
Data visualization is the representation of data through use of common graphics, such as charts, plots, infographics and even animations. These visual displays of information communicate complex data relationships and data-driven insights in a way that is easy to understand.
Data visualization can be utilized for a variety of purposes, and it’s important to note that is not only reserved for use by data teams. Management also leverages it to convey organizational structure and hierarchy while data analysts and data scientists use it to discover and explain patterns and trends. Harvard Business Review (link resides outside ibm.com) categorizes data visualization into four key purposes: idea generation, idea illustration, visual discovery, and everyday dataviz. We’ll delve deeper into these below:
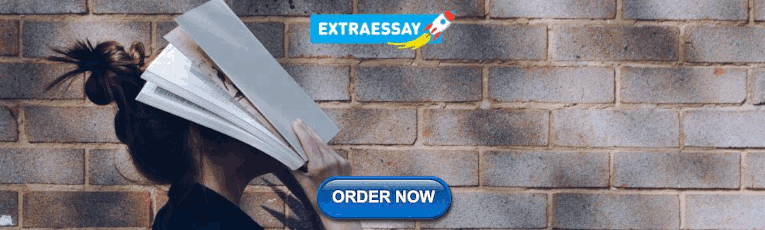
Idea generation
Data visualization is commonly used to spur idea generation across teams. They are frequently leveraged during brainstorming or Design Thinking sessions at the start of a project by supporting the collection of different perspectives and highlighting the common concerns of the collective. While these visualizations are usually unpolished and unrefined, they help set the foundation within the project to ensure that the team is aligned on the problem that they’re looking to address for key stakeholders.
Idea illustration
Data visualization for idea illustration assists in conveying an idea, such as a tactic or process. It is commonly used in learning settings, such as tutorials, certification courses, centers of excellence, but it can also be used to represent organization structures or processes, facilitating communication between the right individuals for specific tasks. Project managers frequently use Gantt charts and waterfall charts to illustrate workflows . Data modeling also uses abstraction to represent and better understand data flow within an enterprise’s information system, making it easier for developers, business analysts, data architects, and others to understand the relationships in a database or data warehouse.
Visual discovery
Visual discovery and every day data viz are more closely aligned with data teams. While visual discovery helps data analysts, data scientists, and other data professionals identify patterns and trends within a dataset, every day data viz supports the subsequent storytelling after a new insight has been found.
Data visualization
Data visualization is a critical step in the data science process, helping teams and individuals convey data more effectively to colleagues and decision makers. Teams that manage reporting systems typically leverage defined template views to monitor performance. However, data visualization isn’t limited to performance dashboards. For example, while text mining an analyst may use a word cloud to to capture key concepts, trends, and hidden relationships within this unstructured data. Alternatively, they may utilize a graph structure to illustrate relationships between entities in a knowledge graph. There are a number of ways to represent different types of data, and it’s important to remember that it is a skillset that should extend beyond your core analytics team.
Use this model selection framework to choose the most appropriate model while balancing your performance requirements with cost, risks and deployment needs.
Register for the ebook on generative AI
The earliest form of data visualization can be traced back the Egyptians in the pre-17th century, largely used to assist in navigation. As time progressed, people leveraged data visualizations for broader applications, such as in economic, social, health disciplines. Perhaps most notably, Edward Tufte published The Visual Display of Quantitative Information (link resides outside ibm.com), which illustrated that individuals could utilize data visualization to present data in a more effective manner. His book continues to stand the test of time, especially as companies turn to dashboards to report their performance metrics in real-time. Dashboards are effective data visualization tools for tracking and visualizing data from multiple data sources, providing visibility into the effects of specific behaviors by a team or an adjacent one on performance. Dashboards include common visualization techniques, such as:
- Tables: This consists of rows and columns used to compare variables. Tables can show a great deal of information in a structured way, but they can also overwhelm users that are simply looking for high-level trends.
- Pie charts and stacked bar charts: These graphs are divided into sections that represent parts of a whole. They provide a simple way to organize data and compare the size of each component to one other.
- Line charts and area charts: These visuals show change in one or more quantities by plotting a series of data points over time and are frequently used within predictive analytics. Line graphs utilize lines to demonstrate these changes while area charts connect data points with line segments, stacking variables on top of one another and using color to distinguish between variables.
- Histograms: This graph plots a distribution of numbers using a bar chart (with no spaces between the bars), representing the quantity of data that falls within a particular range. This visual makes it easy for an end user to identify outliers within a given dataset.
- Scatter plots: These visuals are beneficial in reveling the relationship between two variables, and they are commonly used within regression data analysis. However, these can sometimes be confused with bubble charts, which are used to visualize three variables via the x-axis, the y-axis, and the size of the bubble.
- Heat maps: These graphical representation displays are helpful in visualizing behavioral data by location. This can be a location on a map, or even a webpage.
- Tree maps, which display hierarchical data as a set of nested shapes, typically rectangles. Treemaps are great for comparing the proportions between categories via their area size.
Access to data visualization tools has never been easier. Open source libraries, such as D3.js, provide a way for analysts to present data in an interactive way, allowing them to engage a broader audience with new data. Some of the most popular open source visualization libraries include:
- D3.js: It is a front-end JavaScript library for producing dynamic, interactive data visualizations in web browsers. D3.js (link resides outside ibm.com) uses HTML, CSS, and SVG to create visual representations of data that can be viewed on any browser. It also provides features for interactions and animations.
- ECharts: A powerful charting and visualization library that offers an easy way to add intuitive, interactive, and highly customizable charts to products, research papers, presentations, etc. Echarts (link resides outside ibm.com) is based in JavaScript and ZRender, a lightweight canvas library.
- Vega: Vega (link resides outside ibm.com) defines itself as “visualization grammar,” providing support to customize visualizations across large datasets which are accessible from the web.
- deck.gl: It is part of Uber's open source visualization framework suite. deck.gl (link resides outside ibm.com) is a framework, which is used for exploratory data analysis on big data. It helps build high-performance GPU-powered visualization on the web.
With so many data visualization tools readily available, there has also been a rise in ineffective information visualization. Visual communication should be simple and deliberate to ensure that your data visualization helps your target audience arrive at your intended insight or conclusion. The following best practices can help ensure your data visualization is useful and clear:
Set the context: It’s important to provide general background information to ground the audience around why this particular data point is important. For example, if e-mail open rates were underperforming, we may want to illustrate how a company’s open rate compares to the overall industry, demonstrating that the company has a problem within this marketing channel. To drive an action, the audience needs to understand how current performance compares to something tangible, like a goal, benchmark, or other key performance indicators (KPIs).
Know your audience(s): Think about who your visualization is designed for and then make sure your data visualization fits their needs. What is that person trying to accomplish? What kind of questions do they care about? Does your visualization address their concerns? You’ll want the data that you provide to motivate people to act within their scope of their role. If you’re unsure if the visualization is clear, present it to one or two people within your target audience to get feedback, allowing you to make additional edits prior to a large presentation.
Choose an effective visual: Specific visuals are designed for specific types of datasets. For instance, scatter plots display the relationship between two variables well, while line graphs display time series data well. Ensure that the visual actually assists the audience in understanding your main takeaway. Misalignment of charts and data can result in the opposite, confusing your audience further versus providing clarity.
Keep it simple: Data visualization tools can make it easy to add all sorts of information to your visual. However, just because you can, it doesn’t mean that you should! In data visualization, you want to be very deliberate about the additional information that you add to focus user attention. For example, do you need data labels on every bar in your bar chart? Perhaps you only need one or two to help illustrate your point. Do you need a variety of colors to communicate your idea? Are you using colors that are accessible to a wide range of audiences (e.g. accounting for color blind audiences)? Design your data visualization for maximum impact by eliminating information that may distract your target audience.
An AI-infused integrated planning solution that helps you transcend the limits of manual planning.
Build, run and manage AI models. Prepare data and build models on any cloud using open source code or visual modeling. Predict and optimize your outcomes.
Unlock the value of enterprise data and build an insight-driven organization that delivers business advantage with IBM Consulting.
Your trusted Watson co-pilot for smarter analytics and confident decisions.
Use features within IBM Watson® Studio that help you visualize and gain insights into your data, then cleanse and transform your data to build high-quality predictive models.
Data Refinery makes it easy to explore, prepare, and deliver data that people across your organization can trust.
Learn how to use Apache Superset (a modern, enterprise-ready business intelligence web application) with Netezza database to uncover the story behind the data.
Predict outcomes with flexible AI-infused forecasting and analyze what-if scenarios in real-time. IBM Planning Analytics is an integrated business planning solution that turns raw data into actionable insights. Deploy as you need, on premises or on cloud.
We use essential cookies to make Venngage work. By clicking “Accept All Cookies”, you agree to the storing of cookies on your device to enhance site navigation, analyze site usage, and assist in our marketing efforts.
Manage Cookies
Cookies and similar technologies collect certain information about how you’re using our website. Some of them are essential, and without them you wouldn’t be able to use Venngage. But others are optional, and you get to choose whether we use them or not.
Strictly Necessary Cookies
These cookies are always on, as they’re essential for making Venngage work, and making it safe. Without these cookies, services you’ve asked for can’t be provided.
Show cookie providers
- Google Login
Functionality Cookies
These cookies help us provide enhanced functionality and personalisation, and remember your settings. They may be set by us or by third party providers.
Performance Cookies
These cookies help us analyze how many people are using Venngage, where they come from and how they're using it. If you opt out of these cookies, we can’t get feedback to make Venngage better for you and all our users.
- Google Analytics
Targeting Cookies
These cookies are set by our advertising partners to track your activity and show you relevant Venngage ads on other sites as you browse the internet.
- Google Tag Manager
Infographics
- Daily Infographics
- Popular Templates
- Accessibility
- Graphic Design
- Graphs and Charts
- Data Visualization
- Human Resources
- Beginner Guides
Blog Graphic Design What is Data Visualization? (Definition, Examples, Best Practices)
What is Data Visualization? (Definition, Examples, Best Practices)
Written by: Midori Nediger Jun 05, 2020
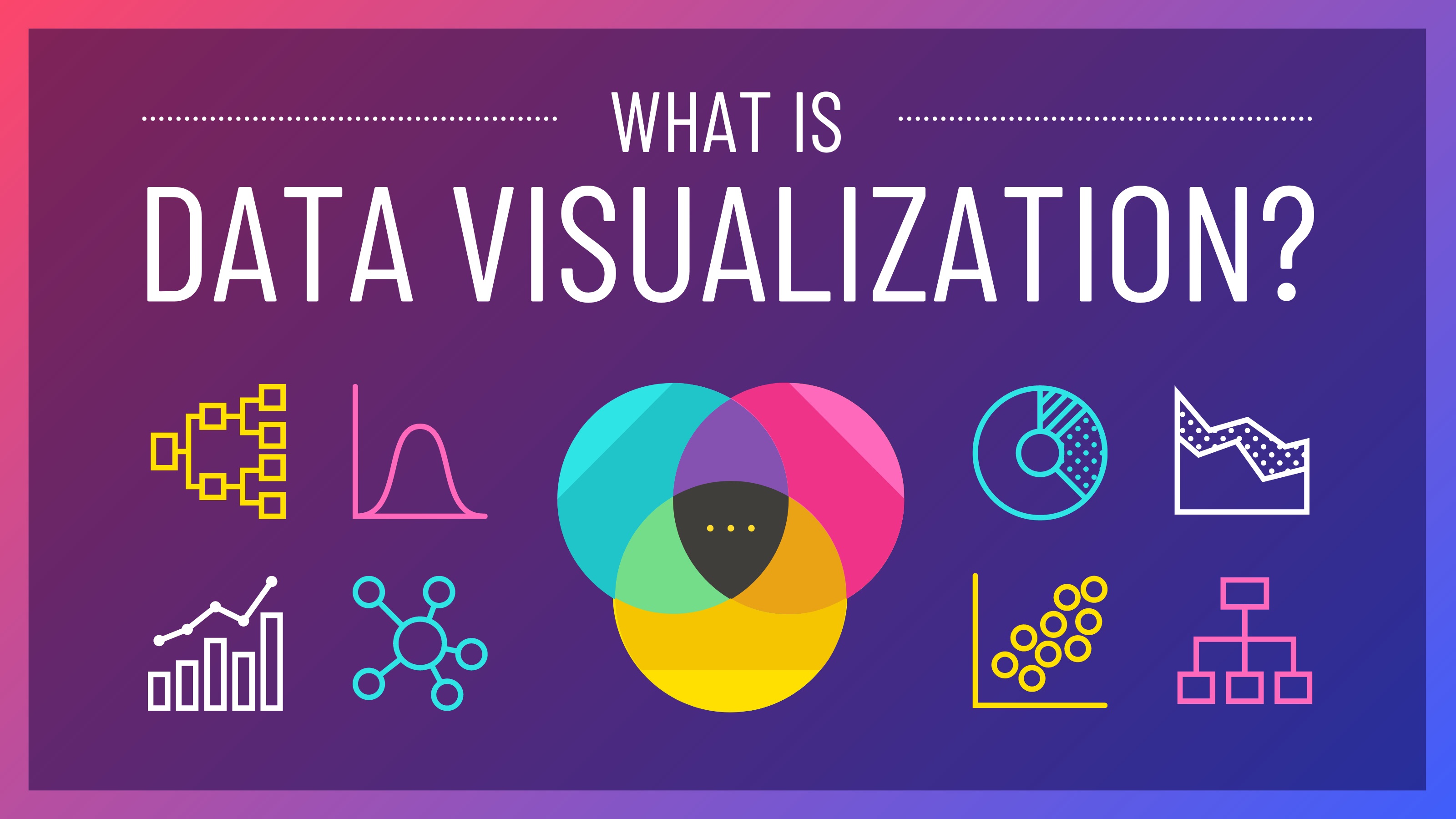
Words don’t always paint the clearest picture. Raw data doesn’t always tell the most compelling story.
The human mind is very receptive to visual information. That’s why data visualization is a powerful tool for communication.
But if “data visualization” sounds tricky and technical don’t worry—it doesn’t have to be.
This guide will explain the fundamentals of data visualization in a way that anyone can understand. Included are a ton of examples of different types of data visualizations and when to use them for your reports, presentations, marketing, and more.
Table of Contents
- What is data visualization?
What is data visualization used for?
Types of data visualizations.
- How to present data visually (for businesses, marketers, nonprofits, and education)
- Data visualization examples
Data visualization is used everywhere.
Businesses use data visualization for reporting, forecasting, and marketing.
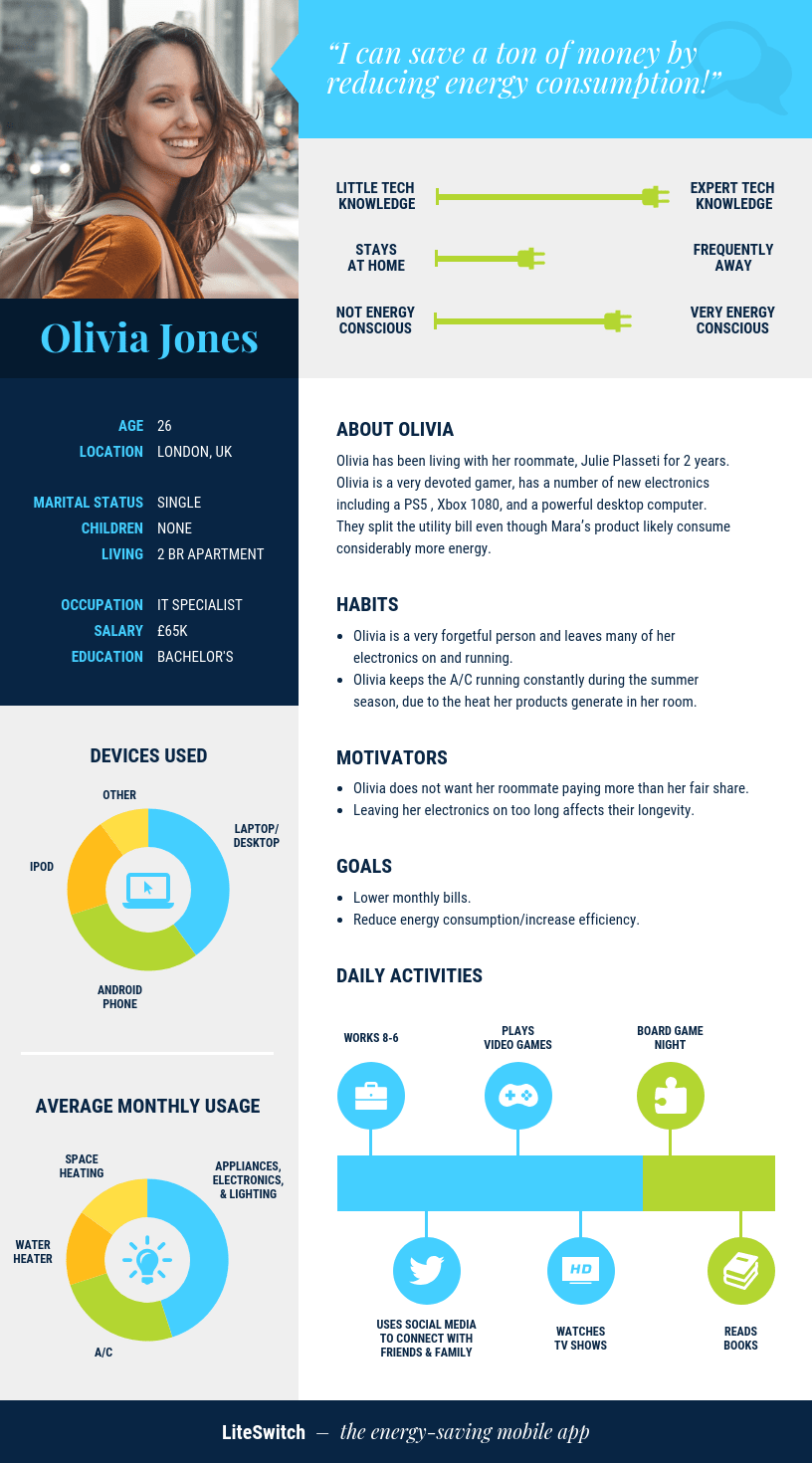
CREATE THIS REPORT TEMPLATE
Nonprofits use data visualizations to put stories and faces to numbers.
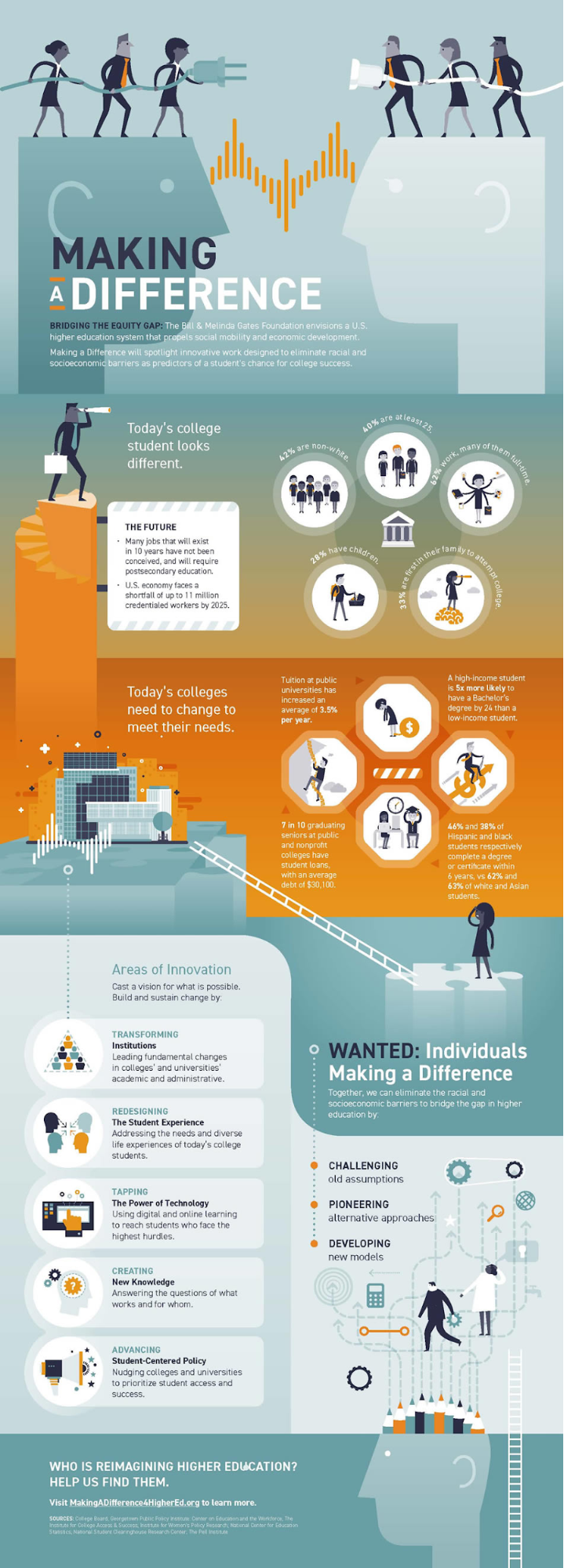
Source: Bill and Melinda Gates Foundation
Scholars and scientists use data visualization to illustrate concepts and reinforce their arguments.
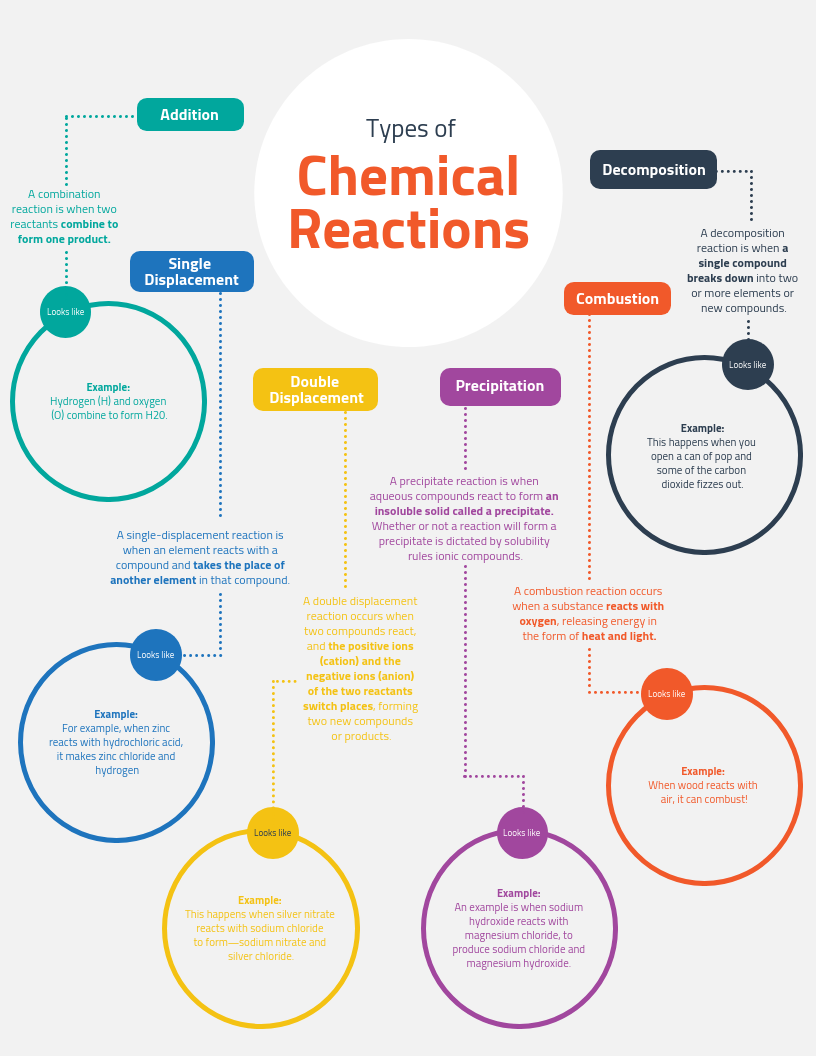
CREATE THIS MIND MAP TEMPLATE
Reporters use data visualization to show trends and contextualize stories.
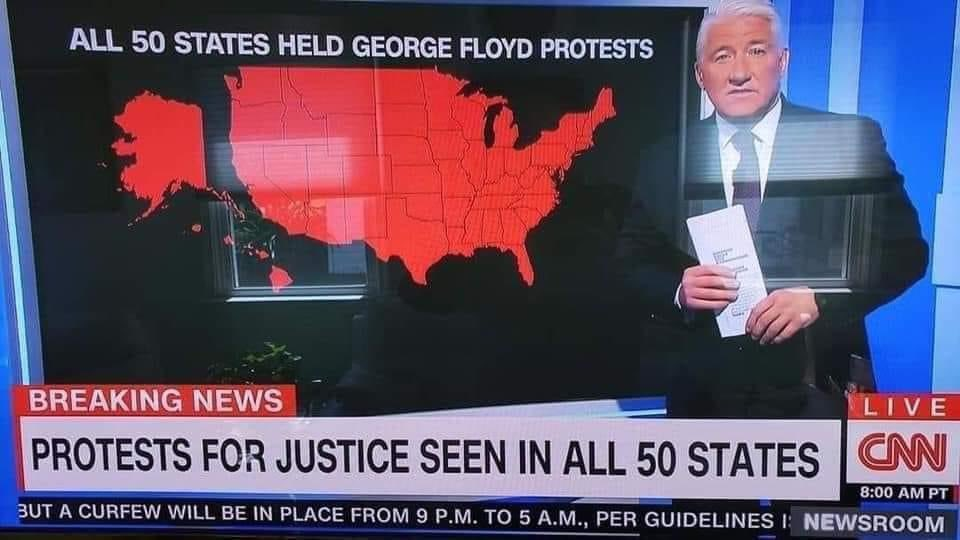
While data visualizations can make your work more professional, they can also be a lot of fun.
What is data visualization? A simple definition of data visualization:
Data visualization is the visual presentation of data or information. The goal of data visualization is to communicate data or information clearly and effectively to readers. Typically, data is visualized in the form of a chart , infographic , diagram or map.
The field of data visualization combines both art and data science. While a data visualization can be creative and pleasing to look at, it should also be functional in its visual communication of the data.
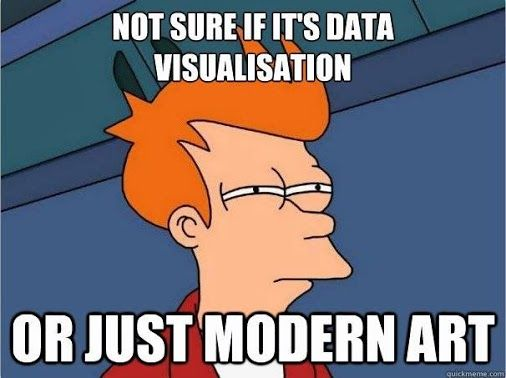
Data, especially a lot of data, can be difficult to wrap your head around. Data visualization can help both you and your audience interpret and understand data.
Data visualizations often use elements of visual storytelling to communicate a message supported by the data.
There are many situations where you would want to present data visually.
Data visualization can be used for:
- Making data engaging and easily digestible
- Identifying trends and outliers within a set of data
- Telling a story found within the data
- Reinforcing an argument or opinion
- Highlighting the important parts of a set of data
Let’s look at some examples for each use case.
1. Make data digestible and easy to understand
Often, a large set of numbers can make us go cross-eyed. It can be difficult to find the significance behind rows of data.
Data visualization allows us to frame the data differently by using illustrations, charts, descriptive text, and engaging design. Visualization also allows us to group and organize data based on categories and themes, which can make it easier to break down into understandable chunks.
Related : How to Use Data Visualization in Your Infographics
For example, this infographic breaks down the concept of neuroplasticity in an approachable way:
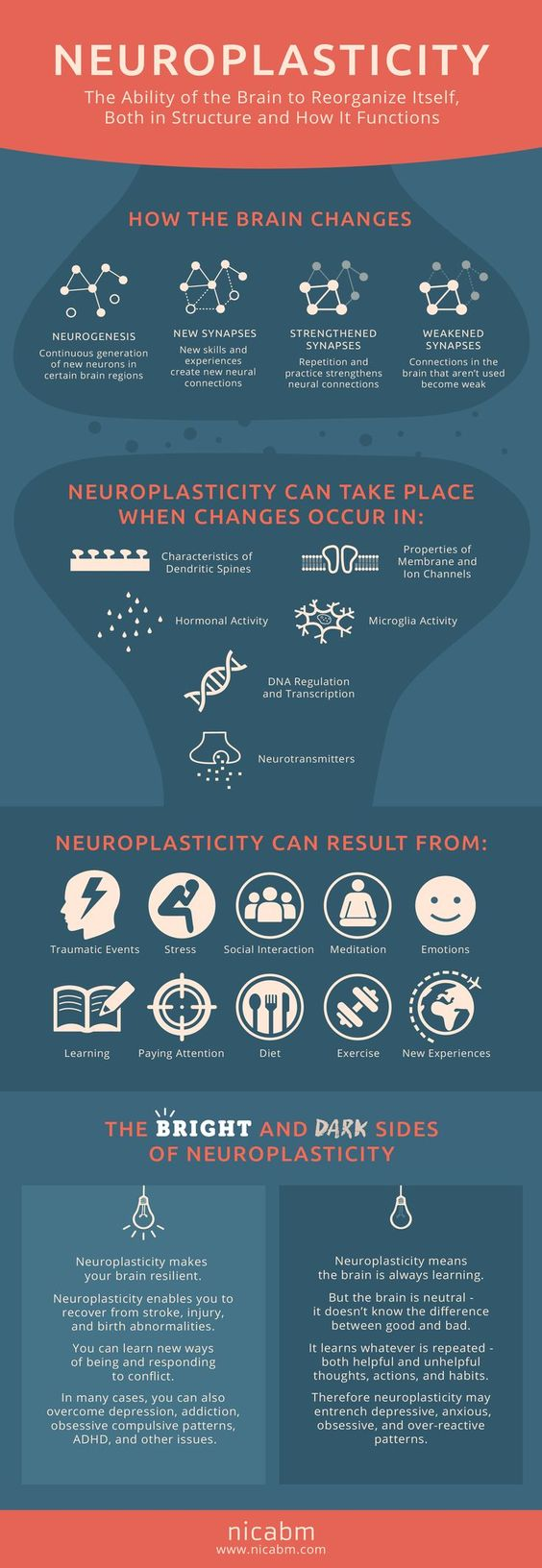
Source: NICABM
The same goes for complex, specialized concepts. It can often be difficult to break down the information in a way that non-specialists will understand. But an infographic that organizes the information, with visuals, can demystify concepts for novice readers.
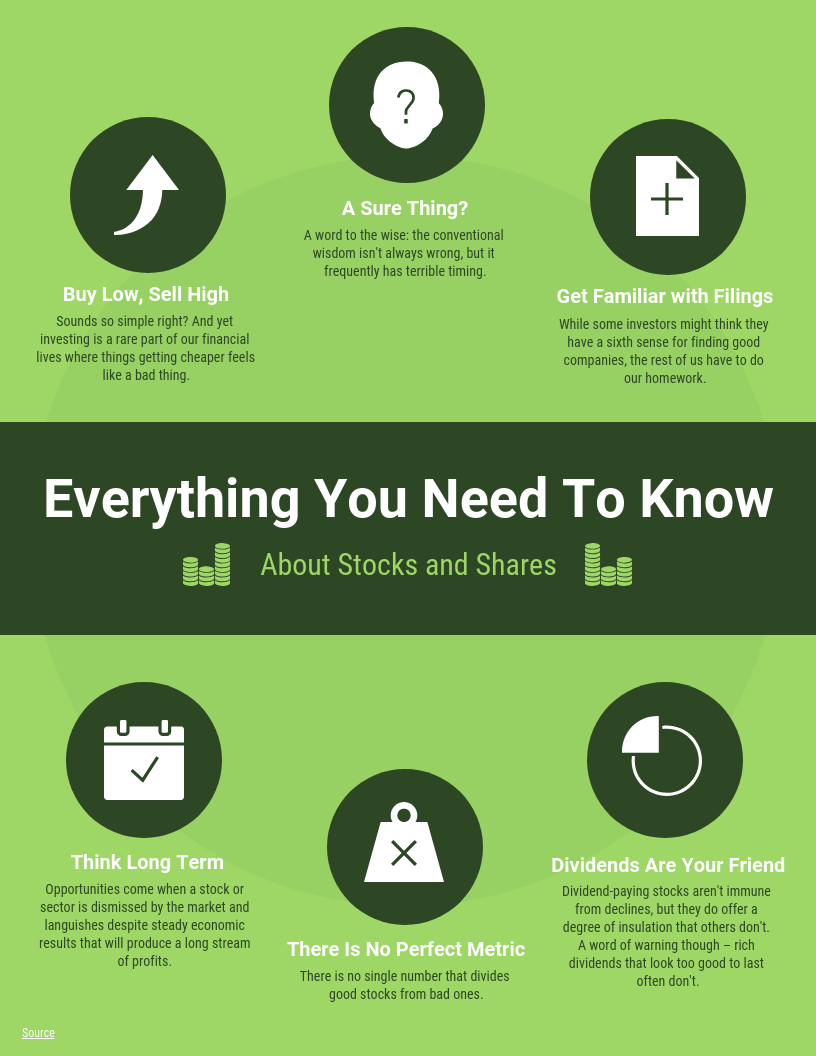
CREATE THIS INFOGRAPHIC TEMPLATE
NEW! Introducing: Marketing Statistics Report 2022
It’s 2022 already. Marketers, are you still using data from pre-COVID times?
Don’t make decisions based on outdated data that no longer applies. It’s time you keep yourself informed of the latest marketing statistics and trends during the past two years, and learn how COVID-19 has affected marketing efforts in different industries — with this FREE marketing statistics report put together by Venngage and HubSpot .
The report uses data gathered from over 100,000 customers of HubSpot CRM. In addition to that, you’ll also know about the trends in using visuals in content marketing and the impacts of the pandemic on visual content, from 200+ marketers all over the world interviewed by Venngage.
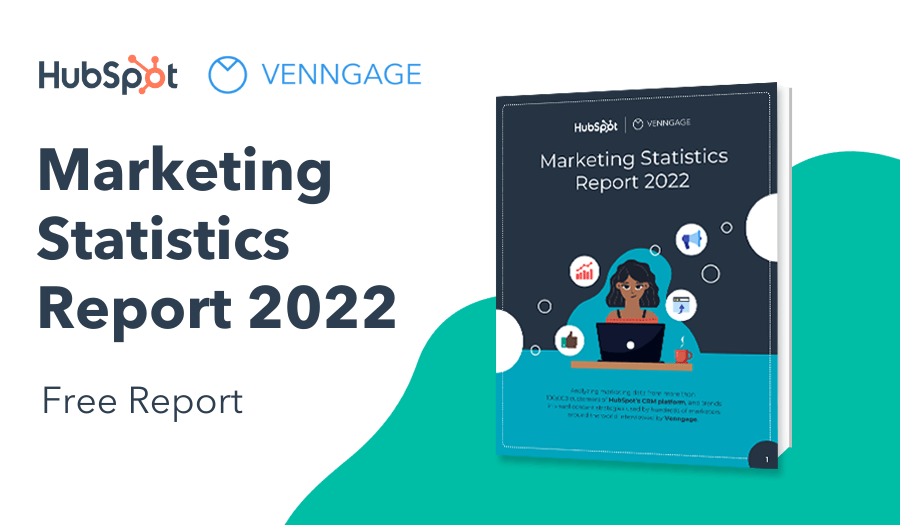
GET YOUR FREE COPY
2. Identify trends and outliers
If you were to sift through raw data manually, it could take ages to notice patterns, trends or outlying data. But by using data visualization tools like charts, you can sort through a lot of data quickly.
Even better, charts enable you to pick up on trends a lot quicker than you would sifting through numbers.
For example, here’s a simple chart generated by Google Search Console that shows the change in Google searches for “toilet paper”. As you can see, in March 2020 there was a huge increase in searches for toilet paper:
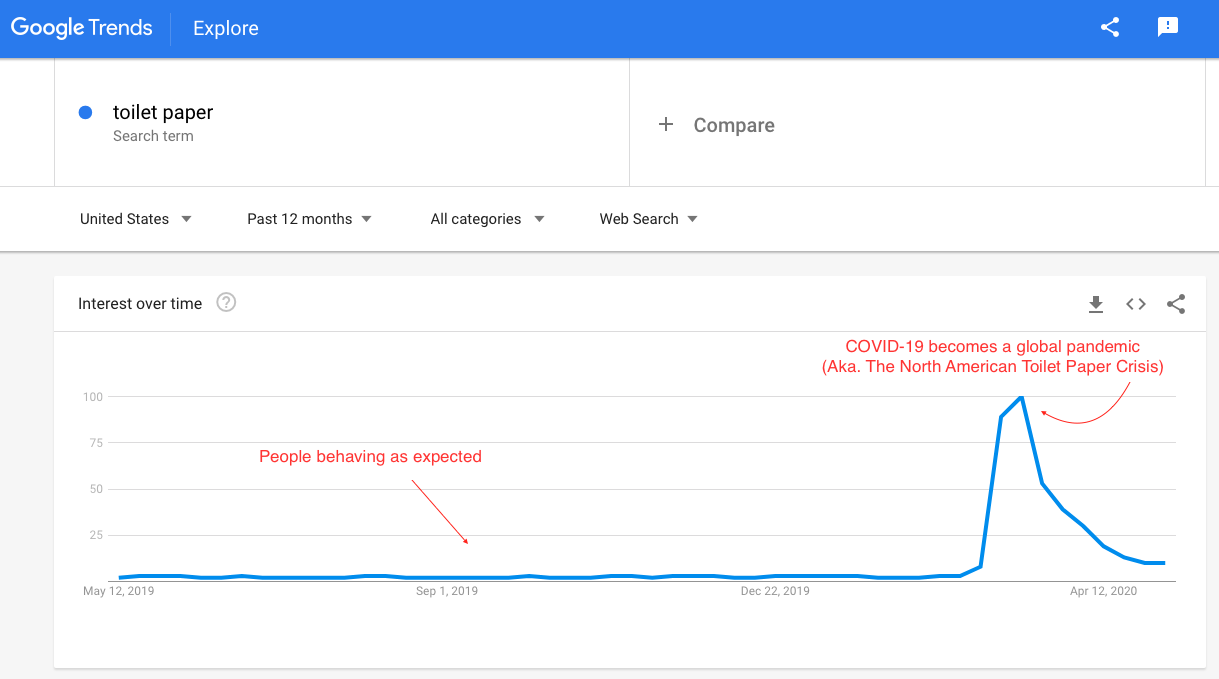
Source: How to Use SEO Data to Fuel Your Content Marketing Strategy in 2020
This chart shows an outlier in the general trend for toilet paper-related Google searches. The reason for the outlier? The outbreak of COVID-19 in North America. With a simple data visualization, we’ve been able to highlight an outlier and hint at a story behind the data.
Uploading your data into charts, to create these kinds of visuals is easy. While working on your design in the editor, select a chart from the left panel. Open the chart and find the green IMPORT button under the DATA tab. Then upload the CSV file and your chart automatically visualizes the information.
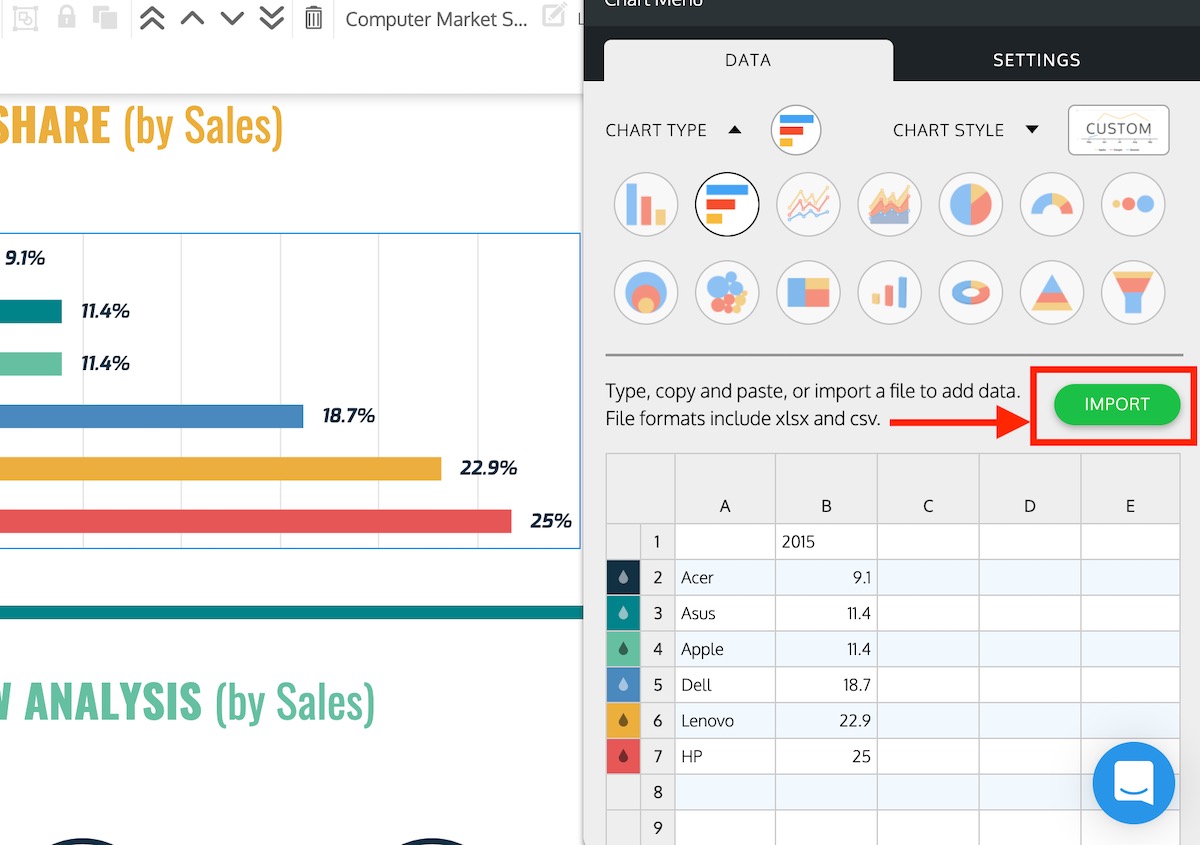
3. Tell a story within the data
Numbers on their own don’t tend to evoke an emotional response. But data visualization can tell a story that gives significance to the data.
Designers use techniques like color theory , illustrations, design style and visual cues to appeal to the emotions of readers, put faces to numbers, and introduce a narrative to the data.
Related : How to Tell a Story With Data (A Guide for Beginners)
For example, here’s an infographic created by World Vision. In the infographics, numbers are visualized using illustrations of cups. While comparing numbers might impress readers, reinforcing those numbers with illustrations helps to make an even greater impact.
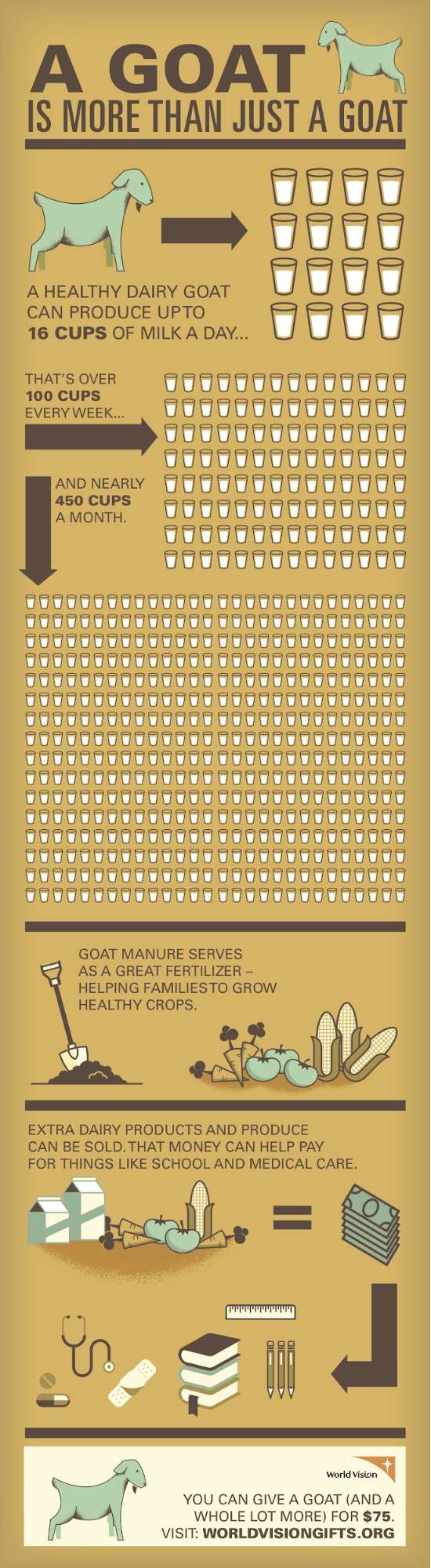
Source: World Vision
Meanwhile, this infographic uses data to draw attention to an often overlooked issue:

Read More: The Coronavirus Pandemic and the Refugee Crisis
4. Reinforce an argument or opinion
When it comes to convincing people your opinion is right, they often have to see it to believe it. An effective infographic or chart can make your argument more robust and reinforce your creativity.
For example, you can use a comparison infographic to compare sides of an argument, different theories, product/service options, pros and cons, and more. Especially if you’re blending data types.
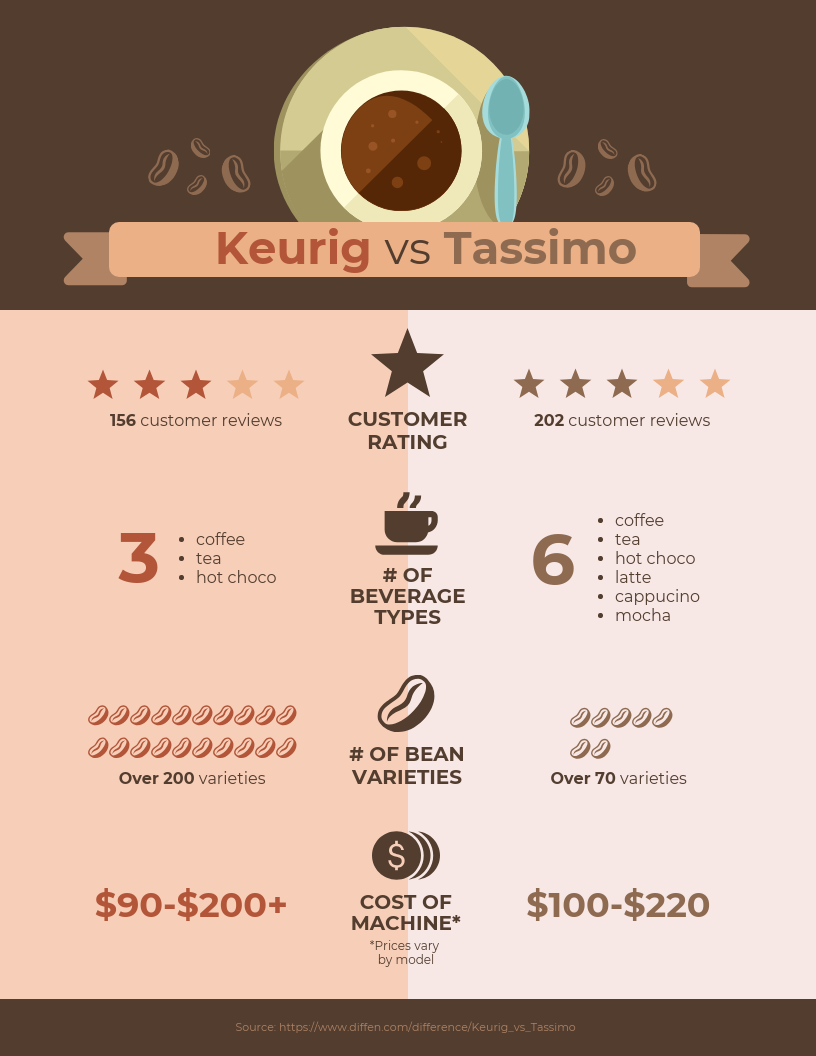
5. Highlight an important point in a set of data
Sometimes we use data visualizations to make it easier for readers to explore the data and come to their own conclusions. But often, we use data visualizations to tell a story, make a particular argument, or encourage readers to come to a specific conclusion.
Designers use visual cues to direct the eye to different places on a page. Visual cues are shapes, symbols, and colors that point to a specific part of the data visualization, or that make a specific part stand out.
For example, in this data visualization, contrasting colors are used to emphasize the difference in the amount of waste sent to landfills versus recycled waste:
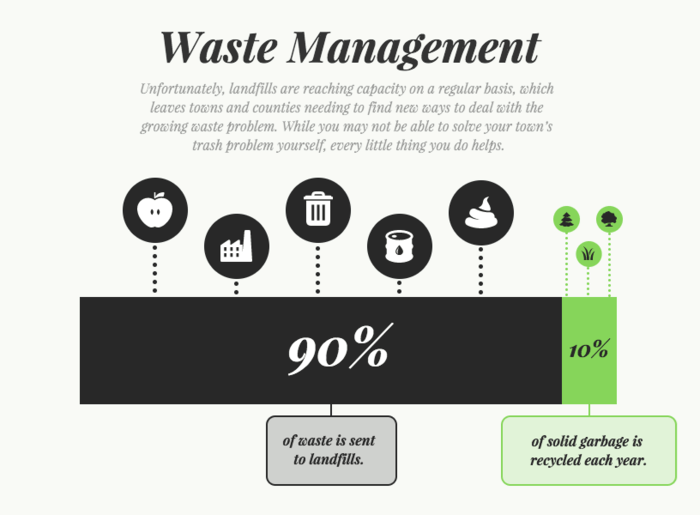
Here’s another example. This time, a red circle and an arrow are used to highlight points on the chart where the numbers show a drop:
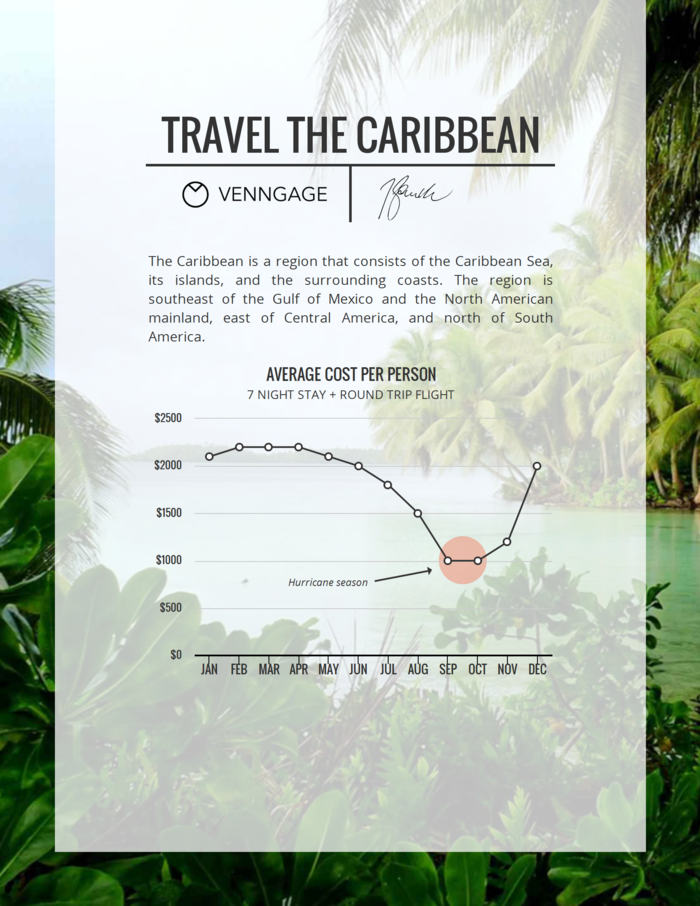
Highlighting specific data points helps your data visualization tell a compelling story.
6. Make books, blog posts, reports and videos more engaging
At Venngage, we use data visualization to make our blog posts more engaging for readers. When we write a blog post or share a post on social media, we like to summarize key points from our content using infographics.
The added benefit of creating engaging visuals like infographics is that it has enabled our site to be featured in publications like The Wall Street Journal , Mashable , Business Insider , The Huffington Post and more.
That’s because data visualizations are different from a lot of other types of content people consume on a daily basis. They make your brain work. They combine concrete facts and numbers with impactful visual elements. They make complex concepts easier to grasp.
Here’s an example of an infographic we made that got a lot of media buzz:
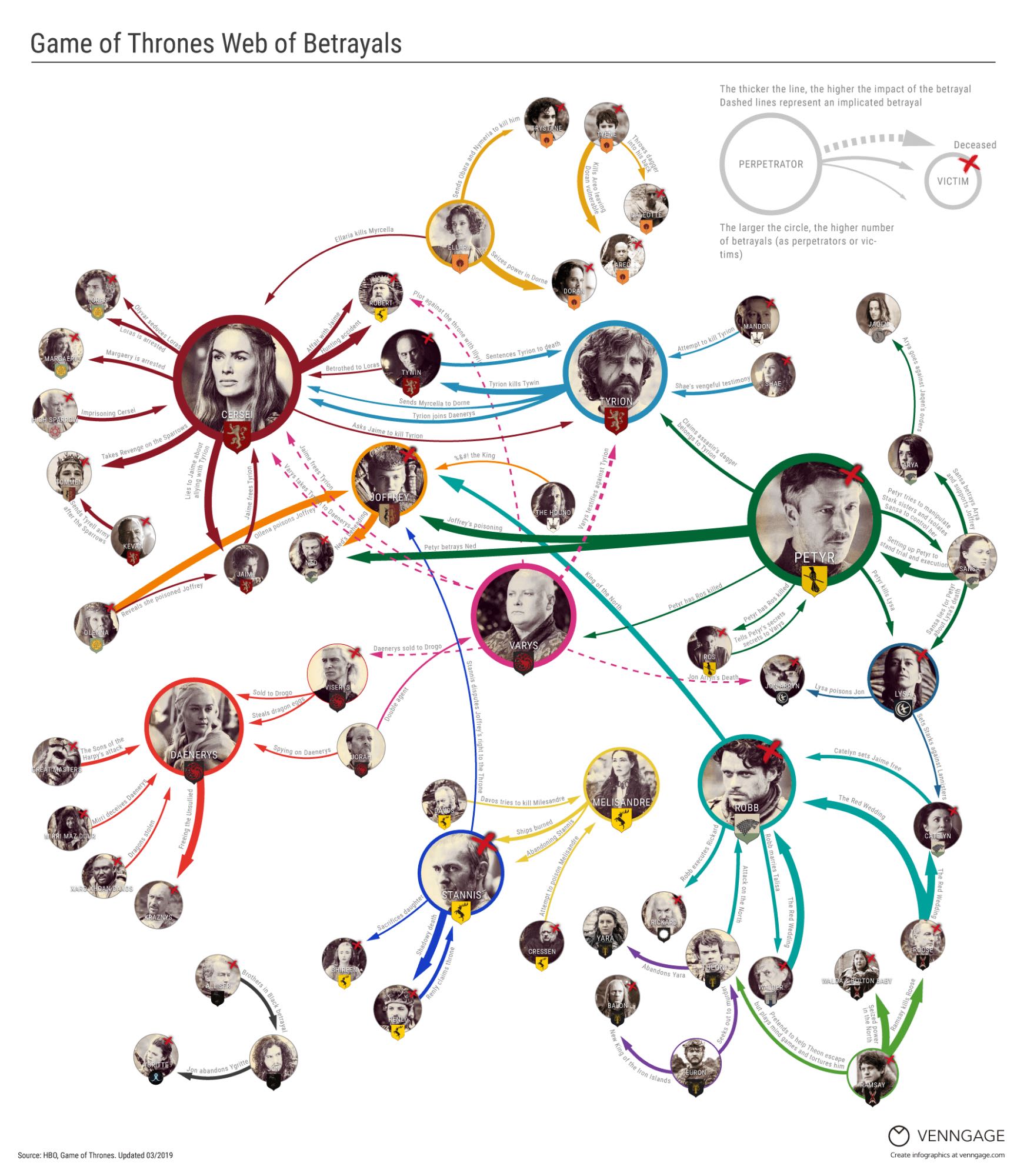
Read the Blog Post: Every Betrayal Ever in Game of Thrones
We created this infographic because a bunch of people on our team are big Game of Thrones fans and we wanted to create a visual that would help other fans follow the show. Because we approached a topic that a lot of people cared about in an original way, the infographic got picked up by a bunch of media sites.
Whether you’re a website looking to promote your content, a journalist looking for an original angle, or a creative building your portfolio, data visualizations can be an effective way to get people’s attention.
Data visualizations can come in many different forms. People are always coming up with new and creative ways to present data visually.
Generally speaking, data visualizations usually fall under these main categories:
An infographic is a collection of imagery, charts, and minimal text that gives an easy-to-understand overview of a topic.
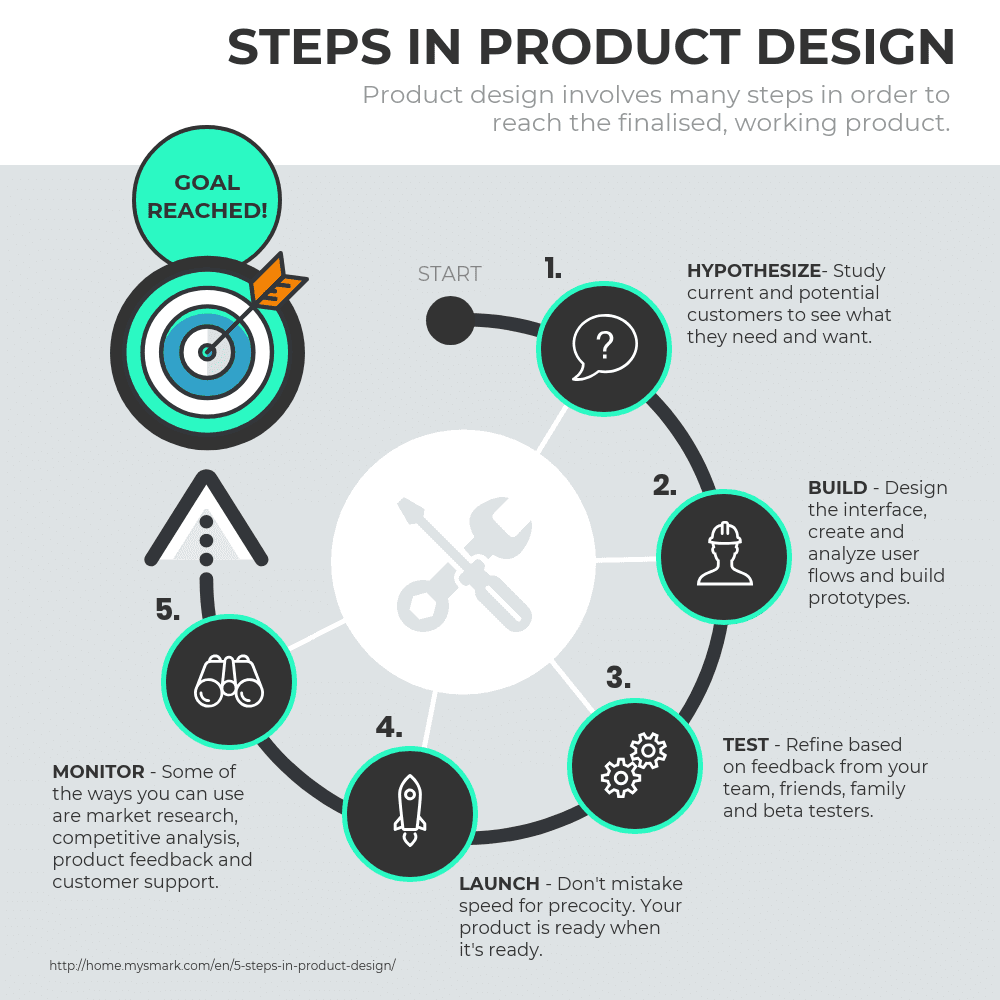
While infographics can take many forms, they can typically be categorized by these infographic types:
- Statistical infographics
- Informational infographics
- Timeline infographics
- Process infographics
- Geographic infographics
- Comparison infographics
- Hierarchical infographics
- List infographics
- Resume infographics
Read More: What is an Infographic? Examples, Templates & Design Tips
Charts
In the simplest terms, a chart is a graphical representation of data. Charts use visual symbols like line, bars, dots, slices, and icons to represent data points.
Some of the most common types of charts are:
- Bar graphs /charts
- Line charts
- Bubble charts
- Stacked bar charts
- Word clouds
- Pictographs
- Area charts
- Scatter plot charts
- Multi-series charts
The question that inevitably follows is: what type of chart should I use to visualize my data? Does it matter?
Short answer: yes, it matters. Choosing a type of chart that doesn’t work with your data can end up misrepresenting and skewing your data.
For example: if you’ve been in the data viz biz for a while, then you may have heard some of the controversy surrounding pie charts. A rookie mistake that people often make is using a pie chart when a bar chart would work better.
Pie charts display portions of a whole. A pie chart works when you want to compare proportions that are substantially different. Like this:
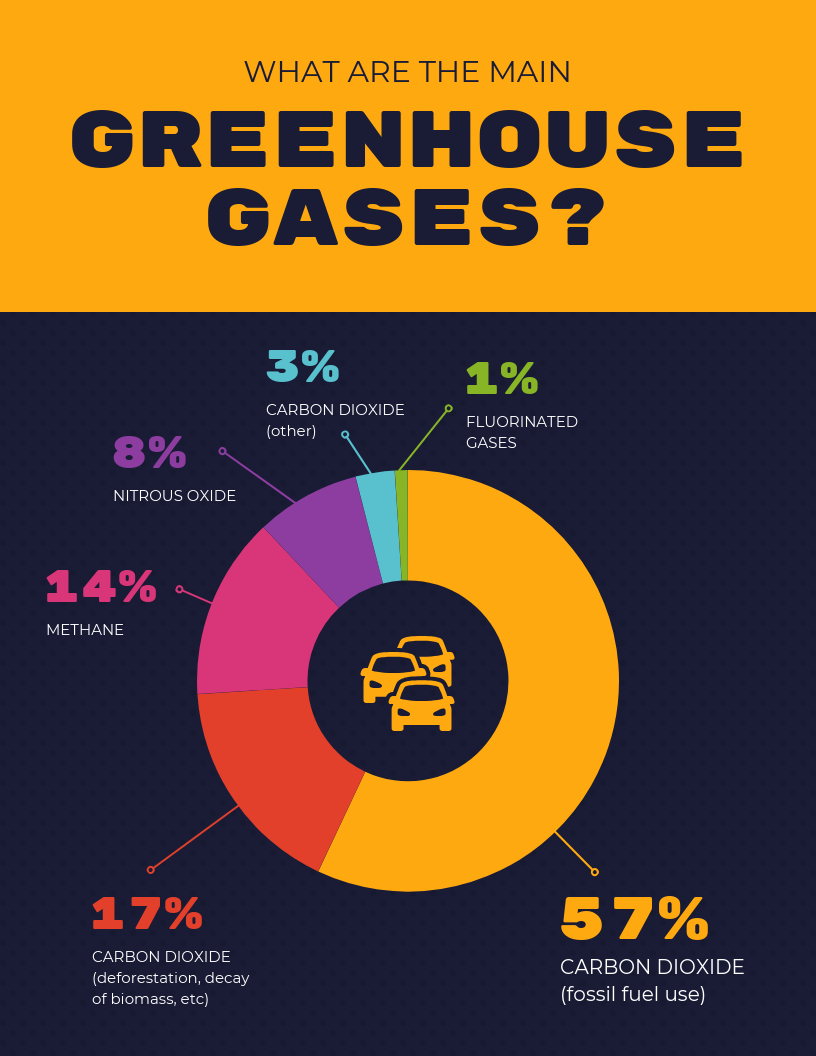
CREATE THIS CHART TEMPLATE
But when your proportions are similar, a pie chart can make it difficult to tell which slice is bigger than the other. That’s why, in most other cases, a bar chart is a safer bet.
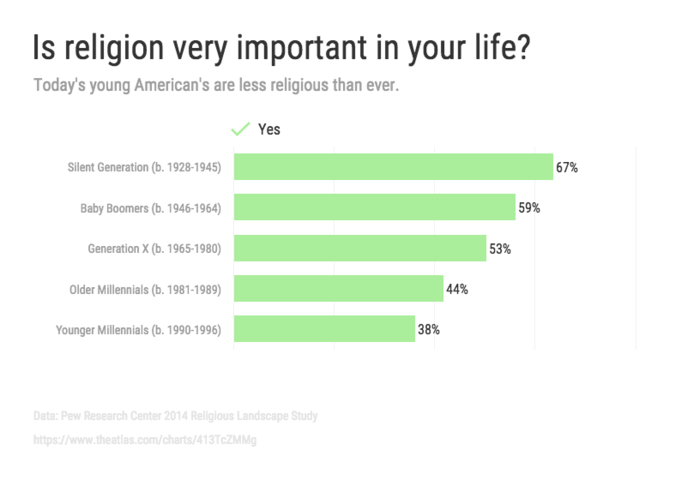
Here is a cheat sheet to help you pick the right type of chart for your data:
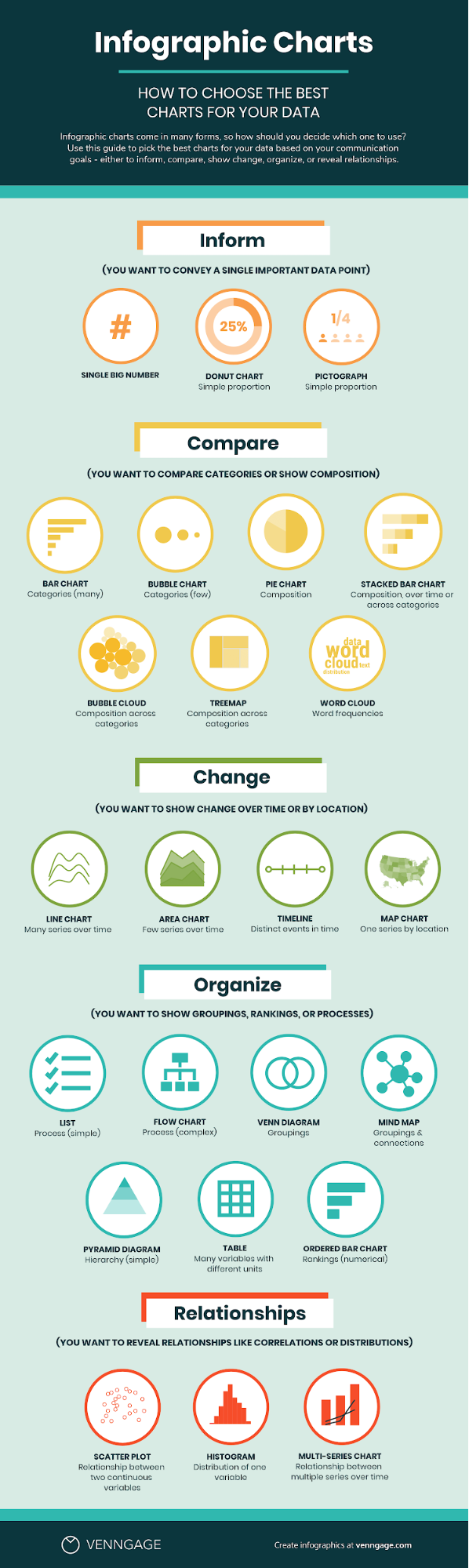
Want to make better charts? Make engaging charts with Venngage’s Chart Maker .
Related : How to Choose the Best Types of Charts For Your Data
Similar to a chart, a diagram is a visual representation of information. Diagrams can be both two-dimensional and three-dimensional.
Some of the most common types of diagrams are:
- Venn diagrams
- Tree diagrams
- SWOT analysis
- Fishbone diagrams
- Use case diagrams
Diagrams are used for mapping out processes, helping with decision making, identifying root causes, connecting ideas, and planning out projects.
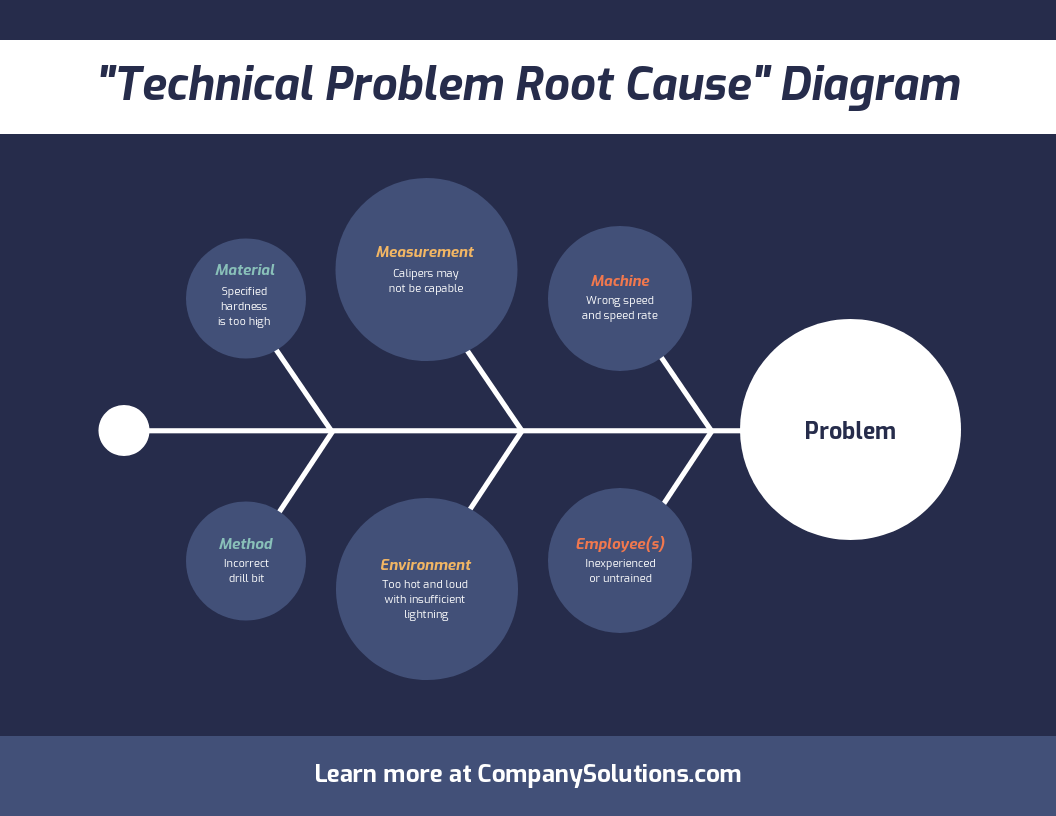
CREATE THIS DIAGRAM TEMPLATE
Want to make a diagram ? Create a Venn diagram and other visuals using our free Venn Diagram Maker .
A map is a visual representation of an area of land. Maps show physical features of land like regions, landscapes, cities, roads, and bodies of water.
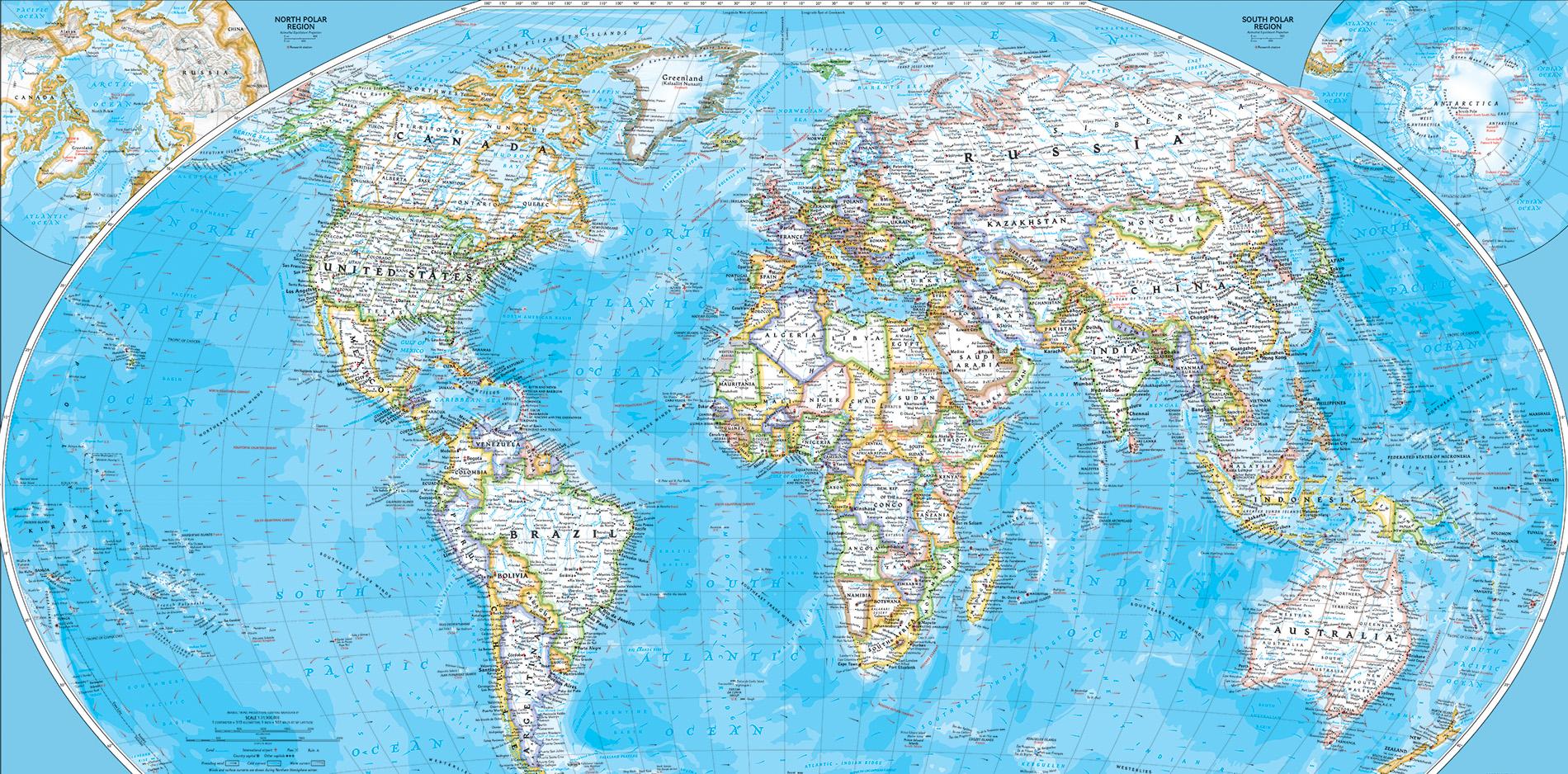
Source: National Geographic
A common type of map you have probably come across in your travels is a choropleth map . Choropleth maps use different shades and colors to indicate average quantities.
For example, a population density map uses varying shades to show the difference in population numbers from region to region:
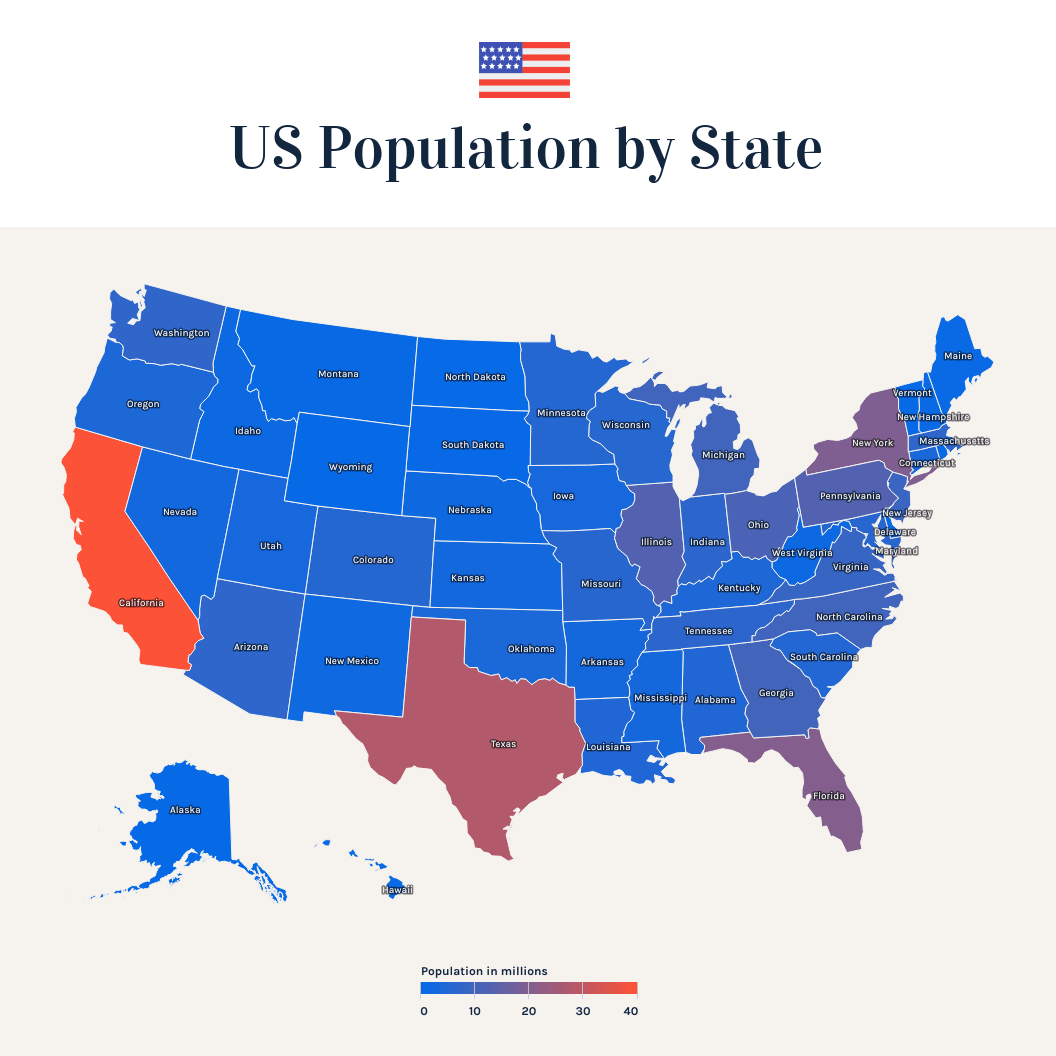
Create your own map for free with Venngage’s Map Maker .
How to present data visually (data visualization best practices)
While good data visualization will communicate data or information clearly and effectively, bad data visualization will do the opposite. Here are some practical tips for how businesses and organizations can use data visualization to communicate information more effectively.
Not a designer? No problem. Venngage’s Graph Maker will help you create better graphs in minutes.
1. Avoid distorting the data
This may be the most important point in this whole blog post. While data visualizations are an opportunity to show off your creative design chops, function should never be sacrificed for fashion.
The chart styles, colors, shapes, and sizing you use all play a role in how the data is interpreted. If you want to present your data accurately and ethically, then you need to take care to ensure that your data visualization does not present the data falsely.
There are a number of different ways data can be distorted in a chart. Some common ways data can be distorted are:
- Making the baselines something other than 0 to make numbers seem bigger or smaller than they are – this is called “truncating” a graph
- Compressing or expanding the scale of the Y-axis to make a line or bar seem bigger or smaller than it should be
- Cherry picking data so that only the data points you want to include are on a graph (i.e. only telling part of the story)
- Using the wrong type of chart, graph or diagram for your data
- Going against standard, expected data visualization conventions
Because people use data visualizations to reinforce their opinions, you should always read data visualizations with a critical eye. Often enough, writers may be using data visualization to skew the data in a way that supports their opinions, but that may not be entirely truthful.

Read More: 5 Ways Writers Use Graphs To Mislead You
Want to create an engaging line graph? Use Venngage’s Line Graph Maker to create your own in minutes.
2. Avoid cluttering up your design with “chartjunk”
When it comes to best practices for data visualization, we should turn to one of the grandfather’s of data visualization: Edward Tufte. He coined the term “ chartjunk ”, which refers to the use of unnecessary or confusing design elements that skews or obscures the data in a chart.
Here’s an example of a data visualization that suffers from chartjunk:
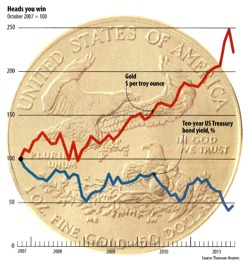
Source: ExcelUser
In this example, the image of the coin is distracting for readers trying to interpret the data. Note how the fonts are tiny – almost unreadable. Mistakes like this are common when a designers tries to put style before function.
Read More : The Worst Infographics of 2020 (With Lessons for 2021)
3. Tell a story with your data
Data visualizations like infographics give you the space to combine data and narrative structure in one page. Visuals like icons and bold fonts let you highlight important statistics and facts.
For example, you could customize this data visualization infographic template to show the benefit of using your product or service (and post it on social media):
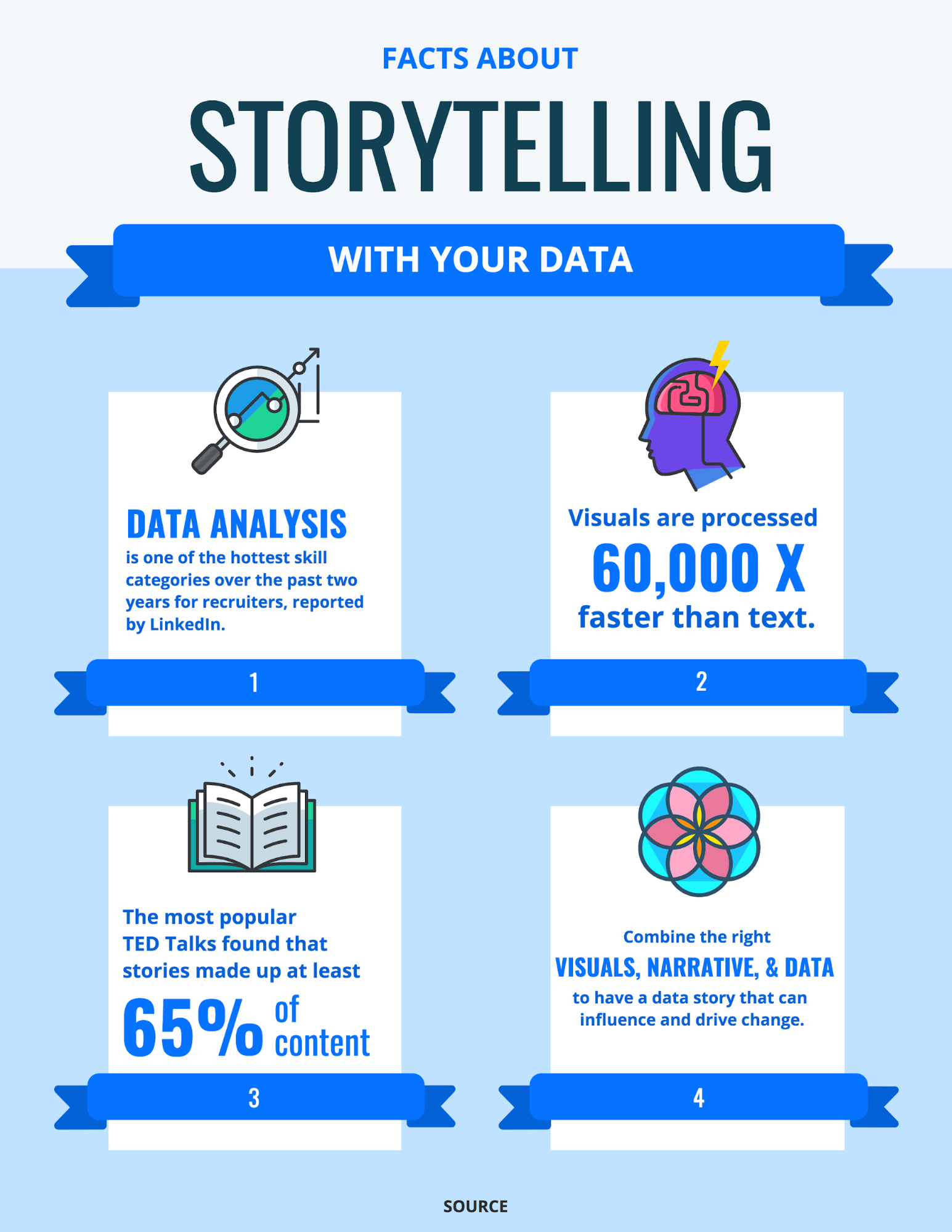
USE THIS TEMPLATE
This data visualization relies heavily on text and icons to tell the story of its data:
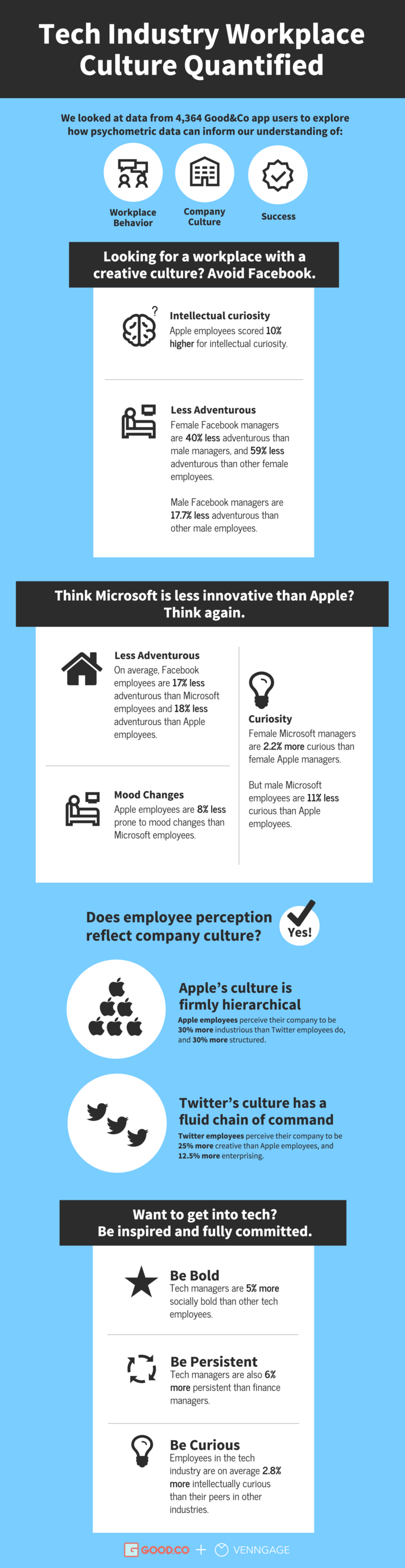
This type of infographic is perfect for those who aren’t as comfortable with charts and graphs. It’s also a great way to showcase original research, get social shares and build brand awareness.
4. Combine different types of data visualizations
While you may choose to keep your data visualization simple, combining multiple types of charts and diagrams can help tell a more rounded story.
Don’t be afraid to combine charts, pictograms and diagrams into one infographic. The result will be a data visualization infographic that is engaging and rich in visual data.
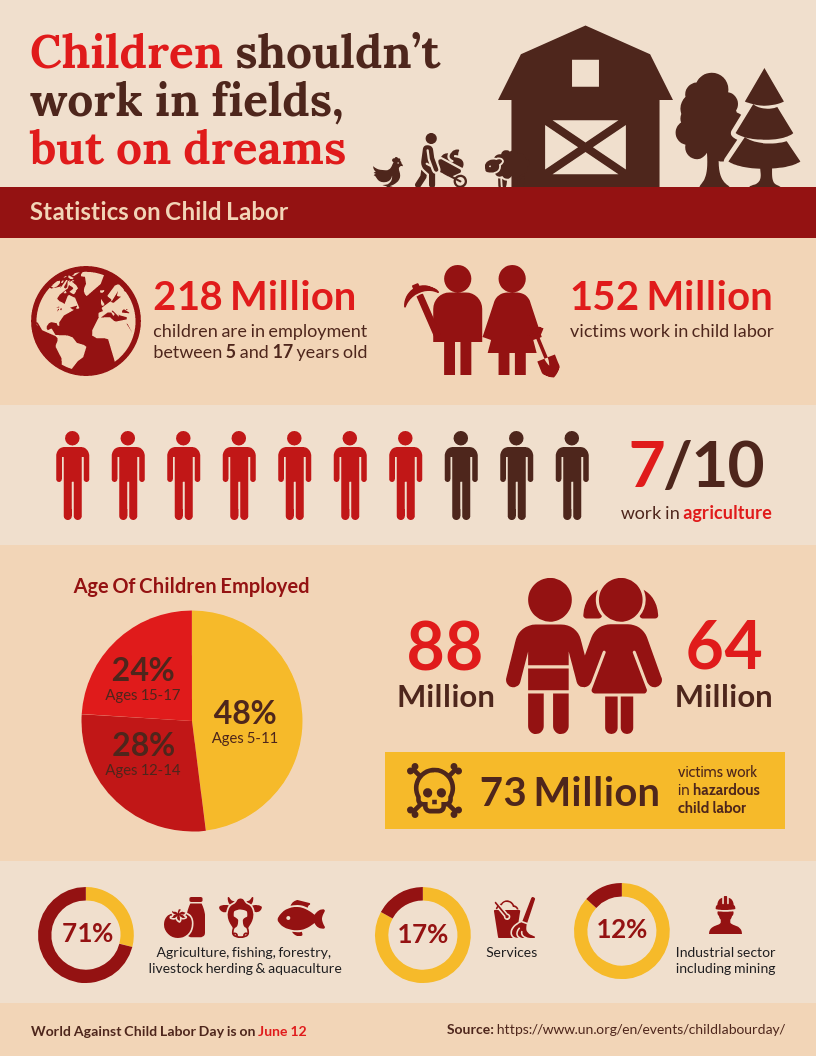
Design Tip: This data visualization infographic would be perfect for nonprofits to customize and include in an email newsletter to increase awareness (and donations).
Or take this data visualization that also combines multiple types of charts, pictograms, and images to engage readers. It could work well in a presentation or report on customer research, customer service scores, quarterly performance and much more:
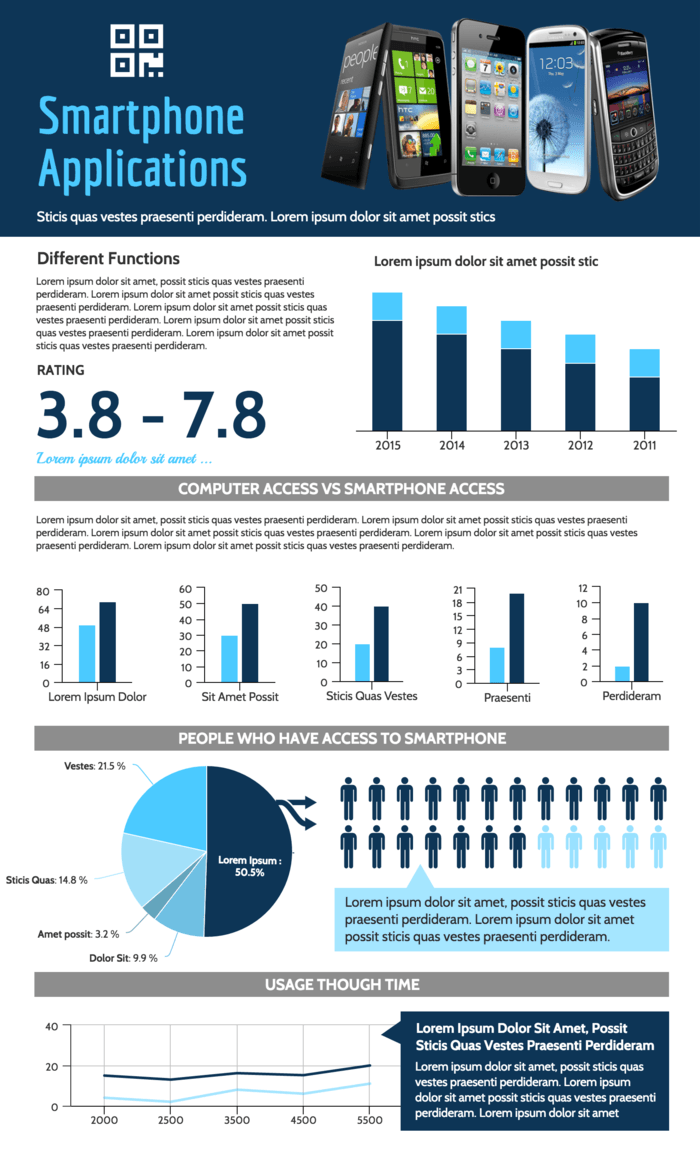
Design Tip: This infographic could work well in a presentation or report on customer research, customer service scores, quarterly performance and much more.
Make your own bar graph in minutes with our free Bar Graph Maker .
5. Use icons to emphasize important points
Icons are perfect for attracting the eye when scanning a page. (Remember: use visual cues!)
If there are specific data points that you want readers to pay attention to, placing an icon beside it will make it more noticeable:

Design Tip: This infographic template would work well on social media to encourage shares and brand awareness.
You can also pair icons with headers to indicate the beginning of a new section.
Meanwhile, this infographic uses icons like bullet points to emphasize and illustrate important points.
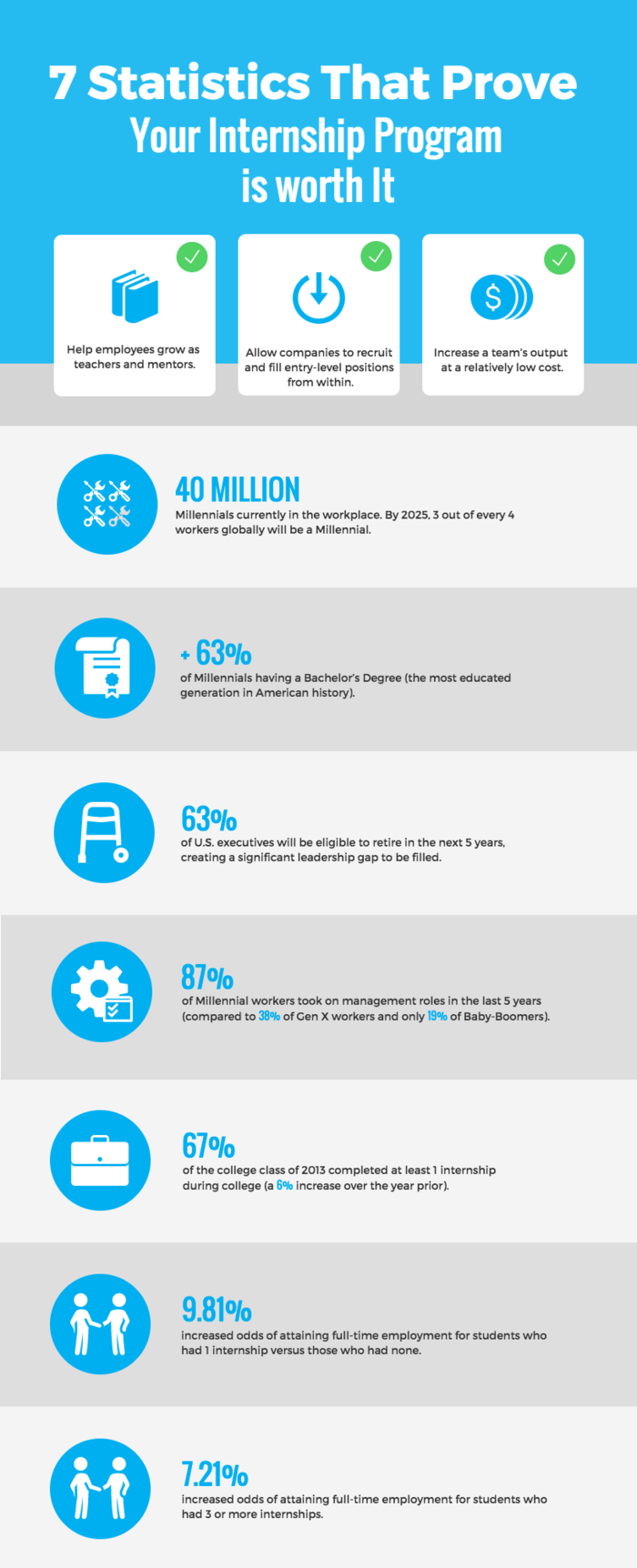
Design Tip: This infographic would make a great sales piece to promote your course or other service.
6. Use bold fonts to make text information engaging
A challenge people often face when setting out to visualize information is knowing how much text to include. After all, the point of data visualization is that it presents information visually, rather than a page of text.
Even if you have a lot of text information, you can still create present data visually. Use bold, interesting fonts to make your data exciting. Just make sure that, above all else, your text is still easy to read.
This data visualization uses different fonts for the headers and body text that are bold but clear. This helps integrate the text into the design and emphasizes particular points:
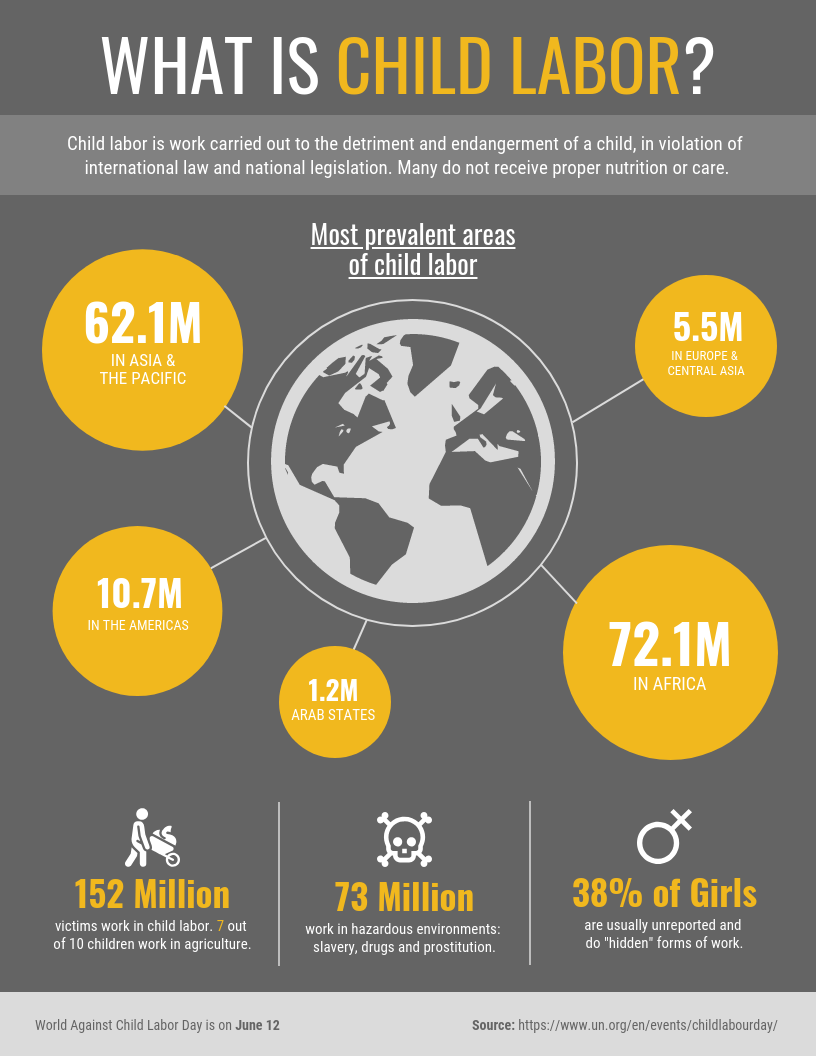
Design Tip: Nonprofits could use this data visualization infographic in a newsletter or on social media to build awareness, but any business could use it to explain the need for their product or service.
As a general rule of thumb, stick to no more than three different font types in one infographic.
This infographic uses one font for headers, another font for body text, and a third font for accent text.
Read More: How to Choose Fonts For Your Designs (With Examples)
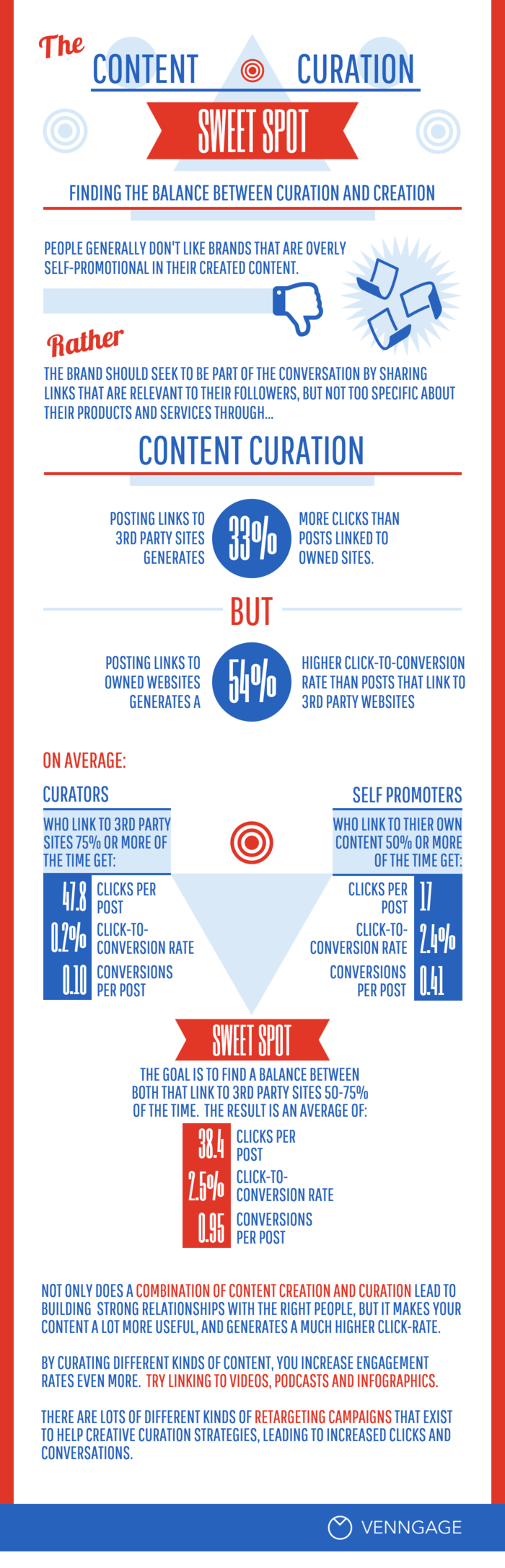
Design Tip: Venngage has a library of fonts to choose from. If you can’t find the icon you’re looking for , you can always request they be added. Our online editor has a chat box with 24/7 customer support.
7. Use colors strategically in your design
In design, colors are as functional as they are fashionable. You can use colors to emphasize points, categorize information, show movement or progression, and more.
For example, this chart uses color to categorize data:
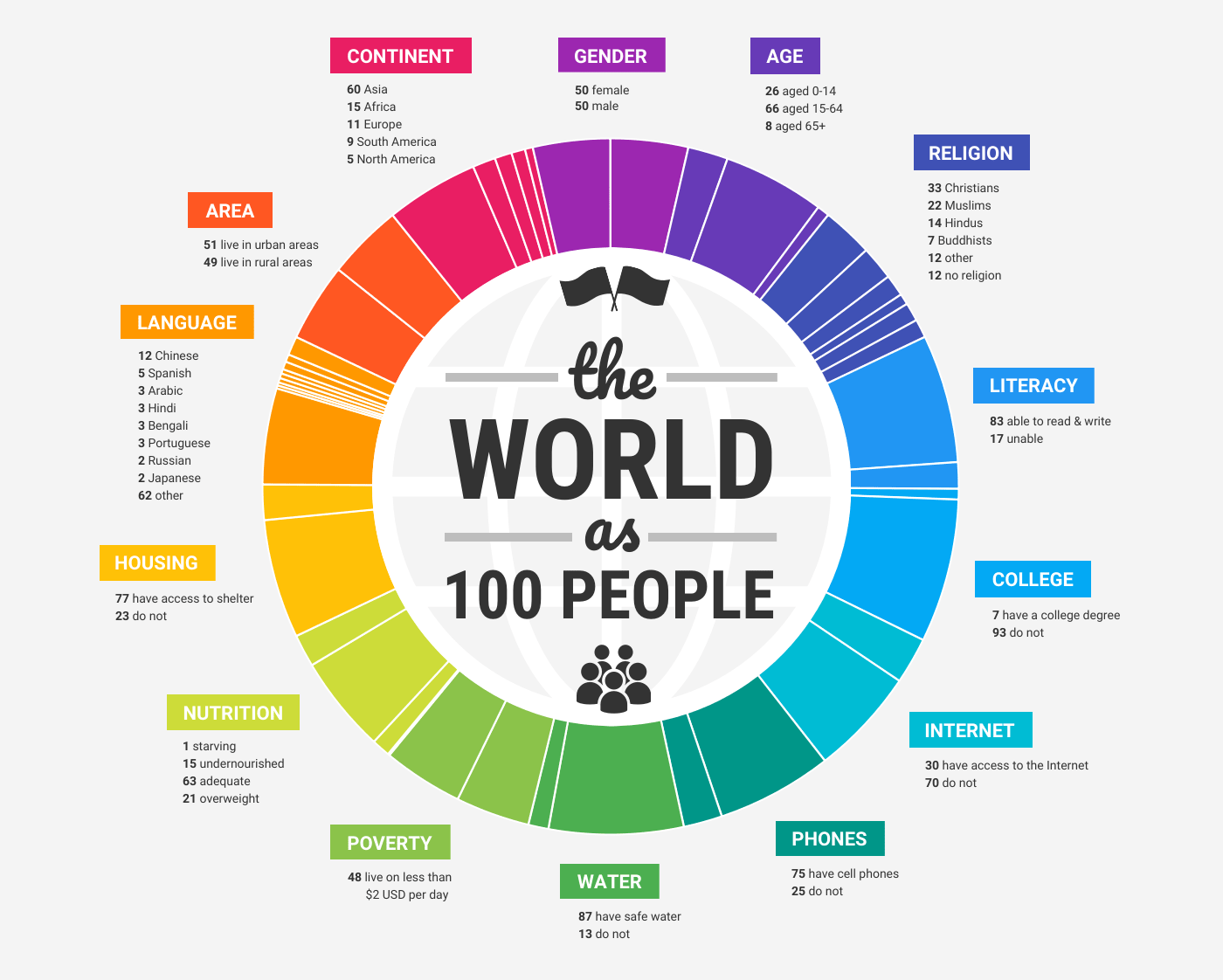
Design Tip : This pie chart can actually be customized in many ways. Human resources could provide a monthly update of people hired by department, nonprofits could show a breakdown of how they spent donations and real estate agents could show the average price of homes sold by neighbourhood.
You can also use light colored text and icons on dark backgrounds to make them stand out. Consider the mood that you want to convey with your infographic and pick colors that will reflect that mood. You can also use contrasting colors from your brand color palette.
This infographic template uses a bold combination of pinks and purples to give the data impact:
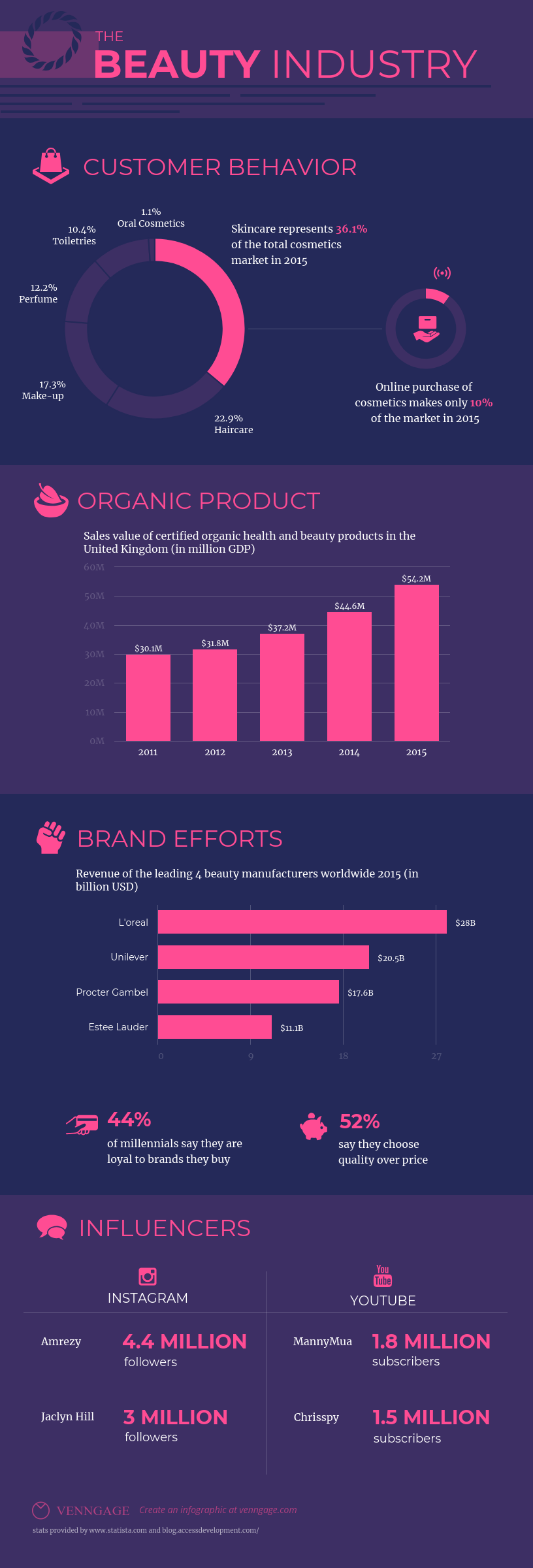
Read More: How to Pick Colors to Captivate Readers and Communicate Effectively
8. Show how parts make up a whole
It can be difficult to break a big topic down into smaller parts. Data visualization can make it a lot easier for people to conceptualize how parts make up a whole.
Using one focus visual, diagram or chart can convey parts of a whole more effectively than a text list can. Look at how this infographic neatly visualizes how marketers use blogging as part of their strategy:
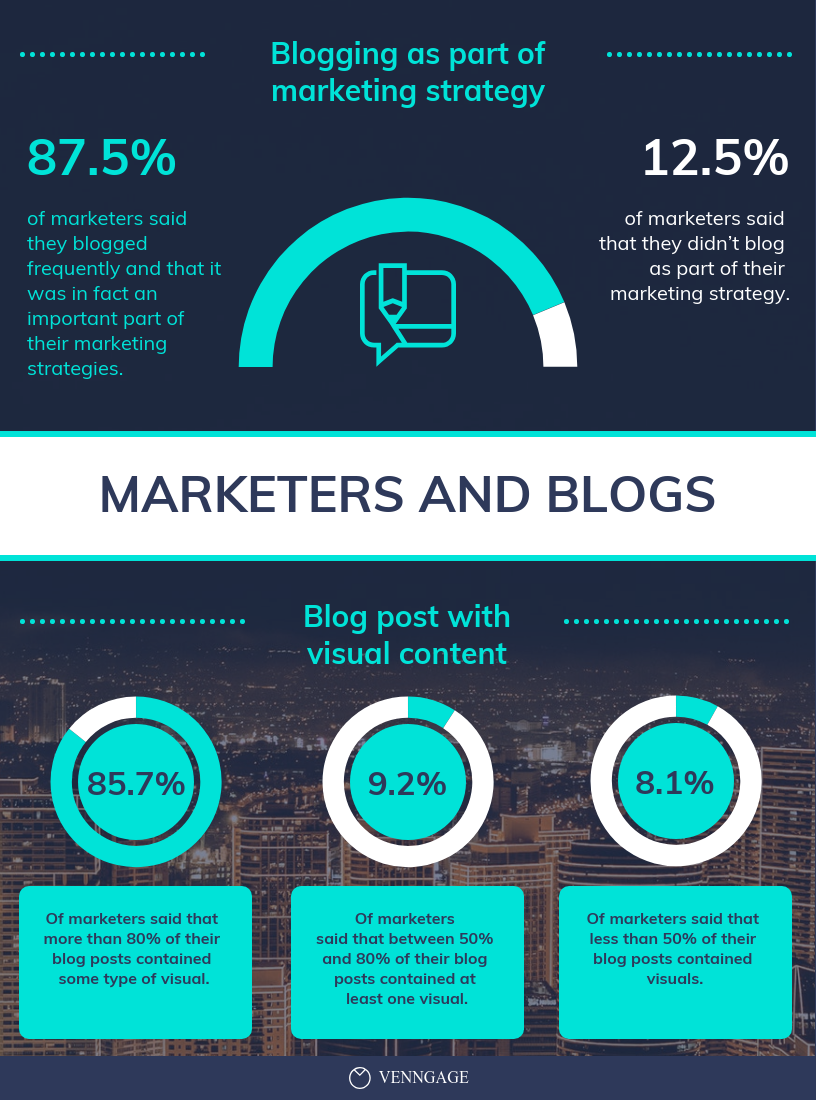
Design Tip: Human resources could use this graphic to show the results of a company survey. Or consultants could promote their services by showing their success rates.
Or look at how this infographic template uses one focus visual to illustrate the nutritional makeup of a banana:
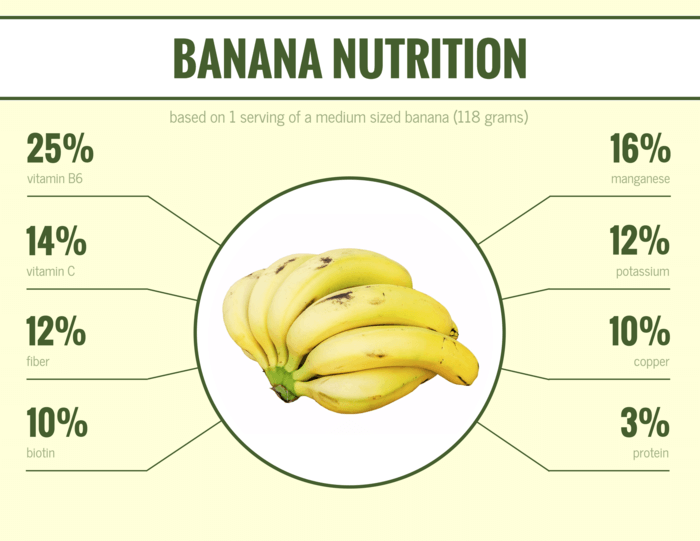
CREATE THIS FLYER TEMPLATE
9. Focus on one amazing statistic
If you are preparing a presentation, it’s best not to try and cram too many visuals into one slide. Instead, focus on one awe-inspiring statistic and make that the focus of your slide.
Use one focus visual to give the statistic even more impact. Smaller visuals like this are ideal for sharing on social media, like in this example:
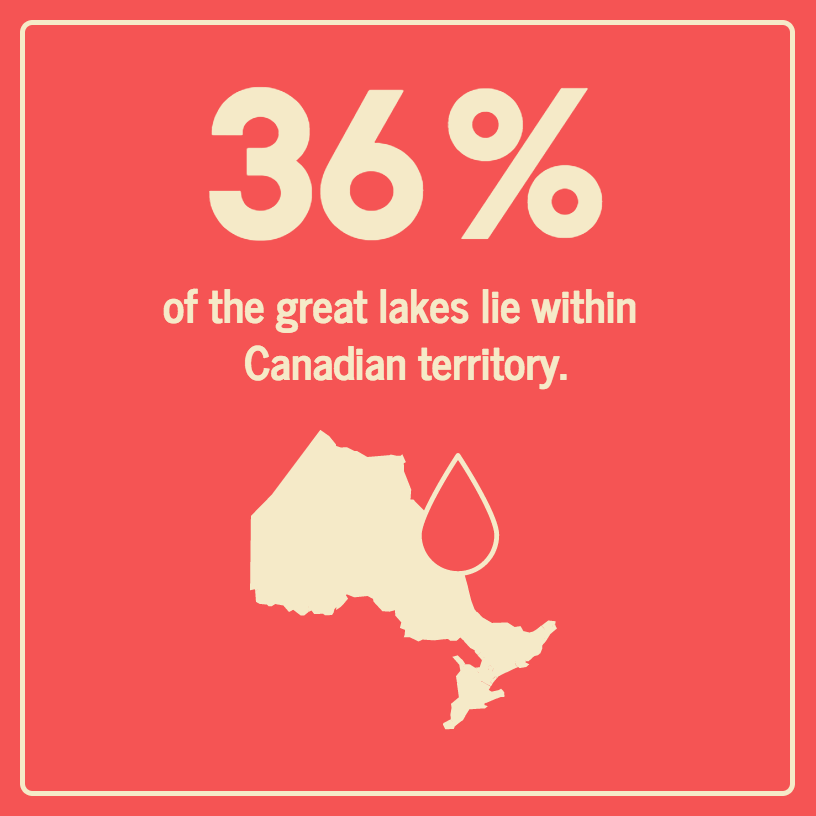
Design Tip: You can easily swap out the icon above (of Ontario, Canada) using Venngage’s drag-and-drop online editor and its in-editor library of icons. Click on the template above to get started.
This template also focuses on one key statistic and offers some supporting information in the bar on the side:
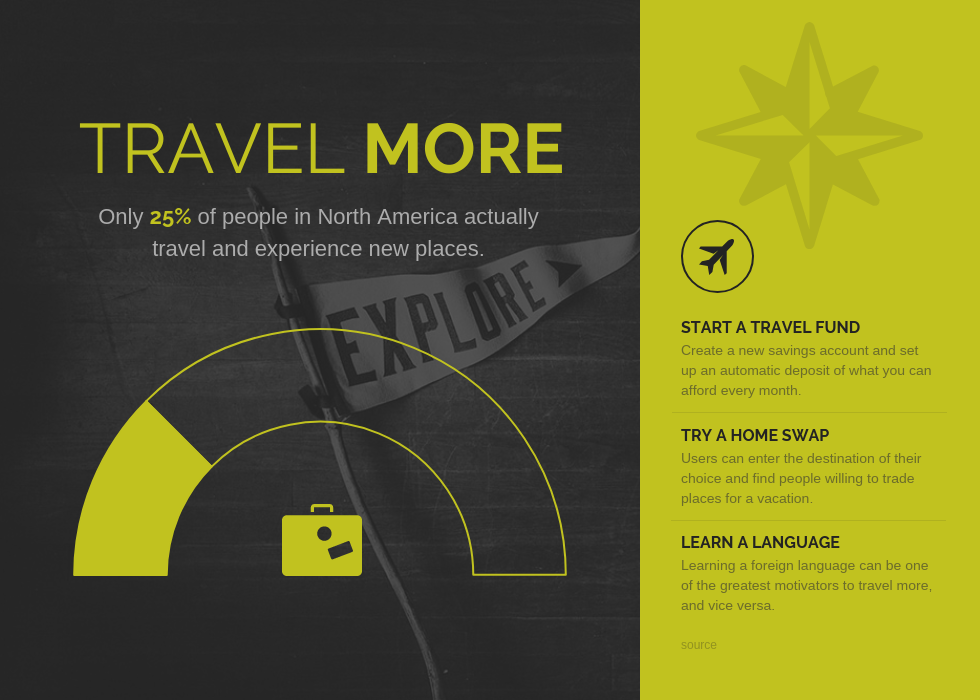
10. Optimize your data visualization for mobile
Complex, information-packed infographics are great for spicing up reports, blog posts, handouts, and more. But they’re not always the best for mobile viewing.
To optimize your data visualization for mobile viewing, use one focus chart or icon and big, legible font. You can create a series of mobile-optimized infographics to share multiple data points in a super original and attention-grabbing way.
For example, this infographic uses concise text and one chart to cut to the core message behind the data:
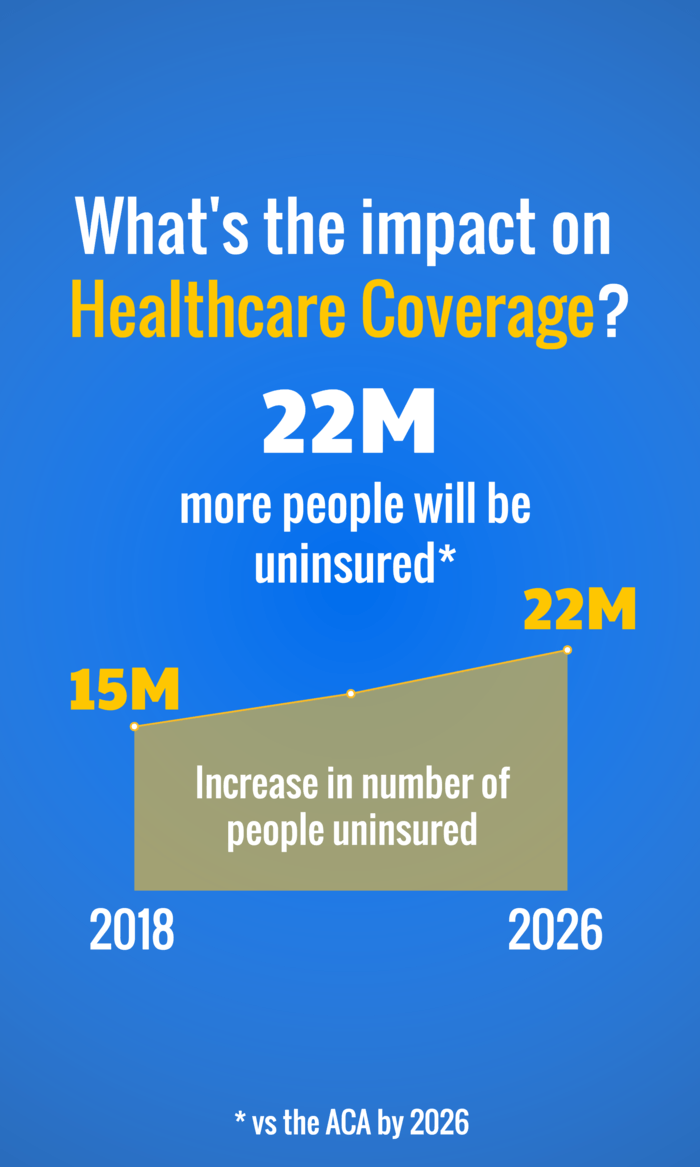
CREATE THIS SOCIAL MEDIA TEMPLATE
Some amazing data visualization examples
Here are some of the best data visualization examples I’ve come across in my years writing about data viz.
Evolution of Marketing Infographic

Graphic Design Trends Infographic
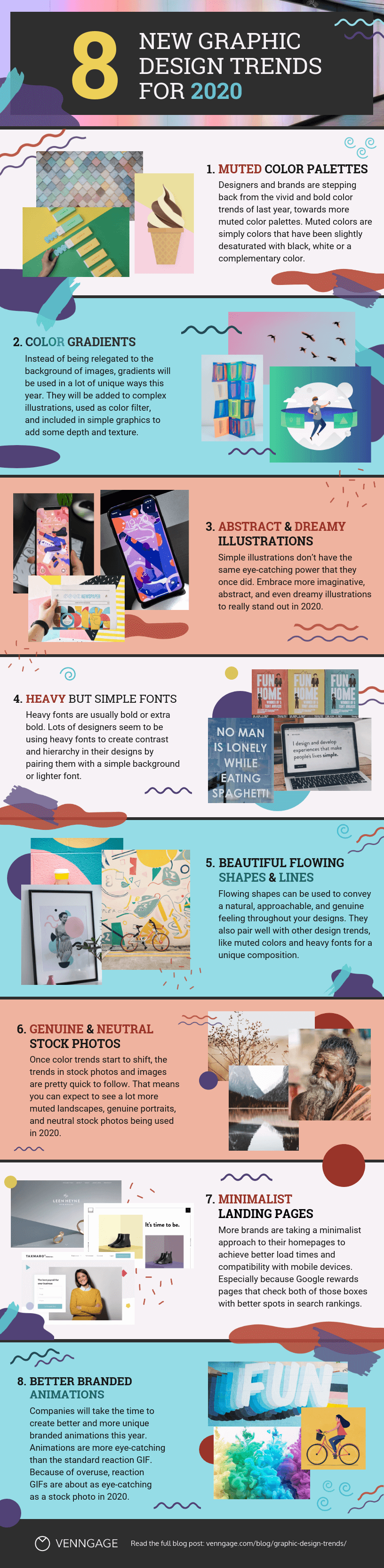
Stop Shark Finning Nonprofit Infographic
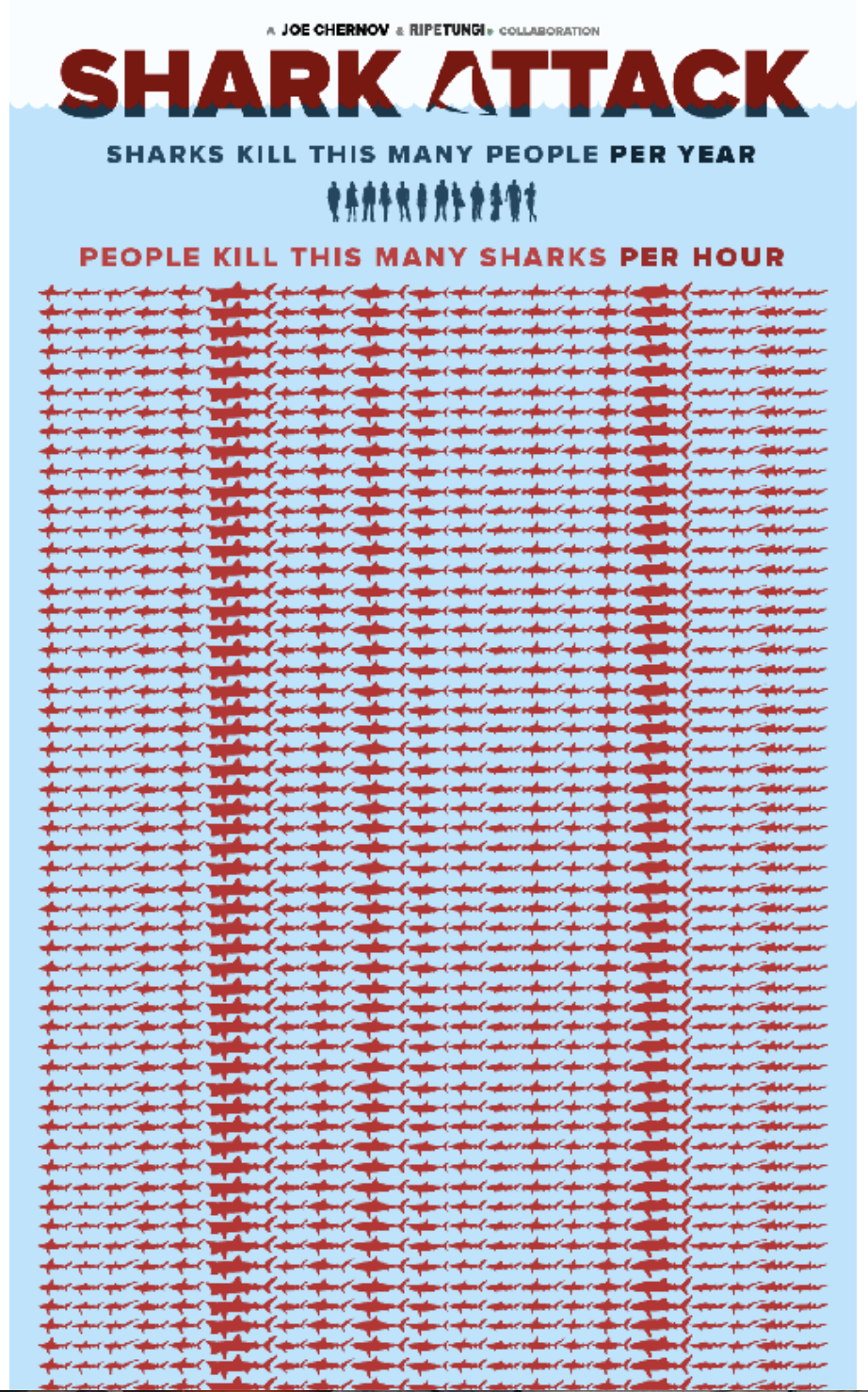
Source: Ripetungi
Coronavirus Impact on Environment Data Visualization

What Disney Characters Tell Us About Color Theory
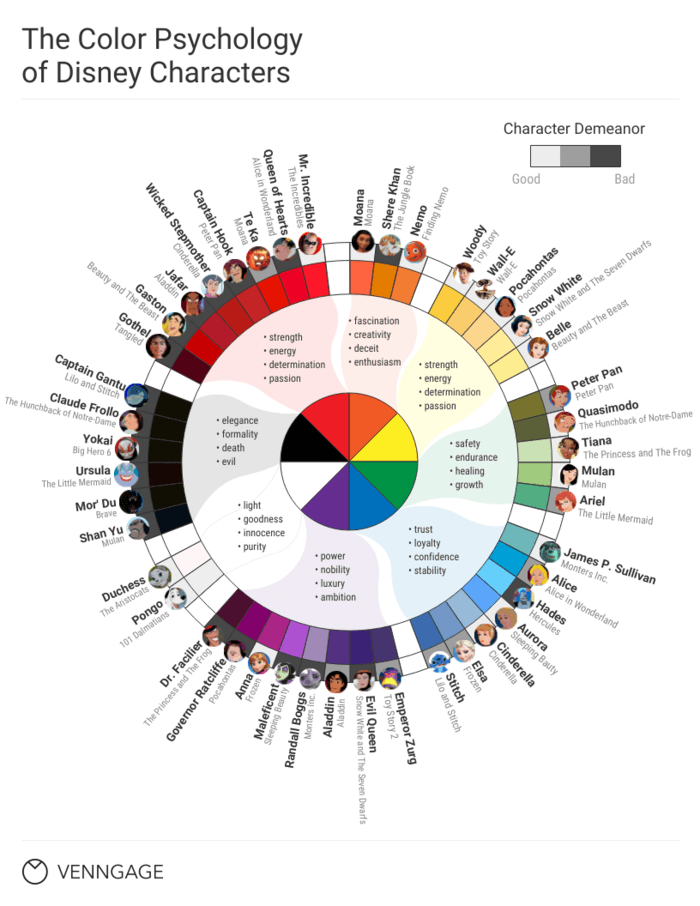
World’s Deadliest Animal Infographic
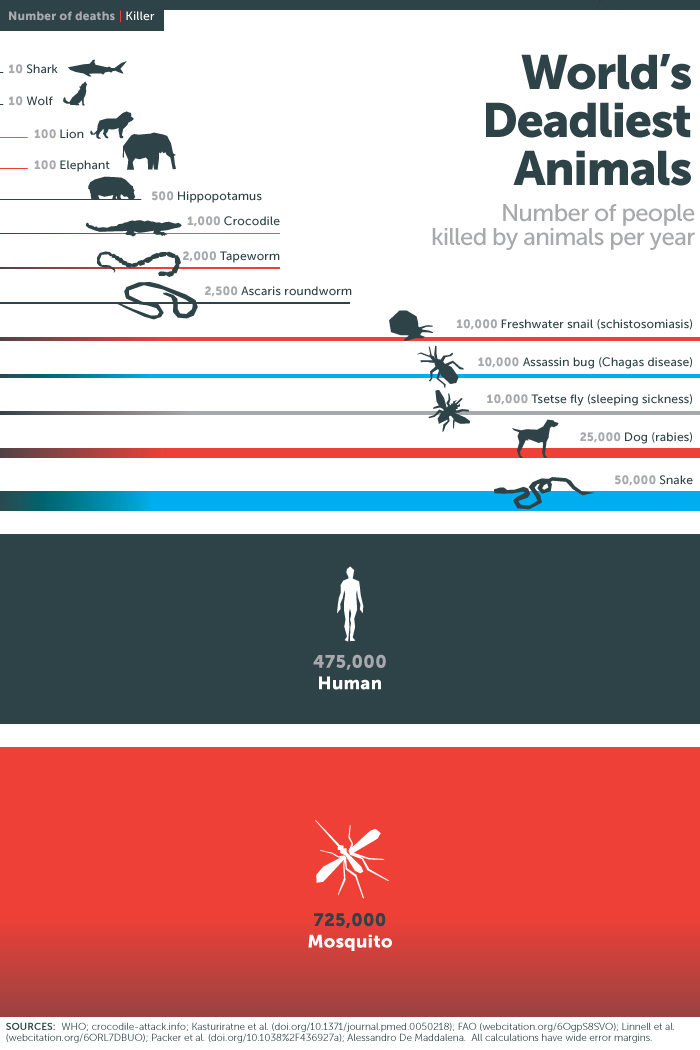
Source: Bill and Melinda Gates Foundation
The Secret Recipe For a Viral Creepypasta

Read More: Creepypasta Study: The Secret Recipe For a Viral Horror Story
The Hero’s Journey Infographic
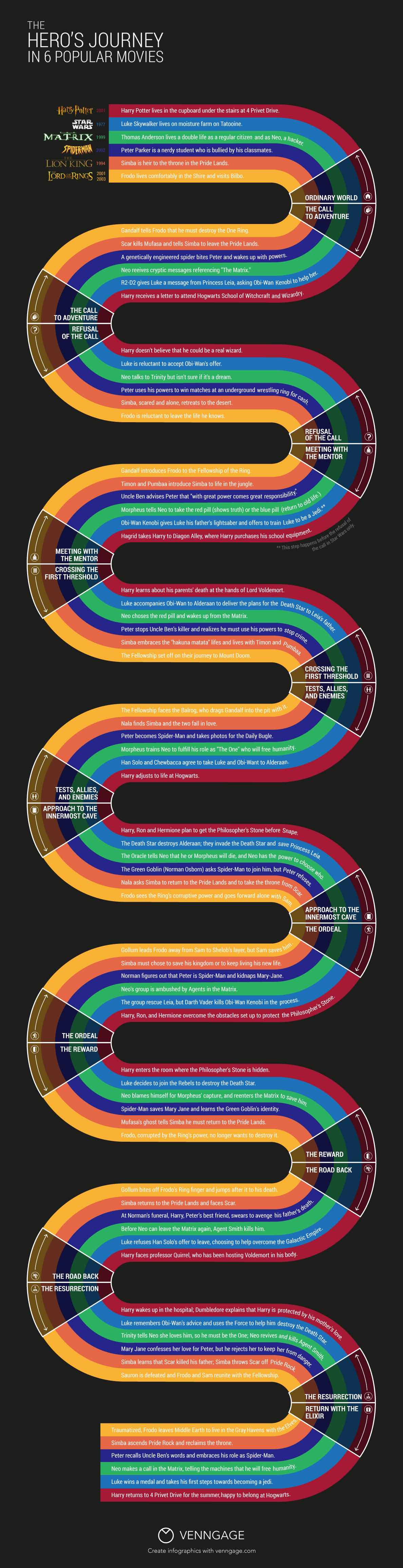
Read More: What Your 6 Favorite Movies Have in Common
Emotional Self Care Guide Infographic
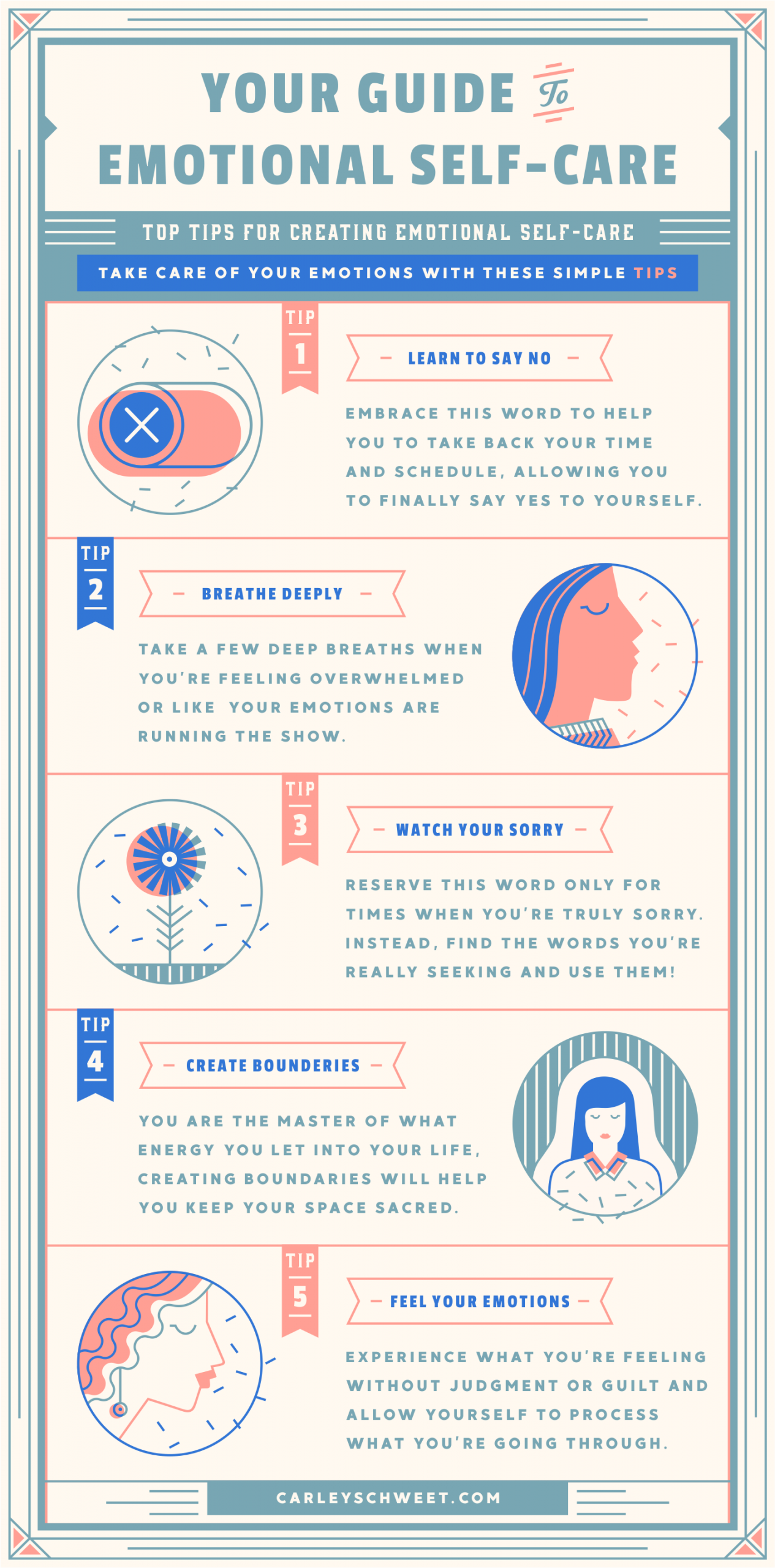
Source: Carley Schweet
Want to look at more amazing data visualization? Read More: 50+ Infographic Ideas, Examples & Templates for 2020 (For Marketers, Nonprofits, Schools, Healthcare Workers, and more)
Discover popular designs
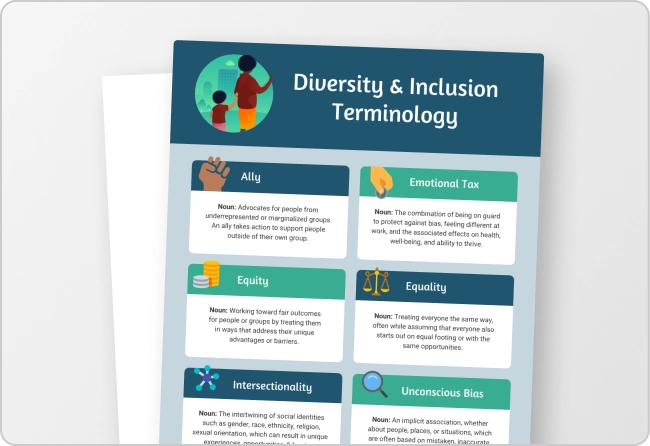
Infographic maker
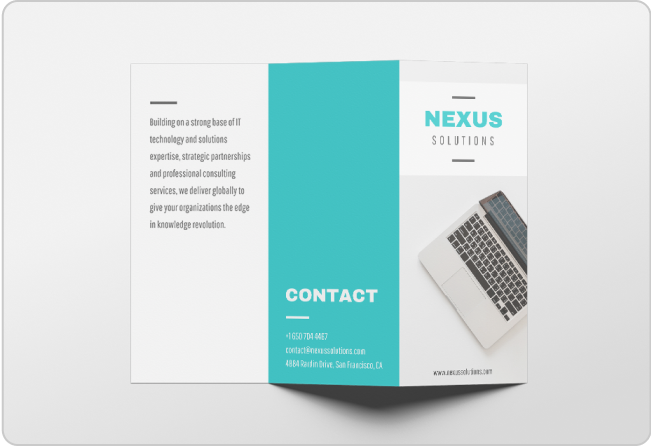
Brochure maker
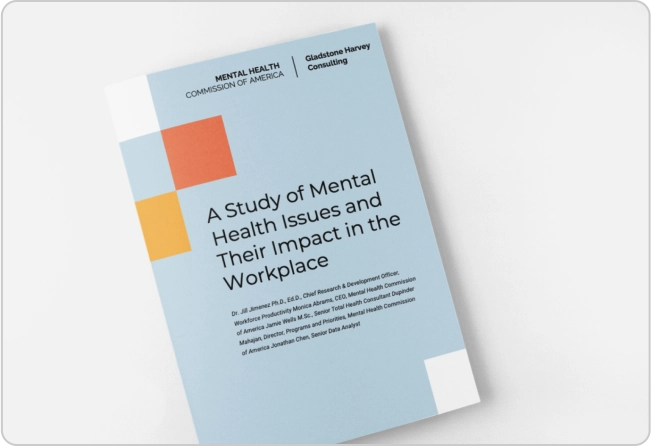
White paper online
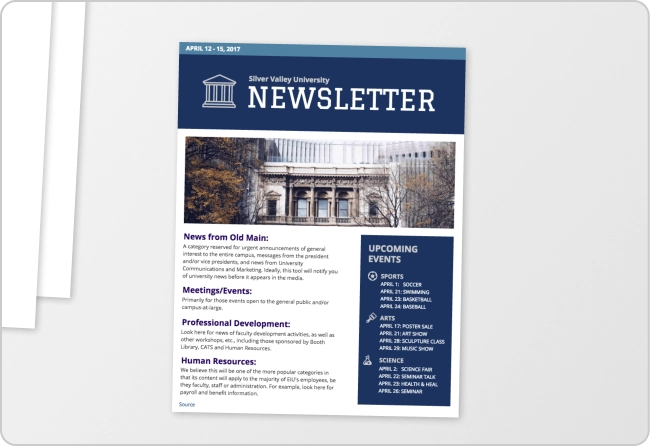
Newsletter creator
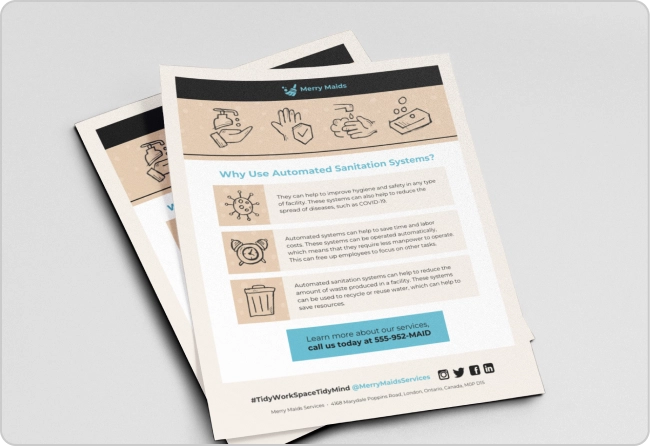
Flyer maker
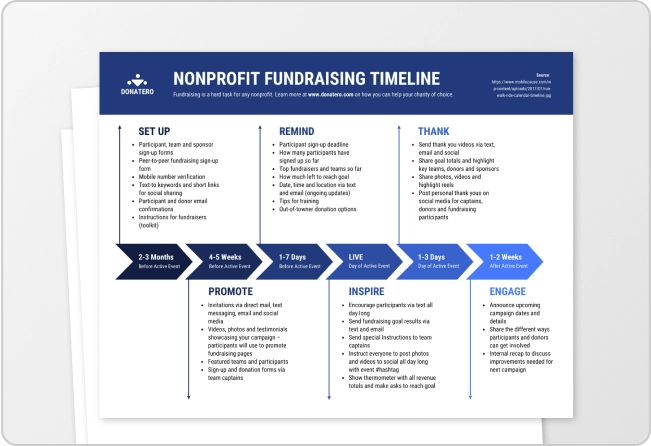
Timeline maker
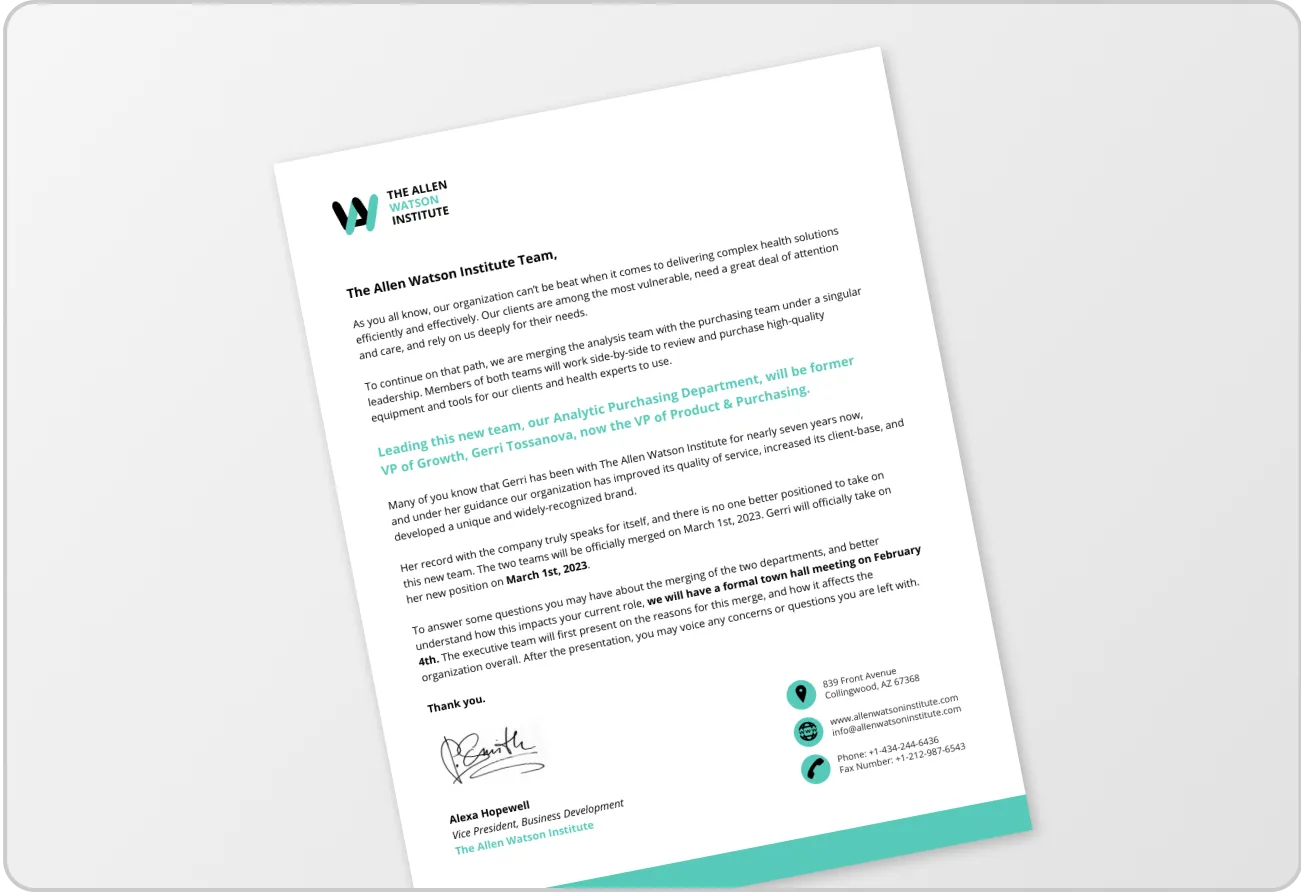
Letterhead maker
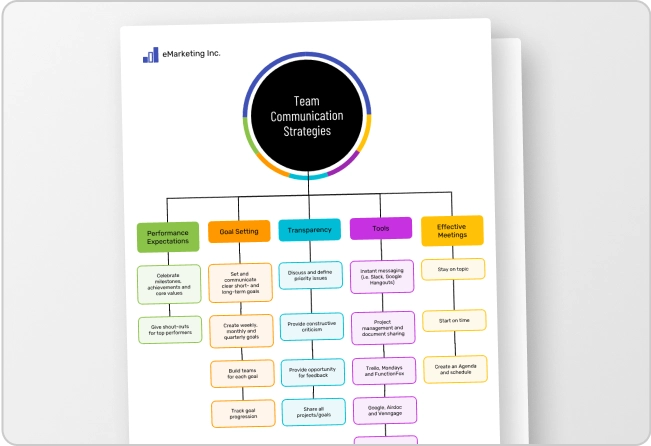
Mind map maker
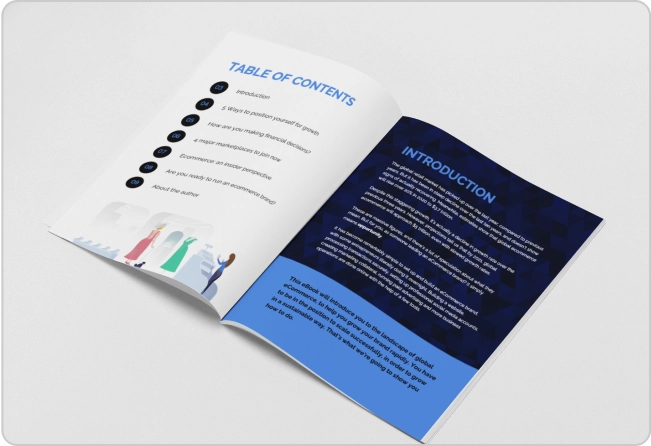
Ebook maker
What Is Data Visualization: Brief Theory, Useful Tips and Awesome Examples
- Share on Facebook
- Share on Twitter
By Al Boicheva
in Insights , Inspiration
4 years ago
Viewed 11,933 times
Spread the word about this article:
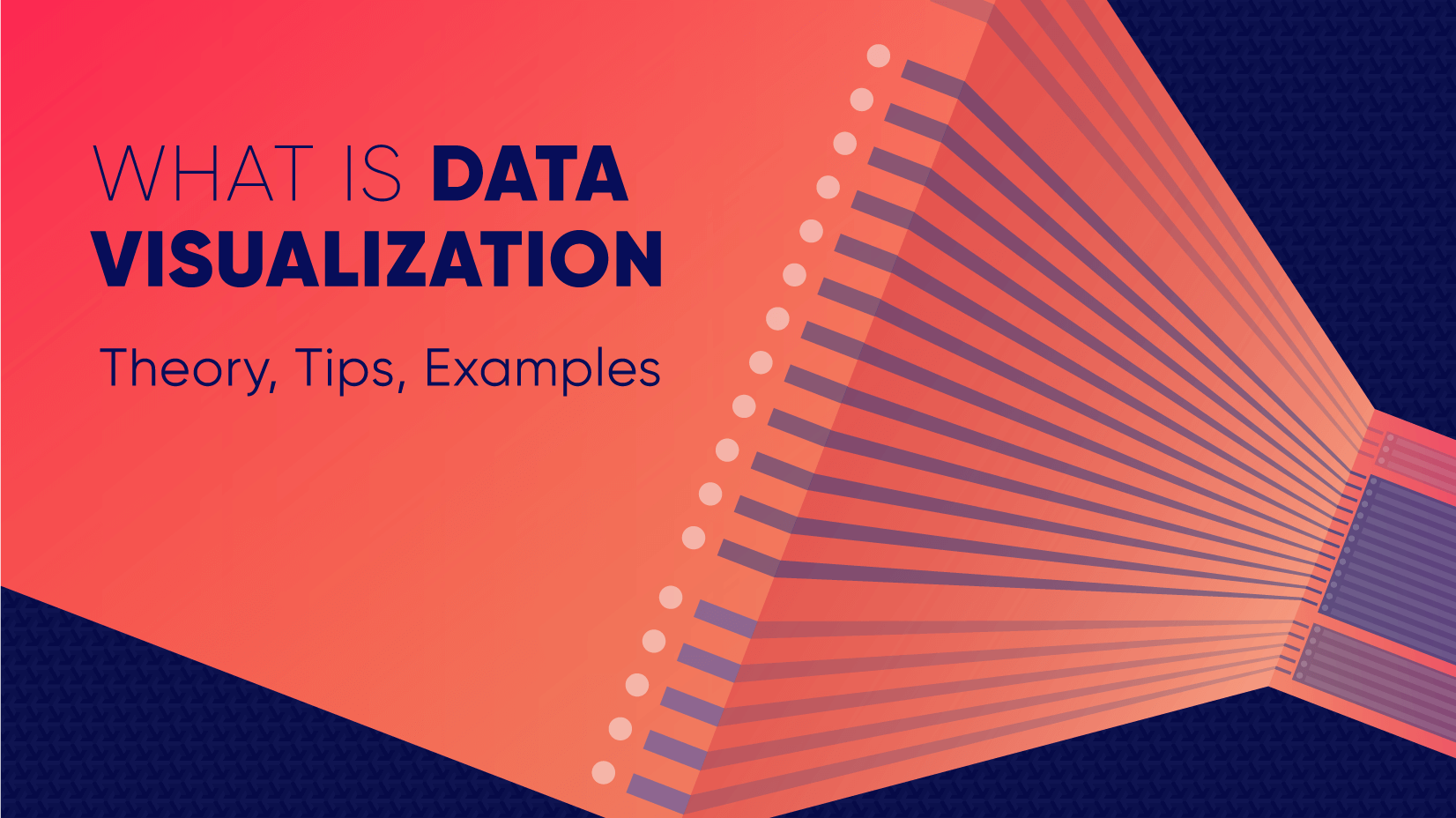
Updated: June 23, 2022
To create data visualization in order to present your data is no longer just a nice to have skill. Now, the skill to effectively sort and communicate your data through charts is a must-have for any business in any field that deals with data. Data visualization helps businesses quickly make sense of complex data and start making decisions based on that data. This is why today we’ll talk about what is data visualization. We’ll discuss how and why does it work, what type of charts to choose in what cases, how to create effective charts, and, of course, end with beautiful examples.
So let’s jump right in. As usual, don’t hesitate to fast-travel to a particular section of your interest.
Article overview: 1. What Does Data Visualization Mean? 2. How Does it Work? 3. When to Use it? 4. Why Use it? 5. Types of Data Visualization 6. Data Visualization VS Infographics: 5 Main Differences 7. How to Create Effective Data Visualization?: 5 Useful Tips 8. Examples of Data Visualization
1. What is Data Visualization?
Data Visualization is a graphic representation of data that aims to communicate numerous heavy data in an efficient way that is easier to grasp and understand . In a way, data visualization is the mapping between the original data and graphic elements that determine how the attributes of these elements vary. The visualization is usually made by the use of charts, lines, or points, bars, and maps.
- Data Viz is a branch of Descriptive statistics but it requires both design, computer, and statistical skills.
- Aesthetics and functionality go hand in hand to communicate complex statistics in an intuitive way.
- Data Viz tools and technologies are essential for making data-driven decisions.
- It’s a fine balance between form and functionality.
- Every STEM field benefits from understanding data.
2. How Does it Work?
If we can see it, our brains can internalize and reflect on it. This is why it’s much easier and more effective to make sense of a chart and see trends than to read a massive document that would take a lot of time and focus to rationalize. We wouldn’t want to repeat the cliche that humans are visual creatures, but it’s a fact that visualization is much more effective and comprehensive.
In a way, we can say that data Viz is a form of storytelling with the purpose to help us make decisions based on data. Such data might include:
- Tracking sales
- Identifying trends
- Identifying changes
- Monitoring goals
- Monitoring results
- Combining data
3. When to Use it?
Data visualization is useful for companies that deal with lots of data on a daily basis. It’s essential to have your data and trends instantly visible. Better than scrolling through colossal spreadsheets. When the trends stand out instantly this also helps your clients or viewers to understand them instead of getting lost in the clutter of numbers.
With that being said, Data Viz is suitable for:
- Annual reports
- Presentations
- Social media micronarratives
- Informational brochures
- Trend-trafficking
- Candlestick chart for financial analysis
- Determining routes
Common cases when data visualization sees use are in sales, marketing, healthcare, science, finances, politics, and logistics.
4. Why Use it?
Short answer: decision making. Data Visualization comes with the undeniable benefits of quickly recognizing patterns and interpret data. More specifically, it is an invaluable tool to determine the following cases.
- Identifying correlations between the relationship of variables.
- Getting market insights about audience behavior.
- Determining value vs risk metrics.
- Monitoring trends over time.
- Examining rates and potential through frequency.
- Ability to react to changes.
5. Types of Data Visualization
As you probably already guessed, Data Viz is much more than simple pie charts and graphs styled in a visually appealing way. The methods that this branch uses to visualize statistics include a series of effective types.
Map visualization is a great method to analyze and display geographically related information and present it accurately via maps. This intuitive way aims to distribute data by region. Since maps can be 2D or 3D, static or dynamic, there are numerous combinations one can use in order to create a Data Viz map.
COVID-19 Spending Data Visualization POGO by George Railean
The most common ones, however, are:
- Regional Maps: Classic maps that display countries, cities, or districts. They often represent data in different colors for different characteristics in each region.
- Line Maps: They usually contain space and time and are ideal for routing, especially for driving or taxi routes in the area due to their analysis of specific scenes.
- Point Maps: These maps distribute data of geographic information. They are ideal for businesses to pinpoint the exact locations of their buildings in a region.
- Heat Maps: They indicate the weight of a geographical area based on a specific property. For example, a heat map may distribute the saturation of infected people by area.
Charts present data in the form of graphs, diagrams, and tables. They are often confused with graphs since graphs are indeed a subcategory of charts. However, there is a small difference: graphs show the mathematical relationship between groups of data and is only one of the chart methods to represent data.
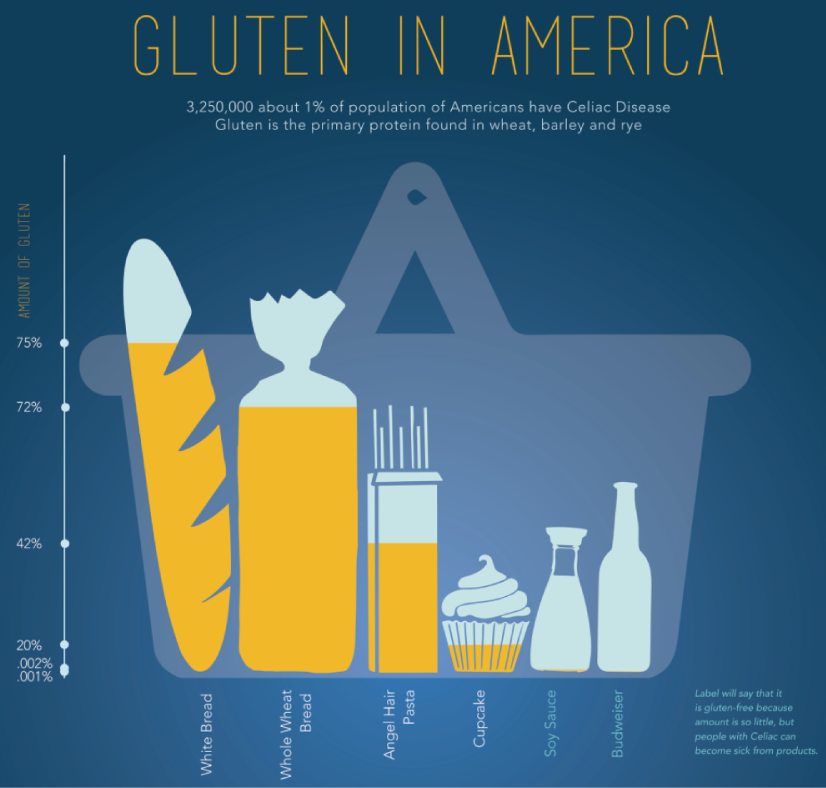
Infographic Data Visualization by Madeline VanRemmen
With that out of the way, let’s talk about the most basic types of charts in data visualization.
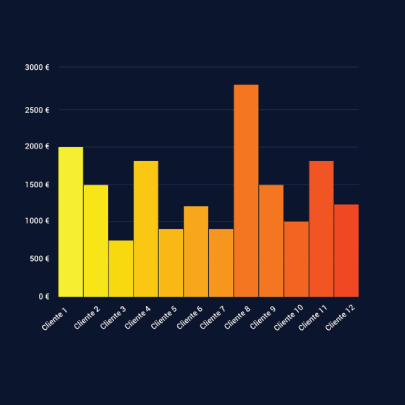
They use a series of bars that illustrate data development. They are ideal for lighter data and follow trends of no more than three variables or else, the bars become cluttered and hard to comprehend. Ideal for year-on-year comparisons and monthly breakdowns.
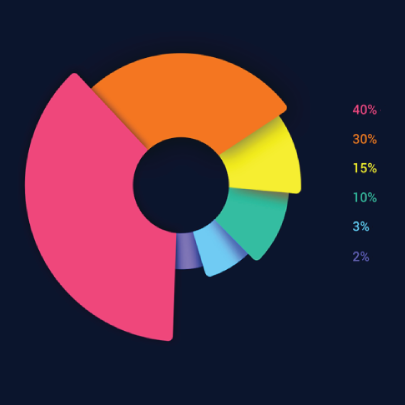
These familiar circular graphs divide data into portions. The bigger the slice, the bigger the portion. They are ideal for depicting sections of a whole and their sum must always be 100%. Avoid pie charts when you need to show data development over time or lack a value for any of the portions. Doughnut charts have the same use as pie charts.
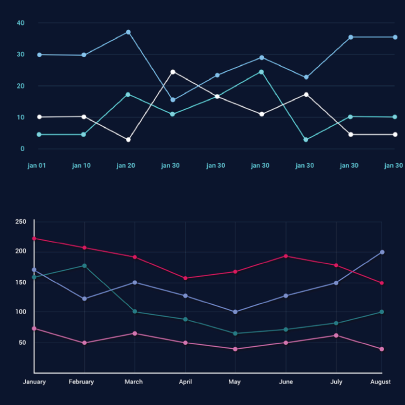
They use a line or more than one lines that show development over time. It allows tracking multiple variables at the same time. A great example is tracking product sales by a brand over the years. Area charts have the same use as line charts.
Scatter Plot
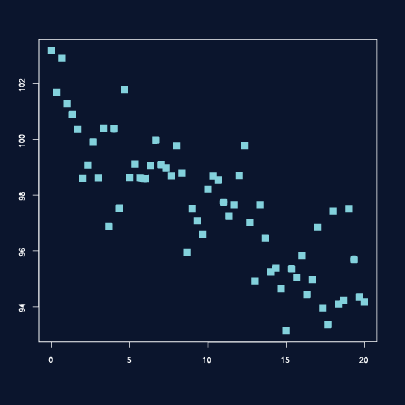
These charts allow you to see patterns through data visualization. They have an x-axis and a y-axis for two different values. For example, if your x-axis contains information about car prices while the y-axis is about salaries, the positive or negative relationship will tell you about what a person’s car tells about their salary.
Unlike the charts we just discussed, tables show data in almost a raw format. They are ideal when your data is hard to present visually and aim to show specific numerical data that one is supposed to read rather than visualize.
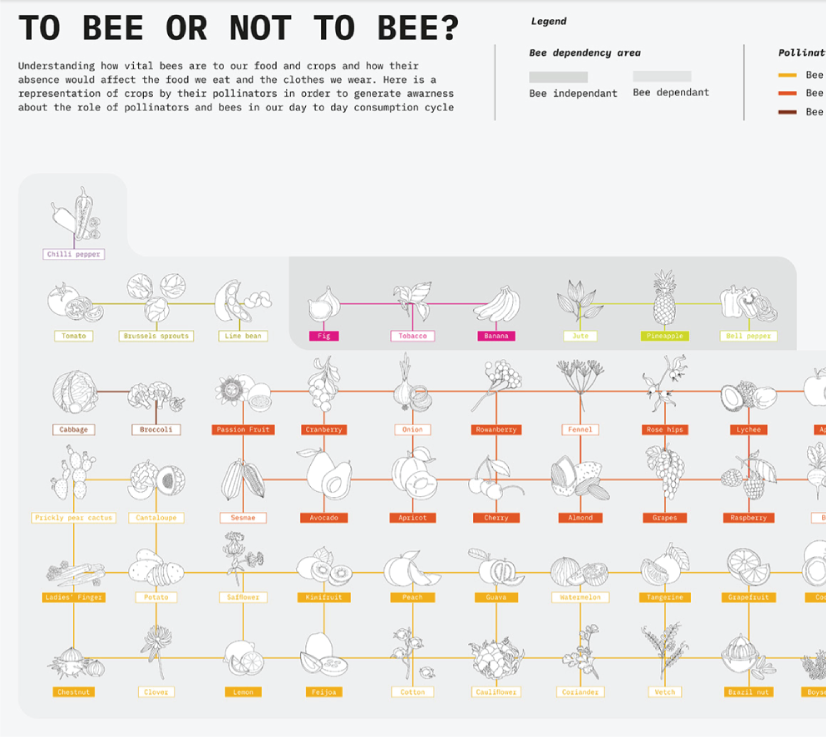
Data Visualisation | To bee or not to bee by Aishwarya Anand Singh
For example, charts are perfect to display data about a particular illness over a time period in a particular area, but a table comes to better use when you also need to understand specifics such as causes, outcomes, relapses, a period of treatment, and so on.
6. Data Visualization VS Infographics
5 main differences.
They are not that different as both visually represent data. It is often you search for infographics and find images titled Data Visualization and the other way around. In many cases, however, these titles aren’t misleading. Why is that?
- Data visualization is made of just one element. It could be a map, a chart, or a table. Infographics , on the other hand, often include multiple Data Viz elements.
- Unlike data visualizations that can be simple or extremely complex and heavy, infographics are simple and target wider audiences. The latter is usually comprehensible even to people outside of the field of research the infographic represents.
- Interestingly enough, data Viz doesn’t offer narratives and conclusions, it’s a tool and basis for reaching those. While infographics, in most cases offer a story and a narrative. For example, a data visualization map may have the title “Air pollution saturation by region”, while an infographic with the same data would go “Areas A and B are the most polluted in Country C”.
- Data visualizations can be made in Excel or use other tools that automatically generate the design unless they are set for presentation or publishing. The aesthetics of infographics , however, are of great importance and the designs must be appealing to wider audiences.
- In terms of interaction, data visualizations often offer interactive charts, especially in an online form. Infographics, on the other hand, rarely have interaction and are usually static images.
While on topic, you could also be interested to check out these 50 engaging infographic examples that make complex data look great.
7. Tips to Create Effective Data Visualization
The process is naturally similar to creating Infographics and it revolves around understanding your data and audience. To be more precise, these are the main steps and best practices when it comes to preparing an effective visualization of data for your viewers to instantly understand.
1. Do Your Homework
Preparation is half the work already done. Before you even start visualizing data, you have to be sure you understand that data to the last detail.
Knowing your audience is undeniable another important part of the homework, as different audiences process information differently. Who are the people you’re visualizing data for? How do they process visual data? Is it enough to hand them a single pie chart or you’ll need a more in-depth visual report?
The third part of preparing is to determine exactly what you want to communicate to the audience. What kind of information you’re visualizing and does it reflect your goal?
And last, think about how much data you’ll be working with and take it into account.
2. Choose the Right Type of Chart
In a previous section, we listed the basic chart types that find use in data visualization. To determine best which one suits your work, there are a few things to consider.
- How many variables will you have in a chart?
- How many items will you place for each of your variables?
- What will be the relation between the values (time period, comparison, distributions, etc.)
With that being said, a pie chart would be ideal if you need to present what portions of a whole takes each item. For example, you can use it to showcase what percent of the market share takes a particular product. Pie charts, however, are unsuitable for distributions, comparisons, and following trends through time periods. Bar graphs, scatter plots,s and line graphs are much more effective in those cases.
Another example is how to use time in your charts. It’s way more accurate to use a horizontal axis because time should run left to right. It’s way more visually intuitive.
3. Sort your Data
Start with removing every piece of data that does not add value and is basically excess for the chart. Sometimes, you have to work with a huge amount of data which will inevitably make your chart pretty complex and hard to read. Don’t hesitate to split your information into two or more charts. If that won’t work for you, you could use highlights or change the entire type of chart with something that would fit better.
Tip: When you use bar charts and columns for comparison, sort the information in an ascending or a descending way by value instead of alphabetical order.
4. Use Colors to Your Advantage
In every form of visualization, colors are your best friend and the most powerful tool. They create contrasts, accents, and emphasis and lead the eye intuitively. Even here, color theory is important.
When you design your chart, make sure you don’t use more than 5 or 6 colors. Anything more than that will make your graph overwhelming and hard to read for your viewers. However, color intensity is a different thing that you can use to your advantage. For example, when you compare the same concept in different periods of time, you could sort your data from the lightest shade of your chosen color to its darker one. It creates a strong visual progression, proper to your timeline.
Things to consider when you choose colors:
- Different colors for different categories.
- A consistent color palette for all charts in a series that you will later compare.
- It’s appropriate to use color blind-friendly palettes.
5. Get Inspired
Always put your inspiration to work when you want to be at the top of your game. Look through examples, infographics, and other people’s work and see what works best for each type of data you need to implement.
This Twitter account Data Visualization Society is a great way to start. In the meantime, we’ll also handpick some amazing examples that will get you in the mood to start creating the visuals for your data.
8. Examples for Data Visualization
As another art form, Data Viz is a fertile ground for some amazing well-designed graphs that prove that data is beautiful. Now let’s check out some.
Dark Souls III Experience Data
We start with Meng Hsiao Wei’s personal project presenting his experience with playing Dark Souls 3. It’s a perfect example that infographics and data visualization are tools for personal designs as well. The research is pretty massive yet very professionally sorted into different types of charts for the different concepts. All data visualizations are made with the same color palette and look great in infographics.
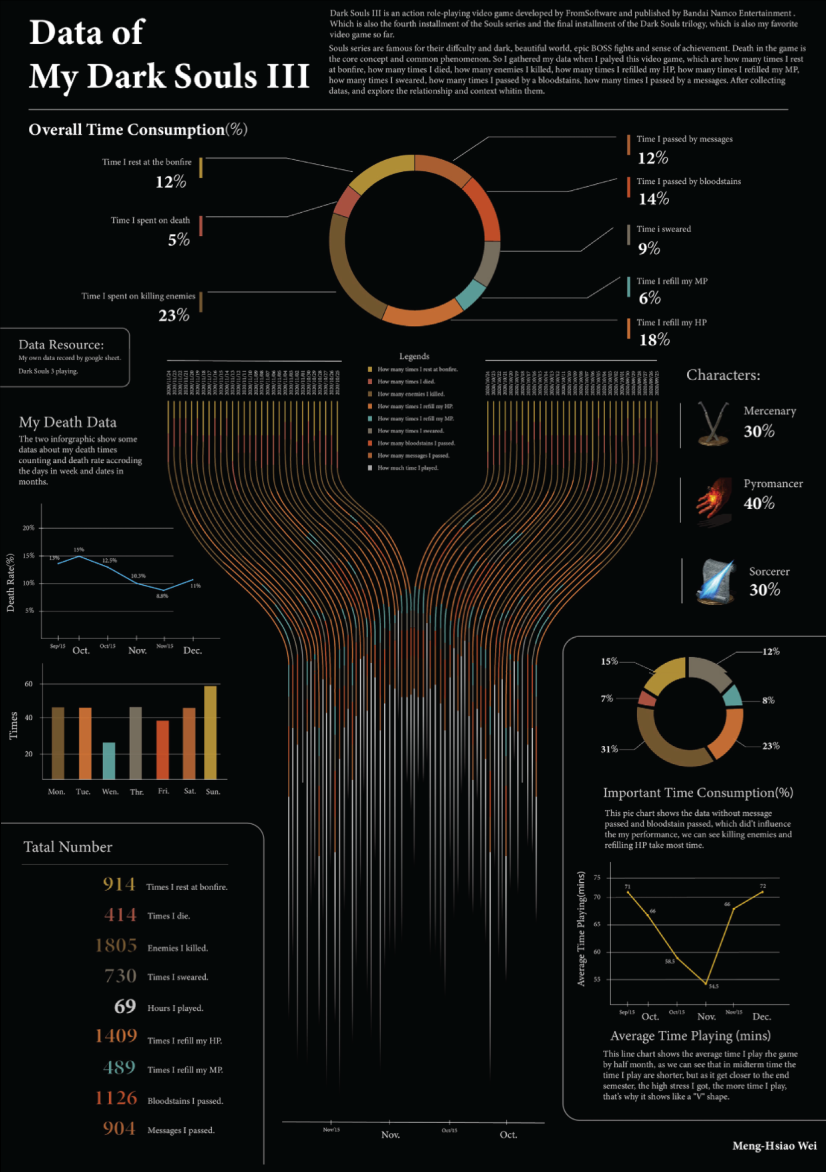
My dark souls 3 playing data by Meng Hsiao Wei
Greatest Movies of all Time
Katie Silver has compiled a list of the 100 greatest movies of all time based on critics and crowd reviews. The visualization shows key data points for every movie such as year of release, oscar nominations and wins, budget, gross, IMDB score, genre, filming location, setting of the film, and production studio. All movies are ordered by the release date.
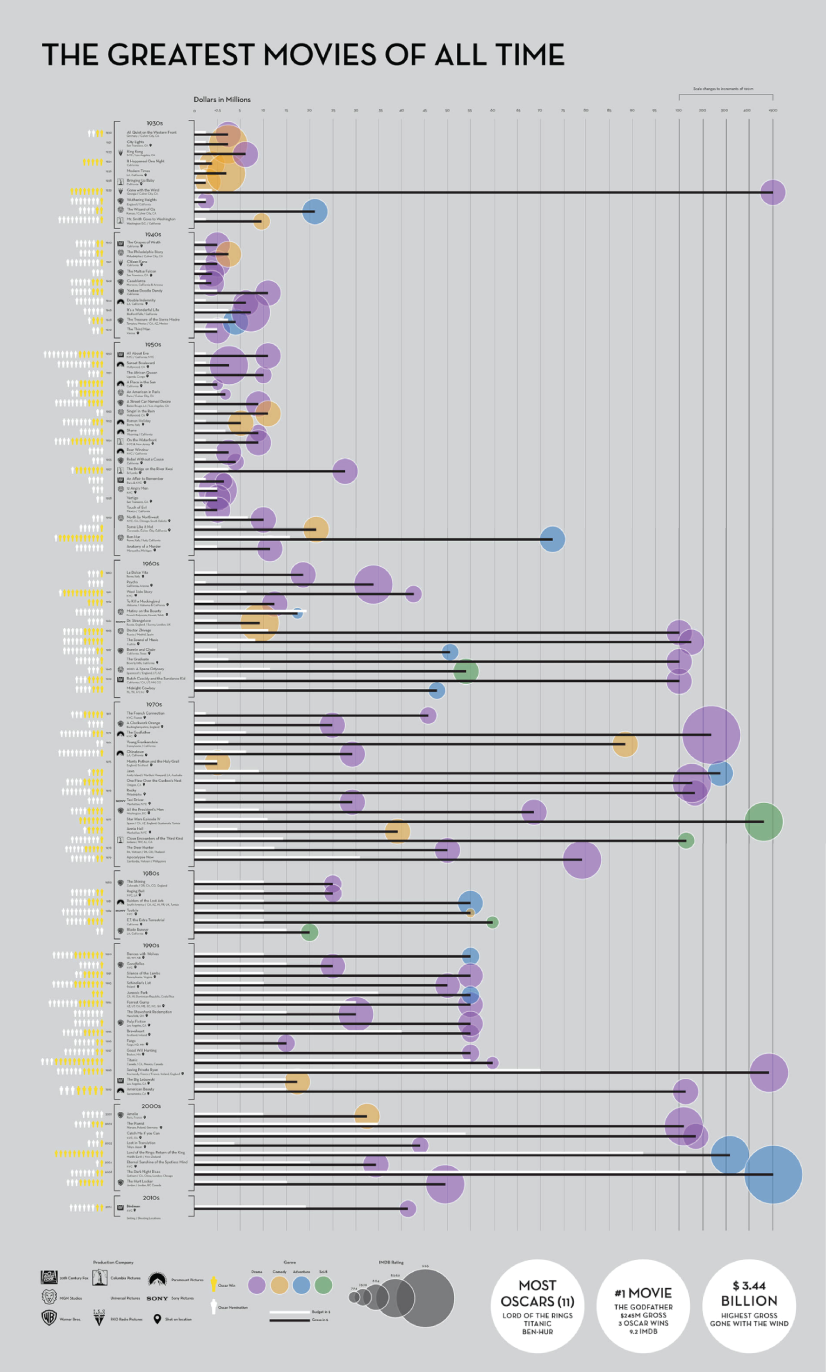
100 Greatest Movies Data Visualization by Katie Silver
The Most Violent Cities
Federica Fragapane shows data for the 50 most violent cities in the world in 2017. The items are arranged on a vertical axis based on population and ordered along the horizontal axis according to the homicide rate.
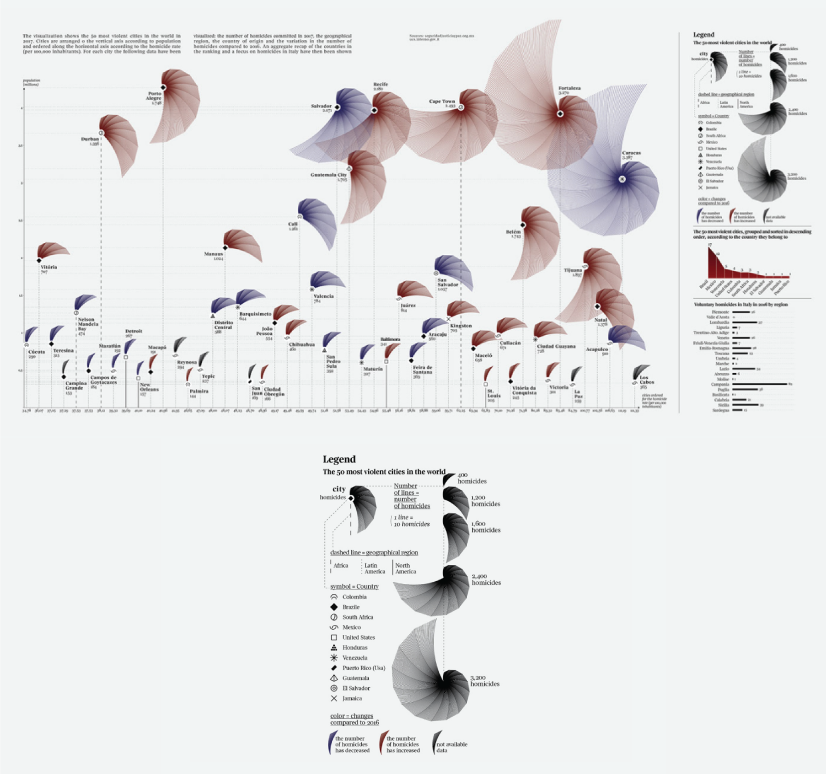
The Most Violent Cities by Federica Fragapane
Family Businesses as Data
These data visualizations and illustrations were made by Valerio Pellegrini for Perspectives Magazine. They show a pie chart with sector breakdown as well as a scatter plot for contribution for employment.
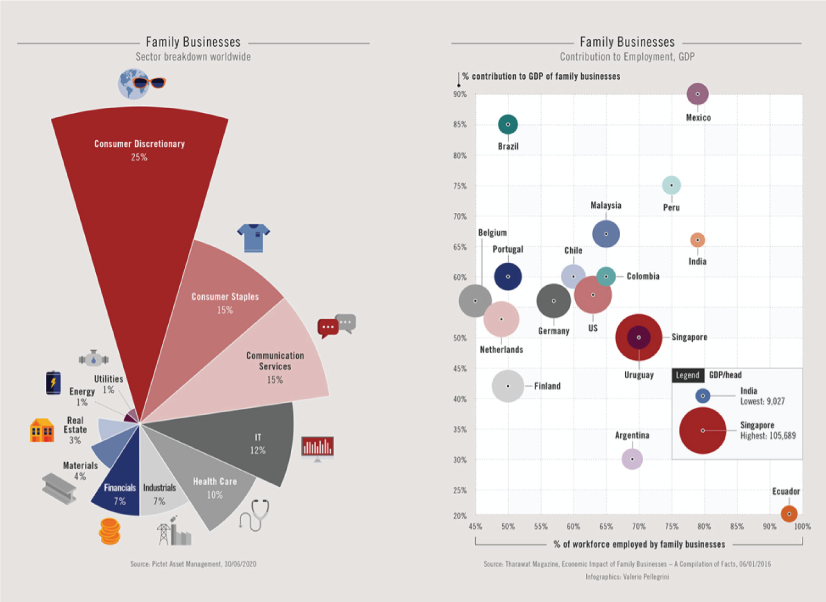
PERSPECTIVES MAGAZINE – Family Businesses by Valerio Pellegrini
Orbit Map of the Solar System
The map shows data on the orbits of more than 18000 asteroids in the solar system. Each asteroid is shown at its position on New Years’ Eve 1999, colored by type of asteroid.
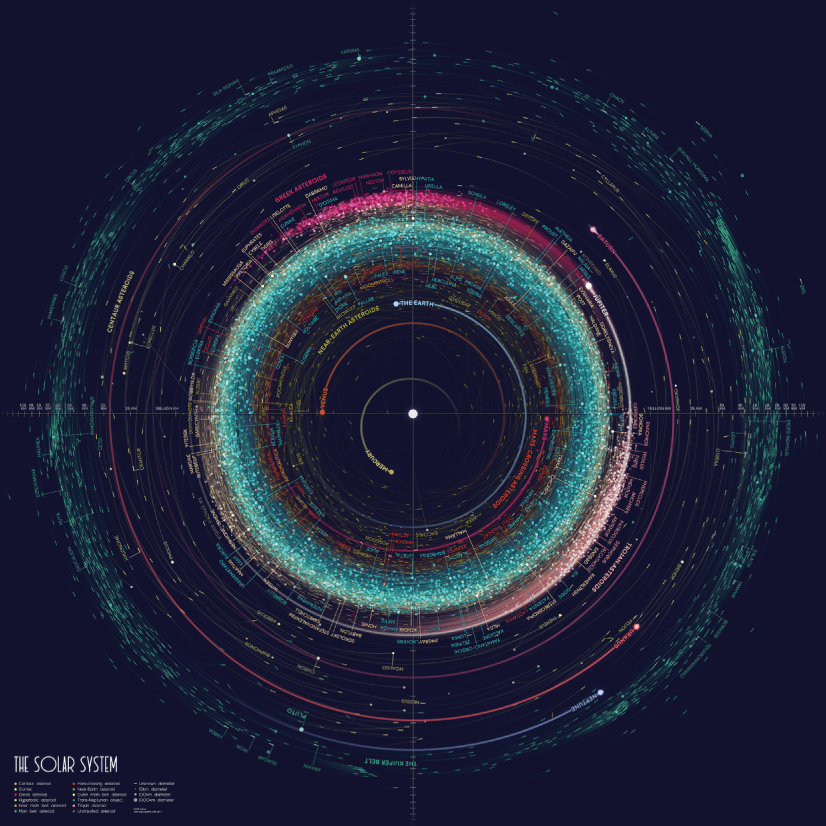
An Orbit Map of the Solar System by Eleanor Lutz
The Semantics Of Headlines
Katja Flükiger has a take on how headlines tell the story. The data visualization aims to communicate how much is the selling influencing the telling. The project was completed at Maryland Institute College of Art to visualize references to immigration and color-coding the value judgments implied by word choice and context.
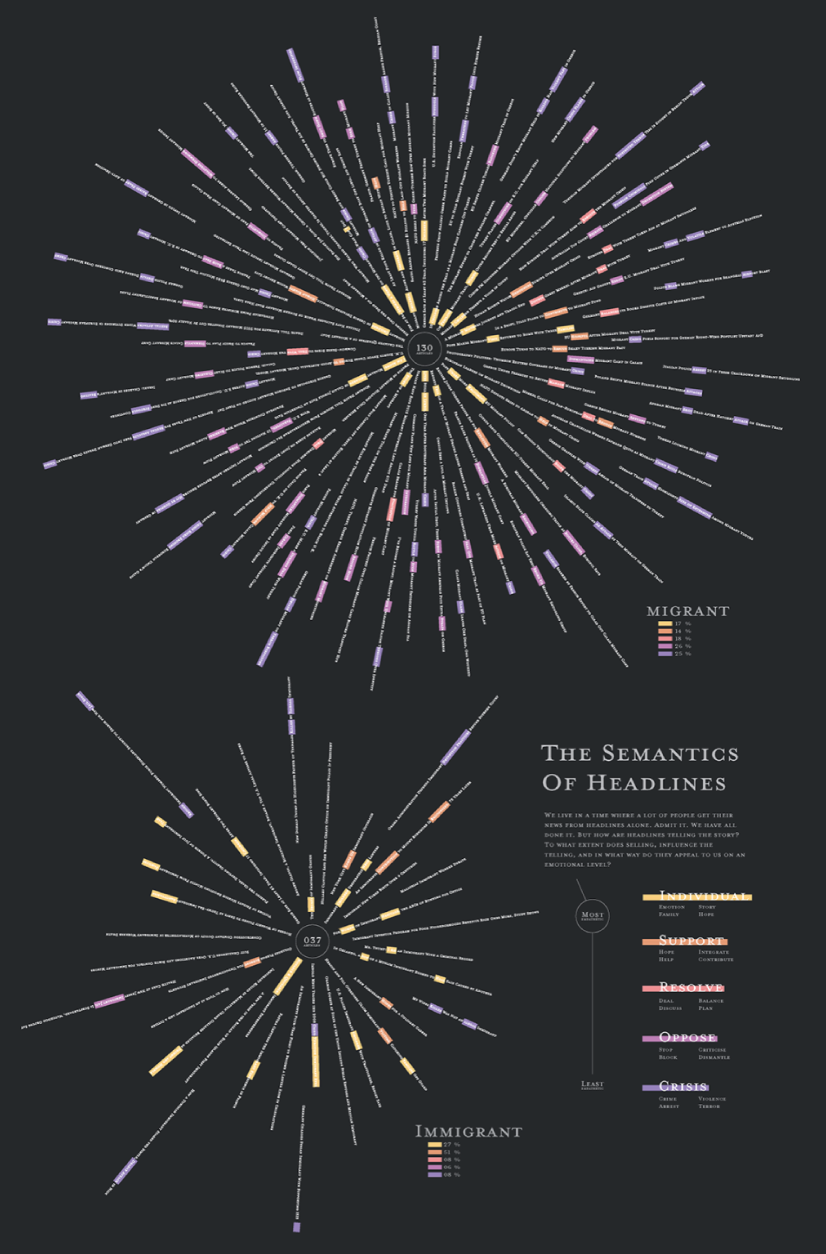
The Semantics of Headlines by Katja Flükiger
Moon and Earthquakes
This data visualization works on answering whether the moon is responsible for earthquakes. The chart features the time and intensity of earthquakes in response to the phase and orbit location of the moon.
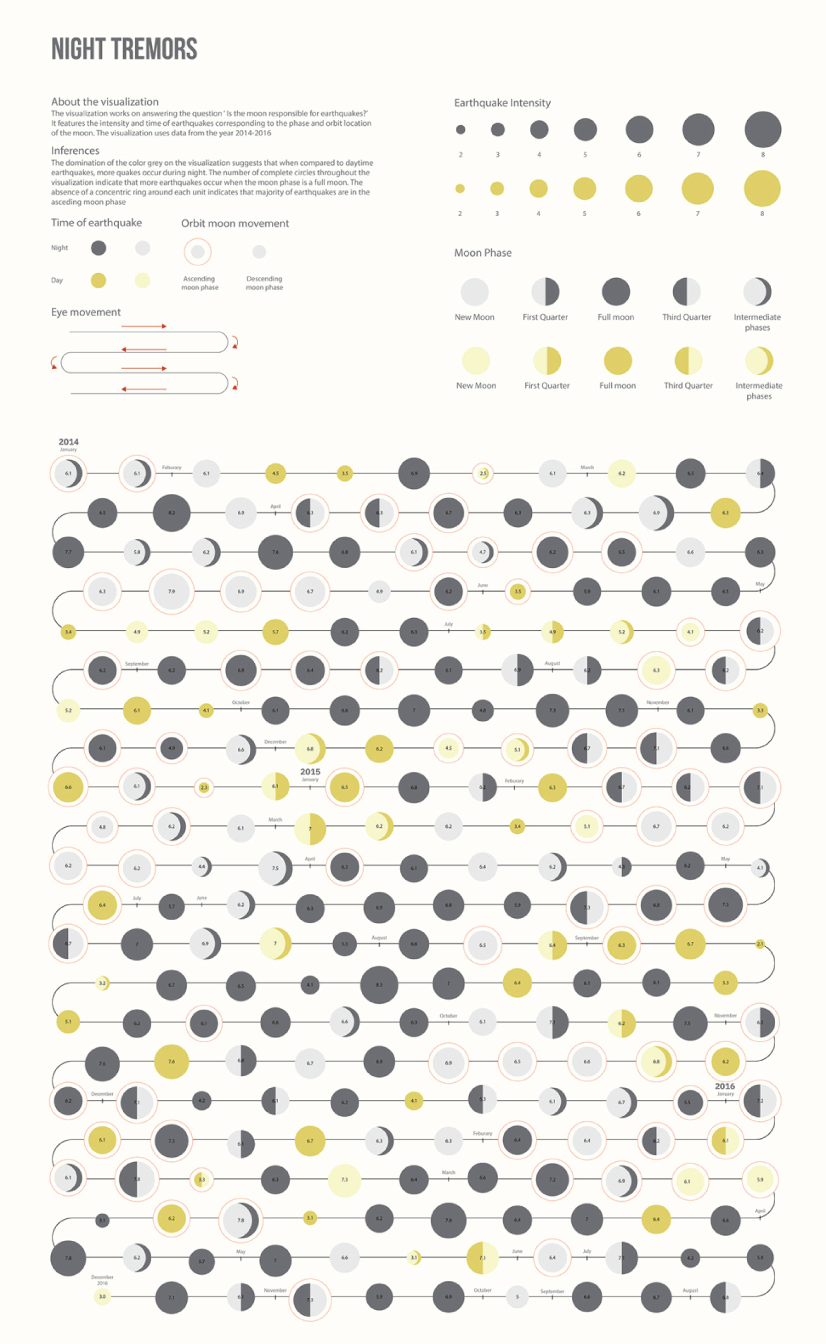
Moon and Earthquakes by Aishwarya Anand Singh
Dawn of the Nanosats
The visualization shows the satellites launched from 2003 to 2015. The graph represents the type of institutions focused on projects as well as the nations that financed them. On the left, it is shown the number of launches per year and satellite applications.
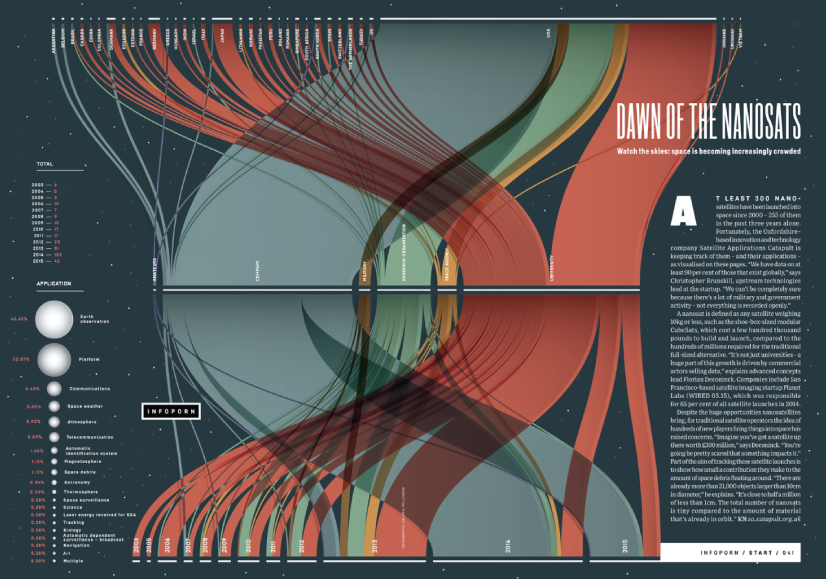
WIRED UK – Dawn of the by Nanosats by Valerio Pellegrini
Final Words
Data visualization is not only a form of science but also a form of art. Its purpose is to help businesses in any field quickly make sense of complex data and start making decisions based on that data. To make your graphs efficient and easy to read, it’s all about knowing your data and audience. This way you’ll be able to choose the right type of chart and use visual techniques to your advantage.
You may also be interested in some of these related articles:
- Infographics for Marketing: How to Grab and Hold the Attention
- 12 Animated Infographics That Will Engage Your Mind from Start to Finish
- 50 Engaging Infographic Examples That Make Complex Ideas Look Great
- Good Color Combinations That Go Beyond Trends: Inspirational Examples and Ideas
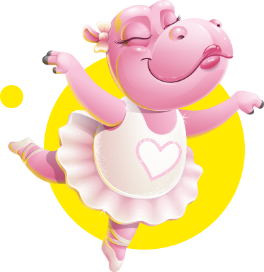
Add some character to your visuals
Cartoon Characters, Design Bundles, Illustrations, Backgrounds and more...
Like us on Facebook
Subscribe to our newsletter
Be the first to know what’s new in the world of graphic design and illustrations.
- [email protected]
Browse High Quality Vector Graphics
E.g.: businessman, lion, girl…
Related Articles
How to make cartoon animation like ted-ed, 21 most famous brand mascot designs of all time, subject matter expert & visuals for elearning projects, 10 tips you should read before hiring a freelancer, 6 trends for the classrooms of the future: what will change, check out our infographics bundle with 500+ infographic templates:, enjoyed this article.
Don’t forget to share!
- Comments (2)
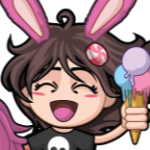
Al Boicheva
Al is an illustrator at GraphicMama with out-of-the-box thinking and a passion for anything creative. In her free time, you will see her drooling over tattoo art, Manga, and horror movies.
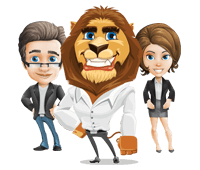
Thousands of vector graphics for your projects.
Hey! You made it all the way to the bottom!
Here are some other articles we think you may like:
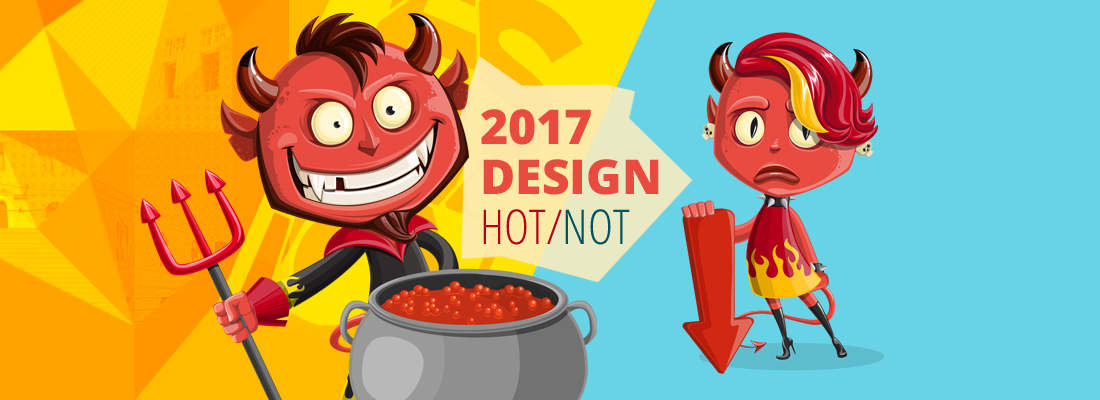
Graphic Design Trends 2017: What’s Hot and What’s Not
by Iveta Pavlova
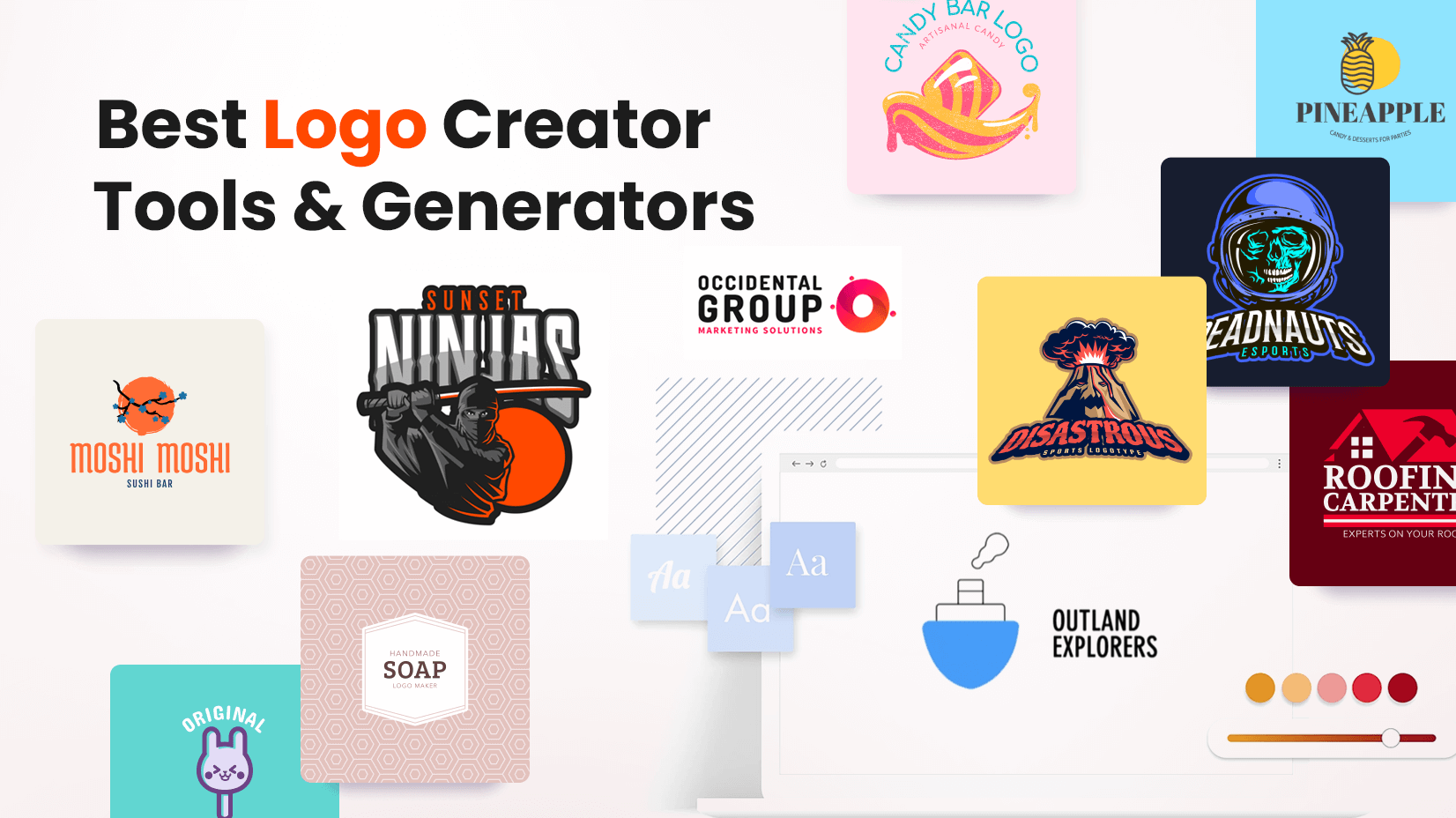
Best Logo Creator Tools & Generators: Fast, Easy, Cost-Effective
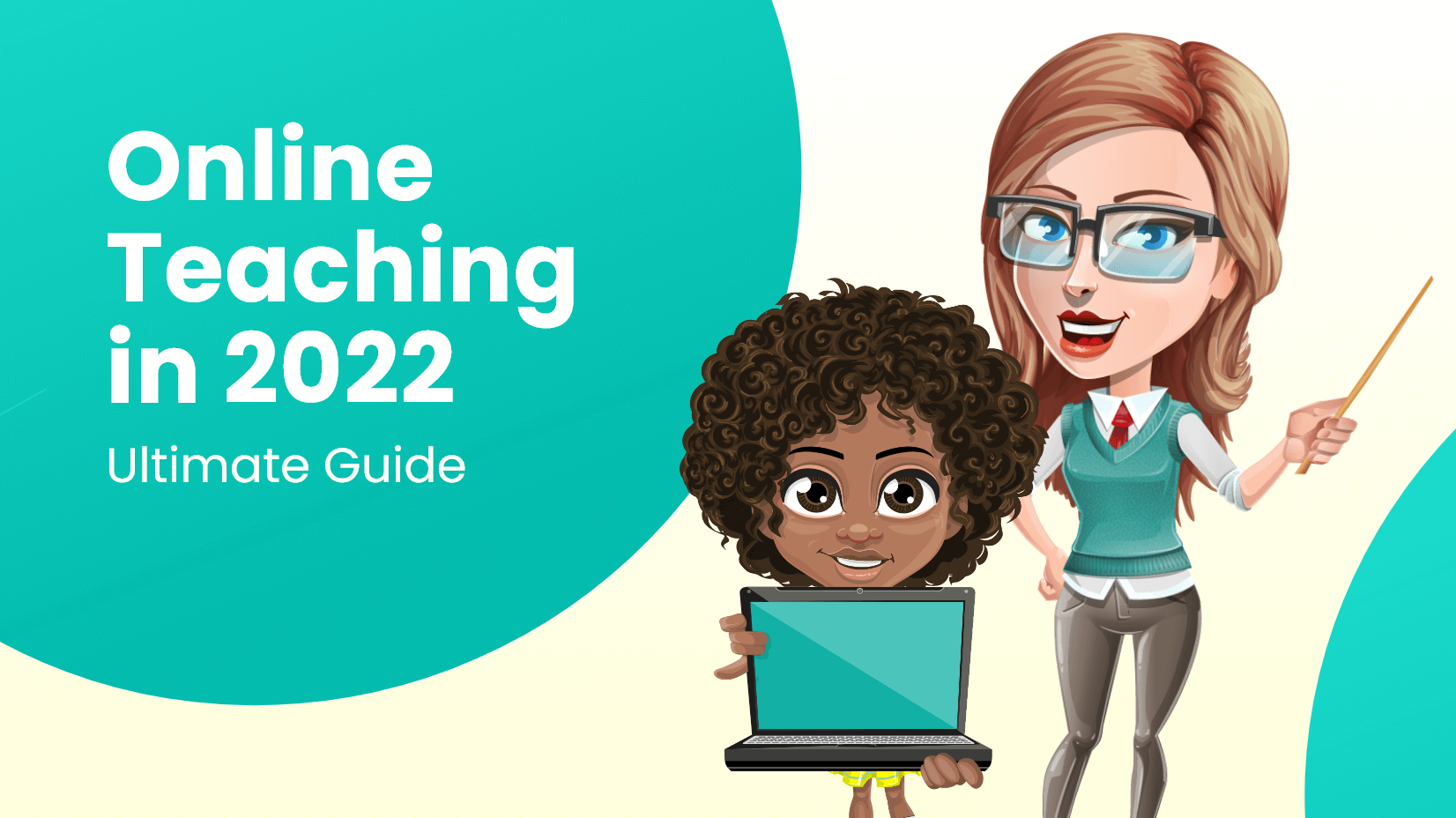
The Ultimate Guide to Online Teaching in 2022
by Boril Obreshkov
Looking for Design Bundles or Cartoon Characters?
A source of high-quality vector graphics offering a huge variety of premade character designs, graphic design bundles, Adobe Character Animator puppets, and more.

These cookies are required for the website to run and cannot be switched off. Such cookies are only set in response to actions made by you such as language, currency, login session, privacy preferences. You can set your browser to block these cookies but this might affect the way our site is working.
These cookies are usually set by our marketing and advertising partners. They may be used by them to build a profile of your interest and later show you relevant ads. If you do not allow these cookies you will not experience targeted ads for your interests.
These cookies enable our website to offer additional functions and personal settings. They can be set by us or by third-party service providers that we have placed on our pages. If you do not allow these cookies, these services may not work properly.
These cookies allow us to measure visitors traffic and see traffic sources by collecting information in data sets. They also help us understand which products and actions are more popular than others.
The Ultimate Guide to Data Visualization
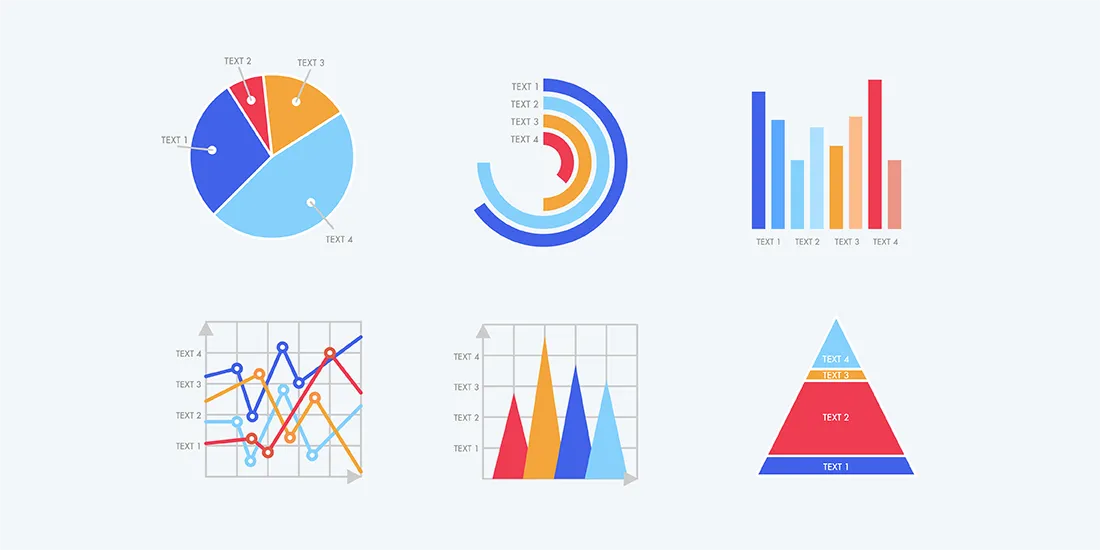
Data visualization is important because it breaks down complex data and extracts meaningful insights in a more digestible way. Displaying the data in a more engaging way helps audiences make sense of the information with a higher chance of retention. But with a variety of charts and graphs, how can you tell which is best for your specific content and audience?
Consider this your ultimate guide to data visualization. We’re breaking down popular charts and graphs and explaining the differences between each so that you can choose the best slide for your story.
Charts vs. graphs
We know that numbers don’t lie and are a strong way to back up your story, but that doesn’t always mean they’re easy to understand. By packaging up complex numbers and metrics in visually appealing graphics you’re telling your audience exactly what they need to know without having to rack their brain to comprehend it. Graphs and charts are important in your presentation because they take your supporting statistics, and story, and make them more relatable.
Charts present data or complex information through tables, infographics , and diagrams, while graphs show a connection between two or more sets of data.
A histogram is a visual representation of the distribution of data. The graph itself consists of a set of rectangles— each rectangle represents a range of values (called a "bin"), while the height corresponds to the numbers of the data that fall within that range.
Histograms are oftentimes used to visualize the frequency distribution of continuous data. Things such as measurements of height, weight, or time can all be organized in the graph. They can also be used to display the distribution of discrete data, like the number of shoes sold in a shoe department during any given period of time.
Histograms are a useful tool for analyzing data, as they allow you to quickly see the shape of the data distribution, the location of the central tendency (the mean or median), and the full spread of the data. They’re a great chart that can also reveal any changes in the data, making it easier to digest.
Need to add a little visual interest to your business presentation? A bar graph slide can display your data easily and effectively. Whether you use a vertical bar graph or horizontal bar graph, a bar graph gives you options to help simplify and present complex data, ensuring you get your point across.
Use it to track long-term changes.
Vertical bar graphs are great for comparing different groups that change over a long period of time. Small or short-term changes may not be as obvious in bar graph form.
Don’t be afraid to play with design .
You can use one bar graph template slide to display a lot of information, as long as you differentiate between data sets. Use colors, spacing, and labels to make the differences obvious.
Use a horizontal graph when necessary.
If your data labels are long, a horizontal bar graph may be easier to read and organize than a vertical bar graph.
Don’t use a horizontal graph to track time.
A vertical bar graph makes more sense when graphing data over time, since the x-axis is usually read from left to right.
Histograms vs. bar graphs
While a histogram is similar to a bar graph, it groups numbers into ranges and displays data in a different way.
Bar graphs are used to represent categorical data, where each bar represents a different category with a height or length proportional to the associated value. The categories of a bar graph don’t overlap, and the bars are usually separated by a gap to differentiate from one another. Bar graphs are ideal when you need to compare the data of different categories.
On the other hand, histograms divide data into a set of intervals or "bins". The bars of a histogram are typically adjacent to each other, with no gaps, as the bins are continuous and can overlap. Histograms are used to visualize the shape, center, and spread of a distribution of numerical data.
A pie chart is a circular graph (hence the name ‘pie’) that’s used to show or compare different segments — or ‘slices’ — of data. Each slice represents a proportion that relates to the whole. When added up, each slice should equal the total. Pie charts are best used for showcasing part-to-whole relationships. In other words, if you have different parts or percentages of a whole, using a pie chart is likely the way to go. Just make sure the total sum equals 100%, or the chart won’t make a lot of sense or convey the message you want it to. Essentially, any type of content or data that can be broken down into comparative categories is suitable to use. Revenue, demographics, market shares, survey results — these are just a few examples of the type of content to use in a pie chart. However, you don’t want to display more than six categories of data or the pie chart can be difficult to read and compare the relative size of slices.
Donut Charts
A donut chart is almost identical to a pie chart, but the center is cut out (hence the name ‘donut’). Donut charts are also used to show proportions of categories that make up the whole, but the center can also be used to display data. Like pie charts, donut charts can be used to display different data points that total 100%. These are also best used to compare a handful of categories at-a-glance and how they relate to the whole. The same type of content you’d use for a pie chart can also work for a donut chart. However, with donut charts, you have room for fewer categories than pie charts — anywhere from 2 to 5. That’s because you want your audience to be able to quickly tell the difference between arc lengths, which can help tell a more compelling story and get your point across more efficiently.
Pie charts vs. donut charts
You may notice that a donut chart and a pie chart look almost identical . While a donut chart is essentially the same as a pie chart in function, with its center cut out, the “slices” in a donut chart are sometimes more clearly defined than in a pie chart.
When deciding between a pie chart or a donut chart for your presentation, make sure the data you’re using is for comparison analysis only. Pie and donut charts are usually limited to just that — comparing the differences between categories. The easiest way to decide which one to use?
The number of categories you’re comparing. If you have more than 4 or 5 categories, go with a pie chart. If you have between 2 and 4 categories, go with a donut chart. Another way to choose? If you have an extra data point to convey (e.g. all of your categories equal an increase in total revenue), use a donut chart so you can take advantage of the space in the middle.
Comparison charts
As its name implies, a comparison chart or comparison graph draws a comparison between two or more items across different parameters. You might use a comparison chart to look at similarities and differences between items, weigh multiple products or services in order to choose one, or present a lot of data in an easy-to-read format.
For a visually interesting twist on a plain bar chart, add a data comparison slide to your presentation. Our data comparison template is similar to a bar graph, using bars of varying lengths to display measured data. The data comparison template, however, displays percentages instead of exact numbers. One of the best things about using Beautiful.ai’s data comparison slide? You can customize it for your presentation. Create a horizontal or vertical slide, remove or add grid lines, play with its design, and more.
Gantt charts
A Gantt chart , named after its early 20th century inventor Henry Gantt, is a birds-eye view of a project. It visually organizes tasks displayed over time. Gantt charts are incredibly useful tools that work for projects and groups of all sizes.
It’s a type of bar chart that you would use to show the start and finish dates of several elements of a project such as what the project tasks are, who is working on each task, how long each task will take, and how tasks group together, overlap, and link with each other. The left side of a Gantt chart lists each task in a project by name. Running along the top of the chart from left to right is a timeline. Depending on the demands and details of your project, the timeline may be broken down by quarter, month, week, or even day.
Project management can be complex, so it’s important to keep your chart simple by using a color scheme with cool colors like blues or greens. You can color code items thematically or by department or person, or even highlight a single task with a contrasting color to call attention to it. You can also choose to highlight important tasks using icons or use images for other annotations. This will make your chart easier to read and more visually appealing.
Additional tips for creating an effective Gantt chart slide .
Use different colors
How many colors you use and how you assign them is up to you. You might choose one color to represent a specific team or department so that you can see who is responsible for which tasks on your chart, for example.
Set milestones
Don’t forget to set milestones where they make sense: deadlines required by clients or customers, when a new department takes over the next phase of the project, or when a long list of tasks is completed.
Label your tasks
When used with a deliberate color scheme, labeling your tasks with its project owner will prevent confusion and make roles clear to everyone.

Jordan Turner
Jordan is a Bay Area writer, social media manager, and content strategist.
Recommended Articles
Bring your business intelligence report to life with data visualizations, when and how to use the venn diagram, the different types of graphs and charts your team needs to know in 2022, guide to doing market analysis: best practices and templates.

- Business Essentials
- Leadership & Management
- Credential of Leadership, Impact, and Management in Business (CLIMB)
- Entrepreneurship & Innovation
- Digital Transformation
- Finance & Accounting
- Business in Society
- For Organizations
- Support Portal
- Media Coverage
- Founding Donors
- Leadership Team
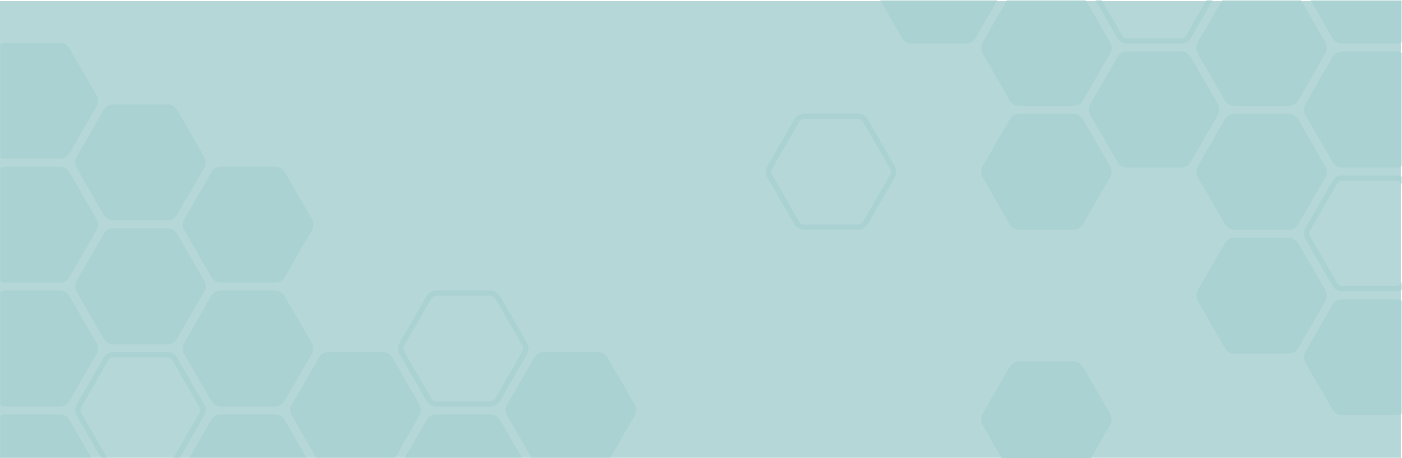
- Harvard Business School →
- HBS Online →
- Business Insights →
Business Insights
Harvard Business School Online's Business Insights Blog provides the career insights you need to achieve your goals and gain confidence in your business skills.
- Career Development
- Communication
- Decision-Making
- Earning Your MBA
- Negotiation
- News & Events
- Productivity
- Staff Spotlight
- Student Profiles
- Work-Life Balance
- AI Essentials for Business
- Alternative Investments
- Business Analytics
- Business Strategy
- Business and Climate Change
- Creating Brand Value
- Design Thinking and Innovation
- Digital Marketing Strategy
- Disruptive Strategy
- Economics for Managers
- Entrepreneurship Essentials
- Financial Accounting
- Global Business
- Launching Tech Ventures
- Leadership Principles
- Leadership, Ethics, and Corporate Accountability
- Leading Change and Organizational Renewal
- Leading with Finance
- Management Essentials
- Negotiation Mastery
- Organizational Leadership
- Power and Influence for Positive Impact
- Strategy Execution
- Sustainable Business Strategy
- Sustainable Investing
- Winning with Digital Platforms
6 Data Visualization Examples To Inspire Your Own
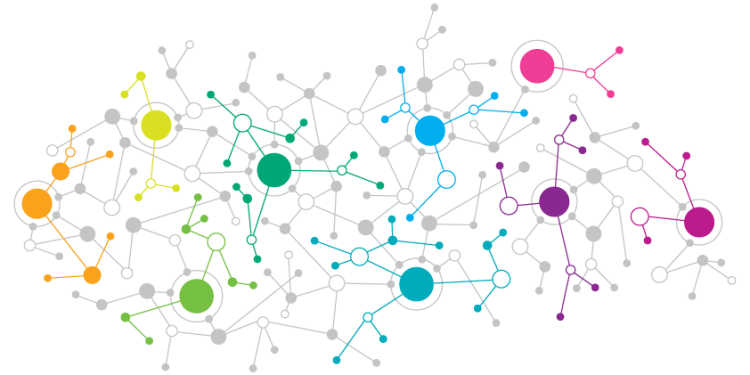
- 12 Jan 2017
Data informs virtually every business decision an organization makes. Because of this, it’s become increasingly important for professionals of all backgrounds to be adept at working with data.
While data can provide immense value, it’s important that professionals are able to effectively communicate the significance of the data to stakeholders. This is where data visualization comes into play. By transforming raw data into engaging visuals using various data visualization tools , it’s much easier to communicate insights gleaned from it.
Here are six real-world examples of data visualization that you can use to inspire your own.
What Is Data Visualization?
Data visualization is the process of turning raw data into graphical representations.
Visualizations make it easy to communicate trends in data and draw conclusions. When presented with a graph or chart, stakeholders can easily visualize the story the data is telling, rather than try to glean insights from raw data.
There are countless data visualization techniques , including:
- Scatter plots
The technique you use will vary based on the type of data you’re handling and what you’re trying to communicate.
6 Real-World Data Visualization Examples
1. the most common jobs by state.

Source: NPR
National Public Radio (NPR) produced a color-coded, interactive display of the most common jobs in each state in each year from 1978 to 2014. By dragging the scroll bar at the bottom of the map, you’re able to visualize occupational changes over time.
If you’re trying to represent geographical data, a map is the best way to go.
2. COVID-19 Hospitalization Rates

Source: CDC
Throughout the COVID-19 pandemic, the Centers for Disease Control and Prevention (CDC) has been transforming raw data into easily digestible visuals. This line graph represents COVID-19 hospitalization rates from March through November 2020.
The CDC tactfully incorporated color to place further emphasis on the stark increase in hospitalization rates, using a darker shade for lower values and a lighter shade for higher values.
3. Forecasted Revenue of Amazon.com

Source: Statista
Data visualizations aren’t limited to historical data. This bar chart created by Statista visualizes the forecasted gross revenue of Amazon.com from 2018 to 2025.
This visualization uses a creative title to summarize the main message that the data is conveying, as well as a darker orange color to spike out the most important data point.
4. Web-Related Statistics

Source: Internet Live Stats
Internet Live Stats has tracked web-related statistics and pioneered methods for visualizing data to show how different digital properties have ebbed and flowed over time.
Simple infographics like this one are particularly effective when your goal is to communicate key statistics rather than visualizing trends or forecasts.
5. Most Popular Food Delivery Items

Source: Eater
Eater, Vox’s food and dining brand, has created this fun take on a “pie” chart, which shows the most common foods ordered for delivery in each of the United States.
To visualize this data, Eater used a specific type of pie chart known as a spie chart. Spie charts are essentially pie charts in which you can vary the height of each segment to further visualize differences in data.
6. Netflix Viewing Patterns

Source: Vox
Vox created this interesting visualization depicting the viewing patterns of Netflix users over time by device type. This Sankey diagram visualizes the tendency of users to switch to streaming via larger device types.

Visualizing Data to Make Business Decisions
The insights and conclusions drawn from data visualizations can guide the decision-making and strategic planning processes for your organization.
To ensure your visualizations are relevant, accurate, and ethical, familiarize yourself with basic data science concepts . With a foundational knowledge in data science, you can maintain confidence in your data and better understand its significance. An online analytics course can help you get started.
Are you interested in improving your data science and analytical skills? Download our Beginner’s Guide to Data & Analytics to learn how you can leverage the power of data for professional and organizational success.
This post was updated on February 26, 2021. It was originally published on January 12, 2017.
18 Best Types of Charts and Graphs for Data Visualization [+ Guide]

Published: May 22, 2024
As a writer for the marketing blog, I frequently use various types of charts and graphs to help readers visualize the data I collect and better understand their significance. And trust me, there's a lot of data to present.
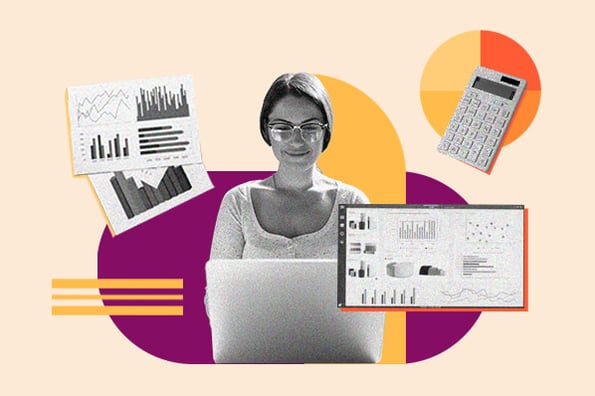
In fact, the volume of data in 2025 will be almost double the data we create, capture, copy, and consume today.

This makes data visualization essential for businesses. Different types of graphs and charts can help you:
- Motivate your team to take action.
- Impress stakeholders with goal progress.
- Show your audience what you value as a business.
Data visualization builds trust and can organize diverse teams around new initiatives. So, I'm going to talk about the types of graphs and charts that you can use to grow your business.
And, if you still need a little more guidance by the end of this post, check out our data visualization guide for more information on how to design visually stunning and engaging charts and graphs.
.png)
Free Excel Graph Templates
Tired of struggling with spreadsheets? These free Microsoft Excel Graph Generator Templates can help.
- Simple, customizable graph designs.
- Data visualization tips & instructions.
- Templates for two, three, four, and five-variable graph templates.
Download Free
All fields are required.
You're all set!
Click this link to access this resource at any time.
Charts vs Graphs: What's the Difference?
A lot of people think charts and graphs are synonymous (I know I did), but they're actually two different things.
Charts visually represent current data in the form of tables and diagrams, but graphs are more numerical in data and show how one variable affects another.
For example, in one of my favorite sitcoms, How I Met Your Mother, Marshall creates a bunch of charts and graphs representing his life. One of these charts is a Venn diagram referencing the song "Cecilia" by Simon and Garfunkle.
Marshall says, "This circle represents people who are breaking my heart, and this circle represents people who are shaking my confidence daily. Where they overlap? Cecilia."
The diagram is a chart and not a graph because it doesn't track how these people make him feel over time or how these variables are influenced by each other.
It may show where the two types of people intersect but not how they influence one another.

Later, Marshall makes a line graph showing how his friends' feelings about his charts have changed in the time since presenting his "Cecilia diagram.
Note: He calls the line graph a chart on the show, but it's acceptable because the nature of line graphs and charts makes the terms interchangeable. I'll explain later, I promise.
The line graph shows how the time since showing his Cecilia chart has influenced his friends' tolerance for his various graphs and charts.

Image source
I can't even begin to tell you all how happy I am to reference my favorite HIMYM joke in this post.
Now, let's dive into the various types of graphs and charts.
Different Types of Graphs for Data Visualization
1. bar graph.
I strongly suggest using a bar graph to avoid clutter when one data label is long or if you have more than 10 items to compare. Also, fun fact: If the example below was vertical it would be a column graph.

Best Use Cases for These Types of Graphs
Bar graphs can help track changes over time. I've found that bar graphs are most useful when there are big changes or to show how one group compares against other groups.
The example above compares the number of customers by business role. It makes it easy to see that there is more than twice the number of customers per role for individual contributors than any other group.
A bar graph also makes it easy to see which group of data is highest or most common.
For example, at the start of the pandemic, online businesses saw a big jump in traffic. So, if you want to look at monthly traffic for an online business, a bar graph would make it easy to see that jump.
Other use cases for bar graphs include:
- Product comparisons.
- Product usage.
- Category comparisons.
- Marketing traffic by month or year.
- Marketing conversions.
Design Best Practices for Bar Graphs
- Use consistent colors throughout the chart, selecting accent colors to highlight meaningful data points or changes over time.
You should also use horizontal labels to improve its readability, and start the y-axis at 0 to appropriately reflect the values in your graph.
2. Line Graph
A line graph reveals trends or progress over time, and you can use it to show many different categories of data. You should use it when you track a continuous data set.
This makes the terms line graphs and line charts interchangeable because the very nature of both is to track how variables impact each other, particularly how something changes over time. Yeah, it confused me, too.
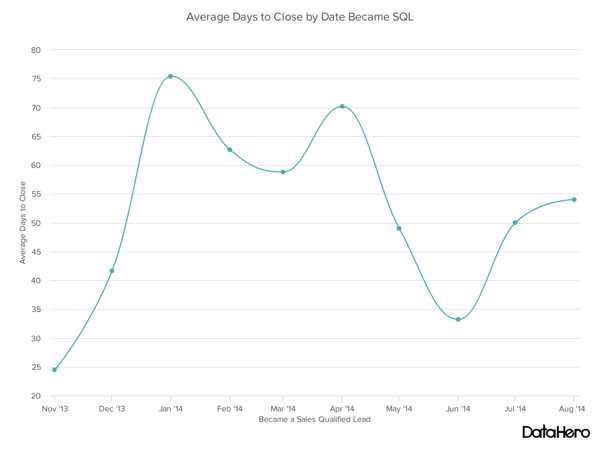
Line graphs help users track changes over short and long periods. Because of this, I find these types of graphs are best for seeing small changes.
Line graphs help me compare changes for more than one group over the same period. They're also helpful for measuring how different groups relate to each other.
A business might use this graph to compare sales rates for different products or services over time.
These charts are also helpful for measuring service channel performance. For example, a line graph that tracks how many chats or emails your team responds to per month.
Design Best Practices for Line Graphs
- Use solid lines only.
- Don't plot more than four lines to avoid visual distractions.
- Use the right height so the lines take up roughly 2/3 of the y-axis' height.
3. Bullet Graph
A bullet graph reveals progress towards a goal, compares this to another measure, and provides context in the form of a rating or performance.
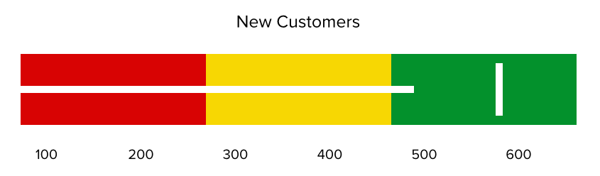
In the example above, the bullet graph shows the number of new customers against a set customer goal. Bullet graphs are great for comparing performance against goals like this.
These types of graphs can also help teams assess possible roadblocks because you can analyze data in a tight visual display.
For example, I could create a series of bullet graphs measuring performance against benchmarks or use a single bullet graph to visualize these KPIs against their goals:
- Customer satisfaction.
- Average order size.
- New customers.
Seeing this data at a glance and alongside each other can help teams make quick decisions.
Bullet graphs are one of the best ways to display year-over-year data analysis. YBullet graphs can also visualize:
- Customer satisfaction scores.
- Customer shopping habits.
- Social media usage by platform.
Design Best Practices for Bullet Graphs
- Use contrasting colors to highlight how the data is progressing.
- Use one color in different shades to gauge progress.
4. Column + Line Graph
Column + line graphs are also called dual-axis charts. They consist of a column and line graph together, with both graphics on the X axis but occupying their own Y axis.
Download our FREE Excel Graph Templates for this graph and more!
Best Use Cases
These graphs are best for comparing two data sets with different measurement units, such as rate and time.
As a marketer, you may want to track two trends at once.
Design Best Practices
Use individual colors for the lines and colors to make the graph more visually appealing and to further differentiate the data.
The Four Basic Types of Charts
Before we get into charts, I want to touch on the four basic chart types that I use the most.
1. Bar Chart
Bar charts are pretty self-explanatory. I use them to indicate values by the length of bars, which can be displayed horizontally or vertically. Vertical bar charts, like the one below, are sometimes called column charts.

2. Line Chart
I use line charts to show changes in values across continuous measurements, such as across time, generations, or categories. For example, the chart below shows the changes in ice cream sales throughout the week.

3. Scatter Plot
A scatter plot uses dotted points to compare values against two different variables on separate axes. It's commonly used to show correlations between values and variables.

4. Pie Chart
Pie charts are charts that represent data in a circular (pie-shaped) graphic, and each slice represents a percentage or portion of the whole.
Notice the example below of a household budget. (Which reminds me that I need to set up my own.)
Notice that the percentage of income going to each expense is represented by a slice.

Different Types of Charts for Data Visualization
To better understand chart types and how you can use them, here's an overview of each:
1. Column Chart
Use a column chart to show a comparison among different items or to show a comparison of items over time. You could use this format to see the revenue per landing page or customers by close date.
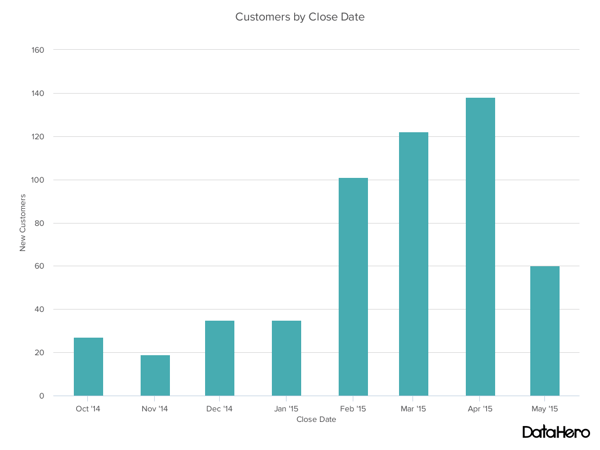
Best Use Cases for This Type of Chart
I use both column charts to display changes in data, but I've noticed column charts are best for negative data. The main difference, of course, is that column charts show information vertically while bar charts show data horizontally.
For example, warehouses often track the number of accidents on the shop floor. When the number of incidents falls below the monthly average, a column chart can make that change easier to see in a presentation.
In the example above, this column chart measures the number of customers by close date. Column charts make it easy to see data changes over a period of time. This means that they have many use cases, including:
- Customer survey data, like showing how many customers prefer a specific product or how much a customer uses a product each day.
- Sales volume, like showing which services are the top sellers each month or the number of sales per week.
- Profit and loss, showing where business investments are growing or falling.
Design Best Practices for Column Charts
- Use horizontal labels to improve readability.
- Start the y-axis at 0 to appropriately reflect the values in your chart .
2. Area Chart
Okay, an area chart is basically a line chart, but I swear there's a meaningful difference.
The space between the x-axis and the line is filled with a color or pattern. It is useful for showing part-to-whole relations, like showing individual sales reps’ contributions to total sales for a year.
It helps me analyze both overall and individual trend information.
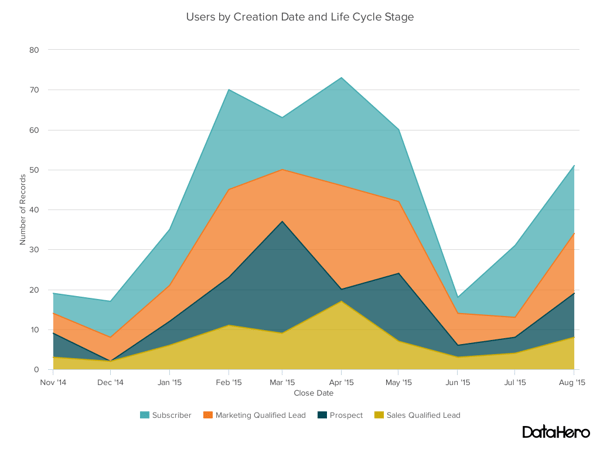
Best Use Cases for These Types of Charts
Area charts help show changes over time. They work best for big differences between data sets and help visualize big trends.
For example, the chart above shows users by creation date and life cycle stage.
A line chart could show more subscribers than marketing qualified leads. But this area chart emphasizes how much bigger the number of subscribers is than any other group.
These charts make the size of a group and how groups relate to each other more visually important than data changes over time.
Area charts can help your business to:
- Visualize which product categories or products within a category are most popular.
- Show key performance indicator (KPI) goals vs. outcomes.
- Spot and analyze industry trends.
Design Best Practices for Area Charts
- Use transparent colors so information isn't obscured in the background.
- Don't display more than four categories to avoid clutter.
- Organize highly variable data at the top of the chart to make it easy to read.
3. Stacked Bar Chart
I suggest using this chart to compare many different items and show the composition of each item you’re comparing.
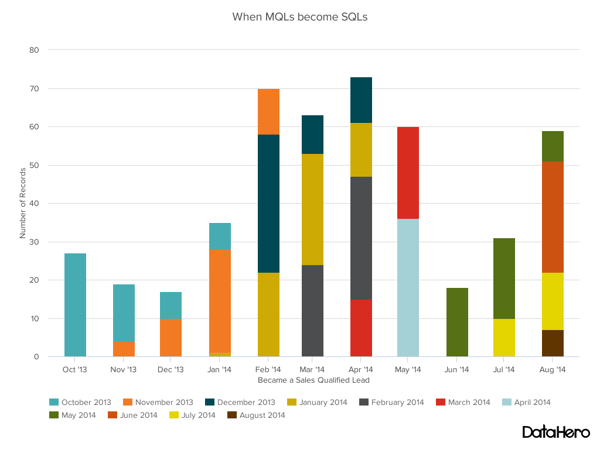
These charts are helpful when a group starts in one column and moves to another over time.
For example, the difference between a marketing qualified lead (MQL) and a sales qualified lead (SQL) is sometimes hard to see. The chart above helps stakeholders see these two lead types from a single point of view — when a lead changes from MQL to SQL.
Stacked bar charts are excellent for marketing. They make it simple to add a lot of data on a single chart or to make a point with limited space.
These charts can show multiple takeaways, so they're also super for quarterly meetings when you have a lot to say but not a lot of time to say it.
Stacked bar charts are also a smart option for planning or strategy meetings. This is because these charts can show a lot of information at once, but they also make it easy to focus on one stack at a time or move data as needed.
You can also use these charts to:
- Show the frequency of survey responses.
- Identify outliers in historical data.
- Compare a part of a strategy to its performance as a whole.
Design Best Practices for Stacked Bar Charts
- Best used to illustrate part-to-whole relationships.
- Use contrasting colors for greater clarity.
- Make the chart scale large enough to view group sizes in relation to one another.
4. Mekko Chart
Also known as a Marimekko chart, this type of chart can compare values, measure each one's composition, and show data distribution across each one.
It's similar to a stacked bar, except the Mekko's x-axis can capture another dimension of your values — instead of time progression, like column charts often do. In the graphic below, the x-axis compares the cities to one another.
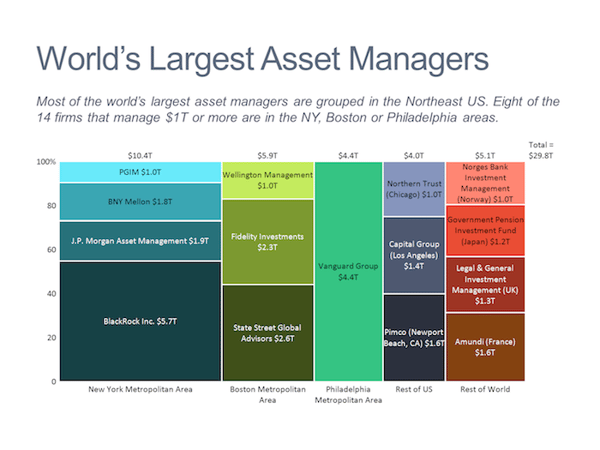
Image Source
I typically use a Mekko chart to show growth, market share, or competitor analysis.
For example, the Mekko chart above shows the market share of asset managers grouped by location and the value of their assets. This chart clarifies which firms manage the most assets in different areas.
It's also easy to see which asset managers are the largest and how they relate to each other.
Mekko charts can seem more complex than other types of charts, so it's best to use these in situations where you want to emphasize scale or differences between groups of data.
Other use cases for Mekko charts include:
- Detailed profit and loss statements.
- Revenue by brand and region.
- Product profitability.
- Share of voice by industry or niche.
Design Best Practices for Mekko Charts
- Vary your bar heights if the portion size is an important point of comparison.
- Don't include too many composite values within each bar. Consider reevaluating your presentation if you have a lot of data.
- Order your bars from left to right in such a way that exposes a relevant trend or message.
5. Pie Chart
Remember, a pie chart represents numbers in percentages, and the total sum of all segments needs to equal 100%.
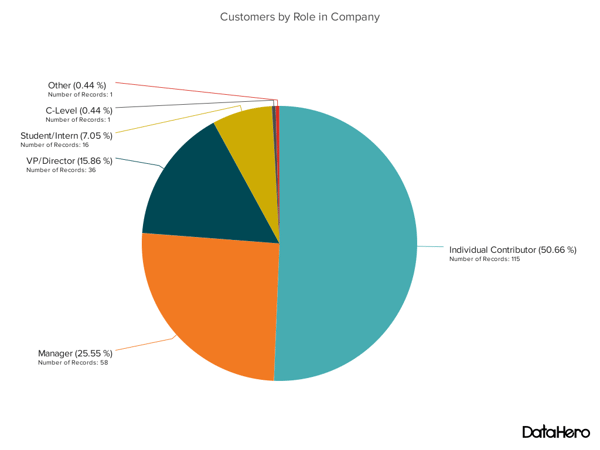
The image above shows another example of customers by role in the company.
The bar chart example shows you that there are more individual contributors than any other role. But this pie chart makes it clear that they make up over 50% of customer roles.
Pie charts make it easy to see a section in relation to the whole, so they are good for showing:
- Customer personas in relation to all customers.
- Revenue from your most popular products or product types in relation to all product sales.
- Percent of total profit from different store locations.
Design Best Practices for Pie Charts
- Don't illustrate too many categories to ensure differentiation between slices.
- Ensure that the slice values add up to 100%.
- Order slices according to their size.
6. Scatter Plot Chart
As I said earlier, a scatter plot or scattergram chart will show the relationship between two different variables or reveal distribution trends.
Use this chart when there are many different data points, and you want to highlight similarities in the data set. This is useful when looking for outliers or understanding your data's distribution.
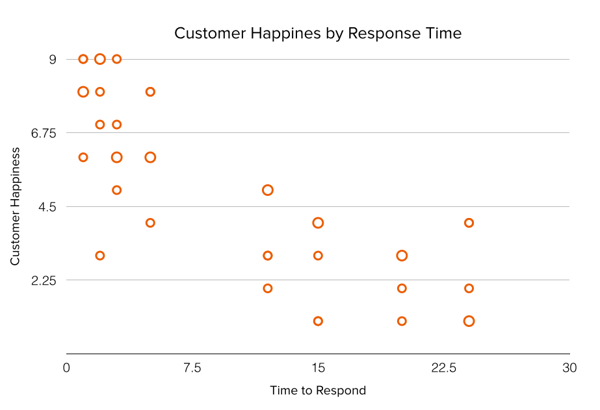
Scatter plots are helpful in situations where you have too much data to see a pattern quickly. They are best when you use them to show relationships between two large data sets.
In the example above, this chart shows how customer happiness relates to the time it takes for them to get a response.
This type of chart makes it easy to compare two data sets. Use cases might include:
- Employment and manufacturing output.
- Retail sales and inflation.
- Visitor numbers and outdoor temperature.
- Sales growth and tax laws.
Try to choose two data sets that already have a positive or negative relationship. That said, this type of chart can also make it easier to see data that falls outside of normal patterns.
Design Best Practices for Scatter Plots
- Include more variables, like different sizes, to incorporate more data.
- Start the y-axis at 0 to represent data accurately.
- If you use trend lines, only use a maximum of two to make your plot easy to understand.
7. Bubble Chart
A bubble chart is similar to a scatter plot in that it can show distribution or relationship. There is a third data set shown by the size of the bubble or circle.
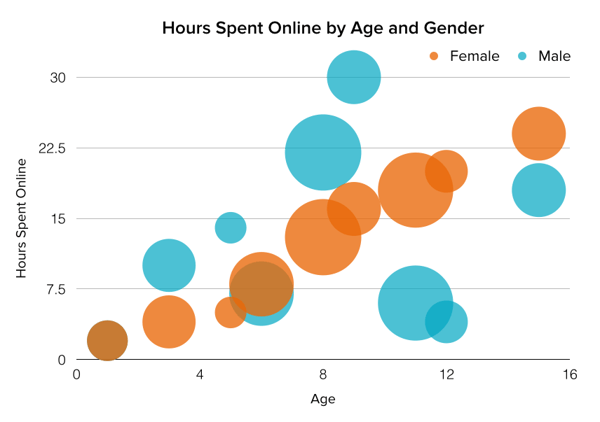
In the example above, the number of hours spent online isn't just compared to the user's age, as it would be on a scatter plot chart.
Instead, you can also see how the gender of the user impacts time spent online.
This makes bubble charts useful for seeing the rise or fall of trends over time. It also lets you add another option when you're trying to understand relationships between different segments or categories.
For example, if you want to launch a new product, this chart could help you quickly see your new product's cost, risk, and value. This can help you focus your energies on a low-risk new product with a high potential return.
You can also use bubble charts for:
- Top sales by month and location.
- Customer satisfaction surveys.
- Store performance tracking.
- Marketing campaign reviews.
Design Best Practices for Bubble Charts
- Scale bubbles according to area, not diameter.
- Make sure labels are clear and visible.
- Use circular shapes only.
8. Waterfall Chart
I sometimes use a waterfall chart to show how an initial value changes with intermediate values — either positive or negative — and results in a final value.
Use this chart to reveal the composition of a number. An example of this would be to showcase how different departments influence overall company revenue and lead to a specific profit number.
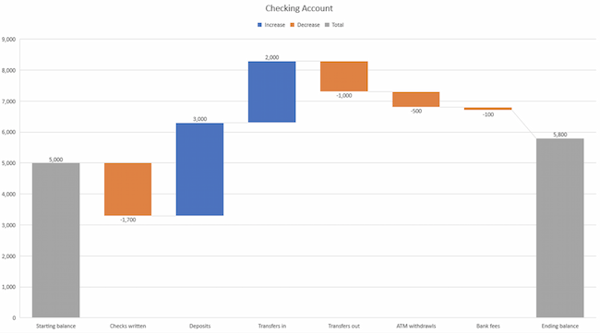
The most common use case for a funnel chart is the marketing or sales funnel. But there are many other ways to use this versatile chart.
If you have at least four stages of sequential data, this chart can help you easily see what inputs or outputs impact the final results.
For example, a funnel chart can help you see how to improve your buyer journey or shopping cart workflow. This is because it can help pinpoint major drop-off points.
Other stellar options for these types of charts include:
- Deal pipelines.
- Conversion and retention analysis.
- Bottlenecks in manufacturing and other multi-step processes.
- Marketing campaign performance.
- Website conversion tracking.
Design Best Practices for Funnel Charts
- Scale the size of each section to accurately reflect the size of the data set.
- Use contrasting colors or one color in graduated hues, from darkest to lightest, as the size of the funnel decreases.
10. Heat Map
A heat map shows the relationship between two items and provides rating information, such as high to low or poor to excellent. This chart displays the rating information using varying colors or saturation.
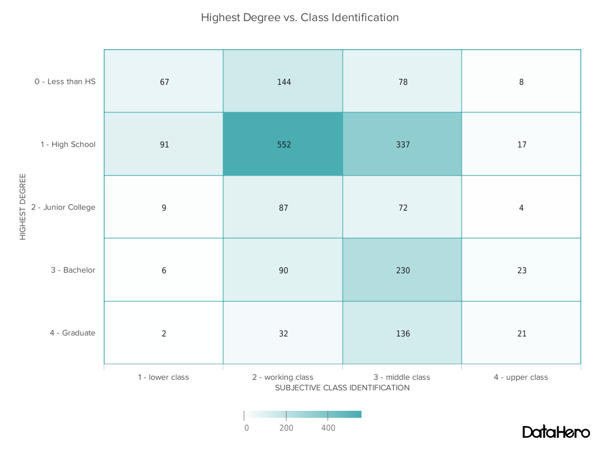
Best Use Cases for Heat Maps
In the example above, the darker the shade of green shows where the majority of people agree.
With enough data, heat maps can make a viewpoint that might seem subjective more concrete. This makes it easier for a business to act on customer sentiment.
There are many uses for these types of charts. In fact, many tech companies use heat map tools to gauge user experience for apps, online tools, and website design .
Another common use for heat map charts is location assessment. If you're trying to find the right location for your new store, these maps can give you an idea of what the area is like in ways that a visit can't communicate.
Heat maps can also help with spotting patterns, so they're good for analyzing trends that change quickly, like ad conversions. They can also help with:
- Competitor research.
- Customer sentiment.
- Sales outreach.
- Campaign impact.
- Customer demographics.
Design Best Practices for Heat Map
- Use a basic and clear map outline to avoid distracting from the data.
- Use a single color in varying shades to show changes in data.
- Avoid using multiple patterns.
11. Gantt Chart
The Gantt chart is a horizontal chart that dates back to 1917. This chart maps the different tasks completed over a period of time.
Gantt charting is one of the most essential tools for project managers. It brings all the completed and uncompleted tasks into one place and tracks the progress of each.
While the left side of the chart displays all the tasks, the right side shows the progress and schedule for each of these tasks.
This chart type allows you to:
- Break projects into tasks.
- Track the start and end of the tasks.
- Set important events, meetings, and announcements.
- Assign tasks to the team and individuals.
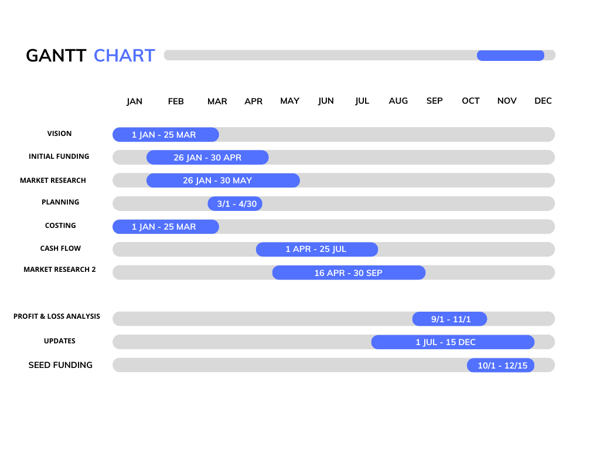
I use donut charts for the same use cases as pie charts, but I tend to prefer the former because of the added benefit that the data is easier to read.
Another benefit to donut charts is that the empty center leaves room for extra layers of data, like in the examples above.
Design Best Practices for Donut Charts
Use varying colors to better differentiate the data being displayed, just make sure the colors are in the same palette so viewers aren't put off by clashing hues.
14. Sankey Diagram
A Sankey Diagram visually represents the flow of data between categories, with the link width reflecting the amount of flow. It’s a powerful tool for uncovering the stories hidden in your data.
As data grows more complex, charts must evolve to handle these intricate relationships. Sankey Diagrams excel at this task.
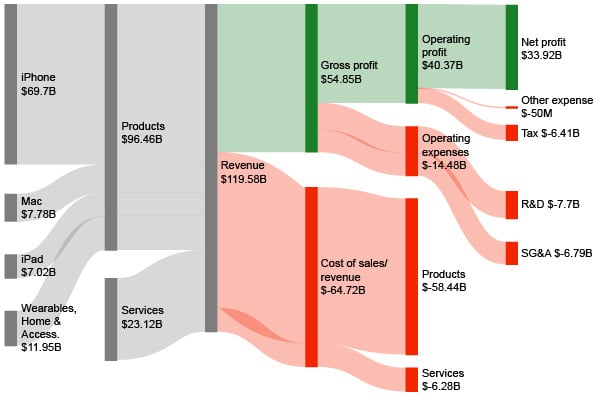
With ChartExpo , you can create a Sankey Chart with up to eight levels, offering multiple perspectives for analyzing your data. Even the most complicated data sets become manageable and easy to interpret.
You can customize your Sankey charts and every component including nodes, links, stats, text, colors, and more. ChartExpo is an add-in in Microsoft Excel, Google Sheets, and Power BI, you can create beautiful Sankey diagrams while keeping your data safe in your favorite tools.
Sankey diagrams can be used to visualize all types of data which contain a flow of information. It beautifully connects the flows and presents the data in an optimum way.
Here are a few use cases:
- Sankey diagrams are widely used to visualize energy production, consumption, and distribution. They help in tracking how energy flows from one source (like oil or gas) to various uses (heating, electricity, transportation).
- Businesses use Sankey diagrams to trace customer interactions across different channels and touchpoints. It highlights the flow of users through a funnel or process, revealing drop-off points and success paths.
- I n supply chain management, these diagrams show how resources, products, or information flow between suppliers, manufacturers, and retailers, identifying bottlenecks and inefficiencies.
Design Best Practices for Sankey Diagrams
When utilizing a Sankey diagram, it is essential to maintain simplicity while ensuring accuracy in proportions. Clear labeling and effective color usage are key factors to consider. Emphasizing the logical flow direction and highlighting significant flows will enhance the visualization.
How to Choose the Right Chart or Graph for Your Data
Channels like social media or blogs have multiple data sources, and managing these complex content assets can get overwhelming. What should you be tracking? What matters most?
How do you visualize and analyze the data so you can extract insights and actionable information?
1. Identify your goals for presenting the data.
Before creating any data-based graphics, I ask myself if I want to convince or clarify a point. Am I trying to visualize data that helped me solve a problem? Or am I trying to communicate a change that's happening?
A chart or graph can help compare different values, understand how different parts impact the whole, or analyze trends. Charts and graphs can also be useful for recognizing data that veers away from what you’re used to or help you see relationships between groups.
So, clarify your goals then use them to guide your chart selection.
2. Figure out what data you need to achieve your goal.
Different types of charts and graphs use different kinds of data. Graphs usually represent numerical data, while charts are visual representations of data that may or may not use numbers.
So, while all graphs are a type of chart, not all charts are graphs. If you don't already have the kind of data you need, you might need to spend some time putting your data together before building your chart.
3. Gather your data.
Most businesses collect numerical data regularly, but you may need to put in some extra time to collect the right data for your chart.
Besides quantitative data tools that measure traffic, revenue, and other user data, you might need some qualitative data.
These are some other ways you can gather data for your data visualization:
- Interviews
- Quizzes and surveys
- Customer reviews
- Reviewing customer documents and records
- Community boards
Fill out the form to get your templates.
4. select the right type of graph or chart..
Choosing the wrong visual aid or defaulting to the most common type of data visualization could confuse your viewer or lead to mistaken data interpretation.
But a chart is only useful to you and your business if it communicates your point clearly and effectively.
Ask yourself the questions below to help find the right chart or graph type.
Download the Excel templates mentioned in the video here.
5 Questions to Ask When Deciding Which Type of Chart to Use
1. do you want to compare values.
Charts and graphs are perfect for comparing one or many value sets, and they can easily show the low and high values in the data sets. To create a comparison chart, use these types of graphs:
- Scatter plot
2. Do you want to show the composition of something?
Use this type of chart to show how individual parts make up the whole of something, like the device type used for mobile visitors to your website or total sales broken down by sales rep.
To show composition, use these charts:
- Stacked bar
3. Do you want to understand the distribution of your data?
Distribution charts help you to understand outliers, the normal tendency, and the range of information in your values.
Use these charts to show distribution:
4. Are you interested in analyzing trends in your data set?
If you want more information about how a data set performed during a specific time, there are specific chart types that do extremely well.
You should choose one of the following:
- Dual-axis line
5. Do you want to better understand the relationship between value sets?
Relationship charts can show how one variable relates to one or many different variables. You could use this to show how something positively affects, has no effect, or negatively affects another variable.
When trying to establish the relationship between things, use these charts:
Featured Resource: The Marketer's Guide to Data Visualization
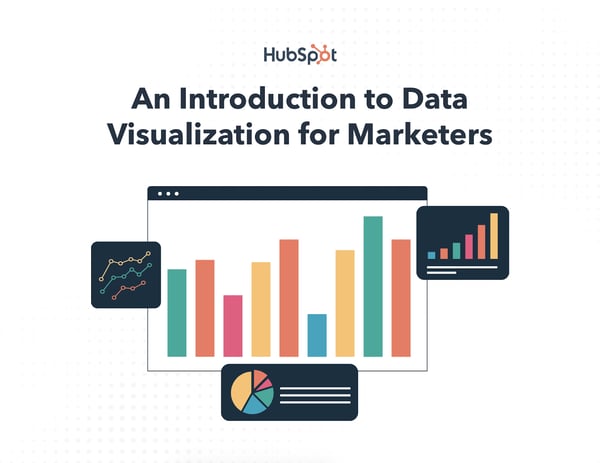
Don't forget to share this post!
Related articles.
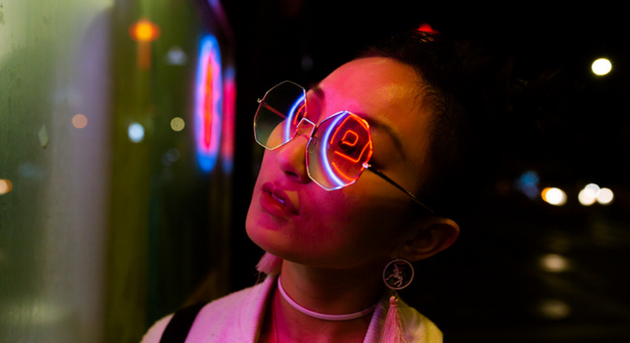
9 Great Ways to Use Data in Content Creation
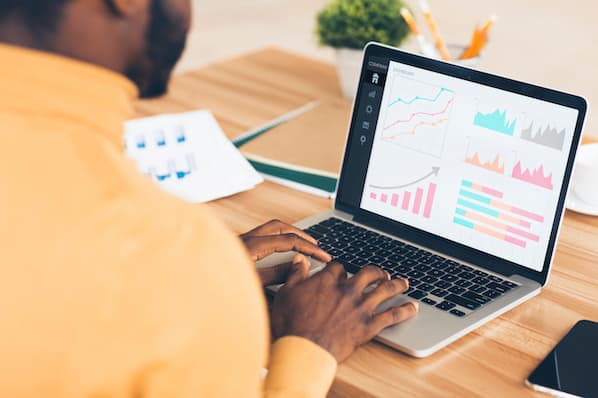
Data Visualization: Tips and Examples to Inspire You
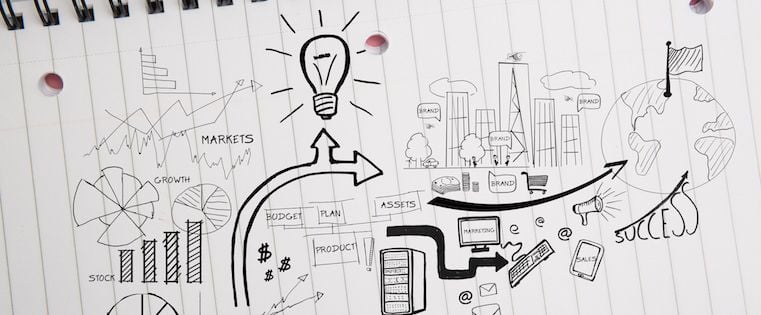
17 Data Visualization Resources You Should Bookmark
![visual representation of data using graphics An Introduction to Data Visualization: How to Create Compelling Charts & Graphs [Ebook]](https://53.fs1.hubspotusercontent-na1.net/hubfs/53/data-visualization-guide.jpg)
An Introduction to Data Visualization: How to Create Compelling Charts & Graphs [Ebook]
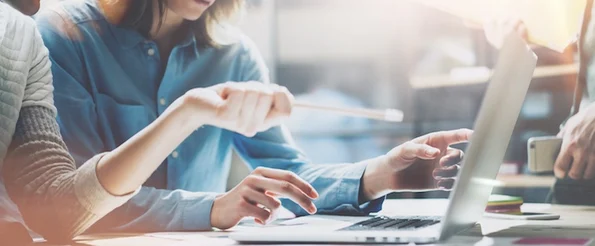
Why Data Is The Real MVP: 7 Examples of Data-Driven Storytelling by Leading Brands
![visual representation of data using graphics How to Create an Infographic Using Poll & Survey Data [Infographic]](https://53.fs1.hubspotusercontent-na1.net/hubfs/53/00-Blog_Thinkstock_Images/Survey_Data_Infographic.jpg)
How to Create an Infographic Using Poll & Survey Data [Infographic]
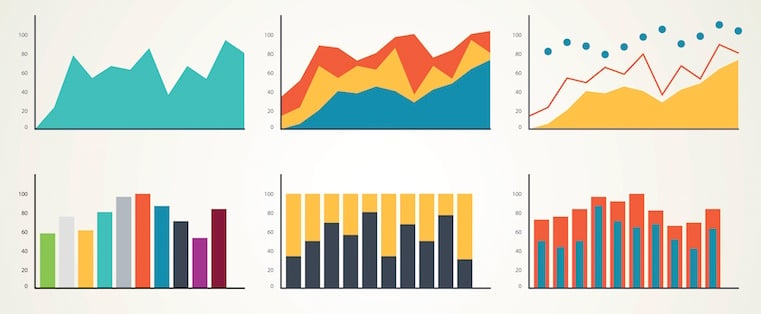
Data Storytelling 101: Helpful Tools for Gathering Ideas, Designing Content & More
Tired of struggling with spreadsheets? These free Microsoft Excel Graph Generator Templates can help
Marketing software that helps you drive revenue, save time and resources, and measure and optimize your investments — all on one easy-to-use platform

- SUGGESTED TOPICS
- The Magazine
- Newsletters
- Managing Yourself
- Managing Teams
- Work-life Balance
- The Big Idea
- Data & Visuals
- Case Selections
- HBR Learning
- Topic Feeds
- Account Settings
- Email Preferences
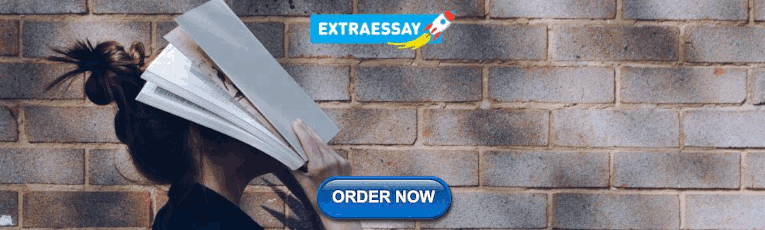
Visualizations That Really Work
- Scott Berinato

Not long ago, the ability to create smart data visualizations (or dataviz) was a nice-to-have skill for design- and data-minded managers. But now it’s a must-have skill for all managers, because it’s often the only way to make sense of the work they do. Decision making increasingly relies on data, which arrives with such overwhelming velocity, and in such volume, that some level of abstraction is crucial. Thanks to the internet and a growing number of affordable tools, visualization is accessible for everyone—but that convenience can lead to charts that are merely adequate or even ineffective.
By answering just two questions, Berinato writes, you can set yourself up to succeed: Is the information conceptual or data-driven? and Am I declaring something or exploring something? He leads readers through a simple process of identifying which of the four types of visualization they might use to achieve their goals most effectively: idea illustration, idea generation, visual discovery, or everyday dataviz.
This article is adapted from the author’s just-published book, Good Charts: The HBR Guide to Making Smarter, More Persuasive Data Visualizations.
Know what message you’re trying to communicate before you get down in the weeds.
Idea in Brief
Knowledge workers need greater visual literacy than they used to, because so much data—and so many ideas—are now presented graphically. But few of us have been taught data-visualization skills.
Tools Are Fine…
Inexpensive tools allow anyone to perform simple tasks such as importing spreadsheet data into a bar chart. But that means it’s easy to create terrible charts. Visualization can be so much more: It’s an agile, powerful way to explore ideas and communicate information.
…But Strategy Is Key
Don’t jump straight to execution. Instead, first think about what you’re representing—ideas or data? Then consider your purpose: Do you want to inform, persuade, or explore? The answers will suggest what tools and resources you need.
Not long ago, the ability to create smart data visualizations, or dataviz, was a nice-to-have skill. For the most part, it benefited design- and data-minded managers who made a deliberate decision to invest in acquiring it. That’s changed. Now visual communication is a must-have skill for all managers, because more and more often, it’s the only way to make sense of the work they do.
- Scott Berinato is a senior editor at Harvard Business Review and the author of Good Charts Workbook: Tips Tools, and Exercises for Making Better Data Visualizations and Good Charts: The HBR Guide to Making Smarter, More Persuasive Data Visualizations .

Partner Center
- Data Visualizations
- Most Recent
- Presentations
- Infographics
- Forms and Surveys
- Video & Animation
- Case Studies
- Design for Business
- Digital Marketing
- Design Inspiration
- Visual Thinking
- Product Updates
- Visme Webinars
- Artificial Intelligence
The 30 Best Data Visualizations of 2024 [Examples]
![visual representation of data using graphics The 30 Best Data Visualizations of 2024 [Examples]](https://visme.co/blog/wp-content/uploads/2021/08/Data-Visualization-Header.jpg)
Written by: Anna Glivinska
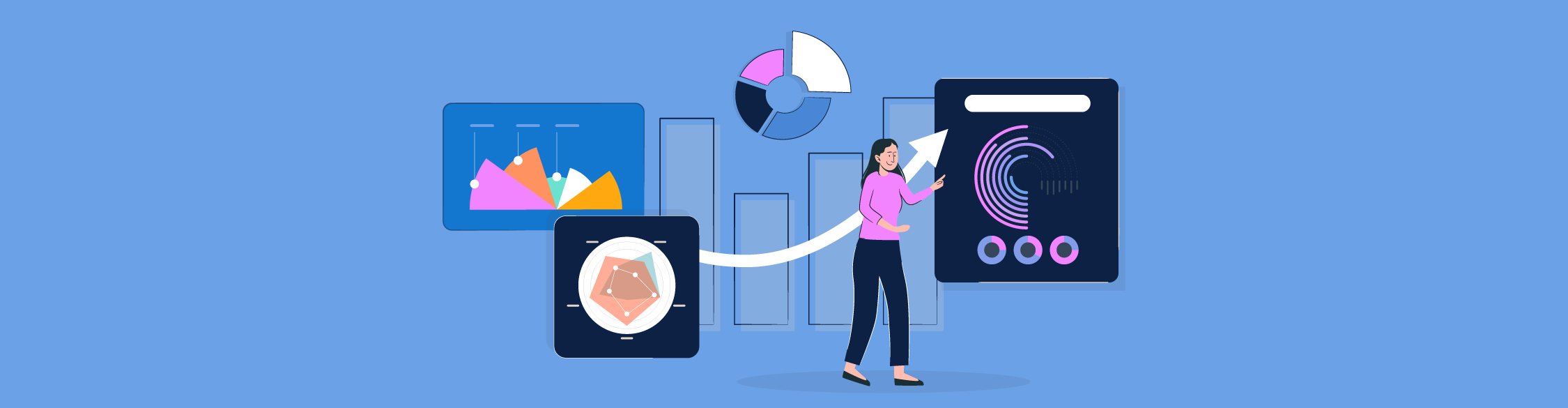
Data is beautiful; it can inspire, improve lives and bring out the best in people. To keep you inspired, we’ve gathered the best data visualizations of 2024.
The chosen works cover a variety of topics from NASA asteroids in space to environmental issue statistics and futuristic LIDAR data graphs.
With over 5.35 billion people using the Internet in 2024, we’re sure to witness even more amazing data visualizations every year. For now, get ready to dive into 2024’s best data visualization examples.
This list includes award winning designs so you can get inspired by the best of the best!
- NASA's Eyes on Asteroids is a good data visualization example that provides a great user experience. The design is simple and intuitive, making it easy for users to navigate the site and find what they're looking for.
- The History of Pandemics is an infographic that presents a visual timeline of every known pandemic and includes information on how many people were affected, where it spread and what caused it.
- Void of the Memories is the rarest data visualization on this list. It's a great combination of calligraphy and data visualization that tells the story of human memory and experience.
- The search for dark matter is one of the most important scientific questions in physics today, and this infographic, “The Search for Dark Matter,” serves as a great introduction to the subject.
- Enhance your data storytelling skills and creatively showcase your data by signing up for Visme's data visualization tools .
1. Nasa’s Eyes on Asteroids
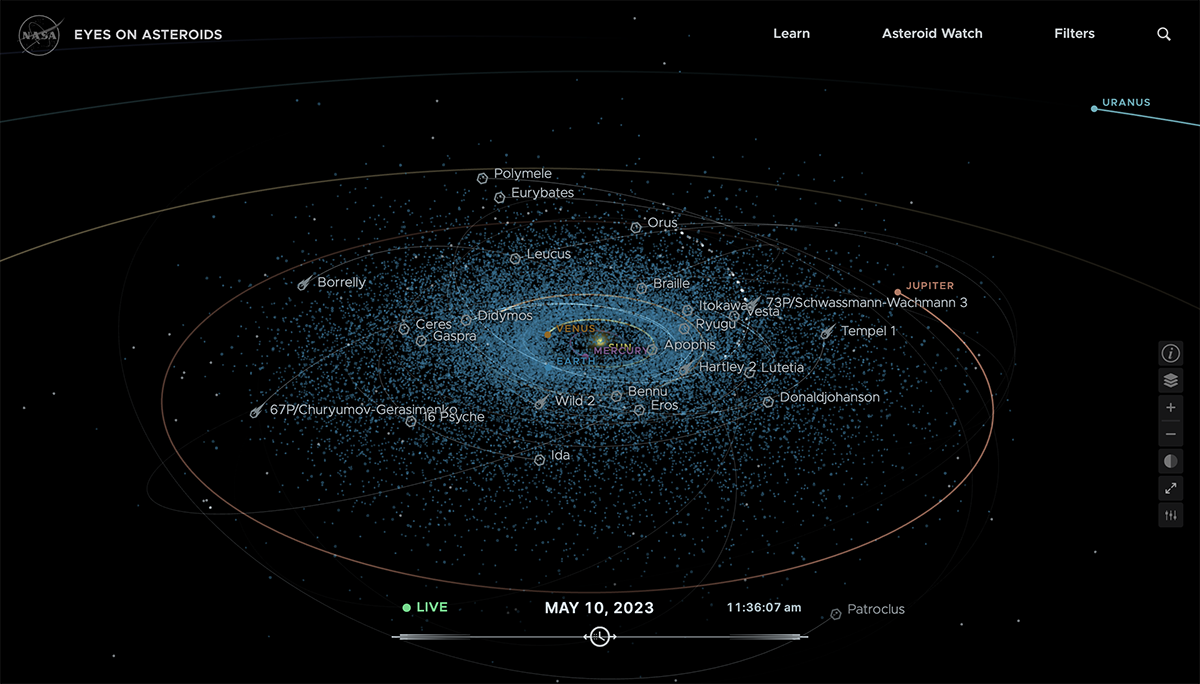
Image Source
If you are interested in exploring data visualization topics in space exploration, check out this striking data visualization created by NASA.
NASA's Eyes on Asteroids is one of the best data visualizations due to its exceptional design and functionality. This interactive visualization allows users to explore the asteroid belt and see the real-time positions of asteroids in our solar system.
The design of this visualization is highly engaging and visually stunning, with a sleek and modern interface that is easy to use. The visualization features a 3D solar system model, allowing users to zoom in and out to explore asteroids and other celestial bodies.
One of the key features of NASA's Eyes on Asteroids visualization is its real-time data feed, which provides up-to-date information on the positions and trajectories of asteroids. This feature makes the visualization highly informative and relevant to current events, allowing users to track potentially hazardous asteroids and see their projected paths over time.
Design your own space exploration infographic using Visme. Allowing you to create data visualizations easier and faster.
Get inspired by one of our loyal Visme users, MacKenzie Stonis , Economic Research Analyst at Greater Memphis Chamber, who said:
"I have enough complications in life; I don’t need my report-building tool to add any fuel to the fire,” she laughs. “I personally had experience with similar applications before Visme and found their tools weren’t as user-friendly as Visme, and their tools didn’t handle data very well. They didn’t provide the solution I really wanted."
2. Launch It
By Shane Mielke
When graphic designer Shane Mielke published his book, Launch It, he also designed an amazing online data visualization depicting where his book could be purchased. This interactive data viz is a spinnable world map showing live data of where the Launch It book can be acquired. To make it more interesting, the user can change the colors of the map and shapes of the markers.
3. Hello Sun app
The Hello Sun app created by Small Multiples, is a live data viz for your phone. The main dashboard visualizes the movement of the sun and moon in the sky in numerous cities in the world.
The movement is depicted in circular sky maps that change color as the sun rises and sets. Each city is illustrated by a major landmark which is only seen when the sun creates a shadow of it.
The app also lets the user know how sun behaves anywhere in the world through GPS coordinates. That way they can plan out a veggie garden or know if the apartment they want to rent will have sun in the afternoon.
4. The World’s Population at 8 Billion
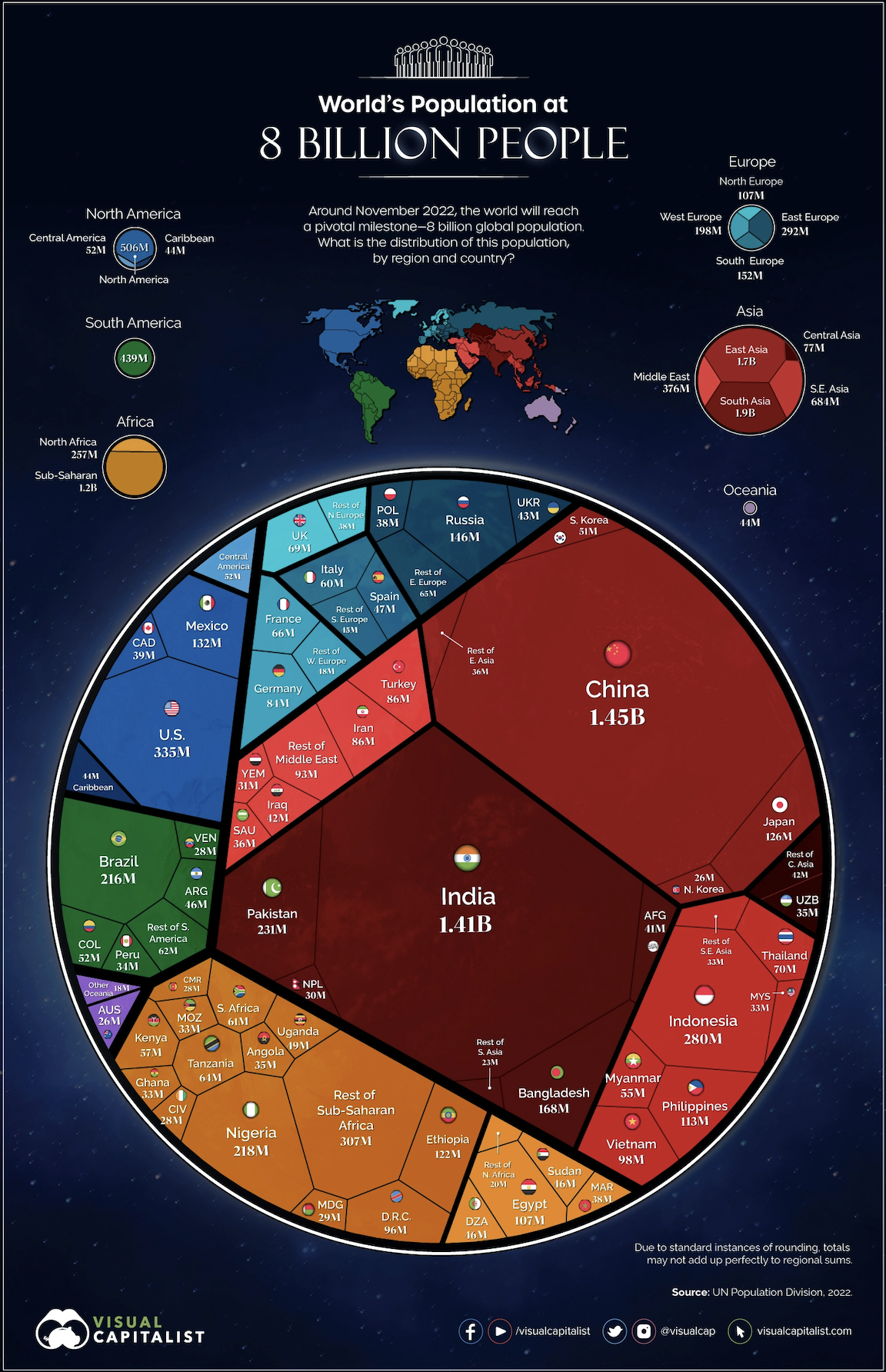
On November 15, 2022, the world’s population reached 8 billion. This is the first time in history that there have been this many people on Earth. And there can't be a more straightforward and visually appealing way to present this data than this visualization.
What makes this big data visualization stand out is its simplicity and effectiveness in conveying the message. Using a circle to represent the earth is a powerful symbol that makes the visualization easy to understand and remember.
By using colors to represent continents and lines to separate countries, the visualization effectively conveys the complexity of the world's population in a simple and visually appealing way.
5. The Top 10 Largest Nuclear Explosions
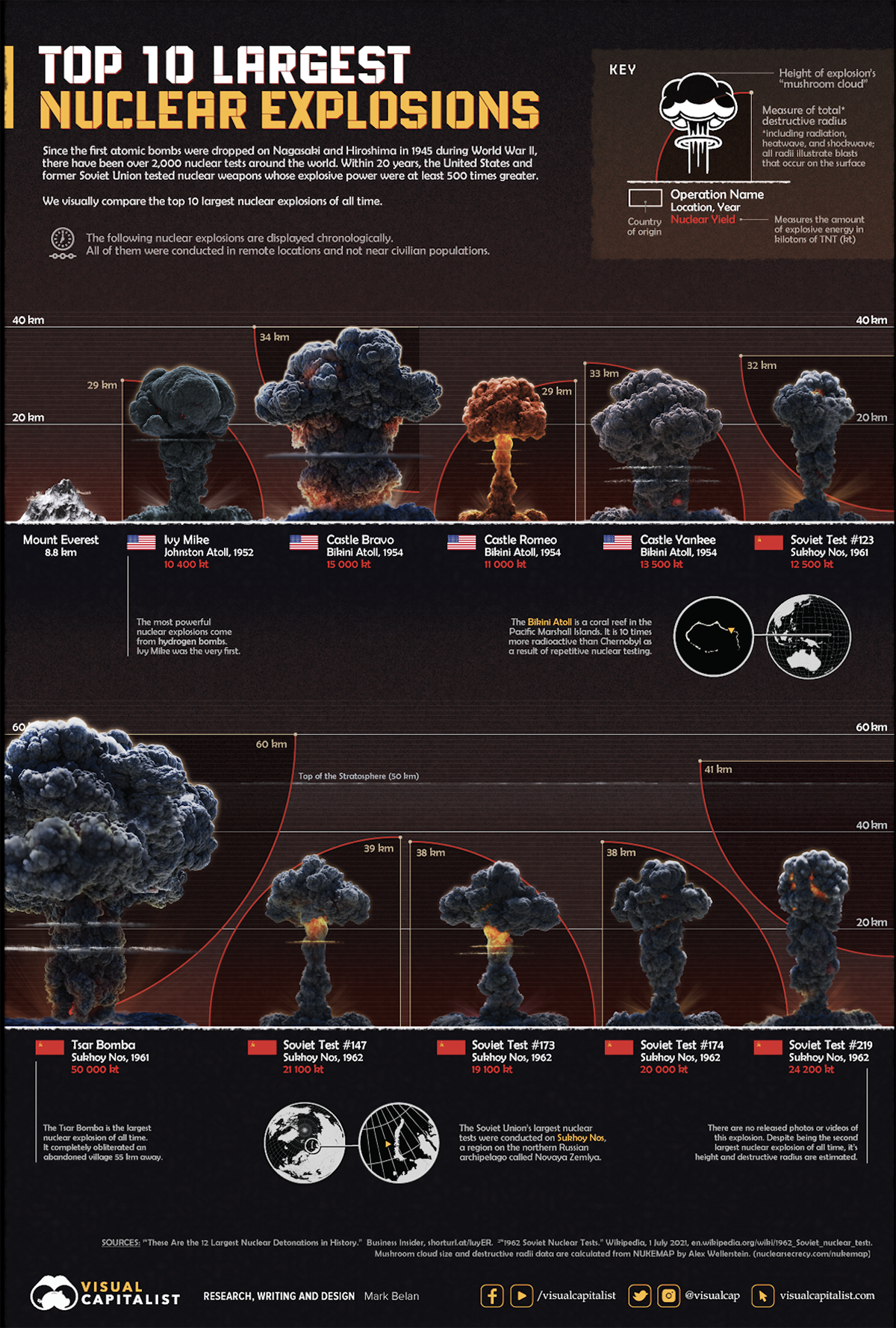
This is a prime example of how creative design can bring data to life. Beyond the interesting data visualization, it uses a unique approach, similar to an infographic, to showcase the impact and size of the largest nuclear explosions ever detonated.
It features a series of explosion image examples that help visualize each explosion's scale and impact. The use of images effectively conveys the destructive power of each blast in a way that is easy to understand and remember.
The data is presented clearly and concisely, with each explosion listed along with its country of origin.
6. Visualizing the History of Pandemics
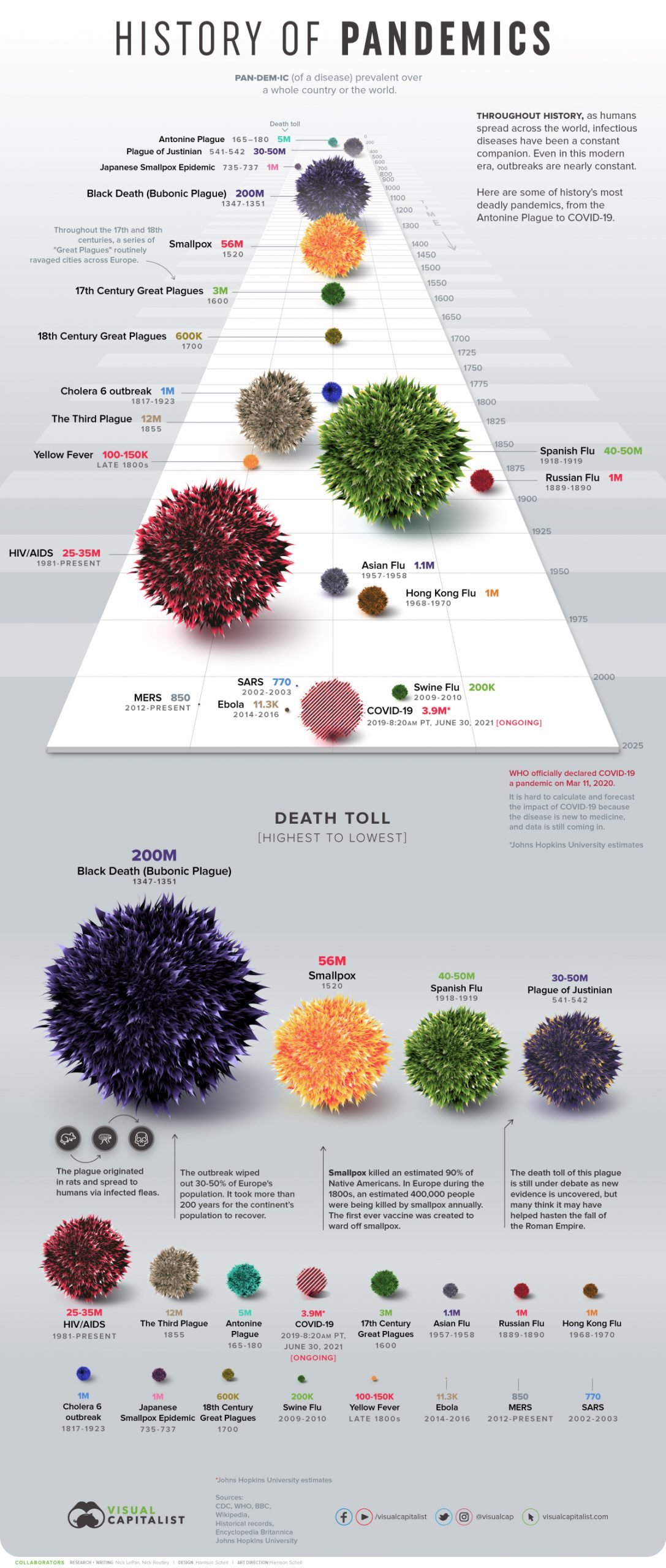
This is an informative graphic named Visualizing the History of Pandemics by Nicholas LePan. It tells the story of all the known pandemics in the history of mankind, including the name of the disease, death toll and the approximate date the pandemic occurred.
While the exact number of victims of every disease is still under question, we can still learn from this graphic that super-spreading infections happened across all history of mankind. Statistical data of this infographic shows some diseases scaling with the growth of the population.
Striking 3D illustrations of diseases are combined with the research data from CDC, WHO, BBC, Wikipedia, Historical records, Encyclopedia Britannica and John Hopkins University. The illustrations scale according to the recorded death toll to allow scanning and recognizing data easily.
7. It Fell From the Sky
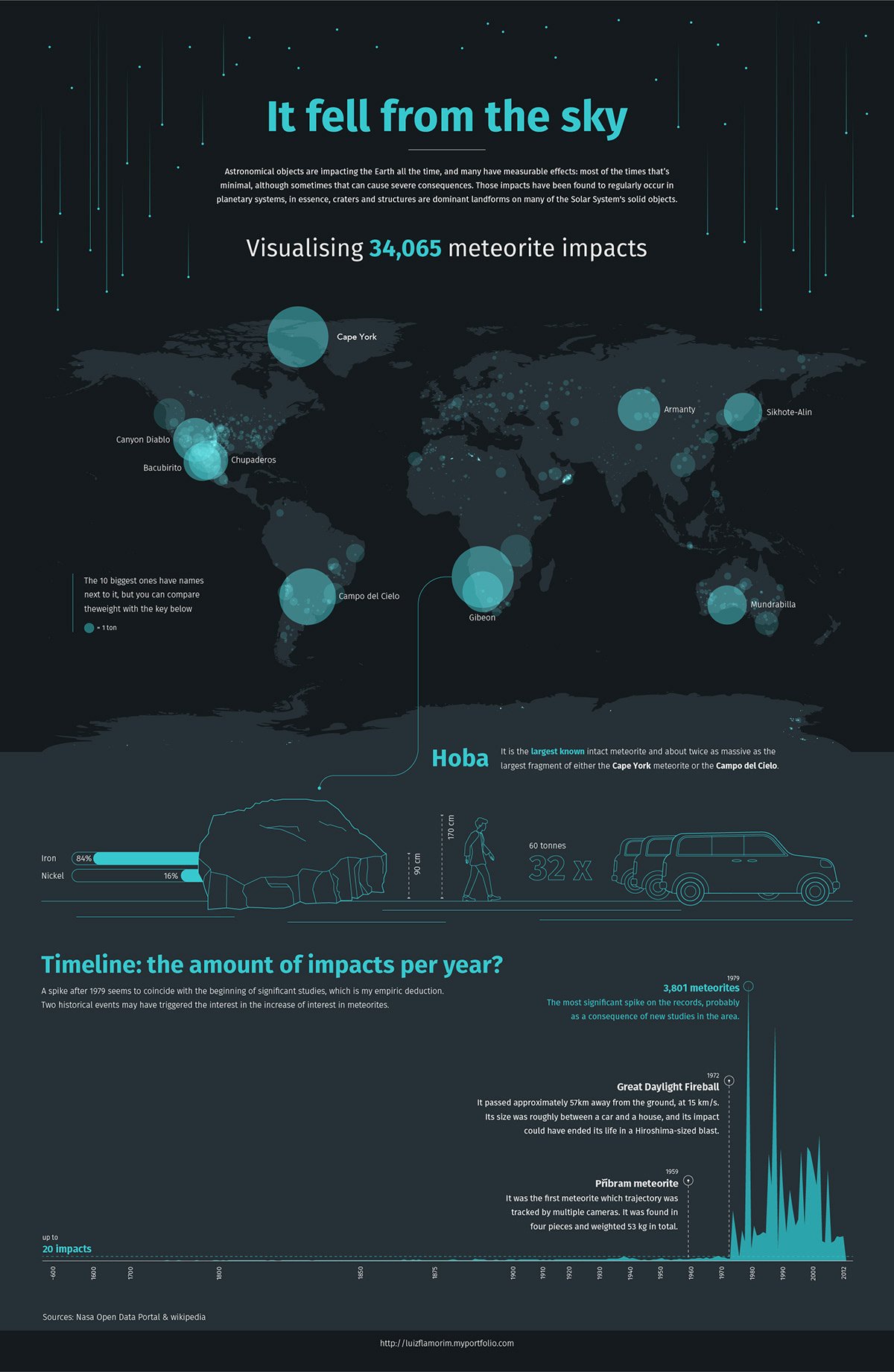
Created by a UK-based designer, this infographic highlights beautiful data visualization of 34,000 meteorites that have fallen on the Earth. You will discover the map and timeline of the impacts per year, wrapped up in clean, stylish graphics. The visualization also shows spikes on the records and comparing the size of the biggest meteorites recorded.
Meteorites hit almost all of Earth’s surface, but some areas seem untouched; this phenomenon could be connected with Earth’s magnetic fields. And who knows – the future may bring us even more meteorites to explore!
If you’re a fan of space and astronomy, you can learn more about meteorites from NASA website or check out this database of the Meteoritical Society.
Try Visme, our all-in-one design for creating stunning visualizations on meteorites in space or other research topics you’re working on.
Get the most out of Visme’s seamless integration with Google Sheets to create visualizations of live, easy-to-update data.
Link to your Google Sheets account or import through a link. Select the page and data range and connect them to your Visme chart. When the data changes in the Google Sheet, it automatically applies to the live project. Simply press the refresh button.
Sign up to Visme for free.
8. Mars Mission 2024 Promo Reel
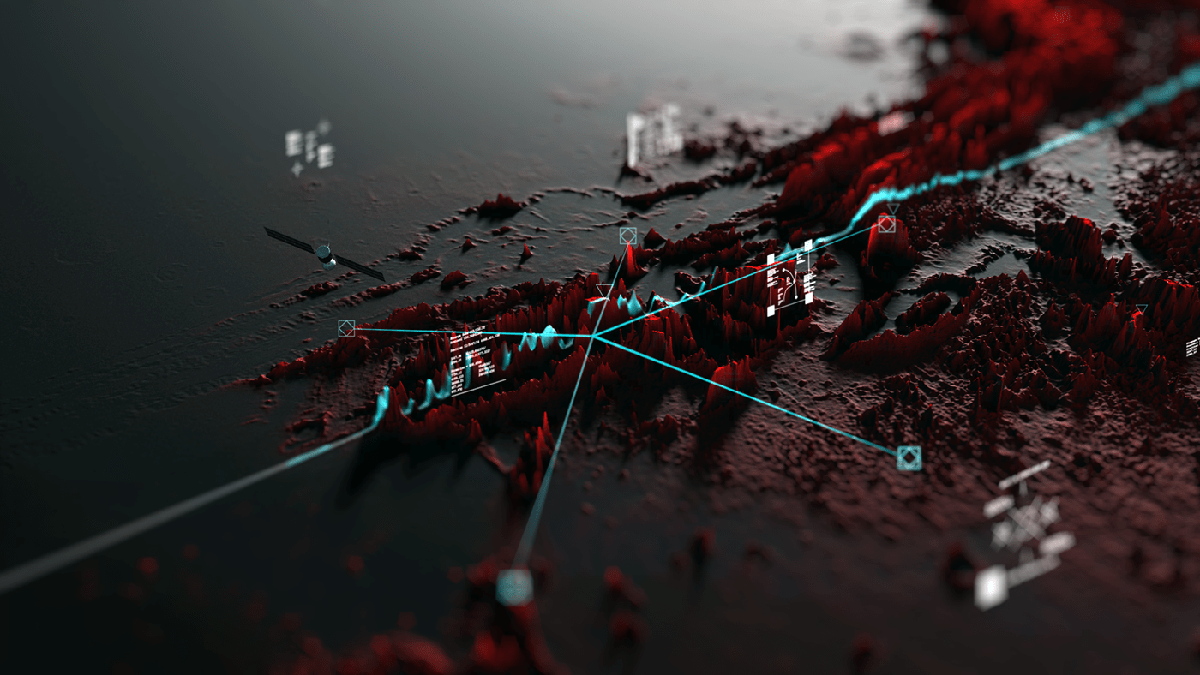
Vivid, rich in details. This 3D graphic uses beautiful data visualizations to share the vision of the future. Space missions and sending people into space are shown in an eye-catching red-grey palette.
The complicated animation of terrain exploration, space module flight and surface graphics are breathtaking. For a moment, you feel like a Mars mission crew member with your eyes on the stars.
9. Codex Atlanticus
By The Visual Agency
The first data viz in our collection is the Codex Atlanticus. The Visual Agency, a data visualization group from Italy, has put together a beautiful digital library of Leonardo Da Vinci’s journals and notebooks. It’s the largest digital collection of his work to date.
But that’s not all—it’s all set up in a way that will amaze both data visualization fans and artists.
The Codex Atlanticus won the Gold Kantar Information is Beautiful Award in the Art and Entertainment category. For history and Leonardo Da Vinci buffs, this digital library is a rich resource for education and research.
For data visualization fans , this library a wonderful example of how history can be digitized in a beautiful way, and it’s no surprise why it's a part of our best data visualizations list.
10. Plastic Waste Pollution
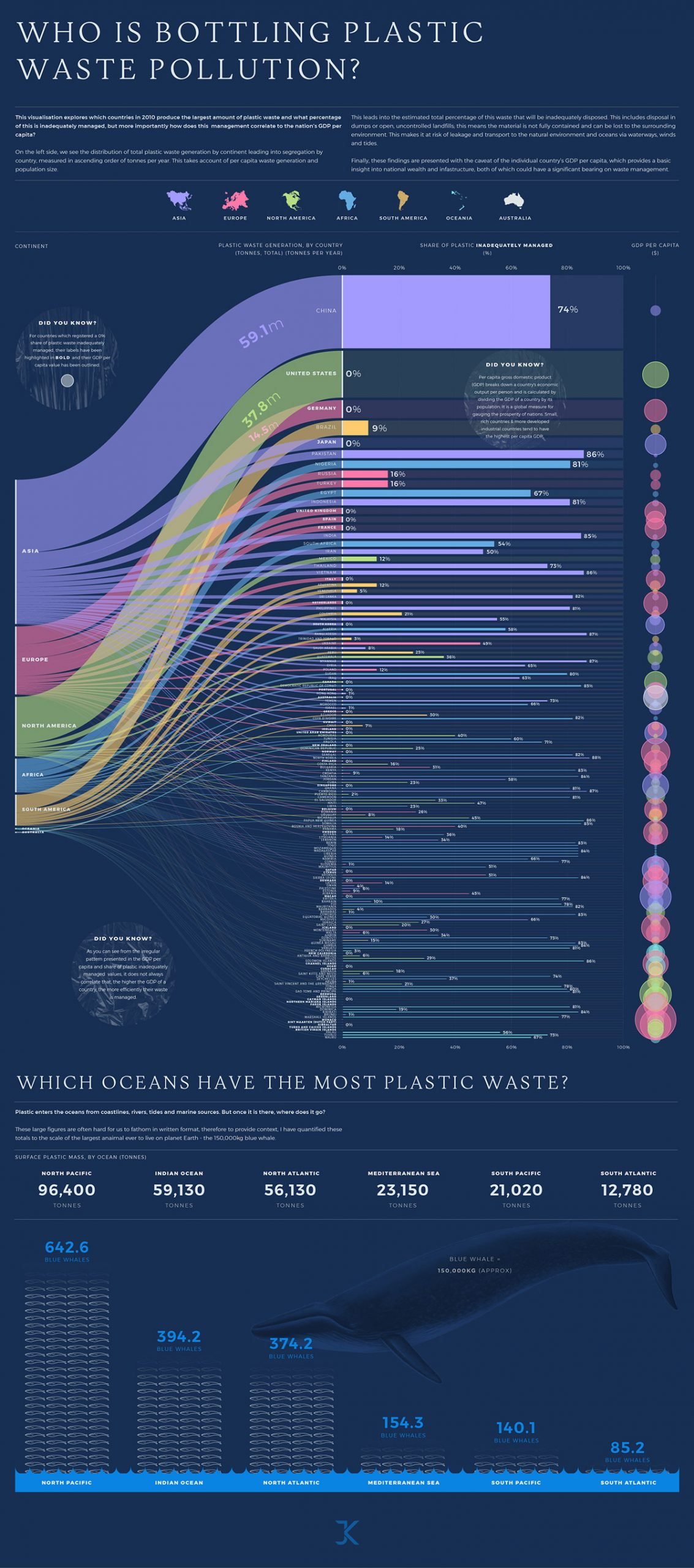
Based on data on the distribution of total plastic waste generation by continent, Jamie Kettle created this personal project to estimate the percentage of plastic waste that was inadequately disposed of.
The infographic provides a clear and precise picture of current surface plastic mass by ocean, measuring it in a creative way. We can see plastic waste management for every country in a colored bar chart. The names of the countries that report 100% of all their plastic waste handled properly are highlighted in bold.
One of the major findings here is that the country's GDP and efficient plastic waste management aren’t always correlated—you can see this by the irregular patterns shown in the infographic.
If you are curious about plastic waste, here are some resources for you: a guide on plastic waste, detailed info on plastic waste pollution from the UN Environment Program and Impacts of Mismanaged Trash by the United States Environmental Protection Agency.
If you’re working on a research topic like waste management, use Visme’s charts and graphs templates to highlight your findings and statistical analysis. Incorporate vertical bar graphs and align the values to the left, right or center to match your overall design.
11. Fossil Fuels
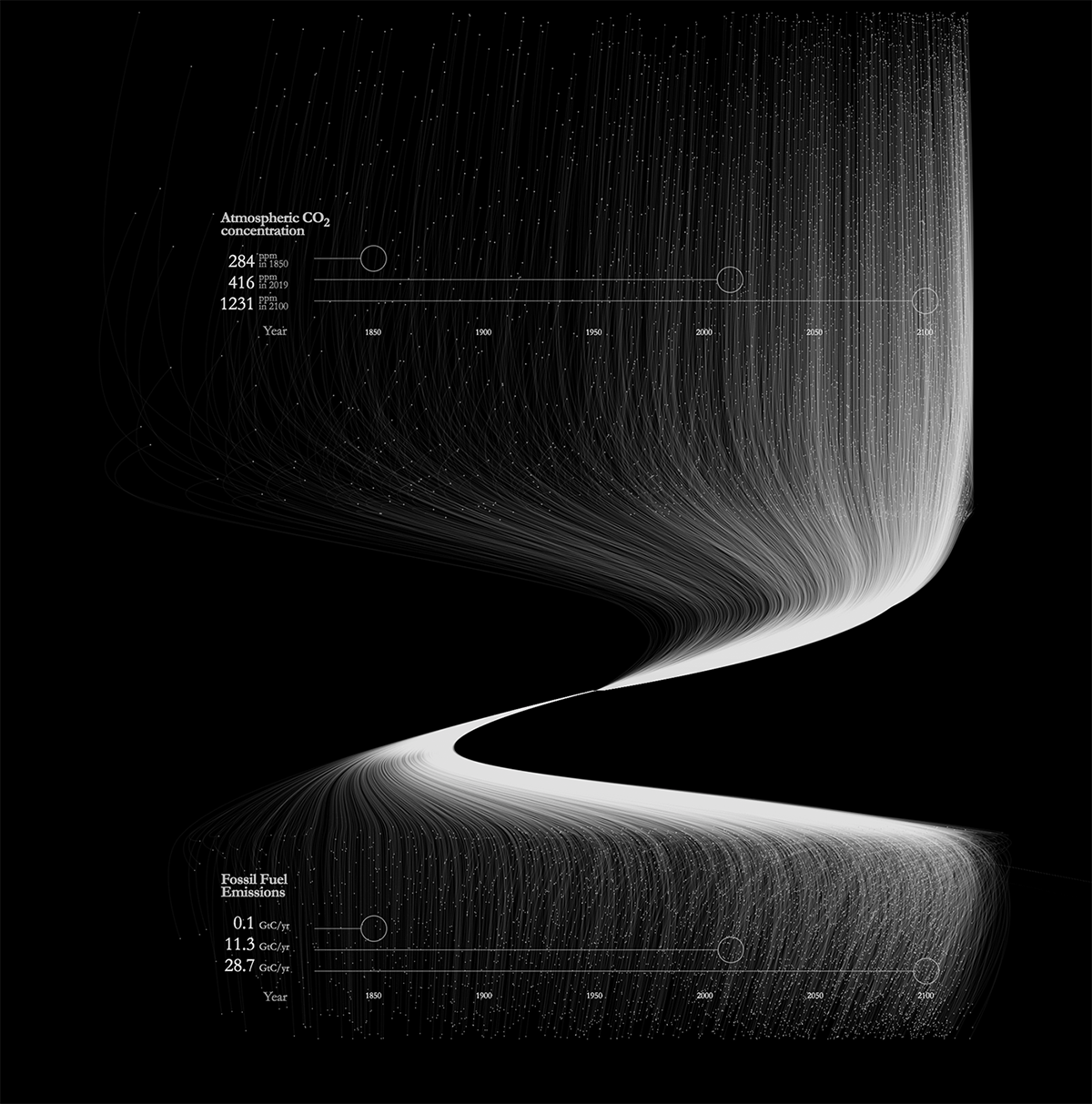
This profound and complex visualization tells us about one of the most pressing environmental issues – the increasing amount of carbon dioxide in the Earth's atmosphere.
While CO 2 buildup is responsible for climate change, the trend is projected to continue, and the infographic provides insight into when this could happen. It’s easy to notice a steady increase in fossil fuel emissions since the Industrial Revolution and the projected sharp rise in the concentration of carbon dioxide until 2100.
Find more data on CO 2 emissions in the Our World in Data research, EPA website and Worldometer stats.
12. IV State of Audio & Voice
:new: Image Source
Prodigious Volcano is a data visualization agency based in Madrid that conducts research focused on human engagement and culture's impact through media.
The IV State of Audio and Voice report is focused on how internet users residing in Spain engage with different types of audio expressions such as podcast, audiobooks, voice assistants and smart speakers. Their methodology involves dividing their analysis into two dimensions, audio as a product and audio as a tool.
The report comes heavily designed with interactive data visualization charts. Each visualization displays the data as a whole or segmented based on the audio method selected. This provides a better user experience for the reader as they can filter information and turns the data into easily digestible content.
This data visualization report has racked up multiple awards including a Webby Award for People's Voice Winner for best data visualization in 2024.
13. Water Consumption
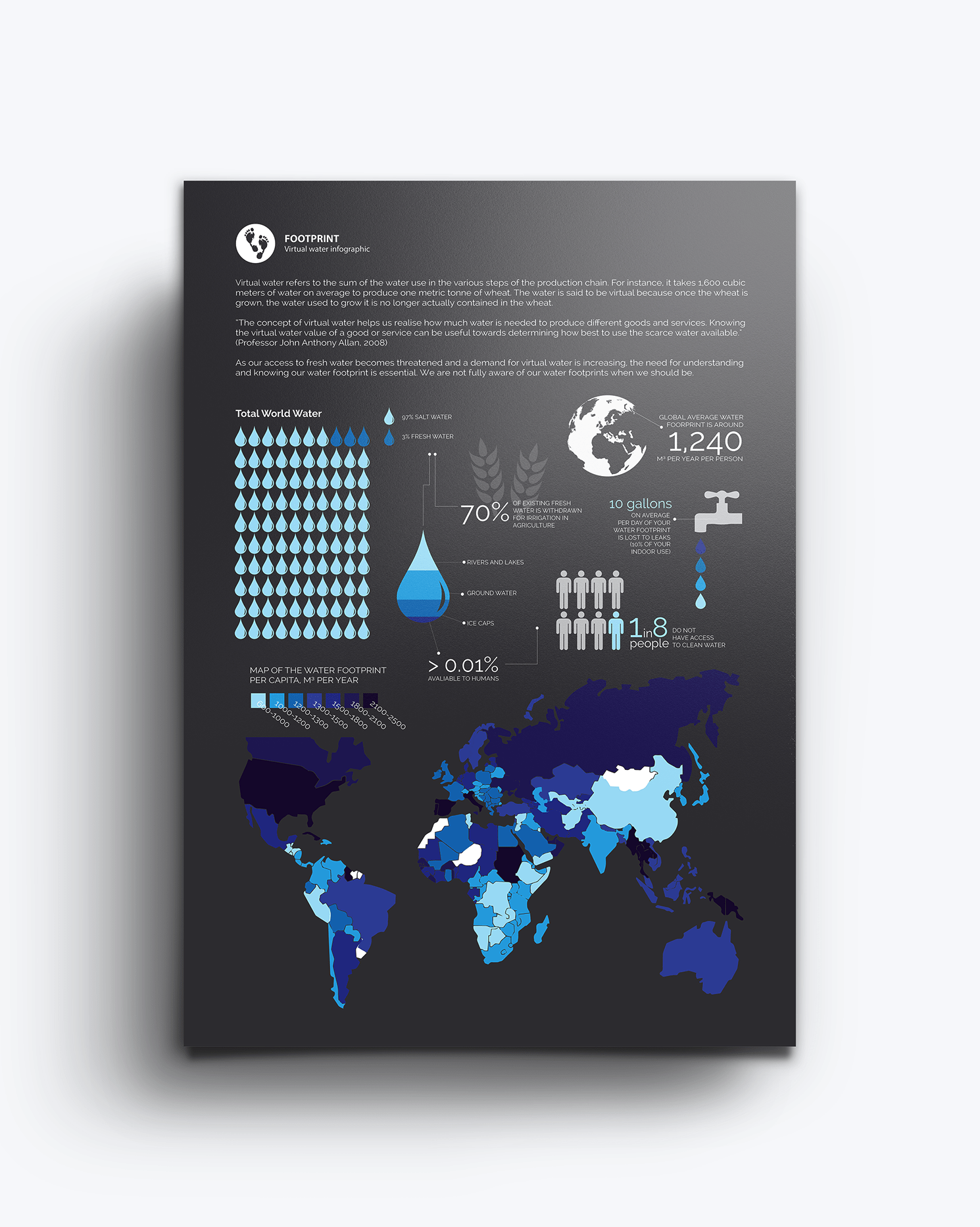
Hidden food production costs involve a great amount of freshwater. This stunning example of visualization created by Chesca Kirkland unfolds a story of water consumption required to produce certain kinds of food.
From chocolate to cheese, coffee and beer, every product requires a certain amount of freshwater to grow or be produced. The second part of the infographic is centered on the water resources available, including the map of the water footprint per capita per year and general availability of clean water to people.
Nominated for two C-Change Environmental and Sustainability Awards, the project won First Class Honours in Final Design Futures. Raising awareness about water sustainability is vital as we move forward to a more intelligent, AI-driven future.
We at Visme are inviting you to take up the challenge and create informative infographics that can invite change to various industry branches. Use our amazing free infographic library to create graphics for your personal projects as well as corporate or brand presentations.
For more detailed info on the infographic creation, watch this video on the 13 major types of infographics .

14. Void of the Memories
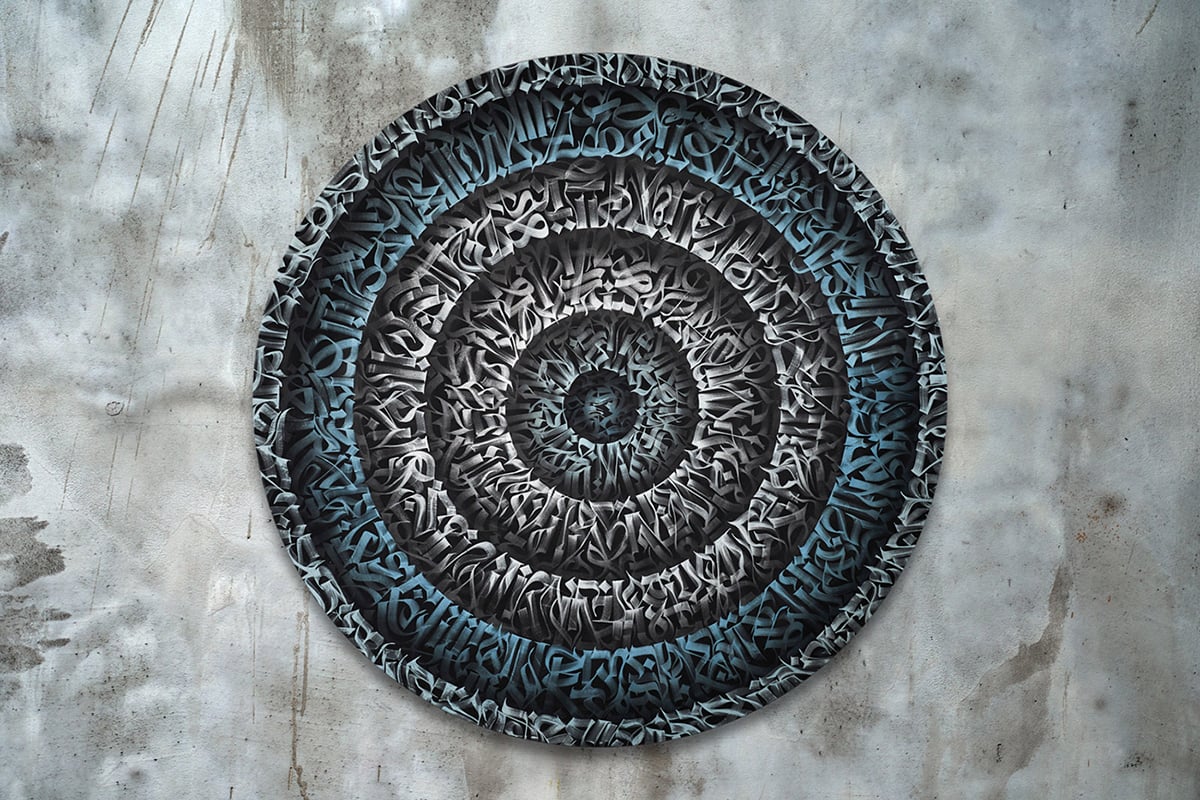
These mesmerizing circles were brought to you by one of the best-in-class street art and calligraphy authors, Pokras Lampas. Whether you would like to decipher this canvas or refer to it as a pure visual object, the unique gothic Calligrafuturism style is an eye magnet for anyone.
The project is focused on the human consciousness and the theme of dreams in the context of human memory and experience. According to the author, the future is for global unity and harmony of cultures – and it’s visible in the fusion of styles, techniques and systems used in the project graphics.
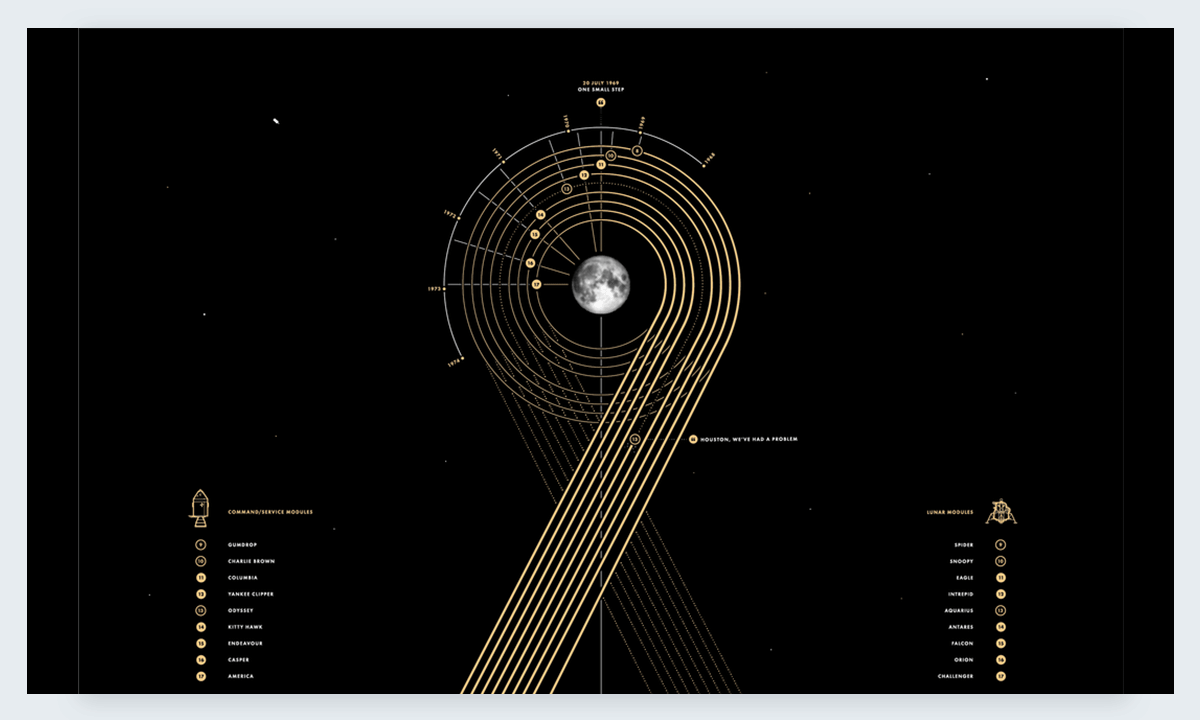
By Paul Button
Paul Button is a graphic designer who loves analyzing data and making it beautiful beyond belief. He has always been interested in the Apollo missions and all things space related. Using collected data about every single Apollo mission, he created this data visualization. He sells it as an art print and is quite successful. The Apollo data viz poster is a real gem, not only for space exploration fanatics but anyone who loves seeing beautiful data on a regular basis.
16. Active Satellites in Space
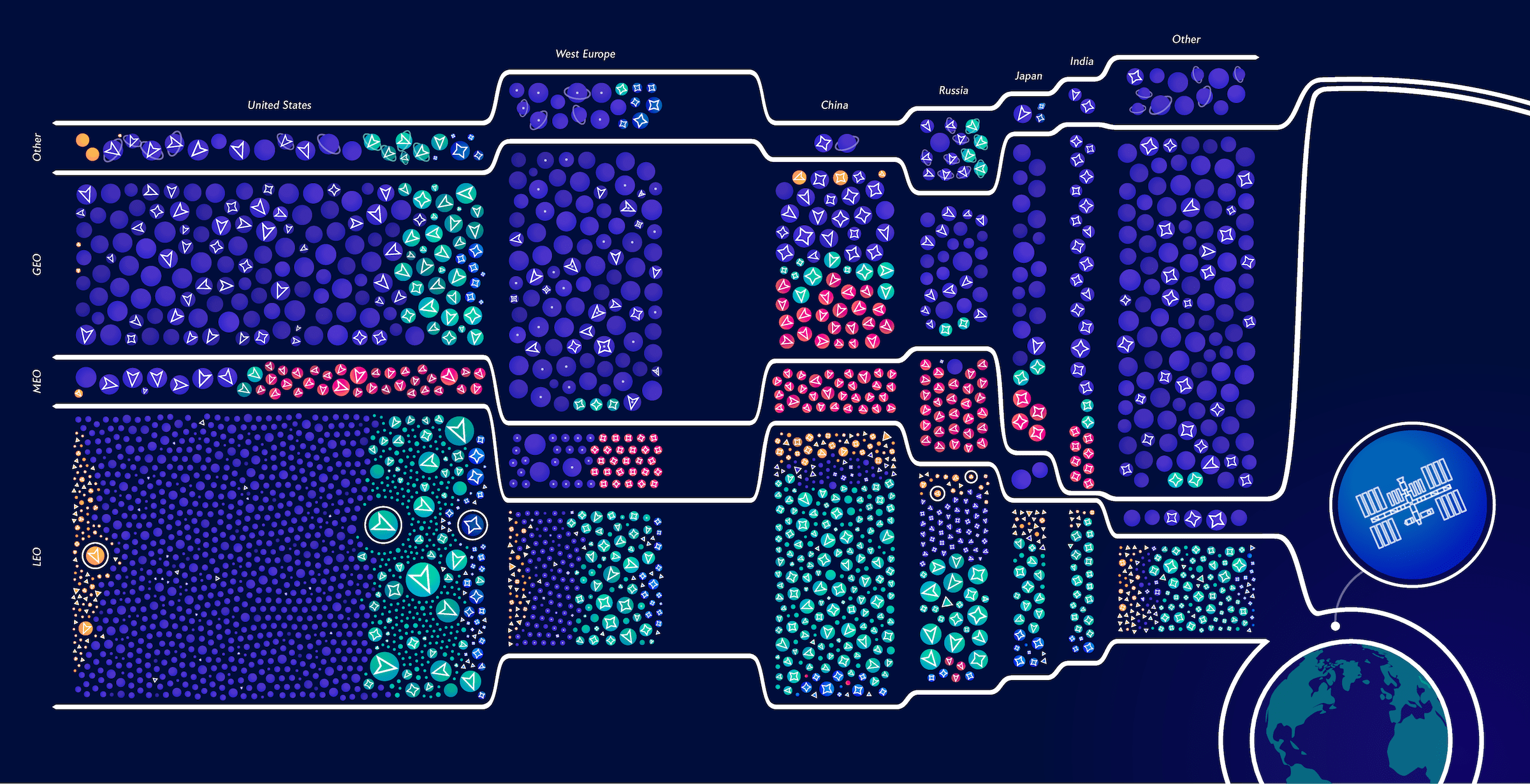
Created for Scientific American, this colorful and bright data visualization displays satellites in an original way. Neat and stylish satellite cluster grids sort them by country, orbit and class – business/commercial, civil, amateur/academic or defense.
The graphic details the mass of the satellites (100 kgs - 5,000 kgs), category (Test and Training, Communications, Images, Surveillance and Meteorology, Navigation and Research) and the launch date, from Nov 1974 till Aug 2020.
According to the graphic, six countries of the world control the largest amount of the satellites in orbit, and the US owns the largest share so far.
17. Covid Vaccination Tracker
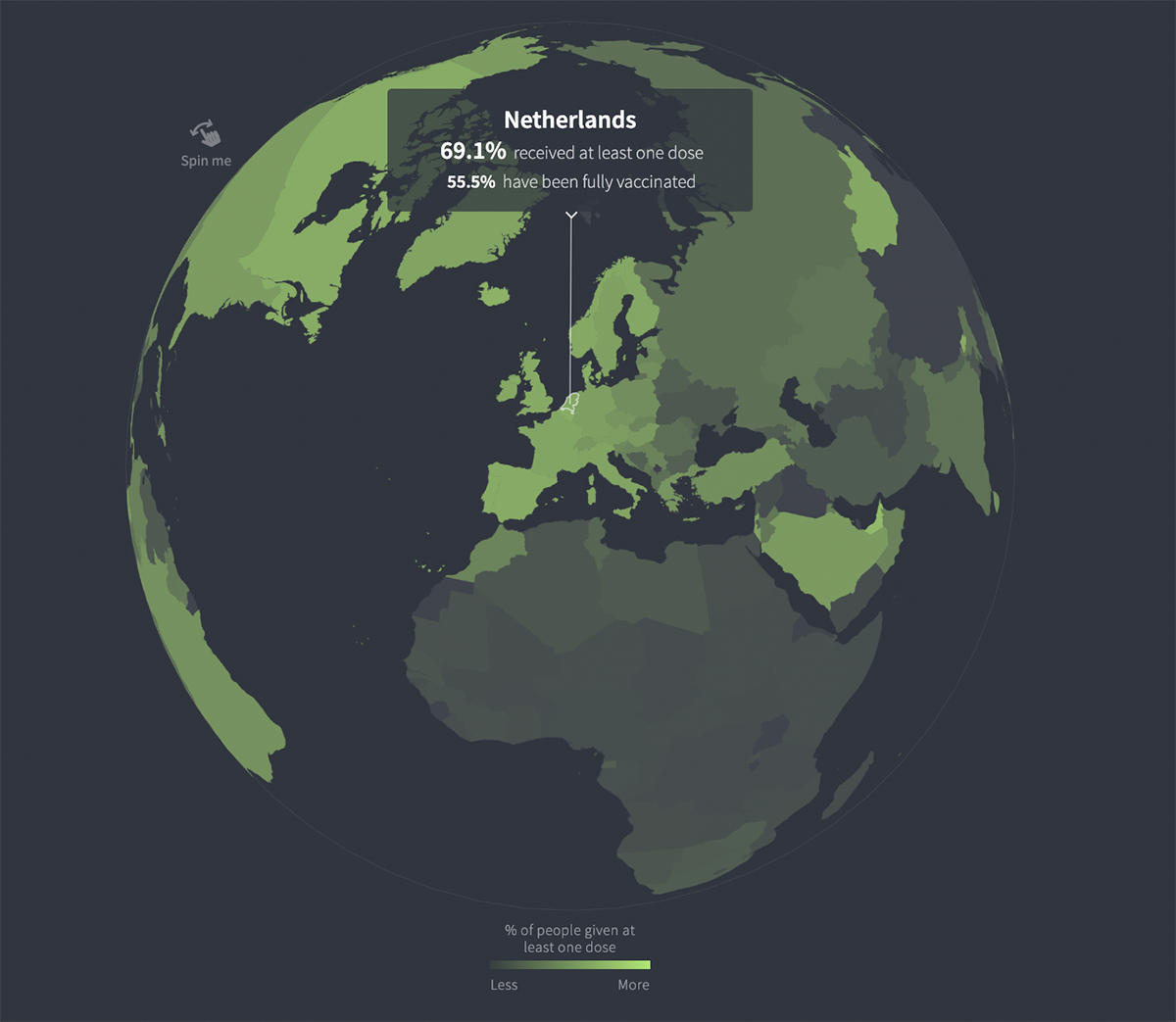
Updated until July 15, 2022, this animated Covid vaccination tracker shows the percentage of people in the world given at least one dose. The infographic and data illustration displays data on the vaccination rollout plan in over 80 countries and 50 US states.
Data presented in this data visualization is sourced from the Our World in Data project at the University of Oxford. Uncluttered, simple graphs show the 7-day Covid vaccination rolling average as well. The interactive charts allow you to sort the percent of population given at least one dose by country or income.
At the bottom of the page we can see the detailed, in-depth Covid-19 vaccination statistics, with type of vaccines offered (Pfizer-BioNTech, Moderna, Sinopharm, CanSino, Oxford-AstraZeneca, Johnson & Johnson, Covishield, Sputnik V, etc.) and vaccination priority groups for various countries separately.
If you’re working on an infographic that includes map data, like this example, try Visme’s map data visualization tool . It comes equipped with a handy hover tooltip that labels country names and square footage. If you don’t need to show this data, you can hide it in the Map settings.
Create demographic visualization easily with Visme’s map templates . If you need to edit your map infographic on the go, you can do so from the mobile app on Android and iOS.
18. Blindsight
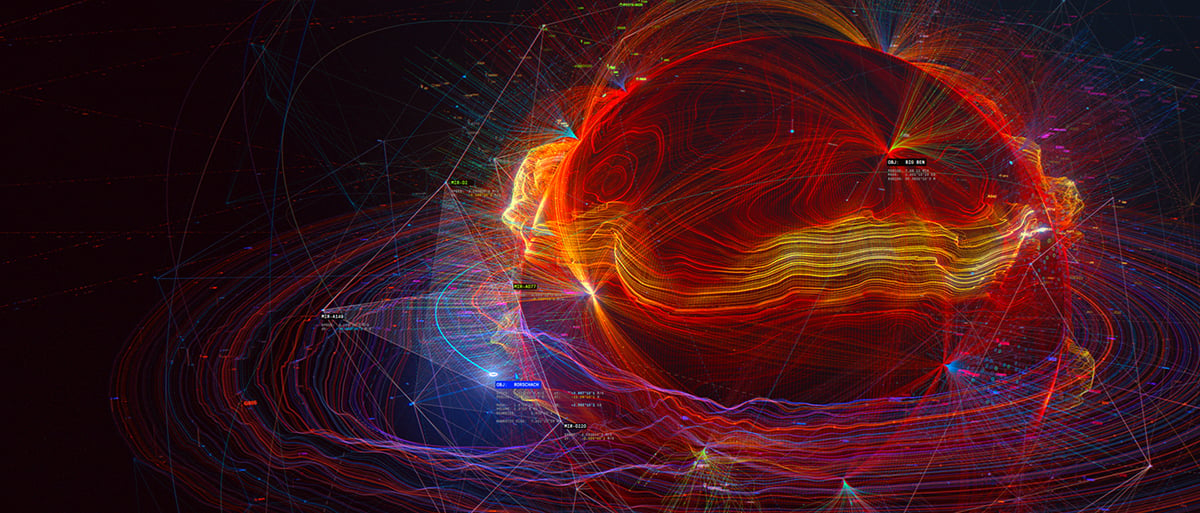
It took 4 years to create this non-commercial self-funded project. Based on the eponymous sci-fi novel by Peter Watts, this visualization row includes breathtaking renders of the solar system, four-dimensional objects as a system of data visualization and manipulation, spacesuit interface renders, cryo capsule graphics and nonhuman species concepts.
The visualization received over a dozen awards and nominations such as Best VFX Screen Power Film Festival 2020, Outstanding Achievement Award (Sci-fi Short) Indie Short Fest LA 2020, Winner Best Sound & Music Fantasy/Sci-fi film Festival 2021, Award Winner Flickfair 2020, Official selection Miami International Sci-fi Film Festival 2021 and so on.
Space mysteries have always tempted mankind. With the outstanding talent of the team behind the project, we hope to enjoy the related movie one day.
19. Gravitational Waves
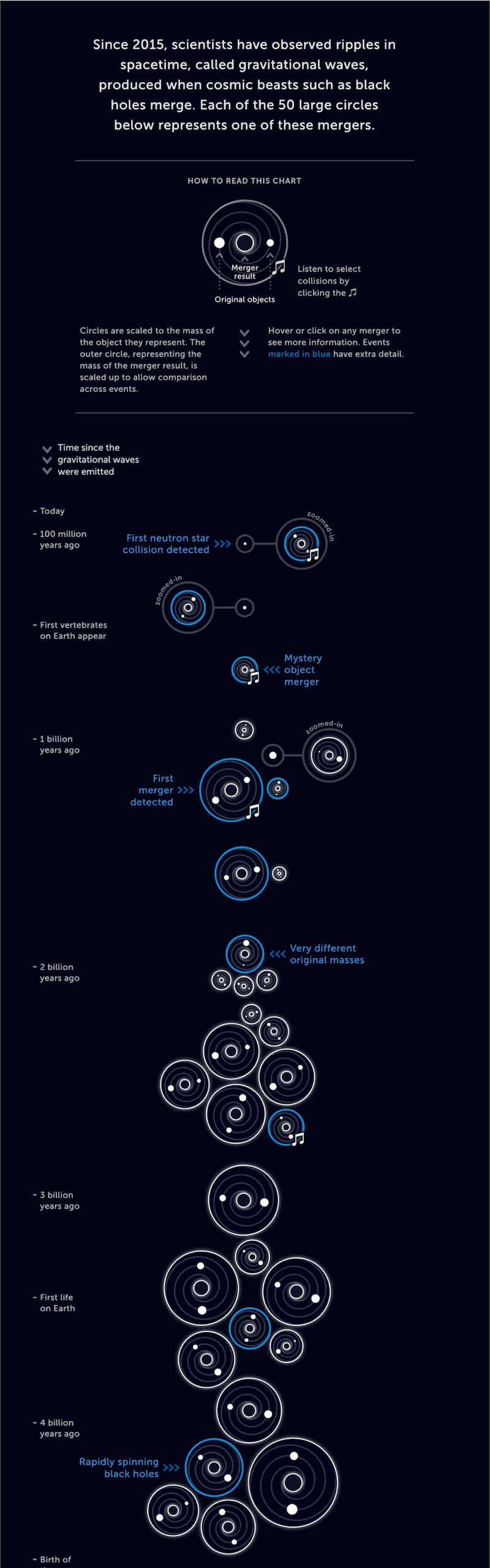
Introducing to you another captivating space-themed project – the interactive visualization of gravitational wave events. Created for Science News, this space-time ripples design is amazingly minimalistic, slick and informative.
This enchanting spiral animation is saturated with useful data about black hole mergers or cosmic smashups. You can learn about the original and final mass of the mergers, total merger size and other details of gravitational wave events.
20. Map of the Lighthouses of Ireland
Updated my map of the lighthouses of Ireland from the #30DayMapChallenge - now with the correct timings/flash patterns etc. Thanks to @IrishLights for providing additional information pic.twitter.com/eLlicP8fw5 — Neil Southall (@neilcfd1) December 8, 2020
This great animation was created as a part of 30 Day Map Challenge and it depicts all lighthouses in Ireland according to their timing and flash patterns. Here, the author visualizes data from the IrishLights – the maritime organization delivering the safety service around the coast of Ireland.
Aside from being a vital part of the water safety of coastal waterways, lighthouses are a symbol of hope and undying light even through the toughest circumstances. That’s one of the reasons why this minimalistic graphic is so appealing.
21. Together [Hierarchical Positions of Employees in a Corporation]
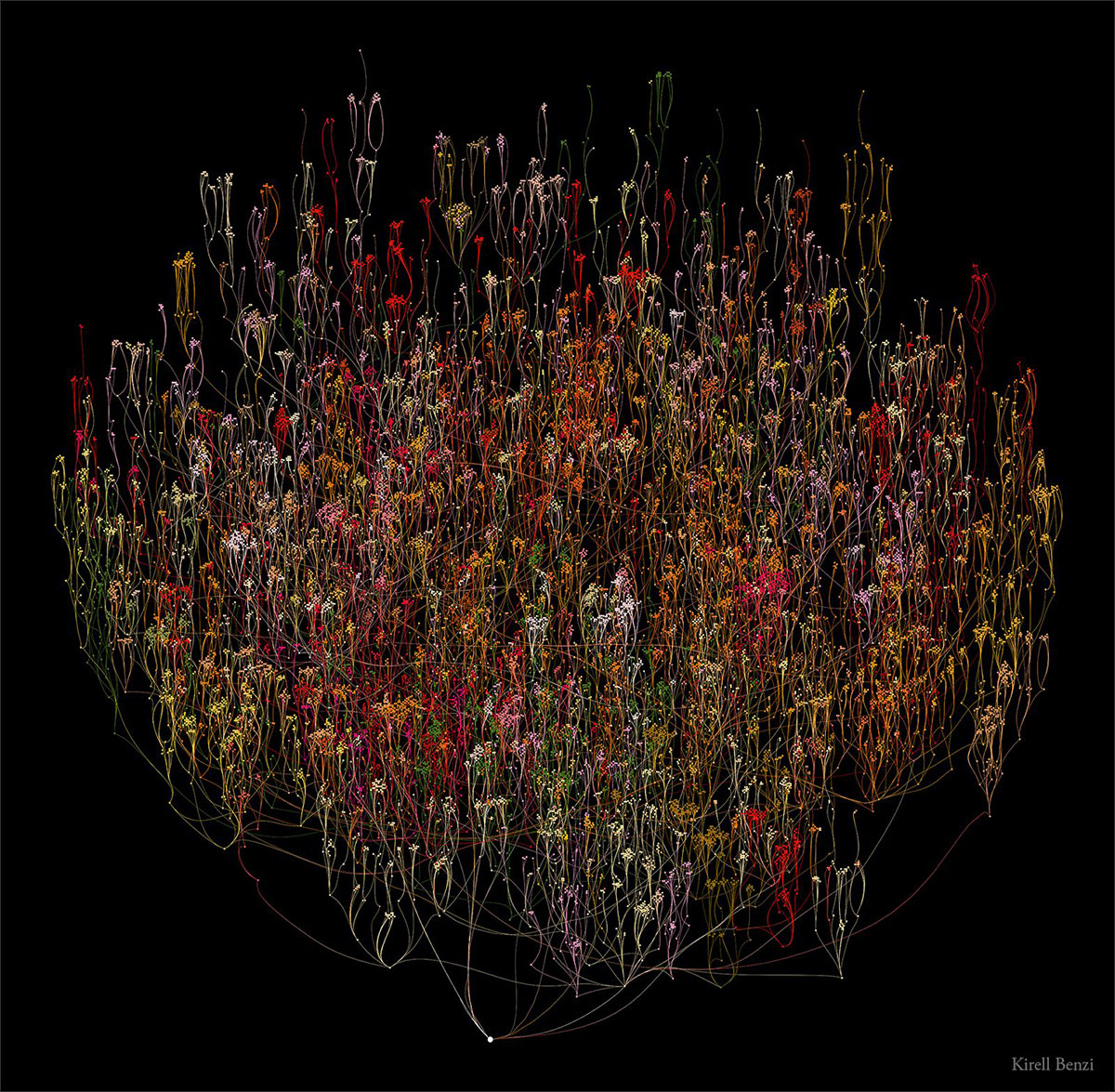
Good data visualizations are essential for conveying complex information in an easily understandable way. Look at this creative way of displaying the hierarchical organization structure in a large corporation with a presence in over 100 countries. This creative data visualization example looks fun and a bit otherworldly, with muffled but contrasting colors.
Linking C-level executives to their subordinates in every branch revealed an intricate and complex corporation structure. It’s suggested that in most cases, flat patterns would fail to represent company structures correctly because of the flexibility of human relations.
22. The Search for Dark Matter
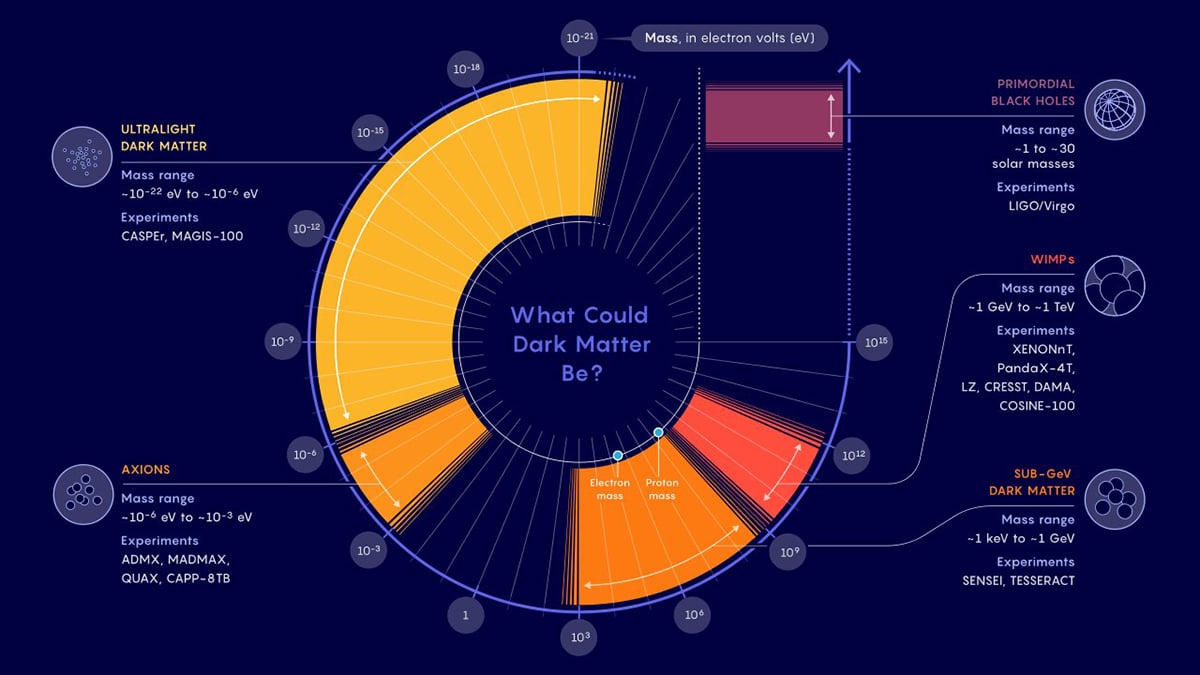
The search for the ever elusive and intriguing dark matter continues. The problem isn’t likely to get solved any time soon – but here is a striking infographic for you to follow the lead.
Quanta Magazine created this interesting data visualization to represent the types of particles that dark matter could be made of. Axions, WIMPs, ultralight dark matter or primordial black holes – any of these could be a star candidate.
Distributing every particle type along the scale according to their mass, the visualization also provides clear, concise descriptions for every type. Additionally, you can dive into the experiments’ data. Are you the one to solve the new puzzle in particle physics?
23. Stolen Paintings
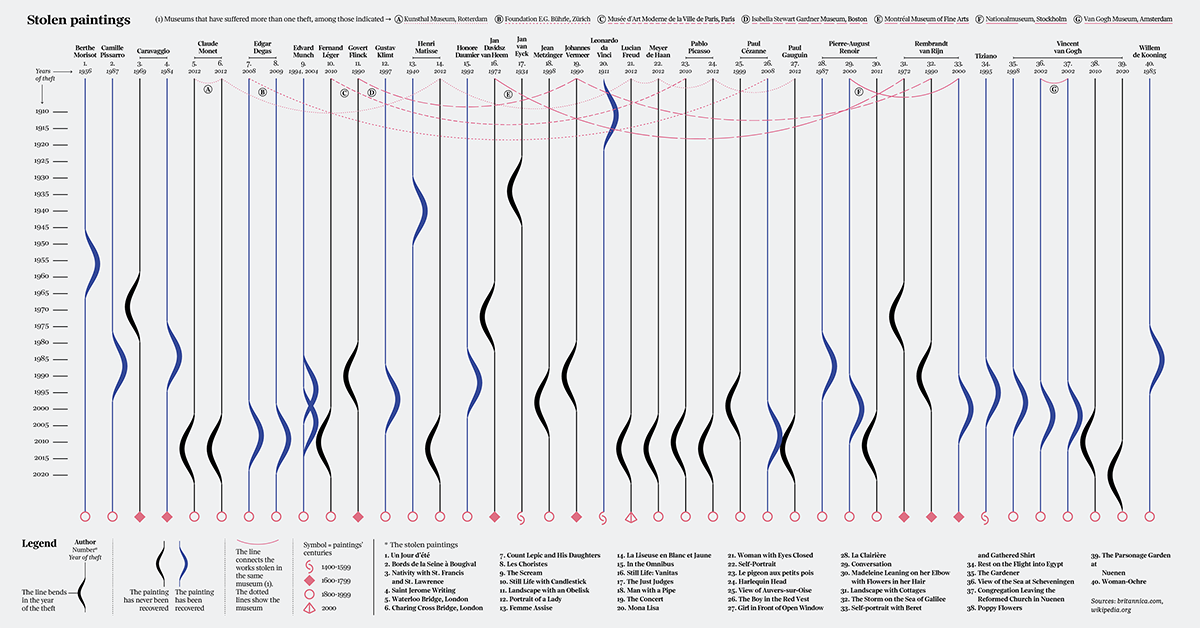
This wonderful visualization was created for Visual Data, a column on "La Lettura," the cultural supplement of "Corriere Della Sera."
From 1900 to the present day, the infographic reveals the details of 40 stolen paintings. Neutral, minimalistic visuals highlight the painting’s artist, the year when the painting was created and the year of theft.
It was shocking to find out that the majority of thefts took place during the last 20 years (2000-2020) – and most of the art works have never been recovered.
24. The U.S. Election Twitter Network Graph Tool
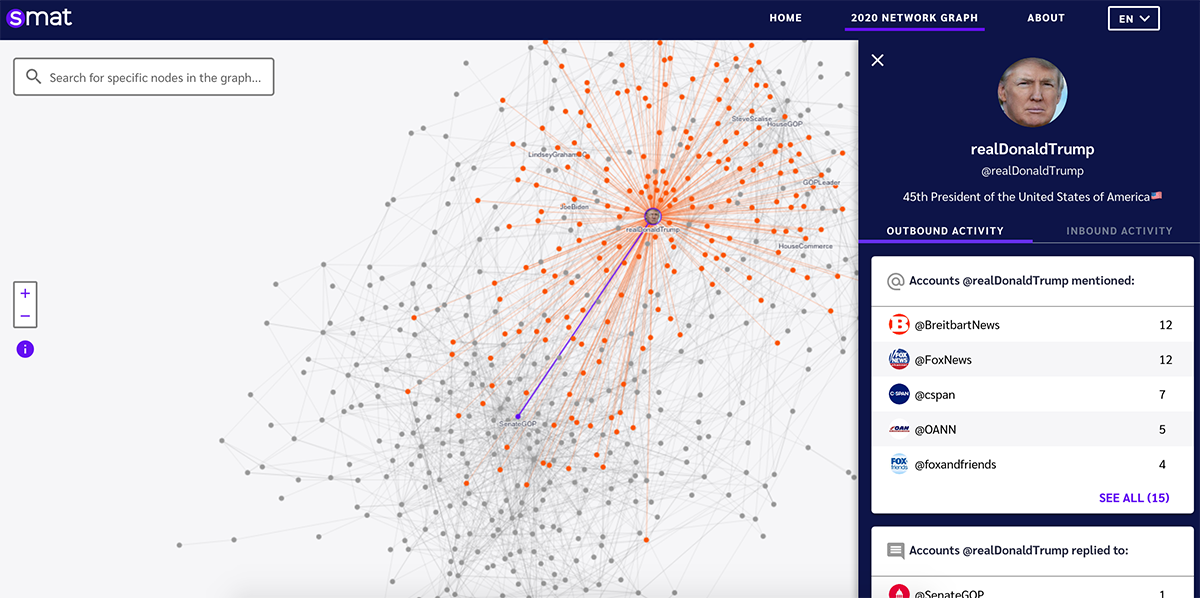
These cutting edge visuals from the U.S. Election Twitter Network Graph Tool enables a viewer to analyze social media interactions that define the online political landscape. In this case, we’re tracking the influence and connections between various political figures.
It’s clearly visible which accounts the target account is most likely to mention or reply to. The network graphs clearly show the potential of certain accounts to generate new connections and influence their followers.
You can search for specific nodes in the interactive map. All information flow between nodes is reflected in the color of the node edges. Working together with other open-source investigation tools, this graph is meant to increase transparency and help fight misinformation in social networks.
25. Map of a Fly Brain
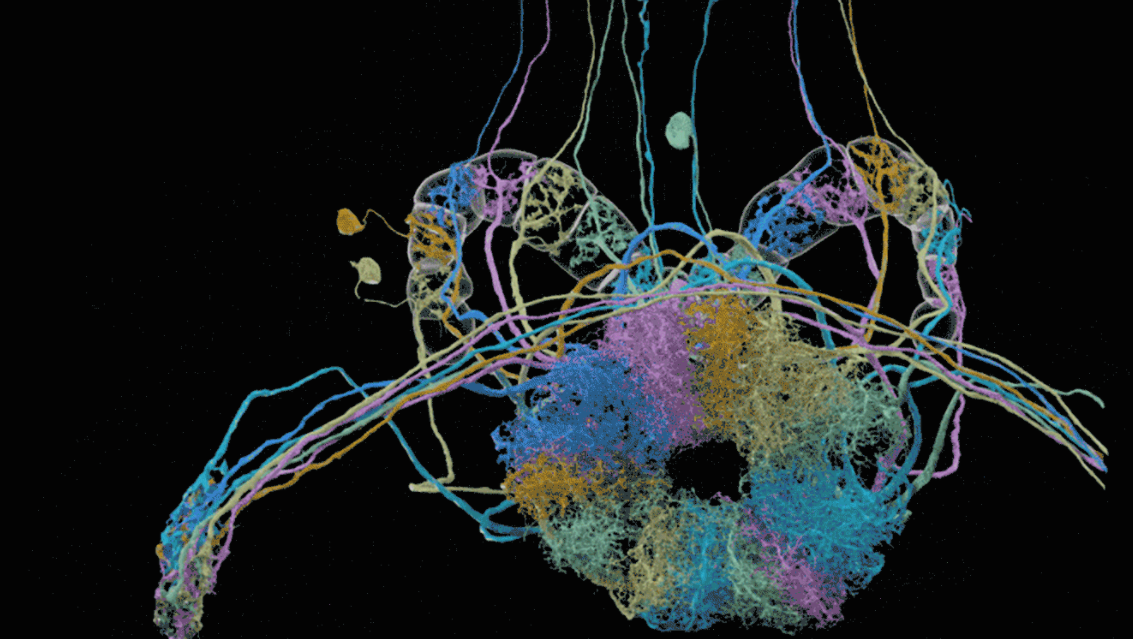
The high-resolution nervous system map represented in the above graphic is a part of the fruit-fly’s brain – yet the complexity and harmony of the structure is astounding.
Millions of connections between 25,000 neurons create a wiring diagram, or connectome, of connections in various parts of a fruit fly’s brain.
It’s estimated that tracking all neuron connections in the fruit fly’s brain manually would need 250 people working for 20 years at least. Google’s computational power has helped to speed up this research, and scientists are aiming to create a full fruit fly brain visualization by 2022.
26. Freight Rail Works
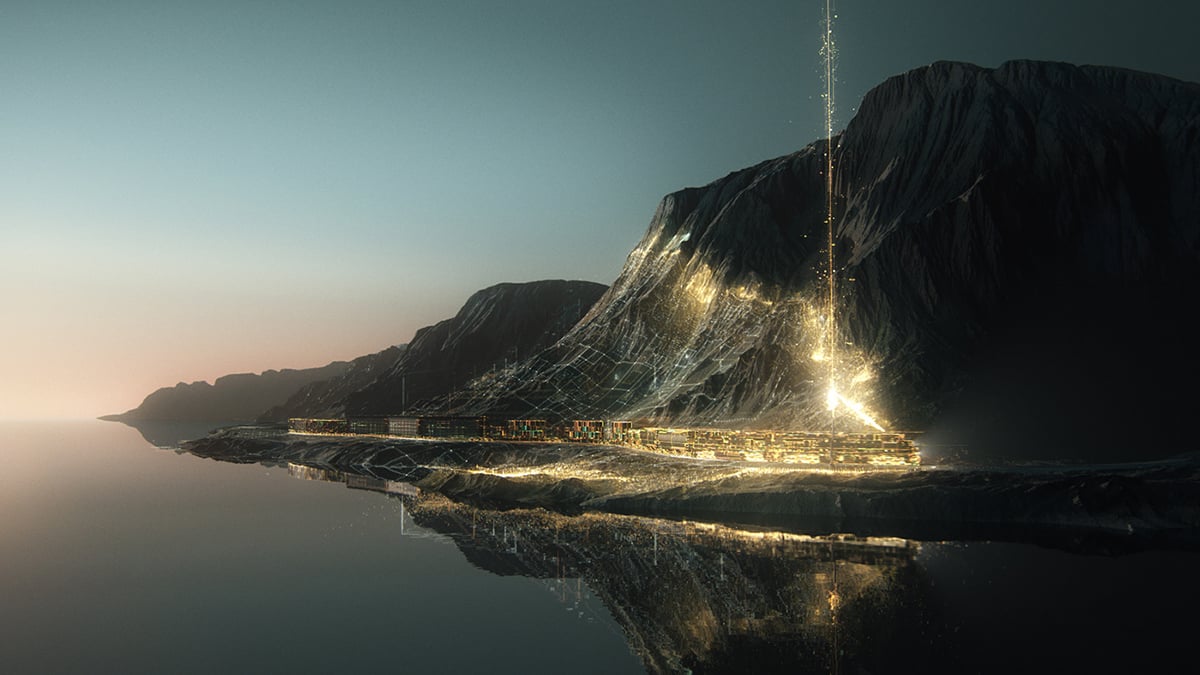
Our next interesting visualization highlights the advanced layers of technology Freight Rail Works uses across its infrastructure. Talented Danil Krivoruchko & Aggressive/Loop teams produced a futuristic and dynamic animation of the data-world around a train in motion.
Magnificent waves of data light up outlines of the objects and then vanish in waves as the train moves forward to the smart city. Graphics of the giant city cluster zoom out to reveal the continent routes and the beauty of a simple railway communications network.
In the era of semi-autonomous aircrafts and drones, the simple, down-to-earth railway system looks stable but innovative in this graphic.
27. The Korean Clusters
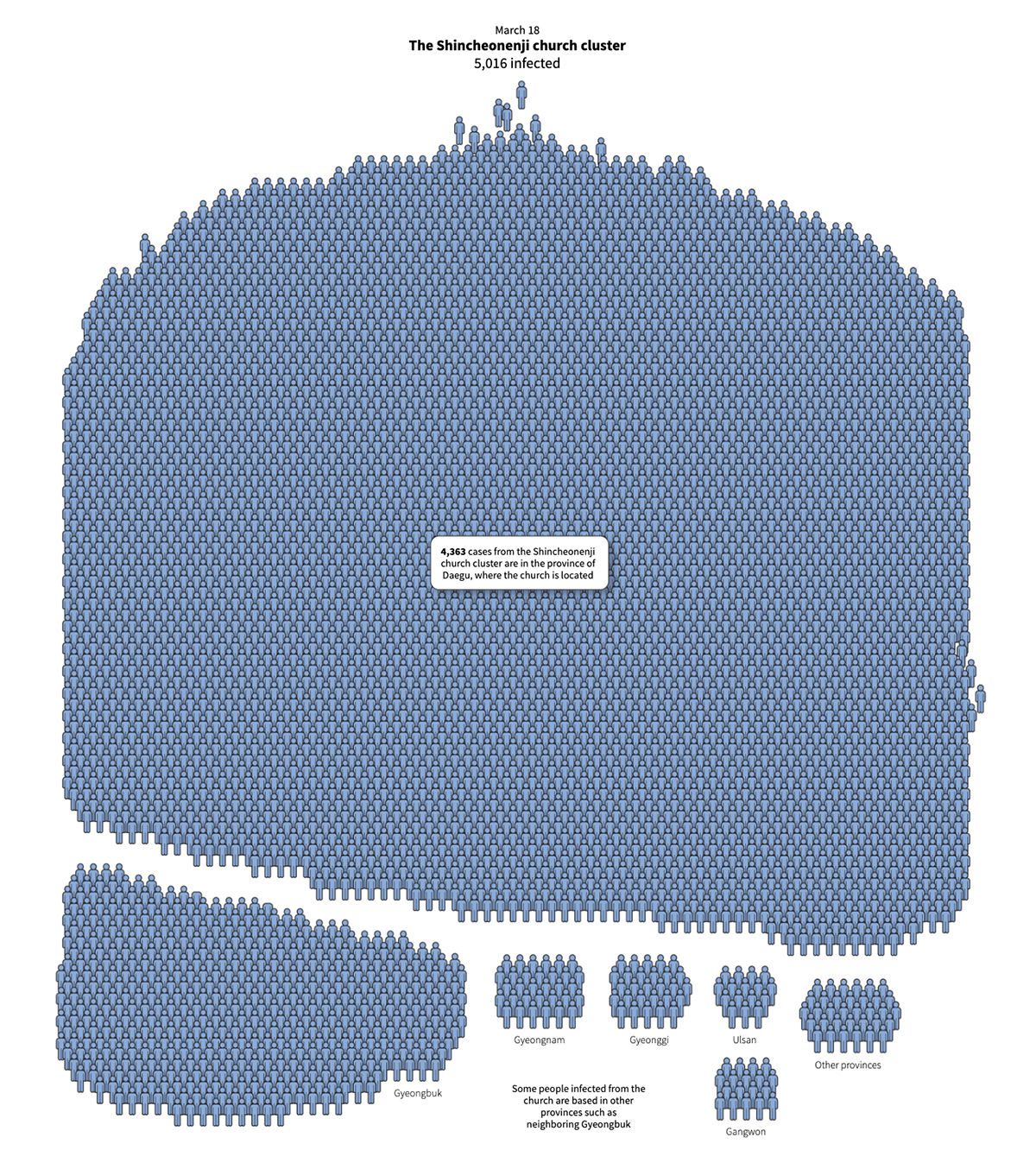
Korean hospitals and churches experienced a burst of Covid infections among their visitors in January 2020. Having linked connections between the confirmed cases, scientists were able to trace back the first case and build a tree of contacts between the affected people.
Tracking the timeline of the first patient’s actions revealed that this person caused thousands of infections. Wandering sick for a few days resulted in over 30 more people infected. Subsequently, the Shincheonji Church cluster with 5,016 infected people accounted for at least 60% of all cases in South Korea at that time.
28. The Biggest Tech Mergers and Acquisitions
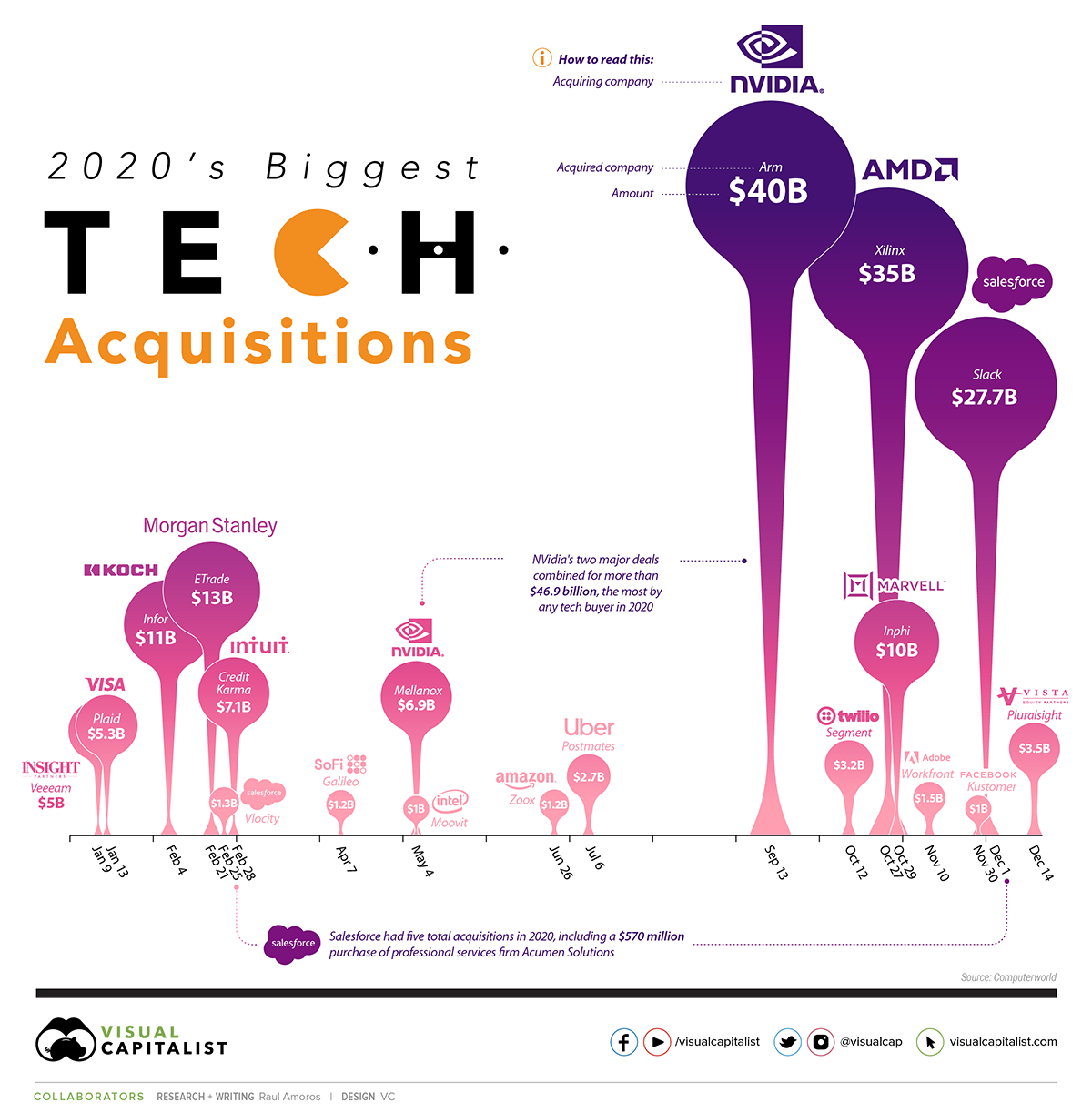
Despite the fact that for most businesses 2020 was a devastating year with grim outcomes, this data visualization shows that Big Tech experienced a growth boost. It’s not surprising that people working remotely increasingly need digital services of all kinds.
The graphic shows the biggest tech mergers and acquisitions closed in 2020, together with the short description of the acquired company, acquiring company, deal amount and deal date. While the chart is visually busy, it’s also innovative and visually appealing.
If you need a market report from your industry area, grab the data from Crunchbase and build your own custom branded infographic via our data visualization tool quickly and easily. Sign up free .
29. The Ancient Seven Wonders of the World
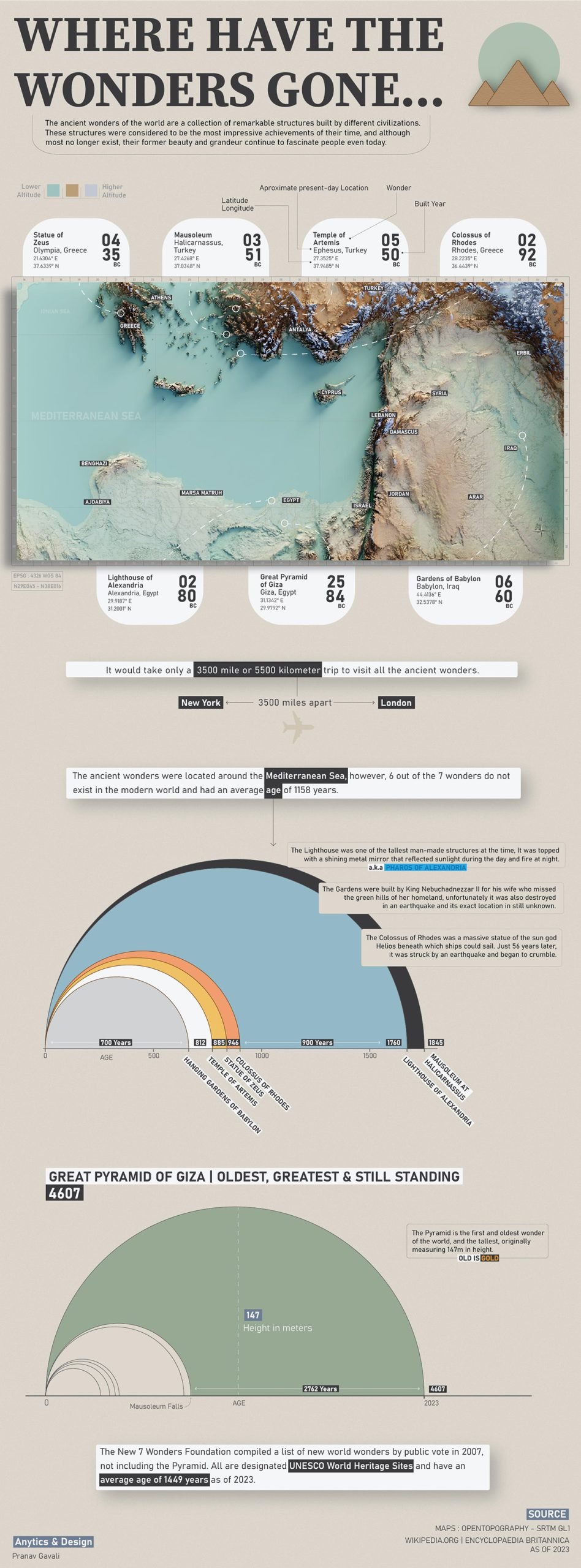
The civil engineering feats of humankind have reached the highest peaks of the mountains and deep into the ocean, and we have built pyramids, temples and statues that are still standing today.
The seven wonders of the ancient world are a collection of man-made structures that are considered to be remarkable feats at the time they were built.
Pranav Gavali, a Data Scientist, created this graphic using data from Encyclopedia Britannica and Wikipedia to visualize the world's seven ancient wonders along with their features and modern-day locations.
The graphic perfectly illustrates how the seven wonders were built and why they are considered a wonder of the world. The Great Pyramid of Giza is the only one of the seven wonders that still stands today.
Design an infographic like this one using Visme’s pre-designed content blocks and infographic templates . Include live data visualizations by connecting to your Google or Excel spreadsheets. When connecting your Visme charts to Excel Online, select full sheets or only a specific range. Plus, when values change in your linked sheet, the chart is This is a prime example of how creative design can bring data to life.
30. Cell Towers Map of the World
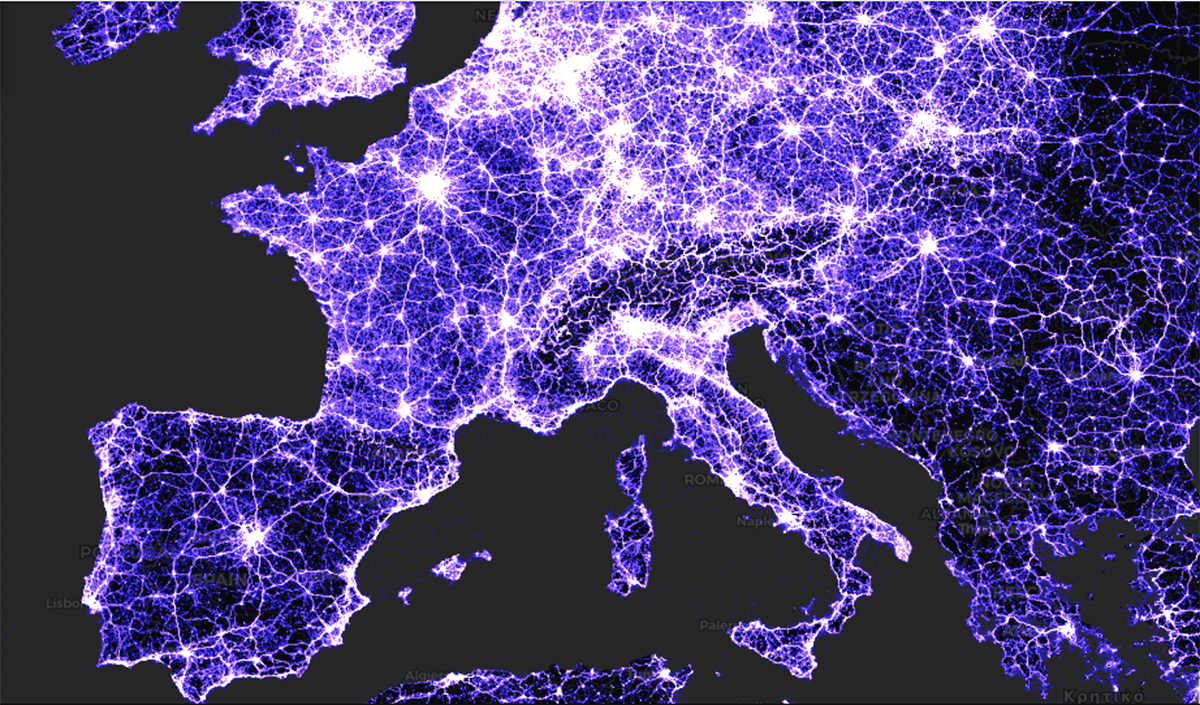
This stunning, elegant and creative visualization of 40 million cell towers is surely an unforgettable view. Based on OpenCelliD, the world's largest open database of cell towers, this interactive map is so far one of the most precise publicly available data sources for telecom-related projects.
We can see how the cell tower network lights up Europe and other big cities of the world; simultaneously, vast areas of “wilderness” are still present on the map. Harsh climate and low population density in the northern regions of Russia and Canada, along with central areas of Africa and Mongolia result in low quantity of cell towers in these areas.
Closeup view of this cell tower map resembles the brain structure. Similar to the neurons, axons and dendrites that create the communication network of the human body – cell towers keep humanity connected.
Bonus Data Visualization:
31. house of cards lidar.
House of Cards from Brendan Dawes on Vimeo .
Take a look at the last cool data visualization in this list – the rework of Radiohead's House of Cards video. This astonishing art was created on the basis of around one minute of the LIDAR data.
Motion graphics of particles scattered around a person’s face create an unforgettable image. The hero of the story in the video is clearly emotional – but we can’t tell anymore whether this person is even human.
AI generated data can be beautiful, but how can you take control?
32. Price of a Pandemic: Poverty Spreads Around the Globe
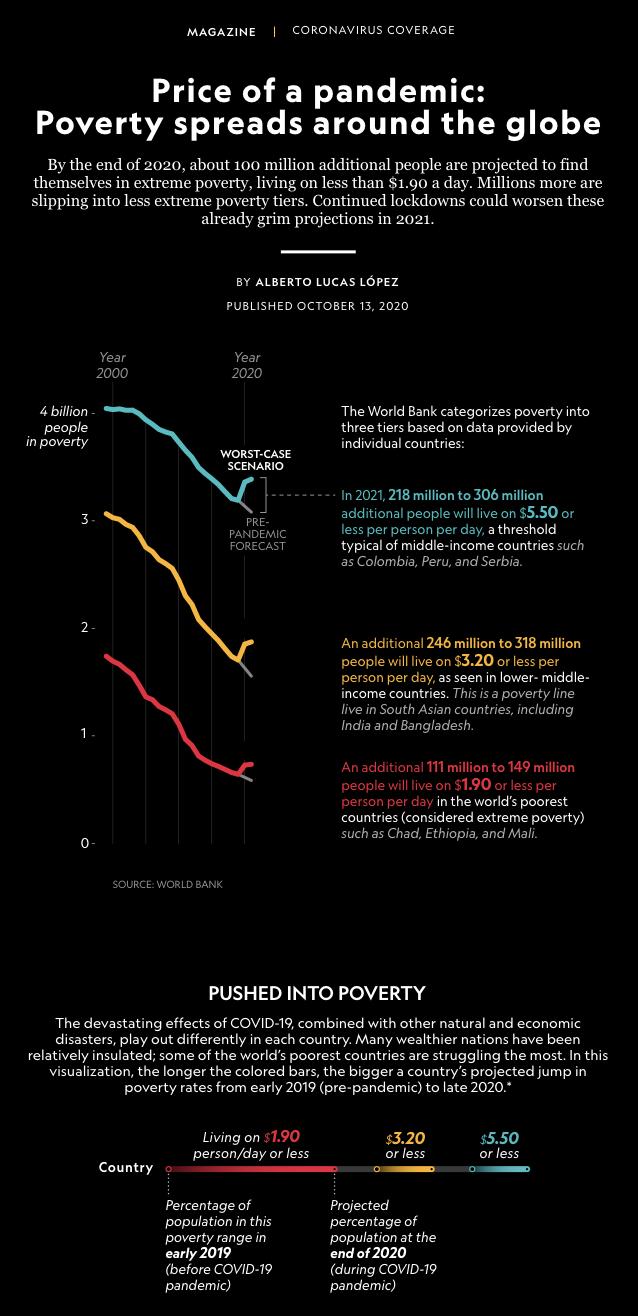
In this classic data visualization by National Geographic, data is placed against the dark background for better contrast and readability. Simple, comprehensive charts show us the effect of the pandemic on the income of people in various countries.
The authors distributed three levels of income range for countries with low and middle class income to provide a clear picture of the current situation. Core findings of the report were that the pandemic pushed a tremendous amount of people to extreme poverty – projected data is 100 million of people living on $1.90 per person/day.
Based on the World Bank data, the infographic provides a wide view of the exact factors influencing people’s wellbeing – from travel restrictions and job loss to wars, displacements and higher food costs. Highlights at the beginning reveal rapid shrinking of income in examined countries across all continents on a mass scale.
33. Selfiecity – The Science of Selfies
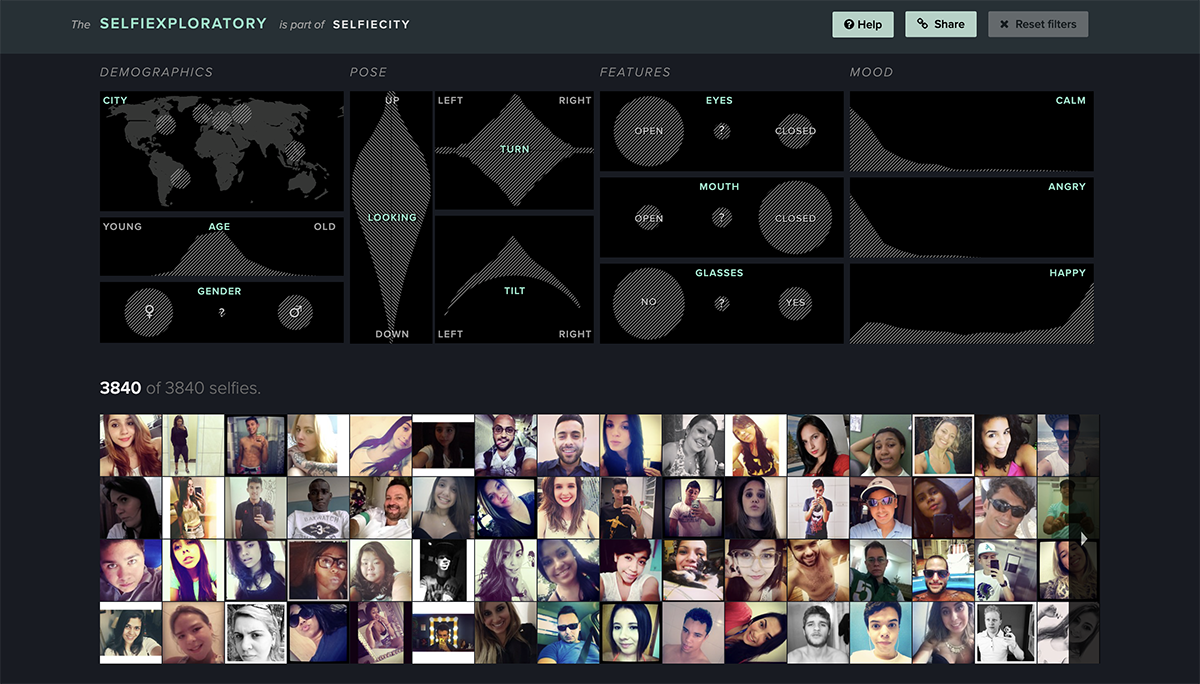
Selfiecity is an innovative and engaging data visualization project exploring the selfies world. It uses a variety of visualizations to analyze selfies from five cities around the world.
They collected over 120,000 selfies from the five cities and selected nearly 1,000 photos from each town. After collecting the images, they analyzed various metrics such as demographics, poses, moods and features.
The project then revealed exciting insights into the culture and social behavior of the people who take selfies. For example, the project shows that women take more selfies than men and that people tend to take selfies in public places rather than private spaces.
The study was quite complex and yielded valuable insights, which presented a challenge when it came to sharing the results . However, the team did an excellent job creating visually appealing data visualizations to present the information.
34. Icebergs and Climate Change
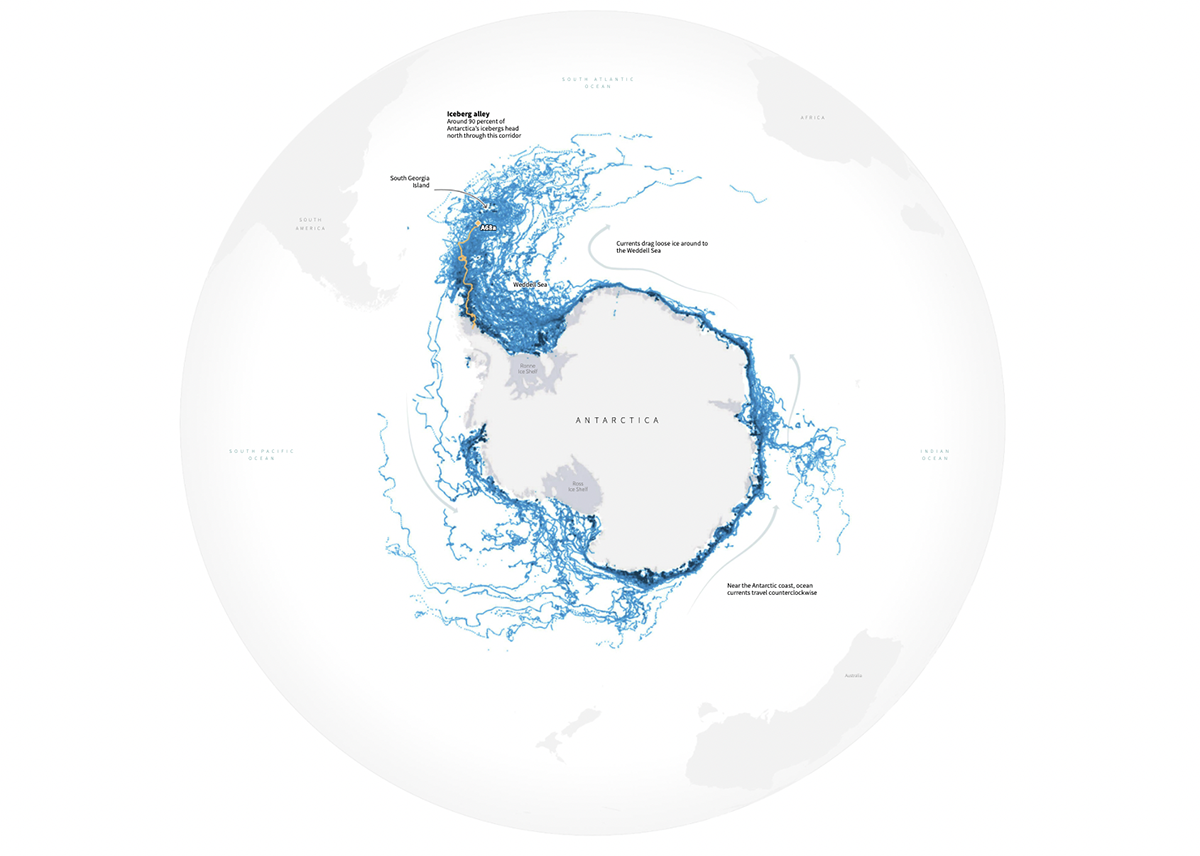
Dedicated to “travel adventures” of this 4,200-square-kilometer iceberg, this infographic alerts people to climate change. A giant chunk of ice the length of Puerto Rico broke off the Antarctic peninsula coast to wander into the wild – and dangerously close to South Georgia Island, packed with wildlife.
The graphic compares the size of the berg with 66 countries or territories and cites that the ice mass is so large that it cannot be captured in one photograph. Besides, we can also see impressive geodata on the wildlife from the IUCN Red List of Threatened Species inhabiting the endangered South Georgia Island.
Data Visualization FAQs
What is the most popular form of data visualization.
Bar graphs, bar charts or column charts are the most popular type of data visualization.
Bar charts are best for comparing numerical values across categories using rectangles (or bars) of equal width and variable height. You can use bar graphs to compare items between different groups, measure changes over time and identify patterns or trends.
Other popular forms of data visualization include pie charts , line graphs , area charts , histograms , pivot tables, boxplots, scatter plots , radar charts and choropleth maps.
What Are the Benefits of Data Visualization?
Here’s how data visualization helps users to make the most of their data.
- Data visualizations make data clear, concise and easy to understand. Users can easily unlock key values from massive data sets, interpret them and draw conclusions.
- Visualization allows business users to identify relationships, patterns and trends between data, giving it greater meaning. You can easily uncover fresh insights and focus areas that require more attention.
- Creative data visualization is about creating compelling narratives through the use of graphics, diagrams and visual analytics. Visualizing data helps users tell better stories and convey messages in an engaging manner.
- Data visualization can significantly increase the pace of decision-making processes since it makes it simple for us to understand visual data. It’s no surprise, as The Wharton School of Business says that data visualization can cut down on meeting time by up to 24% .
Visualizing data helps quickly spot any errors so they can be removed. If you still doubt the importance of data visualization, this article about 50 data visualization statistics might change your thought process.
What are the Best Practices of Data Visualization?
Below are data visualization best practices to help you present data in an engaging and appealing way.
- Specify the audience and their unique needs. Your data visualization should be crafted to communicate, provide real value and meet the needs of the target audience.
- Define a Clear Purpose. Specify what questions you want your data visualizations to answer or the problems you want them to solve.
- Keep your data clean. Before visualizing your data, make sure to fix or remove incomplete, duplicate, incorrect, corrupted and incorrectly formatted data within your dataset.
- Use the right visuals. With so many charts available, identify the best type for presenting the particular data type you’re working on.
- Keep your data organized. At a glance, your audience should be able to view and digest information quickly.
- Use the right color combination.
Read our article to learn more about data visualization best practices.
Create Your Own Data Visualizations
If you are feeling inspired by these cool data visualizations, use our data visualization software to convert disparate data into clean, comprehensive visuals using the best data visualization techniques . You'll find an extensive library of customizable charts and graphs including bubble charts, bar graphs , line charts , scatter plots, and much more.
Wondering if Visme's data visualization tools are right for you? Take a look at what one of our satisfied customers, Cassandra C. | Owner, has to say:
“I also appreciate the wide range of features, including charts, graphs, and other visuals that can be used to present data in a clear and concise way. Overall, I'm very happy with Visme and would highly recommend it to anyone looking for a fun, user-friendly tool to create visuals.”
To learn more about creating your own data visualizations, check out our detailed guide on data visualization types and the introduction to data viz on our blog.
Create beautiful charts, graphs and data visualizations with ease.

Trusted by leading brands
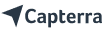
Recommended content for you:
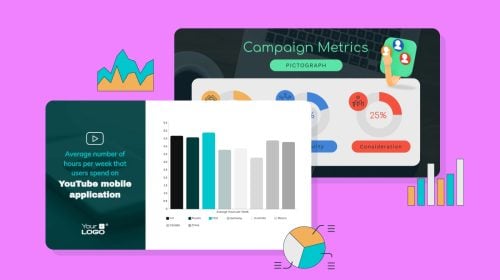
Create Stunning Content!
Design visual brand experiences for your business whether you are a seasoned designer or a total novice.
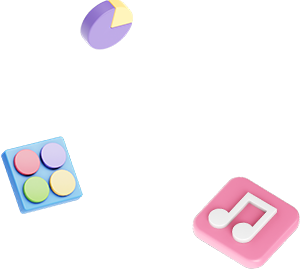
About the Author
Anna enjoys hot weather, collecting shells, and solving challenges in B2B marketing. She delights in thinking about abstract ideas and analyzing complex information to choose the best solution.

An official website of the United States government
The .gov means it’s official. Federal government websites often end in .gov or .mil. Before sharing sensitive information, make sure you’re on a federal government site.
The site is secure. The https:// ensures that you are connecting to the official website and that any information you provide is encrypted and transmitted securely.
- Publications
- Account settings
Preview improvements coming to the PMC website in October 2024. Learn More or Try it out now .
- Advanced Search
- Journal List
- Patterns (N Y)
- v.1(9); 2020 Dec 11

Principles of Effective Data Visualization
Stephen r. midway.
1 Department of Oceanography and Coastal Sciences, Louisiana State University, Baton Rouge, LA 70803, USA
We live in a contemporary society surrounded by visuals, which, along with software options and electronic distribution, has created an increased importance on effective scientific visuals. Unfortunately, across scientific disciplines, many figures incorrectly present information or, when not incorrect, still use suboptimal data visualization practices. Presented here are ten principles that serve as guidance for authors who seek to improve their visual message. Some principles are less technical, such as determining the message before starting the visual, while other principles are more technical, such as how different color combinations imply different information. Because figure making is often not formally taught and figure standards are not readily enforced in science, it is incumbent upon scientists to be aware of best practices in order to most effectively tell the story of their data.
The Bigger Picture
Visuals are an increasingly important form of science communication, yet many scientists are not well trained in design principles for effective messaging. Despite challenges, many visuals can be improved by taking some simple steps before, during, and after their creation. This article presents some sequential principles that are designed to improve visual messages created by scientists.
Many scientific visuals are not as effective as they could be because scientists often lack basic design principles. This article reviews the importance of effective data visualization and presents ten principles that scientists can use as guidance in developing effective visual messages.
Introduction
Visual learning is one of the primary forms of interpreting information, which has historically combined images such as charts and graphs (see Box 1 ) with reading text. 1 However, developments on learning styles have suggested splitting up the visual learning modality in order to recognize the distinction between text and images. 2 Technology has also enhanced visual presentation, in terms of the ability to quickly create complex visual information while also cheaply distributing it via digital means (compared with paper, ink, and physical distribution). Visual information has also increased in scientific literature. In addition to the fact that figures are commonplace in scientific publications, many journals now require graphical abstracts 3 or might tweet figures to advertise an article. Dating back to the 1970s when computer-generated graphics began, 4 papers represented by an image on the journal cover have been cited more frequently than papers without a cover image. 5
Regarding terminology, the terms graph , plot , chart , image , figure , and data visual(ization) are often used interchangeably, although they may have different meanings in different instances. Graph , plot , and chart often refer to the display of data, data summaries, and models, while image suggests a picture. Figure is a general term but is commonly used to refer to visual elements, such as plots, in a scientific work. A visual , or data visualization , is a newer and ostensibly more inclusive term to describe everything from figures to infographics. Here, I adopt common terminology, such as bar plot, while also attempting to use the terms figure and data visualization for general reference.
There are numerous advantages to quickly and effectively conveying scientific information; however, scientists often lack the design principles or technical skills to generate effective visuals. Going back several decades, Cleveland 6 found that 30% of graphs in the journal Science had at least one type of error. Several other studies have documented widespread errors or inefficiencies in scientific figures. 7 , 8 , 9 In fact, the increasing menu of visualization options can sometimes lead to poor fits between information and its presentation. These poor fits can even have the unintended consequence of confusing the readers and setting them back in their understanding of the material. While objective errors in graphs are hopefully in the minority of scientific works, what might be more common is suboptimal figure design, which takes place when a design element may not be objectively wrong but is ineffective to the point of limiting information transfer.
Effective figures suggest an understanding and interpretation of data; ineffective figures suggest the opposite. Although the field of data visualization has grown in recent years, the process of displaying information cannot—and perhaps should not—be fully mechanized. Much like statistical analyses often require expert opinions on top of best practices, figures also require choice despite well-documented recommendations. In other words, there may not be a singular best version of a given figure. Rather, there may be multiple effective versions of displaying a single piece of information, and it is the figure maker's job to weigh the advantages and disadvantages of each. Fortunately, there are numerous principles from which decisions can be made, and ultimately design is choice. 7
The data visualization literature includes many great resources. While several resources are targeted at developing design proficiency, such as the series of columns run by Nature Communications , 10 Wilkinson's The Grammar of Graphics 11 presents a unique technical interpretation of the structure of graphics. Wilkinson breaks down the notion of a graphic into its constituent parts—e.g., the data, scales, coordinates, geometries, aesthetics—much like conventional grammar breaks down a sentence into nouns, verbs, punctuation, and other elements of writing. The popularity and utility of this approach has been implemented in a number of software packages, including the popular ggplot2 package 12 currently available in R. 13 (Although the grammar of graphics approach is not explicitly adopted here, the term geometry is used consistently with Wilkinson to refer to different geometrical representations, whereas the term aesthetics is not used consistently with the grammar of graphics and is used simply to describe something that is visually appealing and effective.) By understanding basic visual design principles and their implementation, many figure authors may find new ways to emphasize and convey their information.
The Ten Principles
Principle #1 diagram first.
The first principle is perhaps the least technical but very important: before you make a visual, prioritize the information you want to share, envision it, and design it. Although this seems obvious, the larger point here is to focus on the information and message first, before you engage with software that in some way starts to limit or bias your visual tools. In other words, don't necessarily think of the geometries (dots, lines) you will eventually use, but think about the core information that needs to be conveyed and what about that information is going to make your point(s). Is your visual objective to show a comparison? A ranking? A composition? This step can be done mentally, or with a pen and paper for maximum freedom of thought. In parallel to this approach, it can be a good idea to save figures you come across in scientific literature that you identify as particularly effective. These are not just inspiration and evidence of what is possible, but will help you develop an eye for detail and technical skills that can be applied to your own figures.
Principle #2 Use the Right Software
Effective visuals typically require good command of one or more software. In other words, it might be unrealistic to expect complex, technical, and effective figures if you are using a simple spreadsheet program or some other software that is not designed to make complex, technical, and effective figures. Recognize that you might need to learn a new software—or expand your knowledge of a software you already know. While highly effective and aesthetically pleasing figures can be made quickly and simply, this may still represent a challenge to some. However, figure making is a method like anything else, and in order to do it, new methodologies may need to be learned. You would not expect to improve a field or lab method without changing something or learning something new. Data visualization is the same, with the added benefit that most software is readily available, inexpensive, or free, and many come with large online help resources. This article does not promote any specific software, and readers are encouraged to reference other work 14 for an overview of software resources.
Principle #3 Use an Effective Geometry and Show Data
Geometries are the shapes and features that are often synonymous with a type of figure; for example, the bar geometry creates a bar plot. While geometries might be the defining visual element of a figure, it can be tempting to jump directly from a dataset to pairing it with one of a small number of well-known geometries. Some of this thinking is likely to naturally happen. However, geometries are representations of the data in different forms, and often there may be more than one geometry to consider. Underlying all your decisions about geometries should be the data-ink ratio, 7 which is the ratio of ink used on data compared with overall ink used in a figure. High data-ink ratios are the best, and you might be surprised to find how much non-data-ink you use and how much of that can be removed.
Most geometries fall into categories: amounts (or comparisons), compositions (or proportions), distributions , or relationships . Although seemingly straightforward, one geometry may work in more than one category, in addition to the fact that one dataset may be visualized with more than one geometry (sometimes even in the same figure). Excellent resources exist on detailed approaches to selecting your geometry, 15 and this article only highlights some of the more common geometries and their applications.
Amounts or comparisons are often displayed with a bar plot ( Figure 1 A), although numerous other options exist, including Cleveland dot plots and even heatmaps ( Figure 1 F). Bar plots are among the most common geometry, along with lines, 9 although bar plots are noted for their very low data density 16 (i.e., low data-ink ratio). Geometries for amounts should only be used when the data do not have distributional information or uncertainty associated with them. A good use of a bar plot might be to show counts of something, while poor use of a bar plot might be to show group means. Numerous studies have discussed inappropriate uses of bar plots, 9 , 17 noting that “because the bars always start at zero, they can be misleading: for example, part of the range covered by the bar might have never been observed in the sample.” 17 Despite the numerous reports on incorrect usage, bar plots remain one of the most common problems in data visualization.

Examples of Visual Designs
(A) Clustered bar plots are effective at showing units within a group (A–C) when the data are amounts.
(B) Histograms are effective at showing the distribution of data, which in this case is a random draw of values from a Poisson distribution and which use a sequential color scheme that emphasizes the mean as red and values farther from the mean as yellow.
(C) Scatterplot where the black circles represent the data.
(D) Logistic regression where the blue line represents the fitted model, the gray shaded region represents the confidence interval for the fitted model, and the dark-gray dots represent the jittered data.
(E) Box plot showing (simulated) ages of respondents grouped by their answer to a question, with gray dots representing the raw data used in the box plot. The divergent colors emphasize the differences in values. For each box plot, the box represents the interquartile range (IQR), the thick black line represents the median value, and the whiskers extend to 1.5 times the IQR. Outliers are represented by the data.
(F) Heatmap of simulated visibility readings in four lakes over 5 months. The green colors represent lower visibility and the blue colors represent greater visibility. The white numbers in the cells are the average visibility measures (in meters).
(G) Density plot of simulated temperatures by season, where each season is presented as a small multiple within the larger figure.
For all figures the data were simulated, and any examples are fictitious.
Compositions or proportions may take a wide range of geometries. Although the traditional pie chart is one option, the pie geometry has fallen out of favor among some 18 due to the inherent difficulties in making visual comparisons. Although there may be some applications for a pie chart, stacked or clustered bar plots ( Figure 1 A), stacked density plots, mosaic plots, and treemaps offer alternatives.
Geometries for distributions are an often underused class of visuals that demonstrate high data density. The most common geometry for distributional information is the box plot 19 ( Figure 1 E), which shows five types of information in one object. Although more common in exploratory analyses than in final reports, the histogram ( Figure 1 B) is another robust geometry that can reveal information about data. Violin plots and density plots ( Figure 1 G) are other common distributional geometries, although many less-common options exist.
Relationships are the final category of visuals covered here, and they are often the workhorse of geometries because they include the popular scatterplot ( Figures 1 C and 1D) and other presentations of x - and y -coordinate data. The basic scatterplot remains very effective, and layering information by modifying point symbols, size, and color are good ways to highlight additional messages without taking away from the scatterplot. It is worth mentioning here that scatterplots often develop into line geometries ( Figure 1 D), and while this can be a good thing, presenting raw data and inferential statistical models are two different messages that need to be distinguished (see Data and Models Are Different Things ).
Finally, it is almost always recommended to show the data. 7 Even if a geometry might be the focus of the figure, data can usually be added and displayed in a way that does not detract from the geometry but instead provides the context for the geometry (e.g., Figures 1 D and 1E). The data are often at the core of the message, yet in figures the data are often ignored on account of their simplicity.
Principle #4 Colors Always Mean Something
The use of color in visualization can be incredibly powerful, and there is rarely a reason not to use color. Even if authors do not wish to pay for color figures in print, most journals still permit free color figures in digital formats. In a large study 20 of what makes visualizations memorable, colorful visualizations were reported as having a higher memorability score, and that seven or more colors are best. Although some of the visuals in this study were photographs, other studies 21 also document the effectiveness of colors.
In today's digital environment, color is cheap. This is overwhelmingly a good thing, but also comes with the risk of colors being applied without intention. Black-and-white visuals were more accepted decades ago when hard copies of papers were more common and color printing represented a large cost. Now, however, the vast majority of readers view scientific papers on an electronic screen where color is free. For those who still print documents, color printing can be done relatively cheaply in comparison with some years ago.
Color represents information, whether in a direct and obvious way, or in an indirect and subtle way. A direct example of using color may be in maps where water is blue and land is green or brown. However, the vast majority of (non-mapping) visualizations use color in one of three schemes: sequential , diverging , or qualitative . Sequential color schemes are those that range from light to dark typically in one or two (related) hues and are often applied to convey increasing values for increasing darkness ( Figures 1 B and 1F). Diverging color schemes are those that have two sequential schemes that represent two extremes, often with a white or neutral color in the middle ( Figure 1 E). A classic example of a diverging color scheme is the red to blue hues applied to jurisdictions in order to show voting preference in a two-party political system. Finally, qualitative color schemes are found when the intensity of the color is not of primary importance, but rather the objective is to use different and otherwise unrelated colors to convey qualitative group differences ( Figures 1 A and 1G).
While it is recommended to use color and capture the power that colors convey, there exist some technical recommendations. First, it is always recommended to design color figures that work effectively in both color and black-and-white formats ( Figures 1 B and 1F). In other words, whenever possible, use color that can be converted to an effective grayscale such that no information is lost in the conversion. Along with this approach, colors can be combined with symbols, line types, and other design elements to share the same information that the color was sharing. It is also good practice to use color schemes that are effective for colorblind readers ( Figures 1 A and 1E). Excellent resources, such as ColorBrewer, 22 exist to help in selecting color schemes based on colorblind criteria. Finally, color transparency is another powerful tool, much like a volume knob for color ( Figures 1 D and 1E). Not all colors have to be used at full value, and when not part of a sequential or diverging color scheme—and especially when a figure has more than one colored geometry—it can be very effective to increase the transparency such that the information of the color is retained but it is not visually overwhelming or outcompeting other design elements. Color will often be the first visual information a reader gets, and with this knowledge color should be strategically used to amplify your visual message.
Principle #5 Include Uncertainty
Not only is uncertainty an inherent part of understanding most systems, failure to include uncertainty in a visual can be misleading. There exist two primary challenges with including uncertainty in visuals: failure to include uncertainty and misrepresentation (or misinterpretation) of uncertainty.
Uncertainty is often not included in figures and, therefore, part of the statistical message is left out—possibly calling into question other parts of the statistical message, such as inference on the mean. Including uncertainty is typically easy in most software programs, and can take the form of common geometries such as error bars and shaded intervals (polygons), among other features. 15 Another way to approach visualizing uncertainty is whether it is included implicitly into the existing geometries, such as in a box plot ( Figure 1 E) or distribution ( Figures 1 B and 1G), or whether it is included explicitly as an additional geometry, such as an error bar or shaded region ( Figure 1 D).
Representing uncertainty is often a challenge. 23 Standard deviation, standard error, confidence intervals, and credible intervals are all common metrics of uncertainty, but each represents a different measure. Expressing uncertainty requires that readers be familiar with metrics of uncertainty and their interpretation; however, it is also the responsibility of the figure author to adopt the most appropriate measure of uncertainty. For instance, standard deviation is based on the spread of the data and therefore shares information about the entire population, including the range in which we might expect new values. On the other hand, standard error is a measure of the uncertainty in the mean (or some other estimate) and is strongly influenced by sample size—namely, standard error decreases with increasing sample size. Confidence intervals are primarily for displaying the reliability of a measurement. Credible intervals, almost exclusively associated with Bayesian methods, are typically built off distributions and have probabilistic interpretations.
Expressing uncertainty is important, but it is also important to interpret the correct message. Krzywinski and Altman 23 directly address a common misconception: “a gap between (error) bars does not ensure significance, nor does overlap rule it out—it depends on the type of bar.” This is a good reminder to be very clear not only in stating what type of uncertainty you are sharing, but what the interpretation is. Others 16 even go so far as to recommend that standard error not be used because it does not provide clear information about standard errors of differences among means. One recommendation to go along with expressing uncertainty is, if possible, to show the data (see Use an Effective Geometry and Show Data ). Particularly when the sample size is low, showing a reader where the data occur can help avoid misinterpretations of uncertainty.
Principle #6 Panel, when Possible (Small Multiples)
A particularly effective visual approach is to repeat a figure to highlight differences. This approach is often called small multiples , 7 and the technique may be referred to as paneling or faceting ( Figure 1 G). The strategy behind small multiples is that because many of the design elements are the same—for example, the axes, axes scales, and geometry are often the same—the differences in the data are easier to show. In other words, each panel represents a change in one variable, which is commonly a time step, a group, or some other factor. The objective of small multiples is to make the data inevitably comparable, 7 and effective small multiples always accomplish these comparisons.
Principle #7 Data and Models Are Different Things
Plotted information typically takes the form of raw data (e.g., scatterplot), summarized data (e.g., box plot), or an inferential statistic (e.g., fitted regression line; Figure 1 D). Raw data and summarized data are often relatively straightforward; however, a plotted model may require more explanation for a reader to be able to fully reproduce the work. Certainly any model in a study should be reported in a complete way that ensures reproducibility. However, any visual of a model should be explained in the figure caption or referenced elsewhere in the document so that a reader can find the complete details on what the model visual is representing. Although it happens, it is not acceptable practice to show a fitted model or other model results in a figure if the reader cannot backtrack the model details. Simply because a model geometry can be added to a figure does not mean that it should be.
Principle #8 Simple Visuals, Detailed Captions
As important as it is to use high data-ink ratios, it is equally important to have detailed captions that fully explain everything in the figure. A study of figures in the Journal of American Medicine 8 found that more than one-third of graphs were not self-explanatory. Captions should be standalone, which means that if the figure and caption were looked at independent from the rest of the study, the major point(s) could still be understood. Obviously not all figures can be completely standalone, as some statistical models and other procedures require more than a caption as explanation. However, the principle remains that captions should do all they can to explain the visualization and representations used. Captions should explain any geometries used; for instance, even in a simple scatterplot it should be stated that the black dots represent the data ( Figures 1 C–1E). Box plots also require descriptions of their geometry—it might be assumed what the features of a box plot are, yet not all box plot symbols are universal.
Principle #9 Consider an Infographic
It is unclear where a figure ends and an infographic begins; however, it is fair to say that figures tend to be focused on representing data and models, whereas infographics typically incorporate text, images, and other diagrammatic elements. Although it is not recommended to convert all figures to infographics, infographics were found 20 to have the highest memorability score and that diagrams outperformed points, bars, lines, and tables in terms of memorability. Scientists might improve their overall information transfer if they consider an infographic where blending different pieces of information could be effective. Also, an infographic of a study might be more effective outside of a peer-reviewed publication and in an oral or poster presentation where a visual needs to include more elements of the study but with less technical information.
Even if infographics are not adopted in most cases, technical visuals often still benefit from some text or other annotations. 16 Tufte's works 7 , 24 provide great examples of bringing together textual, visual, and quantitative information into effective visualizations. However, as figures move in the direction of infographics, it remains important to keep chart junk and other non-essential visual elements out of the design.
Principle #10 Get an Opinion
Although there may be principles and theories about effective data visualization, the reality is that the most effective visuals are the ones with which readers connect. Therefore, figure authors are encouraged to seek external reviews of their figures. So often when writing a study, the figures are quickly made, and even if thoughtfully made they are not subject to objective, outside review. Having one or more colleagues or people external to the study review figures will often provide useful feedback on what readers perceive, and therefore what is effective or ineffective in a visual. It is also recommended to have outside colleagues review only the figures. Not only might this please your colleague reviewers (because figure reviews require substantially less time than full document reviews), but it also allows them to provide feedback purely on the figures as they will not have the document text to fill in any uncertainties left by the visuals.
What About Tables?
Although often not included as data visualization, tables can be a powerful and effective way to show data. Like other visuals, tables are a type of hybrid visual—they typically only include alphanumeric information and no geometries (or other visual elements), so they are not classically a visual. However, tables are also not text in the same way a paragraph or description is text. Rather, tables are often summarized values or information, and are effective if the goal is to reference exact numbers. However, the interest in numerical results in the form of a study typically lies in comparisons and not absolute numbers. Gelman et al. 25 suggested that well-designed graphs were superior to tables. Similarly, Spence and Lewandowsky 26 compared pie charts, bar graphs, and tables and found a clear advantage for graphical displays over tabulations. Because tables are best suited for looking up specific information while graphs are better for perceiving trends and making comparisons and predictions, it is recommended that visuals are used before tables. Despite the reluctance to recommend tables, tables may benefit from digital formats. In other words, while tables may be less effective than figures in many cases, this does not mean tables are ineffective or do not share specific information that cannot always be displayed in a visual. Therefore, it is recommended to consider creating tables as supplementary or appendix information that does not go into the main document (alongside the figures), but which is still very easily accessed electronically for those interested in numerical specifics.
Conclusions
While many of the elements of peer-reviewed literature have remained constant over time, some elements are changing. For example, most articles now have more authors than in previous decades, and a much larger menu of journals creates a diversity of article lengths and other requirements. Despite these changes, the demand for visual representations of data and results remains high, as exemplified by graphical abstracts, overview figures, and infographics. Similarly, we now operate with more software than ever before, creating many choices and opportunities to customize scientific visualizations. However, as the demand for, and software to create, visualizations have both increased, there is not always adequate training among scientists and authors in terms of optimizing the visual for the message.
Figures are not just a scientific side dish but can be a critical point along the scientific process—a point at which the figure maker demonstrates their knowledge and communication of the data and results, and often one of the first stopping points for new readers of the information. The reality for the vast majority of figures is that you need to make your point in a few seconds. The longer someone looks at a figure and doesn't understand the message, the more likely they are to gain nothing from the figure and possibly even lose some understanding of your larger work. Following a set of guidelines and recommendations—summarized here and building on others—can help to build robust visuals that avoid many common pitfalls of ineffective figures ( Figure 2 ).

Overview of the Principles Presented in This Article
The two principles in yellow (bottom) are those that occur first, during the figure design phase. The six principles in green (middle) are generally considerations and decisions while making a figure. The two principles in blue (top) are final steps often considered after a figure has been drafted. While the general flow of the principles follows from bottom to top, there is no specific or required order, and the development of individual figures may require more or less consideration of different principles in a unique order.
All scientists seek to share their message as effectively as possible, and a better understanding of figure design and representation is undoubtedly a step toward better information dissemination and fewer errors in interpretation. Right now, much of the responsibility for effective figures lies with the authors, and learning best practices from literature, workshops, and other resources should be undertaken. Along with authors, journals play a gatekeeper role in figure quality. Journal editorial teams are in a position to adopt recommendations for more effective figures (and reject ineffective figures) and then translate those recommendations into submission requirements. However, due to the qualitative nature of design elements, it is difficult to imagine strict visual guidelines being enforced across scientific sectors. In the absence of such guidelines and with seemingly endless design choices available to figure authors, it remains important that a set of aesthetic criteria emerge to guide the efficient conveyance of visual information.
Acknowledgments
Thanks go to the numerous students with whom I have had fun, creative, and productive conversations about displaying information. Danielle DiIullo was extremely helpful in technical advice on software. Finally, Ron McKernan provided guidance on several principles.
Author Contributions
S.R.M. conceived the review topic, conducted the review, developed the principles, and wrote the manuscript.
Steve Midway is an assistant professor in the Department of Oceanography and Coastal Sciences at Louisiana State University. His work broadly lies in fisheries ecology and how sound science can be applied to management and conservation issues. He teaches a number of quantitative courses in ecology, all of which include data visualization.
Graphical Representation of Data
Graphical representation of data is an attractive method of showcasing numerical data that help in analyzing and representing quantitative data visually. A graph is a kind of a chart where data are plotted as variables across the coordinate. It became easy to analyze the extent of change of one variable based on the change of other variables. Graphical representation of data is done through different mediums such as lines, plots, diagrams, etc. Let us learn more about this interesting concept of graphical representation of data, the different types, and solve a few examples.
1. | |
2. | |
3. | |
4. | |
5. | |
6. | |
7. |
Definition of Graphical Representation of Data
A graphical representation is a visual representation of data statistics-based results using graphs, plots, and charts. This kind of representation is more effective in understanding and comparing data than seen in a tabular form. Graphical representation helps to qualify, sort, and present data in a method that is simple to understand for a larger audience. Graphs enable in studying the cause and effect relationship between two variables through both time series and frequency distribution. The data that is obtained from different surveying is infused into a graphical representation by the use of some symbols, such as lines on a line graph, bars on a bar chart, or slices of a pie chart. This visual representation helps in clarity, comparison, and understanding of numerical data.
Representation of Data
The word data is from the Latin word Datum, which means something given. The numerical figures collected through a survey are called data and can be represented in two forms - tabular form and visual form through graphs. Once the data is collected through constant observations, it is arranged, summarized, and classified to finally represented in the form of a graph. There are two kinds of data - quantitative and qualitative. Quantitative data is more structured, continuous, and discrete with statistical data whereas qualitative is unstructured where the data cannot be analyzed.
Principles of Graphical Representation of Data
The principles of graphical representation are algebraic. In a graph, there are two lines known as Axis or Coordinate axis. These are the X-axis and Y-axis. The horizontal axis is the X-axis and the vertical axis is the Y-axis. They are perpendicular to each other and intersect at O or point of Origin. On the right side of the Origin, the Xaxis has a positive value and on the left side, it has a negative value. In the same way, the upper side of the Origin Y-axis has a positive value where the down one is with a negative value. When -axis and y-axis intersect each other at the origin it divides the plane into four parts which are called Quadrant I, Quadrant II, Quadrant III, Quadrant IV. This form of representation is seen in a frequency distribution that is represented in four methods, namely Histogram, Smoothed frequency graph, Pie diagram or Pie chart, Cumulative or ogive frequency graph, and Frequency Polygon.
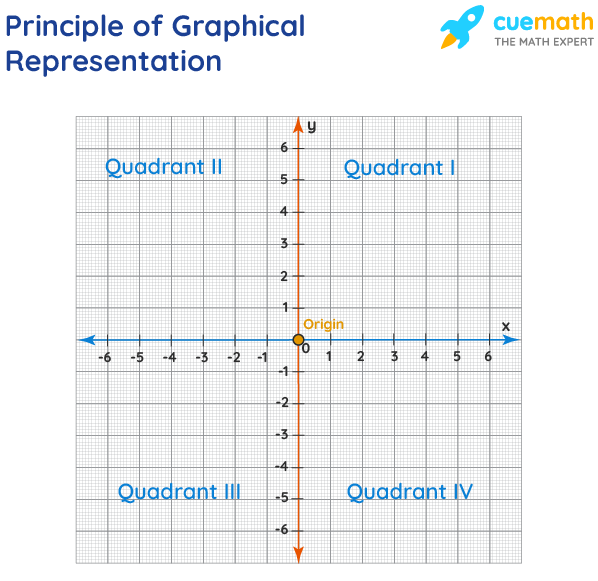
Advantages and Disadvantages of Graphical Representation of Data
Listed below are some advantages and disadvantages of using a graphical representation of data:
- It improves the way of analyzing and learning as the graphical representation makes the data easy to understand.
- It can be used in almost all fields from mathematics to physics to psychology and so on.
- It is easy to understand for its visual impacts.
- It shows the whole and huge data in an instance.
- It is mainly used in statistics to determine the mean, median, and mode for different data
The main disadvantage of graphical representation of data is that it takes a lot of effort as well as resources to find the most appropriate data and then represent it graphically.
Rules of Graphical Representation of Data
While presenting data graphically, there are certain rules that need to be followed. They are listed below:
- Suitable Title: The title of the graph should be appropriate that indicate the subject of the presentation.
- Measurement Unit: The measurement unit in the graph should be mentioned.
- Proper Scale: A proper scale needs to be chosen to represent the data accurately.
- Index: For better understanding, index the appropriate colors, shades, lines, designs in the graphs.
- Data Sources: Data should be included wherever it is necessary at the bottom of the graph.
- Simple: The construction of a graph should be easily understood.
- Neat: The graph should be visually neat in terms of size and font to read the data accurately.
Uses of Graphical Representation of Data
The main use of a graphical representation of data is understanding and identifying the trends and patterns of the data. It helps in analyzing large quantities, comparing two or more data, making predictions, and building a firm decision. The visual display of data also helps in avoiding confusion and overlapping of any information. Graphs like line graphs and bar graphs, display two or more data clearly for easy comparison. This is important in communicating our findings to others and our understanding and analysis of the data.
Types of Graphical Representation of Data
Data is represented in different types of graphs such as plots, pies, diagrams, etc. They are as follows,
Data Representation | Description |
---|---|
A group of data represented with rectangular bars with lengths proportional to the values is a . The bars can either be vertically or horizontally plotted. | |
The is a type of graph in which a circle is divided into Sectors where each sector represents a proportion of the whole. Two main formulas used in pie charts are: | |
The represents the data in a form of series that is connected with a straight line. These series are called markers. | |
Data shown in the form of pictures is a . Pictorial symbols for words, objects, or phrases can be represented with different numbers. | |
The is a type of graph where the diagram consists of rectangles, the area is proportional to the frequency of a variable and the width is equal to the class interval. Here is an example of a histogram. | |
The table in statistics showcases the data in ascending order along with their corresponding frequencies. The frequency of the data is often represented by f. | |
The is a way to represent quantitative data according to frequency ranges or frequency distribution. It is a graph that shows numerical data arranged in order. Each data value is broken into a stem and a leaf. | |
Scatter diagram or is a way of graphical representation by using Cartesian coordinates of two variables. The plot shows the relationship between two variables. |
Related Topics
Listed below are a few interesting topics that are related to the graphical representation of data, take a look.
- x and y graph
- Frequency Polygon
- Cumulative Frequency
Examples on Graphical Representation of Data
Example 1 : A pie chart is divided into 3 parts with the angles measuring as 2x, 8x, and 10x respectively. Find the value of x in degrees.
We know, the sum of all angles in a pie chart would give 360º as result. ⇒ 2x + 8x + 10x = 360º ⇒ 20 x = 360º ⇒ x = 360º/20 ⇒ x = 18º Therefore, the value of x is 18º.
Example 2: Ben is trying to read the plot given below. His teacher has given him stem and leaf plot worksheets. Can you help him answer the questions? i) What is the mode of the plot? ii) What is the mean of the plot? iii) Find the range.
Stem | Leaf |
1 | 2 4 |
2 | 1 5 8 |
3 | 2 4 6 |
5 | 0 3 4 4 |
6 | 2 5 7 |
8 | 3 8 9 |
9 | 1 |
Solution: i) Mode is the number that appears often in the data. Leaf 4 occurs twice on the plot against stem 5.
Hence, mode = 54
ii) The sum of all data values is 12 + 14 + 21 + 25 + 28 + 32 + 34 + 36 + 50 + 53 + 54 + 54 + 62 + 65 + 67 + 83 + 88 + 89 + 91 = 958
To find the mean, we have to divide the sum by the total number of values.
Mean = Sum of all data values ÷ 19 = 958 ÷ 19 = 50.42
iii) Range = the highest value - the lowest value = 91 - 12 = 79
go to slide go to slide
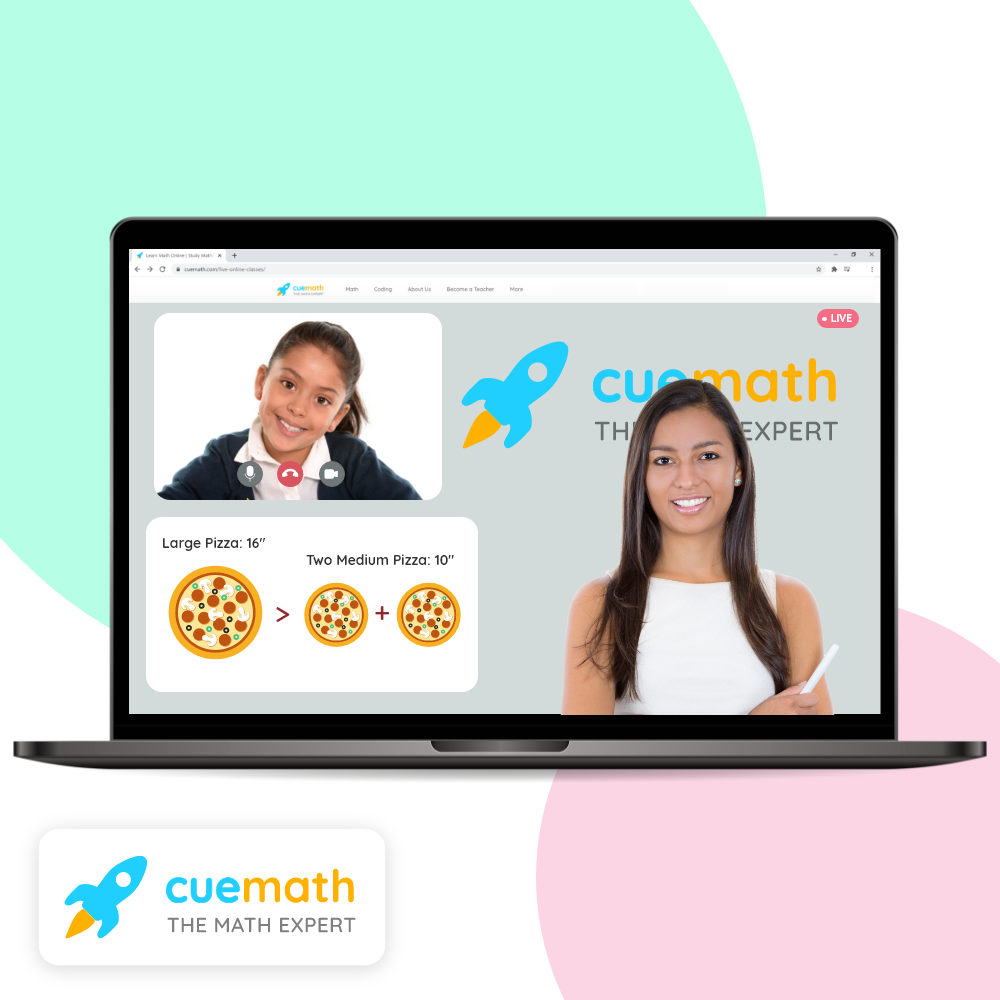
Book a Free Trial Class
Practice Questions on Graphical Representation of Data
Faqs on graphical representation of data, what is graphical representation.
Graphical representation is a form of visually displaying data through various methods like graphs, diagrams, charts, and plots. It helps in sorting, visualizing, and presenting data in a clear manner through different types of graphs. Statistics mainly use graphical representation to show data.
What are the Different Types of Graphical Representation?
The different types of graphical representation of data are:
- Stem and leaf plot
- Scatter diagrams
- Frequency Distribution
Is the Graphical Representation of Numerical Data?
Yes, these graphical representations are numerical data that has been accumulated through various surveys and observations. The method of presenting these numerical data is called a chart. There are different kinds of charts such as a pie chart, bar graph, line graph, etc, that help in clearly showcasing the data.
What is the Use of Graphical Representation of Data?
Graphical representation of data is useful in clarifying, interpreting, and analyzing data plotting points and drawing line segments , surfaces, and other geometric forms or symbols.
What are the Ways to Represent Data?
Tables, charts, and graphs are all ways of representing data, and they can be used for two broad purposes. The first is to support the collection, organization, and analysis of data as part of the process of a scientific study.
What is the Objective of Graphical Representation of Data?
The main objective of representing data graphically is to display information visually that helps in understanding the information efficiently, clearly, and accurately. This is important to communicate the findings as well as analyze the data.
- Back to All Programs /
Data Visualization: Communicating Data and Complex Ideas Visually
Learn how the science of data visualization and the art of graphic design can help you communicate complex information more accurately and effectively.
August 27, 2025
8:30 AM – 4:30 PM ET
2 consecutive days
$3,100 Programs fill quickly — free cancellation up to 14 days prior
Registration Deadline
August 19, 2025
What You'll Learn
Whether you are pitching a new idea, persuading others to take action, building a strategy, or making a decision, data is key. But the amount of information available today is staggering, and it is easy to become overwhelmed by too many statistics, distracted by the wrong set of numbers, and confused by the complexity of data.
This program combines the science of data visualization with the art of graphic design to help you communicate complex information more accurately and effectively. By transforming data sets into visual graphics—such as charts, bar graphs, scatterplots, and heatmaps—you will learn to present complex data in ways your audience can better understand. Through hands-on exercises, you will explore the many types of data in use today, learn how people perceive different graphical displays, and create visual presentations that make a stronger impact on your audience.
Program Benefits
- Critically evaluate visualizations and suggest improvements and refinements
- Avoid creating misleading visual representations of data—and being misled by others
- Apply a structured design process to create effective visualizations
- Conceptualize ideas using sketching and prototyping
- Use principles of human perception and cognition in visualization design
- Communicate more precisely by pinpointing the most relevant information
- Learn how to tell data stories with visualizations
- Earn a Certificate of Completion from the Harvard Division of Continuing Education
Topics Covered
- Creating interactive visualizations using Tableau
- Conceptualizing ideas and interaction techniques using sketching
- Using the visualization design sprint process
- Evaluating strengths and weaknesses of visualization approaches for improvements
- Applying principles of effective visualizations to connect with your audience
Who Should Enroll
This program is ideal for professionals or managers in a wide range of disciplines with an interest in the visual presentation of data.
No programming experience or specific software experience is necessary.
Considering this program?
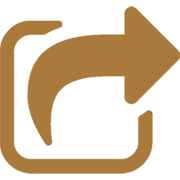
Send yourself the details.
Related Programs
- Foundations of Data-Driven Decision Making with AI
- Digital Marketing Strategy: Data-Driven Strategies for a Modern Marketing Era
- Marketing Analytics Online Course: Strategies for Driving Business Results
August Schedule
- Effective Visualizations
- Sketch and Design
- Storytelling
Hanspeter Pfister
Harvard division of continuing education.
The Division of Continuing Education (DCE) at Harvard University is dedicated to bringing rigorous academics and innovative teaching capabilities to those seeking to improve their lives through education. We make Harvard education accessible to lifelong learners from high school to retirement.
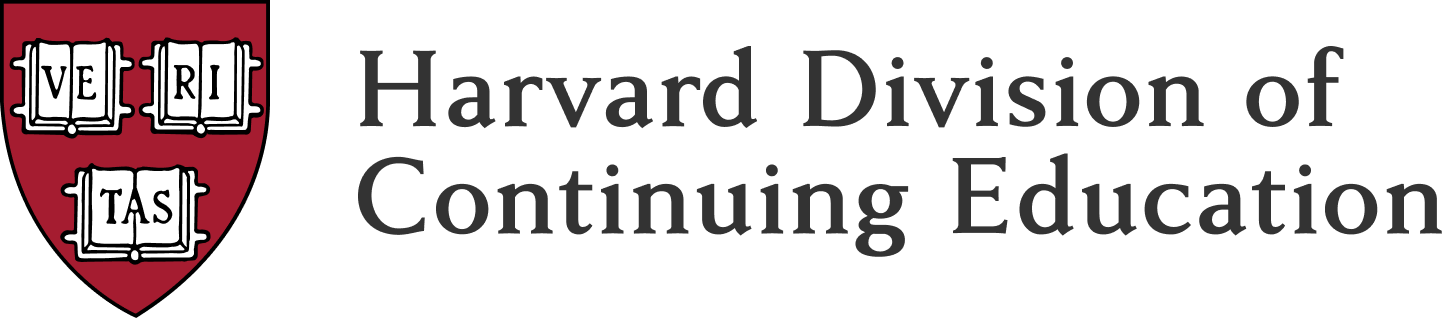
- Data Science
- Data Analysis
- Data Visualization
- Machine Learning
- Deep Learning
- Computer Vision
- Artificial Intelligence
- AI ML DS Interview Series
- AI ML DS Projects series
- Data Engineering
- Web Scrapping
Charts and Graphs for Data Visualization
As companies and groups deal with more and more data, it’s crucial to present it visually. Data is everywhere these days, and it can be overwhelming.
This article is your guide to Data Visualization , which is turning all that data into pictures and charts that are easy to understand. Whether you work in business, marketing, or anything else, these charts can help you explain ideas, track how things are going, and make smart choices.
What is Data Visualization?
Data visualization is taking a bunch of numbers and information and turning it into pictures or any kind of charts that are easier to understand. It takes a big pile of information and sorts it into pictures (like bar charts, line graphs, or pie charts) that make it easier to understand or see patterns and trends. Here are some of the things data visualization can help you see:
- How things are changing over time
- How things compare to each other
- Relationships between things
Different Types of Graphs for Data Visualization
Data can be a jumble of numbers and facts. Charts and graphs turn that jumble into pictures that make sense. 10 prime super useful chart types are:
Bar graphs are one of the most commonly used types of graphs for data visualization. They represent data using rectangular bars where the length of each bar corresponds to the value it represents. Bar graphs are effective for comparing data across different categories or groups.
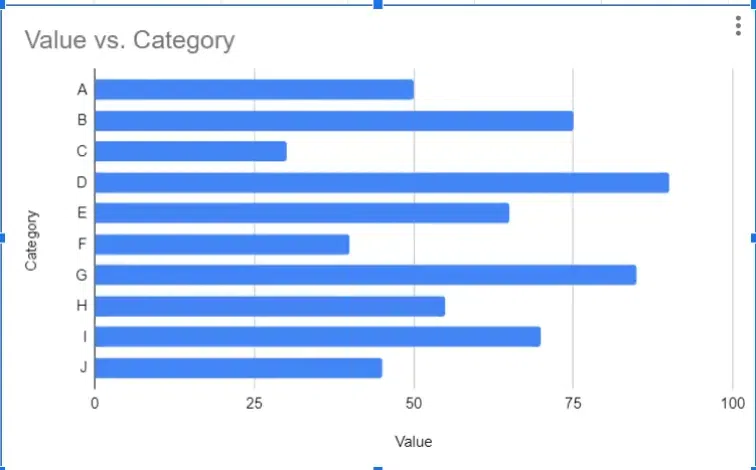
Bar Graph Example
Advantages of Bar Graphs
- Highlighting Trends : Bar graphs are effective at highlighting trends and patterns in data, making it easy for viewers to identify relationships and comparisons between different categories or groups.
- Customizations : Bar graphs can be easily customized to suit specific visualization needs, such as adjusting colors, labels, and styles to enhance clarity and aesthetics.
- Space Efficiency : Bar graphs can efficiently represent large datasets in a compact space, allowing for the visualization of multiple variables or categories without overwhelming the viewer.
Disadvantages of Bar Graphs
- Limited Details : Bar graphs may not provide detailed information about individual data points within each category, limiting the depth of analysis compared to other visualization methods.
- Misleading Scaling : If the scale of the y-axis is manipulated or misrepresented, bar graphs can potentially distort the perception of data and lead to misinterpretation.
- Overcrowding : When too many categories or variables are included in a single bar graph, it can become overcrowded and difficult to read, reducing its effectiveness in conveying clear insights.
Line Graphs
Line graphs are used to display data over time or continuous intervals. They consist of points connected by lines, with each point representing a specific value at a particular time or interval. Line graphs are useful for showing trends and patterns in data. Perfect for showing trends over time, like tracking website traffic or how something changes.
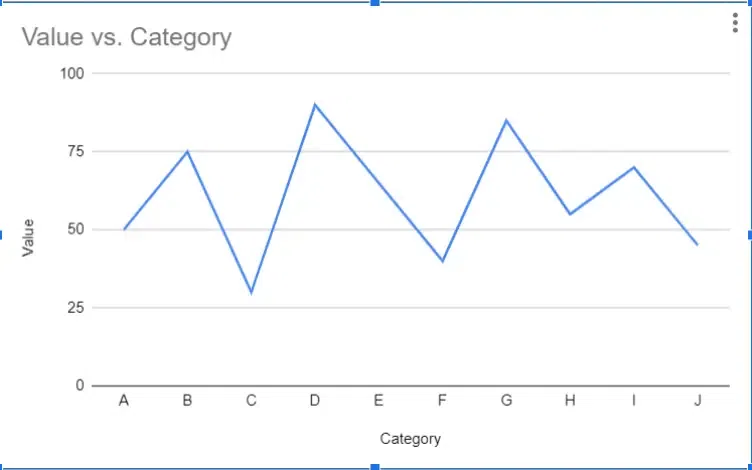
Line Graph Example
Advantages of Line Graphs
- Clarity : Line graphs provide a clear representation of trends and patterns over time or across continuous intervals.
- Visual Appeal : The simplicity and elegance of line graphs make them visually appealing and easy to interpret.
- Comparison : Line graphs allow for easy comparison of multiple data series on the same graph, enabling quick insights into relationships and trends.
Disadvantages of Line Graphs
- Data Simplification: Line graphs may oversimplify complex data sets, potentially obscuring nuances or outliers.
- Limited Representation : Line graphs are most effective for representing continuous data over time or intervals and may not be suitable for all types of data, such as categorical or discrete data.
Different Types of Charts for Data Visualization
Pie charts are circular graphs divided into sectors, where each sector represents a proportion of the whole. The size of each sector corresponds to the percentage or proportion of the total data it represents. Pie charts are effective for showing the composition of a whole and comparing different categories as parts of a whole.
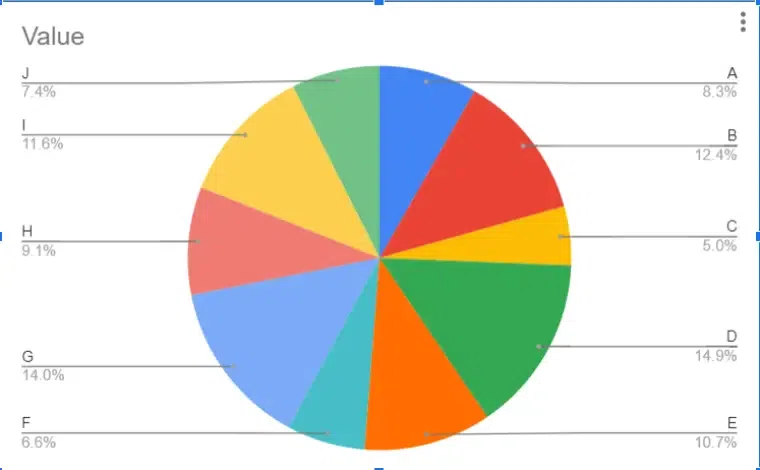
Pie Chart Example
Advantages of Pie Charts
- Easy to create: Pie charts can be quickly generated using various software tools or even by hand, making them accessible for visualizing data without specialized knowledge or skills.
- Visually appealing: The circular shape and vibrant colors of pie charts make them visually appealing, attracting the viewer’s attention and making the data more engaging.
- Simple and easy to understand: Pie charts present data in a straightforward manner, making it easy for viewers to grasp the relative proportions of different categories at a glance.
Disadvantages of Using a Pie Chart
- Limited trend analysis: Pie charts are not ideal for showing trends or changes over time since they represent static snapshots of data at a single point in time.
- Limited data slice: Pie charts become less effective when too many categories are included, as smaller slices can be difficult to distinguish and interpret accurately. They are best suited for representing a few categories with distinct differences in proportions.
Scatter Plots
Scatter plots are used to visualize the relationship between two variables. Each data point in a scatter plot represents a value for both variables, and the position of the point on the graph indicates the values of the variables. Scatter plots are useful for identifying patterns and relationships between variables, such as correlation or trends.
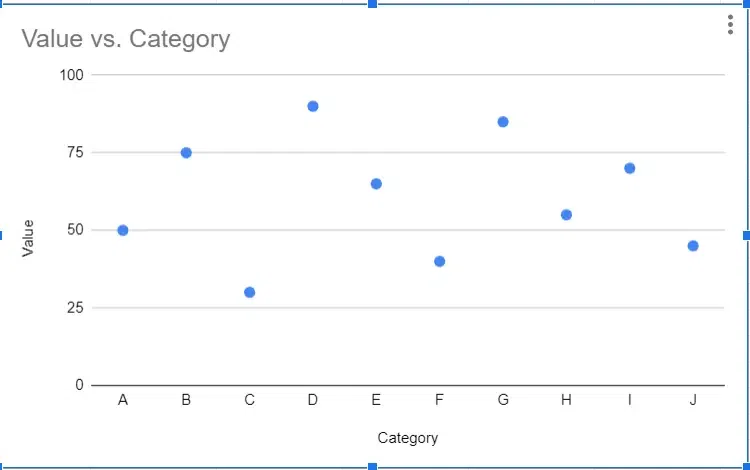
Scatter Chart Example
Advantages of Using Scatter Plots
- Revealing Trends and Relationships: Scatter plots are excellent for visually identifying patterns, trends, and relationships between two variables. They allow for the exploration of correlations and dependencies within the data.
- Easy to Understand: Scatter plots provide a straightforward visual representation of data points, making them easy for viewers to interpret and understand without requiring complex statistical knowledge.
- Highlight Outliers: Scatter plots make it easy to identify outliers or anomalous data points that deviate significantly from the overall pattern. This can be crucial for detecting unusual behavior or data errors within the dataset.
Disadvantages of Using Scatter Plot Charts
- Limited to Two Variables: Scatter plots are limited to visualizing relationships between two variables. While this simplicity can be advantageous for focused analysis, it also means they cannot represent interactions between more than two variables simultaneously.
- Not Ideal for Precise Comparisons: While scatter plots are excellent for identifying trends and relationships, they may not be ideal for making precise comparisons between data points. Other types of graphs, such as bar charts or box plots, may be better suited for comparing specific values or distributions within the data.
Area Charts
Area charts are similar to line graphs but with the area below the line filled in with color. They are used to represent cumulative totals or stacked data over time. Area charts are effective for showing changes in composition over time and comparing the contributions of different categories to the total.
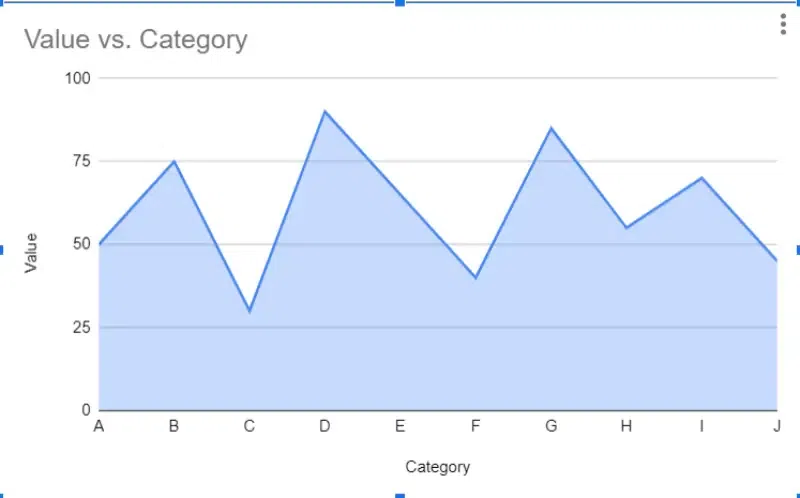
Area Chart Example
Advantages of Using Area Charts
- Visually Appealing: Area charts are aesthetically pleasing and can effectively capture the audience’s attention due to their colorful and filled-in nature.
- Great for Trends: They are excellent for visualizing trends over time, as the filled area under the line emphasizes the magnitude of change, making it easy to identify patterns and fluctuations.
- Compares Well: Area charts allow for easy comparison between different categories or datasets, especially when multiple areas are displayed on the same chart. This comparative aspect aids in highlighting relative changes and proportions.
Disadvantages of Using Area Charts
- Limited Data Sets: Area charts may not be suitable for displaying large or complex datasets, as the filled areas can overlap and obscure details, making it challenging to interpret the data accurately.
- Not for Precise Values: Area charts are less effective for conveying precise numerical values, as the emphasis is on trends and proportions rather than exact measurements. This can be a limitation when precise data accuracy is crucial for analysis or decision-making.
Radar Charts
A radar chart , also known as a spider chart or a web chart, is a graphical method of displaying multivariate data in the form of a two-dimensional chart. It is particularly useful for visualizing the relative values of multiple quantitative variables across several categories. Radar charts compare things across many aspects, like how different employees perform in various skills.
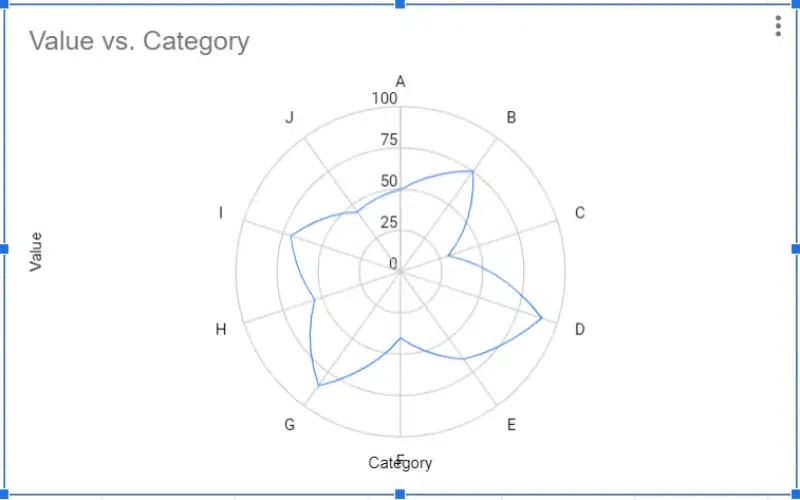
Radar Chart Example
Advantages of Using Radar Chart
- Highlighting Strengths and Weaknesses: Radar charts allow for the clear visualization of strengths and weaknesses across multiple variables, making it easy to identify areas of excellence and areas for improvement.
- Easy Comparisons: The radial nature of radar charts facilitates easy comparison of different variables or categories, as each axis represents a different dimension of the data, enabling quick visual assessment.
- Handling Many Variables: Radar charts are particularly useful for handling datasets with many variables, as each variable can be represented by a separate axis, allowing for comprehensive visualization of multidimensional data.
Disadvantages of Using Radar Chart
- Scaling Issues: Radar charts can present scaling issues, especially when variables have different units or scales. Inaccurate scaling can distort the representation of data, leading to misinterpretation or misunderstanding.
- Misleading Comparisons: Due to the circular nature of radar charts, the area enclosed by each shape can be misleading when comparing variables. Small differences in values can result in disproportionately large visual differences, potentially leading to misinterpretation of data.
Histograms are similar to bar graphs but are used specifically to represent the distribution of continuous data. In histograms, the data is divided into intervals, or bins, and the height of each bar represents the frequency or count of data points within that interval.
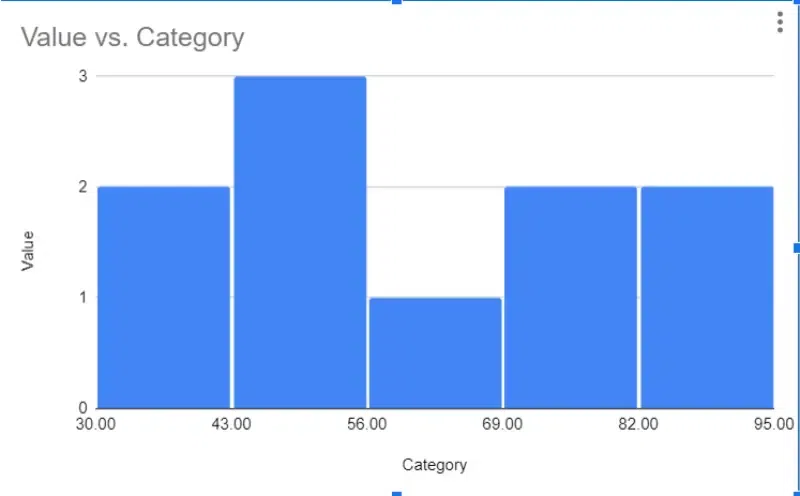
Example of Histogram
Advantages of using Histogram
- Easy to understand: Histograms provide a visual representation of the distribution of data, making it easy for viewers to grasp the overall pattern.
- Identify Patterns: Histograms allow for the identification of patterns and trends within the data, such as skewness, peaks, or gaps.
- Compare Data Sets: Histograms enable comparisons between different datasets, helping to identify similarities or differences in their distributions.
Disadvantages of using Histogram
- Not for small datasets: Histograms may not be suitable for very small datasets as they require a sufficient amount of data to accurately represent the distribution.
- Limited details: Histograms provide a summary of the data distribution but may lack detailed information about individual data points, such as specific values or outliers.
Treemap Charts
Treemap charts are a type of data visualization that represent hierarchical data as a set of nested rectangles. Each rectangle, or “tile,” in the treemap represents a category or subcategory of the data, and the size of the rectangle corresponds to a quantitative value, such as the proportion or absolute value of that category within the dataset.
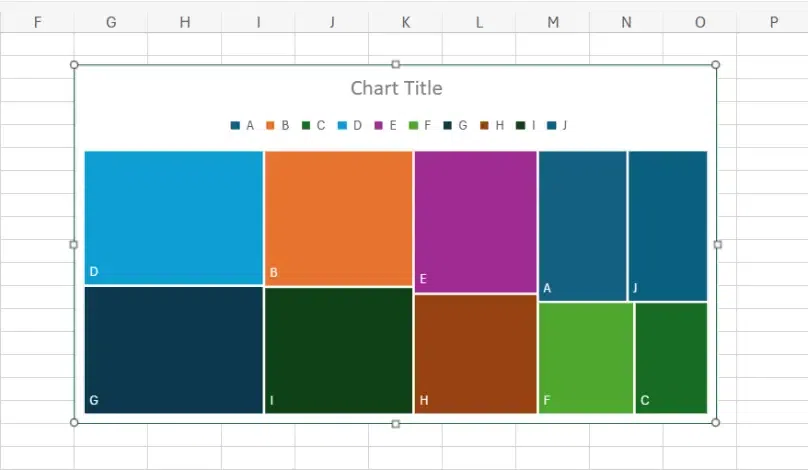
Treemap Charts >>
Advantages of using a Treemap Chart
- Identifying patterns and trends: Treemap charts help in visually identifying patterns and trends within hierarchical data structures by representing data in nested rectangles, making it easier to see how smaller components contribute to the whole.
- Highlighting Proportions: Treemaps effectively highlight proportions by using varying sizes and colors of rectangles to represent different values or categories, making it easy to understand the relative significance of each component.
- Efficient use of space: Treemap charts efficiently utilize space by packing rectangles within larger rectangles, allowing for the visualization of large datasets in a compact and organized manner.
Disadvantages of using a Treemap Chart
- Difficulty comparing exact values: Due to the varying sizes and shapes of the rectangles in a treemap, it can be challenging to accurately compare exact values between different categories or components, especially when the differences are subtle.
- Order dependence: The arrangement of rectangles within a treemap can significantly impact perception. Small changes in sorting or hierarchical structure can lead to different visual interpretations, making it important to carefully consider the ordering of data elements.
Pareto Charts
A Pareto chart is a specific type of chart that combines both bar and line graphs. It’s named after Vilfredo Pareto, an Italian economist who first noted the 80/20 principle, which states that roughly 80% of effects come from 20% of causes. Pareto charts are used to highlight the most significant factors among a set of many factors.
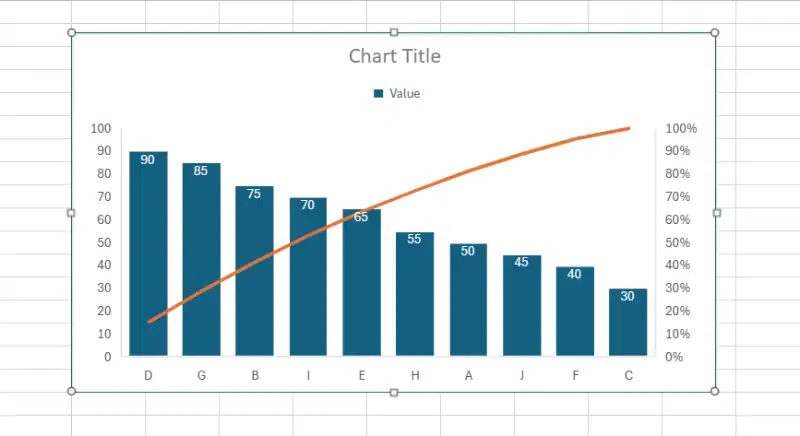
Pareto Chart Example
Advantages of using a Pareto Chart
- Simple to understand: Pareto charts present data in a straightforward manner, making it easy for viewers to grasp the most significant factors at a glance.
- Visually identify key factors: By arranging data in descending order of importance, Pareto charts allow users to quickly identify the most critical factors contributing to a problem or outcome.
- Focus resources effectively: With the ability to prioritize factors based on their impact, Pareto charts help organizations allocate resources efficiently by addressing the most significant issues first.
Disadvantages of Using a Pareto Chart
- Limited Data Exploration: Pareto charts primarily focus on identifying the most critical factors, which may lead to overlooking nuances or subtle trends present in the data.
- Assumes 80/20 rule applies: The Pareto principle, which suggests that roughly 80% of effects come from 20% of causes, is a foundational concept behind Pareto charts. However, this assumption may not always hold true in every situation, potentially leading to misinterpretation or oversimplification of complex data relationships.
Waterfall Charts
Waterfall charts are a type of data visualization tool that display the cumulative effect of sequentially introduced positive or negative values. They are particularly useful for understanding the cumulative impact of different factors contributing to a total or final value.
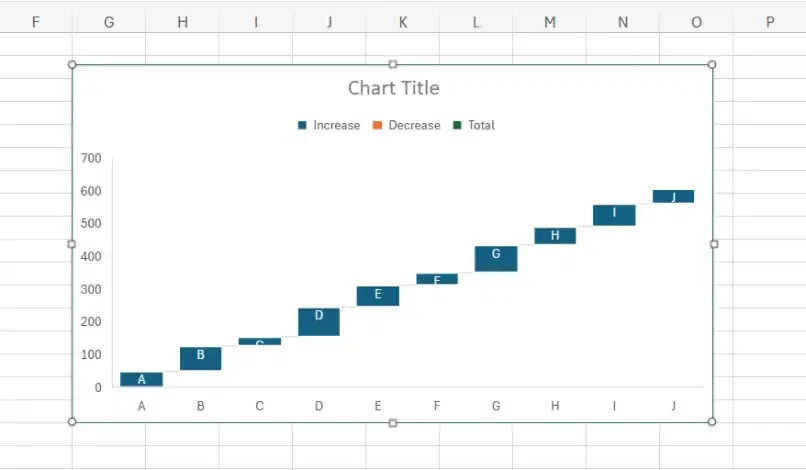
Waterfall Charts Example
Advantages of Using a Waterfall Chart
- Clear Breakdown of Changes: Waterfall charts provide a clear and visual breakdown of changes in data over a series of categories or stages, making it easy to understand the cumulative effect of each change.
- Easy to Identify the Impact: By displaying the incremental additions or subtractions of values, waterfall charts make it easy to identify the impact of each component on the overall total.
- Focus on the Journey: Waterfall charts emphasize the journey of data transformation, showing how values evolve from one stage to another, which can help in understanding the flow of data changes.
Disadvantages of Using a Waterfall Chart
- Complexity with Too Many Categories: Waterfall charts can become complex and cluttered when there are too many categories or stages involved, potentially leading to confusion and difficulty in interpreting the data.
- Not Ideal for Comparisons: While waterfall charts are effective for illustrating changes over a sequence of categories, they may not be suitable for direct comparisons between different datasets or groups, as they primarily focus on showing the cumulative effect of changes rather than individual values.
How to Choose Right Charts or Graphs for Data Visualization?
Choosing the right chart for your data visualization depends on what you want to communicate with your data. Here are some questions provided below to ask yourself before doing Data Visualization,
- How much Data do you have?
- What type of Data are you working with?
- What is the goal of your Visualization?
Also, you can check the general guidelines below to help you pick the right chart type for your reference,
- Distribution of Data
- Relationship between variables
- Comparisons between groups
- Trends over time
- Audience familiarity with different types of Charts and Graphs
The right choice of chart or graph depends on your specific data and the information you want to convey to others. Whether you’re motivating your team, impressing stakeholders, or showcasing your business values, thoughtful data visualization builds trust and drives informed decision-making.
Remember, the key to impactful data visualization lies in choosing the right tool to transform complex data into clear, understanding actionable insights for your audience.
FAQs- Best Types of Charts and Graphs For Data Visualization
Which type of graph is best for data visualization.
The best type of graph depends on the nature of the data. Line graphs are ideal for showing trends over time, bar graphs for comparisons, scatter plots for correlations, and pie charts for proportions.
What type of chart would be best for this visualization?
If you’re comparing categories or groups, a bar chart is often best. It offers a clear visual representation of comparisons between discrete data points.
What are the 4 types of graphs and charts?
Bar Graph Line Graph Pie Chart, and Area Chart
What are the 4 main visualization types?
Spatial, Temporal, Hierarchical, and Network are the 4 main types of visualizations.
Please Login to comment...
Similar reads.
- 105 Funny Things to Do to Make Someone Laugh
- Best PS5 SSDs in 2024: Top Picks for Expanding Your Storage
- Best Nintendo Switch Controllers in 2024
- Xbox Game Pass Ultimate: Features, Benefits, and Pricing in 2024
- #geekstreak2024 – 21 Days POTD Challenge Powered By Deutsche Bank
Improve your Coding Skills with Practice
What kind of Experience do you want to share?

Infographics: Graphic Visual Representations of Information
- April 28, 2020

The Seven Common Types of Infographics
Infographics are graphic visual representations of information, data, or knowledge intended to present information quickly and clearly. Utilizing graphics enhances our ability as humans to recognize patterns and trends and improves cognition. Infographics are made up of three primary elements, the visual, the content, and knowledge. Useful infographics are well designed, they tell a good story, and they are easy to interpret and understand. They combine written words with visual elements to present a significant amount of information in a clear and organized manner.
After completing your research and collecting your data, you need to determine how to demonstrate and communicate your results to your audience clearly. What better way than through an infographic? So, which infographic design will best represent and communicate your information? Below are seven common types of infographics to consider as you think about your infographic design.
List Infographic
The list infographic supports a claim through a series of steps. It is best used to support a specific claim or argument. Your list can go from top to bottom, left to right, or it can move across your canvas. List infographics are one of the most straightforward types of infographics as they engage readers and help them to remember information.

Comparison or Vs. Infographic
The comparison of Vs. Infographic compares two things in a head-to-head study and is typically used to communicate the pros and cons of a product. It is best used to highlight differences between two similar things or highlight similarities between two unlike things. The comparison infographic allows you to display two factors side by side to allow readers to take in all the essential elements and make their comparison in moments. It is also used to prove how one option is superior or inferior to the other option.

Flowchart Infographic
The flowchart infographic provides a specific answer to reader choices and is excellent for showing a process or decision making. It is best used to offer personalized responses to readers, to show how multiple situations can reach the same conclusion, or to display a process flow of your logistic sequence.

Visual Article Infographic
The visual article infographic makes writing more visual. It is best used to cut down on text or make an essay more interesting and enjoyable to consume. It also increases sharing potential as a visual article infographic takes a significant amount of information and presents it visually and enjoyably.

Map Infographic
The map infographic showcases data trends based on location. It is best used to compare places, culture, and people through setting centric data and demographics. A map infographic is used to convey information that is based on location in a visual manner to enhance engagement and comprehension.

Timeline Infographic
The timeline infographic tells a story through a chronological flow. A timeline infographic helps to create a clearer image of a specific timeframe. It is best used to show how something has changed over time or make a long-complicated story easier to understand. These infographics are used to visualize history, highlight essential dates, or display a project timeline.

Data Visualization Infographic
A data visualization infographic communicates data through charts and graphs. It showcases data through design by incorporating pic charts, bar graphs, and line graphs with visual graphics to disclose relevant information. It Is best used to make data-driven arguments easier to understand and makes facts or statistics more enjoyable to absorb.

Infographics are a great tool to communicate information quickly and clearly through graphic visuals, imagery, charts, and minimal texts. They are meant to limit the use of written text by using graphics to engage with your audience and present relevant information visually. Now that you have a basic understanding of the seven commonly used infographics, which type of infographic will you use to represent your information best?

Melina Miller
Marketer Specializing in Digital Marketing, Marketing Analytics, Content Marketing & Personal Branding
- Brand Management
- Consumer Behavior
- Digital Marketing Strategies
- E-Marketing
- Integrated Marketing Communications
- Marketing Analytics
- Marketing Management in a Global Environment
- Marketing Research
- Omnichannel Marketing
- Social Media Marketing

Digital Marketing Interview with Brandon Diaz, Marketing Director

What’s in a (Brand) Name?

Matecumbe Machinery: Brand Strategy Plan

How to Create A Customer Journey Map and Why They Are Important

The Four Types of Marketing Intermediaries
We’d love to hear from You [email protected] 305.942.4559
Got a Project in mind ?
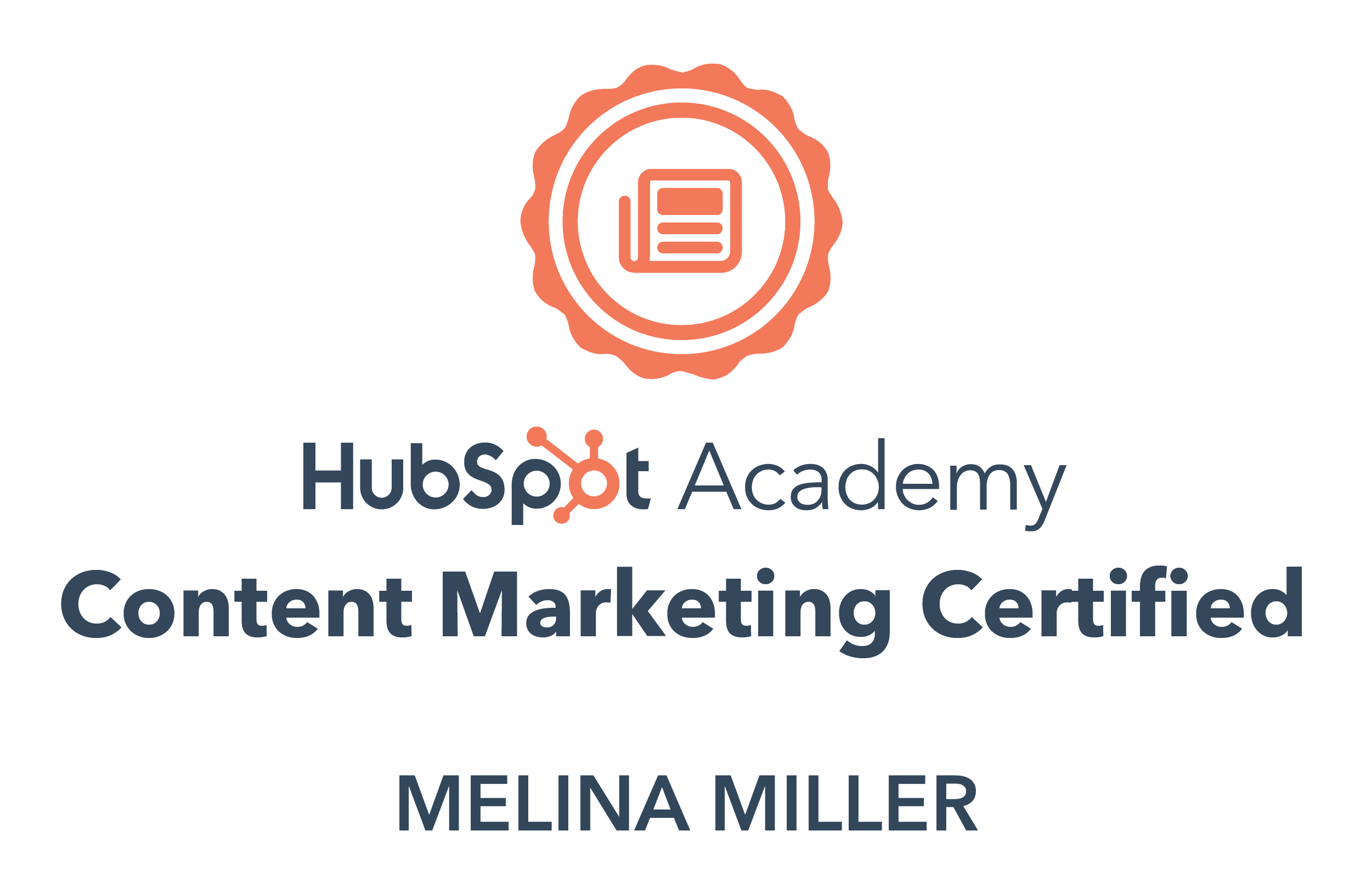
Islamorada, FL
Get Direction
Privacy Policy

Copyright © 2020 Melina K Miller. All rights reserved.
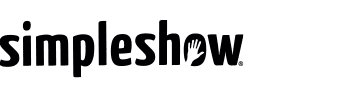
Visualizing complexity: The power of graphics in simplifying difficult concepts
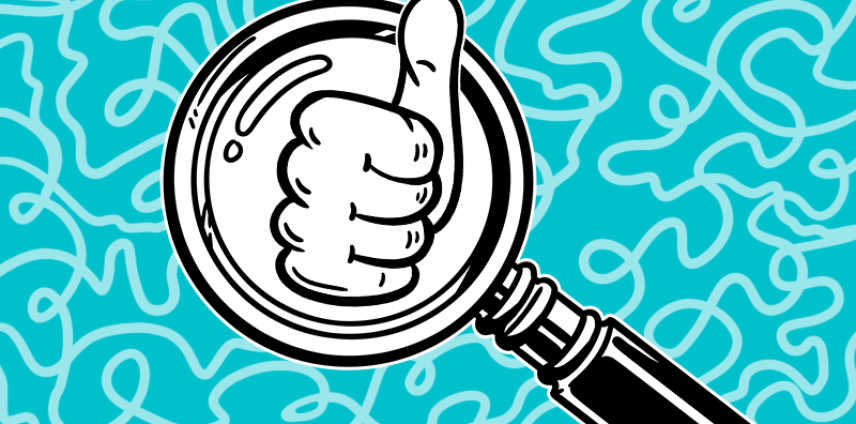
Table of Contents
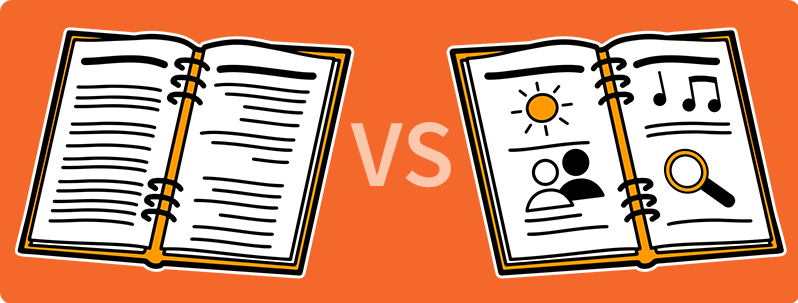
The Power of the Picture
Why visuals work, beyond textbooks, case studies.
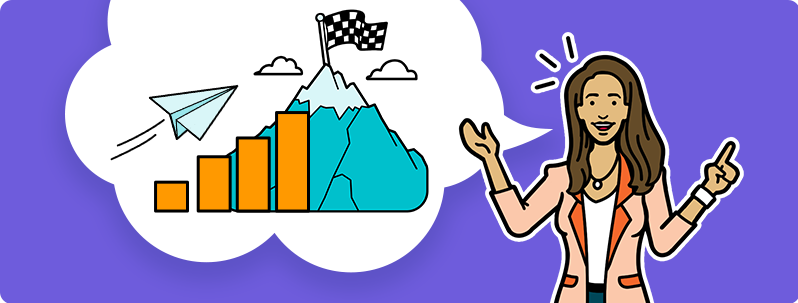
Visual Storytelling
Choosing the right tool, color theory and visual hierarchy.
Storytelling with Graphics
Engagement and action.
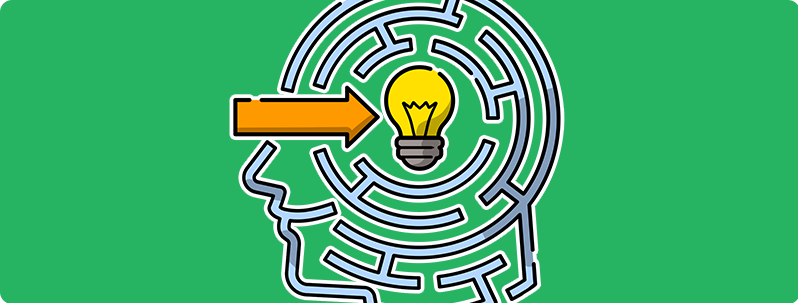
The Nuances of Visual Communication
Avoiding misinterpretation, fact-checking visuals, the art and science.
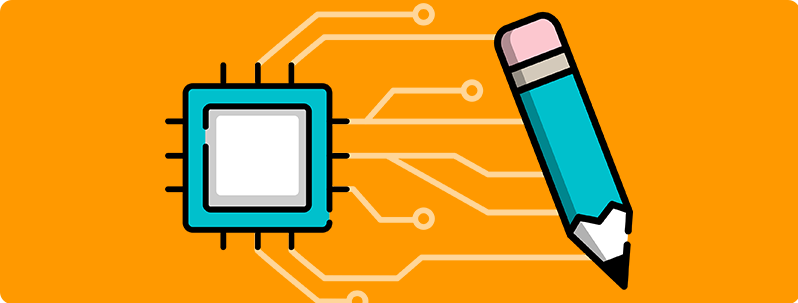
The Future of Visualization
Emerging technologies, engaging the senses, ethical considerations, conclusion: a world illuminated, author's bio.
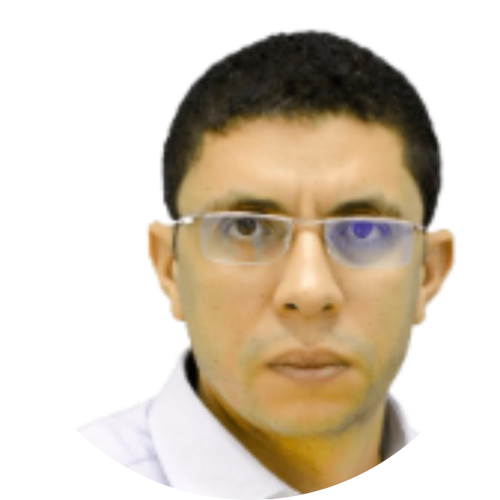
See related articles
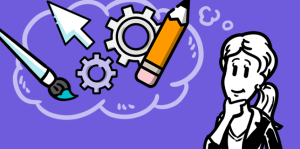
Think like a designer: solving problems with creative design
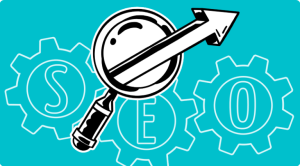
7 effective ways to boost your SEO strategy
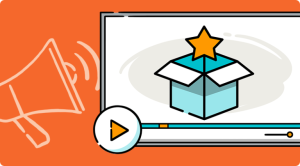
Engage audiences, drive results: Video-centric advertising strategies
Get started with simpleshow today.
Information
- Author Services
Initiatives
You are accessing a machine-readable page. In order to be human-readable, please install an RSS reader.
All articles published by MDPI are made immediately available worldwide under an open access license. No special permission is required to reuse all or part of the article published by MDPI, including figures and tables. For articles published under an open access Creative Common CC BY license, any part of the article may be reused without permission provided that the original article is clearly cited. For more information, please refer to https://www.mdpi.com/openaccess .
Feature papers represent the most advanced research with significant potential for high impact in the field. A Feature Paper should be a substantial original Article that involves several techniques or approaches, provides an outlook for future research directions and describes possible research applications.
Feature papers are submitted upon individual invitation or recommendation by the scientific editors and must receive positive feedback from the reviewers.
Editor’s Choice articles are based on recommendations by the scientific editors of MDPI journals from around the world. Editors select a small number of articles recently published in the journal that they believe will be particularly interesting to readers, or important in the respective research area. The aim is to provide a snapshot of some of the most exciting work published in the various research areas of the journal.
Original Submission Date Received: .
- Active Journals
- Find a Journal
- Proceedings Series
- For Authors
- For Reviewers
- For Editors
- For Librarians
- For Publishers
- For Societies
- For Conference Organizers
- Open Access Policy
- Institutional Open Access Program
- Special Issues Guidelines
- Editorial Process
- Research and Publication Ethics
- Article Processing Charges
- Testimonials
- Preprints.org
- SciProfiles
- Encyclopedia
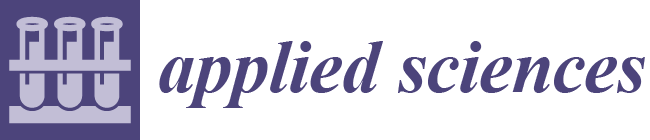
Article Menu

- Subscribe SciFeed
- Recommended Articles
- Author Biographies
- Google Scholar
- on Google Scholar
- Table of Contents
Find support for a specific problem in the support section of our website.
Please let us know what you think of our products and services.
Visit our dedicated information section to learn more about MDPI.
JSmol Viewer
Multimodal emotion recognition using visual, vocal and physiological signals: a review.
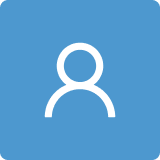
1. Introduction
- RQ1: How can multimodal emotion recognition methods, using visual, vocal and physiological signals be optimized to enhance robustness and accuracy, and what is the impact of deep learning techniques and dynamic expression analysis in overcoming these challenges?
2. Materials and Methods
2.1. search strategy, 2.2. study selection, 3. human emotion categorization models, 4. human emotional expression recognition, 4.1. single modality, 4.1.1. visual modality.
Ref | Year | Database | Features/Classifier | Best Performance |
---|---|---|---|---|
[ ] | 1994 | in-house | Neural network | Acc: to |
[ ] | 2002 | JAFFE | Neural network | Acc: |
[ ] | 2005 | DFAT-504 | Gabor filters + AdaBoost/SVM | Acc: |
[ ] | 2016 | FER2013 + SFEW 2.0 | DNNRL | Acc: |
[ ] | 2017 | CK+ Oulu-CASIA MMI | PHRNN + MSCNN | Acc: Acc: Acc: |
[ ] | 2018 | in-house | Wavelet entropy/Neural network | Acc: |
[ ] | 2018 | KDEF CK+ | CNN/SVM | Acc: Acc: |
[ ] | 2020 | FACES Lifespan CIFE FER2013 | VGG-16/RF | Acc: Acc: Acc: Acc: |
[ ] | 2023 | CK+ FER2013 | CNN + DAISY/RF | Acc: Acc: |
[ ] | 2023 | JAFFE CK+ FER2013 SFEW 2.0 | VGG19 + GoogleNet + ResNet101/SVM | Acc: Acc: Acc: Acc: |
[ ] | 2024 | Aff-Wild2 | Landmarks/GCN | Acc: |
4.1.2. Speech Modality
4.1.3. physiological modality, 4.2. multimodal emotion recognition.
Ref | Year | Multimodal Database | Elicitation | Features | Classifier | Average Accuracy | Fusion Method | Modalities |
---|---|---|---|---|---|---|---|---|
[ ] | 2018 | IEMOCAP, EmotiW | - | BiGRU, attention layer | CNN | Best acc: , WF1 = | Word-level feature-level fusion, CNN | Audio/text |
[ ] | 2015 | eNTERFACE | - | - | (SVM), ELM | Acc: | Feature-level fusion | Audio/facial/ text |
[ ] | 2021 | RAVDESS | Acted | xlsr-Wav2Vec2.0 AUs/ bi-LSTM | - | Acc: | Decision-level fusion using multinomial logistic regression | Audio/facial |
[ ] | 2024 | eNTERFACE’05 | Induced | MobileNetV2 spectrogram/(2D CNN with a federated learning concept) | - | Acc: (subject-dependent) | Decision-level fusion using average probability voting | Audio/facial |
[ ] | 2023 | WESAD CASE k-EmoCon | - | temporal convolution-based modality-specific encoders | FC | Acc: val: , ar: val: , ar: | Feature-level fusion using a transformer | EDA, BVP, TEMP |
[ ] | 2022 | In-house dataset | Induced | CBAM and ResNet34 | MLP | Acc: (subject-dependent) | Data-level fusion | EEG/facial |
[ ] | 2022 | RAVDESS SAVEE | - | ConvLSTM2D and CNN (MFCCs + MS + SC + TZ) CNN | MLP | Acc: Acc: | Feature-level fusion | Audio/video |
[ ] | 2022 | SAVEE RAVDESS RML | - | 2-stream CNN and bi-LSTM (ZC, EN, ENE) CNN | MLP | Acc: Acc: Acc: | Feature-level fusion | Audio/video |
[ ] | 2024 | IEMOCAP (facial/audio) | - | AlexNet with contrastive adversarial learning (facial) MFCC, velocity and acceleration + VGGNet Convolutional autoencoder (teacher), CNN (student) | - | Acc: per emotion state | Adaptive decision-level fusion | Facial/audio |
[ ] | 2023 | ASCERTAIN | Induced | FOX-optimized DDQ | - | Acc: | Optimization-based model fusion | Facial/audio/ GSR |
[ ] | 2023 | RAVDESS CREMA-D | - | 3D CNN with attention mechanism 2D CNN with attention mechanism | - | Acc: Acc: | Cross-attention fusion system (feature-level fusion) | Audio/facial |
[ ] | 2023 | M-LFW-F (facial) and CREMA-D (audio) | - | modified Xception model (spectrogram images extracted from the audio signal) | - | Acc: | Feature-level fusion between entry flow and middle flow | Audio/facial |
[ ] | 2023 | AFEW SFEW MELD AffWild2 | - | VGG19 for face (spectrogram) ResNet50 for audio | - | Acc: Acc: Acc: | Embracenet+, feature-level fusion | Facial/audio |
[ ] | 2024 | In-house dataset | Induced | CNN (EEG topography) CNN | - | Acc: | Decision-level fusion | Facial/EEG |
[ ] | 2024 | FEGE | Acted | 3D-CNN + FC | - | Acc: | model fusion through a shared encoder at feature level and Type-2 fuzzy decision system | Facial/gesture |
5. Deep Learning Challenges and Solutions for High-Quality Emotion Recognition
6. discussion, 7. conclusions, author contributions, institutional review board statement, informed consent statement, data availability statement, conflicts of interest.
- Frijda, N.H. Passions: Emotion and Socially Consequential Behavior. In Emotion: Interdisciplinary Perspectives ; Kavanaugh, R.D., Zimmerberg, B., Fein, S., Eds.; Lawrence Erlbaum Associates, Inc.: Mahwah, NJ, USA, 1996; Volume 1, pp. 1–27. [ Google Scholar ]
- Frijda, N.H. Emotions. In The International Handbook of Psychology ; Pawlik, K., Rosenzweig, M.R., Eds.; Sage Publications: London, UK, 2000; pp. 207–222. [ Google Scholar ]
- Magai, C. Personality theory: Birth, death, and transfiguration. In Emotion: Interdisciplinary Perspectives ; Kavanaugh, R.D., Zimmerberg, B., Fein, S., Eds.; Lawrence Erlbaum Associates, Inc.: Mahwah, NJ, USA, 1996; Volume 1, pp. 171–201. [ Google Scholar ]
- Keltner, D.; Oatley, K.; Jenkins, J.M. Understanding Emotions ; Wiley: Hoboken, NJ, USA, 2014. [ Google Scholar ]
- Scherer, K.R. Emotion. In Introduction to Social Psychology: A European perspective , 3rd ed.; Hewstone, M., Stroebe, W., Eds.; Blackwell Publishing Ltd.: Oxford, UK, 2001; Chapter 6; pp. 151–195. [ Google Scholar ]
- Fredrickson, B.L. The role of positive emotions in positive psychology: The broaden-and-build theory of positive emotions. Am. Psychol. 2001 , 56 , 218–226. [ Google Scholar ] [ CrossRef ]
- Rosenzweig, M.R.; Liang, K.C. Psychology in Biological Perspective. In The International Handbook of Psychology ; Pawlik, K., Rosenzweig, M.R., Eds.; Sage Publications: London, UK, 2000; pp. 54–75. [ Google Scholar ]
- Shuman, V.; Scherer, K.R. Psychological Structure of Emotions. In International Encyclopedia of the Social & Behavioral Sciences ; Wright, J.D., Ed.; Elsevier Ltd.: Waltham, MA, USA, 2015; Volume 7, pp. 526–533. [ Google Scholar ]
- Koolagudi, S.G.; Rao, K.S. Emotion recognition from speech: A review. Int. J. Speech Technol. 2012 , 15 , 99–117. [ Google Scholar ] [ CrossRef ]
- Ko, B.C. A brief review of facial emotion recognition based on visual information. Sensors 2018 , 18 , 401. [ Google Scholar ] [ CrossRef ] [ PubMed ]
- Glowinski, D.; Dael, N.; Camurri, A.; Volpe, G.; Mortillaro, M.; Scherer, K. Toward a minimal representation of affective gestures. IEEE Trans. Affect. Comput. 2011 , 2 , 106–118. [ Google Scholar ] [ CrossRef ]
- Horlings, R.; Datcu, D.; Rothkrantz, L.J. Emotion recognition using brain activity. In Proceedings of the 9th International Conference on Computer Systems and Technologies and Workshop for PhD Students in Computing, Gabrovo, Bulgaria, 12–13 June 2008; p. II–1. [ Google Scholar ]
- Monajati, M.; Abbasi, S.H.; Shabaninia, F.; Shamekhi, S. Emotions states recognition based on physiological parameters by employing of fuzzy-adaptive resonance theory. Int. J. Intell. Sci. 2012 , 2 , 24190. [ Google Scholar ] [ CrossRef ]
- Kim, M.Y.; Bigman, Y.; Tamir, M. Emotional regulation. In International Encyclopedia of the Social & Behavioral Sciences , 2nd ed.; Wright, J.D., Ed.; Elsevier Ltd.: Waltham, MA, USA, 2015; Volume 7, pp. 452–456. [ Google Scholar ]
- Scherer, K.R. What are emotions? And how can they be measured? Soc. Sci. Inf. 2005 , 44 , 695–729. [ Google Scholar ] [ CrossRef ]
- Santhoshkumar, R.; Geetha, M.K. Deep learning approach for emotion recognition from human body movements with feedforward deep convolution neural networks. Procedia Comput. Sci. 2019 , 152 , 158–165. [ Google Scholar ] [ CrossRef ]
- Hassouneh, A.; Mutawa, A.; Murugappan, M. Development of a real-time emotion recognition system using facial expressions and EEG based on machine learning and deep neural network methods. Inform. Med. Unlocked 2020 , 20 , 100372. [ Google Scholar ] [ CrossRef ]
- Shah, M.; Cooper, D.G.; Cao, H.; Gur, R.C.; Nenkova, A.; Verma, R. Action unit models of facial expression of emotion in the presence of speech. In Proceedings of the 2013 Humaine Association Conference on Affective Computing and Intelligent Interaction, Geneva, Switzerland, 2–5 September 2013; IEEE: Piscataway, NJ, USA, 2013; pp. 49–54. [ Google Scholar ]
- Liebrucks, A. The Concept of Social Construction. Theory Psychol. 2001 , 11 , 363–391. [ Google Scholar ] [ CrossRef ]
- Scherer, K.R. Appraisal Theory. In Handbook of Cognition and Emotion ; Dalgleish, T., Power, M.J., Eds.; John Wiley & Sons Ltd.: Chichester, West Sussex, UK, 1999; pp. 637–663. [ Google Scholar ]
- Le Ngo, A.C.; See, J.; Phan, R.C.W. Sparsity in Dynamics of Spontaneous Subtle Emotions: Analysis and Application. IEEE Trans. Affect. Comput. 2017 , 8 , 396–411. [ Google Scholar ] [ CrossRef ]
- Fang, X.; Sauter, D.A.; Van Kleef, G.A. Seeing Mixed Emotions: The Specificity of Emotion Perception From Static and Dynamic Facial Expressions Across Cultures. J. Cross-Cult. Psychol. 2018 , 49 , 130–148. [ Google Scholar ] [ CrossRef ] [ PubMed ]
- Tan, C.B.; Sheppard, E.; Stephen, I.D. A change in strategy: Static emotion recognition in Malaysian Chinese. Cogent Psychol. 2015 , 2 , 1085941. [ Google Scholar ] [ CrossRef ]
- Schmid, P.C.; Schmid Mast, M. Mood effects on emotion recognition. Motiv. Emot. 2010 , 34 , 288–292. [ Google Scholar ] [ CrossRef ]
- Jack, R.E.; Garrod, O.G.; Yu, H.; Caldara, R.; Schyns, P.G. Facial expressions of emotion are not culturally universal. Proc. Natl. Acad. Sci. USA 2012 , 109 , 7241–7244. [ Google Scholar ] [ CrossRef ] [ PubMed ]
- Grainger, S.A.; Henry, J.D.; Phillips, L.H.; Vanman, E.J.; Allen, R. Age deficits in facial affect recognition: The influence of dynamic cues. J. Gerontol. Ser. B: Psychol. Sci. Soc. Sci. 2017 , 72 , 622–632. [ Google Scholar ] [ CrossRef ]
- Martinez, A.M. Visual perception of facial expressions of emotion. Curr. Opin. Psychol. 2017 , 17 , 27–33. [ Google Scholar ] [ CrossRef ]
- Holland, C.A.; Ebner, N.C.; Lin, T.; Samanez-Larkin, G.R. Emotion identification across adulthood using the Dynamic FACES database of emotional expressions in younger, middle aged, and older adults. Cogn. Emot. 2019 , 33 , 245–257. [ Google Scholar ] [ CrossRef ]
- Barrett, L.F.; Adolphs, R.; Marsella, S.; Martinez, A.M.; Pollak, S.D. Emotional expressions reconsidered: Challenges to inferring emotion from human facial movements. Psychol. Sci. Public Interest 2019 , 20 , 1–68. [ Google Scholar ] [ CrossRef ]
- Khosdelazad, S.; Jorna, L.S.; McDonald, S.; Rakers, S.E.; Huitema, R.B.; Buunk, A.M.; Spikman, J.M. Comparing static and dynamic emotion recognition tests: Performance of healthy participants. PLoS ONE 2020 , 15 , e0241297. [ Google Scholar ] [ CrossRef ]
- Krumhuber, E.G.; Kappas, A.; Manstead, A.S. Effects of dynamic aspects of facial expressions: A review. Emot. Rev. 2013 , 5 , 41–46. [ Google Scholar ] [ CrossRef ]
- Kamachi, M.; Bruce, V.; Mukaida, S.; Gyoba, J.; Yoshikawa, S.; Akamatsu, S. Dynamic properties influence the perception of facial expressions. Perception 2013 , 42 , 1266–1278. [ Google Scholar ] [ CrossRef ] [ PubMed ]
- Bassili, J.N. Facial motion in the perception of faces and of emotional expression. J. Exp. Psychol. Hum. Percept. Perform. 1978 , 4 , 373. [ Google Scholar ] [ CrossRef ] [ PubMed ]
- Namba, S.; Kabir, R.S.; Miyatani, M.; Nakao, T. Dynamic displays enhance the ability to discriminate genuine and posed facial expressions of emotion. Front. Psychol. 2018 , 9 , 672. [ Google Scholar ] [ CrossRef ] [ PubMed ]
- Sato, W.; Krumhuber, E.G.; Jellema, T.; Williams, J.H. Dynamic emotional communication. Front. Psychol. 2019 , 10 , 2836. [ Google Scholar ] [ CrossRef ]
- Ghorbanali, A.; Sohrabi, M.K. A comprehensive survey on deep learning-based approaches for multimodal sentiment analysis. Artif. Intell. Rev. 2023 , 56 , 1479–1512. [ Google Scholar ] [ CrossRef ]
- Ahmed, N.; Al Aghbari, Z.; Girija, S. A systematic survey on multimodal emotion recognition using learning algorithms. Intell. Syst. Appl. 2023 , 17 , 200171. [ Google Scholar ] [ CrossRef ]
- Zhang, S.; Yang, Y.; Chen, C.; Zhang, X.; Leng, Q.; Zhao, X. Deep learning-based multimodal emotion recognition from audio, visual, and text modalities: A systematic review of recent advancements and future prospects. Expert Syst. Appl. 2023 , 237 , 121692. [ Google Scholar ] [ CrossRef ]
- Pan, B.; Hirota, K.; Jia, Z.; Dai, Y. A review of multimodal emotion recognition from datasets, preprocessing, features, and fusion methods. Neurocomputing 2023 , 561 , 126866. [ Google Scholar ] [ CrossRef ]
- Gladys, A.A.; Vetriselvi, V. Survey on multimodal approaches to emotion recognition. Neurocomputing 2023 , 556 , 126693. [ Google Scholar ] [ CrossRef ]
- Ezzameli, K.; Mahersia, H. Emotion recognition from unimodal to multimodal analysis: A review. Inf. Fusion 2023 , 99 , 101847. [ Google Scholar ] [ CrossRef ]
- Singh, U.; Abhishek, K.; Azad, H.K. A Survey of Cutting-edge Multimodal Sentiment Analysis. ACM Comput. Surv. 2024 , 56 , 1–38. [ Google Scholar ] [ CrossRef ]
- Hazmoune, S.; Bougamouza, F. Using transformers for multimodal emotion recognition: Taxonomies and state of the art review. Eng. Appl. Artif. Intell. 2024 , 133 , 108339. [ Google Scholar ] [ CrossRef ]
- Liu, H.; Lou, T.; Zhang, Y.; Wu, Y.; Xiao, Y.; Jensen, C.S.; Zhang, D. EEG-based multimodal emotion recognition: A machine learning perspective. IEEE Trans. Instrum. Meas. 2024 , 73 , 4003729. [ Google Scholar ] [ CrossRef ]
- Khan, U.A.; Xu, Q.; Liu, Y.; Lagstedt, A.; Alamäki, A.; Kauttonen, J. Exploring contactless techniques in multimodal emotion recognition: Insights into diverse applications, challenges, solutions, and prospects. Multimed. Syst. 2024 , 30 , 115. [ Google Scholar ] [ CrossRef ]
- Kalateh, S.; Estrada-Jimenez, L.A.; Hojjati, S.N.; Barata, J. A Systematic Review on Multimodal Emotion Recognition: Building Blocks, Current State, Applications, and Challenges. IEEE Access 2024 , 12 , 103976–104019. [ Google Scholar ] [ CrossRef ]
- Poria, S.; Cambria, E.; Bajpai, R.; Hussain, A. A review of affective computing: From unimodal analysis to multimodal fusion. Inf. Fusion 2017 , 37 , 98–125. [ Google Scholar ] [ CrossRef ]
- Kitchenham, B.; Charters, S. Guidelines for Performing Systematic Literature Reviews in Software Engineering ; EBSE Technical Report EBSE-2007-01; Keele University and University of Durham: Keele, UK; Durham, UK, 2007. [ Google Scholar ]
- Bosse, T. On computational models of emotion regulation and their applications within HCI. In Emotions and Affect in Human Factors and Human-Computer Interaction ; Elsevier: Amsterdam, The Netherlands, 2017; pp. 311–337. [ Google Scholar ]
- Scherer, K.R. Psychological Structure of Emotions. In International Encyclopedia of the Social & Behavioral Sciences ; Smelser, N.J., Baltes, P.B., Eds.; Elsevier Ltd.: Amsterdam, The Netherlands, 2001; pp. 4472–4477. [ Google Scholar ] [ CrossRef ]
- Ekman, P. An argument for basic emotions. Cogn. Emot. 1992 , 6 , 169–200. [ Google Scholar ] [ CrossRef ]
- Ekman, P.; Davidson, R.J. (Eds.) The Nature of Emotion: Fundamental Questions ; Oxford University Press: Oxford, UK, 1994. [ Google Scholar ]
- Shaver, P.; Schwartz, J.; Kirson, D.; O’Connor, C. Emotion knowledge: Further exploration of a prototype approach. J. Personal. Soc. Psychol. 1987 , 52 , 1061–1086. [ Google Scholar ] [ CrossRef ]
- Cowen, A.S.; Keltner, D. Self-report captures 27 distinct categories of emotion bridged by continuous gradients. Proc. Natl. Acad. Sci. USA 2017 , 114 , E7900–E7909. [ Google Scholar ] [ CrossRef ]
- Oatley, K.; Johnson-laird, P.N. Towards a Cognitive Theory of Emotions. Cogn. Emot. 1987 , 1 , 29–50. [ Google Scholar ] [ CrossRef ]
- Zeng, Z.; Pantic, M.; Roisman, G.I.; Huang, T.S. A survey of affect recognition methods: Audio, visual, and spontaneous expressions. IEEE Trans. Pattern Anal. Mach. Intell. 2009 , 31 , 39–58. [ Google Scholar ] [ CrossRef ] [ PubMed ]
- Plutchik, R. The nature of emotions: Human emotions have deep evolutionary roots, a fact that may explain their complexity and provide tools for clinical practice. Am. Sci. 2001 , 89 , 344–350. [ Google Scholar ] [ CrossRef ]
- Plutchik, R. A psychoevolutionary theory of emotions. Soc. Sci. Inf. 1982 , 21 , 529–553. [ Google Scholar ] [ CrossRef ]
- Russell, J.A.; Mehrabian, A. Evidence for a three-factor theory of emotions. J. Res. Personal. 1977 , 11 , 273–294. [ Google Scholar ] [ CrossRef ]
- Mehrabian, A. Pleasure-arousal-dominance: A general framework for describing and measuring individual differences in temperament. Curr. Psychol. 1996 , 14 , 261–292. [ Google Scholar ] [ CrossRef ]
- Russell, J.A. A circumplex model of affect. J. Personal. Soc. Psychol. 1980 , 39 , 1161–1178. [ Google Scholar ] [ CrossRef ]
- Whissell, C.M. The dictionary of affect in language. In The Measurement of Emotions ; Elsevier: Amsterdam, The Netherlands, 1989; Chapter 5; pp. 113–131. [ Google Scholar ]
- Ortony, A.; Clore, G.L.; Collins, A. The Cognitive Structure of Emotions ; Cambridge University Press: Cambridge, UK, 1990. [ Google Scholar ]
- Lövheim, H. A new three-dimensional model for emotions and monoamine neurotransmitters. Med. Hypotheses 2012 , 78 , 341–348. [ Google Scholar ] [ CrossRef ]
- Cambria, E.; Livingstone, A.; Hussain, A. The Hourglass of Emotions. In Cognitive Behavioural Systems ; Hutchison, D., Kanade, T., Kittler, J., Kleinberg, J.M., Mattern, F., Mitchell, J.C., Naor, M., Nierstrasz, O., Pandu Rangan, C., Steffen, B., et al., Eds.; Springer: Berlin/Heidelberg, Germany, 2012; Volume 7403, pp. 144–157. [ Google Scholar ] [ CrossRef ]
- Susanto, Y.; Livingstone, A.G.; Ng, B.C.; Cambria, E. The Hourglass Model Revisited. IEEE Intell. Syst. 2020 , 35 , 96–102. [ Google Scholar ] [ CrossRef ]
- Fontaine, J.R.; Scherer, K.R.; Roesch, E.B.; Ellsworth, P.C. The world of emotions is not two-dimensional. Psychol. Sci. 2007 , 18 , 1050–1057. [ Google Scholar ] [ CrossRef ]
- Cochrane, T. Eight dimensions for the emotions. Soc. Sci. Inf. 2009 , 48 , 379–420. [ Google Scholar ] [ CrossRef ]
- Liu, Y.; Fu, Q.; Fu, X. The interaction between cognition and emotion. Chin. Sci. Bull. 2009 , 54 , 4102–4116. [ Google Scholar ] [ CrossRef ]
- Lee, Y.; Seo, Y.; Lee, Y.; Lee, D. Dimensional emotions are represented by distinct topographical brain networks. Int. J. Clin. Health Psychol. 2023 , 23 , 100408. [ Google Scholar ] [ CrossRef ]
- Mauss, I.B.; Robinson, M.D. Measures of emotion: A review. Cogn. Emot. 2009 , 23 , 209–237. [ Google Scholar ] [ CrossRef ] [ PubMed ]
- Kahou, S.E.; Bouthillier, X.; Lamblin, P.; Gulcehre, C.; Michalski, V.; Konda, K.; Jean, S.; Froumenty, P.; Dauphin, Y.; Boulanger-Lewandowski, N.; et al. Emonets: Multimodal deep learning approaches for emotion recognition in video. J. Multimodal User Interfaces 2016 , 10 , 99–111. [ Google Scholar ] [ CrossRef ]
- Davison, A.K.; Merghani, W.; Yap, M.H. Objective Classes for Micro-Facial Expression Recognition. J. Imaging 2018 , 4 , 119. [ Google Scholar ] [ CrossRef ]
- Mehrabian, A. Communication without words. In Communication Theory ; Routledge: London, UK, 2017; Chapter 13; pp. 193–200. [ Google Scholar ]
- Wolfkühler, W.; Majorek, K.; Tas, C.; Küper, C.; Saimed, N.; Juckel, G.; Brüne, M. Emotion recognition in pictures of facial affect: Is there a difference between forensic and non-forensic patients with schizophrenia? Eur. J. Psychiatry 2012 , 26 , 73–85. [ Google Scholar ] [ CrossRef ]
- Yan, W.J.; Wu, Q.; Liang, J.; Chen, Y.H.; Fu, X. How fast are the leaked facial expressions: The duration of micro-expressions. J. Nonverbal Behav. 2013 , 37 , 217–230. [ Google Scholar ] [ CrossRef ]
- Porter, S.; ten Brinke, L. Reading between the lies: Identifying concealed and falsified emotions in universal facial expressions. Psychol. Sci. 2008 , 19 , 508–514. [ Google Scholar ] [ CrossRef ]
- Ekman, P. Darwin, deception, and facial expression. Ann. N. Y. Acad. Sci. 2003 , 1000 , 205–221. [ Google Scholar ] [ CrossRef ]
- Ekman, P. Telling Lies: Clues to Deceit in the Marketplace, Politics, and Marriage ; W.W. Norton & Company Inc.: New York, NY, USA, 2009. [ Google Scholar ]
- Ekman, P. Emotions Revealed: Recognizing Faces and Feelings to Improve Communication and Emotional Life ; Times Books, Henry Holt and Company: New York, NY, USA, 2003. [ Google Scholar ]
- Porter, S.; Ten Brinke, L.; Wallace, B. Secrets and lies: Involuntary leakage in deceptive facial expressions as a function of emotional intensity. J. Nonverbal Behav. 2012 , 36 , 23–37. [ Google Scholar ] [ CrossRef ]
- Frank, M.; Herbasz, M.; Sinuk, K.; Keller, A.; Nolan, C. I see how you feel: Training laypeople and professionals to recognize fleeting emotions. In Proceedings of the Annual Meeting of the International Communication Association, Sheraton New York, New York, NY, USA, 21–25 May 2009; pp. 1–35. [ Google Scholar ]
- Ekman, P.; Freisen, W.V.; Ancoli, S. Facial signs of emotional experience. J. Personal. Soc. Psychol. 1980 , 39 , 1125. [ Google Scholar ] [ CrossRef ]
- Rosenberg, E.L.; Ekman, P. What the Face Reveals: Basic and Applied Studies of Spontaneous Expression Using the Facial Action Coding System (FACS) ; Oxford University Press: Oxford, UK, 2020. [ Google Scholar ]
- Ojala, T.; Pietikainen, M.; Maenpaa, T. Multiresolution gray-scale and rotation invariant texture classification with local binary patterns. IEEE Trans. Pattern Anal. Mach. Intell. 2002 , 24 , 971–987. [ Google Scholar ] [ CrossRef ]
- Ghimire, D.; Lee, J. Geometric feature-based facial expression recognition in image sequences using multi-class Adaboost and support vector machines. Sensors 2013 , 13 , 7714–7734. [ Google Scholar ] [ CrossRef ] [ PubMed ]
- Murugappan, M.; Mutawa, A. Facial geometric feature extraction based emotional expression classification using machine learning algorithms. PLoS ONE 2021 , 16 , e0247131. [ Google Scholar ]
- López-Gil, J.M.; Garay-Vitoria, N. Photogram classification-based emotion recognition. IEEE Access 2021 , 9 , 136974–136984. [ Google Scholar ] [ CrossRef ]
- Rivera, A.R.; Castillo, J.R.; Chae, O.O. Local directional number pattern for face analysis: Face and expression recognition. IEEE Trans. Image Process. 2013 , 22 , 1740–1752. [ Google Scholar ] [ CrossRef ]
- Moore, S.; Bowden, R. Local binary patterns for multi-view facial expression recognition. Comput. Vis. Image Underst. 2011 , 115 , 541–558. [ Google Scholar ] [ CrossRef ]
- Mistry, K.; Zhang, L.; Neoh, S.C.; Jiang, M.; Hossain, A.; Lafon, B. Intelligent Appearance and shape based facial emotion recognition for a humanoid robot. In Proceedings of the 8th International Conference on Software, Knowledge, Information Management and Applications (SKIMA 2014), Dhaka, Bangladesh, 18–20 December 2014; IEEE: Piscataway, NJ, USA, 2014; pp. 1–8. [ Google Scholar ]
- Yang, G.; Ortoneda, J.S.Y.; Saniie, J. Emotion Recognition Using Deep Neural Network with Vectorized Facial Features. In Proceedings of the 2018 IEEE International Conference on Electro/Information Technology (EIT), Rochester, MI, USA, 3–5 May 2018; IEEE: Piscataway, NJ, USA, 2018; pp. 0318–0322. [ Google Scholar ]
- Hai-Duong, N.; Soonja, Y.; Guee-Sang, L.; Hyung-Jeong, Y.; In-Seop, N.; Soo-Hyung, K. Facial Emotion Recognition Using an Ensemble of Multi-Level Convolutional Neural Networks. Int. J. Pattern Recognit. Artif. Intell. 2019 , 33 , 1940015. [ Google Scholar ]
- Agrawal, E.; Christopher, J. Emotion recognition from periocular features. In Proceedings of the Second International Conference on Machine Learning, Image Processing, Network Security and Data Sciences (MIND 2020), Silchar, India, 30–31 July 2020; Springer: Berlin/Heidelberg, Germany, 2020. Part I. pp. 194–208. [ Google Scholar ]
- Dirik, M. Optimized ANFIS model with hybrid metaheuristic algorithms for facial emotion recognition. Int. J. Fuzzy Syst. 2023 , 25 , 485–496. [ Google Scholar ] [ CrossRef ]
- Pfister, T.; Li, X.; Zhao, G.; Pietikäinen, M. Recognising spontaneous facial micro-expressions. In Proceedings of the 2011 International Conference on Computer Vision, Barcelona, Spain, 6–13 November 2011; IEEE: Piscataway, NJ, USA, 2011; pp. 1449–1456. [ Google Scholar ]
- Wang, Y.; See, J.; Phan, R.C.W.; Oh, Y.H. LBP with Six Intersection Points: Reducing Redundant Information in LBP-TOP for Micro-expression Recognition. In Computer Vision—Asian Conference on Computer Vision ACCV 2014 ; Cremers, D., Reid, I., Saito, H., Yang, M.H., Eds.; Springer International Publishing: Cham, Switzerland, 2015; Volume 9003, pp. 525–537. [ Google Scholar ] [ CrossRef ]
- Huang, X.; Wang, S.J.; Zhao, G.; Piteikainen, M. Facial Micro-Expression Recognition Using Spatiotemporal Local Binary Pattern with Integral Projection. In Proceedings of the 2015 IEEE International Conference on Computer Vision Workshop (ICCVW), Santiago, Chile, 7–13 December 2015; pp. 1–9. [ Google Scholar ] [ CrossRef ]
- Wang, Y.; See, J.; Phan, R.C.W.; Oh, Y.H. Efficient spatio-temporal local binary patterns for spontaneous facial micro-expression recognition. PLoS ONE 2015 , 10 , e0124674. [ Google Scholar ]
- Li, X.; Pfister, T.; Huang, X.; Zhao, G.; Pietikäinen, M. A spontaneous micro-expression database: Inducement, collection and baseline. In Proceedings of the 2013 10th IEEE International Conference and Workshops on Automatic Face and Gesture Recognition (FG), Shanghai, China, 22–26 April 2013; IEEE: Piscataway, NJ, USA, 2013; pp. 1–6. [ Google Scholar ]
- Wang, Y.; See, J.; Oh, Y.H.; Phan, R.C.W.; Rahulamathavan, Y.; Ling, H.C.; Tan, S.W.; Li, X. Effective recognition of facial micro-expressions with video motion magnification. Multimed. Tools Appl. 2017 , 76 , 21665–21690. [ Google Scholar ] [ CrossRef ]
- Li, X.; Hong, X.; Moilanen, A.; Huang, X.; Pfister, T.; Zhao, G.; Pietikäinen, M. Towards Reading Hidden Emotions: A Comparative Study of Spontaneous Micro-Expression Spotting and Recognition Methods. IEEE Trans. Affect. Comput. 2018 , 9 , 563–577. [ Google Scholar ] [ CrossRef ]
- Zhao, G.; Pietikainen, M. Dynamic texture recognition using local binary patterns with an application to facial expressions. IEEE Trans. Pattern Anal. Mach. Intell. 2007 , 29 , 915–928. [ Google Scholar ] [ CrossRef ]
- Park, S.Y.; Lee, S.H.; Ro, Y.M. Subtle facial expression recognition using adaptive magnification of discriminative facial motion. In Proceedings of the 23rd ACM International Conference on Multimedia, Brisbane, Australia, 26–30 October 2015; pp. 911–914. [ Google Scholar ]
- Shreve, M.; Godavarthy, S.; Goldgof, D.; Sarkar, S. Macro- and micro-expression spotting in long videos using spatio-temporal strain. In Proceedings of the 2011 IEEE International Conference on Automatic Face & Gesture Recognition (FG), Santa Barbara, CA, USA, 21–25 March 2011; IEEE: Piscataway, NJ, USA, 2011; pp. 51–56. [ Google Scholar ]
- Liong, S.T.; See, J.; Phan, R.C.W.; Le Ngo, A.C.; Oh, Y.H.; Wong, K. Subtle Expression Recognition Using Optical Strain Weighted Features. In Computer Vision—ACCV 2014 Workshops ; Jawahar, C., Shan, S., Eds.; Springer International Publishing: Cham, Switzerland, 2015; Volume 9009, pp. 644–657. [ Google Scholar ] [ CrossRef ]
- Liong, S.T.; See, J.; Phan, R.C.W.; Oh, Y.H.; Le Ngo, A.C.; Wong, K.; Tan, S.W. Spontaneous subtle expression detection and recognition based on facial strain. Signal Process. Image Commun. 2016 , 47 , 170–182. [ Google Scholar ] [ CrossRef ]
- Liong, S.T.; See, J.; Wong, K.; Phan, R.C.W. Less is more: Micro-expression recognition from video using apex frame. Signal Process. Image Commun. 2018 , 62 , 82–92. [ Google Scholar ] [ CrossRef ]
- Xu, F.; Zhang, J.; Wang, J.Z. Microexpression Identification and Categorization Using a Facial Dynamics Map. IEEE Trans. Affect. Comput. 2017 , 8 , 254–267. [ Google Scholar ] [ CrossRef ]
- Zheng, H.; Geng, X.; Yang, Z. A Relaxed K-SVD Algorithm for Spontaneous Micro-Expression Recognition. In PRICAI 2016: Trends in Artificial Intelligence ; Booth, R., Zhang, M.L., Eds.; Springer International Publishing: Cham, Switzerland, 2016; Volume 9810, pp. 692–699. [ Google Scholar ] [ CrossRef ]
- Le Ngo, A.C.; Phan, R.C.W.; See, J. Spontaneous subtle expression recognition: Imbalanced databases and solutions. In Proceedings of the 12th Asian Conference on Computer Vision (ACCV), Singapore, 1–5 November 2014; Springer: Berlin/Heidelberg, Germany, 2014; pp. 33–48. [ Google Scholar ]
- Oh, Y.H.; Le Ngo, A.C.; See, J.; Liong, S.T.; Phan, R.C.W.; Ling, H.C. Monogenic Riesz wavelet representation for micro-expression recognition. In Proceedings of the 2015 IEEE International Conference on Digital Signal Processing (DSP), Singapore, 21–24 July 2015; IEEE: Piscataway, NJ, USA, 2015; pp. 1237–1241. [ Google Scholar ]
- Huang, X.; Wang, S.J.; Liu, X.; Zhao, G.; Feng, X.; Pietikainen, M. Discriminative Spatiotemporal Local Binary Pattern with Revisited Integral Projection for Spontaneous Facial Micro-Expression Recognition. IEEE Trans. Affect. Comput. 2019 , 10 , 32–47. [ Google Scholar ] [ CrossRef ]
- Peng, M.; Wang, C.; Chen, T.; Liu, G.; Fu, X. Dual Temporal Scale Convolutional Neural Network for Micro-Expression Recognition. Front. Psychol. 2017 , 8 , 1745. [ Google Scholar ] [ CrossRef ] [ PubMed ]
- Zhou, L.; Mao, Q.; Xue, L. Cross-database micro-expression recognition: A style aggregated and attention transfer approach. In Proceedings of the 2019 IEEE International Conference on Multimedia & Expo Workshops (ICMEW), Shanghai, China, 8–12 July 2019; IEEE: Piscataway, NJ, USA, 2019; pp. 102–107. [ Google Scholar ]
- Belaiche, R.; Liu, Y.; Migniot, C.; Ginhac, D.; Yang, F. Cost-effective CNNs for real-time micro-expression recognition. Appl. Sci. 2020 , 10 , 4959. [ Google Scholar ] [ CrossRef ]
- Liu, Y.; Du, H.; Zheng, L.; Gedeon, T. A neural micro-expression recognizer. In Proceedings of the 2019 14th IEEE International Conference on Automatic Face & Gesture Recognition (FG 2019), Lille, France, 14–18 May 2019; IEEE: Piscataway, NJ, USA, 2019; pp. 1–4. [ Google Scholar ]
- Avent, R.R.; Ng, C.T.; Neal, J.A. Machine vision recognition of facial affect using backpropagation neural networks. In Proceedings of the 16th Annual International Conference of the IEEE Engineering in Medicine and Biology Society, Baltimore, MD, USA, 3–6 November 1994; IEEE: Piscataway, NJ, USA, 1994; Volume 2, pp. 1364–1365. [ Google Scholar ]
- Gargesha, M.; Kuchi, P.; Torkkola, I. Facial expression recognition using artificial neural networks. Artif. Neural Comput. Syst. 2002 , 8 , 1–6. [ Google Scholar ]
- Bartlett, M.S.; Littlewort, G.; Frank, M.; Lainscsek, C.; Fasel, I.; Movellan, J. Recognizing facial expression: Machine learning and application to spontaneous behavior. In Proceedings of the 2005 IEEE Computer Society Conference on Computer Vision and Pattern Recognition (CVPR’05), San Diego, CA, USA, 20–25 June 2005; IEEE: Piscataway, NJ, USA, 2005; Volume 2, pp. 568–573. [ Google Scholar ]
- Guo, Y.; Tao, D.; Yu, J.; Xiong, H.; Li, Y.; Tao, D. Deep neural networks with relativity learning for facial expression recognition. In Proceedings of the 2016 IEEE International Conference on Multimedia & Expo Workshops (ICMEW), Seattle, WA, USA, 11–15 July 2016; IEEE: Piscataway, NJ, USA, 2016; pp. 1–6. [ Google Scholar ]
- Zhang, K.; Huang, Y.; Du, Y.; Wang, L. Facial expression recognition based on deep evolutional spatial-temporal networks. IEEE Trans. Image Process. 2017 , 26 , 4193–4203. [ Google Scholar ] [ CrossRef ]
- Wang, S.H.; Phillips, P.; Dong, Z.C.; Zhang, Y.D. Intelligent facial emotion recognition based on stationary wavelet entropy and Jaya algorithm. Neurocomputing 2018 , 272 , 668–676. [ Google Scholar ] [ CrossRef ]
- Ruiz-Garcia, A.; Elshaw, M.; Altahhan, A.; Palade, V. A hybrid deep learning neural approach for emotion recognition from facial expressions for socially assistive robots. Neural Comput. Appl. 2018 , 29 , 359–373. [ Google Scholar ] [ CrossRef ]
- Caroppo, A.; Leone, A.; Siciliano, P. Comparison between deep learning models and traditional machine learning approaches for facial expression recognition in ageing adults. J. Comput. Sci. Technol. 2020 , 35 , 1127–1146. [ Google Scholar ] [ CrossRef ]
- Khanbebin, S.N.; Mehrdad, V. Improved convolutional neural network-based approach using hand-crafted features for facial expression recognition. Multimed. Tools Appl. 2023 , 82 , 11489–11505. [ Google Scholar ] [ CrossRef ]
- Boughanem, H.; Ghazouani, H.; Barhoumi, W. Multichannel convolutional neural network for human emotion recognition from in-the-wild facial expressions. Vis. Comput. 2023 , 39 , 5693–5718. [ Google Scholar ] [ CrossRef ]
- Arabian, H.; Abdulbaki Alshirbaji, T.; Chase, J.G.; Moeller, K. Emotion Recognition beyond Pixels: Leveraging Facial Point Landmark Meshes. Appl. Sci. 2024 , 14 , 3358. [ Google Scholar ] [ CrossRef ]
- Kim, D.H.; Baddar, W.J.; Ro, Y.M. Micro-Expression Recognition with Expression-State Constrained Spatio-Temporal Feature Representations. In Proceedings of the 24th ACM International Conference on Multimedia, Amsterdam, The Netherlands, 15–19 October 2016; pp. 382–386. [ Google Scholar ] [ CrossRef ]
- Khor, H.Q.; See, J.; Phan, R.C.W.; Lin, W. Enriched Long-Term Recurrent Convolutional Network for Facial Micro-Expression Recognition. In Proceedings of the 2018 13th IEEE International Conference on Automatic Face & Gesture Recognition (FG 2018), Xi’an, China, 15–19 May 2018; pp. 667–674. [ Google Scholar ] [ CrossRef ]
- Parkhi, O.M.; Vedaldi, A.; Zisserman, A. Deep face recognition. In Proceedings of the BMVC 2015-Proceedings of the British Machine Vision Conference 2015, Swansea, UK, 7–10 September 2015. [ Google Scholar ]
- Zhou, L.; Mao, Q.; Xue, L. Dual-inception network for cross-database micro-expression recognition. In Proceedings of the 2019 14th IEEE International Conference on Automatic Face & Gesture Recognition (FG 2019), Lille, France, 14–18 May 2019; IEEE: Piscataway, NJ, USA, 2019; pp. 1–5. [ Google Scholar ]
- Wang, C.; Peng, M.; Bi, T.; Chen, T. Micro-attention for micro-expression recognition. Neurocomputing 2020 , 410 , 354–362. [ Google Scholar ] [ CrossRef ]
- Gan, Y.S.; Liong, S.T.; Yau, W.C.; Huang, Y.C.; Tan, L.K. OFF-ApexNet on micro-expression recognition system. Signal Process. Image Commun. 2019 , 74 , 129–139. [ Google Scholar ] [ CrossRef ]
- Xia, Z.; Feng, X.; Hong, X.; Zhao, G. Spontaneous facial micro-expression recognition via deep convolutional network. In Proceedings of the 2018 Eighth International Conference on Image Processing Theory, Tools and Applications (IPTA), Xi’an, China, 7–10 November 2018; IEEE: Piscataway, NJ, USA, 2018; pp. 1–6. [ Google Scholar ]
- Liong, S.T.; Gan, Y.S.; See, J.; Khor, H.Q.; Huang, Y.C. Shallow triple stream three-dimensional cnn (ststnet) for micro-expression recognition. In Proceedings of the 2019 14th IEEE International Conference on Automatic Face & Gesture Recognition (FG 2019), Lille, France, 14–18 May 2019; IEEE: Piscataway, NJ, USA, 2019; pp. 1–5. [ Google Scholar ]
- Li, J.; Wang, Y.; See, J.; Liu, W. Micro-expression recognition based on 3D flow convolutional neural network. Pattern Anal. Appl. 2019 , 22 , 1331–1339. [ Google Scholar ] [ CrossRef ]
- Wu, C.; Guo, F. TSNN: Three-Stream Combining 2D and 3D Convolutional Neural Network for Micro-Expression Recognition. IEEJ Trans. Electr. Electron. Eng. 2021 , 16 , 98–107. [ Google Scholar ] [ CrossRef ]
- Peng, M.; Wang, C.; Bi, T.; Shi, Y.; Zhou, X.; Chen, T. A novel apex-time network for cross-dataset micro-expression recognition. In Proceedings of the 2019 8th International Conference on Affective Computing and Intelligent Interaction (ACII), Cambridge, UK, 3–6 September 2019; IEEE: Piscataway, NJ, USA, 2019; pp. 1–6. [ Google Scholar ]
- Van Quang, N.; Chun, J.; Tokuyama, T. CapsuleNet for micro-expression recognition. In Proceedings of the 2019 14th IEEE International Conference on Automatic Face & Gesture Recognition (FG 2019), Lille, France, 14–18 May 2019; IEEE: Piscataway, NJ, USA, 2019; pp. 1–7. [ Google Scholar ]
- Xie, H.X.; Lo, L.; Shuai, H.H.; Cheng, W.H. AU-assisted graph attention convolutional network for micro-expression recognition. In Proceedings of the 28th ACM International Conference on Multimedia, Seattle, WA, USA, 12–16 October 2020; pp. 2871–2880. [ Google Scholar ]
- Polikovsky, S.; Kameda, Y.; Ohta, Y. Facial micro-expressions recognition using high speed camera and 3D-gradient descriptor. In Proceedings of the 3rd International Conference on Imaging for Crime Detection and Prevention (ICDP 2009), London, UK, 3 December 2009. [ Google Scholar ]
- Warren, G.; Schertler, E.; Bull, P. Detecting deception from emotional and unemotional cues. J. Nonverbal Behav. 2009 , 33 , 59–69. [ Google Scholar ] [ CrossRef ]
- Lyons, M.J. “Excavating AI” Re-excavated: Debunking a Fallacious Account of the JAFFE Dataset. arXiv 2021 , arXiv:2107.13998. [ Google Scholar ] [ CrossRef ]
- Yin, L.; Wei, X.; Sun, Y.; Wang, J.; Rosato, M.J. A 3D facial expression database for facial behavior research. In Proceedings of the 7th international conference on automatic face and gesture recognition (FGR06), Southampton, UK, 10–12 April 2006; IEEE: Piscataway, NJ, USA, 2006; pp. 211–216. [ Google Scholar ]
- Li, S.; Deng, W.; Du, J. Reliable crowdsourcing and deep locality-preserving learning for expression recognition in the wild. In Proceedings of the 2017 IEEE Conference on Computer Vision and Pattern Recognition (CVPR), Honolulu, HI, USA, 21–26 July 2017; IEEE: Piscataway, NJ, USA, 2017; pp. 2584–2593. [ Google Scholar ]
- Goeleven, E.; De Raedt, R.; Leyman, L.; Verschuere, B. The Karolinska directed emotional faces: A validation study. Cogn. Emot. 2008 , 22 , 1094–1118. [ Google Scholar ] [ CrossRef ]
- Lucey, P.; Cohn, J.F.; Kanade, T.; Saragih, J.; Ambadar, Z.; Matthews, I. The extended Cohn-Kanade dataset (CK+): A complete dataset for action unit and emotion-specified expression. In Proceedings of the 2010 IEEE Computer Society Conference on Computer Vision and Pattern Recognition-Workshops, San Francisco, CA, USA, 13–18 June 2010; IEEE: Piscataway, NJ, USA, 2010; pp. 94–101. [ Google Scholar ]
- Aifanti, N.; Papachristou, C.; Delopoulos, A. The MUG facial expression database. In Proceedings of the 11th International Workshop on Image Analysis for Multimedia Interactive Services (WIAMIS 10), Desenzano del Garda, Italy, 12–14 April 2010; IEEE: Piscataway, NJ, USA, 2010; pp. 1–4. [ Google Scholar ]
- Chen, L.F.; Yen, Y.S. Taiwanese Facial Expression Image Database ; Brain Mapping Laboratory, Institute of Brain Science, National Yang-Ming University: Taipei, Taiwan, 2007. [ Google Scholar ]
- Langner, O.; Dotsch, R.; Bijlstra, G.; Wigboldus, D.H.; Hawk, S.T.; Van Knippenberg, A. Presentation and validation of the Radboud Faces Database. Cogn. Emot. 2010 , 24 , 1377–1388. [ Google Scholar ] [ CrossRef ]
- Yan, W.J.; Li, X.; Wang, S.J.; Zhao, G.; Liu, Y.J.; Chen, Y.H.; Fu, X. CASME II: An Improved Spontaneous Micro-Expression Database and the Baseline Evaluation. PLoS ONE 2014 , 9 , e86041. [ Google Scholar ] [ CrossRef ]
- Davison, A.K.; Lansley, C.; Costen, N.; Tan, K.; Yap, M.H. SAMM: A Spontaneous Micro-Facial Movement Dataset. IEEE Trans. Affect. Comput. 2018 , 9 , 116–129. [ Google Scholar ] [ CrossRef ]
- Piana, S.; Staglianò, A.; Odone, F.; Verri, A.; Camurri, A. Real-time Automatic Emotion Recognition from Body Gestures. arXiv 2014 , arXiv:1402.5047. [ Google Scholar ]
- Piana, S.; Staglianò, A.; Camurri, A.; Odone, F. A set of full-body movement features for emotion recognition to help children affected by autism spectrum condition. In Proceedings of the IDGEI International Workshop, Chania, Greece, 14 May 2013; Volume 23. [ Google Scholar ]
- Noroozi, F.; Corneanu, C.A.; Kamińska, D.; Sapiński, T.; Escalera, S.; Anbarjafari, G. Survey on emotional body gesture recognition. IEEE Trans. Affect. Comput. 2018 , 12 , 505–523. [ Google Scholar ] [ CrossRef ]
- Zacharatos, H.; Gatzoulis, C.; Chrysanthou, Y.L. Automatic emotion recognition based on body movement analysis: A survey. IEEE Comput. Graph. Appl. 2014 , 34 , 35–45. [ Google Scholar ] [ CrossRef ] [ PubMed ]
- Ly, S.T.; Lee, G.S.; Kim, S.H.; Yang, H.J. Emotion recognition via body gesture: Deep learning model coupled with keyframe selection. In Proceedings of the 2018 International Conference on Machine Learning and Machine Intelligence (MLMI2018), Hanoi, Vietnam, 28–30 September 2018; pp. 27–31. [ Google Scholar ]
- Liu, X.; Shi, H.; Chen, H.; Yu, Z.; Li, X.; Zhao, G. iMiGUE: An identity-free video dataset for micro-gesture understanding and emotion analysis. In Proceedings of the IEEE/CVF Conference on Computer Vision and Pattern Recognition (CVPR), Nashville, TN, USA, 20–25 June 2021; IEEE: Piscataway, NJ, USA, 2021; pp. 10626–10637. [ Google Scholar ]
- Wu, J.; Zhang, Y.; Sun, S.; Li, Q.; Zhao, X. Generalized zero-shot emotion recognition from body gestures. Appl. Intell. 2022 , 52 , 8616–8634. [ Google Scholar ] [ CrossRef ]
- Ekman, P.; Keltner, D. Universal facial expressions of emotion. Calif. Ment. Health Res. Dig. 1970 , 8 , 151–158. [ Google Scholar ]
- Kerkeni, L.; Serrestou, Y.; Mbarki, M.; Raoof, K.; Mahjoub, M.A.; Cleder, C. Automatic speech emotion recognition using machine learning. In Social Media and Machine Learning ; IntechOpen: Rijeka, Croatia, 2019. [ Google Scholar ]
- Murray, I.R.; Arnott, J.L. Toward the simulation of emotion in synthetic speech: A review of the literature on human vocal emotion. J. Acoust. Soc. Am. 1993 , 93 , 1097–1108. [ Google Scholar ] [ CrossRef ]
- Poria, S.; Cambria, E.; Hussain, A.; Huang, G.B. Towards an intelligent framework for multimodal affective data analysis. Neural Netw. 2015 , 63 , 104–116. [ Google Scholar ] [ CrossRef ] [ PubMed ]
- Kamińska, D.; Sapiński, T.; Anbarjafari, G. Efficiency of chosen speech descriptors in relation to emotion recognition. EURASIP J. Audio Speech Music Process. 2017 , 2017 , 3. [ Google Scholar ] [ CrossRef ]
- Vogt, T.; André, E. Comparing feature sets for acted and spontaneous speech in view of automatic emotion recognition. In Proceedings of the 2005 IEEE International Conference on Multimedia and Expo, Amsterdam, The Netherlands, 6 July 2005; IEEE: Piscataway, NJ, USA, 2005; pp. 474–477. [ Google Scholar ]
- Devillers, L.; Vidrascu, L.; Lamel, L. Challenges in real-life emotion annotation and machine learning based detection. Neural Netw. 2005 , 18 , 407–422. [ Google Scholar ] [ CrossRef ]
- Burkhardt, F.; Paeschke, A.; Rolfes, M.; Sendlmeier, W.F.; Weiss, B. A database of German emotional speech. Interspeech 2005 , 5 , 1517–1520. [ Google Scholar ]
- Adigwe, A.; Tits, N.; Haddad, K.E.; Ostadabbas, S.; Dutoit, T. The emotional voices database: Towards controlling the emotion dimension in voice generation systems. arXiv 2018 , arXiv:1806.09514. [ Google Scholar ]
- You, M.; Chen, C.; Bu, J. CHAD: A Chinese affective database. In Proceedings of the International Conference on Affective Computing and Intelligent Interaction, Beijing, China, 22–24 October 2005; Springer: Berlin/Heidelberg, Germany, 2005; pp. 542–549. [ Google Scholar ]
- Palo, H.K.; Mohanty, M.N. Wavelet based feature combination for recognition of emotions. Ain Shams Eng. J. 2018 , 9 , 1799–1806. [ Google Scholar ] [ CrossRef ]
- Kerkeni, L.; Serrestou, Y.; Raoof, K.; Mbarki, M.; Mahjoub, M.A.; Cleder, C. Automatic speech emotion recognition using an optimal combination of features based on EMD-TKEO. Speech Commun. 2019 , 114 , 22–35. [ Google Scholar ] [ CrossRef ]
- Nagarajan, S.; Nettimi, S.S.S.; Kumar, L.S.; Nath, M.K.; Kanhe, A. Speech emotion recognition using cepstral features extracted with novel triangular filter banks based on bark and ERB frequency scales. Digit. Signal Process. 2020 , 104 , 102763. [ Google Scholar ] [ CrossRef ]
- Busso, C.; Bulut, M.; Lee, C.C.; Kazemzadeh, A.; Mower, E.; Kim, S.; Chang, J.N.; Lee, S.; Narayanan, S.S. IEMOCAP: Interactive emotional dyadic motion capture database. Lang. Resour. Eval. 2008 , 42 , 335–359. [ Google Scholar ] [ CrossRef ]
- Snyder, D.; Garcia-Romero, D.; Sell, G.; Povey, D.; Khudanpur, S. X-Vectors: Robust DNN Embeddings for Speaker Recognition. In Proceedings of the 2018 IEEE International Conference on Acoustics, Speech and Signal Processing (ICASSP), Calgary, AB, Canada, 15–20 April 2018; IEEE: Piscataway, NJ, USA, 2018; pp. 5329–5333. [ Google Scholar ] [ CrossRef ]
- Kumawat, P.; Routray, A. Applying TDNN Architectures for Analyzing Duration Dependencies on Speech Emotion Recognition. In Proceedings of the Interspeech 2021 (ISCA), Brno, Czech Republic, 30 August–3 September 2021; pp. 3410–3414. [ Google Scholar ] [ CrossRef ]
- Zhou, S.; Beigi, H. A Transfer Learning Method for Speech Emotion Recognition from Automatic Speech Recognition. arXiv 2020 , arXiv:2008.02863. [ Google Scholar ]
- Morais, E.; Hoory, R.; Zhu, W.; Gat, I.; Damasceno, M.; Aronowitz, H. Speech Emotion Recognition using Self-Supervised Features. arXiv 2022 , arXiv:2202.03896. [ Google Scholar ]
- Ahmed, M.R.; Islam, S.; Islam, A.M.; Shatabda, S. An ensemble 1D-CNN-LSTM-GRU model with data augmentation for speech emotion recognition. Expert Syst. Appl. 2023 , 218 , 119633. [ Google Scholar ] [ CrossRef ]
- Nam, H.J.; Park, H.J. Speech Emotion Recognition under Noisy Environments with SNR Down to- 6 dB Using Multi-Decoder Wave-U-Net. Appl. Sci. 2024 , 14 , 5227. [ Google Scholar ] [ CrossRef ]
- Alkhamali, E.A.; Allinjawi, A.; Ashari, R.B. Combining Transformer, Convolutional Neural Network, and Long Short-Term Memory Architectures: A Novel Ensemble Learning Technique That Leverages Multi-Acoustic Features for Speech Emotion Recognition in Distance Education Classrooms. Appl. Sci. 2024 , 14 , 5050. [ Google Scholar ] [ CrossRef ]
- Sekkate, S.; Khalil, M.; Adib, A. A statistical feature extraction for deep speech emotion recognition in a bilingual scenario. Multimed. Tools Appl. 2023 , 82 , 11443–11460. [ Google Scholar ] [ CrossRef ]
- Huang, Y.; Tian, K.; Wu, A.; Zhang, G. Feature fusion methods research based on deep belief networks for speech emotion recognition under noise condition. J. Ambient Intell. Humaniz. Comput. 2019 , 10 , 1787–1798. [ Google Scholar ] [ CrossRef ]
- Balakrishnan, A.; Rege, A. Reading Emotions from Speech Using Deep Neural Networks ; Technical Report; Computer Science Department, Stanford University: Stanford, CA, USA, 2017. [ Google Scholar ]
- Alu, D.; Zoltan, E.; Stoica, I.C. Voice based emotion recognition with convolutional neural networks for companion robots. Sci. Technol. 2017 , 20 , 222–240. [ Google Scholar ]
- Tzirakis, P.; Zhang, J.; Schuller, B.W. End-to-end speech emotion recognition using deep neural networks. In Proceedings of the 2018 IEEE international conference on acoustics, speech and signal processing (ICASSP), Calgary, AB, Canada, 15–20 April 2018; IEEE: Piscataway, NJ, USA, 2018; pp. 5089–5093. [ Google Scholar ]
- Schuller, B.W. Speech emotion recognition: Two decades in a nutshell, benchmarks, and ongoing trends. Commun. ACM 2018 , 61 , 90–99. [ Google Scholar ] [ CrossRef ]
- Shon, S.; Ali, A.; Glass, J. MIT-QCRI Arabic dialect identification system for the 2017 multi-genre broadcast challenge. In Proceedings of the 2017 IEEE Automatic Speech Recognition and Understanding Workshop (ASRU), Okinawa, Japan, 16–20 December 2017; IEEE: Piscataway, NJ, USA, 2017; pp. 374–380. [ Google Scholar ]
- Shu, L.; Xie, J.; Yang, M.; Li, Z.; Li, Z.; Liao, D.; Xu, X.; Yang, X. A review of emotion recognition using physiological signals. Sensors 2018 , 18 , 2074. [ Google Scholar ] [ CrossRef ] [ PubMed ]
- Wang, X.W.; Nie, D.; Lu, B.L. Emotional state classification from EEG data using machine learning approach. Neurocomputing 2014 , 129 , 94–106. [ Google Scholar ] [ CrossRef ]
- Hosseinifard, B.; Moradi, M.H.; Rostami, R. Classifying depression patients and normal subjects using machine learning techniques and nonlinear features from EEG signal. Comput. Methods Programs Biomed. 2013 , 109 , 339–345. [ Google Scholar ] [ CrossRef ]
- Zhang, Y.; Ji, X.; Zhang, S. An approach to EEG-based emotion recognition using combined feature extraction method. Neurosci. Lett. 2016 , 633 , 152–157. [ Google Scholar ] [ CrossRef ]
- Soroush, M.Z.; Maghooli, K.; Setarehdan, S.K.; Nasrabadi, A.M. A novel method of EEG-based emotion recognition using nonlinear features variability and Dempster–Shafer theory. Biomed. Eng. Appl. Basis Commun. 2018 , 30 , 1850026. [ Google Scholar ] [ CrossRef ]
- Murugappan, M.; Nagarajan, R.; Yaacob, S. Combining spatial filtering and wavelet transform for classifying human emotions using EEG Signals. J. Med. Biol. Eng. 2011 , 31 , 45–51. [ Google Scholar ] [ CrossRef ]
- Jie, X.; Cao, R.; Li, L. Emotion recognition based on the sample entropy of EEG. Bio-Med. Mater. Eng. 2014 , 24 , 1185–1192. [ Google Scholar ] [ CrossRef ]
- Lan, Z.; Sourina, O.; Wang, L.; Liu, Y. Real-time EEG-based emotion monitoring using stable features. Vis. Comput. 2016 , 32 , 347–358. [ Google Scholar ] [ CrossRef ]
- Song, T.; Zheng, W.; Song, P.; Cui, Z. EEG emotion recognition using dynamical graph convolutional neural networks. IEEE Trans. Affect. Comput. 2018 , 11 , 532–541. [ Google Scholar ] [ CrossRef ]
- Gao, Z.; Li, R.; Ma, C.; Rui, L.; Sun, X. Core-brain-network-based multilayer convolutional neural network for emotion recognition. IEEE Trans. Instrum. Meas. 2021 , 70 , 1–9. [ Google Scholar ] [ CrossRef ]
- Yao, L.; Lu, Y.; Wang, M.; Qian, Y.; Li, H. Exploring EEG Emotion Recognition through Complex Networks: Insights from the Visibility Graph of Ordinal Patterns. Appl. Sci. 2024 , 14 , 2636. [ Google Scholar ] [ CrossRef ]
- Álvarez-Jiménez, M.; Calle-Jimenez, T.; Hernández-Álvarez, M. A Comprehensive Evaluation of Features and Simple Machine Learning Algorithms for Electroencephalographic-Based Emotion Recognition. Appl. Sci. 2024 , 14 , 2228. [ Google Scholar ] [ CrossRef ]
- Salama, E.S.; El-Khoribi, R.A.; Shoman, M.E.; Shalaby, M.A.W. EEG-based emotion recognition using 3D convolutional neural networks. Int. J. Adv. Comput. Sci. Appl. 2018 , 9 , 329–337. [ Google Scholar ] [ CrossRef ]
- Kumar, N.; Khaund, K.; Hazarika, S.M. Bispectral analysis of EEG for emotion recognition. Procedia Comput. Sci. 2016 , 84 , 31–35. [ Google Scholar ] [ CrossRef ]
- Quesada Tabares, R.; Molina Cantero, A.J.; Gómez González, I.M.; Merino Monge, M.; Castro García, J.A.; Cabrera Cabrera, R. Emotions Detection based on a Single-electrode EEG Device. In Proceedings of the 4th International Conference on Physiological Computing Systems (PhyCS 2017), Madrid, Spain, 27–28 July 2017; SciTePress: Setubal, Portugal, 2017; pp. 89–95. [ Google Scholar ]
- Van Dyk, D.A.; Meng, X.L. The art of data augmentation. J. Comput. Graph. Stat. 2001 , 10 , 1–50. [ Google Scholar ] [ CrossRef ]
- Khosrowabadi, R.; Quek, C.; Ang, K.K.; Wahab, A. ERNN: A biologically inspired feedforward neural network to discriminate emotion from EEG signal. IEEE Trans. Neural Netw. Learn. Syst. 2013 , 25 , 609–620. [ Google Scholar ] [ CrossRef ]
- Antoniou, A.; Storkey, A.; Edwards, H. Data augmentation generative adversarial networks. arXiv 2017 , arXiv:1711.04340. [ Google Scholar ]
- Pan, Z.; Yu, W.; Wang, B.; Xie, H.; Sheng, V.S.; Lei, J.; Kwong, S. Loss functions of generative adversarial networks (GANs): Opportunities and challenges. IEEE Trans. Emerg. Top. Comput. Intell. 2020 , 4 , 500–522. [ Google Scholar ] [ CrossRef ]
- Harper, R.; Southern, J. End-to-end prediction of emotion from heartbeat data collected by a consumer fitness tracker. In Proceedings of the 2019 8th International Conference on Affective Computing and Intelligent Interaction (ACII), Cambridge, UK, 3–6 September 2019; IEEE: Piscataway, NJ, USA, 2019; pp. 1–7. [ Google Scholar ]
- Sarkar, P.; Etemad, A. Self-supervised learning for ECG-based emotion recognition. In Proceedings of the ICASSP 2020-2020 IEEE International Conference on Acoustics, Speech and Signal Processing (ICASSP), Barcelona, Spain, 4–8 May 2020; IEEE: Piscataway, NJ, USA, 2020; pp. 3217–3221. [ Google Scholar ]
- Li, L.; Chen, J.H. Emotion recognition using physiological signals. In Proceedings of the International Conference on Artificial Reality and Telexistence, Hangzhou, China, 29 November–1 December 2006; Springer: Berlin/Heidelberg, Germany, 2006; pp. 437–446. [ Google Scholar ]
- Lisetti, C.; Nasoz, F.; LeRouge, C.; Ozyer, O.; Alvarez, K. Developing multimodal intelligent affective interfaces for tele-home health care. Int. J. Hum.-Comput. Stud. 2003 , 59 , 245–255. [ Google Scholar ] [ CrossRef ]
- Lisetti, C.L.; Nasoz, F. MAUI: A multimodal affective user interface. In Proceedings of the Tenth ACM International Conference on Multimedia, Juan-les-Pins, France, 1–6 December 2002; pp. 161–170. [ Google Scholar ]
- Shimojo, S.; Shams, L. Sensory modalities are not separate modalities: Plasticity and interactions. Curr. Opin. Neurobiol. 2001 , 11 , 505–509. [ Google Scholar ] [ CrossRef ] [ PubMed ]
- D’mello, S.K.; Kory, J. A review and meta-analysis of multimodal affect detection systems. ACM Comput. Surv. (CSUR) 2015 , 47 , 1–36. [ Google Scholar ] [ CrossRef ]
- Scherer, K.R. Adding the affective dimension: A new look in speech analysis and synthesis. In Proceedings of the ICSLP, Philadelphia, PA, USA, 3–6 October 1996. [ Google Scholar ]
- Lu, Y.; Zheng, W.L.; Li, B.; Lu, B.L. Combining eye movements and EEG to enhance emotion recognition. In Proceedings of the Twenty-Fourth International Joint Conference on Artificial Intelligence, Buenos Aires, Argentina, 25–31 July 2015. [ Google Scholar ]
- Huang, Y.; Yang, J.; Liu, S.; Pan, J. Combining facial expressions and electroencephalography to enhance emotion recognition. Future Internet 2019 , 11 , 105. [ Google Scholar ] [ CrossRef ]
- Van Huynh, T.; Yang, H.J.; Lee, G.S.; Kim, S.H.; Na, I.S. Emotion recognition by integrating eye movement analysis and facial expression model. In Proceedings of the 3rd International Conference on Machine Learning and Soft Computing, Da Lat, Vietnam, 25–28 January 2019; pp. 166–169. [ Google Scholar ]
- Soleymani, M.; Pantic, M.; Pun, T. Multimodal emotion recognition in response to videos. IEEE Trans. Affect. Comput. 2011 , 3 , 211–223. [ Google Scholar ] [ CrossRef ]
- Nguyen, H.D.; Yeom, S.; Oh, I.S.; Kim, K.M.; Kim, S.H. Facial expression recognition using a multi-level convolutional neural network. In Proceedings of the International Conference on Pattern Recognition and Artificial Intelligence, Montréal, Canada, 14–17 May 2018; pp. 217–221. [ Google Scholar ]
- Li, T.H.; Liu, W.; Zheng, W.L.; Lu, B.L. Classification of five emotions from EEG and eye movement signals: Discrimination ability and stability over time. In Proceedings of the 2019 9th International IEEE/EMBS Conference on Neural Engineering (NER), San Francisco, CA, USA, 20–23 March 2019; IEEE: Piscataway, NJ, USA, 2019; pp. 607–610. [ Google Scholar ]
- Zhao, L.M.; Li, R.; Zheng, W.L.; Lu, B.L. Classification of five emotions from EEG and eye movement signals: Complementary representation properties. In Proceedings of the 2019 9th International IEEE/EMBS Conference on Neural Engineering (NER), San Francisco, CA, USA, 20–23 March 2019; IEEE: Piscataway, NJ, USA, 2019; pp. 611–614. [ Google Scholar ]
- Gu, Y.; Yang, K.; Fu, S.; Chen, S.; Li, X.; Marsic, I. Multimodal affective analysis using hierarchical attention strategy with word-level alignment. Proc. Conf. Assoc. Comput. Linguist. Meet. 2018 , 2018 , 2225. [ Google Scholar ]
- Luna-Jiménez, C.; Kleinlein, R.; Griol, D.; Callejas, Z.; Montero, J.M.; Fernández-Martínez, F. A proposal for multimodal emotion recognition using aural transformers and action units on ravdess dataset. Appl. Sci. 2021 , 12 , 327. [ Google Scholar ] [ CrossRef ]
- Simić, N.; Suzić, S.; Milošević, N.; Stanojev, V.; Nosek, T.; Popović, B.; Bajović, D. Enhancing Emotion Recognition through Federated Learning: A Multimodal Approach with Convolutional Neural Networks. Appl. Sci. 2024 , 14 , 1325. [ Google Scholar ] [ CrossRef ]
- Wu, Y.; Daoudi, M.; Amad, A. Transformer-based self-supervised multimodal representation learning for wearable emotion recognition. IEEE Trans. Affect. Comput. 2023 , 15 , 157–172. [ Google Scholar ] [ CrossRef ]
- Li, D.; Liu, J.; Yang, Y.; Hou, F.; Song, H.; Song, Y.; Gao, Q.; Mao, Z. Emotion recognition of subjects with hearing impairment based on fusion of facial expression and EEG topographic map. IEEE Trans. Neural Syst. Rehabil. Eng. 2022 , 31 , 437–445. [ Google Scholar ] [ CrossRef ]
- Middya, A.I.; Nag, B.; Roy, S. Deep learning based multimodal emotion recognition using model-level fusion of audio–visual modalities. Knowl.-Based Syst. 2022 , 244 , 108580. [ Google Scholar ] [ CrossRef ]
- Sharafi, M.; Yazdchi, M.; Rasti, R.; Nasimi, F. A novel spatio-temporal convolutional neural framework for multimodal emotion recognition. Biomed. Signal Process. Control 2022 , 78 , 103970. [ Google Scholar ] [ CrossRef ]
- Kang, D.; Kim, D.; Kang, D.; Kim, T.; Lee, B.; Kim, D.; Song, B.C. Beyond superficial emotion recognition: Modality-adaptive emotion recognition system. Expert Syst. Appl. 2024 , 235 , 121097. [ Google Scholar ] [ CrossRef ]
- Selvi, R.; Vijayakumaran, C. An Efficient Multimodal Emotion Identification Using FOX Optimized Double Deep Q-Learning. Wirel. Pers. Commun. 2023 , 132 , 2387–2406. [ Google Scholar ] [ CrossRef ]
- Mocanu, B.; Tapu, R.; Zaharia, T. Multimodal emotion recognition using cross modal audio-video fusion with attention and deep metric learning. Image Vis. Comput. 2023 , 133 , 104676. [ Google Scholar ] [ CrossRef ]
- Shahzad, H.; Bhatti, S.M.; Jaffar, A.; Rashid, M.; Akram, S. Multi-modal CNN Features Fusion for Emotion Recognition: A Modified Xception Model. IEEE Access 2023 , 11 , 94281–94289. [ Google Scholar ] [ CrossRef ]
- Aguilera, A.; Mellado, D.; Rojas, F. An assessment of in-the-wild datasets for multimodal emotion recognition. Sensors 2023 , 23 , 5184. [ Google Scholar ] [ CrossRef ]
- Roshdy, A.; Karar, A.; Kork, S.A.; Beyrouthy, T.; Nait-ali, A. Advancements in EEG Emotion Recognition: Leveraging Multi-Modal Database Integration. Appl. Sci. 2024 , 14 , 2487. [ Google Scholar ] [ CrossRef ]
- Han, X.; Chen, F.; Ban, J. FMFN: A Fuzzy Multimodal Fusion Network for Emotion Recognition in Ensemble Conducting. IEEE Trans. Fuzzy Syst. 2024 . [ Google Scholar ] [ CrossRef ]
- Wang, Y.; Guan, L.; Venetsanopoulos, A.N. Kernel cross-modal factor analysis for information fusion with application to bimodal emotion recognition. IEEE Trans. Multimed. 2012 , 14 , 597–607. [ Google Scholar ] [ CrossRef ]
- Xie, Z.; Guan, L. Multimodal information fusion of audiovisual emotion recognition using novel information theoretic tools. In Proceedings of the 2013 IEEE International Conference on Multimedia and Expo (ICME), San Jose, CA, USA, 15–19 July 2013; IEEE: Piscataway, NJ, USA, 2013; pp. 1–6. [ Google Scholar ]
- Bota, P.; Wang, C.; Fred, A.; Silva, H. Emotion assessment using feature fusion and decision fusion classification based on physiological data: Are we there yet? Sensors 2020 , 20 , 4723. [ Google Scholar ] [ CrossRef ]
- Arthanarisamy Ramaswamy, M.P.; Palaniswamy, S. Subject independent emotion recognition using EEG and physiological signals—A comparative study. Appl. Comput. Inform. 2022 . [ Google Scholar ] [ CrossRef ]
- Douglas-Cowie, E.; Cowie, R.; Schröder, M. A new emotion database: Considerations, sources and scope. In Proceedings of the ISCA Tutorial and Research Workshop (ITRW) on Speech and Emotion, Newcastle, Northern Ireland, UK, 5–7 September 2000; pp. 39–44. [ Google Scholar ]
- Martin, O.; Kotsia, I.; Macq, B.; Pitas, I. The eNTERFACE’ 05 Audio-Visual Emotion Database. In Proceedings of the 22nd International Conference on Data Engineering Workshops, Atlanta, GA, USA, 3–7 April 2006; p. 8. [ Google Scholar ]
- Douglas-Cowie, E.; Cowie, R.; Sneddon, I.; Cox, C.; Lowry, O.; McRorie, M.; Martin, J.C.; Devillers, L.; Abrilian, S.; Batliner, A.; et al. The HUMAINE Database: Addressing the Collection and Annotation of Naturalistic and Induced Emotional Data. In International Conference on Affective Computing and Intelligent Interaction ; Paiva, A.C.R., Prada, R., Picard, R.W., Eds.; Springer: Berlin/Heidelberg, Germany, 2007; Volume 4738, pp. 488–500. [ Google Scholar ] [ CrossRef ]
- McKeown, G.; Valstar, M.; Cowie, R.; Pantic, M.; Schroder, M. The SEMAINE Database: Annotated Multimodal Records of Emotionally Colored Conversations between a Person and a Limited Agent. IEEE Trans. Affect. Comput. 2012 , 3 , 5–17. [ Google Scholar ] [ CrossRef ]
- Livingstone, S.R.; Russo, F.A. The Ryerson Audio-Visual Database of Emotional Speech and Song (RAVDESS): A dynamic, multimodal set of facial and vocal expressions in North American English. PLoS ONE 2018 , 13 , e0196391. [ Google Scholar ] [ CrossRef ] [ PubMed ]
- Cao, H.; Cooper, D.G.; Keutmann, M.K.; Gur, R.C.; Nenkova, A.; Verma, R. Crema-d: Crowd-sourced emotional multimodal actors dataset. IEEE Trans. Affect. Comput. 2014 , 5 , 377–390. [ Google Scholar ] [ CrossRef ] [ PubMed ]
- Bänziger, T.; Mortillaro, M.; Scherer, K.R. Introducing the Geneva Multimodal expression corpus for experimental research on emotion perception. Emotion 2012 , 12 , 1161. [ Google Scholar ] [ CrossRef ]
- Busso, C.; Parthasarathy, S.; Burmania, A.; AbdelWahab, M.; Sadoughi, N.; Provost, E.M. MSP-IMPROV: An acted corpus of dyadic interactions to study emotion perception. IEEE Trans. Affect. Comput. 2017 , 8 , 67–80. [ Google Scholar ] [ CrossRef ]
- Ngai, W.K.; Xie, H.; Zou, D.; Chou, K.L. Emotion recognition based on convolutional neural networks and heterogeneous bio-signal data sources. Inf. Fusion 2022 , 77 , 107–117. [ Google Scholar ] [ CrossRef ]
- Liu, Y.J.; Zhang, J.K.; Yan, W.J.; Wang, S.J.; Zhao, G.; Fu, X. A main directional mean optical flow feature for spontaneous micro-expression recognition. IEEE Trans. Affect. Comput. 2016 , 7 , 299–310. [ Google Scholar ] [ CrossRef ]
- Xia, Z.; Hong, X.; Gao, X.; Feng, X.; Zhao, G. Spatiotemporal recurrent convolutional networks for recognizing spontaneous micro-expressions. IEEE Trans. Multimed. 2019 , 22 , 626–640. [ Google Scholar ] [ CrossRef ]
- Peng, W.; Hong, X.; Xu, Y.; Zhao, G. A boost in revealing subtle facial expressions: A consolidated eulerian framework. In Proceedings of the 2019 14th IEEE International Conference on Automatic Face & Gesture Recognition (FG 2019), Lille, France, 14–18 May 2019; IEEE: Piscataway, NJ, USA, 2019; pp. 1–5. [ Google Scholar ]
- Sun, Y.; Zheng, L.; Yang, Y.; Tian, Q.; Wang, S. Beyond part models: Person retrieval with refined part pooling (and a strong convolutional baseline). In Proceedings of the 15th European Conference on Computer Vision (ECCV2018), Munich, Germany, 8–14 September 2018; Springer: Berlin/Heidelberg, Germany, 2018; pp. 501–518. [ Google Scholar ]
- Nguyen, H.D.; Yeom, S.; Lee, G.S.; Yang, H.J.; Na, I.S.; Kim, S.H. Facial emotion recognition using an ensemble of multi-level convolutional neural networks. Int. J. Pattern Recognit. Artif. Intell. 2019 , 33 , 1940015. [ Google Scholar ] [ CrossRef ]
- Hu, G.; Liu, L.; Yuan, Y.; Yu, Z.; Hua, Y.; Zhang, Z.; Shen, F.; Shao, L.; Hospedales, T.; Robertson, N.; et al. Deep multi-task learning to recognise subtle facial expressions of mental states. In Proceedings of the 15th European Conference on Computer Vision (ECCV2018), Munich, Germany, 8–14 September 2018; Springer: Berlin/Heidelberg, Germany, 2018; pp. 106–123. [ Google Scholar ]
- Ganin, Y.; Lempitsky, V. Unsupervised domain adaptation by backpropagation. In Proceedings of the 32nd International Conference on Machine Learning (PMLR 37), Lille, France, 7–9 July 2015; Volume 37, pp. 1180–1189. [ Google Scholar ]
- Ganin, Y.; Ustinova, E.; Ajakan, H.; Germain, P.; Larochelle, H.; Laviolette, F.; Marchand, M.; Lempitsky, V. Domain-Adversarial Training of Neural Networks. In Domain Adaptation in Computer Vision Applications ; Csurka, G., Ed.; Springer International Publishing: Cham, Switzerland, 2017; pp. 189–209. [ Google Scholar ] [ CrossRef ]
- Bhattacharya, P.; Gupta, R.K.; Yang, Y. Exploring the contextual factors affecting multimodal emotion recognition in videos. IEEE Trans. Affect. Comput. 2021 , 14 , 1547–1557. [ Google Scholar ] [ CrossRef ]
- Bronstein, M.M.; Bruna, J.; LeCun, Y.; Szlam, A.; Vandergheynst, P. Geometric deep learning: Going beyond euclidean data. IEEE Signal Process. Mag. 2017 , 34 , 18–42. [ Google Scholar ] [ CrossRef ]
- Zheng, J. Geometric Deep Learning with 3D Facial Motion. Master’s Thesis, Imperial College London, London, UK, 2019. [ Google Scholar ]
Click here to enlarge figure
Query Structure: Subexpression 1 AND Subexpression 2 AND Subexpression 3 |
---|
subexpression 1: multimodal OR “multi modal” OR multi-modal OR “multiple modalities” OR multimodality OR multi-modality OR “multiple channels” OR multichannel OR multi-channel OR “multiple sensors” OR multisensor OR multi-sensor OR bimodal OR bi-modal OR bimodality OR bi-modality OR trimodal OR tri-modal OR trimodality OR tri-modality |
subexpression 2: “emotion analysis” OR “emotion recognition” OR “emotion classification” OR “emotion* detection” OR “emotion computing” OR “emotion sensing” OR “emotion assessment” OR “affect recognition” OR “affective computing” OR “emotional state recognition” OR “affective state recognition” |
subexpression 3: visual OR facial OR face OR “body movement*” OR “body motion*” OR gesture* OR posture* OR gesticulation* OR eye OR gaze OR “pupil* dilation” OR “pupil* reflex” OR “pupil* response” OR pupillometry OR pupillogra* OR oculogra* OR lip* OR video* OR audiovisual OR audio-visual OR vocal* OR speech OR audio* OR voice* OR physiological OR biological OR psychophysiological OR biosignal* OR “bio signal*” OR “bio-signal*” OR electroencephalogra* OR eeg OR magnetoencephalogra* OR electrocardiogra* OR ecg OR ekg OR “heart rate” OR “cardiac activit*” OR electromyogra* OR emg OR temg OR “muscle” OR “blood volume” OR bvp OR “blood pressure” OR “blood pulse” OR electrodermal OR eda OR “galvanic skin” OR gsr OR “skin conductance” OR psychogalvanic OR respiration OR accelerometer* OR acceleration* OR electrooculogra* OR eog OR heog OR photoplethysmogra* OR ppg OR “inter-beat interval” OR “interbeat interval” OR “inter beat interval” OR “brain wave*” OR “brain signal*” OR “brain activit*” OR temperature |
Ref | Year | Database | Features/Classifier | Best Performance |
---|---|---|---|---|
[ ] | 2011 | BU3DFE | LGBP+LBP/SVM | Acc: |
[ ] | 2013 | CK CK+ JAFFE MMI CMU-PIE | LDN/SVM | Acc: Acc: Acc: Acc: Acc: |
[ ] | 2013 | CK+ | Geometric/SVM | Acc: |
[ ] | 2014 | CK+ | AAM/NN | Acc: |
[ ] | 2020 | CK+ | AUs/RF | Acc: |
[ ] | 2021 | in-house | ICAT/RF | Acc: |
[ ] | 2021 | CK+ MUG | AUs/Ensemble | Acc: Acc: |
[ ] | 2023 | MUG | AUs and geometric features/ANFIS | Acc: |
Ref | Year | Database | Features/Classifier | Best Performance |
---|---|---|---|---|
[ ] | 2011 | SMIC | LBP-TOP + TIM/MKL | Acc: |
[ ] | 2013 | SMIC-HS | LBP-TOP + TIM/SVM | Acc: |
[ ] | 2014 | SMIC CASME II | STM/AdaBoost | F1: , Acc: F1: , Acc: |
[ ] | 2015 | SMIC CASME II | LBP-SIP/SVM | Acc: Acc: |
[ ] | 2015 | SMIC CASME II | LBP-MOP/SVM | Acc: Acc: |
[ ] | 2015 | SMIC CASME II | STLBP-IP | Acc: Acc: |
[ ] | 2015 | CASME II | Monogenic Riesz Wav./SVM | F1: |
[ ] | 2015 | CASME II | LBP-TOP with adapt. magnification/SVM | Acc: |
[ ] | 2015 | SMIC CASME II | OSW-LBP-TOP/SVM | Acc: Acc: |
[ ] | 2016 | SMIC CASME II | OSF + OSW/SVM | Acc: Acc: |
[ ] | 2016 | CASME CASME II | LBP-TOP/RK-SVD | Acc: Acc: |
[ ] | 2017 | CASME II | LBP-TOP with EVM/SVM | Acc: |
[ ] | 2017 | SMIC CASME II | LBP-TOP with a sparse sampling/SVM | Acc: Acc: 49.00% |
[ ] | 2017 | SMIC-HS CASME CASME II | FDM/SVM | F1: , Acc: F1: , Acc: F1: , Acc: |
[ ] | 2018 | CAS(ME) CASME II SMIC | Bi-WOOF/SVM | F1: , Acc: F1: 0.61 F1: 0.62 |
[ ] | 2018 | SMIC-HS CASME II | HIGO + Magnification/SVM | Acc: 75.00% Acc: 78.14% |
[ ] | 2019 | SMIC CASME CASME II | DiSTLBP-RIP/SVM | Acc: Acc: Acc: |
Ref | Database | Features/Classifier | Best Performance |
---|---|---|---|
2D CNN | |||
[ ] | SMIC, CASME II, and SAMM | EMR | UF1: and UAR: |
[ ] | SMIC, CASME II, and SAMM | Dual-Inception | UF1: and UAR: |
[ ] | SMIC CASME II SAMM | ResNet, Micro-Attention | Acc: Acc: Acc: |
[ ] | SMIC CASME II SAMM | OFF-ApexNet | Acc: Acc: Acc: |
RCNN | |||
[ ] | SMIC CASME CASME II | MER-RCNN | Acc: Acc: Acc: |
3D-CNN | |||
[ ] | CASME I/II | DTSCNN | Acc: |
[ ] | SMIC, CASME II, and SAMM | STSTNet | UF1: and UAR: |
[ ] | SMIC CASME CASME II | 3D-FCNN | Acc: Acc: Acc: |
Combined 2D CNN and 3D CNN | |||
[ ] | SMIC CASME II SAMM + CASME II | TSNN-IF TSNN-LF | F1:0.6631, UAR:0.6566, WAR:0.7547 F1: 0.6921, UAR:0.6833, WAR:0.7632 |
Combined 2D-CNN and LSTM/GRU/RNN | |||
[ ] | SMIC CASME II SAMM | Apex–time network | UF1: and UAR: UF1: and UAR: UF1: and UAR: |
2D-CNN and then LSTM/GRU/RNN | |||
[ ] | CASME II | CNN-LSTM | Acc: |
[ ] | CASME II SAMM | ELRCN | F1 score:0.5 F1 score:0.409 |
Spatial contextual | |||
[ ] | SMIC, CASME II, and SAMM | CapsuleNet | UF1: and UAR: |
[ ] | CASME II SAMM | AU-GACN | Acc: Acc: |
Dataset | No. Samples | No. Characters | Comments |
---|---|---|---|
FER2013 | 35 K (small) [35,887] | 35,887 | Hard: Web/Montreal |
JAFFE [ ] | 213 | 1 actor | Medium |
BU-3DFE [ ] | 2500 | 100 | Video |
RAF-DB [ ] | 30 K | 100 | Medium |
Oulu-CASIA | 30 K | 80 subjects | Medium |
Ferg | 55 K | 6 3D characters | Easy |
KDEF [ ] | 837 | 36 | Image |
SFER | 30 K | - | Subtle |
CK+ [ ] | 327 (593) | 123 subjects | video |
MUG [ ] | 75 K | 476 | Image |
TFEID [ ] | 368 | 4 | Image |
RaFD [ ] | 676 | 18 | Video |
CASME II [ ] | 247 | 26 subjects | Spontaneous subtle: video |
SMIC [ ] | 164 | 16 subjects | Spontaneous subtle |
SAMM [ ] | 159 | 32 subjects | Spontaneous subtle: video |
Ref | Year | Database | Elicitation | Features | Classifier | Average Accuracy |
---|---|---|---|---|---|---|
[ ] | 2018 | FABO | Acted | keyframes HMI + CNN + convLSTM | MLP | |
[ ] | 2019 | GEMEP | Acted | CNN | MLP | |
[ ] | 2021 | iMiGUE (micro-gestures) | Natural | BiLSTM (encoder)/ LSTM (decoder) | BiLSTM (encoder)/ LSTM (decoder) | |
[ ] | 2022 | MASR | Acted | BiLSTM with attention module | HPN/SAE | (seen)/ (unseen) |
Dataset | No. of Emotions | No. of Utterances | Persons | Comments | Difficulty |
---|---|---|---|---|---|
EMO-DB [ ] | 7 | 535 | 10 | Acted | Medium + text |
EVD [ ] | 5 | 9750 | 5 | Acted | Medium |
CHAD [ ] | 7 | 6228 | 42 | Acted | Medium |
Ref | Year | Database | Features | Classifier | Average Accuracy |
---|---|---|---|---|---|
[ ] | 2018 | EMO-DB SAVEE | Combine WLPCCVQ and WMFCCVQ | RBFNN | |
[ ] | 2019 | EMO-DB | AM–FM modulation features (MS, MFF), cepstral features (ECC, EFCC, SMFCC) from THT | SVM | |
[ ] | 2020 | EMO-DB SAVEE | Combine either MFCC, HFCC, TBFCC-B or TBFCC-E | SVM | |
Ref | Year | Database | Features | Classifier | Average Accuracy |
---|---|---|---|---|---|
[ ] | 2008 | Crema-D | CNN | CNN | 60–70% |
[ ] | 2018 | AESDD | LSTM | LSTM | F1 score: 0.6523 |
[ ] | 2021 | LSSED | GRU | GRU | Acc:53.45 |
[ ] | 2020 | ESD | CNN | SVM | Acc:63.78 |
[ ] | 2022 | AESDD | CNN | CNN | |
[ ] | 2023 | TESS EMO-DB RAVDESS SAVEE CREMA-D | Ensemble of 1D CNN, LSTM and GRU | FCN | WAAcc: WAAcc: WAAcc: WAAcc: WAAcc: |
[ ] | 2024 | IEMOCAP NoiseX92 | Wave-U-Net | Wave-U-Net | 62.4% (at 0 dB SNR) |
[ ] | 2024 | EMO-DB RAVDESS SAVEE IEMOCAP SHEIE | Ensemble of Transformer, CNN and LSTM | FCN | |
[ ] | 2023 | RAVDESS EMOVO | Statistical mean of MFCCs | 3-1D CNN | Acc: 87.08 Acc:83.90 (speaker-dependent) |
[ ] | 2019 | EMO-DB | Feature fusion by DBNs: prosody features (fundamental frequency, power), voice quality features (the first, second and third formants with their bandwidths), spectral features (WPCC and W-WPCC) | DBNs/SVM | Acc: |
[ ] | 2019 | EMO-DB | AM–FM modulation features (MS, MFF), cepstral features (ECC, EFCC, SMFCC) from THT | RNN | Acc: |
Ref | Year | Database | Elicitation | Features | Classifier | Accuracy |
---|---|---|---|---|---|---|
[ ] | 2014 | GAMEEMO | Acted | PS wavelet ENT HE FD | SVM | |
[ ] | 2014 | DEAP | Induced | Sample entropy | SVM | val: , ar: |
[ ] | 2016 | DEAP | Induced | Sample entropy of IMFs | SVM | |
[ ] | 2016 | In-house dataset induced by IADS | Induced | FD, 5 statistics and 4 band powers ( , , , ratio) | SVM | |
[ ] | 2011 | in-house dataset induced by audio-visual stimuli | Induced | Wavelet | kNN LDA | |
[ ] | 2018 | DEAP | Induced | Entropy from temporal window | MLPs combined through DST | ar: , val: |
[ ] | 2018 | SEED DREAMER | Induced | Differential entropy PSD | DGCNN | val: , ar: , dom: |
[ ] | 2021 | SEED | Induced | Differential entropy and brain network | CNN | |
[ ] | 2024 | SEED | Induced | AND NDE | SVM | 79.16–91.39% 81.66–85.39% |
[ ] | 2024 | DEAP | Induced | Hybrid of time, frequency, time–frequency and location features | kNN SVM ANN | |
Dataset | No. of Modalities | No. of Emotions | No. of Subjects | Comments |
---|---|---|---|---|
WESAD | BVP, ECG, EDA, EMG, RESP, TEMP, ACC | 6 | 15 | Induced |
SWELL | ECG | Multi-dimensional (val, ar, dom) | 25 | Induced |
AMIGOS | ECG, EEG and GSR | 7 | 40 | Induced |
GAMEEMO | EEG | Multi-dimensional (val, ar) | 28 | Induced |
Ref | Year | Database | Modality | Features | Classifier | Average Accuracy | Fusion Method |
---|---|---|---|---|---|---|---|
[ ] | 2015 | eNTERFACE | Audio/facial (visual)/text combined | - | SVM | Acc: | Feature-level fusion (concatenation) |
[ ] | 2012 | RML eNTERFACE | Visual/video and audio | - | HMM | Acc: | Kernel-based feature-level fusion and decision-level fusion |
[ ] | 2013 | RML eNTERFACE | Facial visual and audio | - | HMM | Acc: | Combination of feature-level (KECA) and decision-level |
[ ] | 2020 | ITMDER WESAD | ECG, EDA, RESP and BVP | - | SVM (ar), RF (val) QDA (ar) SVM (val) | ar: , val: ar: , val: | Feature-level fusion (concatenation) |
[ ] | 2011 | In-house dataset (induced) | Eye gaze and EEG | - | SVM | (FF) ar: , val: (DF) ar: , val: | Feature-level (FF) and decision-level (DF) |
[ ] | 2019 | MAHNOB-HCI DEAP | Facial and EEG | CNN/PSD | CNN/SVM | ar: , val: ar: , val: | AdaBoost for decision-level fusion |
[ ] | 2022 | DEAP | EOG and EMG | PSD, Hjorth activity and complexity | Logit boost | Data-level fusion |
Dataset | No. Emotions | No. Utterances | No. Persons | Comments |
---|---|---|---|---|
Belfast [ ] | 8 | 1440 | 60 | dimensional + categorical |
eNTERFACE [ ] | 7 | 239 | 42 | - |
HUMAINE [ ] | 7 | 50 | 10 | naturalistic and induced data + text |
IEMOCAP [ ] | 10 | 5K-10K | 10 | Actors conversation + text |
SEMAINE [ ] | 7 | 959 | 150 | - |
RAVDESS [ ] | 8 | 1440 | 60 | - |
CREMA-D [ ] | 6 | 7442 | 91 | - |
GEMEP [ ] | 15 | 1260 | 10 | - |
MSP-IMPROV [ ] | - | - | 12 | - |
DEAP | - | EEG and audio | 25 (useful) | Dimensional emotion model description |
The statements, opinions and data contained in all publications are solely those of the individual author(s) and contributor(s) and not of MDPI and/or the editor(s). MDPI and/or the editor(s) disclaim responsibility for any injury to people or property resulting from any ideas, methods, instructions or products referred to in the content. |
Share and Cite
Udahemuka, G.; Djouani, K.; Kurien, A.M. Multimodal Emotion Recognition Using Visual, Vocal and Physiological Signals: A Review. Appl. Sci. 2024 , 14 , 8071. https://doi.org/10.3390/app14178071
Udahemuka G, Djouani K, Kurien AM. Multimodal Emotion Recognition Using Visual, Vocal and Physiological Signals: A Review. Applied Sciences . 2024; 14(17):8071. https://doi.org/10.3390/app14178071
Udahemuka, Gustave, Karim Djouani, and Anish M. Kurien. 2024. "Multimodal Emotion Recognition Using Visual, Vocal and Physiological Signals: A Review" Applied Sciences 14, no. 17: 8071. https://doi.org/10.3390/app14178071
Article Metrics
Article access statistics, further information, mdpi initiatives, follow mdpi.
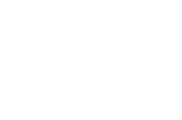
Subscribe to receive issue release notifications and newsletters from MDPI journals
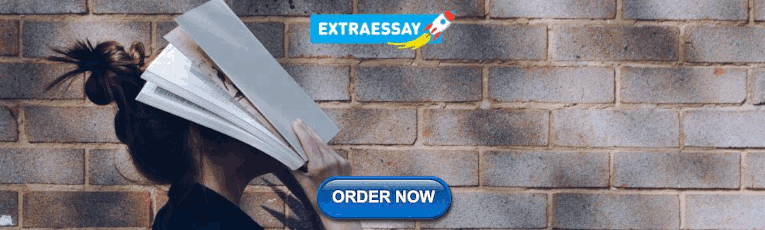
COMMENTS
Data visualization is the graphical representation of information and data. By using v isual elements like charts, graphs, and maps, data visualization tools provide an accessible way to see and understand trends, outliers, and patterns in data. Additionally, it provides an excellent way for employees or business owners to present data to non ...
The Power of Good Data Visualization. Data visualization involves the use of graphical representations of data, such as graphs, charts, and maps. Compared to descriptive statistics or tables, visuals provide a more effective way to analyze data, including identifying patterns, distributions, and correlations and spotting outliers in complex ...
Data visualisation is the graphical representation of information and data. By using visual elements like charts, graphs and maps, data visualisation tools provide an accessible way to see and understand trends, outliers and patterns in data. In the world of big data, data visualisation tools and technologies are essential for analysing massive ...
Data visualization is the representation of data through use of common graphics, such as charts, plots, infographics and even animations. These visual displays of information communicate complex data relationships and data-driven insights in a way that is easy to understand.
A simple definition of data visualization: Data visualization is the visual presentation of data or information. The goal of data visualization is to communicate data or information clearly and effectively to readers. Typically, data is visualized in the form of a chart, infographic, diagram or map. The field of data visualization combines both ...
Data Visualization is a graphic representation of data that aims to communicate numerous heavy data in an efficient way that is easier to grasp and understand. In a way, data visualization is the mapping between the original data and graphic elements that determine how the attributes of these elements vary. The visualization is usually made by ...
Use a horizontal graph when necessary. If your data labels are long, a horizontal bar graph may be easier to read and organize than a vertical bar graph. Don't use a horizontal graph to track time. A vertical bar graph makes more sense when graphing data over time, since the x-axis is usually read from left to right. Histograms vs. bar graphs
Some data visualization tools, however, allow you to add interactivity to your map so the exact values are accessible. 15. Word Cloud. A word cloud, or tag cloud, is a visual representation of text data in which the size of the word is proportional to its frequency. The more often a specific word appears in a dataset, the larger it appears in ...
What is Data Visualization? Data visualization is the graphical representation of different pieces of information or data, using visual elements such as charts, graphs, or maps. Data visualization tools provide the ability to see and understand data trends, outliers, and patterns in an easy, intuitive way. Learn more about data visualization.
6 Real-World Data Visualization Examples. 1. The Most Common Jobs by State. Source: NPR. National Public Radio (NPR) produced a color-coded, interactive display of the most common jobs in each state in each year from 1978 to 2014. By dragging the scroll bar at the bottom of the map, you're able to visualize occupational changes over time.
Use a basic and clear map outline to avoid distracting from the data. Use a single color in varying shades to show changes in data. Avoid using multiple patterns. 11. Gantt Chart. The Gantt chart is a horizontal chart that dates back to 1917. This chart maps the different tasks completed over a period of time.
Data and information visualization (data viz/vis or info viz/vis) [2] is the practice of designing and creating easy-to-communicate and easy-to-understand graphic or visual representations of a large amount [3] of complex quantitative and qualitative data and information with the help of static, dynamic or interactive visual items.
Visualizations That Really Work. Summary. Not long ago, the ability to create smart data visualizations (or dataviz) was a nice-to-have skill for design- and data-minded managers. But now it's a ...
1 Nasa's Eyes on Asteroids. Image Source. If you are interested in exploring data visualization topics in space exploration, check out this striking data visualization created by NASA. NASA's Eyes on Asteroids is one of the best data visualizations due to its exceptional design and functionality.
Introduction. Visual learning is one of the primary forms of interpreting information, which has historically combined images such as charts and graphs (see Box 1) with reading text. 1 However, developments on learning styles have suggested splitting up the visual learning modality in order to recognize the distinction between text and images. 2 Technology has also enhanced visual presentation ...
A graphical representation is a visual representation of data statistics-based results using graphs, plots, and charts. This kind of representation is more effective in understanding and comparing data than seen in a tabular form. Graphical representation helps to qualify, sort, and present data in a method that is simple to understand for a ...
By transforming data sets into visual graphics—such as charts, bar graphs, scatterplots, and heatmaps—you will learn to present complex data in ways your audience can better understand. Through hands-on exercises, you will explore the many types of data in use today, learn how people perceive different graphical displays, and create visual ...
Advantages of Line Graphs. Clarity: Line graphs provide a clear representation of trends and patterns over time or across continuous intervals.; Visual Appeal: The simplicity and elegance of line graphs make them visually appealing and easy to interpret.; Comparison: Line graphs allow for easy comparison of multiple data series on the same graph, enabling quick insights into relationships and ...
The Importance of Visual Data Representation. In the realm of data visualization and graphic communication, the ability to transform complex data into understandable, visual formats has been pivotal. This art and science of making data comprehensible through visual representation have deep roots, tracing back to ancient civilizations.
Thinking and communicating with data visualizations is critical for an educated public (Börner et al., 2019).Science education standards require students to use visualizations to understand relationships, to reason about scientific models, and to communicate data to others (National Governors Association Center for Best Practices and Council of Chief State School Officers, 2010; National ...
Infographics are graphic visual representations of information, data, or knowledge intended to present information quickly and clearly. Utilizing graphics enhances our ability as humans to recognize patterns and trends and improves cognition. Infographics are made up of three primary elements, the visual, the content, and knowledge.
For example, a data visualization campaign about energy use might show how small changes in behavior can lead to significant reductions in energy consumption. Seeing this information in a visual format can make it more compelling and easier to understand than reading a long text description.
Graphical abstracts (GA) and infographics (IG) have revolutionised the way scientific findings are communicated, providing succinct and visually engaging summaries of research. [] Traditionally, the dense and text-heavy nature of scientific publications can be a barrier to the dissemination of knowledge, especially to stakeholders outside the specific field of study.
Edward Rolf Tufte was born in 1942 in Kansas City, Missouri, to Virginia Tufte (1918-2020) and Edward E. Tufte (1912-1999). He grew up in Beverly Hills, California, where his father was a longtime city official.He graduated from the public Beverly Hills High School. [6]Tufte received a BS and a MS in statistics from Stanford University, and a Doctor of Philosophy in political science from ...
Dating back to the 1970s when computer-generated graphics began, 4 papers represented by an image on the journal cover have been cited more frequently than papers without a ... Despite these changes, the demand for visual representations of data and results remains high, as exemplified by graphical abstracts, overview figures, and infographics. ...
The dynamic expressions of emotion convey both the emotional and functional states of an individual's interactions. Recognizing the emotional states helps us understand human feelings and thoughts. Systems and frameworks designed to recognize human emotional states automatically can use various affective signals as inputs, such as visual, vocal and physiological signals.