Jump to navigation

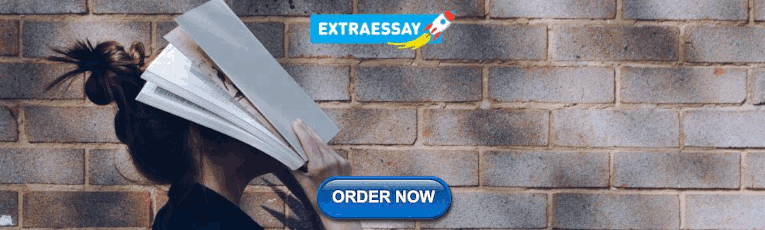
Cochrane Training
Chapter 12: synthesizing and presenting findings using other methods.
Joanne E McKenzie, Sue E Brennan
Key Points:
- Meta-analysis of effect estimates has many advantages, but other synthesis methods may need to be considered in the circumstance where there is incompletely reported data in the primary studies.
- Alternative synthesis methods differ in the completeness of the data they require, the hypotheses they address, and the conclusions and recommendations that can be drawn from their findings.
- These methods provide more limited information for healthcare decision making than meta-analysis, but may be superior to a narrative description where some results are privileged above others without appropriate justification.
- Tabulation and visual display of the results should always be presented alongside any synthesis, and are especially important for transparent reporting in reviews without meta-analysis.
- Alternative synthesis and visual display methods should be planned and specified in the protocol. When writing the review, details of the synthesis methods should be described.
- Synthesis methods that involve vote counting based on statistical significance have serious limitations and are unacceptable.
Cite this chapter as: McKenzie JE, Brennan SE. Chapter 12: Synthesizing and presenting findings using other methods. In: Higgins JPT, Thomas J, Chandler J, Cumpston M, Li T, Page MJ, Welch VA (editors). Cochrane Handbook for Systematic Reviews of Interventions version 6.4 (updated August 2023). Cochrane, 2023. Available from www.training.cochrane.org/handbook .
12.1 Why a meta-analysis of effect estimates may not be possible
Meta-analysis of effect estimates has many potential advantages (see Chapter 10 and Chapter 11 ). However, there are circumstances where it may not be possible to undertake a meta-analysis and other statistical synthesis methods may be considered (McKenzie and Brennan 2014).
Some common reasons why it may not be possible to undertake a meta-analysis are outlined in Table 12.1.a . Legitimate reasons include limited evidence; incompletely reported outcome/effect estimates, or different effect measures used across studies; and bias in the evidence. Other commonly cited reasons for not using meta-analysis are because of too much clinical or methodological diversity, or statistical heterogeneity (Achana et al 2014). However, meta-analysis methods should be considered in these circumstances, as they may provide important insights if undertaken and interpreted appropriately.
Table 12.1.a Scenarios that may preclude meta-analysis, with possible solutions
12.2 Statistical synthesis when meta-analysis of effect estimates is not possible
A range of statistical synthesis methods are available, and these may be divided into three categories based on their preferability ( Table 12.2.a ). Preferable methods are the meta-analysis methods outlined in Chapter 10 and Chapter 11 , and are not discussed in detail here. This chapter focuses on methods that might be considered when a meta-analysis of effect estimates is not possible due to incompletely reported data in the primary studies. These methods divide into those that are ‘acceptable’ and ‘unacceptable’. The ‘acceptable’ methods differ in the data they require, the hypotheses they address, limitations around their use, and the conclusions and recommendations that can be drawn (see Section 12.2.1 ). The ‘unacceptable’ methods in common use are described (see Section 12.2.2 ), along with the reasons for why they are problematic.
Compared with meta-analysis methods, the ‘acceptable’ synthesis methods provide more limited information for healthcare decision making. However, these ‘acceptable’ methods may be superior to a narrative that describes results study by study, which comes with the risk that some studies or findings are privileged above others without appropriate justification. Further, in reviews with little or no synthesis, readers are left to make sense of the research themselves, which may result in the use of seemingly simple yet problematic synthesis methods such as vote counting based on statistical significance (see Section 12.2.2.1 ).
All methods first involve calculation of a ‘standardized metric’, followed by application of a synthesis method. In applying any of the following synthesis methods, it is important that only one outcome per study (or other independent unit, for example one comparison from a trial with multiple intervention groups) contributes to the synthesis. Chapter 9 outlines approaches for selecting an outcome when multiple have been measured. Similar to meta-analysis, sensitivity analyses can be undertaken to examine if the findings of the synthesis are robust to potentially influential decisions (see Chapter 10, Section 10.14 and Section 12.4 for examples).
Authors should report the specific methods used in lieu of meta-analysis (including approaches used for presentation and visual display), rather than stating that they have conducted a ‘narrative synthesis’ or ‘narrative summary’ without elaboration. The limitations of the chosen methods must be described, and conclusions worded with appropriate caution. The aim of reporting this detail is to make the synthesis process more transparent and reproducible, and help ensure use of appropriate methods and interpretation.
Table 12.2.a Summary of preferable and acceptable synthesis methods
12.2.1 Acceptable synthesis methods
12.2.1.1 summarizing effect estimates.
Description of method Summarizing effect estimates might be considered in the circumstance where estimates of intervention effect are available (or can be calculated), but the variances of the effects are not reported or are incorrect (and cannot be calculated from other statistics, or reasonably imputed) (Grimshaw et al 2003). Incorrect calculation of variances arises more commonly in non-standard study designs that involve clustering or matching ( Chapter 23 ). While missing variances may limit the possibility of meta-analysis, the (standardized) effects can be summarized using descriptive statistics such as the median, interquartile range, and the range. Calculating these statistics addresses the question ‘What is the range and distribution of observed effects?’
Reporting of methods and results The statistics that will be used to summarize the effects (e.g. median, interquartile range) should be reported. Box-and-whisker or bubble plots will complement reporting of the summary statistics by providing a visual display of the distribution of observed effects (Section 12.3.3 ). Tabulation of the available effect estimates will provide transparency for readers by linking the effects to the studies (Section 12.3.1 ). Limitations of the method should be acknowledged ( Table 12.2.a ).
12.2.1.2 Combining P values
Description of method Combining P values can be considered in the circumstance where there is no, or minimal, information reported beyond P values and the direction of effect; the types of outcomes and statistical tests differ across the studies; or results from non-parametric tests are reported (Borenstein et al 2009). Combining P values addresses the question ‘Is there evidence that there is an effect in at least one study?’ There are several methods available (Loughin 2004), with the method proposed by Fisher outlined here (Becker 1994).
Fisher’s method combines the P values from statistical tests across k studies using the formula:
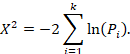
One-sided P values are used, since these contain information about the direction of effect. However, these P values must reflect the same directional hypothesis (e.g. all testing if intervention A is more effective than intervention B). This is analogous to standardizing the direction of effects before undertaking a meta-analysis. Two-sided P values, which do not contain information about the direction, must first be converted to one-sided P values. If the effect is consistent with the directional hypothesis (e.g. intervention A is beneficial compared with B), then the one-sided P value is calculated as
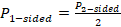
In studies that do not report an exact P value but report a conventional level of significance (e.g. P<0.05), a conservative option is to use the threshold (e.g. 0.05). The P values must have been computed from statistical tests that appropriately account for the features of the design, such as clustering or matching, otherwise they will likely be incorrect.

Reporting of methods and results There are several methods for combining P values (Loughin 2004), so the chosen method should be reported, along with details of sensitivity analyses that examine if the results are sensitive to the choice of method. The results from the test should be reported alongside any available effect estimates (either individual results or meta-analysis results of a subset of studies) using text, tabulation and appropriate visual displays (Section 12.3 ). The albatross plot is likely to complement the analysis (Section 12.3.4 ). Limitations of the method should be acknowledged ( Table 12.2.a ).
12.2.1.3 Vote counting based on the direction of effect
Description of method Vote counting based on the direction of effect might be considered in the circumstance where the direction of effect is reported (with no further information), or there is no consistent effect measure or data reported across studies. The essence of vote counting is to compare the number of effects showing benefit to the number of effects showing harm for a particular outcome. However, there is wide variation in the implementation of the method due to differences in how ‘benefit’ and ‘harm’ are defined. Rules based on subjective decisions or statistical significance are problematic and should be avoided (see Section 12.2.2 ).
To undertake vote counting properly, each effect estimate is first categorized as showing benefit or harm based on the observed direction of effect alone, thereby creating a standardized binary metric. A count of the number of effects showing benefit is then compared with the number showing harm. Neither statistical significance nor the size of the effect are considered in the categorization. A sign test can be used to answer the question ‘is there any evidence of an effect?’ If there is no effect, the study effects will be distributed evenly around the null hypothesis of no difference. This is equivalent to testing if the true proportion of effects favouring the intervention (or comparator) is equal to 0.5 (Bushman and Wang 2009) (see Section 12.4.2.3 for guidance on implementing the sign test). An estimate of the proportion of effects favouring the intervention can be calculated ( p = u / n , where u = number of effects favouring the intervention, and n = number of studies) along with a confidence interval (e.g. using the Wilson or Jeffreys interval methods (Brown et al 2001)). Unless there are many studies contributing effects to the analysis, there will be large uncertainty in this estimated proportion.
Reporting of methods and results The vote counting method should be reported in the ‘Data synthesis’ section of the review. Failure to recognize vote counting as a synthesis method has led to it being applied informally (and perhaps unintentionally) to summarize results (e.g. through the use of wording such as ‘3 of 10 studies showed improvement in the outcome with intervention compared to control’; ‘most studies found’; ‘the majority of studies’; ‘few studies’ etc). In such instances, the method is rarely reported, and it may not be possible to determine whether an unacceptable (invalid) rule has been used to define benefit and harm (Section 12.2.2 ). The results from vote counting should be reported alongside any available effect estimates (either individual results or meta-analysis results of a subset of studies) using text, tabulation and appropriate visual displays (Section 12.3 ). The number of studies contributing to a synthesis based on vote counting may be larger than a meta-analysis, because only minimal statistical information (i.e. direction of effect) is required from each study to vote count. Vote counting results are used to derive the harvest and effect direction plots, although often using unacceptable methods of vote counting (see Section 12.3.5 ). Limitations of the method should be acknowledged ( Table 12.2.a ).
12.2.2 Unacceptable synthesis methods
12.2.2.1 vote counting based on statistical significance.
Conventional forms of vote counting use rules based on statistical significance and direction to categorize effects. For example, effects may be categorized into three groups: those that favour the intervention and are statistically significant (based on some predefined P value), those that favour the comparator and are statistically significant, and those that are statistically non-significant (Hedges and Vevea 1998). In a simpler formulation, effects may be categorized into two groups: those that favour the intervention and are statistically significant, and all others (Friedman 2001). Regardless of the specific formulation, when based on statistical significance, all have serious limitations and can lead to the wrong conclusion.
The conventional vote counting method fails because underpowered studies that do not rule out clinically important effects are counted as not showing benefit. Suppose, for example, the effect sizes estimated in two studies were identical. However, only one of the studies was adequately powered, and the effect in this study was statistically significant. Only this one effect (of the two identical effects) would be counted as showing ‘benefit’. Paradoxically, Hedges and Vevea showed that as the number of studies increases, the power of conventional vote counting tends to zero, except with large studies and at least moderate intervention effects (Hedges and Vevea 1998). Further, conventional vote counting suffers the same disadvantages as vote counting based on direction of effect, namely, that it does not provide information on the magnitude of effects and does not account for differences in the relative sizes of the studies.
12.2.2.2 Vote counting based on subjective rules
Subjective rules, involving a combination of direction, statistical significance and magnitude of effect, are sometimes used to categorize effects. For example, in a review examining the effectiveness of interventions for teaching quality improvement to clinicians, the authors categorized results as ‘beneficial effects’, ‘no effects’ or ‘detrimental effects’ (Boonyasai et al 2007). Categorization was based on direction of effect and statistical significance (using a predefined P value of 0.05) when available. If statistical significance was not reported, effects greater than 10% were categorized as ‘beneficial’ or ‘detrimental’, depending on their direction. These subjective rules often vary in the elements, cut-offs and algorithms used to categorize effects, and while detailed descriptions of the rules may provide a veneer of legitimacy, such rules have poor performance validity (Ioannidis et al 2008).
A further problem occurs when the rules are not described in sufficient detail for the results to be reproduced (e.g. ter Wee et al 2012, Thornicroft et al 2016). This lack of transparency does not allow determination of whether an acceptable or unacceptable vote counting method has been used (Valentine et al 2010).
12.3 Visual display and presentation of the data
Visual display and presentation of data is especially important for transparent reporting in reviews without meta-analysis, and should be considered irrespective of whether synthesis is undertaken (see Table 12.2.a for a summary of plots associated with each synthesis method). Tables and plots structure information to show patterns in the data and convey detailed information more efficiently than text. This aids interpretation and helps readers assess the veracity of the review findings.
12.3.1 Structured tabulation of results across studies
Ordering studies alphabetically by study ID is the simplest approach to tabulation; however, more information can be conveyed when studies are grouped in subpanels or ordered by a characteristic important for interpreting findings. The grouping of studies in tables should generally follow the structure of the synthesis presented in the text, which should closely reflect the review questions. This grouping should help readers identify the data on which findings are based and verify the review authors’ interpretation.
If the purpose of the table is comparative, grouping studies by any of following characteristics might be informative:
- comparisons considered in the review, or outcome domains (according to the structure of the synthesis);
- study characteristics that may reveal patterns in the data, for example potential effect modifiers including population subgroups, settings or intervention components.
If the purpose of the table is complete and transparent reporting of data, then ordering the studies to increase the prominence of the most relevant and trustworthy evidence should be considered. Possibilities include:
- certainty of the evidence (synthesized result or individual studies if no synthesis);
- risk of bias, study size or study design characteristics; and
- characteristics that determine how directly a study addresses the review question, for example relevance and validity of the outcome measures.
One disadvantage of grouping by study characteristics is that it can be harder to locate specific studies than when tables are ordered by study ID alone, for example when cross-referencing between the text and tables. Ordering by study ID within categories may partly address this.
The value of standardizing intervention and outcome labels is discussed in Chapter 3, Section 3.2.2 and Section 3.2.4 ), while the importance and methods for standardizing effect estimates is described in Chapter 6 . These practices can aid readers’ interpretation of tabulated data, especially when the purpose of a table is comparative.
12.3.2 Forest plots
Forest plots and methods for preparing them are described elsewhere ( Chapter 10, Section 10.2 ). Some mention is warranted here of their importance for displaying study results when meta-analysis is not undertaken (i.e. without the summary diamond). Forest plots can aid interpretation of individual study results and convey overall patterns in the data, especially when studies are ordered by a characteristic important for interpreting results (e.g. dose and effect size, sample size). Similarly, grouping studies in subpanels based on characteristics thought to modify effects, such as population subgroups, variants of an intervention, or risk of bias, may help explore and explain differences across studies (Schriger et al 2010). These approaches to ordering provide important techniques for informally exploring heterogeneity in reviews without meta-analysis, and should be considered in preference to alphabetical ordering by study ID alone (Schriger et al 2010).
12.3.3 Box-and-whisker plots and bubble plots
Box-and-whisker plots (see Figure 12.4.a , Panel A) provide a visual display of the distribution of effect estimates (Section 12.2.1.1 ). The plot conventionally depicts five values. The upper and lower limits (or ‘hinges’) of the box, represent the 75th and 25th percentiles, respectively. The line within the box represents the 50th percentile (median), and the whiskers represent the extreme values (McGill et al 1978). Multiple box plots can be juxtaposed, providing a visual comparison of the distributions of effect estimates (Schriger et al 2006). For example, in a review examining the effects of audit and feedback on professional practice, the format of the feedback (verbal, written, both verbal and written) was hypothesized to be an effect modifier (Ivers et al 2012). Box-and-whisker plots of the risk differences were presented separately by the format of feedback, to allow visual comparison of the impact of format on the distribution of effects. When presenting multiple box-and-whisker plots, the width of the box can be varied to indicate the number of studies contributing to each. The plot’s common usage facilitates rapid and correct interpretation by readers (Schriger et al 2010). The individual studies contributing to the plot are not identified (as in a forest plot), however, and the plot is not appropriate when there are few studies (Schriger et al 2006).
A bubble plot (see Figure 12.4.a , Panel B) can also be used to provide a visual display of the distribution of effects, and is more suited than the box-and-whisker plot when there are few studies (Schriger et al 2006). The plot is a scatter plot that can display multiple dimensions through the location, size and colour of the bubbles. In a review examining the effects of educational outreach visits on professional practice, a bubble plot was used to examine visually whether the distribution of effects was modified by the targeted behaviour (O’Brien et al 2007). Each bubble represented the effect size (y-axis) and whether the study targeted a prescribing or other behaviour (x-axis). The size of the bubbles reflected the number of study participants. However, different formulations of the bubble plot can display other characteristics of the data (e.g. precision, risk-of-bias assessments).
12.3.4 Albatross plot
The albatross plot (see Figure 12.4.a , Panel C) allows approximate examination of the underlying intervention effect sizes where there is minimal reporting of results within studies (Harrison et al 2017). The plot only requires a two-sided P value, sample size and direction of effect (or equivalently, a one-sided P value and a sample size) for each result. The plot is a scatter plot of the study sample sizes against two-sided P values, where the results are separated by the direction of effect. Superimposed on the plot are ‘effect size contours’ (inspiring the plot’s name). These contours are specific to the type of data (e.g. continuous, binary) and statistical methods used to calculate the P values. The contours allow interpretation of the approximate effect sizes of the studies, which would otherwise not be possible due to the limited reporting of the results. Characteristics of studies (e.g. type of study design) can be identified using different colours or symbols, allowing informal comparison of subgroups.
The plot is likely to be more inclusive of the available studies than meta-analysis, because of its minimal data requirements. However, the plot should complement the results from a statistical synthesis, ideally a meta-analysis of available effects.
12.3.5 Harvest and effect direction plots
Harvest plots (see Figure 12.4.a , Panel D) provide a visual extension of vote counting results (Ogilvie et al 2008). In the plot, studies based on the categorization of their effects (e.g. ‘beneficial effects’, ‘no effects’ or ‘detrimental effects’) are grouped together. Each study is represented by a bar positioned according to its categorization. The bars can be ‘visually weighted’ (by height or width) and annotated to highlight study and outcome characteristics (e.g. risk-of-bias domains, proximal or distal outcomes, study design, sample size) (Ogilvie et al 2008, Crowther et al 2011). Annotation can also be used to identify the studies. A series of plots may be combined in a matrix that displays, for example, the vote counting results from different interventions or outcome domains.
The methods papers describing harvest plots have employed vote counting based on statistical significance (Ogilvie et al 2008, Crowther et al 2011). For the reasons outlined in Section 12.2.2.1 , this can be misleading. However, an acceptable approach would be to display the results based on direction of effect.
The effect direction plot is similar in concept to the harvest plot in the sense that both display information on the direction of effects (Thomson and Thomas 2013). In the first version of the effect direction plot, the direction of effects for each outcome within a single study are displayed, while the second version displays the direction of the effects for outcome domains across studies . In this second version, an algorithm is first applied to ‘synthesize’ the directions of effect for all outcomes within a domain (e.g. outcomes ‘sleep disturbed by wheeze’, ‘wheeze limits speech’, ‘wheeze during exercise’ in the outcome domain ‘respiratory’). This algorithm is based on the proportion of effects that are in a consistent direction and statistical significance. Arrows are used to indicate the reported direction of effect (for either outcomes or outcome domains). Features such as statistical significance, study design and sample size are denoted using size and colour. While this version of the plot conveys a large amount of information, it requires further development before its use can be recommended since the algorithm underlying the plot is likely to have poor performance validity.
12.4 Worked example
The example that follows uses four scenarios to illustrate methods for presentation and synthesis when meta-analysis is not possible. The first scenario contrasts a common approach to tabulation with alternative presentations that may enhance the transparency of reporting and interpretation of findings. Subsequent scenarios show the application of the synthesis approaches outlined in preceding sections of the chapter. Box 12.4.a summarizes the review comparisons and outcomes, and decisions taken by the review authors in planning their synthesis. While the example is loosely based on an actual review, the review description, scenarios and data are fabricated for illustration.
Box 12.4.a The review
12.4.1 Scenario 1: structured reporting of effects
We first address a scenario in which review authors have decided that the tools used to measure satisfaction measured concepts that were too dissimilar across studies for synthesis to be appropriate. Setting aside three of the 15 studies that reported on the birth partner’s satisfaction with care, a structured summary of effects is sought of the remaining 12 studies. To keep the example table short, only one outcome is shown per study for each of the measurement periods (antenatal, intrapartum or postpartum).
Table 12.4.a depicts a common yet suboptimal approach to presenting results. Note two features.
- Studies are ordered by study ID, rather than grouped by characteristics that might enhance interpretation (e.g. risk of bias, study size, validity of the measures, certainty of the evidence (GRADE)).
- Data reported are as extracted from each study; effect estimates were not calculated by the review authors and, where reported, were not standardized across studies (although data were available to do both).
Table 12.4.b shows an improved presentation of the same results. In line with best practice, here effect estimates have been calculated by the review authors for all outcomes, and a common metric computed to aid interpretation (in this case an odds ratio; see Chapter 6 for guidance on conversion of statistics to the desired format). Redundant information has been removed (‘statistical test’ and ‘P value’ columns). The studies have been re-ordered, first to group outcomes by period of care (intrapartum outcomes are shown here), and then by risk of bias. This re-ordering serves two purposes. Grouping by period of care aligns with the plan to consider outcomes for each period separately and ensures the table structure matches the order in which results are described in the text. Re-ordering by risk of bias increases the prominence of studies at lowest risk of bias, focusing attention on the results that should most influence conclusions. Had the review authors determined that a synthesis would be informative, then ordering to facilitate comparison across studies would be appropriate; for example, ordering by the type of satisfaction outcome (as pre-defined in the protocol, starting with global measures of satisfaction), or the comparisons made in the studies.
The results may also be presented in a forest plot, as shown in Figure 12.4.b . In both the table and figure, studies are grouped by risk of bias to focus attention on the most trustworthy evidence. The pattern of effects across studies is immediately apparent in Figure 12.4.b and can be described efficiently without having to interpret each estimate (e.g. difference between studies at low and high risk of bias emerge), although these results should be interpreted with caution in the absence of a formal test for subgroup differences (see Chapter 10, Section 10.11 ). Only outcomes measured during the intrapartum period are displayed, although outcomes from other periods could be added, maximizing the information conveyed.
An example description of the results from Scenario 1 is provided in Box 12.4.b . It shows that describing results study by study becomes unwieldy with more than a few studies, highlighting the importance of tables and plots. It also brings into focus the risk of presenting results without any synthesis, since it seems likely that the reader will try to make sense of the results by drawing inferences across studies. Since a synthesis was considered inappropriate, GRADE was applied to individual studies and then used to prioritize the reporting of results, focusing attention on the most relevant and trustworthy evidence. An alternative might be to report results at low risk of bias, an approach analogous to limiting a meta-analysis to studies at low risk of bias. Where possible, these and other approaches to prioritizing (or ordering) results from individual studies in text and tables should be pre-specified at the protocol stage.
Table 12.4.a Scenario 1: table ordered by study ID, data as reported by study authors
* All scales operate in the same direction; higher scores indicate greater satisfaction. CI = confidence interval; MD = mean difference; OR = odds ratio; POR = proportional odds ratio; RD = risk difference; RR = risk ratio.
Table 12.4.b Scenario 1: intrapartum outcome table ordered by risk of bias, standardized effect estimates calculated for all studies
* Outcomes operate in the same direction. A higher score, or an event, indicates greater satisfaction. ** Mean difference calculated for studies reporting continuous outcomes. † For binary outcomes, odds ratios were calculated from the reported summary statistics or were directly extracted from the study. For continuous outcomes, standardized mean differences were calculated and converted to odds ratios (see Chapter 6 ). CI = confidence interval; POR = proportional odds ratio.
Figure 12.4.b Forest plot depicting standardized effect estimates (odds ratios) for satisfaction
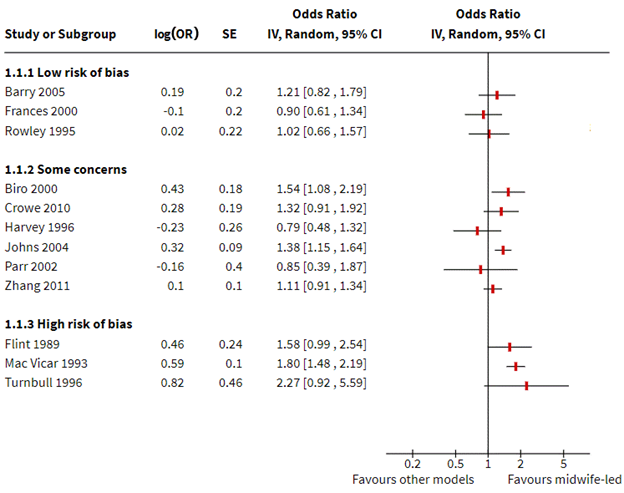
Box 12.4.b How to describe the results from this structured summary
12.4.2 Overview of scenarios 2–4: synthesis approaches
We now address three scenarios in which review authors have decided that the outcomes reported in the 15 studies all broadly reflect satisfaction with care. While the measures were quite diverse, a synthesis is sought to help decision makers understand whether women and their birth partners were generally more satisfied with the care received in midwife-led continuity models compared with other models. The three scenarios differ according to the data available (see Table 12.4.c ), with each reflecting progressively less complete reporting of the effect estimates. The data available determine the synthesis method that can be applied.
- Scenario 2: effect estimates available without measures of precision (illustrating synthesis of summary statistics).
- Scenario 3: P values available (illustrating synthesis of P values).
- Scenario 4: directions of effect available (illustrating synthesis using vote-counting based on direction of effect).
For studies that reported multiple satisfaction outcomes, one result is selected for synthesis using the decision rules in Box 12.4.a (point 2).
Table 12.4.c Scenarios 2, 3 and 4: available data for the selected outcome from each study
* All scales operate in the same direction. Higher scores indicate greater satisfaction. ** For a particular scenario, the ‘available data’ column indicates the data that were directly reported, or were calculated from the reported statistics, in terms of: effect estimate, direction of effect, confidence interval, precise P value, or statement regarding statistical significance (either statistically significant, or not). CI = confidence interval; direction = direction of effect reported or can be calculated; MD = mean difference; NS = not statistically significant; OR = odds ratio; RD = risk difference; RoB = risk of bias; RR = risk ratio; sig. = statistically significant; SMD = standardized mean difference; Stand. = standardized.
12.4.2.1 Scenario 2: summarizing effect estimates
In Scenario 2, effect estimates are available for all outcomes. However, for most studies, a measure of variance is not reported, or cannot be calculated from the available data. We illustrate how the effect estimates may be summarized using descriptive statistics. In this scenario, it is possible to calculate odds ratios for all studies. For the continuous outcomes, this involves first calculating a standardized mean difference, and then converting this to an odds ratio ( Chapter 10, Section 10.6 ). The median odds ratio is 1.32 with an interquartile range of 1.02 to 1.53 (15 studies). Box-and-whisker plots may be used to display these results and examine informally whether the distribution of effects differs by the overall risk-of-bias assessment ( Figure 12.4.a , Panel A). However, because there are relatively few effects, a reasonable alternative would be to present bubble plots ( Figure 12.4.a , Panel B).
An example description of the results from the synthesis is provided in Box 12.4.c .
Box 12.4.c How to describe the results from this synthesis
12.4.2.2 Scenario 3: combining P values
In Scenario 3, there is minimal reporting of the data, and the type of data and statistical methods and tests vary. However, 11 of the 15 studies provide a precise P value and direction of effect, and a further two report a P value less than a threshold (<0.001) and direction. We use this scenario to illustrate a synthesis of P values. Since the reported P values are two-sided ( Table 12.4.c , column 6), they must first be converted to one-sided P values, which incorporate the direction of effect ( Table 12.4.c , column 7).
Fisher’s method for combining P values involved calculating the following statistic:

The combination of P values suggests there is strong evidence of benefit of midwife-led models of care in at least one study (P < 0.001 from a Chi 2 test, 13 studies). Restricting this analysis to those studies judged to be at an overall low risk of bias (sensitivity analysis), there is no longer evidence to reject the null hypothesis of no benefit of midwife-led model of care in any studies (P = 0.314, 3 studies). For the five studies reporting continuous satisfaction outcomes, sufficient data (precise P value, direction, total sample size) are reported to construct an albatross plot ( Figure 12.4.a , Panel C). The location of the points relative to the standardized mean difference contours indicate that the likely effects of the intervention in these studies are small.
An example description of the results from the synthesis is provided in Box 12.4.d .
Box 12.4.d How to describe the results from this synthesis
12.4.2.3 Scenario 4: vote counting based on direction of effect
In Scenario 4, there is minimal reporting of the data, and the type of effect measure (when used) varies across the studies (e.g. mean difference, proportional odds ratio). Of the 15 results, only five report data suitable for meta-analysis (effect estimate and measure of precision; Table 12.4.c , column 8), and no studies reported precise P values. We use this scenario to illustrate vote counting based on direction of effect. For each study, the effect is categorized as beneficial or harmful based on the direction of effect (indicated as a binary metric; Table 12.4.c , column 9).
Of the 15 studies, we exclude three because they do not provide information on the direction of effect, leaving 12 studies to contribute to the synthesis. Of these 12, 10 effects favour midwife-led models of care (83%). The probability of observing this result if midwife-led models of care are truly ineffective is 0.039 (from a binomial probability test, or equivalently, the sign test). The 95% confidence interval for the percentage of effects favouring midwife-led care is wide (55% to 95%).
The binomial test can be implemented using standard computer spreadsheet or statistical packages. For example, the two-sided P value from the binomial probability test presented can be obtained from Microsoft Excel by typing =2*BINOM.DIST(2, 12, 0.5, TRUE) into any cell in the spreadsheet. The syntax requires the smaller of the ‘number of effects favouring the intervention’ or ‘the number of effects favouring the control’ (here, the smaller of these counts is 2), the number of effects (here 12), and the null value (true proportion of effects favouring the intervention = 0.5). In Stata, the bitest command could be used (e.g. bitesti 12 10 0.5 ).
A harvest plot can be used to display the results ( Figure 12.4.a , Panel D), with characteristics of the studies represented using different heights and shading. A sensitivity analysis might be considered, restricting the analysis to those studies judged to be at an overall low risk of bias. However, only four studies were judged to be at a low risk of bias (of which, three favoured midwife-led models of care), precluding reasonable interpretation of the count.
An example description of the results from the synthesis is provided in Box 12.4.e .
Box 12.4.e How to describe the results from this synthesis
Figure 12.4.a Possible graphical displays of different types of data. (A) Box-and-whisker plots of odds ratios for all outcomes and separately by overall risk of bias. (B) Bubble plot of odds ratios for all outcomes and separately by the model of care. The colours of the bubbles represent the overall risk of bias judgement (green = low risk of bias; yellow = some concerns; red = high risk of bias). (C) Albatross plot of the study sample size against P values (for the five continuous outcomes in Table 12.4.c , column 6). The effect contours represent standardized mean differences. (D) Harvest plot (height depicts overall risk of bias judgement (tall = low risk of bias; medium = some concerns; short = high risk of bias), shading depicts model of care (light grey = caseload; dark grey = team), alphabet characters represent the studies)
12.5 Chapter information
Authors: Joanne E McKenzie, Sue E Brennan
Acknowledgements: Sections of this chapter build on chapter 9 of version 5.1 of the Handbook , with editors Jonathan J Deeks, Julian PT Higgins and Douglas G Altman.
We are grateful to the following for commenting helpfully on earlier drafts: Miranda Cumpston, Jamie Hartmann-Boyce, Tianjing Li, Rebecca Ryan and Hilary Thomson.
Funding: JEM is supported by an Australian National Health and Medical Research Council (NHMRC) Career Development Fellowship (1143429). SEB’s position is supported by the NHMRC Cochrane Collaboration Funding Program.
12.6 References
Achana F, Hubbard S, Sutton A, Kendrick D, Cooper N. An exploration of synthesis methods in public health evaluations of interventions concludes that the use of modern statistical methods would be beneficial. Journal of Clinical Epidemiology 2014; 67 : 376–390.
Becker BJ. Combining significance levels. In: Cooper H, Hedges LV, editors. A handbook of research synthesis . New York (NY): Russell Sage; 1994. p. 215–235.
Boonyasai RT, Windish DM, Chakraborti C, Feldman LS, Rubin HR, Bass EB. Effectiveness of teaching quality improvement to clinicians: a systematic review. JAMA 2007; 298 : 1023–1037.
Borenstein M, Hedges LV, Higgins JPT, Rothstein HR. Meta-Analysis methods based on direction and p-values. Introduction to Meta-Analysis . Chichester (UK): John Wiley & Sons, Ltd; 2009. pp. 325–330.
Brown LD, Cai TT, DasGupta A. Interval estimation for a binomial proportion. Statistical Science 2001; 16 : 101–117.
Bushman BJ, Wang MC. Vote-counting procedures in meta-analysis. In: Cooper H, Hedges LV, Valentine JC, editors. Handbook of Research Synthesis and Meta-Analysis . 2nd ed. New York (NY): Russell Sage Foundation; 2009. p. 207–220.
Crowther M, Avenell A, MacLennan G, Mowatt G. A further use for the Harvest plot: a novel method for the presentation of data synthesis. Research Synthesis Methods 2011; 2 : 79–83.
Friedman L. Why vote-count reviews don’t count. Biological Psychiatry 2001; 49 : 161–162.
Grimshaw J, McAuley LM, Bero LA, Grilli R, Oxman AD, Ramsay C, Vale L, Zwarenstein M. Systematic reviews of the effectiveness of quality improvement strategies and programmes. Quality and Safety in Health Care 2003; 12 : 298–303.
Harrison S, Jones HE, Martin RM, Lewis SJ, Higgins JPT. The albatross plot: a novel graphical tool for presenting results of diversely reported studies in a systematic review. Research Synthesis Methods 2017; 8 : 281–289.
Hedges L, Vevea J. Fixed- and random-effects models in meta-analysis. Psychological Methods 1998; 3 : 486–504.
Ioannidis JP, Patsopoulos NA, Rothstein HR. Reasons or excuses for avoiding meta-analysis in forest plots. BMJ 2008; 336 : 1413–1415.
Ivers N, Jamtvedt G, Flottorp S, Young JM, Odgaard-Jensen J, French SD, O’Brien MA, Johansen M, Grimshaw J, Oxman AD. Audit and feedback: effects on professional practice and healthcare outcomes. Cochrane Database of Systematic Reviews 2012; 6 : CD000259.
Jones DR. Meta-analysis: weighing the evidence. Statistics in Medicine 1995; 14 : 137–149.
Loughin TM. A systematic comparison of methods for combining p-values from independent tests. Computational Statistics & Data Analysis 2004; 47 : 467–485.
McGill R, Tukey JW, Larsen WA. Variations of box plots. The American Statistician 1978; 32 : 12–16.
McKenzie JE, Brennan SE. Complex reviews: methods and considerations for summarising and synthesising results in systematic reviews with complexity. Report to the Australian National Health and Medical Research Council. 2014.
O’Brien MA, Rogers S, Jamtvedt G, Oxman AD, Odgaard-Jensen J, Kristoffersen DT, Forsetlund L, Bainbridge D, Freemantle N, Davis DA, Haynes RB, Harvey EL. Educational outreach visits: effects on professional practice and health care outcomes. Cochrane Database of Systematic Reviews 2007; 4 : CD000409.
Ogilvie D, Fayter D, Petticrew M, Sowden A, Thomas S, Whitehead M, Worthy G. The harvest plot: a method for synthesising evidence about the differential effects of interventions. BMC Medical Research Methodology 2008; 8 : 8.
Riley RD, Higgins JP, Deeks JJ. Interpretation of random effects meta-analyses. BMJ 2011; 342 : d549.
Schriger DL, Sinha R, Schroter S, Liu PY, Altman DG. From submission to publication: a retrospective review of the tables and figures in a cohort of randomized controlled trials submitted to the British Medical Journal. Annals of Emergency Medicine 2006; 48 : 750–756, 756 e751–721.
Schriger DL, Altman DG, Vetter JA, Heafner T, Moher D. Forest plots in reports of systematic reviews: a cross-sectional study reviewing current practice. International Journal of Epidemiology 2010; 39 : 421–429.
ter Wee MM, Lems WF, Usan H, Gulpen A, Boonen A. The effect of biological agents on work participation in rheumatoid arthritis patients: a systematic review. Annals of the Rheumatic Diseases 2012; 71 : 161–171.
Thomson HJ, Thomas S. The effect direction plot: visual display of non-standardised effects across multiple outcome domains. Research Synthesis Methods 2013; 4 : 95–101.
Thornicroft G, Mehta N, Clement S, Evans-Lacko S, Doherty M, Rose D, Koschorke M, Shidhaye R, O’Reilly C, Henderson C. Evidence for effective interventions to reduce mental-health-related stigma and discrimination. Lancet 2016; 387 : 1123–1132.
Valentine JC, Pigott TD, Rothstein HR. How many studies do you need?: a primer on statistical power for meta-analysis. Journal of Educational and Behavioral Statistics 2010; 35 : 215–247.
For permission to re-use material from the Handbook (either academic or commercial), please see here for full details.
Analysis vs. Synthesis
What's the difference.
Analysis and synthesis are two fundamental processes in problem-solving and decision-making. Analysis involves breaking down a complex problem or situation into its constituent parts, examining each part individually, and understanding their relationships and interactions. It focuses on understanding the components and their characteristics, identifying patterns and trends, and drawing conclusions based on evidence and data. On the other hand, synthesis involves combining different elements or ideas to create a new whole or solution. It involves integrating information from various sources, identifying commonalities and differences, and generating new insights or solutions. While analysis is more focused on understanding and deconstructing a problem, synthesis is about creating something new by combining different elements. Both processes are essential for effective problem-solving and decision-making, as they complement each other and provide a holistic approach to understanding and solving complex problems.
Further Detail
Introduction.
Analysis and synthesis are two fundamental processes in various fields of study, including science, philosophy, and problem-solving. While they are distinct approaches, they are often interconnected and complementary. Analysis involves breaking down complex ideas or systems into smaller components to understand their individual parts and relationships. On the other hand, synthesis involves combining separate elements or ideas to create a new whole or understanding. In this article, we will explore the attributes of analysis and synthesis, highlighting their differences and similarities.
Attributes of Analysis
1. Focus on details: Analysis involves a meticulous examination of individual components, details, or aspects of a subject. It aims to understand the specific characteristics, functions, and relationships of these elements. By breaking down complex ideas into smaller parts, analysis provides a deeper understanding of the subject matter.
2. Objective approach: Analysis is often driven by objectivity and relies on empirical evidence, data, or logical reasoning. It aims to uncover patterns, trends, or underlying principles through systematic observation and investigation. By employing a structured and logical approach, analysis helps in drawing accurate conclusions and making informed decisions.
3. Critical thinking: Analysis requires critical thinking skills to evaluate and interpret information. It involves questioning assumptions, identifying biases, and considering multiple perspectives. Through critical thinking, analysis helps in identifying strengths, weaknesses, opportunities, and threats, enabling a comprehensive understanding of the subject matter.
4. Reductionist approach: Analysis often adopts a reductionist approach, breaking down complex systems into simpler components. This reductionist perspective allows for a detailed examination of each part, facilitating a more in-depth understanding of the subject matter. However, it may sometimes overlook the holistic view or emergent properties of the system.
5. Diagnostic tool: Analysis is commonly used as a diagnostic tool to identify problems, errors, or inefficiencies within a system. By examining individual components and their interactions, analysis helps in pinpointing the root causes of issues, enabling effective problem-solving and optimization.
Attributes of Synthesis
1. Integration of ideas: Synthesis involves combining separate ideas, concepts, or elements to create a new whole or understanding. It aims to generate novel insights, solutions, or perspectives by integrating diverse information or viewpoints. Through synthesis, complex systems or ideas can be approached holistically, considering the interconnections and interdependencies between various components.
2. Creative thinking: Synthesis requires creative thinking skills to generate new ideas, concepts, or solutions. It involves making connections, recognizing patterns, and thinking beyond traditional boundaries. By embracing divergent thinking, synthesis enables innovation and the development of unique perspectives.
3. Systems thinking: Synthesis often adopts a systems thinking approach, considering the interactions and interdependencies between various components. It recognizes that the whole is more than the sum of its parts and aims to understand emergent properties or behaviors that arise from the integration of these parts. Systems thinking allows for a comprehensive understanding of complex phenomena.
4. Constructive approach: Synthesis is a constructive process that builds upon existing knowledge or ideas. It involves organizing, reorganizing, or restructuring information to create a new framework or understanding. By integrating diverse perspectives or concepts, synthesis helps in generating comprehensive and innovative solutions.
5. Design tool: Synthesis is often used as a design tool to create new products, systems, or theories. By combining different elements or ideas, synthesis enables the development of innovative and functional solutions. It allows for the exploration of multiple possibilities and the creation of something new and valuable.
Interplay between Analysis and Synthesis
While analysis and synthesis are distinct processes, they are not mutually exclusive. In fact, they often complement each other and are interconnected in various ways. Analysis provides the foundation for synthesis by breaking down complex ideas or systems into manageable components. It helps in understanding the individual parts and their relationships, which is essential for effective synthesis.
On the other hand, synthesis builds upon the insights gained from analysis by integrating separate elements or ideas to create a new whole. It allows for a holistic understanding of complex phenomena, considering the interconnections and emergent properties that analysis alone may overlook. Synthesis also helps in identifying gaps or limitations in existing knowledge, which can then be further analyzed to gain a deeper understanding.
Furthermore, analysis and synthesis often involve an iterative process. Initial analysis may lead to the identification of patterns or relationships that can inform the synthesis process. Synthesis, in turn, may generate new insights or questions that require further analysis. This iterative cycle allows for continuous refinement and improvement of understanding.
Analysis and synthesis are two essential processes that play a crucial role in various fields of study. While analysis focuses on breaking down complex ideas into smaller components to understand their individual parts and relationships, synthesis involves integrating separate elements or ideas to create a new whole or understanding. Both approaches have their unique attributes and strengths, and they often complement each other in a cyclical and iterative process. By employing analysis and synthesis effectively, we can gain a comprehensive understanding of complex phenomena, generate innovative solutions, and make informed decisions.
Comparisons may contain inaccurate information about people, places, or facts. Please report any issues.
- Open access
- Published: 11 August 2009
Methods for the synthesis of qualitative research: a critical review
- Elaine Barnett-Page 1 &
- James Thomas 1
BMC Medical Research Methodology volume 9 , Article number: 59 ( 2009 ) Cite this article
198k Accesses
1142 Citations
40 Altmetric
Metrics details
In recent years, a growing number of methods for synthesising qualitative research have emerged, particularly in relation to health-related research. There is a need for both researchers and commissioners to be able to distinguish between these methods and to select which method is the most appropriate to their situation.
A number of methodological and conceptual links between these methods were identified and explored, while contrasting epistemological positions explained differences in approaches to issues such as quality assessment and extent of iteration. Methods broadly fall into 'realist' or 'idealist' epistemologies, which partly accounts for these differences.
Methods for qualitative synthesis vary across a range of dimensions. Commissioners of qualitative syntheses might wish to consider the kind of product they want and select their method – or type of method – accordingly.
Peer Review reports
The range of different methods for synthesising qualitative research has been growing over recent years [ 1 , 2 ], alongside an increasing interest in qualitative synthesis to inform health-related policy and practice [ 3 ]. While the terms 'meta-analysis' (a statistical method to combine the results of primary studies), or sometimes 'narrative synthesis', are frequently used to describe how quantitative research is synthesised, far more terms are used to describe the synthesis of qualitative research. This profusion of terms can mask some of the basic similarities in approach that the different methods share, and also lead to some confusion regarding which method is most appropriate in a given situation. This paper does not argue that the various nomenclatures are unnecessary, but rather seeks to draw together and review the full range of methods of synthesis available to assist future reviewers in selecting a method that is fit for their purpose. It also represents an attempt to guide the reader through some of the varied terminology to spring up around qualitative synthesis. Other helpful reviews of synthesis methods have been undertaken in recent years with slightly different foci to this paper. Two recent studies have focused on describing and critiquing methods for the integration of qualitative research with quantitative [ 4 , 5 ] rather than exclusively examining the detail and rationale of methods for the synthesis of qualitative research. Two other significant pieces of work give practical advice for conducting the synthesis of qualitative research, but do not discuss the full range of methods available [ 6 , 7 ]. We begin our Discussion by outlining each method of synthesis in turn, before comparing and contrasting characteristics of these different methods across a range of dimensions. Readers who are more familiar with the synthesis methods described here may prefer to turn straight to the 'dimensions of difference' analysis in the second part of the Discussion.
Overview of synthesis methods
Meta-ethnography.
In their seminal work of 1988, Noblit and Hare proposed meta-ethnography as an alternative to meta-analysis [ 8 ]. They cited Strike and Posner's [ 9 ] definition of synthesis as an activity in which separate parts are brought together to form a 'whole'; this construction of the whole is essentially characterised by some degree of innovation, so that the result is greater than the sum of its parts. They also borrowed from Turner's theory of social explanation [ 10 ], a key tenet of which was building 'comparative understanding' [[ 8 ], p22] rather than aggregating data.
To Noblit and Hare, synthesis provided an answer to the question of 'how to "put together" written interpretive accounts' [[ 8 ], p7], where mere integration would not be appropriate. Noblit and Hare's early work synthesised research from the field of education.
Three different methods of synthesis are used in meta-ethnography. One involves the 'translation' of concepts from individual studies into one another, thereby evolving overarching concepts or metaphors. Noblit and Hare called this process reciprocal translational analysis (RTA). Refutational synthesis involves exploring and explaining contradictions between individual studies. Lines-of-argument (LOA) synthesis involves building up a picture of the whole (i.e. culture, organisation etc) from studies of its parts. The authors conceptualised this latter approach as a type of grounded theorising.
Britten et al [ 11 ] and Campbell et al [ 12 ] have both conducted evaluations of meta-ethnography and claim to have succeeded, by using this method, in producing theories with greater explanatory power than could be achieved in a narrative literature review. While both these evaluations used small numbers of studies, more recently Pound et al [ 13 ] conducted both an RTA and an LOA synthesis using a much larger number of studies (37) on resisting medicines. These studies demonstrate that meta-ethnography has evolved since Noblit and Hare first introduced it. Campbell et al claim to have applied the method successfully to non-ethnographical studies. Based on their reading of Schutz [ 14 ], Britten et al have developed both second and third order constructs in their synthesis (Noblit and Hare briefly allude to the possibility of a 'second level of synthesis' [[ 8 ], p28] but do not demonstrate or further develop the idea).
In a more recent development, Sandelowski & Barroso [ 15 ] write of adapting RTA by using it to ' integrate findings interpretively, as opposed to comparing them interpretively' (p204). The former would involve looking to see whether the same concept, theory etc exists in different studies; the latter would involve the construction of a bigger picture or theory (i.e. LOA synthesis). They also talk about comparing or integrating imported concepts (e.g. from other disciplines) as well as those evolved 'in vivo'.
Grounded theory
Kearney [ 16 ], Eaves [ 17 ] and Finfgeld [ 18 ] have all adapted grounded theory to formulate a method of synthesis. Key methods and assumptions of grounded theory, as originally formulated and subsequently refined by Glaser and Strauss [ 19 ] and Strauss and Corbin [ 20 , 21 ], include: simultaneous phases of data collection and analysis; an inductive approach to analysis, allowing the theory to emerge from the data; the use of the constant comparison method; the use of theoretical sampling to reach theoretical saturation; and the generation of new theory. Eaves cited grounded theorists Charmaz [ 22 ] and Chesler [ 23 ], as well as Strauss and Corbin [ 20 ], as informing her approach to synthesis.
Glaser and Strauss [ 19 ] foresaw a time when a substantive body of grounded research should be pushed towards a higher, more abstract level. As a piece of methodological work, Eaves undertook her own synthesis of the synthesis methods used by these authors to produce her own clear and explicit guide to synthesis in grounded formal theory. Kearney stated that 'grounded formal theory', as she termed this method of synthesis, 'is suited to study of phenomena involving processes of contextualized understanding and action' [[ 24 ], p180] and, as such, is particularly applicable to nurses' research interests.
As Kearney suggested, the examples examined here were largely dominated by research in nursing. Eaves synthesised studies on care-giving in rural African-American families for elderly stroke survivors; Finfgeld on courage among individuals with long-term health problems; Kearney on women's experiences of domestic violence.
Kearney explicitly chose 'grounded formal theory' because it matches 'like' with 'like': that is, it applies the same methods that have been used to generate the original grounded theories included in the synthesis – produced by constant comparison and theoretical sampling – to generate a higher-level grounded theory. The wish to match 'like' with 'like' is also implicit in Eaves' paper. This distinguishes grounded formal theory from more recent applications of meta-ethnography, which have sought to include qualitative research using diverse methodological approaches [ 12 ].
- Thematic Synthesis
Thomas and Harden [ 25 ] have developed an approach to synthesis which they term 'thematic synthesis'. This combines and adapts approaches from both meta-ethnography and grounded theory. The method was developed out of a need to conduct reviews that addressed questions relating to intervention need, appropriateness and acceptability – as well as those relating to effectiveness – without compromising on key principles developed in systematic reviews. They applied thematic synthesis in a review of the barriers to, and facilitators of, healthy eating amongst children.
Free codes of findings are organised into 'descriptive' themes, which are then further interpreted to yield 'analytical' themes. This approach shares characteristics with later adaptations of meta-ethnography, in that the analytical themes are comparable to 'third order interpretations' and that the development of descriptive and analytical themes using coding invoke reciprocal 'translation'. It also shares much with grounded theory, in that the approach is inductive and themes are developed using a 'constant comparison' method. A novel aspect of their approach is the use of computer software to code the results of included studies line-by-line, thus borrowing another technique from methods usually used to analyse primary research.
Textual Narrative Synthesis
Textual narrative synthesis is an approach which arranges studies into more homogenous groups. Lucas et al [ 26 ] comment that it has proved useful in synthesising evidence of different types (qualitative, quantitative, economic etc). Typically, study characteristics, context, quality and findings are reported on according to a standard format and similarities and differences are compared across studies. Structured summaries may also be developed, elaborating on and putting into context the extracted data [ 27 ].
Lucas et al [ 26 ] compared thematic synthesis with textual narrative synthesis. They found that 'thematic synthesis holds most potential for hypothesis generation' whereas textual narrative synthesis is more likely to make transparent heterogeneity between studies (as does meta-ethnography, with refutational synthesis) and issues of quality appraisal. This is possibly because textual narrative synthesis makes clearer the context and characteristics of each study, while the thematic approach organises data according to themes. However, Lucas et al found that textual narrative synthesis is 'less good at identifying commonality' (p2); the authors do not make explicit why this should be, although it may be that organising according to themes, as the thematic approach does, is comparatively more successful in revealing commonality.
Paterson et al [ 28 ] have evolved a multi-faceted approach to synthesis, which they call 'meta-study'. The sociologist Zhao [ 29 ], drawing on Ritzer's work [ 30 ], outlined three components of analysis, which they proposed should be undertaken prior to synthesis. These are meta-data-analysis (the analysis of findings), meta-method (the analysis of methods) and meta-theory (the analysis of theory). Collectively, these three elements of analysis, culminating in synthesis, make up the practice of 'meta-study'. Paterson et al pointed out that the different components of analysis may be conducted concurrently.
Paterson et al argued that primary research is a construction; secondary research is therefore a construction of a construction. There is need for an approach that recognises this, and that also recognises research to be a product of its social, historical and ideological context. Such an approach would be useful in accounting for differences in research findings. For Paterson et al, there is no such thing as 'absolute truth'.
Meta-study was developed to study the experiences of adults living with a chronic illness. Meta-data-analysis was conceived of by Paterson et al in similar terms to Noblit and Hare's meta-ethnography (see above), in that it is essentially interpretive and seeks to reveal similarities and discrepancies among accounts of a particular phenomenon. Meta-method involves the examination of the methodologies of the individual studies under review. Part of the process of meta-method is to consider different aspects of methodology such as sampling, data collection, research design etc, similar to procedures others have called 'critical appraisal' (CASP [ 31 ]). However, Paterson et al take their critique to a deeper level by establishing the underlying assumptions of the methodologies used and the relationship between research outcomes and methods used. Meta-theory involves scrutiny of the philosophical and theoretical assumptions of the included research papers; this includes looking at the wider context in which new theory is generated. Paterson et al described meta-synthesis as a process which creates a new interpretation which accounts for the results of all three elements of analysis. The process of synthesis is iterative and reflexive and the authors were unwilling to oversimplify the process by 'codifying' procedures for bringing all three components of analysis together.
Meta-narrative
Greenhalgh et al [ 32 ]'s meta-narrative approach to synthesis arose out of the need to synthesise evidence to inform complex policy-making questions and was assisted by the formation of a multi-disciplinary team. Their approach to review was informed by Thomas Kuhn's The Structure of Scientific Revolutions [ 33 ], in which he proposed that knowledge is produced within particular paradigms which have their own assumptions about theory, about what is a legitimate object of study, about what are legitimate research questions and about what constitutes a finding. Paradigms also tend to develop through time according to a particular set of stages, central to which is the stage of 'normal science', in which the particular standards of the paradigm are largely unchallenged and seen to be self-evident. As Greenhalgh et al pointed out, Kuhn saw paradigms as largely incommensurable: 'that is, an empirical discovery made using one set of concepts, theories, methods and instruments cannot be satisfactorily explained through a different paradigmatic lens' [[ 32 ], p419].
Greenhalgh et al synthesised research from a wide range of disciplines; their research question related to the diffusion of innovations in health service delivery and organisation. They thus identified a need to synthesise findings from research which contains many different theories arising from many different disciplines and study designs.
Based on Kuhn's work, Greenhalgh et al proposed that, across different paradigms, there were multiple – and potentially mutually contradictory – ways of understanding the concept at the heart of their review, namely the diffusion of innovation. Bearing this in mind, the reviewers deliberately chose to select key papers from a number of different research 'paradigms' or 'traditions', both within and beyond healthcare, guided by their multidisciplinary research team. They took as their unit of analysis the 'unfolding "storyline" of a research tradition over time' [[ 32 ], p417) and sought to understand diffusion of innovation as it was conceptualised in each of these traditions. Key features of each tradition were mapped: historical roots, scope, theoretical basis; research questions asked and methods/instruments used; main empirical findings; historical development of the body of knowledge (how have earlier findings led to later findings); and strengths and limitations of the tradition. The results of this exercise led to maps of 13 'meta-narratives' in total, from which seven key dimensions, or themes, were identified and distilled for the synthesis phase of the review.
Critical Interpretive Synthesis
Dixon-Woods et al [ 34 ] developed their own approach to synthesising multi-disciplinary and multi-method evidence, termed 'critical interpretive synthesis', while researching access to healthcare by vulnerable groups. Critical interpretive synthesis is an adaptation of meta-ethnography, as well as borrowing techniques from grounded theory. The authors stated that they needed to adapt traditional meta-ethnographic methods for synthesis, since these had never been applied to quantitative as well as qualitative data, nor had they been applied to a substantial body of data (in this case, 119 papers).
Dixon-Woods et al presented critical interpretive synthesis as an approach to the whole process of review, rather than to just the synthesis component. It involves an iterative approach to refining the research question and searching and selecting from the literature (using theoretical sampling) and defining and applying codes and categories. It also has a particular approach to appraising quality, using relevance – i.e. likely contribution to theory development – rather than methodological characteristics as a means of determining the 'quality' of individual papers [ 35 ]. The authors also stress, as a defining characteristic, critical interpretive synthesis's critical approach to the literature in terms of deconstructing research traditions or theoretical assumptions as a means of contextualising findings.
Dixon-Woods et al rejected reciprocal translational analysis (RTA) as this produced 'only a summary in terms that have already been used in the literature' [[ 34 ], p5], which was seen as less helpful when dealing with a large and diverse body of literature. Instead, Dixon-Woods et al adopted a lines-of-argument (LOA) synthesis, in which – rejecting the difference between first, second and third order constructs – they instead developed 'synthetic constructs' which were then linked with constructs arising directly from the literature.
The influence of grounded theory can be seen in particular in critical interpretive synthesis's inductive approach to formulating the review question and to developing categories and concepts, rejecting a 'stage' approach to systematic reviewing, and in selecting papers using theoretical sampling. Dixon-Woods et al also claim that critical interpretive synthesis is distinct in its 'explicit orientation towards theory generation' [[ 34 ], p9].
Ecological Triangulation
Jim Banning is the author of 'ecological triangulation' or 'ecological sentence synthesis', applying this method to the evidence for what works for youth with disabilities. He borrows from Webb et al [ 36 ] and Denzin [ 37 ] the concept of triangulation, in which phenomena are studied from a variety of vantage points. His rationale is that building an 'evidence base' of effectiveness requires the synthesis of cumulative, multi-faceted evidence in order to find out 'what intervention works for what kind of outcomes for what kind of persons under what kind of conditions' [[ 38 ], p1].
Ecological triangulation unpicks the mutually interdependent relationships between behaviour, persons and environments. The method requires that, for data extraction and synthesis, 'ecological sentences' are formulated following the pattern: 'With this intervention, these outcomes occur with these population foci and within these grades (ages), with these genders ... and these ethnicities in these settings' [[ 39 ], p1].
Framework Synthesis
Brunton et al [ 40 ] and Oliver et al [ 41 ] have applied a 'framework synthesis' approach in their reviews. Framework synthesis is based on framework analysis, which was outlined by Pope, Ziebland and Mays [ 42 ], and draws upon the work of Ritchie and Spencer [ 43 ] and Miles and Huberman [ 44 ]. Its rationale is that qualitative research produces large amounts of textual data in the form of transcripts, observational fieldnotes etc. The sheer wealth of information poses a challenge for rigorous analysis. Framework synthesis offers a highly structured approach to organising and analysing data (e.g. indexing using numerical codes, rearranging data into charts etc).
Brunton et al applied the approach to a review of children's, young people's and parents' views of walking and cycling; Oliver et al to an analysis of public involvement in health services research. Framework synthesis is distinct from the other methods outlined here in that it utilises an a priori 'framework' – informed by background material and team discussions – to extract and synthesise findings. As such, it is largely a deductive approach although, in addition to topics identified by the framework, new topics may be developed and incorporated as they emerge from the data. The synthetic product can be expressed in the form of a chart for each key dimension identified, which may be used to map the nature and range of the concept under study and find associations between themes and exceptions to these [ 40 ].
'Fledgling' approaches
There are three other approaches to synthesis which have not yet been widely used. One is an approach using content analysis [ 45 , 46 ] in which text is condensed into fewer content-related categories. Another is 'meta-interpretation' [ 47 ], featuring the following: an ideographic rather than pre-determined approach to the development of exclusion criteria; a focus on meaning in context; interpretations as raw data for synthesis (although this feature doesn't distinguish it from other synthesis methods); an iterative approach to the theoretical sampling of studies for synthesis; and a transparent audit trail demonstrating the trustworthiness of the synthesis.
In addition to the synthesis methods discussed above, Sandelowski and Barroso propose a method they call 'qualitative metasummary' [ 15 ]. It is mentioned here as a new and original approach to handling a collection of qualitative studies but is qualitatively different to the other methods described here since it is aggregative; that is, findings are accumulated and summarised rather than 'transformed'. Metasummary is a way of producing a 'map' of the contents of qualitative studies and – according to Sandelowski and Barroso – 'reflect [s] a quantitative logic' [[ 15 ], p151]. The frequency of each finding is determined and the higher the frequency of a particular finding, the greater its validity. The authors even discuss the calculation of 'effect sizes' for qualitative findings. Qualitative metasummaries can be undertaken as an end in themselves or may serve as a basis for a further synthesis.
Dimensions of difference
Having outlined the range of methods identified, we now turn to an examination of how they compare with one another. It is clear that they have come from many different contexts and have different approaches to understanding knowledge, but what do these differences mean in practice? Our framework for this analysis is shown in Additional file 1 : dimensions of difference [ 48 ]. We have examined the epistemology of each of the methods and found that, to some extent, this explains the need for different methods and their various approaches to synthesis.
Epistemology
The first dimension that we will consider is that of the researchers' epistemological assumptions. Spencer et al [ 49 ] outline a range of epistemological positions, which might be organised into a spectrum as follows:
Subjective idealism : there is no shared reality independent of multiple alternative human constructions
Objective idealism : there is a world of collectively shared understandings
Critical realism : knowledge of reality is mediated by our perceptions and beliefs
Scientific realism : it is possible for knowledge to approximate closely an external reality
Naïve realism : reality exists independently of human constructions and can be known directly [ 49 , 45 , 46 ].
Thus, at one end of the spectrum we have a highly constructivist view of knowledge and, at the other, an unproblematized 'direct window onto the world' view.
Nearly all of positions along this spectrum are represented in the range of methodological approaches to synthesis covered in this paper. The originators of meta-narrative synthesis, critical interpretive synthesis and meta-study all articulate what might be termed a 'subjective idealist' approach to knowledge. Paterson et al [ 28 ] state that meta-study shies away from creating 'grand theories' within the health or social sciences and assume that no single objective reality will be found. Primary studies, they argue, are themselves constructions; meta-synthesis, then, 'deals with constructions of constructions' (p7). Greenhalgh et al [ 32 ] also view knowledge as a product of its disciplinary paradigm and use this to explain conflicting findings: again, the authors neither seek, nor expect to find, one final, non-contestable answer to their research question. Critical interpretive synthesis is similar in seeking to place literature within its context, to question its assumptions and to produce a theoretical model of a phenomenon which – because highly interpretive – may not be reproducible by different research teams at alternative points in time [[ 34 ], p11].
Methods used to synthesise grounded theory studies in order to produce a higher level of grounded theory [ 24 ] appear to be informed by 'objective idealism', as does meta-ethnography. Kearney argues for the near-universal applicability of a 'ready-to-wear' theory across contexts and populations. This approach is clearly distinct from one which recognises multiple realities. The emphasis is on examining commonalities amongst, rather than discrepancies between, accounts. This emphasis is similarly apparent in most meta-ethnographies, which are conducted either according to Noblit and Hare's 'reciprocal translational analysis' technique or to their 'lines-of-argument' technique and which seek to provide a 'whole' which has a greater explanatory power. Although Noblit and Hare also propose 'refutational synthesis', in which contradictory findings might be explored, there are few examples of this having been undertaken in practice, and the aim of the method appears to be to explain and explore differences due to context, rather than multiple realities.
Despite an assumption of a reality which is perhaps less contestable than those of meta-narrative synthesis, critical interpretive synthesis and meta-study, both grounded formal theory and meta-ethnography place a great deal of emphasis on the interpretive nature of their methods. This still supposes a degree of constructivism. Although less explicit about how their methods are informed, it seems that both thematic synthesis and framework synthesis – while also involving some interpretation of data – share an even less problematized view of reality and a greater assumption that their synthetic products are reproducible and correspond to a shared reality. This is also implicit in the fact that such products are designed directly to inform policy and practice, a characteristic shared by ecological triangulation. Notably, ecological triangulation, according to Banning, can be either realist or idealist. Banning argues that the interpretation of triangulation can either be one in which multiple viewpoints converge on a point to produce confirming evidence (i.e. one definitive answer to the research question) or an idealist one, in which the complexity of multiple viewpoints is represented. Thus, although ecological triangulation views reality as complex, the approach assumes that it can be approximately knowable (at least when the realist view of ecological triangulation is adopted) and that interventions can and should be modelled according to the products of its syntheses.
While pigeonholing different methods into specific epistemological positions is a problematic process, we do suggest that the contrasting epistemologies of different researchers is one way of explaining why we have – and need – different methods for synthesis.
Variation in terms of the extent of iteration during the review process is another key dimension. All synthesis methods include some iteration but the degree varies. Meta-ethnography, grounded theory and thematic synthesis all include iteration at the synthesis stage; both framework synthesis and critical interpretive synthesis involve iterative literature searching – in the case of critical interpretive synthesis, it is not clear whether iteration occurs during the rest of the review process. Meta-narrative also involves iteration at every stage. Banning does not mention iteration in outlining ecological triangulation and neither do Lucas or Thomas and Harden for thematic narrative synthesis.
It seems that the more idealist the approach, the greater the extent of iteration. This might be because a large degree of iteration does not sit well with a more 'positivist' ideal of procedural objectivity; in particular, the notion that the robustness of the synthetic product depends in part on the reviewers stating up front in a protocol their searching strategies, inclusion/exclusion criteria etc, and being seen not to alter these at a later stage.
Quality assessment
Another dimension along which we can look at different synthesis methods is that of quality assessment. When the approaches to the assessment of the quality of studies retrieved for review are examined, there is again a wide methodological variation. It might be expected that the further towards the 'realism' end of the epistemological spectrum a method of synthesis falls, the greater the emphasis on quality assessment. In fact, this is only partially the case.
Framework synthesis, thematic narrative synthesis and thematic synthesis – methods which might be classified as sharing a 'critical realist' approach – all have highly specified approaches to quality assessment. The review in which framework synthesis was developed applied ten quality criteria: two on quality and reporting of sampling methods, four to the quality of the description of the sample in the study, two to the reliability and validity of the tools used to collect data and one on whether studies used appropriate methods for helping people to express their views. Studies which did not meet a certain number of quality criteria were excluded from contributing to findings. Similarly, in the example review for thematic synthesis, 12 criteria were applied: five related to reporting aims, context, rationale, methods and findings; four relating to reliability and validity; and three relating to the appropriateness of methods for ensuring that findings were rooted in participants' own perspectives. Studies which were deemed to have significant flaws were excluded and sensitivity analyses were used to assess the possible impact of study quality on the review's findings. Thomas and Harden's use of thematic narrative synthesis similarly applied quality criteria and developed criteria additional to those they found in the literature on quality assessment, relating to the extent to which people's views and perspectives had been privileged by researchers. It is worth noting not only that these methods apply quality criteria but that they are explicit about what they are: assessing quality is a key component in the review process for both of these methods. Likewise, Banning – the originator of ecological triangulation – sees quality assessment as important and adapts the Design and Implementation Assessment Device (DIAD) Version 0.3 (a quality assessment tool for quantitative research) for use when appraising qualitative studies [ 50 ]. Again, Banning writes of excluding studies deemed to be of poor quality.
Greenhalgh et al's meta-narrative review [ 32 ] modified a range of existing quality assessment tools to evaluate studies according to validity and robustness of methods; sample size and power; and validity of conclusions. The authors imply, but are not explicit, that this process formed the basis for the exclusion of some studies. Although not quite so clear about quality assessment methods as framework and thematic synthesis, it might be argued that meta-narrative synthesis shows a greater commitment to the concept that research can and should be assessed for quality than either meta-ethnography or grounded formal theory. The originators of meta-ethnography, Noblit and Hare [ 8 ], originally discussed quality in terms of quality of metaphor, while more recent use of this method has used amended versions of CASP (the Critical Appraisal Skills Programme tool, [ 31 ]), yet has only referred to studies being excluded on the basis of lack of relevance or because they weren't 'qualitative' studies [ 8 ]. In grounded theory, quality assessment is only discussed in terms of a 'personal note' being made on the context, quality and usefulness of each study. However, contrary to expectation, meta-narrative synthesis lies at the extreme end of the idealism/realism spectrum – as a subjective idealist approach – while meta-ethnography and grounded theory are classified as objective idealist approaches.
Finally, meta-study and critical interpretive synthesis – two more subjective idealist approaches – look to the content and utility of findings rather than methodology in order to establish quality. While earlier forms of meta-study included only studies which demonstrated 'epistemological soundness', in its most recent form [ 51 ] this method has sought to include all relevant studies, excluding only those deemed not to be 'qualitative' research. Critical interpretive synthesis also conforms to what we might expect of its approach to quality assessment: quality of research is judged as the extent to which it informs theory. The threshold of inclusion is informed by expertise and instinct rather than being articulated a priori.
In terms of quality assessment, it might be important to consider the academic context in which these various methods of synthesis developed. The reason why thematic synthesis, framework synthesis and ecological triangulation have such highly specified approaches to quality assessment may be that each of these was developed for a particular task, i.e. to conduct a multi-method review in which randomised controlled trials (RCTs) were included. The concept of quality assessment in relation to RCTs is much less contested and there is general agreement on criteria against which quality should be judged.
Problematizing the literature
Critical interpretive synthesis, the meta-narrative approach and the meta-theory element of meta-study all share some common ground in that their review and synthesis processes include examining all aspects of the context in which knowledge is produced. In conducting a review on access to healthcare by vulnerable groups, critical interpretive synthesis sought to question 'the ways in which the literature had constructed the problematics of access, the nature of the assumptions on which it drew, and what has influenced its choice of proposed solutions' [[ 34 ], p6]. Although not claiming to have been directly influenced by Greenhalgh et al's meta-narrative approach, Dixon-Woods et al do cite it as sharing similar characteristics in the sense that it critiques the literature it reviews.
Meta-study uses meta-theory to describe and deconstruct the theories that shape a body of research and to assess its quality. One aspect of this process is to examine the historical evolution of each theory and to put it in its socio-political context, which invites direct comparison with meta-narrative synthesis. Greenhalgh et al put a similar emphasis on placing research findings within their social and historical context, often as a means of seeking to explain heterogeneity of findings. In addition, meta-narrative shares with critical interpretive synthesis an iterative approach to searching and selecting from the literature.
Framework synthesis, thematic synthesis, textual narrative synthesis, meta-ethnography and grounded theory do not share the same approach to problematizing the literature as critical interpretive synthesis, meta-study and meta-narrative. In part, this may be explained by the extent to which studies included in the synthesis represented a broad range of approaches or methodologies. This, in turn, may reflect the broadness of the review question and the extent to which the concepts contained within the question are pre-defined within the literature. In the case of both the critical interpretive synthesis and meta-narrative reviews, terminology was elastic and/or the question formed iteratively. Similarly, both reviews placed great emphasis on employing multi-disciplinary research teams. Approaches which do not critique the literature in the same way tend to have more narrowly-focused questions. They also tend to include a more limited range of studies: grounded theory synthesis includes grounded theory studies, meta-ethnography (in its original form, as applied by Noblit and Hare) ethnographies. The thematic synthesis incorporated studies based on only a narrow range of qualitative methodologies (interviews and focus groups) which were informed by a similarly narrow range of epistemological assumptions. It may be that the authors of such syntheses saw no need for including such a critique in their review process.
Similarities and differences between primary studies
Most methods of synthesis are applicable to heterogeneous data (i.e. studies which use contrasting methodologies) apart from early meta-ethnography and synthesis informed by grounded theory. All methods of synthesis state that, at some level, studies are compared; many are not so explicit about how this is done, though some are. Meta-ethnography is one of the most explicit: it describes the act of 'translation' where terms and concepts which have resonance with one another are subsumed into 'higher order constructs'. Grounded theory, as represented by Eaves [ 17 ], is undertaken according to a long list of steps and sub-steps, includes the production of generalizations about concepts/categories, which comes from classifying these categories. In meta-narrative synthesis, comparable studies are grouped together at the appraisal phase of review.
Perhaps more interesting are the ways in which differences between studies are explored. Those methods with a greater emphasis on critical appraisal may tend (although this is not always made explicit) to use differences in method to explain differences in finding. Meta-ethnography proposes 'refutational synthesis' to explain differences, although there are few examples of this in the literature. Some synthesis methods – for example, thematic synthesis – look at other characteristics of the studies under review, whether types of participants and their context vary, and whether this can explain differences in perspective.
All of these methods, then, look within the studies to explain differences. Other methods look beyond the study itself to the context in which it was produced. Critical interpretive synthesis and meta-study look at differences in theory or in socio-economic context. Critical interpretive synthesis, like meta-narrative, also explores epistemological orientation. Meta-narrative is unique in concerning itself with disciplinary paradigm (i.e. the story of the discipline as it progresses). It is also distinctive in that it treats conflicting findings as 'higher order data' [[ 32 ], p420], so that the main emphasis of the synthesis appears to be on examining and explaining contradictions in the literature.
Going 'beyond' the primary studies
Synthesis is sometimes defined as a process resulting in a product, a 'whole', which is more than the sum of its parts. However, the methods reviewed here vary in the extent to which they attempt to 'go beyond' the primary studies and transform the data. Some methods – textual narrative synthesis, ecological triangulation and framework synthesis – focus on describing and summarising their primary data (often in a highly structured and detailed way) and translating the studies into one another. Others – meta-ethnography, grounded theory, thematic synthesis, meta-study, meta-narrative and critical interpretive synthesis – seek to push beyond the original data to a fresh interpretation of the phenomena under review. A key feature of thematic synthesis is its clear differentiation between these two stages.
Different methods have different mechanisms for going beyond the primary studies, although some are more explicit than others about what these entail. Meta-ethnography proposes a 'Line of Argument' (LOA) synthesis in which an interpretation is constructed to both link and explain a set of parts. Critical interpretive synthesis based its synthesis methods on those of meta-ethnography, developing an LOA using what the authors term 'synthetic constructs' (akin to 'third order constructs' in meta-ethnography) to create a 'synthesising argument'. Dixon-Woods et al claim that this is an advance on Britten et al's methods, in that they reject the difference between first, second and third order constructs.
Meta-narrative, as outlined above, focuses on conflicting findings and constructs theories to explain these in terms of differing paradigms. Meta study derives questions from each of its three components to which it subjects the dataset and inductively generates a number of theoretical claims in relation to it. According to Eaves' model of grounded theory [ 17 ], mini-theories are integrated to produce an explanatory framework. In ecological triangulation, the 'axial' codes – or second level codes evolved from the initial deductive open codes – are used to produce Banning's 'ecological sentence' [ 39 ].
The synthetic product
In overviewing and comparing different qualitative synthesis methods, the ultimate question relates to the utility of the synthetic product: what is it for? It is clear that some methods of synthesis – namely, thematic synthesis, textual narrative synthesis, framework synthesis and ecological triangulation – view themselves as producing an output that is directly applicable to policy makers and designers of interventions. The example of framework synthesis examined here (on children's, young people's and parents' views of walking and cycling) involved policy makers and practitioners in directing the focus of the synthesis and used the themes derived from the synthesis to infer what kind of interventions might be most effective in encouraging walking and cycling. Likewise, the products of the thematic synthesis took the form of practical recommendations for interventions (e.g. 'do not promote fruit and vegetables in the same way in the same intervention'). The extent to which policy makers and practitioners are involved in informing either synthesis or recommendation is less clear from the documents published on ecological triangulation, but the aim certainly is to directly inform practice.
The outputs of synthesis methods which have a more constructivist orientation – meta-study, meta-narrative, meta-ethnography, grounded theory, critical interpretive synthesis – tend to look rather different. They are generally more complex and conceptual, sometimes operating on the symbolic or metaphorical level, and requiring a further process of interpretation by policy makers and practitioners in order for them to inform practice. This is not to say, however, that they are not useful for practice, more that they are doing different work. However, it may be that, in the absence of further interpretation, they are more useful for informing other researchers and theoreticians.
Looking across dimensions
After examining the dimensions of difference of our included methods, what picture ultimately emerges? It seems clear that, while similar in some respects, there are genuine differences in approach to the synthesis of what is essentially textual data. To some extent, these differences can be explained by the epistemological assumptions that underpin each method. Our methods split into two broad camps: the idealist and the realist (see Table 1 for a summary). Idealist approaches generally tend to have a more iterative approach to searching (and the review process), have less a priori quality assessment procedures and are more inclined to problematize the literature. Realist approaches are characterised by a more linear approach to searching and review, have clearer and more well-developed approaches to quality assessment, and do not problematize the literature.
Mapping the relationships between methods
What is interesting is the relationship between these methods of synthesis, the conceptual links between them, and the extent to which the originators cite – or, in some cases, don't cite – one another. Some methods directly build on others – framework synthesis builds on framework analysis, for example, while grounded theory and constant comparative analysis build on grounded theory. Others further develop existing methods – meta-study, critical interpretive synthesis and meta-narrative all adapt aspects of meta-ethnography, while also importing concepts from other theorists (critical interpretive synthesis also adapts grounded theory techniques).
Some methods share a clear conceptual link, without directly citing one another: for example, the analytical themes developed during thematic synthesis are comparable to the third order interpretations of meta-ethnography. The meta-theory aspect of meta-study is echoed in both meta-narrative synthesis and critical interpretive synthesis (see 'Problematizing the literature, above); however, the originators of critical interpretive synthesis only refer to the originators of meta-study in relation to their use of sampling techniques.
While methods for qualitative synthesis have many similarities, there are clear differences in approach between them, many of which can be explained by taking account of a given method's epistemology.
However, within the two broad idealist/realist categories, any differences between methods in terms of outputs appear to be small.
Since many systematic reviews are designed to inform policy and practice, it is important to select a method – or type of method – that will produce the kind of conclusions needed. However, it is acknowledged that this is not always simple or even possible to achieve in practice.
The approaches that result in more easily translatable messages for policy-makers and practitioners may appear to be more attractive than the others; but we do need to take account lessons from the more idealist end of the spectrum, that some perspectives are not universal.
Dixon-Woods M, Agarwhal S, Jones D, Young B, Sutton A: Synthesising qualitative and quantitative evidence: a review of possible methods. J Health Serv Res Pol. 2005, 10 (1): 45-53b. 10.1258/1355819052801804.
Article Google Scholar
Barbour RS, Barbour M: Evaluating and synthesizing qualitative research: the need to develop a distinctive approach. J Eval Clin Pract. 2003, 9 (2): 179-186. 10.1046/j.1365-2753.2003.00371.x.
Article PubMed Google Scholar
Mays N, Pope C, Popay J: Systematically reviewing qualitative and quantitative evidence to inform management and policy-making in the health field. J Health Serv Res Pol. 2005, 10 (Suppl 1): 6-20. 10.1258/1355819054308576.
Dixon-Woods M, Bonas S, Booth A, Jones DR, Miller T, Shaw RL, Smith J, Sutton A, Young B: How can systematic reviews incorporate qualitative research? A critical perspective. Qual Res. 2006, 6: 27-44. 10.1177/1468794106058867.
Pope C, Mays N, Popay J: Synthesizing Qualitative and Quantitative Health Evidence: a Guide to Methods. 2007, Maidenhead: Open University Press
Google Scholar
Thorne S, Jenson L, Kearney MH, Noblit G, Sandelowski M: Qualitative metasynthesis: reflections on methodological orientation and ideological agenda. Qual Health Res. 2004, 14: 1342-1365. 10.1177/1049732304269888.
Centre for Reviews and Dissemination: Systematic Reviews. CRD's Guidance for Undertaking Reviews in Health Care. 2008, York: CRD
Noblit GW, Hare RD: Meta-Ethnography: Synthesizing Qualitative Studies. 1988, London: Sage
Book Google Scholar
Strike K, Posner G: Types of synthesis and their criteria. Knowledge Structure and Use. Edited by: Ward S, Reed L. 1983, Philadelphia: Temple University Press
Turner S: Sociological Explanation as Translation. 1980, New York: Cambridge University Press
Britten N, Campbell R, Pope C, Donovan J, Morgan M, Pill R: Using meta-ethnography to synthesis qualitative research: a worked example. J Health Serv Res. 2002, 7: 209-15. 10.1258/135581902320432732.
Campbell R, Pound P, Pope C, Britten N, Pill R, Morgan M, Donovan J: Evaluating meta-ethnography: a synthesis of qualitative research on lay experiences of diabetes and diabetes care. Soc Sci Med. 2003, 65: 671-84. 10.1016/S0277-9536(02)00064-3.
Pound P, Britten N, Morgan M, Yardley L, Pope C, Daker-White G, Campbell R: Resisting medicines: a synthesis of qualitative studies of medicine taking. Soc Sci Med. 2005, 61: 133-155. 10.1016/j.socscimed.2004.11.063.
Schutz A: Collected Paper. 1962, The Hague: Martinus Nijhoff, 1:
Sandelowski M, Barroso J: Handbook for Synthesizing Qualitative Research. 2007, New York: Springer Publishing Company
Kearney MH: Enduring love: a grounded formal theory of women's experience of domestic violence. Research Nurs Health. 2001, 24: 270-82. 10.1002/nur.1029.
Article CAS Google Scholar
Eaves YD: A synthesis technique for grounded theory data analysis. J Adv Nurs. 2001, 35: 654-63. 10.1046/j.1365-2648.2001.01897.x.
Article CAS PubMed Google Scholar
Finfgeld D: Courage as a process of pushing beyond the struggle. Qual Health Res. 1999, 9: 803-814. 10.1177/104973299129122298.
Glaser BG, Strauss AL: The Discovery of Grounded Theory: Strategies for Qualitative Research. 1967, New York: Aldine De Gruyter
Strauss AL, Corbin J: Basics of Qualitative Research: Grounded Theory Procedures and Techniques. 1990, Newbury Park, CA: Sage
Strauss AL, Corbin J: Basics of Qualitative Research: Techniques and Procedures for Developing Grounded Theory. 1998, Thousand Oaks, CA: Sage
Charmaz K: The grounded theory method: an explication and interpretation. Contemporary Field Research: A Collection of Readings. Edited by: Emerson RM. 1983, Waveland Press: Prospect Heights, IL, 109-126.
Chesler MA: Professionals' Views of the Dangers of Self-Help Groups: Explicating a Grounded Theoretical Approach. 1987, [Michigan]: Department of Sociology, University of Michigan, Ann Arbour Centre for Research on Social Organisation, Working Paper Series
Kearney MH: Ready-to-wear: discovering grounded formal theory. Res Nurs Health. 1988, 21: 179-186. 10.1002/(SICI)1098-240X(199804)21:2<179::AID-NUR8>3.0.CO;2-G.
Thomas J, Harden A: Methods for the thematic synthesis of qualitative research in systematic reviews. BMC Med Res Meth. 2008, 8: 45-10.1186/1471-2288-8-45.
Lucas PJ, Arai L, Baird , Law C, Roberts HM: Worked examples of alternative methods for the synthesis of qualitative and quantitative research in systematic reviews. BMC Med Res Meth. 2007, 7 (4):
Harden A, Garcia J, Oliver S, Rees R, Shepherd J, Brunton G, Oakley A: Applying systematic review methods to studies of people's views: an example from public health research. J Epidemiol Community H. 2004, 58: 794-800. 10.1136/jech.2003.014829.
Paterson BL, Thorne SE, Canam C, Jillings C: Meta-Study of Qualitative Health Research. A Practical Guide to Meta-Analysis and Meta-Synthesis. 2001, Thousand Oaks, CA: Sage Publications
Zhao S: Metatheory, metamethod, meta-data-analysis: what, why and how?. Sociol Perspect. 1991, 34: 377-390.
Ritzer G: Metatheorizing in Sociology. 1991, Lexington, MA: Lexington Books
CASP (Critical Appraisal Skills Programme). date unknown, [ http://www.phru.nhs.uk/Pages/PHD/CASP.htm ]
Greenhalgh T, Robert G, Macfarlane F, Bate P, Kyriakidou O, Peacock R: Storylines of research in diffusion of innovation: a meta-narrative approach to systematic review. Soc Sci Med. 2005, 61: 417-30. 10.1016/j.socscimed.2004.12.001.
Kuhn TS: The Structure of Scientific Revolutions. 1962, Chicago: University of Chicago Press
Dixon-Woods M, Cavers D, Agarwal S, Annandale E, Arthur A, Harvey J, Hsu R, Katbamna S, Olsen R, Smith L, Riley R, Sutton AJ: Conducting a critical interpretive synthesis of the literature on access to healthcare by vulnerable groups. BMC Med Res Meth. 2006, 6 (35):
Gough D: Weight of evidence: a framework for the appraisal of the quality and relevance of evidence. Applied and Practice-based Research. Edited by: Furlong J, Oancea A. 2007, Special Edition of Research Papers in Education, 22 (2): 213-228.
Webb EJ, Campbell DT, Schwartz RD, Sechrest L: Unobtrusive Measures. 1966, Chicago: Rand McNally
Denzin NK: The Research Act: a Theoretical Introduction to Sociological Methods. 1978, New York: McGraw-Hill
Banning J: Ecological Triangulation. [ http://mycahs.colostate.edu/James.H.Banning/PDFs/Ecological%20Triangualtion.pdf ]
Banning J: Ecological Sentence Synthesis. [ http://mycahs.colostate.edu/James.H.Banning/PDFs/Ecological%20Sentence%20Synthesis.pdf ]
Brunton G, Oliver S, Oliver K, Lorenc T: A Synthesis of Research Addressing Children's, Young People's and Parents' Views of Walking and Cycling for Transport. 2006, London: EPPI-Centre, Social Science Research Unit, Institute of Education, University of London
Oliver S, Rees R, Clarke-Jones L, Milne R, Oakley A, Gabbay J, Stein K, Buchanan P, Gyte G: A multidimensional conceptual framework for analysing public involvement in health services research. Health Expect. 2008, 11: 72-84. 10.1111/j.1369-7625.2007.00476.x.
Pope C, Ziebland S, Mays N: Qualitative research in health care: analysing qualitative data. BMJ. 2000, 320: 114-116. 10.1136/bmj.320.7227.114.
Article CAS PubMed PubMed Central Google Scholar
Ritchie J, Spencer L: Qualitative data analysis for applied policy research. Analysing Qualitative Data. Edited by: Bryman A, Burgess R. 1993, London: Routledge, 173-194.
Miles M, Huberman A: Qualitative Data Analysis. 1984, London: Sage
Evans D, Fitzgerald M: Reasons for physically restraining patients and residents: a systematic review and content analysis. Int J Nurs Stud. 2002, 39: 739-743. 10.1016/S0020-7489(02)00015-9.
Suikkala A, Leino-Kilpi H: Nursing student-patient relationships: a review of the literature from 1984–1998. J Adv Nurs. 2000, 33: 42-50. 10.1046/j.1365-2648.2001.01636.x.
Weed M: 'Meta-interpretation': a method for the interpretive synthesis of qualitative research. Forum: Qual Soc Res. 2005, 6: Art 37-
Gough D, Thomas J: Dimensions of difference in systematic reviews. [ http://www.ncrm.ac.uk/RMF2008/festival/programme/sys1 ]
Spencer L, Ritchie J, Lewis J, Dillon L: Quality in Qualitative Evaluation: a Framework for Assessing Research Evidence. 2003, London: Government Chief Social Researcher's Office
Banning J: Design and Implementation Assessment Device (DIAD) Version 0.3: A response from a qualitative perspective. [ http://mycahs.colostate.edu/James.H.Banning/PDFs/Design%20and%20Implementation%20Assessment%20Device.pdf ]
Paterson BL: Coming out as ill: understanding self-disclosure in chronic illness from a meta-synthesis of qualitative research. Reviewing Research Evidence for Nursing Practice. Edited by: Webb C, Roe B. 2007, [Oxford]: Blackwell Publishing Ltd, 73-83.
Chapter Google Scholar
Pre-publication history
The pre-publication history for this paper can be accessed here: http://www.biomedcentral.com/1471-2288/9/59/prepub
Download references
Acknowledgements
The authors would like to acknowledge the helpful contributions of the following in commenting on earlier drafts of this paper: David Gough, Sandy Oliver, Angela Harden, Mary Dixon-Woods, Trisha Greenhalgh and Barbara L. Paterson. We would also like to thank the peer reviewers: Helen J Smith, Rosaline Barbour and Mark Rodgers for their helpful reviews. The methodological development was supported by the Department of Health (England) and the ESRC through the Methods for Research Synthesis Node of the National Centre for Research Methods (NCRM). An earlier draft of this paper currently appears as a working paper on the National Centre for Research Methods' website http://www.ncrm.ac.uk/ .
Author information
Authors and affiliations.
Social Science Research Unit, Evidence for Policy and Practice Information and Co-ordinating (EPPI-) Centre, 18 Woburn Square, London, WC1H 0NS, UK
Elaine Barnett-Page & James Thomas
You can also search for this author in PubMed Google Scholar
Corresponding author
Correspondence to Elaine Barnett-Page .
Additional information
Competing interests.
The authors declare that they have no competing interests.
Authors' contributions
Both authors made substantial contributions, with EBP taking a lead on writing and JT on the analytical framework. Both authors read and approved the final manuscript.
Electronic supplementary material
12874_2009_375_moesm1_esm.doc.
Additional file 1: Dimensions of difference. Ranging from subjective idealism through objective idealism and critical realism to scientific realism to naïve realism (DOC 46 KB)
Rights and permissions
This article is published under license to BioMed Central Ltd. This is an Open Access article distributed under the terms of the Creative Commons Attribution License ( http://creativecommons.org/licenses/by/2.0 ), which permits unrestricted use, distribution, and reproduction in any medium, provided the original work is properly cited.
Reprints and permissions
About this article
Cite this article.
Barnett-Page, E., Thomas, J. Methods for the synthesis of qualitative research: a critical review. BMC Med Res Methodol 9 , 59 (2009). https://doi.org/10.1186/1471-2288-9-59
Download citation
Received : 09 March 2009
Accepted : 11 August 2009
Published : 11 August 2009
DOI : https://doi.org/10.1186/1471-2288-9-59
Share this article
Anyone you share the following link with will be able to read this content:
Sorry, a shareable link is not currently available for this article.
Provided by the Springer Nature SharedIt content-sharing initiative
- Narrative Synthesis
- Theoretical Sampling
- Qualitative Synthesis
- Order Construct
BMC Medical Research Methodology
ISSN: 1471-2288
- General enquiries: [email protected]
Thank you for visiting nature.com. You are using a browser version with limited support for CSS. To obtain the best experience, we recommend you use a more up to date browser (or turn off compatibility mode in Internet Explorer). In the meantime, to ensure continued support, we are displaying the site without styles and JavaScript.
- View all journals
- Explore content
- About the journal
- Publish with us
- Sign up for alerts
- Review Article
- Published: 08 March 2018
Meta-analysis and the science of research synthesis
- Jessica Gurevitch 1 ,
- Julia Koricheva 2 ,
- Shinichi Nakagawa 3 , 4 &
- Gavin Stewart 5
Nature volume 555 , pages 175–182 ( 2018 ) Cite this article
55k Accesses
894 Citations
738 Altmetric
Metrics details
- Biodiversity
- Outcomes research
Meta-analysis is the quantitative, scientific synthesis of research results. Since the term and modern approaches to research synthesis were first introduced in the 1970s, meta-analysis has had a revolutionary effect in many scientific fields, helping to establish evidence-based practice and to resolve seemingly contradictory research outcomes. At the same time, its implementation has engendered criticism and controversy, in some cases general and others specific to particular disciplines. Here we take the opportunity provided by the recent fortieth anniversary of meta-analysis to reflect on the accomplishments, limitations, recent advances and directions for future developments in the field of research synthesis.
This is a preview of subscription content, access via your institution
Access options
Access Nature and 54 other Nature Portfolio journals
Get Nature+, our best-value online-access subscription
24,99 € / 30 days
cancel any time
Subscribe to this journal
Receive 51 print issues and online access
185,98 € per year
only 3,65 € per issue
Buy this article
- Purchase on Springer Link
- Instant access to full article PDF
Prices may be subject to local taxes which are calculated during checkout
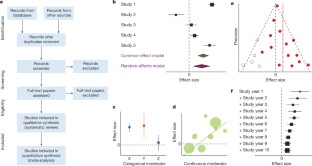
Similar content being viewed by others
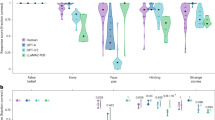
Testing theory of mind in large language models and humans
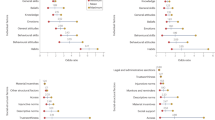
Determinants of behaviour and their efficacy as targets of behavioural change interventions
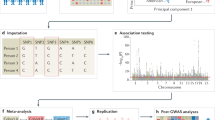
Genome-wide association studies
Jennions, M. D ., Lortie, C. J. & Koricheva, J. in The Handbook of Meta-analysis in Ecology and Evolution (eds Koricheva, J . et al.) Ch. 23 , 364–380 (Princeton Univ. Press, 2013)
Article Google Scholar
Roberts, P. D ., Stewart, G. B. & Pullin, A. S. Are review articles a reliable source of evidence to support conservation and environmental management? A comparison with medicine. Biol. Conserv. 132 , 409–423 (2006)
Bastian, H ., Glasziou, P . & Chalmers, I. Seventy-five trials and eleven systematic reviews a day: how will we ever keep up? PLoS Med. 7 , e1000326 (2010)
Article PubMed PubMed Central Google Scholar
Borman, G. D. & Grigg, J. A. in The Handbook of Research Synthesis and Meta-analysis 2nd edn (eds Cooper, H. M . et al.) 497–519 (Russell Sage Foundation, 2009)
Ioannidis, J. P. A. The mass production of redundant, misleading, and conflicted systematic reviews and meta-analyses. Milbank Q. 94 , 485–514 (2016)
Koricheva, J . & Gurevitch, J. Uses and misuses of meta-analysis in plant ecology. J. Ecol. 102 , 828–844 (2014)
Littell, J. H . & Shlonsky, A. Making sense of meta-analysis: a critique of “effectiveness of long-term psychodynamic psychotherapy”. Clin. Soc. Work J. 39 , 340–346 (2011)
Morrissey, M. B. Meta-analysis of magnitudes, differences and variation in evolutionary parameters. J. Evol. Biol. 29 , 1882–1904 (2016)
Article CAS PubMed Google Scholar
Whittaker, R. J. Meta-analyses and mega-mistakes: calling time on meta-analysis of the species richness-productivity relationship. Ecology 91 , 2522–2533 (2010)
Article PubMed Google Scholar
Begley, C. G . & Ellis, L. M. Drug development: Raise standards for preclinical cancer research. Nature 483 , 531–533 (2012); clarification 485 , 41 (2012)
Article CAS ADS PubMed Google Scholar
Hillebrand, H . & Cardinale, B. J. A critique for meta-analyses and the productivity-diversity relationship. Ecology 91 , 2545–2549 (2010)
Moher, D . et al. Preferred reporting items for systematic reviews and meta-analyses: the PRISMA statement. PLoS Med. 6 , e1000097 (2009). This paper provides a consensus regarding the reporting requirements for medical meta-analysis and has been highly influential in ensuring good reporting practice and standardizing language in evidence-based medicine, with further guidance for protocols, individual patient data meta-analyses and animal studies.
Moher, D . et al. Preferred reporting items for systematic review and meta-analysis protocols (PRISMA-P) 2015 statement. Syst. Rev. 4 , 1 (2015)
Nakagawa, S . & Santos, E. S. A. Methodological issues and advances in biological meta-analysis. Evol. Ecol. 26 , 1253–1274 (2012)
Nakagawa, S ., Noble, D. W. A ., Senior, A. M. & Lagisz, M. Meta-evaluation of meta-analysis: ten appraisal questions for biologists. BMC Biol. 15 , 18 (2017)
Hedges, L. & Olkin, I. Statistical Methods for Meta-analysis (Academic Press, 1985)
Viechtbauer, W. Conducting meta-analyses in R with the metafor package. J. Stat. Softw. 36 , 1–48 (2010)
Anzures-Cabrera, J . & Higgins, J. P. T. Graphical displays for meta-analysis: an overview with suggestions for practice. Res. Synth. Methods 1 , 66–80 (2010)
Egger, M ., Davey Smith, G ., Schneider, M. & Minder, C. Bias in meta-analysis detected by a simple, graphical test. Br. Med. J. 315 , 629–634 (1997)
Article CAS Google Scholar
Duval, S . & Tweedie, R. Trim and fill: a simple funnel-plot-based method of testing and adjusting for publication bias in meta-analysis. Biometrics 56 , 455–463 (2000)
Article CAS MATH PubMed Google Scholar
Leimu, R . & Koricheva, J. Cumulative meta-analysis: a new tool for detection of temporal trends and publication bias in ecology. Proc. R. Soc. Lond. B 271 , 1961–1966 (2004)
Higgins, J. P. T . & Green, S. (eds) Cochrane Handbook for Systematic Reviews of Interventions : Version 5.1.0 (Wiley, 2011). This large collaborative work provides definitive guidance for the production of systematic reviews in medicine and is of broad interest for methods development outside the medical field.
Lau, J ., Rothstein, H. R . & Stewart, G. B. in The Handbook of Meta-analysis in Ecology and Evolution (eds Koricheva, J . et al.) Ch. 25 , 407–419 (Princeton Univ. Press, 2013)
Lortie, C. J ., Stewart, G ., Rothstein, H. & Lau, J. How to critically read ecological meta-analyses. Res. Synth. Methods 6 , 124–133 (2015)
Murad, M. H . & Montori, V. M. Synthesizing evidence: shifting the focus from individual studies to the body of evidence. J. Am. Med. Assoc. 309 , 2217–2218 (2013)
Rasmussen, S. A ., Chu, S. Y ., Kim, S. Y ., Schmid, C. H . & Lau, J. Maternal obesity and risk of neural tube defects: a meta-analysis. Am. J. Obstet. Gynecol. 198 , 611–619 (2008)
Littell, J. H ., Campbell, M ., Green, S . & Toews, B. Multisystemic therapy for social, emotional, and behavioral problems in youth aged 10–17. Cochrane Database Syst. Rev. https://doi.org/10.1002/14651858.CD004797.pub4 (2005)
Schmidt, F. L. What do data really mean? Research findings, meta-analysis, and cumulative knowledge in psychology. Am. Psychol. 47 , 1173–1181 (1992)
Button, K. S . et al. Power failure: why small sample size undermines the reliability of neuroscience. Nat. Rev. Neurosci. 14 , 365–376 (2013); erratum 14 , 451 (2013)
Parker, T. H . et al. Transparency in ecology and evolution: real problems, real solutions. Trends Ecol. Evol. 31 , 711–719 (2016)
Stewart, G. Meta-analysis in applied ecology. Biol. Lett. 6 , 78–81 (2010)
Sutherland, W. J ., Pullin, A. S ., Dolman, P. M . & Knight, T. M. The need for evidence-based conservation. Trends Ecol. Evol. 19 , 305–308 (2004)
Lowry, E . et al. Biological invasions: a field synopsis, systematic review, and database of the literature. Ecol. Evol. 3 , 182–196 (2013)
Article PubMed Central Google Scholar
Parmesan, C . & Yohe, G. A globally coherent fingerprint of climate change impacts across natural systems. Nature 421 , 37–42 (2003)
Jennions, M. D ., Lortie, C. J . & Koricheva, J. in The Handbook of Meta-analysis in Ecology and Evolution (eds Koricheva, J . et al.) Ch. 24 , 381–403 (Princeton Univ. Press, 2013)
Balvanera, P . et al. Quantifying the evidence for biodiversity effects on ecosystem functioning and services. Ecol. Lett. 9 , 1146–1156 (2006)
Cardinale, B. J . et al. Effects of biodiversity on the functioning of trophic groups and ecosystems. Nature 443 , 989–992 (2006)
Rey Benayas, J. M ., Newton, A. C ., Diaz, A. & Bullock, J. M. Enhancement of biodiversity and ecosystem services by ecological restoration: a meta-analysis. Science 325 , 1121–1124 (2009)
Article ADS PubMed CAS Google Scholar
Leimu, R ., Mutikainen, P. I. A ., Koricheva, J. & Fischer, M. How general are positive relationships between plant population size, fitness and genetic variation? J. Ecol. 94 , 942–952 (2006)
Hillebrand, H. On the generality of the latitudinal diversity gradient. Am. Nat. 163 , 192–211 (2004)
Gurevitch, J. in The Handbook of Meta-analysis in Ecology and Evolution (eds Koricheva, J . et al.) Ch. 19 , 313–320 (Princeton Univ. Press, 2013)
Rustad, L . et al. A meta-analysis of the response of soil respiration, net nitrogen mineralization, and aboveground plant growth to experimental ecosystem warming. Oecologia 126 , 543–562 (2001)
Adams, D. C. Phylogenetic meta-analysis. Evolution 62 , 567–572 (2008)
Hadfield, J. D . & Nakagawa, S. General quantitative genetic methods for comparative biology: phylogenies, taxonomies and multi-trait models for continuous and categorical characters. J. Evol. Biol. 23 , 494–508 (2010)
Lajeunesse, M. J. Meta-analysis and the comparative phylogenetic method. Am. Nat. 174 , 369–381 (2009)
Rosenberg, M. S ., Adams, D. C . & Gurevitch, J. MetaWin: Statistical Software for Meta-Analysis with Resampling Tests Version 1 (Sinauer Associates, 1997)
Wallace, B. C . et al. OpenMEE: intuitive, open-source software for meta-analysis in ecology and evolutionary biology. Methods Ecol. Evol. 8 , 941–947 (2016)
Gurevitch, J ., Morrison, J. A . & Hedges, L. V. The interaction between competition and predation: a meta-analysis of field experiments. Am. Nat. 155 , 435–453 (2000)
Adams, D. C ., Gurevitch, J . & Rosenberg, M. S. Resampling tests for meta-analysis of ecological data. Ecology 78 , 1277–1283 (1997)
Gurevitch, J . & Hedges, L. V. Statistical issues in ecological meta-analyses. Ecology 80 , 1142–1149 (1999)
Schmid, C. H . & Mengersen, K. in The Handbook of Meta-analysis in Ecology and Evolution (eds Koricheva, J . et al.) Ch. 11 , 145–173 (Princeton Univ. Press, 2013)
Eysenck, H. J. Exercise in mega-silliness. Am. Psychol. 33 , 517 (1978)
Simberloff, D. Rejoinder to: Don’t calculate effect sizes; study ecological effects. Ecol. Lett. 9 , 921–922 (2006)
Cadotte, M. W ., Mehrkens, L. R . & Menge, D. N. L. Gauging the impact of meta-analysis on ecology. Evol. Ecol. 26 , 1153–1167 (2012)
Koricheva, J ., Jennions, M. D. & Lau, J. in The Handbook of Meta-analysis in Ecology and Evolution (eds Koricheva, J . et al.) Ch. 15 , 237–254 (Princeton Univ. Press, 2013)
Lau, J ., Ioannidis, J. P. A ., Terrin, N ., Schmid, C. H . & Olkin, I. The case of the misleading funnel plot. Br. Med. J. 333 , 597–600 (2006)
Vetter, D ., Rucker, G. & Storch, I. Meta-analysis: a need for well-defined usage in ecology and conservation biology. Ecosphere 4 , 1–24 (2013)
Mengersen, K ., Jennions, M. D. & Schmid, C. H. in The Handbook of Meta-analysis in Ecology and Evolution (eds Koricheva, J. et al.) Ch. 16 , 255–283 (Princeton Univ. Press, 2013)
Patsopoulos, N. A ., Analatos, A. A. & Ioannidis, J. P. A. Relative citation impact of various study designs in the health sciences. J. Am. Med. Assoc. 293 , 2362–2366 (2005)
Kueffer, C . et al. Fame, glory and neglect in meta-analyses. Trends Ecol. Evol. 26 , 493–494 (2011)
Cohnstaedt, L. W. & Poland, J. Review Articles: The black-market of scientific currency. Ann. Entomol. Soc. Am. 110 , 90 (2017)
Longo, D. L. & Drazen, J. M. Data sharing. N. Engl. J. Med. 374 , 276–277 (2016)
Gauch, H. G. Scientific Method in Practice (Cambridge Univ. Press, 2003)
Science Staff. Dealing with data: introduction. Challenges and opportunities. Science 331 , 692–693 (2011)
Nosek, B. A . et al. Promoting an open research culture. Science 348 , 1422–1425 (2015)
Article CAS ADS PubMed PubMed Central Google Scholar
Stewart, L. A . et al. Preferred reporting items for a systematic review and meta-analysis of individual participant data: the PRISMA-IPD statement. J. Am. Med. Assoc. 313 , 1657–1665 (2015)
Saldanha, I. J . et al. Evaluating Data Abstraction Assistant, a novel software application for data abstraction during systematic reviews: protocol for a randomized controlled trial. Syst. Rev. 5 , 196 (2016)
Tipton, E. & Pustejovsky, J. E. Small-sample adjustments for tests of moderators and model fit using robust variance estimation in meta-regression. J. Educ. Behav. Stat. 40 , 604–634 (2015)
Mengersen, K ., MacNeil, M. A . & Caley, M. J. The potential for meta-analysis to support decision analysis in ecology. Res. Synth. Methods 6 , 111–121 (2015)
Ashby, D. Bayesian statistics in medicine: a 25 year review. Stat. Med. 25 , 3589–3631 (2006)
Article MathSciNet PubMed Google Scholar
Senior, A. M . et al. Heterogeneity in ecological and evolutionary meta-analyses: its magnitude and implications. Ecology 97 , 3293–3299 (2016)
McAuley, L ., Pham, B ., Tugwell, P . & Moher, D. Does the inclusion of grey literature influence estimates of intervention effectiveness reported in meta-analyses? Lancet 356 , 1228–1231 (2000)
Koricheva, J ., Gurevitch, J . & Mengersen, K. (eds) The Handbook of Meta-Analysis in Ecology and Evolution (Princeton Univ. Press, 2013) This book provides the first comprehensive guide to undertaking meta-analyses in ecology and evolution and is also relevant to other fields where heterogeneity is expected, incorporating explicit consideration of the different approaches used in different domains.
Lumley, T. Network meta-analysis for indirect treatment comparisons. Stat. Med. 21 , 2313–2324 (2002)
Zarin, W . et al. Characteristics and knowledge synthesis approach for 456 network meta-analyses: a scoping review. BMC Med. 15 , 3 (2017)
Elliott, J. H . et al. Living systematic reviews: an emerging opportunity to narrow the evidence-practice gap. PLoS Med. 11 , e1001603 (2014)
Vandvik, P. O ., Brignardello-Petersen, R . & Guyatt, G. H. Living cumulative network meta-analysis to reduce waste in research: a paradigmatic shift for systematic reviews? BMC Med. 14 , 59 (2016)
Jarvinen, A. A meta-analytic study of the effects of female age on laying date and clutch size in the Great Tit Parus major and the Pied Flycatcher Ficedula hypoleuca . Ibis 133 , 62–67 (1991)
Arnqvist, G. & Wooster, D. Meta-analysis: synthesizing research findings in ecology and evolution. Trends Ecol. Evol. 10 , 236–240 (1995)
Hedges, L. V ., Gurevitch, J . & Curtis, P. S. The meta-analysis of response ratios in experimental ecology. Ecology 80 , 1150–1156 (1999)
Gurevitch, J ., Curtis, P. S. & Jones, M. H. Meta-analysis in ecology. Adv. Ecol. Res 32 , 199–247 (2001)
Lajeunesse, M. J. phyloMeta: a program for phylogenetic comparative analyses with meta-analysis. Bioinformatics 27 , 2603–2604 (2011)
CAS PubMed Google Scholar
Pearson, K. Report on certain enteric fever inoculation statistics. Br. Med. J. 2 , 1243–1246 (1904)
Fisher, R. A. Statistical Methods for Research Workers (Oliver and Boyd, 1925)
Yates, F. & Cochran, W. G. The analysis of groups of experiments. J. Agric. Sci. 28 , 556–580 (1938)
Cochran, W. G. The combination of estimates from different experiments. Biometrics 10 , 101–129 (1954)
Smith, M. L . & Glass, G. V. Meta-analysis of psychotherapy outcome studies. Am. Psychol. 32 , 752–760 (1977)
Glass, G. V. Meta-analysis at middle age: a personal history. Res. Synth. Methods 6 , 221–231 (2015)
Cooper, H. M ., Hedges, L. V . & Valentine, J. C. (eds) The Handbook of Research Synthesis and Meta-analysis 2nd edn (Russell Sage Foundation, 2009). This book is an important compilation that builds on the ground-breaking first edition to set the standard for best practice in meta-analysis, primarily in the social sciences but with applications to medicine and other fields.
Rosenthal, R. Meta-analytic Procedures for Social Research (Sage, 1991)
Hunter, J. E ., Schmidt, F. L. & Jackson, G. B. Meta-analysis: Cumulating Research Findings Across Studies (Sage, 1982)
Gurevitch, J ., Morrow, L. L ., Wallace, A . & Walsh, J. S. A meta-analysis of competition in field experiments. Am. Nat. 140 , 539–572 (1992). This influential early ecological meta-analysis reports multiple experimental outcomes on a longstanding and controversial topic that introduced a wide range of ecologists to research synthesis methods.
O’Rourke, K. An historical perspective on meta-analysis: dealing quantitatively with varying study results. J. R. Soc. Med. 100 , 579–582 (2007)
Shadish, W. R . & Lecy, J. D. The meta-analytic big bang. Res. Synth. Methods 6 , 246–264 (2015)
Glass, G. V. Primary, secondary, and meta-analysis of research. Educ. Res. 5 , 3–8 (1976)
DerSimonian, R . & Laird, N. Meta-analysis in clinical trials. Control. Clin. Trials 7 , 177–188 (1986)
Lipsey, M. W . & Wilson, D. B. The efficacy of psychological, educational, and behavioral treatment. Confirmation from meta-analysis. Am. Psychol. 48 , 1181–1209 (1993)
Chalmers, I. & Altman, D. G. Systematic Reviews (BMJ Publishing Group, 1995)
Moher, D . et al. Improving the quality of reports of meta-analyses of randomised controlled trials: the QUOROM statement. Quality of reporting of meta-analyses. Lancet 354 , 1896–1900 (1999)
Higgins, J. P. & Thompson, S. G. Quantifying heterogeneity in a meta-analysis. Stat. Med. 21 , 1539–1558 (2002)
Download references
Acknowledgements
We dedicate this Review to the memory of Ingram Olkin and William Shadish, founding members of the Society for Research Synthesis Methodology who made tremendous contributions to the development of meta-analysis and research synthesis and to the supervision of generations of students. We thank L. Lagisz for help in preparing the figures. We are grateful to the Center for Open Science and the Laura and John Arnold Foundation for hosting and funding a workshop, which was the origination of this article. S.N. is supported by Australian Research Council Future Fellowship (FT130100268). J.G. acknowledges funding from the US National Science Foundation (ABI 1262402).
Author information
Authors and affiliations.
Department of Ecology and Evolution, Stony Brook University, Stony Brook, 11794-5245, New York, USA
Jessica Gurevitch
School of Biological Sciences, Royal Holloway University of London, Egham, TW20 0EX, Surrey, UK
Julia Koricheva
Evolution and Ecology Research Centre and School of Biological, Earth and Environmental Sciences, University of New South Wales, Sydney, 2052, New South Wales, Australia
Shinichi Nakagawa
Diabetes and Metabolism Division, Garvan Institute of Medical Research, 384 Victoria Street, Darlinghurst, Sydney, 2010, New South Wales, Australia
School of Natural and Environmental Sciences, Newcastle University, Newcastle upon Tyne, NE1 7RU, UK
Gavin Stewart
You can also search for this author in PubMed Google Scholar
Contributions
All authors contributed equally in designing the study and writing the manuscript, and so are listed alphabetically.
Corresponding authors
Correspondence to Jessica Gurevitch , Julia Koricheva , Shinichi Nakagawa or Gavin Stewart .
Ethics declarations
Competing interests.
The authors declare no competing financial interests.
Additional information
Reviewer Information Nature thanks D. Altman, M. Lajeunesse, D. Moher and G. Romero for their contribution to the peer review of this work.
Publisher's note: Springer Nature remains neutral with regard to jurisdictional claims in published maps and institutional affiliations.
PowerPoint slides
Powerpoint slide for fig. 1, rights and permissions.
Reprints and permissions
About this article
Cite this article.
Gurevitch, J., Koricheva, J., Nakagawa, S. et al. Meta-analysis and the science of research synthesis. Nature 555 , 175–182 (2018). https://doi.org/10.1038/nature25753
Download citation
Received : 04 March 2017
Accepted : 12 January 2018
Published : 08 March 2018
Issue Date : 08 March 2018
DOI : https://doi.org/10.1038/nature25753
Share this article
Anyone you share the following link with will be able to read this content:
Sorry, a shareable link is not currently available for this article.
Provided by the Springer Nature SharedIt content-sharing initiative
This article is cited by
Investigate the relationship between the retraction reasons and the quality of methodology in non-cochrane retracted systematic reviews: a systematic review.
- Azita Shahraki-Mohammadi
- Leila Keikha
- Razieh Zahedi
Systematic Reviews (2024)
A meta-analysis on global change drivers and the risk of infectious disease
- Michael B. Mahon
- Alexandra Sack
- Jason R. Rohr
Nature (2024)
Systematic review of the uncertainty of coral reef futures under climate change
- Shannon G. Klein
- Cassandra Roch
- Carlos M. Duarte
Nature Communications (2024)
Meta-analysis reveals weak associations between reef fishes and corals
- Pooventhran Muruga
- Alexandre C. Siqueira
- David R. Bellwood
Nature Ecology & Evolution (2024)
Farming practices to enhance biodiversity across biomes: a systematic review
- Felipe Cozim-Melges
- Raimon Ripoll-Bosch
- Hannah H. E. van Zanten
npj Biodiversity (2024)
By submitting a comment you agree to abide by our Terms and Community Guidelines . If you find something abusive or that does not comply with our terms or guidelines please flag it as inappropriate.
Quick links
- Explore articles by subject
- Guide to authors
- Editorial policies
Sign up for the Nature Briefing newsletter — what matters in science, free to your inbox daily.


- Table of Contents
- Random Entry
- Chronological
- Editorial Information
- About the SEP
- Editorial Board
- How to Cite the SEP
- Special Characters
- Advanced Tools
- Support the SEP
- PDFs for SEP Friends
- Make a Donation
- SEPIA for Libraries
- Entry Contents
Bibliography
Academic tools.
- Friends PDF Preview
- Author and Citation Info
- Back to Top
Analysis has always been at the heart of philosophical method, but it has been understood and practised in many different ways. Perhaps, in its broadest sense, it might be defined as a process of isolating or working back to what is more fundamental by means of which something, initially taken as given, can be explained or reconstructed. The explanation or reconstruction is often then exhibited in a corresponding process of synthesis. This allows great variation in specific method, however. The aim may be to get back to basics, but there may be all sorts of ways of doing this, each of which might be called ‘analysis’. The dominance of ‘analytic’ philosophy in the English-speaking world, and increasingly now in the rest of the world, might suggest that a consensus has formed concerning the role and importance of analysis. This assumes, though, that there is agreement on what ‘analysis’ means, and this is far from clear. On the other hand, Wittgenstein's later critique of analysis in the early (logical atomist) period of analytic philosophy, and Quine's attack on the analytic-synthetic distinction, for example, have led some to claim that we are now in a ‘post-analytic’ age. Such criticisms, however, are only directed at particular conceptions of analysis. If we look at the history of philosophy, and even if we just look at the history of analytic philosophy, we find a rich and extensive repertoire of conceptions of analysis which philosophers have continually drawn upon and reconfigured in different ways. Analytic philosophy is alive and well precisely because of the range of conceptions of analysis that it involves. It may have fragmented into various interlocking subtraditions, but those subtraditions are held together by both their shared history and their methodological interconnections. It is the aim of this article to indicate something of the range of conceptions of analysis in the history of philosophy and their interconnections, and to provide a bibliographical resource for those wishing to explore analytic methodologies and the philosophical issues that they raise.
1.1 Characterizations of Analysis
1.2 guide to this entry.
- Supplementary Document: Definitions and Descriptions of Analysis
- 1. Introduction
- 2. Ancient Greek Geometry
- 4. Aristotle
- 1. Medieval Philosophy
- 2. Renaissance Philosophy
- 2. Descartes and Analytic Geometry
- 3. British Empiricism
5. Modern Conceptions of Analysis, outside Analytic Philosophy
- 5. Wittgenstein
- 6. The Cambridge School of Analysis
- 7. Carnap and Logical Positivism
- 8. Oxford Linguistic Philosophy
- 9. Contemporary Analytic Philosophy
7. Conclusion
Other internet resources, related entries, 1. general introduction.
This section provides a preliminary description of analysis—or the range of different conceptions of analysis—and a guide to this article as a whole.
If asked what ‘analysis’ means, most people today immediately think of breaking something down into its components; and this is how analysis tends to be officially characterized. In the Concise Oxford Dictionary , for example, ‘analysis’ is defined as the “resolution into simpler elements by analysing (opp. synthesis )”, the only other uses mentioned being the mathematical and the psychological [ Quotation ]. And in the Oxford Dictionary of Philosophy , ‘analysis’ is defined as “the process of breaking a concept down into more simple parts, so that its logical structure is displayed” [ Quotation ]. The restriction to concepts and the reference to displaying ‘logical structure’ are important qualifications, but the core conception remains that of breaking something down.
This conception may be called the decompositional conception of analysis (see Section 4 ). But it is not the only conception, and indeed is arguably neither the dominant conception in the pre-modern period nor the conception that is characteristic of at least one major strand in ‘analytic’ philosophy. In ancient Greek thought, ‘analysis’ referred primarily to the process of working back to first principles by means of which something could then be demonstrated. This conception may be called the regressive conception of analysis (see Section 2 ). In the work of Frege and Russell, on the other hand, before the process of decomposition could take place, the statements to be analyzed had first to be translated into their ‘correct’ logical form (see Section 6 ). This suggests that analysis also involves a transformative or interpretive dimension. This too, however, has its roots in earlier thought (see especially the supplementary sections on Ancient Greek Geometry and Medieval Philosophy ).
These three conceptions should not be seen as competing. In actual practices of analysis, which are invariably richer than the accounts that are offered of them, all three conceptions are typically reflected, though to differing degrees and in differing forms. To analyze something, we may first have to interpret it in some way, translating an initial statement, say, into the privileged language of logic, mathematics or science, before articulating the relevant elements and structures, and all in the service of identifying fundamental principles by means of which to explain it. The complexities that this schematic description suggests can only be appreciated by considering particular types of analysis.
Understanding conceptions of analysis is not simply a matter of attending to the use of the word ‘analysis’ and its cognates—or obvious equivalents in languages other than English, such as ‘ analusis ’ in Greek or ‘ Analyse ’ in German. Socratic definition is arguably a form of conceptual analysis, yet the term ‘ analusis ’ does not occur anywhere in Plato's dialogues (see Section 2 below). Nor, indeed, do we find it in Euclid's Elements , which is the classic text for understanding ancient Greek geometry: Euclid presupposed what came to be known as the method of analysis in presenting his proofs ‘synthetically’. In Latin, ‘ resolutio ’ was used to render the Greek word ‘ analusis ’, and although ‘resolution’ has a different range of meanings, it is often used synonymously with ‘analysis’ (see the supplementary section on Renaissance Philosophy ). In Aristotelian syllogistic theory, and especially from the time of Descartes, forms of analysis have also involved ‘reduction’; and in early analytic philosophy it was ‘reduction’ that was seen as the goal of philosophical analysis (see especially the supplementary section on The Cambridge School of Analysis ).
Further details of characterizations of analysis that have been offered in the history of philosophy, including all the classic passages and remarks (to which occurrences of ‘[ Quotation ]’ throughout this entry refer), can be found in the supplementary document on
Definitions and Descriptions of Analysis .
A list of key reference works, monographs and collections can be found in the
Annotated Bibliography, §1 .
This entry comprises three sets of documents:
- The present document
- Six supplementary documents (one of which is not yet available)
- An annotated bibliography on analysis, divided into six documents
The present document provides an overview, with introductions to the various conceptions of analysis in the history of philosophy. It also contains links to the supplementary documents, the documents in the bibliography, and other internet resources. The supplementary documents expand on certain topics under each of the six main sections. The annotated bibliography contains a list of key readings on each topic, and is also divided according to the sections of this entry.
2. Ancient Conceptions of Analysis and the Emergence of the Regressive Conception
The word ‘analysis’ derives from the ancient Greek term ‘ analusis ’. The prefix ‘ ana ’ means ‘up’, and ‘ lusis ’ means ‘loosing’, ‘release’ or ‘separation’, so that ‘ analusis ’ means ‘loosening up’ or ‘dissolution’. The term was readily extended to the solving or dissolving of a problem, and it was in this sense that it was employed in ancient Greek geometry and philosophy. The method of analysis that was developed in ancient Greek geometry had an influence on both Plato and Aristotle. Also important, however, was the influence of Socrates's concern with definition, in which the roots of modern conceptual analysis can be found. What we have in ancient Greek thought, then, is a complex web of methodologies, of which the most important are Socratic definition, which Plato elaborated into his method of division, his related method of hypothesis, which drew on geometrical analysis, and the method(s) that Aristotle developed in his Analytics . Far from a consensus having established itself over the last two millennia, the relationships between these methodologies are the subject of increasing debate today. At the heart of all of them, too, lie the philosophical problems raised by Meno's paradox, which anticipates what we now know as the paradox of analysis, concerning how an analysis can be both correct and informative (see the supplementary section on Moore ), and Plato's attempt to solve it through the theory of recollection, which has spawned a vast literature on its own.
‘Analysis’ was first used in a methodological sense in ancient Greek geometry, and the model that Euclidean geometry provided has been an inspiration ever since. Although Euclid's Elements dates from around 300 BC, and hence after both Plato and Aristotle, it is clear that it draws on the work of many previous geometers, most notably, Theaetetus and Eudoxus, who worked closely with Plato and Aristotle. Plato is even credited by Diogenes Laertius ( LEP , I, 299) with inventing the method of analysis, but whatever the truth of this may be, the influence of geometry starts to show in his middle dialogues, and he certainly encouraged work on geometry in his Academy.
The classic source for our understanding of ancient Greek geometrical analysis is a passage in Pappus's Mathematical Collection , which was composed around 300 AD, and hence drew on a further six centuries of work in geometry from the time of Euclid's Elements :
Now analysis is the way from what is sought—as if it were admitted—through its concomitants ( akolouthôn ) in order[,] to something admitted in synthesis. For in analysis we suppose that which is sought to be already done, and we inquire from what it results, and again what is the antecedent of the latter, until we on our backward way light upon something already known and being first in order. And we call such a method analysis, as being a solution backwards ( anapalin lysin ). In synthesis, on the other hand, we suppose that which was reached last in analysis to be already done, and arranging in their natural order as consequents ( epomena ) the former antecedents and linking them one with another, we in the end arrive at the construction of the thing sought. And this we call synthesis. [ Full Quotation ]
Analysis is clearly being understood here in the regressive sense—as involving the working back from ‘what is sought’, taken as assumed, to something more fundamental by means of which it can then be established, through its converse, synthesis. For example, to demonstrate Pythagoras's theorem—that the square on the hypotenuse of a right-angled triangle is equal to the sum of the squares on the other two sides—we may assume as ‘given’ a right-angled triangle with the three squares drawn on its sides. In investigating the properties of this complex figure we may draw further (auxiliary) lines between particular points and find that there are a number of congruent triangles, from which we can begin to work out the relationship between the relevant areas. Pythagoras's theorem thus depends on theorems about congruent triangles, and once these—and other—theorems have been identified (and themselves proved), Pythagoras's theorem can be proved. (The theorem is demonstrated in Proposition 47 of Book I of Euclid's Elements .)
The basic idea here provides the core of the conception of analysis that one can find reflected, in its different ways, in the work of Plato and Aristotle (see the supplementary sections on Plato and Aristotle ). Although detailed examination of actual practices of analysis reveals more than just regression to first causes, principles or theorems, but decomposition and transformation as well (see especially the supplementary section on Ancient Greek Geometry ), the regressive conception dominated views of analysis until well into the early modern period.
Ancient Greek geometry was not the only source of later conceptions of analysis, however. Plato may not have used the term ‘analysis’ himself, but concern with definition was central to his dialogues, and definitions have often been seen as what ‘conceptual analysis’ should yield. The definition of ‘knowledge’ as ‘justified true belief’ (or ‘true belief with an account’, in more Platonic terms) is perhaps the classic example. Plato's concern may have been with real rather than nominal definitions, with ‘essences’ rather than mental or linguistic contents (see the supplementary section on Plato ), but conceptual analysis, too, has frequently been given a ‘realist’ construal. Certainly, the roots of conceptual analysis can be traced back to Plato's search for definitions, as we shall see in Section 4 below.
Further discussion can be found in the supplementary document on
Ancient Conceptions of Analysis .
Further reading can be found in the
Annotated Bibliography, §2 .
3. Medieval and Renaissance Conceptions of Analysis
Conceptions of analysis in the medieval and renaissance periods were largely influenced by ancient Greek conceptions. But knowledge of these conceptions was often second-hand, filtered through a variety of commentaries and texts that were not always reliable. Medieval and renaissance methodologies tended to be uneasy mixtures of Platonic, Aristotelian, Stoic, Galenic and neo-Platonic elements, many of them claiming to have some root in the geometrical conception of analysis and synthesis. However, in the late medieval period, clearer and more original forms of analysis started to take shape. In the literature on so-called ‘syncategoremata’ and ‘exponibilia’, for example, we can trace the development of a conception of interpretive analysis. Sentences involving more than one quantifier such as ‘Some donkey every man sees’, for example, were recognized as ambiguous, requiring ‘exposition’ to clarify.
In John Buridan's masterpiece of the mid-fourteenth century, the Summulae de Dialectica , we can find all three of the conceptions outlined in Section 1.1 above. He distinguishes explicitly between divisions, definitions and demonstrations, corresponding to decompositional, interpretive and regressive analysis, respectively. Here, in particular, we have anticipations of modern analytic philosophy as much as reworkings of ancient philosophy. Unfortunately, however, these clearer forms of analysis became overshadowed during the Renaissance, despite—or perhaps because of—the growing interest in the original Greek sources. As far as understanding analytic methodologies was concerned, the humanist repudiation of scholastic logic muddied the waters.
Medieval and Renaissance Conceptions of Analysis .
Annotated Bibliography, §3 .
4. Early Modern Conceptions of Analysis and the Development of the Decompositional Conception
The scientific revolution in the seventeenth century brought with it new forms of analysis. The newest of these emerged through the development of more sophisticated mathematical techniques, but even these still had their roots in earlier conceptions of analysis. By the end of the early modern period, decompositional analysis had become dominant (as outlined in what follows), but this, too, took different forms, and the relationships between the various conceptions of analysis were often far from clear.
In common with the Renaissance, the early modern period was marked by a great concern with methodology. This might seem unsurprising in such a revolutionary period, when new techniques for understanding the world were being developed and that understanding itself was being transformed. But what characterizes many of the treatises and remarks on methodology that appeared in the seventeenth century is their appeal, frequently self-conscious, to ancient methods (despite, or perhaps—for diplomatic reasons—because of, the critique of the content of traditional thought), although new wine was generally poured into the old bottles. The model of geometrical analysis was a particular inspiration here, albeit filtered through the Aristotelian tradition, which had assimilated the regressive process of going from theorems to axioms with that of moving from effects to causes (see the supplementary section on Aristotle ). Analysis came to be seen as a method of discovery, working back from what is ordinarily known to the underlying reasons (demonstrating ‘the fact’), and synthesis as a method of proof, working forwards again from what is discovered to what needed explanation (demonstrating ‘the reason why’). Analysis and synthesis were thus taken as complementary, although there remained disagreement over their respective merits.
There is a manuscript by Galileo, dating from around 1589, an appropriated commentary on Aristotle's Posterior Analytics , which shows his concern with methodology, and regressive analysis, in particular (see Wallace 1992a and 1992b). Hobbes wrote a chapter on method in the first part of De Corpore , published in 1655, which offers his own interpretation of the method of analysis and synthesis, where decompositional forms of analysis are articulated alongside regressive forms [ Quotations ]. But perhaps the most influential account of methodology, from the middle of the seventeenth century until well into the nineteenth century, was the fourth part of the Port-Royal Logic , the first edition of which appeared in 1662 and the final revised edition in 1683. Chapter 2 (which was the first chapter in the first edition) opens as follows:
The art of arranging a series of thoughts properly, either for discovering the truth when we do not know it, or for proving to others what we already know, can generally be called method. Hence there are two kinds of method, one for discovering the truth, which is known as analysis , or the method of resolution , and which can also be called the method of discovery . The other is for making the truth understood by others once it is found. This is known as synthesis , or the method of composition , and can also be called the method of instruction . [ Fuller Quotations ]
That a number of different methods might be assimilated here is not noted, although the text does go on to distinguish four main types of ‘issues concerning things’: seeking causes by their effects, seeking effects by their causes, finding the whole from the parts, and looking for another part from the whole and a given part ( ibid ., 234). While the first two involve regressive analysis and synthesis, the third and fourth involve decompositional analysis and synthesis.
As the authors of the Logic make clear, this particular part of their text derives from Descartes's Rules for the Direction of the Mind , written around 1627, but only published posthumously in 1684. The specification of the four types was most likely offered in elaborating Descartes's Rule Thirteen, which states: “If we perfectly understand a problem we must abstract it from every superfluous conception, reduce it to its simplest terms and, by means of an enumeration, divide it up into the smallest possible parts.” ( PW , I, 51. Cf. the editorial comments in PW , I, 54, 77.) The decompositional conception of analysis is explicit here, and if we follow this up into the later Discourse on Method , published in 1637, the focus has clearly shifted from the regressive to the decompositional conception of analysis. All the rules offered in the earlier work have now been reduced to just four. This is how Descartes reports the rules he says he adopted in his scientific and philosophical work:
The first was never to accept anything as true if I did not have evident knowledge of its truth: that is, carefully to avoid precipitate conclusions and preconceptions, and to include nothing more in my judgements than what presented itself to my mind so clearly and so distinctly that I had no occasion to doubt it. The second, to divide each of the difficulties I examined into as many parts as possible and as may be required in order to resolve them better. The third, to direct my thoughts in an orderly manner, by beginning with the simplest and most easily known objects in the order to ascend little by little, step by step, to knowledge of the most complex, and by supposing some order even among objects that have no natural order of precedence. And the last, throughout to make enumerations so complete, and reviews so comprehensive, that I could be sure of leaving nothing out. ( PW , I, 120.)
The first two are rules of analysis and the second two rules of synthesis. But although the analysis/synthesis structure remains, what is involved here is decomposition/composition rather than regression/progression. Nevertheless, Descartes insisted that it was geometry that influenced him here: “Those long chains composed of very simple and easy reasonings, which geometers customarily use to arrive at their most difficult demonstrations, had given me occasion to suppose that all the things which can fall under human knowledge are interconnected in the same way.” ( Ibid . [ Further Quotations ])
Descartes's geometry did indeed involve the breaking down of complex problems into simpler ones. More significant, however, was his use of algebra in developing ‘analytic’ geometry as it came to be called, which allowed geometrical problems to be transformed into arithmetical ones and more easily solved. In representing the ‘unknown’ to be found by ‘ x ’, we can see the central role played in analysis by the idea of taking something as ‘given’ and working back from that, which made it seem appropriate to regard algebra as an ‘art of analysis’, alluding to the regressive conception of the ancients. Illustrated in analytic geometry in its developed form, then, we can see all three of the conceptions of analysis outlined in Section 1.1 above, despite Descartes's own emphasis on the decompositional conception. For further discussion of this, see the supplementary section on Descartes and Analytic Geometry .
Descartes's emphasis on decompositional analysis was not without precedents, however. Not only was it already involved in ancient Greek geometry, but it was also implicit in Plato's method of collection and division. We might explain the shift from regressive to decompositional (conceptual) analysis, as well as the connection between the two, in the following way. Consider a simple example, as represented in the diagram below, ‘collecting’ all animals and ‘dividing’ them into rational and non-rational , in order to define human beings as rational animals.
On this model, in seeking to define anything, we work back up the appropriate classificatory hierarchy to find the higher (i.e., more basic or more general) ‘Forms’, by means of which we can lay down the definition. Although Plato did not himself use the term ‘analysis’—the word for ‘division’ was ‘ dihairesis ’—the finding of the appropriate ‘Forms’ is essentially analysis. As an elaboration of the Socratic search for definitions, we clearly have in this the origins of conceptual analysis. There is little disagreement that ‘Human beings are rational animals’ is the kind of definition we are seeking, defining one concept, the concept human being , in terms of other concepts, the concepts rational and animal . But the construals that have been offered of this have been more problematic. Understanding a classificatory hierarchy extensionally , that is, in terms of the classes of things denoted, the classes higher up are clearly the larger, ‘containing’ the classes lower down as subclasses (e.g., the class of animals includes the class of human beings as one of its subclasses). Intensionally , however, the relationship of ‘containment’ has been seen as holding in the opposite direction. If someone understands the concept human being , at least in the strong sense of knowing its definition, then they must understand the concepts animal and rational ; and it has often then seemed natural to talk of the concept human being as ‘containing’ the concepts rational and animal . Working back up the hierarchy in ‘analysis’ (in the regressive sense) could then come to be identified with ‘unpacking’ or ‘decomposing’ a concept into its ‘constituent’ concepts (‘analysis’ in the decompositional sense). Of course, talking of ‘decomposing’ a concept into its ‘constituents’ is, strictly speaking, only a metaphor (as Quine was famously to remark in §1 of ‘Two Dogmas of Empiricism’), but in the early modern period, this began to be taken more literally.
For further discussion, see the supplementary document on
Early Modern Conceptions of Analysis ,
which contains sections on Descartes and Analytic Geometry, British Empiricism, Leibniz, and Kant.
For further reading, see the
Annotated Bibliography, §4 .
As suggested in the supplementary document on Kant , the decompositional conception of analysis found its classic statement in the work of Kant at the end of the eighteenth century. But Kant was only expressing a conception widespread at the time. The conception can be found in a very blatant form, for example, in the writings of Moses Mendelssohn, for whom, unlike Kant, it was applicable even in the case of geometry [ Quotation ]. Typified in Kant's and Mendelssohn's view of concepts, it was also reflected in scientific practice. Indeed, its popularity was fostered by the chemical revolution inaugurated by Lavoisier in the late eighteenth century, the comparison between philosophical analysis and chemical analysis being frequently drawn. As Lichtenberg put it, “Whichever way you look at it, philosophy is always analytical chemistry” [ Quotation ].
This decompositional conception of analysis set the methodological agenda for philosophical approaches and debates in the (late) modern period (nineteenth and twentieth centuries). Responses and developments, very broadly, can be divided into two. On the one hand, an essentially decompositional conception of analysis was accepted, but a critical attitude was adopted towards it. If analysis simply involved breaking something down, then it appeared destructive and life-diminishing, and the critique of analysis that this view engendered was a common theme in idealism and romanticism in all its main varieties—from German, British and French to North American. One finds it reflected, for example, in remarks about the negating and soul-destroying power of analytical thinking by Schiller [ Quotation ], Hegel [ Quotation ] and de Chardin [ Quotation ], in Bradley's doctrine that analysis is falsification [ Quotation ], and in the emphasis placed by Bergson on ‘intuition’ [ Quotation ].
On the other hand, analysis was seen more positively, but the Kantian conception underwent a certain degree of modification and development. In the nineteenth century, this was exemplified, in particular, by Bolzano and the neo-Kantians. Bolzano's most important innovation was the method of variation, which involves considering what happens to the truth-value of a sentence when a constituent term is substituted by another. This formed the basis for his reconstruction of the analytic/synthetic distinction, Kant's account of which he found defective. The neo-Kantians emphasized the role of structure in conceptualized experience and had a greater appreciation of forms of analysis in mathematics and science. In many ways, their work attempts to do justice to philosophical and scientific practice while recognizing the central idealist claim that analysis is a kind of abstraction that inevitably involves falsification or distortion. On the neo-Kantian view, the complexity of experience is a complexity of form and content rather than of separable constituents, requiring analysis into ‘moments’ or ‘aspects’ rather than ‘elements’ or ‘parts’. In the 1910s, the idea was articulated with great subtlety by Ernst Cassirer [ Quotation ], and became familiar in Gestalt psychology.
In the twentieth century, both analytic philosophy and phenomenology can be seen as developing far more sophisticated conceptions of analysis, which draw on but go beyond mere decompositional analysis. The following Section offers an account of analysis in analytic philosophy, illustrating the range and richness of the conceptions and practices that arose. But it is important to see these in the wider context of twentieth-century methodological practices and debates, for it is not just in ‘analytic’ philosophy—despite its name—that analytic methods are accorded a central role. Phenomenology, in particular, contains its own distinctive set of analytic methods, with similarities and differences to those of analytic philosophy. Phenomenological analysis has frequently been compared to conceptual clarification in the ordinary language tradition, for example, and the method of ‘phenomenological reduction’ that Husserl invented in 1905 offers a striking parallel to the reductive project opened up by Russell's theory of descriptions, which also made its appearance in 1905.
Just like Frege and Russell, Husserl's initial concern was with the foundations of mathematics, and in this shared concern we can see the continued influence of the regressive conception of analysis. According to Husserl, the aim of ‘eidetic reduction’, as he called it, was to isolate the ‘essences’ that underlie our various forms of thinking, and to apprehend them by ‘essential intuition’ (‘ Wesenserschauung ’). The terminology may be different, but this resembles Russell's early project to identify the ‘indefinables’ of philosophical logic, as he described it, and to apprehend them by ‘acquaintance’ (cf. POM , xx). Furthermore, in Husserl's later discussion of ‘explication’ (cf. EJ , §§ 22-4 [ Quotations ]), we find appreciation of the ‘transformative’ dimension of analysis, which can be fruitfully compared with Carnap's account of explication (see the supplementary section on Carnap and Logical Positivism ). Carnap himself describes Husserl's idea here as one of “the synthesis of identification between a confused, nonarticulated sense and a subsequently intended distinct, articulated sense” (1950, 3 [ Quotation ]).
Phenomenology is not the only source of analytic methodologies outside those of the analytic tradition. Mention might be made here, too, of R. G. Collingwood, working within the tradition of British idealism, which was still a powerful force prior to the Second World War. In his Essay on Philosophical Method (1933), for example, he criticizes Moorean philosophy, and develops his own response to what is essentially the paradox of analysis (concerning how an analysis can be both correct and informative), which he recognizes as having its root in Meno's paradox. In his Essay on Metaphysics (1940), he puts forward his own conception of metaphysical analysis, in direct response to what he perceived as the mistaken repudiation of metaphysics by the logical positivists. Metaphysical analysis is characterized here as the detection of ‘absolute presuppositions’, which are taken as underlying and shaping the various conceptual practices that can be identified in the history of philosophy and science. Even among those explicitly critical of central strands in analytic philosophy, then, analysis in one form or another can still be seen as alive and well.
Annotated Bibliography, §5 .
6. Conceptions of Analysis in Analytic Philosophy and the Introduction of the Logical (Transformative) Conception
If anything characterizes ‘analytic’ philosophy, then it is presumably the emphasis placed on analysis. But as the foregoing sections have shown, there is a wide range of conceptions of analysis, so such a characterization says nothing that would distinguish analytic philosophy from much of what has either preceded or developed alongside it. Given that the decompositional conception is usually offered as the main conception today, it might be thought that it is this that characterizes analytic philosophy. But this conception was prevalent in the early modern period, shared by both the British Empiricists and Leibniz, for example. Given that Kant denied the importance of decompositional analysis, however, it might be suggested that what characterizes analytic philosophy is the value it places on such analysis. This might be true of Moore's early work, and of one strand within analytic philosophy; but it is not generally true. What characterizes analytic philosophy as it was founded by Frege and Russell is the role played by logical analysis , which depended on the development of modern logic. Although other and subsequent forms of analysis, such as linguistic analysis, were less wedded to systems of formal logic, the central insight motivating logical analysis remained.
Pappus's account of method in ancient Greek geometry suggests that the regressive conception of analysis was dominant at the time—however much other conceptions may also have been implicitly involved (see the supplementary section on Ancient Greek Geometry ). In the early modern period, the decompositional conception became widespread (see Section 4 ). What characterizes analytic philosophy—or at least that central strand that originates in the work of Frege and Russell—is the recognition of what was called earlier the transformative or interpretive dimension of analysis (see Section 1.1 ). Any analysis presupposes a particular framework of interpretation, and work is done in interpreting what we are seeking to analyze as part of the process of regression and decomposition. This may involve transforming it in some way, in order for the resources of a given theory or conceptual framework to be brought to bear. Euclidean geometry provides a good illustration of this. But it is even more obvious in the case of analytic geometry, where the geometrical problem is first ‘translated’ into the language of algebra and arithmetic in order to solve it more easily (see the supplementary section on Descartes and Analytic Geometry ). What Descartes and Fermat did for analytic geometry, Frege and Russell did for analytic philosophy. Analytic philosophy is ‘analytic’ much more in the sense that analytic geometry is ‘analytic’ than in the crude decompositional sense that Kant understood it.
The interpretive dimension of modern philosophical analysis can also be seen as anticipated in medieval scholasticism (see the supplementary section on Medieval Philosophy ), and it is remarkable just how much of modern concerns with propositions, meaning, reference, and so on, can be found in the medieval literature. Interpretive analysis is also illustrated in the nineteenth century by Bentham's conception of paraphrasis , which he characterized as “that sort of exposition which may be afforded by transmuting into a proposition, having for its subject some real entity, a proposition which has not for its subject any other than a fictitious entity” [ Full Quotation ]. He applied the idea in ‘analyzing away’ talk of ‘obligations’, and the anticipation that we can see here of Russell's theory of descriptions has been noted by, among others, Wisdom (1931) and Quine in ‘Five Milestones of Empiricism’ [ Quotation ].
What was crucial in the emergence of twentieth-century analytic philosophy, however, was the development of quantificational theory, which provided a far more powerful interpretive system than anything that had hitherto been available. In the case of Frege and Russell, the system into which statements were ‘translated’ was predicate logic, and the divergence that was thereby opened up between grammatical and logical form meant that the process of translation itself became an issue of philosophical concern. This induced greater self-consciousness about our use of language and its potential to mislead us, and inevitably raised semantic, epistemological and metaphysical questions about the relationships between language, logic, thought and reality which have been at the core of analytic philosophy ever since.
Both Frege and Russell (after the latter's initial flirtation with idealism) were concerned to show, against Kant, that arithmetic is a system of analytic and not synthetic truths. In the Grundlagen , Frege had offered a revised conception of analyticity, which arguably endorses and generalizes Kant's logical as opposed to phenomenological criterion, i.e., (AN L ) rather than (AN O ) (see the supplementary section on Kant ):
(AN) A truth is analytic if its proof depends only on general logical laws and definitions.
The question of whether arithmetical truths are analytic then comes down to the question of whether they can be derived purely logically. (Here we already have ‘transformation’, at the theoretical level—involving a reinterpretation of the concept of analyticity.) To demonstrate this, Frege realized that he needed to develop logical theory in order to formalize mathematical statements, which typically involve multiple generality (e.g., ‘Every natural number has a successor’, i.e. ‘For every natural number x there is another natural number y that is the successor of x ’). This development, by extending the use of function-argument analysis in mathematics to logic and providing a notation for quantification, was essentially the achievement of his first book, the Begriffsschrift (1879), where he not only created the first system of predicate logic but also, using it, succeeded in giving a logical analysis of mathematical induction (see Frege FR , 47-78).
In his second book, Die Grundlagen der Arithmetik (1884), Frege went on to provide a logical analysis of number statements. His central idea was that a number statement contains an assertion about a concept. A statement such as ‘Jupiter has four moons’ is to be understood not as predicating of Jupiter the property of having four moons, but as predicating of the concept moon of Jupiter the second-level property has four instances , which can be logically defined. The significance of this construal can be brought out by considering negative existential statements (which are equivalent to number statements involving the number 0). Take the following negative existential statement:
(0a) Unicorns do not exist.
If we attempt to analyze this decompositionally , taking its grammatical form to mirror its logical form, then we find ourselves asking what these unicorns are that have the property of non-existence. We may then be forced to posit the subsistence —as opposed to existence —of unicorns, just as Meinong and the early Russell did, in order for there to be something that is the subject of our statement. On the Fregean account, however, to deny that something exists is to say that the relevant concept has no instances: there is no need to posit any mysterious object . The Fregean analysis of (0a) consists in rephrasing it into (0b), which can then be readily formalized in the new logic as (0c):
(0b) The concept unicorn is not instantiated. (0c) ~(∃ x ) Fx .
Similarly, to say that God exists is to say that the concept God is (uniquely) instantiated, i.e., to deny that the concept has 0 instances (or 2 or more instances). On this view, existence is no longer seen as a (first-level) predicate, but instead, existential statements are analyzed in terms of the (second-level) predicate is instantiated , represented by means of the existential quantifier. As Frege notes, this offers a neat diagnosis of what is wrong with the ontological argument, at least in its traditional form ( GL , §53). All the problems that arise if we try to apply decompositional analysis (at least straight off) simply drop away, although an account is still needed, of course, of concepts and quantifiers.
The possibilities that this strategy of ‘translating’ into a logical language opens up are enormous: we are no longer forced to treat the surface grammatical form of a statement as a guide to its ‘real’ form, and are provided with a means of representing that form. This is the value of logical analysis: it allows us to ‘analyze away’ problematic linguistic expressions and explain what it is ‘really’ going on. This strategy was employed, most famously, in Russell's theory of descriptions, which was a major motivation behind the ideas of Wittgenstein's Tractatus (see the supplementary sections on Russell and Wittgenstein ). Although subsequent philosophers were to question the assumption that there could ever be a definitive logical analysis of a given statement, the idea that ordinary language may be systematically misleading has remained.
To illustrate this, consider the following examples from Ryle's classic 1932 paper, ‘Systematically Misleading Expressions’:
(Ua) Unpunctuality is reprehensible. (Ta) Jones hates the thought of going to hospital.
In each case, we might be tempted to make unnecessary reifications, taking ‘unpunctuality’ and ‘the thought of going to hospital’ as referring to objects. It is because of this that Ryle describes such expressions as ‘systematically misleading’. (Ua) and (Ta) must therefore be rephrased:
(Ub) Whoever is unpunctual deserves that other people should reprove him for being unpunctual. (Tb) Jones feels distressed when he thinks of what he will undergo if he goes to hospital.
In these formulations, there is no overt talk at all of ‘unpunctuality’ or ‘thoughts’, and hence nothing to tempt us to posit the existence of any corresponding entities. The problems that otherwise arise have thus been ‘analyzed away’.
At the time that Ryle wrote ‘Systematically Misleading Expressions’, he, too, assumed that every statement had an underlying logical form that was to be exhibited in its ‘correct’ formulation [ Quotations ]. But when he gave up this assumption (for reasons indicated in the supplementary section on The Cambridge School of Analysis ), he did not give up the motivating idea of logical analysis—to show what is wrong with misleading expressions. In The Concept of Mind (1949), for example, he sought to explain what he called the ‘category-mistake’ involved in talk of the mind as a kind of ‘Ghost in the Machine’. His aim, he wrote, was to “rectify the logical geography of the knowledge which we already possess” (1949, 9), an idea that was to lead to the articulation of connective rather than reductive conceptions of analysis, the emphasis being placed on elucidating the relationships between concepts without assuming that there is a privileged set of intrinsically basic concepts (see the supplementary section on Oxford Linguistic Philosophy ).
What these various forms of logical analysis suggest, then, is that what characterizes analysis in analytic philosophy is something far richer than the mere ‘decomposition’ of a concept into its ‘constituents’. But this is not to say that the decompositional conception of analysis plays no role at all. It can be found in the early work of Moore, for example (see the supplementary section on Moore ). It might also be seen as reflected in the approach to the analysis of concepts that seeks to specify the necessary and sufficient conditions for their correct employment. Conceptual analysis in this sense goes back to the Socrates of Plato's early dialogues (see the supplementary section on Plato ). But it arguably reached its heyday in the 1950s and 1960s. As mentioned in Section 2 above, the definition of ‘knowledge’ as ‘justified true belief’ is perhaps the most famous example; and this definition was criticised in Gettier's classic paper of 1963. (For details of this, see the entry in this Encyclopedia on The Analysis of Knowledge .) The specification of necessary and sufficient conditions may no longer be seen as the primary aim of conceptual analysis, especially in the case of philosophical concepts such as ‘knowledge’, which are fiercely contested; but consideration of such conditions remains a useful tool in the analytic philosopher's toolbag.
For a more detailed account of the these and related conceptions of analysis, see the supplementary document on
Conceptions of Analysis in Analytic Philosophy .
Annotated Bibliography, §6 .
The history of philosophy reveals a rich source of conceptions of analysis. Their origin may lie in ancient Greek geometry, and to this extent the history of analytic methodologies might be seen as a series of footnotes to Euclid. But analysis developed in different though related ways in the two traditions stemming from Plato and Aristotle, the former based on the search for definitions and the latter on the idea of regression to first causes. The two poles represented in these traditions defined methodological space until well into the early modern period, and in some sense is still reflected today. The creation of analytic geometry in the seventeenth century introduced a more reductive form of analysis, and an analogous and even more powerful form was introduced around the turn of the twentieth century in the logical work of Frege and Russell. Although conceptual analysis, construed decompositionally from the time of Leibniz and Kant, and mediated by the work of Moore, is often viewed as characteristic of analytic philosophy, logical analysis, taken as involving translation into a logical system, is what inaugurated the analytic tradition. Analysis has also frequently been seen as reductive, but connective forms of analysis are no less important. Connective analysis, historically inflected, would seem to be particularly appropriate, for example, in understanding analysis itself.
What follows here is a selection of thirty classic and recent works published over the last half-century that together cover the range of different conceptions of analysis in the history of philosophy. A fuller bibliography, which includes all references cited, is provided as a set of supplementary documents, divided to correspond to the sections of this entry:
Annotated Bibliography on Analysis
- Baker, Gordon, 2004, Wittgenstein's Method , Oxford: Blackwell, especially essays 1, 3, 4, 10, 12
- Baldwin, Thomas, 1990, G.E. Moore , London: Routledge, ch. 7
- Beaney, Michael, 2004, ‘Carnap's Conception of Explication: From Frege to Husserl?’, in S. Awodey and C. Klein, (eds.), Carnap Brought Home: The View from Jena , Chicago: Open Court, pp. 117-50
- –––, 2005, ‘Collingwood's Conception of Presuppositional Analysis’, Collingwood and British Idealism Studies 11, no. 2, 41-114
- –––, (ed.), 2007, The Analytic Turn: Analysis in Early Analytic Philosophy and Phenomenology , London: Routledge [includes papers on Frege, Russell, Wittgenstein, C.I. Lewis, Bolzano, Husserl]
- Byrne, Patrick H., 1997, Analysis and Science in Aristotle , Albany: State University of New York Press
- Cohen, L. Jonathan, 1986, The Dialogue of Reason: An Analysis of Analytical Philosophy , Oxford: Oxford University Press, chs. 1-2
- Dummett, Michael, 1991, Frege: Philosophy of Mathematics , London: Duckworth, chs. 3-4, 9-16
- Engfer, Hans-Jürgen, 1982, Philosophie als Analysis , Stuttgart-Bad Cannstatt: Frommann-Holzboog [Descartes, Leibniz, Wolff, Kant]
- Garrett, Aaron V., 2003, Meaning in Spinoza's Method , Cambridge: Cambridge University Press, ch. 4
- Gaukroger, Stephen, 1989, Cartesian Logic , Oxford: Oxford University Press, ch. 3
- Gentzler, Jyl, (ed.), 1998, Method in Ancient Philosophy , Oxford: Oxford University Press [includes papers on Socrates, Plato, Aristotle, mathematics and medicine]
- Gilbert, Neal W., 1960, Renaissance Concepts of Method , New York: Columbia University Press
- Hacker, P.M.S., 1996, Wittgenstein's Place in Twentieth-Century Analytic Philosophy , Oxford: Blackwell
- Hintikka, Jaakko and Remes, Unto, 1974, The Method of Analysis , Dordrecht: D. Reidel [ancient Greek geometrical analysis]
- Hylton, Peter, 2005, Propositions, Functions, Analysis: Selected Essays on Russell's Philosophy , Oxford: Oxford University Press
- –––, 2007, Quine , London: Routledge, ch. 9
- Jackson, Frank, 1998, From Metaphysics to Ethics: A Defence of Conceptual Analysis , Oxford: Oxford University Press, chs. 2-3
- Kretzmann, Norman, 1982, ‘Syncategoremata, exponibilia, sophistimata’, in N. Kretzmann et al. , (eds.), The Cambridge History of Later Medieval Philosophy , Cambridge: Cambridge University Press, 211-45
- Menn, Stephen, 2002, ‘Plato and the Method of Analysis’, Phronesis 47, 193-223
- Otte, Michael and Panza, Marco, (eds.), 1997, Analysis and Synthesis in Mathematics , Dordrecht: Kluwer
- Rorty, Richard, (ed.), 1967, The Linguistic Turn , Chicago: University of Chicago Press [includes papers on analytic methodology]
- Rosen, Stanley, 1980, The Limits of Analysis , New York: Basic Books, repr. Indiana: St. Augustine's Press, 2000 [critique of analytic philosophy from a ‘continental’ perspective]
- Sayre, Kenneth M., 1969, Plato's Analytic Method , Chicago: University of Chicago Press
- –––, 2006, Metaphysics and Method in Plato's Statesman , Cambridge: Cambridge University Press, Part I
- Soames, Scott, 2003, Philosophical Analysis in the Twentieth Century , Volume 1: The Dawn of Analysis , Volume 2: The Age of Meaning , New Jersey: Princeton University Press [includes chapters on Moore, Russell, Wittgenstein, logical positivism, Quine, ordinary language philosophy, Davidson, Kripke]
- Strawson, P.F., 1992, Analysis and Metaphysics: An Introduction to Philosophy , Oxford: Oxford University Press, chs. 1-2
- Sweeney, Eileen C., 1994, ‘Three Notions of Resolutio and the Structure of Reasoning in Aquinas’, The Thomist 58, 197-243
- Timmermans, Benoît, 1995, La résolution des problèmes de Descartes à Kant , Paris: Presses Universitaires de France
- Urmson, J.O., 1956, Philosophical Analysis: Its Development between the Two World Wars , Oxford: Oxford University Press
How to cite this entry . Preview the PDF version of this entry at the Friends of the SEP Society . Look up topics and thinkers related to this entry at the Internet Philosophy Ontology Project (InPhO). Enhanced bibliography for this entry at PhilPapers , with links to its database.
- Analysis , a journal in philosophy.
- Bertrand Russell Archives
- Leibniz-Archiv
- Wittgenstein Archives at the University of Bergen
abstract objects | analytic/synthetic distinction | Aristotle | Bolzano, Bernard | Buridan, John [Jean] | Descartes, René | descriptions | Frege, Gottlob | Kant, Immanuel | knowledge: analysis of | Leibniz, Gottfried Wilhelm | logical constructions | logical form | Moore, George Edward | necessary and sufficient conditions | Ockham [Occam], William | Plato | Russell, Bertrand | Wittgenstein, Ludwig
Acknowledgments
In first composing this entry (in 2002-3) and then revising the main entry and bibliography (in 2007), I have drawn on a number of my published writings (especially Beaney 1996, 2000, 2002, 2007b, 2007c; see Annotated Bibliography §6.1 , §6.2 ). I am grateful to the respective publishers for permission to use this material. Research on conceptions of analysis in the history of philosophy was initially undertaken while a Research Fellow at the Institut für Philosophie of the University of Erlangen-Nürnberg during 1999-2000, and further work was carried out while a Research Fellow at the Institut für Philosophie of the University of Jena during 2006-7, in both cases funded by the Alexander von Humboldt-Stiftung. In the former case, the account was written up while at the Open University (UK), and in the latter case, I had additional research leave from the University of York. I acknowledge the generous support given to me by all five institutions. I am also grateful to the editors of this Encyclopedia, and to Gideon Rosen and Edward N. Zalta, in particular, for comments and suggestions on the content and organisation of this entry in both its initial and revised form. I would like to thank John Ongley, too, for reviewing the first version of this entry, which has helped me to improve it (see Annotated Bibliography §1.3 ). In updating the bibliography (in 2007), I am indebted to various people who have notified me of relevant works, and especially, Gyula Klima (regarding §2.1), Anna-Sophie Heinemann (regarding §§ 4.2 and 4.4), and Jan Wolenski (regarding §5.3). I invite anyone who has further suggestions of items to be included or comments on the article itself to email me at the address given below.
Copyright © 2014 by Michael Beaney < michael . beaney @ hu-berlin . de >
- Accessibility
Support SEP
Mirror sites.
View this site from another server:
- Info about mirror sites
The Stanford Encyclopedia of Philosophy is copyright © 2023 by The Metaphysics Research Lab , Department of Philosophy, Stanford University
Library of Congress Catalog Data: ISSN 1095-5054

An official website of the United States government
The .gov means it's official. Federal government websites often end in .gov or .mil. Before sharing sensitive information, make sure you're on a federal government site.
The site is secure. The https:// ensures that you are connecting to the official website and that any information you provide is encrypted and transmitted securely.
- Publications
- Account settings
- Browse Titles
NCBI Bookshelf. A service of the National Library of Medicine, National Institutes of Health.
Methods Guide for Effectiveness and Comparative Effectiveness Reviews [Internet]. Rockville (MD): Agency for Healthcare Research and Quality (US); 2008-.

Methods Guide for Effectiveness and Comparative Effectiveness Reviews [Internet].
Quantitative synthesis—an update.
Investigators: Sally C. Morton , Ph.D., M.Sc., M. Hassan Murad , M.D., M.P.H., Elizabeth O’Connor , Ph.D., Christopher S. Lee , Ph.D., R.N., Marika Booth , M.S., Benjamin W. Vandermeer , M.Sc., Jonathan M. Snowden , Ph.D., Kristen E. D’Anci , Ph.D., Rongwei Fu , Ph.D., Gerald Gartlehner , M.D., M.P.H., Zhen Wang , Ph.D., and Dale W. Steele , M.D., M.S.
Affiliations
Published: February 23, 2018 .
Quantitative synthesis, or meta-analysis, is often essential for Comparative Effective Reviews (CERs) to provide scientifically rigorous summary information. Quantitative synthesis should be conducted in a transparent and consistent way with methodologies reported explicitly. This guide provides practical recommendations on conducting synthesis. The guide is not meant to be a textbook on meta-analysis nor is it a comprehensive review of methods, but rather it is intended to provide a consistent approach for situations and decisions that are commonly faced by AHRQ Evidence-based Practice Centers (EPCs). The goal is to describe choices as explicitly as possible, and in the context of EPC requirements, with an appropriate degree of confidence.
This guide addresses issues in the order that they are usually encountered in a synthesis, though we acknowledge that the process is not always linear. We first consider the decision of whether or not to combine studies quantitatively. The next chapter addresses how to extract and utilize data from individual studies to construct effect sizes, followed by a chapter on statistical model choice. The fourth chapter considers quantifying and exploring heterogeneity. The fifth describes an indirect evidence technique that has not been included in previous guidance – network meta-analysis, also known as mixed treatment comparisons. The final section in the report lays out future research suggestions.
The Agency for Healthcare Research and Quality (AHRQ), through its Evidence-based Practice Centers (EPCs), sponsors the development of evidence reports and technology assessments to assist public- and private-sector organizations in their efforts to improve the quality of health care in the United States. The reports and assessments provide organizations with comprehensive, science-based information on common, costly medical conditions and new health care technologies and strategies. The EPCs systematically review the relevant scientific literature on topics assigned to them by AHRQ and conduct additional analyses when appropriate prior to developing their reports and assessments.
Strong methodological approaches to systematic review improve the transparency, consistency, and scientific rigor of these reports. Through a collaborative effort of the Effective Health Care (EHC) Program, the Agency for Healthcare Research and Quality (AHRQ), the EHC Program Scientific Resource Center, and the AHRQ Evidence-based Practice Centers have developed a Methods Guide for Comparative Effectiveness Reviews. This Guide presents issues key to the development of Systematic Reviews and describes recommended approaches for addressing difficult, frequently encountered methodological issues.
The Methods Guide for Comparative Effectiveness Reviews is a living document, and will be updated as further empiric evidence develops and our understanding of better methods improves. We welcome comments on this Methods Guide paper. They may be sent by mail to the Task Order Officer named below at: Agency for Healthcare Research and Quality, 5600 Fishers Lane, Rockville, MD 20857, or by email to vog.shh.qrha@cpe .
- Gopal Khanna, M.B.A. Director Agency for Healthcare Research and Quality
- Arlene S. Bierman, M.D., M.S. Director Center for Evidence and Practice Improvement Agency for Healthcare Research and Quality
- Stephanie Chang, M.D., M.P.H. Director Evidence-based Practice Center Program Center for Evidence and Practice Improvement Agency for Healthcare Research and Quality
- Elisabeth Kato, M.D., M.R.P. Task Order Officer Evidence-based Practice Center Program Center for Evidence and Practice Improvement Agency for Healthcare Research and Quality
- Peer Reviewers
Prior to publication of the final evidence report, EPCs sought input from independent Peer Reviewers without financial conflicts of interest. However, the conclusions and synthesis of the scientific literature presented in this report does not necessarily represent the views of individual investigators.
Peer Reviewers must disclose any financial conflicts of interest greater than $10,000 and any other relevant business or professional conflicts of interest. Because of their unique clinical or content expertise, individuals with potential non-financial conflicts may be retained. The TOO and the EPC work to balance, manage, or mitigate any potential non-financial conflicts of interest identified.
- Eric Bass, M.D., M.P.H Director, Johns Hopkins University Evidence-based Practice Center Professor of Medicine, and Health Policy and Management Johns Hopkins University Baltimore, MD
- Mary Butler, M.B.A., Ph.D. Co-Director, Minnesota Evidence-based Practice Center Assistant Professor, Health Policy & Management University of Minnesota Minneapolis, MN
- Roger Chou, M.D., FACP Director, Pacific Northwest Evidence-based Practice Center Portland, OR
- Lisa Hartling, M.S., Ph.D. Director, University of Alberta Evidence-Practice Center Edmonton, AB
- Susanne Hempel, Ph.D. Co-Director, Southern California Evidence-based Practice Center Professor, Pardee RAND Graduate School Senior Behavioral Scientist, RAND Corporation Santa Monica, CA
- Robert L. Kane, M.D. * Co-Director, Minnesota Evidence-based Practice Center School of Public Health University of Minnesota Minneapolis, MN
- Jennifer Lin, M.D., M.C.R. Director, Kaiser Permanente Research Affiliates Evidence-based Practice Center Investigator, The Center for Health Research, Kaiser Permanente Northwest Portland, OR
- Christopher Schmid, Ph.D. Co-Director, Center for Evidence Synthesis in Health Professor of Biostatistics School of Public Health Brown University Providence, RI
- Karen Schoelles, M.D., S.M., FACP Director, ECRI Evidence-based Practice Center Plymouth Meeting, PA
- Tibor Schuster, Ph.D. Assistant Professor Department of Family Medicine McGill University Montreal, QC
- Jonathan R. Treadwell, Ph.D. Associate Director, ECRI Institute Evidence-based Practice Center Plymouth Meeting, PA
- Tom Trikalinos, M.D. Director, Brown Evidence-based Practice Center Director, Center for Evidence-based Medicine Associate Professor, Health Services, Policy & Practice Brown University Providence, RI
- Meera Viswanathan, Ph.D. Director, RTI-UNC Evidence-based Practice Center Durham, NC RTI International Durham, NC
- C. Michael White, Pharm. D., FCP, FCCP Professor and Head, Pharmacy Practice School of Pharmacy University of Connecticut Storrs, CT
- Tim Wilt, M.D., M.P.H. Co-Director, Minnesota Evidence-based Practice Center Director, Minneapolis VA-Evidence Synthesis Program Professor of Medicine, University of Minnesota Staff Physician, Minneapolis VA Health Care System Minneapolis, MN
Deceased March 6, 2017
- Introduction
The purpose of this document is to consolidate and update quantitative synthesis guidance provided in three previous methods guides. 1 – 3 We focus primarily on comparative effectiveness reviews (CERs), which are systematic reviews that compare the effectiveness and harms of alternative clinical options, and aim to help clinicians, policy makers, and patients make informed treatment choices. We focus on interventional studies and do not address diagnostic studies, individual patient level analysis, or observational studies, which are addressed elsewhere. 4
Quantitative synthesis, or meta-analysis, is often essential for CERs to provide scientifically rigorous summary information. Quantitative synthesis should be conducted in a transparent and consistent way with methodologies reported explicitly. This guide provides practical recommendations on conducting synthesis. The guide is not meant to be a textbook on meta-analysis nor is it a comprehensive review of methods, but rather it is intended to provide a consistent approach for situations and decisions that are commonly faced by Evidence-based Practice Centers (EPCs). The goal is to describe choices as explicitly as possible and in the context of EPC requirements, with an appropriate degree of confidence.
EPC investigators are encouraged to follow these recommendations but may choose to use alternative methods if deemed necessary after discussion with their AHRQ project officer. If alternative methods are used, investigators are required to provide a rationale for their choices, and if appropriate, to state the strengths and limitations of the chosen methods in order to promote consistency, transparency, and learning. In addition, several steps in meta-analysis require subjective judgment, such as when combining studies or incorporating indirect evidence. For each subjective decision, investigators should fully explain how the decision was reached.
This guide was developed by a workgroup comprised of members from across the EPCs, as well as from the Scientific Resource Center (SRC) of the AHRQ Effective Healthcare Program. Through surveys and discussions among AHRQ, Directors of EPCs, the Scientific Resource Center, and the Methods Steering Committee, quantitative synthesis was identified as a high-priority methods topic and a need was identified to update the original guidance. 1 , 5 Once confirmed as a Methods Workgroup, the SRC solicited EPC workgroup volunteers, particularly those with quantitative methods expertise, including statisticians, librarians, thought leaders, and methodologists. Charged by AHRQ to update current guidance, the workgroup consisted of members from eight of 13 EPCs, the SRC, and AHRQ, and commenced in the fall of 2015. We conducted regular workgroup teleconference calls over the course of 14 months to discuss project direction and scope, assign and coordinate tasks, collect and analyze data, and discuss and edit draft documents. After constructing a draft table of contents, we surveyed all EPCs to ensure no topics of interest were missing.
The initial teleconference meeting was used to outline the draft, discuss the timeline, and agree upon a method for reaching consensus as described below. The larger workgroup then was split into subgroups each taking responsibility for a different chapter. The larger group participated in biweekly discussions via teleconference and email communication. Subgroups communicated separately (in addition to the larger meetings) to coordinate tasks, discuss the literature review results, and draft their respective chapters. Later, chapter drafts were combined into a larger document for workgroup review and discussion on the bi-weekly calls.
Literature Search and Review
A medical research librarian worked with each subgroup to identify a relevant search strategy for each chapter, and then combined these strategies into one overall search conducted for all chapters combined. The librarian conducted the search on the ARHQ SRC Methods Library, a bibliographic database curated by the SRC currently containing more than 16,000 citations of methodological works for systematic reviews and comparative effectiveness reviews, using descriptor and keyword strategies to identify quantitative synthesis methods research publications (descriptor search=all quantitative synthesis descriptors, and the keyword search=quantitative synthesis, meta-anal*, metaanal*, meta-regression in [anywhere field]). Search results were limited to English language and 2009 and later to capture citations published since AHRQ’s previous methods guidance on quantitative synthesis. Additional articles were identified from recent systematic reviews, reference lists of reviews and editorials, and through the expert review process.
The search yielded 1,358 titles and abstracts which were reviewed by all workgroup members using ABSTRACKR software (available at http://abstrackr.cebm.brown.edu ). Each subgroup separately identified articles relevant to their own chapter. Abstract review was done by single review, investigators included anything that could be potentially relevant. Each subgroup decided separately on final inclusion/exclusion based on full text articles.
Consensus and Recommendations
Reaching consensus if possible is of great importance for AHRQ methods guidance. The workgroup recognized this importance in its first meeting and agreed on a process for informal consensus and conflict resolution. Disagreements were thoroughly discussed and if possible, consensus was reached. If consensus was not reached, analytic options are discussed in the text. We did not employ a formal voting procedure to assess consensus.
A summary of the workgroup’s key conclusions and recommendations was circulated for comment by EPC Directors and AHRQ officers at a biannual EPC Director’s meeting in October 2016. In addition, a full draft was circulated to EPC Directors and AHRQ officers prior to peer review, and the manuscript was made available for public review. All comments have been considered by the team in the final preparation of this report.
Chapter 1. Decision to Combine Trials
1.1. goals of the meta-analysis.
Meta-analysis is a statistical method for synthesizing (also called combining or pooling) the benefits and/or harms of a treatment or intervention across multiple studies. The overarching goal of a meta-analysis is generally to provide the best estimate of the effect of an intervention. As part of that aspirational goal, results of a meta-analysis may inform a number of related questions, such as whether that best estimate represents something other than a null effect (is this intervention beneficial?), the range in which the true effect likely lies, whether it is appropriate to provide a single best estimate, and what study-level characteristics may influence the effect estimate. Before tackling these questions, it is necessary to answer a preliminary but fundamental question: Is it appropriate to pool the results of the identified studies? 6
Clinical, methodological, and statistical factors must all be considered when deciding whether to combine studies in a meta-analysis. Figure 1.1 depicts a decision tree to help investigators think through these important considerations, which are discussed below.
Pooling decision tree.
1.2. Clinical and Methodological Heterogeneity
Studies must be reasonably similar to be pooled in a meta-analysis. 1 Even when the review protocol identifies a coherent and fairly narrow body of literature, the actual included studies may represent a wide range of population, intervention, and study characteristics. Variations in these factors are referred to as clinical heterogeneity and methodological heterogeneity. 7 , 8 A third form of heterogeneity, statistical heterogeneity, will be discussed later.
The first step in the decision tree is to explore the clinical and methodological heterogeneity of the included studies (Step A, Figure 1.1 ). The goal is to identify groups of trials that are similar enough that an average effect would make a sensible summary. There is no objective measure or universally accepted standard for deciding whether studies are “similar enough” to pool; this decision is inherently a matter of judgment. 6 Verbeek and colleagues suggest working through key sources of variability in sequence, beginning with the clinical variables of intervention/exposure, control condition, and participants, before moving on to methodological areas such as study design, outcome, and follow-up time. When there is important variability in these areas, investigators should consider whether there are coherent subgroups of trials, rather than the full group, that can be pooled. 6
Clinical heterogeneity refers to characteristics related to the participants, interventions, types of outcomes, and study setting. Some have suggested that pooling may be acceptable when it is plausible that the underlying effects could be similar across subpopulations and variations in interventions and outcomes. 9 For example, in a review of a lipid-lowering medication, researchers might be comfortable combining studies that target younger and middle-aged adults, but expect different effects with older adults, who have high rates of comorbidities and other medication use. Others suggest that it may be acceptable to combine interventions with likely similar mechanisms of action. 6 For example, a researcher may combine studies of depression interventions that use a range of psychotherapeutic approaches, on the logic that they all aim to change a person’s thinking and behavior in order to improve mood, but not want to combine them with trials of antidepressants, whose mechanism of action is presumed to be biochemical.
Methodological heterogeneity refers to variations in study methods (e.g., study design, measures, and study conduct). A common question regarding study design, is whether it is acceptable to combine studies that randomize individual participants with those that randomize clusters (e.g., when clinics, clinicians, or classrooms are randomized and individuals are nested within these units). We believe this is generally acceptable, with appropriate adjustment for cluster randomization as needed. 10 However, closer examination may show that the cluster randomized trials also tend to systematically differ on population or intervention characteristics from the individually-randomized trials. If so, subgroup analyses may be considered.
Outcome measures are a common source of methodological heterogeneity. First, trials may have a wide array of specific instruments and cut-points for a common outcome. For example, a review considering pooling the binary outcome of depression prevalence may find measures that range from a depression diagnosis based on a clinical interview to scores above a cut-point on a screening instrument. One guiding principle is to consider pooling only when it is plausible that the underlying relative effects are consistent across specific definitions of an outcome. In addition, investigators should take steps to harmonize outcomes to the extent possible.
Second, there is also typically substantial variability in the statistics reported across studies (e.g., odds ratios, relative risks, hazard ratios, baseline and mean followup scores, change scores for each condition, between-group differences at followup, etc.). Methods to calculate or estimate missing statistics are available, 5 however the investigators must ultimately weigh the tradeoff of potentially less accurate results (due to assumptions required to estimate missing data) with the potential advantage of pooling a more complete set of studies. If a substantial proportion of the studies require calculations that involve assumptions or estimates (rather than straightforward calculations) in order to combine them, then it may be preferable to show results in a table or forest plot without a pooled estimate
1.3. Best Evidence Versus All Evidence
Sometimes the body of evidence comprises a single trial or small number of trials that clearly represent the best evidence, along with a number of additional trials that are much smaller or with other important limitations (Step B, Figure 1.1 ). The “best evidence” trials are generally very large trials with low risk of bias and with good generalizability to the population of interest. In this case, it may be appropriate to focus on the one or few “best” trials rather than combining them with the rest of the evidence, particularly when addressing rare events that small studies are underpowered to examine. 11 , 12 For example, an evidence base of one large, multi-center trial of an intervention to prevent stroke in patients with heart disease could be preferable to a pooled analysis of 4-5 small trials reporting few events, and combining the small trials with the large trial may introduce unnecessary uncertainty to the pooled estimate.
1.4. Assessing the Risk of Misleading Meta-analysis Results
Next, reviews should explore the risk that the meta-analysis will show results that do not accurately capture the true underlying effect (Step C, Figure 1.1 ). Tables, forest plots (without pooling), and some other preliminary statistical tests are useful tools for this stage. Several patterns can arise that should lead investigators to be cautious about combining studies.
Wide-Ranging Effect Sizes
Sometimes one study may show a large benefit and another study of the same intervention may show a small benefit. This may be due to random error, especially when the studies are small. However, this situation also raises the possibility that observed effects truly are widely variable in different subpopulations or situations. Another look at the population characteristics is warranted in this situation to see if the investigators can identify characteristics that are correlated with effect size and direction, potentially explaining clinical heterogeneity.
Even if no characteristic can be identified that explains why the intervention had such widely disparate effects, there could be unmeasured features that explain the difference. If the intervention really does have widely variable impact in different subpopulations, particularly if it is benefiting some patients and harming others, it would be misleading to report a single average effect.
Suspicion of Publication or Reporting Bias
Sometimes, due to lack of effect, trial results are never published (risking publication bias), or are only published in part (risking reporting bias). These missing results can introduce bias and reduce the precision of meta-analysis. 13 Investigators can explore the risk of reporting bias by comparing trials that do and do not report important outcomes to assess whether outcomes appear to be missing at random. 13 For example, investigators may have 30 trials of weight loss interventions with only 10 reporting blood pressure, which is considered an important outcome for the review. This pattern of results may indicate reporting bias as trials finding group differences in blood pressure were more likely to report blood pressure findings. On the other hand, perhaps most of the studies limited to patients with elevated cardiovascular disease (CVD) risk factors did report blood pressure. In this case, the investigators may decide to combine the studies reporting blood pressure that were conducted in high CVD risk populations. However, investigators should be clear about the applicable subpopulation. An examination of the clinical and methodological features of the subset of trials where blood pressure was reported is necessary to make an informed judgement about whether to conduct a meta-analysis.
Small Studies Effect
If small studies show larger effects than large studies, the pooled results may overestimate the true effect size, possibly due to publication or reporting bias. 14 When investigators have at least 10 trials to combine they should examine small studies effects using standard statistical tests such as the Egger test. 15 If there appears to be a small studies effect, the investigators may decide not to report pooled results since they could be misleading. On the other hand, small studies effects could be happening for other reasons, such as differences in sample characteristics, attrition, or assessment methods. These factors do not suggest bias, but should be explored to the degree possible. See Chapter 4 for more information about exploring heterogeneity.
1.5. Special Considerations When Pooling a Small Number of Studies
When pooling a small number of studies (e.g., <10 studies), a number of considerations arise (Step E, Figure 1.1 ):
Rare Outcomes
Meta-analyses of rare binary outcomes are frequently underpowered, and tend to overestimate the true effect size, so pooling should be undertaken with caution. 11 A small difference in absolute numbers of events can result in large relative differences, usually with low precision (i.e., wide confidence intervals). This could result in misleading effect estimates if the analysis is limited to trials that are underpowered for the rare outcomes. 12 One example is all-cause mortality, which is frequently provided as part of the participant flow results, but may not be a primary outcome, may not have adjudication methods described, and typically occurs very rarely. Studies are often underpowered to detect differences in mortality if it is not a primary outcome. Investigators should consider calculating an optimal information size (OIS) when events are rare to see if the combined group of studies has sufficient power to detect group differences. This could be a concern even for a relatively large number of studies, if the total sample size is not very large. 16 See Chapter 3 for more detail on handling rare binary outcomes.
Small Sample Sizes
When pooling a relatively small number of studies, pooling should be undertaken with caution if the body of evidence is limited only to small studies. Results from small trials are less likely to be reliable than results of large trials, even when the risk of bias is low. 17 First, in small trials it is difficult to balance the proportion of patients in potentially important subgroups across interventions, and a difference between interventions of just a few patients in a subgroup can result in a large proportional difference between interventions. Characteristics that are rare are particularly at risk of being unbalanced in trials with small samples. In such situations there is no way to know if trial effects are due to the intervention or to differences in the intervention groups. In addition, patients are generally drawn from a narrower geographic range in small trials, making replication in other trials more uncertain. Finally, although it is not always the case, large trials are more likely to involve a level of scrutiny and standardization to ensure lower risk of bias than are small trials. Therefore, when the trials have small sample sizes, pooled effects are less likely to reflect the true effects of the intervention. In this case, the required or optimal information size can help the investigators determine whether the sample size is sufficient to conclude that results are likely to be stable and not due to random heterogeneity (i.e., truly significant or truly null results; not a type I or type II error). 16 , 18 An option in this case would be to pool the studies and acknowledge imprecision or other limitations when rating the strength of evidence.
What would be considered a “small” trial varies for different fields and outcomes. For addressing an outcome that only happens in 10% of the population, a small trial might be 100 to 200 per intervention arm, whereas a trial addressing a continuous quality of life measure may be small with 20 to 30 per intervention. Looking carefully at what the studies were powered to detect and the credibility of the power calculations may help determine what constitutes a “small” trial. Investigators should also consider how variable the impact of an intervention may be over different settings and subpopulations when determining how to weigh the importance of small studies. For example, the effects of a counseling intervention that relies on patients to change their behavior in order to reap health benefits may be more strongly influenced by characteristics of the patients and setting than a mechanical or chemical agent.
When the number of trials to be pooled is small, there is a heightened risk that statistical heterogeneity will be substantially underestimated, resulting in 95% confidence intervals that are inappropriately narrow and do not have 95% coverage. This is especially concerning when the number of studies being pooled is fewer than five to seven. 19 – 21
Accounting for these factors should guide an evaluation of whether it is advisable to pool the relatively small group of studies. As with many steps in the multi-stage decision to pool, the conclusion that a given investigator arrives at is subjective, although such evaluations should be guided by the criteria above. If consideration of these factors reassures investigators that the risk of bias associated with pooling is sufficiently low, then pooling can proceed. The next step of pooling, whether for a small, moderate, or large body of studies, is to consider statistical heterogeneity.
1.6. Statistical Heterogeneity
Once clinical and methodological heterogeneity and other factors described above have been deemed acceptable for pooling, investigators should next consider statistical heterogeneity (Step F, Figure 1.1 ). We discuss statistical heterogeneity in general in this chapter, and provide a deeper methodological discussion in Chapter 4 . This initial consideration of statistical heterogeneity is accomplished by conducting a preliminary meta-analysis. Next the investigator must decide if the results of the meta-analysis are valid and should be presented, rather than simply showing tables or forest plots without pooled results. If statistical heterogeneity is very high, the investigators may question whether an “average” effect is really meaningful or useful. If there is a reasonably large number of trials, the investigators may shift to exploring effect modification with high heterogeneity, however this may not be possible if few trials are available. While many would likely agree that pooling (or reporting pooled results) should be avoided when there are few studies and statistical heterogeneity is high, what constitutes “few” studies and “high” heterogeneity is a matter of judgment.
While there are a variety of methods for characterizing statistical heterogeneity, one common method is the I 2 statistic, the proportion of total variance in the pooled trials that is due to inter-study variance, as opposed to random variation. 22 The Cochrane manual proposes ranges for interpreting I 2 : 10 statistical heterogeneity associated with I 2 values of 0-40% might not be important, 30-60% may represent moderate heterogeneity, 50-90% may represent substantial heterogeneity, and 75-100% is considerable heterogeneity. Ranges overlap to reflect that other factors—such as the number and size of the trials and the magnitude and direction of the effect—must be taken into consideration. Other measures of statistical heterogeneity include Cochrane’s Q and τ 2 , but these heterogeneity statistics do not have intrinsic standardized scales that allow specific values to be characterized as “small,” “medium,” or “large” in any meaningful way. 23 However, τ 2 can be interpreted on the scale of the pooled effect, as the variance of the true effect. All these measures are discussed in more detail in Chapter 4 .
Although widely used in quantitative synthesis, the I 2 statistic has come under criticism in recent years. One important issue with I 2 is that it can be an inaccurate reflection of statistical heterogeneity when there are few studies to pool and high statistical heterogeneity. 24 , 25 For example, in random effects models (but not fixed effects models), calculations demonstrate that I 2 tends to underestimate true statistical heterogeneity when there are fewer than about 10 studies and the I 2 is 50% or more. 26 In addition, I 2 is correlated with the sample size of the included studies, generally increasing with larger samples. 27 Complicating this, meta-analyses of continuous measures tend to have higher heterogeneity than those of binary outcomes, and I 2 tends to increase as the number of studies increases when analyzing continuous outcomes, but not binary outcomes. 28 , 29 This has prompted some authors to suggest that different standards may be considered for interpreting I 2 for meta-analyses of continuous and binary outcomes, but I 2 should only be considered reliable when there are a sufficient number of studies. 29 Unfortunately there is not clear consensus regarding what constitutes a sufficient number of studies for a given amount of statistical heterogeneity, nor is it possible to be entirely prescriptive, given the limits of I 2 as a measure of heterogeneity. Thus, I 2 is one piece of information that should be considered, but generally should not be the primary deciding factor for whether to pool.
1.7. Conclusion
In the end, the decision to pool boils down to the question: will the results of a meta-analysis help you find a scientifically valid answer to a meaningful question? That is, will the meta-analysis provide something in addition to what can be understood from looking at the studies individually? Further, do the clinical, methodological, and statistical features of the body of studies permit them to be quantitatively combined and summarized in a valid fashion? Each of these decisions can be broken down into specific considerations (outlined in Figure 1.1 ) There is broad guidance to inform investigators in making each of these decisions, but generally the choices involved are subjective. The investigators’ scientific goal might factor into the evaluation of these considerations: for example, if investigators seek a general summary of the combined effect (e.g., direction only) versus an estimated effect size, the consideration of whether to pool may be weighed differently. In the end, to provide a meaningful result, the trials must be similar enough in content, procedures, and implementation to represent a cohesive group that is relevant to real practice/decision-making.
Recommendations
- Use Figure 1.1 when deciding whether to pool studies
Chapter 2. Optimizing Use of Effect Size Data
2.1. introduction.
The employed methods for meta-analysis will depend upon the nature of the outcome data. The two most common data types encountered in trials are binary/dichotomous (e.g., dead or alive, patient admitted to hospital or not, treatment failure or success, etc.) and continuous (e.g., weight, systolic blood pressure, etc.). Some outcomes (e.g., heart rate, counts of common events) that are not strictly continuous, are often treated as continuous for the purposes of meta-analysis based on assumptions of normality and the belief that statistical methods that are applied to normal distributions can be applicable to other distributions (central limit theory). Continuous outcomes are also frequently analyzed as binary outcomes when there are clinically meaningful cut-points or thresholds (e.g., a patient’s systolic blood pressure may be classified as low or high based on whether it is under or over 130mmHG). While this type of dichotomization may be more clinically meaningful it reduces statistical information, so investigators should provide their rationale for taking this approach.
Other less common data types that do not fit into either the binary or continuous categories include ordinal, categorical, rate, and time to event to data. Meta-analyzing these types of data will usually require reporting of the relevant statistics (e.g., hazard ratio, proportional odds ratio, incident rate ratio) by the study authors.
2.2. Nuances of Binary Effect Sizes
Data needed for binary effect size computation.
Under ideal circumstances, the minimal data necessary for the computation of effect sizes of binary data would be available in published trial documents or from original sources. Specifically, risk difference (RD), relative risk (RR), and odds ratios (OR) can be computed when the number of events (technically the number of cases in whom there was an event) and sample sizes are known for treatment and control groups. A schematic of one common approach to assembling binary data from trials for effect size computation is presented in Table 2.1 . This approach will facilitate conversion to analysis using commercially-available software such as Stata (College Station, TX) or Comprehensive Meta-Analysis (Englewood, NJ).
Assembling binary data for effect size computation.
In many instances, a single study (or subset of studies) to be included in the meta-analysis provides only one measure of association (an odds ratio, for example), and the sample size and event counts are not available. In that case, the meta-analytic effect size will be dictated by the available data. However, choosing the appropriate effect size is important for integrity and transparency, and every effort should be made to obtain all the data presented in Table 2.1 . Note that CONSORT guidance requires that published trial data should include the number of events and sample sizes for both treatment and control groups. 30 And, PRISMA guidance supports describing any processes for obtaining and confirming data from investigators 31 – a frequently required step.
In the event that data are only available in an effect size from the original reports, it is important to extract both the mean effect sizes and the associated 95% confidence intervals. Having raw event data available as in Table 2.1 not only facilitates the computation of various effect sizes, but also allows for the application of either binomial (preferred) or normal likelihood approaches; 32 only normal likelihood can be applied to summary statistics (e.g., an odds ratio and confidence interval in the primary study report).
Choosing Among Effect Size Options
One absolute measure and two relative measures are commonly used in meta-analyses involving binary data. The RD (an absolute measure) is a simple metric that is easily understood by clinicians, patients, and other stakeholders. The relative measures, RR or OR, are also used frequently. All three metrics should be considered additive, just on different scales. That is, RD is additive on a raw scale, RR on a log scale, and OR on a logit scale.
Risk Difference
The RD is easily understood by clinicians and patients alike, and therefore most useful to aid decision making. However, the RD tends to be less consistent across studies compared with relative measures of effect size (RR and OR). Hence, the RD may be a preferred measure in meta-analyses when the proportions of events among control groups are relatively common and similar across studies. When events are rare and/or when event rates differ across studies, however, the RD is not the preferred effect size to be used in meta-analysis because combined estimates based on RD in such instances have more conservative confidence intervals and lower statistical power. The calculation of RD and other effect size metrics using binary data from clinical trials can be performed considering the following labeling ( Table 2.2 ).
Organizing binary data for effect size computation.
Equation Set 2.1. Risk Difference
- RD = risk difference
- V RD = variance of the risk difference
- SE RD = standard error of the risk difference
- LL RD = lower limit of the 95% confidence interval of the risk difference
- UL RD = upper limit of the 95% confidence interval of the risk difference
Number Needed To Treat Related to Risk Difference
- NNT = number needed to treat
In case of a negative RD, the number needed to harm (NNH) or number needed to treat for one patient to be harmed is = − 1/RD.
The Wald method 34 is commonly used to calculate confidence intervals for NNT. It is reasonably adequate for large samples and probabilities not close to either 0 or 1, however it can be less reliable for small samples, probabilities close to either 0 or 1, or unbalanced trial designs. 35 An adjustment to the Wald method (i.e., adding pseudo-observations) helps mitigate concern about its application in small samples, 36 but it doesn’t account for other sources of limitations to this method. The Wilson method of calculating confidence intervals for NNT, as described in detail by Newcome, 37 has better coverage properties irrespective of sample size, is free of implausible results, and is argued to be easier to calculate compared with Wald confidence intervals. 35 Therefore, the Wilson method is preferable to the Wald method for calculating confidence intervals for NNT. When considering using NNT as the effect size in meta-analysis, see commentary by Lesaffre and Pledger.38 When considering using NNT as the effect size in meta-analysis, see commentary on the superior performance of combined NNT on the RD scale as opposed to the NNT scale.
It is important to note that the RR and OR are effectively equivalent for event rates below about 10%. In such cases, the RR is chosen over the OR simply for interpretability (an important consideration) and not substantive differences. A potential drawback to the use of RR over OR (or RD) is that the RR of an event is not the reciprocal of the RR for the non-occurrence of that event (e.g., using survival as the outcome instead of death). In contrast, switching between events and non-occurrence of events is reciprocal in the metric of OR and only entails a change in the sign of OR. If switching between death and survival, for example, is central to the meta-analysis, then the RR is likely not the binary effect size metric of choice unless all raw data are available and re-computation is possible. Moreover, investigators should be particularly attentive to the definition of an outcome event when using a RR.
The calculation of RR using binary data can be performed considering the labeling listed in Table 2.2 . Of particular note, the metrics of dispersion related to the RR are first computed in a natural log metric and then converted to the metric of RR.
Equation Set 2.2. Risk Ratio
- RR = risk ratio
- ln RR = natural log of the risk ratio
- V lnRR = variance of the natural log of the risk ratio
- SE lnRR = standard error of the natural log of the risk ratio
- LLlnRR = lower limit of the 95% confidence interval of the natural log of the risk ratio
- UL lnRR = upper limit of the 95% confidence interval of the natural log of the risk ratio
- LL RR = lower limit of the 95% confidence interval of the risk ratio
- UL RR = upper limit of the 95% confidence interval of the risk ratio
Therefore, while the definition of the outcome event needs to be consistent among the included studies when using any measure, the investigators should be particularly attentive to the definition of an outcome event when using an RR.
Odds Ratios
An alternative relative metric for use with binary data is the OR. Given that ORs are frequently presented in models with covariates, it is important to note that the OR is ‘non-collapsible,’ meaning that effect modification varies depending on the covariates for which control has been made; this favors the reporting of RR over OR, particularly when outcomes are common and covariates are included. 39 The calculation of OR using binary data can be performed considering the labeling listed in Table 2.2 . Similar to the computation of RR, the metrics of dispersion related to the OR are first computed in a natural log metric and then converted to the metric of OR.
Equation Set 2.3. Odds ratios
- OR = odds ratio
- Ln OR = natural log of the odds ratio
- V lnOR = variance of the natural log of the odds ratio
- SE lnoR = standard error of the natural log of the odds ratio
- LLlnOR = lower limit of the 95% confidence interval of the natural log of the odds ratio
- UL lnOR = upper limit of the 95% confidence interval of the natural log of the odds ratio
- LL OR = lower limit of the 95% confidence interval of the odds ratio
- UL OR = upper limit of the 95% confidence interval of the odds ratio
A variation on the calculation of OR is the Peto OR that is commonly referred to as the assumption-free method of calculating OR. The two key differences between the standard OR and the Peto OR is that the latter takes into consideration the expected number of events in the treatment group and also incorporates a hypergeometric variance. Because of these difference, the Peto OR is preferred for binary studies with rare events, especially when event rates are less than 1%. But in contrast, the Peto OR is biased when treatment effects are large, due to centering around the null hypothesis, and in the instance of imbalanced treatment and control groups. 40
Equation Set 2.4. Peto odds ratios
ORpeto = exp [ { A − E ( A ) } / v ] where E(A) is the expected number of events in the treatment group calculated as: E ( A ) = n 1 ( A + E ) N and v is hypergeometric variance, calculated as: v = { n 1 n 2 ( A + C ) ( B + D ) } / { N 2 ( N − 1 ) }
There is no perfect effect size of binary data to choose because each has benefits and disadvantages. Criteria used to compare and contrast these measures include consistency over a set of studies, statistical properties, and interpretability. Key benefits and disadvantages of each are presented in Table 2.3 . In the table, the term “baseline risk” is the proportion of subjects in the control group who experienced the event. The term “control rate” is sometimes used for this measure as well.
Benefits and disadvantages of binary data effect sizes.
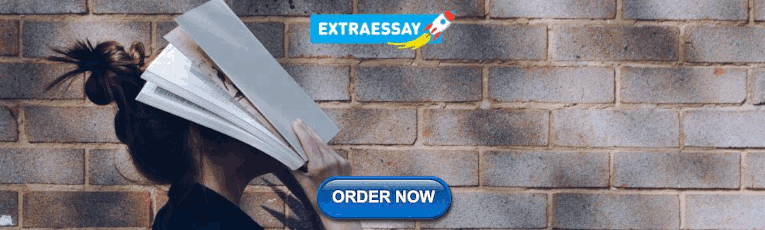
Time-to-Event and Count Outcomes
For time to event data, the effect size measure is a hazard ratio (HR), which is commonly estimated from the Cox proportional hazards model. In the best-case scenario, HR and associated 95% confidence intervals are available from all studies, the time horizon is similar across studies, and there is evidence that the proportional hazards assumption was met in each study to be included in a meta-analysis. When these conditions are not met, an HR and associated dispersion can still be extracted and meta-analyzed. However, this approach raises concerns about reproducibility due to observer variation. 44
Incident rate ratio (IRR) is used for count data and can be estimated from a Poisson or negative binomial regression model. The IRR is a relative metric based on counts of events (e.g., number of hospitalizations, or days of length of stay) over time (i.e., per person-year) compared between trial arms. It is important to consider how IRR estimates were derived in individual studies particularly with respect to adjustments for zero-inflation and/or over-dispersion as these modeling decisions can be sources of between-study heterogeneity. Moreover, studies that include count data may have zero counts in both groups, which may require less common and more nuanced approaches to meta-analysis like Poisson regression with random intervention effects. 45
2.3. Continuous Outcomes
Assembling data needed for effect size computation.
Meta-analysis of studies presenting continuous data requires both estimated differences between the two groups being compared and estimated standard errors of those differences. Estimating the between-group difference is easiest when the study provides the mean difference. While both a standardized mean difference and ratio of means could be given by the study authors, studies more often report means for each group. Thus, a mean difference or ratio of means often must be computed.
If estimates of the standard errors of the mean are not provided studies commonly provide confidence intervals, standard deviations, p-values, z-statistics, and/or t-statistics, which make it possible to compute the standard error of the mean difference. In the absence of any of these statistics, other methods are available to estimate standard error. 45
(Weighted) Mean Difference
The mean difference (formerly known as weighted mean difference) is the most common way of summarizing and pooling a continuous outcome in a meta-analysis. Pooled mean differences can be computed when every study in the analysis measures the outcome on the same scale or on scales that can be easily converted. For example, total weight can be pooled using mean difference even if different studies reported weights in kilograms and pounds; however it is not possible to pool quality of life measured in both Self Perceived Quality of Life scale (SPQL) and the 36-item Short Form Survey Instrument (SF-36), since these are not readily convertible to one format.
Computation of the mean difference is straightforward and explained elsewhere. 5 Most software programs will require the mean, standard deviation, and sample size from each intervention group and for each study in the meta-analysis, although as mentioned above, other pieces of data may also be used.
Some studies report values as change from baseline, or alternatively present both baseline and final values. In these cases, it is possible to pool differences in final values in some studies with differences in change from baseline values in other studies, since they will be estimating the same value in a randomized control trial. If baseline values are unbalanced it may be better to perform ANCOVA analysis (see below). 5
Standardized Mean Difference
Sometimes different studies will assess the same outcome using different scales or metrics that cannot be readily converted to a common measure. In such instances the most common response is to compute a standardized mean difference (SMD) for each study and then pool these across all studies in the meta-analysis. By dividing the mean difference by a pooled estimate of the standard deviation, we theoretically put all scales in the same unit (standard deviation), and are then able to statistically combine all the studies. While the standardized mean difference could be used even when studies use the same metric, it is generally preferred to use mean difference. Interpretation of results is easier when the final pooled estimate is given in the same units as the original studies.
Several methods can compute SMDs. The most frequently used are Cohen’s d and Hedges’ g .
Cohen’s d
Cohen’s d is the simplest S. computation; it is defined as the mean difference divided by the pooled standard deviation of the treatment and control groups. 5 For a given study, Cohen’s d can be computed as: d = m T − m C s p o o l e d
Where m T and m C are the treatment and control means and spooled is essentially the square root of the weighted average of the treatment and control variances.
It has been shown that this estimate is biased in estimating the true population SMD, and the bias decreases as the sample size increases (small sample bias). 46 For this reason, Hedges g is more often used.
Hedges’ g
Hedges’ g is a transformation of Cohen’s d that attempts to adjust for small sample bias. The transformation involves multiplying Cohen’s d by a function of the total sample size.5 This generally results in a slight decrease in value of Hedges’ g compared with Cohen’s d, but the reduction lessens as the total sample size increases. The formula is: d ( 1 − 3 4 N − 9 )
Where N is the total trial sample size.
For very large sample sizes the two estimates will be very similar.
Back Transformation of Pooled SMD
One disadvantages of reporting standardized mean difference is that units of standard deviation are difficult to interpret clinically. Guidelines do exist but are often thought to be arbitrary and not applicable to all situations.47 An alternative is to back transform the pooled SMD into a scale used in the one of the analyses. In theory, by multiplying the SMD (and its upper and lower confidence bounds) by the standard deviation of the original scale, one can obtain a pooled estimate in that original scale. The difficulty is that the true standard deviation is unknown and must be estimated from available data. Alternatives for estimation include using the standard deviation from the largest study or using a pooled estimate of the standard deviations across studies.5 One should include a sensitivity analysis and be transparent about the approach used.
Ratio of Means
Ratio of Means (RoM), also known as response ratio, has been presented as an alternative to the SMD when outcomes are reported in different non-convertible scales. As the name implies the RoM divides the treatment mean by the control mean rather than taking the difference between the two. The ratio can be interpreted as the percentage change in the mean value of the treatment group relative to the control group. By meta-analyzing across studies we are making the assumption that the relative change will be homogeneous across all studies, regardless of which scale was used to measure it. Similar to the risk ratio and odds ratio, the RoM is pooled on the log scale; computational formulas are readily available. 5
For the RoM to have any clinical meaning, it is required that in the scale being used, the values are always positive (or always negative) and that a value of “zero” truly means zero. For example, if the outcome were patient temperature, RoM would be a poor choice since a temperature of 0 degrees does not truly represent what we would think of as zero.
2.4. Special Topics
Crossover trials.
A crossover trial is one where all patients receive, in sequence, both the treatment and control interventions. This results in the final data having the same group of patients represented with both their outcome values while in the treatment and control groups. When computing the standard error of the mean difference of a crossover trial, one must consider the correlation between the two groups—a result of the two measurements on different within-person treatments. 5 For most variables, the correlation will be positive, resulting in a smaller standard error than would be seen with the same values in a parallel trial.
To compute the correct pooled standard error requires an estimate of the correlation between the two groups. If correlation is available, the pooled standard error can be computed using the following formula: S E P = S E T 2 + S E C 2 + 2 r S E T S E C
Where r is the within-patient correlation and SE P , SE T , and SE C are the pooled, treatment, and control standard errors respectively
Most studies do not give the correlation or enough information to compute it, and thus it often has to be estimated based on investigator knowledge or imputed. 5 An imputation of 0.5 has been suggested as a good conservative estimate of correlation in the absence of any other information. 48
If a cross-over study reports its data by period, investigators have sometimes used first period data only when including cross-over trials in their meta-analyses—essentially treating the study as if it were a parallel design. This eliminates correlation issues, but has the disadvantage of omitting half the data from the trial.
Cluster Randomized Trials
Cluster trials occur when patients are randomized to treatment and control in groups (or clusters) rather than individually. If the units/subjects within clusters are positively correlated (as they usually are), then there is a loss of precision compared to a standard (non-clustered) parallel design of the same size. The design effect (DE) of a cluster randomized trial is the multiplicative multiplier needed to adjust the standard error computed as if the trial were a standard parallel design. Reported results from cluster trials may not reflect the design effect, and thus it will need to be computed by the investigator. The formula for computing the design effect is: D E = 1 + ( M − 1 ) I C C
Where M is the average cluster size and ICC is the intra-class correlation coefficient (see below).
Computation of the design effect involves a quantity known as the intra-class correlation coefficient (ICC), which is defined as the proportion of the total variance (i.e., within cluster variance plus between cluster variance) that is due to between cluster variance. 5 ICC’s are often not reported by cluster trials and thus a value must be obtained from external literature or a plausible value must be assumed by the investigator.
Mean Difference and Baseline Imbalance
- Use followup data.
- Use change from baseline data.
- Use an ANCOVA model that adjusts for the effects of baseline imbalance. 49
As long as trials are balanced at baseline, all three methods will give similar unbiased estimates of mean difference. 5 When baseline imbalance is present, it can be shown that using ANCOVA will give the best estimate of the true mean difference; however the parameters required to perform this analysis (mean and standard deviations of baseline, follow-up and change from baseline values) are usually not provided by the study authors. 50 If it is not feasible to perform an ANCOVA analysis, the choice of whether to use follow up or change from baseline values depends on the amount of correlation between baseline and final values. If the correlation is less than or equal to 0.5, then using the follow up values will be less biased (with respect to the estimate in the ANCOVA model) than using the change from baseline values. If the correlation is greater than 0.5, then change from baseline values will be less biased than using the follow up values. 51 There is evidence that these correlations are more often greater than 0.5, so the change from baseline means will usually be preferred if estimates of correlation are totally unobtainable. 52 A recent study 51 showed that all approaches were unbiased when there were both few trials and small sample sizes within the trials.
- The analyst should consider carefully which binary measure to analyze.
- If conversion to NNT or NNH is sought, then the risk difference is the preferred measure.
- The risk ratio and odds ratio are likely to be more consistent than the risk difference when the studies differ in baseline risk.
- The risk difference is not the preferred measure when the event is rare.
- The risk ratio is not the preferred measure if switching between occurrence and non occurrence of the event is important to the meta-analysis.
- The odds ratio can be misleading.
- The mean difference is the preferred measure when studies use the same metric.
- When calculating standardized mean difference, Hedges’ g is preferred over Cohen’s d due to the reduction in bias.
- If baseline values are unbalanced, one should perform an ANCOVA analysis. If ANCOVA cannot be performed and the correlation is greater than 0.5, change from baseline values should be used to compute the mean difference. If the correlation less than or equal to 0.5, follow-up values should be used.
- Data from clustered randomized trials should be adjusted for the design effect.
Chapter 3. Choice of Statistical Model for Combining Studies
3.1. introduction.
Meta-analysis can be performed using either a fixed or a random effects model to provide a combined estimate of effect size. A fixed effects model assumes that there is one single treatment effect across studies and any differences between observed effect sizes are due to sampling error. Under a random effects model, the treatment effects across studies are assumed to vary from study and study and follow a random distribution. The differences between observed effect sizes are not only due to sampling error, but also to variation in the true treatment effects. A random effects model usually assumes that the treatment effects across studies follow a normal distribution, though the validity of this assumption may be difficult to verify, especially when the number of studies is small. Alternative distributions 53 or distribution free models 54 , 55 have also been proposed.
Recent advances in meta-analysis include the development of alternative models to the fixed or random effects models. For example, Doi et al. proposed an inverse variance heterogeneity model (the IVhet model) for the meta-analysis of heterogeneous clinical trials that uses an estimator under the fixed effect model assumption with a quasi-likelihood based variance structure. 56 Stanley and Doucouliagosb proposed an unrestricted weighted least squares (WLS) estimator with multiplicative error for meta-analysis and claimed superiority to both conventional fixed and random effects, 57 though Mawdsley et al. 58 found modest differences when compared with the random effects model. These methods have not been fully compared with the many estimators developed within the framework of the fixed and random effects models and are not readily available in most statistical packages; thus they will not be further considered here.
General Considerations for Model Choice
Considerations for model choice include but are not limited to heterogeneity across treatment effects, the number and size of included studies, the type of outcomes, and potential bias. We recommend against choosing a statistical model based on the significance level of a heterogeneity test, for example, picking a fixed effects model when the p-value for the test of heterogeneity is more than 0.10 and a random effects model when P < 0.10, since such an approach does not take the many factors for model choice into full consideration.
In practice, clinical and methodological heterogeneity are always present across a set of included studies. Variation among studies is inevitable whether or not the test of heterogeneity detects it. Therefore, we recommend random effects models, with special considerations for rare binary outcomes (discussed below in the section on combining rare binary outcomes). For a binary outcome, when the estimate of between-study heterogeneity is zero, a fixed effects model (e.g., the Mantel-Haenszel method, inverse variance method, Peto method (for OR), or fixed effects logistic regression) provides an effect estimate similar to that produced by a random effects model. The Peto method requires that no substantial imbalance exists between treatment and control group sizes within trials and treatment effects are not exceptionally large.
When a systematic review includes both small and large studies and the results of small studies are systematically different from those of the large ones, publication bias may be present and the assumption of a random distribution of effect sizes, in particular, a normal distribution, is not justified. In this case, neither the random effects model nor the fixed effects model provides an appropriate estimate and investigators may choose not to combine all studies. 10 Investigators can choose to combine only the large studies if they are well conducted with good quality and are expected to provide unbiased effect estimates. Other potential differences between small and large studies should also be examined.
Choice of Random Effects Model and Estimator
The most commonly used random effects model for combined effect estimates is based on an estimator developed by DerSimonian and Laird (DL) due to its simplicity and ease of implementation. 59 It is well recognized that the estimator does not adequately reflect the error associated with parameter estimation, in particular, when the number of studies is small, and between-study heterogeneity is high. 40 Refined estimators have been proposed by the original authors. 19 , 60 , 61 Other estimators have also been proposed to improve the DL estimator. Sidik and Jonkman (SJ) and Hartung and Knapp (HK) independently proposed a non-iterative variant of the DL estimator using the t-distribution and an adjusted confidence interval for the overall effect. 62 – 64 We refer to this as the HKSJ method. Biggerstaff–Tweedie (BT) proposed another variant of the DL method by incorporating error in the point estimate of between-study heterogeneity into the estimation of the overall effect. 65 There are also many other likelihood based estimators such as maximum likelihood estimate, restricted maximum likelihood estimate and profile likelihood (PL) methods, which better account for the uncertainty in the estimate of between-study variance. 19
Several simulation studies have been conducted to compare the performance of different estimators for combined effect size. 19 – 21 , 66 , 67 For example, Brockwell et al. showed the PL method provides an estimate with better coverage probability than the DL method. 19 Jackson et al. showed that with a small number of studies, the DL method did not provide adequate coverage probability, in particular, when there was moderate to large heterogeneity. 20 However, these results supported the usefulness of the DL method for larger samples. In contrast, the PL estimates resulted in coverage probability closer to nominal values. IntHout et al. compared the performance of the DL and HKSJ methods and showed that the HKSJ method consistently resulted in more adequate error rates than did the DL method, especially when the number of studies was small, though they did not evaluate coverage probability and power. 67 Kontopantelis and Reeves conducted the most comprehensive simulation studies to compare the performance of nine different methods and evaluated multiple performance measures including coverage probability, power, and overall effect estimation (accuracy of point estimates and error intervals). 21 When the goal is to obtain an accurate estimate of overall effect size and the associated error interval, they recommended using the DL method when heterogeneity is low and using the PL method when heterogeneity is high, where the definition of high heterogeneity varies by the number of studies. The PL method overestimated coverage probability in the absence of between-study heterogeneity. Methods like BT and HKSJ, despite being developed to address the limitations of the DL method, were frequently outperformed by the DL method. Encouragingly, Kontopantelis and Reeves also showed that regardless of the estimation method, results are highly robust against even very severe violations of the assumption of normally distributed effect sizes.
Recently there has been a call to use alternative random-effects estimators to replace the universal use of the Dersimonian-Laird random effects model. 68 Based on the results from the simulation studies, the PL method appears to generally perform best, and provides best performance across more scenarios than other methods, though it may overestimate the confidence intervals in small studies with low heterogeneity. 21 It is appropriate to use the DL method when the heterogeneity is low. Another disadvantage of the PL method is that it does not always converge. In those situations, investigators may choose the DL method with sensitivity analyses using other methods, such as the HKSJ method. If non-convergence is due to high heterogeneity, investigators should also reevaluate the appropriateness of combining studies. The PL method (and the DL method) could be used to combine measures for continuous, count, and time to event data, as well as binary data when events are common. Note that the confidence interval produced by the PL method may not be symmetric. It is also worth noting that OR, RR, HR, and incidence rate ratio statistics should be analyzed on the logarithmic scale when the PL, DL, or HKSJ method is used. Finally, a Bayesian approach can also be used since this approach takes the variations in all parameters into account (see the section on Bayesian methods, below).
Role of Generalized Linear Mixed Effects Models
The different methods and estimators discussed above are generally used to combine effect measures directly (for example, mean difference, SMD, OR, RR, HR, and incidence rate ratio). For study-level aggregated binary data and count data, we also recommend the use of the generalized linear mixed effects model assuming random treatment effects. For aggregated binary data, a combined OR can be generated by assuming the binomial distribution with a logit link. It is also possible to generate a combined RR with the binomial distribution and a log link, though the model does not always converge. For aggregated count data, a combined rate ratio can be generated by assuming the Poisson distribution with a log link. Results from using the generalized linear models and directly combining effect measures are similar when the number of studies and/or the sample sizes are large.
3.2. A Special Case: Combining Rare Binary Outcomes
When combining rare binary outcomes (such as adverse event data), few or zero events often occur in one or both arms in some of the studies. In this case, the binomial distribution is not well-approximated by the normal approximation and choosing an appropriate model becomes complicated. The DL method does not perform well with low-event rate binary data. 43 , 69 A fixed effects model often out performs the DL method even in the presence of heterogeneity. 70 When event rates are less than 1 percent, the Peto OR method has been shown to provide the least biased, most powerful combined estimates with the best confidence interval coverage, 43 if the included studies have moderate effect sizes and the treatment and control group are of relatively similar sizes. The Peto method does not perform well when either the studies are unbalanced or the studies have large ORs (outside the range of 0.2-5). 71 , 72 Otherwise, when treatment and control group sizes are very different, effect sizes are large, or when events become more frequent (5 percent to 10 percent), the Mantel-Haenszel method (without a correction factor) or a fixed effects logistic regression provide better combined estimates.
Within the past few years, many methods have been proposed to analyze sparse data from simple averaging, 73 exact methods, 74 , 75 Bayesian approaches 76 , 77 to various parametric models (e.g., generalized linear mixed effect models, beta-binomial model, Gamma-Poisson model, bivariate Binomial-Normal model etc.). Two dominating opinions are to not use continuity corrections, and to include studies with zero events in both arms in the meta-analysis. Great efforts have been made to develop methods that can include such studies.
Bhaumik et al. proposed the simple (unweighted) average (SA) treatment affect with the 0.5 continuity correction, and found that the bias of the SA estimate in the presence of even significant heterogeneity is minimal compared with the bias of MH estimates (with 0.5 correction). 73 A simple average was also advocated by Shuster. 78 However, potential confounding remains an issue for an unweighted estimator. Spittal et al. showed that Poisson regression works better than the inverse variance method for rare events. 79 Kuss et al. conducted a comprehensive simulation of eleven methods, and recommended the use of the beta-binomial model for the three common effect measures (OR, RR, and RD) as the preferred meta-analysis methods for rare binary events with studies of zero events in one or both arms. 80 The beta-binomial model assumes that the observed events follow a binomial distribution and the binomial probabilities follow a beta distribution. In Kuss’s simulation, using a generalized linear model framework to model the treatment effect, an OR was estimated using a logit link, and an RR, using a log link. Instead of using an identity link, RD was estimated based on the estimated event probabilities from the logit model. This comprehensive simulation examined methods that could incorporate data from studies with zero events from both arms and do not need any continuity correction, and only compared the Peto and MH methods as reference methods.
Given the development of new methods that can handle studies with zero events in both arms, we advise that older methods that use continuity corrections be avoided. Investigators should use valid methods that include studies with zero events in one or both arms. For studies with zero events in one arm, or studies with sparse binary data but no zero events, an estimate can be obtained using the Peto method, the Mantel-Haenszel method, or a logistic regression approach, without adding a correction factor, when the between-study heterogeneity is small. These methods are simple to use and more readily available in standard statistical packages. When the between-study heterogeneity is large and/or there are studies with zero events in both arms, the more recently developed methods, such as beta-binomial model, could be explored and used. However, investigators should note that no method gives completely unbiased estimates when events are rare. Statistical methods can never completely solve the issue of sparse data. Investigators should always conduct sensitivity analyses 81 using alternative methods to check the robustness of results to different methods, and acknowledge the inadequacy of data sources when presenting the meta-analysis results, in particular, when the proportion of studies with zero events in both arms are high. If double-zero studies are to be excluded, they should be qualitatively summarized, by providing information on the confidence intervals for the proportion of events in each arm.
A Note on an Exact Method for Sparse Binary Data
For rare binary events, the normal approximation and asymptotic theory for large sample size does not work satisfactorily and exact inference has been developed to overcome these limitations. Exact methods do not need continuity corrections. However, simulation analyses do not identify a clear advantage of early developed exact methods 75 , 82 over a logistic regression or the Mantel-Haenszel method even in situations where these exact methods would theoretically be advantageous. 43 Recent developments of exact methods include Tian et al.’s method of combining confidence intervals 83 and Liu et al.’s method of combining p-value functions. 84 Yang et al. 85 developed a general framework for meta-analysis of rare events by combining confidence distributions (CDs), and showed that Tian’s and Liu’s methods could be unified under the CD framework. Liu showed that exact methods performed better than the Peto method (except when studies are unbalanced) and the Mantel-Haenszel method, 84 though the comparative performance of these methods has not been thoroughly evaluated. Investigators may choose to use exact methods with considerations for the interpretation of effect measures, but we do not specifically recommend exact methods over other models discussed above.
3.3. Bayesian Methods
A Bayesian framework provides a unified and comprehensive approach to meta-analysis that accommodates a wide variety of outcomes, often, using generalized linear model (GLM) with normal, binomial, Poisson and multinomial likelihoods and various link functions. 86
It should be noted that while these GLM models are routinely implemented in the frequentist framework, and are not specific to the Bayesian framework, extensions to more complex situations are most approachable using the Bayesian framework, for example, allowing for mixed treatment comparisons involving repeated measurements of a continuous outcome that varies over time. 87
There are several specific advantages inherent to the Bayesian framework. First, the Bayesian posterior parameter distributions fully incorporate the uncertainty of all parameters. These posterior distributions need not be assumed to be normal. 88 In random-effects meta-analysis, standard methods use only the most likely value of the between-study variance, 59 rather than incorporating the full uncertainty of each parameter. Thus, Bayesian credible intervals will tend to be wider than confidence intervals produced by some classical random-effects analysis such as the DL method. 89 However, when the number of studies is small, the between-study variance will be poorly estimated by both frequentist and Bayesian methods, and the use of vague priors can lead to a marked variation in results, 90 particularly when the model is used to predict the treatment effect in a future study. 91 A natural alternative is to use an informative prior distribution, based on observed heterogeneity variances in other, similar meta-analyses. 92 – 94
Full posterior distributions can provide a more informative summary of the likely value of parameters than the frequentist approach. When communicating results of meta-analysis to clinicians, the Bayesian framework allows direct probability statements to be made and provides the rank probability that a given treatment is best, second best, or worst (see the section on interpreting ranking probabilities and clinically important results in Chapter 5 below). Another advantage is that posterior distributions of functions of model parameters can be easily obtained such as the NNT. 86 Finally, the Bayesian approach allows full incorporation of parameter uncertainty from meta-analysis into decision analyses. 95
Until recently, Bayesian meta-analysis required specialized software such as WinBUGS, 96 OpenBUGS, 97 and JAGS. 98 , 99 Newer open source software platforms such as Stan 100 and Nimble 101 , 102 provide additional functionality and use BUGS-like modeling languages. In addition, there are user written commands that allow data processing in a familiar environment which then can be passed to WinBUGS, or JAGS for model fitting. 103 For example, in R, the package bmeta currently generates JAGS code to implement 22 models. 104 The R package gemtc similarly automates generation of JAGS code and facilitates assessment of model convergence and inconsistency. 105 , 106 On the other hand, Bayesian meta-analysis can be implemented in commonly used statistical packages. For example, SAS PROC MCMC can now implement at least some Bayesian hierarchical models 107 directly, as can Stata, version 14, via the bayesmh command. 108
When vague prior distributions are used, Bayesian estimates are usually similar to estimates obtained from the above frequentist methods. 90 Use of informative priors requires considerations to avoid undue influence on the posterior estimates. Investigators should provide adequate justifications for the choice of priors and conduct sensitivity analyses. Bayesian methods currently require more work in programming, MCMC simulation and convergence diagnostics.
A Note on Using a Bayesian Approach for Sparse Binary Data
It has been suggested that using a Bayesian approach might be a valuable alternative for sparse event data since Bayesian inference does not depend on asymptotic theory and takes into account all uncertainty in the model parameters. 109 The Bayesian fixed effects model provides good estimates when events are rare for binary data. 70 However, the choice of prior distribution, even when non-informative, may impact results, in particular, when a large proportion of studies have zero events in one or two arms. 80 , 90 , 110 Nevertheless, other simulation studies found that when the overall baseline rate is very small and there is moderate or large heterogeneity, Bayesian hierarchical random effect models can provide less biased estimates for the effect measures and the heterogeneity parameters. 77 To reduce the impact of the prior distributions, objective Bayesian methods have been developed 76 , 111 with special attention paid to the coherence between the prior distributions of the study model parameters and the meta-parameter, 76 though the Bayesian model was developed outside the usual hierarchical normal random effects framework. Further evaluations of these methods are required before recommendations of these objective Bayesian methods might be made.
3.4. Recommendations
- The PL method appears to generally perform best. The DL method is also appropriate when the between-study heterogeneity is low.
- For study-level aggregated binary data and count data, the use of a generalized linear mixed effects model assuming random treatment effects is also recommended.
- Methods that use continuity corrections should be avoided.
- For studies with zero events in one arm, or studies with sparse binary data but no zero events, an estimate can be obtained using the Peto method, the Mantel-Haenszel method, or a logistic regression approach, without adding a correction factor, when the between-study heterogeneity is low.
- When the between-study heterogeneity is high, and/or there are studies with zero events in both arms, more recently developed methods such as a beta-binomial model could be explored and used.
- Sensitivity analyses should be conducted with acknowledgement of the inadequacy of data.
- If investigators choose Bayesian methods, use of vague priors is supported.
Chapter 4. Quantifying, Testing, and Exploring Statistical Heterogeneity
4.1. statistical heterogeneity in meta-analysis.
Statistical heterogeneity was explained in general in Chapter 1 . In this chapter, we provide a deeper discussion from a methodological perspective. Statistical heterogeneity must be expected, quantified and sufficiently addressed in meta-analyses. 112 We recommend performing graphic and quantitative exploration of heterogeneity in combination. 113 In this chapter, it is assumed that a well-specified research question has been posed, the relevant literature has been reviewed, and a set of trials meeting selection criteria have been identified. Even when trial selection criteria are aimed toward identifying studies that are adequately homogenous, it is common for trials included in a meta-analysis to differ considerably as a function of (clinical and/or methodological) heterogeneity that was reviewed in Chapter 1 . Even when these sources of heterogeneity have been accounted for, statistical heterogeneity often remains. Statistical heterogeneity refers to the situation where estimates across studies have greater variability than expected from chance variation alone. 113 , 114
4.2. Visually Inspecting Heterogeneity
Although simple histograms, box plots, and other related graphical methods of depicting effect estimates across studies may be helpful preliminarily, these approaches do not necessarily provide insight into statistical heterogeneity. However, forest and funnel plots can be helpful in the interpretation of heterogeneity particularly when examined in combination with quantitative results. 113 , 115
Forest Plots
Forest plots can help identify potential sources and the extent of statistical heterogeneity. Meta-analyses with limited heterogeneity will produce forest plots with grossly visual overlap of study confidence intervals and the summary estimate. In contrast, a crude sign of statistical heterogeneity would be poor overlap. 115 An important recommendation is to graphically present between-study variance on forest plots of random effects meta-analyses using prediction intervals, which are on the same scale as the outcome. 93 The 95% prediction interval estimates where true effects would be expected for 95% of future studies. 93 When between-study variance is greater than zero, the prediction interval will cover a wider range than the confidence interval of the summary effect. 116 As proposed by Guddat et al. 117 and endorsed by IntHout et al., 116 including the prediction interval as a rectangle at the bottom of forest plots helps differentiate between-study variation from the confidence interval of the summary effect that is commonly depicted as a diamond.
Funnel Plots
Funnel plots are often thought of as representing bias, but they also can aid in detecting sources of heterogeneity. Funnel plots are essentially the plotting of effect sizes observed in each study (x-axis) around the summary effect size versus the degree of precision of each study (typically by standard error, variance, or precision on the y-axis). A meta-analysis that includes studies that estimate the same underlying effect across a range of precision, and has limited bias and heterogeneity would result in a funnel plot that resembles a symmetrical inverted funnel shape with increasing dispersion ranging with less precise (i.e., smaller) studies. 115 In the event of heterogeneity and/or bias, funnel plots will take on an asymmetric pattern around the summary effect size and also provide evidence of scatter outside the bounds of the 95% confidence limits. 115 Asymmetry in funnel plots can be difficult to detect visually, 118 and can be misleading due to multiple contributing factors. 113 , 119 , 120 Formal tests for funnel plot asymmetry (such as Egger’s test 15 for continuous outcomes, or the arcsine test proposed by Rucker et al., 27 for binary data) are available but should not be used with a meta-analysis involving fewer than 10 studies because of limited power. 113 Given the above cautions and considerations, funnel plots should only be used to complement other approaches in the preliminary analysis of heterogeneity.
4.3. Quantifying Heterogeneity
The null hypothesis of homogeneity in meta-analysis is that all studies are evaluating the same effect, 22 (i.e., all studies have the same true effect parameter that may or may not be equivalent to zero) and the alternative hypothesis is that at least one study has an effect that is different from the summary effect.
- Where Q is the heterogeneity statistic,
- w is the study weight based on inverse variance weighting,
- x is the observed effect size in each trial, and
- x ^ w is the summary estimate in a fixed-effect meta-analysis.
The Q statistic is assumed to have an approximate χ 2 distribution with k – 1 degrees of freedom. When Q is in excess over k – 1 and the associated p-value is low (typically, a p-value of <0.10 is used as a cut-off), the null hypothesis of homogeneity can be rejected. 22 , 122 Interpretation of a Q statistic in isolation is not advisable however, because it has low statistical power in meta-analyses involving a limited number of studies 123 , 124 and may detect unimportant heterogeneity when the number of studies included in a meta-analysis is large. Importantly, since heterogeneity is expected in meta-analyses even without statistical tests to support that claim, non-significant Q statistics must not be interpreted as the absence of heterogeneity. Moreover, the interpretation of Q in meta-analyses is more complicated than typically represented, because the actual distribution of Q is dependent on the measure of effect 125 and only approximately χ 2 in large samples. 122 Even if the null distribution of Q were χ 2 , universally interpreting all values of Q greater than the mean of k − 1 as indicating heterogeneity would be an oversimplification. 122 There are expansions to approximate Q for meta-analyses of standardized mean difference, 125 risk difference, 125 and odds ratios 126 that should be used as alternatives to Q , particularly when sample sizes of studies included in a meta-analysis are small. 122 The Q statistic and expansions thereof must be interpreted along with other heterogeneity statistics and with full consideration of their limitations.
Graphical Options for Examining Contributions to Q
Hardy and Thompson proposed using probability plots to investigate the contribution that each study makes to Q . 127 When each study is labeled, those deviating from the normal distribution in a probability plot have the greatest influence on Q . 127 Baujat and colleagues proposed another graphical method to identify studies that have the greatest impact on Q . 128 Baujat proposed plotting the contribution to the heterogeneity statistic for each study on the horizontal axis, and the squared difference between meta-analytic estimates with and without the i th study divided by the estimated variance of the meta-analytic estimate without the i th study along the vertical axis. Because of the Baujat plot presentation, studies that have the greatest influence on Q are located in the upper right corner for easy visual identification. Smaller studies have been shown to contribute more to heterogeneity than larger studies, 129 which would be visually apparent in Baujat plots. We recommend using these graphical approaches only when there is significant heterogeneity, and only when it is important to identify specific studies that are contributing to heterogeneity.
Between-Study Variance
- Where τ 2 is the parameter of between-study variance of the true effects,
- DL is the DerSimonian and Laird approach to τ 2 ,
- Q is the heterogeneity statistic (as above),
- k -1 is the degrees of freedom, and
- w is the weight applied to each study based on inverse variance weighting.
Since variance cannot be less than zero, a τ 2 less than zero is set to zero. The value of τ 2 is integrated into the weights of random-effects meta-analysis as presented in Chapter 3 . Since the DerSimonian and Laird approach to τ 2 is derived in part from Q , the problems with Q described above apply to the τ 2 parameter. 122 There are many alternatives to DerSimonian and Laird when estimating between-study variance. In a recent simulation, Veroniki and colleagues 121 compared 16 estimators of between-study variance; they argued that the Paule and Mandel 130 method of estimating between-study variance is a better alternative to the DerSimonian and Laird parameter for continuous and binary data because it less biased (i.e., yields larger estimates) when between-study variance is moderate-to-large. 121 At the time of this guidance, the Paule and Mandel method of estimating between-study variance is only provisionally recommended as an alternative to DerSimonian and Laird. 129 , 131 Moreover, Veroniki and colleagues provided evidence that the restrictive maximum likelihood estimator 132 is a better alternative to the DerSimonian and Laird parameter of between-study variance for continuous data because it yields similar values for low-to-moderate between-study variance and larger estimates in conditions of high between-study variance. 121
Inconsistency Across Studies
Another statistic that should be generated and interpreted even when Q is not statistically significant is the proportion of variability in effect sizes across studies that is explained by heterogeneity vs. random error or I 2 that is related to Q . 22 , 133
- Where Q is the estimate of between-study variance, and
- k −1 is the degrees of freedom.
- Where τ 2 is the parameter of between-study variance, and
- σ 2 is the within-study variance.
I 2 is a metric of how much heterogeneity is influencing the meta-analysis. With a range from 0% (indicating no heterogeneity) to 100% (indicating that all of the observed variance is attributable to heterogeneity), the I 2 statistic has several advantages over other heterogeneity statistics including its relative simplicity as a signal-to-noise ratio, and focus on how heterogeneity may be influencing interpretation of the meta-analysis. 59 It is important to note that I 2 increases with increasing study precision and hence is dependent on sample size. 27 By various means, confidence/uncertainty intervals can be estimated for I 2 including Higgins’ test-based method. 22 , 23 the assumptions involved in the construction of 95% confidence intervals cannot be justified in all cases, but I 2 confidence intervals based on frequentist assumptions generally provide sufficient coverage of uncertainty in meta-analyses. 133 In small meta-analyses, it has even been proposed that confidence intervals supplement or replace biased point estimates of I 2 . 26 It is important to note that since I 2 is based on Q or τ 2 , any problems that influence Q or τ 2 (most notably the number of trials included in the meta-analysis) will also indirectly interfere with the computation of I 2 . It is also important to consider that I 2 also is dependent on which between-study variance estimator is used. For example, there is a high level of agreement comparing I 2 derived from DerSimonian and Laird vs. Paul and Mandel methods of estimating between-study variance. 131 In contrast, I 2 derived from other methods of estimating between-study variance have low levels of agreement. 131
Based primarily on the observed distributions of I 2 across meta-analyses, there are ranges that are commonly used to further categorize heterogeneity. That is, I 2 values of 25%, 50%, and 75% have been proposed as working definitions of what could be considered low, moderate, and high proportions, respectively, of variability in effect sizes across studies that is explained by heterogeneity. 59 Currently, the Cochrane manual also includes ranges for interpreting I 2 (0%-40% might not be important, 30%-60% may represent moderate heterogeneity, 50-90% may represent substantial heterogeneity and 75-100% may represent considerable heterogeneity). 10 Irrespective of which categorization of I 2 is used, this statistic must be interpreted with the understanding of several nuances, including issues related to a small number of studies (i.e., fewer than 10), 24 – 26 and inherent differences in I 2 comparing binary and continuous effect sizes. 28 , 29 Moreover, I 2 of zero is often misinterpreted in published reports as being synonymous with the absence of heterogeneity despite upper confidence interval limits that most often would exceed 33% when calculated. 134 Finally, a high I 2 does not necessarily mean that dispersion occurs across a wide range of effect sizes, and a low I 2 does not necessarily mean that dispersion occurs across a narrow range of effect sizes; the I 2 is a signal-to-noise metric, not a statistic about the magnitude of heterogeneity.
4.4. Exploring Heterogeneity
Meta-regression.
Meta-regression is a common approach employed to examine the degree to which study-level factors explain statistical heterogeneity. 135 Random effects meta-regression, as compared with fixed effect meta-regression, allows for residual heterogeneity (i.e., between-study variance that is not explained by study-level factors) to incorporated into the model. 136 Because of this feature, among other benefits described below and in Chapter 3 , random effects meta-regression is recommended over fixed effect meta-regression. 137 It is the default of several statistical packages to use a modified estimator of variance in random effects meta-regression that employs a t distribution in lieu of a standard normal distribution when calculating p-values and confidence intervals (i.e., the Knapp-Hartung modification). 138 This approach is recommended to help mitigate false-positive rates that are common in meta-regression. 137 Since the earliest papers on random effects meta-regression, there has been general caution about the inherent low statistical power in analyses when there are fewer than 10 studies for each study-level factor modelled. 136 Currently, the Cochrane manual recommends that there be at least 10 studies per characteristic modelled in meta-regression 10 over the enduring concern about inflated false-positive rates with too few studies. 137 Another consideration that is reasonable to endorse is adjusting the level of statistical significance to account for making multiple comparisons in cases where more than one characteristic is being investigated in meta-regression.
Beyond statistical considerations important in meta-regression, there are also several important conceptual considerations. First, study-level characteristics to be considered in meta-regression should be pre-specified, scientifically defensible and based on hypotheses. 8 , 10 This first consideration will allow investigators to focus on factors that are believed to modify the effect of intervention as opposed to clinically meaningless study-level characteristics. Arguably, it may not be possible to identify all study-level characteristics that may modify intervention effects. The focus of meta-regression should be on factors that are plausible. Second, meta-regression should be carried out under full consideration of ecological bias (i.e., the inherent problems associated with aggregating individual-level data). 139 As classic examples, the mean study age or the proportion of study participants who were female may result in different conclusions in meta-regression as opposed to how these modifying relationships functioned in each trial. 135
Multiple Meta-regression
It may be desirable to examine the influence of more than one study-level factor on the heterogeneity observed in meta-analyses. Recalling general cautions and specific recommendations about the inherent low statistical power in analyses wherein there are fewer than 10 studies for each study-level factors modelled, 10 , 136 , 137 multiple meta-regression (that is, a meta-regression model with more than one study-level factor included) should only be considered when study-level characteristics are pre-specified, scientifically defensible, and based on hypotheses, and when there are 10 or more studies for each study-level factor included in meta-regression.
Subgroup Analysis
Subgroup analysis is another common approach employed to examine the degree to which study-level factors explain statistical heterogeneity. Since subgroup analysis is a type of meta-regression that incorporates a categorical study-level factor as opposed to a continuous study-level factor, it is similarly important that the grouping of studies to be considered in subgroup analysis be pre-specified, scientifically defensible and based on hypotheses. 8 , 10 Like other forms of meta-regression, subgroup analyses have a high false-positive rate. 137 and may be misleading when few studies are included. There are two general approaches to handling subgroups in meta-analysis. First, a common use is to perform meta-analyses within subgroups without any statistical between-group comparisons. A central problem with this approach is the tendency to misinterpret results from within separate groups as being comparative. That is, identification of groups wherein there is a significant summary effect and/or limited heterogeneity and others wherein there is no significant summary effect and/or substantive heterogeneity does not necessarily indicate that the subgroup factor explains overall heterogeneity. 10 Second, it is recommended to incorporate the subgrouping factor into a meta-regression framework. 140 Doing so allows for quantification of both within and among subgroup heterogeneity as well as well as formal statistical testing that informs whether the summary estimates are different across subgroups. Moreover, subgroup analysis in a meta-regression framework will allow for formal testing of residual heterogeneity in a similar fashion to meta-regression using a continuous study-level factor.
Detecting Outlying Studies
Under consideration that removal of one or more studies from a meta-analysis may interject bias in the results, 10 identification of outlier studies may help build the evidence necessary to justify removal. Visual examination of forest, funnel, normal probability and Baujat plots (described in detail earlier in this chapter) alone may be helpful in identifying studies with inherent outlying characteristics. Additional procedures that may be helpful in interpreting the influence of single studies are quantifying the summary effect without each study (often called one study removed), and performing cumulative meta-analyses. One study removed procedures simply involve sequentially estimating the summary effect without each study to determine if single studies are having a large influence on model results. Using cumulative meta-analysis, 141 it is possible to graph the accumulation of evidence of trials reporting at treatment effect. Simply put, this approach integrates all information up to and including each trial into summary estimates. By looking at the graphical output (from Stata’s metacum command or the R metafor cumul() function), one can examine large shifts in the summary effect that may serve as evidence for study removal. Another benefit of cumulative meta-analysis is detecting shifts in practice (e.g., guideline changes, new treatment approval or discontinuation) that would foster subgroup analysis.
Viechtbauer and Chung proposed other methods that should be considered to help identify outliers. One option is to examine extensions of linear regression residual diagnostics by using studentized deleted residuals. 142 Other options are to examine the difference between the predicted average effect with and without each study (indicating by how many standard deviations the average effect changes) or to examine what effect the deletion of each study has on the fitted values of all studies simultaneously (in a metric similar to Cook’s distance). 142 Particularly in combination, there methods serve as diagnostics that are more formal than visual inspection and both one study removed and cumulative meta-analysis procedures.
4.5. Special Topics
Baseline risk (control-rate) meta-regression.
For studies with binary outcomes, the “control rate” refers to the proportion of subjects in the control group who experienced the event. The control rate can be viewed as a surrogate for covariate differences between studies because it is influenced by illness severity, concomitant treatment, duration of follow-up, and/or other factors that may differ across studies. 143 , 144 Groups of patients with higher underlying risk for poor outcomes may experience different benefits and/or harms from treatment compared with groups of patients who have lower underlying risk. 145 Hence, the control-rate can be used to test for interactions between underlying population risk at baseline and treatment benefit.
To examine for an interaction between underlying population risk and treatment benefit, we recommend a simplified approach. First, generate a scatter plot of treatment effect against control rate to visually assess whether there may be a relation between the two. Since the RD tends to be highly correlated with the control rate, 144 we recommend using an RR or OR when examining a treatment effect against the control rate in all steps. The purpose of generating a scatterplot is simply to give preliminary insight into how differences in baseline risk (control rate) may influence the amount of observed variability in effect sizes across studies. Second, use hierarchical meta-regression 144 or Bayesian meta-regression 146 models to formally test the interaction between underlying population risk and treatment benefit. Although a weighted regression has been proposed as an intermediary step between developing a scatter plot and meta-regression, this approach identifies a significant relation between control rate and treatment effect twice as often compared with more suitable approaches (above), 144 , 146 and a negative finding would likely need to be replicated using meta-regression. Hence, the simplified two-step approach may help streamline the process.
Multivariate Meta-analysis
There are both inherent benefits and disadvantages of using meta-analysis to examine multiple outcomes simultaneously (that is, “multivariate meta-analysis”), and much methodological work has been done in both frequentist and Bayesian frameworks in recent years. 147 – 156 . Some of these methods are readily available in statistical packages (for example, Stata mvmeta ).
One of the advantages of multivariate meta-analysis is being able to incorporate multiple outcomes into one model as opposed to the conduct of multiple univariate meta-analyses wherein the outcomes are handled as being independent. 150 Another advantage of multivariate meta-analysis is being able to gain insight into relationships among study outcomes. 150 , 157 An additional advantage of multivariate meta-analysis is that different clinical conclusions may be made; 150 it may be considered easier to present results from a single multivariate meta-analysis than from several univariate analyses that may make different assumptions. Further, multivariate methods may have the potential to reduce the impact of outcome reporting bias. 150 , 158 , 159
- the disconnect between how outcomes are handled within each trial (typically in a univariate fashion) compared with a multivariate meta-analysis;
- estimation difficulties particularly around correlations between outcomes (seldom reported; see Bland 160 for additional commentary);
- overcoming assumptions of normally-distributed random effects with joint outcomes (difficult to justify with joint distributions);
- marginal model improvement in the multivariate vs. univariate case (often not sufficient trade off in effort); and
- amplification of publication bias (e.g., secondary outcomes are not published as frequently). 150
Another potential challenge is the appropriate quantification of heterogeneity in multivariate meta-analysis; but, there are newer alternatives that seem to make this less of a concern. These methods include but are not limited to the multivariate H 2 statistic (the ratio of a generalization of Q and its degrees of freedom, with an accompanying generalization of I 2 ( I H 2 ) ). 163 Finally, limitations to existing software for broad implementation and access to multivariate meta-analysis has been a long-standing barrier to this approach. With currently available add-on or base statistical packages, however, multivariate meta-analysis can be more readily performed, 150 and emerging approaches to multivariate meta-analyses are available to be integrated into standard statistical output. 153 However, the gain in precision of parameter estimates is often modest, and the conclusions from the multivariate meta-analysis are often the same as those from the univariate meta-analysis for individual outcomes, 164 which may not justify the increased complexity and difficulty.
With the exception of diagnostic testing meta-analysis (which provides a natural situation to meta-analyze sensitivity and specificity simultaneously, but which is out of scope for this report) and network meta-analysis (a special case of multivariate meta-analysis with unique challenges, see Chapter 5 ), multivariate meta-analysis has not been widely used in practice. However, we are likely to see multivariate meta-analysis approaches become more accessible to stakeholders involved with systematic reviews. 160 In the interim, however, we do not recommend this approach be used routinely.
Dose-Response Meta-analysis
Considering different exposure or treatment levels has been a longstanding consideration in meta-analyses involving binary outcomes. 165 , 166 and new methods have been developed to extend this approach to differences in means. 167 Meta-regression is commonly employed to test the relationship between exposure or treatment level and the intervention effect (i.e., dose-response). The best-case scenario for testing dose-response using meta-regression is when there are several trials that compared the dose level versus control for each dosing level. That way, subgroup analysis can be performed to provide evidence of effect similarity within groups of study-by-dose in addition to a gradient of treatment effects across groups. 10 Although incorporating study-level average dose can be considered, it should only be conducted in circumstances where there was limited-to-no variation in dosing within intervention arms of the studies included. In many instances, exposure needs to be grouped for effective comparison (e.g., ever vs. never exposed), but doing so raises the issues of non-independence and covariance between estimates. 168 Hamling et al., developed a method of deriving relative effect and precision estimates for such alternative comparisons in meta-analysis that are more reasonable compared with methods that ignore interdependence of estimates by level. 168 In the case of trials involving differences in means, dose-response models are estimated within each study in a first stage and an overall curve is obtained by pooling study-specific dose-response coefficients in a second stage. 167 A key benefit to this emerging approach to differences in means is modeling non-linear dose-response curves in unspecified shapes (including the cubic spline described in the derivation study). 167 Considering the inherent low statistical power associated with meta-regression in general, results of dose-response meta-regression should generally not be used to indicate that a dose response does not exist. 10
- Statistical heterogeneity should be expected, visually inspected and quantified, and sufficiently addressed in all meta-analyses.
- Prediction intervals should be included in all forest plots.
- Investigators should be consider evaluating multiple metrics of heterogeneity, between-study variance, and inconsistency (i.e., Q , τ 2 and I 2 along with their respective confidence intervals when possible).
- A non-significant Q should not be interpreted as the absence of heterogeneity, and there are nuances to the interpretation of Q that carry over to the interpretation of τ 2 and I 2 .
- Random effects is the preferred method for meta-regression that should be used under consideration of low power associated with limited studies (i.e., <10 studies per study-level factor) and the potential for ecological bias.
- We recommend a simplified two-step approach to control-rate meta-regression that involves scatter plotting and then hierarchical or Bayesian meta-regression.
- Routine use of multivariate meta-analysis is not recommended.
Chapter 5. Network Meta-Analysis (Mixed Treatment Comparisons/Indirect Comparisons)
5.1. rationale and definition.
Decision makers, whether patients, providers or policymakers generally want head-to-head estimates of the comparative effectiveness of the different interventions from which they have to choose. However, head-to-head trials are relatively uncommon. The majority of trials compare active agents with placebo, which has left patients and clinicians unable to compare across treatment options with sufficient certainty.
Therefore, an approach has emerged to compare agents indirectly. If we know that intervention A is better than B by a certain amount, and we know how B compares with C; we can indirectly infer the magnitude of effect comparing A with C. Occasionally, a very limited number of head-to-head trials are available (i.e., there may be a small number of trials directly comparing A with C). Such trials will likely produce imprecise estimates due to the small sample size and number of events. In this case, the indirect comparisons of A with C can be pooled with the direct comparisons, to produce what is commonly called a network meta-analysis estimate (NMA). The rationale for producing such an aggregate estimate is to increase precision, and to utilize all the available evidence for decision making.
Frequently, more than two active interventions are available and stakeholders want to compare (rank) many interventions, creating a network of interventions with comparisons accounting for all the permutations of pairings within the network. The following guidance focuses on NMA of randomized controlled trials. NMA of nonrandomized studies is statistically possible; however, without randomization, NMA assumptions would likely not be satisfied and the results would not be reliable.
5.2. Assumptions
There are three key assumptions required for network meta-analysis to be valid:
I. Homogeneity of direct evidence
When important heterogeneity (unexplained differences in treatment effect) across trials is noted, confidence in a pooled estimate decreases. 169 This is true for any meta-analysis. In an NMA, direct evidence (within each pairwise comparison) should be sufficiently homogeneous. This can be evaluated using the standard methods for evaluating heterogeneity ( I 2 statistic, τ 2 , Cochran Q test, and visual inspection of forest plots for consistency of point estimates from individual trials and overlap of confidence intervals).
II. Transitivity, similarity or exchangeability
Patients enrolled in trials of different comparisons in a network need to be sufficiently similar in terms of the distribution of effect modifiers. In other words, patients should be similar to the extent that it is plausible that they were equally likely to have received any of the treatments in the network. 170 Similarly, active and placebo controlled interventions across trials need to be sufficiently similar in order to attribute the observed change in effect size to the change in interventions.
Transitivity cannot be assessed quantitatively. However, it can be evaluated conceptually. Researchers need to identify important effect modifiers in the network and assess whether differences reported by studies are large enough to affect the validity of the transitivity assumption.
III. Consistency (Between Direct and Indirect Evidence)
Comparing direct and indirect estimates in closed loops in a network demonstrates whether the network is consistent (previously called coherent). Important differences between direct and indirect evidence may invalidate combining them in a pooled NMA estimate.
Consistency refers to the agreement between indirect and direct comparison for the same treatment comparison. If a pooled effect size for a direct comparison is similar to the pooled effect size from indirect comparison, we say the network is consistent; otherwise, the network is inconsistent or incoherent. 171 , 172 Multiple causes have been proposed for inconsistency, such as differences in patients, treatments, settings, timing, and other factors.
Statistical models have been developed to assume consistency in the network (consistency models) or account for inconsistency between direct and indirect comparison (inconsistency models). Consistency is a key assumption/prerequisite for a valid network meta-analysis and should always be evaluated. If there is substantial inconsistency between direct and indirect evidence, a network meta-analysis should not be performed. Fortunately, inconsistency can be evaluated statistically.
5.3. Statistical Approaches
The simplest indirect comparison approach is to qualitatively compare the point estimates and the overlap of confidence intervals from two direct comparisons that use a common comparator. Two treatments are likely to have comparable effectiveness if their direct effects relative to a common comparator (e.g., placebo) have the same direction and magnitude, and if there is considerable overlap in their confidence intervals. However, such qualitative comparisons have to be interpreted cautiously because the degree to which confidence intervals overlap is not a reliable substitute for formal hypothesis testing. Formal testing methods adjust the comparison of the interventions by the results of their direct comparison with a common control group and at least partially preserve the advantages of randomization of the component trials. 173
Many statistical models for network meta-analysis have been developed and applied in the literature. These models range from simple indirect comparisons to more complex mixed effects and hierarchical models, developed in both Bayesian and frequentist frameworks, and using both contrast level and arm level data.
Simple Indirect Comparisons
Simple indirect comparisons apply when there is no closed loop in the evidence network. A closed loop means that each comparison in a particular loop has both direct and indirect evidence. At least three statistical methods are available to conduct simple indirect comparisons: (1) the adjusted indirect comparison method proposed by Bucher et al, 174 (2) logistic regression, and (3) random effects meta-regression.
When there are only two sets of trials, say, A vs. B and B vs. C, Bucher‘s method is sufficient to provide the indirect estimate of A vs. C as: log(OR AC )=log(OR AB )-log(OR BC ) and
Var(Log(OR AC )) = Var(Log(OR AB )) + Var(Log(OR BC )), where OR is the odds ratio. Bucher’s method is valid only under a normality assumption on the log scale.
Logistic regression uses arm-level dichotomous outcomes data and is limited to odds ratios as the measure of effect. By contrast, meta-regression and adjusted indirect comparisons typically use contrast-level data and can be extended to risk ratios, risk differences, mean difference and any other effect measures. Under ideal circumstances (i.e., no differences in prognostic factors exist among included studies), all three methods result in unbiased estimates of direct effects. 175 Meta-regression (as implemented in Stata, metareg ) and adjusted indirect comparisons are the most convenient approaches for comparing trials with two treatment arms. A simulation study supports the use of random effects for either of these approaches. 175
Mixed Effects and Hierarchical Models
More complex statistical models are required for more complex networks with closed loops where a treatment effect could be informed by both direct and indirect evidence. These models typically assume random treatment effects and take the complex data structure into account, and may be broadly categorized as mixed effects, or hierarchical models.
Frequentist Approach
Lumley proposed the term “network meta-analysis” and the first network meta-analysis model in the frequentist framework, and constructed a random-effects inconsistency model by incorporating sampling variability, heterogeneity, and inconsistency. 176 The inconsistency follows a common random-effects distribution with mean of 0. It can use arm-level and contrast-level data and can be easily implemented in statistical software, including R’s lme package. However, studies included in the meta-analysis cannot have more than two arms.
Further development of network meta-analysis models in the frequentist framework addressed how to handle multi-armed trials as well as new methods of assessing inconsistency. 171 , 177 – 179 Salanti et al. provided a general network meta-analysis formulation with either contrast-based data or arm-based data, and defined the inconsistency in a standard way as the difference between ‘direct’ evidence and ‘indirect’ evidence. 177 In contrast, White et al. and Higgins et al. proposed to use a treatment-by-design interaction to evaluate inconsistency of evidence, and developed consistency and inconsistency models based on contrast-based multivariate random effects meta-regression. 171 , 178 These models can be implemented using network , a suite of commands in Stata with input data being either arm-level or contrast level.
Bayesian Approach
Lu and Ades proposed the first Bayesian network meta-analysis model for multi-arm studies that included both direct and indirect evidence. 180 The treatment effects are represented by basic parameters and functional parameters. Basic parameters are effect parameters that are directly compared to the baseline treatment, and functional parameters are represented as functions of basic parameters. Evidence inconsistency is defined as a function of a functional parameter and at least two basic parameters. The Bayesian model has been extended to incorporate study-level covariates in an attempt to explain between-study heterogeneity and reduce inconsistency, 181 to allow for repeated measurements of a continuous endpoint that varies over time, 87 or to appraise novelty effects. 182 A Bayesian multinomial network meta-analysis model was also developed for unordered (nominal) categorical outcomes allowing for partially observed data in which exact event counts may not be known for each category. 183 Additionally, Dias et al. set out a generalized linear model framework for the synthesis of data from randomized controlled trials, which could be applied to binary outcomes, continuous outcomes, rate models, competing risks, or ordered category outcomes. 86
Commonly, a vague (flat) prior is chosen for the treatment effect and heterogeneity parameters in Bayesian network meta-analysis. A vague prior distribution for heterogeneity however may not be appropriate when the number of studies is small. 184 An informative prior for heterogeneity can be obtained from the empirically derived predictive distributions for the degree of heterogeneity as expected in various settings (depending on the outcomes assessed and comparisons made). 185 In the NMA framework, frequentist and Bayesian approaches often provide similar results; particularly because of the common practice to use non-informative priors in the Bayesian analysis. 186 – 188 Frequentist approaches, when implemented in a statistical package, are easily applied in real-life data analysis. Bayesian approaches are highly adaptable to complex evidence structures and provide a very flexible modeling framework, but need a better understanding of the model specification and specialized programing skills.
Arm-Based Versus Contrast-Based Models
It is important to differentiate arm-based/contrast-based models from arm-level/contrast-level data. Arm-level and contrast-level data describe how outcomes are reported in the original studies. Arm-level data represent raw data per study arm (e.g., the number of events from a trial per group); while contrast-level data show the difference in outcomes between arms in the form of absolute or relative effect size (e.g., mean difference or the odds ratio of events).
Contrast-based models resemble the traditional approaches used in meta-analysis of direct comparisons. Absolute or relative effect sizes and associated variances are first estimated (per study) and then pooled to produce an estimate of the treatment comparison. Contrast-based models preserve randomization and, largely, alleviate risk of observed and unobserved imbalance between arms within a study. They use effect sizes relative to the comparison group and reduce the variability of outcomes across studies. Contrast-based models are the dominant approach used in direct meta-analysis and network meta-analysis in current practice.
Arm-based models depend on directly combining the observed absolute effect size in individual arms across studies; thereby producing a pooled rate or mean of the outcome per arm. Estimates can be compared among arms to produce a comparative effect size. Arm-based models break randomization; therefore, the comparative estimate will likely be at an increased risk of bias. Following this approach, nonrandomized studies or even noncomparative studies can be included in the analysis. Multiple models have been proposed for the arm-based approach, especially in the Bayesian framework. 177 , 189 – 192 However, the validity of arm-based methods is under debate. 178 , 193 , 194
Assessing Consistency
Network meta-analysis generates results for all pairwise comparisons; however, consistency can only be evaluated when at least one closed loop exists in the network. In other words, the network must have at least one treatment comparison with direct evidence. Many statistical methods are available to assess consistency. 173 , 174 , 176 , 195 – 200
These methods can generally be categorized into two types: (1) an overall consistency measure for the whole network; and (2) a loop-based approach in which direct and indirect estimates are compared. In the following section, we will focus on a few widely used methods in the literature.
- Single Measure for Network Consistency : These approaches use a single measure that represents consistency for the whole network. Lumley assumes that, for each treatment comparison (with or without direct evidence), there is a different inconsistency factor; and the inconsistency factor varies for all treatment comparisons and follows a common random-effects distribution. The variance of the differences, ω, also called incoherence, measures the overall inconsistency of the network. 176 A ω above 0.25 suggests substantial inconsistency and in this case, network meta-analysis may be considered inappropriate. 201
- Global Wald Test : Another approach is to use global Wald test, which tests an inconsistency factor that follows a Χ 2 distribution under the null consistency assumption. 178 A p-value less than 0.10 can be used to determine statistical significance. Rejection of the null is evidence that the model is not consistent.
- Z-test : A simple z-test can be used to compare the difference of the pooled effect sizes between direct and indirect comparisons. 174 Benefits of this approach include simplicity, ease of application, and the ability to identify specific loops with large inconsistency. Limitations include the need for multiple correlated tests.
- Side-splitting: A “node” is a treatment and a “side” (or edge) is a comparison. Dias et al. suggests that each comparison can be assessed by comparing the difference of the pooled estimate from direct evidence to the pooled estimate without direct evidence. 196 Side-splitting (sometimes referred to as node-splitting) can be implemented using the Stata network sidesplit command or R gemtc package.
Several graphical tools have been developed to describe inconsistency. One is the inconsistency plot developed by Chaimani et al. 197 Similar to a forest plot, the inconsistency plot graphically presents an inconsistency factor (the absolute difference between the direct and indirect estimates) and related confidence interval for each of the triangular and quadratic loops in the network. The Stata ifplot command can be used for this purpose.
It is important to understand the limitations of these methods. Lack of statistical significance of an inconsistency test does not prove consistency in the network. Similar to Cochran’s Q test of heterogeneity testing in traditional meta-analysis (which is often underpowered), statistical tests for inconsistency in NMA are also commonly underpowered due to the limited number of studies in direct comparisons.
- Abandon NMA and only perform traditional meta-analysis;
- Present the results from inconsistency models (that incorporate inconsistency) and acknowledge the limited trustworthiness of the NMA estimates;
- Split the network to eliminate the inconsistent nodes;
- Attempt to explain the causes of inconsistency by conducting network meta-regression to test for possible covariates causing the inconsistency: and
- Use only direct estimates for the pairwise NMA comparisons that show inconsistency (i.e., use direct estimates for inconsistent comparisons and use NMA estimates for consistent comparisons).
5.4. Considerations of Model Choice and Software
Consideration of indirect evidence.
Empirical explorations suggest that direct and indirect comparisons often agree, 174 – 176 , 202 – 204 but with notable exceptions. 205 In principle, the validity of combining direct and indirect evidence relies on the transitivity assumption. However, in practice, trials can vary in numerous ways including population characteristics, interventions, and cointerventions, length of follow-up, loss to follow-up, study quality, etc. Given the limited information in many publications and the inclusion of multiple treatments, the validity of combining direct and indirect evidence is often unverifiable. The statistical methods to evaluate inconsistency generally have low power, and are confounded by the presence of statistical heterogeneity. They often fail to detect inconsistency in the evidence network.
Moreover, network meta-analysis, like all other meta-analytic approaches, constitutes an observational study, and residual confounding can always be present. Systematic differences in characteristics among trials in a network can bias network meta-analysis results. In addition, all other considerations for meta-analyses, such as the choice of effect measures or heterogeneity, also apply to network meta-analysis. Therefore, in general, investigators should compare competing interventions based on direct evidence from head-to-head RCTs whenever possible. When head-to-head RCT data are sparse or unavailable but indirect evidence is sufficient, investigators may consider incorporating indirect evidence and network meta-analysis as an additional analytical tool. If the investigators choose to ignore indirect evidence, they should explain why.
Choice of Method
Although the development of network meta-analysis models has exploded in the last 10 years, there has been no systematic evaluation of their comparative performance, and the validity of the model assumptions in practice is generally hard to verify.
Investigators may choose a frequentist or Bayesian mode of inference based on the research team expertise, the complexity of the evidence network, and/or the research question. If investigators believe that the use of prior information is needed and that the data are insufficient to capture all the information available, then they should use a Bayesian model. On the other hand, a frequentist model is appropriate if one wants inferences to be based only on the data that can be incorporated into a likelihood.
Whichever method the investigators choose, they should assess the consistency of the direct and indirect evidence, and the invariance of treatment effects across studies and the appropriateness of the chosen method on a case-by-case basis, paying special attention to comparability across different sets of trials. Investigators should explicitly state assumptions underlying indirect comparisons and conduct sensitivity analysis to check those assumptions. If the results are not robust, findings from indirect comparisons should be considered inconclusive. Interpretation of findings should explicitly address these limitations. Investigators should also note that simple adjusted indirect comparisons are generally underpowered, needing four times as many equally sized studies to achieve the same power as direct comparisons, and frequently lead to indeterminate results with wide confidence intervals. 174 , 175
When the evidence of a network of interventions is consistent, investigators can combine direct and indirect evidence using network meta-analysis models. Conversely, they should refrain from combining multiple sources of evidence from an inconsistent (i.e., incoherent) network where there are substantial differences between direct and indirect evidence that cannot be resolved by conditioning on the known covariates. Investigators should make efforts to explain the differences between direct and indirect evidence based upon study characteristics, though little guidance and consensus exists on how to interpret the results.
Lastly, the network geometry ( Figure 5.1 ) can also affect the choice of analysis method as demonstrated in Table 5.1 .
Common network geometry (simple indirect comparison, star, network with at least one closed loop).
Impact of network geometry on choice of analysis method.
Commonly Used Software
Many statistical packages are available to implement NMA. BUGS software (Bayesian inference Using Gibbs Sampling, WINBUGS, OPENBUGS) is a popular choice for conducting Bayesian NMA 206 that offers flexible model specification including NMA meta-regression. JAGS and STAN are alternative choices for Bayesian NMA. Stata provides user-written routines ( http://www.mtm.uoi.gr/index.php/stata-routines-for-network-meta-analysis ) that can be used to conduct frequentist NMA. In particular, the Stata command network is a suite of programs for importing data for network meta-analysis, running a contrast-based network meta-analysis, assessing inconsistency, and graphing the data and results. Further, in the R environment, three packages, gemtc ( http://cran.r-project.org/web/packages/gemtc/index.html ), pcnetmeta ( http://cran.r-project.org/web/packages/pcnetmeta/index.html ), and netmeta ( http://cran.r-project.org/web/packages/netmeta/index.html ), have been developed for Bayesian ( gemtc, pcnetmeta ) or frequestist ( netmeta ) NMA. The packages also include methods to assess heterogeneity and inconsistency, and data visualizations, and allow users to perform NMA with minimal programming. 207
5.5. Inference From Network Meta-analysis
Stakeholders (users of evidence) require a rating of the strength of a body of evidence. The strength of evidence demonstrates how much certainty we should have in the estimates.
The general framework for assessing the strength of evidence used by the EPC program is described elsewhere. However; for NMA, guidance is evolving and may require some additional computations; therefore, we briefly discuss the possible approaches to rating the strength of evidence. We also discuss inference from rankings and probabilities commonly presented with a network meta-analysis.
Approaches for Rating the Strength of Evidence
The original EPC and GRADE guidance was simple and involved rating down all evidence derived from indirect comparisons (or NMA with mostly indirect evidence) for indirectness. Therefore, following this original GRADE guidance, evidence derived from most NMAs would be rated to have moderate strength at best. 208 Subsequently, Salanti et al. evaluated the transitivity assumption and network inconsistency under the indirectness and inconsistency domains of GRADE respectively. They judged the risk of bias based on a ‘contribution matrix’ which gives the percentage contribution of each direct estimate to each network meta-analysis estimate. 209 A final global judgment of the strength of evidence is made for the overall rankings in a network.
More recently, GRADE published a new approach that is based on evaluating the strength of evidence for each comparison separately rather than making a judgment on the whole network. 210 The rationale for not making such an overarching judgment is that the strength of evidence (certainty in the estimates) is expected to be different for different comparisons. The approach requires presenting the three estimates for each comparison (direct, indirect, and network estimates), then rating the strength of evidence separately for each one.
In summary, researchers conducting NMA should present their best judgment on the strength of evidence to facilitate decision-making. Innovations and newer methodology are constantly evolving in this area.
Interpreting Ranking Probabilities and Clinical Importance of Results
Network meta-analysis results are commonly presented as probabilities of being most effective and as rankings of treatments. Results are also presented as the surface under the cumulative ranking curve (SUCRA). SUCRA is a simple transformation of the mean rank that is used to provide a hierarchy of the treatments accounting both for the location and the variance of all relative treatment effects. SUCRA would be 1 when a treatment is certain to be the best and 0 when a treatment is certain to be the worst. 211 Such presentations should be interpreted with caution since they can be quite misleading.
- Such estimates are usually very imprecise. An empirical evaluation of 58 NMAs showed that the median width of the 95% CIs of SUCRA estimates was 65% (the first quartile was 38%; the third quartile was 80%). In 28% of networks, there was a 50% or greater probability that the best-ranked treatment was actually not the best. No evidence showed a difference between the best-ranked intervention and the second or third best-ranked interventions in 90% and 71% of comparisons, respectively.
- When rankings suggest superiority of an agent over others, the absolute difference between this intervention and other active agents could be trivial. Converting the relative effect to an absolute effect is often needed to present results that are meaningful to clinical practice and relevant to decision making. 212 Such results can be presented for patient groups with varying baseline risks. The source of baseline risk can be obtained from observational studies judged to be most representative of the population of interest, from the average baseline risk of the control arms of the randomized trials included in meta-analysis, or from a risk stratification tool if one is known and commonly used in practice. 213
- Rankings hide the fact that each comparison may have its own risk of bias, limitations, and strength of evidence.
5.6. Presentation and Reporting
- Rationale for conducting an NMA, the mode of inference (e.g., Bayesian, Frequentist), and the model choice (random effects vs. fixed effects; consistency vs inconsistency model, common heterogeneity assumption, etc.);
- Software and syntax/commands used;
- Choice of priors for any Bayesian analyses;
- Graphical presentation of the network structure and geometry;
- Pairwise effect sizes to allow comparative effectiveness inference; and
- Assessment of the extent of consistency between the direct and indirect estimates.
- A network meta-analysis should always be based on a rigorous a rigorous systematic review.
- Homogeneity of direct evidence
- Transitivity, similarity, or exchangeability
- Consistency (between direct and indirect evidence)
- Investigators may choose a frequentist or Bayesian mode of inference based on the research team’s expertise, the complexity of the evidence network, and the research question.
- Evaluating inconsistency is a major and mandatory component of network meta-analysis.
- Evaluating inconsistency should not be only based on a conducting a global test. A loop-based approach can identify the comparisons that cause inconsistency.
- Inference based on the rankings and probabilities of treatments being most effective should be used cautiously. Rankings and probabilities can be misleading and should be interpreted based on the magnitude of pairwise effect sizes. Differences across interventions may not be clinically important despite such rankings.
- Future Research Suggestions
The following are suggestions for directions in future research for each of the topics by chapter.
Chapter 1. Decision To Combine Trials
- Guidance regarding the minimum number of trials one can validly pool at given levels of statistical heterogeneity
- Research on ratio of means—both clinical interpretability and mathematical consistency across studies compared with standardized mean difference
- Research on use of ANCOVA models for adjusting baseline imbalance
- Software packages that more easily enable use of different information
- Methods to handle zeros in the computation of binary outcomes
- Evidence on which metrics, and language used to describe these metrics, are most helpful in conveying meta-analysis results to multiple stakeholders
- Evaluate newly developed statistical models for combining typical effect measures (e.g., mean difference, OR, RR, and/or RD) and compare with current methods
- Heterogeneity statistics for meta-analyses involving a small number of studies
- Guidance on specification of hypotheses in meta-regression
- Guidance on reporting of relationships among study outcomes to facilitate multivariate meta-analysis
Chapter 5. Network Meta-analysis (Mixed Treatment Comparisons/Indirect Comparisons)
- Methods for combining individual patient data with aggregated data
- Methods for integrating evidence from RCTs and observational studies
- Models for time-to-event data
- User friendly software similar to that available for traditional meta-analysis
- Evidence to support model choice
This report is based on research conducted by the Agency for Healthcare Research and Quality (AHRQ) Evidence-based Practice Centers’ 2016 Methods Workgroup. The findings and conclusions in this document are those of the authors, who are responsible for its contents; the findings and conclusions do not necessarily represent the views of AHRQ. Therefore, no statement in this report should be construed as an official position of AHRQ or of the U.S. Department of Health and Human Services.
None of the investigators have any affiliations or financial involvement that conflicts with the material presented in this report.
This research was funded through contracts from the Agency for Healthcare Research and Quality to the following Evidence-based Practice Centers: Mayo Clinic (290-2015-00013-I); Kaiser Permanente (290-2015-00007-I); RAND Corporation (290-2015-00010-I); Alberta (290-2015-00001-I); Pacific Northwest (290-2015-00009-I); RTI (290-2015-00011-I); Brown (290-2015-00002-I); and the Scientific Resource Center (290-2012-00004-C).
The information in this report is intended to help health care decisionmakers—patients and clinicians, health system leaders, and policy makers, among others—make well-informed decisions and thereby improve the quality of health care services. This report is not intended to be a substitute for the application of clinical judgment. Anyone who makes decisions concerning the provision of clinical care should consider this report in the same way as any medical reference and in conjunction with all other pertinent information (i.e., in the context of available resources and circumstances presented by individual patients).
This report is made available to the public under the terms of a licensing agreement between the author and the Agency for Healthcare Research and Quality. This report may be used and reprinted without permission except those copyrighted materials that are clearly noted in the report. Further reproduction of those copyrighted materials is prohibited without the express permission of copyright holders.
AHRQ or U.S. Department of Health and Human Services endorsement of any derivative products that may be developed from this report, such as clinical practice guidelines, other quality enhancement tools, or reimbursement or coverage policies may not be stated or implied.
Persons using assistive technology may not be able to fully access information in this report. For assistance, contact vog.shh.qrha@cpe .
Suggested citation: Morton SC, Murad MH, O’Connor E, Lee CS, Booth M, Vandermeer BW, Snowden JM, D’Anci KE, Fu R, Gartlehner G, Wang Z, Steele DW. Quantitative Synthesis—An Update. Methods Guide for Comparative Effectiveness Reviews. (Prepared by the Scientific Resource Center under Contract No. 290-2012-0004-C). AHRQ Publication No. 18-EHC007-EF. Rockville, MD: Agency for Healthcare Research and Quality; February 2018. Posted final reports are located on the Effective Health Care Program search page . https://doi.org/ 10 .23970/AHRQEPCMETHGUIDE3 .
Prepared for: Agency for Healthcare Research and Quality, U.S. Department of Health and Human Services, 5600 Fishers Lane, Rockville, MD 20857, www.ahrq.gov Contract No.: 290-2012-00004-C . Prepared by: Scientific Resource Center, Portland, OR
- Cite this Page Morton SC, Murad MH, O’Connor E, et al. Quantitative Synthesis—An Update. 2018 Feb 23. In: Methods Guide for Effectiveness and Comparative Effectiveness Reviews [Internet]. Rockville (MD): Agency for Healthcare Research and Quality (US); 2008-.
- PDF version of this page (702K)
In this Page
- Decision to Combine Trials
- Optimizing Use of Effect Size Data
- Choice of Statistical Model for Combining Studies
- Quantifying, Testing, and Exploring Statistical Heterogeneity
- Network Meta-Analysis (Mixed Treatment Comparisons/Indirect Comparisons)
Other titles in these collections
- AHRQ Methods for Effective Health Care
- Health Services/Technology Assessment Text (HSTAT)
Related information
- PMC PubMed Central citations
- PubMed Links to PubMed
Similar articles in PubMed
- Folic acid supplementation and malaria susceptibility and severity among people taking antifolate antimalarial drugs in endemic areas. [Cochrane Database Syst Rev. 2022] Folic acid supplementation and malaria susceptibility and severity among people taking antifolate antimalarial drugs in endemic areas. Crider K, Williams J, Qi YP, Gutman J, Yeung L, Mai C, Finkelstain J, Mehta S, Pons-Duran C, Menéndez C, et al. Cochrane Database Syst Rev. 2022 Feb 1; 2(2022). Epub 2022 Feb 1.
- Review Conducting Quantitative Synthesis When Comparing Medical Interventions: AHRQ and the Effective Health Care Program. [Methods Guide for Effectivenes...] Review Conducting Quantitative Synthesis When Comparing Medical Interventions: AHRQ and the Effective Health Care Program. Fu R, Gartlehner G, Grant M, Shamliyan T, Sedrakyan A, Wilt TJ, Griffith L, Oremus M, Raina P, Ismaila A, et al. Methods Guide for Effectiveness and Comparative Effectiveness Reviews. 2008
- Conducting quantitative synthesis when comparing medical interventions: AHRQ and the Effective Health Care Program. [J Clin Epidemiol. 2011] Conducting quantitative synthesis when comparing medical interventions: AHRQ and the Effective Health Care Program. Fu R, Gartlehner G, Grant M, Shamliyan T, Sedrakyan A, Wilt TJ, Griffith L, Oremus M, Raina P, Ismaila A, et al. J Clin Epidemiol. 2011 Nov; 64(11):1187-97. Epub 2011 Apr 7.
- The future of Cochrane Neonatal. [Early Hum Dev. 2020] The future of Cochrane Neonatal. Soll RF, Ovelman C, McGuire W. Early Hum Dev. 2020 Nov; 150:105191. Epub 2020 Sep 12.
- Review Grading the Strength of a Body of Evidence When Assessing Health Care Interventions for the Effective Health Care Program of the Agency for Healthcare Research and Quality: An Update. [Methods Guide for Effectivenes...] Review Grading the Strength of a Body of Evidence When Assessing Health Care Interventions for the Effective Health Care Program of the Agency for Healthcare Research and Quality: An Update. Berkman ND, Lohr KN, Ansari M, McDonagh M, Balk E, Whitlock E, Reston J, Bass E, Butler M, Gartlehner G, et al. Methods Guide for Effectiveness and Comparative Effectiveness Reviews. 2008
Recent Activity
- Quantitative Synthesis—An Update - Methods Guide for Effectiveness and Comparati... Quantitative Synthesis—An Update - Methods Guide for Effectiveness and Comparative Effectiveness Reviews
Your browsing activity is empty.
Activity recording is turned off.
Turn recording back on
Connect with NLM
National Library of Medicine 8600 Rockville Pike Bethesda, MD 20894
Web Policies FOIA HHS Vulnerability Disclosure
Help Accessibility Careers

- University of Texas Libraries
- UT Libraries
Systematic Reviews & Evidence Synthesis Methods
- Types of Reviews
- Formulate Question
- Find Existing Reviews & Protocols
- Register a Protocol
- Searching Systematically
- Supplementary Searching
- Managing Results
- Deduplication
- Critical Appraisal
- Glossary of terms
- Librarian Support
- Video tutorials This link opens in a new window
- Systematic Review & Evidence Synthesis Boot Camp
Once you have completed your analysis, you will want to both summarize and synthesize those results. You may have a qualitative synthesis, a quantitative synthesis, or both.
Qualitative Synthesis
In a qualitative synthesis, you describe for readers how the pieces of your work fit together. You will summarize, compare, and contrast the characteristics and findings, exploring the relationships between them. Further, you will discuss the relevance and applicability of the evidence to your research question. You will also analyze the strengths and weaknesses of the body of evidence. Focus on where the gaps are in the evidence and provide recommendations for further research.
Quantitative Synthesis
Whether or not your Systematic Review includes a full meta-analysis, there is typically some element of data analysis. The quantitative synthesis combines and analyzes the evidence using statistical techniques. This includes comparing methodological similarities and differences and potentially the quality of the studies conducted.
Summarizing vs. Synthesizing
In a systematic review, researchers do more than summarize findings from identified articles. You will synthesize the information you want to include.
While a summary is a way of concisely relating important themes and elements from a larger work or works in a condensed form, a synthesis takes the information from a variety of works and combines them together to create something new.
Synthesis :
"The goal of a systematic synthesis of qualitative research is to integrate or compare the results across studies in order to increase understanding of a particular phenomenon, not to add studies together. Typically the aim is to identify broader themes or new theories – qualitative syntheses usually result in a narrative summary of cross-cutting or emerging themes or constructs, and/or conceptual models."
Denner, J., Marsh, E. & Campe, S. (2017). Approaches to reviewing research in education. In D. Wyse, N. Selwyn, & E. Smith (Eds.), The BERA/SAGE Handbook of educational research (Vol. 2, pp. 143-164). doi: 10.4135/9781473983953.n7
- Approaches to Reviewing Research in Education from Sage Knowledge
Data synthesis (Collaboration for Environmental Evidence Guidebook)
Interpreting findings and and reporting conduct (Collaboration for Environmental Evidence Guidebook)
Interpreting results and drawing conclusions (Cochrane Handbook, Chapter 15)
Guidance on the conduct of narrative synthesis in systematic reviews (ESRC Methods Programme)
- Last Updated: May 16, 2024 11:05 AM
- URL: https://guides.lib.utexas.edu/systematicreviews
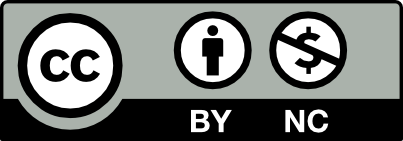
Design Thinking - Analysis Vs Synthesis
In this chapter, we will see the difference between two ways of solution-based thinking, i.e. Analysis and Synthesis, and also get to know how it helps in design thinking.
Analysis is derived from the Greek word ‘analusis’, which translates into ‘breaking up’ in English. Analysis is older than the times of great philosophers like Aristotle and Plato. As discussed in the previous section, analysis is the process of breaking down a big single entity into multiple fragments. It is a deduction where a bigger concept is broken down to smaller ones . This breaking down into smaller fragments is necessary for improved understanding.
So, how does analysis help in design thinking? During analysis, design thinkers are required to break down the problem statement into smaller parts and study each one of them separately. The different smaller components of the problem statement are to be solved one-by-one, if possible. Then, solutions are thought for each of the small problems. Brainstorming is done over each of the solutions.
Later, a feasibility check is done to include the feasible and viable solutions. The solutions that don’t stand firm on the grounds of feasibility and viability are excluded from the set of solutions to be considered.
Design thinkers are, then, encouraged to connect with the diverse ideas and examine the way each idea was composed. This process of breaking down the bigger problem statement at hand into multiple smaller problem statements and examining each as a separate entity is called analysis.
Reductionism
The underlying assumption in analysis is reductionism . Reductionism states that the reality around us can be reduced down to invisible parts. The embodiment of this principle is found in basic axioms of analytic geometry, which says “the whole is equal to the sum of its parts”. However, understanding of a system cannot be developed by analysis alone. Hence, synthesis is required following analysis.
Synthesis refers to the process of combining the fragmented parts into an aggregated whole. It is an activity that is done at the end of the scientific or creative inquiry. This process leads to creation of a coherent bigger entity, which is something new and fresh. How does synthesis come into picture in design thinking?
Once the design thinkers have excluded the non-feasible and non-viable solutions and have zeroed-in on the set of feasible and viable solutions, it is time for the thinkers to put together their solutions.
Out of 10 available solutions, around 2-3 solutions may need to be excluded since they may not fit into the larger picture, i.e. the actual solution. This is where synthesis helps.
The design thinkers start from a big entity called the problem statement and then end up with another bigger entity, i.e. the solution. The solution is completely different from the problem statement. During synthesis, it is ensured that the different ideas are in sync with each other and do not lead to conflicts.
Analysis + Synthesis = Design Thinking
Analysis and synthesis, thus, form the two fundamental tasks to be done in design thinking. Design thinking process starts with reductionism, where the problem statement is broken down into smaller fragments. Each fragment is brainstormed over by the team of thinkers, and the different smaller solutions are then put together to form a coherent final solution. Let us take a look at an example.
Problem Statement − Suppose the problem statement at hand is to contain the attrition that happens in companies worldwide. High quality employees leave the organization, mainly after the appraisal cycle. As a result, an average company loses its valuable human resources and suffers from an overhead of transferring the knowledge to a new employee. This takes time and additional human resource in the form of a trainer, which adds to the company’s costs. Devise a plan to contain attrition in the company.
Analysis − Now, let’s break down the problem statement into various constituent parts. Following are the subparts of the same problem statement, broken down to elementary levels.
- The employees are not motivated anymore to work in the company.
- Appraisal cycle has something to do with attrition.
- Knowledge transfer is necessary for new employees.
- Knowledge transfer adds to the cost of the company.
Synthesis − Now, let's start solving each problem individually. In this step, we will do synthesis. Let's look at one problem at a time and try to find a solution only for that problem statement, without thinking of other problem statements.
To solve the problem of lack of motivation, the management can plan some sort of incentives that can be given on a regular basis. The efforts put in by the employees must be rewarded well. This will keep the employees motivated.
To solve the issue of occurrence of attrition during appraisal cycle, the management can conduct a meeting with the employees leaving the organization, and take their insight as to what led them to leave the company.
For knowledge transfer, the management can hire only those people who are experts in a domain.
Regarding concerns for budget of knowledge transfer, the management can have a document prepared by experts in a domain and this document can be uploaded on intranet. This can be made available to new joinees. Hence, additional human resource is not required for knowledge transfer and this will reduce the figures in the company's budget.
Now, if we observe carefully, the third solution may not be feasible all the time. We cannot be assured of expert professionals coming for interviews all the time. Moreover, expert professionals demand more compensation than not-so-expert professionals. This will increase the company's budget.
Hence, we will now combine the other three solutions to form a coherent one. The final solution will be for the management to first have a talk with the employees leaving the organization to know the reasons behind attrition, then come up with awards in suitable categories and then, create an easily and universally accessible document in the organization for knowledge transfer.
This way, analysis and synthesis together help in design thinking process. Design thinkers start with breaking down a problem into smaller problems that can be handled and studied easily. Then, the different solutions are combined to form a coherent single solution.
To Continue Learning Please Login

Want to create or adapt books like this? Learn more about how Pressbooks supports open publishing practices.
Chapter Six: Analysis and Synthesis
What does it mean to know something? How would you explain the process of thinking? In the 1950s, educational theorist Benjamin Bloom proposed that human cognition, thinking and knowing, could be classified by six categories. 1 Hierarchically arranged in order of complexity, these steps were:
Since his original model, the taxonomy has been revised, as illustrated in the diagram below:
- Each word is an action verb instead of a noun (e.g., “applying” instead of “application”);
- Some words have been changed for different synonyms;
- One version holds “creating” above “evaluating”;
- And, most importantly, other versions are reshaped into a circle, as pictured above. 2
What do you think the significance of these changes is?
I introduce this model of cognition to contextualize analysis as a cognitive tool which can work in tandem with other cognitive tasks and behaviors. Analysis is most commonly used alongside synthesis . To proceed with the LEGO® example from Chapter 4, consider my taking apart the castle as an act of analysis. I study each face of each block intently, even those parts that I can’t see when the castle is fully constructed. In the process of synthesis, I bring together certain blocks from the castle to instead build something else—let’s say, a racecar. By unpacking and interpreting each part , I’m able to build a new whole . 3
In a text wrestling essay, you’re engaging in a process very similar to my castle-to-racecar adventure. You’ll encounter a text and unpack it attentively, looking closely at each piece of language, its arrangement, its signification, and then use it to build an insightful, critical insight about the original text. I might not use every original block, but by exploring the relationship of part-to-whole, I better understand how the castle is a castle. In turn, I am better positioned to act as a sort of tour guide for the castle or a mechanic for the racecar, able to show my readers what about the castle or racecar is important and to explain how it works.
In this chapter, you’ll learn about crafting a thesis for a text wrestling essay and using evidence to support that thesis . As you will discover, an analytical essay involves every tier of Bloom’s Taxonomy, arguably even including “judgement” because your thesis will present an interpretation that is evidence-based and arguable.
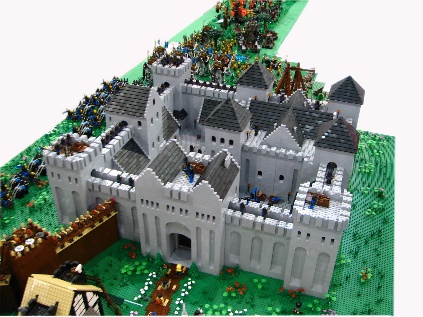
Chapter Vocabulary
So What? Turning Observations into a Thesis
It’s likely that you’ve heard the term “thesis statement” multiple times in your writing career. Even though you may have some idea what a thesis entails already, it is worth reviewing and unpacking the expectations surrounding a thesis, specifically in a text wrestling essay.
A thesis statement is a central, unifying insight that drives your analysis or argument. In a typical college essay, this insight should be articulated in one to three sentences, placed within the introductory paragraph or section. As we’ll see below, this is not always the case, but it is what many of your audiences will expect. To put it simply, a thesis is the “So what?” of an analytical or persuasive essay. It answers your audience when they ask, Why does your writing matter? What bigger insights does it yield about the subject of analysis? About our world?
Thesis statements in most rhetorical situations advocate for a certain vision of a text, phenomenon, reality, or policy. Good thesis statements support such a vision using evidence and thinking that confirms, clarifies, demonstrates, nuances, or otherwise relates to that vision. In other words, a thesis is “a proposition that you can prove with evidence…, yet it’s one you have to prove, that isn’t obviously true or merely factual.” 4
In a text wrestling analysis, a thesis pushes beyond basic summary and observation. In other words, it’s the difference between:
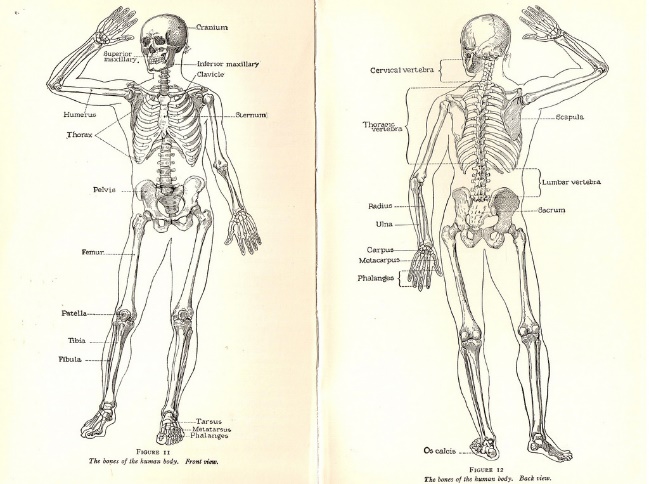
If you think of your essay as the human body, the thesis is the spine. Yes, the body can still exist without a spine, but its functionings will be severely limited. Furthermore, everything comes back to and radiates out from the spine: trace back from your fingertips to your backbone and consider how they relate. In turn, each paragraph should tie back to your thesis, offering support and clear connections so your reader can see the entire “body” of your essay. In this way, a thesis statement serves two purposes: it is not only about the ideas of your paper, but also the structure .
The Purdue Online Writing Lab (OWL) 5 suggests this specific process for developing your thesis statement:
- Once you’ve read the story or novel closely, look back over your notes for patterns of questions or ideas that interest you. Have most of your questions been about the characters, how they develop or change?
For example: If you are reading Conrad’s The Secret Agent , do you seem to be most interested in what the author has to say about society? Choose a pattern of ideas and express it in the form of a question and an answer such as the following:
Question: What does Conrad seem to be suggesting about early twentieth-century London society in his novel The Secret Agent ? Answer: Conrad suggests that all classes of society are corrupt.
Pitfalls: Choosing too many ideas. Choosing an idea without any support.
- Once you have some general points to focus on, write your possible ideas and answer the questions that they suggest.
For example: Question : How does Conrad develop the idea that all classes of society are corrupt? Answer: He uses images of beasts and cannibalism whether he’s describing socialites, policemen or secret agents.
- To write your thesis statement, all you have to do is turn the question and answer around. You’ve already given the answer, now just put it in a sentence (or a couple of sentences) so that the thesis of your paper is clear.
For example: In his novel, The Secret Agent , Conrad uses beast and cannibal imagery to describe the characters and their relationships to each other. This pattern of images suggests that Conrad saw corruption in every level of early twentieth-century London society.
- Now that you’re familiar with the story or novel and have developed a thesis statement, you’re ready to choose the evidence you’ll use to support your thesis. There are a lot of good ways to do this, but all of them depend on a strong thesis for their direction.
For example: Here’s a student’s thesis about Joseph Conrad’s The Secret Agent .
In his novel, The Secret Agent, Conrad uses beast and cannibal imagery to describe the characters and their relationships to each other. This pattern of images suggests that Conrad saw corruption in every level of early twentieth-century London society.
This thesis focuses on the idea of social corruption and the device of imagery. To support this thesis, you would need to find images of beasts and cannibalism within the text.
There are many ways to write a thesis, and your construction of a thesis statement will become more intuitive and nuanced as you become a more confident and competent writer. However, there are a few tried-and-true strategies that I’ll share with you over the next few pages.
The T3 Strategy
T3 is a formula to create a thesis statement. The T (for Thesis) should be the point you’re trying to make—the “So what?” In a text wrestling analysis, you are expected to advocate for a certain interpretation of a text: this is your “So what?” Examples might include:
In “A Wind from the North,” Bill Capossere conveys the loneliness of isolated life or Kate Chopin’s “The Story of an Hour” suggests that marriage can be oppressive to women
But wait—there’s more! In a text wrestling analysis, your interpretation must be based on evidence from that text. Therefore, your thesis should identify both a focused statement of the interpretation (the whole) and also the particular subjects of your observation (the parts of the text you will focus on support that interpretation). A complete T3 thesis statement for a text wrestling analysis might look more like this:
In “A Wind from the North,” Bill Capossere conveys the loneliness of an isolated lifestyle using the motif of snow, the repeated phrase “five or six days” (104), and the symbol of his uncle’s car. or “The Story of an Hour” suggests that marriage can be oppressive to women. To demonstrate this theme, Kate Chopin integrates irony, foreshadowing, and symbols of freedom in the story.
Notice the way the T3 allows for the part-to-whole thinking that underlies analysis:
This is also a useful strategy because it can provide structure for your paper: each justifying support for your thesis should be one section of your paper.
- Thesis: In “A Wind from the North,” Bill Capossere conveys the loneliness of an isolated lifestyle using the motif of snow, the repeated phrase “five or six days” (104), and the symbol of his uncle’s car.
- Section on ‘the motif of snow.’ Topic sentence: The recurring imagery of snow creates a tone of frostiness and demonstrates the passage of time.
- Section on ‘the repeated phrase “five or six days” (104).’ Topic sentence: When Capossere repeats “five or six days” (104), he reveals the ambiguity of death in a life not lived.
- Section on ‘the symbol of his uncle’s car.’ Topic sentence: Finally, Capossere’s uncle’s car is symbolic of his lifestyle.
Once you’ve developed a T3 statement, you can revise it to make it feel less formulaic. For example:
In “A Wind from the North,” Bill Capossere conveys the loneliness of an isolated lifestyle by symbolizing his uncle with a “untouchable” car. Additionally, he repeats images and phrases in the essay to reinforce his uncle’s isolation. or “The Story of an Hour,” a short story by Kate Chopin, uses a plot twist to imply that marriage can be oppressive to women. The symbols of freedom in the story create a feeling of joy, but the attentive reader will recognize the imminent irony.
The O/P Strategy
An occasion/position thesis statement is rhetorically convincing because it explains the relevance of your argument and concisely articulates that argument. Although you should already have your position in mind, your rhetorical occasion will lead this statement off: what sociohistorical conditions make your writing timely, relevant, applicable? Continuing with the previous examples:
As our society moves from individualism to isolationism, Bill Capossere’s “A Wind from the North” is a salient example of a life lived alone. or Although Chopin’s story was written over 100 years ago, it still provides insight to gender dynamics in American marriages.
Following your occasion, state your position—again, this is your “So What?” It is wise to include at least some preview of the parts you will be examining.
As our society moves from individualism to isolationism, Bill Capossere’s “A Wind from the North” is a salient example of a life lived alone. Using recurring images and phrases, Capossere conveys the loneliness of his uncle leading up to his death. or Although Chopin’s story was written over 100 years ago, it still provides insight to gender dynamics in American marriages. “The Story of an Hour” reminds us that marriage has historically meant a surrender of freedom for women.
Research Question and Embedded Thesis
There’s one more common style of thesis construction that’s worth noting, and that’s the inquiry-based thesis. (Read more about inquiry-based research writing in Chapter Eight). For this thesis, you’ll develop an incisive and focused question which you’ll explore throughout the course of the essay. By the end of the essay, you will be able to offer an answer (perhaps a complicated or incomplete answer, but still some kind of answer) to the question. This form is also referred to as the “embedded thesis” or “delayed thesis” organization.
Although this model of thesis can be effectively applied in a text wrestling essay, it is often more effective when combined with one of the other methods above.
Consider the following examples:
Bill Capossere’s essay “A Wind from the North” suggests that isolation results in sorrow and loneliness; is this always the case? How does Capossere create such a vision of his uncle’s life? or Many people would believe that Kate Chopin’s story reflects an outdated perception of marriage—but can “The Story of an Hour” reveal power imbalances in modern relationships, too?
Synthesis: Using Evidence to Explore Your Thesis
Now that you’ve considered what your analytical insight might be (articulated in the form of a thesis), it’s time to bring evidence in to support your analysis—this is the synthesis part of Bloom’s Taxonomy earlier in this chapter. Synthesis refers to the creation of a new whole (an interpretation) using smaller parts (evidence from the text you’ve analyzed).
There are essentially two ways to go about collecting and culling relevant support from the text with which you’re wrestling. In my experience, students are split about evenly on which option is better for them:
Option #1: Before writing your thesis, while you’re reading and rereading your text, annotate the page and take notes. Copy down quotes, images, formal features, and themes that are striking, exciting, or relatable. Then, try to group your collection of evidence according to common traits. Once you’ve done so, choose one or two groups on which to base your thesis. Or Option #2: After writing your thesis , revisit the text looking for quotes, images, and themes that support, elaborate, or explain your interpretation. Record these quotes, and then return to the drafting process.
Once you’ve gathered evidence from your focus text, you should weave quotes, paraphrases, and summaries into your own writing. A common misconception is that you should write “around” your evidence, i.e. choosing the direct quote you want to use and building a paragraph around it. Instead, you should foreground your interpretation and analysis, using evidence in the background to explore and support that interpretation. Lead with your idea, then demonstrate it with evidence; then, explain how your evidence demonstrates your idea.
The appropriate ratio of evidence (their writing) to exposition (your writing) will vary depending on your rhetorical situation, but I advise my students to spend at least as many words unpacking a quote as that quote contains. (I’m referring here to Step #4 in the table below.) For example, if you use a direct quote of 25 words, you ought to spend at least 25 words explaining how that quote supports or nuances your interpretation.
There are infinite ways to bring evidence into your discussion, 6 but for now, let’s take a look at a formula that many students find productive as they find their footing in analytical writing: Front-load + Quote/Paraphrase/Summarize + Cite + Explain/elaborate/analyze.
What might this look like in practice?
The recurring imagery of snow creates a tone of frostiness and demonstrates the passage of time. (1) Snow brings to mind connotations of wintery cold, quiet, and death (2) as a “sky of utter clarity and simplicity” lingers over his uncle’s home and “it [begins] once more to snow” ( (3) Capossere 104). (4) Throughout his essay, Capossere returns frequently to weather imagery, but snow especially, to play on associations the reader has. In this line, snow sets the tone by wrapping itself in with “clarity,” a state of mind. Even though the narrator still seems ambivalent about his uncle, this clarity suggests that he is reflecting with a new and somber understanding.
- Front-load Snow brings to mind connotations of wintery cold, quiet, and death
- Quote as a “sky of utter clarity and simplicity” lingers over his uncle’s home and “it [begins] once more to snow”
- Cite (Capossere 104).
- Explain/elaborate/analysis Throughout his essay, Capossere returns frequently to weather imagery, but snow especially, to play on associations the reader has. In this line, snow sets the tone by wrapping itself in with “clarity,” a state of mind. Even though the narrator still seems ambivalent about his uncle, this clarity suggests that he is reflecting with a new and somber understanding.
This might feel formulaic and forced at first, but following these steps will ensure that you give each piece of evidence thorough attention. Some teachers call this method a “quote sandwich” because you put your evidence between two slices of your own language and interpretation.
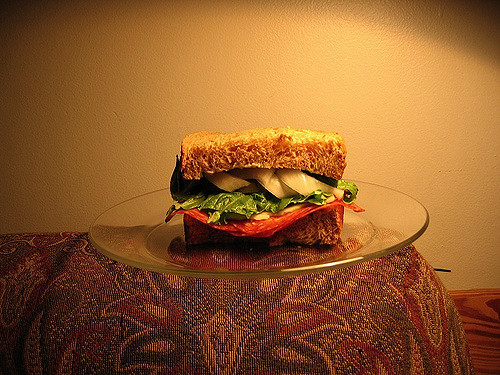
For more on front-loading (readerly signposts or signal phrases), see the subsection titled “Readerly Signposts” in Chapter Nine.
Idea Generation: Close Reading Graphic Organizer
The first time you read a text, you most likely will not magically stumble upon a unique, inspiring insight to pursue as a thesis. As discussed earlier in this section, close reading is an iterative process, which means that you must repeatedly encounter a text (reread, re-watch, re-listen, etc.) trying to challenge it, interrogate it, and gradually develop a working thesis.
Very often, the best way to practice analysis is collaboratively, through discussion. Because other people will necessarily provide different perspectives through their unique interpretive positions, reading groups can help you grow your analysis. By discussing a text, you open yourself up to more nuanced and unanticipated interpretations influenced by your peers. Your teacher might ask you to work in small groups to complete the following graphic organizer in response to a certain text. (You can also complete this exercise independently, but it might not yield the same results.)
Thesis Builder
Your thesis statement can and should evolve as you continue writing your paper: teachers will often refer to a thesis as a “working thesis” because the revision process should include tweaking, pivoting, focusing, expanding, and/or rewording your thesis. The exercise on the next two pages, though, should help you develop a working thesis to begin your project. Following the examples, identify the components of your analysis that might contribute to a thesis statement.
Model Texts by Student Authors
(A text wrestling analysis of “Proofs” by Richard Rodriguez)
Songs are culturally important. In the short story “Proofs” by Richard Rodriguez, a young Mexican American man comes to terms with his bi-cultural life. This young man’s father came to America from a small and poverty-stricken Mexican village. The young man flashes from his story to his father’s story in order to explore his Mexican heritage and American life. Midway through the story Richard Rodriguez utilizes the analogies of songs to represent the cultures and how they differ. Throughout the story there is a clash of cultures. Because culture can be experienced through the arts and teachings of a community, Rodriguez uses the songs of the two cultures to represent the protagonist’s bi-cultural experience.
According to Rodriguez, the songs that come from Mexico express an emotional and loving culture and community: “But my mama says there are no songs like the love songs of Mexico” (50). The songs from that culture can be beautiful. It is amazing the love and beauty that come from social capital and community involvement. The language Richard Rodriguez uses to explain these songs is beautiful as well. “—it is the raw edge of sentiment” (51). The author explains how it is the men who keep the songs. No matter how stoic the men are, they have an outlet to express their love and pain as well as every emotion in between. “The cry of a Jackal under the moon, the whistle of a phallus, the maniacal song of the skull” (51). This is an outlet for men to express themselves that is not prevalent in American culture. It expresses a level of love and intimacy between people that is not a part of American culture. The songs from the American culture are different. In America the songs get lost. There is assimilation of cultures. The songs of Mexico are important to the protagonist of the story. There is a clash between the old culture in Mexico and the subject’s new American life represented in these songs.
A few paragraphs later in the story, on page 52, the author tells us the difference in the American song. America sings a different tune. America is the land of opportunity. It represents upward mobility and the ability to “make it or break it.” But it seems there is a cost for all this material gain and all this opportunity. There seems to be a lack of love and emotion, a lack of the ability to express pain and all other feelings, the type of emotion which is expressed in the songs of Mexico. The song of America says, “You can be anything you want to be” (52). The song represents the American Dream. The cost seems to be the loss of compassion, love and emotion that is expressed through the songs of Mexico. There is no outlet quite the same for the stoic men of America. Rodriguez explains how the Mexican migrant workers have all that pain and desire, all that emotion penned up inside until it explodes in violent outbursts. “Or they would come into town on Monday nights for the wrestling matches or on Tuesdays for boxing. They worked over in Yolo County. They were men without women. They were Mexicans without Mexico” (49).
Rodriguez uses the language in the story almost like a song in order to portray the culture of the American dream. The phrase “I will send for you or I will come home rich,” is repeated twice throughout the story. The gain for all this loss of love and compassion is the dream of financial gain. “You have come into the country on your knees with your head down. You are a man” (48). That is the allure of the American Dream.
The protagonist of the story was born in America. Throughout the story he is looking at this illusion of the American Dream through a different frame. He is also trying to come to terms with his own manhood in relation to his American life and Mexican heritage. The subject has the ability to see the two songs in a different light. “The city will win. The city will give the children all the village could not-VCR’s, hairstyles, drumbeat. The city sings mean songs, dirty songs” (52). Part of the subject’s reconciliation process with himself is seeing that all the material stuff that is dangled as part of the American Dream is not worth the love and emotion that is held in the old Mexican villages and expressed in their songs.
Rodriguez represents this conflict of culture on page 53. The protagonist of the story is taking pictures during the arrest of illegal border-crossers. “I stare at the faces. They stare at me. To them I am not bearing witness; I am part of the process of being arrested”(53). The subject is torn between the two cultures in a hazy middle ground. He is not one of the migrants and he is not one of the police. He is there taking pictures of the incident with a connection to both of the groups and both of the groups see him connected with the other.
The old Mexican villages are characterized by a lack of : “Mexico is poor” (50). However, this is not the reason for the love and emotion that is held. The thought that people have more love and emotion because they are poor is a misconception. There are both rich people and poor people who have multitudes of love and compassion. The defining elements in creating love and emotion for each other comes from the level of community interaction and trust—the ability to sing these love songs and express emotion towards one another. People who become caught up in the American Dream tend to be obsessed with their own personal gain. This diminishes the social interaction and trust between fellow humans. There is no outlet in the culture of America quite the same as singing love songs towards each other. It does not matter if they are rich or poor, lack of community, trust, and social interaction; lack of songs can lead to lack of love and emotion that is seen in the old songs of Mexico.
The image of the American Dream is bright and shiny. To a young boy in a poor village the thought of power and wealth can dominate over a life of poverty with love and emotion. However, there is poverty in America today as well as in Mexico. The poverty here looks a little different but many migrants and young men find the American Dream to be an illusion. “Most immigrants to America came from villages.
The America that Mexicans find today, at the decline of the century, is a closed-circuit city of ramps and dark towers, a city without God. The city is evil. Turn. Turn” (50). The song of America sings an inviting tune for young men from poor villages. When they arrive though it is not what they dreamed about. The subject of the story can see this. He is trying to come of age in his own way, acknowledging America and the Mexico of old. He is able to look back and forth in relation to the America his father came to for power and wealth and the America that he grew up in. All the while, he watches this migration of poor villages, filled with love and emotion, to a big heartless city, while referring back to his father’s memory of why he came to America and his own memories of growing up in America. “Like wandering Jews. They carried their home with them, back and forth: they had no true home but the tabernacle of memory” (51). The subject of the story is experiencing all of this conflict of culture and trying to compose his own song.
Works Cited
Rodriguez, Richard. “Proofs.” In Short: A Collection of Brief Creative Nonfiction , edited by Judith Kitchen and Mary Paumier Jones, Norton, 1996, pp. 48-54.
Normal Person: An Analysis of the Standards of Normativity in “A Plague of Tics” 9
David Sedaris’ essay “A Plague of Tics” describes Sedaris’ psychological struggles he encountered in his youth, expressed through obsessive-compulsive tics. These abnormal behaviors heavily inhibited his functionings, but more importantly, isolated and embarrassed him during his childhood, adolescence, and young adult years. Authority figures in his life would mock him openly, and he constantly struggled to perform routine simple tasks in a timely manner, solely due to the amount of time that needed to be set aside for carrying out these compulsive tics. He lacked the necessary social support an adolescent requires because of his apparent abnormality. But when we look at the behaviors of his parents, as well as the socially acceptable tics of our society more generally, we see how Sedaris’ tics are in fact not too different, if not less harmful than those of the society around him. By exploring Sedaris’ isolation, we can discover that socially constructed standards of normativity are at best arbitrary, and at worst violent.
As a young boy, Sedaris is initially completely unaware that his tics are not socially acceptable in the outside world. He is puzzled when his teacher, Miss Chestnut, correctly guesses that he is “going to hit [himself] over the head with [his] shoe” (361), despite the obvious removal of his shoe during their private meeting. Miss Chestnut continues by embarrassingly making fun out of the fact that Sedaris’ cannot help but “bathe her light switch with [his] germ-ridden tongue” (361) repeatedly throughout the school day. She targets Sedaris with mocking questions, putting him on the spot in front of his class; this behavior is not ethical due to Sedaris’ age. It violates the trust that students should have in their teachers and other caregivers. Miss Chestnut criticizes him excessively for his ambiguous, child-like answers. For example, she drills him on whether it is “healthy to hit ourselves over the head with our shoes” (361) and he “guess[es] that it was not,” (361) as a child might phrase it. She ridicules his use of the term “guess,” using obvious examples of instances when guessing would not be appropriate, such as “[running] into traffic with a paper sack over [her] head” (361). Her mockery is not only rude, but ableist and unethical. Any teacher—at least nowadays—should recognize that Sedaris needs compassion and support, not emotional abuse.
These kinds of negative responses to Sedaris’ behavior continue upon his return home, in which the role of the insensitive authority figure is taken on by his mother. In a time when maternal support is crucial for a secure and confident upbringing, Sedaris’ mother was never understanding of his behavior, and left little room for open, honest discussion regarding ways to cope with his compulsiveness. She reacted harshly to the letter sent home by Miss Chestnut, nailing Sedaris, exclaiming that his “goddamned math teacher” (363) noticed his strange behaviors, as if it should have been obvious to young, egocentric Sedaris. When teachers like Miss Chestnut meet with her to discuss young David’s problems, she makes fun of him, imitating his compulsions; Sedaris is struck by “a sharp, stinging sense of recognition” upon viewing this mockery (365). Sedaris’ mother, too, is an authority figure who maintains ableist standards of normativity by taunting her own son. Meeting with teachers should be an opportunity to truly help David, not tease him.
On the day that Miss Chestnut makes her appearance in the Sedaris household to discuss his behaviors with his mother, Sedaris watches them from the staircase, helplessly embarrassed. We can infer from this scene that Sedaris has actually become aware of that fact that his tics are not considered to be socially acceptable, and that he must be “the weird kid” among his peers—and even to his parents and teachers. His mother’s cavalier derision demonstrates her apparent disinterest in the well-being of he son, as she blatantly brushes off his strange behaviors except in the instance during which she can put them on display for the purpose of entertaining a crowd. What all of these pieces of his mother’s flawed personality show us is that she has issues too—drinking and smoking, in addition to her poor mothering—but yet Sedaris is the one being chastised while she lives a normal life. Later in the essay, Sedaris describes how “a blow to the nose can be positively narcotic” (366), drawing a parallel to his mother’s drinking and smoking. From this comparison, we can begin to see flawed standards of “normal behavior”: although many people drink and smoke (especially at the time the story takes place), these habits are much more harmful than what Sedaris does in private.
Sedaris’ father has an equally harmful personality, but it manifests differently. Sedaris describes him as a hoarder, one who has, “saved it all: every last Green Stamp and coupon, every outgrown bathing suit and scrap of linoleum” (365). Sedaris’ father attempts to “cure [Sedaris] with a series of threats” (366). In one scene, he even enacts violence upon David by slamming on the brakes of the car while David has his nose pressed against a windshield. Sedaris reminds us that his behavior might have been unusual, but it wasn’t violent: “So what if I wanted to touch my nose to the windshield? Who was I hurting?” (366). In fact, it is in that very scene that Sedaris draws the aforementioned parallel to his mother’s drinking: when Sedaris discovers that “a blow to the nose can be positively narcotic,” it is while his father is driving around “with a lapful of rejected, out-of-state coupons” (366). Not only is Sedaris’ father violating the trust David places in him as a caregiver; his hoarding is an arguably unhealthy habit that simply happens to be more socially acceptable than licking a concrete toadstool. Comparing Sedaris’s tics to his father’s issues, it is apparent that his father’s are much more harmful than his own. None of the adults in Sedaris’ life are innocent—“mother smokes and Miss Chestnut massaged her waist twenty, thirty times a day—and here I couldn’t press my nose against the windshield of a car” (366)—but nevertheless, Sedaris’s problems are ridiculed or ignored by the ‘normal’ people in his life, again bringing into question what it means to be a normal person.
In high school, Sedaris’ begins to take certain measures to actively control and hide his socially unacceptable behaviors. “For a time,” he says, “I thought that if I accompanied my habits with an outlandish wardrobe, I might be viewed as eccentric rather than just plain retarded” (369). Upon this notion, Sedaris starts to hang numerous medallions around his neck, reflecting that he “might as well have worn a cowbell” (369) due to the obvious noises they made when he would jerk his head violently, drawing more attention to his behaviors (the opposite of the desired effect). He also wore large glasses, which he now realizes made it easier to observe his habit of rolling his eyes into his head, and “clunky platform shoes [that] left lumps when used to discreetly tap [his] forehead” (369). Clearly Sedaris was trying to appear more normal, in a sense, but was failing terribly. After high school, Sedaris faces the new wrinkle of sharing a college dorm room. He conjures up elaborate excuses to hide specific tics, ensuring his roommate that “there’s a good chance the brain tumor will shrink” (369) if he shakes his head around hard enough and that specialists have ordered him to perform “eye exercises to strengthen what they call he ‘corneal fibers’” (369). He eventually comes to a point of such paranoid hypervigilance that he memorizes his roommate’s class schedule to find moments to carry out his tics in privacy. Sedaris worries himself sick attempting to approximate ‘normal’: “I got exactly fourteen minutes of sleep during my entire first year of college” (369). When people are pressured to perform an identity inconsistent with their own—pressured by socially constructed standards of normativity—they harm themselves in the process. Furthermore, even though the responsibility does not necessarily fall on Sedaris’ peers to offer support, we can assume that their condemnation of his behavior reinforces the standards that oppress him.
Sedaris’ compulsive habits peak and begin their slow decline when he picks up the new habit of smoking cigarettes, which is of course much more socially acceptable while just as compulsive in nature once addiction has the chance to take over. He reflects, from the standpoint of an adult, on the reason for the acquired habit, speculating that “maybe it was coincidental, or perhaps … much more socially acceptable than crying out in tiny voices” (371). He is calmed by smoking, saying that “everything’s fine as long I know there’s a cigarette in my immediate future” (372). (Remarkably, he also reveals that he has not truly been cured, as he revisits his former tics and will “dare to press [his] nose against the doorknob or roll his eyes to achieve that once-satisfying ache” [372.]) Sedaris has officially achieved the tiresome goal of appearing ‘normal’, as his compulsive tics seemed to “[fade] out by the time [he] took up with cigarettes” (371). It is important to realize, however, that Sedaris might have found a socially acceptable way to mask his tics, but not a healthy one. The fact that the only activity that could take place of his compulsive tendencies was the dangerous use of a highly addictive substance, one that has proven to be dangerously harmful with frequent and prolonged use, shows that he is conforming to the standards of society which do not correspond with healthy behaviors.
In a society full of dangerous, inconvenient, or downright strange habits that are nevertheless considered socially acceptable, David Sedaris suffered through the psychic and physical violence and negligence of those who should have cared for him. With what we can clearly recognize as a socially constructed disability, Sedaris was continually denied support and mocked by authority figures. He struggled to socialize and perform academically while still carrying out each task he was innately compelled to do, and faced consistent social hardship because of his outlandish appearance and behaviors that are viewed in our society as “weird.” Because of ableist, socially constructed standards of normativity, Sedaris had to face a long string of turmoil and worry that most of society may never come to completely understand. We can only hope that as a greater society, we continue sharing and studying stories like Sedaris’ so that we critique the flawed guidelines we force upon different bodies and minds, and attempt to be more accepting and welcoming of the idiosyncrasies we might deem to be unfavorable.
Teacher Takeaways
“The student clearly states their thesis in the beginning, threading it through the essay, and further developing it through a synthesized conclusion. The student’s ideas build logically through the essay via effective quote integration: the student sets up the quote, presents it clearly, and then responds to the quote with thorough analysis that links it back to their primary claims. At times this thread is a bit difficult to follow; as one example, when the student talks about the text’s American songs, it’s not clear how Rodriguez’s text illuminates the student’s thesis. Nor is it clear why the student believes Rodriguez is saying the “American Dream is not worth the love and emotion.” Without this clarification, it’s difficult to follow some of the connections the student relies on for their thesis, so at times it seems like they may be stretching their interpretation beyond what the text supplies.”– Professor Dannemiller
“I like how this student follows their thesis through the text, highlighting specific instances from Sedaris’s essay that support their analysis. Each instance of this evidence is synthesized with the student’s observations and connected back to their thesis statement, allowing for the essay to capitalize on the case being built in their conclusion. At the ends of some earlier paragraphs, some of this ‘spine-building’ is interrupted with suggestions of how characters in the essay should behave, which doesn’t always clearly link to the thesis’s goals. Similarly, some information isn’t given a context to help us understand its relevance, such as what violating the student-teacher trust has to do with normativity being a social construct, or how Sedaris’s description of ‘a blow to the nose’ being a narcotic creates a parallel to his mother’s drinking and smoking. Without further analysis and synthesis of this information the reader is left to guess how these ideas connect.”– Professor Dannemiller
Sedaris, David. “A Plague of Tics.” 50 Essays: A Portable Anthology , 4 th edition, edited by Samuel Cohen, Bedford, 2013, pp. 359-372.
Analyzing “Richard Cory” 10
In the poem “Richard Cory” by Edward Arlington Robinson, a narrative is told about the character Richard Cory by those who admired him. In the last stanza, the narrator, who uses the pronoun “we,” tells us that Richard Cory commits suicide. Throughout most of the poem, though, Cory had been described as a wealthy gentleman. The “people on the pavement” (2), the speakers of the poem, admired him because he presented himself well, was educated, and was wealthy. The poem presents the idea that, even though Cory seemed to have everything going for him, being wealthy does not guarantee happiness or health.
Throughout the first three stanzas Cory is described in a positive light, which makes it seem like he has everything that he could ever need. Specifically, the speaker compares Cory directly and indirectly to royalty because of his wealth and his physical appearance: “He was a gentleman from sole to crown, / Clean favored and imperially slim” (Robinson 3-4). In line 3, the speaker is punning on “soul” and “crown.” At the same time, Cory is both a gentleman from foot (sole) to head (crown) and also soul to crown. The use of the word “crown” instead of head is a clever way to show that Richard was thought of as a king to the community. The phrase “imperially slim” can also be associated with royalty because imperial comes from “empire.” The descriptions used gave clear insight that he was admired for his appearance and manners, like a king or emperor.
In other parts of the poem, we see that Cory is ‘above’ the speakers. The first lines, “When Richard Cory went down town, / We people on the pavement looked at him” (1-2), show that Cory is not from the same place as the speakers. The words “down” and “pavement” also suggest a difference in status between Cory and the people. The phrase “We people on the pavement” used in the first stanza (Robinson 2), tells us that the narrator and those that they are including in their “we” may be homeless and sleeping on the pavement; at the least, this phrase shows that “we” are below Cory.
In addition to being ‘above,’ Cory is also isolated from the speakers. In the second stanza, we can see that there was little interaction between Cory and the people on the pavement: “And he was always human when he talked; / But still fluttered pulses when he said, / ‘Good- morning’” (Robinson 6-8). Because people are “still fluttered” by so little, we can speculate that it was special for them to talk to Cory. But these interactions gave those on the pavement no insight into Richard’s real feelings or personality. Directly after the descriptions of the impersonal interactions, the narrator mentions that “he was rich—yes, richer than a king” (Robinson 9). At the same time that Cory is again compared to royalty, this line reveals that people were focused on his wealth and outward appearance, not his personal life or wellbeing.
The use of the first-person plural narration to describe Cory gives the reader the impression that everyone in Cory’s presence longed to have the life that he did. Using “we,” the narrator speaks for many people at once. From the end of the third stanza to the end of the poem, the writing turns from admirable description of Richard to a noticeably more melancholy, dreary description of what those who admired Richard had to do because they did not have all that Richard did. These people had nothing, but they thought that he was everything. To make us wish that we were in his place. So on we worked, and waited for the light,
And went without the meat, and cursed the bread…. (Robinson 9-12)
They sacrificed their personal lives and food to try to rise up to Cory’s level. They longed to not be required to struggle. A heavy focus on money and materialistic things blocked their ability to see what Richard Cory was actually feeling or going through. I suggest that “we” also includes the reader of the poem. If we read the poem this way, “Richard Cory” critiques the way we glorify wealthy people’s lives to the point that we hurt ourselves. Our society values financial success over mental health and believes in a false narrative about social mobility.
Though the piece was written more than a century ago, the perceived message has not been lost. Money and materialistic things do not create happiness, only admiration and alienation from those around you. Therefore, we should not sacrifice our own happiness and leisure for a lifestyle that might not make us happy. The poem’s message speaks to our modern society, too, because it shows a stigma surrounding mental health: if people have “everything / To make us wish that we were in [their] place” (11-12), we often assume that they don’t deal with the same mental health struggles as everyone. “Richard Cory” reminds us that we should take care of each other, not assume that people are okay because they put up a good front.
“I enjoy how this author uses evidence: they use a signal phrase (front-load) before each direct quote and take plenty of time to unpack the quote afterward. This author also has a clear and direct thesis statement which anticipates the content of their analysis. I would advise them, though, to revise that thesis by ‘previewing’ the elements of the text they plan to analyze. This could help them clarify their organization, since a thesis should be a road-map.”– Professor Wilhjelm
Robinson, Edward Arlington. “Richard Cory.” The Norton Introduction to Literature , Shorter 12 th edition, edited by Kelly J. Mays, Norton, 2017, p. 482.
the cognitive process and/or rhetorical mode of studying constituent parts to demonstrate an interpretation of a larger whole.
a part or combination of parts that lends support or proof to an arguable topic, idea, or interpretation.
a cognitive and rhetorical process by which an author brings together parts of a larger whole to create a unique new product. Examples of synthesis might include an analytical essay, found poetry, or a mashup/remix.
a 1-3 sentence statement outlining the main insight(s), argument(s), or concern(s) of an essay; not necessary in every rhetorical situation; typically found at the beginning of an essay, though sometimes embedded later in the paper. Also referred to as a “So what?” statement.
EmpoWORD: A Student-Centered Anthology and Handbook for College Writers Copyright © 2018 by Shane Abrams is licensed under a Creative Commons Attribution-NonCommercial 4.0 International License , except where otherwise noted.
Share This Book
- Systematic Review
- Open access
- Published: 05 June 2024
Effects of Stretching or Strengthening Exercise on Spinal and Lumbopelvic Posture: A Systematic Review with Meta-Analysis
- Konstantin Warneke 1 ,
- Lars Hubertus Lohmann ORCID: orcid.org/0000-0002-5990-2290 2 &
- Jan Wilke 1
Sports Medicine - Open volume 10 , Article number: 65 ( 2024 ) Cite this article
123 Accesses
53 Altmetric
Metrics details
Abnormal posture (e.g. loss of lordosis) has been associated with the occurrence of musculoskeletal pain. Stretching tight muscles while strengthening the antagonists represents the most common method to treat the assumed muscle imbalance. However, despite its high popularity, there is no quantitative synthesis of the available evidence examining the effectiveness of the stretch-and-strengthen approach.
A systematic review with meta-analysis was conducted, searching PubMed, Web of Science and Google Scholar. We included controlled clinical trials investigating the effects of stretching or strengthening on spinal and lumbopelvic posture (e.g., pelvic tilt, lumbar lordosis, thoracic kyphosis, head tilt) in healthy individuals. Effect sizes were pooled using robust variance estimation. To rate the certainty about the evidence, the GRADE approach was applied.
A total of 23 studies with 969 participants were identified. Neither acute (d = 0.01, p = 0.97) nor chronic stretching (d=-0.19, p = 0.16) had an impact on posture. Chronic strengthening was associated with large improvements (d=-0.83, p = 0.01), but no study examined acute effects. Strengthening was superior (d = 0.81, p = 0.004) to stretching. Sub-analyses found strengthening to be effective in the thoracic and cervical spine (d=-1.04, p = 0.005) but not in the lumbar and lumbopelvic region (d=-0.23, p = 0.25). Stretching was ineffective in all locations ( p > 0.05).
Moderate-certainty evidence does not support the use of stretching as a treatment of muscle imbalance. In contrast, therapists should focus on strengthening programs targeting weakened muscles.
• Stretching of tight muscles and strengthening of weak muscles is popular in treating muscular imbalance of the pelvis and spine. While combined interventions have previously been meta-analyzed and shown to be effective, the effectiveness of both used in isolation has not been investigated.
• This meta-analysis found no effects of stretching on posture while strengthening can improve imbalances/posture.
• Additional studies including higher stretching volumes and intensities are warranted.
Spinal alignment and posture have been investigated for about 250 years [ 1 , 2 ]. Evidence syntheses from recent decades suggest that deviations from the assumed physiological norm may be associated with the occurrence of musculoskeletal pain. Chun et al. [ 3 ] found a strong cross-sectional relationship of reduced lumbar lordosis and low back pain. In a meta-analysis of prospective cohort studies, limited lordosis predicted the development of low back pain with an odds ratio of 1.27 [ 4 ]. With regard to the neck, patients with pain displayed a forward head posture (FHP) when compared to asymptomatic individuals. Interestingly, the magnitude of FHP correlated with neck pain intensity and subjective disability [ 5 ], which is frequently associated with, for instance, early fatigue, neck and shoulder pain, decreased respiratory capacity, as well as reduced aerobic endurance [ 6 , 7 ]. Barrett et al. [ 5 ] focused on thoracic kyphosis. The authors found that persons with excessive spinal curvature exhibited reductions in shoulder range of motion. This is of relevance because restricted shoulder mobility has been shown to increase the risk for upper extremity pain and injury [ 8 , 9 ].
Changes of lumbopelvic or spinal posture are commonly related to muscle imbalance [ 10 ]. Such imbalance is suggested to originate from extended periods of biomechanical, psychological and social stresses as well as repetitive activities [ 11 , 12 ] While some muscles respond with tightness or shortening, their antagonists may become too weak to maintain the normal joint position [ 13 , 14 , 15 , 16 , 17 , 18 ]. As an example for muscle imbalance, Janda [ 13 , 14 ] hypothesized that shortening of the pectoralis major, upper trapezius and levator scapulae muscles in conjunction with weakness of the deep neck flexors, lower trapezius and rhomboids causes excessive kyphosis and FHP.
Besides various other methods including mobilization [ 19 , 20 ], yoga [ 21 ], Pilates [ 22 , 23 ], manual therapy [ 24 ], or taping [ 25 ], stretching of tight muscles and strengthening of weak muscles has gained high popularity in the treatment of muscle imbalance. A survey by Perriman and colleagues from 2012 [ 26 ] revealed that 71% and 64% of the physiotherapists use stretching and strengthening, respectively, to treat excessive kyphosis, while in 2024, 60% of the physiotherapists and sport scientists attending an Austrian training convention assumed stretching to be effective in treating muscular imbalance [ 27 ]. Despite the frequent use of the stretch-and-strengthen approach, the effectiveness of corrective exercise routines on posture is questionable [ 15 , 16 ]. A systematic review with meta-analysis by Gonzalez-Galvez et al. [ 18 ] reported a positive influence of exercise programs in general, mostly when combining stretch and strengthening exercise. Interestingly, they concluded that strengthening may be superior to stretching. Yet, this assumption was based on the analysis of only 10 studies and, more importantly, no investigation of the isolated effects of stretching and stretching was performed. Withers et al. [ 28 ] included different training approaches. Among these, they examined stretching as a stand-alone treatment for hyperkyphosis. Since only one isolated static stretching was found, further research seems necessary. In view of the lack of evidence on the individual components of the stretch-and-strengthen approach, the present systematic review with meta-analysis was conducted to summarize the evidence on isolated stretch and strengthening treatments aiming to modify spinal or lumbopelvic posture.
A systematic review with meta-analysis was performed adhering to the PRISMA (Preferred Reporting Items for Systematic Reviews and Meta-Analyses) guidelines. We considered ethical publishing standards [ 29 ] and registered the study in the PROSPERO database (CRD42023412854).
Literature Search
Two authors (KW & LHL) conducted a systematic literature search using MEDLINE/PubMed and Web of Science (inception to April, 2023) and assessed all records independently. Disagreements at each screening level (title, abstract + full-text) were resolved by discussion (see Fig. 1 ). Database queries were supplemented by a hand search using Google Scholar as well as citation searching in eligible studies. The following criteria were applied for study inclusion: (1) randomized or non-randomized controlled intervention study design, (2) assessment of acute (post-testing immediately following the intervention) or chronic (intervention period of at least one week) effects, (3) comparison of stretching vs. strengthening, stretching vs. non-intervention control, or strengthening vs. non-intervention control, (4) measurement of pelvic tilt, lumbar lordosis, kyphosis, and/or forward head/forward shoulder posture using objective and quantifiable measurements (e.g., radiographs or camera systems), (5) inclusion of healthy adults. Patients with a history of musculoskeletal, neurologic, or cardiopulmonary disorders, joint replacements, osteoporosis, specific back pain or other pathologies were excluded from this analysis to improve homogeneity. Trials combining different interventions (i.e., stretching plus strengthening) were excluded as well.
Stretching interventions eligible for inclusion were static, dynamic and ballistic stretching and proprioceptive neuromuscular facilitation in accordance with Warneke & Lohmann [ 30 ] and Behm [ 31 ]. Static stretching was defined as muscle lengthening until onset of a stretch sensation or to the point of discomfort. By definition, this position is to be held and can be performed passively via partner, external weight or a tool, or actively via movement. Proprioceptive neuromuscular facilitation includes a (sub-) maximal voluntary contraction to a stretching bout with or without antagonist contraction. Dynamic stretching was defined as controlled back-and-forth movement in the end range of motion with ballistic stretching as a sub-category and less controlled, bouncing movements [ 32 ]. Strengthening interventions were considered eligible if the authors stated the application of dynamic or isometric muscle actions sufficient to increase strength capacity, while the control group was considered to be inactive if no structured intervention was performed within the study.
The search terms were created based on the requirements of each database (see Appendix S1 ). In addition to the database searches, the reference lists of all included studies were screened for further eligible articles [ 33 ].
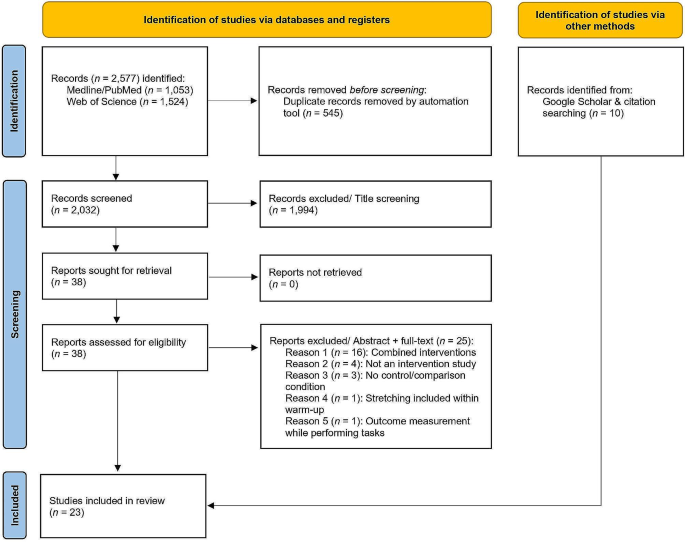
Flow-chart of literature search for studies assessing the influence of stretching or strengthening on posture
Methodological Study Quality and Risk of Bias
We used the PEDro scale for the assessment of methodological study quality [ 34 , 35 ]. Scoring was performed by two independent investigators (KW & LHL). If both did not reach consensus, a third examiner provided the decisive vote (JW) [ 28 ]. To estimate the risk of publication bias, funnel plots, created using the modification of Fernandez-Castilla et al. [ 36 ] for multiple study outcomes, were visually inspected. In addition, we performed Egger’s regression test with the extension for dependent effect sizes [ 36 ].
To rate the certainty about the evidence, we applied the GRADE working group criteria [ 37 ]. Briefly, the quality of evidence of randomized, controlled trials was initially classified as high and adjusted afterwards, considering the GRADE framework. In detail, in case of limitations in study design or execution, inconsistency of results, indirectness of evidence, imprecision or publication bias, one point was subtracted for each weakness. On the contrary, large magnitude effects or a dose-response gradient led to improvements of the quality of evidence by one point each. This resulted in a final rating of the certainty about the evidence as very low, low, moderate, or high.
Data Processing and Statistics
The means (M) and standard deviations (SD) from pre- and post-tests were extracted for all parameters (e.g. lordotic angle). In case of missing data, the authors of the primary studies were contacted. KW and LHL extracted data from eligible studies cooperatively, meaning that one read the values aloud and checked the shared screen while the other entered the numbers in a Microsoft Excel sheet. Additionally, KW double-checked the entered values for accuracy at the end of the extraction process. Changes from pre- to post-test were calculated as M (posttest) – M (pretest) and standard deviations were pooled as
A meta-analysis with robust variance estimation, accounting for the dependency of effect sizes (e.g. in case of multiple outcomes in the same study), was performed to pool the standardized mean differences (SMD) and 95% confidence intervals (CI) between the intervention (stretching or strengthening) and control groups [ 38 ]. The between-study variance component was estimated using τ 2 . Pooled effect sizes (ES) were interpreted as follows: 0 ≤ ES < 0.2 trivial, 0.2 ≤ ES < 0.5 small, 0.5 ≤ ES < 0.8 moderate and ES ≥ 0.8 large [ 39 ]. Besides the omnibus analyses on the effects of stretching and strengthening, we performed sub-analyses for different body regions (1: forward head posture/thoracic kyphosis, 2: pelvic angle/lordotic angle). All calculations were performed using R and the robumeta package [ 40 ].
Search Results and Study Characteristics
Figure 1 shows the flow-chart of the literature search.
A total of 23 studies [ 41 , 42 , 43 , 44 , 45 , 46 , 47 , 48 , 49 , 50 , 51 , 52 , 53 , 54 , 55 , 56 , 57 , 58 , 59 , 60 , 61 , 62 , 63 ] ( n = 969 participants, 48 ES) were found eligible. Fourteen of the papers examined the effects of stretching [ 41 , 43 , 45 , 46 , 51 , 52 , 53 , 54 , 55 , 57 , 58 , 59 , 61 , 62 ] while fifteen studies [ 42 , 43 , 44 , 46 , 47 , 48 , 49 , 50 , 51 , 52 , 56 , 59 , 60 , 62 , 63 ] investigated the effects of strengthening. The majority of the studies ( n = 21) focused on chronic treatment effects while only 2 studies explored acute effects. These were quantified via the Cobb angle, kyphosis angle, lordosis angle, head tilt angle, neck flexion angle, hip extension angle, acromion process vertical distance and assessed with marker-based camera (three-dimensional) motion capture systems, radiography, the spinal mouse system, steel ruler, photographs, flexible rulers, inclinometers and goniometers. Most studies ( n = 17) included participants without pain. While patients were generally excluded, six studies included participants with unspecific back ( n = 2) [ 54 , 59 ] or neck ( n = 4) [ 51 , 52 , 60 , 63 ] pain. Table 1 provides information about the studies’ characteristics.
Methodological Quality, Risk of Bias and Certainty About the Evidence
For stretching studies, the average risk of bias was rated as fair with a PEDro score of 4.1 ± 1.3 (range: 3 to 8 points). The same applied to strengthening studies, which averaged 4.3 ± 1.4 points (range: 2 to 7). Almost all studies used random group allocation, reported statistical between-group comparisons and provided both, point measures and measures of variability. In contrast, blinding of the participants was only reported in one study, and not at all for therapist blinding. Also, very few studies ( n = 2) declared application of the intention-to-treat principle (see Table 2 ).
Visual inspection of funnel plots suggested absence of a publication bias (Figures A-C in Supplemental material). These results were confirmed by Egger’s regression tests (t = 2.26, p = 0.16, 95% CI -0.32–0.99) for chronic stretching, (t=-0.88, p = 0.206, 95% CI -2.40–0.64), strengthening, and (t = 0.76, p = 0.532, 95% CI -2.06–2.84) chronic stretching vs. strengthening.
With regard to the stretching studies, the certainty about the evidence was downgraded by 1 level (high to moderate) due to (1) risk of bias classified as fair via the PEDro score. For the strengthening studies, due to (1) risk of bias and (2) heterogeneity, certainty was downgraded by 2 levels (high to low) but upgraded one level due to the large effect size. Therefore, in sum, for both stretching and strengthening, the certainty about the evidence was moderate.
Quantitative Synthesis
Neither acute stretching (d = 0.013, -3.33, 3.36 95% CI, p = 0.97, τ²=0.01, 2 studies, 3 ES) nor chronic stretching (ES=-0.19, 95%CI -0.47 to 0.1, p = 0.16, τ²=0.0, 8 studies, 15 ES) had an effect on posture. Likewise, subgroup analyses showed no impact of stretching in any of the tested body regions (pelvis/lumbar spine: ES=-0.04, 95% CI -0.17 to 0.09, p = 0.43, τ²=0.0, 5 studies, 7 ES; thoracic/cervical spine: ES=-0.44, 95% CI -1.03 to 0.16, p = 0.101, τ²=0.02, 4 studies, 8 ES; see Table 3 ; Fig. 2 ). The certainty about the evidence was moderate.
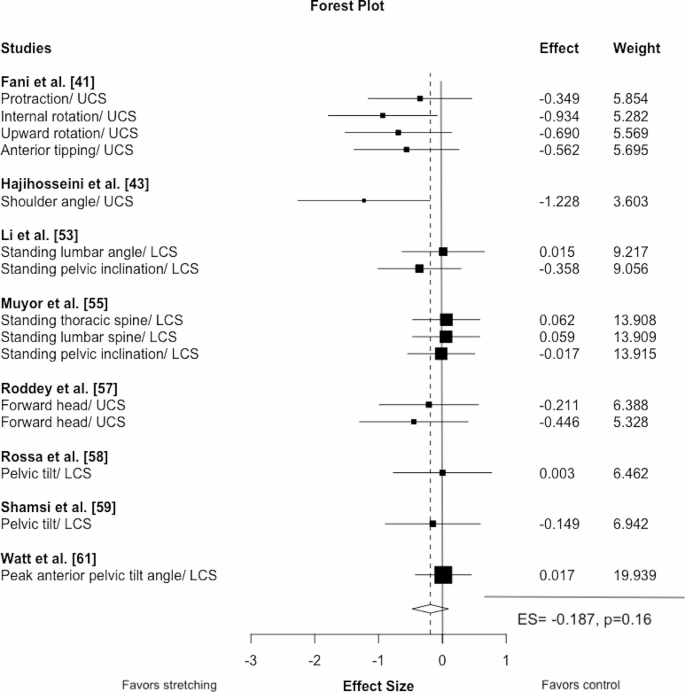
Forest plot for chronic stretching interventions on posture. Negative values illustrate effects favoring stretching compared to control. The effect size includes the 95% confidence interval
Chronic Strengthening
No study examined acute strengthening effects. Chronic strengthening had a large beneficial effect on posture (ES=-0.87, 95% CI -1.58 to -0.17, p = 0.02, τ²=0.4, 10 studies, 19 ES). According to the sub-analysis, no impact was identified in the pelvis and lumbar spine (ES=-0.23, 95% CI -1.45 to 0.98 p = 0.25, τ²=0.00, 2 studies, 5 ES), while a very large effect was found for the thoracic/cervical spine (ES=-1.04, 95% CI -1.69, -0.40, p = 0.005 τ²=0.19, 10 studies, 14 ES; Fig. 3 ). The certainty about the evidence was moderate.
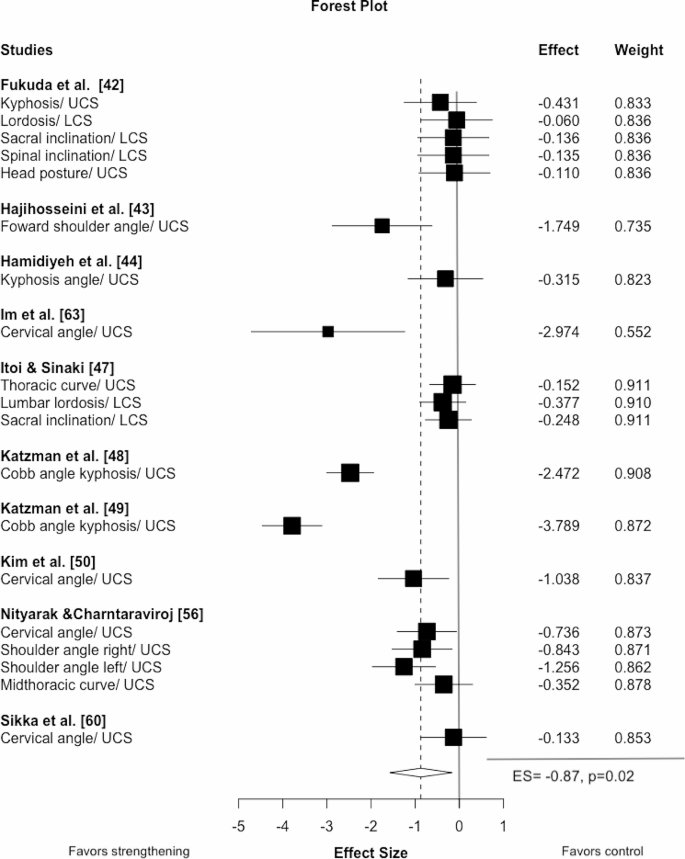
Forest plot for chronic strengthening interventions on posture. Negative values illustrate effects favoring strengthening compared to control. The effect size includes the 95% confidence interval
Stretching vs. Strengthening
No study comparing acute stretch and strengthening interventions was found. For chronic interventions, a large effect in favour of strengthening exercise (d = 0.81, 0.4, 1.22 95% CI, p = 0.004, τ²=0.02, 6 studies, 9 ES) was detected. Since all studies but one focused on the thoracic/cervical spine region, no sub-analysis of body locations was possible.
Stretching of tight or shortened skeletal muscles represents one of the most popular strategies used to tackle muscle imbalance and postural impairments [ 26 ]. As early as 1997, Spring et al. [ 64 ] recommended it as the gold standard of posture treatment and twenty years later, the application of stretch was still described a viable method preventing hypertonia-induced muscular imbalance [ 65 ]. While recent reviews did not consider stretching as a stand-alone intervention [ 18 , 66 ], Withers et al. [ 28 ] were only able to include one stretching study in their meta-analysis. Summarizing the effects of 12 chronic stretching studies, our systematic review is the first to extensively examine the foundation of this approach. Of note, in contrast to popular beliefs in practice, moderate-certainty evidence does not support the use of stretching when aiming to tackle imbalance-related posture deficits (e.g. hyperkyphosis or forward head posture). However, our analysis revealed a large effect of strengthening which also was superior in direct comparison to stretching. This finding confirms earlier speculations by Gonzalez-Galvez et al. [ 18 ] who reported combined stretching and strengthening to improve spinal posture, but suggested that only strengthening may be effective. As a consequence, exercise therapy for posture can be substantially economized by forgoing stretching tight muscles, and instead focusing on strengthening weakened muscles.
From a physiological point of view, it has been argued that chronic stretching of a tight or shortened muscle would lower its stiffness or tone. While stretching of two to eight minutes acutely reduced muscle stiffness [ 67 , 68 , 69 , 70 , 71 ], a rapid return to baseline occurred after a short recovery of only up to 20 min. This is highly plausible considering the mechanical role of the titin filament. The protein, which is attached to the myosin filament and the z-disk, has substantial elastic properties and after being lengthened (e.g., during a stretch), it helps to restore the original passive resting length. Acting as a molecular spring [ 72 , 73 , 74 ], it hence regulates the mechanical behavior of the muscle fiber [ 75 ]. Data collected in rabbits revealed that titin contributes up to 60% of the total passive stiffness of a skeletal muscle [ 76 ]. Experimentally disrupting the filament decreased passive tension by 50 to 100% [ 77 ]. Considering the elastic properties of titin and its role in passive muscle tension, the acute reductions in stiffness after stretching as well as the fast restoration of baseline values seem logical. Interestingly, the evidence of potential stiffness changes following chronic stretching treatments seems controversial. While in 2018, Freitas and colleagues [ 78 ] found stretch-mediated stiffness reduction in response to weekly volumes of up to 20 min over up to eight weeks unlikely, more recent literature found opposing results [ 79 ]. Yet, even if long-term stretching could reduce muscle stiffness, the causal relationship between decreasing stiffness of shortened muscles and improvements in posture remains speculative, calling for further exploration. While there is currently no evidence for positive chronic effects of stretching on posture, this might potentially be due to a lack of investigations that use sufficient stretching volumes meaning further research is necessary. Irrespective, it needs to be acknowledged that only two studies were available on acute stretch application. Additional research evaluating the immediate impact on posture is therefore warranted as well.
Besides reduced stiffness, another suggested effect of chronic stretching is an increase in muscle length. As such, one might expect the formation of new serial sarcomeres within the muscle-tendon-unit [ 80 , 81 ]. Indeed, Williams and Goldspink et al. [ 82 ] observed a higher sarcomere number following long-term immobilization of animal limbs. However, on the one hand, immobilization cannot be readily compared to stretching and, on the other hand, the applicability of animal findings to humans is disputed [ 80 ]. Interestingly, titin does not only regulate the resting tension of the skeletal muscle but also appears to play an important role in structural adaptations. Van der Pjil et al. [ 83 ] described the importance of titin unfolding at high muscle lengths for sarcomerogenesis and with this, longitudinal (and parallel) hypertrophy. Even though viable, observations indicating a possible influence of chronic stretch training on structural properties were, to the best of our knowledge, exclusively made in animals [ 84 , 85 ]. However, again, no transfer of longitudinal hypertrophy effects to humans was found [ 86 ]. Before 2020, stretch-induced chronic structural stretching adaptations were classified unlikely [ 78 , 86 ], but within the past 5 years, evidence emerged that large stretching volumes (≥ 15 min per day, ≥6 weeks intervention period) have the potential to induce muscle hypertrophy, and with this, changes in tissue morphology [ 87 , 88 ]. As, to date, no evidence could be found for longitudinal hypertrophy, it could be speculated that the studies matching the inclusion criteria of this systematic review did not perform stretching with the required stretching duration and/or intensity [ 87 , 88 , 89 ].
Contrarily to stretching, we found a large beneficial influence of strengthening on posture. However, the underlying mechanisms are a matter of debate. Surprisingly, there is a lack of conclusive research on resistance training-induced changes of the muscle’s passive mechanical properties [ 90 ]. In 1998, the hypothesis of increases in passive muscle stiffness as an adaptation to resistance training arose [ 91 ], leading to the recommendation to strengthen lengthened or weak muscle groups in muscle imbalance. The authors argued that hypertrophy would be associated with a larger number of parallel titin-myosin filaments, which, in agreement with the above-described evidence, would lead to a higher resting tension [ 91 ]. Indeed, in a ten-week strength training study, the authors reported a 30%-increase in passive tension without decreases in extensibility of the muscle. In another study, isometric resistance training led to an increase in core stiffness [ 92 ]. However, a recent systematic review found no stiffness changes in the long-term as a response to resistance training [ 93 ]. Of note, the review only included measurements with ultrasound elastography which allows assumptions on compressive tissue stiffness. Assuming specific resistance training adaptations occur following induction of tensile/shortening stress to the muscle, it seems necessary to distinguish between compressive and tensile or strain stiffness. Research on foam rolling effects revealed that decreases in compressive stiffness could be detected using elastography and indentometric methods, while this was not the case for tensile stiffness using passive resistive torque during stretch [ 94 , 95 ]. As a consequence, it may be assumed that stiffness changes are specific to the applied stimulus (compression in foam rolling, but stretch-shortening in resistance exercise). Following this theory, it would still be possible that resistance training does only modify tensile stiffness, which would also align with the role of titin as a serial agent for passive tension regulation. In sum, more research is warranted in order to gain further insight into the mechanisms of strengthening-induced improvements of posture.
Implications
Our findings have implications for clinical practice. As indicated, stretching is highly popular among therapists aiming to treat muscle imbalance and frequently recommended in the scientific literature [ 26 , 64 , 65 ]. Yet, the available evidence speaks strongly against this approach. In line with earlier speculations of Gonzalez-Galvez et al. [ 18 ], beneficial exercise effects seem rather attributable to strengthening, while stretching programs are ineffective. Consequently, when aiming to counteract muscular imbalances and to improve spinal and lumbopelvic posture, no evidence-based recommendation for the implementation of stretching can be given. Interestingly, we found a beneficial influence of strengthening for the thoracic and cervical spine region, while no changes were detected in the lumbar and pelvic region. On the one hand, effect sizes were in fact trivial to small for the lumbar spine and pelvis. On the other hand, with a total of only 5 ES from two studies, this region is under-researched. Future investigations, besides aiming to better understand the physiological adaptions of stretching and strengthening with regard to passive tissue properties (muscle, tendons, fascia) [ 90 ] and neuromuscular aspects [ 10 ], should be geared to provide more data on exercise treatments in the lumbar spine region.
The common recommendation of stretching tight or shortened skeletal muscle to improve muscle imbalance and posture lacks scientific evidence (moderate certainty). In contrast, our review reinforces the role of strengthening weak antagonists which, however, was only effective in the thoracic and cervical but not in the lumbar spine (moderate certainty). Further well-designed RCTs, e.g. applying high stretch durations and experimental studies elaborating the underlying physiological mechanisms, are required to conclusively judge the role of treatments aiming to modify postural abnormalities.
Data Availability
Data can be provided on reasonable request.
Abbreviations
Confidence interval
Effect size
Standard difference
Standardized mean difference.
Jones P. An Essay on Crookedness, or Distortions of the Spine. 1788.
Sayre LA. Deformities of spine. Atlanta Med Surg J. 1875;13:405–10.
PubMed PubMed Central Google Scholar
Chun S-W, Lim C-Y, Kim K, Hwang J, Chung SG. The relationships between low back pain and lumbar lordosis: a systematic review and meta-analysis. Spine J. 2017;17:1180–91.
Article PubMed Google Scholar
Sadler SG, Spink MJ, Ho A, De Jonge XJ, Chuter VH. Restriction in lateral bending range of motion, lumbar lordosis, and hamstring flexibility predicts the development of low back pain: a systematic review of prospective cohort studies. BMC Musculoskelet Disord. 2017;18:179.
Article PubMed PubMed Central Google Scholar
Barrett E, O’Keeffe M, O’Sullivan K, Lewis J, McCreesh K. Is thoracic spine posture associated with shoulder pain, range of motion and function? A systematic review. Man Ther. 2016;26:38–46.
Mahmoud NF, Hassan KA, Abdelmajeed SF, Moustafa IM, Silva AG. The relationship between Forward Head posture and Neck Pain: a systematic review and Meta-analysis. Curr Rev Musculoskelet Med. 2019;12:562–77.
Fatima A, Ashraf HS, Sohail M, Akram S, Khan M, Azam H. Prevalence of Upper Cross Syndrome and Associated Postural deviations in Computer operators; a qualitative study. J Allied Health Sci (AJAHS). 2022;7.
Bullock GS, Faherty MS, Ledbetter L, Thigpen CA, Sell TC. Shoulder range of motion and baseball arm injuries: a systematic review and Meta-analysis. J Athl Train. 2018;53:1190–9.
Hill L, Collins M, Posthumus M. Risk factors for shoulder pain and injury in swimmers: a critical systematic review. Phys Sportsmed. 2015;43:412–20.
Morris CE, Bonnefin D, Darville C. The Torsional Upper crossed syndrome: a multi-planar update to Janda’s model, with a case series introduction of the mid-pectoral fascial lesion as an associated etiological factor. J Bodyw Mov Ther. 2015;19:681–9.
Salsali M, Sheikhhoseini R, Sayyadi P, Hides JA, Dadfar M, Piri H. Association between physical activity and body posture: a systematic review and meta-analysis. BMC Public Health. 2023;23:1670.
Lynch SS, Thigpen CA, Mihalik JP, Prentice WE, Padua D. The effects of an exercise intervention on forward head and rounded shoulder postures in elite swimmers. Br J Sports Med. 2010;44:376–81.
Janda V. Some aspects of extracranial causes of facial pain. J Prosthet Dent. 1986;56:484–7.
Article CAS PubMed Google Scholar
Janda V. On the Concept of Postural Muscles and posture in Man. Australian J Physiotherapy. 1983;29:83–4.
Article CAS Google Scholar
Hrysomallis C. Effectiveness of strengthening and stretching exercises for the Postural correction of abducted scapulae: a review. J Strength Cond Res. 2010;24:567–74.
Hrysomallis C, Goodman C. A review of resistance exercise and posture realignment. J Strength Cond Res. 2001;15:385–90.
CAS PubMed Google Scholar
Jang H-J, Hughes LC, Oh D-W, Kim S-Y. Effects of Corrective Exercise for thoracic hyperkyphosis on posture, Balance, and well-being in older women: a Double-Blind, Group-Matched Design. J Geriatr Phys Ther. 2019;42:E17–27.
González-Gálvez N, Gea-García GM, Marcos-Pardo PJ. Effects of exercise programs on kyphosis and lordosis angle: a systematic review and meta-analysis. PLoS ONE. 2019;14:e0216180.
Osama M, Tassadaq N, Malik R. Effect of Muscle Energy Techniques and facet joint mobilization on spinal curvature in patients with mechanical neck pain: a pilot study. J Pak Med Assoc. 2019;1.
Park SJ, Kim SH, Kim SH. Effects of thoracic mobilization and extension Exercise on thoracic alignment and shoulder function in patients with Subacromial Impingement Syndrome: a Randomized Controlled Pilot Study. Healthcare. 2020;8:316.
Brämberg EB, Bergström G, Jensen I, Hagberg J, Kwak L. Effects of yoga, strength training and advice on back pain: a randomized controlled trial. BMC Musculoskelet Disord. 2017;18:132.
Ahmadi F, Safari Variani A, Saadatian A, Varmazyar S. The impact of 10 weeks of Pilates exercises on the thoracic and lumbar curvatures of female college students. Sport Sci Health. 2021;17:989–97.
Article Google Scholar
Lee S-M, Lee C-H, O’Sullivan D, Jung J-H, Park J-J. Clinical effectiveness of a pilates treatment for forward head posture. J Phys Ther Sci. 2016;28:2009–13.
Fathollahnejad K, Letafatkar A, Hadadnezhad M. The effect of manual therapy and stabilizing exercises on forward head and rounded shoulder postures: a six-week intervention with a one-month follow-up study. BMC Musculoskelet Disord. 2019;20:86.
Han J-T, Lee J-H, Yoon C-H. The mechanical effect of kinesiology tape on rounded shoulder posture in seated male workers: a single-blinded randomized controlled pilot study. Physiother Theory Pract. 2015;31:120–5.
Perriman DM, Scarvell JM, Hughes AR, Lueck CJ, Dear KBG, Smith PN. Thoracic hyperkyphosis: a survey of Australian physiotherapists. Physiotherapy Res Int. 2012;17:167–78.
Warneke K, Konrad A, Wilke J. The knowledge of movement experts about stretching effects: does the science reach practice? PLoS ONE. 2024;19:e0295571.
Article CAS PubMed PubMed Central Google Scholar
Withers RA, Plesh CR, Skelton DA. Does stretching of anterior structures alone, or in combination with strengthening of posterior structures, decrease hyperkyphosis and improve posture in adults? A systematic review and Meta-analysis. J Frailty Sarcopenia Falls. 2023;8:174–87.
Wager E, Wiffen PJ. Ethical issues in preparing and publishing systematic reviews. J Evid Based Med. 2011;4:130–4.
Warneke K, Lohmann LH. Revisiting the stretch-induced force deficit - A systematic review with meta-analysis of acute effects. J Sport Health Sci. 2024.
Behm DG. The Science and Physiology of Flexibility and stretching: implications and applications in Sport Performance and Health. London. UK: Routledge; 2018.
Book Google Scholar
Behm DG, Chaouachi A. A review of the acute effects of static and dynamic stretching on performance. Eur J Appl Physiol. 2011;111:2633–51.
Horsley T, Dingwall O, Sampson M. Checking reference lists to find additional studies for systematic reviews. Cochrane Database Syst Rev. 2011;2011.
de Morton NA. The PEDro Scale is a valid measure of the Methodological Quality of clinical trials: a demographic study. Aust J Physiother. 2009;55:129–33.
Maher CG, Sherrington C, Herbert RD, Moseley AM, Elkins M. Reliability of the PEDro scale for rating quality of randomized controlled trials. Phys Ther. 2003;83:713–21.
Fernández-Castilla B, Declercq L, Jamshidi L, Beretvas SN, Onghena P, van den Noortgate W. Visual representations of meta-analyses of multiple outcomes: extensions to forest plots, funnel plots, and caterpillar plots. Methodology. 2020;16:299–315.
Atkins D, Best D, Briss PA, Eccles M, Falck-Ytter Y, Flottorp S, et al. Grading quality of evidence and strength of recommendations. BMJ. 2004;328:1490.
Fu R, Gartlehner G, Grant M, Shamliyan T, Sedrakyan A, Wilt TJ, et al. Conducting quantitative synthesis when comparing medical interventions: AHRQ and the Effective Health Care Program. J Clin Epidemiol. 2011;64:1187–97.
Faraone SV. Interpreting estimates of treatment effects: implications for managed care. P T. 2008;33:700–3.
Fisher Z, Tipton E, Robumeta. An R-package for robust variance estimation in meta analysis. arXiv:1503.02220. 2015.
Fani M, Ebrahimi S, Ghanbari A. Evaluation of scapular mobilization and comparison to pectoralis minor stretching in individuals with rounded shoulder posture: a randomized controlled trial. J Bodyw Mov Ther. 2020;24:367–72.
Fukuda A, Tsushima E, Wada K, Ishibashi Y. Effects of back extensor strengthening exercises on postural alignment, physical function and performance, self-efficacy, and quality of life in Japanese community-dwelling older adults: a controlled clinical trial. Phys Ther Res. 2020;23:132–42.
Hajihosseini E, Norasteh A, Shamsi A, Daneshmandi H. The effects of strengthening, stretching and Comprehensive exercises on Forward Shoulder posture correction. Phys Treatments. 2014;4:123–32.
Google Scholar
Hamidiyeh M, Naserpour H, Chogan M. Change in Erector Spinae muscle strength and Kyphosis Angle following an eight weeks TRX training in middle-age men. Int J Aging Health Mov. 2021;3:13–20.
Hammonds ALD, Laudner KG, McCaw S, McLoda TA. Acute lower extremity running Kinematics after a Hamstring Stretch. J Athl Train [Internet]. 2012;47:5–14. Available from: www.nata.orgljat.
Hassan D, Irfan T, Butt S, Hashim M, Waseem A, Affiliation, ’. Exercise in forward head posture and rounded shoulder: stretching or strengthening? Ann Allied Health Sci [Internet]. 2022;8:3–8. Available from:. www.aahs.kmu.edu.pk .
Itoi E, Sinaki M. Effect of back-strengthening Exercise on posture in healthy women 49 to 65 years of age. Mayo Clin Proc. 1994;69:1054–9.
Katzman WB, Parimi N, Gladin A, Poltavskiy EA, Schafer AL, Long RK et al. Sex differences in response to targeted kyphosis specific exercise and posture training in community-dwelling older adults: a randomized controlled trial. BMC Musculoskelet Disord. 2017;18.
Katzman WB, Vittinghoff E, Lin F, Schafer A, Long RK, Wong S, et al. Targeted spine strengthening exercise and posture training program to reduce hyperkyphosis in older adults: results from the study of hyperkyphosis, exercise, and function (SHEAF) randomized controlled trial. Osteoporos Int. 2017;28:2831–41.
Kim S, Jung J, Kim N. The effects of McKenzie Exercise on Forward Head posture and respiratory function. J Korean Phys Therapy. 2019;31:351–7.
Lee SH, Lee JH. Effects of strengthening and stretching exercises on the forward head posture. J Int Acad Phys Therapy Res. 2016;7:1046–50.
Lee M-H, Park S-J, Kim J-S. Effects of Neck Exercise on High-School Students’ Neck–Shoulder posture. J Phys Ther Sci. 2013;25:571–4.
Li Y, McClure PW, Pratt N. The Effect of Hamstring Muscle Stretching on Standing Posture and on Lumbar and Hip Motions During Forward Bending. Phys Ther [Internet]. 1996;76:836–45. https://academic.oup.com/ptj/article/76/8/836/2633037 .
Malai S, Pichaiyongwongdee Msc S, Phd S. Immediate Effect of Hold-Relax Stretching of Iliopsoas Muscle on Transversus Abdominis Muscle Activation in Chronic Non-Specific Low Back Pain with Lumbar Hyperlordosis. Journal of medical association of Thailand [Internet]. 2015;98:S6–11. http://www.jmatonline.com .
Muyor JM, López-Miñarro PA, Casimiro AJ. Effect of stretching program in an industrial workplace on hamstring flexibility and sagittal spinal posture of adult women workers: a randomized controlled trial. J Back Musculoskelet Rehabil. 2012;25:161–9.
Nitayarak H, Charntaraviroj P. Effects of scapular stabilization exercises on posture and muscle imbalances in women with upper crossed syndrome: a randomized controlled trial. J Back Musculoskelet Rehabil. 2021;34:1031–40.
Roddey TS, Olson SL, Grant SE. The Effect of Pectoralis muscle stretching on the resting position of the scapula in persons with varying degrees of Forward Head/ Rounded Shoulder posture. J Man Manip Ther. 2002.
Rossa M, Meinar Sari G, Novembri Utomo D. The Effect of Static stretching hamstring on increasing hamstring muscle extensibility and pelvic Tilt Angle on Hamstring tightness. Int J Res Publications. 2021;86.
Shamsi MB, Shahsavari S, Safari A, Mirzaei M. A randomized clinical trial for the effect of static stretching and strengthening exercise on pelvic tilt angle in LBP patients. J Bodyw Mov Ther. 2020;24:15–20.
Sikka I, Chawla C, Seth S, Alghadir AH, Khan M. Effects of Deep Cervical Flexor Training on Forward Head Posture, Neck Pain, and Functional Status in Adolescents Using Computer Regularly. Biomed Res Int. 2020;2020.
Watt JR, Jackson K, Franz JR, Dicharry J, Evans J, Kerrigan DC. Effect of a supervised hip flexor stretching program on gait in elderly individuals. PM R. 2011;3:324–9.
Yoo W. Comparison of the effects of pectoralis muscles stretching exercise and scapular retraction strengthening exercise on forward shoulder. J Phys Ther Sci. 2018;30:584–5.
Im B, Kim Y, Chung Y, Hwang S. Effects of scapular stabilization exercise on neck posture and muscle activation in individuals with neck pain and forward head posture. J Phys Ther Sci. 2016;28:951–5.
Spring H, Schneider W, Tritschler T, Stretching. Orthopade. 1997;26:981–6.
Evans SH, Cameron MW, Burton JMichael. Hypertonia Curr Probl Pediatr Adolesc Health Care. 2017;47:161–6.
PubMed Google Scholar
Sepehri S, Sheikhhoseini R, Piri H, Sayyadi P. The effect of various therapeutic exercises on forward head posture, rounded shoulder, and hyperkyphosis among people with upper crossed syndrome: a systematic review and meta-analysis. BMC Musculoskelet Disord. 2024;25:105.
Bouvier T, Opplert J, Cometti C, Babault N. Acute effects of static stretching on muscle–tendon mechanics of quadriceps and plantar flexor muscles. Eur J Appl Physiol. 2017;117:1309–15.
Maeda N, Urabe Y, Tsutsumi S, Sakai S, Fujishita H, Kobayashi T, et al. The acute effects of static and cyclic stretching on muscle stiffness and hardness of medial gastrocnemius muscle. J Sports Sci Med. 2017;16:514–20.
Nakamura M, Ikezoe T, Takeno Y, Ichihashi N. Acute and prolonged effect of static stretching on the passive stiffness of the human gastrocnemius muscle tendon unit in vivo. J Orthop Res. 2011;29:1759–63.
Ryan ED, Beck TW, Herda TJ, Hull HR, Hartman MJ, Costa PB, et al. The Time Course of Musculotendinous stiffness responses following different durations of Passive stretching. J Orthop Sports Phys Therapy. 2008;38:632–9.
Fukaya T, Sato S, Yahata K, Yoshida R, Takeuchi K, Nakamura M. Effects of stretching intensity on range of motion and muscle stiffness: a narrative review. J Bodyw Mov Ther. 2022;32:68–76.
Wang K, McCarter R, Wright J, Beverly J, Ramirez-Mitchell R. Viscoelasticity of the sarcomere matrix of skeletal muscles. The titin-myosin composite filament is a dual-stage molecular spring. Biophys J. 1993;64:1161–77.
Wang K, McCarter R, Wright J, Beverly J, Ramirez-Mitchell R. Regulation of skeletal muscle stiffness and elasticity by titin isoforms: a test of the segmental extension model of resting tension. Proc Natl Acad Sci. 1991;88:7101–5.
Granzier H, Wu Y, Siegfried L, LeWinter M. Titin: physiological function and role in Cardiomyopathy and failure. Heart Fail Rev. 2005;10:211–23.
Linke WA. Stretching the story of titin and muscle function. J Biomech. 2023;152:111553.
Prado LG, Makarenko I, Andresen C, Krüger M, Opitz CA, Linke WA. Isoform Diversity of Giant Proteins in Relation to Passive and active Contractile properties of rabbit skeletal muscles. J Gen Physiol. 2005;126:461–80.
Nishikawa K. Titin: a tunable spring in active muscle. Physiology. 2020;35:209–17.
Freitas SR, Mendes B, Le Sant G, Andrade RJ, Nordez A, Milanovic Z. Can chronic stretching change the muscle-tendon mechanical properties? A review. Scand J Med Sci Sports. 2018;28:794–806.
Takeuchi K, Nakamura M, Konrad A, Mizuno T. Long-term static stretching can decrease muscle stiffness: a systematic review and meta‐analysis. Scand J Med Sci Sports. 2023;33:1294–306.
Zöllner AM, Abilez OJ, Böl M, Kuhl E. Stretching skeletal muscle: chronic muscle lengthening through Sarcomerogenesis. PLoS ONE. 2012;7:e45661.
Kruse A, Rivares C, Weide G, Tilp M, Jaspers RT. Stimuli for adaptations in muscle length and the length range of active force Exertion—A narrative review. Front Physiol. 2021;12.
Williams PE, Goldspink G. Changes in sarcomere length and physiological properties in immobilized muscle. J Anat. 1978;127:459–68.
CAS PubMed PubMed Central Google Scholar
van der Pijl RJ, Hudson B, Granzier-Nakajima T, Li F, Knottnerus AM, Smith J et al. Deleting Titin’s C-Terminal PEVK exons increases Passive Stiffness, alters splicing, and induces cross-sectional and longitudinal hypertrophy in skeletal muscle. Front Physiol. 2020;11.
Antonio J, Gonyea WJ. Progressive stretch overload of skeletal muscle results in hypertrophy before hyperplasia. J Appl Physiol. 1993;75:1263–71.
Alway SE. Contractile properties of aged avian muscle after stretch-overload. Mech Ageing Dev. 1994;73:97–112.
Nunes JP, Schoenfeld BJ, Nakamura M, Ribeiro AS, Cunha PM, Cyrino ES. Does stretch training induce muscle hypertrophy in humans? A review of the literature. Clin Physiol Funct Imaging. 2020;40:148–56.
Warneke K, Lohmann LH, Behm DG, Wirth K, Keiner M, Schiemann S et al. Effects of Chronic Static Stretching on Maximal Strength and Muscle Hypertrophy: A Systematic Review and Meta-Analysis. Sports Med Open. 2024;accepted.
Panidi I, Donti O, Konrad A, Petros CD, Terzis G, Mouratidis A et al. Muscle architecture adaptations to static stretching training: a systematic review with meta-analysis. Sports Med Open. 2023;9.
Apostolopoulos N, Metsios GS, Flouris AD, Koutedakis Y, Wyon MA. The relevance of stretch intensity and position—a systematic review. Front Psychol. 2015;6:1–25.
Blazevich AJ. Adaptations in the passive mechanical properties of skeletal muscle to altered patterns of use. J Appl Physiol. 2019;126:1483–91.
Wiemann K, Klee A, Startmann M. Fibrillar sources of the muscle resting tension and the therapy of muscular imbalances. Muskelphysiologie. 1998;49:111–8.
Lee BCY, McGill SM. Effect of long-term isometric training on Core/Torso stiffness. J Strength Cond Res. 2015;29:1515–26.
Dankel SJ, Razzano BM. The impact of acute and chronic resistance exercise on muscle stiffness: a systematic review and meta-analysis. J Ultrasound. 2020;23:473–80.
Schroeder J, Wilke J, Hollander K. Effects of Foam Rolling Duration on tissue stiffness and perfusion: a randomized cross-over trial. J Sports Sci Med. 2021;626–34.
Wilke J, Niemeyer P, Niederer D, Schleip R, Banzer W. Influence of Foam Rolling velocity on knee range of motion and tissue stiffness: a Randomized, controlled crossover trial. J Sport Rehabil. 2019;28:711–5.
Download references
Acknowledgements
Not applicable.
Open Access funding enabled and organized by Projekt DEAL.
Author information
Authors and affiliations.
Institute of Sport Science, Department of Movement Sciences, Alpen-Adrian-University Klagenfurt, Klagenfurt, Austria
Konstantin Warneke & Jan Wilke
Department of Human Movement Science and Exercise Physiology, Institute of Sport Science, Friedrich Schiller University, Jena, Germany
Lars Hubertus Lohmann
You can also search for this author in PubMed Google Scholar
Contributions
KW wrote the first draft, contributed in the screening of studies and performed the meta-analytic procedure. LHL contributed in study screening, quality assessment and assisted in the writing. JW supervised the project, included critical feedback and advised on statistical matters. All authors contributed to the manuscript, discussed and approved the final version.
Corresponding author
Correspondence to Lars Hubertus Lohmann .
Ethics declarations
Ethics approval and consent to participate, consent for publication, registration of the study.
The study was registered in the PROSPERO data base using the number CRD42023412854 and the title “Effects of stretching and strengthening exercise on spinal and lumbopelvic posture: a systematic review with meta-analysis”.
Competing Interests
The authors declare that they have no competing interests.
Additional information
Publisher’s note.
Springer Nature remains neutral with regard to jurisdictional claims in published maps and institutional affiliations.
Electronic Supplementary Material
Below is the link to the electronic supplementary material.
Supplementary Material 1
Rights and permissions.
Open Access This article is licensed under a Creative Commons Attribution 4.0 International License, which permits use, sharing, adaptation, distribution and reproduction in any medium or format, as long as you give appropriate credit to the original author(s) and the source, provide a link to the Creative Commons licence, and indicate if changes were made. The images or other third party material in this article are included in the article’s Creative Commons licence, unless indicated otherwise in a credit line to the material. If material is not included in the article’s Creative Commons licence and your intended use is not permitted by statutory regulation or exceeds the permitted use, you will need to obtain permission directly from the copyright holder. To view a copy of this licence, visit http://creativecommons.org/licenses/by/4.0/ .
Reprints and permissions
About this article
Cite this article.
Warneke, K., Lohmann, L.H. & Wilke, J. Effects of Stretching or Strengthening Exercise on Spinal and Lumbopelvic Posture: A Systematic Review with Meta-Analysis. Sports Med - Open 10 , 65 (2024). https://doi.org/10.1186/s40798-024-00733-5
Download citation
Received : 04 September 2023
Accepted : 22 May 2024
Published : 05 June 2024
DOI : https://doi.org/10.1186/s40798-024-00733-5
Share this article
Anyone you share the following link with will be able to read this content:
Sorry, a shareable link is not currently available for this article.
Provided by the Springer Nature SharedIt content-sharing initiative
- Muscular Imbalance
- Strengthening
- Forward head Posture
- Pelvic tilt
- Forward Shoulder
Synthesis, rietveld refinement, and microstructural characterization of bulk zinc gallium telluride
- Published: 05 June 2024
- Volume 245 , article number 99 , ( 2024 )
Cite this article
- S. D. Dhruv 1 ,
- Jayant Kolte 2 ,
- Pankaj Solanki 3 ,
- Vanaraj Solanki 4 ,
- J. H. Markna 3 ,
- Bharat Kataria 3 ,
- B. A. Amin 5 ,
- Naveen Agrawal 1 &
- D. K. Dhruv 1
Explore all metrics
After thoroughly evaluating its properties, the suggested semiconducting material for a thin film semiconductor electronic device is selected. A thorough analysis of Zinc gallium telluride (ZnGa 2 Te 4 ) in bulk can be helpful for researchers working on electronic device production. ZnGa 2 Te 4 (ZGT) is a ternary semiconducting compound that belongs to the II-III 2 -VI 4 family [II: zinc (Zn), cadmium (Cd); III: indium (In), gallium (Ga); and III: selenium (Se), tellurium (Te), sulphur (S)]. The microcontroller-based programmable rotary tube furnace synthesized homogeneous bulk ZGT ternary semiconducting compound. A vigorous XRD peak intensity and a low full breadth/width at half maximum ( β ) (FWHM) of the diffraction peak value in the synthesized ZGT bulk indicate high levels of crystallinity, which were examined using X-ray diffraction (XRD). The ZGT bulk's d -interplanar spacings (as determined by Bragg's law and Bravais theory), stacking fault (SF), texture coefficient ( C i ), degree of preferred orientation ( σ ), lattice constants ( a and c ), and unit cell volume ( V ) have all been computed. To understand the thermal and structural characteristics of ZGT, the XRD data is analyzed using the Rietveld refinement (RR) method in the Fullprof programme. Various microstructural parameters of ZGT bulk, such as lattice parameters ( a and c ), crystallite size ( D ), strain ( ε ), microstrain ( \({\varepsilon }_{\text{rms}}\) ), dislocation density ( \(\delta\) ), Young's modulus ( y h k l ), stress ( \(\sigma\) ), and energy density \((u)\) are calculated. Calculations have been made for the bulk, Voigt shear, Young's modulus, and Poisson's ratio elastic moduli of ZGT. Equipped with its transverse and longitudinal sound velocities, the ZGT bulk's Debye temperature has been calculated and depicted. The ZGT's bulk density is calculated using a pycnometer. The implications are discussed.
This is a preview of subscription content, log in via an institution to check access.
Access this article
Price includes VAT (Russian Federation)
Instant access to the full article PDF.
Rent this article via DeepDyve
Institutional subscriptions
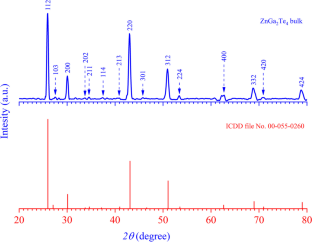
Data availability
This declaration is "Not Applicable".
Mamedova, I.A., Jahangirli, Z.A., Kerimova, T.G., Abdullayev, N.A.: Bulk Modulus, Elastic Constants, and Force Constants of Interatomic Bonds of II-III 2 -VI 4 Compounds. Physica Status Solidi (b) 260 , 2200441 (2023). https://doi.org/10.1002/pssb.202200441
Article ADS Google Scholar
Rashmi Dhawan, U.: X-ray powder diffraction study of ZnGa 2 Te 4 . Powder Diffr. 17 , 41–43 (2002). https://doi.org/10.1154/1.1424263
Fouad, S.S., Sakr, G.B., Yahia, I.S., Basset, D.M.A.: Structural characterization and novel optical properties of defect chalcopyrite ZnGa 2 Te 4 thin films. Mater. Res. Bull. 46 , 2141–2146 (2011). https://doi.org/10.1016/j.materresbull.2011.06.002
Article Google Scholar
Errandonea, D., Kumar, R.S., Gomis, O., Manjón, F.J., Ursaki, V.V., Tiginyanu, I.M.: X-ray diffraction study on pressure-induced phase transformations and the equation of state of ZnGa 2 Te 4 . J. Appl. Phys. 114 , 233507 (2013). https://doi.org/10.1063/1.4851735
Mayengbam, R., Tripathy, S.K., Palai, G., Dhar, S.S.: First-principles study of phase transition, electronic, elastic and optical properties of defect chalcopyrite ZnGa 2 Te 4 semiconductor under different pressures. J. Phys. Chem. Solids 119 , 193–201 (2018). https://doi.org/10.1016/j.jpcs.2018.03.027
Akl, A.A., El Radaf, I.M., Hassanien, A.S.: Intensive comparative study using X-Ray diffraction for investigating microstructural parameters and crystal defects of the novel nanostructural ZnGa 2 S 4 thin films. Superlattices Microstruct. 143 , 106544 (2020). https://doi.org/10.1016/j.spmi.2020.106544
Hasan, M.A.A., Jasim, K.A., Miran, H.A.J.: Synthesis and Comparative Analysis of Crystallite Size and Lattice Strain of Pb 2 Ba 1.7 Sr 0.3 Ca 2 Cu 3 O 10+δ Superconductor. Korean. J. Mater. Res. 32 , 66–71 (2022). https://doi.org/10.3740/MRSK.2022.32.2.66
Chandra, S., Sinha, A., Kumar, V.: Electronic and elastic properties of A II B 2 III C 4 VI defect-chalcopyrite semiconductors. Int. J. Mod. Phys. B 33 , 1950340 (2019). https://doi.org/10.1142/S0217979219503405
Sakr, G.B., Fouad, S.S., Yahia, I.S., Abdel Basset, D.M.: Memory switching of ZnGa 2 Te 4 thin films. J. Mater. Sci. 48 , 1134–1140 (2013). https://doi.org/10.1007/s10853-012-6850-z
Sakr, G.B., Fouad, S.S., Yahia, I.S., Abdel Basset, D.M., Yakuphanoglu, F.: Nano-crystalline p-ZnGa 2 Te 4 /n-Si as a new heterojunction diode. Mater. Res. Bull. 48 , 752–759 (2013). https://doi.org/10.1016/j.materresbull.2012.11.013
Fouad, S.S., Sakr, G.B., Yahia, I.S., Abdel-Basset, D.M., Yakuphanoglu, F.: Impedance spectroscopy of p-ZnGa 2 Te 4 /n-Si nano-HJD. Physica B 415 , 82–91 (2013). https://doi.org/10.1016/j.physb.2013.01.014
Wartak, M.S., Fong, C.-Y.: Bragg's Law. In: Field Guide to Solid State Physics. SPIE (2019)
Hahn, H., Frank, G., Klingler, W., Strger, A.D., Storger, G.: Untersuchungen uber ternare Chalkogenide. VI. Uber Ternare Chalkogenide des Aluminiums, Galliums und Indiums mit Zink, Cadmium und Quecksilber. Z Anorg Allg Chem. 279 , 241–270 (1955). https://doi.org/10.1002/zaac.19552790502
Suresh, R., Ponnuswamy, V., Mariappan, R.: Effect of annealing temperature on the microstructural, optical and electrical properties of CeO 2 nanoparticles by chemical precipitation method. Appl. Surf. Sci. 273 , 457–464 (2013). https://doi.org/10.1016/j.apsusc.2013.02.062
Choudhury, B., Choudhury, A.: Ce3 + and oxygen vacancy mediated tuning of structural and optical properties of CeO 2 nanoparticles. Mater. Chem. Phys. 131 , 666–671 (2012). https://doi.org/10.1016/j.matchemphys.2011.10.032
El-Habib, A., Addou, M., Aouni, A., Diani, M., Zimou, J., Bakkali, H.: Synthesis, structural and optical characteristics of vanadium doped cerium dioxide layers. Materialia. 18 , 101143 (2021). https://doi.org/10.1016/j.mtla.2021.101143
Zhang, M., Chen, Y., Qiu, C., Fan, X., Chen, C., Wang, Z.: Synthesis and atomic-scale characterization of CeO 2 nano-octahedrons. Physica E 64 , 218–223 (2014). https://doi.org/10.1016/j.physe.2014.08.002
Momma, K., Izumi, F.: VESTA3 for three-dimensional visualization of crystal, volumetric and morphology data. J. Appl. Crystallogr. 44 , 1272–1276 (2011). https://doi.org/10.1107/S0021889811038970
Vashistha, I., Rohilla, S.: Structural characterization and rietveld refinement of CeO 2 /CoFe 2 O 4 nanocomposites prepared via coprecipitation method. IOP Conf. Ser.: Mater. Sci. Eng. 872 , 012170 (2020). https://doi.org/10.1088/1757-899X/872/1/012170
Singh, O., Agarwal, A., Sanghi, S., Singh, J.: Variation of crystal structure, magnetization, and dielectric properties of Nd and Ba co-doped BiFeO 3 multiferroics. Int J Applied Ceramic Tech. 16 , 119–129 (2019). https://doi.org/10.1111/ijac.13052
Canchanya-Huaman, Y., Mayta-Armas, A.F., Pomalaya-Velasco, J., Bendezú-Roca, Y., Guerra, J.A., Ramos-Guivar, J.A.: Strain and Grain Size Determination of CeO 2 and TiO 2 Nanoparticles: Comparing Integral Breadth Methods versus Rietveld, μ-Raman, and TEM. Nanomaterials 11 , 2311 (2021). https://doi.org/10.3390/nano11092311
Magdalane, C.M., Kaviyarasu, K., Vijaya, J.J., Siddhardha, B., Jeyaraj, B.: Photocatalytic activity of binary metal oxide nanocomposites of CeO 2 /CdO nanospheres: Investigation of optical and antimicrobial activity. J. Photochem. Photobiol., B 163 , 77–86 (2016). https://doi.org/10.1016/j.jphotobiol.2016.08.013
Amutha, T., Rameshbabu, M., Muthupandi, S., Prabha, K.: Theoretical comparison of lattice parameter and particle size determination of pure tin oxide nanoparticles from powder X-ray diffraction. Materials Today: Proceedings. 49 , 2624–2627 (2022). https://doi.org/10.1016/j.matpr.2021.08.044
Basak, M., Rahman, Md.L., Ahmed, Md.F., Biswas, B., Sharmin, N.: The use of X-ray diffraction peak profile analysis to determine the structural parameters of cobalt ferrite nanoparticles using Debye-Scherrer, Williamson-Hall, Halder-Wagner and Size-strain plot: Different precipitating agent approach. J. Alloy. Compd. 895 , 162694 (2022). https://doi.org/10.1016/j.jallcom.2021.162694
Parveen, B., Mahmood-ul-Hassan Khalid, Z., Riaz, S., Naseem, S.: Room-temperature ferromagnetism in Ni-doped TiO 2 diluted magnetic semiconductor thin films. J. Appl. Res. Technol. 15 , 132–139 (2017). https://doi.org/10.1016/j.jart.2017.01.009
ShunmugaSundaram, P., Sangeetha, T., Rajakarthihan, S., Vijayalaksmi, R., Elangovan, A., Arivazhagan, G.: XRD structural studies on cobalt doped zinc oxide nanoparticles synthesized by coprecipitation method: Williamson-Hall and size-strain plot approaches. Physica B 595 , 412342 (2020). https://doi.org/10.1016/j.physb.2020.412342
Rabiei, M., Palevicius, A., Monshi, A., Nasiri, S., Vilkauskas, A., Janusas, G.: Comparing Methods for Calculating Nano Crystal Size of Natural Hydroxyapatite Using X-Ray Diffraction. Nanomaterials 10 , 1627 (2020). https://doi.org/10.3390/nano10091627
Monshi, A., Foroughi, M.R., Monshi, M.R.: Modified Scherrer Equation to Estimate More Accurately Nano-Crystallite Size Using XRD. WJNSE. 02 , 154–160 (2012). https://doi.org/10.4236/wjnse.2012.23020
Nath, D., Singh, F., Das, R.: X-ray diffraction analysis by Williamson-Hall, Halder-Wagner and size-strain plot methods of CdSe nanoparticles-a comparative study. Mater. Chem. Phys. 239 , 122021 (2020). https://doi.org/10.1016/j.matchemphys.2019.122021
Himabindu, B., Latha Devi, N.S.M.P., RajiniKanth, B.: Microstructural parameters from X-ray peak profile analysis by Williamson-Hall models; A review. Mater. Today: Proc. 47 , 4891–4896 (2021). https://doi.org/10.1016/j.matpr.2021.06.256
Jamal, M., JalaliAsadabadi, S., Ahmad, I., Rahnamaye Aliabad, H.A.: Elastic constants of cubic crystals. Comput. Mater. Sci. 95 , 592–599 (2014). https://doi.org/10.1016/j.commatsci.2014.08.027
Vinjamuri, K.B., Viswanadha, S., Basireddy, H., Borra, R.K.: X-Ray Diffraction Analysis by Williamson-Hall, Size-Strain, Halder-Wagner Plot Methods for Ni Doped CdS Nanoparticles. AMM. 903 , 27–32 (2021). https://doi.org/10.4028/www.scientific.net/AMM.903.27
Deshpande, M.P., Garg, N., Bhatt, S.V., Soni, B., Chaki, S.H.: Study on CdSe Nanoparticles Synthesized by Chemical Method. AMR. 665 , 267–282 (2013). https://doi.org/10.4028/www.scientific.net/AMR.665.267
Singh, S., Rath, M.C., Singh, A.K., Mukherjee, T., Jayakumar, O.D., Tyagi, A.K., Sarkar, S.K.: CdSe nanoparticles grown via radiolytic methods in aqueous solutions. Radiat. Phys. Chem. 80 , 736–741 (2011). https://doi.org/10.1016/j.radphyschem.2011.01.015
Panda, K.B., Ravi Chandran, K.S.: Determination of elastic constants of titanium diboride (TiB 2 ) from first principles using FLAPW implementation of the density functional theory. Comput. Mater. Sci. 35 , 134–150 (2006). https://doi.org/10.1016/j.commatsci.2005.03.012
Download references
Author information
Authors and affiliations.
Natubhai V. Patel College of Pure and Applied Sciences, The Charutar Vidya Mandal University, Vallabh Vidyanagar, 388120, Anand, Gujarat, India
S. D. Dhruv, Naveen Agrawal & D. K. Dhruv
School of Physics and Materials Science, Thapar Institute of Engineering and Technology, Patiala, 147004, Punjab, India
Jayant Kolte
Department of Nanoscience and Advanced Materials, Saurashtra University, Rajkot, 360005, Gujarat, India
Pankaj Solanki, J. H. Markna & Bharat Kataria
Dr. K.C. Patel R & D Centre, Charotar University of Science and Technology, Changa, 388421, Gujarat, India
Vanaraj Solanki
College of Agricultural Engineering and Technology, Anand Agricultural University, Godhra, 389001, Panchmahal, Gujarat, India
You can also search for this author in PubMed Google Scholar
Contributions
S.D. Dhruv: Data curation, Writing-Review Jayant Kolte: Resources Pankaj Solanki: Formal analysis Vanaraj Solanki: Visualization J.H. Markna: Investigation, Methodology Bharat Kataria: Project administration, Validation B.A. Amin: Editing Naveen Agrawal: Formal analysis D.K. Dhruv: Writing original draft, Conceptualization, Supervision
Corresponding author
Correspondence to D. K. Dhruv .
Ethics declarations
Ethical approval, competing interests.
The authors declare no competing interests.
Additional information
Publisher's note.
Springer Nature remains neutral with regard to jurisdictional claims in published maps and institutional affiliations.
Rights and permissions
Springer Nature or its licensor (e.g. a society or other partner) holds exclusive rights to this article under a publishing agreement with the author(s) or other rightsholder(s); author self-archiving of the accepted manuscript version of this article is solely governed by the terms of such publishing agreement and applicable law.
Reprints and permissions
About this article
Dhruv, S.D., Kolte, J., Solanki, P. et al. Synthesis, rietveld refinement, and microstructural characterization of bulk zinc gallium telluride. Hyperfine Interact 245 , 99 (2024). https://doi.org/10.1007/s10751-024-01948-4
Download citation
Accepted : 26 May 2024
Published : 05 June 2024
DOI : https://doi.org/10.1007/s10751-024-01948-4
Share this article
Anyone you share the following link with will be able to read this content:
Sorry, a shareable link is not currently available for this article.
Provided by the Springer Nature SharedIt content-sharing initiative
- ZnGa 2 Te 4
- X-ray diffraction
- Rietveld refinement
- Lattice parameters
- Crystallite size
- Dislocation density
Advertisement
- Find a journal
- Publish with us
- Track your research
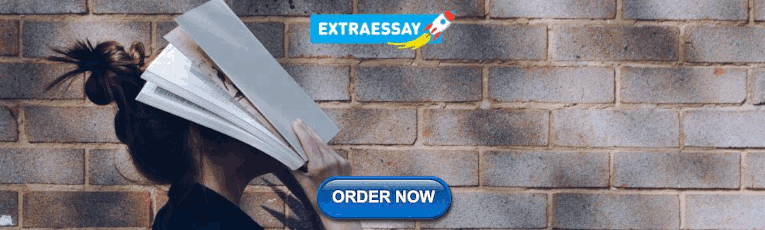
IMAGES
VIDEO
COMMENTS
We used the terms above combined with the term "method* (e.g., "realist synthesis" and "method*) in the database Health ... to narrative-interpretive reasoning. The unit of analysis for the synthesis is the unfolding "storyline" of a research tradition over time. Five key principles underlie the methodology: pragmatism, pluralism ...
12.2 Statistical synthesis when meta-analysis of effect estimates is not possible. A range of statistical synthesis methods are available, and these may be divided into three categories based on their preferability (Table 12.2.a).Preferable methods are the meta-analysis methods outlined in Chapter 10 and Chapter 11, and are not discussed in detail here.
On the other hand, synthesis involves combining different elements or ideas to create a new whole or solution. It involves integrating information from various sources, identifying commonalities and differences, and generating new insights or solutions. While analysis is more focused on understanding and deconstructing a problem, synthesis is ...
This is the Method of Analysis: and the Synthesis consists in assuming the Causes discover'd, and establish'd as Principles, and by them explaining the Phænomena proceeding from them, and proving the Explanations. (Opticks, Book Three, Part I, 404-5) Nietzsche, Friedrich
The range of different methods for synthesising qualitative research has been growing over recent years [1, 2], alongside an increasing interest in qualitative synthesis to inform health-related policy and practice [].While the terms 'meta-analysis' (a statistical method to combine the results of primary studies), or sometimes 'narrative synthesis', are frequently used to describe how ...
Meta-analysis is the quantitative, scientific synthesis of research results. Since the term and modern approaches to research synthesis were first introduced in the 1970s, meta-analysis has had a ...
3 Cauchy and the continuum: the significance of non-standard analysis for the history and philosophy of mathematics (edited by J. P. Cleave) 4 What does a mathematical proof prove? 5 The method of analysis-synthesis
Typically, meta-synthesis research begins with research questions, which drive the entire process, including: who is a member of the research team, the type of synthesis study conducted, how broad or narrow the search for literature is, the analysis methods (if collecting and analyzing ethnographic studies, analysis methods used in ethnography ...
Authors can select from a range of analysis and synthesis methods. Tables 1.1 and 1.2 from Chapter 1 presented examples. The myriad of analysis and synthesis practices vary in the breadth of their coverage. Some methods, such as meta-analyses and thematic summaries, focus specifically on data manipulation. Other procedures, such as meta ...
This document aims to assist authors in planning their narrative analysis at protocol stage, and to highlight some issues for authors to consider at review stage. Narrative forms of synthesis are an area of emerging research, and so advice is likely to be adapted as methods develop. This document sits alongside the RevMan templates for ...
Analysis. First published Mon Apr 7, 2003; substantive revision Wed Mar 19, 2014. Analysis has always been at the heart of philosophical method, but it has been understood and practised in many different ways. Perhaps, in its broadest sense, it might be defined as a process of isolating or working back to what is more fundamental by means of ...
Steps taken in grounded theory meta-synthesis of qualitative research. The figure displays the five consecutive steps for the use of grounded theory during meta-synthesis. The first step is the extraction of data from retrieved studies. This is followed by the analysis of these data using memos and open codes.
Research Synthesis Methods, the official journal of the Society for Research Synthesis Methodology, is a multidisciplinary peer reviewed journal devoted to the development and dissemination of methods for designing, conducting, analyzing, interpreting, reporting, and applying systematic research synthesis.It aims to facilitate the creation and exchange of knowledge about research synthesis ...
Analysis by Synthesis: Mumford & Grenander • Grenander (1960's) had proposed that vision could be formulated as pattern theory and proposed the idea of "analysis by synthesis". This is naturally expressed in Bayesian terms. (S. Geman was a student of Grenander). • Mumford embraced Analysis by Synthesis and Pattern Theory.
Quantitative synthesis, or meta-analysis, is often essential for Comparative Effective Reviews (CERs) to provide scientifically rigorous summary information. Quantitative synthesis should be conducted in a transparent and consistent way with methodologies reported explicitly. This guide provides practical recommendations on conducting synthesis. The guide is not meant to be a textbook on meta ...
Once you have completed your analysis, you will want to both summarize and synthesize those results. You may have a qualitative synthesis, a quantitative synthesis, or both. Qualitative Synthesis. In a qualitative synthesis, you describe for readers how the pieces of your work fit together.
Analysis is the first step towards synthesis, which requires not only thinking critically and investigating a topic or source, but combining thoughts and ideas to create new ones. As you synthesize, you will draw inferences and make connections to broader themes and concepts. It's this step that will really help add substance, complexity, and ...
The analysis in interpretive synthesis is conceptual both in process and outcome, and "the product is not aggregations of data, but theor y" [49 , p.12]. Interpretive syntheses involve
The book discusses the main interpretations of the classical distinction between analysis and synthesis with respect to mathematics. In the first part, this is discussed from a historical point of view, by considering different examples from the history of mathematics. In the second part, the question is considered from a philosophical point of view, and some new interpretations are proposed.
Analysis + Synthesis = Design Thinking. Analysis and synthesis, thus, form the two fundamental tasks to be done in design thinking. Design thinking process starts with reductionism, where the problem statement is broken down into smaller fragments. Each fragment is brainstormed over by the team of thinkers, and the different smaller solutions ...
Some teachers call this method a "quote sandwich" because you put your evidence between two slices of your own language and interpretation. ... my analysis and synthesis require my intellectual discretion. 4 Mays 1258.Mays, Kelly J. "The Literature Essay." The Norton Introduction to Literature, Portable 12th edition, Norton, 2017, pp ...
Abstract. Data analysis is a challenging stage of the integrative review process as it requires the reviewer to synthesize data from diverse methodological sources. Although established approaches to data analysis and synthesis of integrative review findings continue to evolve, adherence to systematic methods during this stage is essential to ...
1 Summary. Research synthesis is a set of related methods that integrate the ndings of separate empirical. studies. It is a tool for understanding a body of literature and characteristics that ...
The Future of Research Synthesis. Research synthesis exists at a fascinating time, with new tools and technologies emerging faster than news cycles can cover them. Frameworks like Method's own Journey Map Kit are gaining traction because they offer a robust structure for creating large-scale deliverables. Transcription tools allow researchers ...
Framework analysis was developed for applied policy research but has increasingly been adapted as a method for evidence synthesis (Brunton et al., Citation 2020; Ritchie & Spencer, Citation 2002). Based on the steps proposed by Ritchie and Spencer ( Citation 2002 ) the lead author first familiarized herself with the studies, focussing on ...
Thus, we optimized a single-step method for obtaining 1-(1-isocyanoethyl)adamantane 2 with a yield of 92% by reacting the amine with chloroform and t-BuOK in a dichloromethane and tert-butanol (1:1) mixture.The developed synthesis method has several advantages. Firstly, the reaction proceeds in a single step, significantly reducing labor costs.
Abnormal posture (e.g. loss of lordosis) has been associated with the occurrence of musculoskeletal pain. Stretching tight muscles while strengthening the antagonists represents the most common method to treat the assumed muscle imbalance. However, despite its high popularity, there is no quantitative synthesis of the available evidence examining the effectiveness of the stretch-and-strengthen ...
The SSP method considers the XRD line analysis a heterogeneous function involving Lorentzian and Gaussian components, ... Hasan, M.A.A., Jasim, K.A., Miran, H.A.J.: Synthesis and Comparative Analysis of Crystallite Size and Lattice Strain of Pb 2 Ba 1.7 Sr 0.3 Ca 2 Cu 3 O 10+ ...