This website uses cookies to ensure you get the best experience on our website Learn More


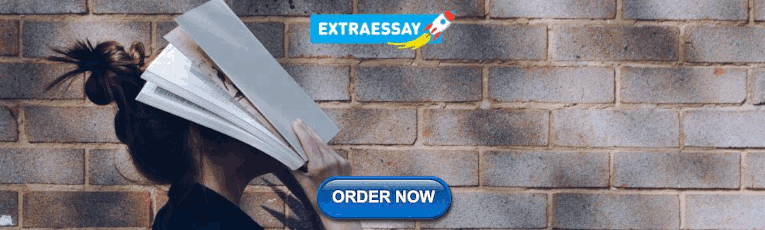
Super charge your college essay

Elevate Your Essay to Perfection

Admissions expertise

Impression analysis

Actionable insights

Instant feedback
Get started free.

Recent blog posts

Frequently Asked Questions
- Skip to main content
- Skip to primary sidebar
- Skip to footer
Additional menu
Khan Academy Blog
Introducing Khanmigo’s New Academic Essay Feedback Tool
posted on November 29, 2023
By Sarah Robertson , senior product manager at Khan Academy
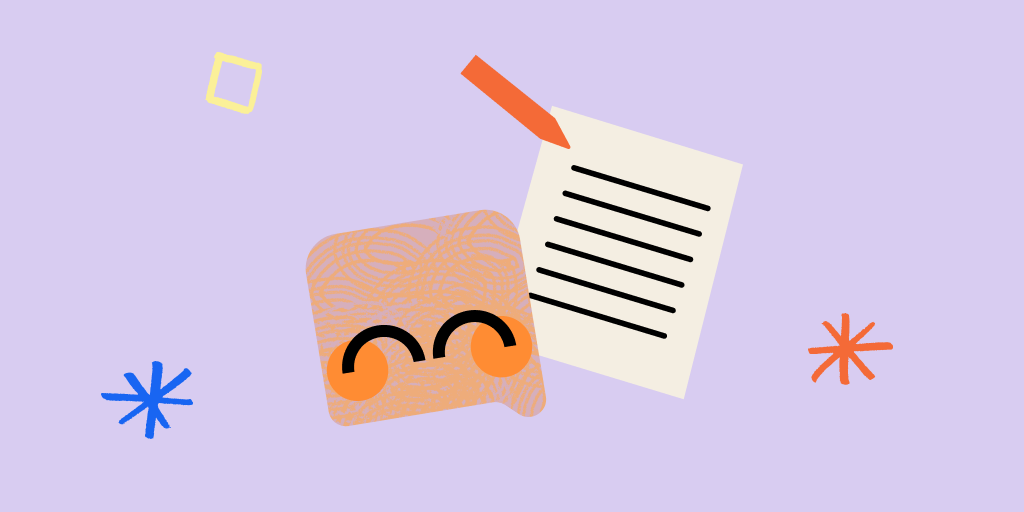
Khan Academy has always been about leveraging technology to deliver world-class educational experiences to students everywhere. We think the newest AI-powered feature in our Khanmigo pilot—our Academic Essay Feedback tool—is a groundbreaking step toward revolutionizing how students improve their writing skills.
The reality of writing instruction
Here’s a word problem for you: A ninth-grade English teacher assigns a two-page essay to 100 students. If she limits herself to spending 10 minutes per essay providing personalized, detailed feedback on each draft, how many hours will it take her to finish reviewing all 100 essays?
The answer is that it would take her nearly 17 hours —and that’s just for the first draft!
Research tells us that the most effective methods of improving student writing skills require feedback to be focused, actionable, aligned to clear objectives, and delivered often and in a timely manner .
The unfortunate reality is that teachers are unable to provide this level of feedback to students as often as students need it—and they need it now more than ever. Only 25% of eighth and twelfth graders are proficient in writing, according to the most recent NAEP scores .
An AI writing tutor for every student
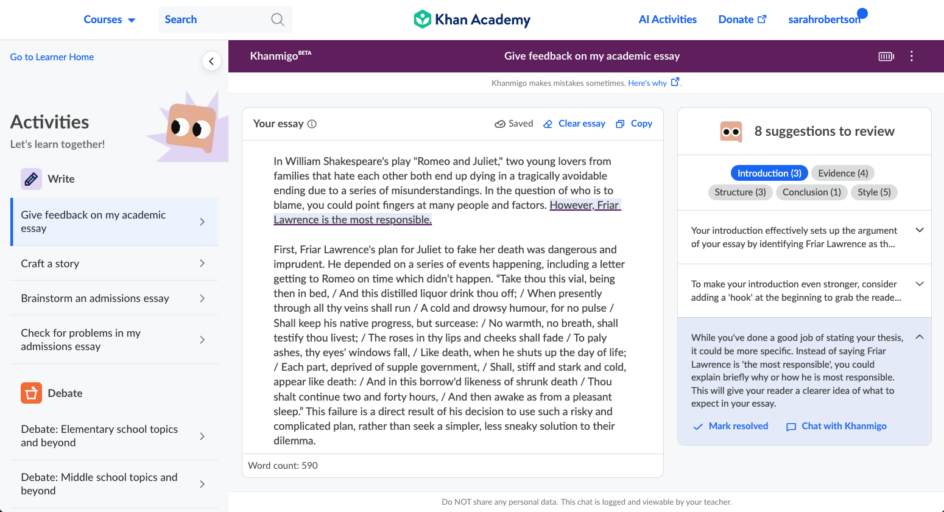
Developed by experts in English Language Arts (ELA) and writing instruction, the pilot Khanmigo Academic Essay Feedback tool uses AI to offer students specific, immediate, and actionable feedback on their argumentative, expository, or literary analysis essays.
Unlike other AI-powered writing tools, the Academic Essay Feedback tool isn’t limited to giving feedback on sentence- or language-level issues alone, like grammar or spelling. Instead, it provides feedback on areas like essay structure and organization, how well students support their arguments, introduction and conclusion, and style and tone.
The tool also doesn’t just stop at providing feedback, it also guides students through the revision process. Students can view highlighted feedback, ask clarifying questions, see exemplar writing, make revisions, and ask for further review—without the AI doing any actual writing for them.
Unique features of Khanmigo pilot Academic Essay Feedback tool
- Immediate, personalized feedback: within seconds, students get detailed, actionable, grade-level-appropriate feedback (both praise and constructive) that is personalized to their specific writing assignment and tied directly to interactive highlights in their essay.
- Comprehensive approach: feedback covers a wide range of writing skills, from crafting an engaging yet focused introduction and thesis, to overall essay structure and organization, to style and tone, to alignment and use of evidence.
- Interactive revision process: students can interact with Khanmigo to ask questions about specific pieces of feedback, get examples of model writing, make immediate revisions based on the feedback, and see if their revisions addressed the suggestion.
- Support for various essay types: the tool is versatile and assists with multi-paragraph persuasive, argumentative, explanatory, and literary analysis essay assignments for grades 8-12 (and more, coming soon).
- Focus on instruction and growth: like all Khanmigo features, the Academic Essay Feedback tool will not do the work for the student. Teachers and parents can rest assured that Khanmigo is there to improve the students’ independent writing skills, not provide one-click suggested revisions.
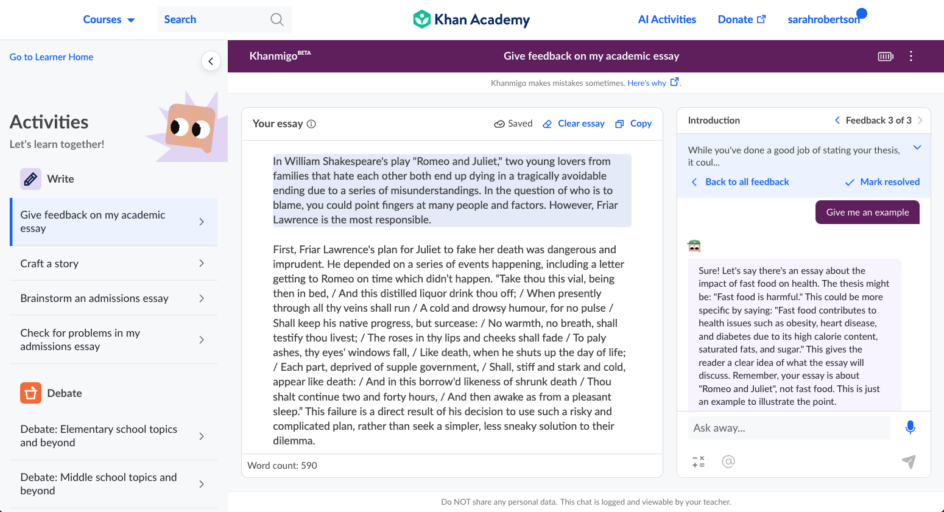
How parents can use Khanmigo’s Academic Essay Feedback tool
Any student with Khanmigo access can find the feedback tool under the “Write” category on their AI Activities menu.
For academic essays, students should simply paste their first draft into the essay field, select their grade level and essay type, and provide the essay instructions from the teacher.
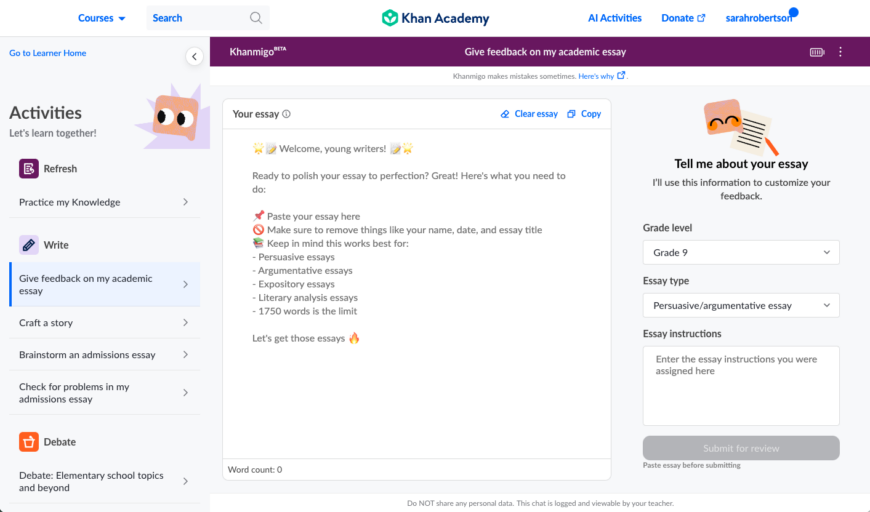
Students then click “Submit” and feedback begins generating. Once Khanmigo is done generating feedback, students can work their way through the suggestions for each category, chat with Khanmigo for help, make revisions, and resolve feedback. They can then submit their second draft for another round of feedback, or copy the final draft to submit to their teacher.
Bringing Khanmigo to your classroom, school, or district
Teachers in Khan Academy Districts partnerships can begin using the Khanmigo Academic Essay Feedback tool with their students right away. Simply direct students to the feedback tool under the “Write” category on their AI Activities menu.
Like all other Khanmigo activities, students’ interactions are monitored and moderated for safety. Teachers or parents can view the student’s initial draft, AI-generated feedback, chat history, and final draft in the student’s chat history. If anything is flagged for moderation, teachers or parents will receive an email notification.
Looking ahead
With the Academic Essay Feedback tool in our Khanmigo pilot, teachers and parents can empower students to take charge of their writing.The tool helps facilitate a deeper understanding of effective writing techniques and encourages self-improvement. For teachers, we think this tool is a valuable ally, enabling them to provide more frequent, timely, detailed, and actionable feedback for students on multiple drafts.
In the coming months, we’ll be launching exciting improvements to the tool and even more writing resources for learners, parents, teachers, and administrators:
- The ability for teachers to create an essay-revision assignment for their students on Khan Academy
- More varied feedback areas and flexibility in what feedback is given
- Support for students in essay outlining and drafting
- Insights for teachers and parents into their students’ full writing process
Stay tuned!
Sarah Robertson is a senior product manager at Khan Academy. She has a M.Ed. in Curriculum and Instruction and over a decade of experience teaching English, developing curriculum, and creating software products that have helped tens of millions of students improve their reading and writing skills.
Get Khanmigo
The best way to learn and teach with AI is here. Ace the school year with our AI-powered guide, Khanmigo.
For learners For teachers For parents
AI-Powered Essays & Papers For Academic Success
Trusted by students and professionals across the world, see what samwell can do.
Example of essays and research papers written with Samwell AI
Analysis of Marketing Mix Strategies for Wal-Mart Company
Performance Evaluation of Portfolio Managers of Mutual and Hedge Funds
Continuity and Change in USA 1950’s to Present
Write, Cite, Learn
Samwell makes writing and researching for essays easy, fast, and fun while delivering the best results
Guided Papers
Get a guided and optimal overview of your research paper.
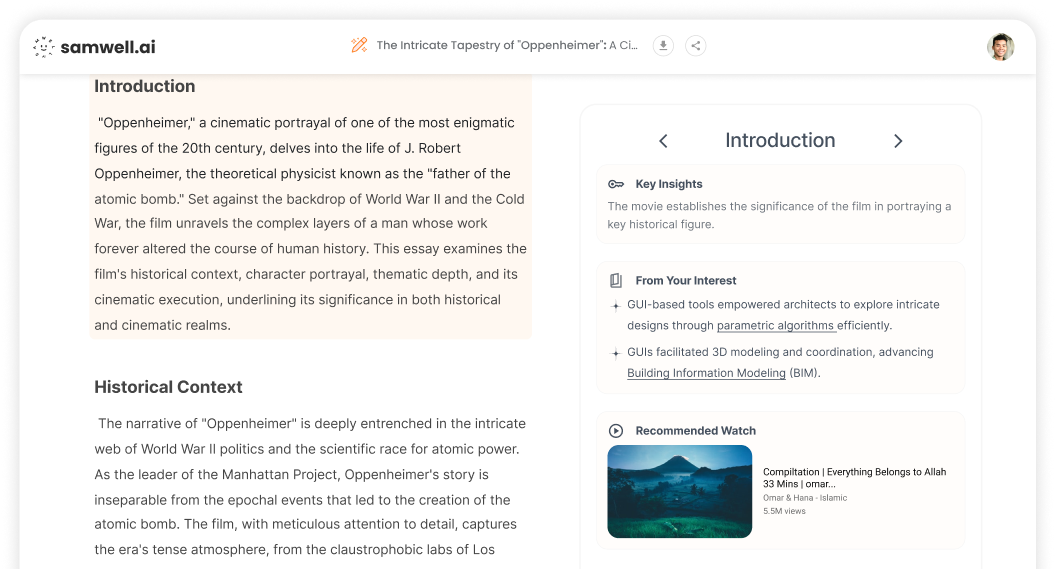
Citation mode
Switch References
Need a reference that better aligns with your essay's requirements? Samwell AI provides a variety of citation choices for each paragraph, ensuring your references are tailored and diverse
Academic Integrity
Samwell AI champions academic integrity, offering suggestions that inspire true creativity within academic standards. Our tool simplifies information access and writing with AI, while keeping the focus on learning - the heart of our mission.
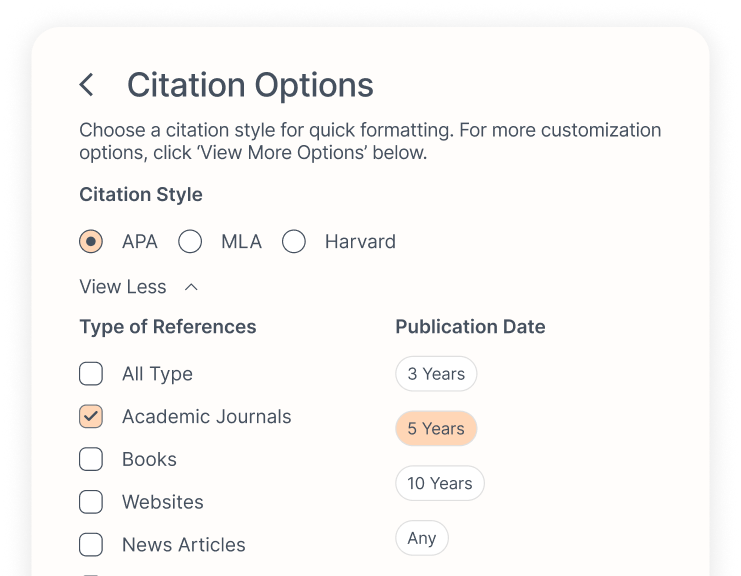
Overview mode
Personalized Research
Discover insights into how the highlights of your new research paper connect with your existing knowledge.
A million empowered writers
Samwell has written over 1.2 billion words from essays to market analysis to blog posts.

Samwell is the app I would have DREAM of when I was in high school, bachelor, master and PhD. So fast and intuitive. We can learn about 10 topics in a day now.

In a world where writing doesn't mean much anymore, Samwell AI offers academics and students what really matters today: quick access to information, references, overview. Introducing it to my students right away.

I considered ChatGPT to be a helpful writing assistant. However, upon discovering Samwell AI, I was amazed. Its capabilities exceeded my expectations by tenfold.

What’s the point to write with AI, now that AI writes for you. Samwell AI is the smartest AI I’ve see, it helps to learn faster and have access to information in a second. No bullshit of you writing, this isn't the point anymore

Samwell provide the best references on the market, the longer essay too (up to 50 pages). Everything in a second and (finally) design friendly. Big up!

A big thank you to Samwell Ai for genuinely saving my life. 🙏❤️

Samwell AI is truly astounding as it generates the entire first draft of your master's thesis or any research paper instantaneously. Furthermore, it provides various options for references and in-text citations, along with a guided tour of the paper. It's absolutely mind-blowing!

Samwell, the AI-driven helper for academic research, has recently improved and become perfect.

Samwell AI is a dream, it guide you through the research paper, you have access to the best accurate sources, it recommend Youtube videos to highlight some important topic. It’s huge!! Congrats!
You’re in control
Choose the package that suits you best. You can switch anytime.
1,000 words
15,000 words per month
90,000 words per month
200,000 words per month
Start Writing Your Free Essay!
More about samwell.
Have a question that needs answering? Our team is here to help! Get in touch with us via email at [email protected]
What are you writing about today?
Write better essays, in less time, with your ai writing assistant.
Thank you for visiting nature.com. You are using a browser version with limited support for CSS. To obtain the best experience, we recommend you use a more up to date browser (or turn off compatibility mode in Internet Explorer). In the meantime, to ensure continued support, we are displaying the site without styles and JavaScript.
- View all journals
- My Account Login
- Explore content
- About the journal
- Publish with us
- Sign up for alerts
- Open access
- Published: 25 January 2021
AI-assisted peer review
- Alessandro Checco ORCID: orcid.org/0000-0002-0981-3409 1 ,
- Lorenzo Bracciale 2 ,
- Pierpaolo Loreti 2 ,
- Stephen Pinfield 1 &
- Giuseppe Bianchi 2
Humanities and Social Sciences Communications volume 8 , Article number: 25 ( 2021 ) Cite this article
27k Accesses
66 Citations
65 Altmetric
Metrics details
- Library science
- Science, technology and society
The scientific literature peer review workflow is under strain because of the constant growth of submission volume. One response to this is to make initial screening of submissions less time intensive. Reducing screening and review time would save millions of working hours and potentially boost academic productivity. Many platforms have already started to use automated screening tools, to prevent plagiarism and failure to respect format requirements. Some tools even attempt to flag the quality of a study or summarise its content, to reduce reviewers’ load. The recent advances in artificial intelligence (AI) create the potential for (semi) automated peer review systems, where potentially low-quality or controversial studies could be flagged, and reviewer-document matching could be performed in an automated manner. However, there are ethical concerns, which arise from such approaches, particularly associated with bias and the extent to which AI systems may replicate bias. Our main goal in this study is to discuss the potential, pitfalls, and uncertainties of the use of AI to approximate or assist human decisions in the quality assurance and peer-review process associated with research outputs. We design an AI tool and train it with 3300 papers from three conferences, together with their reviews evaluations. We then test the ability of the AI in predicting the review score of a new, unobserved manuscript, only using its textual content. We show that such techniques can reveal correlations between the decision process and other quality proxy measures, uncovering potential biases of the review process. Finally, we discuss the opportunities, but also the potential unintended consequences of these techniques in terms of algorithmic bias and ethical concerns.
Similar content being viewed by others
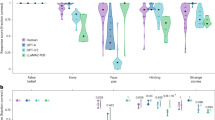
Testing theory of mind in large language models and humans
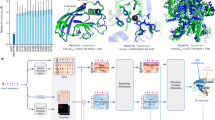
Highly accurate protein structure prediction with AlphaFold
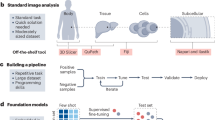
A guide to artificial intelligence for cancer researchers
Introduction.
The scholarly communication process is under strain, particularly because of increasing demands on peer reviewers and their time. Manuscript submissions to peer-review journals have seen an unprecedented 6.1% annual growth since 2013 and a considerable increase in retraction rates (Publons, 2018 ). It is estimated over 15 million hours are spent every year on reviewing of manuscripts previously rejected and then resubmitted to other journals (AJE, 2018 ).
Developments that can make the quality control/assurance process associated with research outputs, particularly the peer review process, more efficient are likely to be welcomed by the research community. There are already a number of initiatives making use of automated screening tools in areas such as plagiarism prevention, requirements compliance checks, and reviewer-manuscript matching and scoring. Many of these tools make use of artificial intelligence (AI), machine learning and natural language processing of big datasets. Some notable examples are:
Statcheck, software that assesses the consistency of authors’ statistics reporting, focusing on p -values (Nuijten et al., 2017 ).
Penelope.ai, a commercial tool able to examine whether the references and the structure of a manuscript meet a journal’s requirements.
UNSILO, software able to automatically pull out key concepts to summarise manuscript content.
StatReviewer, which checks the soundness of statistics and methods in manuscripts (Shanahan, 2016 ).
Automated tools used in the grant-review processes of the National Natural Science Foundation of China, to reduce bias and the load on the selection panels (Cyranoski, 2019 ).
Online system to manage the grant application process, introduced in 2012 by the Canadian Institutes of Health Research (CIHR), removing the need for face-to-face meetings, to reduce reviewer fatigue and improve quality, fairness and transparency.
Automated Essay Scoring (AES) application, used by EdX, MIT and Harvard’s non-profit MOOC federation to assess written work in their MOOCs.
Such initiatives are not without controversy, however. Some doubts have been expressed about the reliability of the Statcheck tool (Schmidt, 2017 ). The CIHR application system received heavy criticism from some reviewers (Akst, 2016 ). Other MOOC producers have been skeptical of the AES scoring application (Balfour, 2013 ).
It is, therefore, helpful to investigate further the potential of big data and AI to support the quality control process in general, and peer review process in particular, and investigate specifically how the time of peer reviewers might be saved, especially in the more tedious parts of the review process, which require less intellectual input or domain expertise. That is what we aim to do in this study.
Peer review is also under strain in the sense that it is coming under increasing scrutiny from those who are concerned that it may often reinforce pre-existing biases in the academy. Biases associated with gender, language or institutional affiliation are examples of those, which may be evident in decisions made within the peer review system (Lee et al., 2013 ). Such biases may arguably come to the fore, particularly if unconscious, when reviewers are time pressured and do not adequately reflect on their own decision-making. Investigation of the system using AI tools may help, therefore, not merely to save reviewers’ time, but also to uncover biases in decision-making. Uncovering such biases may help to develop approaches to reducing or eliminating their impact.
The quality control/assurance process for research publishing typically consists of a number of different components, as delineated by Spezi et al. ( 2018 ). Their work focuses on peer-reviewed journals but applies to other quality-controlled research outputs, e.g., conference proceedings. They divide the normal process that takes place prior to publication in two stages:
Pre-peer review screening: consisting of a number of checks, including plagiarism detection, formatting checks, scope verification etc, plus checking of language and quality of expression. In many cases, if a paper does not meet these checks, it will be “desk rejected” before peer review. However, the extent of pre-peer review screening varies considerably across different publications.
Peer review: normally consisting of assessment of four main criteria: novelty or originality, significance or importance, relevance or scope, rigour or soundness. In addition, peer reviewers are also asked to comment on the quality of the language and argumentation (overlapping with but also extending the language checks carried out in the pre-peer review screening).
Spezi et al. ( 2018 ) also discuss various post-publication quality identifiers, including citation and usage metrics, and reader commenting, as well formal post-publication peer review, as carried out by, e.g., F1000 Research . Post-publication commenting and community-based analysis can in extreme circumstances result in retraction of articles where it becomes evident a study was flawed.
In Fig. 1 , we recast the model developed by Spezi et al. to visualise the different dimensions of the peer review process. We continue to use the framework of pre-publication screening, pre-publication peer review, and post-publication quality indicators, but have attempted to show more clearly where criteria used in pre-screening and peer review intersect, the point that is the focus of the research presented in this paper.
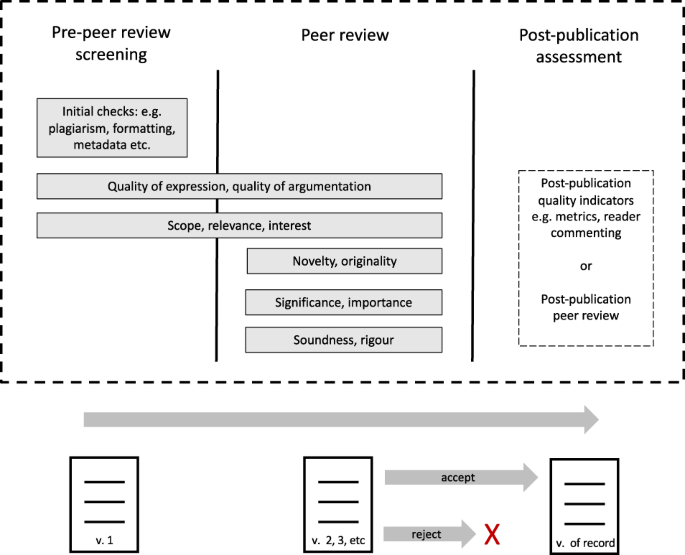
Quality metrics and their relevance along the phases of the peer review process.
Our research covers some aspects of the pre-peer review screening, particularly formatting, language and expression. Pre-peer review screening includes a variety of checks shown in Fig. 1 , including formatting checks. Assessment is also made at the pre-screening phase of quality of expression and scope issues. Consideration of these issues is also undertaken by peer reviewers, who assess quality of expression and argumentation and issues of relevance and interest to a particular subject community as part of their work. The submitted document may undergo several revisions during the process, and will then be formally accepted or rejected for publication. Published documents are normally fixed in the form of a “version of record”.
These two phases are then followed by a post-publication phase (than can affect a paper’s status, e.g., retraction).
Our goal is to make an early contribution to the discussion on the potential, pitfalls and uncertainties of the use of AI to assist pre-peer review screening as well as some of the aspects of the peer review process, based on the results of an empirical investigation aiming to reproduce outcomes of reviewer decision-making using AI methods.
We are interested in understanding the extent to which AI can assist reviewers and authors, rather than in attempting to replace human decision-making processes. At the same time, we are also interested in investigating the ways in which using AI as a rudimentary tool to model human reviewer decision-making can uncover apparent biases in the human decision-making process, and particularly, the extent to which human decision-making may make use of different quality proxy measures, which could produce inequitable assessments. Using AI tools to identify such biases could then help in addressing them.
More specifically, our research questions are:
RQ1: To what extent can AI approximate human decisions in the quality assessment and peer-review process?
RQ2: Can AI play a role in the decreasing time reviewers need to spend assessing papers?
RQ3: Can AI uncover common biases in the review process?
RQ4: What are the ethical implications of the use of such tools?
RQ1 is important since AI approaches may sometimes encounter major problems in trying to imitate abstract and complex intellectual activity, such as peer review, so their accuracy in modelling human decision-making needs to be carefully evaluated. RQ2 raises the possibility of AI tools being used to address some of the strains in the peer review process by potentially avoiding redundant reviews, and removing or at least reducing, the burden of standardised checking (AJE, 2018 ). RQ3 focuses on the extent to which (potential) biases may be evident in review outcomes, in particular how human decision-making may make use of proxy measures of quality, which may reflect bias, and how AI tools may uncover this. RQ4 is important since it encourages reflection on the ethical implications of using AI tools in assisting human decision-making, in particular whether such tools can help address issues of bias or, in fact, whether their use may even risk perpetuating bias.
We address RQ1 by performing and evaluating our experiment in sections “Methodology” and “Results” of this paper, while section “Explainability” reports the reasoning behind our model and its limitations. We address RQ2 in section “Impact.”, where we show how AI can potentially reduce redundant reviews, administrative functions and standardised checks. RQ3 is addressed in sections “Analysis of the experiment outcome” and “Bias”, and RQ4 in section “The ethics of (Semi) automated peer review”, where we analyse the implications of the experiment. We observe that care needs to be taken in how AI tools are used in this space.
Our approach
In this paper, we focus on peer-reviewed conference proceedings, and report an experiment designed to investigate how well a neural network can approximate the known recommendations of peer reviewers. To do that, we trained the neural network using a collection of submitted manuscripts, together with their associated peer review decision (acceptance/rejection or average review score), as outlined in Fig. 2 . The AI tool analysed the manuscripts using a set of features: the textual content (word frequencies), together with readability scores (measuring factors such as word sizes, sentence lengths, and vocabulary complexity, indicating how easy it is to understand the text) and formatting measures—features that might be considered somewhat separate from the substance of the research reported in the document. The analysis therefore covers the parts of the quality assurance process where pre-peer-review screening and peer review itself overlap (Fig. 1 ), covering aspects of pre-peer-review checks, e.g., formatting, and peer review, e.g., quality of expression.
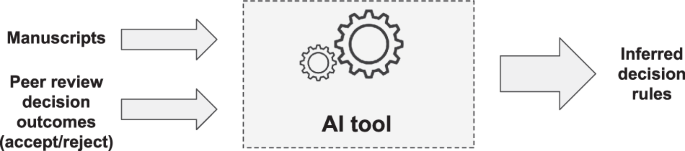
Manuscripts and peer review decision outcomes are inputs of the AI tool to infer decision rules.
The objective underlying the use of the AI tool is to find a set of empirical rules to correlate a posteriori the document features with the final peer review decision. This approach is explained in more detail in section “Methodology”.
Once the learning phase was completed, we evaluated how accurate such empirical rules were in predicting the peer review outcome of a previously unobserved manuscript. Finally, we examined the AI decision criteria to understand what a machine can learn about the quality assessment and peer review process (section “Explainability”). Asking “Why the AI tool has marked papers as accepted or rejected?” is particularly relevant where its “decision” correlates well with the recommendations of reviewers since it may give us insight into human decision-making.
Perhaps surprisingly, even using only rather superficial metrics to perform the training, the machine-learning system was often able to successfully predict the peer review outcome reached as a result of human reviewers’ recommendations. In other words, there was a strong correlation between word distribution, readability and formatting scores, and the outcome of the review process as a whole. This correlation between simple proxy quality measures and the final accept/reject decision is interesting, and merits further discussion and investigation.
We discuss in section “Analysis of the experiment outcome” the significance of this finding, particularly in relation to what it tells us about the quality control process in general and the peer review process in particular.
Limitations of our approach
The approach we take does not cover all the aspects of the peer review process, nor does it attempt to replace human reviewers with AI. However, it suggests that there are some components of the quality assessment and peer review process, which could reasonably to assisted or replaced by AI-assisted tools. These could potentially include readability assessment of the text, and formatting checks, as well as more established checks, e.g., plagiarism detection. Conversely, we do not envisage any relevant contribution from AI on the processes requiring significant domain expertise and intellectual effort, at least for the foreseeable future. The possible model of quality assessment we are exploring is then a semi-automated one, where AI informs decision-making, rather than alone determining outcomes. It is acknowledged the extent to which this is possible will vary depending on a number of factors, not least the nature of the research output itself and its approach to presenting research results. One key variable here is in disciplinary norms. There is likely to be considerable variation across different disciplines in the ways AI assessment tools can be designed and applied to research outputs.
Structure of this paper
The rest of this article is structured as follows. We first introduce related work, in particular studies on peer review and relevant aspects of AI. We then present our methodology, and discuss the accuracy and the explainability of the obtained models. Following that, we analyse the experiment results. We go on to discuss the significance of our findings, the applicability of the proposed system to publishing practice, and some of the key ethical implications. Finally, we draw conclusions and suggest possible future work.
Related work
The peer review process is complex, and itself takes place in a complex wider research system. Judgements of quality take place as part of a system “managed by a hyper-competitive publishing industry and integrated with academic career progression” (Tennant, 2018 ). It is a system that combines extensive collaboration with intense competition between academic researchers and institutions (Tennant, 2018 ). Nevertheless, the “invisible hand” of peer review is still considered to be what keeps the quality of refereed journal literature high (Harnad, 1999 ; Mulligan et al., 2013 ; Nicholas et al., 2015 ). While a future with different approaches to scholarly communication can easily be envisioned (Priem, 2013 ), it is hard to imagine one without peer review (Bornmann, 2011 ; Harnad, 1998 ).
Several studies have analysed how potentially “problematic publications” (e.g., those containing fraudulent research) may be identified through peer review and have provided good practice guidelines for editors (Horbach & Halffman, 2019 ). Problems with the peer review system have been observed focusing a wide variety of problems, ranging from the opportunistic (or even adversarial) rejection of high-quality work, to the acceptance of low-quality manuscripts without a careful review (D’Andrea & O’Dwyer, 2017 ).
A number of recent initiatives have experimented with major changes to the peer review process. Most notably, open peer review is being more widely introduced as an alternative paradigm of interaction between authors and reviewers (Ford, 2013 ; Ross-Hellauer, 2017 ). In the case of open-access mega-journals (OAMJs), the review policies are pared down to focus on rigour and soundness only, leaving to “the community to decide” on issues of novelty, significance and relevance following publication (Spezi et al., 2018 ). Other approaches have included quality judgements being made following publication, sometimes shifting ideas of peer review to potentially include post-publication commenting by readers (Pontille & Torny, 2015 ). A wide range of alternative peer review processes, systems and online solutions (from Reddit-like voting systems to block-chain models) are explored by Tennant et al. ( 2017 ).
While the number of studies of peer review systems is vast, less quantitative analysis of the actual process of reviewing manuscripts has been carried out. Piech et al. ( 2013 ) studied how to identify and correct for the bias of reviewers in Massive Open Online Courses (MOOCs). Some MOOCs have already started to use machine-based Automated Essay Scoring (AES) applications to assess work, although others have pointed out potential problems in using such tools (Balfour, 2013 ).
To understand how the peer review process may be supported by AI tools, an important precondition is understanding how the quality and readability in textual data can be assessed. Readability formulas and cognitive indices has been studied extensively (Crossley et al., 2011 , 2008 ), and Natural Language Processing (NLP) has proven to be a powerful tool for text quality assessment (Cozza et al., 2016 ). However, assessing the quality of complex documents by automated means is still a challenging problem (Sonntag, 2004 ).
Modelling of the peer review process has been attempted in other contexts, such as education research (Goldin & Ashley, 2011 ), and in legal education contexts (Ashley & Goldin, 2011 ), which may be relevant for our study.
One thing that is apparent, however, is that many socio-cultural biases are present in peer review (Lee et al., 2013 ), and some of them could potentially propagate to AI systems, as described in the studies on algorithmic bias (Garcia, 2016 ; Mittelstadt et al., 2016 ). Many studies have shown that biased algorithms can inadvertently discriminate against specific groups (Barocas & Selbst, 2016 ; Zarsky, 2016 ).
Bias in the review process may take different forms. These include “first-impression” bias, the Doctor Fox effect, ideological/theoretical orientation, language, perceived social identity and prestige biases (Hojat et al., 2003 ; Lee et al., 2013 ; Siler et al., 2015 ). Such biases are evident in many contexts, such as websites (Lindgaard et al., 2006 ; SWEOR, 2019 ), examinations (Wood et al., 2018 ) or staff recruitment (Florea et al., 2019 ). In the area of document assessment, the typographical layout has been proven to have an important role in the “first-impression bias”, where initial impressions of the document colour further judgements about its overall quality (Moys, 2014 ). Challenges remain in modelling this, although there are some pioneering studies that show how AI techniques can be used to model first-impression bias in relation to human encounters, e.g. job interviews (Gucluturk et al., 2017 ).
As the peer review process is a highly complex and demanding set of tasks, we suggest that, especially when time is at a premium, reviewers may tend to employ heuristics (D’Andrea & O’Dwyer, 2017 ) to assess a paper (e.g., more superficial features of the document, like language, formatting, etc.). Such heuristics can potentially be approximated using AI. Indeed, recent trends demonstrate the ability of AI to approximate human cognition in some simple tasks in a way that is similar to the way humans use their senses to relate to the world around them (Russell & Norvig, 2016 ). However, understanding how far we are from machine approximation to the full task of peer review, with all of its complex intellectual input, is still an open question.
Methodology
To investigate how the review process works, it is necessary to have access to a set of submitted papers and their corresponding review reports (including the specific scores assigned by reviewers). This itself is not easy, as the reviews, and especially the content of rejected manuscripts are usually confidential. In section “Data collection”. we explain in detail how we overcame this challenge in the data collection process we employed. Once a set of papers has been acquired together with their review scores, it is necessary to perform a set of transformations on the data to obtain relevant features. The process we carried to do this is described in section “Feature extraction”. After that, some statistics on the documents also need to be collected to help the modelling process, as shown in section “Feature augmentation—macroscopic features”. Finally, the features can be used to train a neural network, as described in section “Neural network design”. We include a significant level of technical detail in this section for reasons of transparency and in order to enable the replicability of our experimental setup. The process we followed is represented in Fig. 2 , a schematic of the training of the AI tool. By inputting both the submitted manuscripts and peer reviewer recommendations/decisions into the AI tool, we were able to produce a set of inferred decision rules, which underpinned the decisions made.
Data collection
We employed two different strategies to obtain the review data. Firstly, we obtained submitted manuscripts, numerical reviewers’ scores and editorial decisions from the general chair of the 2018 IEEE wireless communications and networking conference (WCNC). Secondly, we employed data from openreview.net (aka OpenReview), which provides “a flexible cloud-based web interface and underlying database API enabling [...] open peer review, access, discussion and publishing” Footnote 1 . We selected two conferences with the largest number of publications from openreview.net, that is the International Conference on Learning Representations (ICLR) for the years 2018 and 2019. All the papers from both ICLR and WCNC had been reviewed by two to five reviewers. We cleaned the data to remove any information that had been added after manuscript acceptance, e.g., author names and affiliations. For simplicity we did not use the textual reviews, but rather we focused only on the numerical scores of the review. In summary, for all of the datasets we had: a paper (pdf file), an editorial decision (accepted/rejected), and numerical reviewers’ scores (e.g., 3.5).
In Table 1 , a summary of the data is shown, together with the dimensions of the training set used to build the model and the test set used to evaluate its predictive capabilities. We can observe that the datasets are fairly balanced. Figs. 3 and 4 show that the separation between accepted and rejected papers in terms of score is quite apparent. However, in a small number of cases, there is a rather strong discrepancy between the editorial decision and the average score of the reviewers. This is particularly true for OpenReview data: after a preliminary analysis on the dataset, we did not find any rule or correlation that appreciably binds the final acceptance decision with the document content, apparently corroborating Colman ( 1982 ), who also observed a lack of correlation between paper quality, authors’ reputation/affiliation and the final accept-reject decisions for journal papers. We did, however, identify some patterns when using the average reviewers scores (previous step of the review process). For this reason, we decided to focus on predicting the average reviewers score for OpenReview data.
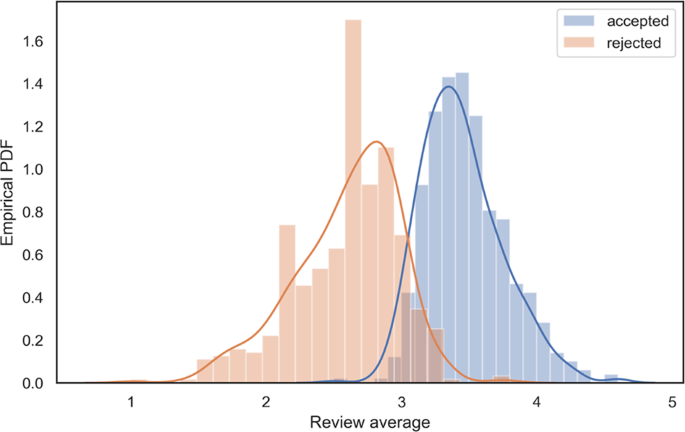
Empirical probability distribution function of the average review score for accepted (in blue) and rejected (in orange) WCNC papers.
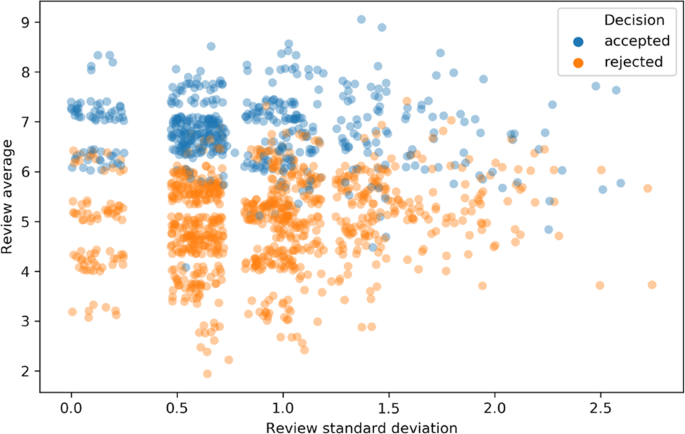
Scatterplot of average vs. standard deviation of accepted and rejected OpenReview papers, with added jitter to improve visibility.
Feature extraction
The pdf documents were converted to textual data. Then, each document text was tokenised Footnote 2 using binary encoding of the top 20,000 words in terms of frequency for the WCNC conference and term frequency-inverse document frequency (TFIDF) of the top 2000 words for OpenReview.
Feature augmentation—macroscopic features
As further discussed in sections “Related work” and “Analysis of the experiment outcome”, the layout of the document and its graphical components could affect the first-impression of the reviewer, and thus are important in our modelling process. For this reason, we added “macroscopic features” like text/image ratio, file size and textual length to our analysis. We also incorporated the most commonly-used text quality and readability metrics (Crossley et al., 2011 ), as shown in Table 2 .
Neural network design
For all the datasets, we used well-accepted standards of developing neural networks, technical details of which are given below. We employed a dense neural network with a 32 neurons layer, followed by a dropout layer to reduce overfitting, feeding to a layer of 16 neurons. The two layers used Rectified Linear Unit (ReLU) activation. The final layer comprises a single node with a sigmoid activation function when the network is trained to predict paper acceptance or rejection or to predict the reviewer’s scores. The resulting networks include 640,577 total trainable parameters for WCNC, 64,577 total trainable parameters for OpenReview. The difference is accountable to the different number of input features for the two analysed cases. The loss function was the binary cross entropy for the classification problem (WCNC) and the Mean Squared Error (MSE) for the regression problem (OpenReview). To train the network we made use of Stochastic Gradient Descent (SGD) with Nesterov update.
Using a standard machine-learning approach, we split the dataset in a training set on which the learning algorithm builds its model, and a test set used to evaluate the model accuracy. The model accuracy is defined as the ability to predict, respectively: (i) whether a previously unobserved paper would be accepted or not (for the WCNC dataset); (ii) the reviewers average score (for the OpenReview dataset).
In this section, we show the results in terms of prediction performance of the designed models with respect to the final editorial decision. For an analysis of the models, see section “Analysis of the experiment outcome”.
WCNC dataset
For this dataset, we used as baseline a random classifier, that would obtain an F1-score Footnote 3 of about 50% since the dataset is balanced. We measured accuracy, precision, recall and F1-score. Depending on the context in which the model is used, one of these metrics on its own might be more appropriate to assess the usability of the model. For example, recall might be the best measure if the tool was meant to signal problematic papers to assign additional reviewers: in that case, a false negative (a high-quality paper signalled as low quality) might create an additional burden on the reviewer and, at the same time, reduce the confidence on the tool. Conversely, F1-score might be more appropriate in assessing the quality of the prediction if the cost of false positives and false negatives are expected to be the same. The results are shown in Table 3 .
By focusing on the first layer of the neural network, we can observe that the stronger features in activating the neurons are the Linsear write formula (Crossley et al., 2011 ), the text length and the number of pages, together with the following list of words: address, approach, approximately, conclusion, constant, correlation, deployed, drawn, easy, efficient, illustrates, increased, issue, knowledge, level, obtain, page, potential, previously, process, respectively, types , γ , τ . However, it is important to note that the interactions between the features are more complex than a simple independent activation, and involve multiple layers in the neural network. This is why we dedicate section “Analysis of the experiment outcome” to an extensive analysis of the interpretability of the model.
OpenReview dataset
As discussed before, here we focused on the prediction of the reviewer average score, using the Mean Absolute Error (MAE) and the Mean Squared Error (MSE) as reference metrics. As baseline we selected a naive classifier that chose the median score of the training dataset. In Fig. 5 , the training behaviour of the network is shown, in terms of MSE of the validation and training set over the different training epochs. The performance of the trained regressor is shown in Table 4 .
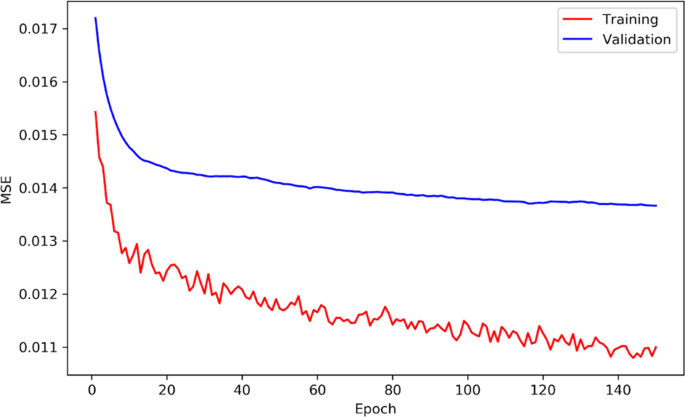
Mean Squared Error vs. number of training epochs for OpenReview training process, with batches of 32 samples.
As we can see, the trained regressor is able to improve a naive one.
Even more importantly, Fig. 6 shows the empirical distribution of the MAE over the test set: we can see that 75% of the samples have an error of under 1.2 (over a total score of 10), and the median error is only 0.79. This means that we can expect this model to predict the average reviewers score with a median error of 0.79 over 10. While the reduction in the error rate is promising, it is worth noting that its low magnitude (for both approaches) is in part explained by the low variance of the scores used by reviewers, who tend not to use the whole scale available.
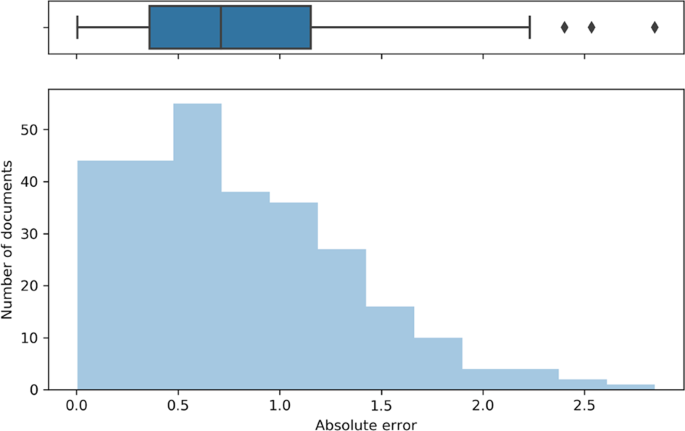
75% of the samples have an error of under 1.2, and the median error is 0.79.
As for the previous dataset, we can observe that the stronger features activating the first neural layer are the Lix index, the Flesch Kincaid grade (Crossley et al., 2011 ), the text length, number of pages and file size, together with the following list of words: 256, actor, buffer, causal, coefficients, curve, demonstrate, dnn, exploration, github, gpu, idea, imagenet, measures, message, perturbations, precision, produce, quantised, query, regression, review, selected, sentence, standard, state, supervision, tensor, token, width . We refer to the next section for a more detailed analysis of the explainability of the model.
Explainability
In the case of black-box models like deep-learning systems, such as the one we developed, it is important to attempt to interpret the reasoning of the model, or in other words, the rationale for an automated decision, to allow practitioners to decide the level of trust given to a model. This is of fundamental importance to reduce the opacity of such tools, enabling an informed evaluation of their performance and, therefore, allowing greater trust in their outputs.
Explaining models depending on half a million parameters is practically impossible using standard tools. For example, the presence of a specific keyword or a specific document statistics can affect the model decision in a non-linear and document-dependent way, making it very challenging to identify a set of simple rules that can make the model understandable to a non-specialist.
However, recent studies have shown that local interpretable model-agnostic explanations (LIME) are able to effectively explain the model decision on a specific document (Ribeiro et al., 2016 ). The technique is based on the local perturbation of an instance and the development of a linear model. We used this method to create explanations on multiple documents, and repeat this approach on the whole document space, with the goal of picking a set of exemplar documents distant enough from each other to obtain a representative set of rules of the whole model (submodular pick technique).
In Figs. 7 and 8 examples of the local explanation for an accepted paper of the OpenReview dataset are shown. In orange the top features influencing the decision towards a positive decision are represented, while the blue colour represents factors associated with a negative decision. This summary is simple enough to be presented as is to a non-specialist. In Fig. 7 , the absence of the word “quadratic”, a low sentence count, and a high number of difficult words positively affects the model score, while a low number of pages, a small number of average syllables per word and a low text length affect the model score negatively. In some cases, the local explanation can expose potential biases or signal overfitting of the model. Overfitting occurs where the fits to the model is based closely on the specifics of the training set but would be a poor fit further related data. For example, in Fig. 8 , we can observe that the absence of the word “decoding” is affecting negatively the model decision. This might reasonably be considered an overfitting problem caused by the over-abundance of documents related to decoding in this conference. The choice of whether this contingent explanation is satisfactory is highly context dependent, but it can increase the transparency of the model and allow the practitioners to assess the trust on the model. Another example of overfitting has been observed in the early stages of the model building, using a less than optimal hyperparameter set. In that case, the presence of some specific first names was regarded as a positive signal for the final decision.
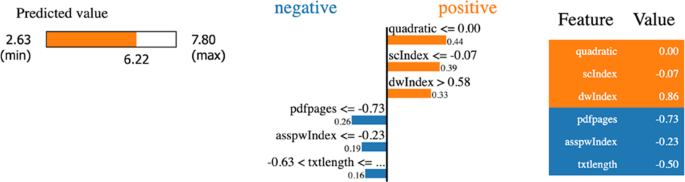
The top features affecting the model are: the word “quadratic”, the sentence count scIndex , the number of difficult words dwIndex , the number of pages pdfPages , the average number of syllables per word asspwIndex , and the text length txtlength .
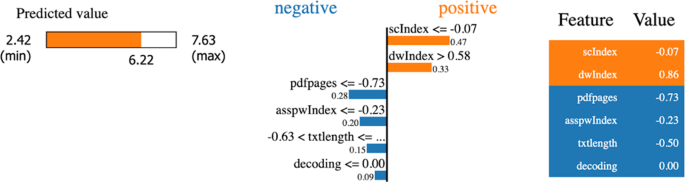
The top features are: the word “decoding”, the sentence count scIndex , the number of difficult words dwIndex , the number of pages pdfPages , the average number of syllables per word asspwIndex , and the text length txtlength .
Often local rules do not generalise for documents that are considerably different. For example, high text length can increase the predicted score when some keywords are present, while it could be decrease it in other contexts. This is clearly shown after running a submodular pick analysis of the whole space, as shown in Fig. 9 , after identifying a group of exemplar documents covering the training space. Some keywords like “hyperparameters” and “quadratic” can be modelled as positive or negative depending on the context of the specific paper.
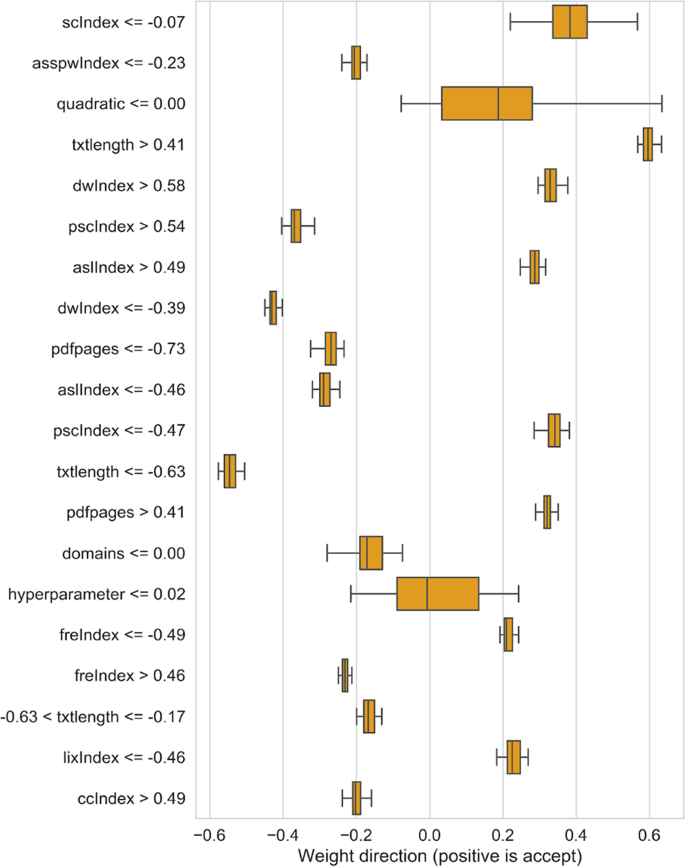
Boxplot representing the weight direction for each feature (vertical black line is the median).
Analysis of the experiment outcome
Despite the focusing on rather superficial document features, like word distribution, readability and formatting scores, the machine-learning system was often able to successfully predict the peer review outcome. In other words, those documents that scored highly in those areas (e.g., they achieved high scores in readability and were formatted as required) were more likely to be recommended by reviewers for acceptance, and those that achieved lower scores in those areas, more likely to be recommended for rejection. There are a number of possible explanations for this.
One possible explanation is that a correlation between such superficial features and the outcome of the review process as a whole might indicate that they are in fact a good indicator of the overall quality of the paper. In other words, if a paper is presented and reads badly, it is likely to be of lower quality in other, more substantial, ways, making these more superficial features proxy useful metrics for quality. In that case, assessing a paper taking into particular account of those superficial features may be a reasonable heuristic in making overall decisions about the quality of the paper. If that was the case, it would be reasonable to use AI to screen papers before peer review process, using AI as a tool to desk reject papers based on these macroscopic features as part of the pre-peer review screening referred to earlier. This would save the time of peer reviewers who would have to review papers, which were highly likely to low quality. Even if low-scoring papers are not desk rejected, it could be that their scores are flagged to peer reviewers to assist them in making their decisions—also a potential time saver.
However, it may be that papers that score less well on these superficial features create a “first-impression bias” on the part of peer reviewers, who then are more inclined to reject papers based on this negative first-impression derived from what are arguably relatively superficial problems. Reviewers may be unduly influenced by, e.g., formatting or grammatical issues and become unconsciously influenced by this in their judgements of more substantive issues in the submission. Examples of such issues in papers include the presence of typos, the presence of references to papers from regions under-represented in the scientific literature, or the use of methods that have been associated with rejected papers in the past.
In that case, an AI tool that screens papers prior to peer review in the way described, could be used to advise authors to rework their paper before it is sent on for peer review, since it is likely that peer reviewers may reject the paper or at least be negatively influenced by the macroscopic features of the paper, which could be relatively easily corrected.
This might be of particular benefit to authors for whom English is not a first language, for example, and whose work, therefore, may be likely to be adversely affected by first-impression bias.
Table 5 lists several aspects and the potential role how AI-based tools, such as the one we describe in this study, can (or already do) impact the different dimensions of the peer review process.
Tools of this kind have the potential to be of direct benefit in assisting editors of journals and conference proceedings in decision-making (and similarly, the role of making funding decisions, as described in section “Conclusions and future work”). Such tools have the potential to save the time of reviewers, when used as decision support systems. We suggest there may be potential positive impacts in the following specific processes.
Reducing desk rejects
By catching the “first eye impression”, the approach we have explored in this paper has the potential to detect early superficial problems, like formatting issues and quality of the graphs. Authors could be made aware of such problems immediately without any further review, or the outcome could be used to pre-empt/inform desk rejects. Even though this technique could wrongly signal high quality (but unusual) typographical choices, a notification about potential issues would help authors to evaluate whether or not they should correct their presentation. Removing superficial problems before peer review could help to avoid reviewer decisions being unduly informed by first-impression biases, and allow them to focus more on the scientific content. On the other hand, AI could also provide inexperienced reviewers with an impartial point of view of the work, providing some performance indicators and synthetic parameters such as a measure of deviation from past authors in terms of style, language and typographic format.
Explaining decisions by data-driven modelling
By analysing review decisions via a data-driven predictor/classifier, it is possible to investigate the extent to which the complex reviewing process can be modelled at scale. Although complex (our preliminary neural network has half a million parameters), such models can be inspected to derive justifications for and explanations of decisions. While completely replicating the cognitive tasks required for the peer review process would be demanding, an analysis of the human decision process through data analysis and AI replication could potentially more easily imitate the more superficial parts of the decision-making processes involved. This could in turn potentially expose biases and similar issues in the decision-making process.
Discovering latent motivations
Motivations behind a decision are not always clear, even to the person making the decision. Producing a model for predictors/classifiers potentially exposes hidden motivations underlying a decision. This idea has been a particular feature of marketing research as a way of identifying and (and then exploiting) “gut reactions”. Exposing such motivations in the context of peer reviewing would help reviewers and editors to increase awareness in and transparency of the peer review process, and this may again help to identify possible biases in decision-making.
Machine-learning techniques are inherently conservative, as they are trained with data from the past. This could lead to bias and other unintended consequences when a tool based on machine learning is used to inform decision-making in the future. For example, papers with characteristics associated with countries historically under-represented in the scientific literature might have a higher rejection rate using AI methods, since the automated reviews may not adequately take account of rising quality of submissions from such sources over time. Biases might also be introduced by the fact that historically, editors have disproportionately selected reviewers from high-income regions of the world, while low-income regions are under-represented among reviewers. The USA dominates the contribution to peer review, with 32.9% of all reviews vs. 25.4% of published article output (Publons, 2018 ). We suggest that AI systems can be used to expose possible biases and to inform actions taken to prevent their replication in future use of automated tools.
The ethics of (semi) automated peer review
As shown in section “Results”, overfitting and other issues with the model we have used could lead to unintended consequences, like the creation of biased rules that could penalise under-represented groups or even individuals if a tool such as the one we have developed is used in peer review. Following the categories delineated by Mittelstadt et al. ( 2016 ) and considering the most relevant of them to our study, we can identify three key examples of ethical concerns arising from our work:
Inscrutable evidence leading to opacity
When the link between the original data and the way they affect the model prediction is not easy to interpret, there is a problem of algorithm opacity, that can in turn lead to mistrust towards the algorithm and the data processors. An author will not trust a review if there is no transparency on the rationale for the decision taken. If tools are used to assist in decision-making of the sort we have described in future, it is crucial that there is as great a level of transparency as possible about how the models work to explain and justify decisions made.
Misguided evidence leading to bias
Models are the result of a particular design path, that has been selected following the values and goals of the designer. These values and goals will inevitably be “frozen into the code” (Macnish, 2012 ). Moreover, models based on machine learning, like the one described in this work, rely on past results (in this case, past reviews), and thus a model may propagate cultural and organisational biases already present in the learning set (Diakopoulos, 2016 ). Other sources of bias can be technical constraints or emergent contexts of usage. In review systems, a tool such as the one we have developed could in practice adversely affect decisions on papers produced by authors from low-income countries and or those on innovative topics if used without taking such possibilities into account.
Transformative effects leading to challenges for autonomy
Even using such models only to signal problematic papers or to assist reviewers could affect the agency of reviewers by creating new forms of understanding and conceptualisation. This may result in a specular effect to the one discussed in the previous point: the way the model interprets the manuscript could propagate to the reviewer, potentially creating an unintended biased outcome. For example, should the model identify as potential issues the presence of typos, the presence of references to papers from under-represented regions, or the usage of techniques that have been associated with previously rejected papers, the potential effect of the signalling of such issues to the reviewers could be an increase of the importance of such factors in the mind of the reviewers and influence their authority bias/status quo bias.
All of these ethical concerns need to be considered carefully in the way AI tools are designed and deployed in practice, and in determining the role they play in decision-making. Continued research in these areas is crucial in helping to ensure that the role AI tools play processes like peer review is a positive one.
Conclusions and future work
In this paper, we have reported an experiment involving three peer-reviewed conference proceedings, training a machine-learning system to infer a set of rules able to match the peer review outcome, ultimately providing an acceptance probability for other manuscripts. We focused on a rather superficial set of features of the submitted manuscripts, like word distribution, readability scores and document format.
Nevertheless, the machine-learning system was often able to successfully predict the peer review outcome: we found a strong correlation between such superficial features and the outcome of the review process as a whole.
We have seen how tools could be developed based on such systems, which could be used to create greater efficiency in the quality control and peer review process. We have also seen how such tools could be used to gain insight on the reviewing process: our results suggest that such tools can create measurable benefits for scientometric studies, because of their explainability capability.
While the application of such AI tools is still in its infancy, we have observed some of the possible implications in terms of biases and ethics. Our findings point in the direction of a new type of analysis of typical human process, conducted with the help of machine-learning systems, one which is cognisant of ethical dimensions of the work as well as technical capabilities.
The following future work is suggested.
Feedback loop
We are interested in exploring the behaviour of reviewers when using these AI-powered support tools. We intend in future to carry out controlled experiments with academic reviewers, to understand the biases introduced by the AI signals on the reviewers. As discussed in “The ethics of (semi) automated peer review”, understanding potential effects on the reviewers is fundamental to ensuring ethical usage of such tools.
Review process
When using openreview.net, we would be interested in taking into account the full text of the review itself (rather than only the review outcome) to better train the AI tools. A great deal of useful information is contained in the text of the reviews and rebuttals that inform the final decision.
Work on the first-impression bias needs to be extended, including more complex typographic layout indicators. Similarly, a more detailed analysis of the model could expose additional decision rules like language issues and formatting issues.
Disciplinary variation
We would like to explore how the design and application of AI tools carrying out semi-automated quality assessment can take place in the context of different disciplines, taking into account different disciplinary norms of communicating research results.
Grant applications
Funders might use such decision support systems to assess grant applications. Grant applications have a different structure (as they are proposing projects not reporting them), thus the content heterogeneity might be higher. We plan to investigate further the application of the methods discussed here to that domain.
Data availability
The datasets generated during the current study are not publicly available due to de-anonymisation risks, but are available from the corresponding author on reasonable request. The OpenReview data are available on https://openreview.net/ .
Openreview is available at https://openreview.net/about .
Tokenisation is a standard machine-learning process, which consists in chopping a text into words (tokens), throwing away punctuation.
In statistics, F1-score is a measure of a test’s accuracy, which considers both the precision and the recall.
AJE (2018) Peer review: how we found 15 million hours of lost time. URL https://www.aje.com/en/arc/peer-review-process-15-million-hours-lost-time , Accessed 20 Dec 2019
Akst J (2016) Researchers to CIHR: reverse peer review changes. URL https://www.the-scientist.com/the-nutshell/researchers-to-cihr-reverse-peer-review-changes-33236 .
Ashley KD, Goldin IM (2011) Toward AI-enhanced computer-supported peer review in legal education. In: Biswas G, Bull S, Kay J, Mitrovic A (eds) JURIX. pp. 3–12
Balfour SP (2013) Assessing writing in MOOCs: automated essay scoring and calibrated peer review. Res Pract Assess 8:40–48
Google Scholar
Barocas S, Selbst AD (2016) Big data’s disparate impact. Cal Law Rev 104:671
Bornmann L (2011) Scientific peer review. Ann Rev Inform Sci Technol 45:197–245
Article Google Scholar
Colman AM (1982) Manuscript evaluation by journal referees and editors: randomness or bias? Behav Brain Sci 5:205–206
Cozza V, Petrocchi M, Spognardi A (2016) A matter of words: NLP for quality evaluation of Wikipedia medical articles. In: Bozzon A, Cudré-Maroux P, Pautasso C (eds) International Conference on Web Engineering. Springer, pp. 448–456
Crossley SA, Allen DB, McNamara DS (2011) Text readability and intuitive simplification: a comparison of readability formulas. Read Foreign Lang 23:84–101
Crossley SA, Greenfield J, McNamara DS (2008) Assessing text readability using cognitively based indices. Tesol Quart 42:475–493
Cyranoski D (2019) Artificial intelligence is selecting grant reviewers in China. URL https://www.nature.com/articles/d41586-019-01517-8 , Accessed 20 Dec 2019
D’Andrea R, O’Dwyer JP (2017) Can editors save peer review from peer reviewers? PLoS ONE 12:e0186111
Diakopoulos N (2016) Accountability in algorithmic decision making. Commun ACM 59:56–62
Florea L et al. (2019) From first impressions to selection decisions: the role of dispositional cognitive motivations in the employment interview. Person Rev 48:249–272
Ford E (2013) Defining and characterizing open peer review: a review of the literature. J Scholar Publish 44:311–326
Garcia M (2016) Racist in the machine: the disturbing implications of algorithmic bias. World Policy J 33:111–117
Goldin IM, Ashley KD (2011) Peering inside peer review with bayesian models. In: Biswas G, Bull S, Kay J and Mitrovic A (eds) International Conference on Artificial Intelligence in Education. Springer, pp. 90–97
Güçlütürk Y et al. (2017) Multimodal first impression analysis with deep residual networks. IEEE Trans Affect Comput 9:316–329
Harnad S (1999) Free at last: the future of peer-reviewed journals. D-Lib Magaz 5:12
Harnad S (1998) The invisible hand of peer review. Nature 5. https://doi.org/10.1038/nature28029 .
Hojat M, Gonnela JS, Caelleigh AS (2003) Impartial judgment by the "gatekeepers" of science: fallibility and accountability in the peer review process. Adv Health Sci Educ 8:75–96
Horbach SPJM, Halffman W (2019) The ability of different peer review procedures to flag problematic publications. Scientometrics 118:339–373
Article CAS Google Scholar
Lee CJ et al. (2013) Bias in peer review. J Am Soc Inform Sci Technol 64:2–17
Lindgaard G et al. (2006) Attention web designers: you have 50 milliseconds to make a good first impression! Behav Inform Technol 25:115–126
Macnish K (2012) Unblinking eyes: the ethics of automating surveillance. Ethics Inform Technol 14:151–167
Mittelstadt BD et al. (2016) The ethics of algorithms: mapping the debate. Big Data Soc 3:68
Moys JL (2014) Typographic layout and first impressions: testing how changes in text layout influence reader’s judgments of documents. Vis Lang 48(1): 881
Mulligan A, Hall L, Raphael E (2013) Peer review in a changing world: an international study measuring the attitudes of researchers. J Am Socr Inform Sci Technol 64:132–161
Nicholas D et al. (2015) Peer review: still king in the digital age. Learn Publ 28:15–21
Nuijten MB, Van Assen MALA, Hartgerink CHJ, Epskamp S, Wicherts JM et al. (2017) The validity of the tool "statcheck” in discovering statistical reporting inconsistencies. Preprint retrieved from https://psyarxiv.com/tcxaj/
Piech C, Huang J, Chen Z et al. (2013) Tuned models of peer assessment in MOOCs. In: D’Mello SK, Calvo RA and Olney A (eds) 6th International Conference on Educational Data Mining (EDM 2013). International Educational Data Mining Society, pp. 153–160
Pontille D, Torny D (2015) From manuscript evaluation to article valuation: the changing technologies of journal peer review. Human Stud 38:57–79
Preim J (2013) Beyond the paper. Nature 495:437–440
Article ADS Google Scholar
Publons (2018) Global state of peer review 2018. URL https://publons.com/static/Publons-Global-State-Of-Peer-Review-2018.pdf , Accessed 20 Dec 2019.
Ribeiro MT, Singh S, Guestrin, C (2016) Why should I trust you?: Explaining the predictions of any classifier. In: Balaji K, Mohak S (eds) Proceedings of the 22nd ACM SIGKDD international conference on knowledge discovery and data mining. ACM, pp. 1135–1144
Ross-Hellauer T (2017) What is open peer review? A systematic review. F1000Res 6.588. https://doi.org/10.12688/f1000research.11369.2
Russell SJ, Norvig P (2016) Artificial intelligence: a modern approach. Pearson Education Limited, Malaysia
MATH Google Scholar
Schmidt T (2017) Statcheck does not work: All the numbers. Reply to Nuijten et al. (2017). PsyArXiv. http://psyarxiv.com/hr6qy .
Shanahan D (2016) A peerless review? Automating methodological and statistical review. Springer Nature BioMed Central, Research in progress blog. Available at: https://blogs.biomedcentral.com/bmcblog/2016/05/23/peerless-review-automating-methodological-statistical-review Accessed 6 Jan 2020
Siler K, Lee K, Bero L (2015) Measuring the effectiveness of scientific gatekeeping. Proc Natl Acad Sci 112:360–365
Article ADS CAS Google Scholar
Sonntag D (2004) Assessing the quality of natural language text data. In: Dadam P, Reichert M (eds) GI Jahrestagung. pp. 259–263
Spezi V et al. (2018) Let the community decide? The vision and reality of soundness-only peer review in open-access mega-journals. J Document 74:137–161
SWEOR (2019) 27 eye-opening website statistics: is your website costing you clients? URL https://www.sweor.com/firstimpressions , Accessed 20 Dec 2019
Tennant JP (2018) The state of the art in peer review. FEMS Microbiol Lett 365(19). https://doi.org/10.1093/femsle/fny204 .
Tennant JP, Dugan JM, Graziotin D et al. (2017) A multi-disciplinary perspective on emergent and future innovations in peer review. F1000Res 6:1151. https://doi.org/10.12688/f1000research.12037.3
Wood TJ et al. (2018) Can physician examiners overcome their first impression when examinee performance changes? Adv Health Sci Educ 23:721–732
Zarsky T (2016) The trouble with algorithmic decisions: an analytic road map to examine efficiency and fairness in automated and opaque decision making. Sci Technol Human Value 41:118–132
Download references
Author information
Authors and affiliations.
Information School, The University of Sheffield, Sheffield, UK
Alessandro Checco & Stephen Pinfield
Department of Electronic Engineering, University of Rome Tor Vergata, Rome, Italy
Lorenzo Bracciale, Pierpaolo Loreti & Giuseppe Bianchi
You can also search for this author in PubMed Google Scholar
Corresponding authors
Correspondence to Alessandro Checco , Lorenzo Bracciale or Stephen Pinfield .
Ethics declarations
Competing interests.
The authors declare no competing interests.
Additional information
Publisher’s note Springer Nature remains neutral with regard to jurisdictional claims in published maps and institutional affiliations.
Rights and permissions
Open Access This article is licensed under a Creative Commons Attribution 4.0 International License, which permits use, sharing, adaptation, distribution and reproduction in any medium or format, as long as you give appropriate credit to the original author(s) and the source, provide a link to the Creative Commons license, and indicate if changes were made. The images or other third party material in this article are included in the article’s Creative Commons license, unless indicated otherwise in a credit line to the material. If material is not included in the article’s Creative Commons license and your intended use is not permitted by statutory regulation or exceeds the permitted use, you will need to obtain permission directly from the copyright holder. To view a copy of this license, visit http://creativecommons.org/licenses/by/4.0/ .
Reprints and permissions
About this article
Cite this article.
Checco, A., Bracciale, L., Loreti, P. et al. AI-assisted peer review. Humanit Soc Sci Commun 8 , 25 (2021). https://doi.org/10.1057/s41599-020-00703-8
Download citation
Received : 23 December 2019
Accepted : 29 October 2020
Published : 25 January 2021
DOI : https://doi.org/10.1057/s41599-020-00703-8
Share this article
Anyone you share the following link with will be able to read this content:
Sorry, a shareable link is not currently available for this article.
Provided by the Springer Nature SharedIt content-sharing initiative
This article is cited by
Artificial intelligence and illusions of understanding in scientific research.
- Lisa Messeri
- M. J. Crockett
Nature (2024)
A GAN-BERT based decision making approach in peer review
- Imen Boukhris
- Chayma Zaâbi
Social Network Analysis and Mining (2024)
Emerging plagiarism in peer-review evaluation reports: a tip of the iceberg?
- Mikołaj Piniewski
- Zbigniew W. Kundzewicz
Scientometrics (2024)
Artificial intelligence is the new chief editor of Critical Care (maybe?)
- Michele Salvagno
- Fabio Silvio Taccone
Critical Care (2023)
The role of the big geographic sort in online news circulation among U.S. Reddit users
- Lia Bozarth
- Daniele Quercia
- Sanja Šćepanović
Scientific Reports (2023)
Quick links
- Explore articles by subject
- Guide to authors
- Editorial policies

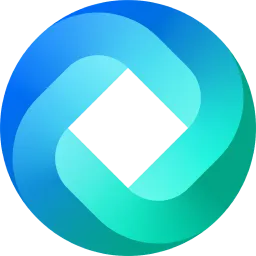
AI Literature Review Generator
Generate high-quality literature reviews fast with ai.
- Academic Research: Create a literature review for your thesis, dissertation, or research paper.
- Professional Research: Conduct a literature review for a project, report, or proposal at work.
- Content Creation: Write a literature review for a blog post, article, or book.
- Personal Research: Conduct a literature review to deepen your understanding of a topic of interest.
New & Trending Tools
Brand management tutor, lesson plan maker, marketing research tutor.
DevOpsSchool.com
Hix essaygpt review: the ai essay writing copilot for academic excellence.
In a world increasingly driven by artificial intelligence, the integration of AI in the realm of academic writing has opened up new avenues for students, researchers, and academics alike. HIX EssayGPT stands at the forefront of this innovation, offering a suite of tools designed to enhance the essay writing process. This review seeks to explore the myriad ways in which HIX EssayGPT serves as a copilot in academic writing, its capabilities in generating and refining essays, and the broader implications of AI assistance in academic integrity and research.
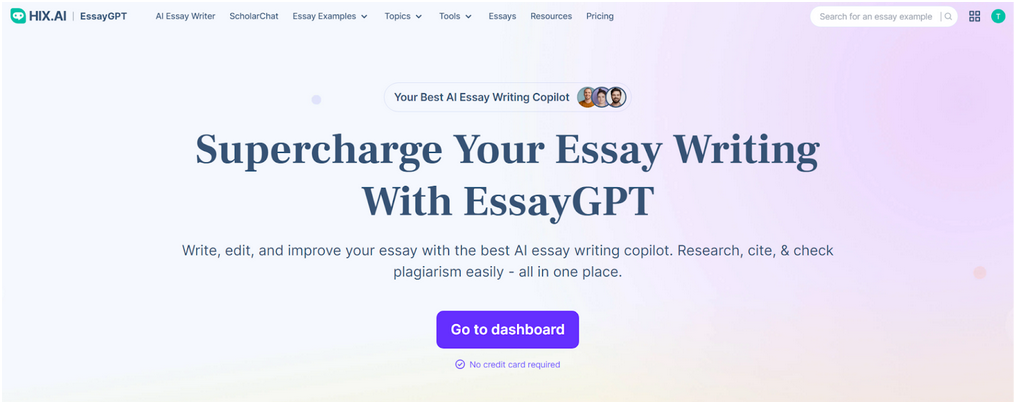
Table of Contents
Understanding HIX EssayGPT: The AI-powered Academic Companion
At its core, HIX EssayGPT is an AI essay writer developed by HIX.AI to assist in all facets of academic writing. From generating initial drafts to refining and polishing a final piece, it serves an array of functionalities aimed at easing the often daunting task of essay writing. But what sets HIX EssayGPT apart in a sea of digital tools and resources?
The Mechanics Behind the Magic
HIX EssayGPT operates on a sophisticated AI platform capable of understanding and generating text across a wide range of topics and academic disciplines. It doesn’t simply regurgitate information but is designed to analyze, synthesize, and present arguments in a coherent and academically sound manner.
Generative Capabilities
From creating outlines to drafting comprehensive essays, HIX EssayGPT’s generative capabilities are designed to save time and foster creativity among students and scholars. It generates content that can be seamlessly integrated into the writing process, tailored to the specific requirements of various academic fields.
Refined Customization
What makes HIX EssayGPT particularly intriguing is its ability to adapt its writing style to suit the needs of individual users. Whether it’s crafting a narrative, argumentative, or analytical essay, the tool provides a structured approach that can be customized to match the user’s tone, style, and argumentative flow.
Bridging the Gap: HIX EssayGPT’s Role in Academic Writing
Navigating the landscape of academic writing can be challenging, with hurdles ranging from writer’s block to the rigorous demand for original, well-researched content. HIX EssayGPT proposes a novel solution to these challenges.
Overcoming Writer’s Block
HIX EssayGPT offers an antidote to writer’s block by generating ideas, prompts, and content that can spark creativity and facilitate the writing process. Its ability to provide real-time suggestions and edits enables a smoother writing experience, free from the stalls of cognitive overload.
Research Assistance
Among its standout features is ScholarChat, a tool designed to simplify the research process. By accessing an extensive database of scholarly articles, HIX EssayGPT makes it possible for users to integrate credible sources and citations directly into their essays, thereby enriching the content with authoritative voices and evidence.
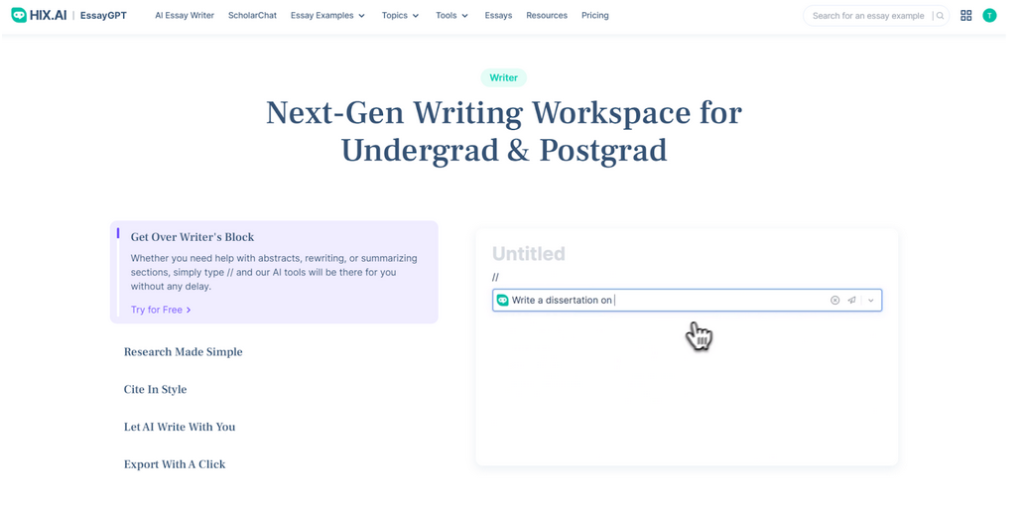
The Essay Shortener
HIX EssayGPT’s Essay Shortener tool is designed to streamline academic writing by condensing essays into more concise versions without losing the essence. It efficiently eliminates redundant content, ensuring the final product retains its critical arguments and main points, making it an indispensable tool for enhancing readability and adhering to word count requirements.
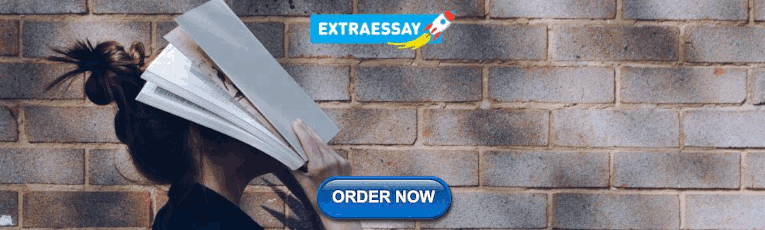
Ethical Considerations and Academic Authenticity
In an era where the line between AI-generated content and human creativity is increasingly blurred, HIX EssayGPT recognizes the significance of maintaining academic integrity. The tool encourages users to use AI-generated drafts as a foundation upon which to build and refine their original ideas, promoting a collaborative rather than substitutive approach to AI assistance in writing.
Encouraging Original Thought
HIX EssayGPT’s aim is not to replace the student or researcher but to act as a catalyst for original thought and writing. It emphasizes the importance of adding a personal touch to AI-generated drafts, thereby ensuring that the final submission is a product of the user’s own analysis, reflection, and creativity.
Conclusion: The Prospective Horizon of AI in Academic Writing
HIX EssayGPT signifies a leap towards the integration of AI into the academic domain, offering a glimpse into the potential for AI to augment human capabilities rather than replace them. Its array of tools, from essay generators to research assistants, positions HIX EssayGPT as a valuable ally in the pursuit of academic excellence.
While there are legitimate concerns regarding the ethical use of AI in academic writing, platforms like HIX EssayGPT highlight the potential for a balanced coexistence between artificial intelligence and human creativity. As we tread further into the realm of AI-assisted education, the focus should not solely rest on the capabilities of these technologies but also on fostering a responsible approach to their use, ensuring that the essence of academic writing—critical thinking, analysis, and originality—remains at the forefront of education.
Recent Posts
- Top 10 AI Tutor Platforms: Revolutionizing Homework Help with Free AI Homework Solvers May 29, 2024
- HIX EssayGPT Review: The AI Essay Writing Copilot for Academic Excellence May 29, 2024
- How I sold my apartment to invest in bonds May 29, 2024
- icinga: Commands May 27, 2024
- icinga: icinga object types May 27, 2024
- Adobe Photoshop 1
- Amazon Web Services 28
- Angular JS 4
- AnthillPro 1
- Apache Ant 48
- Apache Continuum 3
- App / Web Servers 17
- Artifactory 5
- Artificial Intelligence 13
- Bangalore, India 1
- Batch Scripting 2
- Big Data 15
- Bootstrap 4
- Build Management 13
- Build Management & Tools 5
- Build Tools 17
- BuildForge 4
- ClearCase 5
- CLOUD COMPUTING AND OPERATING SOFTWARE 48
- Code Analysis 17
- Code Coverage 7
- Concept & Definition 34
- CONTAINERS 11
- Continuous Delivery 3
- Continuous Inspection 1
- Continuous Integration 10
- Data Analytics 15
- Data Science 15
- Deep Learning 17
- DevOps Community 30
- DevOps Practice and Process 53
- DevOps Tooling 24
- DevOpsSchool 17
- DevSecOps 14
- Elastic Stack 20
- Elasticsearch 9
- Framework 11
- General 410
- Hacking & Security 2
- Hyderabad, India 1
- IDEs and Editor 8
- Incident and Bug Tracking Systems 2
- Internet of things 20
- Interview Questions & Answers 120
- IT Infrastructure Monitoring Tools 12
- Javascript 22
- Kubernetes 82
- Laravel 164
- Linkedin Groups 2
- Log Monitoring Tools 1
- Machine Learning 17
- Microservices 22
- monitoring 2
- Multimedia 26
- Netherland, Amsterdam 6
- Networking 18
- News & Events 1
- Octopus Deploy 12
- OpenShift 4
- OpenStake 1
- Operating Systems 10
- Package Management 4
- Perforce 100
- Php Valiable 14
- Practice of Software Configuration Management 24
- Programming Scripting Languages 41
- Prometheus 11
- Quality Management 2
- Release Concept 2
- Release, News & Updates 16
- Repository Manager Tools 1
- REST API 18
- Reveal JS 3
- Robotics 19
- Scripting 23
- Security Practices 1
- Security Tools 10
- sh404SEF custom content 1
- Shell Script 117
- Shortcuts 3
- Site Reliability Engineering 12
- Social Media 31
- Software Engineering 2
- SonarQube 12
- SonaType Nexus 7
- Source Code Management 6
- SVN Subversion 16
- Team Foundation Server 1
- Teamcity 12
- Tech Jobs 3
- Teradata 18
- Teradata Database 10
- Terraform 59
- Test Coverage Tools 3
- Testing Tools 23
- Tips and Tricks 57
- Tools Comparison 21
- Trainers 10
- Training 70
- Uncategorised 3,303
- UrbanCode Deploy 3
- Version Control Systems 11
- Virtualization 8
- Websites 23
Answers AWS Best Build certification Configuration Devops DevOpsSchool Docker Google Guide How Interview Interview Questions & Answers Jenkins LARAVEL Linux list Online Perforce Php Process Questions SEO software Tools Top Training Tutorial Tutorials
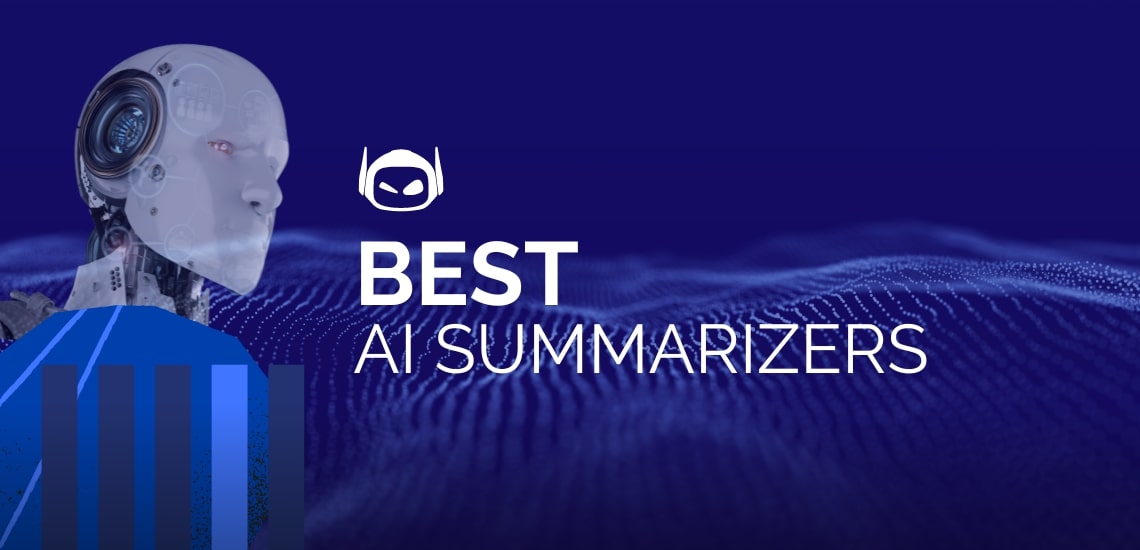
15 Best AI Summarizers
- Smodin Editorial Team
- Published: May 30, 2024
When you’re doing in-depth research, reading through an entire document can be a real chore, especially if you need to do it quickly and effectively. Thankfully, AI summarizers are here to help!
AI summarizers use AI technology to concisely summarize lengthy articles, web pages, or research papers. They pull all the key points, so you don’t have to.
We’re talking about platforms like Smodin, Jasper, and QuillBot, which are some of the best summary generators out there. But there are other tools that may spark your interest, too, so this guide will explore the benefits, limitations, and features of the 15 best AI summarizes available in the market.
Here’s a quick snapshot of the best AI summarizers:
- Hypotenuse AI
- Any Summary
- Semrush AI Summarizer
- Summarizer,org
1. Smodin – Best Overall
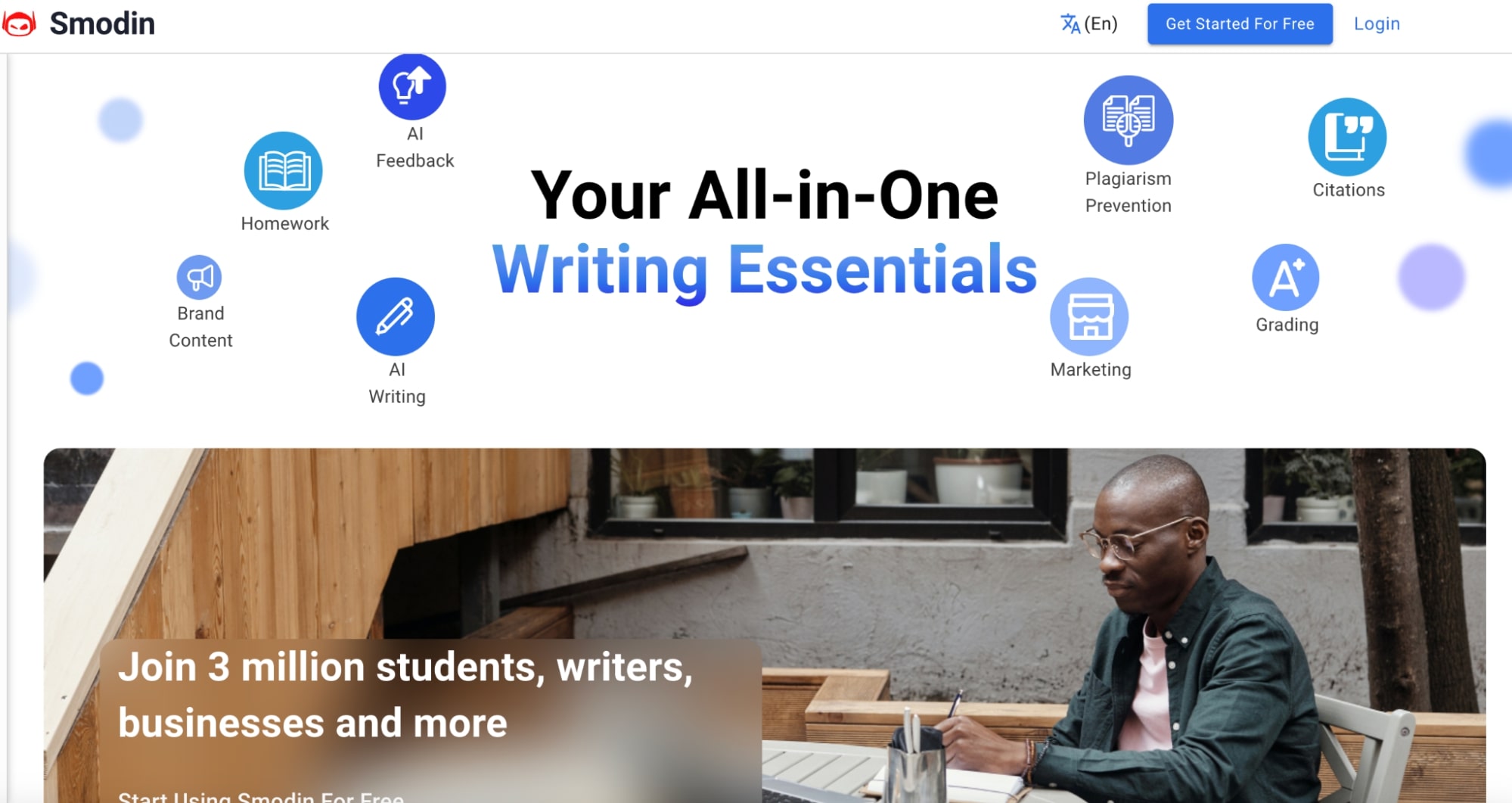
We should also mention that Smodin has the ability to support multiple languages. This means that users across the globe can benefit from its advanced technology.
- Smodin Writer – an AI writer that can quickly produce elegantly structured content.
- Multi-language support, including over 50 languages.
- Integrates directly with platforms like Microsoft Word and Google Docs.
- Offers a free mode with basic functionalities.
- MLA and APA citation generation.
- Easy-to-use tool with a clean interface.
- Fast processing speed for generating summaries.
- Accessible across multiple devices and platforms.
- The free version has limited features.
- Sometimes oversimplifies complex content.
System compatability: Windows, macOS, Linux, iOS, Android
2. Jasper – Best For Advanced Summary Features
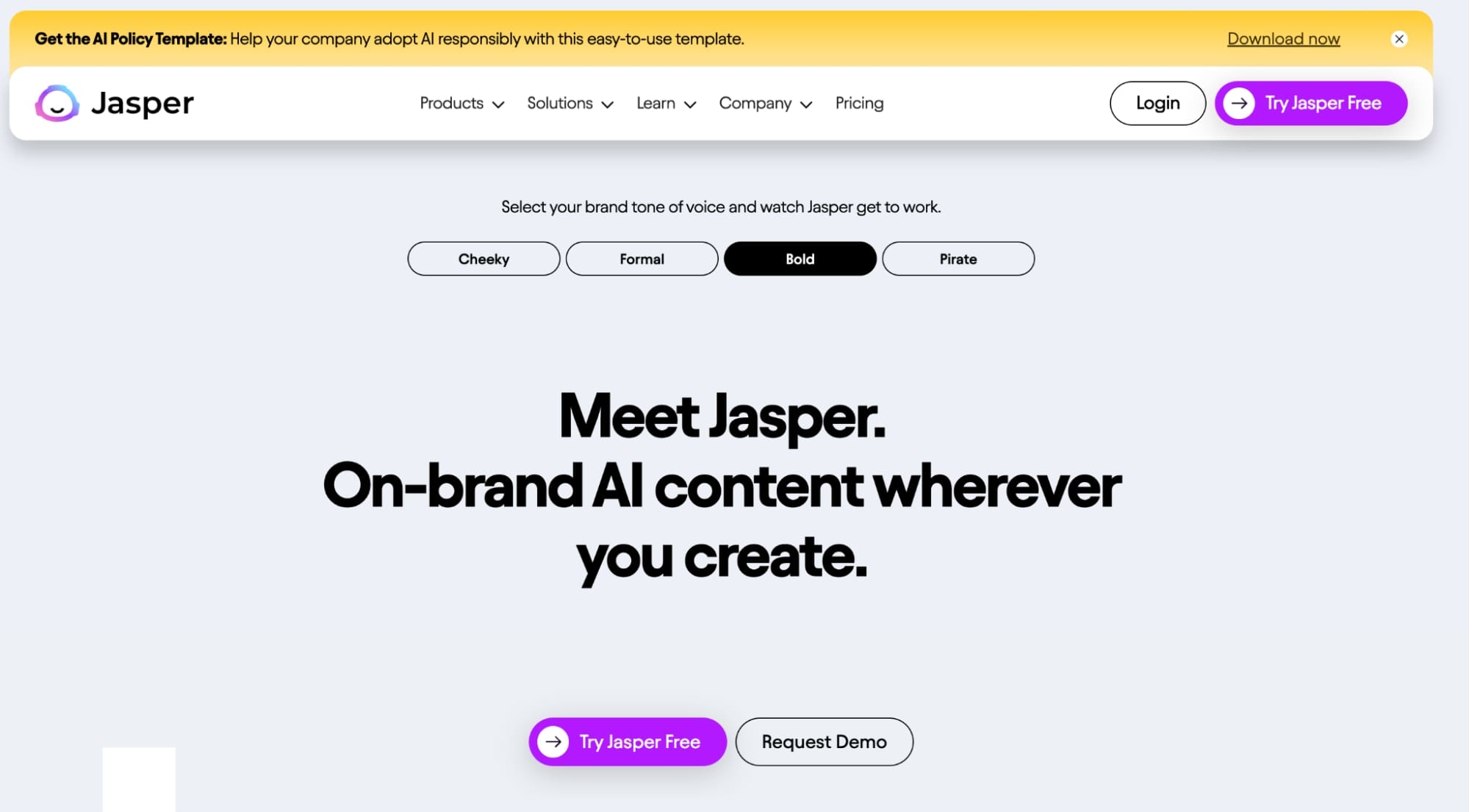
Users can input text, choose their target audience, and generate summaries without breaking a sweat. It’s another platform that’s well-suited for busy professionals and students.
- Customizable brand voice for a consistent tone.
- Templates for specific summary formats.
- Team collaboration and company knowledge system.
- Saves time with quick, accurate summaries.
- Repurposes summaries for social media or emails.
- Could impact document import capabilities.
- Premium plan is needed for extra features.
System compatability: Web browser, Google Docs, Chrome extension
3. QuillBot – Best For Versatility
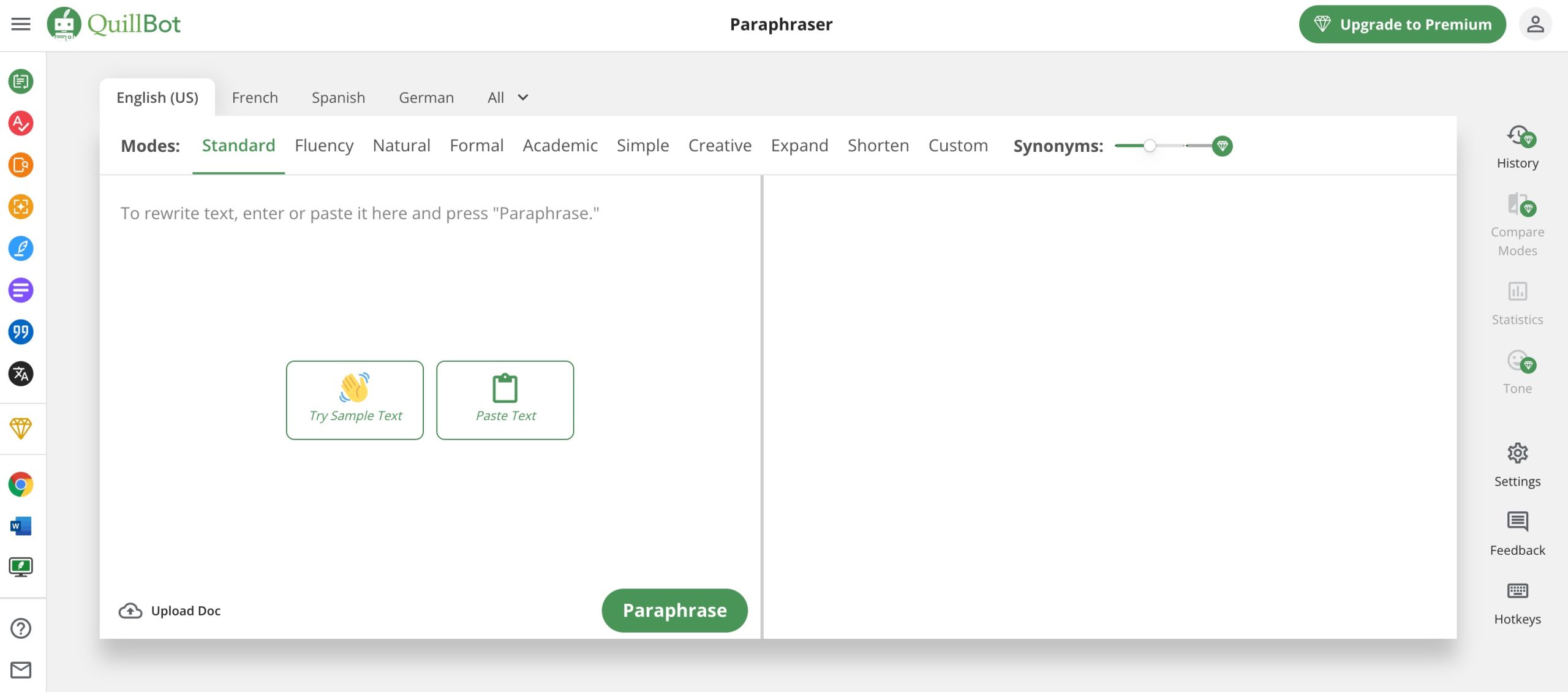
It’s also worth mentioning that this summarizing tool uses natural language processing (NLP) to accurately extract key points while maintaining the original context.
- Summary mode selection.
- Grammar checking tools.
- Free version with unlimited summarization.
- Simple writing process.
- Different modes for tailored summaries.
- The free version lacks advanced functionalities.
- Needs Microsoft Word or Chrome extension for seamless workflow.
System compatability: Web browser, Microsoft Word, Chome extension
4. Frase.io – Best For Handling Various Content Types
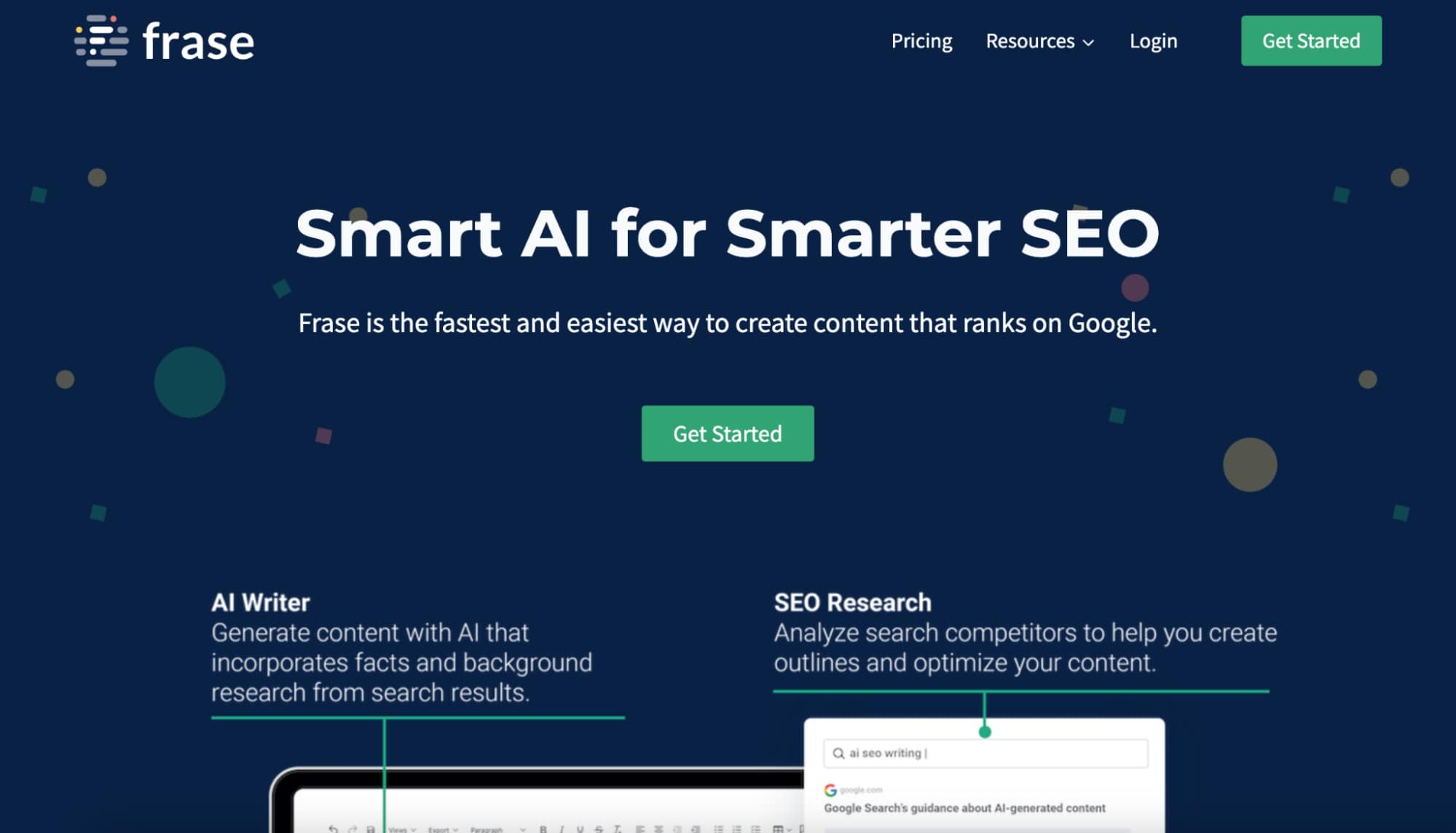
The tool is ideal for bloggers, marketers, and researchers who need effective summaries for various content types. With it, readers can grasp important points in no time.
- Efficiently solves writer’s block with suggestions.
- Offers concise summaries to come before calls to action.
- Uses BLUF (Bottom Line Up Front) and Inverted Pyramid summary techniques.
- Easy to use for writing process productivity.
- Generates accurate summaries with minimal editing.
- Limited to 700 words per input.
- Needs refinement for niche topics.
- Full access to advanced algorithms requires a premium plan.
System compatability: Web browser
5. Scholarcy – Best For Summarizing Long-form Documents
If you’re a student, scholar, or researcher, Scholarcy’s AI summary generator tool might be a good option. It creates structured summaries using flashcards, highlighting key concepts and allowing users to either skim or dive deep.
Scholarcy also has a pretty fun interface but many users cite it as lacking more advanced features to take summaries to another level. But, it still does a good job of breaking down complex content into simple summaries.
- Identifies key terms and findings in academic papers.
- Supports PDFs, book chapters, online articles, and more.
- Provides snapshot summaries for quick reading.
- Free version available for up to three summaries daily.
- Accurately identifies essential points.
- Advanced features require a Scholarcy Plus plan.
- Doesn’t summarize documents over a certain size.
System compatability: Web browser, Chrome extension
6. genei – Best For Detailed Summaries
genei isn’t exactly the most exciting summary tool on our list but this doesn’t mean it should be ignored. It still does a good job of empowering students, professionals, and researchers to speed up their research and content production. genei summarizes documents, analyzes web pages, and extracts keywords. So, with it, you’ll have no trouble finding crucial information quickly.
- Summarization for PDFs and web pages.
- Multi-document summarization and question answering.
- Advanced GPT-3-based language generation.
- Offers a free trial and basic plan.
- Facilitates advanced research with customizable folders.
- Chrome extension allows for content to be saved.
- Premium features are only accessible in genei Pro.
- Some features may need to be worked on for very specific research topics.
7. Notta – Best For Summarizing Non-text Content (Audio, Video, Calls, etc.)
Notta’s AI summary tool can turn audio and video content into rich summaries in just a few clicks. It works well for meetings, interviews, and podcasts. Notta automatically generates actionable chapters and the most important points. This helps a lot in terms of taking in a lot of content in a short timeframe.
Notta might be a good solution if you’re an educator, content creator, or part of a business team.
- Transcribes and summarizes video and audio files.
- Generates chapters and key action items.
- AI templates for various meeting types.
- Advanced summary creation for efficient content digestion.
- Streamlines summarization across multiple platforms.
- Free plan offers very limited transcription length.
- Advanced templates require a subscription plan.
- Not suitable for transcribing poor-quality audio.
System compatability: Web browser, Mobile app, Chrome extension
8. Glasp – Best For Summarizing User Notes
For anyone looking to highlight important quotes from web pages and PDFs, Glasp’s AI summary tool is your answer. Its AI-generated summaries are personalized according to your highlights and notes. This makes it a great tool for learners, as it helps them retain key insights and share their findings. It also helps them connect with like-minded individuals.
- AI summarization of web pages, PDFs, and YouTube videos.
- Knowledge graph for visualizing learning progress.
- Integrates with Kindle, Roam Research, and other apps.
- Offers a free version with decent features.
- Enables meaningful connections through social learning.
- Full range of features may be complex for new users.
- Requires Chome or Safari extension for full features.
- Some users may find the community feed to be overwhelming.
System compatability: Web browser, Chrome extension, Safari extension
9. Hypotenuse AI – Best For Removing Unnecessary Information
Hypotenuse AI’s summarizing tool doesn’t falter when it comes to providing accurate summaries for paragraphs, articles, and videos. It simplifies long text by condensing the essential information into concise bullet points or paragraphs through advanced AI. The great thing about it is that it does a good job of cutting through fluff, providing a clear view of what matters.
- Offers a free trial.
- Summarizes up to 200,000 characters (50,000 words).
- Advanced AI analyzes and generates summaries from scratch.
- Optimized for repurposing into social media posts or infographics.
- Reduces the writing process into simple steps.
- Lets writers refine content with better key points.
- Full features require a premium plan.
- Doesn’t accept documents over 50,000 words.
- May require editing for technical accuracy.
System compatability: Web browser, integrates with Shopify and WordPress
10. Sharly AI – Best For Concise Summaries
Sharly AI is another comprehensive summarizing tool. It can handle articles, PDFs, and many document formats. With its AI summary generators, you can quickly get a summary that does a good job of balancing brevity and detail. Overall, this tool is best suited for professionals who need a to-the-point summary.
- Supports multiple file types.
- Allows cross-document analysis for improved insights.
- Customizable summary formats and lengths.
- Generate accurate summaries in just minutes.
- Saves academics and professionals significant time.
- Advanced features are only part of the premium plan.
- Summary quality may vary depending on the source material.
- Limited compatibility with non-text-based documents.
11. Wordtune – Best For Smart Translation
Wordtune is another solid option, although arguably not as popular as the ones mentioned earlier. It does a good of summarizing documents, articles, and YouTube videos, helping users maximize productivity. With section summaries and a personalized library, Wordtune ensures a streamlined workflow for researchers looking to cut down on analysis time.
- Browser extension for summarizing a web page.
- Offers notes for research points and insights.
- Stores links, video files, and summaries in a personal library.
- Allows users to expand on specific sections for detailed exploration.
- Enhances the research process with organized summaries.
- Integrates well with browser extensions for seamless working.
- Focused mainly on English content.
- Advanced features require a paid subscription.
- May require further editing for detailed reports.
System compatability: Web browser, Chrome extension, Microsoft Edge extension
12. Any Summary – Best For Various Input Methods
The name says it all really. Any Summary can generate tight summaries for various documents, audio, and video files. By accepting multiple formats and letting users choose between bullet points or abstracts, this tool simplifies lengthy content. It can work well for a variety of people but researchers and professionals will typically get the most out of Any Summary.
- Powered by ChatGPT for more accurate insights.
- Accepts URLs and files up to 100 MB in size.
- Supports personalized summary instructions or automated AI summaries.
- Handles many different files for versatile usage.
- Customizable summaries offer greater control.
- Quite a number of features require a paid plan.
- File size limits can be frustrating depending on the format.
System compatability: Web browser.
13. Semrush AI Summarizer – Best For Customizable Summary Format
Semrush has thrown its hat in the AI arena and has been doing a pretty good job since. Their AI generator transforms articles, reports, and paragraphs into very digestible summaries. It can simplify content into either paragraph or bullet-point formats – nothing incredibly ground-breaking.
One of its greatest features is that it allows users to customize summaries based on their preferred length and style.
- Summarizes any length of content with no character limit.
- Adjustable summary length: short, medium, long.
- Free to use, no limits.
- Provides plagiarism-free summaries.
- Allows users to own and freely use their generated summaries.
- Currently only supports English.
- May need manual review for industry-specific jargon.
14. Summarizer.org – Best For Simplicity
Summarizer.org doesn’t have that much to offer in terms of features. It’s simple but it does simple very well. Much like QuillBot, it uses natural language processing techniques to provide brief summaries for many types of content. The tool easily identifies the main points from the text, providing outputs that are easily digestible. Users also get to control the length and structure of the output to fit their needs.
- Summarizes text in multiple languages.
- Generates bullet-point summaries or one-line statements.
- Provides word count before and after summarization.
- Provides ample flexibility for adjusting the summary length.
- Ads on the website.
- Limited amount of features.
15. BooksAI – Best Free Summarizer
And last but not least, we have BooksAI. It provides AI-generated book summaries and recommendations, both powered by ChatGPT.
The app does well at condensing complex ideas and helping readers find their next book based on their preferences, which could be very useful in academia. It also lets you create a personalized reading list and explore book summaries in various languages.
- Offer summaries for over 40 million books.
- Provides spoiler-free synopses for popular books.
- Supports summaries in nine languages.
- Expands reading choices with curated suggestions.
- Supports multi-language summaries for global accessibility.
- Requires app installation for full access.
- Focused mainly on popular book titles.
- Some features require user data sharing.
System compatability: iOS, Android
How to Choose an AI Summarizer Tool
We’ve given you a lot of AI tools to choose from. So, you might be a little confused about how to settle on one text summarizer tool. Here are some key features you should pay attention to:
- Summary format options: An AI summarizer should provide lots of formatting options, like bullet points, paragraphs, and abstracts. This flexibility will help you meet different project needs.
- Accuracy and relevance: Ensure the tool generates accurate summaries that capture the main points without mixing up the original meaning.
- Multi-language support: If your documents are in multiple languages, look for a summarizer offering translation. Multi-language summaries are also a great bonus.
- Customization and length control: Choose an AI summarizer that lets you adjust the summary length to suit specific needs.
- Data privacy: The tool must protect your data to prevent unauthorized access or misuse.
- Pricing: Consider tools that offer a free version or at least a trial period to test its features before signing up for a paid plan.
Summarize and More with Smodin
Studies have found that 88% of students believe that taking notes is key to academic success. But remember that taking notes is the easy part, the hard part is summing them up to determine what’s most important.
You could spend countless hours summarizing your notes and conducting research, or you could let AI do the heavy lifting for you. Save yourself the headache – let a tool like Smodin change the way you summarize, forever!
Smodin is an AI tool that many others simply can’t measure up to. Its advanced algorithms and versatility make it an ideal AI summary writer. Offering key features like customizable summary formats and citation generation, Smodin is pretty much an academic’s best friend.
You can use its summarizer to adjust the summary length and format for detailed reports or quick bullets. This flexibility ensures accuracy, whether you’re handling academic texts, business reports, or creative projects.
Plus, Smodin’s comprehensive language support and automated plagiarism detection make it one of the best AI tools available.
Subscribe to the PwC Newsletter
Join the community, edit social preview.
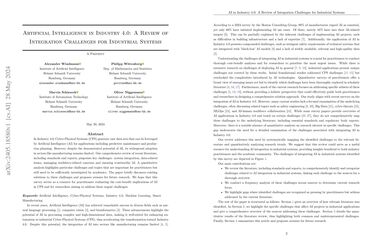
Add a new code entry for this paper
Remove a code repository from this paper, mark the official implementation from paper authors, add a new evaluation result row, remove a task, add a method, remove a method, edit datasets, artificial intelligence in industry 4.0: a review of integration challenges for industrial systems.
28 May 2024 · Alexander Windmann , Philipp Wittenberg , Marvin Schieseck , Oliver Niggemann · Edit social preview
In Industry 4.0, Cyber-Physical Systems (CPS) generate vast data sets that can be leveraged by Artificial Intelligence (AI) for applications including predictive maintenance and production planning. However, despite the demonstrated potential of AI, its widespread adoption in sectors like manufacturing remains limited. Our comprehensive review of recent literature, including standards and reports, pinpoints key challenges: system integration, data-related issues, managing workforce-related concerns and ensuring trustworthy AI. A quantitative analysis highlights particular challenges and topics that are important for practitioners but still need to be sufficiently investigated by academics. The paper briefly discusses existing solutions to these challenges and proposes avenues for future research. We hope that this survey serves as a resource for practitioners evaluating the cost-benefit implications of AI in CPS and for researchers aiming to address these urgent challenges.
Code Edit Add Remove Mark official
Tasks edit add remove, datasets edit, results from the paper edit add remove, methods edit add remove.
A systematic literature review of empirical research on ChatGPT in education
- Open access
- Published: 26 May 2024
- Volume 3 , article number 60 , ( 2024 )
Cite this article
You have full access to this open access article
- Yazid Albadarin ORCID: orcid.org/0009-0005-8068-8902 1 ,
- Mohammed Saqr 1 ,
- Nicolas Pope 1 &
- Markku Tukiainen 1
237 Accesses
Explore all metrics
Over the last four decades, studies have investigated the incorporation of Artificial Intelligence (AI) into education. A recent prominent AI-powered technology that has impacted the education sector is ChatGPT. This article provides a systematic review of 14 empirical studies incorporating ChatGPT into various educational settings, published in 2022 and before the 10th of April 2023—the date of conducting the search process. It carefully followed the essential steps outlined in the Preferred Reporting Items for Systematic Reviews and Meta-Analyses (PRISMA 2020) guidelines, as well as Okoli’s (Okoli in Commun Assoc Inf Syst, 2015) steps for conducting a rigorous and transparent systematic review. In this review, we aimed to explore how students and teachers have utilized ChatGPT in various educational settings, as well as the primary findings of those studies. By employing Creswell’s (Creswell in Educational research: planning, conducting, and evaluating quantitative and qualitative research [Ebook], Pearson Education, London, 2015) coding techniques for data extraction and interpretation, we sought to gain insight into their initial attempts at ChatGPT incorporation into education. This approach also enabled us to extract insights and considerations that can facilitate its effective and responsible use in future educational contexts. The results of this review show that learners have utilized ChatGPT as a virtual intelligent assistant, where it offered instant feedback, on-demand answers, and explanations of complex topics. Additionally, learners have used it to enhance their writing and language skills by generating ideas, composing essays, summarizing, translating, paraphrasing texts, or checking grammar. Moreover, learners turned to it as an aiding tool to facilitate their directed and personalized learning by assisting in understanding concepts and homework, providing structured learning plans, and clarifying assignments and tasks. However, the results of specific studies (n = 3, 21.4%) show that overuse of ChatGPT may negatively impact innovative capacities and collaborative learning competencies among learners. Educators, on the other hand, have utilized ChatGPT to create lesson plans, generate quizzes, and provide additional resources, which helped them enhance their productivity and efficiency and promote different teaching methodologies. Despite these benefits, the majority of the reviewed studies recommend the importance of conducting structured training, support, and clear guidelines for both learners and educators to mitigate the drawbacks. This includes developing critical evaluation skills to assess the accuracy and relevance of information provided by ChatGPT, as well as strategies for integrating human interaction and collaboration into learning activities that involve AI tools. Furthermore, they also recommend ongoing research and proactive dialogue with policymakers, stakeholders, and educational practitioners to refine and enhance the use of AI in learning environments. This review could serve as an insightful resource for practitioners who seek to integrate ChatGPT into education and stimulate further research in the field.
Similar content being viewed by others
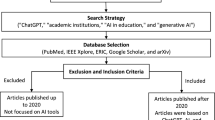
Empowering learners with ChatGPT: insights from a systematic literature exploration
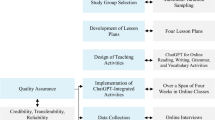
Incorporating AI in foreign language education: An investigation into ChatGPT’s effect on foreign language learners
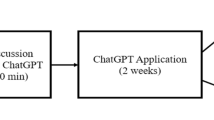
Large language models in education: A focus on the complementary relationship between human teachers and ChatGPT
Avoid common mistakes on your manuscript.
1 Introduction
Educational technology, a rapidly evolving field, plays a crucial role in reshaping the landscape of teaching and learning [ 82 ]. One of the most transformative technological innovations of our era that has influenced the field of education is Artificial Intelligence (AI) [ 50 ]. Over the last four decades, AI in education (AIEd) has gained remarkable attention for its potential to make significant advancements in learning, instructional methods, and administrative tasks within educational settings [ 11 ]. In particular, a large language model (LLM), a type of AI algorithm that applies artificial neural networks (ANNs) and uses massively large data sets to understand, summarize, generate, and predict new content that is almost difficult to differentiate from human creations [ 79 ], has opened up novel possibilities for enhancing various aspects of education, from content creation to personalized instruction [ 35 ]. Chatbots that leverage the capabilities of LLMs to understand and generate human-like responses have also presented the capacity to enhance student learning and educational outcomes by engaging students, offering timely support, and fostering interactive learning experiences [ 46 ].
The ongoing and remarkable technological advancements in chatbots have made their use more convenient, increasingly natural and effortless, and have expanded their potential for deployment across various domains [ 70 ]. One prominent example of chatbot applications is the Chat Generative Pre-Trained Transformer, known as ChatGPT, which was introduced by OpenAI, a leading AI research lab, on November 30th, 2022. ChatGPT employs a variety of deep learning techniques to generate human-like text, with a particular focus on recurrent neural networks (RNNs). Long short-term memory (LSTM) allows it to grasp the context of the text being processed and retain information from previous inputs. Also, the transformer architecture, a neural network architecture based on the self-attention mechanism, allows it to analyze specific parts of the input, thereby enabling it to produce more natural-sounding and coherent output. Additionally, the unsupervised generative pre-training and the fine-tuning methods allow ChatGPT to generate more relevant and accurate text for specific tasks [ 31 , 62 ]. Furthermore, reinforcement learning from human feedback (RLHF), a machine learning approach that combines reinforcement learning techniques with human-provided feedback, has helped improve ChatGPT’s model by accelerating the learning process and making it significantly more efficient.
This cutting-edge natural language processing (NLP) tool is widely recognized as one of today's most advanced LLMs-based chatbots [ 70 ], allowing users to ask questions and receive detailed, coherent, systematic, personalized, convincing, and informative human-like responses [ 55 ], even within complex and ambiguous contexts [ 63 , 77 ]. ChatGPT is considered the fastest-growing technology in history: in just three months following its public launch, it amassed an estimated 120 million monthly active users [ 16 ] with an estimated 13 million daily queries [ 49 ], surpassing all other applications [ 64 ]. This remarkable growth can be attributed to the unique features and user-friendly interface that ChatGPT offers. Its intuitive design allows users to interact seamlessly with the technology, making it accessible to a diverse range of individuals, regardless of their technical expertise [ 78 ]. Additionally, its exceptional performance results from a combination of advanced algorithms, continuous enhancements, and extensive training on a diverse dataset that includes various text sources such as books, articles, websites, and online forums [ 63 ], have contributed to a more engaging and satisfying user experience [ 62 ]. These factors collectively explain its remarkable global growth and set it apart from predecessors like Bard, Bing Chat, ERNIE, and others.
In this context, several studies have explored the technological advancements of chatbots. One noteworthy recent research effort, conducted by Schöbel et al. [ 70 ], stands out for its comprehensive analysis of more than 5,000 studies on communication agents. This study offered a comprehensive overview of the historical progression and future prospects of communication agents, including ChatGPT. Moreover, other studies have focused on making comparisons, particularly between ChatGPT and alternative chatbots like Bard, Bing Chat, ERNIE, LaMDA, BlenderBot, and various others. For example, O’Leary [ 53 ] compared two chatbots, LaMDA and BlenderBot, with ChatGPT and revealed that ChatGPT outperformed both. This superiority arises from ChatGPT’s capacity to handle a wider range of questions and generate slightly varied perspectives within specific contexts. Similarly, ChatGPT exhibited an impressive ability to formulate interpretable responses that were easily understood when compared with Google's feature snippet [ 34 ]. Additionally, ChatGPT was compared to other LLMs-based chatbots, including Bard and BERT, as well as ERNIE. The findings indicated that ChatGPT exhibited strong performance in the given tasks, often outperforming the other models [ 59 ].
Furthermore, in the education context, a comprehensive study systematically compared a range of the most promising chatbots, including Bard, Bing Chat, ChatGPT, and Ernie across a multidisciplinary test that required higher-order thinking. The study revealed that ChatGPT achieved the highest score, surpassing Bing Chat and Bard [ 64 ]. Similarly, a comparative analysis was conducted to compare ChatGPT with Bard in answering a set of 30 mathematical questions and logic problems, grouped into two question sets. Set (A) is unavailable online, while Set (B) is available online. The results revealed ChatGPT's superiority in Set (A) over Bard. Nevertheless, Bard's advantage emerged in Set (B) due to its capacity to access the internet directly and retrieve answers, a capability that ChatGPT does not possess [ 57 ]. However, through these varied assessments, ChatGPT consistently highlights its exceptional prowess compared to various alternatives in the ever-evolving chatbot technology.
The widespread adoption of chatbots, especially ChatGPT, by millions of students and educators, has sparked extensive discussions regarding its incorporation into the education sector [ 64 ]. Accordingly, many scholars have contributed to the discourse, expressing both optimism and pessimism regarding the incorporation of ChatGPT into education. For example, ChatGPT has been highlighted for its capabilities in enriching the learning and teaching experience through its ability to support different learning approaches, including adaptive learning, personalized learning, and self-directed learning [ 58 , 60 , 91 ]), deliver summative and formative feedback to students and provide real-time responses to questions, increase the accessibility of information [ 22 , 40 , 43 ], foster students’ performance, engagement and motivation [ 14 , 44 , 58 ], and enhance teaching practices [ 17 , 18 , 64 , 74 ].
On the other hand, concerns have been also raised regarding its potential negative effects on learning and teaching. These include the dissemination of false information and references [ 12 , 23 , 61 , 85 ], biased reinforcement [ 47 , 50 ], compromised academic integrity [ 18 , 40 , 66 , 74 ], and the potential decline in students' skills [ 43 , 61 , 64 , 74 ]. As a result, ChatGPT has been banned in multiple countries, including Russia, China, Venezuela, Belarus, and Iran, as well as in various educational institutions in India, Italy, Western Australia, France, and the United States [ 52 , 90 ].
Clearly, the advent of chatbots, especially ChatGPT, has provoked significant controversy due to their potential impact on learning and teaching. This indicates the necessity for further exploration to gain a deeper understanding of this technology and carefully evaluate its potential benefits, limitations, challenges, and threats to education [ 79 ]. Therefore, conducting a systematic literature review will provide valuable insights into the potential prospects and obstacles linked to its incorporation into education. This systematic literature review will primarily focus on ChatGPT, driven by the aforementioned key factors outlined above.
However, the existing literature lacks a systematic literature review of empirical studies. Thus, this systematic literature review aims to address this gap by synthesizing the existing empirical studies conducted on chatbots, particularly ChatGPT, in the field of education, highlighting how ChatGPT has been utilized in educational settings, and identifying any existing gaps. This review may be particularly useful for researchers in the field and educators who are contemplating the integration of ChatGPT or any chatbot into education. The following research questions will guide this study:
What are students' and teachers' initial attempts at utilizing ChatGPT in education?
What are the main findings derived from empirical studies that have incorporated ChatGPT into learning and teaching?
2 Methodology
To conduct this study, the authors followed the essential steps of the Preferred Reporting Items for Systematic Reviews and Meta-Analyses (PRISMA 2020) and Okoli’s [ 54 ] steps for conducting a systematic review. These included identifying the study’s purpose, drafting a protocol, applying a practical screening process, searching the literature, extracting relevant data, evaluating the quality of the included studies, synthesizing the studies, and ultimately writing the review. The subsequent section provides an extensive explanation of how these steps were carried out in this study.
2.1 Identify the purpose
Given the widespread adoption of ChatGPT by students and teachers for various educational purposes, often without a thorough understanding of responsible and effective use or a clear recognition of its potential impact on learning and teaching, the authors recognized the need for further exploration of ChatGPT's impact on education in this early stage. Therefore, they have chosen to conduct a systematic literature review of existing empirical studies that incorporate ChatGPT into educational settings. Despite the limited number of empirical studies due to the novelty of the topic, their goal is to gain a deeper understanding of this technology and proactively evaluate its potential benefits, limitations, challenges, and threats to education. This effort could help to understand initial reactions and attempts at incorporating ChatGPT into education and bring out insights and considerations that can inform the future development of education.
2.2 Draft the protocol
The next step is formulating the protocol. This protocol serves to outline the study process in a rigorous and transparent manner, mitigating researcher bias in study selection and data extraction [ 88 ]. The protocol will include the following steps: generating the research question, predefining a literature search strategy, identifying search locations, establishing selection criteria, assessing the studies, developing a data extraction strategy, and creating a timeline.
2.3 Apply practical screen
The screening step aims to accurately filter the articles resulting from the searching step and select the empirical studies that have incorporated ChatGPT into educational contexts, which will guide us in answering the research questions and achieving the objectives of this study. To ensure the rigorous execution of this step, our inclusion and exclusion criteria were determined based on the authors' experience and informed by previous successful systematic reviews [ 21 ]. Table 1 summarizes the inclusion and exclusion criteria for study selection.
2.4 Literature search
We conducted a thorough literature search to identify articles that explored, examined, and addressed the use of ChatGPT in Educational contexts. We utilized two research databases: Dimensions.ai, which provides access to a large number of research publications, and lens.org, which offers access to over 300 million articles, patents, and other research outputs from diverse sources. Additionally, we included three databases, Scopus, Web of Knowledge, and ERIC, which contain relevant research on the topic that addresses our research questions. To browse and identify relevant articles, we used the following search formula: ("ChatGPT" AND "Education"), which included the Boolean operator "AND" to get more specific results. The subject area in the Scopus and ERIC databases were narrowed to "ChatGPT" and "Education" keywords, and in the WoS database was limited to the "Education" category. The search was conducted between the 3rd and 10th of April 2023, which resulted in 276 articles from all selected databases (111 articles from Dimensions.ai, 65 from Scopus, 28 from Web of Science, 14 from ERIC, and 58 from Lens.org). These articles were imported into the Rayyan web-based system for analysis. The duplicates were identified automatically by the system. Subsequently, the first author manually reviewed the duplicated articles ensured that they had the same content, and then removed them, leaving us with 135 unique articles. Afterward, the titles, abstracts, and keywords of the first 40 manuscripts were scanned and reviewed by the first author and were discussed with the second and third authors to resolve any disagreements. Subsequently, the first author proceeded with the filtering process for all articles and carefully applied the inclusion and exclusion criteria as presented in Table 1 . Articles that met any one of the exclusion criteria were eliminated, resulting in 26 articles. Afterward, the authors met to carefully scan and discuss them. The authors agreed to eliminate any empirical studies solely focused on checking ChatGPT capabilities, as these studies do not guide us in addressing the research questions and achieving the study's objectives. This resulted in 14 articles eligible for analysis.
2.5 Quality appraisal
The examination and evaluation of the quality of the extracted articles is a vital step [ 9 ]. Therefore, the extracted articles were carefully evaluated for quality using Fink’s [ 24 ] standards, which emphasize the necessity for detailed descriptions of methodology, results, conclusions, strengths, and limitations. The process began with a thorough assessment of each study's design, data collection, and analysis methods to ensure their appropriateness and comprehensive execution. The clarity, consistency, and logical progression from data to results and conclusions were also critically examined. Potential biases and recognized limitations within the studies were also scrutinized. Ultimately, two articles were excluded for failing to meet Fink’s criteria, particularly in providing sufficient detail on methodology, results, conclusions, strengths, or limitations. The review process is illustrated in Fig. 1 .
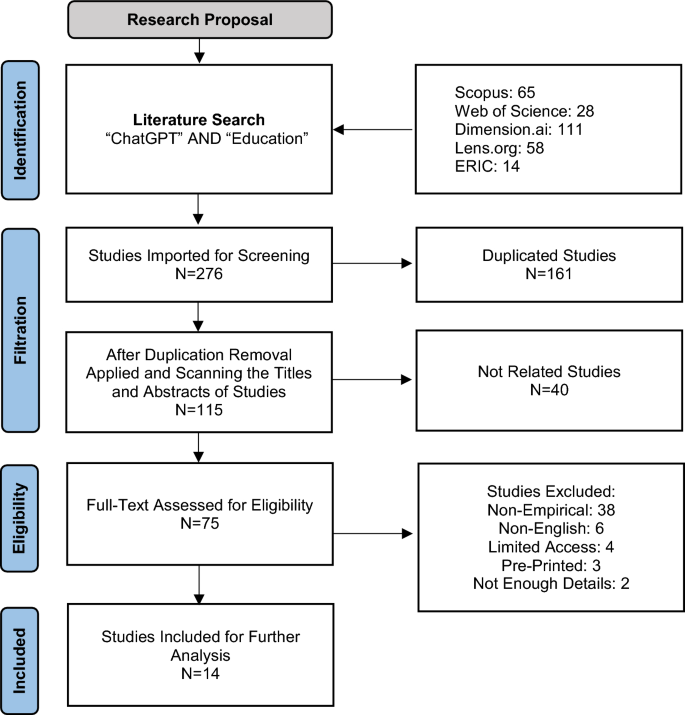
The study selection process
2.6 Data extraction
The next step is data extraction, the process of capturing the key information and categories from the included studies. To improve efficiency, reduce variation among authors, and minimize errors in data analysis, the coding categories were constructed using Creswell's [ 15 ] coding techniques for data extraction and interpretation. The coding process involves three sequential steps. The initial stage encompasses open coding , where the researcher examines the data, generates codes to describe and categorize it, and gains a deeper understanding without preconceived ideas. Following open coding is axial coding , where the interrelationships between codes from open coding are analyzed to establish more comprehensive categories or themes. The process concludes with selective coding , refining and integrating categories or themes to identify core concepts emerging from the data. The first coder performed the coding process, then engaged in discussions with the second and third authors to finalize the coding categories for the first five articles. The first coder then proceeded to code all studies and engaged again in discussions with the other authors to ensure the finalization of the coding process. After a comprehensive analysis and capturing of the key information from the included studies, the data extraction and interpretation process yielded several themes. These themes have been categorized and are presented in Table 2 . It is important to note that open coding results were removed from Table 2 for aesthetic reasons, as it included many generic aspects, such as words, short phrases, or sentences mentioned in the studies.
2.7 Synthesize studies
In this stage, we will gather, discuss, and analyze the key findings that emerged from the selected studies. The synthesis stage is considered a transition from an author-centric to a concept-centric focus, enabling us to map all the provided information to achieve the most effective evaluation of the data [ 87 ]. Initially, the authors extracted data that included general information about the selected studies, including the author(s)' names, study titles, years of publication, educational levels, research methodologies, sample sizes, participants, main aims or objectives, raw data sources, and analysis methods. Following that, all key information and significant results from the selected studies were compiled using Creswell’s [ 15 ] coding techniques for data extraction and interpretation to identify core concepts and themes emerging from the data, focusing on those that directly contributed to our research questions and objectives, such as the initial utilization of ChatGPT in learning and teaching, learners' and educators' familiarity with ChatGPT, and the main findings of each study. Finally, the data related to each selected study were extracted into an Excel spreadsheet for data processing. The Excel spreadsheet was reviewed by the authors, including a series of discussions to ensure the finalization of this process and prepare it for further analysis. Afterward, the final result being analyzed and presented in various types of charts and graphs. Table 4 presents the extracted data from the selected studies, with each study labeled with a capital 'S' followed by a number.
This section consists of two main parts. The first part provides a descriptive analysis of the data compiled from the reviewed studies. The second part presents the answers to the research questions and the main findings of these studies.
3.1 Part 1: descriptive analysis
This section will provide a descriptive analysis of the reviewed studies, including educational levels and fields, participants distribution, country contribution, research methodologies, study sample size, study population, publication year, list of journals, familiarity with ChatGPT, source of data, and the main aims and objectives of the studies. Table 4 presents a comprehensive overview of the extracted data from the selected studies.
3.1.1 The number of the reviewed studies and publication years
The total number of the reviewed studies was 14. All studies were empirical studies and published in different journals focusing on Education and Technology. One study was published in 2022 [S1], while the remaining were published in 2023 [S2]-[S14]. Table 3 illustrates the year of publication, the names of the journals, and the number of reviewed studies published in each journal for the studies reviewed.
3.1.2 Educational levels and fields
The majority of the reviewed studies, 11 studies, were conducted in higher education institutions [S1]-[S10] and [S13]. Two studies did not specify the educational level of the population [S12] and [S14], while one study focused on elementary education [S11]. However, the reviewed studies covered various fields of education. Three studies focused on Arts and Humanities Education [S8], [S11], and [S14], specifically English Education. Two studies focused on Engineering Education, with one in Computer Engineering [S2] and the other in Construction Education [S3]. Two studies focused on Mathematics Education [S5] and [S12]. One study focused on Social Science Education [S13]. One study focused on Early Education [S4]. One study focused on Journalism Education [S9]. Finally, three studies did not specify the field of education [S1], [S6], and [S7]. Figure 2 represents the educational levels in the reviewed studies, while Fig. 3 represents the context of the reviewed studies.
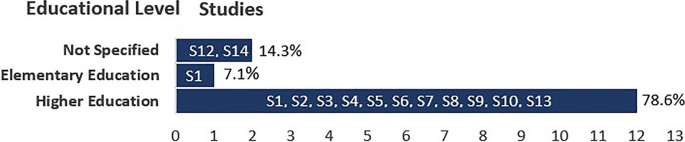
Educational levels in the reviewed studies
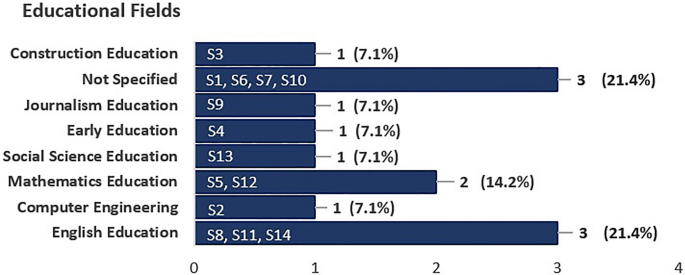
Context of the reviewed studies
3.1.3 Participants distribution and countries contribution
The reviewed studies have been conducted across different geographic regions, providing a diverse representation of the studies. The majority of the studies, 10 in total, [S1]-[S3], [S5]-[S9], [S11], and [S14], primarily focused on participants from single countries such as Pakistan, the United Arab Emirates, China, Indonesia, Poland, Saudi Arabia, South Korea, Spain, Tajikistan, and the United States. In contrast, four studies, [S4], [S10], [S12], and [S13], involved participants from multiple countries, including China and the United States [S4], China, the United Kingdom, and the United States [S10], the United Arab Emirates, Oman, Saudi Arabia, and Jordan [S12], Turkey, Sweden, Canada, and Australia [ 13 ]. Figures 4 and 5 illustrate the distribution of participants, whether from single or multiple countries, and the contribution of each country in the reviewed studies, respectively.
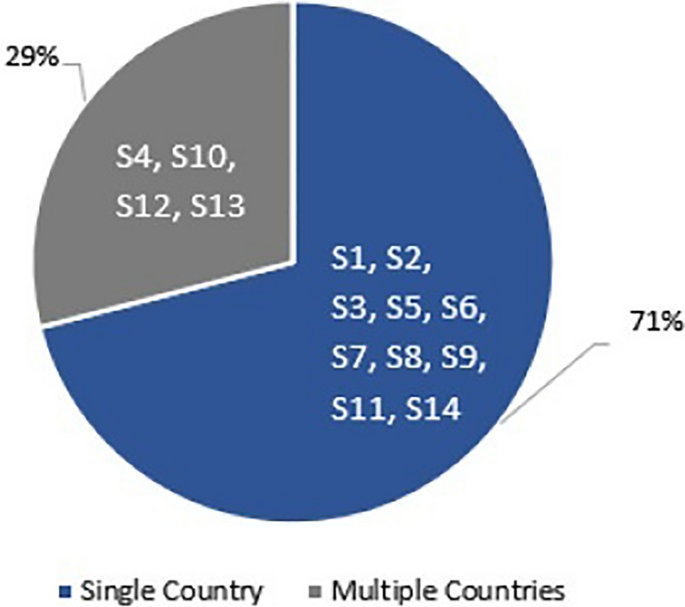
The reviewed studies conducted in single or multiple countries
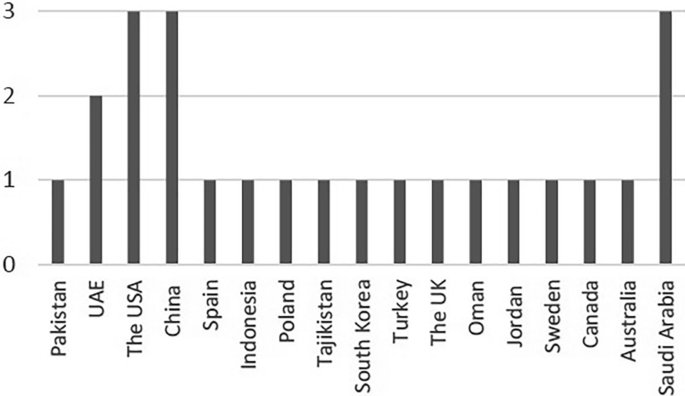
The Contribution of each country in the studies
3.1.4 Study population and sample size
Four study populations were included: university students, university teachers, university teachers and students, and elementary school teachers. Six studies involved university students [S2], [S3], [S5] and [S6]-[S8]. Three studies focused on university teachers [S1], [S4], and [S6], while one study specifically targeted elementary school teachers [S11]. Additionally, four studies included both university teachers and students [S10] and [ 12 , 13 , 14 ], and among them, study [S13] specifically included postgraduate students. In terms of the sample size of the reviewed studies, nine studies included a small sample size of less than 50 participants [S1], [S3], [S6], [S8], and [S10]-[S13]. Three studies had 50–100 participants [S2], [S9], and [S14]. Only one study had more than 100 participants [S7]. It is worth mentioning that study [S4] adopted a mixed methods approach, including 10 participants for qualitative analysis and 110 participants for quantitative analysis.
3.1.5 Participants’ familiarity with using ChatGPT
The reviewed studies recruited a diverse range of participants with varying levels of familiarity with ChatGPT. Five studies [S2], [S4], [S6], [S8], and [S12] involved participants already familiar with ChatGPT, while eight studies [S1], [S3], [S5], [S7], [S9], [S10], [S13] and [S14] included individuals with differing levels of familiarity. Notably, one study [S11] had participants who were entirely unfamiliar with ChatGPT. It is important to note that four studies [S3], [S5], [S9], and [S11] provided training or guidance to their participants before conducting their studies, while ten studies [S1], [S2], [S4], [S6]-[S8], [S10], and [S12]-[S14] did not provide training due to the participants' existing familiarity with ChatGPT.
3.1.6 Research methodology approaches and source(S) of data
The reviewed studies adopted various research methodology approaches. Seven studies adopted qualitative research methodology [S1], [S4], [S6], [S8], [S10], [S11], and [S12], while three studies adopted quantitative research methodology [S3], [S7], and [S14], and four studies employed mixed-methods, which involved a combination of both the strengths of qualitative and quantitative methods [S2], [S5], [S9], and [S13].
In terms of the source(s) of data, the reviewed studies obtained their data from various sources, such as interviews, questionnaires, and pre-and post-tests. Six studies relied on interviews as their primary source of data collection [S1], [S4], [S6], [S10], [S11], and [S12], four studies relied on questionnaires [S2], [S7], [S13], and [S14], two studies combined the use of pre-and post-tests and questionnaires for data collection [S3] and [S9], while two studies combined the use of questionnaires and interviews to obtain the data [S5] and [S8]. It is important to note that six of the reviewed studies were quasi-experimental [S3], [S5], [S8], [S9], [S12], and [S14], while the remaining ones were experimental studies [S1], [S2], [S4], [S6], [S7], [S10], [S11], and [S13]. Figures 6 and 7 illustrate the research methodologies and the source (s) of data used in the reviewed studies, respectively.
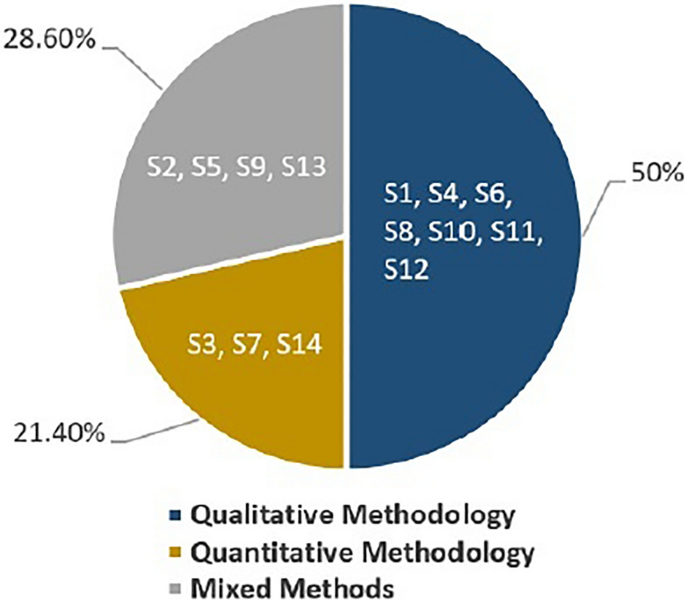
Research methodologies in the reviewed studies
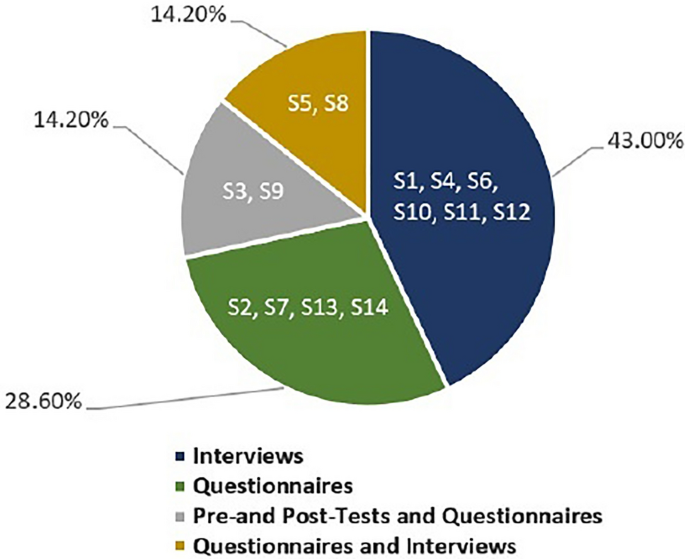
Source of data in the reviewed studies
3.1.7 The aim and objectives of the studies
The reviewed studies encompassed a diverse set of aims, with several of them incorporating multiple primary objectives. Six studies [S3], [S6], [S7], [S8], [S11], and [S12] examined the integration of ChatGPT in educational contexts, and four studies [S4], [S5], [S13], and [S14] investigated the various implications of its use in education, while three studies [S2], [S9], and [S10] aimed to explore both its integration and implications in education. Additionally, seven studies explicitly explored attitudes and perceptions of students [S2] and [S3], educators [S1] and [S6], or both [S10], [S12], and [S13] regarding the utilization of ChatGPT in educational settings.
3.2 Part 2: research questions and main findings of the reviewed studies
This part will present the answers to the research questions and the main findings of the reviewed studies, classified into two main categories (learning and teaching) according to AI Education classification by [ 36 ]. Figure 8 summarizes the main findings of the reviewed studies in a visually informative diagram. Table 4 provides a detailed list of the key information extracted from the selected studies that led to generating these themes.
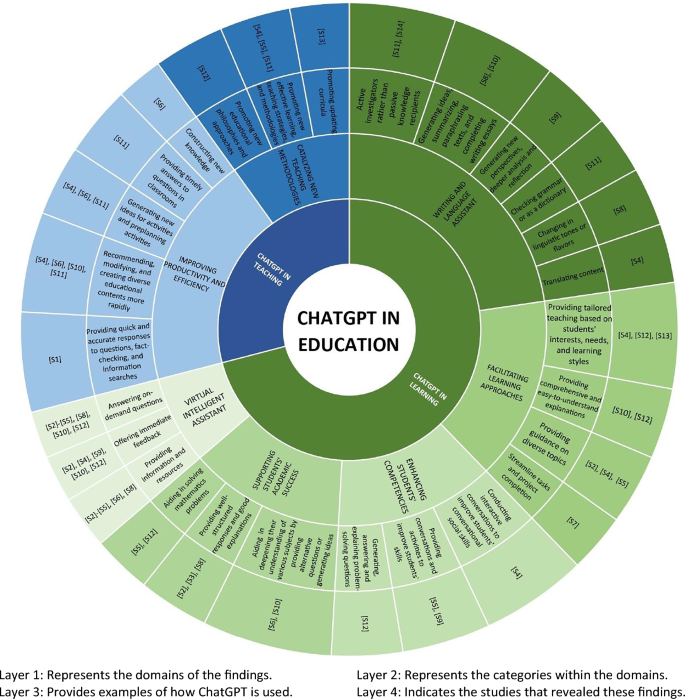
The main findings in the reviewed studies
4 Students' initial attempts at utilizing ChatGPT in learning and main findings from students' perspective
4.1 virtual intelligent assistant.
Nine studies demonstrated that ChatGPT has been utilized by students as an intelligent assistant to enhance and support their learning. Students employed it for various purposes, such as answering on-demand questions [S2]-[S5], [S8], [S10], and [S12], providing valuable information and learning resources [S2]-[S5], [S6], and [S8], as well as receiving immediate feedback [S2], [S4], [S9], [S10], and [S12]. In this regard, students generally were confident in the accuracy of ChatGPT's responses, considering them relevant, reliable, and detailed [S3], [S4], [S5], and [S8]. However, some students indicated the need for improvement, as they found that answers are not always accurate [S2], and that misleading information may have been provided or that it may not always align with their expectations [S6] and [S10]. It was also observed by the students that the accuracy of ChatGPT is dependent on several factors, including the quality and specificity of the user's input, the complexity of the question or topic, and the scope and relevance of its training data [S12]. Many students felt that ChatGPT's answers were not always accurate and most of them believed that it requires good background knowledge to work with.
4.2 Writing and language proficiency assistant
Six of the reviewed studies highlighted that ChatGPT has been utilized by students as a valuable assistant tool to improve their academic writing skills and language proficiency. Among these studies, three mainly focused on English education, demonstrating that students showed sufficient mastery in using ChatGPT for generating ideas, summarizing, paraphrasing texts, and completing writing essays [S8], [S11], and [S14]. Furthermore, ChatGPT helped them in writing by making students active investigators rather than passive knowledge recipients and facilitated the development of their writing skills [S11] and [S14]. Similarly, ChatGPT allowed students to generate unique ideas and perspectives, leading to deeper analysis and reflection on their journalism writing [S9]. In terms of language proficiency, ChatGPT allowed participants to translate content into their home languages, making it more accessible and relevant to their context [S4]. It also enabled them to request changes in linguistic tones or flavors [S8]. Moreover, participants used it to check grammar or as a dictionary [S11].
4.3 Valuable resource for learning approaches
Five studies demonstrated that students used ChatGPT as a valuable complementary resource for self-directed learning. It provided learning resources and guidance on diverse educational topics and created a supportive home learning environment [S2] and [S4]. Moreover, it offered step-by-step guidance to grasp concepts at their own pace and enhance their understanding [S5], streamlined task and project completion carried out independently [S7], provided comprehensive and easy-to-understand explanations on various subjects [S10], and assisted in studying geometry operations, thereby empowering them to explore geometry operations at their own pace [S12]. Three studies showed that students used ChatGPT as a valuable learning resource for personalized learning. It delivered age-appropriate conversations and tailored teaching based on a child's interests [S4], acted as a personalized learning assistant, adapted to their needs and pace, which assisted them in understanding mathematical concepts [S12], and enabled personalized learning experiences in social sciences by adapting to students' needs and learning styles [S13]. On the other hand, it is important to note that, according to one study [S5], students suggested that using ChatGPT may negatively affect collaborative learning competencies between students.
4.4 Enhancing students' competencies
Six of the reviewed studies have shown that ChatGPT is a valuable tool for improving a wide range of skills among students. Two studies have provided evidence that ChatGPT led to improvements in students' critical thinking, reasoning skills, and hazard recognition competencies through engaging them in interactive conversations or activities and providing responses related to their disciplines in journalism [S5] and construction education [S9]. Furthermore, two studies focused on mathematical education have shown the positive impact of ChatGPT on students' problem-solving abilities in unraveling problem-solving questions [S12] and enhancing the students' understanding of the problem-solving process [S5]. Lastly, one study indicated that ChatGPT effectively contributed to the enhancement of conversational social skills [S4].
4.5 Supporting students' academic success
Seven of the reviewed studies highlighted that students found ChatGPT to be beneficial for learning as it enhanced learning efficiency and improved the learning experience. It has been observed to improve students' efficiency in computer engineering studies by providing well-structured responses and good explanations [S2]. Additionally, students found it extremely useful for hazard reporting [S3], and it also enhanced their efficiency in solving mathematics problems and capabilities [S5] and [S12]. Furthermore, by finding information, generating ideas, translating texts, and providing alternative questions, ChatGPT aided students in deepening their understanding of various subjects [S6]. It contributed to an increase in students' overall productivity [S7] and improved efficiency in composing written tasks [S8]. Regarding learning experiences, ChatGPT was instrumental in assisting students in identifying hazards that they might have otherwise overlooked [S3]. It also improved students' learning experiences in solving mathematics problems and developing abilities [S5] and [S12]. Moreover, it increased students' successful completion of important tasks in their studies [S7], particularly those involving average difficulty writing tasks [S8]. Additionally, ChatGPT increased the chances of educational success by providing students with baseline knowledge on various topics [S10].
5 Teachers' initial attempts at utilizing ChatGPT in teaching and main findings from teachers' perspective
5.1 valuable resource for teaching.
The reviewed studies showed that teachers have employed ChatGPT to recommend, modify, and generate diverse, creative, organized, and engaging educational contents, teaching materials, and testing resources more rapidly [S4], [S6], [S10] and [S11]. Additionally, teachers experienced increased productivity as ChatGPT facilitated quick and accurate responses to questions, fact-checking, and information searches [S1]. It also proved valuable in constructing new knowledge [S6] and providing timely answers to students' questions in classrooms [S11]. Moreover, ChatGPT enhanced teachers' efficiency by generating new ideas for activities and preplanning activities for their students [S4] and [S6], including interactive language game partners [S11].
5.2 Improving productivity and efficiency
The reviewed studies showed that participants' productivity and work efficiency have been significantly enhanced by using ChatGPT as it enabled them to allocate more time to other tasks and reduce their overall workloads [S6], [S10], [S11], [S13], and [S14]. However, three studies [S1], [S4], and [S11], indicated a negative perception and attitude among teachers toward using ChatGPT. This negativity stemmed from a lack of necessary skills to use it effectively [S1], a limited familiarity with it [S4], and occasional inaccuracies in the content provided by it [S10].
5.3 Catalyzing new teaching methodologies
Five of the reviewed studies highlighted that educators found the necessity of redefining their teaching profession with the assistance of ChatGPT [S11], developing new effective learning strategies [S4], and adapting teaching strategies and methodologies to ensure the development of essential skills for future engineers [S5]. They also emphasized the importance of adopting new educational philosophies and approaches that can evolve with the introduction of ChatGPT into the classroom [S12]. Furthermore, updating curricula to focus on improving human-specific features, such as emotional intelligence, creativity, and philosophical perspectives [S13], was found to be essential.
5.4 Effective utilization of CHATGPT in teaching
According to the reviewed studies, effective utilization of ChatGPT in education requires providing teachers with well-structured training, support, and adequate background on how to use ChatGPT responsibly [S1], [S3], [S11], and [S12]. Establishing clear rules and regulations regarding its usage is essential to ensure it positively impacts the teaching and learning processes, including students' skills [S1], [S4], [S5], [S8], [S9], and [S11]-[S14]. Moreover, conducting further research and engaging in discussions with policymakers and stakeholders is indeed crucial for the successful integration of ChatGPT in education and to maximize the benefits for both educators and students [S1], [S6]-[S10], and [S12]-[S14].
6 Discussion
The purpose of this review is to conduct a systematic review of empirical studies that have explored the utilization of ChatGPT, one of today’s most advanced LLM-based chatbots, in education. The findings of the reviewed studies showed several ways of ChatGPT utilization in different learning and teaching practices as well as it provided insights and considerations that can facilitate its effective and responsible use in future educational contexts. The results of the reviewed studies came from diverse fields of education, which helped us avoid a biased review that is limited to a specific field. Similarly, the reviewed studies have been conducted across different geographic regions. This kind of variety in geographic representation enriched the findings of this review.
In response to RQ1 , "What are students' and teachers' initial attempts at utilizing ChatGPT in education?", the findings from this review provide comprehensive insights. Chatbots, including ChatGPT, play a crucial role in supporting student learning, enhancing their learning experiences, and facilitating diverse learning approaches [ 42 , 43 ]. This review found that this tool, ChatGPT, has been instrumental in enhancing students' learning experiences by serving as a virtual intelligent assistant, providing immediate feedback, on-demand answers, and engaging in educational conversations. Additionally, students have benefited from ChatGPT’s ability to generate ideas, compose essays, and perform tasks like summarizing, translating, paraphrasing texts, or checking grammar, thereby enhancing their writing and language competencies. Furthermore, students have turned to ChatGPT for assistance in understanding concepts and homework, providing structured learning plans, and clarifying assignments and tasks, which fosters a supportive home learning environment, allowing them to take responsibility for their own learning and cultivate the skills and approaches essential for supportive home learning environment [ 26 , 27 , 28 ]. This finding aligns with the study of Saqr et al. [ 68 , 69 ] who highlighted that, when students actively engage in their own learning process, it yields additional advantages, such as heightened motivation, enhanced achievement, and the cultivation of enthusiasm, turning them into advocates for their own learning.
Moreover, students have utilized ChatGPT for tailored teaching and step-by-step guidance on diverse educational topics, streamlining task and project completion, and generating and recommending educational content. This personalization enhances the learning environment, leading to increased academic success. This finding aligns with other recent studies [ 26 , 27 , 28 , 60 , 66 ] which revealed that ChatGPT has the potential to offer personalized learning experiences and support an effective learning process by providing students with customized feedback and explanations tailored to their needs and abilities. Ultimately, fostering students' performance, engagement, and motivation, leading to increase students' academic success [ 14 , 44 , 58 ]. This ultimate outcome is in line with the findings of Saqr et al. [ 68 , 69 ], which emphasized that learning strategies are important catalysts of students' learning, as students who utilize effective learning strategies are more likely to have better academic achievement.
Teachers, too, have capitalized on ChatGPT's capabilities to enhance productivity and efficiency, using it for creating lesson plans, generating quizzes, providing additional resources, generating and preplanning new ideas for activities, and aiding in answering students’ questions. This adoption of technology introduces new opportunities to support teaching and learning practices, enhancing teacher productivity. This finding aligns with those of Day [ 17 ], De Castro [ 18 ], and Su and Yang [ 74 ] as well as with those of Valtonen et al. [ 82 ], who revealed that emerging technological advancements have opened up novel opportunities and means to support teaching and learning practices, and enhance teachers’ productivity.
In response to RQ2 , "What are the main findings derived from empirical studies that have incorporated ChatGPT into learning and teaching?", the findings from this review provide profound insights and raise significant concerns. Starting with the insights, chatbots, including ChatGPT, have demonstrated the potential to reshape and revolutionize education, creating new, novel opportunities for enhancing the learning process and outcomes [ 83 ], facilitating different learning approaches, and offering a range of pedagogical benefits [ 19 , 43 , 72 ]. In this context, this review found that ChatGPT could open avenues for educators to adopt or develop new effective learning and teaching strategies that can evolve with the introduction of ChatGPT into the classroom. Nonetheless, there is an evident lack of research understanding regarding the potential impact of generative machine learning models within diverse educational settings [ 83 ]. This necessitates teachers to attain a high level of proficiency in incorporating chatbots, such as ChatGPT, into their classrooms to create inventive, well-structured, and captivating learning strategies. In the same vein, the review also found that teachers without the requisite skills to utilize ChatGPT realized that it did not contribute positively to their work and could potentially have adverse effects [ 37 ]. This concern could lead to inequity of access to the benefits of chatbots, including ChatGPT, as individuals who lack the necessary expertise may not be able to harness their full potential, resulting in disparities in educational outcomes and opportunities. Therefore, immediate action is needed to address these potential issues. A potential solution is offering training, support, and competency development for teachers to ensure that all of them can leverage chatbots, including ChatGPT, effectively and equitably in their educational practices [ 5 , 28 , 80 ], which could enhance accessibility and inclusivity, and potentially result in innovative outcomes [ 82 , 83 ].
Additionally, chatbots, including ChatGPT, have the potential to significantly impact students' thinking abilities, including retention, reasoning, analysis skills [ 19 , 45 ], and foster innovation and creativity capabilities [ 83 ]. This review found that ChatGPT could contribute to improving a wide range of skills among students. However, it found that frequent use of ChatGPT may result in a decrease in innovative capacities, collaborative skills and cognitive capacities, and students' motivation to attend classes, as well as could lead to reduced higher-order thinking skills among students [ 22 , 29 ]. Therefore, immediate action is needed to carefully examine the long-term impact of chatbots such as ChatGPT, on learning outcomes as well as to explore its incorporation into educational settings as a supportive tool without compromising students' cognitive development and critical thinking abilities. In the same vein, the review also found that it is challenging to draw a consistent conclusion regarding the potential of ChatGPT to aid self-directed learning approach. This finding aligns with the recent study of Baskara [ 8 ]. Therefore, further research is needed to explore the potential of ChatGPT for self-directed learning. One potential solution involves utilizing learning analytics as a novel approach to examine various aspects of students' learning and support them in their individual endeavors [ 32 ]. This approach can bridge this gap by facilitating an in-depth analysis of how learners engage with ChatGPT, identifying trends in self-directed learning behavior, and assessing its influence on their outcomes.
Turning to the significant concerns, on the other hand, a fundamental challenge with LLM-based chatbots, including ChatGPT, is the accuracy and quality of the provided information and responses, as they provide false information as truth—a phenomenon often referred to as "hallucination" [ 3 , 49 ]. In this context, this review found that the provided information was not entirely satisfactory. Consequently, the utilization of chatbots presents potential concerns, such as generating and providing inaccurate or misleading information, especially for students who utilize it to support their learning. This finding aligns with other findings [ 6 , 30 , 35 , 40 ] which revealed that incorporating chatbots such as ChatGPT, into education presents challenges related to its accuracy and reliability due to its training on a large corpus of data, which may contain inaccuracies and the way users formulate or ask ChatGPT. Therefore, immediate action is needed to address these potential issues. One possible solution is to equip students with the necessary skills and competencies, which include a background understanding of how to use it effectively and the ability to assess and evaluate the information it generates, as the accuracy and the quality of the provided information depend on the input, its complexity, the topic, and the relevance of its training data [ 28 , 49 , 86 ]. However, it's also essential to examine how learners can be educated about how these models operate, the data used in their training, and how to recognize their limitations, challenges, and issues [ 79 ].
Furthermore, chatbots present a substantial challenge concerning maintaining academic integrity [ 20 , 56 ] and copyright violations [ 83 ], which are significant concerns in education. The review found that the potential misuse of ChatGPT might foster cheating, facilitate plagiarism, and threaten academic integrity. This issue is also affirmed by the research conducted by Basic et al. [ 7 ], who presented evidence that students who utilized ChatGPT in their writing assignments had more plagiarism cases than those who did not. These findings align with the conclusions drawn by Cotton et al. [ 13 ], Hisan and Amri [ 33 ] and Sullivan et al. [ 75 ], who revealed that the integration of chatbots such as ChatGPT into education poses a significant challenge to the preservation of academic integrity. Moreover, chatbots, including ChatGPT, have increased the difficulty in identifying plagiarism [ 47 , 67 , 76 ]. The findings from previous studies [ 1 , 84 ] indicate that AI-generated text often went undetected by plagiarism software, such as Turnitin. However, Turnitin and other similar plagiarism detection tools, such as ZeroGPT, GPTZero, and Copyleaks, have since evolved, incorporating enhanced techniques to detect AI-generated text, despite the possibility of false positives, as noted in different studies that have found these tools still not yet fully ready to accurately and reliably identify AI-generated text [ 10 , 51 ], and new novel detection methods may need to be created and implemented for AI-generated text detection [ 4 ]. This potential issue could lead to another concern, which is the difficulty of accurately evaluating student performance when they utilize chatbots such as ChatGPT assistance in their assignments. Consequently, the most LLM-driven chatbots present a substantial challenge to traditional assessments [ 64 ]. The findings from previous studies indicate the importance of rethinking, improving, and redesigning innovative assessment methods in the era of chatbots [ 14 , 20 , 64 , 75 ]. These methods should prioritize the process of evaluating students' ability to apply knowledge to complex cases and demonstrate comprehension, rather than solely focusing on the final product for assessment. Therefore, immediate action is needed to address these potential issues. One possible solution would be the development of clear guidelines, regulatory policies, and pedagogical guidance. These measures would help regulate the proper and ethical utilization of chatbots, such as ChatGPT, and must be established before their introduction to students [ 35 , 38 , 39 , 41 , 89 ].
In summary, our review has delved into the utilization of ChatGPT, a prominent example of chatbots, in education, addressing the question of how ChatGPT has been utilized in education. However, there remain significant gaps, which necessitate further research to shed light on this area.
7 Conclusions
This systematic review has shed light on the varied initial attempts at incorporating ChatGPT into education by both learners and educators, while also offering insights and considerations that can facilitate its effective and responsible use in future educational contexts. From the analysis of 14 selected studies, the review revealed the dual-edged impact of ChatGPT in educational settings. On the positive side, ChatGPT significantly aided the learning process in various ways. Learners have used it as a virtual intelligent assistant, benefiting from its ability to provide immediate feedback, on-demand answers, and easy access to educational resources. Additionally, it was clear that learners have used it to enhance their writing and language skills, engaging in practices such as generating ideas, composing essays, and performing tasks like summarizing, translating, paraphrasing texts, or checking grammar. Importantly, other learners have utilized it in supporting and facilitating their directed and personalized learning on a broad range of educational topics, assisting in understanding concepts and homework, providing structured learning plans, and clarifying assignments and tasks. Educators, on the other hand, found ChatGPT beneficial for enhancing productivity and efficiency. They used it for creating lesson plans, generating quizzes, providing additional resources, and answers learners' questions, which saved time and allowed for more dynamic and engaging teaching strategies and methodologies.
However, the review also pointed out negative impacts. The results revealed that overuse of ChatGPT could decrease innovative capacities and collaborative learning among learners. Specifically, relying too much on ChatGPT for quick answers can inhibit learners' critical thinking and problem-solving skills. Learners might not engage deeply with the material or consider multiple solutions to a problem. This tendency was particularly evident in group projects, where learners preferred consulting ChatGPT individually for solutions over brainstorming and collaborating with peers, which negatively affected their teamwork abilities. On a broader level, integrating ChatGPT into education has also raised several concerns, including the potential for providing inaccurate or misleading information, issues of inequity in access, challenges related to academic integrity, and the possibility of misusing the technology.
Accordingly, this review emphasizes the urgency of developing clear rules, policies, and regulations to ensure ChatGPT's effective and responsible use in educational settings, alongside other chatbots, by both learners and educators. This requires providing well-structured training to educate them on responsible usage and understanding its limitations, along with offering sufficient background information. Moreover, it highlights the importance of rethinking, improving, and redesigning innovative teaching and assessment methods in the era of ChatGPT. Furthermore, conducting further research and engaging in discussions with policymakers and stakeholders are essential steps to maximize the benefits for both educators and learners and ensure academic integrity.
It is important to acknowledge that this review has certain limitations. Firstly, the limited inclusion of reviewed studies can be attributed to several reasons, including the novelty of the technology, as new technologies often face initial skepticism and cautious adoption; the lack of clear guidelines or best practices for leveraging this technology for educational purposes; and institutional or governmental policies affecting the utilization of this technology in educational contexts. These factors, in turn, have affected the number of studies available for review. Secondly, the utilization of the original version of ChatGPT, based on GPT-3 or GPT-3.5, implies that new studies utilizing the updated version, GPT-4 may lead to different findings. Therefore, conducting follow-up systematic reviews is essential once more empirical studies on ChatGPT are published. Additionally, long-term studies are necessary to thoroughly examine and assess the impact of ChatGPT on various educational practices.
Despite these limitations, this systematic review has highlighted the transformative potential of ChatGPT in education, revealing its diverse utilization by learners and educators alike and summarized the benefits of incorporating it into education, as well as the forefront critical concerns and challenges that must be addressed to facilitate its effective and responsible use in future educational contexts. This review could serve as an insightful resource for practitioners who seek to integrate ChatGPT into education and stimulate further research in the field.
Data availability
The data supporting our findings are available upon request.
Abbreviations
- Artificial intelligence
AI in education
Large language model
Artificial neural networks
Chat Generative Pre-Trained Transformer
Recurrent neural networks
Long short-term memory
Reinforcement learning from human feedback
Natural language processing
Preferred Reporting Items for Systematic Reviews and Meta-Analyses
AlAfnan MA, Dishari S, Jovic M, Lomidze K. ChatGPT as an educational tool: opportunities, challenges, and recommendations for communication, business writing, and composition courses. J Artif Intell Technol. 2023. https://doi.org/10.37965/jait.2023.0184 .
Article Google Scholar
Ali JKM, Shamsan MAA, Hezam TA, Mohammed AAQ. Impact of ChatGPT on learning motivation. J Engl Stud Arabia Felix. 2023;2(1):41–9. https://doi.org/10.56540/jesaf.v2i1.51 .
Alkaissi H, McFarlane SI. Artificial hallucinations in ChatGPT: implications in scientific writing. Cureus. 2023. https://doi.org/10.7759/cureus.35179 .
Anderson N, Belavý DL, Perle SM, Hendricks S, Hespanhol L, Verhagen E, Memon AR. AI did not write this manuscript, or did it? Can we trick the AI text detector into generated texts? The potential future of ChatGPT and AI in sports & exercise medicine manuscript generation. BMJ Open Sport Exerc Med. 2023;9(1): e001568. https://doi.org/10.1136/bmjsem-2023-001568 .
Ausat AMA, Massang B, Efendi M, Nofirman N, Riady Y. Can chat GPT replace the role of the teacher in the classroom: a fundamental analysis. J Educ. 2023;5(4):16100–6.
Google Scholar
Baidoo-Anu D, Ansah L. Education in the Era of generative artificial intelligence (AI): understanding the potential benefits of ChatGPT in promoting teaching and learning. Soc Sci Res Netw. 2023. https://doi.org/10.2139/ssrn.4337484 .
Basic Z, Banovac A, Kruzic I, Jerkovic I. Better by you, better than me, chatgpt3 as writing assistance in students essays. 2023. arXiv preprint arXiv:2302.04536 .
Baskara FR. The promises and pitfalls of using chat GPT for self-determined learning in higher education: an argumentative review. Prosiding Seminar Nasional Fakultas Tarbiyah dan Ilmu Keguruan IAIM Sinjai. 2023;2:95–101. https://doi.org/10.47435/sentikjar.v2i0.1825 .
Behera RK, Bala PK, Dhir A. The emerging role of cognitive computing in healthcare: a systematic literature review. Int J Med Inform. 2019;129:154–66. https://doi.org/10.1016/j.ijmedinf.2019.04.024 .
Chaka C. Detecting AI content in responses generated by ChatGPT, YouChat, and Chatsonic: the case of five AI content detection tools. J Appl Learn Teach. 2023. https://doi.org/10.37074/jalt.2023.6.2.12 .
Chiu TKF, Xia Q, Zhou X, Chai CS, Cheng M. Systematic literature review on opportunities, challenges, and future research recommendations of artificial intelligence in education. Comput Educ Artif Intell. 2023;4:100118. https://doi.org/10.1016/j.caeai.2022.100118 .
Choi EPH, Lee JJ, Ho M, Kwok JYY, Lok KYW. Chatting or cheating? The impacts of ChatGPT and other artificial intelligence language models on nurse education. Nurse Educ Today. 2023;125:105796. https://doi.org/10.1016/j.nedt.2023.105796 .
Cotton D, Cotton PA, Shipway JR. Chatting and cheating: ensuring academic integrity in the era of ChatGPT. Innov Educ Teach Int. 2023. https://doi.org/10.1080/14703297.2023.2190148 .
Crawford J, Cowling M, Allen K. Leadership is needed for ethical ChatGPT: Character, assessment, and learning using artificial intelligence (AI). J Univ Teach Learn Pract. 2023. https://doi.org/10.53761/1.20.3.02 .
Creswell JW. Educational research: planning, conducting, and evaluating quantitative and qualitative research [Ebook]. 4th ed. London: Pearson Education; 2015.
Curry D. ChatGPT Revenue and Usage Statistics (2023)—Business of Apps. 2023. https://www.businessofapps.com/data/chatgpt-statistics/
Day T. A preliminary investigation of fake peer-reviewed citations and references generated by ChatGPT. Prof Geogr. 2023. https://doi.org/10.1080/00330124.2023.2190373 .
De Castro CA. A Discussion about the Impact of ChatGPT in education: benefits and concerns. J Bus Theor Pract. 2023;11(2):p28. https://doi.org/10.22158/jbtp.v11n2p28 .
Deng X, Yu Z. A meta-analysis and systematic review of the effect of Chatbot technology use in sustainable education. Sustainability. 2023;15(4):2940. https://doi.org/10.3390/su15042940 .
Eke DO. ChatGPT and the rise of generative AI: threat to academic integrity? J Responsib Technol. 2023;13:100060. https://doi.org/10.1016/j.jrt.2023.100060 .
Elmoazen R, Saqr M, Tedre M, Hirsto L. A systematic literature review of empirical research on epistemic network analysis in education. IEEE Access. 2022;10:17330–48. https://doi.org/10.1109/access.2022.3149812 .
Farrokhnia M, Banihashem SK, Noroozi O, Wals AEJ. A SWOT analysis of ChatGPT: implications for educational practice and research. Innov Educ Teach Int. 2023. https://doi.org/10.1080/14703297.2023.2195846 .
Fergus S, Botha M, Ostovar M. Evaluating academic answers generated using ChatGPT. J Chem Educ. 2023;100(4):1672–5. https://doi.org/10.1021/acs.jchemed.3c00087 .
Fink A. Conducting research literature reviews: from the Internet to Paper. Incorporated: SAGE Publications; 2010.
Firaina R, Sulisworo D. Exploring the usage of ChatGPT in higher education: frequency and impact on productivity. Buletin Edukasi Indonesia (BEI). 2023;2(01):39–46. https://doi.org/10.56741/bei.v2i01.310 .
Firat, M. (2023). How chat GPT can transform autodidactic experiences and open education. Department of Distance Education, Open Education Faculty, Anadolu Unive . https://orcid.org/0000-0001-8707-5918
Firat M. What ChatGPT means for universities: perceptions of scholars and students. J Appl Learn Teach. 2023. https://doi.org/10.37074/jalt.2023.6.1.22 .
Fuchs K. Exploring the opportunities and challenges of NLP models in higher education: is Chat GPT a blessing or a curse? Front Educ. 2023. https://doi.org/10.3389/feduc.2023.1166682 .
García-Peñalvo FJ. La percepción de la inteligencia artificial en contextos educativos tras el lanzamiento de ChatGPT: disrupción o pánico. Educ Knowl Soc. 2023;24: e31279. https://doi.org/10.14201/eks.31279 .
Gilson A, Safranek CW, Huang T, Socrates V, Chi L, Taylor A, Chartash D. How does ChatGPT perform on the United States medical Licensing examination? The implications of large language models for medical education and knowledge assessment. JMIR Med Educ. 2023;9: e45312. https://doi.org/10.2196/45312 .
Hashana AJ, Brundha P, Ayoobkhan MUA, Fazila S. Deep Learning in ChatGPT—A Survey. In 2023 7th international conference on trends in electronics and informatics (ICOEI) . 2023. (pp. 1001–1005). IEEE. https://doi.org/10.1109/icoei56765.2023.10125852
Hirsto L, Saqr M, López-Pernas S, Valtonen T. (2022). A systematic narrative review of learning analytics research in K-12 and schools. Proceedings . https://ceur-ws.org/Vol-3383/FLAIEC22_paper_9536.pdf
Hisan UK, Amri MM. ChatGPT and medical education: a double-edged sword. J Pedag Educ Sci. 2023;2(01):71–89. https://doi.org/10.13140/RG.2.2.31280.23043/1 .
Hopkins AM, Logan JM, Kichenadasse G, Sorich MJ. Artificial intelligence chatbots will revolutionize how cancer patients access information: ChatGPT represents a paradigm-shift. JNCI Cancer Spectr. 2023. https://doi.org/10.1093/jncics/pkad010 .
Househ M, AlSaad R, Alhuwail D, Ahmed A, Healy MG, Latifi S, Sheikh J. Large Language models in medical education: opportunities, challenges, and future directions. JMIR Med Educ. 2023;9: e48291. https://doi.org/10.2196/48291 .
Ilkka T. The impact of artificial intelligence on learning, teaching, and education. Minist de Educ. 2018. https://doi.org/10.2760/12297 .
Iqbal N, Ahmed H, Azhar KA. Exploring teachers’ attitudes towards using CHATGPT. Globa J Manag Adm Sci. 2022;3(4):97–111. https://doi.org/10.46568/gjmas.v3i4.163 .
Irfan M, Murray L, Ali S. Integration of Artificial intelligence in academia: a case study of critical teaching and learning in Higher education. Globa Soc Sci Rev. 2023;8(1):352–64. https://doi.org/10.31703/gssr.2023(viii-i).32 .
Jeon JH, Lee S. Large language models in education: a focus on the complementary relationship between human teachers and ChatGPT. Educ Inf Technol. 2023. https://doi.org/10.1007/s10639-023-11834-1 .
Khan RA, Jawaid M, Khan AR, Sajjad M. ChatGPT—Reshaping medical education and clinical management. Pak J Med Sci. 2023. https://doi.org/10.12669/pjms.39.2.7653 .
King MR. A conversation on artificial intelligence, Chatbots, and plagiarism in higher education. Cell Mol Bioeng. 2023;16(1):1–2. https://doi.org/10.1007/s12195-022-00754-8 .
Kooli C. Chatbots in education and research: a critical examination of ethical implications and solutions. Sustainability. 2023;15(7):5614. https://doi.org/10.3390/su15075614 .
Kuhail MA, Alturki N, Alramlawi S, Alhejori K. Interacting with educational chatbots: a systematic review. Educ Inf Technol. 2022;28(1):973–1018. https://doi.org/10.1007/s10639-022-11177-3 .
Lee H. The rise of ChatGPT: exploring its potential in medical education. Anat Sci Educ. 2023. https://doi.org/10.1002/ase.2270 .
Li L, Subbareddy R, Raghavendra CG. AI intelligence Chatbot to improve students learning in the higher education platform. J Interconnect Netw. 2022. https://doi.org/10.1142/s0219265921430325 .
Limna P. A Review of Artificial Intelligence (AI) in Education during the Digital Era. 2022. https://ssrn.com/abstract=4160798
Lo CK. What is the impact of ChatGPT on education? A rapid review of the literature. Educ Sci. 2023;13(4):410. https://doi.org/10.3390/educsci13040410 .
Luo W, He H, Liu J, Berson IR, Berson MJ, Zhou Y, Li H. Aladdin’s genie or pandora’s box For early childhood education? Experts chat on the roles, challenges, and developments of ChatGPT. Early Educ Dev. 2023. https://doi.org/10.1080/10409289.2023.2214181 .
Meyer JG, Urbanowicz RJ, Martin P, O’Connor K, Li R, Peng P, Moore JH. ChatGPT and large language models in academia: opportunities and challenges. Biodata Min. 2023. https://doi.org/10.1186/s13040-023-00339-9 .
Mhlanga D. Open AI in education, the responsible and ethical use of ChatGPT towards lifelong learning. Soc Sci Res Netw. 2023. https://doi.org/10.2139/ssrn.4354422 .
Neumann, M., Rauschenberger, M., & Schön, E. M. (2023). “We Need To Talk About ChatGPT”: The Future of AI and Higher Education. https://doi.org/10.1109/seeng59157.2023.00010
Nolan B. Here are the schools and colleges that have banned the use of ChatGPT over plagiarism and misinformation fears. Business Insider . 2023. https://www.businessinsider.com
O’Leary DE. An analysis of three chatbots: BlenderBot, ChatGPT and LaMDA. Int J Intell Syst Account, Financ Manag. 2023;30(1):41–54. https://doi.org/10.1002/isaf.1531 .
Okoli C. A guide to conducting a standalone systematic literature review. Commun Assoc Inf Syst. 2015. https://doi.org/10.17705/1cais.03743 .
OpenAI. (2023). https://openai.com/blog/chatgpt
Perkins M. Academic integrity considerations of AI large language models in the post-pandemic era: ChatGPT and beyond. J Univ Teach Learn Pract. 2023. https://doi.org/10.53761/1.20.02.07 .
Plevris V, Papazafeiropoulos G, Rios AJ. Chatbots put to the test in math and logic problems: A preliminary comparison and assessment of ChatGPT-3.5, ChatGPT-4, and Google Bard. arXiv (Cornell University) . 2023. https://doi.org/10.48550/arxiv.2305.18618
Rahman MM, Watanobe Y (2023) ChatGPT for education and research: opportunities, threats, and strategies. Appl Sci 13(9):5783. https://doi.org/10.3390/app13095783
Ram B, Verma P. Artificial intelligence AI-based Chatbot study of ChatGPT, google AI bard and baidu AI. World J Adv Eng Technol Sci. 2023;8(1):258–61. https://doi.org/10.30574/wjaets.2023.8.1.0045 .
Rasul T, Nair S, Kalendra D, Robin M, de Oliveira Santini F, Ladeira WJ, Heathcote L. The role of ChatGPT in higher education: benefits, challenges, and future research directions. J Appl Learn Teach. 2023. https://doi.org/10.37074/jalt.2023.6.1.29 .
Ratnam M, Sharm B, Tomer A. ChatGPT: educational artificial intelligence. Int J Adv Trends Comput Sci Eng. 2023;12(2):84–91. https://doi.org/10.30534/ijatcse/2023/091222023 .
Ray PP. ChatGPT: a comprehensive review on background, applications, key challenges, bias, ethics, limitations and future scope. Internet Things Cyber-Phys Syst. 2023;3:121–54. https://doi.org/10.1016/j.iotcps.2023.04.003 .
Roumeliotis KI, Tselikas ND. ChatGPT and Open-AI models: a preliminary review. Future Internet. 2023;15(6):192. https://doi.org/10.3390/fi15060192 .
Rudolph J, Tan S, Tan S. War of the chatbots: Bard, Bing Chat, ChatGPT, Ernie and beyond. The new AI gold rush and its impact on higher education. J Appl Learn Teach. 2023. https://doi.org/10.37074/jalt.2023.6.1.23 .
Ruiz LMS, Moll-López S, Nuñez-Pérez A, Moraño J, Vega-Fleitas E. ChatGPT challenges blended learning methodologies in engineering education: a case study in mathematics. Appl Sci. 2023;13(10):6039. https://doi.org/10.3390/app13106039 .
Sallam M, Salim NA, Barakat M, Al-Tammemi AB. ChatGPT applications in medical, dental, pharmacy, and public health education: a descriptive study highlighting the advantages and limitations. Narra J. 2023;3(1): e103. https://doi.org/10.52225/narra.v3i1.103 .
Salvagno M, Taccone FS, Gerli AG. Can artificial intelligence help for scientific writing? Crit Care. 2023. https://doi.org/10.1186/s13054-023-04380-2 .
Saqr M, López-Pernas S, Helske S, Hrastinski S. The longitudinal association between engagement and achievement varies by time, students’ profiles, and achievement state: a full program study. Comput Educ. 2023;199:104787. https://doi.org/10.1016/j.compedu.2023.104787 .
Saqr M, Matcha W, Uzir N, Jovanović J, Gašević D, López-Pernas S. Transferring effective learning strategies across learning contexts matters: a study in problem-based learning. Australas J Educ Technol. 2023;39(3):9.
Schöbel S, Schmitt A, Benner D, Saqr M, Janson A, Leimeister JM. Charting the evolution and future of conversational agents: a research agenda along five waves and new frontiers. Inf Syst Front. 2023. https://doi.org/10.1007/s10796-023-10375-9 .
Shoufan A. Exploring students’ perceptions of CHATGPT: thematic analysis and follow-up survey. IEEE Access. 2023. https://doi.org/10.1109/access.2023.3268224 .
Sonderegger S, Seufert S. Chatbot-mediated learning: conceptual framework for the design of Chatbot use cases in education. Gallen: Institute for Educational Management and Technologies, University of St; 2022. https://doi.org/10.5220/0010999200003182 .
Book Google Scholar
Strzelecki A. To use or not to use ChatGPT in higher education? A study of students’ acceptance and use of technology. Interact Learn Environ. 2023. https://doi.org/10.1080/10494820.2023.2209881 .
Su J, Yang W. Unlocking the power of ChatGPT: a framework for applying generative AI in education. ECNU Rev Educ. 2023. https://doi.org/10.1177/20965311231168423 .
Sullivan M, Kelly A, McLaughlan P. ChatGPT in higher education: Considerations for academic integrity and student learning. J ApplLearn Teach. 2023;6(1):1–10. https://doi.org/10.37074/jalt.2023.6.1.17 .
Szabo A. ChatGPT is a breakthrough in science and education but fails a test in sports and exercise psychology. Balt J Sport Health Sci. 2023;1(128):25–40. https://doi.org/10.33607/bjshs.v127i4.1233 .
Taecharungroj V. “What can ChatGPT do?” analyzing early reactions to the innovative AI chatbot on Twitter. Big Data Cognit Comput. 2023;7(1):35. https://doi.org/10.3390/bdcc7010035 .
Tam S, Said RB. User preferences for ChatGPT-powered conversational interfaces versus traditional methods. Biomed Eng Soc. 2023. https://doi.org/10.58496/mjcsc/2023/004 .
Tedre M, Kahila J, Vartiainen H. (2023). Exploration on how co-designing with AI facilitates critical evaluation of ethics of AI in craft education. In: Langran E, Christensen P, Sanson J (Eds). Proceedings of Society for Information Technology and Teacher Education International Conference . 2023. pp. 2289–2296.
Tlili A, Shehata B, Adarkwah MA, Bozkurt A, Hickey DT, Huang R, Agyemang B. What if the devil is my guardian angel: ChatGPT as a case study of using chatbots in education. Smart Learn Environ. 2023. https://doi.org/10.1186/s40561-023-00237-x .
Uddin SMJ, Albert A, Ovid A, Alsharef A. Leveraging CHATGPT to aid construction hazard recognition and support safety education and training. Sustainability. 2023;15(9):7121. https://doi.org/10.3390/su15097121 .
Valtonen T, López-Pernas S, Saqr M, Vartiainen H, Sointu E, Tedre M. The nature and building blocks of educational technology research. Comput Hum Behav. 2022;128:107123. https://doi.org/10.1016/j.chb.2021.107123 .
Vartiainen H, Tedre M. Using artificial intelligence in craft education: crafting with text-to-image generative models. Digit Creat. 2023;34(1):1–21. https://doi.org/10.1080/14626268.2023.2174557 .
Ventayen RJM. OpenAI ChatGPT generated results: similarity index of artificial intelligence-based contents. Soc Sci Res Netw. 2023. https://doi.org/10.2139/ssrn.4332664 .
Wagner MW, Ertl-Wagner BB. Accuracy of information and references using ChatGPT-3 for retrieval of clinical radiological information. Can Assoc Radiol J. 2023. https://doi.org/10.1177/08465371231171125 .
Wardat Y, Tashtoush MA, AlAli R, Jarrah AM. ChatGPT: a revolutionary tool for teaching and learning mathematics. Eurasia J Math, Sci Technol Educ. 2023;19(7):em2286. https://doi.org/10.29333/ejmste/13272 .
Webster J, Watson RT. Analyzing the past to prepare for the future: writing a literature review. Manag Inf Syst Quart. 2002;26(2):3.
Xiao Y, Watson ME. Guidance on conducting a systematic literature review. J Plan Educ Res. 2017;39(1):93–112. https://doi.org/10.1177/0739456x17723971 .
Yan D. Impact of ChatGPT on learners in a L2 writing practicum: an exploratory investigation. Educ Inf Technol. 2023. https://doi.org/10.1007/s10639-023-11742-4 .
Yu H. Reflection on whether Chat GPT should be banned by academia from the perspective of education and teaching. Front Psychol. 2023;14:1181712. https://doi.org/10.3389/fpsyg.2023.1181712 .
Zhu C, Sun M, Luo J, Li T, Wang M. How to harness the potential of ChatGPT in education? Knowl Manag ELearn. 2023;15(2):133–52. https://doi.org/10.34105/j.kmel.2023.15.008 .
Download references
The paper is co-funded by the Academy of Finland (Suomen Akatemia) Research Council for Natural Sciences and Engineering for the project Towards precision education: Idiographic learning analytics (TOPEILA), Decision Number 350560.
Author information
Authors and affiliations.
School of Computing, University of Eastern Finland, 80100, Joensuu, Finland
Yazid Albadarin, Mohammed Saqr, Nicolas Pope & Markku Tukiainen
You can also search for this author in PubMed Google Scholar
Contributions
YA contributed to the literature search, data analysis, discussion, and conclusion. Additionally, YA contributed to the manuscript’s writing, editing, and finalization. MS contributed to the study’s design, conceptualization, acquisition of funding, project administration, allocation of resources, supervision, validation, literature search, and analysis of results. Furthermore, MS contributed to the manuscript's writing, revising, and approving it in its finalized state. NP contributed to the results, and discussions, and provided supervision. NP also contributed to the writing process, revisions, and the final approval of the manuscript in its finalized state. MT contributed to the study's conceptualization, resource management, supervision, writing, revising the manuscript, and approving it.
Corresponding author
Correspondence to Yazid Albadarin .
Ethics declarations
Competing interests.
The authors declare no competing interests.
Additional information
Publisher's note.
Springer Nature remains neutral with regard to jurisdictional claims in published maps and institutional affiliations.
See Table 4
The process of synthesizing the data presented in Table 4 involved identifying the relevant studies through a search process of databases (ERIC, Scopus, Web of Knowledge, Dimensions.ai, and lens.org) using specific keywords "ChatGPT" and "education". Following this, inclusion/exclusion criteria were applied, and data extraction was performed using Creswell's [ 15 ] coding techniques to capture key information and identify common themes across the included studies.
Rights and permissions
Open Access This article is licensed under a Creative Commons Attribution 4.0 International License, which permits use, sharing, adaptation, distribution and reproduction in any medium or format, as long as you give appropriate credit to the original author(s) and the source, provide a link to the Creative Commons licence, and indicate if changes were made. The images or other third party material in this article are included in the article's Creative Commons licence, unless indicated otherwise in a credit line to the material. If material is not included in the article's Creative Commons licence and your intended use is not permitted by statutory regulation or exceeds the permitted use, you will need to obtain permission directly from the copyright holder. To view a copy of this licence, visit http://creativecommons.org/licenses/by/4.0/ .
Reprints and permissions
About this article
Albadarin, Y., Saqr, M., Pope, N. et al. A systematic literature review of empirical research on ChatGPT in education. Discov Educ 3 , 60 (2024). https://doi.org/10.1007/s44217-024-00138-2
Download citation
Received : 22 October 2023
Accepted : 10 May 2024
Published : 26 May 2024
DOI : https://doi.org/10.1007/s44217-024-00138-2
Share this article
Anyone you share the following link with will be able to read this content:
Sorry, a shareable link is not currently available for this article.
Provided by the Springer Nature SharedIt content-sharing initiative
- Large language models
- Educational technology
- Systematic review
Advertisement
- Find a journal
- Publish with us
- Track your research
US South Carolina
Recently viewed courses
Recently viewed.
Find Your Dream School
This site uses various technologies, as described in our Privacy Policy, for personalization, measuring website use/performance, and targeted advertising, which may include storing and sharing information about your site visit with third parties. By continuing to use this website you consent to our Privacy Policy and Terms of Use .
COVID-19 Update: To help students through this crisis, The Princeton Review will continue our "Enroll with Confidence" refund policies. For full details, please click here.
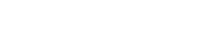
- College Admissions Essay Counseling College Admissions Essay Counseling
- Homework Essay Feedback Homework Essay Feedback

AI Meets World-class Experts
40+ years of experience infused into the latest technology
Why The Princeton Review with AI
By leveraging AI, we can help more students more effectively and efficiently.
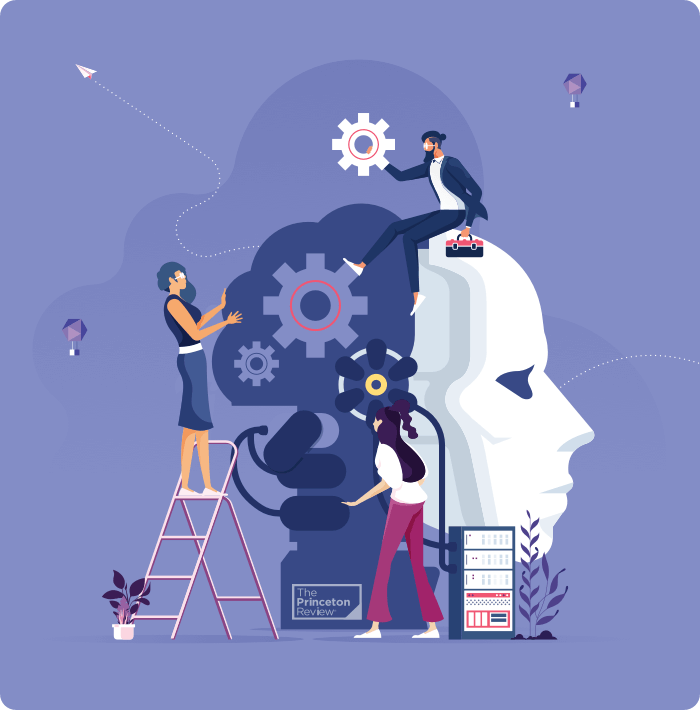
How We Built It
40+ years of expertise built into the latest tech. We are the next evolution of learning.
Our Vision in AI
This is just the beginning of what we aim to accomplish. Many more areas await the smartest way to achieve your goal.
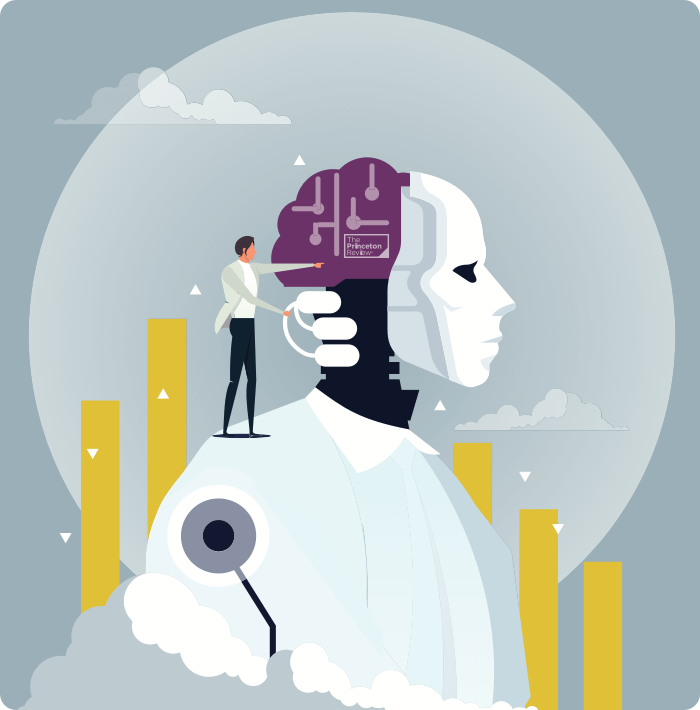
We've been busy bringing these great tools into your life
Ai college admissions essay counseling, ai homework essay review.
Enrollment Advisor
1-800-2REVIEW (800-273-8439) ext. 1
1-877-LEARN-30
Mon-Fri 9AM-10PM ET
Sat-Sun 9AM-8PM ET
Student Support
1-800-2REVIEW (800-273-8439) ext. 2
Mon-Fri 9AM-9PM ET
Sat-Sun 8:30AM-5PM ET
Partnerships
- Teach or Tutor for Us
College Readiness
International
Advertising
Affiliate/Other
- Enrollment Terms & Conditions
- Accessibility
- Cigna Medical Transparency in Coverage
Register Book
Local Offices: Mon-Fri 9AM-6PM
- SAT Subject Tests
Academic Subjects
- Social Studies
Find the Right College
- College Rankings
- College Advice
- Applying to College
- Financial Aid
School & District Partnerships
- Professional Development
- Advice Articles
- Private Tutoring
- Mobile Apps
- International Offices
- Work for Us
- Affiliate Program
- Partner with Us
- Advertise with Us
- International Partnerships
- Our Guarantees
- Accessibility – Canada
Privacy Policy | CA Privacy Notice | Do Not Sell or Share My Personal Information | Your Opt-Out Rights | Terms of Use | Site Map
©2024 TPR Education IP Holdings, LLC. All Rights Reserved. The Princeton Review is not affiliated with Princeton University
TPR Education, LLC (doing business as “The Princeton Review”) is controlled by Primavera Holdings Limited, a firm owned by Chinese nationals with a principal place of business in Hong Kong, China.
Help | Advanced Search
Computer Science > Machine Learning
Title: application of machine learning in agriculture: recent trends and future research avenues.
Abstract: Food production is a vital global concern and the potential for an agritech revolution through artificial intelligence (AI) remains largely unexplored. This paper presents a comprehensive review focused on the application of machine learning (ML) in agriculture, aiming to explore its transformative potential in farming practices and efficiency enhancement. To understand the extent of research activity in this field, statistical data have been gathered, revealing a substantial growth trend in recent years. This indicates that it stands out as one of the most dynamic and vibrant research domains. By introducing the concept of ML and delving into the realm of smart agriculture, including Precision Agriculture, Smart Farming, Digital Agriculture, and Agriculture 4.0, we investigate how AI can optimize crop output and minimize environmental impact. We highlight the capacity of ML to analyze and classify agricultural data, providing examples of improved productivity and profitability on farms. Furthermore, we discuss prominent ML models and their unique features that have shown promising results in agricultural applications. Through a systematic review of the literature, this paper addresses the existing literature gap on AI in agriculture and offers valuable information to newcomers and researchers. By shedding light on unexplored areas within this emerging field, our objective is to facilitate a deeper understanding of the significant contributions and potential of AI in agriculture, ultimately benefiting the research community.
Submission history
Access paper:.
- HTML (experimental)
- Other Formats
References & Citations
- Google Scholar
- Semantic Scholar
BibTeX formatted citation

Bibliographic and Citation Tools
Code, data and media associated with this article, recommenders and search tools.
- Institution
arXivLabs: experimental projects with community collaborators
arXivLabs is a framework that allows collaborators to develop and share new arXiv features directly on our website.
Both individuals and organizations that work with arXivLabs have embraced and accepted our values of openness, community, excellence, and user data privacy. arXiv is committed to these values and only works with partners that adhere to them.
Have an idea for a project that will add value for arXiv's community? Learn more about arXivLabs .
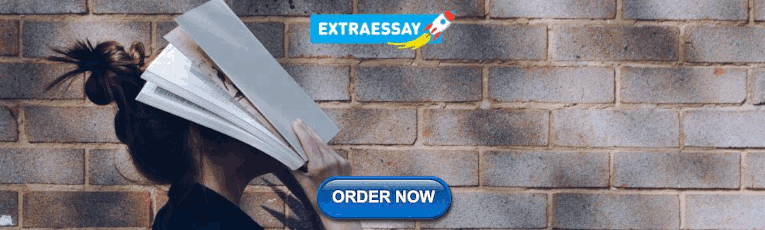
IMAGES
VIDEO
COMMENTS
AI-powered essay analysis and feedback. HyperWrite's AI Essay Reviewer is an innovative tool that analyzes your essay and provides comprehensive feedback on its structure, grammar, coherence, and relevance. Leveraging the power of AI, it offers an overall rating and detailed feedback on each aspect of your essay, making it an invaluable tool ...
Our free essay checking tool gives your essay one final review of usage, grammar, spelling, and punctuation. You can feel great every time you write an essay. Utilize our AI-powered essay and paper checker for precise analysis and correction. Enhance your writing with our efficient AI essay and paper checker tool.
Popular. $5.99 / month. Review all your essays multiple times for insightful feedback along your writing journey. Great for students and teachers. ∼200,000 words / month. 1,000,000 characters / month. Ultimate. $14.99 / month. The power plan for teachers and students reviewing hundreds of essays a month and saving days of work.
The Princeton Review 2023 AI Essay users rate us a solid 4 out of 5 stars! 3 Easy Steps to Writing a Better Essay. Upload your essay. Our AI-powered tutor will instantly go to work. Use actionable feedback to write a better essay! Launching Special! 50% OFF. 10 Essay edits. Tailored Feedback. Spelling & Grammar Review.
Popular. $5.99 / month. Review all your essays multiple times for insightful feedback along your writing journey. Great for students and teachers. ∼200,000 words / month. 1,000,000 characters / month. Ultimate. $14.99 / month. The power plan for teachers and students reviewing hundreds of essays a month and saving days of work.
Review all corrections in detail by unlocking unlimited access ... You won't pay more based on the number of words or characters. Our AI Proofreader is ideal for academic papers and dissertations! What is the turnaround time for the AI proofreader? The exact time depends on the length of your document, but, in most cases, the proofreading ...
College essay checker designed by admissions experts: detailed review, admission rubric scores, and personalized recommendations. Get free beta access now. ... Say goodbye to long waits for essay feedback. Cutting-edge AI tool will provide you assessment and suggest next steps in seconds. Get started free.
Unlike other AI-powered writing tools, the Academic Essay Feedback tool isn't limited to giving feedback on sentence- or language-level issues alone, like grammar or spelling. Instead, it provides feedback on areas like essay structure and organization, how well students support their arguments, introduction and conclusion, and style and tone.
Scribbr is committed to protecting academic integrity. Our plagiarism checker, AI Detector, Citation Generator, proofreading services, paraphrasing tool, grammar checker, summarizer, and free Knowledge Base content are designed to help students produce quality academic papers. We make every effort to prevent our software from being used for ...
Improve your writing with AI. Improve your academic performance and write like a pro without cheating or jeopardizing your degree. Get access to free AI writing tools, useful ChatGPT prompts, the latest articles about AI, and more. Start writing.
Example of essays and research papers written with Samwell AI. 5,000 words and. MLA Citation. Analysis of Marketing Mix Strategies for Wal-Mart Company. View essay. 15,000 words and. APA Citation. Uploaded PDFs. Performance Evaluation of Portfolio Managers of Mutual and Hedge Funds.
1. Informality: The essay is written in a somewhat casual and informal tone. While this makes the essay sound conversational and genuine, an overly casual tone might not be optimal for a college application. For example, phrases like "Hope that helps and brings a smile to your face." may seem too relaxed.
You can unlock unlimited credits, unlimited autocomplete, unlimited sources, and more for $14 per month. Conclusions. Overall, EssayGenius and JotBot were the best AI tools I tested. I was ...
Free proofreading tool. With QuillBot, you have the best free proofreading tool in your pocket, on your browser, or in Word whenever you need it.. Writing is a lot of work, and doing it well means revising and revising again. For writers with high standards in every genre and field, online proofreading is an invaluable resource—a must-have.
Here are a few of them —. 1. Saves time. Using best AI for essay writing has its own benefits. Students can take care of the research process while these AI tools write the essays for them. Be it an essay topic or a full-length essay generation, it saves a bunch of students' time. 2. Boosts productivity.
Experience seamless content evaluation with our AI-powered Peer Review Generator. Improve your writing quality, receive unbiased feedback, and fast-track your path to publication. Harness the power of artificial intelligence to drive your academic and professional success. Try it today and elevate your writing to new heights!
Write better essays, in half the time, with your AI writing assistant. EssayGenius uses cutting-edge AI to help you write your essays like never before. Generate ideas, rephrase sentences, and have your essay structure built for you. EssayGenius lets you write better essays, in less time. Our AI tools help you generate new paragraphs, complete ...
Literature review generators minimize errors by ensuring access to the most accurate data, hence enhancing the quality of the review. Pedagogical Benefits: Using a Literature review generator does not only provide a quick fix for students but it also serves as a tool for learning. It allows the users to understand how professional literature ...
If that was the case, it would be reasonable to use AI to screen papers before peer review process, using AI as a tool to desk reject papers based on these macroscopic features as part of the pre ...
Creates a comprehensive academic literature review with scholarly resources based on a specific research topic. HyperWrite's AI Literature Review Generator is a revolutionary tool that automates the process of creating a comprehensive literature review. Powered by the most advanced AI models, this tool can search and analyze scholarly articles, books, and other resources to identify key themes ...
Understanding HIX EssayGPT: The AI-powered Academic Companion. At its core, HIX EssayGPT is an AI essay writer developed by HIX.AI to assist in all facets of academic writing. From generating initial drafts to refining and polishing a final piece, it serves an array of functionalities aimed at easing the often daunting task of essay writing.
Artificial Intelligence Review is a fully open access journal publishing state-of-the-art research in artificial intelligence and cognitive science. Publishes critical evaluations of applications, techniques, and algorithms in the field. Provides a platform for researchers and application developers. Presents refereed survey and tutorial ...
Jasper's AI summary generator provides lightning-fast summaries for articles, research papers, and pretty much any lengthy content. Its AI summarizing tool stands out by condensing up to 5,000 characters in over 25 languages. Also, it has customizable formality levels and a whole host of advanced features.
However, despite the demonstrated potential of AI, its widespread adoption in sectors like manufacturing remains limited. Our comprehensive review of recent literature, including standards and reports, pinpoints key challenges: system integration, data-related issues, managing workforce-related concerns and ensuring trustworthy AI.
Artificial intelligence (AI) has been widely adopted in the service sector to enhance the customer experience and gain a competitive advantage. However, there are a limited number of papers that focus on the relationship between AI and customer experience, and there is no clear framework to reveal how AI influences the customer experience.
Over the last four decades, studies have investigated the incorporation of Artificial Intelligence (AI) into education. A recent prominent AI-powered technology that has impacted the education sector is ChatGPT. This article provides a systematic review of 14 empirical studies incorporating ChatGPT into various educational settings, published in 2022 and before the 10th of April 2023—the ...
This synergy of techniques ensures minimal loss of capability, supporting efficient AI deployments across various settings . Computational efficiency. This is another domain where new research papers are pushing the boundaries of LLMs. Here are some key research papers published recently which are improving the computation efficiency of LLMs. 1.
AI Homework Essay Review Learn More Learn More . AI College Admissions Essay Counseling Learn More . AI Homework Essay Review Learn More . Enrollment Advisor. 1-800-2REVIEW (800-273-8439) ext. 1. 1-877-LEARN-30. FAQ. Hours. Mon-Fri 9AM-10PM ET. Sat-Sun 9AM-8PM ET. Student Support. 1-800-2REVIEW (800-273-8439) ext. 2.
Background: Artificial intelligence (AI) can radically change almost every aspect of the human experience. In the medical field, there are numerous applications of AI and subsequently, in a relatively short time, significant progress has been made. Cardiology is not immune to this trend, this fact being supported by the exponential increase in the number of publications in which the algorithms ...
Food production is a vital global concern and the potential for an agritech revolution through artificial intelligence (AI) remains largely unexplored. This paper presents a comprehensive review focused on the application of machine learning (ML) in agriculture, aiming to explore its transformative potential in farming practices and efficiency enhancement. To understand the extent of research ...