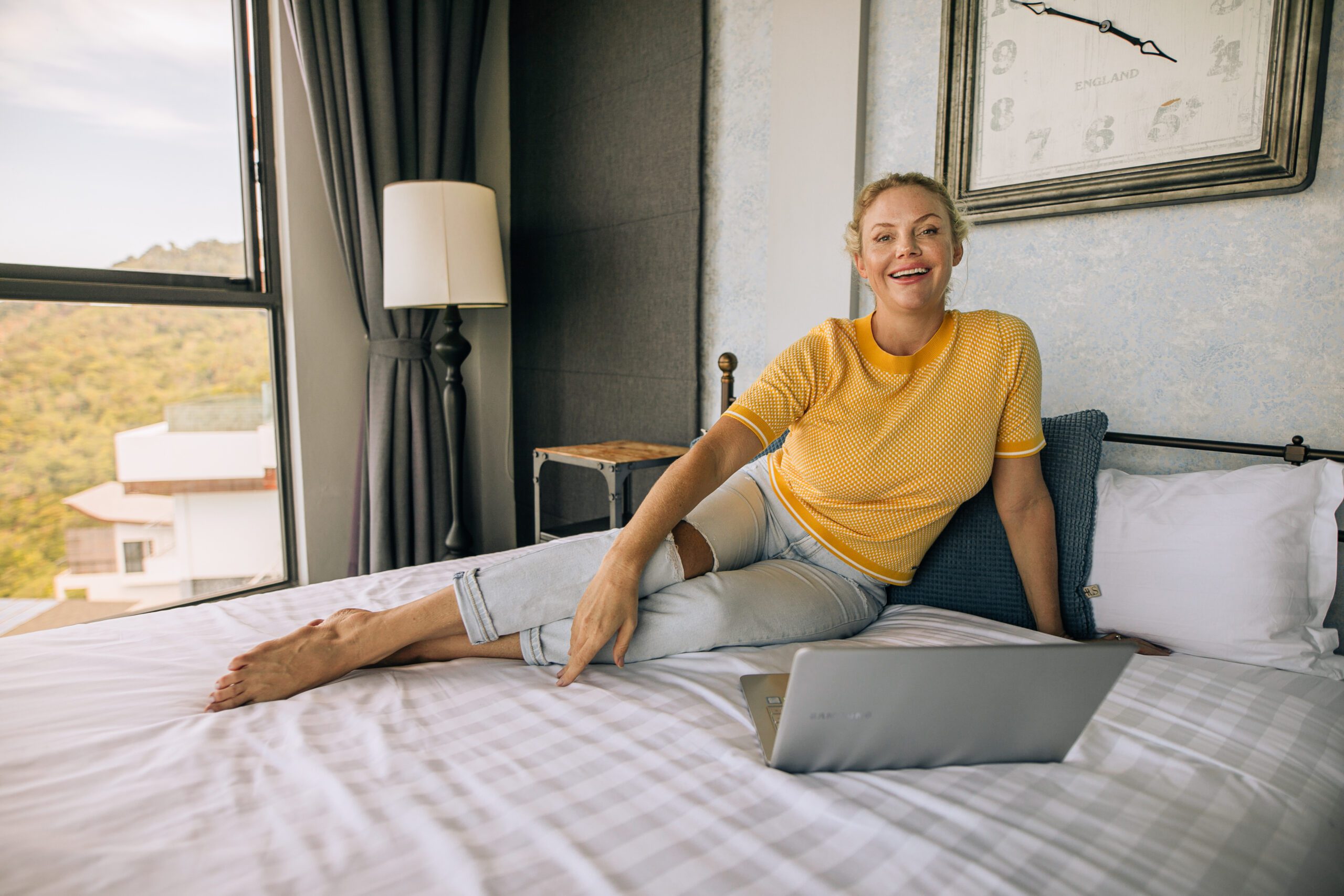
Get more actionable advice by joining The Convergence Newsletter for free below.
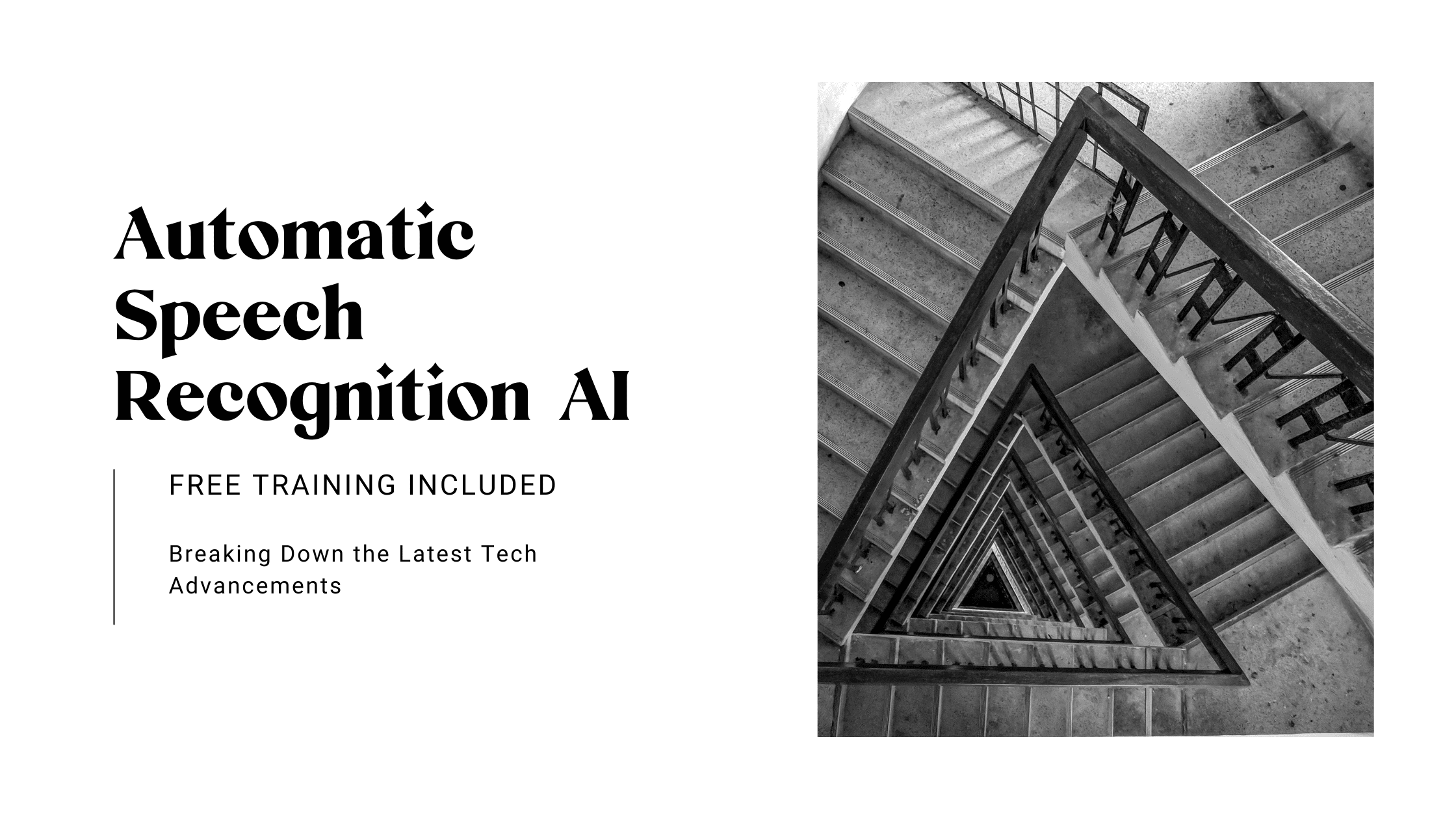
Automatic Speech Recognition AI: Breaking Down the Latest Tech Advancements [Free Training Included]
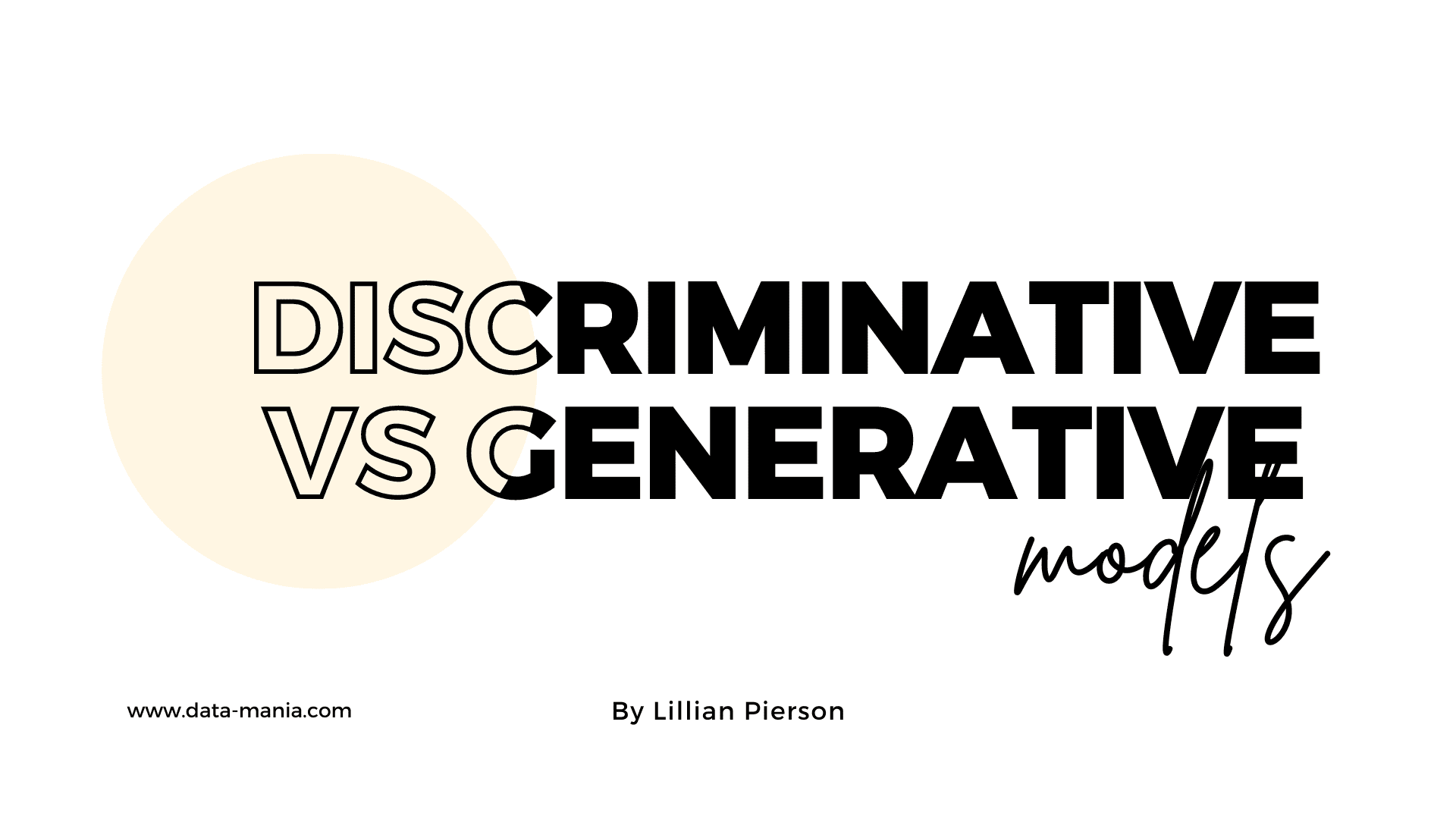
Choosing Between Discriminative vs Generative Models
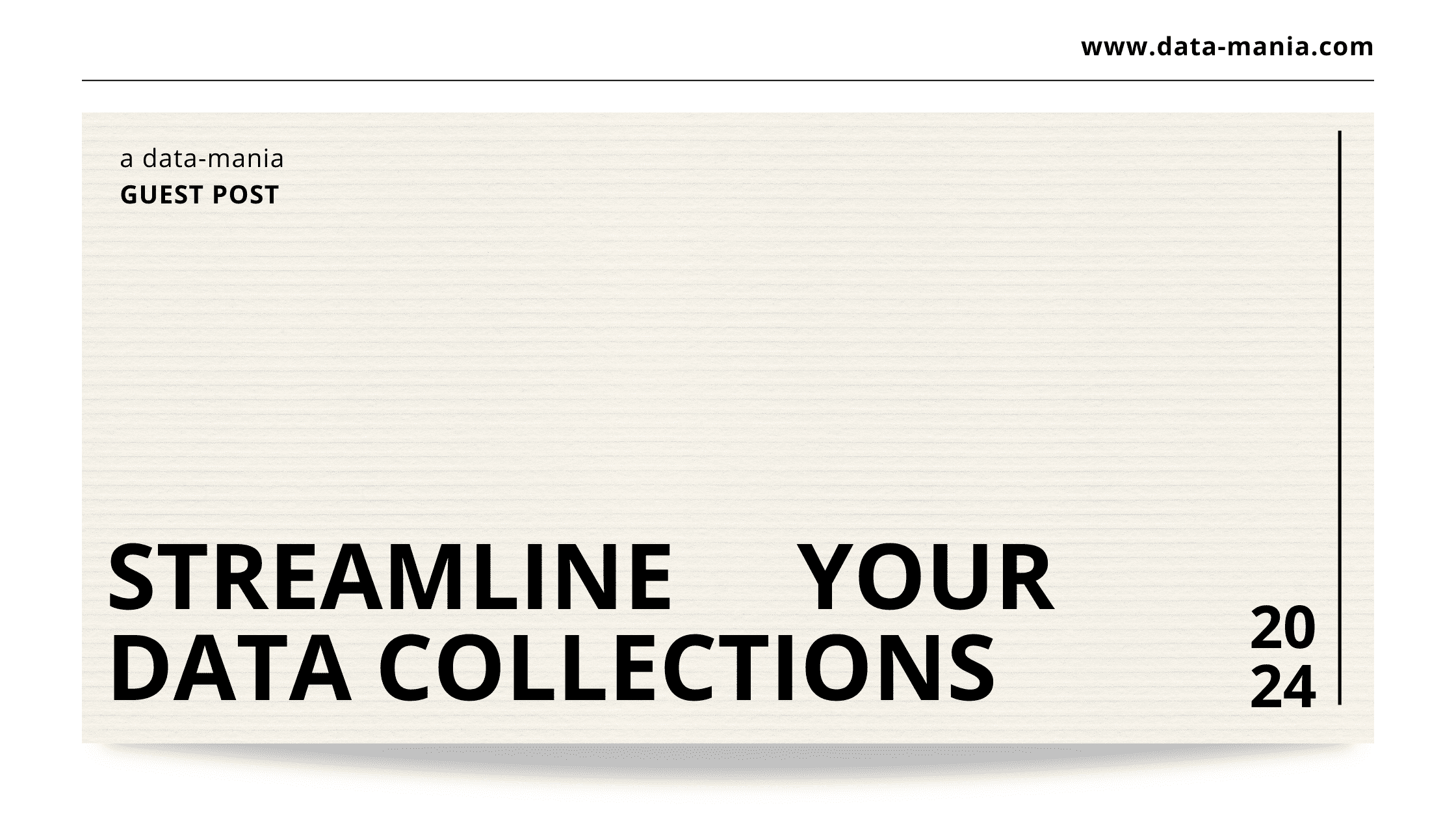
5 Ways AI Helps Streamline Data Collection
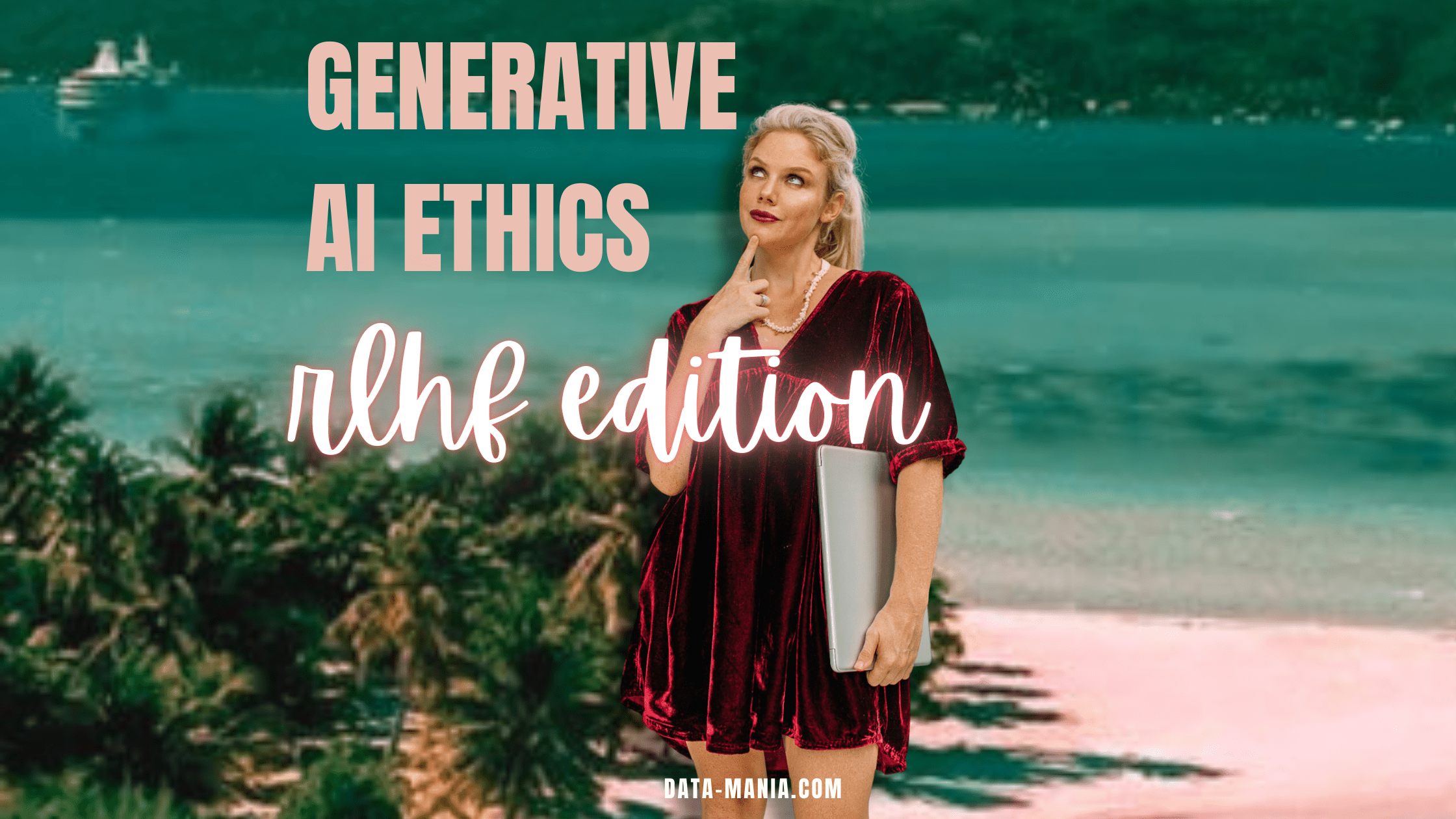
Ugly Generative AI Ethics Concerns: RLHF Edition
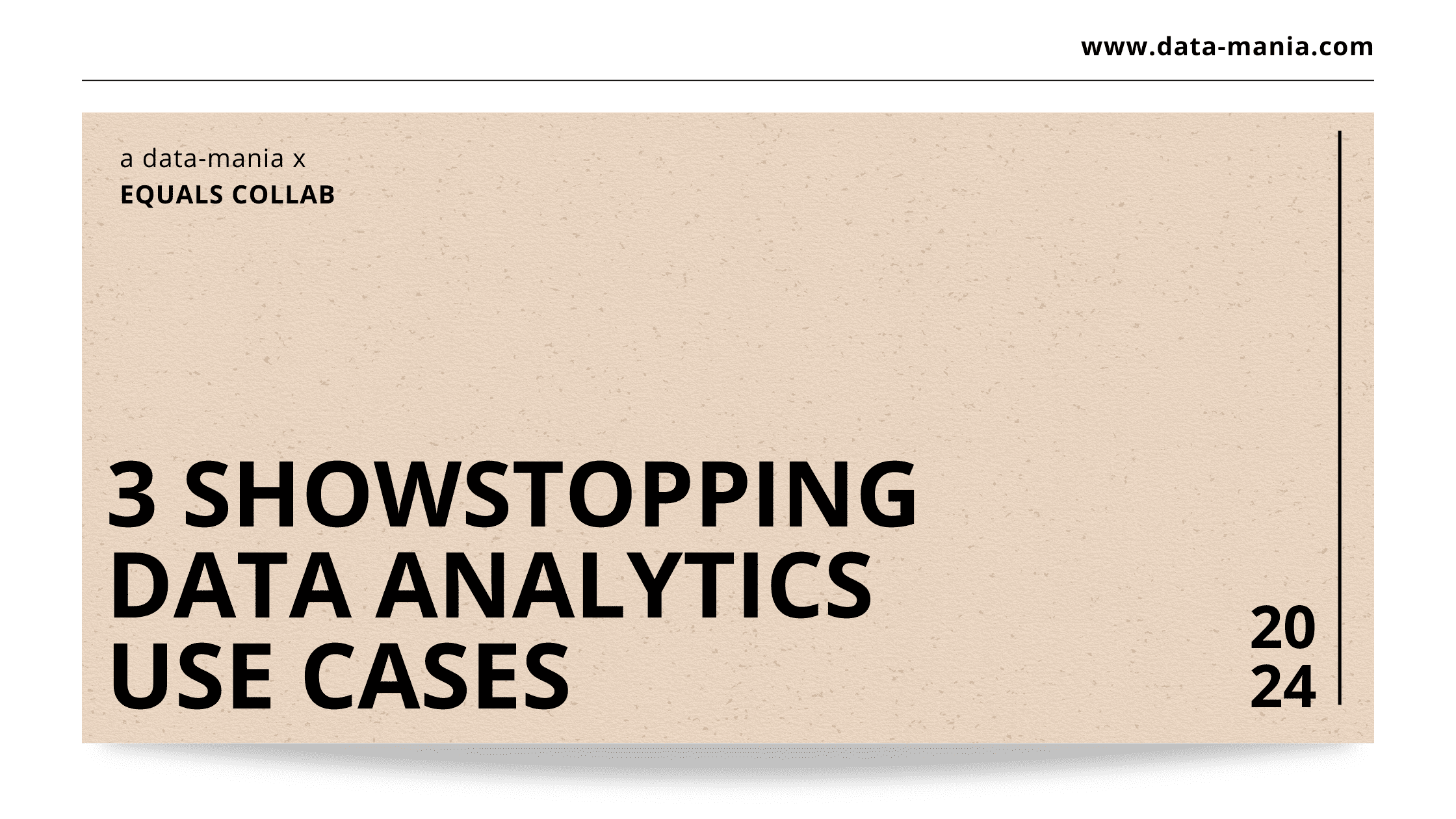
3 Showstopping Data Analytics Use Cases To Uplevel Your Startup Profit-Margins
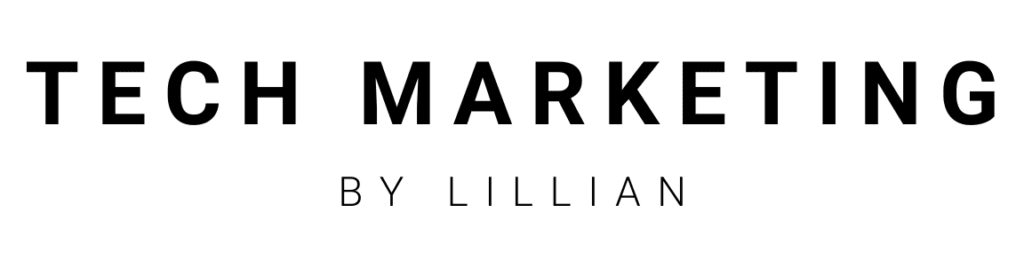
Fractional CMO for deep tech B2B businesses. Specializing in go-to-market strategy, SaaS product growth, and consulting revenue growth. American expat serving clients worldwide since 2012.
Get connected, © data-mania, 2012 - 2024+, all rights reserved - terms & conditions - privacy policy | products protected by copyscape, privacy overview.
Get The Newsletter
Top 20 Analytics Case Studies in 2024

Cem is the principal analyst at AIMultiple since 2017. AIMultiple informs hundreds of thousands of businesses (as per Similarweb) including 60% of Fortune 500 every month.
Cem's work focuses on how enterprises can leverage new technologies in AI, automation, cybersecurity(including network security, application security), data collection including web data collection and process intelligence.
Although the potential of Big Data and business intelligence are recognized by organizations, Gartner analyst Nick Heudecker says that the failure rate of analytics projects is close to 85%. Uncovering the power of analytics improves business operations, reduces costs, enhances decision-making , and enables the launching of more personalized products.
In this article, our research covers:
How to measure analytics success?
What are some analytics case studies.
According to Gartner CDO Survey, the top 3 critical success factors of analytics projects are:
- Creation of a data-driven culture within the organization,
- Data integration and data skills training across the organization,
- And implementation of a data management and analytics strategy.
The success of the process of analytics depends on asking the right question. It requires an understanding of the appropriate data required for each goal to be achieved. We’ve listed 20 successful analytics applications/case studies from different industries.
During our research, we examined that partnering with an analytics consultant helps organizations boost their success if organizations’ tech team lacks certain data skills.
*Vendors have not shared the client name
For more on analytics
If your organization is willing to implement an analytics solution but doesn’t know where to start, here are some of the articles we’ve written before that can help you learn more:
- AI in analytics: How AI is shaping analytics
- Edge Analytics in 2022: What it is, Why it matters & Use Cases
- Application Analytics: Tracking KPIs that lead to success
Finally, if you believe that your business would benefit from adopting an analytics solution, we have data-driven lists of vendors on our analytics hub and analytics platforms
We will help you choose the best solution tailored to your needs:

Cem's work has been cited by leading global publications including Business Insider, Forbes, Washington Post, global firms like Deloitte, HPE, NGOs like World Economic Forum and supranational organizations like European Commission. You can see more reputable companies and media that referenced AIMultiple.
Cem's hands-on enterprise software experience contributes to the insights that he generates. He oversees AIMultiple benchmarks in dynamic application security testing (DAST), data loss prevention (DLP), email marketing and web data collection. Other AIMultiple industry analysts and tech team support Cem in designing, running and evaluating benchmarks.
Throughout his career, Cem served as a tech consultant, tech buyer and tech entrepreneur. He advised enterprises on their technology decisions at McKinsey & Company and Altman Solon for more than a decade. He also published a McKinsey report on digitalization.
He led technology strategy and procurement of a telco while reporting to the CEO. He has also led commercial growth of deep tech company Hypatos that reached a 7 digit annual recurring revenue and a 9 digit valuation from 0 within 2 years. Cem's work in Hypatos was covered by leading technology publications like TechCrunch and Business Insider.
Cem regularly speaks at international technology conferences. He graduated from Bogazici University as a computer engineer and holds an MBA from Columbia Business School.
AIMultiple.com Traffic Analytics, Ranking & Audience , Similarweb. Why Microsoft, IBM, and Google Are Ramping up Efforts on AI Ethics , Business Insider. Microsoft invests $1 billion in OpenAI to pursue artificial intelligence that’s smarter than we are , Washington Post. Data management barriers to AI success , Deloitte. Empowering AI Leadership: AI C-Suite Toolkit , World Economic Forum. Science, Research and Innovation Performance of the EU , European Commission. Public-sector digitization: The trillion-dollar challenge , McKinsey & Company. Hypatos gets $11.8M for a deep learning approach to document processing , TechCrunch. We got an exclusive look at the pitch deck AI startup Hypatos used to raise $11 million , Business Insider.
To stay up-to-date on B2B tech & accelerate your enterprise:
Next to Read
14 case studies of manufacturing analytics in 2024, what is data virtualization benefits, case studies & top tools [2024], iot analytics: benefits, challenges, use cases & vendors [2024].
Your email address will not be published. All fields are required.
Related research
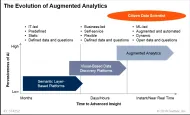
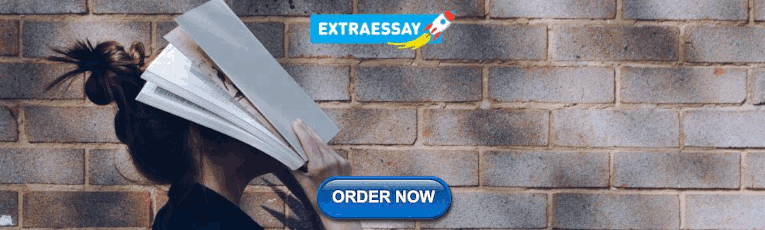
How Do Businesses Democratize Analytics With AI in 2024?
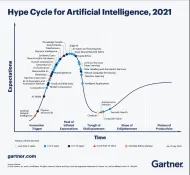
Exploring Analytics & AI in 2024: A Detailed Primer
Qualitative case study data analysis: an example from practice
Affiliation.
- 1 School of Nursing and Midwifery, National University of Ireland, Galway, Republic of Ireland.
- PMID: 25976531
- DOI: 10.7748/nr.22.5.8.e1307
Aim: To illustrate an approach to data analysis in qualitative case study methodology.
Background: There is often little detail in case study research about how data were analysed. However, it is important that comprehensive analysis procedures are used because there are often large sets of data from multiple sources of evidence. Furthermore, the ability to describe in detail how the analysis was conducted ensures rigour in reporting qualitative research.
Data sources: The research example used is a multiple case study that explored the role of the clinical skills laboratory in preparing students for the real world of practice. Data analysis was conducted using a framework guided by the four stages of analysis outlined by Morse ( 1994 ): comprehending, synthesising, theorising and recontextualising. The specific strategies for analysis in these stages centred on the work of Miles and Huberman ( 1994 ), which has been successfully used in case study research. The data were managed using NVivo software.
Review methods: Literature examining qualitative data analysis was reviewed and strategies illustrated by the case study example provided. Discussion Each stage of the analysis framework is described with illustration from the research example for the purpose of highlighting the benefits of a systematic approach to handling large data sets from multiple sources.
Conclusion: By providing an example of how each stage of the analysis was conducted, it is hoped that researchers will be able to consider the benefits of such an approach to their own case study analysis.
Implications for research/practice: This paper illustrates specific strategies that can be employed when conducting data analysis in case study research and other qualitative research designs.
Keywords: Case study data analysis; case study research methodology; clinical skills research; qualitative case study methodology; qualitative data analysis; qualitative research.
- Case-Control Studies*
- Data Interpretation, Statistical*
- Nursing Research / methods*
- Qualitative Research*
- Research Design
- Privacy Policy

Home » Case Study – Methods, Examples and Guide
Case Study – Methods, Examples and Guide
Table of Contents

A case study is a research method that involves an in-depth examination and analysis of a particular phenomenon or case, such as an individual, organization, community, event, or situation.
It is a qualitative research approach that aims to provide a detailed and comprehensive understanding of the case being studied. Case studies typically involve multiple sources of data, including interviews, observations, documents, and artifacts, which are analyzed using various techniques, such as content analysis, thematic analysis, and grounded theory. The findings of a case study are often used to develop theories, inform policy or practice, or generate new research questions.
Types of Case Study
Types and Methods of Case Study are as follows:
Single-Case Study
A single-case study is an in-depth analysis of a single case. This type of case study is useful when the researcher wants to understand a specific phenomenon in detail.
For Example , A researcher might conduct a single-case study on a particular individual to understand their experiences with a particular health condition or a specific organization to explore their management practices. The researcher collects data from multiple sources, such as interviews, observations, and documents, and uses various techniques to analyze the data, such as content analysis or thematic analysis. The findings of a single-case study are often used to generate new research questions, develop theories, or inform policy or practice.
Multiple-Case Study
A multiple-case study involves the analysis of several cases that are similar in nature. This type of case study is useful when the researcher wants to identify similarities and differences between the cases.
For Example, a researcher might conduct a multiple-case study on several companies to explore the factors that contribute to their success or failure. The researcher collects data from each case, compares and contrasts the findings, and uses various techniques to analyze the data, such as comparative analysis or pattern-matching. The findings of a multiple-case study can be used to develop theories, inform policy or practice, or generate new research questions.
Exploratory Case Study
An exploratory case study is used to explore a new or understudied phenomenon. This type of case study is useful when the researcher wants to generate hypotheses or theories about the phenomenon.
For Example, a researcher might conduct an exploratory case study on a new technology to understand its potential impact on society. The researcher collects data from multiple sources, such as interviews, observations, and documents, and uses various techniques to analyze the data, such as grounded theory or content analysis. The findings of an exploratory case study can be used to generate new research questions, develop theories, or inform policy or practice.
Descriptive Case Study
A descriptive case study is used to describe a particular phenomenon in detail. This type of case study is useful when the researcher wants to provide a comprehensive account of the phenomenon.
For Example, a researcher might conduct a descriptive case study on a particular community to understand its social and economic characteristics. The researcher collects data from multiple sources, such as interviews, observations, and documents, and uses various techniques to analyze the data, such as content analysis or thematic analysis. The findings of a descriptive case study can be used to inform policy or practice or generate new research questions.
Instrumental Case Study
An instrumental case study is used to understand a particular phenomenon that is instrumental in achieving a particular goal. This type of case study is useful when the researcher wants to understand the role of the phenomenon in achieving the goal.
For Example, a researcher might conduct an instrumental case study on a particular policy to understand its impact on achieving a particular goal, such as reducing poverty. The researcher collects data from multiple sources, such as interviews, observations, and documents, and uses various techniques to analyze the data, such as content analysis or thematic analysis. The findings of an instrumental case study can be used to inform policy or practice or generate new research questions.
Case Study Data Collection Methods
Here are some common data collection methods for case studies:
Interviews involve asking questions to individuals who have knowledge or experience relevant to the case study. Interviews can be structured (where the same questions are asked to all participants) or unstructured (where the interviewer follows up on the responses with further questions). Interviews can be conducted in person, over the phone, or through video conferencing.
Observations
Observations involve watching and recording the behavior and activities of individuals or groups relevant to the case study. Observations can be participant (where the researcher actively participates in the activities) or non-participant (where the researcher observes from a distance). Observations can be recorded using notes, audio or video recordings, or photographs.
Documents can be used as a source of information for case studies. Documents can include reports, memos, emails, letters, and other written materials related to the case study. Documents can be collected from the case study participants or from public sources.
Surveys involve asking a set of questions to a sample of individuals relevant to the case study. Surveys can be administered in person, over the phone, through mail or email, or online. Surveys can be used to gather information on attitudes, opinions, or behaviors related to the case study.
Artifacts are physical objects relevant to the case study. Artifacts can include tools, equipment, products, or other objects that provide insights into the case study phenomenon.
How to conduct Case Study Research
Conducting a case study research involves several steps that need to be followed to ensure the quality and rigor of the study. Here are the steps to conduct case study research:
- Define the research questions: The first step in conducting a case study research is to define the research questions. The research questions should be specific, measurable, and relevant to the case study phenomenon under investigation.
- Select the case: The next step is to select the case or cases to be studied. The case should be relevant to the research questions and should provide rich and diverse data that can be used to answer the research questions.
- Collect data: Data can be collected using various methods, such as interviews, observations, documents, surveys, and artifacts. The data collection method should be selected based on the research questions and the nature of the case study phenomenon.
- Analyze the data: The data collected from the case study should be analyzed using various techniques, such as content analysis, thematic analysis, or grounded theory. The analysis should be guided by the research questions and should aim to provide insights and conclusions relevant to the research questions.
- Draw conclusions: The conclusions drawn from the case study should be based on the data analysis and should be relevant to the research questions. The conclusions should be supported by evidence and should be clearly stated.
- Validate the findings: The findings of the case study should be validated by reviewing the data and the analysis with participants or other experts in the field. This helps to ensure the validity and reliability of the findings.
- Write the report: The final step is to write the report of the case study research. The report should provide a clear description of the case study phenomenon, the research questions, the data collection methods, the data analysis, the findings, and the conclusions. The report should be written in a clear and concise manner and should follow the guidelines for academic writing.
Examples of Case Study
Here are some examples of case study research:
- The Hawthorne Studies : Conducted between 1924 and 1932, the Hawthorne Studies were a series of case studies conducted by Elton Mayo and his colleagues to examine the impact of work environment on employee productivity. The studies were conducted at the Hawthorne Works plant of the Western Electric Company in Chicago and included interviews, observations, and experiments.
- The Stanford Prison Experiment: Conducted in 1971, the Stanford Prison Experiment was a case study conducted by Philip Zimbardo to examine the psychological effects of power and authority. The study involved simulating a prison environment and assigning participants to the role of guards or prisoners. The study was controversial due to the ethical issues it raised.
- The Challenger Disaster: The Challenger Disaster was a case study conducted to examine the causes of the Space Shuttle Challenger explosion in 1986. The study included interviews, observations, and analysis of data to identify the technical, organizational, and cultural factors that contributed to the disaster.
- The Enron Scandal: The Enron Scandal was a case study conducted to examine the causes of the Enron Corporation’s bankruptcy in 2001. The study included interviews, analysis of financial data, and review of documents to identify the accounting practices, corporate culture, and ethical issues that led to the company’s downfall.
- The Fukushima Nuclear Disaster : The Fukushima Nuclear Disaster was a case study conducted to examine the causes of the nuclear accident that occurred at the Fukushima Daiichi Nuclear Power Plant in Japan in 2011. The study included interviews, analysis of data, and review of documents to identify the technical, organizational, and cultural factors that contributed to the disaster.
Application of Case Study
Case studies have a wide range of applications across various fields and industries. Here are some examples:
Business and Management
Case studies are widely used in business and management to examine real-life situations and develop problem-solving skills. Case studies can help students and professionals to develop a deep understanding of business concepts, theories, and best practices.
Case studies are used in healthcare to examine patient care, treatment options, and outcomes. Case studies can help healthcare professionals to develop critical thinking skills, diagnose complex medical conditions, and develop effective treatment plans.
Case studies are used in education to examine teaching and learning practices. Case studies can help educators to develop effective teaching strategies, evaluate student progress, and identify areas for improvement.
Social Sciences
Case studies are widely used in social sciences to examine human behavior, social phenomena, and cultural practices. Case studies can help researchers to develop theories, test hypotheses, and gain insights into complex social issues.
Law and Ethics
Case studies are used in law and ethics to examine legal and ethical dilemmas. Case studies can help lawyers, policymakers, and ethical professionals to develop critical thinking skills, analyze complex cases, and make informed decisions.
Purpose of Case Study
The purpose of a case study is to provide a detailed analysis of a specific phenomenon, issue, or problem in its real-life context. A case study is a qualitative research method that involves the in-depth exploration and analysis of a particular case, which can be an individual, group, organization, event, or community.
The primary purpose of a case study is to generate a comprehensive and nuanced understanding of the case, including its history, context, and dynamics. Case studies can help researchers to identify and examine the underlying factors, processes, and mechanisms that contribute to the case and its outcomes. This can help to develop a more accurate and detailed understanding of the case, which can inform future research, practice, or policy.
Case studies can also serve other purposes, including:
- Illustrating a theory or concept: Case studies can be used to illustrate and explain theoretical concepts and frameworks, providing concrete examples of how they can be applied in real-life situations.
- Developing hypotheses: Case studies can help to generate hypotheses about the causal relationships between different factors and outcomes, which can be tested through further research.
- Providing insight into complex issues: Case studies can provide insights into complex and multifaceted issues, which may be difficult to understand through other research methods.
- Informing practice or policy: Case studies can be used to inform practice or policy by identifying best practices, lessons learned, or areas for improvement.
Advantages of Case Study Research
There are several advantages of case study research, including:
- In-depth exploration: Case study research allows for a detailed exploration and analysis of a specific phenomenon, issue, or problem in its real-life context. This can provide a comprehensive understanding of the case and its dynamics, which may not be possible through other research methods.
- Rich data: Case study research can generate rich and detailed data, including qualitative data such as interviews, observations, and documents. This can provide a nuanced understanding of the case and its complexity.
- Holistic perspective: Case study research allows for a holistic perspective of the case, taking into account the various factors, processes, and mechanisms that contribute to the case and its outcomes. This can help to develop a more accurate and comprehensive understanding of the case.
- Theory development: Case study research can help to develop and refine theories and concepts by providing empirical evidence and concrete examples of how they can be applied in real-life situations.
- Practical application: Case study research can inform practice or policy by identifying best practices, lessons learned, or areas for improvement.
- Contextualization: Case study research takes into account the specific context in which the case is situated, which can help to understand how the case is influenced by the social, cultural, and historical factors of its environment.
Limitations of Case Study Research
There are several limitations of case study research, including:
- Limited generalizability : Case studies are typically focused on a single case or a small number of cases, which limits the generalizability of the findings. The unique characteristics of the case may not be applicable to other contexts or populations, which may limit the external validity of the research.
- Biased sampling: Case studies may rely on purposive or convenience sampling, which can introduce bias into the sample selection process. This may limit the representativeness of the sample and the generalizability of the findings.
- Subjectivity: Case studies rely on the interpretation of the researcher, which can introduce subjectivity into the analysis. The researcher’s own biases, assumptions, and perspectives may influence the findings, which may limit the objectivity of the research.
- Limited control: Case studies are typically conducted in naturalistic settings, which limits the control that the researcher has over the environment and the variables being studied. This may limit the ability to establish causal relationships between variables.
- Time-consuming: Case studies can be time-consuming to conduct, as they typically involve a detailed exploration and analysis of a specific case. This may limit the feasibility of conducting multiple case studies or conducting case studies in a timely manner.
- Resource-intensive: Case studies may require significant resources, including time, funding, and expertise. This may limit the ability of researchers to conduct case studies in resource-constrained settings.
About the author
Muhammad Hassan
Researcher, Academic Writer, Web developer
You may also like

Survey Research – Types, Methods, Examples

Applied Research – Types, Methods and Examples

Quantitative Research – Methods, Types and...

Ethnographic Research -Types, Methods and Guide

Textual Analysis – Types, Examples and Guide

Focus Groups – Steps, Examples and Guide
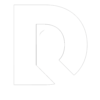
Table of Contents
Data Analysis Using Excel Case Study
Data analysis is an essential skill in today’s business world. As organizations deal with increasing amounts of data, it becomes crucial for professionals to make sense of this information and derive useful insights. Excel is a powerful and versatile tool that can assist in analyzing and presenting data effectively, particularly through the use of case studies.
A case study is a detailed examination of a specific situation or problem in order to better understand the complexities involved. By using Excel for data analysis, individuals can explore and analyze the data related to the case study in a comprehensive and structured manner. Excel offers various tools and functionalities, such as PivotTables, slicers, and data visualization features, which allow users to assess patterns, trends, and relationships within the data.
Applying these techniques for data analysis in Excel case studies enables professionals to make well-informed business decisions and communicate their findings effectively. By leveraging the capabilities of Excel in conjunction with case studies, individuals can unlock valuable insights that drive organizational success and contribute to an enhanced understanding of the overall data landscape.
Excel Basics for Data Analysis
Dataset preparation.
When working with Excel, the first step in data analysis is dataset preparation . This process involves setting up the data in a structured format, with clearly defined headers and cells. To start, you must import or enter your data into an Excel spreadsheet, ensuring that each record is represented by a row and each variable by a column. Headers should be placed in the top row and provide descriptive labels for each column. Proper organization of your dataset helps to ensure accurate analysis and interpretation .
For example, suppose you have a dataset that contains the following information:
In this dataset, the headers are “Year,” “Category,” “Sales,” and “Profit.” Each row represents a record, and the cells contain the corresponding data.
Data Cleaning
The next step in data analysis using Excel is data cleaning . Data cleaning is the process of identifying and correcting errors, inconsistencies, and inaccuracies in your dataset. Common data cleaning tasks include:
- Removing duplicate records,
- Filling in missing values,
- Correcting data entry errors,
- Standardizing and formatting variable names and values.
To perform data cleaning in Excel, you can use various functions and tools:
- Remove duplicates: To remove duplicate records, select your dataset and navigate to the Data tab. Click the “Remove Duplicates” button and select the columns to be used for identifying duplicate rows.
- Fill in missing values: Use Excel functions such as VLOOKUP , HLOOKUP , and INDEX-MATCH to fill in missing values based on other data in your dataset. You can also use the IFERROR function to handle errors when looking up values.
- Correct data entry errors: Use Excel’s “Find and Replace” tool (Ctrl + F) to search for and correct errors in your dataset. You may need to perform this multiple times for different errors.
- Standardize and format variable names and values: Use Excel functions such as UPPER , LOWER , PROPER , and TRIM to standardize text data. Format numerical values using the Number Format options in the Home tab.
By ensuring your dataset is clean and well-organized, you can confidently move forward with more advanced data analysis tasks in Excel.
Powerful Excel Functions
Excel is a versatile tool when it comes to data analysis. There are many powerful functions that can help you perform complex calculations and analysis easily. In this section, we will explore some of the top functions in three categories: Text Functions, Date Functions, and Lookup Functions.
Text Functions
Text Functions are crucial when working with large sets of data containing text. These functions help in cleaning, extracting, and modifying text data. Some key text functions include:
- LEFT : Extracts a specified number of characters from the beginning of a text string.
- RIGHT : Extracts a specified number of characters from the end of a text string.
- MID : Extracts a specified number of characters from a text string, starting at a specified position.
- TRIM : Removes extra spaces from text, leaving a single space between words and no space at the beginning or end of the text.
- CONCATENATE : Joins multiple text strings into one single string.
- FIND : Locates the position of a specific character or text string within another text string.
Date Functions
Date Functions are essential for dealing with dates and times in data analysis. These functions help in calculating the difference between dates, extracting parts of a date, and performing various date-related calculations. Some notable date functions include:
- TODAY : Returns the current date.
- NOW : Returns the current date and time.
- DATEDIF : Calculates the difference between two dates in days, months, or years.
- DATE : Creates a date by combining individual day, month, and year values.
- WEEKDAY : Returns the day of the week corresponding to a specific date, as an integer between 1 (Sunday) and 7 (Saturday).
- EOMONTH : Returns the last day of the month for a given date.
Lookup Functions
Lookup Functions are powerful tools used to search and retrieve data from a specific range or table in Excel. These functions can save time and effort when working with large datasets. Some essential lookup functions include:
- VLOOKUP : Searches for a specific value in the first column of a range and returns a corresponding value from a specified column.
- HLOOKUP : Searches for a specific value in the first row of a range and returns a corresponding value from a specified row.
- INDEX : Returns a value from a specific cell within a range, using row and column numbers.
- MATCH : Searches for a specific value in a range and returns its relative position within that range.
- XLOOKUP : Performs a lookup by searching for a specific value in a range or table and returning a corresponding value from another column or row (available only in Excel 365 and Excel 2019).
These powerful Excel functions can help make the process of data analysis more efficient and accurate. In combination with appropriate formatting, tables, and other visual aids, these functions can greatly enhance your ability to process and understand large datasets.
Related Article: Excel Functions for Data Analysts.
Data Exploration and Visualization
In the process of data analysis using Excel, data exploration and visualization play essential roles in revealing patterns, trends, and relationships within the data. This section will cover two primary techniques for data visualization in Excel: Charts and Trends, and Pivot Tables and Pivot Charts.
Charts and Trends
Charts in Excel are a highly effective method of uncovering patterns and relationships within the dataset. There are various types of charts available in Excel that cater to different use cases, such as bar charts, line charts, and scatter plots. These chart types can be customized to suit the needs of the analysis and to emphasize specific trends or patterns.
Trends in the data can be identified with the help of charts, and Excel offers trend lines functionalities to visualize these trends more clearly. By applying a trend line, one can easily identify the overall direction (positive or negative) of the dataset and make predictions based on this information. Additionally, Excel offers built-in formatting options that can help emphasize certain data points or highlight particular trends for easier interpretation.
Pivot Tables and Pivot Charts
Pivot Tables are another powerful data analysis feature in Excel. They allow the user to summarize, reorganize, and filter data by dragging and dropping columns into different areas. This enables the user to analyze data across multiple dimensions, revealing hidden insights and patterns.
To complement Pivot Tables, Excel also offers Pivot Charts, which allow users to create dynamic visualizations derived from the Pivot Table data. Pivot Charts offer the same chart types as regular Excel charts but with the added capability to update the chart when the Pivot Table data is altered. This makes Pivot Charts ideal for creating interactive and easily updatable visualizations.
Overall, incorporating these techniques into the data analysis process can enhance understanding and unveil valuable insights from the dataset. When using Excel for data analysis, data exploration and visualization with Charts and Trends, as well as Pivot Tables and Pivot Charts, can provide a comprehensive and insightful overview of the data in question.
Case Study: Covid-19 Data Analysis
Data collection and cleaning.
The Covid-19 pandemic has generated vast amounts of data, requiring researchers and analysts to collect, clean, and organize data sets to gain valuable insights. Several sources, such as the World Health Organization and Johns Hopkins University , provide updated information on confirmed cases, recoveries, and deaths.
Data collection starts with gathering raw data from various sources. These data sets may have inconsistencies, missing values, or discrepancies, which need to be addressed to ensure accurate analysis. Data cleaning is a critical step in this process, involving tasks such as removing duplicates, filling in missing values, and correcting errors.
Exploratory Data Analysis
Once the data is clean and organized, exploratory data analysis (EDA) can be conducted using tools like Excel. EDA helps analysts understand the data, identify patterns, and generate hypotheses for further investigation.
Some useful techniques in conducting EDA in Excel include:
- Pivot Tables : These allow users to summarize and reorganize data quickly, providing aggregated views of the data.
- Charts and Graphs : Visual representations of data, such as bar charts or line graphs, can display trends, correlations, or patterns more clearly than raw numbers.
- Descriptive Statistics : Excel’s built-in functions allow easy calculation of measures such as mean, median, and standard deviation, providing a preliminary statistical analysis of the data.
In the context of Covid-19 data, EDA can help reveal important information about the pandemic’s progression. For example, analysts can:
- Compare infection rates across countries or regions
- Monitor changes in case numbers over time
- Evaluate the effectiveness of public health interventions and policies
The insights gained from exploratory data analysis can guide further research, inform decision-making, and contribute to a better understanding of the pandemic’s impact on public health.
Case Study: Stock Market Data Analysis
Data collection and preparation.
The first step in the stock market data analysis case study is collecting and preparing the data. This process involves gathering historical stock prices, trading volumes, and other relevant financial metrics from reliable sources. The data can be cleaned and organized in Excel, removing any errors or inconsistencies. It’s essential to verify the collected data’s accuracy to ensure the analysis’s validity.
After preparing the financial data, the next step is to compute essential measures and ratios. These may include:
- Price-to-Earnings (P/E) Ratio
- Dividends Yield
- Total Return
- Moving Averages
Calculating these ratios and measures provides a general overview of a company’s performance in the stock market, which can be further analyzed with Excel tools.
Profit and Loss Analysis
In this stage of the case study, profit and loss analysis is conducted to assess the stock’s performance. Using Excel PivotTables, we can summarize the data to identify trends or patterns in the stock market. For instance, we can analyze the historical profits and losses of multiple stocks during a specific state or market condition.
Analyzing profit and loss data can also be done with natural language capabilities in Excel. This feature allows us to ask questions about the dataset, and Excel will produce relevant results. For example, we could pose a question like “Which stocks had the highest profit margins in the last quarter?” or “What is the average loss for the technology sector?”
After exploring the profits and losses of the stocks, we can gain insights into which stocks or sectors are more profitable or risky. This information can help potential investors make informed decisions about their investment strategies. Additionally, the insights from the case study can serve as a reference point for future stock market analyses.
Remember, this case study only serves as an example of how to conduct stock market data analysis using Excel. By adapting and expanding on these techniques, one can harness the power of Excel to explore various aspects of financial markets and derive valuable insights.
Case Study: San Diego Burrito Ratings
Data gathering and cleaning.
The main objective of this case study is to evaluate and analyze the various factors that contribute to the ratings of San Diego burritos. The data used in this analysis is collected from different sources, which include customer reviews and ratings from Yelp, along with other relevant information about burrito sales and geographical distribution. The raw data is then compiled and cleaned to ensure that it is consistent and free from any discrepancies or errors. This process involves standardizing the fields and records, as well as filtering out any irrelevant information. The cleaned data is then organized into a structured format, which is suitable for further analysis using Excel PivotTables and Charts.
Use of Pivot Tables and Charts
After cleaning and organizing the data, Excel PivotTables are utilized to analyze the regional distribution of San Diego burrito ratings. By categorizing the data based on regions, such as East and West, it becomes convenient to identify the ratings and sales trends across these regions. The organized data is then sorted based on the ratings and popularity of burrito establishments within specific densely populated areas.
Using Pivot Charts, a graphical representation of the data is created to provide a clear and comprehensive visual of the ratings distribution in different regions of San Diego. It becomes easier to discern patterns and trends, allowing for the development of informed conclusions on the factors influencing the popularity and success of burrito establishments.
Throughout the analysis, various parameters are investigated, which include the relationship between ratings and sales, the potential impact of particular fields on popularity, and the apparent differences between densely populated regions in terms of burrito preferences. By utilizing PivotTables and Charts confidently, it is possible to draw insights and conclusions that can help optimize marketing strategies, guide customer preferences, and influence the overall success of burrito establishments across San Diego.
Case Study: Shark Attack Records Analysis
Data collection and pre-processing.
In this case study, the primary focus is on the analysis of shark attack records recorded between 1900 and 2016, consisting of just under 5,300 records or observations. To begin the analysis, the data needs to be collected from a reliable source and pre-processed to ensure its accuracy and relevance.
Data pre-processing is an essential step to prepare the dataset for analysis. It involves checking for missing values, outliers, and inconsistencies in the data. Additionally, it may also require converting the data into a suitable format, such as categorizing dates or splitting location information into separate columns (latitude and longitude).
Identifying Trends and Patterns
Once the dataset has been pre-processed, it’s time to dive into the analysis using Microsoft Excel. Excel offers a fast and central way to analyze data and search for trends and patterns within shark attack records. One powerful tool for this purpose is Excel’s PivotTables, which allows users to easily aggregate and summarize data.
Some possible trends and patterns that can be identified through the analysis of shark attack records include:
- Temporal Trends: Analyzing the frequency of shark attacks over time to identify any patterns in the occurrence of attacks, such as seasonality or specific years with higher attack rates.
- Geographical Patterns: Identifying areas with a higher concentration of shark attacks, which can provide insights into hotspots and potentially dangerous locations.
- Victim Demographics: Examining the demographics of shark attack victims, such as age, gender, and activity type, to determine if certain groups are more prone to attacks.
- Species Involved: Investigating the types of shark species responsible for attacks and their relative frequency in the dataset.
By utilizing Excel’s data analysis tools and PivotTables, researchers can confidently and clearly identify trends and patterns in the shark attack records, providing valuable insights into shark behavior and risk factors associated with shark attacks. This analysis can be helpful in understanding and managing the risks associated with shark encounters for both public safety and conservation efforts.
Related Article: How to Solve Data Analysis Real World Problems.
Additional Resources and Exercises
Kaggle and data analysis courses.
Kaggle is a popular platform that offers data science competitions, datasets, and courses to help you improve your data analysis skills in Excel. The courses are designed for various skill levels, and they cover essential concepts like PivotTables and data visualization. The comprehensive exercises and practical case studies provide a real-world context for mastering data analysis techniques.
The course reviews on Kaggle are usually quite positive, with many users appreciating the knowledgeable instructors and engaging content. If you’re looking to become a data analyst or enhance your existing skills, exploring the data analysis courses on Kaggle is a great starting point.
Power Query in Excel
Power Query is a powerful data analysis tool in Excel that enables you to import, transform, and combine data from various sources. This feature is particularly useful when working with large datasets or preparing data for analysis. There are numerous resources available to learn how to use Power Query effectively.
To practice using Power Query, consider working on exercises that focus on data cleansing, data transformation, and data integration. As you progress, you will gain a deeper understanding of the various Power Query functionalities and become more confident in your data analysis abilities.
In conclusion, engaging with additional resources like Kaggle courses and Power Query exercises will help you hone your Excel data analysis skills and enable you to tackle complex case studies with ease.
Frequently Asked Questions
How can excel be used for effective case study analysis.
Excel is a versatile tool that can be utilized for effective case study analysis. By organizing and transforming data into easily digestible formats, users can better identify trends, patterns, and insights within their data sets. Excel also offers various functions and tools, such as pivot tables, data tables, and data visualization, which enable users to analyze case study data more efficiently and uncover valuable information.
Which Excel functions are most useful for data analysis in case studies?
There are numerous Excel functions that can be highly useful for data analysis in case studies. These include:
- VLOOKUP, which allows users to search for specific information in large data sets
- INDEX-MATCH, a more advanced alternative to VLOOKUP that’s capable of handling more complex data structures
- IF, which helps in making conditional statements and decisions in data analysis
- AVERAGE, MAX, MIN, and COUNT for basic data aggregation
- SUMIFS and COUNTIFS, which allow users to perform conditional aggregation based on predefined criteria
What are some examples of data analysis projects using Excel?
Many different projects can benefit from data analysis using Excel, such as financial analysis, market research, sales performance tracking, and customer behavior analysis. Businesses across industries are known to use Excel for evaluating their case studies and forming data-driven decisions based on their insights.
How can Excel pivot tables aid in analyzing case study data?
Pivot tables in Excel are powerful, enabling users to summarize and analyze large data sets quickly and efficiently. They allow users to group and filter data based on different dimensions, making it much easier to identify trends, patterns, and relationships within the data. Additionally, pivot tables provide user-friendly drag-and-drop functionalities, allowing for easy customization and requiring minimal Excel proficiency.
In which industries is Excel data analysis most commonly applied in case studies?
Excel data analysis is widely used across various industries for case studies, including:
- Finance and banking, for analyzing investment portfolios, risk management, and financial performance
- Healthcare, for patient data analysis and identifying patterns in disease occurrence
- Marketing and sales, to analyze customer data and product performance
- Retail, for inventory management and sales forecasting
- Manufacturing, to evaluate the efficiency and improve production processes
What steps should be followed for a successful data analysis process in Excel?
A successful data analysis process in Excel typically involves the following steps:
- Data collection: Gather relevant data from various sources and consolidate it in Excel.
- Data cleaning and preprocessing: Remove any errors, duplicate records, or missing values in the data, and reformat it as necessary.
- Data exploration: Familiarize with the data, identify patterns, and spot trends through descriptive analysis and visualization techniques.
- Data analysis: Use relevant functions, formulas, and tools such as pivot tables to analyze the data and extract valuable insights.
- Data visualization: Create charts, graphs, or dashboard reports to effectively visualize the findings for improved understanding and decision-making.
What you should know:
- Our Mission is to Help you to Become a Professional Data Analyst.
- This Website is a Home for Data Analysts. Get our latest in-depth Data Analysis and Artificial Intelligence Lessons and Updates in your Inbox.
Tech Writer | Data Analyst | Digital Creator
Get Our Professional Data Analyst Roadmap for free
You may also like
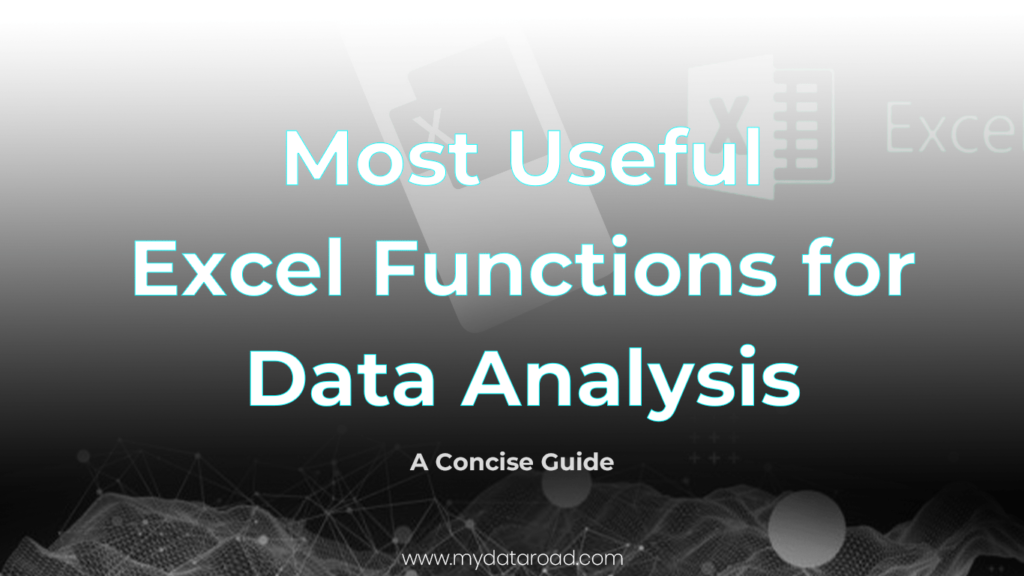
Harvard Business Analytics Program
Designed for aspiring and established leaders in any industry, HBAP equips participants with the machine learning and data analysis tools they need to incorporate innovative tech into their business strategy, at the top levels of their organization.
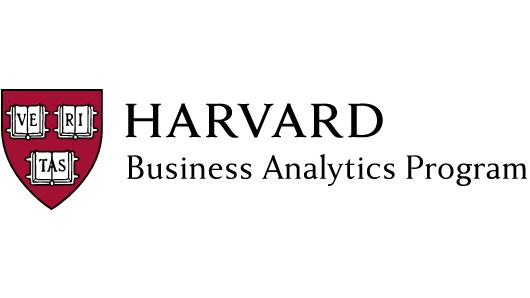
Associated Schools
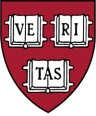
Harvard Faculty of Arts & Sciences
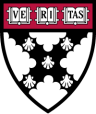
Harvard Business School
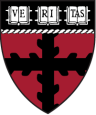
Harvard School of Engineering and Applied Sciences
What you'll learn.
Capabilities in technical, analytical, and operational areas to advance your firm’s position in the global market.
Core data analysis and management skills.
How to keep up with a business world that is constantly impacted by machine learning and artificial intelligence.
An in-depth understanding of modern technologies and practices in next-generation analytics, such as blockchain, digital strategy, and AI/ML.
How to interpret your findings and use them to uncover valuable business insights.
How to apply these concepts to your organization, with a mind toward maximizing efficiencies and outcomes.
Course description
Unlike many other offerings, the Harvard Business Analytics Program features a blended format with live online and in-person components.
The Harvard Business Analytics Program consists of six core courses, two seminars, and two in-person immersions. The rigorous curriculum delivers an authentic Harvard experience and consists of entirely new courses that are frequently updated to adapt to industry changes and emerging technologies. The program can be completed in as little as nine months.
Participants who are accepted into the upcoming cohort can apply for an income-based scholarship of up to 30%. To secure the scholarship offer, participants who haven't already made an enrollment deposit must do so immediately, as scholarships are awarded on a first-come, first-served basis to a limited number of applicants.
Course Outline
Competing in the Age of AI
Artificial intelligence (AI) is revolutionizing the way today's businesses compete and operate. By putting AI and data at the center of their capabilities, companies are redefining how they create, capture, and share value—and are achieving impressive growth as a result. This course will delve into new AI-based business models and operational approaches. Through global case studies on market leaders and innovative startups in diverse industries, the course will explore a range of capabilities that can help your business succeed in today's data-driven environments. Along with learning how to unlock new growth potential, you will deepen your understanding of ethical challenges that come with leveraging massive amounts of data and sophisticated analytics. You will further examine the ethics of AI through real-world case studies that have widespread debate about issues such as academic freedom, corporate responsibility, and bias in AI systems. Recent developments in generative AI are discussed including how large language models are developed and how they can be used by individuals and businesses. Course faculty demonstrate conversing and performing data analytics with ChatGPT.
Programming and Data Systems
Combining technical guidance, analysis of real-world cases, and hands-on coding assignments each week, this initiative enables individuals to navigate technological choices effectively, even without a technical background. Covering areas such as artificial intelligence, cloud computing, networking, privacy, scalability, and security, the program places a special focus on web and mobile technologies. Participants will learn the basics of coding with SQL and Python, and be introduced to fundamental concepts in decision trees, neural networks, LLMs, other types of AI models, and generative AI in order to understand what AI can do for their organization.
Leadership, Innovation, and Change
This course places a strong emphasis on the leader’s role in enhancing the execution of their existing strategy to outperform competitors, alongside their involvement in driving strategic innovation. We employ the congruence model that links strategy to execution through alignment of culture, people, tasks, structure, and executive leadership. Because ambidexterity requires leaders that can deal with punctuated change and paradoxical strategies, our program concludes with what we know about ambidextrous leadership and leading large system change.
Operations and Supply Chain Management
This program will focus on overseeing product availability, particularly within the dynamic landscape of swift product expansion, brief product life cycles, and interconnected networks of suppliers and customers on a global scale. The key topics examined include inventory management, distribution economics, demand forecasting, supplier management, and the potential benefits of using AI and machine learning to work with data.
Foundations of Quantitative Analysis
This program serves as an initiation into employing statistical methodologies for tackling business challenges. Key elements of the curriculum include methods for describing and summarizing data, the fundamentals of probability, the basics of study design and data collection, and statistical inference. Data analyses, simulation, and design issues are implemented in the statistical computing package R run within the RStudio interface.
Leadership and People Analytics
Participants will develop practical skills to analyze data in a manner that compliments the frameworks and intuitions typically employed to steer their managerial decisions on people issues. Anchored in data, this program will equip participants with an analytic approach for assessing the diverse factors impacting individual, team, and organizational performance, ultimately resulting in more impactful interventions and initiatives.
Data-Driven Marketing
The once qualitative and instinct-driven aspect of business functions (think “Mad Men”) has evolved into a data-driven profession that relies on quantitative insights on how best to optimize ad creation and placement and influence consumer purchase behavior. This course focuses on the benefits that AI and machine learning bring to marketing including enhanced personalization and customization, as well as pricing optimization and automation. Participants will also learn how AI affects change management and algorithmic bias. This course will examine the ways in which marketing has changed and the new skills and capabilities needed to succeed in this function.
Data Science Pipeline and Critical Thinking
This program will take a holistic approach to helping participants understand the key factors involved in the data science pipeline, from data collection to analysis to prediction and insight. The curriculum will expand on the application of AI in data science by looking at the role of machine learning. Topics such as large language models, supervised learning, unsupervised learning, Bayes’ Theorem, and deep learning will be explored throughout the program. Projects will give participants hands-on experience developing and running a data science pipeline to ensure that the correct business predictions are being made.
In addition to the online learning components of the program, you will have the opportunity to attend two 2.5-day, on-site and in-person immersions held at the Harvard Business School campus in Boston.
During these experiences, you'll get to engage in direct interactions with your classmates, establish connections with faculty members, and connect with influential figures in the industry during evening events. You’ll also have the chance to tour the Harvard campus and participate in hands-on guided learning exercises. Throughout your experience, you’ll gain an in-depth understanding of deep learning and neural networks, and use the HBS case method to formulate solutions to real-world business scenarios as a way to understand relevant challenges in the industry. Recent topics include fairness in algorithms, data privacy, leading transformational change, and the evolving landscape of AI.
Instructors
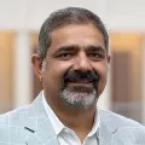
Karim Lakhani
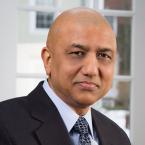
Sunil Gupta
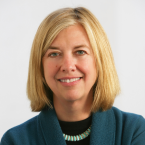
Janice Hammond
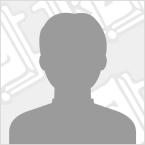
Hanspeter Pfister
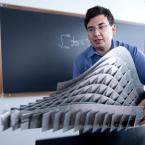
Joseph Blitzstein
Mark glickman.
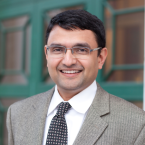
V. Narayanan
Kevin rader, michael i. parzen.
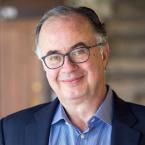
Marco Iansiti
Henry h. leitner, margo seltzer.
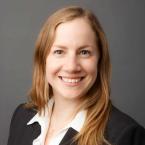
Ayelet Israeli
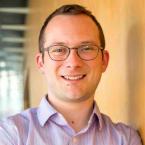
David Parkes
You may also like.
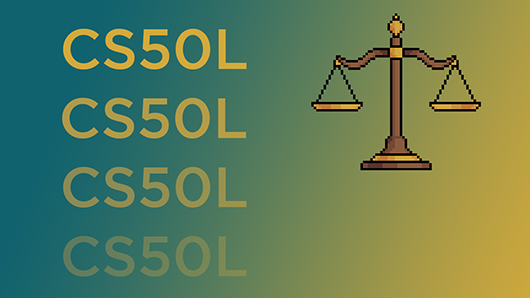
CS50 for Lawyers
This course is a variant of Harvard University's introduction to computer science, CS50, designed especially for lawyers (and law students).
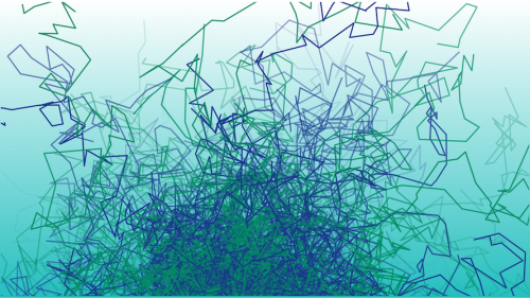
Using Python for Research
Take your introductory knowledge of Python programming to the next level and learn how to use Python 3 for your research.
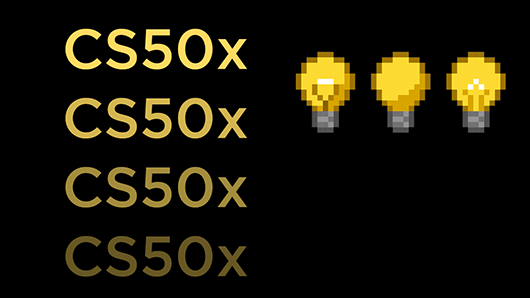
CS50: Introduction to Computer Science
An introduction to the intellectual enterprises of computer science and the art of programming.
Join our list to learn more
We use cookies on our website to support technical features that enhance your user experience, and to help us improve our website. By continuing to use this website, you accept our privacy policy .
- Student Login
- No-Cost Professional Certificates
- Call Us: 888-549-6755
- 888-559-6763
- Search site Search our site Search Now Close
- Request Info
Skip to Content (Press Enter)
How to Become a Data Analyst and Change Your Career
By Brianna Flavin on 05/30/2024

Data analytics has been booming lately. That growth and excitement has lots of people wondering how to become a data analyst. It's a great career to consider! Whether you want to change careers or just set your life on a new track, data analysis could be the dawning of a whole new era in your life.
But learning data analysis takes training, effort and time. We asked data analytics professors to explain how to become a data analyst, along with some important things you'll want to keep in mind as you decide. Before you make any concrete plans, get a bird's eye view of the steps you'd need to take to get into the data industry. You might also be wondering " is data analyst a good career ?".
Step 1: Consider your ideal career outcome
Data analysis isn't just one job.
In the same way that a teacher's job looks totally different if they teach preschoolers vs. college undergraduates, a data analyst will need different skills depending on where they want to work.
While you will definitely learn more about the field if you enroll in a data analytics program, doing a little preemptory research can help you narrow down those program options to make sure you choose a good fit.
"I think students need to have a clear understanding of the type of work a prospective degree program offers, and if it’s in the line of work they want to pursue," says Kevin Watts, Data Analytics Lead and Professor of Technology at Rasmussen University.
"There’s a difference between business analytics, data analytics and data science, for example," Watts points out. "These terms have been used quite loosely in the industry. But if data scientists will be doing heavy programming." In contrast, Watts says students in a data analytics program will focus more on tools and techniques to analyze data.
Of course, lots of crossover happens in data roles. "I find, just in looking at the job market, that students have to have some basic programming skills for a lot of data analyst jobs, even in a business analyst role, Watts says.
"Plan on taking at least an introductory course in tools like Tableau ® (a data visualization platform) and Python ® (a programming language)."
If you lean toward the work of a data scientist or would love to learn multiple programming languages, choose a data science or computer science program over a data analytics program.
If you see yourself in the corporate world giving presentation about findings to help guide your company, look into programs that will give you some exposure to business analytics.
If you want to graduate with solid, technical data analyst skills, choosing a data analytics program is your best bet. Be sure to research enrollment requirements and prerequisites for any program you are considering.
For example, at Rasmussen, Data Analytics Bachelor’s degree program applicants must already have conferred associate’s or bachelor’s degree or completion of at least 60 quarter or 40 semester credits of college-level coursework with a grade of C or higher in each course.
Step 2: Decide the level of education you need
Now that you have an idea of what type of data analyst you might want to be, you should start researching program options.
There are so many jobs out there that you can definitely find a variance in what employers require, but for marketing analyst roles (a type of business analyst) the Bureau of Labor Statistics (BLS) writes that a bachelor's degree is the most common education requirement. 1
Since data analysts need a thorough understanding of how to work with huge volumes of data, as well as the technical skills to collect, refine, evaluate and explain data insights—these degree programs are pretty crucial. Data analysis tools make a lot more sense when you understand how a successful data analyst uses them.
If you already have a bachelor's degree in another field, it's worth asking if you can transfer some credits over into a data analytics program. Or you could look into certifications that would give you the training data professionals need to pair with your current degree.
If you don't yet have a bachelor's degree, choosing a bachelor's program in data analytics is your next step.
Step 3: Vet your data analytics program options (contact them directly)
Data analytics programs at different schools will all be a bit different, with their own nuances. Here are a few things to consider as you research.
*Note, since some of these things might be hard to find online, call any school program you are serious about to ask your questions. Or at least contact the program directly. It's extra effort, yes, but when you consider the expense of a university program, ten minutes on the phone or filling out a "tell me more form" to decide if program leaders know what they're doing is probably worth it.
What is the cost of tuition?
These are often the two most important questions at the beginning. It's awesome when you can find tuition information online, but some schools don't post tuition prices because their programs will have different costs depending on each student.
Admissions representatives also know about the different aid options available to students, so contacting a program directly can help you work out a more accurate true cost than the numbers they post online.
How long does the data analytics program take?
The same kind of thing can be true for how long programs take. But with full time enrollment, the bachelor's degree program in data analytics at Rasmussen can be completed in as few as 18 months. 2
How often do they evaluate the data analytics curriculum?
If you think about how quickly data analysts need to adjust to change and new technologies, you'll understand that data analytics programs need to stay on their toes to keep curriculum current.
"One thing I like about the program at Rasmussen is that it’s constantly evolving," Watts says. "We look at courses on a frequent rotation, taking in student and faculty feedback." Watts explains that they make changes to data analytics courses when technology changes, as well as when something isn't working well for the students.
"There was a class I taught where the assignments required students to go to an external website's data set, but the site was creating constant frustrations," Watts recalls. "After the course, I gave my feedback that the site was causing extra work and aggravation, so we changed the course to build on a data set we provided."
While he was rewriting that course, Watts also adjusted the assignment parameters to make the whole experience more intuitive.
As you can imagine, programs that frequently evaluate their course material wind up incorporating all sorts of learning improvements—from the specific data analytics tools and objectives to the overall student experience.
How will the data analytics courses work?
In the old days, academia had a pretty template routine. Show up to lectures, take exams, write papers. But technology and more awareness of what succeeds in different areas of study (thank you data analytics!) has changed education in a major way.
You can find online programs, in-person programs, maybe even some that are a mixture of both.
You can also find programs that keep a tight, synchronous schedule (ex: everyone has to log in or show up at the same times throughout the week, assignments are all due at midnight on a Sunday, etc...).
Or you can choose programs that allow students more leeway to work through course content. Ex: Log in once a week, watch recorded lectures, complete course content as you go, etc...
The last thing you want is to go through all the work to enroll in a program, then find out the pace is totally incompatible with your other responsibilities—or find out the format is making everything harder because of the way you learn.
Ask about it ahead of time, then you can prepare accordingly.
Step 4: Enroll in a program and make the time count
Once you choose, apply and gain acceptance to a program, whether that's a bachelor's degree program or a certificate program to add skills to your current background, it's time to rock. (Rasmussen does not offer certificate programs in data analytics.)
Pay attention to the type of work you like best as you learn. There are so many ways data analysts can specialize in their careers. And whether you gravitate toward quantitative work, like statistical analysis or the critical thinking and creative thinking components—push yourself to become a well-rounded data analyst.
Giving students transferable skills like communication and professionalism on top of the technical skills data analysts need is a priority at Rasmussen.
"Degree programs should give you broad exposure to the industry," Watts explains. "It's when you get into the field in corporate America that you'll see more specified roles and the chance to pursue areas of interest."
While he's teaching, Watts loves to bring in examples from his own work experience, to help students make further connections to their future careers. "A lot of my work experience comes through in my lectures. I bring that content in, and I considered those real-life experiences while I wrote these courses," Watts says.
In one of his courses, he gives students data that is flawed, intentionally. "They only have a sample and have to do some math, make some assumptions to statistically forecast what things would be like." This mirrors some of Watt's work experience where the analysts lacked access to all the raw data for every client.
"We came up with ways to make sound business decisions based only on samples," Watts recalls. These problem-solving skills are the bread and butter of data analysis.
Step 5 extra credit : Do an internship or volunteer your skill
Doing the actual work of a data analyst as soon as possible will only strengthen your competitive advantage in this field. Ideally, your program assignments involve real world scenarios, but if you can take on an extra project to add to your resume, you'll impress potential employers.
"Take advantage of internships or volunteer to use what you are learning," says Asia Anuwa, Data Analytics Professor at Rasmussen University. Reach out to a local business or a friend or family member and see if they'd want some free data-driven insights. (Wouldn't the answer always be a yes?)
A data analyst collects data, refines it, analyzes it and presents it. Offer to do that for someone, and add the project to your resume or portfolio.
Step 6: Find a data analyst job
When you graduate, you should have a skill set that is valuable in many different countries. Data analysis skills are so useful that it's hard to imagine any medium to large company going without them. Update your LinkedIn profile, check out job postings for companies that interest you, and keep networking with other people from your program.
You never know when a connection with a peer or a professor might blossom into career opportunities. This is a good reason to truly connect with people in your program. "Stand out while still in school, don't isolate yourself," Anuwa says.
Afterall, you are working and paying for this program. Don't limit yourself to analyzing data and completing the coursework. Ask questions, connect with fellow students and let your network grow.
Is a data analyst role on your radar?
If you want to become a data analyst, one of the best things you can do is contact a data analytics program to ask some questions and evaluate if they are the right fit for you.
If you already have a degree in another field, Rasmussen's data analytics program might fit your needs.
Check out the Data Analytics Bachelor's program degree page for more information on start dates, program structure and a form you can use to hear directly from a admissions advisor.
Tableau ® is a registered trademark of SALESFORCE, INC. Python ® is a registered trademark of PYTHON SOFTWARE FOUNDATION 1 Bureau of Labor Statistics, U.S. Department of Labor, Occupational Outlook Handbook, Market Research Analysts, (accessed May 2024), Market Research Analysts : Occupational Outlook Handbook: : U.S. Bureau of Labor Statistics (bls.gov) 2 Completion time is dependent on transfer credits accepted and the number of courses completed each term
- Share on Facebook
- Share on Twitter
- Share on Pinterest
- Share on LinkedIn
Request More Information
Talk with an admissions advisor today. Fill out the form to receive information about:
- Program Details and Applying for Classes
- Financial Aid and FAFSA (for those who qualify)
- Customized Support Services
- Detailed Program Plan
There are some errors in the form. Please correct the errors and submit again.
Please enter your first name.
Please enter your last name.
There is an error in email. Make sure your answer has:
- An "@" symbol
- A suffix such as ".com", ".edu", etc.
There is an error in phone number. Make sure your answer has:
- 10 digits with no dashes or spaces
- No country code (e.g. "1" for USA)
There is an error in ZIP code. Make sure your answer has only 5 digits.
Please choose a School of study.
Please choose a program.
Please choose a degree.
The program you have selected is not available in your ZIP code. Please select another program or contact an Admissions Advisor (877.530.9600) for help.
The program you have selected requires a nursing license. Please select another program or contact an Admissions Advisor (877.530.9600) for help.
Rasmussen University is not enrolling students in your state at this time.
By selecting "Submit," I authorize Rasmussen University to contact me by email, phone or text message at the number provided. There is no obligation to enroll. This site is protected by reCAPTCHA and the Google Privacy Policy and Terms of Service apply.
About the author
Brianna Flavin
Brianna is a senior content manager who writes student-focused articles for Rasmussen University. She holds an MFA in poetry and worked as an English Professor before diving into the world of online content.
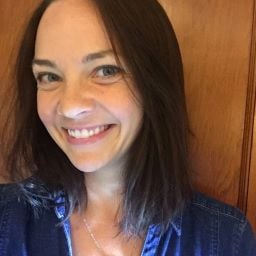
Posted in Data Analytics
- tech careers
- data science
- tech education
Related Content

Brianna Flavin | 05.30.2024
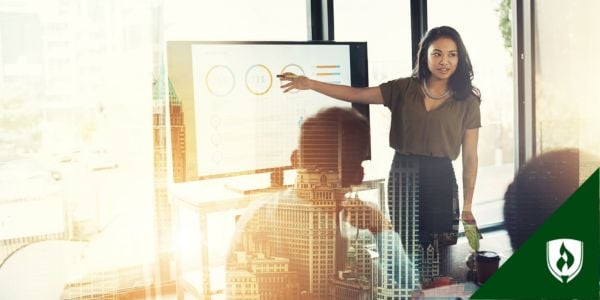
Brianna Flavin | 05.02.2024
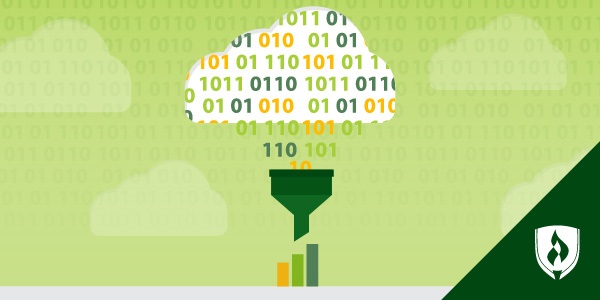
Kirsten Slyter | 09.19.2022
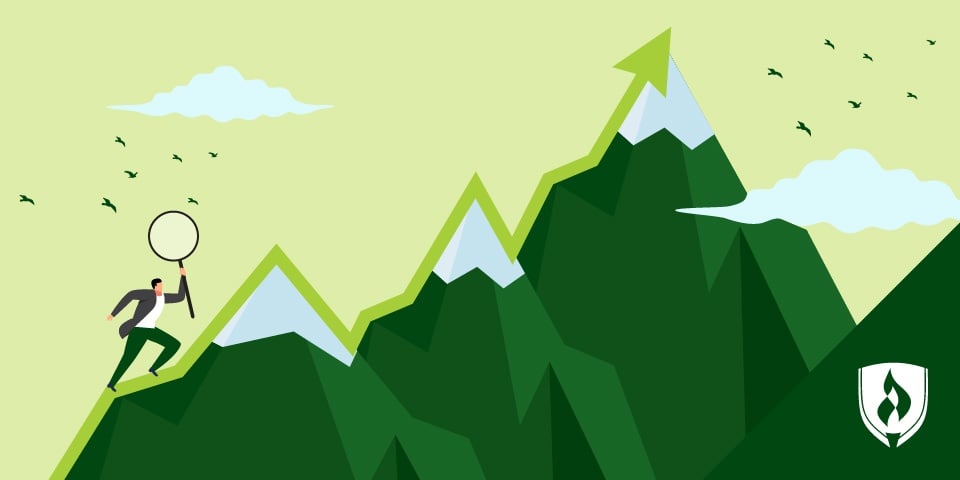
Brianna Flavin | 06.28.2022
This piece of ad content was created by Rasmussen University to support its educational programs. Rasmussen University may not prepare students for all positions featured within this content. Please visit www.rasmussen.edu/degrees for a list of programs offered. External links provided on rasmussen.edu are for reference only. Rasmussen University does not guarantee, approve, control, or specifically endorse the information or products available on websites linked to, and is not endorsed by website owners, authors and/or organizations referenced. Rasmussen University is accredited by the Higher Learning Commission, an institutional accreditation agency recognized by the U.S. Department of Education.
- Open access
- Published: 29 May 2024
Cannabis use in a Canadian long-term care facility: a case study
- Lynda G. Balneaves 1 , 4 ,
- Abeer A. Alraja 1 ,
- Genevieve Thompson 1 ,
- Jamie L. Penner 1 ,
- Philip St. John 2 ,
- Daniella Scerbo 1 &
- Joanne van Dyck 3
BMC Geriatrics volume 24 , Article number: 467 ( 2024 ) Cite this article
300 Accesses
2 Altmetric
Metrics details
Following the legalization of cannabis in Canada in 2018, people aged 65 + years reported a significant increase in cannabis consumption. Despite limited research with older adults regarding the therapeutic benefits of cannabis, there is increasing interest and use among this population, particularly for those who have chronic illnesses or are at end of life. Long-term Care (LTC) facilities are required to reflect on their care and policies related to the use of cannabis, and how to address residents’ cannabis use within what they consider to be their home.
Using an exploratory case study design, this study aimed to understand how one LTC facility in western Canada addressed the major policy shift related to medical and non-medical cannabis. The case study, conducted November 2021 to August 2022, included an environmental scan of existing policies and procedures related to cannabis use at the LTC facility, a quantitative survey of Healthcare Providers’ (HCP) knowledge, attitudes, and practices related to cannabis, and qualitative interviews with HCPs and administrators. Quantitative survey data were analyzed using descriptive statistics and content analysis was used to analyze the qualitative data.
A total of 71 HCPs completed the survey and 12 HCPs, including those who functioned as administrators, participated in the interview. The largest knowledge gaps were related to dosing and creating effective treatment plans for residents using cannabis. About half of HCPs reported providing care in the past month to a resident who was taking medical cannabis (54.9%) and a quarter (25.4%) to a resident that was taking non-medical cannabis. The majority of respondents (81.7%) reported that lack of knowledge, education or information about medical cannabis were barriers to medical cannabis use in LTC. From the qualitative data, we identified four key findings regarding HCPs’ attitudes, cannabis access and use, barriers to cannabis use, and non-medical cannabis use.
Conclusions
With the legalization of medical and non-medical cannabis in jurisdictions around the world, LTC facilities will be obligated to develop policies, procedures and healthcare services that are able to accommodate residents’ use of cannabis in a respectful and evidence-informed manner.
Peer Review reports
In October 2018, Canada became the second country to legalize non-medical cannabis [ 1 ]. Despite the increasing interest in cannabis among Canadians of all ages [ 2 ], the percentage of individuals over the age of 15 years reporting cannabis use a year following legalization remained relatively unchanged at 18% [3]. The only age group to report a significant increase in cannabis consumption was those aged 65 + years, with 7.6% reporting cannabis use in the past 3 months [ 3 ] in 2019 compared to 4% in 2018. This upward trend in cannabis use among Canadians 65 years or older was also observed in 2021 [ 4 ].
This increase may reflect a growing acceptance of cannabis among older populations who were previously dissuaded from taking cannabis due to its illegal status as well as limited accessibility through legal means. In addition, the rise in cannabis use among older adults may reflect a harm reduction approach, substituting cannabis for other recreational substances with substantial health risks, such as alcohol [ 5 ]. Moreover, the belief in the potential therapeutic benefits of cannabis [ 6 , 7 , 8 ], such as the management of pain and sleep issues, is becoming increasingly prevalent among older adults. There has been limited research, however, among older adults in Canada to understand this progressive trend in cannabis use and the influencing factors [ 9 ].
Canada has been a world leader in cannabis legalization, launching a federal medical cannabis program in 2001. Since this time, the medical cannabis program has undergone numerous revisions, including how authorization is obtained, what types of products are available, and where cannabis is purchased. Currently, Canadians can seek medical authorization from either a physician or a nurse practitioner, and access a variety of cannabis products, including dried flower, capsules, and oils, which are purchased online through a licensed producer (LP). Some individuals also apply for a personal or designated grow license to produce their own supply of dried cannabis. Outside of the medical authorization program, individuals can access non-medical cannabis through an authorized storefront. It is estimated that over 1 million Canadians are using cannabis for therapeutic purposes [4], with 247,548 individuals officially registered as of March 2022 [ 10 ]. Among the 479,400 individuals over the age of 65 who reported cannabis use in the third quarter of 2019, 52% utilized cannabis exclusively for medical reasons, and another 24% reported using cannabis for both recreational and medical purposes [ 3 ].
Despite the growing interest in cannabis as a therapeutic agent, there has been limited human research due to its illegal status in many countries, as well as the challenges posed by the complexity of the cannabis plant compared to single agent, pharmaceutical forms of cannabis (e.g., nabilone) [ 11 , 12 ]. Notwithstanding these challenges, there is emergent research on the potential role of cannabis-based medicines in the management of health conditions common among older adults, including osteoarthritis [ 13 ], sleep disorders [ 14 ], dementia [ 15 ], and Parkinson’s [ 16 , 17 ], which are also prevalent among individuals residing at long-term care (LTC) facilities. For example, several studies have found cannabis-based medicines to significantly reduce neuropsychiatric symptoms and improve quality of life among people living with Alzheimer’s Disease [ 18 , 19 , 20 ]. Cannabis may also play a significant role at end of life in not only alleviating physical symptoms, such as pain, nausea and vomiting, and appetite loss, but also addressing the emotional and existential issues that may arise [ 21 ]. It has also been proposed that cannabis may have a therapeutic role among rehabilitative populations who often reside in LTC settings, including those with spinal cord injuries [ 22 , 23 ] and traumatic brain injury [ 24 ]. The evidence base surrounding cannabis as a therapeutic agent, however, remains limited with few large randomized clinical trials conducted to date.
Cannabis is not a benign substance and may pose risk to older adults, especially those living with frailty or cognitive impairment. Given the known cognitive effects of tetrahydrocannabinol (THC), a cannabinoid found in many forms of cannabis, adults living in long-term and rehabilitative care settings may experience somnolence, confusion, and fatigue [ 25 ]. Cannabis high in THC may also negatively impact motor coordination and increase the risk of falls, especially among those with impaired balance and walking ability [ 25 ]. As research advances on cannabis, there has been growing awareness of its negative interactions with certain medications [ 26 ], which can pose a significant issue among older clients prone to polypharmacy. Lastly, numerous health conditions are contraindicated with cannabis use, including heart disease, and a personal or family history of psychosis, schizophrenia, or bipolar disorder [ 27 ].
Despite limited research with older adults regarding the therapeutic benefits of cannabis, there is increasing interest and use among this population, particularly for those who have chronic illnesses. As adults age, they are more likely to experience multimorbidity, and a significant number of older adults spend their last years of life residing in a LTC facility [ 28 , 29 ]. LTC facilities are, thus, placed in a unique position. While these facilities are considered medical institutions that provide evidence-informed supportive health care, they have also become home for individuals who are no longer able to reside safely in the community. Increasingly, these types of facilities are challenged to create home-like environments and offer residents the opportunity and autonomy to engage in potentially risky health behaviours [ 30 ]; behaviours that individuals in the community have the independence and legal right to choose, such as alcohol or tobacco consumption. With the legalization of non-medical cannabis and the growing interest in the potential of cannabis to manage challenging health conditions, it behooves LTC facilities to reflect on their care and policies related to the use of legal substances, such as cannabis, and how to address residents’ cannabis use within what they consider to be their home.
The overarching aim of this case study was to understand how one LTC facility, and its healthcare professionals (HCPs) and administrators, addressed the major policy shift in Canada related to medical and non-medical cannabis. Specific research questions included: (1) What are the experiences and perceptions of HCPs and administrators regarding the use of medical and non-medical cannabis at LTC settings?; (2) What are the perceived barriers/facilitators to medical and non-medical cannabis use at LTC facilities from the perspective of HCPs and administrators?; and (3) What are the educational needs, attitudes, and practices of HCPs at LTC facilities related to medical and non-medical cannabis?
Research design and setting
An exploratory case study design was utilized in this study. This type of case study is used to explore those situations in which the phenomenon being evaluated has no clear or single set of outcomes [ 31 ]. The case selected for this study was a large LTC facility in Western Canada. This 387-bed residential facility provides 24/7 care to a diverse population, including older adults with cognitive and physical disabilities, individuals recovering from stroke and traumatic brain injury, and those requiring end-of-life care. Individuals with these various conditions may reside in several units, including palliative care, rehabilitation, personal care home, and complex chronic care. The case study included an environmental scan of existing policies and procedures related to medical and non-medical cannabis use at the LTC facility, a quantitative survey of HCPs’ knowledge, attitudes, and practices related to medical and non-medical cannabis, and qualitative interviews with HCPs and administrators. The qualitative interviews were informed by qualitative descriptive methodology [ 32 ] and explored HCPs’ and administrators’ experiences, beliefs, perceptions regarding cannabis use in LTC, and the related barriers and facilitators.
Sample and recruitment
For the survey, a convenience sample was drawn from the entire population of accredited HCPs working in the selected facility. Eligibility criteria included being 18 + years, able to read/speak English, currently employed and providing care at the LTC facility, and able to provide informed written consent. Study participants were recruited through an emailed letter of invitation, posters placed in staff areas, and in-person presentations by a research assistant. From participants who took part in the survey, a subsample of HCPs, including administrators, who expressed interest in taking part in an interview was selected. The data collection period was from November 2021 and August 2022.
Data collection
For the environmental scan, facility administrators were approached via an emailed letter and asked to identify relevant policies and procedures related to cannabis use within their LTC facility. Policies relevant to both residents’ use of cannabis and HCPs’ practice related to medical and non-medical cannabis were requested. Provincial and federal cannabis policies were also collected.
The survey was modified from a questionnaire utilized in two national studies that examined Canadian physicians’ and nurse practitioners’ knowledge, attitudes, and perceptions of the associated barriers and facilitators related to medical cannabis use, as well as their preferences regarding medical cannabis education [ 33 , 34 ]. This survey has been found to be internally consistent, with Cronbach’s alphas of 0.70 to 0.92 reported across subscales [ 33 , 34 ]. Slight word changes were made to reflect the fact that individuals living in LTC facility are referred to as residents, not patients, and the name of the facility was used to orientate the questions towards HCPs’ attitudes and practices related to cannabis use within the LTC setting.
Survey items were added that assessed HCPs’ practices related to addressing residents’ and family members’ questions about cannabis, as well as requests for medical cannabis authorization and follow-up care. A demographic survey that assessed gender, age, professional designation, years in practice, area(s) of practice, and education related to medical cannabis was included. The survey was available in hard copy (Supplementary Material 1 ) as well as online through the software program, Qualtrics®.
An interview guide was developed by the research team, which included a facility administrator and HCP, and was informed by the literature and previous cannabis research conducted by members of the research team [ 35 ] (Supplementary Material 2 ). Due to the COVID-19 pandemic, all but one interview was conducted by the project coordinator (AAA) via Zoom, with one interview occurring over the phone. The interviews were 20–30 min in length and were digitally recorded and transcribed verbatim. Both the survey and interview were completed at times preferred by the respondents, including within and outside work time. No honoraria were provided for study participants.
Data analysis
The policies identified through the environmental scan were reviewed and summarized in table format, with similarities, contradictions and gaps identified.
Quantitative survey data was uploaded into the statistical program, SPSS® v.25. Descriptive statistics were used to summarize demographic information, knowledge about medical cannabis and related attitudes, perceived barriers and facilitators, practice experiences, and preferred educational approaches.
Perceived knowledge gap was calculated by computing the difference between perceived current and desired knowledge levels (i.e., “the level of knowledge you desire” about medical cannabis). Rather than using averages, the knowledge gap was calculated based on how much greater an individual’s desired knowledge level was compared to their current knowledge level [ 36 ]. Only response pairs (i.e., current and desired knowledge) were used, and responses where the desired level was lower than the current level were excluded. To further elucidate, the knowledge gap was calculated by having each respondent’s current knowledge level response subtracted from their desired knowledge level response.
Prior to the onset of qualitative data analysis, the accuracy of the transcripts was checked by listening to the digital recordings. Content analysis was used to analyze the qualitative data [ 37 ], with two team members (AAA and LGB) independently reading the transcripts and developing a preliminary coding scheme. Constant comparison of new and existing data ensured consistency, relevance, and comprehensiveness of emerging codes. Several strategies were applied to ensure rigour in the qualitative analysis. To increase credibility, a team member with expertise in qualitative inquiry (LGB) monitored the qualitative data and its analysis. Confirmability was addressed by using the participants’ own words throughout the process of data analysis, interpretation, and description. An audit trail was kept documenting the activities of the study, including data analysis decisions.
Environmental scan of cannabis-related policies
Administrators at the LTC facility provided the research team with the policies and procedures that addressed the management and use of medical and non-medical cannabis within the facility. The guiding policy adopted by the LTC facility was a generic policy applicable to all sites and facilities governed by a regional health authority. This policy, entitled “Patient Use of Medical Cannabis (Marijuana)” was issued in June 2020. The policy, which aimed to provide individuals with “reasonable access to medical cannabis”, outlined numerous issues that might arise with institutional cannabis use, including “ordering, labeling, packaging, storage, security, administration, documentation and monitoring requirements for the use of medical cannabis”. Key aspects of the policy are summarised in a table found in the Supplementary Material section (Supplementary Material 3 ).
Other relevant policies that were reviewed included the standards of practice issued by the provincial college of nurses and the college of physicians and surgeons [ 38 , 39 , 40 ], which provided direction to HCPs working in LTC about their scope of practice regarding medical and non-medical cannabis. The regional health authority’s smoke-free policy [ 41 ] also informed how inhaled forms of medical and non-medical cannabis were addressed, requiring residents to leave the facility grounds to smoke or vape cannabis. Lastly, the overarching federal Cannabis Act and Regulations provided guidance to both administrators and HCPs regarding the Canadian regulations specific to medical and non-medical cannabis [ 1 , 42 ]. Together, existing facility, regional, and national policies created a context in which cannabis was framed as neither a medicine nor a controlled substance, but something unique and complex that must be navigated by residents, family members and staff in LTC settings.
Quantitative survey
Demographic characteristics.
From the approximately 318 eligible HCPs employed at the LTC facility, a total of 71 participants consented and completed the survey, yielding a response rate of 22.3%. With regards to response rate by profession, pharmacists (50.0%) and social workers (42.9%) were best represented, followed by physicians (23.1%), nurses (21.0%), and PT/OT (11.4%).
Most respondents were women (71.8%), registered nurses (62.0%) and worked within the palliative care unit (76.1%) at the facility. The average age of the sample was 40.9 years and the largest proportion of the sample had worked in the LTC facility for 5 or less years. See Table 1 for additional details.
Knowledge about medical cannabis
HCPs reported being most knowledgeable about the therapeutic potential of cannabis (3.1/5.0), potential risks of medical cannabis (2.9/5.0), and the different ways to administer medical cannabis (2.9/5.0). They reported being least knowledgeable about the dosing of medical cannabis (2.0/5.0), how to create effective treatment plans related to medical cannabis (2.1/5.0), and the similarities and differences between different forms of cannabis products and prescription cannabinoid medications (2.2/5.0). The top three ranked knowledge gaps mirrored the items ranked lowest with regards to knowledge (see Table 2 ). Overall, there was high interest in gaining more medical cannabis knowledge, with all knowledge items scoring greater than 4 on desired knowledge level.
Practice experiences with medical cannabis
About half of HCPs reported providing care in the past month to a resident who was taking medical cannabis (54.9%) and a quarter (25.4%) to a resident that was taking non-medical cannabis. Over 60% had been approached by a resident and/or a family member to discuss the potential use of medical cannabis; however, few HCPs reported initiating these conversations. Moreover, when asked if they felt comfortable discussing medical cannabis, 32.4% of HCPs disagreed (data not shown). Less than 20% reported helping residents, either directly or indirectly, to use medical cannabis and a very small proportion (1.3–2.8%) reported assisting residents’ consumption of non-medical cannabis. With regards to authorizing the use of medical cannabis or prescribing cannabinoid medication, which in Canada can be done by either a physician or nurse practitioner, just over half of physicians reported supporting residents’ access to these types of treatment. See Table 3 for additional details.
Barriers to medical cannabis use in long-term care
Lack of knowledge, education or information about medical cannabis were reported to be barriers to medical cannabis use in LTC by most HCPs (81.7%). Moreover, the uncertain risks and benefits of medical cannabis and the lack of clinical guidelines were also perceived as barriers by 66.2% and 63.4% of HCPs, respectively. The complete list of barriers is presented in Table 4 .
Education about medical cannabis
Most of the HCPs agreed that additional education on medical cannabis would increase their comfort with discussing this treatment option with residents and family members (87.4%; data not shown). With regards to indirectly or directly administering medical cannabis to a resident, most HCPs for which this fell within their scope of practice also reported they would feel more comfortable if they had further education (59.2% and 56.4%, respectively; data not shown).
Over half of HCPs had not received any prior education related to medical cannabis (54.9%). Those that had, received it from conferences or workshops (65.6%), books or journal articles (43.8%) or through a colleague (37.5%). While almost half the sample (49.3%) reported receiving information from peer-reviewed sources, nearly a quarter received information about medical cannabis from a non-peer reviewed source or from a resident or family member. Some participants also received information from a cannabis industry source. Table 5 provides additional details.
The preferred sources of medical cannabis education were online learning programs (i.e., continuing education) (74.6%), monographs (66.2%), and topic-specific one-pagers (64.8%). See Fig. 1 for further details.
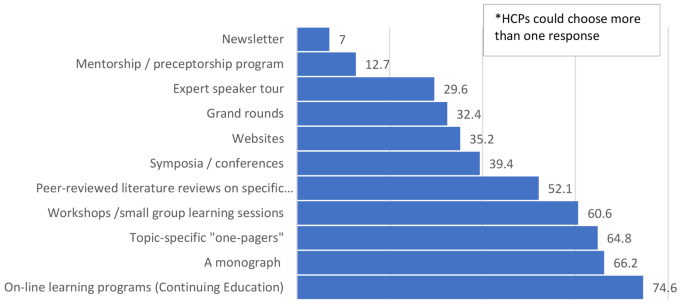
Percentage of respondents indicating prefered method of cannabis education*
Qualitative findings
A total of 12 HCPs were interviewed regarding their perceptions and experiences related to medical and non-medical cannabis in the LTC facility. This included 3 HCPs who were administrators, 6 nurses, 1 physician, 1 social worker and 1 pharmacist. Four main themes were identified.
Attitudes regarding medical cannabis: cautious support
There were mixed attitudes regarding the potential role of medical cannabis in general and in LTC populations. While some HCPs felt medical cannabis was a “good idea” for which there was beginning research regarding its health benefits, other HCPs believed additional high-quality evidence was needed prior to medical cannabis becoming a therapeutic option.
I think it’s [medical cannabis] the fair option, it helps some people, but it doesn’t help others. So, I think we need a bit more evidence and a bit more research and having it available sort of allows for that research to occur (Physician; PC07).
There appeared to be greater acceptance for medical cannabis use by individuals at end of life compared to those not considered immediately palliative (i.e., living with dementia, stroke, or traumatic brain injury), the latter of which comprise the majority of the people living in LTC settings. For individuals receiving palliative care, some HCPs perceived medical cannabis to be beneficial in managing pain, nausea, and anxiety, as well as reducing the use of other medications that may be problematic (e.g., opioids) due to their side effects. The potential value of medical cannabis in “adding quality of life and living” at the end of life was also mentioned.
I’m working on the palliative care unit right now. A lot of patients that I’ve seen use it [medical cannabis] for anxiety purposes, or for nausea… some people find beneficial. So, I’ve seen it – people find it helpful for those reasons, and then they have to take less of their other medications. So, if it’s worked well for them and that’s what they prefer to do, then I think it should be an option for people, especially if some people find it beneficial. (Registered nurse; PC03)
Within the context of LTC, several HCPs also spoke of the importance of respecting residents’ autonomy and previous experiences taking medical cannabis. The reality of a LTC facility being a resident’s “home” was particularly influential in HCPs’ support of medical cannabis being included as part of a holistic approach to care.
I guess because people live at [LTC facility’s name], that is their home and if they were at home in the community, they would be able to access it [medical cannabis]. (Registered nurse, PC02)
I think it’s a part of people’s lives. And I think if we’re allowing people to have certain things and keeping it as part of their treatment because if you look at a holistic view, preventing somebody from doing something that they’ve been doing for many years is not going to help them be accepting of other types of therapies. (Pharmacist, PC09)
Some HCPs also perceived medical cannabis as offering an alternative to medical treatments that were not consistently effective in managing challenging health conditions, such as dementia and agitation.
HCPs’ attitudes towards medical cannabis varied across different products and routes of administration. Given the existing smoke-free policy at the facility, HCPs were more supportive of edibles, oils, oral sprays or topical creams and lotions than any form of inhaled medical cannabis (i.e., smoking and vaping). They were concerned not only about lung health, environmental exposure, and maintaining a scent-free facility, but also about how to safely manage vulnerable residents travelling off the facility’s property to smoke or vape.
Medical cannabis access and use: concern, confusion, and limited conversations
According to HCPs interviewed, most residents using medical cannabis obtained their authorization prior to moving to LTC. Individuals who sought authorization after arriving at the facility struggled to have their requests acknowledged or addressed by the health care team. As one nurse shared:
I do remember I had a resident that did ask about it [medical cannabis]. And whenever it was kind of brought up, it didn’t seem to be acknowledged all the time. Or there were people who didn’t like the idea of having a resident on it. (Registered nurse; PC06)
Conversations about medical cannabis were perceived to be severely limited by the culture surrounding medical cannabis at the LTC facility. The lack of open discussion about medical cannabis was seen by some to create conflict and negatively impact the development of trust between residents, family members, and the health care team: “ Without that discussion, it does create conflict within the team and between the physician and family, and perhaps that could impact the trusting relationship” (Administrator; PA03). Further, several HCPs expressed the belief that conversations about non-pharmacological forms of medical cannabis could not be initiated by them due to policy issues; residents who expressed interest but did not have prior authorization were instead directed towards pharmaceutical forms of cannabis.
There have been residents who have asked about using cannabis. And as I said, you can’t initiate it, if they’re going to get it on their own, fair enough. That’s pretty much been the experience I’ve had with residents with just non-pharmaceutical medical cannabis . (Physician, PC07)
The only HCP-initiated conversations about medical cannabis mentioned were those occurring between pharmacists and residents, which focused on the potential side effects, benefits, and “red flags” to watch out for, such as allergic reactions.
HCPs shared that for those residents with authorization, they or a support person were responsible for ordering the medical cannabis product from an LP, which would then send the product to either the resident at the LTC facility or to their support person’s home. The cannabis product was then stored in a locked drawer in the resident’s room if they were self-administering or in a medication room if nursing staff were assisting with administration. According to one pharmacist, the pharmacy department was not permitted, due to existing federal regulations, to either directly order or dispense medical cannabis:
No, we don’t dispense any cannabis. It’s considered resident’s own. So, we don’t acquire it for them. They have to directly be the holders of it and have it provided to them directly. And I think that has more to do with the regulations within Canada, the resident has to have certain type of documentation in order to have medical cannabis. So, it’s directly to them, we’re not able to order it for them or anything like that on their behalf. (Pharmacist; PC09)
With regards to the type of medical cannabis products permitted in the facility, due to non-smoking policies and concerns about safety issues and the “smell”, combustible forms and inhaled routes of administration (i.e., joints, vaporizers, vape pens) were not allowed; instead, ingestible forms were mentioned most frequently by HCPs.
There was some confusion and concerns expressed regarding the storage and disposal of medical cannabis, which may have reflected changes in facility policies over time. Some HCPs expressed concerns about the storage of cannabis in residents’ rooms and the lack of “safeguards” to limit potential diversion and allow an accurate “count” of medical cannabis.
We have to go into our Pyxis machine to retrieve a key to open that drawer. So, by going by that you’re able to know who’s actually accessed the key, but once the key is out you have no idea how many people have used that key and accessed that drawer before it’s gone back. You have no way of knowing how much cannabis has been taken out [of the drawer] or used, because you know there’s no way to measure it. So that’s a huge problem, I find. (Registered nurse; PC01)
This nurse was particularly concerned about the potential risk of being accused of diversion:
I’m not worried about people abusing it, it’s more the worry of being accused. You know, like, if a resident says, ‘why is my cannabis running out already, I thought I had enough for a few more weeks?’ and we’re like, ‘I don’t know’, right? There’s the potential for that sort of thing to happen. (Registered nurse; PC01)
There was also a perception that there was a lack of direction from the facility regarding the appropriate disposal of medical cannabis. Most believed residents or family members were expected to remove any unused product once the resident was no longer at the facility. When such disposal was not possible, the policy was to destroy the cannabis product in a manner similar to narcotics or other controlled substances. However, variations in practice occurred with some HCPs described “throwing it in the trash” or using a medical waste disposal bin with or without a witness.
Barriers to medical cannabis use: safety, stigma and lack of knowledge
Numerous barriers to the use of medical cannabis by LTC residents were identified by HCPs. Foremost, the policies related to how cannabis products were ordered, accessed, stored, and administered were perceived to be complicated and created barriers to residents wanting to take medical cannabis, particularly those without family support. The inability of the LTC facility to order medical cannabis on behalf of a resident was perceived to be especially problematic, as described by one registered nurse:
I know when it became legal, there were a few residents who have inquired about it, but they didn’t have the family resources in place to be able to get it because I believe there’s some hoops that you have to go through to be able to have it medically prescribed in getting it on to the unit. And so, the ones who were interested in it didn’t have those supports in place, so they weren’t able to get it prescribed for them. (Registered nurse; PC05)
The lack of awareness and understanding of the regional policies related to medical cannabis by some of the clinical staff was also seen as being problematic. As one registered nurse shared:
My only concern is that there’s a lot of rules around being able to administer and how it’s [medical cannabis] administered, which can again make things a bit complicated. I would say that’s probably my biggest concern is just it’s hard to remember everything that you have to do when you’re trying to administer it or helping a resident. So, you don’t get involved. (Registered nurse; PC06)
Several HCPs attributed the lack of awareness about cannabis policy to the onset of the COVID-19 pandemic, which overshadowed all other health issues within their facility: “ Everybody’s been so focused on COVID for a year and a half that there hasn’t been really time to really think about or educate on other things. ” (Registered nurse; PC01).
HCPs suggested that more “straight forward” and tailored policies were needed that simplified how medical cannabis was managed. Having facility-specific policies would acknowledge the uniqueness of the LTC population, who may have cognitive impairment, limited social support, and complex healthcare plans. As one nurse shared: “ If it’s a dementia patient, they can’t really administer it on their own. So how do we follow the policy to help the patient take the cannabis? How would we know when they would want to take it PRN?” (Registered nurse; PC03). It was also recommended that the policy that prevented the facility from directly ordering and supplying medical cannabis required revision so that LTC residents were not reliant on family members to gain access. Lastly, several HCPs suggested that medical cannabis policies need to be well advertised and additional training developed for clinical staff to enhance their awareness and comfort level in providing appropriate and supportive care.
There needs to be a training session… staff have to read through them [cannabis policies] and get instructions about them, sort of like a self-learning activity. But that is not part of what we do when orienting. (Registered nurse; PC02)
Another perceived barrier frequently mentioned by HCPs was their lack of knowledge regarding the potential risks and benefits of medical cannabis. There was limited understanding about the effects of medical cannabis, how it may interact with other medications and health conditions, what side effects could arise, as well as basic information about starting dose, titration, and difference between THC and cannabidiol (CBD). Without such information, HCPs were perceived to be very hesitant about recommending or supporting medical cannabis as a treatment alternative for LTC residents:
There’s lots of unknown, that’s the problem. If there were more specifics about the recreational and the medical use of cannabis, then I think health care professionals would be more likely to want to provide it to the residents. But if not, then that’s kind of what’s hindering health care professionals to provide it. (Registered nurse; PC08)
There was also substantial discussion by HCPs regarding the “stigma” that they perceived to exist within the facility regarding medical cannabis. As described by one pharmacist: “ I think the understanding of cannabis, regardless of if it’s medical or anything, it’s still considered in many people’s minds as an illicit drug. It hasn’t shaken that. And I think there’s a lot of stereotypes around the type of people that use cannabis” (Pharmacist; PC09). The stigmatization of medical cannabis was perceived to be particularly pronounced among the medical staff, which led to what was described as a “hands-off approach” with regards to authorizing medical cannabis.
Almost all HCPs and administrators interviewed recommended that education programming and resources for HCPs be developed to address the lingering stigma associated with cannabis and the knowledge gaps that exist about medical cannabis and associated policies. Several participants recommended that education initiatives should first target physicians, who were responsible for authorizing medical cannabis in the facility. Physicians were perceived to need education on when and for whom medical cannabis would be appropriate, the latest evidence regarding efficacy and safety (i.e., drug interactions), and what their obligations and responsibilities were as the authorizing HCP. Participants also thought that all HCPs could benefit from additional training regarding medical cannabis, including the different types of cannabinoids and products, the process of titration, and dosing. Some of the nurses interviewed also expressed the need for education about the legal implications of medical cannabis and their role regarding provision and administration:
I think the legal implications of cannabis use, I think that would be a good focus for the nursing group – so that they understood what their obligations were, what they could be held accountable for, those kinds of things. (Administrator; PA02)
Finally, numerous HCPs spoke of the need for “safeguards” and clear policies and procedures to ensure that clinical staff were aware of what type of medical cannabis products residents were taking, what was the “right dose”, and the possibility of cannabis interacting with other medications. As shared by one pharmacist:
So that we know that this patient is on it because there are potential drug interactions with other things that patients are taking. So, we just have to be cautious and aware that patients are doing this. Because especially right now with studies, there haven’t been a lot of great studies on drug interactions. (Pharmacist; PC09)
Non-medical cannabis use: balancing autonomy and safety
HCPs were asked about their attitudes and experiences about residents’ use of non-medical cannabis in the facility. Two disparate points of view became apparent – those that perceived non-medical cannabis as a legal substance that should be available to LTC residents given the facility was their home and those that saw non-medical cannabis as a stigmatized substance that could lead to problematic use and disruptions in the care environment.
Because it is somebody’s home and so you’re trying to honour and match what their lifestyle and aspects of their life at home were and matching that here [LTC facility]. The bad is, while it is somebody’s home, it’s the next person’s home too, and so it’s trying to balance that, right? In an institutional setting, trying to make it as home-like as possible but, at the same time, you know, monitoring and matching for what everyone’s needs are. (Registered nurse; PA01)
Professionally, I think that it creates issues in terms of trying to police the use of recreational cannabis. In terms of smoking cigarette tobacco, that’s an issue in itself. We’re a non-smoking facility. So, adding cannabis to the mix creates issues…having staff perhaps exposed or other people exposed if people are using cannabis indoors or where they’re not supposed. Or if they want to access and use cannabis outside, who’s going to take them for that? Because that creates exposure too for staff or others who may have to escort them. (Registered nurse; PA03)
HCPs frequently mentioned the complexity of managing residents’ non-medical use of cannabis given the facility’s non-smoking policy that required residents to leave the facility grounds to use inhaled forms of cannabis. With staff unable to transport residents outside, concerns were raised regarding the safety of residents, particularly in the winter months, and who would be responsible for their transfer in and out of the facility as well as monitoring how much cannabis was consumed. In addition, residents’ access to non-medical cannabis was again dependent on having a support person that was able and willing to transport the product to the facility, posing a potential equity issue for some residents:
If someone’s wanting to go smoke outside, then mobility might be an issue. If they don’t have the right wheelchair or family to take them outside for that. If they have the access. Like, if they need family to go and buy it and bring it to them, that could be more of an access issue depending on their family support. (Registered nurse; PC03)
There was specific concern expressed for individuals in the rehabilitation units who may have pre-existing substance use issues. For these individuals, HCPs were concerned that allowing access to non-medical cannabis could add to an already complex care plan. In addition, with many vulnerable residents living at the facility, concerns were raised regarding them being “incredibly suggestible” to others encouraging their consumption of cannabis:
These people – they have an addiction. For sure they’re making choices, but those choices are influenced by physical withdrawal or influenced by stress; they’re influenced by lots of things. So, I would hate to put residents in a position where that was one other [non-medical cannabis] thing they had to contend with during the rehab stay. (Administrator, PA02)
The use of cannabis for therapeutic and recreational purposes is becoming more prevalent within older adult populations, both in the community as well as within healthcare institutions. There has also been growing interest in the possible role of medical cannabis for select chronic, rehabilitative, and palliative health conditions, frequently found among individuals residing within LTC settings. LTC facilities, thus, face the complex practice and policy implications associated with a substance that has been surrounded in controversy for close to a century. This case study is among the first to explore in one LTC facility in Western Canada how cannabis use is being addressed following the legalization of non-medical cannabis products, and what challenges exist. It provides an important snapshot of the complexities surrounding cannabis use in LTC and a foundation for future research.
Cannabis use in LTC settings: a clash of cultures
One challenge experienced by people residing in LTC facilities is the tension that exists between social and medical models of care that most facilities are founded on. Historically, LTC facilities have operated as what Goffman [ 43 ] termed “total institutions”, places where every aspect of a person’s life was controlled by others, paternalism dominated, and the medical needs of people were what drove care practices. Aspects of the total institution still exist, as noted in this case study, whereby cannabis use is in the control of the HCPs; it is dispensed during medication administration times rather than being freely available for use by the resident when they so desire as would be in a person’s home. In trying to create more home-like environments and meet the broad range of social and emotional needs of residents, resident-centred care practices and relational models of care have emerged [ 44 ]. Within this milieu, resident autonomy and choice are at the forefront and HCPs are there to assist, rather than take control of residents’ daily lives. In the most ideal settings, behaviours that are considered ‘risky’, like alcohol consumption, are treated as social experiences, not care tasks to be managed [ 45 ]. The tension arises, however, that despite the desire to be resident-centred, most LTC facilities are highly regulated by governments, putting limits to resident choice and, therefore, their autonomy [ 45 ]. While HCPs in our study acknowledged that residents should have the right to use medical or non-medical cannabis, the regional and institutional policies surrounding safety and the rights of other residents and staff to not be exposed to potentially risky behaviour underscored many of their views. LTC facilities would be wise to consider the principles of dignity of risk [ 46 ] with relation to cannabis consumption/use along the frail elderly population that reside in the home.
Cannabis policies and LTC: one size doesn’t fit all
The cannabis policies developed at the advent of legalization, without consideration of the unique populations and healthcare challenges that exist within LTC facilities, created numerous barriers to residents accessing and using cannabis, as well as for HCPs attempting to provide appropriate care. One of the most significant challenges experienced by LTC residents in our study was the inability to obtain a medical cannabis authorization from a physician working in the facility. Another significant challenge was the regional policy that medical cannabis could not be couriered directly to the LTC pharmacy; instead, the resident or their support persons were responsible for ordering and bringing cannabis products into the facility. Both challenges created enormous inequity in which residents that lacked the physical and cognitive ability to obtain authorization and order medical cannabis from an LP or were without a support person willing and able to obtain medical cannabis on their behalf, were unable to access medical cannabis. Given the nature of LTC populations, these policies led to only a few residents being able to access and use medical cannabis as part of their care.
Another policy that had substantial safety implications for residents wanting to use inhaled forms of cannabis was the regional and institutional no smoking policies that prevented both tobacco and cannabis products from being consumed within the centre as well as on the grounds. As a result, residents had to make their own way, or be accompanied by a support person, to walk approximately 300 m to the public sidewalk where they were allowed to smoke or vape cannabis. With the LTC facility located in a region where winter temperatures can reach − 35 Celsius and sidewalks are covered in snow and ice, this poses significant risk for residents who may be at heightened risk of falls and utilizing assisted walking devices. Similar safety implications of smoke-free policies have been identified in previous research [ 47 ].
Lastly, the policies surrounding the storage and self-administration of medical cannabis for those residents with the physical and emotional capacity (or with a support person willing to administer) may pose potential safety and liability risks and contribute to the concerns held by some HCPs about the use of cannabis in LTC. While residents’ autonomy must be respected, as well as their own expertise with regards to medical cannabis use, the value of standardized medication protocols to ensure the safety of residents as well as to inform care decisions must be acknowledged. The tension experienced in balancing LTC residents’ autonomy with health and safety concerns in the context of substance use has been cited in a recent scoping review [ 48 ] as well as prior research that has examined the use of tobacco in residential care settings [ 49 ].
The policy-related challenges identified by study participants suggest that consultations with LTC residents, families and HCPs are urgently needed to develop and refine cannabis policies that address the needs and reality of individuals living and receiving care in LTC. Future policy reviews must balance LTC residents’ autonomy with the safety issues associated with cannabis use (i.e., dignity of risk), particularly among older adults and those with cognitive and physical impairments. Approaching cannabis policies and procedures in LTC from a harm reduction perspective [ 50 ] with regards to supporting safer consumption of medical cannabis (e.g., route of administration, designated consumption areas) may also be important. Further, the unique context of LTC must also be acknowledged in that for many residents, a LTC facility is their home, and will continue to be so until the end of their lives. But the shared nature of a LTC setting requires that some boundaries be established to protect all residents, as well as those working within LTC. From a staff perspective, a review of policies related to the administration and documentation of cannabis use is needed to protect them from claims of diversion as well as other medicolegal challenges.
Cannabis knowledge gap and stigma in LTC
Across both the quantitative and qualitative data, the gap in knowledge regarding cannabis and the need for continuing education for HCPs working in LTC were readily apparent. When HCPs are unfamiliar about the various forms of medical cannabis, appropriate dosing and titration schedules, and routes of administration, they are hindered in their ability to engage in shared decision making with LTC residents as well as provide high-quality care [ 51 , 52 , 53 , 54 ]. Education is particularly needed that is tailored to the unique risks and benefits of medical cannabis use among LTC populations, including those living with physical and cognitive impairment. Older adults may be more sensitive to the side effects of cannabis due to changes in how medications and drugs are metabolised, and the predominance of polypharmacy among those residing in LTC may further complicate how individuals respond to cannabis [ 55 ]. Therefore, HCPs working in LTC must be aware of how cannabis use may impact individuals’ mobility, memory, and behaviour, as well as the potential for dependency, particularly among those who have experienced substance use issues in the past.
Beyond basic education regarding cannabis and its effects, HCPs must also become aware and informed about existing federal, regional, and institutional policies as well as professional practice standards regarding both medical and non-medical cannabis. The study findings highlighted the uncertainty many HCPs experienced regarding how medical and non-medical cannabis was to be accessed, authorized, administered, stored, and disposed within the LTC facility and what was within their professional scope of practice. Legal concerns about liability, workplace safety, and diversion were also raised.
It is important that future cannabis education programs targeting LTC settings also address the underlying stigma and stereotypes that still surround cannabis use [ 56 , 57 ], despite the existence of a medical cannabis program in Canada for over 20 years and the recent legalization of non-medical cannabis. Experiential training that promotes non-judgmental communication that avoids stigmatizing language (e.g., user, addict, marijuana) and considers both the risks and benefits of cannabis use, particularly within the context of end-of-life care, will help address the stigma that HCPs and LTC residents and families may hold towards cannabis.
With the legalization of cannabis in many regions around the world, it is imperative that undergraduate health professional training programs include information about both medical and non-medical cannabis. Currently, there is a knowledge gap among HCPs due to the lack of standardized curriculum for medical cannabis across nursing or medical schools [ 35 , 58 ]. Understanding such foundational knowledge such as the endocannabinoid system, the different forms and types of cannabis, and the potential health effects will enable physicians, nurses, pharmacists and other HCPs to engage in informed conversations with individuals and families both within and beyond LTC [ 33 ]. In addition, the development of continuing education programs focused on cannabis will ensure practicing HCPs have current knowledge about cannabis, including existing policies and programs relevant to medical and non-medical cannabis. For example, the Canadian Coalition for Seniors’ Mental Health created asynchronous e-learning modules to provide evidence-based knowledge for various clinicians [ 59 ].
Non-medical cannabis use in LTC: it’s legal but…
Despite non-medical cannabis being a legal substance for over three years in Canada at the time of the case study, the use of non-medical cannabis by LTC residents was considered controversial amongst the HCPs interviewed. Not only were HCPs limited in their ability to support the use of non-medical cannabis due to regional policies that prohibited non-medical cannabis consumption at any healthcare facility and surrounding grounds but concerns about potential safety risks and disruptions to the care environment made some HCPs hesitant about supporting residents’ use of non-medical cannabis.
Notwithstanding these challenges, at least a quarter of HCPs surveyed reported providing care to a LTC resident who used non-medical cannabis, which suggests that regulatory and policy changes are required to ensure there is equity across LTC residents who may express interest in non-medical cannabis, as well as to address the unique safety and care issues associated with recreational cannabis use in LTC populations. Similar to medical cannabis, LTC residents’ autonomy must be considered in future policy changes related to non-medical cannabis to facilitate care that is free from stigma and bias, respects residents’ rights to make informed decisions and to live with risk, and to create a home-like environment where residents can engage in activities that were an important part of their lives before entering LTC.
Lessons can be drawn from literature that has examined the use of other legal substances, such as alcohol and tobacco in LTC [ 48 , 60 ], and the need to develop person-centered care plans that ensure the safety of the individual, fellow residents, and the healthcare team.
Limitations
Like all case studies, the findings cannot be extrapolated to other LTC settings and populations. Given that this study was undertaken in Canada, which has a socialized healthcare system and legalized both medical and non-medical cannabis, the experiences and attitudes of HCPs who participated may be unique and limit the generalizability of the findings. However, there are lessons to be learned regarding the challenges that residents in LTC facilities face in using medical and non-medical cannabis, as well as the potential need for both education and policy reform to better support HCPs in providing appropriate, safe, and person-centred care of LTC residents. In addition, the collection of both quantitative and qualitative data allowed triangulation during the data analysis and helped improved the rigor of the findings [ 61 ]. Recruitment and data collection for this study also occurred during the height of the COVID-19 pandemic. Therefore, the response rate was lower than desired and there was limited diversity among study participants with regards health profession designation. However, the proportion of physicians, nurses, pharmacists, and other allied health professions reflected the overall staff composition of the LTC facility.
Implications for future research
Beyond the policy and practice implications discussed earlier, the study findings also point to the urgent need for research focused on cannabis use among populations commonly found within LTC settings. The lack of evidence regarding the potential health effects of cannabis in the management of diseases such as dementia, arthritis, Parkinson’s, traumatic brain injury, and multiple sclerosis led many of the HCPs interviewed to be hesitant about authorizing and supervising cannabis use for LTC residents living with these conditions. While there is a growing number of studies being undertaken focused on medical cannabis, many are limited by their sample size and study design. It is only through high-quality clinical trials that evaluate the efficacy and safety of medical cannabis that a change in practice will occur.
Future medical cannabis research must also be developed in a manner that is inclusive of older adults and those living in LTC. The exclusion of such populations from clinical research has been previously identified as problematic [ 62 ], resulting in research findings that lack generalizability and pose challenges in determining the applicability of research to older adults who may be living with numerous co-morbidities and using multiple medications. While the inclusion of older adults in medical cannabis clinical trials may be more methodologically and ethically challenging, it will lead to evidence that will inform both future policies and practices.
Lastly, our case study offers insight into the reality and challenges of cannabis use by residents of one LTC facility. Additional research across different jurisdictions is needed to explore how LTC settings are addressing cannabis use and to learn from their experiences. We encourage the continued use of mixed methods study designs to ensure the experiences and perspectives of residents, family members and HCPs are captured alongside administrative data related to medical and non-medical cannabis use.
With the legalization of medical and non-medical cannabis in jurisdictions around the world, LTC facilities will be obligated to develop policies, procedures and healthcare services that are able to accommodate residents’ use of cannabis in a respectful and evidence-informed manner. Balancing the safety concerns against the potential therapeutic value of cannabis, as well as considering residents’ autonomy and the home-like environment of LTC, will be important considerations in how cannabis use is addressed and regulated. Our case study highlights the lack of knowledge, inequities, and stigma that continue to surround cannabis in LTC. There is an urgent need for research that not only explores the potential risks and benefits of cannabis, but also informs the development of more nuanced and equitable policies and education resources that will support reasonable and informed access to medical and non-medical cannabis for older adults and others living in LTC.
Data availability
The datasets generated and analysed during the current study are not publicly available due to the small sample size drawn from one health care facility but are available from the corresponding author on reasonable request.
Abbreviations
Cannabidiol
Healthcare provider
Long–term care
Tetrahydrocannabinol
Licensed Producer
Government of Canada. Cannabis Act (S.C. 2018, c.16). 2018. http://canlii.ca/t/5392s retrieved on 2019-10-07.
Sandhu HS, Anderson LN, Busse JW. Characteristics of canadians likely to try or increase cannabis use following legalization for nonmedical purposes: a cross-sectional study. CMAJ Open. 2019;7(2):E399–404.
Article PubMed PubMed Central Google Scholar
Statistics Canada. National Cannabis Survey, third quarter 2019. https://www150.statcan.gc.ca/n1/daily-quotidien/191030/dq191030a-eng.htm
Statistics Canada. Research to Insights: Cannabis in Canada. 2023. https://www150.statcan.gc.ca/n1/pub/11-631-x/11-631-x2023006-eng.htm
Lau N, Sales P, Averill S, Murphy F, Sato SO, Murphy S. A safer alternative: cannabis substitution as harm reduction. Drug Alcohol Rev. 2015;34(6):654–9.
Bobitt J, Qualls SH, Schuchman M, Wickersham R, Lum HD, Arora K, et al. Qualitative analysis of cannabis use among older adults in Colorado. Drugs Aging. 2019;36(7):655–66.
Article PubMed Google Scholar
Reynolds IR, Fixen DR, Parnes BL, Lum HD, Shanbhag P, Church S, et al. Characteristics and patterns of marijuana use in community-dwelling older adults. J Am Geriatr Soc. 2018;66(11):2167–71.
Baumbusch J, Sloan Yip I. Exploring new use of cannabis among older adults. Clin Gerontol. 2021;44(1):25–31.
Tumati S, Lanctôt KL, Wang RD, Li A, Davis A, Herrmann N. Medical cannabis use among older adults in Canada: self-reported data on types and amount used, and perceived effects. Drugs Aging. 2022;39(2):153–63.
Government of Canada. Data on cannabis for medical purposes. 2022. https://www.canada.ca/en/health-canada/services/drugs-medication/cannabis/research-data/medical-purpose.html
Cooper ZD, Abrams DI, Gust S, Salicrup A, Throckmorton DC. Challenges for clinical cannabis and cannabinoid research in the United States. J Natl Cancer Inst Monogr. 2021;2021(58):114–22.
Piomelli D, Solomon R, Abrams D, Balla A, Grant I, Marcotte T, et al. Regulatory barriers to research on cannabis and cannabinoids: a proposed path forward. Cannabis Cannabinoid Res. 2019;4(1):21–32.
O’Brien M, McDougall JJ. Cannabis and joints: scientific evidence for the alleviation of osteoarthritis pain by cannabinoids. Curr Opin Pharmacol. 2018;40:104–9.
Babson KA, Sottile J, Morabito D. Cannabis, cannabinoids, and sleep: a review of the literature. Curr Psychiatry Rep. 2017;19(4):23.
Hillen JB, Soulsby N, Alderman C, Caughey GE. Safety and effectiveness of cannabinoids for the treatment of neuropsychiatric symptoms in dementia: a systematic review. Ther Adv Drug Saf. 2019;10:2042098619846993.
Chagas MHN, Zuardi AW, Tumas V, Pena-Pereira MA, Sobreira ET, Bergamaschi MM, et al. Effects of cannabidiol in the treatment of patients with Parkinson’s disease: an exploratory double-blind trial. J Psychopharmacol. 2014;28(11):1088–98.
Shohet A, Khlebtovsky A, Roizen N, Roditi Y, Djaldetti R. Effect of medical cannabis on thermal quantitative measurements of pain in patients with Parkinson’s disease. Eur J Pain. 2017;21(3):486–93.
Article CAS PubMed Google Scholar
Herrmann N, Ruthirakuhan M, Gallagher D, Verhoeff NPLG, Kiss A, Black SE, et al. Randomized placebo-controlled trial of nabilone for agitation in Alzheimer’s disease. Am J Geriatr Psychiatry. 2019;27(11):1161–73.
Shelef A, Barak Y, Berger U, Paleacu D, Tadger S, Plopsky I, et al. Safety and efficacy of medical cannabis oil for behavioral and psychological symptoms of dementia: an open label, add-on, pilot study. J Alzheimer’s Dis. 2016;51(1):15–9.
Article CAS Google Scholar
Volicer L, Stelly M, Morris J, Mclaughlin J, Volicer BJ. Effects of dronabinol on anorexia and disturbed behavior in patients with Alzheimer’s disease. Int J Geriatr Psychiatry. 1997;12(9):913–9.
Cyr C, Arboleda MF, Aggarwal SK, Balneaves LG, Daeninck P, Néron A, et al. Cannabis in palliative care: current challenges and practical recommendations. Ann Palliat Med. 2018;7(4):463–77.
Pooyania S, Ethans K, Szturm T, Casey A, Perry D. A randomized, double-blinded, crossover pilot study assessing the effect of nabilone on spasticity in persons with spinal cord injury. Arch Phys Med Rehabil. 2010;91(5):703–7.
Wilsey B, Marcotte TD, Deutsch R, Zhao H, Prasad H, Phan A. An exploratory human laboratory experiment evaluating vaporized cannabis in the treatment of neuropathic pain from spinal cord injury and disease. J Pain. 2016;17(9):982–1000.
Article CAS PubMed PubMed Central Google Scholar
Grenier K, Ponnambalam F, Lee D, Lauwers R, Bhalerao S. Cannabis in the treatment of traumatic brain injury: a primer for clinicians. Can J Neurol Sci. 2019;47(1):11–7.
Minerbi A, Hauser W, Fitzcharles MA. Medical cannabis for older patients. Drugs Aging. 2019;36(1):39–51.
MacCallum CA, Russo EB. Practical considerations in medical cannabis administration and dosing. Eur J Int Med. 2018;49:12–9.
Bertram JR, Porath A, Seitz D, Kalant H, Krishnamoorthy A, Nickerson J, et al. Canadian guidelines on cannabis use disorder among older adults. Can Geriatr J. 2020;23(1):135–42.
Banerjee S. Multimorbidity–older adults need health care that can count past one. Lancet. 2015;385(9968):587–9.
Bom J, Bakx P, Rellstab S. Well-being right before and after a permanent nursing home admission. Health Econ. 2022;31(12):2558–74.
Calkins M, Brush J. Honoring individual choice in long-term residential communities when it involves risk: a person-centered approach. J Gerontol Nurs. 2016;42(8):12–7.
Yin R. Case study research and applications: design and methods. 6th ed. Thousand Oaks, CA: Sage; 2018.
Google Scholar
Sandelowski M. Whatever happened to qualitative description? Res Nurs Health. 2000;23(4):334–40.
Balneaves LG, Alraja A, Ziemianski D, McCuaig F, Ware M. A national needs assessment of Canadian nurse practitioners regarding cannabis for therapeutic purposes. Cannabis Cannabinoid Res. 2018;3(1):66–73.
Ziemianski D, Capler R, Tekanoff R, Lacasse A, Luconi F, Ware MA. Cannabis in medicine: a national educational needs assessment among Canadian physicians. BMC Med Educ. 2015;15:50–2.
Article Google Scholar
Balneaves LG, Alraja AA. Guarding their practice: a descriptive study of Canadian nursing policies and education related to medical cannabis. BMC Nurs. 2019;18(1):66.
Fox RD. Revisiting discrepancy analysis in continuing medical education: a conceptual model 1983. J Contin Health Prof. 2011;31(1):71–6.
Hsieh HF, Shannon SE. Three approaches to qualitative content analysis. Qual Health Res. 2005;15(9):1277–88.
College of Registered Nurses of Manitoba. Medical cannabis: Guidelines for Manitoba nurses. 2019. https://www.crnm.mb.ca/uploads/document/document_file_247.pdf?t=1549645011
College of Registered Nurses of Manitoba. Practice direction: RN(NP) authorization of medical cannabis. 2018. https://www.crnm.mb.ca/uploads/document/document_file_252.pdf?t=1538755351
The College of Physicians & Surgeons of Manitoba. Standard of practice: Cannabis for medical purpose. 2020. https://cpsm.mb.ca/assets/Standards of Practice/Standard of Practice Authorizing Cannabis for Medical Purposes.pdf.
Winnipeg Regional Health Authority. Smoke-Free Policy. 2014. https://wrha.mb.ca/files/wrha-policy-10-00-010.pdf
Government of Canada. Cannabis regulations (SOR/2018 – 144) [. 2018. https://laws-lois.justice.gc.ca/eng/Regulations/SOR-2018-144/index.html
Goffman E. Asylums: essays on the social situations of mental patients and other inmates. Oxford, England: Doubleday (Anchor); 1961.
White-Chu EF, Graves WJ, Godfrey SM, Bonner A, Sloane P. Beyond the medical model: the culture change revolution in long-term care. J Am Med Dir Assoc. 2009;10(6):370–8.
Chivers S. Make mine a double. In: Armstrong P, Daly T, editors. Exercising choice in long-term residential care. Montreal, QC: RR Donnelley; 2017. pp. 55–8.
Woolford MH, de Lacy-Vawdon C, Bugeja L, Weller C, Ibrahim JE. Applying dignity of risk principles to improve quality of life for vulnerable persons. Int J Geriatr Psychiatry. 2020;35(1):122–30.
Schultz ASH, Finegan B, Nykiforuk CIJ, Kvern MA. A qualitative investigation of smoke-free policies on hospital property. CMAJ. 2011;183(18):2085–6.
De Graaf L, Janssen M, Roelofs T, Luijkx K. Substance use and misuse of older adults living in residential care facilities: a scoping review from a person-centred care approach. Ageing Soc. 2021;43(6):1–27.
Adler G, Greeman M, Parker H, Kuskowski M. Self-determination and residents who smoke. J Social Work Long-Term Care. 2002;1(4):19–30.
Lau N, Sales P, Averill S, Murphy F, Sato SO, Murphy S. Responsible and controlled use: older cannabis users and harm reduction. Int J Drug Policy. 2015;26(8):709–18.
Clobes TA, Palmier LA, Gagnon M, Klaiman C, Arellano M. The impact of education on attitudes toward medical cannabis. PEC Innov. 2022;1:100009.
Croker JA, Bobitt J, Sanders S, Arora K, Mueller K, Kaskie B. Cannabis and end-of-life care: a snapshot of hospice planning and experiences among Illinois medical cannabis patients with a terminal diagnosis. Am J Hosp Palliat Med. 2022;39(3):345–52.
Hachem Y, Abdallah SJ, Rueda S, Wiese JL, Mehra K, Rup J, et al. Healthcare practitioner perceptions on barriers impacting cannabis prescribing practices. BMC Complement Med Ther. 2022;22(1):1–12.
Hope J, Schoonhoven L, Griffiths P, Gould L, Bridges J. I’ll put up with things for a long time before I need to call anybody: face work, the Total Institution and the perpetuation of care inequalities. Sociol Health Illn. 2022;44(2):469–87.
Williams AR. Ethical dilemmas in counseling older adults on cannabis use: less science, more quandaries. Generations J. 2020;44(4):1–5.
Skliamis K, Benschop A, Korf DJ. Cannabis users and stigma: a comparison of users from European countries with different cannabis policies. Eur J Crimin. 2022;19(6):1483–500.
Troup LJ, Erridge S, Ciesluk B, Sodergren MH. Perceived stigma of patients undergoing treatment with cannabis-based medicinal products. Int J Env Res Public Health. 2022;19(12).
Evanoff AB, Quan T, Dufault C, Awad M, Bierut LJ. Physicians-in-training are not prepared to prescribe medical marijuana. Drug Alcohol Depend. 2017;180:151–5.
Canadian Coalition for Seniors’ Mental Health. Cannabis and older adults. https://ccsmh.ca/cannabis-and-older-adults-project/
Grossi A, Holmes A, Ibrahim JE. Use of alcohol in long term care settings: a comparative analysis of personal choice, public health advice and the law. J Am Med Dir Assoc. 2021;22(1):9–e141.
Crowe S, Cresswell K, Robertson A, Huby G, Avery A, Sheikh A. The case study approach. BMC Med Res Methodol. 2011;11:100.
Crome P, Lally F, Cherubini A, Oristrell J, Beswick AD, Clarfield AM, et al. Exclusion of older people from clinical trials: professional views from nine European countries participating in the PREDICT study. Drugs Aging. 2011;28(8):667–77.
Download references
Acknowledgements
The authors would like to thank the healthcare professionals that graciously took the time to share their thoughts about cannabis use in long-term care settings. In addition, Ms. Sina Barkman, Chief Human Resources Officer, Riverview Health Centre, helped the research team navigate the complexity of conducting research in long-term care settings during the COVID-19 pandemic.
Funding for this study was received from the Riverview Health Centre Foundation.
Author information
Authors and affiliations.
College of Nursing, University of Manitoba, Winnipeg, MB, Canada
Lynda G. Balneaves, Abeer A. Alraja, Genevieve Thompson, Jamie L. Penner & Daniella Scerbo
Max Rady College of Medicine, University of Manitoba, Winnipeg, MB, Canada
Philip St. John
Riverview Health Centre, Winnipeg, MB, Canada
Joanne van Dyck
College of Nursing, Rady Faculty of Health Sciences, University of Manitoba, 89 Curry Place, Winnipeg, MB, R3T 2N2, Canada
Lynda G. Balneaves
You can also search for this author in PubMed Google Scholar
Contributions
“L.G.B, G.T, J.P and P.StJ. conceptualised the study. A.A.A. and D.S. engaged in recruitment and data collection activities. L.G.B. and A.A.A. analysed and interpreted the quantitative and qualitative data and developed a first draft of the manuscript, with assistance from G.T. All authors read and approved the final manuscript.”
Corresponding author
Correspondence to Lynda G. Balneaves .
Ethics declarations
Ethics approval and consent to participate.
Ethical approval for the study was obtained from the University of Manitoba Research Ethics Board (R1-2021:011 (HS24693)) and was approved by the Riverview Health Centre Research Committee. Implied consent was received from participants who completed the survey and written informed consent was obtained from all participants who completed an interview. We confirm that all methods were performed in accordance with the relevant ethical guidelines and regulations, (i.e., Tri-Council Policy Statement).
Consent for publication
Not applicable.
Competing interests
The authors declare no competing interests.
Additional information
Publisher’s note.
Springer Nature remains neutral with regard to jurisdictional claims in published maps and institutional affiliations.
Electronic supplementary material
Below is the link to the electronic supplementary material.
Supplementary Material 1
Rights and permissions.
Open Access This article is licensed under a Creative Commons Attribution 4.0 International License, which permits use, sharing, adaptation, distribution and reproduction in any medium or format, as long as you give appropriate credit to the original author(s) and the source, provide a link to the Creative Commons licence, and indicate if changes were made. The images or other third party material in this article are included in the article’s Creative Commons licence, unless indicated otherwise in a credit line to the material. If material is not included in the article’s Creative Commons licence and your intended use is not permitted by statutory regulation or exceeds the permitted use, you will need to obtain permission directly from the copyright holder. To view a copy of this licence, visit http://creativecommons.org/licenses/by/4.0/ . The Creative Commons Public Domain Dedication waiver ( http://creativecommons.org/publicdomain/zero/1.0/ ) applies to the data made available in this article, unless otherwise stated in a credit line to the data.
Reprints and permissions
About this article
Cite this article.
Balneaves, L.G., Alraja, A.A., Thompson, G. et al. Cannabis use in a Canadian long-term care facility: a case study. BMC Geriatr 24 , 467 (2024). https://doi.org/10.1186/s12877-024-05074-2
Download citation
Received : 11 July 2023
Accepted : 13 May 2024
Published : 29 May 2024
DOI : https://doi.org/10.1186/s12877-024-05074-2
Share this article
Anyone you share the following link with will be able to read this content:
Sorry, a shareable link is not currently available for this article.
Provided by the Springer Nature SharedIt content-sharing initiative
- Medical cannabis
- Non-medical cannabis
- Long-term care
- Residential care
- Older adults
BMC Geriatrics
ISSN: 1471-2318
- Submission enquiries: [email protected]
- General enquiries: [email protected]
Temporal and spatial evolution simulation and attribution analysis of vegetation photosynthesis over the past 21 years based on satellite SIF data: a case study from Asia
- Published: 06 June 2024
- Volume 196 , article number 597 , ( 2024 )
Cite this article
- Haixiang Si 1 ,
- Ruiyan Wang 1 , 2 &
- Xiaoteng Li 1
Photosynthesis in vegetation is one of the key processes in maintaining regional ecological balance and climate stability, and it is of significant importance for understanding the health of regional ecosystems and addressing climate change. Based on 2001–2021 Global OCO-2 Solar-Induced Fluorescence (GOSIF) dataset, this study analyzed spatiotemporal variations in Asian vegetation photosynthesis and its response to climate and human activities. Results show the following: (1) From 2001 to 2021, the overall photosynthetic activity of vegetation in the Asian region has shown an upward trend, exhibiting a stable distribution pattern with higher values in the eastern and southern regions and lower values in the central, western, and northern regions. In specific regions such as the Turgen Plateau in northwestern Kazakhstan, Cambodia, Laos, and northeastern Syria, photosynthesis significantly declined. (2) Meteorological factors influencing photosynthesis exhibit differences based on latitude and vertical zones. In low-latitude regions, temperature is the primary driver, while in mid-latitude areas, solar radiation and precipitation are crucial. High-latitude regions are primarily influenced by temperature, and high-altitude areas depend on precipitation and solar radiation. (3) Human activities (56.44%) have a slightly greater impact on the dynamics of Asian vegetation photosynthesis compared to climate change (43.56%). This research deepens our comprehension of the mechanisms behind the fluctuations in Asian vegetation photosynthesis, offering valuable perspectives for initiatives in environmental conservation, sustainability, and climate research.
This is a preview of subscription content, log in via an institution to check access.
Access this article
Price includes VAT (Russian Federation)
Instant access to the full article PDF.
Rent this article via DeepDyve
Institutional subscriptions
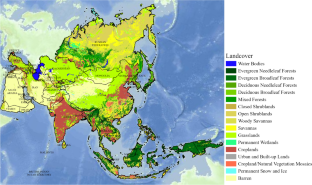
Data availability
No datasets were generated or analysed during the current study.
Andrade, A. M. D., Michel, R. F. M., Bremer, U. F., Schaefer, C. E. G. R., & Simões, J. C. (2018). Relationship between solar radiation and surface distribution of vegetation in Fildes Peninsula and Ardley Island, Maritime Antarctica. International Journal of Remote Sensing, 39 (8), 2238–2254. https://doi.org/10.1080/01431161.2017.1420937
Article Google Scholar
Ascensão, F., Fahrig, L., Clevenger, A. P., Corlett, R. T., Jaeger, J. A. G., Laurance, W. F., & Pereira, H. M. (2018). Environmental challenges for the Belt and Road Initiative. Nature Sustainability, 1 (5), 206–209. https://doi.org/10.1038/s41893-018-0059-3
Bai, X., Zhang, S., Li, C., Xiong, L., Song, F., Du, C., et al. (2023). A carbon-neutrality-capacity index for evaluating carbon sink contributions. Environmental Science and Ecotechnology, 15 , 100237. https://doi.org/10.1016/j.ese.2023.100237
Article CAS Google Scholar
Bashir, B., Cao, C., Naeem, S., ZamaniJoharestani, M., Bo, X., Afzal, H., et al. (2020). Spatio-temporal vegetation dynamic and persistence under climatic and anthropogenic factors. Remote Sensing, 12 (16), 2612. https://doi.org/10.3390/rs12162612
Cooper, M., & Messina, C. D. (2023). Breeding crops for drought-affected environments and improved climate resilience. The Plant Cell, 35 (1), 162–186. https://doi.org/10.1093/plcell/koac321
Dan, S., Li, H., Ping, L., & De, X. (2013). Effects of climate change on vegetation in desert steppe Inner Mongolia. Natural Resources, 04 (04), 319–322. https://doi.org/10.4236/nr.2013.44038
Estoque, R. C., Ooba, M., Avitabile, V., Hijioka, Y., DasGupta, R., Togawa, T., & Murayama, Y. (2019). The future of Southeast Asia’s forests. Nature Communications, 10 (1), 1829. https://doi.org/10.1038/s41467-019-09646-4
Fu, L., Cao, Y., Kuang, S.-Y., & Guo, H. (2021). Index for climate change adaptation in China and its application. Advances in Climate Change Research, 12 (5), 723–733. https://doi.org/10.1016/j.accre.2021.06.006
Ge, W., Deng, L., Wang, F., & Han, J. (2021). Quantifying the contributions of human activities and climate change to vegetation net primary productivity dynamics in China from 2001 to 2016. Science of the Total Environment, 773 , 145648. https://doi.org/10.1016/j.scitotenv.2021.145648
Green, J. K., Konings, A. G., Alemohammad, S. H., Berry, J., Entekhabi, D., Kolassa, J., et al. (2017). Regionally strong feedbacks between the atmosphere and terrestrial biosphere. Nature Geoscience, 10 (6), 410–414. https://doi.org/10.1038/ngeo2957
Hussain, S., Ulhassan, Z., Brestic, M., Zivcak, M., Zhou, W., Allakhverdiev, S. I., et al. (2021). Photosynthesis research under climate change. Photosynthesis Research, 150 (1–3), 5–19. https://doi.org/10.1007/s11120-021-00861-z
Kashyap, R., Kuttippurath, J., & Kumar, P. (2023). Browning of vegetation in efficient carbon sink regions of India during the past two decades is driven by climate change and anthropogenic intrusions. Journal of Environmental Management, 336 , 117655. https://doi.org/10.1016/j.jenvman.2023.117655
Li, X., & Xiao, J. (2019). A global, 0.05-degree product of solar-induced chlorophyll fluorescence derived from OCO-2, MODIS, and reanalysis data. Remote Sensing, 11 (5), 517. https://doi.org/10.3390/rs11050517
Li, A., Wu, J., & Huang, J. (2012). Distinguishing between human-induced and climate-driven vegetation changes: A critical application of RESTREND in inner Mongolia. Landscape Ecology, 27 (7), 969–982. https://doi.org/10.1007/s10980-012-9751-2
Li, P., Qian, H., Howard, K. W. F., & Wu, J. (2015). Building a new and sustainable “Silk Road economic belt.” Environmental Earth Sciences, 74 (10), 7267–7270. https://doi.org/10.1007/s12665-015-4739-2
Li, G., Chen, W., Zhang, X., Bi, P., Yang, Z., Shi, X., & Wang, Z. (2022). Spatiotemporal dynamics of vegetation in China from 1981 to 2100 from the perspective of hydrothermal factor analysis. Environmental Science and Pollution Research, 29 (10), 14219–14230. https://doi.org/10.1007/s11356-021-16664-7
Liu, X., Zhu, Z., Yu, M., & Liu, X. (2021). Drought-induced productivity and economic losses in grasslands from Inner Mongolia vary across vegetation types. Regional Environmental Change, 21 (2), 59. https://doi.org/10.1007/s10113-021-01789-9
Lu, C., Hou, M., Liu, Z., Li, H., & Lu, C. (2021). Variation characteristic of NDVI and its response to climate change in the middle and upper reaches of Yellow River Basin, China. IEEE Journal of Selected Topics in Applied Earth Observations and Remote Sensing, 14 , 8484–8496. https://doi.org/10.1109/JSTARS.2021.3105897
Ma, P., Zhao, J., Zhang, H., Zhang, L., & Luo, T. (2023). Increased precipitation leads to earlier green-up and later senescence in Tibetan alpine grassland regardless of warming. Science of the Total Environment, 871 , 162000. https://doi.org/10.1016/j.scitotenv.2023.162000
Meroni, M., Rossini, M., Picchi, V., Panigada, C., Cogliati, S., Nali, C., & Colombo, R. (2008). Assessing steady-state fluorescence and PRI from hyperspectral proximal sensing as early indicators of plant stress: The case of ozone exposure. Sensors, 8 (3), 1740–1754. https://doi.org/10.3390/s8031740
Misal, H., Hoare, V. H. C., & Miles, V. (2022). Responding to the climate crisis – Taking action on the IPCC 6th Assessment Report . Weather, 77 (4), 149–150. https://doi.org/10.1002/wea.4162
Mohammat, A., Wang, X., Xu, X., Peng, L., Yang, Y., Zhang, X., et al. (2013). Drought and spring cooling induced recent decrease in vegetation growth in Inner Asia. Agricultural and Forest Meteorology, 178–179 , 21–30. https://doi.org/10.1016/j.agrformet.2012.09.014
Mohammed, G. H., Colombo, R., Middleton, E. M., Rascher, U., van der Tol, C., Nedbal, L., et al. (2019). Remote sensing of solar-induced chlorophyll fluorescence (SIF) in vegetation: 50 years of progress. Remote Sensing of Environment, 231 , 111177. https://doi.org/10.1016/j.rse.2019.04.030
Nakamura, Y., Krestov, P. V., & Omelko, A. M. (2007). Bioclimate and zonal vegetation in Northeast Asia: First approximation to an integrated study. Phytocoenologia, 37 (3–4), 443–470. https://doi.org/10.1127/0340-269X/2007/0037-0443
Nanzad, L., Zhang, J., Tuvdendorj, B., Yang, S., Rinzin, S., Prodhan, F. A., & Sharma, T. P. P. (2021). Assessment of drought impact on net primary productivity in the terrestrial ecosystems of Mongolia from 2003 to 2018. Remote Sensing, 13 (13), 2522. https://doi.org/10.3390/rs13132522
Paul-Limoges, E., Damm, A., Hueni, A., Liebisch, F., Eugster, W., Schaepman, M. E., & Buchmann, N. (2018). Effect of environmental conditions on sun-induced fluorescence in a mixed forest and a cropland. Remote Sensing of Environment, 219 , 310–323. https://doi.org/10.1016/j.rse.2018.10.018
Piao, S., Cui, M., Chen, A., Wang, X., Ciais, P., Liu, J., & Tang, Y. (2011). Altitude and temperature dependence of change in the spring vegetation green-up date from 1982 to 2006 in the Qinghai-Xizang Plateau. Agricultural and Forest Meteorology, 151 (12), 1599–1608. https://doi.org/10.1016/j.agrformet.2011.06.016
Piao, S., Yue, C., Ding, J., & Guo, Z. (2022). Perspectives on the role of terrestrial ecosystems in the ‘carbon neutrality’ strategy. Science China Earth Sciences, 65 (6), 1178–1186. https://doi.org/10.1007/s11430-022-9926-6
Porcar-Castell, A., Tyystjärvi, E., Atherton, J., van der Tol, C., Flexas, J., Pfündel, E. E., et al. (2014). Linking chlorophyll a fluorescence to photosynthesis for remote sensing applications: Mechanisms and challenges. Journal of Experimental Botany, 65 (15), 4065–4095. https://doi.org/10.1093/jxb/eru191
Reichstein, M., Ciais, P., Papale, D., Valentini, R., Running, S., Viovy, N., et al. (2007). Reduction of ecosystem productivity and respiration during the European summer 2003 climate anomaly: A joint flux tower, remote sensing and modelling analysis. Global Change Biology, 13 (3), 634–651. https://doi.org/10.1111/j.1365-2486.2006.01224.x
Seddon, A. W. R., Macias-Fauria, M., Long, P. R., Benz, D., & Willis, K. J. (2016). Sensitivity of global terrestrial ecosystems to climate variability. Nature, 531 (7593), 229–232. https://doi.org/10.1038/nature16986
Song, L., Guanter, L., Guan, K., You, L., Huete, A., Ju, W., & Zhang, Y. (2018). Satellite sun-induced chlorophyll fluorescence detects early response of winter wheat to heat stress in the Indian Indo-Gangetic Plains. Global Change Biology, 24 (9), 4023–4037. https://doi.org/10.1111/gcb.14302
Srivastava, S., Mehta, L., & Naess, L. O. (2022). Increased attention to water is key to adaptation. Nature Climate Change, 12 (2), 113–114. https://doi.org/10.1038/s41558-022-01277-w
Stavi, I. (2023). Urgent reduction in greenhouse gas emissions is needed to avoid irreversible tipping points: Time is running out. All Earth, 35 (1), 38–45. https://doi.org/10.1080/27669645.2023.2178127
Su, Y., Yang, X., Gentine, P., Maignan, F., Shang, J., & Ciais, P. (2022). Observed strong atmospheric water constraints on forest photosynthesis using eddy covariance and satellite-based data across the Northern Hemisphere. International Journal of Applied Earth Observation and Geoinformation, 110 , 102808. https://doi.org/10.1016/j.jag.2022.102808
Tewari, V. P., Verma, R. K., & Von Gadow, K. (2017). Climate change effects in the Western Himalayan ecosystems of India: Evidence and strategies. Forest Ecosystems, 4 (1), 13. https://doi.org/10.1186/s40663-017-0100-4
Umuhoza, J., Jiapaer, G., Tao, Y., Jiang, L., Zhang, L., Gasirabo, A., et al. (2023). Analysis of fluctuations in vegetation dynamic over Africa using satellite data of solar-induced chlorophyll fluorescence. Ecological Indicators, 146 , 109846. https://doi.org/10.1016/j.ecolind.2022.109846
van Leeuwen, W. J. D., Orr, B. J., Marsh, S. E., & Herrmann, S. M. (2006). Multi-sensor NDVI data continuity: Uncertainties and implications for vegetation monitoring applications. Remote Sensing of Environment, 100 (1), 67–81. https://doi.org/10.1016/j.rse.2005.10.002
Wang, X., Wang, T., Xu, J., Shen, Z., Yang, Y., Chen, A., et al. (2022). Enhanced habitat loss of the Himalayan endemic flora driven by warming-forced upslope tree expansion. Nature Ecology & Evolution, 6 (7), 890–899. https://doi.org/10.1038/s41559-022-01774-3
Wang, Y., Liu, J., Wennberg, P. O., He, L., Bonal, D., Köhler, P., et al. (2023). Elucidating climatic drivers of photosynthesis by tropical forests. Global Change Biology, 29 (17), 4811–4825. https://doi.org/10.1111/gcb.16837
Wei, Z. F., Huang, Q. Y., & Zhang, R. (2019). Dynamics of vegetation coverage and response to climate change in China-South Asia-Southeast Asia during 1982–2013. Applied Ecology and Environmental Research, 17 (2), 2865–2879. https://doi.org/10.15666/aeer/1702_28652879
Wu, K., Chen, J., Yang, H., Yang, Y., & Hu, Z. (2023). Spatiotemporal variations in the sensitivity of vegetation growth to typical climate factors on the Qinghai-Tibet Plateau. Remote Sens, 15 , 2355. https://doi.org/10.3390/rs15092355
Zhang, Y., Gentine, P., Luo, X., Lian, X., Liu, Y., Zhou, S., et al. (2022). Increasing sensitivity of dryland vegetation greenness to precipitation due to rising atmospheric CO2. Nature Communications, 13 (1), 4875. https://doi.org/10.1038/s41467-022-32631-3
Zhang, R., Zhou, Y., Hu, T., Sun, W., Zhang, S., Wu, J., & Wang, H. (2023). Detecting the spatiotemporal variation of vegetation phenology in northeastern China based on MODIS NDVI and solar-induced chlorophyll fluorescence dataset. Sustainability, 15 (7), 6012. https://doi.org/10.3390/su15076012
Zhong, R., Wang, P., Mao, G., Chen, A., & Liu, J. (2021). Spatiotemporal variation of enhanced vegetation index in the Amazon Basin and its response to climate change. Physics and Chemistry of the Earth, Parts A/B/C, 123 , 103024. https://doi.org/10.1016/j.pce.2021.103024
Zhou, L., Tian, Y., Myneni, R. B., Ciais, P., Saatchi, S., Liu, Y. Y., et al. (2014). Widespread decline of Congo rainforest greenness in the past decade. Nature, 509 (7498), 86–90. https://doi.org/10.1038/nature13265
Zhu, L., Sun, S., Li, Y., Liu, X., & Hu, K. (2023). Effects of climate change and anthropogenic activity on the vegetation greening in the Liaohe River Basin of northeastern China. Ecological Indicators, 148 , 110105. https://doi.org/10.1016/j.ecolind.2023.110105
Download references
This research was funded by the Shandong Province Graduate Education Quality Improvement Plan (SDYAL21044) and the Funds of the Natural Science Foundation of Shandong Province (ZR2020MD003).
Author information
Authors and affiliations.
College of Resources and Environment, Shandong Agricultural University, Tai’an, 271018, China
Haixiang Si, Ruiyan Wang & Xiaoteng Li
National Engineering Research Center for Efficient Utilization of Soil and Fertilizer Resources, Shandong Agricultural University, Tai’an, 271018, China
Ruiyan Wang
You can also search for this author in PubMed Google Scholar
Contributions
Haixiang Si: conceptualization, methodology, validation, writing—original draft, writing—review and editing, formal analysis, visualization.
Ruiyan Wang: conceptualization, methodology, writing—original draft, formal analysis, visualization, writing—review and editing, supervision, project administration, funding acquisition.
Xiaoteng Li: writing—original draft, writing—review and editing, formal analysis, visualization.
All authors have read and agreed to the published version of the manuscript.
Corresponding author
Correspondence to Ruiyan Wang .
Ethics declarations
Ethical approval.
Not applicable, as this work did not involve humans or animals in any way, but only atmospheric dust.
Competing interests
The authors declare no competing interests.
Additional information
Publisher's note.
Springer Nature remains neutral with regard to jurisdictional claims in published maps and institutional affiliations.
Rights and permissions
Springer Nature or its licensor (e.g. a society or other partner) holds exclusive rights to this article under a publishing agreement with the author(s) or other rightsholder(s); author self-archiving of the accepted manuscript version of this article is solely governed by the terms of such publishing agreement and applicable law.
Reprints and permissions
About this article
Si, H., Wang, R. & Li, X. Temporal and spatial evolution simulation and attribution analysis of vegetation photosynthesis over the past 21 years based on satellite SIF data: a case study from Asia. Environ Monit Assess 196 , 597 (2024). https://doi.org/10.1007/s10661-024-12755-3
Download citation
Received : 29 January 2024
Accepted : 25 May 2024
Published : 06 June 2024
DOI : https://doi.org/10.1007/s10661-024-12755-3
Share this article
Anyone you share the following link with will be able to read this content:
Sorry, a shareable link is not currently available for this article.
Provided by the Springer Nature SharedIt content-sharing initiative
- Vegetation photosynthesis
- Solar-induced chlorophyll fluorescence
- Spatiotemporal variations
- Climate change
- Find a journal
- Publish with us
- Track your research
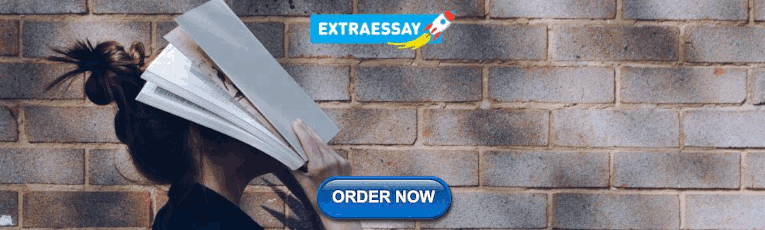
IMAGES
VIDEO
COMMENTS
Step 1: With Data Analytics Case Studies, Start by Making Assumptions. Hint: Start by making assumptions and thinking out loud. With this question, focus on coming up with a metric to support the hypothesis. If the question is unclear or if you think you need more information, be sure to ask.
4 Case Study Questions for Interviewing Data Analysts at a Startup. A good data analyst is one who has an absolute passion for data, he/she has a strong understanding of the business/product you are running, and will be always seeking meaningful insights to help the team make better decisions. Anthony Thong Do.
Data Analytics Case Studies in Oil and Gas 9) Shell Data Analyst Case Study Project. Shell is a global group of energy and petrochemical companies with over 80,000 employees in around 70 countries. Shell uses advanced technologies and innovations to help build a sustainable energy future.
Step 1: Clarify. Clarifying is used to gather more information. More often than not, these case studies are designed to be confusing and vague. There will be unorganized data intentionally supplemented with extraneous or omitted information, so it is the candidate's responsibility to dig deeper, filter out bad information, and fill gaps.
Inventory Analysis Case Study Instructor files: Instructor guide. Phase 1 - Data Collection and Preparation. Phase 2 - Data Discovery and Visualization. Phase 3 - Introduction to Statistical Analysis. Stay up to date.
A data analytics case study comprises essential elements that structure the analytical journey: Problem Context: A case study begins with a defined problem or question. It provides the context for the data analysis, setting the stage for exploration and investigation.. Data Collection and Sources: It involves gathering relevant data from various sources, ensuring data accuracy, completeness ...
Having a clear sense of purpose will guide your analysis. Gather Data: If the case study provides data, gather and organize it. This may involve cleaning and preprocessing the data, handling missing values, and converting it into a suitable format for analysis. Explore Data: Conduct exploratory data analysis (EDA) to gain insights into the data ...
There are 4 modules in this course. This course is the eighth and final course in the Google Data Analytics Certificate. You'll have the opportunity to complete a case study, which will help prepare you for your data analytics job hunt. Case studies are commonly used by employers to assess analytical skills. For your case study, you'll ...
Most of the time, you will be given a 30-45 min interview with a single data scientist or a hiring manager in which you'll answer a multifaceted business problem that's likely related to the organization's daily work. When I first started to prepare for the case study interview, I didn't know there are different types of case studies.
There are 4 modules in this course. This course is the eighth and final course in the Google Data Analytics Certificate. You'll have the opportunity to complete a case study, which will help prepare you for your data analytics job hunt. Case studies are commonly used by employers to assess analytical skills. For your case study, you'll ...
In this course, you'll learn how to use Data, Analytics, Statistics, Probability, and basic Data Science to give an edge in your career and everyday life. Being able to see through the noise within data, and explain it to others will make you invaluable in any career. We will examine over 2 dozen real-world data sets and show how to obtain ...
Humana's Automated Data Analysis Case Study. The key thing to note here is that the approach to creating a successful data program varies from industry to industry. Let's start with one to demonstrate the kind of value you can glean from these kinds of success stories. Humana has provided health insurance to Americans for over 50 years.
7 Top Data Science Case Studies . Here are 7 top case studies that show how companies and organizations have approached common challenges with some seriously inventive data science solutions: Geosciences. Data science is a powerful tool that can help us to understand better and predict geoscience phenomena.
Feb 21, 2021. --. 1. Solving a Data Science case study means analyzing and solving a problem statement intensively. Solving case studies will help you show unique and amazing data science use ...
Advantages of Using Case Studies for Data Analysts. Problem-solving (PS) - PS plays an important role for DS ( data scientists )/DA (data analyst)/BA (business analyst). The magnitude of efforts used on PS can vary across organizations and projects, with some teams working mainly on PS about 80% of the time, while others work 20% of the time.
Case study protocol is a formal document capturing the entire set of procedures involved in the collection of empirical material . It extends direction to researchers for gathering evidences, empirical material analysis, and case study reporting . This section includes a step-by-step guide that is used for the execution of the actual study.
Top 20 Analytics Case Studies in 2024. Updated on Jan 10. 4 min read. Written by. Cem Dilmegani. Although the potential of Big Data and business intelligence are recognized by organizations, Gartner analyst Nick Heudecker says that the failure rate of analytics projects is close to 85%. Uncovering the power of analytics improves business ...
A case study is one of the most commonly used methodologies of social research. This article attempts to look into the various dimensions of a case study research strategy, the different epistemological strands which determine the particular case study type and approach adopted in the field, discusses the factors which can enhance the effectiveness of a case study research, and the debate ...
Data sources: The research example used is a multiple case study that explored the role of the clinical skills laboratory in preparing students for the real world of practice. Data analysis was conducted using a framework guided by the four stages of analysis outlined by Morse ( 1994 ): comprehending, synthesising, theorising and recontextualising.
A single-case study is an in-depth analysis of a single case. This type of case study is useful when the researcher wants to understand a specific phenomenon in detail. ... Rich data: Case study research can generate rich and detailed data, including qualitative data such as interviews, observations, and documents. This can provide a nuanced ...
propose an approach to the analysis of case study data by logically linking the data to a series of propositions and then interpreting the subsequent information. Like the Yin (1994) strategy, the Miles and Huberman (1994) process of analysis of case study data, although quite detailed, may still be insufficient to guide the novice researcher.
There are 4 modules in this course. This course is the eighth and final course in the Google Data Analytics Certificate. You'll have the opportunity to complete a case study, which will help prepare you for your data analytics job hunt. Case studies are commonly used by employers to assess analytical skills. For your case study, you'll ...
Data Analysis Using Excel Case Study Data analysis is an essential skill in today's business world. As organizations deal with increasing amounts of data, it becomes crucial for professionals to make sense of this information and derive useful insights. Excel is a powerful and versatile tool that can assist in analyzing and presenting data effectively, […]
Designed for aspiring and established leaders in any industry, HBAP equips participants with the machine learning and data analysis tools they need to incorporate innovative tech into their business strategy, at the top levels of their organization. Learn More. Duration. Available Anytime. Price. $51,500.
Rasmussen University is accredited by the Higher Learning Commission, an institutional accreditation agency recognized by the U.S. Department of Education. Data is a thrilling industry to get into. Whether you're a career changer or a high school student, if you're asking how to become a data analyst, here are 6 steps you should take.
COVID-19 Risk Analysis Based on Population Migration Big Data: A Case Study of Wuhan. Pages 940-946. Previous Chapter Next Chapter. ABSTRACT. Population movement between regions is one of the main ways for the spread of COVID-19. The Chinese government has adopted unprecedented population movement controls to restrain the spread of COVID-19.
Background Following the legalization of cannabis in Canada in 2018, people aged 65 + years reported a significant increase in cannabis consumption. Despite limited research with older adults regarding the therapeutic benefits of cannabis, there is increasing interest and use among this population, particularly for those who have chronic illnesses or are at end of life. Long-term Care (LTC ...
We utilized 2006-2015 claims data of the Taiwan National Health Insurance Program as a data source. A case-control study was conducted, involving individuals 20 years or older with and without colon polyps. ... However, more studies combining the biomarker analysis and molecular pathways are needed to prompt a comprehensive exploration of ...
Photosynthesis in vegetation is one of the key processes in maintaining regional ecological balance and climate stability, and it is of significant importance for understanding the health of regional ecosystems and addressing climate change. Based on 2001-2021 Global OCO-2 Solar-Induced Fluorescence (GOSIF) dataset, this study analyzed spatiotemporal variations in Asian vegetation ...
As the medium of geological information, groundwater provides an indirect method to solve the secondary disasters of mining activities. Identifying the groundwater regime of overburden aquifers induced by the mining disturbance is significant in mining safety and geological environment protection. This study proposes the novel data-driven algorithm based on the combination of machine learning ...