
An official website of the United States government
The .gov means it’s official. Federal government websites often end in .gov or .mil. Before sharing sensitive information, make sure you’re on a federal government site.
The site is secure. The https:// ensures that you are connecting to the official website and that any information you provide is encrypted and transmitted securely.
- Publications
- Account settings
Preview improvements coming to the PMC website in October 2024. Learn More or Try it out now .
- Advanced Search
- Journal List
- J Diabetes Investig
- v.12(11); 2021 Nov
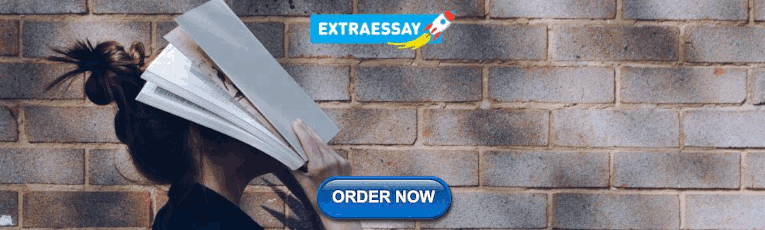
Recent updates and future perspectives on gestational diabetes mellitus: An important public health challenge
Leo check hei chu.
1 Department of Medicine and Therapeutics, The Chinese University of Hong Kong, Hong Kong China
Takashi Sugiyama
2 Department of Obstetrics and Gynecology, Ehime University Graduate School of Medicine, Toon, Ehime Japan
Ronald Ching Wan Ma
3 Hong Kong Institute of Diabetes and Obesity, The Chinese University of Hong Kong, Hong Kong China
4 Li Ka Shing Institute of Health Sciences, The Chinese University of Hong Kong, Hong Kong China
Leo Chu, Takashi Sugiyama, and Ronald Ma provide their perspective on recent updates on research in gestational diabetes mellitus, including updates on the epidemiology, diagnosis, and long‐term follow‐up of women with gestational diabetes mellitus. They also provide a summary of current challenges and a perspective on research gaps on this important clinical and public health problem.

Gestational diabetes mellitus (GDM) is the most common form of dysglycemia during pregnancy. It is defined as hyperglycemia diagnosed for the first time during pregnancy, most commonly during the second or third trimester. GDM can be harmful to both the mother and the offspring. Complications to fetuses include excessive birthweight and preterm birth, while women with GDM are more susceptible to gestational hypertension, pre‐eclampsia, and postpartum abnormal glucose intolerance (pAGT). Due to much debate (and confusion) over diagnostic criteria over the past decade 1 , studies on the prevalence and incidence of GDM have been somewhat limited, and sometimes difficult to compare across different populations.
As a result of the rapid economic developments in recent years, the prevalence of diabetes mellitus (DM) and GDM have increased in many Asian countries 2 . Furthermore, improved healthcare awareness and the impact of GDM have also led to more screening and diagnosis, including an increasing number of countries adopting the universal screening of GDM in pregnant women. China has a large population and diverse ethnicity. People living in different provinces adopt a different lifestyle and diet. Thus, the prevalence of GDM in mainland China might differ from area to area. Previously, there have been regional studies analyzing the prevalence of GDM in mainland China, but no systematic nationwide analysis has been conducted. A recent study conducted a systemic meta‐analysis on the prevalence of GDM in mainland China 3 using the 2010 International Association of the Diabetes and Pregnancy Study Groups (IADPSG) diagnostic standard, which has subsequently been adopted by the World Health Organization (WHO) in 2013 and the International Diabetes Federation (IDF), International Federation of Gynecology and Obstetrics (FIGO) in 2015 1 . The systematic review included 25 studies from 21 regions in mainland China. Data from 79,064 participants spanning the time period from 2010 to 2017 were included, and the total incidence rate was 14.8%. Subgroup data revealed that women with a higher maternal age and weight are more susceptible to GDM. Moreover, those with a family history of DM are three times more likely to have GDM compared with those who do not, highlighting the importance of genetic factors. This study is one of the first of its kind, providing a comprehensive view of the prevalence of GDM in China. This can be crucial for strategic public health planning at the national level.
Another recent study investigated the prevalence of GDM (according to the 2014 National Health and Family Planning Commission of the People’s Republic of China criteria, which is consistent with the IADPSG 2010/WHO 2013 criteria) among 78,572 pregnant women in Xiamen, China, and revealed a prevalence rate of 17.6% 4 . The minor difference in prevalence estimates between this and the earlier mentioned study may be attributed to regional, ethnic, dietary, and lifestyle differences. Results from the subgroup analysis in this study is similar to that in the systematic review, with the incidence rate higher among pregnant women with a higher maternal age, BMI, and a family history of diabetes or hypertension. These results further highlight maternal age, weight gain in early pregnancy and systolic blood pressure as important determinants of GDM.
Gestational diabetes mellitus can lead to metabolic complications in both the mother and the offspring. Therefore, prevention, earlier identification, and treatment in clinical practice are especially important. There have been many studies that have investigated the effectiveness of using conventional risk markers such as age, weight, family history, etc. for the prediction of GDM. However, no risk scores have been universally accepted for use in different populations. Some other biomarkers being proposed include body mass index (BMI), hemoglobin A1c (HbA1C), and different hormones, but these factors alone are not sufficiently strong to provide a robust prediction of GDM. A recent study from Japan aimed to identify new predictive biomarkers for GDM using a metabolomic approach 5 . Levels of metabolites in serum and urine samples of 121 participants with GDM were analyzed using hydrophilic interaction chromatography tandem mass spectrometry. Their samples were then compared with that of the control group. The investigators identified three metabolites, namely glutamine in serum, and ethanolamine and 1,3‐diphosphoglycerate in the urine, which are associated with the risk of GDM. When combined, the panel of biomarkers had an area under the receiver operating characteristic curves of >0.8. The metabolites identified in urine could play an important role in amino acid metabolism and the pentose phosphate pathway and relate to subsequent GDM, but the exact relationship between the metabolites, metabolic pathways, and the pathogenesis of GDM still remains unclear. Nonetheless, with more research and independent validation, this new metabolomic approach could open a new avenue in predicting GDM accurately and, thus making early prevention possible. It is also important to note that metabolomic markers among subjects with GDM of different ethnicities may differ, as well as according to the underlying pathophysiology of GDM. Therefore, much work remains to be done to identify biomarkers predictive of GDM, including a systematic comparison with established risk factors.
There has been a long‐standing debate on the diagnostic criteria of GDM, though it appears that a consensus is finally emerging, with most national and international guidelines now adopting the 2010 IADPSG criteria for diagnosis 1 . Other factors such as at what gestational age the test is taken can also affect the diagnostic results. At <24 weeks, the IAPDSG criteria do not recommend performing a 75 g oral glucose tolerance test (OGTT), due to insufficient data regarding thresholds 6 . Instead, the IADPSG defines a fasting plasma glucose of 92–125 mg/dL (5.1–7.0 mmol/L) at <24 weeks as diagnostic of GDM. At 24–28 weeks gestation, a 75 g OGTT is performed and GDM is defined when at least one of the following three values are met: fasting plasma glucose (PG) level 92–125 mg/dL, 1 h PG level ≥180 mg/dL (10.0 mmol/L), 2 h PG level ≥153 mg/dL (8.5mmol/L) 6 . The 2010 Japanese Society of Obstetrics and Gynecology (JSOG) criteria has adopted these diagnostic thresholds for OGTT, regardless of gestational age. It states that GDM is defined when at least one of the following three values is met at any gestational age: fasting PG level 92–125 mg/dL, 1 h PG level ≥180 mg/dL (10.0 mmol/L), 2 h PG level ≥153 mg/dL (8.5mmol/L). A recent study conducted in Japan examined how the gestational age at the time of the 75 g OGTT could affect the prevalence of GDM 7 . The study compared 2,578 pregnant women according to the timing of their OGTT, into three groups: <14 weeks, 14–23 weeks, and 24–32 weeks. The results indicated that fasting glucose was higher among the early OGTT group (<14 weeks), and participants had higher chances of being diagnosed with GDM based on the 2010 JSOG criteria if they had undergone a 75 g OGTT at <14 weeks gestation compared with those who had undergone the test at 24–32 weeks gestation (adjusted odds ratio 1.25, 95% confidence interval 1.07–1.46). On the other hand, based on the 2010 IADPSG criteria, women whose fasting PG levels were examined at <24 weeks had a lower chance of being diagnosed with GDM than those who did a 75 g OGTT at 24–28 weeks gestation. Several previous studies highlighted that fasting PG levels, 1 h and 2 h PG levels at 75 g OGTT all change during pregnancy depending on the gestational age. Therefore, applying the same threshold to diagnose GDM at earlier gestational age might not be accurate. More studies are required in order to evaluate what would be appropriate cut‐off values for the 75 g OGTT should it be implemented earlier in pregnancy, and the potential implications of earlier screening and earlier intervention.
Gestational diabetes mellitus is associated with long term metabolic disorders in the mother. In particular, women with GDM are at substantially increased risk of type 2 diabetes 8 . Thus, follow‐up is recommended for those who had a history of GDM. A long‐term study was conducted in Hong Kong to determine the maternal cardiometabolic outcomes 22 years after GDM diagnosis (by WHO 1999 criteria) 9 . Eighty participants with normal glucose tolerance (NGT) during pregnancy and 38 others with GDM/gestational impaired glucose tolerance (GIGT) completed the 22 years follow up. At 8 years postpartum, 40.3% of those in the GDM/GIGT group developed pAGT. The percentage increases to approximately 53% at 22 years postpartum. At the same time, for the NGT group, the percentage of the group having pAGT later on also increased from 17.7% at 8 years postpartum to 30% at 22 years postpartum. Remarkably, the fasting and 2 h glucose values during OGTT in pregnancy were associated with different glucose parameters and total glucose area under the curve at OGTT 22 years later. Those with a GDM/GIGT history had lower indices of β‐cell function at OGTT at both 8 and 15 years postpartum, highlighting the role of impaired β‐cell function.
The identification of risk factors for postpartum abnormal glucose tolerance (pAGT) could help to identify those who have a high risk of developing pAGT, and facilitate the implementation of measures such as dietary and lifestyle changes to prevent the onset of pAGT after pregnancy. A recent study analyzed the clinical and genetic characteristics of 213 Japanese women who had a recent history of GDM, and determined how these characteristics were associated with pAGT 10 . In this study cohort, the prevalence of pAGT was 28% on OGTT at a median 24.9 weeks postpartum. As aforementioned, the 2010 IADPSG criteria could be used to diagnose GDM based on the three threshold values of 75 g OGTT. Results from this study showed that among the 142 women who had a single abnormal OGTT value, 33 of them (23.2%) had pAGT. This indicated that even for patients who had a relatively less severe case of GDM with only one abnormal value during OGTT, the risks of pAGT should not be overlooked. The study identified four metabolic parameters from the antepartum OGTT profile that could serve as antenatal predictors of pAGT, including 1 h PG, 2 h PG, insulinogenic index (IGI) and insulin secretion‐sensitivity index‐2 (ISSI‐2). The IGI in the pAGT group was significantly lower than that in the normal glucose tolerance (NGT) group, highlighting the relationship between impaired β‐cell function and a later risk of pAGT. The other three parameters are also closely related to β ‐cell capacity for insulin secretion. Additionally, the genotyping of single‐nucleotide polymorphism (SNPs) at 45 type 2 diabetes susceptibility loci were conducted to investigate the relationship between these candidate genetic variants and pAGT. Four SNPs showed a nominally significant association with the occurrence of pAGT, including rs266729 ( ADIPOQ ), rs6017317 ( HNF4A ), rs5215 ( KCNJ11 ), and rs7177055 ( HMG20A ). A genetic risk score of 0–8 was constructed according to the number of risk alleles each individual carried at these four SNPs. The risk of pAGT increased according to the number of risk alleles (OR 1.91, 95% CI 1.40–2.61, P = 0.00005). Of note, three of the four aforementioned candidate genes were related to insulin‐secretion, highlighting the link between impaired insulin secretion and pAGT. Although there is much ongoing work exploring how to improve the prediction of pAGT, this study demonstrated the potential to utilize clinical characteristics and genetic markers to predict future pAGT.
Although GDM represents the main form of hyperglycemia in pregnancy, glucokinase‐maturity‐onset diabetes of the young (GCK‐MODY) is another form of hyperglycemia caused by inherited mutations in the glucokinase gene. Generally, complications are rare and medical treatments are seldom needed, except for pregnant women with GCK‐MODY because the disorder may affect the offspring, resulting in perinatal complications. A recent study analyzed the pregnancy outcome of Japanese patients with GCK‐MODY, who were treated with either insulin injections or diet intervention during pregnancy. The study revealed that for the unaffected offspring born to an affected mother, insulin treatment would result in a lower birthweight (3025 g vs 3593 g) 11 . For affected offspring born to affected mothers, birthweight was also lower in the insulin‐treated group (2532 g vs 2800 g). Importantly, perinatal complications, including small‐for‐gestational age, occurred only among affected offspring born to insulin‐treated mothers. The authors therefore do not recommend routine insulin treatment in mothers with GCK‐MODY during pregnancy, and suggest close monitoring with serial ultrasound to monitor fetal growth remains an appropriate way to approach GCK‐MODY during pregnancy.
The present article highlights some findings from recent studies on GDM, especially from the pages of Journal of Diabetes Investigation (JDI). The large burden of GDM, whereby more than 1 in every 10 pregnancies in the region are affected, will present significant public health challenge to most countries. Some of the challenges and key research questions relating to GDM are summarized in Figure 1 . Given the large burden and significant long‐term consequences to the mother and offspring, strategies to identify at‐risk individuals early, and to implement intervention to reduce the associated complications, seem warranted but require formal evaluation in clinical trial settings. Reducing hyperglycemia in pregnancy may also have beneficial effects on reducing the intergenerational effects on the risk of obesity and diabetes in the offspring. Some biomarkers such as metabolomic markers are emerging as promising candidates to facilitate the identification of individuals at risk of GDM. Given the increased risk of diabetes and other non‐communicable diseases later in the life course in women with GDM, how to improve postpartum screening, and engage women to reduce future progression to pAGT and cardiovascular disease are most important, with a significant public health impact.

Current challenges and research gaps in relation to gestational diabetes. Whilst pregnancy only represents a short time window in the life course of a woman, it represents a particularly important window of opportunity to address the risk of diabetes and other NCDs, especially among women affected by gestational diabetes mellitus. Some of the current challenges and research gaps in GDM are highlighted according to the different stages of pregnancy. The schematic diagram of the pregnant mother with the fetus highlights the potential for intergenerational effects on the future risk of diabetes and obesity in offspring of mothers with GDM. There is much that can be achieved post‐partum, including the need to encourage breastfeeding, to remind mothers to undergo a postpartum oral glucose tolerance test (OGTT), followed by subsequent periodic testing for dysglycemia, and efforts in diabetes prevention given the increased risk of diabetes among women with a history of GDM. GDM, gestational diabetes mellitus; DM, diabetes mellitus; MODY, maturity‐onset diabetes of the young; NCDs, non‐communicable diseases. Pregnancy 1, first pregnancy; Pregnancy 2, second pregnancy.
The author declares no conflict of interest.
Approval of the research protocol: N/A.
Informed consent: N/A.
Registry and the registration no. of the study/trial: N/A.
Animal studies: N/A.
ACKNOWLEDGMENT
RCWM acknowledge support from a Research Grants Council General Research Fund (ref. 14118718).
J Diabetes Investig . 2021; 11 : 1944–1947 [ PMC free article ] [ PubMed ] [ Google Scholar ]
Contributor Information
Takashi Sugiyama, Email: pj.ca.u-emihe.m@amayigus .
Ronald Ching Wan Ma, Email: kh.ude.khuc@amwcr .
- Open access
- Published: 15 March 2024
Prevalence of gestational diabetes in the United States and Canada: a systematic review and meta-analysis
- Claire E. Eades 1 ,
- Katherine A. Burrows 1 ,
- Roza Andreeva 1 ,
- Daniel R. Stansfield 2 &
- Josie MM. Evans 1 , 3
BMC Pregnancy and Childbirth volume 24 , Article number: 204 ( 2024 ) Cite this article
1073 Accesses
Metrics details
An understanding of the prevalence of gestational diabetes mellitus among pregnant women is essential at local, national and international level so that appropriate health care interventions can be planned, financed and delivered.
A systematic review and meta-analysis of primary research reporting the prevalence of gestational diabetes mellitus in Canada or the United States were carried out according to Meta-analysis of Observational Studies in Epidemiology guidelines. Four electronic databases were systematically searched in June 2023 to identify articles that reported gestational diabetes mellitus prevalence using universal screening in pregnant women from eligible general population samples. Estimates were combined using a random effects model, and the effects of moderator variables analysed.
There were 36 separate samples of women or deliveries (total sample size 1,550,917). Overall mean prevalence of gestational diabetes mellitus was 6.9% (95% CI: 5.7–8.3); 13.7% (95% CI: 10.7–17.3) in studies using a one-step screening strategy, and 5.2% (95% CI: 4.4–6.1) in those using a two-step strategy. Heterogeneity in technical methods between studies produced differences in estimates, as did different diagnostic thresholds used.
Conclusions
The meta-analysis suggests a slightly higher prevalence of gestational diabetes mellitus in Canada and the United States, compared to Europe, but highlights the need for standardised protocols for estimating gestational diabetes mellitus prevalence.
Peer Review reports
Gestational diabetes mellitus (GDM) is defined as elevated blood glucose levels that are first diagnosed in pregnancy [ 1 ]. Women with GDM are more likely to experience caesarean section or preterm delivery and babies born to women with GDM are at a greater risk of macrosomia, respiratory distress, neonatal jaundice, admission to neonatal care and type 2 diabetes in later life [ 2 , 3 ]. In addition to the adverse outcomes during pregnancy and delivery, women with GDM are at an estimated 8-fold risk of developing type 2 diabetes compared to women who have not had GDM [ 4 ]. Up to 70% of women with GDM develop type 2 diabetes, with the risk being greatest in the first five years following pregnancy and then plateauing at around 10 years [ 5 , 6 ], but a diagnosis of GDM represents an opportunity for interventions to reduce type 2 diabetes risk [ 7 ].
It is thought that around 14% of pregnant women worldwide are affected by GDM but differences in screening approaches and diagnostic criteria result in variable estimates [ 8 ]. The diagnostic criteria used by clinicians for the diagnosis of GDM vary considerably worldwide, and have also changed over time. In the past diagnostic criteria were based on criteria for glucose intolerance in non-pregnant individuals or thresholds were decided based upon prediction of future type 2 diabetes risk in the mother, but more recently there has been an increasing focus on diagnostic thresholds that are based upon their predictive value for adverse outcomes in pregnancy [ 9 ].
A clear understanding of GDM is essential at local and national level so that health care interventions can be planned, financed and delivered for this group. A recent study of 51 population-based studies worldwide estimated global prevalence to be 4.4% (95% CI 4.3–4.4%) [ 10 ]. Our recent meta-analysis in developed countries in Europe yielded a prevalence estimate of 5.4% (95% CI 3.8–7.8%) [ 11 ] and another reviewing data from all European countries reported prevalence of 10.9% (95% CI 10-11.8%) [ 12 ].A meta-analysis in Eastern and South-eastern Asia yielded an estimate of 10.1% (95% 6.5–15.7%) [ 13 ] and another in Africa reported prevalence of 13.6% (95% CI 11-16.2%) [ 14 ]. However, there has been no review of prevalence of GDM specifically in the US or Canada. We have therefore conducted a systematic review and meta-analysis of observational studies that have assessed the prevalence of GDM in the general population of pregnant women in the US or Canada, regardless of the specific diagnostic criteria used. We have calculated an overall prevalence estimate for GDM and examined variables that could have influenced this estimate.
The systematic review and meta-analysis were conducted according to the Meta-analysis of Observational Studies in Epidemiology (MOOSE) guidelines [ 13 ].
Data sources
A search was carried out in the databases MEDLINE, CINAHL, Health Source and PsycInfo in June 2023 with no limit on the age of articles For each database the following search terms were used: (prevalence or incidence) and (gestational diabetes or diabetes in pregnancy or gestational diabetes mellitus) and (United States or America or US*or Canada).
Study selection
The titles and abstracts of all articles were screened by one author (DS) and independent screening was split between two other authors, with JE screening half and CE screening the other half. The full texts of papers were retrieved for studies that were considered relevant, but also for those that contained insufficient information to allow judgement of relevance. These were checked against the inclusion criteria by CE and independently by JE. Where there were disagreements between authors about the inclusion of a paper, the full text of the paper was retrieved, and a consensus was reached through discussion. The reference lists of included papers were checked to identify any other potentially relevant papers but experts in the field were not contacted due to the time-consuming nature of this process.
Articles were required to meet the following inclusion criteria.
Study Design
Observational study published in English.
General population of pregnant women living in the US or Canada. In this context, general population referred to a sample of women not defined by clinical or other non-demographic characteristics.
Outcome measures
Prevalence of GDM diagnosed using universal screening carried out in the second or third trimester, using either an Oral Glucose Tolerance Test (OGTT) alone or two step screening with glucose challenge test (GCT) followed by an OGTT.
Data extraction and quality assessment
Data from included papers were extracted by two authors (half by CE and half by JE) using a data extraction form based on the template provided by the Centre for Reviews and Dissemination [ 14 ]. The extracted data were independently checked by two other authors (KB,RA). The following information was recorded for each included study: first author, journal name, year of publication, country, dates of data collection, study sample type, study design, age range of sample, ethnicity, body mass index (BMI), sample size, type of screening and diagnostic test carried out, and diagnostic criteria used for GDM.
The outcome measures extracted were the number and proportion of the sample with GDM and, where reported, these measures stratified by demographic factors such as ethnicity and age. Ethnic make-up of the sample was defined as unknown or mixed, unless one ethnic group comprised more than 70% of the sample, in which case it was allocated to that ethnic grouping.
Where possible, confidence intervals for prevalence estimates were calculated by the authors if these were not reported. Where there was more than one paper published from the same sample, only the paper reporting the most complete and definitive results was included. In cases where a study reported prevalence estimates according to different diagnostic criteria only one prevalence estimate was included in the analysis to avoid dependency effects. The prevalence estimate selected was that derived from the criteria that were most commonly used in other papers included in the review, to maximise comparability. For studies reporting multiple prevalence estimates by other factors, such as age or year, an average of the estimates was calculated and used in the analysis.
Included studies quality assessed using a checklist based upon the example published by the Joanna Briggs Institute [ 15 ] which was specifically designed for assessment of quality in systematic reviews of prevalence and incidence. Quality assessment was completed for all included papers by one author (CE) and a list of all identified weaknesses was compiled. The list was then discussed by two authors (CE and JE). A decision was made to exclude any papers with significant weaknesses, one of which was a participation rate of less than 70%. Participation rates can be defined in many ways but for this review the participation rate (recoded during data extraction if necessary and possible) was the proportion of eligible women sampled who completed testing for GDM. Papers were also excluded if sample size was less than 500, if it was not clear that screening was universal, or if it was not possible to determine whether the population was a ‘general’ population. Other less important weaknesses were common in the papers. These included not explicitly reporting women’s gestation at testing, limited description of the study sample, not reporting differences between participants and non-participants, not reporting details of who carried out glucose testing and not reporting confidence intervals. Papers with these weaknesses were retained.
Data synthesis and analysis
The meta-analysis was carried out using the Comprehensive Meta-Analysis software version 3.3.070 (Biostat, Englewood, NJ). The proportion of women or deliveries with GDM in each study was transformed into a logit event rate effect size and the standard error was calculated [ 16 ]. After analysis, the logits were retransformed to proportions. Combined effect sizes were calculated, and analyses were carried out that either included or excluded outlying logit event rates. No significant differences were found between these analyses, so the outliers were initially retained.
A random effects model was used to combine studies for significance testing and moderator analysis in a meta-regression, thereby allowing for the possibility that there were random differences between studies due to factors such as variation in procedures, measures or settings, alongside differences due to sampling error. This accords with evidence suggesting that the variability in reported prevalence for GDM may be the due to different methodologies and criteria [ 2 ]. The Q test was used to assess the homogeneity of studies, for which the null hypothesis states that variability of the effect sizes is due to sampling error only. If the assumption of homogeneity is violated, sources of variation can be explored by studying moderator variables. Categorical moderator variables in this study were analysed using an analysis of variance for meta-analysis, and tests of interaction used to explore differences between subgroups of these variables. The between study homogeneity statistic (Q B ) reflects the amount of heterogeneity that can be attributed to the moderator variable. The within study homogeneity statistic indicates the degree of heterogeneity that remains in the category in question (Q W ) and the I 2 statistic shows the proportion of the variation that is due to heterogeneity rather than sampling error. Finally, a weighted multiple regression was carried out to assess which moderator variables made the greatest contribution to the variability in prevalence of GDM.
Description of included studies
Figure 1 shows a PRISMA flow diagram of studies identified by the search. The search identified 4,229 abstracts of which 504 were potentially relevant after title and abstract screening. The full text articles were retrieved and assessed against the inclusion criteria, with 54 retained for quality assessment. Following assessment, a further 25 articles were excluded for the following reasons: eight were subsets or repeated samples of other included studies [ 17 , 18 , 19 , 20 , 21 , 22 , 23 , 24 ], six were cohort studies in which participants were invited to take part but eligibility criteria and/or participation rate were unclear [ 25 , 26 , 27 , 28 , 29 , 30 ], two had sample sizes of less than 500 [ 31 , 32 ], four provided insufficient information on how the sample was derived [ 33 , 34 , 35 , 36 ], four used different methods to diagnose GDM within the same study without separate reporting [ 37 , 38 , 39 , 40 ], and one did not provide the required unadjusted data [ 41 ].
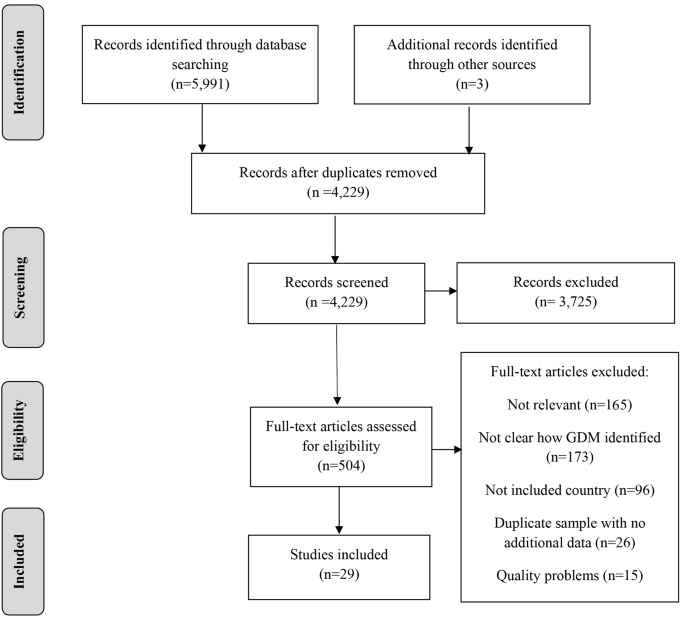
PRISMA flow diagram showing study selection
The resulting 29 studies yielded prevalence estimates for 36 separate samples of women, pregnancies or deliveries, giving a total sample size of 1,550,917 [ 42 , 43 , 44 , 45 , 46 , 47 , 48 , 49 , 50 , 51 , 52 , 53 , 54 , 55 , 56 , 57 , 58 , 59 , 60 , 61 , 62 , 63 , 64 , 65 , 66 , 67 , 68 , 69 , 70 , 71 ]. The characteristics of the studies are presented in Table 1 . In general, studies tended to fall into one of two categories. Some studies reported data that had been collected specifically for the estimation of GDM prevalence or were available through other related ad hoc research projects. Alternatively, some studies reported analyses of routinely collected data that were available either as part of national datasets or to support the operation of large Health Maintenance Organisations (HMOs).
Nine studies (11 samples) were from Canada; the rest were from the US. Most of the studies used a two single step screening strategy, with all women screened first with a GCT, followed by an oral glucose challenge test (OGTT) if indicated. A one-step screening strategy was used in nine samples. Thresholds for GDM diagnosis with an OGTT also varied. We divided the studies into five categories, according to the diagnostic cut-offs that were used in the study (Table 1 ).
The most commonly used diagnostic criteria [ 72 ] were those of the National Diabetes Data Group (NDDG) which were used to diagnose GDM in ten studies as part of two-step screening and one study using a one-step strategy. Carpenter-Coustan criteria were used in eight studies, all using a two-step strategy. Two studies used O’Sullivan criteria within a two-step strategy, and five used thresholds according to Canadian guidelines (1998) [ 73 ], all of which one used a two-step strategy. The IADPSG criteria were applied in three studies, all using a one-step strategy. The diagnostic thresholds used by studies in this meta-analysis are shown in Table 2 .
Mean prevalence of GDM
The overall mean prevalence of GDM in the meta-analysis including all studies was 6.9% (95% CI: 5.7–8.3). There were three outliers identified: studies that yielded prevalence estimates of 23.3%, 24.1% and 27.4%, all of which used IADPSG diagnostic thresholds. When these outliers were excluded, the prevalence estimate was 5.8% (95% CI: 5.0-6.8). Because this difference was not statistically significant, the outliers were initially retained in subsequent analyses. However, there was a statistically significant difference between studies that used a one-step or two-step screening strategy. The mean GDM prevalence using a one-step strategy was 13.7% (95% CI: 10.7–17.3) compared to 5.2% (95% CI: 4.4–6.1) for studies using a two-step strategy. For this reason, all subsequent analyses were conducted using studies that used a two-step strategy only (with the result that the outliers were also excluded).
Moderator analyses
Table 2 shows the effect of different moderators on the prevalence estimate. As would be expected, the estimate varied by the diagnostic criteria used. The highest prevalence of GDM was observed when the Carpenter-Coustan criteria were used, and the lowest with the NDDG criteria. There were no statistically significant differences in mean GDM between studies carried out in the US and Canada. There was a trend of increasing prevalence estimates the later the data collection period started but the trend according to when the data collection period ended was not significant. Only 15 and 11 studies respectively reported on the mean age and proportion of nulliparous women in the sample, and those studies with higher proportions of nulliparous women and a mean age of under 30 had lower GDM prevalence estimates, but these differences were not statistically significant. The ethnic composition of 19 of the samples was mixed or unknown. However, GDM prevalence estimates were slightly higher for five samples comprising over 70% First Nations women, and three comprising over 70% Hispanic or Latino women although these differences were not statistically significant.
The 15 studies using routinely collected data yielded prevalence estimates that were approximately 2% lower than those from the other studies. The GDM prevalence estimate in studies where the denominator did not include women with pre-existing diabetes was 1.6% higher than studies that included these women but the difference was not statistically significant. The estimate in the 12 studies when the sample was defined as pregnancies or deliveries, and pregnant women could be included more than once was similar to those where it was stated or implied that women could only be included in the study for one pregnancy or delivery ( n = 13).
Multivariate analysis
On the basis of the moderator analysis, a weighted multiple regression was performed in order to explore which important moderator variables made the greatest contribution to the variability in prevalence of GDM (Table 3 ). Correlations between the different variables were explored to inform variable selection for the multivariate analysis but no statistically significant correlations were found. Diagnostic criteria, start of data collection period, whether routinely-collected data were used, and how the sample was defined were statistically significant in moderator analyses and included in the final model of the multiple regression.
The results of the meta-regression indicated that overall, the covariates were able to explain 57% of the total observed variability (R 2 analog = 0.57 (QR [ 8 ] = 71.97, p < 0.001). However, the residual model was statistically significant (QE [ 14 ] = 1163.83, p < 0.001, I2 = 98.8%) confirming that there was variability in the data that was not explained by the moderator variables. Of the variables that were significant in the univariate analysis (diagnostic criteria, start period of data collection, routine dataset, how sample was defined) only diagnostic criteria and period of data collection remained significant when the other variables were held constant.
This meta-analysis of 32 samples of pregnant women in the US and Canada yielded prevalence estimates for GDM of 11.8% using a one-step screening strategy and 5.0% using a two-step screening strategy; with an overall estimate of 5.9%. The overall estimate was higher than estimates from meta-analyses in Europe (5.4%) [ 9 ] and globally (4.4%) [ 11 ], but lower than that for Eastern and South Eastern Asia (10.1%) [ 10 ]. A higher estimate associated with a one-step screening strategy was also observed within the European and Asian studies, with US one-step and two-step estimates again higher than respective estimates in Europe but lower than those from Asia [ 9 , 11 ]. The methods of this systematic review were robust and followed a pre-determined protocol. Independent reviewers screened all results returned by the search and decisions on the inclusion of papers were discussed and made by two authors. Limitations of the review include that only non-English language papers were excluded, experts in the field were not contacted, grey literature was not identified, and data extraction was only carried out by one author. The increased prevalence observed in women in the US and Canada in the present review compared to Europe [ 9 ] may reflect difference in prevalence of obesity in these populations. Women who are obese have significantly increased odds of developing gestational diabetes even after confounders are controlled for [ 74 ]. In 2021 41.8% of women in the USA and 22% of women in Canada were obese. Rates in developed European countries included in the European systematic review discussed [ 9 ] were between 9.7% in Italy and 20.4% in the UK with an average figure of 16.3% [World Obesity 2021]. Differences in prevalence estimates between studies in this review were not only related to the screening approach (one-step or two-step) but can also be attributed to the use of different diagnostic thresholds, with estimates obtained using NDDG thresholds and those from Canadian guidelines significantly lower than those using Carpenter-Coustan thresholds. The IADPSG thresholds yielded very high estimates, as has consistently been reported [ 75 ]. When stratified by diagnostic categories, US and Canadian estimates in our meta-analysis were higher for two out of three categories that could be directly compared with the European study further supporting the suggestions of underlying differences in GDM prevalence between these areas linked to obesity prevalence. The effect of diagnostic category on GDM prevalence is less pronounced in the multivariate meta-regression. This was also the case for later start of data collection which was univariately associated with increased prevalence of GDM, but no independent effect of this variable was evident after adjustment for diagnostic category in the multivariate analysis. But the defined periods of data collection were relatively wide, so a temporal trend of increasing prevalence cannot be ruled out. Samples of women with mean age over 30 years yielded higher estimates of GDM than samples with a lower mean age although the difference was not statistically significant. Fewer than half of the studies reported age, making it difficult to assess the effect of age on our results, or indeed to compare with other studies.
One of the challenges of a meta-analysis is the heterogeneity of methods used in different studies. We attempted to include studies that used similar methods in order to minimise differences in prevalence estimates that could be due to differences in settings, procedures and clinical factors. We defined a general population of pregnant women as one which was not considered to be high risk or defined according to other clinical characteristics. This could mean a geographical (neighbourhood, regional or national) population, or the catchment population of either one, or a group of, medical centres or hospitals, provided that they did not serve a high-risk group. However, there was a difference between studies that used routinely-collected data where the denominator could be very large and included all enrolled women, and those where the data were collected within the context of a specific research study, often when women needed to be recruited and consented. Studies using routinely collected data tended to produce lower estimates. Furthermore, some of these studies used data from large Health Maintenance Organisations, and these populations are not necessarily socio-demographically representative of the overall population, but tend to be relatively affluent.
This review has shown that technical differences in the way that the denominator or the sample is defined can also have substantial effects on prevalence estimates. Most studies in this review used pregnant women as the sample, with some restricting this to primiparous women. Where the number of pregnancies or deliveries was the sampling unit, either the first or a randomly-selected delivery in the study period might be selected, while other studies could include the same woman twice. Furthermore, not all studies excluded stillbirths, or explicitly indicated that analyses were restricted to singleton pregnancies. It was not possible to perform moderator analyses on all these differences, given that the requisite information was not always available, but we did show that studies using pregnancies or deliveries as the sampling unit yielded lower estimates overall, and that excluding women with pre-existing diabetes from the denominator substantially increased the prevalence estimate. Given the increasing prevalence of prediabetes and diabetes in reproductive age women, the effects of this particular methodological detail could become increasingly important. The complexities of defining and diagnosing GDM that are highlighted in this review are likely to continue and as technology in this area develops. Continuous glucose monitoring has recently been shown to be able to potentially detect abnormal glucose levels in women who have a negative OGTT result [ 76 ] and previously HbA1c had been considered and used as a diagnostic tool [ 77 ]. These developments further highlight the need for clarity in the conduct and reporting of epidemiological research on GDM to allow new technology to be evaluated and compared to more established diagnostic tools.
This meta-analysis points to a slightly higher prevalence of GDM in the US and Canada, compared to Europe. However, much of the variability observed between estimates in the meta-regression remains unexplained. The combined effects of technical methodological differences and variation in the composition of different samples clearly account for a high proportion of residual variability. This strengthens the case for standardised epidemiological protocols for estimating the prevalence of GDM, so that trends over time can be monitored accurately, and that meaningful local, national and international comparisons can be made.
Data availability
The datasets used for this study are available from the corresponding author on reasonable request.
Abbreviations
United States
Gestational diabetes mellitus
Glucose challenge test
Oral glucose tolerance test
Health maintenance organisation
Buckley BS, Harreiter J, Damm P, Corcoy R, Chico A, Simmons D, et al. Gestational diabetes mellitus in Europe: prevalence, current screening practice and barriers to screening. A review. Diabet Med. 2012;29:844–54.
Article CAS PubMed Google Scholar
Ye W, Luo C, Huhang J, Li C, Liu Z, Liu F. Gestational diabetes mellitus and adverse pregnancy outcomes: systematic review and meta-analysis. BMJ. 2022;377:e06946.
Google Scholar
Wenland E, Torloni MR, Falavigna M, Trujillo J, Dode MA, Campos MA, Duncan BB, Schmidt MI. Gestational diabetes and pregnancy outcomes - a systematic review of the World Health Organization (WHO) and the International Association of Diabetes in pregnancy study groups (IADPSG) diagnostic criteria. BMC Pregnancy Childb. 2012;12:23.
Article Google Scholar
Dennison RA, Chen ES, Green ME, Legard C, Kotecha D, Farmer G, Sharp SJ, Ward RJ, Usher-Smith JA, Griffin SJ. The absolute and relative risk of type 2 diabetes after gestational diabetes; a systematic review and meta-analysis of 129 studies. Diabetes Res Clin Pr. 2021;17:108625.
Ferrara A. Increasing prevalence of gestational diabetes mellitus: a public health perspective. Diabetes Care. 2007;30:141–6.
Kim C, Newton KM, Knopp RH. Gestational diabetes and the incidence of type 2 diabetes. Diabetes Care. 2002;25:1862–8.
Article PubMed Google Scholar
Unwin N, Shaw J, Zimmet P, Alberti KGMM. Impaired glucose tolerance and impaired fasting glycaemia: the current status on definition and intervention. Diabet Med. 2002;19:708–23.
Wang H, Li N, Chivese T, Werfalli M, Sun H, Yuen L, et al. IDF Diabetes Atlas: estimation of Global and Regional Gestational Diabetes Mellitus Prevalence for 2021 by International Association of Diabetes in Pregnancy Study Group’s Criteria. Diabetes Res Clin Pr. 2022;183:109050.
Hadar E, Oats J, Hod M. Towards new diagnostic criteria for diagnosing GDM – the HAPO Study. J Perinat Med. 2009;37:447–9.
Behboudi-Gandevani S, Amiri M, Yarandi RB, Tehrani FR. The impact of diagnostic criteria for gestational diabetes on its prevalence: a systematic review and meta-analysis. Diabetol Metab Synd 2019; 11.
Eades CE, Cameron DM, Evans JMM. Prevalence of gestational diabetes mellitus in Europe: a meta-analysis. Diabetes Res Clin Pract. 2017;129:173–81.
Paulo MS, Abdo NM, Bettencourt-Silva R, Al-Rifai RH. Gestational diabetes Mellitus in Europe: a systematic review and Meta-analysis of Prevalence studies. Front Endocrinol. 2021;12:691033.
Nguyen CL, Pham NM, Binns CW, Duong DV, Lee AH. Prevalence of gestational diabetes mellitus in eastern and southeastern Asia: a systematic review and meta-analysis. J Diabetes Res. 2018;6536974.
Much A, Olayemi O, Gete Y. Prevalence and determinants of gestational diabetes mellitus in Africa based on the updated international diagnostic criteria: a systematic review and meta-analysis. Arch Public Health. 2019;77:36.
Stroup D, Berlin J, Morton S, Olkin I, Williams G, Rennie D, et al. Meta-analysis of observational studies in epidemiology. A proposal for reporting. JAMA. 2000;283(15):2008–12.
Centre for Reviews and Dissemination. Systematic Reviews . CRD’s guidance for undertaking reviews in healthcare. 2009.
Joanna Briggs Institute. The Joanna Briggs Institute reviewer’s manual 2014. The systematic review of prevalence and incidence data. 2014.
Lipsey MW, Wilson DB. Practical meta-analysis. California: Sage; 2000.
Butalia S, Donovan L, Savu A, Johnson J, Edwards A, Kaul P. Postpartum diabetes testing rates after gestational diabetes mellitus in Canadian women: a population-based study. Can J Diabetes. 2017;41:613–20.
Lai FY, Johnson JA, Dover D, Kaul P. Outcomes of singleton and twin pregnancies complicated by pre-existing diabetes and gestational diabetes: a population-based study in Alberta, Canada, 2005–2011. J Diabetes. 2006;8:45–55.
Lo JC, Feigenbaum SL, Escobar GJ, Yang J, Crites YM, Ferrara A. Increased prevalence of gestational diabetes mellitus among women with diagnosed polycystic ovary syndrome. Diabetes Care. 2006;29:1915–7.
Rodrigues S, Robinson EJ, Gray-Donald K. Prevalence of gestational diabetes mellitus among James Bay Cree women in northern Quebec. CMAJ. 1999;160:1293–7.
CAS PubMed PubMed Central Google Scholar
Sacks DA, Black MH, Li X, Montor MN, Lawrence JM. Adverse pregnancy outcomes using the International Association of the diabetes and pregnancy study groups criteria. Obstet Gynecol. 2015;126:67–70.
Mackenzie ML, Yuan Y, Shen Y, Toth EL, Bell RC, Oster RT. Pregnancy and development of diabetes in First Nations and non-first nations women in Alberta, Canada. Diabet Med. 2021;38:e14372.
Xiang AH, Wang X, Martinez MP, Page K, Buchanan TA, Feldman RK. Maternal type 1 diabetes and risk of autism in offspring. JAMA. 2018;320:89–91.
Article PubMed PubMed Central Google Scholar
Xiang BH, Li BH, Black MH, Sacks DA, Buchanan TA, Jacobsen SJ, Lawrence JM. Racial and ethnic disparities in diabetes risk after gestational diabetes. Diabetologia. 2011;54:3016–21.
Anand SS, Gupta M, Teo KK, Schulze KM, Desai D, Abdalla N, et al. For the south Asian birth cohort (START). Causes and consequences of gestational diabetes in South asians living in Canada: results from a prospective cohort study. CMAJ Open. 2017;5:E604–11.
Anderson V, Sermer M, Connelly PW, Hanley AJG, Zinman B, Retnakaran R. Fasting capillary glucose as a screening test for ruling out gestational diabetes. J Obstet Gynecol Can. 2013;35:515–22.
MacGregor C, Freedman A, Keenan-Devlin L, Grobman W, Wadwha P, Simhan H, et al. Maternal perceived discrimination and association with gestational diabetes. Am J Obstet Gynaecol MFM. 2020;2:100222.
Kew S, Ye C, Mehmood S, Hanley AJ, Sermer M, Zinman B, et al. Neighorhood walkability and risk of gestational diabetes. BMJ Open Diab Res Care. 2020;8:e000938.
Shaffer RM, Ferguson KK, Sheppard L, James-Todd T, Butts S, Chandrasekaran S, et al. Maternal urinary phthalate metabolites in relation to gestational diabetes and glucose intolerance during pregnancy. Environ Int. 2019;123:588–96.
Article CAS PubMed PubMed Central Google Scholar
Dietrich ML, Dolnicek CF, Rayburn WF. Gestational diabetes screening in a private, midwestern American population. Am J Obstet Gynaecol. 1987;156:1403–8.
Article CAS Google Scholar
Wang Y, Chen L, Horswell R, Xiao K, Besse J, Johnson J, Ryan DH, Hu G. Racial differences in the association between gestational diabetes mellitus and risk of type 2 diabetes. J Women’s Health. 2012;21:628–33.
Rey E, Monier D, Lemonnier M-C. Carbohydrate intolerance during pregnancy: incidence and neonatal outcomes. Clin Invest Med. 1996;19:6.
Shah L, Kwapong Y, Boakye E, Ogunwole M, Bennett W, Blumenthal R, et al. Maternal nativity and length of US residence in an urban low income population in the United States. CJC Open. 2022;4:540–50.
North S, Zinn C, Crofts C. Hyperinsulinemia during pregnancy across varying degrees of glucose tolerance: an examination of the Kraft database. J Obst Gyn Res. 2021;47(5):1719–26.
Kieffer EC, Nolan GH, Carman WJ, Sanborn CZ, Guzman R, Ventura A. Glucose tolerance during pregnancy and birth weight in a hispanic population. Obstet Gynecol. 1999;94:741–6.
CAS PubMed Google Scholar
Yoo T, Chao J. Screening for gestational diabetes. J Fam Pract. 1989;29:41–4.
Hiersch L, Shah B, Berger H, Geary M, McDonald S, Murray-Davis B. Oral glucose tolerance test results in pregnancy can be used to individualize the risk of future maternal type 2 diabetes mellitus in women with gestational diabetes mellitus. Diabetes Care. 2021;44:1860–7.
Retnakaran R, Shah B. Mediating effect of vascular risk factors underlying the link between gestational diabetes and cardiovascular disease. BMC Med. 2022;20:389.
Ogunleye OK, Davidson KD, Gregg AR, Egerman RS. Perinatal outcomes after adopting 1- versus 2-step approach to diagnosing gestational diabetes. J Matern Fetal Neonatal Med. 2017;30:186–90.
Rudra CB, Sorensen TK, Leisenring WM, Dashow E, Williams MA. Weight characteristics and height in relation to risk of gestational diabetes. Am J Epidemiol. 2006;165:302–8.
Acosta I, Aponte Z, De-Jesus Z, De-Leon A, Gonzalez M, Hernandez J, et al. Prevalence of diabetes mellitus among pregnant women receiving health services at the Puerto Rico University hospital, Puerto Rico 1997–1998. PRHSJ. 2001;20:165–70.
Aljohani N, Rempel BM, Ludwig S, Morris M, McQuillen K, Cheang M, et al. Gestational diabetes in Manitoba during a twenty-year period. Clin Invest Med. 2008;31:E131–7.
Alunni ML, Roeder HA, Moore TR, Ramos GA. First trimester gestational diabetes screening – change in incidence and pharmacotherapy need. Diab Res Clin Pract. 2015;109:135–40.
Berkowitz GS, Lapinski RH, Wein R, Lee D. Race/ethnicity and other risk factors for gestational diabetes. Am J Epidemiol. 1992;135:965–73.
Black MH, Sacks DA, Xiang AH, Lawrence JM. The relative contribution of prepregnancy overweight and obesity, gestational weight gain, and IADPSG-defined gestational diabetes mellitus to fetal overgrowth. Diabetes Care. 2013;36:56–62.
Bodmer-Roy S, Morin L, Cousineau J, Rey E. Pregnancy outcomes in women with and without gestational diabetes mellitus according to the International Association of the Diabetes and pregnancy study groups criteria. Obstet Gynecol. 2012;120:746–52.
Dabelea D, Snell-Bergeon JK, Hartsfield CL, Bischoff K, Hamman RF, McDuffie RS. Increasing prevalence of gestational diabetes mellitus (GDM) over time and by birth cohort. Diabetes Care. 2005;28:579–84.
Donovan LE, Savu A, Edwards AL, Johnson JA, Kaul P. Prevalence and timing of screening and diagnostic testing for gestational diabetes mellitus: a population-based study in Alberta, Canada. Diabetes Care. 2016;39:55–60.
Dooley SL, Metzger BE, Cho NH. Gestational diabetes mellitus. Influence of race on disease prevalence and perinatal outcome in a U.S. population. Diabetes. 1991;40(Suppl 2):25–9.
Feldman KR, Tieu RS, Yasumura L. Gestational diabetes screening. The International Association of the Diabetes and Pregnancy Study Groups compared with Carpenter-Coustan screening. Obstet Gynecol. 2016;127:10–7.
Ghaffari N, Gonzalez J, Rosenstein M. Does the 1-step method of gestational diabetes mellitus screening improve pregnancy outcomes? Am J Obstet Gynecol MFM. 2020;2:100199.
Harris SB, Caulfield LE, Sugamori ME, Whalen EA, Henning B. The epidemiology of diabetes in pregnant native canadians. Diabetes Care. 1997;20:1422–5.
Hedderson MM, Darbinian JE, Ferrara A. Disparities in the risk of gestational diabetes by race-ethnicity and country of birth. Paediatr Perinat Epidemiol. 2010;24:441–8.
Hillier T, Pedula K, Ogasawara K, Vesco K, Oshiro C, Lubarsky S, et al. A pragmatic, randomized clinical trial of gestational diabetes screening. NEJM. 2021;2021(384 10):895–904.
Hsu S, Selen D, James K, Li S, Camargo C Jr, Kaimal A, et al. Assessment of the validity of administrative data for gestational diabetes ascertainment. Am J Obstet Gynecol MFM. 2023;5:100814.
Kalamegham R, Nuwayhid BS, Mulla ZD. Prevalence of gestational fasting and postload single dysglycemia in Mexican-American women and their relative significance in identifying carbohydrate intolerance. Am J Perinat. 2010;27:697–704.
Kieffer EC, Tabaei B, Carman WJ, Nolan GH, Guzman JR, Herman WH. The influence of maternal weight and glucose intolerance on infant birthweight in latino mother-infant pairs. Am J Public Health. 2006;96:2201–7.
Murphy NJ, Bulkow LR, Schraer CD, Lanier AP. Prevalence of diabetes mellitus in pregnancy among yup’Ik eskimos, 1987–1988. Diabetes Care. 1993;16:315–7.
Oster RT, King M, Morrish DW, Mayan MJ, Toth EL. Diabetes in pregnancy among First Nations women in Alberta, Canada: a retrospective analysis. BMC Pregnancy Childbirth. 2014;14:136.
Palatnik A, Swanson K, Churchill T, Bilski A, Grobman WA, Miller ES. Association between types of screening for gestational diabetes mellitus and cesarean delivery. Obstet Gynecol. 2017;130:539–44.
Pedula KL, Hillier TA, Schmidt MM, Mullen JA, Charles M-A, Pettitt DJ. Ethnic differences in gestational oral glucose screening in a large US population. Ethnic Dis. 2009;19:414–9.
Pocobelli G, Yu O, Fuller S, Fraser JR, Wartko PD, Chen L, Newton KM, et al. One-step approach to identifying gestational diabetes mellitus. Obstet Gynecol. 2018;132:859–67.
Pouliot A, Elmahboubi R, Adam C. Incidence and outcomes of gestational diabetes mellitus using the new international association of diabetes in pregnancy study group criteria in Hôpital Maisonneuve-Rosemont. Can J Diabet. 2019;43:594–9.
Schwartz ML, Ray WN, Lubarsky SL. The diagnosis and classification of gestational diabetes mellitus: is it time to change our tune? Am J Obstet Gynecol. 1999;180:1560–9.
Schwartz DB, Daoud Y, Zazula P, Goyert G, Bronsteen R, Wright D, Copes J. Gestational diabetes mellitus: metabolic and blood glucose parameters in singleton versus twin pregnancies. Am J Obstet Gynecol. 1991;181:912–4.
Tuleda CM, Casey BM, McIntire DD, Cunningham FG. Relationship of subclinical thyroid disease to the incidence of gestational diabetes. Obstet Gynecol. 2012;119:983–8.
Willows ND, Sanou D, Bell RC. Assessment of Canadian Cree infants’ birth size using the WHO child growth standards. Am J Hum Biology. 2011;23:126–31.
Yeung RO, Savu A, Kinniburgh B, Lee L, Dzakpasu S, Nelson C, et al. Prevalence of gestational diabetes among Chinese and south asians: a Canadian population-based analysis. J Diabetes its Complications. 2017;31:529–36.
Xiong X, Saunders LD, Wang FL, Demianczuk NN. Gestational diabetes mellitus: prevalence, risk factors, maternal and infant outcomes. Int J Gynaecol Obstet. 2001;75:221–8.
Houshmand A, Jensen DM, Mathiesen ER, Damm P. Evolution of diagnostic criteria for gestational diabetes mellitus. AOGS. 2013;92:739–45.
Meltzer S, Leiter L, Daneman D, Gerstein HC, Lau D, Ludwig S, Yale J-F, Zinman B, Lillie D. 1998 clinical practice guidelines for the management of diabetes in Canada. CMAJ 1998;159:(8 Suppl).
Yao D, Chang Q, Wu Q, Gao S, Zhao H, Liu Y et al. Relationship between Maternal Central Obesity and the Risk of Gestational Diabetes Mellitus: A Systematic Review and Meta-Analysis of Cohort Studies. J Diab Res. 2020; 2020; 6303820.
O’Sullivan EP, Avalos G, O’Reilly M, Dennedy MC, Gaffney G, Dunne F. Atlantic diabetes in pregnancy (DIP): the prevalence and outcomes of gestational diabetes mellitus using new diagnostic criteria. Diabetologia. 2011;54:1670–5.
Tartaglione L, di Stasio E, Sirico A, Di Leo M, Caputo S, Rizzi A et al. Continuous Glucose Monitoring in Women with Normal OGTT in Pregnancy. J Diab Res. 2021; 2021: 9987646.
Lai Y, Chen H, Du Z, Zhou S, Xu W, Li T. The diagnostic accuracy of HbA1c in detecting gestational diabetes mellitus among Chinese pregnant individuals. Ann Transl Med. 2020;8(16):1014.
Download references
Acknowledgements
Not applicable.
This research did not receive any specific grant from funding agencies in the public, commercial, or not-for-profit sectors.
Author information
Authors and affiliations.
Faculty of Health Sciences and Sport, University of Stirling, Stirling, FK9 4LA, Scotland, UK
Claire E. Eades, Katherine A. Burrows, Roza Andreeva & Josie MM. Evans
University of Edinburgh Medical School, Edinburgh, Scotland, UK
Daniel R. Stansfield
Public Health Scotland, Edinburgh, Scotland
Josie MM. Evans
You can also search for this author in PubMed Google Scholar
Contributions
CE: Conceptualization, Methodology, Investigation, Supervision, Writing - review and editing, Project administration, Supervision. KB: Validation RA: Validation DS: Investigation, Validation JE: Conceptualisation, Methodology, Investigation, Formal analysis, Writing - original draft of paper.
Corresponding author
Correspondence to Claire E. Eades .
Ethics declarations
Ethics approval and consent to participate.
No ethical approval required.
Consent for publication
Not required.
Competing interests
The authors declare no competing interests.
Additional information
Publisher’s note.
Springer Nature remains neutral with regard to jurisdictional claims in published maps and institutional affiliations.
Rights and permissions
Open Access This article is licensed under a Creative Commons Attribution 4.0 International License, which permits use, sharing, adaptation, distribution and reproduction in any medium or format, as long as you give appropriate credit to the original author(s) and the source, provide a link to the Creative Commons licence, and indicate if changes were made. The images or other third party material in this article are included in the article’s Creative Commons licence, unless indicated otherwise in a credit line to the material. If material is not included in the article’s Creative Commons licence and your intended use is not permitted by statutory regulation or exceeds the permitted use, you will need to obtain permission directly from the copyright holder. To view a copy of this licence, visit http://creativecommons.org/licenses/by/4.0/ . The Creative Commons Public Domain Dedication waiver ( http://creativecommons.org/publicdomain/zero/1.0/ ) applies to the data made available in this article, unless otherwise stated in a credit line to the data.
Reprints and permissions
About this article
Cite this article.
Eades, C.E., Burrows, K.A., Andreeva, R. et al. Prevalence of gestational diabetes in the United States and Canada: a systematic review and meta-analysis. BMC Pregnancy Childbirth 24 , 204 (2024). https://doi.org/10.1186/s12884-024-06378-2
Download citation
Received : 16 December 2022
Accepted : 28 February 2024
Published : 15 March 2024
DOI : https://doi.org/10.1186/s12884-024-06378-2
Share this article
Anyone you share the following link with will be able to read this content:
Sorry, a shareable link is not currently available for this article.
Provided by the Springer Nature SharedIt content-sharing initiative
- Epidemiology
- Gestational diabetes
BMC Pregnancy and Childbirth
ISSN: 1471-2393
- Submission enquiries: [email protected]
- General enquiries: [email protected]
REVIEW article
Gestational diabetes mellitus—innovative approach to prediction, diagnosis, management, and prevention of future ncd—mother and offspring.
- 1 Mater Research, The University of Queensland, South Brisbane, QLD, Australia
- 2 World Diabetes Foundation, Bagsvaerd, Denmark
- 3 Divakars Specialty Hospital, Bengaluru, India
- 4 Mor Women’s Health Care Center, Tel Aviv, Israel
Gestational diabetes mellitus (GDM) is the commonest medical complication of pregnancy. The association of GDM with immediate pregnancy complications including excess fetal growth and adiposity with subsequent risk of birth trauma and with hypertensive disorders of pregnancy is well recognized. However, the associations with wide ranges of longer-term health outcomes for mother and baby, including the lifetime risks of obesity, pre-diabetes, and diabetes and cardiovascular disease have received less attention and few health systems address these important issues in a systematic way. This article reviews historical and recent data regarding prediction of GDM using demographic, clinical, and biochemical parameters. We evaluate current and potential future diagnostic approaches designed to most effectively identify GDM and extend this analysis into a critical evaluation of lifestyle and nutritional/pharmacologic interventions designed to prevent the development of GDM. The general approach to management of GDM during pregnancy is then discussed and the major final focus of the article revolves around the importance of a GDM diagnosis as a future marker of the risk of non-communicable disease (NCD), in particular pre-diabetes, diabetes, and cardiovascular disease, both in mother and offspring.
On a worldwide basis, both Hyperglycemia in Pregnancy (HIP) and obesity among women of childbearing age are increasing to epidemic proportions ( 1 , 2 ). For our current report, we are following the diagnostic framework for HIP as recommended by the International Federation of Gynecology and Obstetrics (FIGO) ( 3 ) which considers any degree of glucose elevation in pregnancy as part of the umbrella definition of HIP. This broad group is then further divided into those women with either known pre-pregnancy diabetes or markedly elevated glucose levels which would qualify for a diagnosis of diabetes outside pregnancy. This condition is termed “Diabetes in Pregnancy” (DIP). The far larger group of women with elevated glucose levels below these thresholds is classified as “Gestational Diabetes Mellitus” (GDM). Many reports have attempted to dissect the relative importance of HIP and overweight/obesity relating both to pregnancy complications and longer term health of both GDM mothers and their children. Clear determination of causality is challenging, GDM and overweight/obesity frequently affect the same people, and causality is bidirectional—obesity lies on the causal pathway toward hyperglycemia and HIP is causally related to obesity in the offspring. Also, hyperglycemia (pre-diabetes or diabetes) may well exist before pregnancy as demonstrated by large surveys such as NHANES ( 4 , 5 ). However, it is generally asymptomatic at this point and may only be detected when (and if) systematic testing occurs in the pregnancy context.
The Hyperglycemia and Adverse Pregnancy Outcome (HAPO) study ( 6 , 7 ), demonstrated that both maternal BMI and hyperglycemia share similar associations with complications of pregnancy. Both were associated with increased rates of excess fetal growth, primary cesarean birth, clinical neonatal hypoglycemia and fetal adiposity, neonatal hyperinsulinemia, and hypertensive disorder of pregnancy. The association of hyperglycemia with adverse outcomes is generally linear, while that of BMI follows a quadratic pattern with decreasing increments in the highest BMI categories ( 8 ).
HAPO also reported BMI and GDM considered together with pregnancy complications ( 6 ). In HAPO, obesity was present in 13.7% and GDM by IADPSG criteria ( 9 ) in 16.1% of those who remained blinded. Obesity was present in 25% of GDM women but prevalence varied greatly between centers. Compared to women with neither factor, adverse outcomes were increased on both groups. Pre-eclampsia was more frequent in the “obesity/non GDM group”, while excess fetal growth and fetal hyperinsulinemia were slightly more common in the “GDM/no obesity group” than in the “obesity/non GDM group”. The combination of both factors was accompanied by an additive increase in pregnancy complications.
Thus, both maternal BMI and glycemia contribute independently and additively to suboptimal pregnancy outcomes. While not ignoring obesity as a major health problem, our current review will focus primarily on the prediction and diagnosis of GDM and outline optimal management both during and following pregnancy.
Prediction of GDM
As noted above, many women currently considered as “GDM” may actually have undiagnosed hyperglycemia before pregnancy. In high prevalence countries, ideally screening for hyperglycemia would happen as part of well-organized and well- resourced preconception care. However, this approach has limitations as only around 40% of pregnancies worldwide are “intended” ( 10 ). Lack of preconception testing means that we cannot strictly assert that testing in early pregnancy is “predicting” GDM. However, early testing does represent an opportunity to recognize some women as having likely pre-existing abnormalities of glucose metabolism. In India, for example, it has been reported that over 70% of GDM women can be identified at their first antenatal visit ( 11 ).
Further, it may be possible, by using clinical characteristics and biochemical tests, to identify a separate group of pregnant women whose glucose levels are in the normal range in early gestation, but who carry a high risk of progression to “standard GDM”, which is generally diagnosed at around 24 to 28 weeks’ gestation. In pragmatic terms, it appears reasonable to consider women with pre-pregnancy hyperglycemia, those with early GDM and those at high risk of GDM as target groups for early intervention.
GDM is frequently an antecedent of later Type 2 diabetes and a marker for (premature) cardiovascular (CV) disease in women. In addition to their sine qua non of hyperglycemia, GDM and Type 2 diabetes share a range of underlying processes including insulin resistance, chronic metabolic inflammation, changes in adipocytokines, and alterations in many areas of metabolism ( 12 , 13 ).
The simplest models for prediction of GDM involve the use of single or multiple clinical characteristics to stratify GDM risk. The performance of these models has recently been evaluated by van Hoorn et al. ( 14 ), who concluded that models which included both multiple clinical characteristics and early pregnancy glucose measurements performed best in prediction. Recently, machine learning or artificial intelligence methods using demographic variables and previous laboratory results have been applied to improve predictive power ( 15 ).
GDM is also associated with abnormalities of placentation and early pregnancy markers commonly used in aneuploidy prediction such as pregnancy associated plasma protein A (PAPP-A) and free β HCG have also been incorporated into predictive models ( 16 ). Sweeting et al., using stored serum samples from a first trimester screening program in Sydney, have reported that use of multiple biochemical markers in combination with clinical features, is able to predict GDM with high accuracy [area under receiver operator curve (AUROC) 0.91–0.93]. However, these findings have yet to be validated in independent cohorts.
Proteomic screening in early pregnancy has revealed multiple potential protein markers, including a cluster associated with insulin secretion, binding, resistance, and signaling for later GDM ( 17 , 18 ). Ravnsborg et al. have reported that vitronectin, which is also associated with metabolic syndrome outside pregnancy, significantly augments the predictive power of maternal risk factors ( 17 ) and may prove to be a valuable predictor in clinical use. However, proteomic methods are too complex and expensive for routine use and must progress to automated, low cost laboratory tests before they are widely applicable.
Recently, the role of extracellular vesicles (ECVs) as GDM markers has been explored ( 19 , 20 ). These circulating particles, derived in pregnancy primarily from placenta and adipose tissue, “package” multiple potential protein and RNA molecules and transport them to specific sites. James-Allan et al. ( 21 ) have demonstrated that specific small ECVs are associated with GDM and that infusion of human ECVs from GDM women produces both insulin resistance and reduced insulin secretion in rodents, reminiscent of the pathophysiology of GDM.
Micro RNAs are a major component in ECVs and are associated with glucose metabolism. An exploratory case-control study by Yoffe et al. suggested that micro RNA-223 and micro RNA 23a in first trimester blood samples were strongly predictive of later GDM (AUROC 0.91) ( 22 ). Another recent cohort study has confirmed this finding for micro RNA-233 ( 23 ). These results are promising and the overall associations between non coding RNAs and GDM have recently been reviewed in detail ( 24 ). However, as is the case for other biomarkers, these positive findings from small studies need to be validated in independent cohorts. The required assays will also need to be modified to allow low cost, high throughput use in routine diagnostic laboratories.
In summary, cohort studies have revealed multiple potential early pregnancy predictors of later GDM ( 13 ). These range from single or multiple clinical or demographic measures to including early pregnancy glycemic measurement and extending to measurement of complex network of molecular biomarkers. To be valuable for routine clinical practice, molecular biomarkers need both to perform better than clinical risk factors and simple glucose measurements in predicting GDM and pregnancy outcomes and to demonstrate cost-effectiveness. In practical terms, they should also be suited to non-fasting testing at the same time as other routine early pregnancy health screening tests. While many biomarkers have a strong association with later GDM, none have yet been sufficiently developed as automated and low-cost assays to allow their routine clinical use. However, they offer valuable insights into the pathophysiology of GDM and may, in time, be ready for the clinic.
Diagnosis of GDM
GDM is generally diagnosed with an oral glucose tolerance test (OGTT) administered at 24–28 weeks’ gestation. This timing has generally been preferred for routine GDM diagnosis as most of the physiologic insulin resistance of pregnancy will be well established. However, with globally increasing levels of obesity, rising maternal age and other environmental risk factors this assumption may no longer be valid as evidenced by high GDM detection rates in early pregnancy witnessed in recent studies from different parts of the world. The rising prevalence of undiagnosed dysglycaemia (diabetes and pre-diabetes) in reproductive age women enhances the need to rule out pre-existing undiagnosed diabetes at the earliest possible moment, thus bringing into question the old norm of testing between 24 and 28 weeks. The exact process and criteria for OGTT diagnosis of GDM vary widely across the world. The International Association of Diabetes in Pregnancy Study Groups (IADPSG) ( 9 ), World Health Organization (WHO) ( 2 , 25 ) and FIGO ( 3 ) have all endorsed “one step” OGTT testing, using thresholds ≥ 5.1 mmol/L fasting; 10.0 mmol/L at 1 h and 8.5 mmol/L at 2 h following a 75 gram glucose load for diagnosis of GDM. However, the FIGO pragmatic recommendations, recognizing differing health care contexts, allow for alternative diagnostic approaches for China, India, South America, and the United Kingdom ( 3 ). There is a major variance in diagnostic approach in the USA ( 26 ) and Canada ( 27 ) which generally prefer two step testing using a non-fasting, 1 h “glucose challenge” test (GCT), followed by OGTT (100 gram or 75 gram) if the GCT result falls above predefined thresholds. IADPSG, WHO, and FIGO have all also endorsed the need for early testing as well as testing in the traditional 24 to 28 week window.
A further major variation in worldwide testing protocols for GDM is the ongoing debate regarding whether testing should be universal (for all pregnant women) or targeted only toward women with identified risk factors which are associated with a higher risk of a positive test. FIGO ( 3 ), the IADPSG ( 9 ) and the American College of Obstetricians and Gynecologists (ACOG) ( 26 ) all recommend universal testing. The HAPO study ( 7 ) clearly demonstrated that OGTT glucose values are independently associated with adverse pregnancy outcomes, even after correction for multiple additional maternal characteristics including BMI. GDM is almost uniformly asymptomatic, so a testing strategy based on symptoms is clearly untenable. A cost utility analysis for the United Kingdom (UK) concluded that if the population frequency of GDM is >4.2%, then universal OGTT is the most cost effective strategy ( 28 ). No current credible estimates of GDM frequency fall below this threshold. Nonetheless, some authorities, notably the National Institute for Clinical Excellence (NICE) in the UK, still promote risk factor based screening ( 29 ). The most recent Cochrane review ( 30 ) is inconclusive, but a recent systematic review of economic evaluations of GDM screening again concluded that universal screening was the most effective approach ( 31 ). Compliance with officially endorsed risk factor based screening protocols appears to be poor in countries as diverse as Sweden ( 32 ), where only 31% of women received the screening test deemed appropriate for their documented risk factor profile, the UK (61% appropriate screening according to risk factors) ( 33 ) and South Africa ( 34 ), where Adam et al. reported that, although use of risk factors would reduce OGTTs by 46%, this protocol would miss 41% of GDM diagnoses ( 34 ). A further study from Italy reported that 23% of GDM cases would be missed by risk factor based screening ( 34 ). By contrast, a report from Sri Lanka, a country with a high background frequency of diabetes, noted that 80% of women would still require screening if risk factors were used to determine the need for OGTT, but only 13% of GDM cases would be missed ( 35 ). Thus, the effects of implementing risk factor–based screening differ between various populations. However, given that most countries have an increasing prevalence of both GDM and associated risk factors and that correct implementation of risk factor based screening is poor, we favor uniform biochemical testing.
Clearly, the glucose tolerance test is inconvenient, resource intensive and also quite poorly reproducible ( 36 ) and an alternative cheap, reproducible, non-fasting test would be preferable. Self-administered home OGTTs offer increased convenience and appear to perform as well as laboratory testing ( 37 ). For fasting glucose testing, specific meters with tight laboratory based quality control may also provide acceptable accuracy ( 38 ) and this approach has been endorsed by FIGO for use in low resource settings ( 3 ).
Glycosylated haemoglobin (HbA1c) is an obvious alternative and is widely used for diagnosis of diabetes outside pregnancy. However, it performs poorly both in prediction of OGTT diagnosed GDM and in prediction of pregnancy outcomes ( 39 ) and appears to be of limited value except in early pregnancy detection of undiagnosed hyperglycemia ( 40 ).
A variety of other markers of overall glycemia with shorter half-lives (thus more reflective of short term glycemia changes) including fructosamine (FA), glycated albumin (GA) and 1.5 anhydroglucitrol (1.5 AHG) have been evaluated ( 41 ). None of these markers are optimally suited to use in pregnancy. FA is easily measured but is affected by the dilutional anaemia of pregnancy. FA and GA vary in the presence of albuminuria (as in pre-eclampsia) and 1.5 AHG is inaccurate in pregnancy due to reduced renal threshold for glycosuria ( 41 ). More recently, the glycated complement fraction GCD59 ( 42 ) has been shown in retrospective studies to be associated with early GDM and with large for gestational age (LGA) infants ( 43 ) in an obese pregnant population. Larger prospective evaluations are now underway ( 44 ). GCD59 is currently the most promising, non-fasting marker of overall glycemia in the context of pregnancy.
Prevention of GDM—Before and During Pregnancy
Ideally, GDM rates in the population could be reduced by both individual and societal measures designed to promote healthy lifestyle changes including optimal dietary intake and increased physical activity in the general population with a focus on health and fitness of women of reproductive age. Maternal age at conception is an important marker of pregnancy complications including GDM ( 45 ), but is strongly influenced both by individual choices and societal factors and unlikely to be a useful target for preventative measures. Maternal overweight and obesity are also very important risks that need to be addressed pre-pregnancy through lifestyle measures ( 45 ). For marked obesity, bariatric surgery is the best proven means of reducing body weight. Although a full consideration of its effects on pregnancy are beyond the scope of this article, a recent review ( 46 ) showed benefits reduced GDM prevalence, lower rates of LGA infants and reduced prevalence of pregnancy hypertension. However, there was an increase in impaired fetal growth reflected by higher rates of SGA infants. Preterm deliveries were also more frequent. Surgical treatments which cause malabsorption are followed by greater weight reduction and demonstrate lower rates of LGA, but conversely also more SGA, suggesting that clinical decisions must balance potential risks and benefits.
The most recent overview of multiple GDM prevention strategies from the Cochrane group ( 47 ) reports that no single lifestyle or medication-based intervention is of proven benefit and that the overall evidence base is weak, but we shall consider some widely discussed potential options in more detail.
Lifestyle Interventions
Despite the negative Cochrane findings outlined above, which specifically concluded that diet alone or exercise alone had no effect in preventing GDM and that combined diet and exercise had only a “possible” (non-significant) effect in preventing GDM, one systematic review by Song et al. ( 48 ) has suggested that lifestyle intervention before the 15 th gestational week may reduce GDM by 20% [RR 0.80 (95% 9I 0.66–0.97)]. This provides glimmer of hope for lifestyle based interventions, but the weight of evidence is negative for any lifestyle intervention after the first trimester.
Metformin is commonly used in early pregnancy for patients with polycystic ovarian syndrome. It is also used in the second and third trimester as drug treatment for GDM if lifestyle modifications fail to achieve glycemic goals. A recent review has summarized many aspects of metformin use in pregnancy ( 49 ). However, current evidence does not support metformin as a preventative option for GDM. The (EMPOWaR) study randomised 449 obese women with normal baseline glucose tolerance to the addition of metformin of up to 2,500 g per day vs. placebo between 12- and 16-week gestation and continued until delivery of the infant ( 50 ). EMPOWaR noted no difference GDM prevalence, maternal weight gain or maternal lipid metabolism. Birth weight and birthweight SD (Z) score were comparable between the groups. Metformin was associated with more reported diarrhoea (42% vs 19%, P < 0.0001).
Syngelaki et al. randomized women with BMI > 35 kg/m 2 to metformin 3 g per day or placebo from 12- to 18-week gestation until delivery ( 51 ). A total of 202 women on metformin and 198 on placebo completed the study. There was no difference in fetal growth. Maternal GWG was reduced by 1.7 kg (P < 0.001) with metformin. GDM rates were similar between groups and other pregnancy outcomes were also similar.
The GRoW RCT of metformin for overweight and obese women from early pregnancy, conducted by Dodds et al., has also reported negative results, with no reduction in GDM or other pregnancy complications ( 52 ).
Myoinositol
Myoinositol enhances insulin sensitivity outside pregnancy. In GDM, a trial of supplementation of 69 women randomised to myoinositol 4 g/day with folic acid 400mcg daily compared to folic acid alone showed reduced insulin resistance in the myoinositol group ( 53 ). Another study compared myoinositol 2 g with 200 mcg folic acid daily vs. 200 mcg folic acid daily alone in 220 obese women starting at 12- to 13-week gestation and continued throughout pregnancy ( 54 ). GDM prevalence was reportedly reduced from 33.6% to 14% (P = 0.001), in the myoinositol group.
However, another RCT which recruited women with family history of diabetes and compared women randomised to 1,100 mg myoinositol, 27.6 mg D-chiro-inositol plus 400 mcg folic acid, or to 400 mcg folic acid only, from early pregnancy until 24- to 28-week gestation demonstrated no reduction in GDM frequency ( 55 ).
Resveratrol was compared to inositol in another RCT involving overweight pregnant women. The three arms of the study included resveratrol plus inositol, inositol alone and placebo ( 56 ). Resveratrol was associated with reduced lipid and glucose measures. The NiPPeR study plans to compare twice-daily intake of a control nutritional drink, enriched with standard micronutrients, to a twice-daily nutritional drink enriched with additional micronutrients, myoinositol, and probiotics. Results are not yet available ( 57 ).
Probiotics were found reduce GDM in normal weight women in a study from Finland ( 58 ), a result which stimulated great interest in their use as a low risk therapeutic agent in GDM prevention. However, results in women at higher risk due to pre-pregnancy obesity have been less promising. The Probiotics in Pregnancy Study randomised 175 obese women to probiotic vs. placebo capsule for a four week period from 24 until 28 weeks gestation, with the primary outcomes being change in fasting glucose. Treatment groups differed in baseline BMI. After adjustment for BMI, there were no discernible clinical benefits in the major outcome of fasting glucose. Infant birthweight and other metabolic parameters did not improve ( 59 ). More recently, the SPRING study ( 60 ), HUMBA study ( 61 ) and studies from Finland ( 62 ) and Denmark ( 63 ) have reported negative results for probiotic supplementation, so the weight of current evidence is certainly negative.
Dietary fatty acid has also been suggested as a therapy which might reduce GDM and also improve the rates of preterm delivery. The DHA to optimize mother infant outcome (DOMInO) RCT included 2399 women who were randomized before 21-week gestation to either (1) DHA enriched fish oil 800 mg/day or (2) vegetable oil capsules without DHA until the time of birth ( 64 ). GDM and preeclampsia were not reduced and there were no differences in neonatal size or adiposity. Subsequent assessment of the offspring at Age 7 years showed no differences in anthropometry ( 65 ).
Low serum 25 hydroxy vitamin D levels are clearly a risk factor for development of GDM ( 66 ), but the results of therapeutic trials have been mixed. The most recent Cochrane review ( 67 ), including primarily studies from the Middle East, reported that supplementation with Vitamin D alone “probably” reduces the population frequency of GDM [RR 0.51 (95% CI 0.27–0.97)] and pre-eclampsia [RR 0.48 (95% CI 0.30–0.79)]. However, no benefit was noted for Vitamin D + calcium or Vitamin D + calcium + other minerals. The overall quality of available evidence has been considered “low” by the Cochrane Group in their overview of GDM prevention studies ( 47 ). Noting the overall divergence in populations studied, baseline Vitamin D levels and therapeutic Vitamin D doses used in the studies included in/excluded from various reviews, we consider the following conclusion from Corcoy et al. ( 68 ) as a balanced summary: “High dose vitamin D supplementation during pregnancy halves the rate of GDM in pregnant women with baseline Vitamin D < 50 nmol/L”. Toxicity from Vitamin D supplementation up to 5,000 IU/day appears low ( 69 , 70 ). Therefore, in practical terms, Vitamin D therefore appears to be a reasonable option in populations with low baseline levels. Hopefully, future studies will clarify its true therapeutic role.
Management of GDM—During Pregnancy and Post-Partum
Treatment of GDM during pregnancy, centers on dietary modulation, promotion of healthy physical activity and pharmacologic management, primarily with insulin as well as oral hypoglycemic agents (OHA), if glycemic control cannot be achieved with lifestyle measures alone. The details of the therapeutic approach, in particular regarding various dietary approaches and the potential use of OHAs such as metformin and glyburide (glibenclamide) differ widely between and within countries and detailed discussion of these ongoing issues of contention is beyond the scope of our current review, but has recently been covered in detail ( 71 ).
Two landmark prospective RCTs have confirmed that detection of and medical therapy for GDM carries benefits for both mother and baby in terms of immediate pregnancy outcomes ( 72 , 73 ). Women in the intervention arm of the Australian (Crowther) study showed lower rates of fetal macrosomia, reduced frequency of LGA and reduced preeclampsia ( 72 ). In the US (Landon) study, women who received treatment for GDM demonstrated lower GWG and lower rates of pre-eclampsia. Reduced frequency of LGA and macrosomia were noted in infants of treated mothers.
Prevention of Future NCD—Mother and Offspring
Apart from its short term immediate associations with adverse perinatal outcomes, antecedent GDM is the strongest available historical marker for future Type 2 diabetes. GDM women carry an almost tenfold increased risk of progressing to later diagnosis of type 2 diabetes [RR 9.51 (95% CI 7.14–12.67)] ( 74 ). Women with GDM are also at heightened risk of CV disease ( 75 ). This increased risk exists even in those women who do not progress to overt Type 2 diabetes themselves. In the overall population of women with Type 2 diabetes, those with antecedent GDM also appear to carry higher risks than those without such a history ( 76 ). Compared with those who did not have GDM, women with GDM had a twofold higher risk of future CV events [RR 1.98 (95% CI 1.57–2.50)].
Meta-regression analysis demonstrates that this relationship is independent of Type 2 diabetes incidence across various studies (P = 0.34). Even when considering only those women who did progress to overt Type 2 diabetes, GDM was associated with an elevated chance of suffering future CV events [RR 1.56 (95% CI 1.04–2.32)]. Much of this risk occurs early in the life course post pregnancy, with a 2.3-fold increased noted for CV events in the first decade post-partum [RR 2.31 (95% CI 1.57–3.39)] ( 77 ).
The therapeutic opportunity for delaying/preventing type 2 diabetes through post-partum lifestyle modifications and possible use of pharmacotherapy in women identified as being “at risk” due to prior GDM ( 78 ) has been conclusively shown. A German study reported that breastfeeding (BF) was associated with a > 40% reduction in diabetes also appeared to delay the onset of T2DM by a further 10 years. These effects were not dependent on known high risk maternal characteristics such as baseline obesity or a need for insulin treatment during the index pregnancy. The greatest reduction in later diabetes risk was seen in women who breast fed for >3 months ( 79 ). A systematic review and meta-analysis of the association of BF with the post-partum risk of progression from GDM to overt type 2 diabetes reported reduced risks for both pre-diabetes and T2DM. Pre-DM was substantially reduced (OR = 0.66; 95% CI, 0.51–0.86 and T2DM was also less frequent (OR = 0.79; 95% CI, 0.68–0.92). Positive effects were noted among women with longer duration of any BF following a GDM pregnancy. These were even more evident with longer follow-up. Compared with women with shorter duration of BF, longer BF duration was associated with improvement in glucometabolic parameters, including lower fasting glucose and enhanced insulin sensitivity, lower BMI at follow up improved lipid metabolism as demonstrated by lower triglyceride levels. Thus, BF is potentially of great value as a low cost preventative measure in preventing both T2DM and related metabolic derangements in women with a history of GDM, in addition to its other well recognized benefits ( 80 ). Evidence for prevention of CV disease in women with history of GDM through post-partum interventions is currently lacking; but logically breast feeding and improved post-partum lifestyle would be predicted to reduce the risk significantly.
Excessive increase in maternal weight from one pregnancy to the next is often associated with a failure to return to pre-pregnancy weight. This in turn is clearly related to a higher frequency of adverse outcomes in subsequent pregnancies. Documented risks include higher rates of stillbirth ( 81 ). Further this ongoing weight gain adds to the “vicious cycle” of weight gain and heightened risks of development of T2DM and CV disease. However, the precise contribution of interpregnancy weight gain to the overall CV risk profile been quantified in clinical studies.
Offspring developing in a hyperglycemic environment in utero , carry higher risks of obesity developing early in the life course, progression to early impaired glucose tolerance, later development of T2DM and long term risks of development of overt CV disease. These risks are independent of maternal obesity and broadly fall into the conceptual framework of “developmental origins of health and disease” (DoHaD) ( 82 – 85 ). German researchers comparing GDM and non GDM offspring described higher risks for development of childhood disorders, even after adjustment for maternal BMI. They reported adjusted OR of 1.81 (95% CI, 1.23–2.65) for childhood overweight and 2.80 (95% CI, 1.58–4.99) for obesity ( 83 ). Maternal GDM also increased the risk of childhood abdominal adiposity (OR, 1.64; 95% CI, 1.16–2.33). Israeli researchers have reported an association between mild GDM (diet treated) and offspring CV morbidity (relative risk, 1.6; 95% CI, 1.2–2.2) ( 86 ).
Other researchers have suggested a link between GDM and neuro psychiatric disorders in offspring ( 87 ).
The efficacy of current standard GDM interventions during pregnancy in attenuating the risks of obesity and impaired glucose metabolism in children of GDM mothers is much less clear. Existing report suffer from methodologic difficulties related to post hoc design and incomplete cohort follow-up. Specifically designed prospective follow up studies would be of great value, but these are clearly difficult to design, difficult to fund and difficult to conduct. Follow up from the landmark GDM RCTs which demonstrated benefits of GDM treatment during pregnancy have been conducted. Gillman et al. ( 88 ) reported follow up from the Crowther study ( 72 ), but this report was comprised of limited follow up through school based databases rather than direct clinical contact. No benefit was shown for the offspring after maternal treatment of GDM. Landon et al. ( 89 ) reported follow up of their North American study ( 73 ) and despite detailed clinic visits, they again descried no clear offspring benefits from maternal GDM therapy. Landon et al. study did describe some minor improvement in glucometabolic status for girls whose mothers were treated for GDM. This contrasted with their previous findings related to immediate pregnancy outcomes, which had suggested that males were more likely to benefit from GDM treatment ( 90 ). The reason for this disparity remains unclear.
Gunderson et al., describing a cohort based in the USA, have recently reported that breast feeding may reduce some of the offspring risks associated with maternal GDM. They note that offspring weight for length Z score was reduced by 0.36–0.45 standard deviation units at 12 months of Age in those babies of GDM mothers who were intensively breast fed ( 91 ). At this stage, data regarding potential longer term effects is not available.
Post-Partum Glucose Testing
All women with HIP (GDM and overt DIP), should have their glycemic status reassessed with a 75 g OGTT at 6–12 weeks after delivery ( 3 ). However, compliance with this recommendation is poor in most health care systems ( 92 ) and alternative strategies based on testing in the early post-partum period are supported to some extent by physiologic ( 93 ) research demonstrating that changes in maternal insulin sensitivity occur rapidly following delivery. Early testing appears adequate to exclude ongoing Type 2 diabetes at this time point ( 94 ), but appears insufficient to exclude impaired fasting glucose (IFG) or impaired glucose tolerance (IGT) ( 94 ). Diagnosis at 6–12 weeks should conform to the local non-pregnant criteria for diabetes, IFG, and IGT. Those women whose test results fall into the normal range at this point should undergo ongoing surveillance for diabetes, while those with abnormal results should receive structured interventions for pre-diabetes or diabetes depending on their findings ( 95 ).
Irrespective early post-partum glucose results, all women with GDM should be considered to carry higher risks of future diabetes and CV disease. They should be advised to breast feed as long as possible, institute healthy eating patterns and habitual physical activity and seek to achieve a normal body weight. Ideally, they should receive support through consultation and ongoing follow-up with health care professionals knowledgeable about diabetes prevention. It must be recognized that this post-partum care is often the best (and only) opportunity to attempt to improve overall maternal health before the next pregnancy and represents an important opportunity to influence maternal and infant health over the life course.
The biggest stumbling block in the care of GDM mother and her offspring lies in post-partum follow up and inability to set up programs that can help intergenerational prevention of NCDs. There are many barriers in achieving these objectives ( 96 ). Following delivery, women with GDM (usually) no longer have glucose levels in the pre-diabetic or diabetic range. Further, they are no longer pregnancy and thus the maternal health care system rarely provides for ongoing care beyond 6- to 12-week post-partum. Thus, they are less likely to attend for regular follow up of their own health issues. Furthermore, the question of responsibility for ongoing care is often left “open”, without a clear plan for ongoing follow-up ( 97 ).
However, baby health care is often a major priority at this stage and women are generally very diligent in ensuring that their baby receives routine health checks, growth and developmental assessments and scheduled vaccinations. These often occur in a well-organized fashion for at least five years after birth ( 98 ). Therefore, obstetricians, family physicians, internists, and pediatricians must develop systems to link post-partum follow up women with GDM with the recommended routine care of their child as this appears to give the best chance of high quality care and health care engagement for the mother-baby dyad ( 3 ).
All offspring of mothers with HIP are at a heightened risk of glucometabolic and CV disease. However, the female offspring carry the additional risk of developing HIP themselves when they reach reproductive age and thus compounding the intergenerational “vicious cycle” of NCD transmission. Pregnant women with a maternal GDM history themselves are at higher risk of GDM compared to those with such a history from the paternal side ( 99 ). It is therefore important to test these women for hyperglycemia before conception or as early as possible during pregnancy and repeat testing in each trimester.
In summary, GDM represents a major challenge both in the short and long term. In the immediate pregnancy context, GDM detection and treatment are clearly valuable in improving outcomes. The associations with long term health of mothers and babies are also clear, but the optimal approach to treatment remains to be demonstrated. This is a global problem! Prevention and intervention, both during and following pregnancy are urgently needed to reduce the NCD burden of GDM as manifest for affected women and for their offspring. Despite expanding knowledge in this area, practical implementation of proven strategies remains limited. There is great potential for reduction in NCD burden from widespread implementation of relatively simple strategies, to stem the tide of the “slow motion disaster” of obesity and diabetes as identified by Doctor Margaret Chan, Director of the World Health Organization, in 2017 ( 100 ).
Author Contributions
HM wrote the first draft of the manuscript and is guarantor of the work. AK, HD, and MH all reviewed and edited the manuscript and approved the final draft. All authors contributed to the article and approved the submitted version.
Conflict of Interest
The authors declare that the research was conducted in the absence of any commercial or financial relationships that could be construed as a potential conflict of interest.
1. Cho NH, Shaw JE, Karuranga S, Huang Y, da Rocha Fernandes JD, Ohlrogge AW, et al. IDF Diabetes Atlas: Global estimates of diabetes prevalence for 2017 and projections for 2045. Diabetes Res Clin Pract (2018) 138:271–81. doi: 10.1016/j.diabres.2018.02.023
PubMed Abstract | CrossRef Full Text | Google Scholar
2. Colagiuri S, Falavigna M, Agarwal MM, Boulvain M, Coetzee E, Hod M, et al. Strategies for implementing the WHO diagnostic criteria and classification of hyperglycaemia first detected in pregnancy. Diabetes Res Clin Pract (2014) 103:364–72. doi: 10.1016/j.diabres.2014.02.012
3. Hod M, Kapur A, Sacks DA, Hadar E, Agarwal M, Di Renzo GC, et al. The International Federation of Gynecology and Obstetrics (FIGO) Initiative on gestational diabetes mellitus: A pragmatic guide for diagnosis, management, and care. Int J Gynaecol Obstet (2015) 131 Suppl 3:S173–211. doi: 10.1016/S0020-7292(15)30033-3
CrossRef Full Text | Google Scholar
4. Menke A, Casagrande S, Geiss L, Cowie CC. Prevalence of and Trends in Diabetes Among Adults in the United States, 1988-2012. Jama (2015) 314:1021–9. doi: 10.1001/jama.2015.10029
5. Menke A, Casagrande S, Cowie CC. Prevalence of Diabetes in Adolescents Aged 12 to 19 Years in the United States, 2005-2014. Jama (2016) 316:344–5. doi: 10.1001/jama.2016.8544
6. Catalano PM, McIntyre HD, Cruickshank JK, McCance DR, Dyer AR, Metzger BE, et al. The hyperglycemia and adverse pregnancy outcome study: associations of GDM and obesity with pregnancy outcomes. Diabetes Care (2012) 35:780–6. doi: 10.2337/dc11-1790
7. Metzger BE, Lowe LP, Dyer AR, Trimble ER, Chaovarindr U, Coustan DR, et al. Hyperglycemia and adverse pregnancy outcomes. N Engl J Med (2008) 358:1991–2002.
PubMed Abstract | Google Scholar
8. HAPO Study Cooperative Research Group. Hyperglycaemia and Adverse Pregnancy Outcome (HAPO) Study: associations with maternal body mass index. BJOG (2010) 117:575–84. doi: 10.1111/j.1471-0528.2009.02486.x
9. Metzger BE, Gabbe SG, Persson B, Buchanan TA, Catalano PA, Damm P, et al. International association of diabetes and pregnancy study groups recommendations on the diagnosis and classification of hyperglycemia in pregnancy. Diabetes Care (2010) 33:676–82. doi: 10.2337/dc10-0719
10. Sedgh G, Singh S, Hussain R. Intended and unintended pregnancies worldwide in 2012 and recent trends. Stud Fam Plann (2014) 45:301–14. doi: 10.1111/j.1728-4465.2014.00393.x
11. Seshiah V, Balaji V, Balaji MS, Panneerselvam A, Thamizharasi M, Arthi T. Glycemic level at the first visit and prediction of GDM. J Assoc Physicians India (2007) 55:630–2.
12. Lorenzo-Almorós A, Hang T, Peiró C, Soriano-Guillén L, Egido J, Tuñón J, et al. Predictive and diagnostic biomarkers for gestational diabetes and its associated metabolic and cardiovascular diseases. Cardiovasc Diabetol (2019) 18:140. doi: 10.1186/s12933-019-0935-9
13. Powe CE. Early Pregnancy Biochemical Predictors of Gestational Diabetes Mellitus. Curr Diabetes Rep (2017) 17:12. doi: 10.1007/s11892-017-0834-y
14. van Hoorn F, Koster M, Naaktgeboren CA, Groenendaal F, Kwee A, Lamain-de Ruiter M, et al. Prognostic models versus single risk factor approach in first-trimester selective screening for gestational diabetes mellitus: a prospective population-based multicentre cohort study. Bjog (2020). doi: 10.1111/1471-0528.16446
15. Artzi NS, Shilo S, Hadar E, Rossman H, Barbash-Hazan S, Ben-Haroush A, et al. Prediction of gestational diabetes based on nationwide electronic health records. Nat Med (2020) 26:71–6. doi: 10.1038/s41591-019-0724-8
16. Sweeting AN, Wong J, Appelblom H, Ross GP, Kouru H, Williams PF, et al. A first trimester prediction model for gestational diabetes utilizing aneuploidy and pre-eclampsia screening markers. J Matern Fetal Neonatal Med (2018) 31:2122–30. doi: 10.1080/14767058.2017.1336759
17. Ravnsborg T, Svaneklink S, Andersen LLT, Larsen MR, Jensen DM, Overgaard M. First-trimester proteomic profiling identifies novel predictors of gestational diabetes mellitus. PLoS One (2019) 14:e0214457. doi: 10.1371/journal.pone.0214457
18. Zhou T, Huang L, Wang M, Chen D, Chen Z, Jiang SW. A Critical Review of Proteomic Studies in Gestational Diabetes Mellitus. J Diabetes Res (2020) 2020:6450352. doi: 10.1155/2020/6450352
19. Jayabalan N, Lai A, Nair S, Guanzon D, Scholz-Romero K, Palma C, et al. Quantitative Proteomics by SWATH-MS Suggest an Association Between Circulating Exosomes and Maternal Metabolic Changes in Gestational Diabetes Mellitus. Proteomics (2019) 19:e1800164. doi: 10.1002/pmic.201800164
20. Herrera-Van Oostdam AS, Salgado-Bustamante M, López JA, Herrera-Van Oostdam DA, López-Hernández Y. Placental exosomes viewed from an ‘omics’ perspective: implications for gestational diabetes biomarkers identification. Biomark Med (2019) 13:675–84. doi: 10.2217/bmm-2018-0468
21. James-Allan LB, Rosario FJ, Barner K, Lai A, Guanzon D, McIntyre HD, et al. Regulation of glucose homeostasis by small extracellular vesicles in normal pregnancy and in gestational diabetes. FASEB J (2020) 34:5724–39. doi: 10.1096/fj.201902522RR
22. Yoffe L, Polsky A, Gilam A, Raff C, Mecacci F, Ognibene A, et al. Early diagnosis of gestational diabetes mellitus using circulating microRNAs. Eur J Endocrinol (2019) 181:565–77. doi: 10.1530/EJE-19-0206
23. Abdeltawab A, Zaki ME, Abdeldayem Y, Mohamed AA, Zaied SM. Circulating micro RNA-223 and angiopoietin-like protein 8 as biomarkers of gestational diabetes mellitus. Br J BioMed Sci (2020) 1–6. doi: 10.1080/09674845.2020.1764211
24. Filardi T, Catanzaro G, Mardente S, Zicari A, Santangelo C, Lenzi A, et al. Non-Coding RNA: Role in Gestational Diabetes Pathophysiology and Complications. Int J Mol Sci (2020) 21:4020. doi: 10.3390/ijms21114020
25. World Health Organization. Diagnostic Criteria and Classification of Hyperglycaemia First Detected in Pregnancy . Geneva: WHO Press (2013).
Google Scholar
26. A.C.o.P. Bulletins. ACOG Practice Bulletin No. 190: Gestational Diabetes Mellitus. Obstet Gynecol (2018) 131:e49–64. doi: 10.1097/AOG.0000000000002501
27. Canadian Diabetes Association. Canadian Diabetes Association 2008 Clinical Practice Guidelines for the Prevention and Management of Diabetes in Canada. Can J Diabetes (2008) 32:S168–81.
28. Round JA, Jacklin P, Fraser RB, Hughes RG, Mugglestone MA, Holt RI. Screening for gestational diabetes mellitus: cost-utility of different screening strategies based on a woman’s individual risk of disease. Diabetologia (2011) 54:256–63. doi: 10.1007/s00125-010-1881-y
29. NICE guidelines, Diabetes in pregnancy: management from preconception to the postnatal period . London, UK: National Institute for Health and Care Excellence: Clinical Guidelines (2015).
30. Tieu J, McPhee AJ, Crowther CA, Middleton P, Shepherd E. Screening for gestational diabetes mellitus based on different risk profiles and settings for improving maternal and infant health. Cochrane Database Syst Rev (2017) 8:Cd007222. doi: 10.1002/14651858.CD007222.pub4
31. Mo X, Tobe RG, Takahashi Y, Arata N, Liabsuetrakul T, Nakayama T, et al. Economic evaluations of gestational diabetes mellitus screening: A systematic review. J Epidemiol (2020). doi: 10.2188/jea.JE20190338
32. Persson M, Winkvist A, Mogren I. Surprisingly low compliance to local guidelines for risk factor based screening for gestational diabetes mellitus - A population-based study. BMC Pregnancy Childbirth (2009) 9:53. doi: 10.1186/1471-2393-9-53
33. Murphy NM, McCarthy FP, Khashan AS, Myers JE, Simpson NA, Kearney PM, et al. Compliance with National Institute of Health and Care Excellence risk-based screening for Gestational Diabetes Mellitus in nulliparous women. Eur J Obstet Gynecol Reprod Biol (2016) 199:60–5. doi: 10.1016/j.ejogrb.2016.01.044
34. Adam S, Rheeder P. Screening for gestational diabetes mellitus in a South African population: Prevalence, comparison of diagnostic criteria and the role of risk factors. S Afr Med J (2017) 107:523–7. doi: 10.7196/SAMJ.2017.v107i6.12043
35. Meththananda Herath HM, Weerarathna TP, Weerasinghe NP. Is Risk Factor-based Screening Good Enough to Detect Gestational Diabetes Mellitus in High-Risk Pregnant Women? A Sri Lankan Experience. Int J Prev Med (2016) 7:99. doi: 10.4103/2008-7802.188084
36. Bonongwe P, Lindow SW, Coetzee EJ. Reproducibility of a 75G oral glucose tolerance test in pregnant women. J Perinatal Med (2015) 43:333–8. doi: 10.1515/jpm-2014-0208
37. Dunseath GJ, Bright D, Jones C, Dowrick S, Cheung WY, Luzio SD. Performance evaluation of a self-administered home oral glucose tolerance test kit in a controlled clinical research setting. Diabetes Med (2019) 36:862–7. doi: 10.1111/dme.13961
38. Dhatt GS, Agarwal MM, Othman Y, Nair SC. Performance of the Roche Accu-Chek active glucose meter to screen for gestational diabetes mellitus using fasting capillary blood. Diabetes Technol Ther (2011) 13:1229–33.
39. Lowe LP, Metzger BE, Dyer AR, Lowe J, McCance DR, Lappin TR, et al. Hyperglycemia and Adverse Pregnancy Outcome (HAPO) Study: associations of maternal A1C and glucose with pregnancy outcomes. Diabetes Care (2012) 35:574–80.
40. Hughes RC, Moore MP, Gullam JE, Mohamed K, Rowan J. An early pregnancy HbA1c >/=5.9% (41 mmol/mol) is optimal for detecting diabetes and identifies women at increased risk of adverse pregnancy outcomes. Diabetes Care (2014) 37:2953–9.
41. Mendes N, Tavares Ribeiro R, Serrano F. Beyond self-monitored plasma glucose and HbA1c: the role of non-traditional glycaemic markers in gestational diabetes mellitus. J Obstet Gynaecol (2018) 38:762–9.
42. Ghosh P, Luque-Fernandez MA, Vaidya A, Ma D, Sahoo R, Chorev M, et al. Plasma Glycated CD59, a Novel Biomarker for Detection of Pregnancy-Induced Glucose Intolerance. Diabetes Care (2017) 40:981–4.
43. Ma D, Luque-Fernandez MA, Bogdanet D, Desoye G, Dunne F, Halperin JA. Plasma Glycated CD59 Predicts Early Gestational Diabetes and Large for Gestational Age Newborns. J Clin Endocrinol Metab (2020) 105:e1033–40. doi: 10.1210/clinem/dgaa087
44. Bogdanet D, O’Shea PM, Halperin J, Dunne F. Plasma glycated CD59 (gCD59), a novel biomarker for the diagnosis, management and follow up of women with Gestational Diabetes (GDM) - protocol for prospective cohort study. BMC Pregnancy Childbirth (2020) 20:412. doi: 10.1186/s12884-020-03090-9
45. Zhang C, Rawal S, Chong YS. Risk factors for gestational diabetes: is prevention possible? Diabetologia (2016) 59:1385–90. doi: 10.1007/s00125-016-3979-3
46. Kwong W, Tomlinson G, Feig DS. Maternal and neonatal outcomes after bariatric surgery; a systematic review and meta-analysis: do the benefits outweigh the risks? Am J Obstet Gynecol (2018) 218:573–80. doi: 10.1016/j.ajog.2018.02.003
47. Griffith RJ, Alsweiler J, Moore AE, Brown S, Middleton P, Shepherd E, et al. Interventions to prevent women from developing gestational diabetes mellitus: an overview of Cochrane Reviews. Cochrane Database Syst Rev (2020) 6:Cd012394. doi: 10.1002/14651858.CD012394.pub3
48. Song C, Li J, Leng J, Ma RC, Yang X. Lifestyle intervention can reduce the risk of gestational diabetes: a meta-analysis of randomized controlled trials. Obes Rev (2016) 10:960–9. doi: 10.1111/obr.12442
49. Lindsay RS, Loeken MR. Metformin use in pregnancy: promises and uncertainties. Diabetologia (2017) 60:1612–9. doi: 10.1007/s00125-017-4351-y
50. Chiswick C, Reynolds RM, Denison F, Drake AJ, Forbes S, Newby DE, et al. Effect of metformin on maternal and fetal outcomes in obese pregnant women (EMPOWaR): a randomised, double-blind, placebo-controlled trial. Lancet Diabetes Endocrinol (2015) 3:778–86. doi: 10.1016/S2213-8587(15)00219-3
51. Syngelaki A, Nicolaides KH, Balani J, Hyer S, Akolekar R, Kotecha R, et al. Metformin versus Placebo in Obese Pregnant Women without Diabetes Mellitus. N Engl J Med (2016) 374:434–43. doi: 10.1056/NEJMoa1509819
52. Dodd JM, Louise J, Deussen AR, Grivell RM, Dekker G, McPhee AJ, et al. Effect of metformin in addition to dietary and lifestyle advice for pregnant women who are overweight or obese: the GRoW randomised, double-blind, placebo-controlled trial. Lancet Diabetes Endocrinol (2019) 7:15–24. doi: 10.1016/S2213-8587(18)30310-3
53. Corrado F, D’Anna R, Di Vieste G, Giordano D, Pintaudi B, Santamaria A, et al. The effect of myoinositol supplementation on insulin resistance in patients with gestational diabetes. Diabetes Med (2011) 28:972–5. doi: 10.1111/j.1464-5491.2011.03284.x
54. D’Anna R, Scilipoti A, Giordano D, Caruso C, Cannata ML, Interdonato ML, et al. myo-Inositol supplementation and onset of gestational diabetes mellitus in pregnant women with a family history of type 2 diabetes: a prospective, randomized, placebo-controlled study. Diabetes Care (2013) 36:854–7. doi: 10.2337/dc12-1371
55. Farren M, Daly N, McKeating A, Kinsley B, Turner MJ, Daly S. The Prevention of Gestational Diabetes Mellitus With Antenatal Oral Inositol Supplementation: A Randomized Controlled Trial. Diabetes Care (2017) 40:759–63. doi: 10.2337/dc16-2449
56. Malvasi A, Kosmas I, Mynbaev OA, Sparic R, Gustapane S, Guido M, et al. Can trans resveratrol plus d-chiro-inositol and myo-inositol improve maternal metabolic profile in overweight pregnant patients? Clin Ter (2017) 168:e240–7.
57. Godfrey KM, Cutfield W, Chan SY, Baker PN, Chong YS. Nutritional Intervention Preconception and During Pregnancy to Maintain Healthy Glucose Metabolism and Offspring Health (“NiPPeR”): study protocol for a randomised controlled trial. Trials (2017) 18:131. doi: 10.1186/s13063-017-1875-x
58. Isolauri E, Rautava S, Collado MC, Salminen S. Role of probiotics in reducing the risk of gestational diabetes. Diabetes Obes Metab (2015) 17:713–9. doi: 10.1111/dom.12475
59. Lindsay KL, Kennelly M, Culliton M, Smith T, Maguire OC, Shanahan F, et al. Probiotics in obese pregnancy do not reduce maternal fasting glucose: a double-blind, placebo-controlled, randomized trial (Probiotics in Pregnancy Study). Am J Clin Nutr (2014) 99:1432–9. doi: 10.3945/ajcn.113.079723
60. Callaway LK, McIntyre HD, Barrett HL, Foxcroft K, Tremellen A, Lingwood BE, et al. Probiotics for the Prevention of Gestational Diabetes Mellitus in Overweight and Obese Women: Findings From the SPRING Double-blind Randomized Controlled Trial. Diabetes Care (2019) 42:364–71. doi: 10.2337/dc18-2248
61. Okesene-Gafa KAM, Li M, McKinlay CJD, Taylor RS, Rush EC, Wall CR, et al. Effect of antenatal dietary interventions in maternal obesity on pregnancy weight-gain and birthweight: Healthy Mums and Babies (HUMBA) randomized trial. Am J Obstet Gynecol (2019) 221:152.e1–152.e13.
62. Pellonperä O, Mokkala K, Houttu N, Vahlberg T, Koivuniemi E, Tertti K, et al. Efficacy of Fish Oil and/or Probiotic Intervention on the Incidence of Gestational Diabetes Mellitus in an At-Risk Group of Overweight and Obese Women: A Randomized, Placebo-Controlled, Double-Blind Clinical Trial. Diabetes Care (2019) 42:1009–17. doi: 10.2337/dc18-2591
63. Halkjær SI, de Knegt VE, Lo B, Nilas L, Cortes D, Pedersen AE, et al. Multistrain Probiotic Increases the Gut Microbiota Diversity in Obese Pregnant Women: Results from a Randomized, Double-Blind Placebo-Controlled Study. Curr Dev Nutr (2020) 4:nzaa095. doi: 10.1093/cdn/nzaa095
64. Luo X, Ma X, Hu H, Li C, Cao S, Huang L, et al. Kinetic study of pentosan solubility during heating and reacting processes of steam treatment of green bamboo. Bioresour Technol (2013) 130:769–76. doi: 10.1016/j.biortech.2012.12.088
65. Wood K, Mantzioris E, Lingwood B, Couper J, Makrides M, Gibson RA, et al. The effect of maternal DHA supplementation on body fat mass in children at 7 years: follow-up of the DOMInO randomized controlled trial. Prostaglandins Leukot Essent Fatty Acids (2017) 139:49–54. doi: 10.1016/j.plefa.2017.09.013
66. Sadeghian M, Asadi M, Rahmani S, Akhavan Zanjani M, Sadeghi O, Hosseini SA, et al. Circulating vitamin D and the risk of gestational diabetes: a systematic review and dose-response meta-analysis. Endocrine (2020) 70:36–47. doi: 10.1007/s12020-020-02360-y
67. Palacios C, Kostiuk LK, Peña-Rosas JP. Vitamin D supplementation for women during pregnancy. Cochrane Database Syst Rev (2019) 7:Cd008873. doi: 10.1002/14651858.CD008873.pub4
68. Corcoy R, Mendoza LC, Simmons D, Desoye G, Mathiesen ER, Kautzky-Willer A, et al. Vitamin D and gestational diabetes mellitus: a systematic review based on data free of Hawthorne effect. BJOG (2018) 125:1338–9. doi: 10.1111/1471-0528.15278
69. Bokharee N, Khan YH, Wasim T, Mallhi TH, Alotaibi NH, Iqbal MS, et al. Daily versus stat vitamin D supplementation during pregnancy; A prospective cohort study. PLoS One (2020) 15:e0231590. doi: 10.1371/journal.pone.0231590
70. Cannell JJ. Vitamin D and autism, what’s new? Rev Endocr Metab Disord (2017) 18:183–93. doi: 10.1007/s11154-017-9409-0
71. McIntyre HD, Catalano P, Zhang C, Desoye G, Mathiesen ER, Damm P. Gestational diabetes mellitus. Nat Rev Dis Primers (2019) 5:47. doi: 10.1038/s41572-019-0098-8
72. Crowther CA, Hiller JE, Moss JR, McPhee AJ, Jeffries WS, Robinson JS. Effect of treatment of gestational diabetes mellitus on pregnancy outcomes. N Engl J Med (2005) 352:2477–86. doi: 10.1056/NEJMoa042973
73. Landon MB, Spong CY, Thom E, Carpenter MW, Ramin SM, Casey B, et al. A multicenter, randomized trial of treatment for mild gestational diabetes. N Engl J Med (2009) 361:1339–48. doi: 10.1056/NEJMoa0902430
74. Vounzoulaki E, Khunti K, Abner SC, Tan BK, Davies MJ, Gillies CL. Progression to type 2 diabetes in women with a known history of gestational diabetes: systematic review and meta-analysis. BMJ (2020) 369:m1361. doi: 10.1136/bmj.m1361
75. Retnakaran R, Shah BR. Mild glucose intolerance in pregnancy and risk of cardiovascular disease: a population-based cohort study. CMAJ (2009) 181:371–6. doi: 10.1503/cmaj.090569
76. Retnakaran R, Shah BR. Role of Type 2 Diabetes in Determining Retinal, Renal, and Cardiovascular Outcomes in Women With Previous Gestational Diabetes Mellitus. Diabetes Care (2017) 40:101–8. doi: 10.2337/dc16-1400
77. Kramer CK, Campbell S, Retnakaran R. Gestational diabetes and the risk of cardiovascular disease in women: a systematic review and meta-analysis. Diabetologia (2019) 62:905–14. doi: 10.1007/s00125-019-4840-2
78. Bao W, Tobias DK, Bowers K, Chavarro J, Vaag A, Grunnet LG, et al. Physical activity and sedentary behaviors associated with risk of progression from gestational diabetes mellitus to type 2 diabetes mellitus: a prospective cohort study. JAMA Internal Med (2014) 174:1047–55. doi: 10.1001/jamainternmed.2014.1795
79. Much D, Beyerlein A, Roßbauer M, Hummel S, Ziegler AG. Beneficial effects of breastfeeding in women with gestational diabetes mellitus. Mol Metab (2014) 3:284–92. doi: 10.1016/j.molmet.2014.01.002
80. Ma S, Hu S, Liang H, Xiao Y, Tan H. Metabolic effects of breastfeed in women with prior gestational diabetes mellitus: A systematic review and meta-analysis. Diabetes Metab Res Rev (2019) 35:e3108. doi: 10.1002/dmrr.3108
81. Cnattingius S, Villamor E. Weight change between successive pregnancies and risks of stillbirth and infant mortality: a nationwide cohort study. Lancet (2016) 387:558–65. doi: 10.1016/S0140-6736(15)00990-3
82. Lowe WL Jr., Scholtens DM, Kuang A, Linder B, Lawrence JM, Lebenthal Y, et al. Hyperglycemia and Adverse Pregnancy Outcome Follow-up Study (HAPO FUS): Maternal Gestational Diabetes and Childhood Glucose Metabolism. Diabetes Care (2019) 42:372–80. doi: 10.2337/dc18-1646
83. Nehring I, Chmitorz A, Reulen H, von Kries R, Ensenauer R. Gestational diabetes predicts the risk of childhood overweight and abdominal circumference independent of maternal obesity. Diabetes Med (2013) 30:1449–56. doi: 10.1111/dme.12286
84. Dabelea D, Mayer-Davis EJ, Lamichhane AP, D’Agostino RB Jr., Liese AD, Vehik KS, et al. Association of intrauterine exposure to maternal diabetes and obesity with type 2 diabetes in youth: the SEARCH Case-Control Study. Diabetes Care (2008) 31:1422–6. doi: 10.2337/dc07-2417
85. Osgood ND, Dyck RF, Grassmann WK. The inter- and intragenerational impact of gestational diabetes on the epidemic of type 2 diabetes. Am J Public Health (2011) 101:173–9. doi: 10.2105/AJPH.2009.186890
86. Leybovitz-Haleluya N, Wainstock T, Landau D, Sheiner E. Maternal gestational diabetes mellitus and the risk of subsequent pediatric cardiovascular diseases of the offspring: a population-based cohort study with up to 18 years of follow up. Acta Diabetol (2018) 55:10037–42. doi: 10.1016/j.reprotox.2018.03.009
87. Nahum Sacks K, Friger M, Shoham-Vardi I, Abokaf H, Spiegel E, Sergienko R, et al. Prenatal exposure to gestational diabetes mellitus as an independent risk factor for long-term neuropsychiatric morbidity of the offspring. Am J Obstet Gynecol (2016) 215:380.e1–7.
88. Gillman MW, Oakey H, Baghurst PA, Volkmer RE, Robinson JS, Crowther CA. Effect of treatment of gestational diabetes mellitus on obesity in the next generation. Diabetes Care (2010) 33:964–8. doi: 10.2337/dc09-1810
89. Landon MB, Rice MM, Varner MW, Casey BM, Reddy UM, Wapner RJ, et al. Mild gestational diabetes mellitus and long-term child health. Diabetes Care (2015) 38:445–52. doi: 10.2337/dc14-2159
90. Bahado-Singh RO, Mele L, Landon MB, Ramin SM, Carpenter MW, Casey B, et al. Fetal male gender and the benefits of treatment of mild gestational diabetes mellitus. Am J Obstet Gynecol (2012) 206:422.e1–5.
91. Gunderson EP, Greenspan LC, Faith MS, Hurston SR, Quesenberry CP Jr. Breastfeeding and growth during infancy among offspring of mothers with gestational diabetes mellitus: a prospective cohort study. Pediatr Obes (2018) 13:492–504. doi: 10.1111/ijpo.12277
92. Gabbe SG, Landon MB, Warren-Boulton E, Fradkin J. Promoting health after gestational diabetes: a National Diabetes Education Program call to action. Obstet Gynecol (2012) 119:171–6. doi: 10.1097/AOG.0b013e3182393208
93. Waters TP, Minium J, Hagiac M, Schnellinger P, Ryan D, Haugel-de Mouzon S, et al. Does maternal insulin sensitivity improve immediately after delivery or do we need to wait unti six weeks postpartum? Am J Obstet Gynecol (2015) 212:S20. doi: 10.1016/j.ajog.2014.10.074
94. Waters TP, Kim SY, Werner E, Dinglas C, Carter EB, Patel R, et al. Should women with gestational diabetes be screened at delivery hospitalization for type 2 diabetes? Am J Obstet Gynecol (2020) 222:73.e1–73.e11.
95. A.D. Association. 2. Classification and Diagnosis of Diabetes: Standards of Medical Care in Diabetes-2020. Diabetes Care (2020) 43:S14–s31. doi: 10.2337/dc20-S002
96. Nielsen KK, Kapur A, Damm P, de Courten M, Bygbjerg IC. From screening to postpartum follow-up - the determinants and barriers for gestational diabetes mellitus (GDM) services, a systematic review. BMC Pregnancy Childbirth (2014) 14:41. doi: 10.1186/1471-2393-14-41
97. Wilkinson SA, Lim SS, Upham S, Pennington A, O’Reilly SL, Asproloupos D, et al. Who’s responsible for the care of women during and after a pregnancy affected by gestational diabetes? Med J Aust (2014) 201:S78–81. doi: 10.5694/mja14.00251
98. Kapur A. Pregnancy: a window of opportunity for improving current and future health. Int J Gynaecol Obstet (2011) 115:S50–1. doi: 10.1016/S0020-7292(11)60015-5
99. Kragelund Nielsen K, Damm P, Kapur A, Balaji V, Balaji MS, Seshiah V, et al. Risk Factors for Hyperglycaemia in Pregnancy in Tamil Nadu, India. PLoS One (2016) 11:e0151311. doi: 10.1371/journal.pone.0151311
100. Chan M. Obesity and Diabetes: The Slow-Motion Disaster. Milbank Q (2017) 95:11–4. doi: 10.1111/1468-0009.12238
Keywords: gestational diabetes, prediction, diagnosis, prevention, management, pregnancy, non-communicable disease
Citation: McIntyre HD, Kapur A, Divakar H and Hod M (2020) Gestational Diabetes Mellitus—Innovative Approach to Prediction, Diagnosis, Management, and Prevention of Future NCD—Mother and Offspring. Front. Endocrinol. 11:614533. doi: 10.3389/fendo.2020.614533
Received: 06 October 2020; Accepted: 04 November 2020; Published: 03 December 2020.
Reviewed by:
Copyright © 2020 McIntyre, Kapur, Divakar and Hod. This is an open-access article distributed under the terms of the Creative Commons Attribution License (CC BY) . The use, distribution or reproduction in other forums is permitted, provided the original author(s) and the copyright owner(s) are credited and that the original publication in this journal is cited, in accordance with accepted academic practice. No use, distribution or reproduction is permitted which does not comply with these terms.
*Correspondence: H. David McIntyre, [email protected]
Disclaimer: All claims expressed in this article are solely those of the authors and do not necessarily represent those of their affiliated organizations, or those of the publisher, the editors and the reviewers. Any product that may be evaluated in this article or claim that may be made by its manufacturer is not guaranteed or endorsed by the publisher.
- Introduction
- Conclusions
- Article Information
a Values are rounded either up or down to a multiple of 5 for patient confidentiality purposes.
b Fatal events occurring at any point between 20 weeks’ gestation in the second pregnancy and 12 weeks post partum. Five deaths were related to a fatal cardiovascular disease (CVD) event while the remaining 15 fatalities were related to obstetrical complications related to childbirth, major trauma, and suicide.
The log-rank test suggested significant differences in event-free survival across exposure groups. GD indicates gestational diabetes.
The corresponding unadjusted hazard ratios (HRs) among women with no GD, GD in first pregnancy, GD in second pregnancy and GD in both pregnancies, in Panel A were 4.80 (95% CI, 4.48-5.14), 9.09 (95% CI, 8.67-9.53), and 19.05 (95% CI, 18.10-19.91) for GD in first pregnancy, GD in second pregnancy, and GD in both pregnancies, respectively. The corresponding unadjusted HRs among those in panel B were 1.89 (95% CI, 1.75-2.05) and 3.96 (95% CI, 3.66-4.28) for GD in second pregnancy and GD in both pregnancies, respectively. The corresponding unadjusted HR among those in panel C was 2.09 (95% CI, 1.97-2.22) for GD in both pregnancies.
eTable 1. ICD Codes
eTable 2. Comparing Effect Estimates of the Diabetes Outcome, With and Without Inclusion of Years of Pregnancy, with Account for Temporal Trends in the Screening and Diagnosis of Gestational Diabetes
eTable 3. Associations of Incident Diabetes With Gestational Diabetes Occurrences When Including Women With Stillbirth Pregnancies in Cohort
eFigure 1. Timeline of Applied Exclusions and Exposure Ascertainment Windows
eFigure 2. Distribution of Gestational Diabetes Exposure Categories
Data Sharing Statement
See More About
Sign up for emails based on your interests, select your interests.
Customize your JAMA Network experience by selecting one or more topics from the list below.
- Academic Medicine
- Acid Base, Electrolytes, Fluids
- Allergy and Clinical Immunology
- American Indian or Alaska Natives
- Anesthesiology
- Anticoagulation
- Art and Images in Psychiatry
- Artificial Intelligence
- Assisted Reproduction
- Bleeding and Transfusion
- Caring for the Critically Ill Patient
- Challenges in Clinical Electrocardiography
- Climate and Health
- Climate Change
- Clinical Challenge
- Clinical Decision Support
- Clinical Implications of Basic Neuroscience
- Clinical Pharmacy and Pharmacology
- Complementary and Alternative Medicine
- Consensus Statements
- Coronavirus (COVID-19)
- Critical Care Medicine
- Cultural Competency
- Dental Medicine
- Dermatology
- Diabetes and Endocrinology
- Diagnostic Test Interpretation
- Drug Development
- Electronic Health Records
- Emergency Medicine
- End of Life, Hospice, Palliative Care
- Environmental Health
- Equity, Diversity, and Inclusion
- Facial Plastic Surgery
- Gastroenterology and Hepatology
- Genetics and Genomics
- Genomics and Precision Health
- Global Health
- Guide to Statistics and Methods
- Hair Disorders
- Health Care Delivery Models
- Health Care Economics, Insurance, Payment
- Health Care Quality
- Health Care Reform
- Health Care Safety
- Health Care Workforce
- Health Disparities
- Health Inequities
- Health Policy
- Health Systems Science
- History of Medicine
- Hypertension
- Images in Neurology
- Implementation Science
- Infectious Diseases
- Innovations in Health Care Delivery
- JAMA Infographic
- Law and Medicine
- Leading Change
- Less is More
- LGBTQIA Medicine
- Lifestyle Behaviors
- Medical Coding
- Medical Devices and Equipment
- Medical Education
- Medical Education and Training
- Medical Journals and Publishing
- Mobile Health and Telemedicine
- Narrative Medicine
- Neuroscience and Psychiatry
- Notable Notes
- Nutrition, Obesity, Exercise
- Obstetrics and Gynecology
- Occupational Health
- Ophthalmology
- Orthopedics
- Otolaryngology
- Pain Medicine
- Palliative Care
- Pathology and Laboratory Medicine
- Patient Care
- Patient Information
- Performance Improvement
- Performance Measures
- Perioperative Care and Consultation
- Pharmacoeconomics
- Pharmacoepidemiology
- Pharmacogenetics
- Pharmacy and Clinical Pharmacology
- Physical Medicine and Rehabilitation
- Physical Therapy
- Physician Leadership
- Population Health
- Primary Care
- Professional Well-being
- Professionalism
- Psychiatry and Behavioral Health
- Public Health
- Pulmonary Medicine
- Regulatory Agencies
- Reproductive Health
- Research, Methods, Statistics
- Resuscitation
- Rheumatology
- Risk Management
- Scientific Discovery and the Future of Medicine
- Shared Decision Making and Communication
- Sleep Medicine
- Sports Medicine
- Stem Cell Transplantation
- Substance Use and Addiction Medicine
- Surgical Innovation
- Surgical Pearls
- Teachable Moment
- Technology and Finance
- The Art of JAMA
- The Arts and Medicine
- The Rational Clinical Examination
- Tobacco and e-Cigarettes
- Translational Medicine
- Trauma and Injury
- Treatment Adherence
- Ultrasonography
- Users' Guide to the Medical Literature
- Vaccination
- Venous Thromboembolism
- Veterans Health
- Women's Health
- Workflow and Process
- Wound Care, Infection, Healing
Get the latest research based on your areas of interest.
Others also liked.
- Download PDF
- X Facebook More LinkedIn
Mussa J , Rahme E , Dahhou M , Nakhla M , Dasgupta K. Incident Diabetes in Women With Patterns of Gestational Diabetes Occurrences Across 2 Pregnancies. JAMA Netw Open. 2024;7(5):e2410279. doi:10.1001/jamanetworkopen.2024.10279
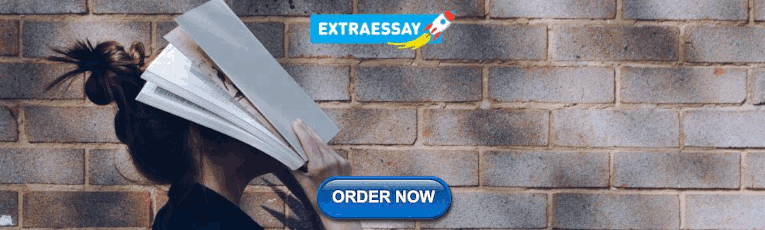
Manage citations:
© 2024
- Permissions
Incident Diabetes in Women With Patterns of Gestational Diabetes Occurrences Across 2 Pregnancies
- 1 Department of Medicine, McGill University, Montreal, Quebec, Canada
- 2 Centre for Outcomes Research and Evaluation (CORE), Research Institute of the McGill University Health Centre (RI-MUHC), Montreal, Quebec, Canada
- 3 Department of Pediatrics, McGill University, Montreal, Quebec, Canada
Question Across 2 pregnancies, are the order and number of gestational diabetes occurrences linked to maternal diabetes risk in the years after second pregnancy?
Findings In this cohort study of 431 980 women, those with a first occurrence of gestational diabetes in a second pregnancy had a 76% higher risk for diabetes development than women who had gestational diabetes in the first pregnancy but not in the second, a statistically significant difference. The highest risk was in women with gestational diabetes in both pregnancies.
Meaning These findings suggest that considering gestational diabetes history in each pregnancy results in more accurate diabetes risk estimation than a simple yes/no dichotomy of past gestational diabetes occurrence.
Importance Gestational diabetes is a type 2 diabetes risk indicator, and recurrence further augments risk. In women with a single occurrence across 2 pregnancies, it is unclear whether first- vs second-pregnancy gestational diabetes differ in terms of risk.
Objective To compare the hazards of incident diabetes among those with gestational diabetes in the first, in the second, and in both pregnancies with women without gestational diabetes in either.
Design, Setting, and Participants This was a retrospective cohort study with cohort inception from April 1, 1990, to December 31, 2012. Follow-up was April 1, 1990, to April 1, 2019. Participants were mothers with 2 singleton deliveries between April 1, 1990, and December 31, 2012, without diabetes before or between pregnancies, who were listed in public health care insurance administrative databases and birth, stillbirth, and death registries in Quebec, Canada. Data were analyzed from July to December 2023.
Exposure Gestational diabetes occurrence(s) across 2 pregnancies.
Main outcomes and measures Incident diabetes from the second delivery until a third pregnancy, death, or the end of the follow-up period, whichever occurred first.
Results The 431 980 women with 2 singleton deliveries studied had a mean (SD) age of 30.1 (4.5) years at second delivery, with a mean (SD) of 2.8 (1.5) years elapsed between deliveries; 373 415 (86.4%) were of European background, and 78 770 (18.2%) were at the highest quintile of material deprivation. Overall, 10 920 women (2.5%) had gestational diabetes in their first pregnancy, 16 145 (3.7%) in their second, and 8255 (1.9%) in both (12 205 incident diabetes events; median [IQR] follow-up 11.5 [5.3-19.4] years). First pregnancy–only gestational diabetes increased hazards 4.35-fold (95% CI, 4.06-4.67), second pregnancy–only increased hazards 7.68-fold (95% CI, 7.31-8.07), and gestational diabetes in both pregnancies increased hazards 15.8-fold (95% CI, 15.0-16.6). Compared with first pregnancy–only gestational diabetes, second pregnancy–only gestational diabetes increased hazards by 76% (95% CI, 1.63-1.91), while gestational diabetes in both pregnancies increased it 3.63-fold (95% CI, 3.36-3.93).
Conclusions and relevance In this retrospective cohort study of nearly half a million women with 2 singleton pregnancies, both the number and ordinal pregnancy of any gestational diabetes occurrence increased diabetes risk. These considerations offer greater nuance than an ever or never gestational diabetes dichotomy.
Gestational diabetes (GD) affects 14% of pregnancies globally. 1 A recent meta-analysis 2 estimates its occurrence is associated with a 10-fold risk increase for type 2 diabetes. Whether risks vary with the order of GD occurrences is not well-studied. We hypothesized that new GD occurrence in a second pregnancy implies transition to a higher risk profile, while a single occurrence in a first pregnancy implies the converse.
One challenge is that GD is conditional on pregnancy (ie, cannot occur without pregnancy) and the number of pregnancies itself is associated with type 2 diabetes risk. The lowest risk occurs in those with 1 pregnancy. 3 Two previous studies 4 , 5 tried to improve comparability among participants by requiring that all have at least 2 pregnancies. Both reported a 2.4-fold increase in hazards with GD recurrence compared with its absence in the second pregnancy. They did not include women without any GD or women with a new occurrence of GD in a second pregnancy.
In the longer term, first-pregnancy GD may motivate some to adopt behaviors demonstrated to reduce diabetes risk, 6 , 7 lowering GD recurrence rates and type 2 diabetes development. In contrast, some women without GD in the first pregnancy may enter a higher risk trajectory, related to excess gestational weight gain, 8 postpartum weight retention, 9 weight gain between pregnancies, 10 , 11 and motherhood demands impeding healthy eating and physical activity. 12 The delineation of differences in future incident type 2 diabetes risk between GD occurrence in a first pregnancy compared with new occurrence in a second could allow further personalization of approaches to type 2 diabetes prevention. 13 We therefore examined patterns of GD absence, occurrence, and recurrence across 2 pregnancies and their associations with diabetes.
The McGill University Health Centre’s research ethics board and Quebec Access to Information Commission approved the protocol. These bodies waived informed consent because the study involved deidentified data, analyses at the Quebec Statistical Institute’s secure data centers, and rounded frequencies to multiples of 5. This cohort study followed the Strengthening the Reporting of Observational Studies in Epidemiology ( STROBE ) reporting guidelines.
We conducted a retrospective cohort study in Quebec, Canada. We examined health administrative databases of the public health insurance plan linked to birth, stillbirth, and death registries by the Quebec Statistical Institute (probabilistic linkage). We obtained mothers’ residential territory and month and year of birth from the public health insurance registry. The Physician Services Claims and Hospitalization Discharge Databases include diagnostic codes (eTable 1 in Supplement 1 ) and hospitalization dates; we used these to define outcomes, exposures, and other variables alongside data from birth and stillbirth registries (offspring birthdates, gestational age at birth, birthweight, parental country of birth and first language, and years of maternal education). We also had access to the mothers’ Institut national de santé publique du Québec (INSPQ) material and social deprivation index, derived from the 6-digit postal code in the public health registry. 14 The Institut national de santé publique du Québec (INSPQ) material and social deprivation index is computed from small-area census data. Specifically, the material indices are derived from average income, proportions without high school diploma, and employment to population ratio among those 15 years and older. The social indices are derived from the proportion of the population who are single-parent families, aged 15 years and older living alone, and aged 15 years and older who are separated, divorced, or widowed. To assign the INSPQ index for each woman, we first checked availability of this variable in the index year (year of second delivery).
We considered women with 2 or more consecutive singleton deliveries between April 1, 1990, and December 31, 2012, who were alive at 12 weeks following the second delivery (index date) (eFigure 1 in Supplement 1 ). We excluded mothers with missing offspring gestational age (required to distinguish diabetes from GD), 15 and those with diabetes or hypertension before or between pregnancies. We applied the validated Canadian Chronic Disease Surveillance System (CCDSS) diabetes 16 , 17 and hypertension 18 definitions of 2 outpatient or 1 hospitalization diagnostic code(s) to (1) the 2-year period before 20 weeks’ gestation in first pregnancy and (2) the period from 12 weeks after the first delivery until 20 weeks’ gestation in the second pregnancy. All required an opportunity to develop GD for both of the pregnancies considered, thus gestation 20 or more weeks was required. In the primary analysis, we required the same partner for each offspring to minimize heterogeneity of within-household factors. 19 - 21 This resulted in the removal of stillbirths, for whom paternal data were unavailable; in a sensitivity analysis, we removed the paternal data requirement. Lastly, we excluded those with 2 outpatient visits or 1 hospitalization for cardiovascular disease, the most common diabetes consequence, before the index date.
We adapted a validated health administrative database GD definition 22 that applies diabetes and GD diagnostic codes to a pregnancy-specific period. We started this period at 20 weeks’ gestation instead of the 120-day predelivery date used in the validation study, as the information we had on gestational age allowed us to conform with clinical definitions, which considers type 2 diabetes before 20 weeks’ gestation as preexisting. 15 We extended the period beyond delivery to 12 weeks post partum, as screening for type 2 diabetes after pregnancy is generally advised by this time. 23 , 24 We required 2 outpatient and/or 1 hospitalization code to maximize specificity (99.5%) and maintain sensitivity (94.1%), as recommended in the validation study. 22 Our 4 mutually exclusive exposure categories were absence of GD, its presence in only first pregnancy, in only the second, and in both.
Our primary outcome was incident diabetes, using the previously described CCDSS definition. 16 , 17 These diagnostic codes cannot differentiate between type 1 and type 2 diabetes, but because 95% of incident diabetes among adults is type 2 diabetes, they primarily capture incident type 2 diabetes. Follow-up was until the first of incident diabetes, the 120-day time point before a third delivery (we did not have gestational age data for any third pregnancy), death, or the end of the study period (April 1, 2019).
For both the first and second pregnancies, we considered other pregnancy and offspring-related factors associated with type 2 diabetes development, specifically, gestational hypertension (with or without preeclampsia), preterm delivery (<37 weeks), and small-for-gestational-age (SGA) and large-for-gestational-age (LGA) status. 25 , 26 For gestational hypertension, we applied the CCDSS hypertension definition to the same pregnancy periods for which we defined GD, and we also considered diagnostic codes for gestational hypertension and preeclampsia.
We considered time between deliveries, comorbid conditions, maternal age at index date, deprivation level (see Table 1 footnotes), 14 preexisting paternal diabetes and hypertension (validated CCDSS definitions 16 , 17 applied from 2 years before 20 weeks’ gestation in the first pregnancy to 12 weeks following the second delivery), and ethnocultural background (African/Caribbean [if born in West/South/East/Central Africa or first language was of Caribbean or African descent], Arabic [if born in the Arab league or first language was of Arabic or other North African or South-West Asian descent], Asian [if born in West/East/Central/South/Southeast/Pacific Asia or first language descends from these regions], European [if born in North America, South America, Central America, Mexico, East/South/Southern/West Europe, or Australia and first language was English, French, or other European language], and other [if first language was of Indigenous descent) based on participant-reported place of birth and primary language recorded on the mandatory birth declaration and incorporated into the birth registry. Ethnocultural background was assessed in this study because those with background other than European have a higher baseline risk for diabetes.
We computed baseline characteristics (counts and proportions for categorical variables and mean [SD] for continuous variables) and compared them across exposure groups (Pearson χ 2 tests for proportions; 1-way analysis of variance for means, as applicable). We calculated type 2 diabetes incidence rates (IR). We assessed for interactions ( P < .05 for interaction terms) and multicollinearity (Cramer V > 0.10) among exposures and covariates. We constructed Kaplan-Meier curves and compared these through log-rank testing. We evaluated proportional hazards assumptions (log-minus-log survival plots, Schoenfeld residuals) and performed some transformations to fulfill these (age as a spline variable and binary gestational hypertension category defined as presence in either or both pregnancies vs neither).
We constructed multivariable Cox proportional hazards models to compute hazard ratios (HR) for type 2 diabetes, first with GD absence in either pregnancy as the reference group. We then examined models with GD in only the first pregnancy as the reference group and finally with GD in only the second as the reference group. We retained covariates based on univariate association with type 2 diabetes where P ≤ .25, multivariable association (stepwise selection) where P ≤ .05, and reduced bayesian information criteria values with inclusion (see eMethods in Supplement 1 for omitted variables).
In a sensitivity analysis, we evaluated the change in associations when including calendar years of each pregnancy in our models to account for temporal trends in the screening and diagnosis of GD over the years. 27 In another sensitivity analysis, we retained women with stillbirth deliveries and accounted for stillbirths in the model, along with miscarriages between pregnancies. In a third sensitivity analysis, we applied indirect adjustments for obesity and smoking status to our main results, using established methods. 28 , 29 This bias analysis required external estimates for the HRs of obesity and of smoking with incident type 2 diabetes in women, which we respectively estimated as 3.90 (obesity vs no obesity) 30 and 1.13 (smoking vs not smoking). 31 This method also required external cohort data for obesity and smoking prevalence in groups of women corresponding to our main exposure categories. We used the Canadian Community Health Survey (Cycle 2.2) for this purpose 32 ; 13% were in the obesity category and 24% smoked cigarettes. We applied the following formula for the indirect obesity adjustment:
HR (corrected for obesity) = HR (from our analysis) / HR (related to obesity, from literature) P oe − P e × P o
(see eMethods in Supplement 1 , similar formula applied for smoking; P oe = proportion within specific GD category who have obesity; P e = proportion of those with specific GD category among all women with 2 consecutive singleton pregnancies; P o = proportion with obesity among all women with 2 consecutive singleton pregnancies). We performed analyses with SAS version 9.4 (SAS Institute). Data were analyzed from July 2023 to December 2023.
The 431 980 women analyzed ( Figure 1 ) had a mean (SD) age of 30.1 (4.5) years, and a mean (SD) of 2.8 (1.5) years elapsed between deliveries. Overall, 8550 women were African or Caribbean, 17 315 were from Arab-speaking regions, 14 615 were Asian, 373 415 were of European background, 78 770 (18.2%) at the highest material deprivation level (INSPQ deprivation index: quintile 5), 62 605 (14.5%) had 1 or more SGA offspring, 64 195 (14.9%) had 1 or more LGA offspring, 35 290 (8.2%) had 1 or more preterm delivery, and 34 145 (7.9%) had 1 or more gestational hypertension occurrence. In terms of the main exposure, 10 920 (2.5%) had GD in only their first pregnancy, 16 145 (3.7%) had GD in only their second, and 8255 (1.9%) in both (eFigure 2 in Supplement 1 ). Those without GD in either pregnancy (396 660 participants) ( Table 1 ) were younger with higher proportions of European background and lower proportions with deprivation, comorbid conditions, LGA offspring, preterm births, and partners with diabetes and hypertension.
Over a median (IQR) of 11.5 (5.3-19.4) years (5 298 940 total person years), 12 205 mothers developed type 2 diabetes. The IRs per 1000 person-years rose across the no GD (IR, 1.4), GD in first pregnancy only (IR, 6.7), GD in second pregnancy only (IR, 12.4), and GD in both pregnancies (IR, 25.5) categories. Kaplan-Meier curves suggested significant differences in event-free survival across groups ( Figure 2 ). The proportional hazards assumption applied. We did not detect interactions or multicollinearity.
In adjusted models, compared with those without GD, those with GD in first pregnancy had a 4.35-fold higher hazard for type 2 diabetes (95% CI, 4.06-4.67) ( Figure 3 A), those with GD in the second pregnancy had a 7.68-fold increase (95% CI, 7.31-8.07), and those with GD in both pregnancies demonstrated a 15.80-fold increase (95% CI, 15.00-16.61). Compared with those with GD in the first pregnancy, women with GD in the second had 76% higher hazards (95% CI, 1.63-1.91) ( Figure 3 B) and those with GD in both pregnancies had a 3.63-fold increase (95% CI, 3.36-3.93). Hazards were 2.06-fold higher among women with GD in both pregnancies (95% CI, 1.94-2.19) ( Figure 3 C) compared with those with GD in the second pregnancy.
Inclusion of calendar years for each pregnancy did not importantly alter HRs (eTable 2 in Supplement 1 ). In another sensitivity analysis including women with stillbirth pregnancies in our study cohort (435 685 participants; 12 415 events), stillbirths were associated with 19% increased hazards (HR, 1.19; 95% CI, 1.04-1.38), compared with women without stillbirth deliveries (eTable 3 in Supplement 1 ). Miscarriages between pregnancies were not conclusively associated with incident diabetes (HR, 1.03; 95% CI, 0.97-1.09). Furthermore, retaining stillbirths among the 2 pregnancies examined and considering miscarriages between pregnancies did not importantly alter the association between GD occurrences and incident diabetes.
Indirect adjustments for obesity (no GD: reference; GD in first pregnancy HR, 2.72; 95% CI, 2.46-2.83; GD in second pregnancy HR, 5.48; 95% CI, 5.22-5.76; GD in both pregnancies HR, 9.62; 95% CI, 9.15-10.10) (see eMethods in Supplement 1 for other comparisons) somewhat attenuated the HRs for the incident type 2 diabetes outcome. Indirect adjustments for smoking (no GD: reference; GD in first pregnancy HR, 4.23; 95% CI, 3.94-4.53; GD in second pregnancy HR, 7.44; 95% CI, 7.09-7.83; GD in both pregnancies HR, 15.50; 95% CI, 14.70-16.21) did not significantly alter the HRs.
Gestational hypertension in either or both pregnancies was associated with a 65% increase in hazards for diabetes development ( Table 2 ). Compared with appropriate size for gestational age of both offspring, LGA was consistently associated with diabetes development, with a 60% increase in hazards whether it occurred in the first or second pregnancies, and a doubling when it occurred in both pregnancies or when LGA occurred in 1 pregnancy and SGA in the other. Preterm delivery in 1 or both pregnancies was associated with a 10% to 20% increase in hazards of diabetes compared with full-term delivery in both pregnancies. Deprivation levels were associated with a stepwise increase in hazards. All ethnocultural groups other than European had higher diabetes hazards compared with European women, and all comorbid conditions considered were associated with increased hazards. Paternal diabetes was associated with a 43% increase in hazards for maternal diabetes development.
Among nearly half a million mothers with 2 consecutive singleton pregnancies, our analyses demonstrate that GD in only the second pregnancy was associated with higher hazards for type 2 diabetes development than GD in only the first pregnancy. The highest hazards are with GD occurrence in both pregnancies. Compared with women without GD in either pregnancy, there were 4.35-fold, 7.68-fold, and 15.80-fold greater hazards for type 2 diabetes with GD in the first, in the second, and in both pregnancies, respectively. Indirect adjustments for obesity somewhat attenuated these values to 2.72-fold, 5.48-fold, and 9.62-fold, respectively, but the magnitude remained high, and the differences persisted. Direct comparisons between GD groups were also conclusive. For example, compared with first pregnancy–only GD, second pregnancy–only GD increased hazards by 76%, while GD in both pregnancies increased hazards 3.63-fold.
We did not identify any prior study that compared GD in a first pregnancy with GD in a second among women with 2 pregnancies. Our specific estimate for the increase in hazards associated with GD recurrence (HR, 3.63; HR, 2.21 with indirect adjustment for obesity) compared with women with a GD occurrence in only a first pregnancy was similar to the greater than 2-fold increase in hazards reported in 2 previous studies 4 , 5 that restricted analyses to women with at least 2 pregnancies. Other studies that examined GD recurrence had a higher degree of variability in numbers of pregnancies. One reported a 16% increase in hazards with GD recurrence 33 while the other estimated a 2-fold increase. 34 We identified a single study that examined hazards of type 2 diabetes after pregnancy in relationship to numbers of prior GD pregnancies (Sister Study). 35 It differed in several other respects from ours. As such, its interpretation applies to an older group of women, several years beyond pregnancy. In the Sister Study, women with a history of 2 GD pregnancies experienced 6.2-fold higher hazards for type 2 diabetes in middle age compared with women with no GD pregnancies. The reference group included women without pregnancies. The investigators accounted for time since last GD pregnancy and self-reported weight. In our study, women with 2 pregnancies and GD in both had a 15.8-fold increase in hazards for type 2 diabetes development between their 30s and 40s, starting soon after their second pregnancy, compared with women without GD in either pregnancy. We indirectly adjusted for obesity and demonstrated that the association was attenuated to a 10-fold increase in hazards.
Our key discovery is that a single GD occurrence in a first pregnancy is associated with lower hazards for type 2 diabetes than a single GD occurrence in a second pregnancy. A subgroup analysis of the American Diabetes Prevention Program among women with a GD history showed that healthy diet-induced weight loss and higher physical activity levels could reduce type 2 diabetes risk. 6 , 7 Women with a first GD pregnancy may be motivated to adopt behavioral changes that both prevent GD in a second pregnancy and lower hazards of incident type 2 diabetes development thereafter. Supporting this, a recent cohort study 11 determined that among women without GD in their first pregnancy, weight loss between pregnancies was associated with reduced risk for new occurrence of GD in a subsequent pregnancy. In another study 10 among women with excess weight and GD in a first pregnancy, weight loss between pregnancies lowered the risk for GD recurrence in a second pregnancy.
In our analyses, the women without GD in the first pregnancy who developed GD in the second may have gained excess weight in the first pregnancy and had difficulty losing it 9 or gained weight between pregnancies. 10 , 11 In an Australian investigation 11 among women without GD in their first pregnancy, higher levels of weight gain between pregnancies were associated with stepwise increases in the risk of new occurrence of GD in second pregnancy. For many women, the additional responsibilities of motherhood 12 may challenge efforts to engage in behaviors to enhance personal health. Furthermore, the metabolic stresses inherent to pregnancy may impair their β-cell function, 36 making them more susceptible to developing GD in the second pregnancy, and ultimately to type 2 diabetes development.
Alongside health behaviors and physiological changes, our analyses reinforce the importance of social factors, including material and social deprivation and non-European background. The underpinnings of such associations likely stem from other related upstream characteristics, such as food insecurity, 37 local environments not conducive to physical activity, 38 , 39 and structural inequity. 40 Partner diabetes was another risk indicator for maternal type 2 diabetes development in our analyses, with a 43% increase in hazards. This is consistent with our prior studies 41 , 42 demonstrating increases in hazards for the development of diabetes in fathers whose partners had GD compared with those whose partners did not. Shared partner type 2 diabetes risk may be related to shared health behaviors, resources, social factors, and household environments. 20 , 43 - 46 Assortative mating (similar demographics, attitudes, behaviors, and traits at the outset) may also play a role. 46 - 49
Our large sample size of nearly half a million women was possible through linkage of Quebec’s health administrative and vital statistics databases. Limitations to these data include lack of information on GD management, prepartum weight status, gestational weight gain, smoking status, and laboratory values. GD and weight excess are intimately associated. However, all of our models accounted for LGA in both the first and second pregnancies, a strong correlate of prepregnancy and gestational weight gain. 50 , 51 Furthermore, we performed indirect adjustments for obesity using established methods. 28 , 29 We could not corroborate International Statistical Classification of Diseases and Related Health Problems, Ninth and Tenth Revision –coded diagnoses of diabetes with laboratory data, but to mitigate for misclassification, we applied validated definitions of GD and diabetes. 16 , 17 The diagnostic codes do not reliably distinguish between type 1 and type 2 diabetes, but given that 95% of diabetes onset among adults is type 2 diabetes, the majority of events captured with our codes are type 2 diabetes. We acknowledge that women with more than 1 GD occurrence may already be undergoing more frequent screening for diabetes than women with only 1 occurrence, perhaps partly accounting for their higher diabetes hazards. We also acknowledge potential misclassification of ethnocultural background, as second- and third-generation women and/or Indigenous women would have been classified as European if their first language was English, French, or another European language. Lastly, we did not examine women without pregnancies or women with a single pregnancy, but our focus was women with 2 consecutive deliveries; restriction to women with 2 or more deliveries overcame some methodological challenges, as discussed.
Our retrospective cohort study suggests that among women with 2 consecutive singleton pregnancies, without diabetes before or between pregnancies, the absence of GD in a second pregnancy following GD in the first suggests that the mother is taking effective diabetes prevention measures. If confirmed, she should be encouraged to continue. New onset GD or recurrent GD in a second pregnancy, however, should inspire urgent action for prevention or adjustments to ongoing efforts. We also confirmed the importance of material deprivation and ethnocultural background in type 2 diabetes risk estimation, and we identified paternal diabetes as a factor associated with risk for type 2 diabetes development in mothers. Our results provide a personalized medicine–oriented pathway to diabetes risk estimation in women. This should be coupled with tailored prevention programs and equitable referral pathways to reduce the burden of type 2 diabetes and its complications.
Accepted for Publication: March 7, 2024.
Published: May 9, 2024. doi:10.1001/jamanetworkopen.2024.10279
Open Access: This is an open access article distributed under the terms of the CC-BY License . © 2024 Mussa J et al. JAMA Network Open .
Corresponding Author: Kaberi Dasgupta, MD, Centre for Outcomes Research and Evaluation of the RI-MUHC, 5252 boul de Maisonneuve Ouest, Office 3E.09, Montréal, QC H4A 3S5, Canada ( [email protected] ).
Author Contributions: Dr Dasgupta had full access to all of the data in the study and takes responsibility for the integrity of the data and the accuracy of the data analysis.
Concept and design: Mussa, Rahme, Dasgupta.
Acquisition, analysis, or interpretation of data: All authors.
Drafting of the manuscript: Mussa, Dasgupta.
Critical review of the manuscript for important intellectual content: All authors.
Statistical analysis: Mussa, Rahme, Dahhou.
Obtained funding: Dasgupta.
Administrative, technical, or material support: Mussa.
Supervision: Rahme, Dasgupta.
Conflict of Interest Disclosures: None reported.
Funding/Support: The Heart and Stroke Foundation of Canada funded this study (grant No. HSF G-12-000251 to Dr Dasgupta). The Société Québécoise d’Hypertension Artérielle and McGill’s Faculty of Medicine provided additional support through doctoral studentships for Mr Mussa.
Role of the Funder/Sponsor: The funders had no role in the design and conduct of the study; collection, management, analysis, and interpretation of the data; preparation, review, or approval of the manuscript; and decision to submit the manuscript for publication.
Data Sharing Statement: See Supplement 2 .
Additional Contributions: The authors thank the Quebec Statistical Institute (Institut de la statistique du Québec) for data linkage and hosting us at their secured Centers for Access to Research Data.
- Register for email alerts with links to free full-text articles
- Access PDFs of free articles
- Manage your interests
- Save searches and receive search alerts
Thank you for visiting nature.com. You are using a browser version with limited support for CSS. To obtain the best experience, we recommend you use a more up to date browser (or turn off compatibility mode in Internet Explorer). In the meantime, to ensure continued support, we are displaying the site without styles and JavaScript.
- View all journals
- My Account Login
- Explore content
- About the journal
- Publish with us
- Sign up for alerts
- Review Article
- Open access
- Published: 21 May 2024
Association of gastrointestinal microbiome and obesity with gestational diabetes mellitus-an updated globally based review of the high-quality literatures
- Jiahui Li 1 ,
- Min Wang 1 ,
- Shuai Ma 1 ,
- Zhong Jin 1 ,
- Haonan Yin 1 &
- Shuli Yang ORCID: orcid.org/0009-0002-7297-5424 1
Nutrition & Diabetes volume 14 , Article number: 31 ( 2024 ) Cite this article
248 Accesses
1 Altmetric
Metrics details
- Gestational diabetes
The purpose of this review is to investigate the relationship between gastrointestinal microbiome, obesity, and gestational diabetes mellitus (GDM) in an objective manner.
We conducted a thorough and comprehensive search of the English language literatures published in PubMed, Web of Science, and the Cochrane Library from the establishment of the library until 12 December 2023. Our search strategy included both keywords and free words searches, and we strictly applied inclusion and exclusion criteria. Meta-analyses and systematic reviews were prepared.
Six high-quality literature sources were identified for meta-analysis. However, after detailed study and analysis, a certain degree of heterogeneity was found, and the credibility of the combined analysis results was limited. Therefore, descriptive analyses were conducted. The dysbiosis of intestinal microbiome, specifically the ratio of Firmicutes/Bacteroides, is a significant factor in the development of metabolic diseases such as obesity and gestational diabetes. Patients with intestinal dysbiosis and obesity are at a higher risk of developing GDM.
Conclusions
During pregnancy, gastrointestinal microbiome disorders and obesity may contribute to the development of GDM, with all three factors influencing each other. This finding could aid in the diagnosis and management of patients with GDM through further research on their gastrointestinal microbiome.
Similar content being viewed by others
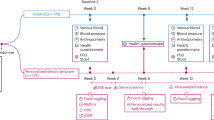
Effects of a personalized nutrition program on cardiometabolic health: a randomized controlled trial
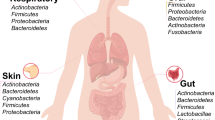
Microbiota in health and diseases
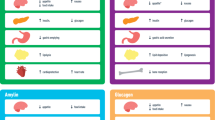
What is the pipeline for future medications for obesity?
Introduction.
Trillions of microbial cells essential to human health are present in the human body. The gastrointestinal microbiome, located on the surface of the intestinal mucosa, is the largest microbial ecosystem in the human body and is involved in epithelial homeostasis, energy harvesting and immune development [ 1 , 2 ], and has a major impact on nutrient and energy metabolism, with significant implications for human health [ 3 ]. Based on their relationship to host health, the gastrointestinal microbiome can be categorized into beneficial, harmful and conditionally pathogenic bacteria [ 4 ]. There is no single optimal gastrointestinal microbiome composition, as it varies from individual to individual, and it is important to maintain a healthy balance between the host and the pathogens in order to achieve optimal metabolic and immune functions [ 5 ]. Changes or ecological disturbances in the normal composition of the gastrointestinal microbiome are defined as an imbalance between commensal and pathogenic bacteria [ 6 ], and the gastrointestinal microbiome can regulate each other to maintain the abundance and diversity of the microbiome in a dynamic equilibrium [ 4 ]. Due to changes in the body’s endocrine status, immune function, age profile, dietary structure, environmental and genetic factors, the gastrointestinal microbiome can become dysbiotic and show changes in abundance and diversity, this can lead to the development of metabolic disorders such as obesity and diabetes [ 6 ].
Overweight and obesity are significant global health issues and are significant risk factors for GDM [ 7 ]. Overweight and obesity have a strong association with adverse pregnancy outcomes. An increase in pre-pregnancy body mass index (BMI) significantly increases the risk of fetal miscarriage, neonatal hypoglycemia, fetal malformations, large for gestational age, macrosomia, and cesarean section [ 8 , 9 ]. Obesity reduces the number of insulin receptors and causes receptor defects, leading to insulin resistance (IR) and higher fasting insulin levels. This affects glucose transport, utilization, and protein synthesis. Women are particularly susceptible to GDM due to excessive nutritional intake during pregnancy.
GDM is one of the most common complications of pregnancy and is defined as the first occurrence of diabetes during pregnancy due to abnormal glucose metabolism [ 7 , 10 ]. The International Diabetes Federation estimates that the global prevalence of diabetes will increase to 10.2 percent (578 million people) by 2030 and to 10.9 percent (700 million people) by 2045 [ 11 ]. In normal pregnancy, especially towards the third trimester of pregnancy(T3), beta(β) cells secrete more insulin to compensate and maintain normal blood glucose levels due to IR. However, in GDM, certain changes lead to a reduction in insulin sensitivity, impairment of insulin secretion and the development of carbohydrate intolerance [ 12 ]. The molecular mechanisms by which these changes take place are still not clear.
In recent years, research into the relationship among the gastrointestinal microbiome and obesity and GDM has become a major topic in medicine, but the results of these studies on the upregulation or downregulation of the gastrointestinal microbiome are not consistent because the subjects were from different countries and races, and the tests used were different. Due to the high degree of heterogeneity of the cohort studies in these areas, it was not possible to perform combined analyses. Therefore, this review aims to provide a comprehensive overview of these three areas of research, to further our understanding of GDM, to identify new targets for treatment of GDM, and ultimately to provide guidelines for clinicians.
Composition and characteristics of the gastrointestinal microbiome during pregnancy
The microbiome, which is the collection of all gastrointestinal microbial genes in an individual, is an order of magnitude larger than the human genome [ 13 , 14 ]. The adult intestinal is colonized by at least 1800 genera and approximately 15–36,000 species of bacteria [ 15 ]. The human gastrointestinal microbiome consists of four main groups: Firmicutes, Bacteroides, Actinomycetes, and Proteus [ 16 ]. The ratio of Firmicutes to Bacteroides(F/B) is a crucial parameter that reflects dysbiosis of the gastrointestinal microbiome [ 17 ]. According to molecular analyses targeting 16S rRNA, the fecal samples of healthy human volunteers contained bacteria primarily from two phyla: Firmicutes and Bacteroidetes. These bacteria were predominantly anaerobic species [ 18 ]. Compositionally, the gastrointestinal microbiome of the entire pregnant population is also dominated by members of Firmicutes and Bacteroidetes, which account for approximately 90% of the microbial environment in the intestine [ 5 , 19 ]. Currently, there is a great deal of variation in the study of gastrointestinal microbiome in various countries, possibly due to factors such as study populations from different races, different ages, the use of different test methods, contamination of study reagents, and statistical differences.
The role of bile acids
It is worth noting that bile acids (BA), which are a type of amphiphilic steroid produced in the liver and modified by the microbiome, are increasingly recognized as contributing to the pathogenesis and progression of metabolic diseases such as obesity and diabetes. BAs promote the absorption of intestinal fat. They also have hormone-like functions by activating nuclear and membrane-bound receptors, which regulate glucose, lipid and energy metabolism, intestinal integrity and immunity [ 20 ]. The BA biotransformation process is shown in Fig. 1 [ 21 ].
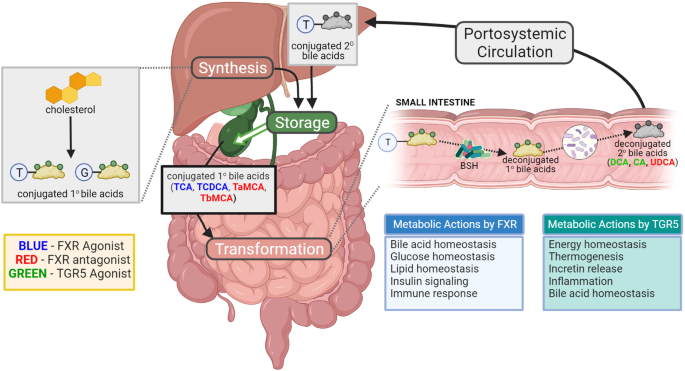
Cholesterol is converted to primary BAs in the liver. Primary BAs are conjugated with primarily taurine in mice or glycine in humans before being transported to the gallbladder for storage in the form of bile. On ingestion of dietary fats, primary conjugated BAs (within bile) are released into the gut lumen to aid lipid absorption. Bacteria with BSH deconjugate BAs, thereby weakening their soap-like qualities. This allows other microbiome members to further modify them into secondary BAs. Some secondary BAs can be transported back to the liver, where they are then conjugated. The interaction between the gut microbiome and BAs leads to modulation of FXR and TGR5 agonists and antagonists, and thus, allows the gut microbiome to affect host metabolism (Note: T taurine, G glycine. In humans: TCA taurocholic acid, TCDCA taurochenodeoxycholic acid. In mice: TαMCA tauro-α-uricholic acid, FXR farnesoid X receptor, TGR5 G protein-coupled BA receptor 1).
Glucose homeostasis is a crucial physiological process affected by BAs. BA signaling pathways, such as the nuclear hormone receptor FXR [ 22 ] and TGR5 [ 23 ], are highly conserved metabolic regulatory pathways between mouse models and humans, and they affect IR. Additionally, BA regulates dozens of genes involved in metabolic homeostasis through the activation of fibroblast growth factor 19 in humans [ 24 ]. Studies in mice have shown that FXR regulates the release of glucagon-like peptide-1 (GLP-1) [ 25 ], This peptide promotes gluconeogenesis [ 26 ] and browning of white adipose tissue in the liver and muscle [ 27 ]. The influence of diet on BA signaling is also evident. Studies on FXR in various animal models of metabolic disorders have yielded inconsistent results. However, several studies have demonstrated that mice lacking FXR on a regular diet develop hyperglycemia and hypercholesterolemia [ 28 ]. Additionally, feeding FXR-deficient mice a high-fat diet prevented obesity and improved glucose homeostasis [ 29 , 30 ]. TGR5 is expressed in various organs including the gallbladder, lung, spleen, liver, bone marrow, and placenta [ 28 ]. It is also present in intestinal L cells, immune cells such as Kupffer cells, muscle, and brown adipose tissue [ 21 ]. TGR5 activation in brown adipose tissue promotes calorie production from stored fat. In L cells, TGR5 activation affects glucose homeostasis primarily through the secretion of GLP-1 [ 21 ].
Circadian rhythms also affect human glucose metabolism, with a 34% decrease in insulin sensitivity at night compared to the morning [ 31 ]. Disturbances in circadian rhythms are considered a significant factor in metabolic disorders. The roles of intestinal microbiome and BA metabolism have received significant attention. A recent study highlights the importance of maintaining proper oscillations of Ursodeoxycholic acid in a lean state to synchronize insulin sensitivity oscillations [ 32 ]. However, the function of the gut microbiota-bile acid axis in regulating circadian rhythms of metabolic homeostasis remains largely unknown and requires further exploration in the future.
The relationship among gastrointestinal microbiome, obesity and GDM
During pregnancy, around 20% of patients develop pre-diabetes or type 2 diabetes Mellitus [ 33 ]. Hormone levels undergo various changes during pregnancy, particularly with a significant increase in luteinizing hormone and estrogen levels, which produce many physiological effects. These hormone levels may affect the composition of the microbiome [ 34 ]. In addition, changes in modern lifestyles and the use of antimicrobial drugs have led to a decrease in the diversity of the gastrointestinal microbiome in many populations in developed countries [ 35 ]. Dysbiosis of the gastrointestinal microbiome can have several adverse effects on the organism, such as imbalances between Firmicutes and Bacteroidetes, which can lead to obesity and diabetes [ 36 ].
This review follows the Preferred Reporting Items for Systematic Reviews and Meta-Analyses protocol. We conducted a comprehensive search for both published and unpublished literature in English up to 12 December 2023, using databases such as PubMed, Web of Science, and the Cochrane Library. In order to gather the literature, we established rigorous inclusion and exclusion criteria and employed a search strategy that combined keywords with free words or synonyms.
Inclusion criteria
The study compared women during pregnancy with GDM (experimental group) to those with normal glucose tolerance (control group), while also examining differences between women with normal weight and those who were overweight or obese.
Fresh feces were collected from the subjects in the early morning, and fasting venous blood was drawn to analyze gastrointestinal microbiome and measure BMI.
The study included both experimental and control groups to investigate indicators of gastrointestinal microbiome, levels of obesity, and blood glucose levels.
Make sure that experimental and control groups were comparable.
Use randomized controlled trials, case-control studies, or cohort studies.
The literatures included in this study were written in English.
Exclusion criteria
Studies on women with multifetal pregnancies or less than 10 cases.
Studies for which the full text was not available or from which no valid data could be extracted.
This section excludes studies in the form of case studies, reviews, and lectures.
Considering that this is the first systematic review so far to study the association of gastrointestinal microbiome and obesity with GDM, this direction was registered with PROSPERO (registration number: CRD42023486272). After conducted a thorough literature search and analysis (see Table 1 for the search process), a total of 6 relevant documents were identified for this study (see Table 2 for the basic characteristics of the included documents), and there was less comparability and more heterogeneity between the groups, which made it impossible to perform a combined analysis. Considering the above, a descriptive analysis of these few high-quality documents is presented below.
It has been found that most studies on the involvement of gastrointestinal microbiome in metabolic diseases rely on the use of 16S rRNA marker genes [ 37 ]. The 16S rRNA genes of bacteria are made up of conserved and variable parts, the latter being specific to a given strain. Sequences from the V3 to V4 parts of the 16S rRNA gene are generally accepted as representative of all 16S rRNA sequences, and the analysis of sequences from the V3 to V4 parts of the 16S rRNA gene is used to determine the taxonomy of each bacterial species [ 38 ]. Only one of the above six papers [ 39 ] sequenced the V6–V8 variable region of 16S rRNA, but ultimately it was the 16S rRNA that was investigated as a means to speculate on its interaction with host metabolism.
Two studies have suggested a correlation between the composition of gastrointestinal microbiome and both GDM and weight status [ 36 , 40 ]. Based on different periods of pregnancy, Zhi-ying Song [ 4 ] concluded that significant differences in the species composition of the gastrointestinal microbiome existed between the purely overweight group and the group of normal women in T3. In a study of a group of people who were both obese or overweight, Marketa [ 41 ] concluded that significant differences in the gastrointestinal bacterial microbiota of different GDM pathogenesis groups existed in the first trimester of pregnancy. The two remaining studies [ 39 , 42 ] comparing the composition of gastrointestinal microbiome between GDM and women in pregnancy without GDM (non-GDM) groups found no significant differences. In their analysis of the intestinal microbiome and weight, Yao Su and colleagues [ 36 ] discovered that the ratio of F/B in the ultra-restructured gastrointestinal microbiome of GDM was approximately 3:5, which is the opposite of other groups. Zhi-ying Song [ 4 ] found a negative correlation between pre-pregnancy BMI and Lactobacillus, as well as between weight gain during pregnancy and Desulfovibrio in Proteobacteria. (Table 3 presents the specific differences in the indicators of gastrointestinal microbiome between the groups.)
The diagnosis of GDM is primarily based on a glucose tolerance test. This involves measuring fasting glucose levels, as well as glucose levels at 1 h (OGTT_1h) and 2 h (OGTT_2h) after consuming a glucose solution. (Criteria: At least one of the three blood glucose values must be equal to or greater than 5.1 mmol/L, 10.0 mmol/L, and 8.5 mmol/L, respectively.) The test is typically performed between 24 and 28 weeks of gestation and follows the guidelines developed by the International Association of Diabetes and Pregnancy Research Groups [ 43 ]. The correlation analyses conducted by Zhi-ying Song and others [ 4 ] found that the composition and abundance of gastrointestinal microbiome have an impact on GDM. Specifically, blood glucose values at OGTT_1 h and OGTT_2 h were positively correlated with Bacteroides, and negatively correlated with Prevotella. It is important to note that these findings are based on objective data and do not include any subjective evaluations. The study In Patricia [ 42 ] found a positive correlation between the abundance of Christensenellaceae and Enterobacteriaceae with plasma glucose levels one hour after OGTT. Conversely, Enterococci were negatively correlated with plasma glucose levels two hours after OGTT. These findings suggest potential implications for the diagnosis and treatment of GDM.
A cohort study by Dualib et al. [ 42 ] concluded that in relation to the development of GDM, alpha(α) and β diversity did not differ between the GDM and Non-GDM groups, despite differences in the relative abundance of specific bacteria. In our study, Thomas et al. [ 39 ] found that no significant changes in the relative abundance of major bacterial taxa were detected between women in health and women with GDM at 28 weeks’ gestation, and that the occurrence of GDM was associated with a decrease in Shannon diversity ( p = 0.02) but no different clustering as measured by β-diversity, and that at 28 weeks’ gestation the women with GDM had a decreased microbial richness and evenness.
In Patricia’s study [ 42 ], the abundance of bifidobacteria and peptidococci increased in the third trimester of pregnancy, but there was no difference in α-diversity or overall microbiota structure between the two groups. These results suggested a degree of inconsistency, which could be due to differences in the subject populations, experimental error, or the comparability of the groups. Given that Patricia [ 42 ] included women who were overweight or obese regardless of when GDM was diagnosed by abnormal blood glucose, it is possible that the difference in BMI contributed to the increased gastrointestinal microbiome abundance, but this is only a preliminary hypothesis and further studies with larger sample sizes are needed to support this conclusion.
Research has indicated that individuals with a low abundance of gastrointestinal microbiome are more susceptible to dyslipidemia [ 44 ]. In this study, Thomas and colleagues [ 39 ] found that the GDM group had elevated levels of VLDL, triglycerides, venous glucose, HOMA-IR, and C-peptide at 28 weeks of gestation when compared to the control group. In a randomized controlled trial, Marketa [ 41 ] also found that Escherichia/Shigella had a positive correlation with plasma lipid levels, while Subdoligranulum had a negative correlation with plasma lipid levels in the GDM group. Coprococcus, Akkermansia, Methanobrevibacter, Phascolarctobacterium, and Alistipes were found to have a positive correlation with acetate, valerate, 2-hydroxybutyrate, and 2-methylbutyrate levels, respectively. In summary, these lipid metabolism abnormalities contribute to the development of obesity and GDM, but the specific indicators may vary among individuals.
Short-chain fatty acids (SCFAs) play a crucial role in glucose homeostasis by providing additional energy from undigested food. Butyrate, acetate, and propionate are the three main SCFAs produced by gastrointestinal microbes during the fermentation of nondigestible dietary fiber in the large intestine. SCFAs are most concentrated in the cecum and proximal colon, with concentrations decreasing towards the distal colon [ 21 ]. The type of SCFAs produced and the diet determine the metabolic pathways triggered through various receptors. SCFAs influence the regulation of host lipid and glucose metabolism through G protein-coupled receptors (GPCRs) linkages, such as GPR41 and GPR43 [ 45 ], as shown in Fig. 2 . Disturbances in gastrointestinal microbiome can lead to a reduced intestinal anti-inflammatory response. Low levels of SCFAs can also reduce the activation of GPCRs, leading to reduced activation of GPR41 and GPR43, which can generate intestinal inflammation, insulin resistance, and ultimately, diabetes [ 46 ]. Studies have shown that GPR43-deficient mice become obese even on a normal diet, while mice that specifically overexpress this receptor in adipose tissue remain lean [ 47 ]. Additionally, GPR43 activation promotes the secretion of GLP-1 in the intestine, enhancing insulin sensitivity [ 48 ]. SCFAs deficiency can cause a loss of tight junctions and increased enterocyte permeability. This can lead to increased absorption of bacterial endotoxins, such as lipopolysaccharide, which in turn can cause the production of pro-inflammatory cytokines. These factors can predispose women to IR and GDM [ 49 ].
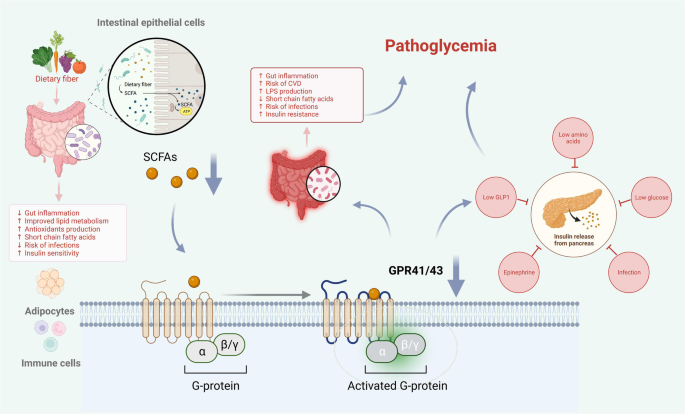
Lipid molecules regulate host metabolism through GPR41 and GPR43 receptor linkage.
Normal gastrointestinal microbiome has a positive impact on host metabolism. SCFAs activate GPCRs like GPR41 and GPR43. These receptors are expressed in various cell types, including intestinal epithelial cells, adipocytes, and immune cells [ 33 ]. Disruptions in the gastrointestinal microbiota can weaken the intestinal anti-inflammatory response. Low levels of SCFAs can also decrease GPCR activation, potentially resulting in intestinal inflammation, IR, and ultimately GDM.
Relationship between intestinal microbiome and GDM
Microbial abundance in women with GDM compared to non-GDM is either reduced [ 50 , 51 , 52 ], unchanged, or elevated [ 19 ]. However, there is currently no specific microbiota that can predict the development of GDM. Generally, pregnancy leads to increased bacterial loads and significant alterations in the composition of the intestinal microbiome [ 53 ]. The composition of intestinal microbiome during the first trimester of pregnancy(T1) is similar to that of women in health and nonpregnancy [ 53 ]. Microbiome disorders are highly characteristic in patients with GDM, particularly in mid-pregnancy(T2), and can be used as a predictor of GDM [ 54 ]. Significant alterations in the composition of the intestinal microbiome were observed in women during pregnancy compared to non-pregnant women, and from T1 to T3 [ 55 , 56 ]. The late pregnancy intestinal microbiome has been found to cause weight gain, insulin resistance, and a greater inflammatory response when transferred to germ-free mice compared to the early pregnancy microbiota [ 55 ]. In T3, Koren et al. found an increase in the abundance of the Actinobacteria and Aspergillus phyla, and a decrease in the Faecalibacterium [ 55 ]. Ferrocino et al. [ 54 ] discovered that individuals with GDM had an increased abundance of Blautia, Butyricicoccus, and Clostridium, as well as a decreased abundance of Bacteroides, Collinsella, and Rikenellaceae during T2 compared to T3 [ 19 ].
However, the gastrointestinal microbiome of patients with GDM may be abnormal at several levels, including the phylum and genus levels. According to a study [ 19 ], the GDM cohort had a higher abundance of Actinobacteria at the phylum level and Collinsella, Roseburia and Desulfovibrio at the genus level. According to Koren [ 55 ], significant changes are identified by a decrease in individual richness (α-diversity), an increase in intersubject diversity (β-diversity), and altered abundance of certain species.
An operational taxonomic unit (OTU) is a set of uniform markers used to represent taxonomic units, such as phylum, order, family, genus, and species. OTUs are created for the purpose of facilitating analysis in phylogenetic or population genetics studies [ 26 , 57 ]. In a comparative study of intestinal microbiome differences between healthy pregnant women and GDM patients, two studies analyzed 18 [ 58 ], and 17 [ 19 ] bacterial OUTs, respectively. The major differences in dysbiosis OUTs were attributed to the Firmicutes (72.2% and 88.2%). This suggests that alterations in the Firmicutes are a characteristic hallmark of GDM. Therefore, strains specific to the Firmicutes require urgent exploration in the future.
In a comparative study between women during pregnany in a normal state and those with diabetes, Xing et al. found that GDM subjects had a higher abundance of Lachnospiraceae family OTUs and a lower abundance of Enterobacteriaceae and Rumatococcaceae. Two Lachnospiraceae OTUs (247 and 672) were positively correlated with OGTT_1h at 24–28 weeks of gestation, and bacterial OTUs (e.g., Enterobacteriaceae_OTU 123 and Rumatococcaceae_OTU 93) were associated with FBG levels at 12 weeks of gestation [ 58 ]. Intestinal Lachnospiraceae bacteria have been suggested to be positively associated with type 2 diabetes Mellitus [ 59 ]. A study conducted in China found that, at the species level, the relative abundance of Clostridium_spiroforme, Eubacterium_dolichum, and Ruminococcus_gnavus was positively correlated with FBG, while Pyramidobacter_piscolens was negatively correlated with FBG [ 57 ]. A previous animal study also reported a positive correlation between Lachnospiraceae OTUs and blood glucose levels [ 60 ].
Ruminococcus gnavus is an anaerobic bacterium that is Gram-positive. It belongs to the Firmicutes, it is also a member of the family Lachnospiraceae. An increasing number of enteric and extra-enteric diseases are associated with this bacterium [ 61 ]. Zhi-ying Song [ 4 ] and Yao Su [ 36 ] found that g-Ruminococcus was a characteristic biomarker for the normal pregnant women group and Ruminococcaceae_UCG014 was a characteristic biomarker for the GDM-only group, respectively. The study suggests that Ruminococcaceae play a role in energy metabolism, insulin signaling, and inflammatory processes. It also found that an increase in the relative abundance of Ruminococcaceae is associated with higher FBG concentrations and TR, which increases the risk of developing GDM [ 62 ]. These findings indicate the potential predictive value of specific microbial combinations for GDM.
There is no predictive value of blood glucose values for the development of severe GDM. Furthermore, differences in the severity of GDM correspond to changes in gastrointestinal microbiome [ 63 ]. In a prospective longitudinal study conducted in Chiang Mai, Thailand, there were no differences in FBG, OGTT_2h, and glycated hemoglobin levels at diagnosis between patients with diet-controlled GDM and those requiring insulin therapy [ 63 ]. However, unlike the literature included in this study, GDM patients who ultimately required insulin therapy had higher levels of Clostridium difficile [ 63 ]. Lactobacillus has long been considered beneficial to the host by attenuating intestinal mucosal barrier dysfunction, remodeling intestinal microbiota composition, and reducing systemic inflammation [ 64 , 65 , 66 ]. However, there are conflicting accounts of its effects on GDM patients.
This study concludes that g_Lactobacillus may be a characteristic gastrointestinal microbiome of overweight GDM patients, distinguishing them from other patient groups [ 4 ]. A cross-sectional study found that GDM patients had lower levels of Lactobacillus casei than non-GDM controls before delivery [ 67 ]. Another study showed that the relative abundance of specific Lactobacillus at diagnosis was higher in women with GDM than non-GDM [ 68 ]. The varying results regarding different bacilli and blood glucose levels can be attributed to different subgroups of bacilli sequences. Therefore, it is important to explore the microbiome further in order to gain a better understanding of the role of various bacilli in female patients with GDM.
The relationship between gastrointestinal microbiome and obesity
Animal and human studies have shown that obesity is associated with an imbalance or ecological dysbiosis of the intestinal microbiome [ 69 ], as the imbalance between energy consumption and depletion favors the prevalence of disease-causing bacteria [ 70 ], but the role of the intestinal microbiome in the development of this disease and whether there is a causal relationship remains controversial [ 37 ]. The gastrointestinal microbiome plays a crucial role in the absorption of nutrients, in digestion and metabolic activities, as well as in the efficiency and storage of energy [ 71 ]. However, changes in the composition of various factors caused by the microbiota (ecological dysbiosis) may have adverse long-term effects, leading to diseases such as obesity, intestinal inflammation, diabetes and metabolic syndrome in the host organism and in future generations [ 72 ]. The effect of the intestinal microbiota on host metabolism was first demonstrated in a 2004 study of germ-free mice, which found that conventionally raised mice had more total body fat than those raised in germ-free conditions [ 73 ]. Studies evaluating the increased ratio of F/B following microbiota transplantation in obese individuals have not yet produced consistent results [ 74 , 75 ].
The maternal intestinal microbiota composition changes during pregnancy and breastfeeding due to maternal metabolism adjustments caused by the increased demands of the developing fetus and postnatal infant, as well as the mother’s own physiological changes [ 76 ]. Dysbiosis of the intestinal microbiome is prevalent in obesity and is characterized by a reduction in the diversity [ 44 ] and abundance of the intestinal microbiome in obese individuals [ 77 ]. Studies have shown that individuals with low intestinal microbiome abundance are more susceptible to obesity, IR [ 78 ], and dyslipidaemia [ 44 ]. In patients with obesity, the levels of mucinophilic Akkermansia muciniphila, Faecalibacterium prausnitzii, and Bacteroides were found to be decreased [ 79 , 80 , 81 ], while the abundance of fungal phyla was significantly increased [ 82 , 83 , 84 ].
A follow-up study was conducted in Finland with 256 women. The study found that overweight and obese mothers had a higher relative abundance of the Firmicutes, and there was a trend towards a higher ratio of F/B [ 85 ]. Additionally, the ratio of F/B decreased after weight loss in obese individuals [ 83 ]. It has been suggested that the higher relative abundance of Prevotella detected in women during pregnancy with obesity, compared to those who are overweight, may contribute to glucose metabolism through the metabolites produced [ 86 ].
Akkermansia is a Gram-negative, anaerobic, elliptical bacterium that degrades mucin and inhabits the outer mucus layer of the intestinal barrier [ 37 , 87 ]. The mechanisms by which mucin regulates obesity and glucose levels have not been fully elucidated. In humans, its abundance and genetic richness are positively correlated with healthy metabolic states, including better body fat distribution and absence of metabolic syndrome [ 88 , 89 ]. A previous study demonstrated that Akkermansia enhances thermogenesis and GLP-1 secretion, while reducing the expression of proteins involved in adipocyte differentiation. Additionally, it decreases the gene expression of glucose and fructose transporter proteins in the jejunum, indicating a reduction in carbohydrate absorption [ 90 , 91 , 92 ]. In conclusion, while most studies suggest a beneficial role for Akkermansia in metabolic profiling, its effects may be dual depending on dietary patterns [ 37 ]. Moran noted that consistent observations in the human intestinal microbiota and its interactions with diet and genetics suggest that the microbial diversity of individuals with a high BMI or obese individuals is lower. According to the study, Christensenellaceae, Oscillospira, and Rikenellaceae were more prevalent in individuals with a normal body weight, while Bifidobacteria and Akkermansia were less abundant in those with altered metabolism [ 37 ].
Relationship between obesity and GDM
Maternal adiposity increases significantly during pregnancy due to the increased nutritional needs of the fetus and the demands of the mother’s own metabolism. This can lead to GDM due to the development of IR. Obesity can also affect GDM through other mechanisms, including impaired β-cell function and chronic low-grade inflammation [ 93 , 94 ]. This inflammation is mainly manifested as dyslipidemia and a pro-inflammatory state during pregnancy [ 95 , 96 ]. Prospective studies have linked a range of fatty acids, phospholipids, lipoproteins, certain glycolipids, and cholesterol with incident GDM [ 97 ]. In a recent study of 1008 women’s lipidomic, it was observed that seven out of ten lipids associated with BMI (four LPCs, two TGs, and one SM) were linked to the risk of GDM, even after adjusting for maternal BMI [ 98 ]. Additionally, gestational weight gain has been experimentally confirmed to be associated with an increased risk of developing GDM [ 99 ]. A Danish study found that 11 OTUs were associated with gestational weight gain, with the majority being Clostridiales (7 of 11 OTUs), when diabetic status was not taken into account. Lower weight gain was associated with 7 OTUs, including a Christensenella OTU (OTU_63) and an Alistipes OTU (OTU_128). Weight gain was associated with 4 OTUs, including an Eisenbergiella OTU (OTU_258) and a Lactobacillus OTU (OTU_80) [ 19 ].
Stored upper body fat in pregnant women with obesity can increase the concentration of free fatty acids and lipotoxicity. This can lead to inflammation, endothelial dysfunction, and reduced trophoblastic invasion, ultimately decreasing placental metabolism and function [ 100 ]. The placenta serves as the sole interface between the mother and the fetus, making it a crucial organ for the exchange of gases and nutrients between the two. Specific changes occur in the structure of the placenta in pregnant women who are obese and diabetic, including increased weight, angiogenesis, and slower chorionic villus maturation. These structural abnormalities lead to functional abnormalities, which worsen metabolic abnormalities during pregnancy [ 101 ]. Abnormal protein expression in the placenta can cause insulin antagonism, resulting in abnormal insulin resistance and glucose metabolism [ 102 ]. Five proteins, namely very low density lipoprotein receptor, aquaporin-1, platelet factor 4, peptidyl prolyl isomerase, and malonyl cofactor-acyl carrier protein transacylase, have been associated with altered placental function, placental vascular dysfunction, and placental inflammation and its complications in patients with GDM [ 103 , 104 , 105 ]. Reduced very low density lipoprotein receptor levels may promote GDM by inhibiting the placenta’s ability to remove cholesterol [ 103 ]. Maternal IR is a pathophysiological condition that causes changes in the growth and efficiency of the placenta in pregnant women, especially those who are obese and diabetic [ 106 ]. IR may enhance chorionic cell proliferation and increase placental size, but expansion of immature chorionic villi may reduce the efficiency of placental transport mechanisms, leading to placental insufficiency [ 107 , 108 ]. Furthermore, during pregnancy, the placenta secretes pregnancy-specific hormones such as human chorionic gonadotropin, human placental lactogen, and human placental growth hormone, as well as increased levels of prolactin, estradiol, and cortisol into the maternal circulation. These hormones can affect glucose metabolism and lead to the development of diabetes. It is important to note that this is a complex process and further research is needed to fully understand the mechanisms involved. The rapid recovery of glucose homeostasis immediately after placenta expulsion at delivery demonstrates the significant role of the placenta in GDM with obese patients.
Recent data suggest that exosomes, which are membrane-derived nanovesicles, may play a role throughout pregnancy. This includes mediating placental responses to hyperglycemia and insulin sensitivity. Patients with GDM have been found to have higher levels of circulating exosomes, both overall and of placental origin, during gestation compared to normal pregnancies [ 109 ]. Additionally, hyperglycemia has been shown to increase the release of exosomes from trophoblast cells in early pregnancy [ 110 ], indicating a correlation between maternal metabolic status during pregnancy and circulating levels of placental exosomes.
It has also been suggested that hyperactivation of adipose tissue plays an important role in the pathogenesis of GDM. Lipocalin is a protein produced in large quantities by adipose tissue. It enhances insulin sensitivity, exerts anti-inflammatory effects, and reduces plasma glucose levels [ 111 ]. Lipocalin is thought to play a pivotal role in the regulation of systemic glucose homeostasis [ 112 ]. Lipocalins are expressed and synthesized primarily in maternal adipose tissue, but not via the placenta, and do not enter the fetal circulation [ 113 ]. Deletion of the lipocalin gene leads to impaired insulin tolerance [ 114 ]. Previous studies have shown that low levels of lipocalin in pregnant women are associated with reduced maternal insulin sensitivity during pregnancy [ 115 ]. These findings suggest that lipocalin may play a role in insulin sensitivity during pregnancy. In studies conducted in various populations, including South India [ 116 ] and Iran [ 117 ] maternal serum lipocalin levels were significantly lower in patients with GDM. The use of lipocalin as a prognostic biomarker for GDM risk is currently a topic of debate due to inconsistent results.
This review presents an overview of the gastrointestinal microbiota and its connection to obesity and diabetes (refer to Fig. 3 ), with a causal relationship between these three metabolic conditions. Differences between countries, regions, and ethnicities were analyzed. Currently, no specific combination of gastrointestinal microbiota has an absolute advantage to the host. However, there is some predictive significance for the degree of obesity and the severity of GDM based on the up- or down-regulation, expression or non-expression, and changes in abundance and diversity of different gastrointestinal microbiomes. The placenta plays a pivotal role during this particular period of pregnancy. Additionally, research on BA and intestinal flora mechanisms is a current topic in the field of metabolic diseases. The detection of gastrointestinal microbiome is becoming increasingly important. It can be used as a clinical indicator to assist in the diagnosis of obstetrics and gynecology, especially in patients with GDM.
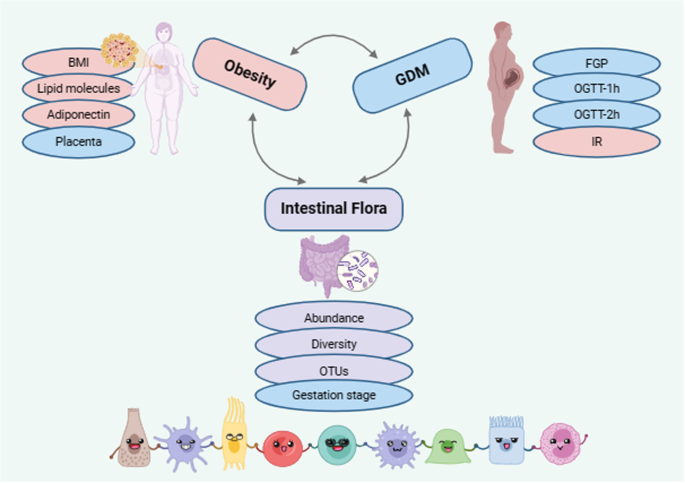
Association of intestinal microbiota and obesity with GDM.
However, this study also has some limitations: The investigation did not cover whether the offspring of mothers with GDM have abnormal intestinal microbiome; Additionally, this review did not discuss the potential of probiotics to treat intestinal dysbiosis due to length constraints; Furthermore, the limited number of current literatures on the relationship between the three factors makes it impossible to carry out a meta-analysis. Considering the aforementioned limitations, it is expected that future studies will concentrate on this aspect to provide advantages to patients with GDM.
Throughout the extensive history of studying gastrointestinal microbiome, it has been discovered that certain combinations or individual microbes have significant effects on host metabolism. This study analyzed high-quality and related literatures to identify that an imbalance in the F/B ratio may be a characteristic feature of intestinal microbiome dysbiosis, as outlined below: 1. An imbalance of the F/B ratio may lead to metabolic disorders such as obesity and diabetes; 2. The F/B ratio has been found to decrease with age, which may result in decreased glucose tolerance; 3. It can be concluded that the alteration of the Firmicutes is a characteristic marker of both GDM and obesity. Additionally, a characteristic biomarker of GDM, Ruminococcus gnavus, was found. However, the up- or down-regulation of the Firmicutes did not consistently affect the development of the disease. Studies have shown conflicting results regarding the F/B ratio in obese and GDM patients, with some suggesting a decrease and others suggesting a trend towards an increase in overweight or obesity. Despite some results from animal studies contradicting the transplantation of microbiota in obese populations, further research has shown promise. With the deepening and refinement of animal studies, as well as large-scale population-based studies (which are mainly retrospective at present), it is believed that specific changes in human intestinal microbiome (especially in pregnant populations) will be explored. This will be a milestone for early identification of clinical diseases and effective monitoring of health status.
Kolodziejczyk AA, Zheng D, Elinav E. Diet-microbiota interactions and personalized nutrition. Nat Rev Microbiol. 2019;17:742–53.
Article CAS PubMed Google Scholar
Qin Y, Roberts JD, Grimm SA, Lih FB, Deterding LJ, Li R, et al. An obesity-associated gut microbiome reprograms the intestinal epigenome and leads to altered colonic gene expression. Genome Biol. 2018;19:7.
Article PubMed PubMed Central Google Scholar
Gilbert JA, Blaser MJ, Caporaso JG, Jansson JK, Lynch SV, Knight R. Current understanding of the human microbiome. Nat Med. 2018;24:392–400.
Article CAS PubMed PubMed Central Google Scholar
Song Z, Li S, Li R. An Investigation into the Correlation of Intestinal Flora with Obesity and Gestational Diabetes Mellitus. Comput Math Methods Med. 2022;2022:5677073.
Rinninella E, Raoul P, Cintoni M, Franceschi F, Miggiano GAD, Gasbarrini A, et al. What is the Healthy Gut Microbiota Composition? A Changing Ecosystem across Age, Environment, Diet, and Diseases. Microorganisms. 2019;7:14.
Li D, Wang P, Wang P, Hu X, Chen F. The gut microbiota: A treasure for human health. Biotechnol Adv. 2016;34:1210–24.
Article PubMed Google Scholar
McIntyre HD, Catalano P, Zhang C, Desoye G, Mathiesen ER, Damm P. Gestational diabetes mellitus. Nat Rev Dis Prim. 2019;5:47.
Zheng QX, Wang HW, Jiang XM, Lin Y, Liu GH, Pan M, et al. Prepregnancy body mass index and gestational weight gain are associated with maternal and infant adverse outcomes in Chinese women with gestational diabetes. Sci Rep. 2022;12:2749.
Committee on Practice Bulletins—Obstetrics. ACOG Practice Bulletin No. 190: Gestational Diabetes Mellitus. Obstet Gynecol. 2018;131: e49–e64.
Pervjakova N, Moen GH, Borges MC, Ferreira T, Cook JP, Allard C, et al. Multi-ancestry genome-wide association study of gestational diabetes mellitus highlights genetic links with type 2 diabetes. Hum Mol Genet. 2022;31:3377–91.
Saeedi P, Petersohn I, Salpea P, Malanda B, Karuranga S, Unwin N, et al. Global and regional diabetes prevalence estimates for 2019 and projections for 2030 and 2045: Results from the International Diabetes Federation Diabetes Atlas, 9th edition. Diabetes Res Clin Pr. 2019;157:107843.
Article Google Scholar
Fakhrul-Alam M, Sharmin-Jahan, Mashfiqul-Hasan, Nusrat-Sultana, Mohona-Zaman, Rakibul-Hasan M, et al. Insulin secretory defect may be the major determinant of GDM in lean mothers. J Clin Transl Endocrinol. 2020;20:100226.
CAS PubMed PubMed Central Google Scholar
Lynch SV, Pedersen O. The Human Intestinal Microbiome in Health and Disease. N Engl J Med. 2016;375:2369–79.
Abbasalizad Farhangi M, Vajdi M. Gut microbiota-associated trimethylamine N-oxide and increased cardiometabolic risk in adults: a systematic review and dose-response meta-analysis. Nutr Rev. 2021;79:1022–42.
Ziętek M, Celewicz Z, Szczuko M. Short-Chain Fatty Acids, Maternal Microbiota and Metabolism in Pregnancy. Nutrients. 2021;13:1244.
Frank DN, St Amand AL, Feldman RA, Boedeker EC, Harpaz N, Pace NR. Molecular-phylogenetic characterization of microbial community imbalances in human inflammatory bowel diseases. Proc Natl Acad Sci USA. 2007;104:13780–5.
Thaiss CA, Itav S, Rothschild D, Meijer MT, Levy M, Moresi C, et al. Persistent microbiome alterations modulate the rate of post-dieting weight regain. Nature. 2016;540:544–51.
Gomes AC, Hoffmann C, Mota JF. The human gut microbiota: Metabolism and perspective in obesity. Gut Microbes. 2018;9:308–25.
Crusell MKW, Hansen TH, Nielsen T, Allin KH, Rühlemann MC, Damm P, et al. Gestational diabetes is associated with change in the gut microbiota composition in third trimester of pregnancy and postpartum. Microbiome. 2018;6:89.
Li R, Andreu-Sánchez S, Kuipers F, Fu J. Gut microbiome and bile acids in obesity-related diseases. Best Pr Res Clin Endocrinol Metab. 2021;35:101493.
Article CAS Google Scholar
Fogelson KA, Dorrestein PC, Zarrinpar A, Knight R. The Gut Microbial Bile Acid Modulation and Its Relevance to Digestive Health and Diseases. Gastroenterology. 2023;164:1069–85.
Matsubara T, Li F, Gonzalez FJ. FXR signaling in the enterohepatic system. Mol Cell Endocrinol. 2013;368:17–29.
Pols TW, Noriega LG, Nomura M, Auwerx J, Schoonjans K. The bile acid membrane receptor TGR5 as an emerging target in metabolism and inflammation. J Hepatol. 2011;54:1263–72.
Watanabe M, Houten SM, Mataki C, Christoffolete MA, Kim BW, Sato H, et al. Bile acids induce energy expenditure by promoting intracellular thyroid hormone activation. Nature. 2006;439:484–9.
Fiorucci S, Mencarelli A, Palladino G, Cipriani S. Bile-acid-activated receptors: targeting TGR5 and farnesoid-X-receptor in lipid and glucose disorders. Trends Pharm Sci. 2009;30:570–80.
Katsuma S, Hirasawa A, Tsujimoto G. Bile acids promote glucagon-like peptide-1 secretion through TGR5 in a murine enteroendocrine cell line STC-1. Biochem Biophys Res Commun. 2005;329:386–90.
Duran-Sandoval D, Cariou B, Percevault F, Hennuyer N, Grefhorst A, van Dijk TH, et al. The farnesoid X receptor modulates hepatic carbohydrate metabolism during the fasting-refeeding transition. J Biol Chem. 2005;280:29971–9.
Wahlström A, Sayin SI, Marschall HU, Bäckhed F. Intestinal Crosstalk between Bile Acids and Microbiota and Its Impact on Host Metabolism. Cell Metab. 2016;24:41–50.
Ma K, Saha PK, Chan L, Moore DD. Farnesoid X receptor is essential for normal glucose homeostasis. J Clin Invest. 2006;116:1102–9.
Lambert G, Amar MJ, Guo G, Brewer HB Jr, Gonzalez FJ, Sinal CJ. The farnesoid X-receptor is an essential regulator of cholesterol homeostasis. J Biol Chem. 2003;278:2563–70.
Lee A, Ader M, Bray GA, Bergman RN. Diurnal variation in glucose tolerance. Cyclic suppression of insulin action and insulin secretion in normal-weight, but not obese, subjects. Diabetes. 1992;41:750–9.
Guo X, Wang J, Xu H, Wang Y, Cao Y, Wen Y, et al. Obesity induced disruption on diurnal rhythm of insulin sensitivity via gut microbiome-bile acid metabolism. Biochim Biophys Acta Mol Cell Biol Lipids. 2024;1869:159419.
Tilg H, Adolph TE. Influence of the human intestinal microbiome on obesity and metabolic dysfunction. Curr Opin Pediatr. 2015;27:496–501.
Neuman H, Koren O. The Pregnancy Microbiome. Nestle Nutr Inst Workshop Ser. 2017;88:1–9.
Clemente JC, Pehrsson EC, Blaser MJ, Sandhu K, Gao Z, Wang B, et al. The microbiome of uncontacted Amerindians. Sci Adv. 2015;1:e1500183.
Su Y, Chen L, Zhang DY, Gan XP, Cao YN, Cheng DC, et al. The characteristics of intestinal flora in overweight pregnant women and the correlation with gestational diabetes mellitus. Endocr Connect. 2021;10:1366–76.
Moran-Ramos S, López-Contreras BE, Canizales-Quinteros S. Gut Microbiota in Obesity and Metabolic Abnormalities: A Matter of Composition or Functionality? Arch Med Res. 2017;48:735–53.
Jovel J, Patterson J, Wang W, Hotte N, O’Keefe S, Mitchel T, et al. Characterization of the Gut Microbiome Using 16S or Shotgun Metagenomics. Front Microbiol. 2016;7:459.
Mullins TP, Tomsett KI, Gallo LA, Callaway LK, McIntyre HD, Dekker Nitert M, et al. Maternal gut microbiota displays minor changes in overweight and obese women with GDM. Nutr Metab Cardiovasc Dis. 2021;31:2131–9.
Abdullah B, Daud S, Aazmi MS, Idorus MY, Mahamooth MIJ. Gut microbiota in pregnant Malaysian women: a comparison between trimesters, body mass index and gestational diabetes status. BMC Pregnancy Childbirth. 2022;22:152.
Vavreckova M, Galanova N, Kostovcik M, Krystynik O, Ivanovova E, Roubalova R, et al. Specific gut bacterial and fungal microbiota pattern in the first half of pregnancy is linked to the development of gestational diabetes mellitus in the cohort including obese women. Front Endocrinol. 2022;13:970825.
Dualib PM, Taddei CR, Fernandes G, Carvalho CRS, Sparvoli LG, Silva IT, et al. Gut Microbiota across Normal Gestation and Gestational Diabetes Mellitus: A Cohort Analysis. Metabolites. 2022;12:796.
International Association of Diabetes and Pregnancy Study Groups Consensus Panel, Metzger BE, Gabbe SG, Persson B, Buchanan TA, Catalano PA, et al. International association of diabetes and pregnancy study groups recommendations on the diagnosis and classification of hyperglycemia in pregnancy. Diabetes Care. 2010;33:676–82.
Article PubMed Central Google Scholar
Le Chatelier E, Nielsen T, Qin J, Prifti E, Hildebrand F, Falony G, et al. Richness of human gut microbiome correlates with metabolic markers. Nature. 2013;500:541–6.
Blaut M. Gut microbiota and energy balance: role in obesity. Proc Nutr Soc. 2015;74:227–34.
Ma Q, Li Y, Li P, Wang M, Wang J, Tang Z, et al. Research progress in the relationship between type 2 diabetes mellitus and intestinal flora. Biomed Pharmacother. 2019;117:109138.
Kimura I, Ozawa K, Inoue D, Imamura T, Kimura K, Maeda T, et al. The gut microbiota suppresses insulin-mediated fat accumulation via the short-chain fatty acid receptor GPR43. Nat Commun. 2013;4:1829.
Tolhurst G, Heffron H, Lam YS, Parker HE, Habib AM, Diakogiannaki E, et al. Short-chain fatty acids stimulate glucagon-like peptide-1 secretion via the G-protein-coupled receptor FFAR2. Diabetes. 2012;61:364–71.
Ye G, Zhang L, Wang M, Chen Y, Gu S, Wang K, et al. The Gut Microbiota in Women Suffering from Gestational Diabetes Mellitus with the Failure of Glycemic Control by Lifestyle Modification. J Diabetes Res. 2019;2019:6081248.
Kuang YS, Lu JH, Li SH, Li JH, Yuan MY, He JR, et al. Connections between the human gut microbiome and gestational diabetes mellitus. Gigascience. 2017;6:1–12.
Cortez RV, Taddei CR, Sparvoli LG, Ângelo AGS, Padilha M, Mattar R, et al. Microbiome and its relation to gestational diabetes. Endocrine. 2019;64:254–64.
Gao B, Zhong M, Shen Q, Wu Y, Cao M, Ju S, et al. Gut microbiota in early pregnancy among women with Hyperglycaemia vs. Normal blood glucose. BMC Pregnancy Childbirth. 2020;20:284.
Nuriel-Ohayon M, Neuman H, Koren O. Microbial Changes during Pregnancy, Birth, and Infancy. Front Microbiol. 2016;7:1031.
Ferrocino I, Ponzo V, Gambino R, Zarovska A, Leone F, Monzeglio C, et al. Changes in the gut microbiota composition during pregnancy in patients with gestational diabetes mellitus (GDM). Sci Rep. 2018;8:12216.
Koren O, Goodrich JK, Cullender TC, Spor A, Laitinen K, Bäckhed HK, et al. Host remodeling of the gut microbiome and metabolic changes during pregnancy. Cell. 2012;150:470–80.
Liu J, Yang H, Yin Z, Jiang X, Zhong H, Qiu D, et al. Remodeling of the gut microbiota and structural shifts in Preeclampsia patients in South China. Eur J Clin Microbiol Infect Dis. 2017;36:713–9.
Li G, Yin P, Chu S, Gao W, Cui S, Guo S, et al. Correlation Analysis between GDM and Gut Microbial Composition in Late Pregnancy. J Diabetes Res. 2021;2021:8892849.
Wang X, Liu H, Li Y, Huang S, Zhang L, Cao C, et al. Altered gut bacterial and metabolic signatures and their interaction in gestational diabetes mellitus. Gut Microbes. 2020;12:1–13.
Qin J, Li Y, Cai Z, Li S, Zhu J, Zhang F, et al. A metagenome-wide association study of gut microbiota in type 2 diabetes. Nature. 2012;490:55–60.
Huang X, Fang S, Yang H, Gao J, He M, Ke S, et al. Evaluating the contribution of gut microbiome to the variance of porcine serum glucose and lipid concentration. Sci Rep. 2017;7:14928.
Juge N. Microbe Profile: Ruminococcus gnavus: the yin and yang of human gut symbionts. Microbiology. 2023;169:001383.
Ponzo V, Fedele D, Goitre I, Leone F, Lezo A, Monzeglio C, et al. Diet-Gut Microbiota Interactions and Gestational Diabetes Mellitus (GDM). Nutrients. 2019;11:330.
Huang L, Sililas P, Thonusin C, Tongsong T, Luewan S, Chattipakorn N, et al. Association Between Gut Microbiota and Insulin Therapy in Women With Gestational Diabetes Mellitus. Can J Diabetes. 2022;46:804–812.e2.
Li H, Shi J, Zhao L, Guan J, Liu F, Huo G, et al. Lactobacillus plantarum KLDS1.0344 and Lactobacillus acidophilus KLDS1.0901 Mixture Prevents Chronic Alcoholic Liver Injury in Mice by Protecting the Intestinal Barrier and Regulating Gut Microbiota and Liver-Related Pathways. J Agric Food Chem. 2021;69:183–97.
Liu J, Li T, Wu H, Shi H, Bai J, Zhao W, et al. Lactobacillus rhamnosus GG strain mitigated the development of obstructive sleep apnea-induced hypertension in a high salt diet via regulating TMAO level and CD4+ T cell induced-type I inflammation. Biomed Pharmacother. 2019;112:108580.
Jang HM, Han SK, Kim JK, Oh SJ, Jang HB, Kim DH. Lactobacillus sakei Alleviates High-Fat-Diet-Induced Obesity and Anxiety in Mice by Inducing AMPK Activation and SIRT1 Expression and Inhibiting Gut Microbiota-Mediated NF-κB Activation. Mol Nutr Food Res. 2019;63:e1800978.
Wu Y, Bible PW, Long S, Ming WK, Ding W, Long Y, et al. Metagenomic analysis reveals gestational diabetes mellitus-related microbial regulators of glucose tolerance. Acta Diabetol. 2020;57:569–81.
Chen F, Gan Y, Li Y, He W, Wu W, Wang K, et al. Association of gestational diabetes mellitus with changes in gut microbiota composition at the species level. BMC Microbiol. 2021;21:147.
Nehra V, Allen JM, Mailing LJ, Kashyap PC, Woods JA. Gut Microbiota: Modulation of Host Physiology in Obesity. Physiology. 2016;31:327–35.
Carlos D, Pérez MM, Leite JA, Rocha FA, Martins LMS, Pereira CA, et al. NOD2 Deficiency Promotes Intestinal CD4+ T Lymphocyte Imbalance, Metainflammation, and Aggravates Type 2 Diabetes in Murine Model. Front Immunol. 2020;11:1265.
Garcia-Mantrana I, Collado MC. Obesity and overweight: Impact on maternal and milk microbiome and their role for infant health and nutrition. Mol Nutr Food Res. 2016;60:1865–75.
Spor A, Koren O, Ley R. Unravelling the effects of the environment and host genotype on the gut microbiome. Nat Rev Microbiol. 2011;9:279–90.
Bäckhed F, Ding H, Wang T, Hooper LV, Koh GY, Nagy A, et al. The gut microbiota as an environmental factor that regulates fat storage. Proc Natl Acad Sci USA. 2004;101:15718–23.
Arumugam M, Raes J, Pelletier E, Le Paslier D, Yamada T, Mende DR, et al. Enterotypes of the human gut microbiome. Nature. 2011;473:174–80.
Sehgal K, Khanna S. Gut microbiota: a target for intervention in obesity. Expert Rev Gastroenterol Hepatol. 2021;15:1169–79.
Edwards SM, Cunningham SA, Dunlop AL, Corwin EJ. The Maternal Gut Microbiome During Pregnancy. MCN Am J Matern Child Nurs. 2017;42:310–7.
Riva A, Borgo F, Lassandro C, Verduci E, Morace G, Borghi E, et al. Pediatric obesity is associated with an altered gut microbiota and discordant shifts in Firmicutes populations. Environ Microbiol. 2017;19:95–105.
Ley RE, Bäckhed F, Turnbaugh P, Lozupone CA, Knight RD, Gordon JI. Obesity alters gut microbial ecology. Proc Natl Acad Sci USA. 2005;102:11070–5.
Kasai C, Sugimoto K, Moritani I, Tanaka J, Oya Y, Inoue H, et al. Comparison of the gut microbiota composition between obese and non-obese individuals in a Japanese population, as analyzed by terminal restriction fragment length polymorphism and next-generation sequencing. BMC Gastroenterol. 2015;15:100.
Armougom F, Henry M, Vialettes B, Raccah D, Raoult D. Monitoring bacterial community of human gut microbiota reveals an increase in Lactobacillus in obese patients and Methanogens in anorexic patients. PLoS One. 2009;4:e7125.
Million M, Thuny F, Angelakis E, Casalta JP, Giorgi R, Habib G, et al. Lactobacillus reuteri and Escherichia coli in the human gut microbiota may predict weight gain associated with vancomycin treatment. Nutr Diabetes. 2013;3:e87.
Vallianou N, Stratigou T, Christodoulatos GS, Dalamaga M. Understanding the Role of the Gut Microbiome and Microbial Metabolites in Obesity and Obesity-Associated Metabolic Disorders: Current Evidence and Perspectives. Curr Obes Rep. 2019;8:317–32.
Ley RE, Turnbaugh PJ, Klein S, Gordon JI. Microbial ecology: human gut microbes associated with obesity. Nature. 2006;444:1022–3.
Turnbaugh PJ, Ley RE, Mahowald MA, Magrini V, Mardis ER, Gordon JI. An obesity-associated gut microbiome with increased capacity for energy harvest. Nature. 2006;444:1027–31.
Zacarías MF, Collado MC, Gómez-Gallego C, Flinck H, Aittoniemi J, Isolauri E, et al. Pregestational overweight and obesity are associated with differences in gut microbiota composition and systemic inflammation in the third trimester. PLoS One. 2018;13:e0200305.
Houttu N, Mokkala K, Laitinen K. Overweight and obesity status in pregnant women are related to intestinal microbiota and serum metabolic and inflammatory profiles. Clin Nutr. 2018;37:1955–66.
Rodrigues VF, Elias-Oliveira J, Pereira ÍS, Pereira JA, Barbosa SC, Machado MSG, et al. Akkermansia muciniphila and Gut Immune System: A Good Friendship That Attenuates Inflammatory Bowel Disease, Obesity, and Diabetes. Front Immunol. 2022;13:934695.
Lim MY, You HJ, Yoon HS, Kwon B, Lee JY, Lee S, et al. The effect of heritability and host genetics on the gut microbiota and metabolic syndrome. Gut. 2017;66:1031–8.
Dao MC, Everard A, Aron-Wisnewsky J, Sokolovska N, Prifti E, Verger EO, et al. Akkermansia muciniphila and improved metabolic health during a dietary intervention in obesity: relationship with gut microbiome richness and ecology. Gut. 2016;65:426–36.
Depommier C, Van Hul M, Everard A, Delzenne NM, De Vos WM, Cani PD. Pasteurized Akkermansia muciniphila increases whole-body energy expenditure and fecal energy excretion in diet-induced obese mice. Gut Microbes. 2020;11:1231–45.
Yoon HS, Cho CH, Yun MS, Jang SJ, You HJ, Kim JH, et al. Akkermansia muciniphila secretes a glucagon-like peptide-1-inducing protein that improves glucose homeostasis and ameliorates metabolic disease in mice. Nat Microbiol. 2021;6:563–73.
Lee JS, Song WS, Lim JW, Choi TR, Jo SH, Jeon HJ, et al. An integrative multiomics approach to characterize anti-adipogenic and anti-lipogenic effects of Akkermansia muciniphila in adipocytes. Biotechnol J. 2022;17:e2100397.
Plows JF, Stanley JL, Baker PN, Reynolds CM, Vickers MH. The Pathophysiology of Gestational Diabetes Mellitus. Int J Mol Sci. 2018;19:3342.
Pantham P, Aye IL, Powell TL. Inflammation in maternal obesity and gestational diabetes mellitus. Placenta. 2015;36:709–15.
Parrettini S, Caroli A, Torlone E. Nutrition and Metabolic Adaptations in Physiological and Complicated Pregnancy: Focus on Obesity and Gestational Diabetes. Front Endocrinol. 2020;11:611929.
Gregor MF, Hotamisligil GS. Inflammatory mechanisms in obesity. Annu Rev Immunol. 2011;29:415–45.
Wang Y, Pan XF, Pan A. Lipidomics in gestational diabetes mellitus. Curr Opin Lipido. 2023;34:1–11.
Wang Y, Wu P, Huang Y, Ye Y, Yang X, Sun F, et al. BMI and lipidomic biomarkers with risk of gestational diabetes in pregnant women. Obesity. 2022;30:2044–54.
MacDonald SC, Bodnar LM, Himes KP, Hutcheon JA. Patterns of Gestational Weight Gain in Early Pregnancy and Risk of Gestational Diabetes Mellitus. Epidemiology. 2017;28:419–27.
Jarvie E, Hauguel-de-Mouzon S, Nelson SM, Sattar N, Catalano PM, Freeman DJ. Lipotoxicity in obese pregnancy and its potential role in adverse pregnancy outcome and obesity in the offspring. Clin Sci. 2010;119:123–9.
Kampmann U, Knorr S, Fuglsang J, Ovesen P. Determinants of Maternal Insulin Resistance during Pregnancy: An Updated Overview. J Diabetes Res. 2019;2019:5320156.
Chatuphonprasert W, Jarukamjorn K, Ellinger I. Physiology and Pathophysiology of Steroid Biosynthesis, Transport and Metabolism in the Human Placenta. Front Pharm. 2018;9:1027.
Dubé E, Ethier-Chiasson M, Lafond J. Modulation of cholesterol transport by insulin-treated gestational diabetes mellitus in human full-term placenta. Biol Reprod. 2013;88:16.
Bouvier D, Rouzaire M, Marceau G, Prat C, Pereira B, Lemarié R, et al. Aquaporins and Fetal Membranes From Diabetic Parturient Women: Expression Abnormalities and Regulation by Insulin. J Clin Endocrinol Metab. 2015;100:E1270–9.
Zhang Y, Ye J, Fan J. Regulation of malonyl-CoA-acyl carrier protein transacylase network in umbilical cord blood affected by intrauterine hyperglycemia. Oncotarget. 2017;8:75254–63.
Tanaka K, Yamada K, Matsushima M, Izawa T, Furukawa S, Kobayashi Y, et al. Increased maternal insulin resistance promotes placental growth and decreases placental efficiency in pregnancies with obesity and gestational diabetes mellitus. J Obstet Gynaecol Res. 2018;44:74–80.
Huang L, Liu J, Feng L, Chen Y, Zhang J, Wang W. Maternal prepregnancy obesity is associated with higher risk of placental pathological lesions. Placenta. 2014;35:563–9.
Huynh J, Dawson D, Roberts D, Bentley-Lewis R. A systematic review of placental pathology in maternal diabetes mellitus. Placenta. 2015;36:101–14.
Salomon C, Scholz-Romero K, Sarker S, Sweeney E, Kobayashi M, Correa P, et al. Gestational Diabetes Mellitus Is Associated With Changes in the Concentration and Bioactivity of Placenta-Derived Exosomes in Maternal Circulation Across Gestation. Diabetes. 2016;65:598–609.
Rice GE, Scholz-Romero K, Sweeney E, Peiris H, Kobayashi M, Duncombe G, et al. The Effect of Glucose on the Release and Bioactivity of Exosomes From First Trimester Trophoblast Cells. J Clin Endocrinol Metab. 2015;100:E1280–8.
Greenhill C. Diabetes: The role of adiponectin in gestational diabetes mellitus. Nat Rev Endocrinol. 2017;13:128.
PubMed Google Scholar
Miehle K, Stepan H, Fasshauer M. Leptin, adiponectin and other adipokines in gestational diabetes mellitus and pre-eclampsia. Clin Endocrinol. 2012;76:2–11.
Haghiac M, Basu S, Presley L, Serre D, Catalano PM, Hauguel-de Mouzon S. Patterns of adiponectin expression in term pregnancy: impact of obesity. J Clin Endocrinol Metab. 2014;99:3427–34.
Qiao L, Wattez JS, Lee S, Nguyen A, Schaack J, Hay WW Jr, et al. Adiponectin Deficiency Impairs Maternal Metabolic Adaptation to Pregnancy in Mice. Diabetes. 2017;66:1126–35.
Turer AT, Scherer PE. Adiponectin: mechanistic insights and clinical implications. Diabetologia. 2012;55:2319–26.
Bhograj A, Suryanarayana KM, Nayak A, Murthy NS, Dharmalingam M, Kalra P. Serum adiponectin levels in gestational diabetes mellitus. Indian J Endocrinol Metab. 2016;20:752–5.
Mohammadi T, Paknahad Z. Adiponectin Concentration in Gestational Diabetic Women: a Case-Control Study. Clin Nutr Res. 2017;6:267–76.
Download references
Acknowledgements
The Fig. 1 was reproduced with permission from [Fogelson KA, Dorrestein PC, Zarrinpar A, Knight R], [The Gut Microbial Bile Acid Modulation and Its Relevance to Digestive Health and Diseases]; published by [Gastroenterology], [2023]. And no changes were made. Please refer to https://creativecommons.org/licenses/by/4.0/ . The Figs. 2 and 3 used in this review were created with BioRender.com.
Funding for this work was provided by the Jilin Provincial Department of Science and Technology (No. 20240305067YY).
Author information
Authors and affiliations.
Department of Gynecology and Obstetrics, The Second Hospital of Jilin University, Changchun, 130000, Jilin, China
Jiahui Li, Min Wang, Shuai Ma, Zhong Jin, Haonan Yin & Shuli Yang
You can also search for this author in PubMed Google Scholar
Contributions
LJ, MW and SM devised the manuscript; ZJ and YH performed literature searches and selected the studies. LJ wrote the manuscript., YS made subsequent amendments and provided financial support. All authors read and approved the final manuscript and contributed to the conception of this review.
Corresponding author
Correspondence to Shuli Yang .
Ethics declarations
Competing interests.
The authors declare no competing interests.
Additional information
Publisher’s note Springer Nature remains neutral with regard to jurisdictional claims in published maps and institutional affiliations.
Supplementary information
Abbreviations, rights and permissions.
Open Access This article is licensed under a Creative Commons Attribution 4.0 International License, which permits use, sharing, adaptation, distribution and reproduction in any medium or format, as long as you give appropriate credit to the original author(s) and the source, provide a link to the Creative Commons licence, and indicate if changes were made. The images or other third party material in this article are included in the article’s Creative Commons licence, unless indicated otherwise in a credit line to the material. If material is not included in the article’s Creative Commons licence and your intended use is not permitted by statutory regulation or exceeds the permitted use, you will need to obtain permission directly from the copyright holder. To view a copy of this licence, visit http://creativecommons.org/licenses/by/4.0/ .
Reprints and permissions
About this article
Cite this article.
Li, J., Wang, M., Ma, S. et al. Association of gastrointestinal microbiome and obesity with gestational diabetes mellitus-an updated globally based review of the high-quality literatures. Nutr. Diabetes 14 , 31 (2024). https://doi.org/10.1038/s41387-024-00291-5
Download citation
Received : 07 January 2024
Revised : 27 April 2024
Accepted : 03 May 2024
Published : 21 May 2024
DOI : https://doi.org/10.1038/s41387-024-00291-5
Share this article
Anyone you share the following link with will be able to read this content:
Sorry, a shareable link is not currently available for this article.
Provided by the Springer Nature SharedIt content-sharing initiative
Quick links
- Explore articles by subject
- Guide to authors
- Editorial policies

Risk of Neonatal Hypoglycemia in Infants of Mothers With Gestational Glucose Intolerance

C.E.P. and S.S. contributed equally to this work.
- Split-Screen
- Article contents
- Figures & tables
- Supplementary Data
- Peer Review
- Open the PDF for in another window
- Cite Icon Cite
- Get Permissions
Chloe Andrews , Jacqueline Maya , Carolin C.M. Schulte , Sarah Hsu , Tanayott Thaweethai , Kaitlyn E. James , Jose Halperin , Camille E. Powe , Sarbattama Sen; Risk of Neonatal Hypoglycemia in Infants of Mothers With Gestational Glucose Intolerance. Diabetes Care 2024; dc232239. https://doi.org/10.2337/dc23-2239
Download citation file:
- Ris (Zotero)
- Reference Manager
To examine the relationship between gestational glucose intolerance (GGI) and neonatal hypoglycemia.
This was a secondary analysis of 8,262 mother-infant dyads, with delivery at two hospitals between 2014 and 2023. We categorized maternal glycemic status as normal glucose tolerance (NGT), GGI, or gestational diabetes mellitus (GDM). We defined NGT according to a normal glucose load test result, GGI according to an abnormal glucose load test result with zero (GGI-0) or one (GGI-1) abnormal value on the 100-g oral glucose tolerance test, and GDM according to an abnormal glucose load test result with two or more abnormal values on the glucose tolerance test. Neonatal hypoglycemia was defined according to blood glucose <45 mg/dL or ICD-9 or ICD-10 diagnosis of neonatal hypoglycemia. We used logistic regression analysis to determine associations between maternal glucose tolerance category and neonatal hypoglycemia and conducted a sensitivity analysis using Δ-adjusted multiple imputation, assuming for unscreened infants a rate of neonatal hypoglycemia as high as 33%.
Of infants, 12% had neonatal hypoglycemia. In adjusted models, infants born to mothers with GGI-0 had 1.28 (95% 1.12, 1.65), GGI-1 1.58 (95% CI 1.11, 2.25), and GDM 4.90 (95% CI 3.81, 6.29) times higher odds of neonatal hypoglycemia in comparison with infants born to mothers with NGT. Associations in sensitivity analyses were consistent with the primary analysis.
GGI is associated with increased risk of neonatal hypoglycemia. Future research should include examination of these associations in a cohort with more complete neonatal blood glucose ascertainment and determination of the clinical significance of these findings on long-term child health.
Graphical Abstract
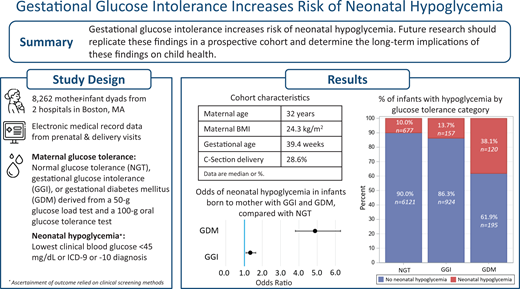
This article contains supplementary material online at https://doi.org/10.2337/figshare.25653348 .
Email alerts
- Online ISSN 1935-5548
- Print ISSN 0149-5992
- Diabetes Care
- Clinical Diabetes
- Diabetes Spectrum
- Standards of Medical Care in Diabetes
- Scientific Sessions Abstracts
- BMJ Open Diabetes Research & Care
- ShopDiabetes.org
- ADA Professional Books
Clinical Compendia
- Clinical Compendia Home
- Latest News
- DiabetesPro SmartBrief
- Special Collections
- DiabetesPro®
- Diabetes Food Hub™
- Insulin Affordability
- Know Diabetes By Heart™
- About the ADA
- Journal Policies
- For Reviewers
- Advertising in ADA Journals
- Reprints and Permission for Reuse
- Copyright Notice/Public Access Policy
- ADA Professional Membership
- ADA Member Directory
- Diabetes.org
- X (Twitter)
- Cookie Policy
- Accessibility
- Terms & Conditions
- Get Adobe Acrobat Reader
- © Copyright American Diabetes Association
This Feature Is Available To Subscribers Only
Sign In or Create an Account

Risk for T2D after gestational diabetes quantified
Posted May 14, 2024 by University of Washington Diabetes Research Center
A large study of women with two singleton pregnancies found an association between gestational diabetes and an increased risk for subsequent diabetes, according to a study in JAMA Network Open . The risk was 15.8 times higher for women with gestational diabetes in both pregnancies and 4.35 times higher for women with gestational diabetes only in the first pregnancy.
Full Story: Physician’s Weekly (5/10)

A .gov website belongs to an official government organization in the United States.
A lock ( ) or https:// means you've safely connected to the .gov website. Share sensitive information only on official, secure websites.
- Risk Factors
- Providing Care
- Living with Diabetes
- Clinical Guidance
- DSMES for Health Care Providers
- Prevent Type 2 Diabetes: Talking to Your Patients About Lifestyle Change
- Employers and Insurers
- Community-based Organizations (CBOs)
- Toolkits for Diabetes Educators and Community Health Workers
- National Diabetes Statistics Report
- Reports and Publications
- Data and Statistics
- Current Research Projects
- National Diabetes Prevention Program
- State, Local, and National Partner Diabetes Programs for Public Health
- Diabetes Self-Management Education and Support (DSMES) Toolkit
About Gestational Diabetes
- Gestational diabetes can develop during pregnancy in women who don't already have diabetes.
- It usually develops around the 24th week of pregnancy.
- Gestational diabetes may not cause symptoms, so testing for it between 24 and 28 weeks is important.
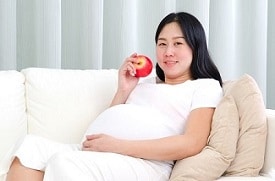
Gestational diabetes can develop during pregnancy in women who don't already have diabetes. Every year, 5% to 9% of U.S. pregnancies are affected by gestational diabetes. Managing gestational diabetes can help make sure you have a healthy pregnancy and a healthy baby.
Gestational diabetes often doesn't have any symptoms. If you do have symptoms, they may be mild, such as being thirstier than normal or having to urinate more often. You'll need to be tested to know for sure if you have gestational diabetes.
Risk factors
You're at higher risk for gestational diabetes if you:
- Had gestational diabetes during a previous pregnancy.
- Have given birth to a baby who weighed over 9 pounds.
- Are overweight.
- Have a family history of type 2 diabetes.
- Have a hormone disorder called polycystic ovary syndrome (PCOS).
- Are an African American, Hispanic or Latino, American Indian, Alaska Native, Native Hawaiian, or Pacific Islander person.
Gestational diabetes happens when your body can't make enough insulin during your pregnancy. Insulin is a hormone made by your pancreas. It acts like a key to let blood sugar into the cells in your body for use as energy.
During pregnancy, your body makes more hormones and goes through other changes, such as weight gain. These changes cause your body's cells to use insulin less well, a condition called insulin resistance . Insulin resistance increases your body's need for insulin.
All pregnant women have some insulin resistance during late pregnancy. However, some women have insulin resistance even before they get pregnant. They start pregnancy with an increased need for insulin and are more likely to develop gestational diabetes.
Before you get pregnant, you may be able to prevent gestational diabetes with lifestyle changes. These include losing weight if you're overweight, eating a healthy diet, and getting regular physical activity .
Don't try to lose weight if you're already pregnant. You'll need to gain some weight for your baby to be healthy. Talk to your doctor about how much weight you should gain for a healthy pregnancy.
Prevent type 2 diabetes
It's important to be tested for gestational diabetes so you can begin treatment to protect your baby's health and your own.
Gestational diabetes usually develops around the 24 th week of pregnancy. You'll probably be tested between 24 and 28 weeks.
If you're at higher risk for gestational diabetes, your doctor may test you earlier. If your blood sugar is higher than normal early in your pregnancy, you may not have gestational diabetes. You may have type 1 or type 2 diabetes instead.
You can do a lot to manage your gestational diabetes. Go to all your prenatal appointments and follow your treatment plan, including:
- Checking your blood sugar to make sure your levels stay in a healthy range.
- Being active lowers your blood sugar and makes you more sensitive to insulin.
- Eating healthy food in the right amounts at the right times.
If healthy eating and being active aren't enough to manage your blood sugar, your doctor may prescribe insulin, metformin, or other medication.
Diabetes is a chronic disease that affects how your body turns food into energy. About 1 in 10 Americans has diabetes.
For Everyone
Health care providers, public health.
Association between GLO1 variants and gestational diabetes mellitus susceptibility in a Chinese population: a preliminary study
Affiliations.
- 1 Department of Internal Medicine, Shunde Women and Children's Hospital (Maternity and Child Healthcare Hospital of Shunde Foshan), Guangdong Medical University, Foshan, Guangdong, China.
- 2 Key Laboratory of Research in Maternal and Child Medicine and Birth Defects, Guangdong Medical University, Foshan, Guangdong, China.
- 3 Maternal and Child Research Institute, Shunde Women and Children's Hospital (Maternity and Child Healthcare Hospital of Shunde Foshan), Guangdong Medical University, Foshan, Guangdong, China.
- 4 State Key Laboratory of Quality Research in Chinese Medicine, School of Pharmacy, Macau University of Science and Technology, Taipa, Macao, Macao SAR, China.
- 5 Guangdong Engineering Research Center of Chinese Medicine & Disease Susceptibility, Jinan University, Guangzhou, Guangdong, China.
- 6 Department of Ultrasound, Shunde Women and Children's Hospital (Maternity and Child Healthcare Hospital of Shunde Foshan), Guangdong Medical University, Foshan, Guangdong, China.
- 7 Department of Ultrasound, Affiliated Hospital of Guangdong Medical University, Zhanjiang, Guangdong, China.
- 8 Department of Obstetric, Shunde Women and Children's Hospital (Maternity and Child Healthcare Hospital of Shunde Foshan), Guangdong Medical University, Foshan, Guangdong, China.
- 9 Department of Gynecology, Shunde Women and Children's Hospital (Maternity and Child Healthcare Hospital of Shunde Foshan), Guangdong Medical University, Foshan, Guangdong, China.
- 10 Department of Endocrinology, Affiliated Hospital of Guangdong Medical University, Zhanjiang, Guangdong, China.
- PMID: 38027126
- PMCID: PMC10656739
- DOI: 10.3389/fendo.2023.1235581
Background: Glyoxalase 1 ( GLO1 ) plays a crucial role in defending against glycation. Single nucleotide polymorphism (SNP) variants in the GLO1 gene may affect gene expression and alter enzyme activity. However, there have been limited studies evaluating the association between GLO1 and diabetes, especially gestational diabetes mellitus (GDM). Therefore, this study is the first to explore the association of GLO1 SNPs and GDM risk.
Methods: The study included a total of 500 GDM patients and 502 control subjects. The SNPscan™ genotyping assay was used to genotype rs1781735, rs4746 and rs1130534. To assess the disparities in genotype, allele, and haplotype distributions and their correlation with GDM risk, the independent sample t-test, logistic regression, and chi-square test were employed during the data processing phase. Furthermore, one-way ANOVA was conducted to determine the differences in genotype and blood glucose and methylglyoxal(MG) levels.
Results: Significant differences were observed in prepregnancy body mass index (pre-BMI), age, systolic blood pressure (SBP), diastolic blood pressure (DBP), and parity between GDM and healthy subjects ( P < 0.05). After adjusting for these factors, GLO1 rs1130534 TA remained associated with an increased risk of GDM (TA vs . TT + AA: OR = 1.320; 95% CI: 1.008-1.728; P = 0.044), especially in the pre-BMI ≥ 24 subgroup (TA vs . TT + AA: OR = 2.424; 95% CI: 1.048-5.607; P = 0.039), with fasting glucose levels being significantly elevated in the TA genotype compared to the TT genotype ( P < 0.05). Conversely, the GLO1 rs4746 TG was associated with a decreased risk of GDM (TG vs . TT: OR = 0.740; 95% CI: 0.548-0.999; P = 0.049; TG vs . TT + GG: OR = 0.740; 95% CI: 0.548-0.998; P = 0.048). Additionally, the haplotype T-G-T of rs1781735, rs4746 and rs1130534 was associated with a decreased risk of GDM among individuals with a pre-BMI ≥ 24 (OR = 0.423; 95% CI: 0.188-0.955; P = 0.038). Furthermore, the rs1781735 GG genotype was found to be more closely related to maternal MG accumulation and neonatal weight gain ( P < 0.05).
Conclusion: Our findings suggested that GLO1 rs1130534 was associated with an increased susceptibility to GDM and higher blood glucose levels, but GLO1 rs4746 was associated with a decreased risk of GDM. The rs1781735 has been associated with the accumulation of maternal MG and subsequent weight gain in neonates.
Keywords: GLO1; MG; gestational diabetes mellitus (GDM); rs1130534; rs1781735; rs4746.
Copyright © 2023 Zeng, Yang, Wei, Zou, Wei, Han, He, Huang and Guo.
Publication types
- Research Support, Non-U.S. Gov't
- Blood Glucose / metabolism
- Diabetes, Gestational* / epidemiology
- Diabetes, Gestational* / genetics
- East Asian People
- Infant, Newborn
- Lactoylglutathione Lyase* / genetics
- Lactoylglutathione Lyase* / metabolism
- Polymorphism, Single Nucleotide
- Weight Gain
- Blood Glucose
- Lactoylglutathione Lyase
- GLO1 protein, human
Grants and funding
- Undergraduate
- High School
- Architecture
- American History
- Asian History
- Antique Literature
- American Literature
- Asian Literature
- Classic English Literature
- World Literature
- Creative Writing
- Linguistics
- Criminal Justice
- Legal Issues
- Anthropology
- Archaeology
- Political Science
- World Affairs
- African-American Studies
- East European Studies
- Latin-American Studies
- Native-American Studies
- West European Studies
- Family and Consumer Science
- Social Issues
- Women and Gender Studies
- Social Work
- Natural Sciences
- Pharmacology
- Earth science
- Agriculture
- Agricultural Studies
- Computer Science
- IT Management
- Mathematics
- Investments
- Engineering and Technology
- Engineering
- Aeronautics
- Medicine and Health
- Alternative Medicine
- Communications and Media
- Advertising
- Communication Strategies
- Public Relations
- Educational Theories
- Teacher's Career
- Chicago/Turabian
- Company Analysis
- Education Theories
- Shakespeare
- Canadian Studies
- Food Safety
- Relation of Global Warming and Extreme Weather Condition
- Movie Review
- Admission Essay
- Annotated Bibliography
- Application Essay
- Article Critique
- Article Review
- Article Writing
- Book Review
- Business Plan
- Business Proposal
- Capstone Project
- Cover Letter
- Creative Essay
- Dissertation
- Dissertation - Abstract
- Dissertation - Conclusion
- Dissertation - Discussion
- Dissertation - Hypothesis
- Dissertation - Introduction
- Dissertation - Literature
- Dissertation - Methodology
- Dissertation - Results
- GCSE Coursework
- Grant Proposal
- Marketing Plan
- Multiple Choice Quiz
- Personal Statement
- Power Point Presentation
- Power Point Presentation With Speaker Notes
- Questionnaire
- Reaction Paper
Research Paper
Research Proposal
- SWOT analysis
- Thesis Paper
- Online Quiz
Literature Review
- Movie Analysis
- Statistics problem
- Math Problem
- All papers examples
- How It Works
- Money Back Policy
- Terms of Use
- Privacy Policy
- We Are Hiring
Gestational Diabetes, Research Proposal Example
Pages: 12
Words: 3190
Hire a Writer for Custom Research Proposal
Use 10% Off Discount: "custom10" in 1 Click 👇
You are free to use it as an inspiration or a source for your own work.
Does Two Hours Walking of Gestational Diabetes (GD) Women During Pregnancy Can Improve the Treatment of GD and the Quality of Life Compared to Those With Low Physical Activities (PA)?
Gestational Diabetes (GD) is the most common medical condition in pregnancy (Artal, 2003a; Dye, Knox, Artal, Aubry, & Wojtowycx, 2007). GD is defined as an “abnormal carbohydrate intolerance of variable severity that is first manifested during pregnancy and ends with childbirth” (Artal, 2003b; Catalano, Kirwan, Hagel-de Mouzon, & King, 2003). Even though GD begins and ends with pregnancy, GD may be a risk factor for diabetes mellitus after this pregnancy. GD is associated with the maternal development of type 2 diabetes mellitus (T2DM) later in life (Kim, Newton, & Knopp, 2002; Verma et al., 2002). Up to 40% of women with GD develop T2DM within four to fifteen years after experiencing GD (Albaeda et al., 2013; Brody, Harris, & Lohr, 2003).
GD increases perinatal morbidity (Artal, 2003b). Pregnant women with GD have increased rates of hypertension and cesarean birth. Infants of women with GD experience increased birth injuries, metabolic deviations, and are more likely to become obese and develop T2DM during adolescence (National Diabetes Data Group, 2015). Little is known about the effects of long-hours walking during pregnancy with diagnosed GD as compared to those pregnant women with low physical activities (PA).
Rationale
Physical exercise such as brisk walking is a well-known adjunctive or alternative therapy for the control of elevated blood glucose for nonpregnant individuals with Type 2 Diabetes Mellitus (T2DM) (Nelson, Reiber, & Boyko, 2002; Reusch, 2002). Pregnant women sometimes do not participate in physical exercise because of fear of risk to the fetus (Artal, 2003b). As it has become apparent that risk to the fetus is minimal (Kennelly, McCaffrey, McLoughlin, Lyons, & McKenna, 2002), researchers have begun to investigate the possible benefits of physical exercise for maternal glycemic control during pregnancy. Recent studies suggest that physical exercise for women with GD improves glycemic control thereby reducing maternal and fetal morbidity
(Artal, 2003b).
The effects of maternal physical exercise are probably through enhanced glucose uptake by skeletal muscle. Physical exercise promotes the glucose uptake in skeletal muscle, thus reducing hyperglycemia (Artal, 2003b; Booth, Gordon, Carlson, & Hamilton, 2000). Improving maternal
glycemic control reduces the risk of fetal hyperglycemia during pregnancy (Artal, 2003b; Bessinger & McMurray, 2003; Jovanovic, 2000). Improved glucose levels are a reflection of reduced insulin resistance. Thus maternal physical exercise behaviour has the potential to reduce the complications associated with insulin resistance.
Physical exercise counteracts the insulin resistance of T2DM and increases glucose transport into the muscle cells (Goodpaster, He, Watkins, & Kelley, 2001). Pregnant women might also benefit from long walks as a means to control blood glucose either in normal or Gestational Diabetes (GD) pregnancies (Artal, 2003; Bessinger & McMurray, 2003). The focus on this research is the comparison of the effects of two-hours walking of GD pregnant women to those pregnant women with low physical activities as to improved GD treatment and quality of life.
Research Question
Does two hours walking of gestational diabetes (GD) women during pregnancy improve the treatment of GD and quality of life compared to those with low physical activities (PA)?
Objectives and Hypothesis
The primary objective of this research is to compare the effect of two-hours walking of GD women during pregnancy to those with low physical activities (PA).
Hypothesis : Women who perform two-hours walking improve treatment of GD than those women with low PA as to.
- The interval between the initial clinic visit and need for insulin.
Hypothesis: Pregnant women participating in two-hours walking will have a longer interval between the onset of therapy and initiation of insulin treatment than women with low PA.
- The amount of insulin required.
Hypothesis: The amount of insulin required will be lower in women performing two-hours walking than those women with low PA.
The secondary objective is to compare the effect of two-hours walking of Gestational Diabetic (GD) women during pregnancy to those with low physical activities (PA) on the quality of life. Thus, qualitative analysis will help clarify the relationships between two-hours walking to the treatment of GD, and the quality of life.
Plain English Summary
Currently there are studies published for the benefits of physical exercise to pregnant women with GD; however, evidence-based studies for the extent two-hours walking is beneficial for the improvement of GD treatment is lacking. Hence, this study is designed to compare the quality of life among GD pregnant women who do two-hours walking a day to those with low physical activities. Specifically, the study would like to find out the effect of long walking and low physical activities through a randomized controlled trial consisting of two groups, a group of GD women who performs two-hours walking a day for six weeks then compare their health results in, particular, the improved treatment of GD with a control group who does low physical activities only. Moreover, to find out pregnant GD women perceptions of the quality of life brought by the two-hours walking impact on their health, this study will assess the use and attitude of pregnant GD women towards physical exercise through a qualitative and a quantitative questionnaire and a semi-structured interview.
Research Plan
This proposed study will utilize a mixed-methods design to answer this study’s main question, “Does two hours walking of gestational diabetes (GD) women during pregnancy improve the treatment of GD and quality of life compared to those with low physical activities (PA)?”
To build on the strengths of both quantitative and qualitative approaches, a randomized controlled trial design will be chosen for this study. An emphasis is placed on the quantitative method, while the qualitative section will serve to clarify and expand upon the quantitative results (Creswell 2011). The quantitative methods will be used to develop instruments, measure variables, and statistically assess the impact of two-hours walking of GD women on the treatment of GD during pregnancy to provide valid, objective, and generalizable data. The second, qualitative phase of the study will utilize a semi-structured interview to assess GD women’s’ personal experiences of the quality of life perceived due to high or low physical activities.
Sample Size, power calculations and sample inclusion / exclusion criteria
Approximately 50% of pregnant women with GD in the ____ area are prescribed insulin treatment. To calculate the sample size, it is estimated that the incidence of insulin use would need to be reduced to 25% to be considered statistically significant. For 0.8 power, alpha = 0.05 the sample size has been calculated at N=32 subjects equally randomized into each of the two groups.
So the subjects will be 32 otherwise healthy, non-smoking pregnant women with a documented diagnosis of GD between week 26 and 32 of gestation. Clearance to enter the study will be provided by the physician monitoring each subject’s pregnancy. All subjects will be provided with written informed consent before entering the study.
Subjects will be recruited from the Diabetic Outpatient Clinics at the _____Hospital.
The Inclusion criteria for the study will include the maternal age between 20-40 years, gestational age between 26-32 weeks, body mass index below 40, and non-smokers who are not involved in a regular exercise program. The study design, information form, and informed consent form will be approved by the University of ____ Ethics Board. Site approval will be obtained from both the _____ Hospital.
Subjects will be approached upon their first visit to the Diabetes Outpatient Clinic. Interested individuals will be given an information form and will be asked to complete a medical screening form as well as the informed consent form. Subjects will be randomized using a random numbers table. The allocation will be concealed in sequentially numbered opaque envelopes. Each new subject entering the study will receive the next envelope in sequence.
Inclusion Criteria
Age 20-40 years
Physician diagnosis of GDM
Between 26 and 32 weeks gestation
Pre-pregnant body mass index (BMI) below 40
No current regular exercise regimen
Exclusion Criteria
Clinically significant valvular or ischemic heart disease
Type I diabetes mellitus, peripheral vascular disease, thyroid disease or uncontrolled hypertension, other serious system disorder
An incompetent cervix
A history of two or more spontaneous abortions
Bleeding or placenta previa ruptured membranes, premature labour
Toxemia or pre-eclampsia (current pregnancy)
Evidence of fetal growth retardation (current pregnancy)
Multiple pregnancies (i.e. twins)
The ability to determine the effects of two-hours walking of GD women on the various outcomes will be limited by subjects’ compliance to the diet advice as well as the walking program.
Data Collection
During the Study Protocol
Glucose monitoring will include a daily fasting and 1- or 2-hour postprandial measurements with portable glucometers. Nurse educators will be provided instruction on home glucose monitoring. Instruction in cleaning, calibration, and troubleshooting of the monitors was included in the subjects’ education. Blood glucose values will be recorded in a logbook so that mean blood glucose values could be determined. A random glucose measurement will be taken in a reference laboratory at least once during treatment to ensure the accuracy of the meter.
Insulin therapy will be initiated if at any time during therapy two of the following three values were exceeded:
- Mean fasting > 5.3 m mohl- or Mean 1 -hour postprandial > 7.8 m rnol ‘L- or 2-hour postprandial > 6.7 mm nM,-
- Subjects will be encouraged to continue the walking exercise protocol for the measurement of the secondary endpoints.
Goal of treatment
The goals for treatment will be as follows:
- < 5.3 mmol’L- fasting glucose
- < 7.8 mmol»L-1 hour postprandial glucose
- < 6.7 mmo M / 2 hour postprandial glucose
- >3.5 mmo M / = minimum glucose concentration
Subjects will be instructed to stop the walking exercise and consult the physician if any of the following warning signs or symptoms will occur:
- Shortness of breath
- Palpitations
- Tachycardia
- Difficulty walking
Data Analysis Strategies
The primary endpoint of this study is the requirement for insulin in GD. The number of pregnant women requiring administration of insulin will be compared between the two groups. As the intent is to treat trial, subjects will be analysed according to the groups to which they will be randomized. Secondary endpoints will include the length of time that insulin treatment will be delayed and the amount of insulin that would be administered.
A sub-group analysis will be performed to determine the requirement for insulin between the treatment groups only in women with a body mass index above 25 kg/m2.
A chi-square test will be used to analyse the primary endpoint of the requirement for insulin. The independent samples t-test will be used to analyse the secondary endpoints.
The power to detect a significant difference will be calculated using the Pearson-Hartley chart. Variables that are not normally distributed will be analysed with the Mann- Whitney U-test.
Ethical considerations and Concerns
This study is to be approved by the Human Subjects Committee of the University of _____ prior to commencement of the study (see Appendix A). Permission will then be obtained to recruit subjects from an obstetrician’s clinic in. This clinic serves several obstetricians who oversee the majority of yearly ____ births. The clinic will be the primary investigator with an office out of which to work and in which to store blank and completed questionnaires.
GD pregnant women will be invited and provided with information describing the study and notified that they are under no obligation to participate.
GD pregnant women who indicated that they are interested in participating will be given a clipboard and a pen. The clipboard contained the Letter of Invitation and Letter of Informed Consent (see Appendices B and C). Each interested GD pregnant woman will complete an informed consent document prior to her participation. They participants will be assured that they will be assigned a coded numbers, and that individuals will not be identified by name but by numbers. A potential risk of participation is a breach of privacy by study personnel. In order to safeguard confidentiality, all data will be treated in strict confidence and will be locked in a filing cabinet in the clinic.
Finally, the investigator will always be available at the clinic to answer any questions.
Potential difficulties and Risks
Because no existing research is yet available to answer the research question, “Does two hours walking of gestational diabetes (GD) women during pregnancy improve the treatment of GD and quality of life compared to those with low physical activities (PA)”, a new RCT should be done.
For the quantitative part of this study, low response rate is a potential risk since the participants will be asked to return their completed questionnaires to the clinic. Thus, in an attempt to improve the response rate, several procedures will be conducted (Ransdell, 2006). First, the cover letters were printed on department letterhead, and they were personalized with a hand written note requesting the women’s participation. Second, the questionnaire jackets were printed on bright colored paper (red., blue and yellow) to make the survey more attractive. Third, for those participants not returning the questionnaire, a post-card reminder will be emailed out to participants one week after their second trimester mailing. If the participant did not return the questionnaire within the following week, another second trimester packet will be sent. If the participant still will not return her second trimester questionnaire, her data were coded “did not return,” and she will then be eliminated from the third trimester emailing.
The qualitative semi-structured interview questions on the other hand, may run the risk of reliability and internal validity. Hence, experts from a specific area of specialization—diabetic nutritionists or dieticians from a national certifying organization will critically evaluate the questions.
PPI/E Involvement
GD pregnant women involvement/engagement are applicable to this research proposal. Finding and selectin GD pregnant women involvement for a study can be a challenge. The researcher would have preferred random sampling as preferred for many research strategies, but is very difficult in reality. Because childbearing years are restricted by nature, selecting the population of GD pregnant women to participate was somewhat self-constricting.
Parity was another consideration in selection of GD pregnant women volunteers. Efforts will be made to find the involvement of both parous and non-parous volunteers to ensure that specific feedback concerning the pregnancy experience could be obtained. Women of differing parity involvement is needed to provide a diverse range of experiences with the pregnant condition.
Another involvement feature that will be considered is the choice of sample size. It is necessary for the decision of sample size to take into account various pragmatic factors including population availability, time restrictions, and budget constraints. In consideration of these factors, a sample of 30 GD pregnant women subjects will be chosen as minimum numbers of volunteers to be used in this study (McMillan & Schmacher, 2001, p. 177).
Anticipated Impact
The importance of exercise to pregnant women is evident. Hence, this study may provide valuable insight as to how much walking may improve the treatment and quality of life of GD women during pregnancy. Despite women’s concerns about the impact of exercising during their pregnancy, it contributes positively to women’s health (USDHHS). In addition, the ACOG recommends that healthy pregnant women exercise moderately for at least 15 min per day, 3 to 5 days per week. Thus, to promote physical activity during pregnancy, it is necessary to examine the impact of longer duration of walking during this time. Moreover, it is important to study the impact of exercising and pregnancy because the literature is scant, and it is limited by several factors, including;
- Small sample sizes (Berg, 2009).
- A lack of theoretical research explaining the determinants of exercising during pregnancy (Walker, Cooney, & Riggs, 2000).
- Methodological concerns with using nonstandardized exercise measures (Eisen,
Rield, & Larason, 2001).
Proposed Methods of Dissemination
Walking as an exercise session every other day may prove to be more acceptable to many GD pregnant women than daily insulin injections. In an effort to adhere to the theory guidelines developed by Ajzen and Fishbein (1980) and to achieve the general objective of this proposal, two groups of women studies will be conducted.
Diet Alone Without Walking Exercise
All subjects will be managed with a standard diet that consisted of 40% carbohydrate, 20% protein, and 40% fat to be calculated at 24 to 30 kcal/kg/day divided into three meals and three snacks. A registered dietician will administer each subject’s diet plan. Meal plans will be individualized to account for the ethnic background and food preferences of each subject. Subjects will be monitored weekly to ensure appropriate weight gain.
The women in the diet alone group will be asked not to walk more than an hour a day and to engage in low physical activities only during the remainder of the pregnancy.
Diet Plus Two-hours Walking Exercise
The two-hours walking exercise group will be followed the same standard diet while participating in a two-hours walking exercise program. An experienced instructor will supervise the first three sessions to ensure the safety of participants. Subjects in the walking program group will be instructed to do walking exercise two-hours per day. Subjects will be taught to monitor their heart rate during exercise to ensure that it did not rise above 140 beats per minute. All walking exercise sessions will be recorded in a logbook. Thus, the remainder of this paper will examine the evidence that walking exercise can be used as an alternative treatment to insulin in gestational diabetes.
The researcher proposed dissemination through Obstetrician clinics` and diabetic organizations` websites.
Albaeda MD, Cabellero AC, Badell GC, Piquer SA, Ortiz AT, de Leiva, AL, Corcoy RC. Diabetes and abnormal gucose tolerance in women with previous gestational diabetes. Diabetes Care, 2013; 6:1199-1205.
Artal RA. Exercise and pregnancy. Clinical Obstetrics and Gynecology, 2003a; 377-378.
Artal, RA. Exercise: The alternative therapeutic intervention for gestational diabetes. Clinical Obstetrics and Gynecology, 2003b; 479-487.
Bessinger RC, McMurray RG. Substrate utilization and hormonal responses to exercise in pregnancy. Clinical Obstetrics and Gynecology, 2003; 46: 467-478.
Booth FW, Gordon SE, Carlson CJ, Hamilton MT. Waging war on modern chronic diseases: primary prevention through exercise biology. Journal of Applied Physiology, 2000; 88: 774-787.
Brody SC, Harris RK, Lohr KM. Screening for gestational diabetes: A summary of the evidence for the U. S. Preventive Services Task Force. Obstetrics & Gynecology, 2003; 101: 380-392.
Catalano PM, Kirwan JP, Haygel-deMouzon SJ, King JL. Gestational diabetes and insulin resistance: Role in short and long-term implications for mother and fetus. Journal of Nutrition, 2003; 133:1674S-1683S.
Dye TDV, Knox R, Artal RA, Aubry, RH, Wojtowycx, MA. Physical activity, obesity, and diabetes in pregnancy. American Journal of Epidemiology, 2007; 46: 961-965.
Goodpaster BH, He JC, Watkins SP, Kelley DE. Skeletal muscle lipid content and insulin resistance: evidence for a paradox in endurancetrained athletes. The Journal of Clinical Endocrinology & Metabolism, 2001; 86: 5755-5761.
Jovanovic LB. The role of continuous glucose monitoring in gestational diabetes mellitus. Diabetes Technology & Therapeutics, 2000; 2: S-67-71.
Kennelly MM, Geary MJ, McCaffrey NA, McLoughlin PA, Staine AM, McKenna PG. Exercise-related changes in umbilical and uterine artery waveforms as assessed by Doppler ultrasound scans. American Journal of Obstetrics and Gynecology, 2002; 187: 661-666.
Kim CB, Newton KM, Knopp, RH. Gestational diabetes and the incidence of type 2 diabetes. Diabetes Care, 2002; 25:1862-1868.
National Diabetes Data Group. National Institute of Diabetes and Digestive and Kidney Diseases. Available from: http://diabetes.niddk.nih.gov/dm/pubs/america/citation.htm
Nelson KM, Reiber GC, Boyko EJ. Diet and exercise among adults with type 2 diabetes: Findings from the Third National Health and Nutrition Examination Survey (NHANES III). Diabetes Care, 2002; 25: 1722-1728.
Reusch JEB. Current concepts in insulin resistance, type 2 diabetes mellitus, and the metabolic syndrome. American Journal of Cardiology, 2002; 90:19G-26G.
Verma AG, Boney CM, Tucker RH, Vohr BR. Insulin resistance syndrome in women with prior history of gestational diabetes mellitus. The Journal of Endocrinology & Metabolism, 2002; 87: 3227-3235.
Stuck with your Research Proposal?
Get in touch with one of our experts for instant help!
The Transition of Blues and Jazz Music Into the Modern Hip-Hop Genre, Research Paper Example
The Middlesteins, Essay Example
Time is precious
don’t waste it!
Plagiarism-free guarantee
Privacy guarantee
Secure checkout
Money back guarantee
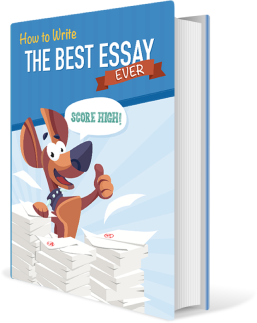
Related Research Proposal Samples & Examples
Censorship of internet users and online protestors in china, research proposal example.
Pages: 6
Words: 1720
Electronic Cigarettes vs Commercial Cigarettes, Research Proposal Example
Pages: 9
Words: 2600
Examining Student Satisfaction With Wholly Online Learning, Research Proposal Example
Pages: 5
Words: 1340
The Incidence of Sexual Harassment in the Workplace, Research Proposal Example
Pages: 10
Words: 2663
To What Extent Should Indigenous Entrepreneurship Be Diversified in Jamaica? Research Proposal Example
Pages: 4
Words: 1064
How Innovation Can Help Business and Be a Factor for Growth, Research Proposal Example
Words: 3382
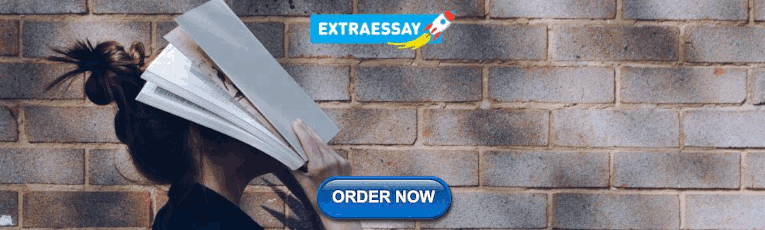
IMAGES
VIDEO
COMMENTS
Current challenges and research gaps in relation to gestational diabetes. Whilst pregnancy only represents a short time window in the life course of a woman, it represents a particularly important window of opportunity to address the risk of diabetes and other NCDs, especially among women affected by gestational diabetes mellitus.
Gestational diabetes mellitus, one of the most common complications of pregnancy, 1,2 affects 6 to 25% of pregnant women (depending on diagnostic criteria) 3,4 and is associated with increased ...
Gestational diabetes is a complex metabolic condition thought to have a strong genetic predisposition. A large genome-wide association study of participants from Finland sheds light on the genetic ...
Women from South Asia (Indian sub-continent) have a high risk of developing gestational diabetes mellitus (GDM) [1,2,3], and are almost four times more likely than other women in Australia to develop GDM.It is defined as glucose intolerance with onset or first recognition during pregnancy [] and affects up to 14-19% of all pregnancies in some populations, such as South Asians, South East ...
Gestational diabetes mellitus (GDM) is a condition associated with pregnancy that engenders additional healthcare demand. A growing body of research includes empirical studies focused on pregnant women's GDM healthcare experiences. The aim of this scoping review is to map findings, highlight gaps and investigate the way research has been conducted into the healthcare experiences of women ...
Diabetes Research and Clinical Practice. Volume 162, April 2020, ... and the proposal for reporting Meta-analysis of Observational Studies in Epidemiology [19]. 2.1. ... gestational diabetes mellitus; OR, odds ratio; GCT, glucose challenge test; OGTT, oral glucose tolerance test; ...
An understanding of the prevalence of gestational diabetes mellitus among pregnant women is essential at local, national and international level so that appropriate health care interventions can be planned, financed and delivered. A systematic review and meta-analysis of primary research reporting the prevalence of gestational diabetes mellitus in Canada or the United States were carried out ...
Objective To investigate the association between gestational diabetes mellitus and adverse outcomes of pregnancy after adjustment for at least minimal confounding factors. Design Systematic review and meta-analysis. Data sources Web of Science, PubMed, Medline, and Cochrane Database of Systematic Reviews, from 1 January 1990 to 1 November 2021. Review methods Cohort studies and control arms of ...
GDM, gestational diabetes mellitus; SGA, small for gestational age; LGA, large for gestational age. Data are n (%) unless otherwise speci fi ed. Li et al. 10.3389/fendo.2023.1158969
Gestational diabetes mellitus (GDM) is the most. common medical complication of pregnancy. It. is associated with maternal and neonatal adverse. outcomes. Maintaining adequate blood glucose levels ...
1 Mater Research, The University of Queensland, South Brisbane, QLD, Australia; 2 World Diabetes Foundation, Bagsvaerd, Denmark; 3 Divakars Specialty Hospital, Bengaluru, India; 4 Mor Women's Health Care Center, Tel Aviv, Israel; Gestational diabetes mellitus (GDM) is the commonest medical complication of pregnancy. The association of GDM with immediate pregnancy complications including ...
Objective To compare the hazards of incident diabetes among those with gestational diabetes in the first, in the second, and in both pregnancies with women without gestational diabetes in either. Design, Setting, and Participants This was a retrospective cohort study with cohort inception from April 1, 1990, to December 31, 2012.
Gestational diabetes is diabetes first detected during pregnancy. This disorder is associated with. pregnancy complications that can damage the health of mother and baby. The placenta joins mother ...
The purpose of this review is to investigate the relationship between gastrointestinal microbiome, obesity, and gestational diabetes mellitus (GDM) in an objective manner. We conducted a thorough ...
Gestational Diabetes Mellitus studies. The prevalence of gestational diabetes mellitus is influenced by the race or ethnicity, age, and obesity among women in the delivery population as well as the criteria used to make the diagnosis. Our researchers study the causes, genetics, treatment, and outcomes of women with gestational diabetes and ...
Published by Elsevier Inc. 1 TITLE: Impact of Hypertensive Disorders of Pregnancy and Gestational Diabetes Mellitus 1 on Offspring Cardiovascular Health in Early Adolescence 2 3 AUTHORS: Kartik K ... (NUCATS) 35 Institute, Northwestern Memorial Foundation Dixon Translational Research Grant, American 36 Heart Association 17SFRN33660752 ...
To examine the relationship between gestational glucose intolerance (GGI) and neonatal hypoglycemia. RESEARCH DESIGN AND METHODS This was a secondary analysis of 8,262 mother-infant dyads, with delivery at two hospitals between 2014 and 2023.
A large study of women with two singleton pregnancies found an association between gestational diabetes and an increased risk for subsequent diabetes, according to a study in JAMA Network Open.The risk was 15.8 times higher for women with gestational diabetes in both pregnancies and 4.35 times higher for women with gestational diabetes only in the first pregnancy.
The human microbiome, a complex ecosystem of bacteria, viruses, and protozoans living in symbiosis with the host, plays a crucial role in human health, influencing everything from metabolism to immune function. Dysbiosis, or an imbalance in this ecosystem, has been linked to various health issues, including diabetes and gestational diabetes (GD). In diabetes, dysbiosis affects the function of ...
Gestational diabetes happens when your body can't make enough insulin during your pregnancy. Insulin is a hormone made by your pancreas. It acts like a key to let blood sugar into the cells in your body for use as energy. During pregnancy, your body makes more hormones and goes through other changes, such as weight gain.
Background: Glyoxalase 1 (GLO1) plays a crucial role in defending against glycation.Single nucleotide polymorphism (SNP) variants in the GLO1 gene may affect gene expression and alter enzyme activity. However, there have been limited studies evaluating the association between GLO1 and diabetes, especially gestational diabetes mellitus (GDM). ). Therefore, this study is the first to explore the ...
Gestational diabetes in high risk women in Assiut Ahmed et al. 427 Mothers may have a considerably elevated risk of preeclampsia, CS, and type 2 DM later on [2].
Background. Gestational Diabetes (GD) is the most common medical condition in pregnancy (Artal, 2003a; Dye, Knox, Artal, Aubry, & Wojtowycx, 2007). GD is defined as an "abnormal carbohydrate intolerance of variable severity that is first manifested during pregnancy and ends with childbirth" (Artal, 2003b; Catalano, Kirwan, Hagel-de Mouzon ...