Click through the PLOS taxonomy to find articles in your field.
For more information about PLOS Subject Areas, click here .
Loading metrics
Open Access
Peer-reviewed
Research Article
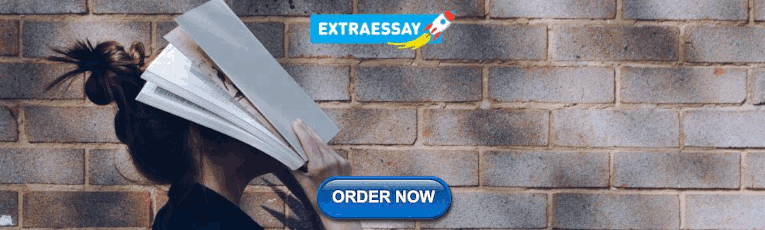
Differentiating the learning styles of college students in different disciplines in a college English blended learning setting
Roles Conceptualization, Formal analysis, Funding acquisition, Methodology, Supervision, Writing – original draft, Writing – review & editing
* E-mail: [email protected]
Affiliations Department of Linguistics, School of International Studies, Zhejiang University, Hangzhou City, Zhejiang Province, China, Center for College Foreign Language Teaching, Zhejiang University, Hangzhou City, Zhejiang Province, China, Institute of Asian Civilizations, Zhejiang University, Hangzhou City, Zhejiang Province, China

Roles Formal analysis, Project administration, Writing – review & editing
Affiliation Department of Linguistics, School of International Studies, Zhejiang University, Hangzhou City, Zhejiang Province, China
Roles Formal analysis, Writing – original draft
Roles Writing – review & editing
- Jie Hu,
- Yi Peng,
- Xueliang Chen,
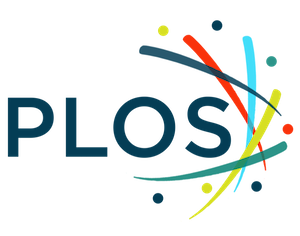
- Published: May 20, 2021
- https://doi.org/10.1371/journal.pone.0251545
- Peer Review
- Reader Comments
Learning styles are critical to educational psychology, especially when investigating various contextual factors that interact with individual learning styles. Drawing upon Biglan’s taxonomy of academic tribes, this study systematically analyzed the learning styles of 790 sophomores in a blended learning course with 46 specializations using a novel machine learning algorithm called the support vector machine (SVM). Moreover, an SVM-based recursive feature elimination (SVM-RFE) technique was integrated to identify the differential features among distinct disciplines. The findings of this study shed light on the optimal feature sets that collectively determined students’ discipline-specific learning styles in a college blended learning setting.
Citation: Hu J, Peng Y, Chen X, Yu H (2021) Differentiating the learning styles of college students in different disciplines in a college English blended learning setting. PLoS ONE 16(5): e0251545. https://doi.org/10.1371/journal.pone.0251545
Editor: Haoran Xie, Lingnan University, HONG KONG
Received: May 15, 2020; Accepted: April 29, 2021; Published: May 20, 2021
Copyright: © 2021 Hu et al. This is an open access article distributed under the terms of the Creative Commons Attribution License , which permits unrestricted use, distribution, and reproduction in any medium, provided the original author and source are credited.
Data Availability: All relevant data are within the paper and its Supporting Information files.
Funding: This research was supported by the Philosophical and Social Sciences Planning Project of Zhejiang Province in 2020 [grant number 20NDJC01Z] with the recipient Jie Hu, Second Batch of 2019 Industry-University Collaborative Education Project of Chinese Ministry of Education [grant number 201902016038] with the recipient Jie Hu, SUPERB College English Action Plan with the recipient Jie Hu, and the Fundamental Research Funds for the Central Universities of Zhejiang University with the recipient Jie Hu.
Competing interests: The authors have declared that no competing interests exist.
Introduction
Research background.
Learning style, as an integral and vital part of a student’s learning process, has been constantly discussed in the field of education and pedagogy. Originally developed from the field of psychology, psychological classification, and cognitive research several decades ago [ 1 ], the term “learning style” is generally defined as the learner’s innate and individualized preference for ways of participation in learning practice [ 2 ]. Theoretically, learning style provides a window into students’ learning processes [ 3 , 4 ], predicts students’ learning outcomes [ 5 , 6 ], and plays a critical role in designing individualized instruction [ 7 ]. Knowing a student’s learning style and personalizing instruction to students’ learning style could enhance their satisfaction [ 8 ], improve their academic performance [ 9 ], and even reduce the time necessary to learn [ 10 ].
Researchers in recent years have explored students’ learning styles from various perspectives [ 11 – 13 ]. However, knowledge of the learning styles of students from different disciplines in blended learning environments is limited. In an effort to address this gap, this study aims to achieve two major objectives. First, it investigates how disciplinary background impacts students’ learning styles in a blended learning environment based on data collected in a compulsory college English course. Students across 46 disciplines were enrolled in this course, providing numerous disciplinary factor resources for investigating learning styles. Second, it introduces a novel machine learning method named the SVM to the field of education to identify an optimal set of factors that can simultaneously differentiate students of different academic disciplines. Based on data for students from 46 disciplines, this research delves into the effects of a massive quantity of variables related to students’ learning styles with the help of a powerful machine learning algorithm. Considering the convergence of a wide range of academic disciplines and the detection of latent interactions between a large number of variables, this study aims to provide a clear picture of the relationship between disciplinary factors and students’ learning styles in a blended learning setting.
Literature review
Theories of learning styles..
Learning style is broadly defined as the inherent preferences of individuals as to how they engage in the learning process [ 2 ], and the “cognitive, affective and physiological traits” of students have received special attention [ 14 ]. To date, there has been a proliferation of learning style definitions proposed to explain people’s learning preferences, each focusing on different aspects. Efforts to dissect learning style have been contested, with some highlighting the dynamic process of the learner’s interaction with the learning environment [ 14 ] and others underlining the individualized ways of information processing [ 15 ]. One vivid explication involved the metaphor of an onion, pointing out the multilayer nature of learning styles. It was proposed that the outermost layer of the learning style could change in accordance with the external environment, while the inner layer is relatively stable [ 16 , 17 ]. In addition, a strong concern in this field during the last three decades has led to a proliferation of models that are germane to learning styles, including the Kolb model [ 18 ], the Myers-Briggs Type Indicator model [ 19 ] and the Felder-Silverman learning style model (FSLSM) [ 20 ]. These learning style models have provided useful analytical lenses for analyzing students’ learning styles. The Kolb model focuses on learners’ thinking processes and identifies four types of learning, namely, diverging, assimilating, converging, and accommodating [ 18 ]. The Myers-Briggs Type Indicator model classifies learners into extraversion and introversion types, with the former preferring to learn from interpersonal communication and the latter inclining to benefit from personal experience [ 19 ]. As the most popular available model, the FSLSM identifies eight categories of learners according to the four dimensions of perception, input, processing and understanding [ 20 ]. In contrast to other learning style models that divided students into only a few groups, the FSLSM describes students’ learning styles in a more detailed manner. The four paired dimensions delicately distinguish students’ engagement in the learning process, providing a solid basis for a steady and reliable learning style analysis [ 21 ]. In addition, it has been argued that the FSLSM is the most appropriate model for a technology-enhanced learning environment because it involves important theories of cognitive learning behaviors [ 22 , 23 ]. Therefore, a large number of scholars have based their investigations of students’ learning styles in the e-learning/computer-aided learning environment on FSLSM [ 24 – 28 ].
Learning styles and FSLSM.
Different students receive, process, and respond to information with different learning styles. A theoretical model of learning style can be used to categorize people according to their idiosyncratic learning styles. In this study, the FSLSM was adopted as a theoretical framework to address the collective impacts of differences in students’ learning styles across different disciplines (see Fig 1 ).
- PPT PowerPoint slide
- PNG larger image
- TIFF original image
This model specifies the four dimensions of the construct of learning style: visual/verbal, sensing/intuitive, active/reflective, and sequential/global. These four dimensions correspond to four psychological processes: input, perception, processing, and understanding.
https://doi.org/10.1371/journal.pone.0251545.g001
The FSLSM includes learning styles scattered among four dimensions.
Visual learners process information best when it is presented as graphs, pictures, etc., while verbal learners prefer spoken cues and remember best what they hear. Sensory learners like working with facts, data, and experimentation, while intuitive learners prefer abstract principles and theories. Active learners like to try things and learn through experimentation, while reflective learners prefer to think things through before taking action. Sequential learners absorb knowledge in a linear fashion and make progress step by step, while global learners tend to grasp the big picture before filling in all the details.
Learning styles and academic disciplines.
Learning styles vary depending on a series of factors, including but not limited to age [ 29 ], gender [ 30 ], personality [ 2 , 31 ], learning environment [ 32 ] and learning experience [ 33 ]. In the higher education context, the academic discipline seems to be an important variable that influences students’ distinctive learning styles, which echoes a multitude of investigations [ 29 , 34 – 41 ]. One notable study explored the learning styles of students from 4 clusters of disciplines in an academic English language course and proposed that the academic discipline is a significant predictor of students’ learning styles, with students from the soft-pure, soft-applied, hard-pure and hard-applied disciplines each favoring different learning modes [ 42 ]. In particular, researchers used the Inventory of Learning Styles (ILS) questionnaire and found prominent disparities in learning styles between students from four different disciplinary backgrounds in the special educational field of vocational training [ 43 ]. These studies have found significant differences between the learning styles of students from different academic disciplines, thus supporting the concept that learning style could be domain dependent.
Learning styles in an online/blended learning environment.
Individuals’ learning styles reflect their adaptive orientation to learning and are not fixed personality traits. Consequently, learning styles can vary among diverse contexts, and related research in different contexts is vital to understanding learning styles in greater depth. Web-based technologies eliminate barriers of space and time and have become integrated in individuals’ daily lives and learning habits. Online and blended learning have begun to pervade virtually every aspect of the education landscape [ 40 ], and this warrants close attention. In addition to a series of studies that reflected upon the application of information and communication technology in the learning process [ 44 , 45 ], recent studies have found a mixed picture of whether students in a web-based/blended learning environment have a typical preference for learning.
Online learning makes it possible for students to set their goals and develop an individualized study plan, equipping them with more learning autonomy [ 46 ]. Generally, students with a more independent learning style, greater self-regulating behavior and stronger self-efficacy are found to be more successful in an online environment [ 47 ]. For now, researchers have made substantial contributions to the identification and prediction of learning styles in an online learning environment [ 27 , 48 – 51 ]. For instance, an inspiring study focused on the manifestation of college students’ learning styles in a purely computer-based learning environment to evaluate the different learning styles of web-learners in the online courses, indicating that students’ learning styles were significantly related to online participation [ 49 ]. Students’ learning styles in interactive E-learning have also been meticulously investigated, from which online tutorials have been found to be contributive to students’ academic performance regardless of their learning styles [ 51 ].
As a flexible learning method, blended courses have combined the advantages of both online learning and traditional teaching methods [ 52 ]. Researchers have investigated students’ learning styles within this context and have identified a series of prominent factors, including perceived satisfaction and technology acceptance [ 53 ], the dynamics of the online/face-to-face environment [ 54 ], and curriculum design [ 55 ]. Based on the Visual, Aural, Reading or Write and Kinesthetic model, a comprehensive study scrutinized the learning styles of K12 students in a blended learning environment, elucidating the effect of the relationship between personality, learning style and satisfaction on educational outcomes [ 56 ]. A recent study underscored the negative effects of kinesthetic learning style, whereas the positive effects of visual or auditory learning styles on students’ academic performance, were also marked in the context of blended learning [ 57 ].
Considering that academic disciplines and learning environment are generally regarded as essential predictors of students’ learning styles, some studies have also concentrated on the effects of academic discipline in a blended learning environment. Focusing on college students’ learning styles in a computer-based learning environment, an inspiring study evaluated the different learning styles of web learners, namely, visual, sensing, global and sequential learners, in online courses. According to the analysis, compared with students from other colleges, liberal arts students, are more susceptible to the uneasiness that may result from remote teaching because of their learning styles [ 11 ]. A similar effort was made with the help of the CMS tool usage logs and course evaluations to explore the learning styles of disciplinary quadrants in the online learning environment. The results indicated that there were noticeable differences in tool preferences between students from different domains [ 12 ]. In comparison, within the context of blended learning, a comprehensive study employed chi-square statistics on the basis of the Community of Inquiry (CoI) presences framework, arguing that soft-applied discipline learners in the blended learning environment prefer the kinesthetic learning style, while no correlations between the learning style of soft-pure and hard-pure discipline students and the CoI presences were identified. However, it is noted that students’ blended learning experience depends heavily on academic discipline, especially for students in hard-pure disciplines [ 13 ].
Research gaps and research questions
Overall, the research seems to be gaining traction, and new perspectives are continually introduced. The recent literature on learning styles mostly focuses on the exploration of the disciplinary effects on the variation in learning styles, and some of these studies were conducted within the blended environment. However, most of the studies focused only on several discrete disciplines or included only a small group of student samples [ 34 – 41 ]. Data in these studies were gathered through specialized courses such as academic English language [ 42 ] rather than the compulsory courses available to students from all disciplines. Even though certain investigations indeed boasted a large number of samples [ 49 ], the role of teaching was emphasized rather than students’ learning style. In addition, what is often overlooked is that a large number of variables related to learning styles could distinguish students from different academic disciplines in a blended learning environment, whereas a more comprehensive analysis that takes into consideration the effects of a great quantity of variables related to learning styles has remained absent. Therefore, one goal of the present study is to fill this gap and shed light on this topic.
Another issue addressed in this study is the selection of an optimal measurement that can effectively identify and differentiate individual learning styles [ 58 ]. The effective identification and differentiation of individual learning styles can not only help students develop greater awareness of their learning but also provide teachers with the necessary input to design tailor-made instructions in pedagogical practice. Currently, there are two general approaches to identify learning styles: a literature-based approach and a data-driven approach. The literature-based approach tends to borrow established rules from the existing literature, while the data-driven approach tends to construct statistical models using algorithms from fields such as machine learning, artificial intelligence, and data mining [ 59 ]. Research related to learning styles has been performed using predominantly traditional instruments, such as descriptive statistics, Spearman’s rank correlation, coefficient R [ 39 ], multivariate analysis of variance [ 56 ] and analysis of variance (ANOVA) [ 38 , 43 , 49 , 57 ]. Admittedly, these instruments have been applied and validated in numerous studies, in different disciplines, and across multiple timescales. Nevertheless, some of the studies using these statistical tools did not identify significant results [ 36 , 53 , 54 ] or reached only loose conclusions [ 60 ]; this might be because of the inability of these methods to probe into the synergistic effects of variables. However, the limited functions of comparison, correlation, prediction, etc. are being complemented by a new generation of technological innovations that promise more varied approaches to addressing social and scientific issues. Machine learning is one such approach that has received much attention both in academia and beyond. As a subset of artificial intelligence, machine learning deals with algorithms and statistical models on computer systems, performing tasks based on patterns and inference instead of explicit instruction. As such, it can deal with high volumes of data at the same time, perform tasks automatically and independently, and continuously improve its performance based on past experience [ 54 ]. Similar machine learning approaches have been proposed and tested by different scholars to identify students’ learning styles, with varying results regarding the classification of learning styles. For instance, a study that examined the precision levels of four computational intelligence approaches, i.e., artificial neural network, genetic algorithm, ant colony system and particle swarm optimization, found that the average precision of learning style differentiation ranged between 66% and 77% [ 61 ]. Another study that classified learning styles through SVM reported accuracy levels ranging from 53% to 84% [ 62 ]. A comparison of the prediction performance of SVM and artificial neural networks found that SVM has higher prediction accuracy than the latter [ 63 ]. This was further supported by another study, which yielded a similar result between SVM and the particle swarm optimization algorithm [ 64 ]. Moreover, when complemented by a genetic algorithm [ 65 ] and ant colony system [ 66 ], SVM has also shown improved results. These findings across different fields point to the reliability of SVM as an effective statistical tool for identification and differentiation analysis.
Therefore, a comprehensive investigation across the four general disciplines in Biglan’s taxonomy using a strong machine learning approach is needed. Given the existence of the research gaps discussed above, this exploratory study seeks to address the following questions:
- Can students’ learning styles be applied to differentiate various academic disciplines in the blended learning setting? If so, what are the differentiability levels among different academic disciplines based on students’ learning styles?
- What are the key features that can be selected to determine the collective impact on differentiation by a machine learning algorithm?
- What are the collective impacts of optimal feature sets?
Materials and methods
This study adopted a quantitative approach for the analysis. First, a modified and translated version of the original ILS questionnaire was administered to collect scores for students’ learning styles. Then, two alternate data analyses were performed separately. One analysis involved a traditional ANOVA, which tested the main effect of discipline on students’ learning styles in each ILS dimension. The other analysis involved the support vector machine (SVM) technique to test its performance in classifying students’ learning styles in the blended learning course among 46 specializations. Then, SVM-based recursive feature elimination (SVM-RFE) was employed to specify the impact of students’ disciplinary backgrounds on their learning styles in blended learning. By referencing the 44 questions (operationalized as features in this study) in the ILS questionnaire, SVM-RFE could rank these features based on their relative importance in differentiating different disciplines and identify the key features that collectively differentiate the students’ learning style. These steps are intended to not only identify students’ learning style differences but also explain such differences in relation to their academic disciplinary backgrounds.
Participants
The participants included 790 sophomores taking the blended English language course from 46 majors at Z University. Sophomore students were selected for this study for two reasons. First, sophomores are one of the only two groups of students (the other group being college freshmen) who take a compulsory English language course, namely, the College English language course. Second, of these two groups of students, sophomores have received academic discipline-related education, while their freshmen counterparts have not had disciplinary training during the first year of college. In the College English language course, online activities, representing 55% of the whole course, include e-course teaching designed by qualified course teachers or professors, courseware usage for online tutorials, forum discussion and essay writing, and two online quizzes. Offline activities, which represent 45% of the whole course, include role-playing, ice-breaker activities, group presentations, an oral examination, and a final examination. Therefore, the effects of the academic discipline on sophomores’ learning styles might be sufficiently salient to warrant a comparison in a blended learning setting [ 67 ]. Among the participants, 420 were male, and 370 were female. Most participants were aged 18 to 19 years and had taken English language courses for at least 6 years. Based on Biglan’s typology of disciplinary fields, the students’ specializations were classified into the four broad disciplines of hard-applied (HA, 289/37.00%), hard-pure (HP, 150/19.00%), soft-applied (SA, 162/20.00%), and soft-pure (SP, 189/24.00%).
Biglan’s classification scheme of academic disciplines (hard (H) vs. soft (S) disciplines and pure (P) vs. applied (A) disciplines) has been credited as the most cited organizational system of academic disciplines in tertiary education [ 68 – 70 ]. Many studies have also provided evidence supporting the validity of this classification [ 69 ]. Over the years, research has indicated that Biglan’s typology is correlated with differences in many other properties and serves as an appropriate mechanism to organize discipline-specific knowledge or epistemologies [ 38 ] and design and deliver courses for students with different learning style preferences [ 41 ]. Therefore, this classification provides a convenient framework to explore differences across disciplinary boundaries. In general, HA disciplines include engineering, HP disciplines include the so-called natural sciences, SA disciplines include the social sciences, and SP disciplines include the humanities [ 41 , 68 , 71 ].
In learning style research, it is difficult to select an instrument to measure the subjects’ learning styles [ 72 ]. The criteria used for the selection of a learning style instrument in this study include the following: 1) successful use of the instrument in previous studies, 2) demonstrated validity and reliability, 3) a match between the purpose of the instrument and the aim of this study and 4) open access to the questionnaire.
The Felder and Soloman’s ILS questionnaire, which was built based on the FSLSM, was adopted in the present study to investigate students’ learning styles across different disciplines. First, the FSLSM is recognized as the most commonly used model for measuring individual learning styles on a general scale [ 73 ] in higher education [ 74 ] and has remained popular for many years across different disciplines in university settings and beyond. In the age of personalized instruction, this model has breathed new life into areas such as blended learning [ 75 ], online distance learning [ 76 ], courseware design [ 56 ], and intelligent tutoring systems [ 77 , 78 ]. Second, the FSLSM is based on previous learning style models; the FSLSM integrates all their advantages and is, thus, more comprehensive in delineating students’ learning styles [ 79 , 80 ]. Third, the FSLSM has a good predictive ability with independent testing sets (i.e., unknown learning style objects) [ 17 ], which has been repeatedly proven to be a more accurate, reliable, and valid model than most other models for predicting students’ learning performance [ 10 , 80 ]. Fourth, the ILS is a free instrument that can be openly accessed online (URL: https://www.webtools.ncsu.edu/learningstyles/ ) and has been widely used in the research context [ 81 , 82 ].
The modified and translated version of the original ILS questionnaire includes 44 questions in total, and 11 questions correspond to each dimension of the Felder-Silverman model as follows: questions 1–11 correspond to dimension 1 (active vs. reflective), questions 12–22 correspond to dimension 2 (sensing vs. intuitive), questions 23–33 correspond to dimension 3 (visual vs. verbal), and questions correspond 34–44 to dimension 4 (sequential vs. global). Each question is followed by five choices on a five-point Likert scale ranging from “strongly agree with A (1)”, “agree with A (2)”, “neutral (3)”, “agree with B (4)” and “strongly agree with B (5)”. Option A and option B represent the two choices offered in the original ILS questionnaire.
Ethics statements
The free questionnaires were administered in a single session by specialized staff who collaborated on the investigation. The participants completed all questionnaires individually. The study procedures were in accordance with the ethical standards of the Helsinki Declaration and were approved by the Ethics Committee of the School of International Studies, Zhejiang University. All participants signed written informed consent to authorize their participation in this research. After completion of the informed consent form, each participant was provided a gift (a pen) in gratitude for their contribution and participation.
Data collection procedure
Before the questionnaires were distributed, the researchers involved in this study contacted faculty members from various departments and requested their help. After permission was given, the printed questionnaires were administered to students under the supervision of their teachers at the end of their English language course. The students were informed of the purpose and importance of the study and asked to carefully complete the questionnaires. The students were also assured that their personal information would be used for research purposes only. All students provided written informed consent (see S2 File ). After the questionnaires were completed and returned, they were thoroughly examined by the researchers such that problematic questionnaires could be identified and excluded from further analysis. All questionnaires eligible for the data analysis had to meet the following two standards: first, all questions must be answered, and second, the answered questions must reflect a reasonable logic. Regarding the few missing values, the median number of a given individual’s responses on 11 questions per dimension included in the ILS questionnaire was used to fill the void in each case. In statistics, using the median number to impute missing values is common and acceptable because missing values represent only a small minority of the entire dataset and are assumed to not have a large impact on the final results [ 83 , 84 ].
In total, 850 questionnaires were administered to the students, and 823 of these questionnaires were retrieved. Of the retrieved questionnaires, the remaining 790 questionnaires were identified as appropriate for further use. After data screening, these questionnaires were organized, and their respective results were translated into an Excel format.
Data analysis method
During the data analysis, as a library of the SVM, the free package LIBSVM ( https://www.csie.ntu.edu.tw/~cjlin/libsvm/ ) was first applied as an alternative method of data analysis. Then, a traditional ANOVA was performed to examine whether there was a main effect of academic discipline on Chinese students’ learning styles. ANOVA could be performed using SPSS, a strong data analysis software that supports a series of statistical analyses. In regard to the examination of the effect of a single or few independent variables, SPSS ANOVA can produce satisfactory results. However, SVM, a classic data mining algorithm, outperforms ANOVA for dataset in which a large number of variables with multidimensions are intertwined and their combined/collective effects influence the classification results. In this study, the research objective was to efficiently differentiate and detect the key features among the 44 factors. Alone, a single factor or few factors might not be significant enough to discriminate the learning styles among the different disciplines. Selected by the SVM, the effects of multiple features may collectively enhance the classification performance. Therefore, the reason for selecting SVM over ANOVA is that in the latter case, the responses on all questions in a single dimension are summed instead of treated as individual scores; thus, the by-item variation is concealed. In addition, the SVM is especially suitable for statistical analysis with high-dimensional factors (usually > 10; 44-dimensional factors were included in this study) and can detect the effects collectively imposed by a feature set [ 85 ].
Originally proposed in 1992 [ 86 ], the SVM is a supervised learning model related to machine learning algorithms that can be used for classification, data analysis, pattern recognition, and regression analysis. The SVM is an efficient classification model that optimally divides data into two categories and is ranked among the top methods in statistical theory due to its originality and practicality [ 85 ]. Due to its robustness, accurate classification, and prediction performance [ 87 – 89 ], the SVM has high reproducibility [ 90 , 91 ]. Due to the lack of visualization of the computing process of the SVM, the SVM has been described as a “black box” method [ 92 ]; however, future studies in the emerging field of explainable artificial intelligence can help solve this problem and convert this approach to a “glass box” method [ 67 ]. This algorithm has proven to have a solid theoretical foundation and excellent empirical application in the social sciences, including education [ 93 ] and natural language processing [ 94 ]. The mechanism underlying the SVM is also presented in Fig 2 .
Hyperplanes 1 and 2 are two regression lines that divide the data into two groups. Hyperplane 1 is considered the best fitting line because it maximizes the distance between the two groups.
https://doi.org/10.1371/journal.pone.0251545.g002
The SVM contains the following two modules: one module is a general-purpose machine learning method, and the other module is a domain-specific kernel function. The SVM training algorithm is used to build a training model that is then used to predict the category to which a new sample instance belongs [ 95 ]. When a set of training samples is given, each sample is given the label of one of two categories. To evaluate the performance of SVM models, a confusion matrix, which is a table describing the performance of a classifier on a set of test data for which the true values are known, is used (see Table 1 ).
https://doi.org/10.1371/journal.pone.0251545.t001
ACC represents the proportion of true results, including both positive and negative results, in the selected population;
SPE represents the proportion of actual negatives that are correctly identified as such;
SEN represents the proportion of actual positives that are correctly identified as such;
AUC is a ranking-based measure of classification performance that can distinguish a randomly chosen positive example from a randomly chosen negative example; and
F-measure is the harmonic mean of precision (another performance indicator) and recall.
The ACC is a good metric frequently applied to indicate the measurement of classification performance, but the combination of the SPE, SEN, AUC, F-measure and ACC may be a measure of enhanced performance assessment and was frequently applied in current studies [ 96 ]. In particular, the AUC is a good metric frequently applied to validate the measurement of the general performance of models [ 97 ]. The advantage of this measure is that it is invariant to relative class distributions and class-specific error costs [ 98 , 99 ]. Moreover, to some extent, the AUC is statistically consistent and more discriminating than the ACC with balanced and imbalanced real-world data sets [ 100 ], which is especially suitable for unequal samples, such as the HA-HP model in this study. After all data preparations were completed, the data used for the comparisons were extracted separately. First, the processed data of the training set were run by using optimized parameters. Second, the constructed model was used to predict the test set, and the five indicators of the fivefold cross-validation and fivefold average were obtained. Cross-validation is a general validation procedure used to assess how well the results of a statistical analysis generalize to an independent data set, which is used to evaluate the stability of the statistical model. K-fold cross-validation is commonly used to search for the best hyperparameters of SVM to achieve the highest accuracy performance [ 101 ]. In particular, fivefold, tenfold, and leave-one-out cross-validation are typically used versions of k-fold cross-validation [ 102 , 103 ]. Fivefold cross-validation was selected because fivefold validation can generally achieve a good prediction performance [ 103 , 104 ] and has been commonly used as a popular rule of thumb supported by empirical evidence [ 105 ]. In this study, five folds (groups) of subsets were randomly divided from the entire set by the SVM, and four folds (training sample) of these subsets were randomly selected to develop a prediction model, while the remaining one fold (test sample) was used for validation. The above functions were all implemented with Python Programming Language version 3.7.0 (URL: https://www.python.org/ ).
Then, SVM-RFE, which is an embedded feature selection strategy that was first applied to identify differentially expressed genes between patients and healthy individuals [ 106 ], was adopted. SVM-RFE has proven to be more robust to data overfitting than other feature selection techniques and has shown its power in many fields [ 107 ]. This approach works by removing one feature each time with the smallest weight iteratively to a feature rank until a group of highly weighted features were selected. After this feature selection procedure, several SVM models were again constructed based on these selected features. The performance of the new models is compared to that of the original models with all features included. The experimental process is provided in Fig 3 for the ease of reference.
https://doi.org/10.1371/journal.pone.0251545.g003
The classification results produced by SVM and the ranking of the top 20 features produced by SVM-RFE were listed in Table 2 . Twenty variables have been selected in this study for two reasons: a data-based reason and a literature-based reason. First, it is clear that models composed of 20 features generally have a better performance than the original models. The performance of models with more than 20 is negatively influenced. Second, SVM-based studies in the social sciences have identified 20 to 30 features as a good number for an optimal feature set [ 108 ], and 20 features were selected for inclusion in the optimal feature set [ 95 ]. Therefore, in this study, the top 20 features were selected for subsequent analysis, as proposed in previous analyses that yielded accepted measurement rates. These 20 features retained most of the useful information from all 44 factors but with fewer feature numbers, which showed satisfactory representation [ 96 ].
https://doi.org/10.1371/journal.pone.0251545.t002
Results of RQ (1) What are the differentiability levels among different academic disciplines based on students’ learning styles?
To further measure the performance of the differentiability among students’ disciplines, the collected data were examined with the SVM algorithm. As shown in Table 2 , the five performance indicators, namely, the ACC, SPE, SEN, AUC and F-measure, were utilized to measure the SVM models. Regarding the two general performance indicators, i.e., the ACC value and AUC value, the HA-HP, HA-SA, and HA-SP-based models yielded a classification capacity of approximately 70.00%, indicating that the students in these disciplines showed a relatively large difference. In contrast, the models based on the H-S, A-P, HP-SA, HP-SP, and SA-SP disciplines only showed a moderate classification capacity (above 55.00%). This finding suggests that these five SVM models were not as effective as the other three models in differentiating students among these disciplines based on their learning styles. The highest ACC and AUC values were obtained in the model based on the HA-HP disciplines, while the lowest values were obtained in the model based on the HP-SA disciplines. As shown in Table 2 , the AUCs of the different models ranged from 57.76% (HP-SA) to 73.97% (HA-HP).
To compare the results of the SVM model with another statistical analysis, an ANOVA was applied. Prior to the main analysis, the students’ responses in each ILS dimension were summed to obtain a composite score. All assumptions of ANOVA were checked, and no serious violations were observed. Then, an ANOVA was performed with academic discipline as the independent variable and the students’ learning styles as the dependent variable. The results of the ANOVA showed that there was no statistically significant difference in the group means of the students’ learning styles in Dimension 1, F(3, 786) = 2.56, p = .054, Dimension 2, F(3, 786) = 0.422, p = .74, or Dimension 3, F(3, 786) = 0.90, p = .443. However, in Dimension 4, a statistically significant difference was found in the group means of the students’ learning styles, F (3, 786) = 0.90, p = .005. As the samples in the four groups were unbalanced, post hoc comparisons using Scheffé’s method were performed, demonstrating that the means of the students’ learning styles significantly differed only between the HA (M = 31.04, SD = 4.986) and SP (M = 29.55, SD = 5.492) disciplines, 95.00% CI for MD [0.19, 2.78], p = .016, whereas the other disciplinary models showed no significant differences. When compared with the results obtained from the SVM models, the three models (HA-HP, HA-SA, and HA-SP models) presented satisfactory differentiability capability of approximately 70.00% based on the five indicators.
In the case of a significant result, it was difficult to determine which questions were representative of the significant difference. With a nonsignificant result, it was possible that certain questions might be relevant in differentiating the participants. However, this problem was circumvented in the SVM, where each individual question was treated as a variable and a value was assigned to indicate its relative importance in the questionnaire. Using SVM also circumvented the inherent problems with traditional significance testing, especially the reliance on p-values, which might become biased in the case of multiple comparisons [ 109 ].
Results of RQ (2) What are the key features that can be selected to determine the collective impact on differentiation by a machine learning algorithm?
To examine whether the model performance improved as a result of this feature selection procedure, the 20 selected features were submitted to another round of SVM analysis. The same five performance indicators were used to measure the model performance (see Table 2 ). By comparing the performance of the SVM model and that of the SVM-RFE model presented in Table 2 , except for the HA-SP model, all other models presented a similar or improved performance after the feature selection process. In particular, the improvement in the HA-HP and HP-SA models was quite remarkable. For instance, in the HA-HP model, the ACC value increased from 69.32% in the SVM model to 82.59% in the SVM-RFE model, and the AUC score substantially increased from 73.97% in the SVM model to 89.13% in the SVM-RFE model. This finding suggests that the feature selection process refined the model’s classification accuracy and that the 20 features selected, out of all 44 factors, carry substantive information that might be informative for exploring disciplinary differences. Although results for the indicators of the 20 selected features were not very high, all five indicators above 65.00% showed that the model was still representative because only 20 of 44 factors could present the classification capability. Considering that there was a significant reduction in the number of questions used for the model construction in SVM-RFE (compared with those used for the SVM model), the newly identified top 20 features by SVM-RFE were effective enough to preserve the differential ability of all 44 questions. Thus, these newly identified top 20 factors could be recognized as key differential features for distinguishing two distinct disciplines.
To identify these top 20 features in eight models (see Table 2 ), SVM-RFE was applied to rank order all 44 features contained in the ILS questionnaire. To facilitate a detailed understanding of what these features represent, the questions related to the top 20 features in the HA-HP model are listed in Table 3 for ease of reference.
https://doi.org/10.1371/journal.pone.0251545.t003
Results of RQ (3) What are the collective impacts of optimal feature sets?
The collective impacts of optimal feature sets could be interpreted from four aspects, namely, the complexities of students’ learning styles, the appropriate choice of SVM, the ranking of SVM-RFE and multiple detailed comparisons between students from different disciplines. First, the FSLSM considers the fact that students’ learning styles are shaped by a series of factors during the growth process, which intertwine and interact with each other. Considering the complex dynamics of the learning style, selecting an approach that could detect the combined effects of a group of variables is needed. Second, recent years have witnessed the emergence of data mining approaches to explore students learning styles [ 28 , 48 – 50 , 110 ]. Specifically, as one of the top machine learning algorithms, the SVM excels in identifying the combined effects of high-order factors [ 87 ]. In this study, the SVM has proven to perform well in classifying students’ learning styles across different disciplines, with every indicator being acceptable. Third, the combination of SVM with RFE could enable the simultaneous discovery of multiple features that collectively determine classification. Notably, although SVM-FRE could rank the importance of the features, they should be regarded as an entire optimal feature set. In other words, the combination of these 20 features, rather than a single factor, could differentiate students’ learning styles across different academic disciplines. Last but not least, the multiple comparisons between different SVM models of discipline provide the most effective learning style factors, giving researchers clues to the nuanced differences between students’ learning styles. It can be seen that students from different academic disciplines understand, see and reflect things from individualized perspectives. The 20 most effective factors for all models scattered within 1 to 44, verifying students’ different learning styles in 4 dimensions. Therefore, the FSLSM provides a useful and effective tool for evaluating students’ learning styles from a rather comprehensive point of view.
The following discussions address the three research questions explored in the current study.
Levels of differentiability among various academic disciplines based on students’ learning styles with SVM
The results suggest that SVM is an effective approach for classification in the blended learning context in which students with diverse disciplinary backgrounds can be distinguished from each other according to their learning styles. All performance indicators presented in Tables 2 and 3 remain above the baseline of 50.00%, suggesting that between each two disciplines, students’ learning style differences can be identified. To some extent, these differences can be identified with a relatively satisfactory classification capability (e.g., 69.32% of the ACC and 73.97% of the AUC in the HA-HP model shown in Table 2 ). Further support for the SVM algorithm is obtained from the SVM-RFE constructed to assess the rank of the factors’ classification capacity, and all values also remained above the baseline value, while some values reached a relatively high classification capability (e.g., 82.59% of the ACC and 89.13% of the AUC in the HA-HP model shown in Table 2 ). While the results obtained mostly show a moderate ACC and AUC, they still provide some validity evidence supporting the role of SVM as an effective binary classifier in the educational context. However, while these differences are noteworthy, the similarities among students in different disciplines also deserve attention. The results reported above indicate that in some disciplines, the classification capacity is not relatively high; this was the case for the model based on the SA-SP disciplines.
Regarding low differentiability, one explanation might be the indistinct classification of some emerging “soft disciplines.” It was noted that psychology, for example, could be identified as “a discipline that can be considered predominantly ‘soft’ and slightly ‘purer’ than ‘applied’ in nature” [ 111 ] (p. 43–53), which could have blurred the line between the SA and SP disciplines. As there is now no impassable gulf separating the SA and SP disciplines, their disciplinary differences may have diminished in the common practice of lecturing in classrooms. Another reason comes from the different cultivation models of “soft disciplines” and “hard disciplines” for sample students. In their high school, sample students are generally divided into liberal art students and science students and are then trained in different environments of knowledge impartation. The two-year unrelenting and intensive training makes it possible for liberal art students to develop a similar thinking and cognitive pattern that is persistent. After the college entrance examination, most liberal art students select SA or SP majors. However, a year or more of study in university does not exert strong effects on their learning styles, which explains why a multitude of researchers have traditionally investigated the SA and SP disciplines together, calling them simply “social science” or “soft disciplines” compared with “natural science” or “hard disciplines”. There have been numerous contributions pointing out similarities in the learning styles of students from “soft disciplines” [ 37 , 112 – 114 ]. However, students majoring in natural science exhibit considerable differences in learning styles, demonstrating that the talent cultivation model of “hard disciplines” in universities is to some extent more influential on students’ learning styles than that of the “soft disciplines”. Further compelling interpretations of this phenomenon await only the development of a sufficient level of accumulated knowledge among scholars in this area.
In general, these results are consistent with those reported in many previous studies based on the Felder-Silverman model. These studies tested the precision of different computational approaches in identifying and differentiating the learning styles of students. For example, by means of a Bayesian network (BN), an investigation obtained an overall precision of 58.00% in the active/reflective dimension, 77.00% in the sensing/intuitive dimension and 63.00% in the sequential/global dimension (the visual/verbal dimension was not considered) [ 81 ]. With the help of the keyword attributes of learning objects selected by students, a precision of 70.00% in the active/reflective dimension, 73.30% in the sensing/intuitive dimension, 73.30% in the sequential/global dimension and 53.30% in the visual/verbal dimension was obtained [ 115 ].
These results add to a growing body of evidence expanding the scope of the application of the SVM algorithm. Currently, the applications of the SVM algorithm still reside largely in engineering or other hard disciplines despite some tentative trials in the humanities and social sciences [ 26 ]. In addition, as cross-disciplines increase in current higher education, it is essential to match the tailored learning styles of students and researchers studying interdisciplinary subjects, such as the HA, HP, SA and SP disciplines. Therefore, the current study is the first to incorporate such a machine learning algorithm into interdisciplinary blended learning and has broader relevance to further learning style-related theoretical or empirical investigations.
Verification of the features included in the optimal feature sets
Features included in the optimal feature sets provided mixed findings compared with previous studies. Some of the 20 identified features are verified and consistent with previous studies. A close examination of the individual questions included in the feature sets can offer some useful insights into the underlying psychological processes. For example, in six of the eight models constructed, Question 1 (“I understand something better after I try it out/think it through”) appears as the feature with the number 1 ranking, highlighting the great importance attached to this question. This question mainly reflects the dichotomy between experimentation and introspection. A possible revelation is that students across disciplines dramatically differ in how they process tasks, with the possible exception of the SA-SP disciplines. This difference has been supported by many previous studies. For example, it was found that technical students tended to be more tactile than those in the social sciences [ 116 ], and engineering students (known as HA in this study) were more inclined toward concrete and pragmatic learning styles [ 117 ]. Similarly, it was explored that engineering students prefer “a logical learning style over visual, verbal, aural, physical or solitary learning styles” [ 37 ] (p. 122), while social sciences (known as SA in this study) students prefer a social learning style to a logical learning style. Although these studies differ in their focus to a certain degree, they provide an approximate idea of the potential differences among students in their relative disciplines. In general, students in the applied disciplines show a tendency to experiment with tasks, while those in the pure disciplines are more inclined towards introspective practices, such as an obsession with theories. For instance, in Biglan’s taxonomy of academic disciplines, students in HP disciplines prefer abstract rules and theories, while students in SA disciplines favor application [ 67 ]. Additionally, Question 10 (“I find it easier to learn facts/to learn concepts”) is similar to Question 1, as both questions indicate a certain level of abstraction or concreteness. The difference between facts and concepts is closely related to the classification difference between declarative knowledge and procedural knowledge in cognitive psychology [ 35 , 38 ]. Declarative knowledge is static and similar to facts, while procedural knowledge is more dynamic and primarily concerned with operational steps. Students’ preferences for facts or concepts closely correspond to this psychological distinction.
In addition, Questions 2, 4, 7, and 9 also occur frequently in the 20 features selected for the different models. Question 2 (“I would rather be considered realistic/innovative”) concerns taking chances. This question reflects a difference in perspective, i.e., whether the focus should be on obtaining pragmatic results or seeking original solutions. This difference cannot be easily connected to the disciplinary factor. Instead, there are numerous factors, e.g., genetic, social and psychological factors, that may play a strong role in defining this trait. The academic discipline only serves to strengthen or diminish this difference. For instance, decades of research in psychology have shown that males are more inclined towards risk taking than females [ 118 – 121 ]. A careful examination of the current academic landscape reveals a gender difference; more females choose soft disciplines than males, and more males choose hard disciplines than females. This situation builds a disciplinary wall classifying students into specific categories, potentially strengthening the disciplinary effect. For example, Question 9 (“In a study group working on difficult material, I am more likely to jump in and contribute ideas/sit back and listen”) emphasizes the distinction between active participation and introspective thinking, reflecting an underlying psychological propensity in blended learning. Within this context, the significance of this question could also be explained by the psychological evaluation of “loss and gain”, as students’ different learning styles are associated with expected reward values and their internal motivational drives, which are determined by their personality traits [ 122 ]. When faced with the risk of “losing face”, whether students will express their ideas in front of a group of people depends largely on their risk and stress management capabilities and the presence of an appropriate motivation system.
The other two questions also convey similar messages regarding personality differences. Question 4 concerns how individuals perceive the world, while Question 7 concerns the preferred modality of information processing. Evidence of disciplinary differences in these respects was also reported [ 35 , 123 – 125 ]. The other questions, such as Questions 21, 27, and 39, show different aspects of potential personality differences and are mostly consistent with the previous discussion. This might also be a vivid reflection of the multi-faceted effects of blended learning, which may differ in their consonance with the features of each discipline. First, teachers from different domains use technology in different ways, and student from different disciplines may view blended learning differently. For instance, the characteristics of soft-applied fields entail specialized customization in blended courses, further broadening the gulf between different subjects [ 126 ]. Second, although blended learning is generally recognized as a stimulus to students’ innovation [ 127 ], some students who are used to an instructivist approach in which the educator acts as a ‘sage on the stage’ will find it difficult to adapt to a social constructivist approach in which the educator serves as a ‘guide on the side’ [ 128 ]. This difficulty might not only negatively affect students’ academic performance but also latently magnify the effects of different academic disciplines.
Interpretation of the collective impact of optimal feature sets
In each SVM model based on a two-discipline model, the 20 key features (collectively known as an optimal feature set) selected exert a concerted effect on students’ learning styles across different disciplines (see Table 2 ). A broad examination of the distribution of collective impact of each feature set with 20 features in the eight discipline models suggests that it is especially imperative considering the emerging cross-disciplines in academia. Current higher education often involves courses with crossed disciplines and students with diverse disciplinary backgrounds. In addition, with the rise of technology-enhanced learning, the design of personalized tutoring systems requires more nuanced information related to student attributes to provide greater adaptability [ 59 ]. By identifying these optimal feature sets, such information becomes accessible. Therefore, understanding such interdisciplinary factors and designing tailor-made instructions are essential for promoting learning success [ 9 ]. For example, in an English language classroom in which the students are a blend of HP and SP disciplines, instructors might consider integrating a guiding framework at the beginning of the course and stepwise guidelines during the process such that the needs of both groups are met. With the knowledge that visual style is dominant across disciplines, instructors might include more graphic presentations (e.g., Question 11) in language classrooms rather than continue to use slides or boards filled with words. Furthermore, to achieve effective communication with students and deliver effective teaching, instructors may target these students’ combined learning styles. While some methods are already practiced in real life, this study acts as a further reminder of the rationale underlying these practices and thus increases the confidence of both learners and teachers regarding these practices. Therefore, the practical implications of this study mainly concern classroom teachers and educational researchers, who may draw some inspiration for interdisciplinary curriculum design and the tailored application of learning styles to the instructional process.
Conclusions
This study investigated learning style differences among students with diverse disciplinary backgrounds in a blended English language course based on the Felder-Silverman model. By introducing a novel machine learning algorithm, namely, SVM, for the data analysis, the following conclusions can be reached. First, the multiple performance indicators used in this study confirm that it is feasible to apply learning styles to differentiate various disciplines in students’ blended learning processes. These disciplinary differences impact how students engage in their blended learning activities and affect students’ ultimate blended learning success. Second, some questions in the ILS questionnaire carry more substantive information about students’ learning styles than other questions, and certain underlying psychological processes can be derived. These psychological processes reflect students’ discipline-specific epistemologies and represent the possible interaction between the disciplinary background and learning style. In addition, the introduction of SVM in this study can provide inspiration for future studies of a similar type along with the theoretical significance of the above findings.
Despite the notable findings of this study, it is subject to some limitations that may be perfected in further research. First, the current analysis examined the learning styles without allowing for the effects of other personal or contextual factors. The educational productivity model proposed by Walberg underlines the significance of the collected influence of contextual factors on individuals’ learning [ 129 ]. For example, teachers from different backgrounds and academic disciplines are inclined to select various teaching methods and to create divergent learning environments [ 130 ], which should also be investigated thoroughly. The next step is therefore to take into account the effects of educational background, experience, personality and learning experience to gain a more comprehensive understanding of students’ learning process in the blended setting.
In conclusion, the findings of this research validate previous findings and offer new perspectives on students’ learning styles in a blended learning environment, which provides future implications for educational researchers, policy makers and educational practitioners (i.e., teachers and students). For educational researchers, this study not only highlights the merits of using machine learning algorithms to explore students’ learning styles but also provides valuable information on the delicate interactions between blended learning, academic disciplines and learning styles. For policy makers, this analysis provides evidence for a more inclusive but personalized educational policy. For instance, in addition to learning styles, the linkage among students’ education in different phases should be considered. For educational practitioners, this study plays a positive role in promoting student-centered and tailor-made teaching. The findings of this study can help learners of different disciplines develop a more profound understanding of their blended learning tendencies and assist teachers in determining how to bring students’ learning styles into full play pedagogically, especially in interdisciplinary courses [ 131 – 134 ].
Supporting information
https://doi.org/10.1371/journal.pone.0251545.s001
S2 File. Informed consent for participants.
https://doi.org/10.1371/journal.pone.0251545.s002
S1 Dataset.
https://doi.org/10.1371/journal.pone.0251545.s003
Acknowledgments
The authors would like to thank the anonymous reviewers for their constructive comments on this paper and Miss Ying Zhou for her suggestions during the revision on this paper.
- View Article
- Google Scholar
- 15. Dunn R, Dunn K, Perrin J. Teaching young children through their individual learning styles. Boston, MA: Allyn & Bacon, Inc;1994.
- 17. Curry L. Integrating concepts of cognitive or learning style: A review with attention to psychometric standards. Ottawa, ON: Canadian College of Health Service Executives;1987.
- 18. Kolb DA. Experiential learning: experience as the source of learning and development. Englewood Cliffs, NJ: Prentice-Hall;1984.
- 21. Anitha D, Deisy C, Lakshmi SB, Meenakshi MK. Proposing a Classification Methodology to Reduce Learning Style Combinations for Better Teaching and Learning. 2014 IEEE Sixth International Conference on Technology for Education, Amritapuri, India, 2014; 208–211. https://doi.org/10.1109/T4E.2014.5
- 23. Kuljis J, Liu F. A comparison of learning style theories on the suitability for e-learning. Web Technologies, Applications, and Services, 2005; 191–197. Retrieved from: https://www.mendeley.com/catalogue/da014340-bfb5-32d1-b144-73545a86d440/
- 30. Richardson JTE. Researching student learning. Buckingham: SRHE and Open University Press; 2000.
- 33. Marton F, Säljö R. Approaches to learning. Edinburgh: Scottish Academic Press; 1984.
- PubMed/NCBI
- 52. Thorne K. Blended learning: How to integrate online and traditional learning, London: Kogan Page; 2003.
- 59. Graf S. Adaptivity in learning management systems focusing on learning styles. Vienna, Austria: Vienna University of Technology;2007.
- 79. Crockett K, Latham A, Mclean D, Bandar Z, O’Shea J. On predicting learning styles in conversational intelligent tutoring systems using fuzzy classification trees. IEEE International Conference on Fuzzy Systems. 2011; 2481–2488. https://doi.org/10.1109/FUZZY.2011.6007514
- 82. Coffield, Ecclestone K, Moseley, Hall E. Learning styles and pedagogy in post 16 education: A critical and systematic review. London, UK: Learning and Skills Research Centre;2004.
- 86. Acuna E, Rodriguez C. The Treatment of Missing Values and its Effect on Classifier Accuracy. In Banks D, House L, McMorris FR, Arabie P, Gaul W, editors. Classification, Clustering, and Data Mining Applications. Springer Berlin Heidelberg;2004. p. 639–647.
- 88. Boser BE, Guyon IM, Vapnik VN. A training algorithm for optimal margin classifiers. A training algorithm for optimal margin classifiers. Proceedings of The Fifth Annual Workshop on Computational Learning Theory. New York: ACM Press, 1992: 144–152. https://doi.org/10.1145/130385.130401
- 100. Maloof MA. Learning when data sets are imbalanced and when costs are unequal and unknown. Proceedings of the 20th International Conference on Machine Learning (ICML-2003). 2003. Retrieved from: http://www.site.uottawa.ca/~nat/Workshop2003/maloof-icml03-wids.pdf
- 101. Yan L, Rodier R, Mozer M, Wolniewicz R. Optimizing classifier performance via the Wilcoxon-Mann-Withney statistics. Proceedings of the 20th International Conference on Machine Learning (ICML-2003). 2003. Retrieved from: http://citeseerx.ist.psu.edu/viewdoc/download?doi=10.1.1.324.1091&rep=rep1&type=pdf
- 105. James G, Witten D, Hastie T, Tibshirani R. An introduction to statistical learning: with applications in R (Springer Texts in Statistics). 1st ed. New York, NY: Springer Verlag;2013.
- 118. Anderson JR. Language, memory, and thought. Mahwah, NJ: Lawrence Erlbaum Associates;1976.
- 119. Anderson JR. The architecture of cognition. Cambridge, Massachusetts: Harvard University Press;1983.
Academia.edu no longer supports Internet Explorer.
To browse Academia.edu and the wider internet faster and more securely, please take a few seconds to upgrade your browser .
Enter the email address you signed up with and we'll email you a reset link.
- We're Hiring!
- Help Center
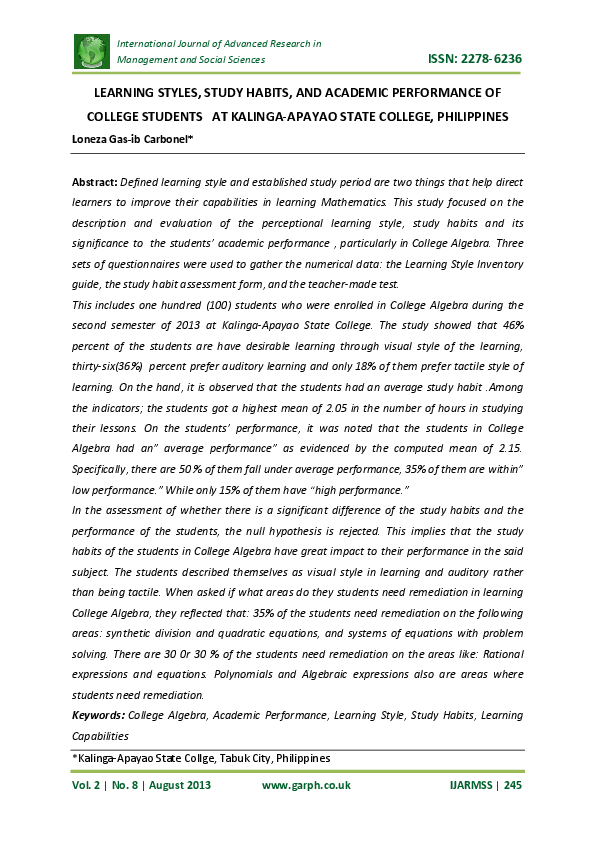
LEARNING STYLES, STUDY HABITS, AND ACADEMIC PERFORMANCE OF COLLEGE STUDENTS AT KALINGA-APAYAO STATE COLLEGE, PHILIPPINES Loneza Gas-ib Carbonel* INTRODUCTION

Related Papers
Journal of Education and Practice
Lina S Calucag
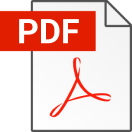
School Science and Mathematics
Robert Reys
Zenodo (CERN European Organization for Nuclear Research)
Francis Jose Bearneza
Psychology and Education: A Multidisciplinary Journal
Psychology and Education , Joan Alendajao
During the twentieth century, the meaning of successful Mathematics learning underwent several shifts in response to changes in both society and schooling. For roughly the first half of the century, success in learning the mathematics of pre-kindergarten to eighth grade usually meant facility in using the computational procedures of arithmetic, with many educators emphasizing the need for skilled performance and others emphasizing the need for students to learn procedures with understanding. To this end, the researchers is conducting a study to identify academic predictors of learning proficiency across strands of mathematics of grade 8 students of a public secondary school in Marikina City, the Marikina High School. The study is descriptive in nature and makes use of 6 groups of subjects: they were composed of 42 Grade 8 of Marikina High School, a secondary public school in the Schools Division of Marikina City, Metro Manila. From the research findings, it can be concluded that the majority of the respondents got a high rating in Algebra and Geometry, most of the student respondents fell above the passing rate of the subject Mathematics, and most of the student respondents accumulated a high score rating in Statistics and Probability. The null hypothesis is rejected; therefore, there is a significant difference between students' proficiency levels in Mathematics along with Algebra and Geometry. In academic characteristics related to the proficiency level of the students along study strategy in terms of Rehearsal, analyzing its contents again and again in studying math materials is strongly agreed by the student-respondents; Organization, reading through the class notes textbook and finding out the most important parts is strongly agreed; Self-regulation, listing down related formula first is strongly agreed; Study Environment, making it a hobby to study Math at a fixed time is agreed; Attitude towards Mathematics, student-respondents treat Mathematics difficult but an interesting subject. There is no significant difference in the Academic Characteristics of the respondents except in terms of Attitude towards Mathematics and Time and Study Environment, which have a strong positive/negative difference in the academic characteristics of the respondents. Moreover, a significant relationship exists between academic characteristics and students' proficiency levels.
Abdul Taram
This study is based on students' low learning outcomes, low learning preparation, and lack of optimization of learning style math learning causes less effective mathematics study. This research aims to determine whether there is influence toward math learning outcomes among students who have a high or low learning preparation and learning style visual, auditorial, or kinesthetic in the students Class VII of State Junior High School (SMP Negeri) 1 Banjarmangu Banjarnegara Regency in Even Semester Academic Year 2017/2018. This research population was the students of class VII SMP Negeri 1 Banjarmangu Banjarnegara Regency in even semester academic year 2017/2018, which consists of 7 classes. With Random Sampling Technique to the class and obtained class VII G as a class of research sample and class VII F as a class of research trial. Data collection techniques used a questionnaire form and test. A multiple-choice test is a matter of learning mathematics outcomes in the form of a qu...
Jurnal Magister Pendidikan Matematika (JUMADIKA)
Louise M Saija
This descriptive and comparative study aims to see the students learning styles and mathematics achievements. The respondent are fifty junior high school students in Lembang, West Bandung, Indonesia. The research instruments are Perceptual Learning Style Preference Questionnaire (PLSPQ) and mathematics problem solving test. The result of this study are: (1) Only twenty percent of the students have exactly one learning style and most of the students has kinesthetic learning style; (2) Students who have three or less major learning styles perform good mathematics achievement, and students who have minor learning styles only, perform better mathematics achievements averagely, comparing with students who have major learning styles; (3) Students perform better mathematics achievement when they have more than one learning style, (4) There is no significant difference in the students’ mathematics achievement, between students who have three or less major learning styles and students ...
Nelviyanti Podilito
wigati indah sari
The purpose of the research was to determine how far the influence of the ability of students’ mathematical understanding learning style. The subjects in this study were 140 11th grade students of SMK Bina Am-Mak'mur Tangerang Regency Year 2019/2020 which was classified into three learning style groups, which was visual learning style consisting of 32 students, auditorial consisting of 23 students, and kinesthetic consisting of 85 students. The method in this study was ex post facto to know clearly the data on students’ ability of mathematical understanding learning style. The data were taken and processed using the normality test and homogeneity test with Anava One Way, to determine the influence of students ' learning style more clearly. After the test, it can be concluded that the average value of the results of the mathematical understanding of students with visual learning style was better than the average value of the results of the mathematical understanding of studen...
Enrique B Picardal Jr
The Normal Lights
Rene Belecina
The study aimed to determine and describe the cognitive processes and learning styles of 12 eight graders in performing problem-solving tasks. The cognitive processes of students with different learning styles were studied in terms of the cognitive behaviors they manifested as they were given problem-solving tasks. These cognitive processes were understanding the tasks, specializing, generalizing, conjecturing, justifying, and looking back. Forty-one of the original 75 students from two sections of a government-owned secondary school in Quezon City were classified as active, reflective, sequential, and global, based on learning styles as revealed by the Felder and Soloman’s Index of Learning Styles Questionnaire. Consequently, three students from each learning style were randomly chosen as participants of the study. The three phases of the study involved audio taping the students while doing the problem-solving activities and interviewing them afterwards, coding all the transcribe...
RELATED TOPICS
- We're Hiring!
- Help Center
- Find new research papers in:
- Health Sciences
- Earth Sciences
- Cognitive Science
- Mathematics
- Computer Science
- Academia ©2024
- Open access
- Published: 11 May 2024
Nursing students’ stressors and coping strategies during their first clinical training: a qualitative study in the United Arab Emirates
- Jacqueline Maria Dias 1 ,
- Muhammad Arsyad Subu 1 ,
- Nabeel Al-Yateem 1 ,
- Fatma Refaat Ahmed 1 ,
- Syed Azizur Rahman 1 , 2 ,
- Mini Sara Abraham 1 ,
- Sareh Mirza Forootan 1 ,
- Farzaneh Ahmad Sarkhosh 1 &
- Fatemeh Javanbakh 1
BMC Nursing volume 23 , Article number: 322 ( 2024 ) Cite this article
470 Accesses
Metrics details
Understanding the stressors and coping strategies of nursing students in their first clinical training is important for improving student performance, helping students develop a professional identity and problem-solving skills, and improving the clinical teaching aspects of the curriculum in nursing programmes. While previous research have examined nurses’ sources of stress and coping styles in the Arab region, there is limited understanding of these stressors and coping strategies of nursing students within the UAE context thereby, highlighting the novelty and significance of the study.
A qualitative study was conducted using semi-structured interviews. Overall 30 students who were undergoing their first clinical placement in Year 2 at the University of Sharjah between May and June 2022 were recruited. All interviews were recorded and transcribed verbatim and analyzed for themes.
During their first clinical training, nursing students are exposed to stress from different sources, including the clinical environment, unfriendly clinical tutors, feelings of disconnection, multiple expectations of clinical staff and patients, and gaps between the curriculum of theory classes and labatories skills and students’ clinical experiences. We extracted three main themes that described students’ stress and use of coping strategies during clinical training: (1) managing expectations; (2) theory-practice gap; and (3) learning to cope. Learning to cope, included two subthemes: positive coping strategies and negative coping strategies.
Conclusions
This qualitative study sheds light from the students viewpoint about the intricate interplay between managing expectations, theory practice gap and learning to cope. Therefore, it is imperative for nursing faculty, clinical agencies and curriculum planners to ensure maximum learning in the clinical by recognizing the significance of the stressors encountered and help students develop positive coping strategies to manage the clinical stressors encountered. Further research is required look at the perspective of clinical stressors from clinical tutors who supervise students during their first clinical practicum.
Peer Review reports
Nursing education programmes aim to provide students with high-quality clinical learning experiences to ensure that nurses can provide safe, direct care to patients [ 1 ]. The nursing baccalaureate programme at the University of Sharjah is a four year program with 137 credits. The programmes has both theoretical and clinical components withs nine clinical courses spread over the four years The first clinical practicum which forms the basis of the study takes place in year 2 semester 2.
Clinical practice experience is an indispensable component of nursing education and links what students learn in the classroom and in skills laboratories to real-life clinical settings [ 2 , 3 , 4 ]. However, a gap exists between theory and practice as the curriculum in the classroom differs from nursing students’ experiences in the clinical nursing practicum [ 5 ]. Clinical nursing training places (or practicums, as they are commonly referred to), provide students with the necessary experiences to ensure that they become proficient in the delivery of patient care [ 6 ]. The clinical practicum takes place in an environment that combines numerous structural, psychological, emotional and organizational elements that influence student learning [ 7 ] and may affect the development of professional nursing competencies, such as compassion, communication and professional identity [ 8 ]. While clinical training is a major component of nursing education curricula, stress related to clinical training is common among students [ 9 ]. Furthermore, the nursing literature indicates that the first exposure to clinical learning is one of the most stressful experiences during undergraduate studies [ 8 , 10 ]. Thus, the clinical component of nursing education is considered more stressful than the theoretical component. Students often view clinical learning, where most learning takes place, as an unsupportive environment [ 11 ]. In addition, they note strained relationships between themselves and clinical preceptors and perceive that the negative attitudes of clinical staff produce stress [ 12 ].
The effects of stress on nursing students often involve a sense of uncertainty, uneasiness, or anxiety. The literature is replete with evidence that nursing students experience a variety of stressors during their clinical practicum, beginning with the first clinical rotation. Nursing is a complex profession that requires continuous interaction with a variety of individuals in a high-stress environment. Stress during clinical learning can have multiple negative consequences, including low academic achievement, elevated levels of burnout, and diminished personal well-being [ 13 , 14 ]. In addition, both theoretical and practical research has demonstrated that increased, continual exposure to stress leads to cognitive deficits, inability to concentrate, lack of memory or recall, misinterpretation of speech, and decreased learning capacity [ 15 ]. Furthermore, stress has been identified as a cause of attrition among nursing students [ 16 ].
Most sources of stress have been categorized as academic, clinical or personal. Each person copes with stress differently [ 17 ], and utilizes deliberate, planned, and psychological efforts to manage stressful demands [ 18 ]. Coping mechanisms are commonly termed adaptation strategies or coping skills. Labrague et al. [ 19 ] noted that students used critical coping strategies to handle stress and suggested that problem solving was the most common coping or adaptation mechanism used by nursing students. Nursing students’ coping strategies affect their physical and psychological well-being and the quality of nursing care they offer. Therefore, identifying the coping strategies that students use to manage stressors is important for early intervention [ 20 ].
Studies on nursing students’ coping strategies have been conducted in various countries. For example, Israeli nursing students were found to adopt a range of coping mechanisms, including talking to friends, engaging in sports, avoiding stress and sadness/misery, and consuming alcohol [ 21 ]. Other studies have examined stress levels among medical students in the Arab region. Chaabane et al. [ 15 ], conducted a systematic review of sudies in Arab countries, including Saudi Arabia, Egypt, Jordan, Iraq, Pakistan, Oman, Palestine and Bahrain, and reported that stress during clinical practicums was prevalent, although it could not be determined whether this was limited to the initial clinical course or occurred throughout clinical training. Stressors highlighted during the clinical period in the systematic review included assignments and workload during clinical practice, a feeling that the requirements of clinical practice exceeded students’ physical and emotional endurance and that their involvement in patient care was limited due to lack of experience. Furthermore, stress can have a direct effect on clinical performance, leading to mental disorders. Tung et al. [ 22 ], reported that the prevalence of depression among nursing students in Arab countries is 28%, which is almost six times greater than the rest of the world [ 22 ]. On the other hand, Saifan et al. [ 5 ], explored the theory-practice gap in the United Arab Emirates and found that clinical stressors could be decreased by preparing students better for clinical education with qualified clinical faculty and supportive preceptors.
The purpose of this study was to identify the stressors experienced by undergraduate nursing students in the United Arab Emirates during their first clinical training and the basic adaptation approaches or coping strategies they used. Recognizing or understanding different coping processes can inform the implementation of corrective measures when students experience clinical stress. The findings of this study may provide valuable information for nursing programmes, nurse educators, and clinical administrators to establish adaptive strategies to reduce stress among students going clinical practicums, particularly stressors from their first clinical training in different healthcare settings.
A qualitative approach was adopted to understand clinical stressors and coping strategies from the perspective of nurses’ lived experience. Qualitative content analysis was employed to obtain rich and detailed information from our qualitative data. Qualitative approaches seek to understand the phenomenon under study from the perspectives of individuals with lived experience [ 23 ]. Qualitative content analysis is an interpretive technique that examines the similarities and differences between and within different areas of text while focusing on the subject [ 24 ]. It is used to examine communication patterns in a repeatable and systematic way [ 25 ] and yields rich and detailed information on the topic under investigation [ 23 ]. It is a method of systematically coding and categorizing information and comprises a process of comprehending, interpreting, and conceptualizing the key meanings from qualitative data [ 26 ].
Setting and participants
This study was conducted after the clinical rotations ended in April 2022, between May and June in the nursing programme at the College of Health Sciences, University of Sharjah, in the United Arab Emirates. The study population comprised undergraduate nursing students who were undergoing their first clinical training and were recruited using purposive sampling. The inclusion criteria for this study were second-year nursing students in the first semester of clinical training who could speak English, were willing to participate in this research, and had no previous clinical work experience. The final sample consisted of 30 students.
Research instrument
The research instrument was a semi structured interview guide. The interview questions were based on an in-depth review of related literature. An intensive search included key words in Google Scholar, PubMed like the terms “nursing clinical stressors”, “nursing students”, and “coping mechanisms”. Once the questions were created, they were validated by two other faculty members who had relevant experience in mental health. A pilot test was conducted with five students and based on their feedback the following research questions, which were addressed in the study.
How would you describe your clinical experiences during your first clinical rotations?
In what ways did you find the first clinical rotation to be stressful?
What factors hindered your clinical training?
How did you cope with the stressors you encountered in clinical training?
Which strategies helped you cope with the clinical stressors you encountered?
Data collection
Semi-structured interviews were chosen as the method for data collection. Semi structured interviews are a well-established approach for gathering data in qualitative research and allow participants to discuss their views, experiences, attitudes, and beliefs in a positive environment [ 27 ]. This approach allows for flexibility in questioning thereby ensuring that key topics related to clinical learning stressors and coping strategies would be explored. Participants were given the opportunity to express their views, experiences, attitudes, and beliefs in a positive environment, encouraging open communication. These semi structured interviews were conducted by one member of the research team (MAS) who had a mental health background, and another member of the research team who attended the interviews as an observer (JMD). Neither of these researchers were involved in teaching the students during their clinical practicum, which helped to minimize bias. The interviews took place at the University of Sharjah, specifically in building M23, providing a familiar and comfortable environment for the participant. Before the interviews were all students who agreed to participate were provided with an explanation of the study’s purpose. The time and location of each interview were arranged. Before the interviews were conducted, all students who provided consent to participate received an explanation of the purpose of the study, and the time and place of each interview were arranged to accommodate the participants’ schedules and preferences. The interviews were conducted after the clinical rotation had ended in April, and after the final grades had been submitted to the coordinator. The timings of the interviews included the month of May and June which ensured that participants have completed their practicum experience and could reflect on the stressors more comprehensively. The interviews were audio-recorded with the participants’ consent, and each interview lasted 25–40 min. The data were collected until saturation was reached for 30 students. Memos and field notes were also recorded as part of the data collection process. These additional data allowed for triangulation to improve the credibility of the interpretations of the data [ 28 ]. Memos included the interviewers’ thoughts and interpretations about the interviews, the research process (including questions and gaps), and the analytic progress used for the research. Field notes were used to record the interviewers’ observations and reflections on the data. These additional data collection methods were important to guide the researchers in the interpretation of the data on the participants’ feelings, perspectives, experiences, attitudes, and beliefs. Finally, member checking was performed to ensure conformability.
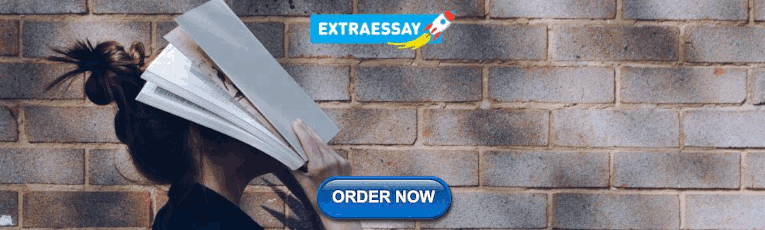
Data analysis
The study used the content analysis method proposed by Graneheim and Lundman [ 24 ]. According to Graneheim and Lundman [ 24 ], content analysis is an interpretive technique that examines the similarities and differences between distinct parts of a text. This method allows researchers to determine exact theoretical and operational definitions of words, phrases, and symbols by elucidating their constituent properties [ 29 ]. First, we read the interview transcripts several times to reach an overall understanding of the data. All verbatim transcripts were read several times and discussed among all authors. We merged and used line-by-line coding of words, sentences, and paragraphs relevant to each other in terms of both the content and context of stressors and coping mechanisms. Next, we used data reduction to assess the relationships among themes using tables and diagrams to indicate conceptual patterns. Content related to stress encountered by students was extracted from the transcripts. In a separate document, we integrated and categorized all words and sentences that were related to each other in terms of both content and context. We analyzed all codes and units of meaning and compared them for similarities and differences in the context of this study. Furthermore, the emerging findings were discussed with other members of the researcher team. The final abstractions of meaningful subthemes into themes were discussed and agreed upon by the entire research team. This process resulted in the extraction of three main themes in addition to two subthemes related to stress and coping strategies.
Ethical considerations
The University of Sharjah Research Ethics Committee provided approval to conduct this study (Reference Number: REC 19-12-03-01-S). Before each interview, the goal and study procedures were explained to each participant, and written informed consent was obtained. The participants were informed that participation in the study was voluntary and that they could withdraw from the study at any time. In the event they wanted to withdraw from the study, all information related to the participant would be removed. No participant withdrew from the study. Furthermore, they were informed that their clinical practicum grade would not be affected by their participation in this study. We chose interview locations in Building M23that were private and quiet to ensure that the participants felt at ease and confident in verbalizing their opinions. No participant was paid directly for involvement in this study. In addition, participants were assured that their data would remain anonymous and confidential. Confidentiality means that the information provided by participants was kept private with restrictions on how and when data can be shared with others. The participants were informed that their information would not be duplicated or disseminated without their permission. Anonymity refers to the act of keeping people anonymous with respect to their participation in a research endeavor. No personal identifiers were used in this study, and each participant was assigned a random alpha-numeric code (e.g., P1 for participant 1). All digitally recorded interviews were downloaded to a secure computer protected by the principal investigator with a password. The researchers were the only people with access to the interview material (recordings and transcripts). All sensitive information and materials were kept secure in the principal researcher’s office at the University of Sharjah. The data will be maintained for five years after the study is completed, after which the material will be destroyed (the transcripts will be shredded, and the tapes will be demagnetized).
In total, 30 nursing students who were enrolled in the nursing programme at the Department of Nursing, College of Health Sciences, University of Sharjah, and who were undergoing their first clinical practicum participated in the study. Demographically, 80% ( n = 24) were females and 20% ( n = 6) were male participants. The majority (83%) of study participants ranged in age from 18 to 22 years. 20% ( n = 6) were UAE nationals, 53% ( n = 16) were from Gulf Cooperation Council countries, while 20% ( n = 6) hailed from Africa and 7% ( n = 2) were of South Asian descent. 67% of the respondents lived with their families while 33% lived in the hostel. (Table 1 )
Following the content analysis, we identified three main themes: (1) managing expectations, (2) theory-practice gap and 3)learning to cope. Learning to cope had two subthemes: positive coping strategies and negative coping strategies. An account of each theme is presented along with supporting excerpts for the identified themes. The identified themes provide valuable insight into the stressors encountered by students during their first clinical practicum. These themes will lead to targeted interventions and supportive mechanisms that can be built into the clinical training curriculum to support students during clinical practice.
Theme 1: managing expectations
In our examination of the stressors experienced by nursing students during their first clinical practicum and the coping strategies they employed, we identified the first theme as managing expectations.
The students encountered expectations from various parties, such as clinical staff, patients and patients’ relatives which they had to navigate. They attempted to fulfil their expectations as they progressed through training, which presented a source of stress. The students noted that the hospital staff and patients expected them to know how to perform a variety of tasks upon request, which made the students feel stressed and out of place if they did not know how to perform these tasks. Some participants noted that other nurses in the clinical unit did not allow them to participate in nursing procedures, which was considered an enormous impediment to clinical learning, as noted in the excerpt below:
“…Sometimes the nurses… They will not allow us to do some procedures or things during clinical. And sometimes the patients themselves don’t allow us to do procedures” (P5).
Some of the students noted that they felt they did not belong and felt like foreigners in the clinical unit. Excerpts from the students are presented in the following quotes;
“The clinical environment is so stressful. I don’t feel like I belong. There is too little time to build a rapport with hospital staff or the patient” (P22).
“… you ask the hospital staff for some guidance or the location of equipment, and they tell us to ask our clinical tutor …but she is not around … what should I do? It appears like we do not belong, and the sooner the shift is over, the better” (P18).
“The staff are unfriendly and expect too much from us students… I feel like I don’t belong, or I am wasting their (the hospital staff’s) time. I want to ask questions, but they have loads to do” (P26).
Other students were concerned about potential failure when working with patients during clinical training, which impacted their confidence. They were particularly afraid of failure when performing any clinical procedures.
“At the beginning, I was afraid to do procedures. I thought that maybe the patient would be hurt and that I would not be successful in doing it. I have low self-confidence in doing procedures” (P13).
The call bell rings, and I am told to answer Room No. XXX. The patient wants help to go to the toilet, but she has two IV lines. I don’t know how to transport the patient… should I take her on the wheelchair? My eyes glance around the room for a wheelchair. I am so confused …I tell the patient I will inform the sister at the nursing station. The relative in the room glares at me angrily … “you better hurry up”…Oh, I feel like I don’t belong, as I am not able to help the patient… how will I face the same patient again?” (P12).
Another major stressor mentioned in the narratives was related to communication and interactions with patients who spoke another language, so it was difficult to communicate.
“There was a challenge with my communication with the patients. Sometimes I have communication barriers because they (the patients) are of other nationalities. I had an experience with a patient [who was] Indian, and he couldn’t speak my language. I did not understand his language” (P9).
Thus, a variety of expectations from patients, relatives, hospital staff, and preceptors acted as sources of stress for students during their clinical training.
Theme 2: theory-practice gap
Theory-practice gaps have been identified in previous studies. In our study, there was complete dissonance between theory and actual clinical practice. The clinical procedures or practices nursing students were expected to perform differed from the theory they had covered in their university classes and skills lab. This was described as a theory–practice gap and often resulted in stress and confusion.
“For example …the procedures in the hospital are different. They are different from what we learned or from theory on campus. Or… the preceptors have different techniques than what we learned on campus. So, I was stress[ed] and confused about it” (P11).
Furthermore, some students reported that they did not feel that they received adequate briefing before going to clinical training. A related source of stress was overload because of the volume of clinical coursework and assignments in addition to clinical expectations. Additionally, the students reported that a lack of time and time management were major sources of stress in their first clinical training and impacted their ability to complete the required paperwork and assignments:
“…There is not enough time…also, time management at the hospital…for example, we start at seven a.m., and the handover takes 1 hour to finish. They (the nurses at the hospital) are very slow…They start with bed making and morning care like at 9.45 a.m. Then, we must fill [out] our assessment tool and the NCP (nursing care plan) at 10 a.m. So, 15 only minutes before going to our break. We (the students) cannot manage this time. This condition makes me and my friends very stressed out. -I cannot do my paperwork or assignments; no time, right?” (P10).
“Stressful. There is a lot of work to do in clinical. My experiences are not really good with this course. We have a lot of things to do, so many assignments and clinical procedures to complete” (P16).
The participants noted that the amount of required coursework and number of assignments also presented a challenge during their first clinical training and especially affected their opportunity to learn.
“I need to read the file, know about my patient’s condition and pathophysiology and the rationale for the medications the patient is receiving…These are big stressors for my learning. I think about assignments often. Like, we are just focusing on so many assignments and papers. We need to submit assessments and care plans for clinical cases. We focus our time to complete and finish the papers rather than doing the real clinical procedures, so we lose [the] chance to learn” (P25).
Another participant commented in a similar vein that there was not enough time to perform tasks related to clinical requirements during clinical placement.
“…there is a challenge because we do not have enough time. Always no time for us to submit papers, to complete assessment tools, and some nurses, they don’t help us. I think we need more time to get more experiences and do more procedures, reduce the paperwork that we have to submit. These are challenges …” (P14).
There were expectations that the students should be able to carry out their nursing duties without becoming ill or adversely affected. In addition, many students reported that the clinical environment was completely different from the skills laboratory at the college. Exposure to the clinical setting added to the theory-practice gap, and in some instances, the students fell ill.
One student made the following comment:
“I was assisting a doctor with a dressing, and the sight and smell from the oozing wound was too much for me. I was nauseated. As soon as the dressing was done, I ran to the bathroom and threw up. I asked myself… how will I survive the next 3 years of nursing?” (P14).
Theme 3: learning to cope
The study participants indicated that they used coping mechanisms (both positive and negative) to adapt to and manage the stressors in their first clinical practicum. Important strategies that were reportedly used to cope with stress were time management, good preparation for clinical practice, and positive thinking as well as engaging in physical activity and self-motivation.
“Time management. Yes, it is important. I was encouraging myself. I used time management and prepared myself before going to the clinical site. Also, eating good food like cereal…it helps me very much in the clinic” (P28).
“Oh yeah, for sure positive thinking. In the hospital, I always think positively. Then, after coming home, I get [to] rest and think about positive things that I can do. So, I will think something good [about] these things, and then I will be relieved of stress” (P21).
Other strategies commonly reported by the participants were managing their breathing (e.g., taking deep breaths, breathing slowly), taking breaks to relax, and talking with friends about the problems they encountered.
“I prefer to take deep breaths and breathe slowly and to have a cup of coffee and to talk to my friends about the case or the clinical preceptor and what made me sad so I will feel more relaxed” (P16).
“Maybe I will take my break so I feel relaxed and feel better. After clinical training, I go directly home and take a long shower, going over the day. I will not think about anything bad that happened that day. I just try to think about good things so that I forget the stress” (P27).
“Yes, my first clinical training was not easy. It was difficult and made me stressed out…. I felt that it was a very difficult time for me. I thought about leaving nursing” (P7).
I was not able to offer my prayers. For me, this was distressing because as a Muslim, I pray regularly. Now, my prayer time is pushed to the end of the shift” (P11).
“When I feel stress, I talk to my friends about the case and what made me stressed. Then I will feel more relaxed” (P26).
Self-support or self-motivation through positive self-talk was also used by the students to cope with stress.
“Yes, it is difficult in the first clinical training. When I am stress[ed], I go to the bathroom and stand in the front of the mirror; I talk to myself, and I say, “You can do it,” “you are a great student.” I motivate myself: “You can do it”… Then, I just take breaths slowly several times. This is better than shouting or crying because it makes me tired” (P11).
Other participants used physical activity to manage their stress.
“How do I cope with my stress? Actually, when I get stressed, I will go for a walk on campus” (P4).
“At home, I will go to my room and close the door and start doing my exercises. After that, I feel the negative energy goes out, then I start to calm down… and begin my clinical assignments” (P21).
Both positive and negative coping strategies were utilized by the students. Some participants described using negative coping strategies when they encountered stress during their clinical practice. These negative coping strategies included becoming irritable and angry, eating too much food, drinking too much coffee, and smoking cigarettes.
“…Negative adaptation? Maybe coping. If I am stressed, I get so angry easily. I am irritable all day also…It is negative energy, right? Then, at home, I am also angry. After that, it is good to be alone to think about my problems” (P12).
“Yeah, if I…feel stress or depressed, I will eat a lot of food. Yeah, ineffective, like I will be eating a lot, drinking coffee. Like I said, effective, like I will prepare myself and do breathing, ineffective, I will eat a lot of snacks in between my free time. This is the bad side” (P16).
“…During the first clinical practice? Yes, it was a difficult experience for us…not only me. When stressed, during a break at the hospital, I will drink two or three cups of coffee… Also, I smoke cigarettes… A lot. I can drink six cups [of coffee] a day when I am stressed. After drinking coffee, I feel more relaxed, I finish everything (food) in the refrigerator or whatever I have in the pantry, like chocolates, chips, etc” (P23).
These supporting excerpts for each theme and the analysis offers valuable insights into the specific stressors faced by nursing students during their first clinical practicum. These insights will form the basis for the development of targeted interventions and supportive mechanisms within the clinical training curriculum to better support students’ adjustment and well-being during clinical practice.
Our study identified the stressors students encounter in their first clinical practicum and the coping strategies, both positive and negative, that they employed. Although this study emphasizes the importance of clinical training to prepare nursing students to practice as nurses, it also demonstrates the correlation between stressors and coping strategies.The content analysis of the first theme, managing expectations, paves the way for clinical agencies to realize that the students of today will be the nurses of tomorrow. It is important to provide a welcoming environment where students can develop their identities and learn effectively. Additionally, clinical staff should foster an environment of individualized learning while also assisting students in gaining confidence and competence in their repertoire of nursing skills, including critical thinking, problem solving and communication skills [ 8 , 15 , 19 , 30 ]. Another challenge encountered by the students in our study was that they were prevented from participating in clinical procedures by some nurses or patients. This finding is consistent with previous studies reporting that key challenges for students in clinical learning include a lack of clinical support and poor attitudes among clinical staff and instructors [ 31 ]. Clinical staff with positive attitudes have a positive impact on students’ learning in clinical settings [ 32 ]. The presence, supervision, and guidance of clinical instructors and the assistance of clinical staff are essential motivating components in the clinical learning process and offer positive reinforcement [ 30 , 33 , 34 ]. Conversely, an unsupportive learning environment combined with unwelcoming clinical staff and a lack of sense of belonging negatively impact students’ clinical learning [ 35 ].
The sources of stress identified in this study were consistent with common sources of stress in clinical training reported in previous studies, including the attitudes of some staff, students’ status in their clinical placement and educational factors. Nursing students’ inexperience in the clinical setting and lack of social and emotional experience also resulted in stress and psychological difficulties [ 36 ]. Bhurtun et al. [ 33 ] noted that nursing staff are a major source of stress for students because the students feel like they are constantly being watched and evaluated.
We also found that students were concerned about potential failure when working with patients during their clinical training. Their fear of failure when performing clinical procedures may be attributable to low self-confidence. Previous studies have noted that students were concerned about injuring patients, being blamed or chastised, and failing examinations [ 37 , 38 ]. This was described as feeling “powerless” in a previous study [ 7 , 12 ]. In addition, patients’ attitudes towards “rejecting” nursing students or patients’ refusal of their help were sources of stress among the students in our study and affected their self-confidence. Self-confidence and a sense of belonging are important for nurses’ personal and professional identity, and low self-confidence is a problem for nursing students in clinical learning [ 8 , 39 , 40 ]. Our findings are consistent with a previous study that reported that a lack of self-confidence was a primary source of worry and anxiety for nursing students and affected their communication and intention to leave nursing [ 41 ].
In the second theme, our study suggests that students encounter a theory-practice gap in clinical settings, which creates confusion and presents an additional stressors. Theoretical and clinical training are complementary elements of nursing education [ 40 ], and this combination enables students to gain the knowledge, skills, and attitudes necessary to provide nursing care. This is consistent with the findings of a previous study that reported that inconsistencies between theoretical knowledge and practical experience presented a primary obstacle to the learning process in the clinical context [ 42 ], causing students to lose confidence and become anxious [ 43 ]. Additionally, the second theme, the theory-practice gap, authenticates Safian et al.’s [ 5 ] study of the theory-practice gap that exists United Arab Emirates among nursing students as well as the need for more supportive clinical faculty and the extension of clinical hours. The need for better time availability and time management to complete clinical tasks were also reported by the students in the study. Students indicated that they had insufficient time to complete clinical activities because of the volume of coursework and assignments. Our findings support those of Chaabane et al. [ 15 ]. A study conducted in Saudi Arabia [ 44 ] found that assignments and workload were among the greatest sources of stress for students in clinical settings. Effective time management skills have been linked to academic achievement, stress reduction, increased creativity [ 45 ], and student satisfaction [ 46 ]. Our findings are also consistent with previous studies that reported that a common source of stress among first-year students was the increased classroom workload [ 19 , 47 ]. As clinical assignments and workloads are major stressors for nursing students, it is important to promote activities to help them manage these assignments [ 48 ].
Another major challenge reported by the participants was related to communicating and interacting with other nurses and patients. The UAE nursing workforce and population are largely expatriate and diverse and have different cultural and linguistic backgrounds. Therefore, student nurses encounter difficulty in communication [ 49 ]. This cultural diversity that students encounter in communication with patients during clinical training needs to be addressed by curriculum planners through the offering of language courses and courses on cultural diversity [ 50 ].
Regarding the third and final theme, nursing students in clinical training are unable to avoid stressors and must learn to cope with or adapt to them. Previous research has reported a link between stressors and the coping mechanisms used by nursing students [ 51 , 52 , 53 ]. In particular, the inability to manage stress influences nurses’ performance, physical and mental health, attitude, and role satisfaction [ 54 ]. One such study suggested that nursing students commonly use problem-focused (dealing with the problem), emotion-focused (regulating emotion), and dysfunctional (e.g., venting emotions) stress coping mechanisms to alleviate stress during clinical training [ 15 ]. Labrague et al. [ 51 ] highlighted that nursing students use both active and passive coping techniques to manage stress. The pattern of clinical stress has been observed in several countries worldwide. The current study found that first-year students experienced stress during their first clinical training [ 35 , 41 , 55 ]. The stressors they encountered impacted their overall health and disrupted their clinical learning. Chaabane et al. [ 15 ] reported moderate and high stress levels among nursing students in Bahrain, Egypt, Iraq, Jordan, Oman, Pakistan, Palestine, Saudi Arabia, and Sudan. Another study from Bahrain reported that all nursing students experienced moderate to severe stress in their first clinical placement [ 56 ]. Similarly, nursing students in Spain experienced a moderate level of stress, and this stress was significantly correlated with anxiety [ 30 ]. Therefore, it is imperative that pastoral systems at the university address students’ stress and mental health so that it does not affect their clinical performance. Faculty need to utilize evidence-based interventions to support students so that anxiety-producing situations and attrition are minimized.
In our study, students reported a variety of positive and negative coping mechanisms and strategies they used when they experienced stress during their clinical practice. Positive coping strategies included time management, positive thinking, self-support/motivation, breathing, taking breaks, talking with friends, and physical activity. These findings are consistent with those of a previous study in which healthy coping mechanisms used by students included effective time management, social support, positive reappraisal, and participation in leisure activities [ 57 ]. Our study found that relaxing and talking with friends were stress management strategies commonly used by students. Communication with friends to cope with stress may be considered social support. A previous study also reported that people seek social support to cope with stress [ 58 ]. Some students in our study used physical activity to cope with stress, consistent with the findings of previous research. Stretching exercises can be used to counteract the poor posture and positioning associated with stress and to assist in reducing physical tension. Promoting such exercise among nursing students may assist them in coping with stress in their clinical training [ 59 ].
Our study also showed that when students felt stressed, some adopted negative coping strategies, such as showing anger/irritability, engaging in unhealthy eating habits (e.g., consumption of too much food or coffee), or smoking cigarettes. Previous studies have reported that high levels of perceived stress affect eating habits [ 60 ] and are linked to poor diet quality, increased snacking, and low fruit intake [ 61 ]. Stress in clinical settings has also been linked to sleep problems, substance misuse, and high-risk behaviors’ and plays a major role in student’s decision to continue in their programme.
Implications of the study
The implications of the study results can be grouped at multiple levels including; clinical, educational, and organizational level. A comprehensive approach to addressing the stressors encountered by nursing students during their clinical practicum can be overcome by offering some practical strategies to address the stressors faced by nursing students during their clinical practicum. By integrating study findings into curriculum planning, mentorship programs, and organizational support structures, a supportive and nurturing environment that enhances students’ learning, resilience, and overall success can be envisioned.
Clinical level
Introducing simulation in the skills lab with standardized patients and the use of moulage to demonstrate wounds, ostomies, and purulent dressings enhances students’ practical skills and prepares them for real-world clinical scenarios. Organizing orientation days at clinical facilities helps familiarize students with the clinical environment, identify potential stressors, and introduce interventions to enhance professionalism, social skills, and coping abilities Furthermore, creating a WhatsApp group facilitates communication and collaboration among hospital staff, clinical tutors, nursing faculty, and students, enabling immediate support and problem-solving for clinical situations as they arise, Moreover, involving chief nursing officers of clinical facilities in the Nursing Advisory Group at the Department of Nursing promotes collaboration between academia and clinical practice, ensuring alignment between educational objectives and the needs of the clinical setting [ 62 ].
Educational level
Sharing study findings at conferences (we presented the results of this study at Sigma Theta Tau International in July 2023 in Abu Dhabi, UAE) and journal clubs disseminates knowledge and best practices among educators and clinicians, promoting awareness and implementation of measures to improve students’ learning experiences. Additionally we hold mentorship training sessions annually in January and so we shared with the clinical mentors and preceptors the findings of this study so that they proactively they are equipped with strategies to support students’ coping with stressors during clinical placements.
Organizational level
At the organizational we relooked at the available student support structures, including counseling, faculty advising, and career advice, throughout the nursing program emphasizing the importance of holistic support for students’ well-being and academic success as well as retention in the nursing program. Also, offering language courses as electives recognizes the value of communication skills in nursing practice and provides opportunities for personal and professional development.
For first-year nursing students, clinical stressors are inevitable and must be given proper attention. Recognizing nursing students’ perspectives on the challenges and stressors experienced in clinical training is the first step in overcoming these challenges. In nursing schools, providing an optimal clinical environment as well as increasing supervision and evaluation of students’ practices should be emphasized. Our findings demonstrate that first-year nursing students are exposed to a variety of different stressors. Identifying the stressors, pressures, and obstacles that first-year students encounter in the clinical setting can assist nursing educators in resolving these issues and can contribute to students’ professional development and survival to allow them to remain in the profession. To overcome stressors, students frequently employ problem-solving approaches or coping mechanisms. The majority of nursing students report stress at different levels and use a variety of positive and negative coping techniques to manage stress.
The present results may not be generalizable to other nursing institutions because this study used a purposive sample along with a qualitative approach and was limited to one university in the Middle East. Furthermore, the students self-reported their stress and its causes, which may have introduced reporting bias. The students may also have over or underreported stress or coping mechanisms because of fear of repercussions or personal reasons, even though the confidentiality of their data was ensured. Further studies are needed to evaluate student stressors and coping now that measures have been introduced to support students. Time will tell if these strategies are being used effectively by both students and clinical personnel or if they need to be readdressed. Finally, we need to explore the perceptions of clinical faculty towards supervising students in their first clinical practicum so that clinical stressors can be handled effectively.
Data availability
The data sets are available with the corresponding author upon reasonable request.
Almarwani AM. The effect of integrating a nursing licensure examination preparation course into a nursing program curriculum: a quasi-experimental study. Saudi J Health Sci. 2022;11:184–9.
Article Google Scholar
Horntvedt MT, Nordsteien A, Fermann T, Severinsson E. Strategies for teaching evidence-based practice in nursing education: a thematic literature review. BMC Med Educ. 2018;18:172.
Article PubMed PubMed Central Google Scholar
Larsson M, Sundler AJ, Blomberg K, Bisholt B. The clinical learning environment during clinical practice in postgraduate district nursing students’ education: a cross-sectional study. Nurs Open. 2023;10:879–88.
Article PubMed Google Scholar
Sellberg M, Palmgren PJ, Möller R. A cross-sectional study of clinical learning environments across four undergraduate programs using the undergraduate clinical education environment measure. BMC Med Educ. 2021;21:258.
Saifan A, Devadas B, Mekkawi M, Amoor H, Matizha P, James J, et al. Managing the theory-practice gap in nursing education and practice: hearing the voices of nursing students in the United Arab Emirates. J Nurs Manag. 2021;29:1869–79.
Flott EA, Linden L. The clinical learning environment in nursing education: a concept analysis. J Adv Nurs. 2016;72:501–13.
Kalyani MN, Jamshidi N, Molazem Z, Torabizadeh C, Sharif F. How do nursing students experience the clinical learning environment and respond to their experiences? A qualitative study. BMJ Open. 2019;9:e028052.
Mahasneh D, Shoqirat N, Alsaraireh A, Singh C, Thorpe L. From learning on mannequins to practicing on patients: nursing students’ first-time experience of clinical placement in Jordan. SAGE Open Nurs. 2021;7:23779608211004298.
PubMed PubMed Central Google Scholar
Stubin C. Clinical stress among undergraduate nursing students: perceptions of clinical nursing faculty. Int J Nurs Educ Scholarsh. 2020;17:20190111.
Ahmed WAM. Anxiety and related symptoms among critical care nurses in Albaha, Kingdom of Saudi Arabia. AIMS Med Sci. 2015;2:303–9.
Alhassan. Duke Phillips. 2024.
Ekstedt M, Lindblad M, Löfmark A. Nursing students’ perception of the clinical learning environment and supervision in relation to two different supervision models - a comparative cross-sectional study. BMC Nurs. 2019;18:49.
Bradshaw C, Murphy Tighe S, Doody O. Midwifery students’ experiences of their clinical internship: a qualitative descriptive study. Nurse Educ Today. 2018;68:213–7.
McCarthy B, Trace A, O’Donovan M, O’Regan P, Brady-Nevin C, O’Shea M, et al. Coping with stressful events: a pre-post-test of a psycho-educational intervention for undergraduate nursing and midwifery students. Nurse Educ Today. 2018;61:273–80.
Chaabane S, Chaabna K, Bhagat S, Abraham A, Doraiswamy S, Mamtani R, et al. Perceived stress, stressors, and coping strategies among nursing students in the Middle East and North Africa: an overview of systematic reviews. Syst Rev. 2021;10:136.
Pines EW, Rauschhuber ML, Norgan GH, Cook JD, Canchola L, Richardson C, et al. Stress resiliency, psychological empowerment and conflict management styles among baccalaureate nursing students. J Adv Nurs. 2012;68:1482–93.
Lazarus RS. Coping theory and research: past, present, and future. Psychosom Med. 1993;55:234–47.
Article CAS PubMed Google Scholar
Boyd MA. Essentials of psychiatric nursing. Philadelphia, PA: Wolters Kluwer; 2017.
Google Scholar
Labrague LJ, McEnroe-Petitte DM, Gloe D, Thomas L, Papathanasiou IV, Tsaras K. A literature review on stress and coping strategies in nursing students. J Ment Health. 2017;26:471–80.
Ni C, Lo D, Liu X, Ma J, Xu S, Li L. Chinese female nursing students’ coping strategies, self-esteem and related factors in different years of school. J Nurs Educ Pract. 2012;2:33–41.
Jan LK, Popescu L. Israel’s nursing students’ stress sources and coping strategies during their first clinical experience in hospital wards-a qualitative research. Soc Work Rev / Rev Asistenta Soc. 2014;13:163–88.
Tung YJ, Lo KKH, Ho RCM, Tam WSW. Prevalence of depression among nursing students: a systematic review and meta-analysis. Nurse Educ Today. 2018;63:119–29.
Speziale HS, Streubert HJ, Carpenter DR. Qualitative research in nursing: advancing the humanistic imperative. Philadelphia, PA: Lippincott Williams & Wilkins; 2011.
Graneheim UH, Lundman B. Qualitative content analysis in nursing research: concepts, procedures and measures to achieve trustworthiness. Nurse Educ Today. 2004;24:105–12.
Bryman A. Integrating quantitative and qualitative research: how is it done? Qual Res. 2006;6:97–113.
Holloway I, Wheeler S. Qualitative research in nursing and healthcare. New York, NY: Wiley; 2013.
Richards L, Morse J. A user’s guide to qualitative methods. London, UK: Sage; 2007.
Lincoln Y, Guba EG. The SAGE handbook of qualitative research. Newbury Park, CA: SAGE Publications Inc; 2017.
Park S, Park KS. Family stigma: a concept analysis. Asian Nurs Res. 2014;8:165–71.
Onieva-Zafra MD, Fernández-Muñoz JJ, Fernández-Martínez E, García-Sánchez FJ, Abreu-Sánchez A, Parra-Fernández ML. Anxiety, perceived stress and coping strategies in nursing students: a cross-sectional, correlational, descriptive study. BMC Med Educ. 2020;20:370.
Albloushi M, Ferguson L, Stamler L, Bassendowski S, Hellsten L, Kent-Wilkinson A. Saudi female nursing students experiences of sense of belonging in the clinical settings: a qualitative study. Nurse Educ Pract. 2019;35:69–74.
Arkan B, Ordin Y, Yılmaz D. Undergraduate nursing students’ experience related to their clinical learning environment and factors affecting to their clinical learning process. Nurse Educ Pract. 2018;29:127–32.
Bhurtun HD, Azimirad M, Saaranen T, Turunen H. Stress and coping among nursing students during clinical training: an integrative review. J Nurs Educ. 2019;58:266–72.
Jamshidi N, Molazem Z, Sharif F, Torabizadeh C, Kalyani MN. The challenges of nursing students in the clinical learning environment: a qualitative study. ScientificWorldJournal. 2016;2016:1846178.
Porter SL. First year nursing students’ perceptions of stress and resilience during their initial clinical placement and the introduction of a stress management app: a mixed methods approach. A thesis submitted in partial fulfilment of the requirements of Edinburgh Napier University, for the award of Doctor of Philosophy. 2019. https://www.napier.ac.uk/~/media/worktribe/output-2086663/first-year-nursing-students-perceptions-of-stress-and-resilience-during-their-initial.pdf
Panda S, Dash M, John J, Rath K, Debata A, Swain D, et al. Challenges faced by student nurses and midwives in clinical learning environment - A systematic review and meta-synthesis. Nurse Educ Today. 2021;101:104875.
Ahmadi G, Shahriari M, Keyvanara M, Kohan S. Midwifery students’ experiences of learning clinical skills in Iran: a qualitative study. Int J Med Educ. 2018;9:64–71.
Harrison-White K, Owens J. Nurse link lecturers’ perceptions of the challenges facing student nurses in clinical learning environments: a qualitative study. Nurse Educ Pract. 2018;32:78–83.
Grobecker PA. A sense of belonging and perceived stress among baccalaureate nursing students in clinical placements. Nurse Educ Today. 2016;36:178–83.
Msiska G, Kamanga M, Chilemba E, Msosa A, Munkhondya TE. Sources of stress among undergraduate nursing students during clinical practice: a Malawian perspective. Open J Nurs. 2019;9:1.
Joolaee S, Amiri SRJ, Farahani MA, Varaei S. Iranian nursing students’ preparedness for clinical training: a qualitative study. Nurse Educ Today. 2015;35:e13–7.
Günay U, Kılınç G. The transfer of theoretical knowledge to clinical practice by nursing students and the difficulties they experience: a qualitative study. Nurse Educ Today. 2018;65:81–6.
Farzi S, Shahriari M, Farzi S. Exploring the challenges of clinical education in nursing and strategies to improve it: a qualitative study. J Educ Health Promot. 2018;7:115.
Hamaideh SH, Al-Omari H, Al-Modallal H. Nursing students’ perceived stress and coping behaviors in clinical training in Saudi Arabia. J Ment Health. 2017;26:197–203.
Yaghoobi A, Mohagheghi H, Zade MY, Ganji K, Olfatii N. The effect of time management training on test anxiety and academic achievement motivation among high school students. J Sch Psychol. 2014;3:131–44.
Kebriaei A, Bidgoli MS, Saeedi A. Relationship between use of time management skills and satisfaction with spending time among students of Zahedan University of Medical Sciences. J Med Educ Dev. 2014;6:79–88.
Chen YW, Hung CH. Predictors of Taiwanese baccalaureate nursing students’ physio-psycho-social responses during clinical practicum. Nurse Educ Today. 2014;34:73–7.
Ab Latif R, Mat Nor MZ. Stressors and coping strategies during clinical practice among diploma nursing students. Malays J Med Sci. 2019;26:88–98.
Al-Yateem N, Almarzouqi A, Dias JM, Saifan A, Timmins F. Nursing in the United Arab Emirates: current challenges and opportunities. J Nurs Manag. 2021;29:109–12.
Baraz-Pordanjani S, Memarian R, Vanaki Z. Damaged professional identity as a barrier to Iranian nursing students’ clinical learning: a qualitative study. J Clin Nurs Midwifery. 2014;3:1–15.
Labrague LJ, McEnroe-Petitte DM, Papathanasiou IV, Edet OB, Tsaras K, Leocadio MC, et al. Stress and coping strategies among nursing students: an international study. J Ment Health. 2018;27:402–8.
Madian AAEM, Abdelaziz MM, Ahmed HAE. Level of stress and coping strategies among nursing students at Damanhour University, Egypt. Am J Nurs Res. 2019;7:684–96.
Wu CS, Rong JR, Huang MZ. Factors associated with perceived stress of clinical practice among associate degree nursing students in Taiwan. BMC Nurs. 2021;20:89.
Zhao FF, Lei XL, He W, Gu YH, Li DW. The study of perceived stress, coping strategy and self-efficacy of Chinese undergraduate nursing students in clinical practice. Int J Nurs Pract. 2015;21:401–9.
Bektaş H, Terkes N, Özer Z. Stress and ways of coping among first year nursing students: a Turkish perspective. J Hum Sci. 2018;15:319–30.
John B, Al-Sawad M. Perceived stress in clinical areas and emotional intelligence among baccalaureate nursing students. J Indian Acad Appl Psychol. 2015;41:76–85.
Mapfumo JS, Chitsiko N, Chireshe R. Teaching practice generated stressors and coping mechanisms among student teachers in Zimbabwe. S Afr J Educ. 2012;32:155–66.
Timmins F, Corroon AM, Byrne G, Mooney B. The challenge of contemporary nurse education programmes. Perceived stressors of nursing students: mental health and related lifestyle issues. J Psychiatr Ment Health Nurs. 2011;18:758–66.
Hegberg NJ, Tone EB. Physical activity and stress resilience: considering those at-risk for developing mental health problems. Ment Health Phys Act. 2015;8:1–7.
Shudifat RM, Al-Husban RY. Perceived sources of stress among first-year nursing students in Jordan. J Psychosoc Nurs Ment Health Serv. 2015;53:37–43.
El Ansari W, Adetunji H, Oskrochi R. Food and mental health: relationship between food and perceived stress and depressive symptoms among university students in the United Kingdom. Cent Eur J Public Health. 2014;22:90–7.
Dias JM, Aderibigbe SA, Abraham MS. Undergraduate nursing students’ mentoring experiences in the clinical practicum: the United Arab Emirates (UAE) perspective. J Nurs Manag. 2022;30:4304–13.
Download references
Acknowledgements
The authors are grateful to all second year nursing students who voluntarily participated in the study.
No funding was received. Not applicable.
Author information
Authors and affiliations.
Department of Nursing, College of Health Sciences, University of Sharjah, POBox, Sharjah, 272272, UAE
Jacqueline Maria Dias, Muhammad Arsyad Subu, Nabeel Al-Yateem, Fatma Refaat Ahmed, Syed Azizur Rahman, Mini Sara Abraham, Sareh Mirza Forootan, Farzaneh Ahmad Sarkhosh & Fatemeh Javanbakh
Health Care Management, College of Health Sciences, University of Sharjah, Sharjah, United Arab Emirates
Syed Azizur Rahman
You can also search for this author in PubMed Google Scholar
Contributions
JMD conceptualized the idea and designed the methodology, formal analysis, writing original draft and project supervision and mentoring. MAS prepared the methodology and conducted the qualitative interviews and analyzed the methodology and writing of original draft and project supervision. NY, FRA, SAR, MSA writing review and revising the draft. SMF, FAS, FJ worked with MAS on the formal analysis and prepared the first draft.All authors reviewed the final manuscipt of the article.
Corresponding author
Correspondence to Jacqueline Maria Dias .
Ethics declarations
Ethics approval and consent to participate.
The Research Ethics Committee (REC) under) the Office of the Vice Chancellor for Research and Graduate Studies UOS approved this study (REC 19-12-03-01-S). Additionally, a written consent was obtained from all participants and the process followed the recommended policies and guidelines of the Declaration of Helsinki.
Consent for publication
Not applicable.
Competing interests
Dr Fatma Refaat Ahmed is an editorial board member in BMC Nursing. Other authors do not have any conflict of interest
Additional information
Publisher’s note.
Springer Nature remains neutral with regard to jurisdictional claims in published maps and institutional affiliations.
Electronic supplementary material
Below is the link to the electronic supplementary material.
Supplementary Material 1
Rights and permissions.
Open Access This article is licensed under a Creative Commons Attribution 4.0 International License, which permits use, sharing, adaptation, distribution and reproduction in any medium or format, as long as you give appropriate credit to the original author(s) and the source, provide a link to the Creative Commons licence, and indicate if changes were made. The images or other third party material in this article are included in the article’s Creative Commons licence, unless indicated otherwise in a credit line to the material. If material is not included in the article’s Creative Commons licence and your intended use is not permitted by statutory regulation or exceeds the permitted use, you will need to obtain permission directly from the copyright holder. To view a copy of this licence, visit http://creativecommons.org/licenses/by/4.0/ . The Creative Commons Public Domain Dedication waiver ( http://creativecommons.org/publicdomain/zero/1.0/ ) applies to the data made available in this article, unless otherwise stated in a credit line to the data.
Reprints and permissions
About this article
Cite this article.
Dias, J.M., Subu, M.A., Al-Yateem, N. et al. Nursing students’ stressors and coping strategies during their first clinical training: a qualitative study in the United Arab Emirates. BMC Nurs 23 , 322 (2024). https://doi.org/10.1186/s12912-024-01962-5
Download citation
Received : 06 January 2024
Accepted : 22 April 2024
Published : 11 May 2024
DOI : https://doi.org/10.1186/s12912-024-01962-5
Share this article
Anyone you share the following link with will be able to read this content:
Sorry, a shareable link is not currently available for this article.
Provided by the Springer Nature SharedIt content-sharing initiative
- Clinical practicums
- Coping strategies
- Nursing students
BMC Nursing
ISSN: 1472-6955
- General enquiries: [email protected]
Learning Styles of ESTP, ESFP, ENTP, ENFP Students
Learning styles of estp, esfp, entp, enfp students.
4 min. read
Colleges and universities face an enormous amount of pressure to demonstrate value and prepare students for the real world. Likewise, students are under a different (but related) kind of pressure to blaze their own trail and get to the next milestone. But that’s easier said than done for some Myers-Briggs Type Indicator® (MBTI) personality types.
We recently revealed the four personality types that are least likely to graduate college — ESTP, ESFP, ENTP and ENFP. It’s a nuanced topic, but here’s the main issue: not all students understand why they’re in college.
Sure, they know college is one step on their road to a career, but there’s a deeper sense of purpose and alignment that’s often missing. (Much of this can be applied to the workforce too. When an employee or leader understands why they’re in a particular role or industry, it’s easier for them to stay committed and engaged.)
The preferred learning styles of these types don’t match the traditional instruction models of higher education. Think of a typical class lecture where the professor talks for an hour straight. If you’re easily distracted or prefer hands-on learning, you might feel stifled in this setting. To succeed, those four MBTI types (and all types, honestly) need to experience a greater sense of purpose, different teaching styles, and campus involvement that aligns with their preferences.
The MBTI can tell us a lot about what a person’s college experience could be like – which is why it’s important for academic advisors, professors, and career counselors to use it as a tool for student development. If student success and persistence are important, the MBTI can offer some clarity about how to meet them where they’re at.
What are the learning styles of ESTP, ESFP, ENTP, and ENFP personality types?
In the first post about student persistence, we covered how different MBTI types can garner a sense of purpose while in college. Now we’ll explore teaching style and campus involvement. Research from Astin and Tinto points to the need for different kinds of instruction to address different learning styles. In turn, this affects students’ involvement in the campus community – whether that’s through extracurricular activities (i.e., clubs) or student support services (i.e., tutoring, skills labs). Let’s look at the learning styles and needs of the four MBTI types least likely to graduate:
For an ESTP , learning is meaningful when:
- It's active and hands-on
- Expectations are clear and feedback is efficient
- Professors are straightforward and businesslike
- There are sequential “how to” steps with real-life applications
In the classroom or on campus, ESTP students need:
- Clearly stated goals and timelines
- Evidence that the process works
- Practical solutions to problems
- To be actively involved
For an ESFP , learning is meaningful when:
- It’s active and collaborative
- Expectations are clear and feedback is personal
- Professors are friendly and available to help
- There are sequential “how to” steps with personal applications
In the classroom or on campus, ESFP students need:
- Practical solutions for their immediate needs
- Access to helpful information and resources
- To feel like they matter
- Group interaction
For an ENTP , learning is meaningful when:
- It’s active and makes room for debate or exploration of different ideas
- Out-of-the-box thinking and experimentation are encouraged
- Professors are grounded in theory and research
- There are intellectual challenges to solve
In the classroom or on campus, ENTP students need:
- Interdisciplinary activities that are intellectually stimulating
- Freedom to argue and debate various possibilities
- Non-traditional ways to solve problems
- Lively group interaction
For an ENFP , learning is meaningful when:
- It’s active and allows opportunities for collaborative input
- Personal growth and self-expression are prioritized
- Professors are creative and personal
- Creativity is encouraged
In the classroom or on campus, ENFP students need:
- Unique experiences that are personally empowering
- Help bringing their personal vision to reality
- Opportunities to explore possibilities
For ESTPs and ESFPs, learning isn’t meaningful when it involves too much sitting still, out-of-the-box thinking, hypothetical challenges, research, experimentation, conceptual creativity, and self-expression.
For ENTPs and ENFPs, learning isn’t meaningful when it emphasizes memorization, repetition, sequential steps, structure, efficiency, practical applications, measurable outcomes, and individual reflection.
This doesn’t mean that these four MBTI types should always avoid learning styles they dislike. After all, exposure and adaptation to differences is part of life. It just means certain types of instruction aren’t immediately engaging to students who need active, real-life, personal applications. And when the first two years of general education courses are delivered in a certain way (often counter to what these four types prefer), it doesn’t bode well for their success.
Colleges and universities can use the MBTI to increase student success
When students understand how their personality preferences impact their college experiences, they’re in a better position to graduate. And face the world that awaits them. When colleges and universities implement the MBTI into student advisement, it helps uncover natural learning styles and offers clarity into which courses, majors, or activities will make the most impact. In a world filled with enough pressure, it’s the confidence boost and value-add students and institutions need.
College Student Irene Hwang Walks Us Through MBTIonline Careers How to plan your summer vacation 3 Ways to use your MBTI personality type to manage your stress
More From Forbes
How you learn to lead matters.
- Share to Facebook
- Share to Twitter
- Share to Linkedin
A part of National Library of Copenhagen, the mirrors reflected the sky and clouds.
How personalized learning strategies foster better leadership at any career stage
When Eleanor* embarked on her leadership journey with me, it quickly became apparent that she was a high performer and had a work ethic many would envy. However, when I discovered how she interacted with her team and peers, I realized she was blind to her Achilles heel.
A few years ago, Harvard Business Review examined the executive coaching engagements of more than 70 executives. Their findings highlight executives' distinct and often nuanced coaching needs based on their career stage. Specifically, this analysis found a clear contrast between the experience of younger (30s) and older (40s and 50s) leaders.
The study found that younger executives often seek guidance on tactical and operational issues. They want clear, actionable advice to help them navigate their early career stages. In contrast, older executives look for strategic insights and personal growth opportunities, aiming to refine their leadership style and leave a lasting impact. I've witnessed this dichotomy firsthand in my executive coaching practice, even if these groups have significant variability and nuance.
Eleanor was often immature, gossiping and engaging in petty conflict with colleagues. She was unaware of how this impacted her reputation across the organization. When her behavior was brought to her attention, she insisted she was doing the right thing or played down the consequences.
Developing robust self-awareness is critical for all executives, especially those early in their leadership journey. Success is often measured by an individual’s capacity for following prescribed steps and executing tactically in their higher education and even in the early stages of their careers.
When Is ‘The Voice’ Season 25 Finale? All About The Star-Studded Event
Wayfair outdoor furniture sale the best early memorial day deals this week, what’s next for ether prices after today’s sharp gains.
However, as they advance in their careers, developing a more nuanced understanding of human behavior and organizational dynamics is crucial. Young-in-career executives must get out of the executional weeds and develop a new skill set. I’ve found that many of my younger clients often lack the experience to interpret complex interpersonal dynamics accurately. Moreover, each individual’s personal background and core values will add a lens to how they communicate and interpret their interactions. Our coaching work often focuses on bridging this gap by providing them with the right tools to build self-awareness and understand the subtleties of leading and influencing others effectively.
Around the same time I worked with Eleanor, I also began working with Daniel*. His manager had come to me seeking support. Like Eleanor, he was a high performer with immense potential, but his communication strategy was uniformly aggressive. While Daniel was delivering results, he was also alienating the people around him.
A somewhat pervasive trait among high-potential young executives is the belief that they have it all figured out. This confidence can be a double-edged sword. While it fuels ambition and drives performance, it can also camouflage blind spots. Eleanor and Daniel had strong beliefs about their effectiveness, but how they responded to stakeholder feedback and coaching made a difference in their longer-term success.
At the beginning of a coaching engagement, I seek feedback from key stakeholders for each client to better understand the core areas we’ll focus on. We then work to align their core values with the path forward.
Eleanor resisted her stakeholder feedback and justified her behavior, refusing to acknowledge that there might be some truth to it. She doggedly insisted her behavior was fine despite the damage she inflicted on her reputation, not to mention her relationships with others. Last I heard, her professional advancement was flattening.
Daniel claimed that one of his highest priorities was cultivating deep relationships, yet he didn't do that at work with his peers or his team. Although it was uncomfortable, with coaching, Daniel began to explore being more vulnerable, open, and curious in his work interactions. This required increased self-awareness, intentional connections, and empathetic communication at work and home. The result was improved relationships with his colleagues and a well-deserved significant promotion.
Regardless of age or career stage, my most successful clients are those who are more receptive to different approaches and continuous learning. They understand that there isn't always a "right" or perfect way and are more willing to experiment and adapt to embrace transformational, root-cause changes. While quick fixes and tactical solutions seem the fastest way forward, sophisticated leadership development often requires a fundamental behavior, understanding, and awareness shift.
*Names have been changed to protect privacy.
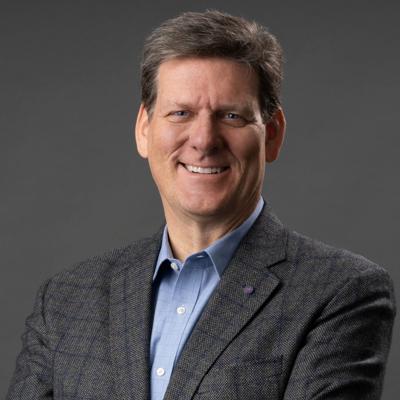
- Editorial Standards
- Reprints & Permissions
Join The Conversation
One Community. Many Voices. Create a free account to share your thoughts.
Forbes Community Guidelines
Our community is about connecting people through open and thoughtful conversations. We want our readers to share their views and exchange ideas and facts in a safe space.
In order to do so, please follow the posting rules in our site's Terms of Service. We've summarized some of those key rules below. Simply put, keep it civil.
Your post will be rejected if we notice that it seems to contain:
- False or intentionally out-of-context or misleading information
- Insults, profanity, incoherent, obscene or inflammatory language or threats of any kind
- Attacks on the identity of other commenters or the article's author
- Content that otherwise violates our site's terms.
User accounts will be blocked if we notice or believe that users are engaged in:
- Continuous attempts to re-post comments that have been previously moderated/rejected
- Racist, sexist, homophobic or other discriminatory comments
- Attempts or tactics that put the site security at risk
- Actions that otherwise violate our site's terms.
So, how can you be a power user?
- Stay on topic and share your insights
- Feel free to be clear and thoughtful to get your point across
- ‘Like’ or ‘Dislike’ to show your point of view.
- Protect your community.
- Use the report tool to alert us when someone breaks the rules.
Thanks for reading our community guidelines. Please read the full list of posting rules found in our site's Terms of Service.
The world is getting “smarter” every day, and to keep up with consumer expectations, companies are increasingly using machine learning algorithms to make things easier. You can see them in use in end-user devices (through face recognition for unlocking smartphones) or for detecting credit card fraud (like triggering alerts for unusual purchases).
Within artificial intelligence (AI) and machine learning , there are two basic approaches: supervised learning and unsupervised learning. The main difference is that one uses labeled data to help predict outcomes, while the other does not. However, there are some nuances between the two approaches, and key areas in which one outperforms the other. This post clarifies the differences so you can choose the best approach for your situation.
Supervised learning is a machine learning approach that’s defined by its use of labeled data sets. These data sets are designed to train or “supervise” algorithms into classifying data or predicting outcomes accurately. Using labeled inputs and outputs, the model can measure its accuracy and learn over time.
Supervised learning can be separated into two types of problems when data mining : classification and regression:
- Classification problems use an algorithm to accurately assign test data into specific categories, such as separating apples from oranges. Or, in the real world, supervised learning algorithms can be used to classify spam in a separate folder from your inbox. Linear classifiers, support vector machines, decision trees and random forest are all common types of classification algorithms.
- Regression is another type of supervised learning method that uses an algorithm to understand the relationship between dependent and independent variables. Regression models are helpful for predicting numerical values based on different data points, such as sales revenue projections for a given business. Some popular regression algorithms are linear regression, logistic regression, and polynomial regression.
Unsupervised learning uses machine learning algorithms to analyze and cluster unlabeled data sets. These algorithms discover hidden patterns in data without the need for human intervention (hence, they are “unsupervised”).
Unsupervised learning models are used for three main tasks: clustering, association and dimensionality reduction:
- Clustering is a data mining technique for grouping unlabeled data based on their similarities or differences. For example, K-means clustering algorithms assign similar data points into groups, where the K value represents the size of the grouping and granularity. This technique is helpful for market segmentation, image compression, and so on.
- Association is another type of unsupervised learning method that uses different rules to find relationships between variables in a given data set. These methods are frequently used for market basket analysis and recommendation engines, along the lines of “Customers Who Bought This Item Also Bought” recommendations.
- Dimensionality reduction is a learning technique that is used when the number of features (or dimensions) in a given data set is too high. It reduces the number of data inputs to a manageable size while also preserving the data integrity. Often, this technique is used in the preprocessing data stage, such as when autoencoders remove noise from visual data to improve picture quality.
The main distinction between the two approaches is the use of labeled data sets. To put it simply, supervised learning uses labeled input and output data, while an unsupervised learning algorithm does not.
In supervised learning, the algorithm “learns” from the training data set by iteratively making predictions on the data and adjusting for the correct answer. While supervised learning models tend to be more accurate than unsupervised learning models, they require upfront human intervention to label the data appropriately. For example, a supervised learning model can predict how long your commute will be based on the time of day, weather conditions and so on. But first, you must train it to know that rainy weather extends the driving time.
Unsupervised learning models, in contrast, work on their own to discover the inherent structure of unlabeled data. Note that they still require some human intervention for validating output variables. For example, an unsupervised learning model can identify that online shoppers often purchase groups of products at the same time. However, a data analyst would need to validate that it makes sense for a recommendation engine to group baby clothes with an order of diapers, applesauce, and sippy cups.
- Goals: In supervised learning, the goal is to predict outcomes for new data. You know up front the type of results to expect. With an unsupervised learning algorithm, the goal is to get insights from large volumes of new data. The machine learning itself determines what is different or interesting from the data set.
- Applications: Supervised learning models are ideal for spam detection, sentiment analysis, weather forecasting and pricing predictions, among other things. In contrast, unsupervised learning is a great fit for anomaly detection, recommendation engines, customer personas and medical imaging.
- Complexity: Supervised learning is a simple method for machine learning, typically calculated by using programs like R or Python. In unsupervised learning, you need powerful tools for working with large amounts of unclassified data. Unsupervised learning models are computationally complex because they need a large training set to produce intended outcomes.
- Drawbacks: Supervised learning models can be time-consuming to train, and the labels for input and output variables require expertise. Meanwhile, unsupervised learning methods can have wildly inaccurate results unless you have human intervention to validate the output variables.
Choosing the right approach for your situation depends on how your data scientists assess the structure and volume of your data, as well as the use case. To make your decision, be sure to do the following:
- Evaluate your input data: Is it labeled or unlabeled data? Do you have experts that can support extra labeling?
- Define your goals: Do you have a recurring, well-defined problem to solve? Or will the algorithm need to predict new problems?
- Review your options for algorithms: Are there algorithms with the same dimensionality that you need (number of features, attributes, or characteristics)? Can they support your data volume and structure?
Classifying big data can be a real challenge in supervised learning, but the results are highly accurate and trustworthy. In contrast, unsupervised learning can handle large volumes of data in real time. But, there’s a lack of transparency into how data is clustered and a higher risk of inaccurate results. This is where semi-supervised learning comes in.
Can’t decide on whether to use supervised or unsupervised learning? Semi-supervised learning is a happy medium, where you use a training data set with both labeled and unlabeled data. It’s particularly useful when it’s difficult to extract relevant features from data—and when you have a high volume of data.
Semi-supervised learning is ideal for medical images, where a small amount of training data can lead to a significant improvement in accuracy. For example, a radiologist can label a small subset of CT scans for tumors or diseases so the machine can more accurately predict which patients might require more medical attention.
Machine learning models are a powerful way to gain the data insights that improve our world. To learn more about the specific algorithms that are used with supervised and unsupervised learning, we encourage you to delve into the Learn Hub articles on these techniques. We also recommend checking out the blog post that goes a step further, with a detailed look at deep learning and neural networks.
- What is Supervised Learning?
- What is Unsupervised Learning?
- AI vs. Machine Learning vs. Deep Learning vs. Neural Networks: What’s the difference?
To learn more about how to build machine learning models, explore the free tutorials on the IBM® Developer Hub .
Get the latest tech insights and expert thought leadership in your inbox.
The Data Differentiator: Learn how to weave a single technology concept into a holistic data strategy that drives business value.
Get our newsletters and topic updates that deliver the latest thought leadership and insights on emerging trends.
- Election 2024
- Entertainment
- Newsletters
- Photography
- Personal Finance
- AP Investigations
- AP Buyline Personal Finance
- AP Buyline Shopping
- Press Releases
- Israel-Hamas War
- Russia-Ukraine War
- Global elections
- Asia Pacific
- Latin America
- Middle East
- Election Results
- Delegate Tracker
- AP & Elections
- Auto Racing
- 2024 Paris Olympic Games
- Movie reviews
- Book reviews
- Personal finance
- Financial Markets
- Business Highlights
- Financial wellness
- Artificial Intelligence
- Social Media
‘Constantly learning’ Imanaga off to impressive start with the Chicago Cubs
Chicago Cubs pitcher Shota Imanaga heads to the tunnel after pitching in the fifth inning of a baseball game against the Pittsburgh Pirates, Saturday, May 18, 2024, in Chicago. (AP Photo/Charles Rex Arbogast)
Chicago Cubs pitcher Shota Imanaga is greeted in the dugout as he heads to the tunnel after pitching in the fifth inning of a baseball game against the Pittsburgh Pirates, Saturday, May 18, 2024, in Chicago. (AP Photo/Charles Rex Arbogast)
Chicago Cubs pitcher Shota Imanaga delivers during the fourth inning of a baseball game against the Pittsburgh Pirates, Saturday, May 18, 2024, in Chicago. (AP Photo/Charles Rex Arbogast)
Chicago Cubs starting pitcher Shota Imanaga winds up during the first inning of a baseball game against the Pittsburgh Pirates, Saturday, May 18, 2024, in Chicago. (AP Photo/Charles Rex Arbogast)
Chicago Cubs pitcher Shota Imanaga delivers during the fifth inning of a baseball game against the Pittsburgh Pirates, Saturday, May 18, 2024, in Chicago. (AP Photo/Charles Rex Arbogast)
Chicago Cubs pitcher Shota Imanaga waits to pitch during the fourth inning of a baseball game against the Pittsburgh Pirates, Saturday, May 18, 2024, in Chicago. (AP Photo/Charles Rex Arbogast)
Chicago Cubs pitcher Shota Imanaga and the infield listen to pitching coach Tommy Hottovy during a mound visit in the seventh inning of a baseball game against the Pittsburgh Pirates, Saturday, May 18, 2024, in Chicago. (AP Photo/Charles Rex Arbogast)
- Copy Link copied

CHICAGO (AP) — The road for Shota Imanaga from Japan to the major leagues included at least one sharp observation that has served him well in his transition to life with the Chicago Cubs.
“Watching foreign players in Japan and how they try to figure out how to get support from the fans, essentially I’m just doing the opposite of that, coming over here,” Imanaga said through a translator. “It was something I thought about.”
From his entertaining pitching style to his trips to Dunkin’ Donuts — “Either I order a small iced latte or a medium,” he said — Imanaga has moved with a purpose in his acclimation to the big leagues. And he is making it look easy at the moment.
Relying on a deceptive four-seam fastball that he usually locates at the top of the strike zone, along with a splitter that plays at the bottom, Imanaga is 5-0 with a 0.84 ERA for the contending Cubs. The left-hander also has 58 strikeouts and nine walks in 53 2/3 innings — thrusting himself into the early conversation for NL Rookie of the Year and the Cy Young Award.
Imanaga’s ERA is the lowest for a major leaguer for his first nine career starts — excluding openers — since baseball started tracking earned runs in 1913. Fernando Valenzuela had the previous mark at 0.91 in his second year with the Los Angeles Dodgers in 1981.
It’s the fourth-lowest ERA since 1920 for any pitcher in his first nine starts in a season, trailing Jacob DeGrom (0.62 in 2021), Hall of Famer Juan Marichal (0.69 in 1966) and Zack Greinke (0.82 in 2009).
So far, Imanaga has been better than Chicago ever could have expected when it signed him to a $53 million, four-year contract in January.
“The way he thinks about himself as a pitcher, the way he talks through his first few starts here, he’s thoughtful about everything,” said Jed Hoyer, the president of baseball operations for the Cubs. “I think he knows he can continue to get better and continue to refine stuff. It’s been a great start, and I am glad people have sort of gravitated towards watching him.”
Have they ever.
Imanaga, who takes the mound in throwback-style high socks, is already a fan favorite in sports-crazy Chicago. But his early success is only part of the growing connection between the Japanese lefty and the passionate residents of Wrigley Field.
It started when Imanaga — wearing No. 18 in honor of Ben Zobrist, who was the World Series MVP when the Cubs won the title in 2016 — recited a couple of lines from the song “Go Cubs Go” during his introductory news conference.
He warms up to The Fratellis’ “Chelsea Dagger,” the goal-scoring song for the NHL’s Chicago Blackhawks. He has openly mused about switching his alarm to the sound of cheering fans at Wrigley to help him get started in the morning.
“What impresses me the most is how (much) fun he’s having here,” San Diego Padres pitcher Yu Darvish said through a translator. “Not just pitching ... but how he’s adjusting to life in the U.S. I think he’s really taking it in and enjoying it, so that part makes me happy, too.”
As Imanaga prepared for life in the majors, he spoke with Darvish about his experience. Imanaga and Darvish were teammates when Japan won last year’s World Baseball Classic , beating the U.S. in the final. The 37-year-old Darvish, who played for Chicago for three seasons, made his big league debut with Texas in 2012.
Imanaga, 30, also did his homework on Chicago and the Cubs organization before signing with the team.
“He’s in a place in life where he’s very sure who he is, and that’s been evident kind of from early on,” Cubs manager Craig Counsell said. “He’s very confident in his choices, very confident in the people he puts around him. ... I think he is looking for the experience of what can I enjoy from a new country, and I think you take that perspective and it makes the experience a really good one.”
Imanaga went 7-4 with a 2.80 ERA in 22 starts in his final season with the Yokohama BayStars of Japan’s Central League, finishing with 174 strikeouts and 24 walks in 148 innings. Nicknamed “The Throwing Philosopher,” he also wrote a book on pitching that was published last year.
“I’m always constantly learning,” Imanaga said about the book-writing process. “But the fact that I’m trying to put out all the information, I think that helped me understand more about myself.”
The 5-foot-10 Imanaga averages 92 mph on his four-seamer — not exactly noteworthy in a sea of high-90s fastballs — but it has a high spin rate and an unusual amount of vertical movement. Hitters likely will adjust over time, forcing Imanaga to take a closer look at his approach.
But Imanaga and the Cubs are confident he is up to the challenge, especially with how he embraces the advanced metrics of the sport.
“Compared to Japan, I mean they do hand out data, but here, they’ll give us data and they’ll have a plan going along with it,” Imanaga said. “I like that.”
AP MLB: https://apnews.com/hub/mlb
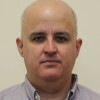
'Constantly learning' Imanaga off to impressive start with the Chicago Cubs
From his entertaining pitching style to his trips to Dunkin’ Donuts, Chicago Cubs pitcher Shota Imanaga has moved with a purpose in his transition to the major leagues
CHICAGO — The road for Shota Imanaga from Japan to the major leagues included at least one sharp observation that has served him well in his transition to life with the Chicago Cubs.
“Watching foreign players in Japan and how they try to figure out how to get support from the fans, essentially I’m just doing the opposite of that, coming over here,” Imanaga said through a translator. “It was something I thought about.”

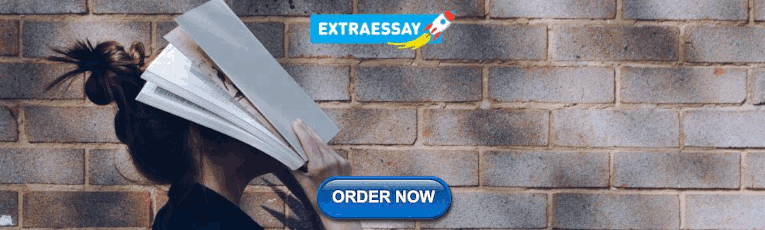
IMAGES
VIDEO
COMMENTS
her learning style preference. This questionnaire consists of thirty items that represent six learning style preferences, namely Visual, Auditory, Kinesthetic, Tactile, Group, and Individual. Five items for each learning style category were arranged randomly. Items 6,10,12,24,29 are Visual, items 1,7,9,17,20 are Auditory, items 2,8,15,19,26 are
Universities strive to ensure quality education focused on the diversity of the student body. According to experiential learning theory, students display different learning preferences. This study has a three-fold objective: to compare learning styles based on personal and educational variables, to analyze the association between learning styles, the level of academic performance, and ...
LEARNING STYLES ON ACADEMIC ACHIEVEMENT: THE MEDIATION EFFECTS OF LEARNING STYLES IN POST SECONDARY EDUCATION A dissertation submitted in partial fulfillment of the requirements for the degree of DOCTOR OF PSYCHOLOGY to the faculty of the DEPARTMENT OF PSYCHOLOGY of ST. JOHN'S COLLEGE OF LIBERAL ARTS AND SCIENCES at ST. JOHN'S UNIVERSITY
Thesis (Ed. D.)--Texas Tech University, 1999. Includes bibliographical references (leaves 108-116). ... Learning styles has been delineated as a sophisticated method within which the learner ought ...
The learning styles of students should influence the assessment and the teaching of students. Assessment works as a continuum. Aiding students with their individual ... institutions of higher learning. The thesis of learning styles is that individuals vary considerably in how they learn. Any given person has academic or learning strengths that ...
Learning styles are critical to educational psychology, especially when investigating various contextual factors that interact with individual learning styles. Drawing upon Biglan's taxonomy of academic tribes, this study systematically analyzed the learning styles of 790 sophomores in a blended learning course with 46 specializations using a novel machine learning algorithm called the ...
Learning styles research is used in human resource management, sales, team development, counseling, academic applications, and many other fields (Kevin, 2010). Within the Academic applications of learning styles research, there are two general applications of learning styles information that affect classroom instruction and student learning.
Riding and Cheema's Fundamental Dimensions. Having identified in excess of 30 labels used to describe a variety of cognitive and learning styles, Riding and Cheema (Citation 1991) propose a broad categorisation of style according to two fundamental dimensions representing the way in which information is processed and represented: wholist-analytic and verbaliser-imager.
their learning styles, the dominant ones are participant with a coefficient value of 0.36 and collaborative with a coefficient value of 0.3162. The least one is inde-pendent learning style as indicated by the coefficient value of 0.01. This finding discloses that the majority of the students in THE TLE program are both partici-
This study aimed to determine the relationship of pupils' learning styles and their academic performance in their major subjects namely: English, Filipino, Mathematics and Science. The respondents consisted of the 313 intermediate pupils of Central Philippine University Elementary School. The instrument used in this study was a teacher- made questionnaire which was administered personally by ...
a study of the preferred learning styles of students taking the english 1119 paper in smk tengku intan zaharah: are the teachers aware of these learning styles? November 2012 DOI: 10.13140/RG.2.1 ...
A learning style is defined as the characteristics, strengths and preferences in how people receive and process information (Felder & Silverman, 1988;Allinson & Hayes, 1996;Felder & Brent, 2005 ...
The purpose of this study is to investigate learning styles in the elementary music classroom. You will be given a fourth grade general music lesson to teach over the course of two weeks. You and your selected fourth graders will be observed (by video record) during a two-week treatment period.
Learning styles are different between the two sexes. There is evidence that shows woman's learning style depends more on sympathy, collaboration and careful listening (Grasha, 1996). Learning styles theory suggests changing the traditional instructional methods to benefit from the individuals learning styles.
Understanding learning styles can become an essential tool to implement new learning procedures. Knowledge of individual learning style preferences will help students see themselves as learners and the awareness of their learning style preferences can lead to improving performance and learning outcomes (Claxton & Murrell, 1987).
ScholarWorks@GVSU | Grand Valley State University Research
A learning style is a term used to describe the ways in which people gather, interpret, and store information. Each style can be broken down into a category based on sensory needs: auditory, visual, and tactile. The presumption is that you will best retain the information presented to you if the conditions of your learning style meet.
Understanding different learning styles is important for creating an optimal educational and professional environment. Recognising if someone or oneself is a visual, auditory, kinesthetic, or reading/writing learner allows you to adjust your teaching approaches for better understanding and retention. This article delves into these major ...
"The Journey to Learning: Through the Learning Styles of the Senior High School Academic Strand Students A.Y. 2019-2020" March 2020 DOI: 10.13140/RG.2.2.10443.62240
Understanding the stressors and coping strategies of nursing students in their first clinical training is important for improving student performance, helping students develop a professional identity and problem-solving skills, and improving the clinical teaching aspects of the curriculum in nursing programmes. While previous research have examined nurses' sources of stress and coping styles ...
Let's look at the learning styles and needs of the four MBTI types least likely to graduate: For an ESTP, learning is meaningful when: It's active and hands-on. Expectations are clear and feedback is efficient. Professors are straightforward and businesslike. There are sequential "how to" steps with real-life applications.
Your thesis must be something that can be argued and proved by your research. I like the idea a previous poster had connecting the need to teach to learning styles even though standardized testing ...
How personalized learning strategies foster better leadership at any career stage When Eleanor* embarked on her leadership journey with me, it quickly became apparent that she was a high performer ...
The main difference between supervised and unsupervised learning: Labeled data. The main distinction between the two approaches is the use of labeled data sets. To put it simply, supervised learning uses labeled input and output data, while an unsupervised learning algorithm does not. In supervised learning, the algorithm "learns" from the ...
As Imanaga prepared for life in the majors, he spoke with Darvish about his experience. Imanaga and Darvish were teammates when Japan won last year's World Baseball Classic, beating the U.S. in the final. The 37-year-old Darvish, who played for Chicago for three seasons, made his big league debut with Texas in 2012.
From his entertaining pitching style to his trips to Dunkin' Donuts, Chicago Cubs pitcher Shota Imanaga has moved with a purpose in his transition to the major leagues