
An official website of the United States government
The .gov means it’s official. Federal government websites often end in .gov or .mil. Before sharing sensitive information, make sure you’re on a federal government site.
The site is secure. The https:// ensures that you are connecting to the official website and that any information you provide is encrypted and transmitted securely.
- Publications
- Account settings
Preview improvements coming to the PMC website in October 2024. Learn More or Try it out now .
- Advanced Search
- Journal List
- J Biomed Phys Eng
- v.9(6); 2019 Dec
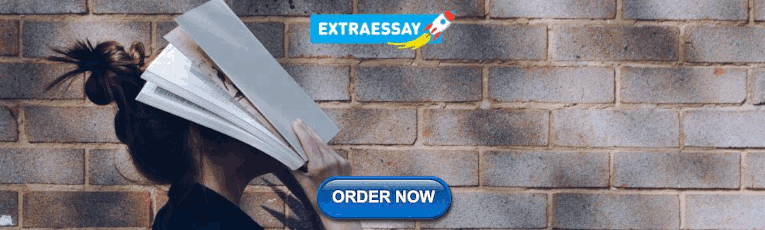
A Systematic Review of Nutrition Recommendation Systems: With Focus on Technical Aspects
1 PhD Candidate, Health Information Management Department, School of Allied Medical Sciences, Tehran University of Medical Sciences, Tehran, Iran;
2 PhD, Professor, Health Information Management Department, School of Allied Medical Sciences, Tehran University of Medical Sciences, Tehran, Iran;
L. Azadbakht
3 PhD, Professor, Department of Community Nutrition, School of Nutritional Sciences and Dietetics, Tehran University of Medical Sciences, Tehran, Iran;
K. B. Lankarani
4 MD, Professor, Health Policy Research Center, Institute of Health, Shiraz University of Medical Sciences, Shiraz, Iran;
Sh. R. Niakan Kalhori
5 PhD, Associate Professor, Health Information Management Department, School of Allied Medical Sciences, Tehran University of Medical Sciences, Tehran, Iran;
B. Honarvar
6 MD, Associate Professor, Health Policy Research Center, Institute of Health, Shiraz University of Medical Sciences, Shiraz, Iran;
7 PhD, Department of Food Science and Technology, National Nutrition and Food Technology Research Institute, Faculty of Nutrition Sciences and Food Technology, Shahid Beheshti University of Medical Sciences, Tehran, Iran
S. M. Ayyoubzadeh
S. zakerabasali, y. jalilpiran.
8 PhD student, Department of Clinical Nutrition, School of Nutritional Sciences and Dietetics, Tehran University of Medical Sciences, Tehran, Iran;
Background:
Nutrition informatics has become a novel approach for registered dietitians to practice in this field and make a profit for health care. Recommendation systems considered as an effective technology into aid users to adjust their eating behavior and achieve the goal of healthier food and diet. The purpose of this study is to review nutrition recommendation systems (NRS) and their characteristics for the first time.
Material and Methods:
The systematic review was conducted using a comprehensive selection of scientific databases as reference sources, allowing access to diverse publications in the field. The process of articles selection was based on the PRISMA strategy. We identified keywords from our initial research, MeSH database and expert’s opinion. Databases of PubMed, Web of Sciences, Scopus, Embase, and IEEE were searched. After evaluating, they obtained records from databases by two independent reviewers and inclusion and exclusion criteria were applied to each retrieved work to select those of interest. Finally, 25 studies were included.
Hybrid recommender systems and knowledge-based recommender systems with 40% and 32%, respectively, were the mostly recommender types used in NRS. In NRS, rule-based and ontology techniques were used frequently. The frequented platform that applied in NRS was a mobile application with 28%.
Conclusion:
If NRS was properly designed, implemented and finally evaluated, it could be used as an effective tool to improve nutrition and promote a healthy lifestyle. This study can help to inform specialists in the nutrition informatics domain, which was necessary to design and develop NRS.
Introduction
Nutrition is the essential basis for health and development of human life from the earliest stage of fetal development into old age. Healthy food and proper nutrition are indubitable requirements for survival, physical development, mental growth, performance and productivity, health, and well-being [ 1 - 5 ].
The combination of information technology (IT), information sciences and nutrition has generated the concept of nutrition informatics. This branch of health informatics has become a novel approach for registered dietitians and dietetic technicians to practice in this field and make a profit for health care. The first scientific use of term ‘nutrition informatics’ referred to 1996; however, the dietitians have been applying the nutrition information and assuming technology for decades. The first paper exhibiting the potential usage of the computer to analyze dietary intake was published in 1962. Health care has included IT to establish patient care by the use of electronic health records, gather population data through the collection of personal health records and support clinical research via the reuse of data. Moreover, dietitians have involved particular electronic tools developed for managing patient tray service, indexing, and evaluation of nutrients [ 6 - 17 ].
Though most people have been informed about the significance of healthy eating habits, they incline to neglect suitable behaviors because of urban lifestyle and/or unwillingness to spend cognitive attempt on food provision. Those barriers prevent people from healthy food intake [ 18 - 20 ]. Nutrition recommendation systems (NRS) are one of the main technologies applyed in nutrition informatics scope. They are explored as an effective tool in order to support users for changing their eating behavior and reaching the goal of healthier food choices. NRS not only recommends users’ preferences for food, but also recommends healthy diet choices; in addition, it can advise appropriate diet and keep pursue of eating behavior, realize health problems, and lead into alteration user behavior [ 21 - 24 ].
Generally, recommendation systems have developed an efficient and effective technology to extract valuable information and then use it in an effectual way. A recommender system can predict the preferences of users for unrated items and recommend new items to users. The capability of these systems depends on technical requirements and proper design based on system types and functionality. Several various techniques have been proposed for developing personalized recommendations; these varieties in applied techniques and design may create various types of recommendation systems, including collaborative filtering recommender systems (CF), content-based recommender systems (CB), knowledge-based recommender systems (KBS) and hybrid recommender systems (HRS) [ 21 , 24 - 28 ].
There has been no detailed investigation to review NRS and their characteristics. The purpose of this study is to review recommendation systems in the nutrition field with focus on characteristics, types and evaluation methods. Moreover, this study uses a systematic review approach based on PRISMA guideline.
This systematic review responds to the following research questions:
- RQ1: Which types of recommendation systems are mostly used in the field of nutrition?
- RQ2: Which Artificial Intelligence (AI) techniques or intelligent methods are applied in NRS?
- RQ3: What are the main modules of these NRS?
- RQ4: Which platforms design NRS?
Material and Methods
This systematic review was conducted based on the preferred reporting items for systematic reviews and meta-analysis (PRISMA). Figure 1 displays the process of PRISMA for data collection and analysis.

Process of PRISMA for data collection and analysis
Search strategy
The papers from PubMed, Scopus, Embase, Web of Science, IEEE (Institute of Electrical and Electronics Engineers) and Google Scholar databases were searched without time limitation (up 10 January 2019).
The PICO (population, intervention, comparison, and outcome) criteria were used to define the search string: population (P), intervention (I), comparison (C), and outcome (O). The population was defined based on whom systems were designed for. The intervention was the usage of recommendation systems in the nutrition field. The comparison was excluded and the outcomes were the characteristics and types of developed NRS as well as their methods of evaluation.
We identified keywords from our initial research and matched them with those existing on the database of Medical Subject Headings (MeSH), which is managed by the US National Library of Medicine. In addition, we used an expert working in the medical branch libraries to improve the quality of searches. The search strings based on MeSH and title, abstract and keywords were as follows: (nutrition* OR diet OR food* OR meal) and (recommend* system*).
Inclusion and exclusion criteria
The inclusion criteria were original articles and proceedings. Moreover, just articles which focus on NRS and have health-promotion goals, and papers up 10 January 2019 were included. Exclusion criteria were Non-English papers, any types of publication other than journal articles and proceedings (review papers, letters, etc.), and non-human study papers. Furthermore, papers focusing on systems other than recommendation systems such as expert, clinical decision support systems and those only working with business and marketing purposes were excluded.
Selection process
In the primary screening, two individual reviewers screened titles and abstracts of the articles based on the research and PICO questions and the papers were classified into three sets completely. The first set (number 1) contained the papers with certainly inclusion criteria. The next set (number 2) contained the papers that their inclusion criteria were not clear for reviewers. Lastly, set (number 0) referred to the papers with no inclusion criteria, which were ineligible and excluded from the screening. The reviewers assessed the papers with different assigned numbers and discussed them. All the papers with similar numbers (one or two) were involved for the next stage. Following, the full text of the involved papers was achieved for the second-stage of screening and then evaluated by two reviewers. Finally, we were certain about 25 papers, then summarized and reported them in tables and charts according to the aims of the study.
Data extraction
In this phase, 6 variables were extracted in order to response to the research questions. These variables contained the name of the system, type of recommendation system, artificial intelligence (AI) or intelligent techniques applied in recommendation system, system modules and type of system’s platform.
Table 1 shows data extracted from the 25 articles reviewed [ 23 , 29 - 52 ]. The sixth variables of the selected papers are presented in Table 1 .
A brief Summary of Evaluated Technical Result for each Unit
Type of recommendation system
As presented in the fourth column of Table 1 , the types of recommendation system were studied. The frequency (%) of recommendation system types is presented in Figure 2 .

The frequency (%) of recommendation system types
AI or intelligent techniques apply in recommendation systems
As shown in the fifth column of Table 1 , various AI or intelligent techniques have been applied in recommendation systems. The types of these techniques with their frequency in the researches are stated in Figure 3 .

Frequncy of AI or intelligent techniques applied in recommendation systems
The seventh column of Table 1 illustrates the type of system’s platform. Figure 4 demonstrates the frequency of the type of system’s platform. Based on the chart, the frequented platform applied in NRS was a mobile application with 28%.

Frequncy of type of system’s platform
Despite the various application of recommendation systems in many domains, there is not much evidence for using recommendation systems in health informatics or medicine [ 53 ]. According to the Valdez framework [ 53 ], if we want to design or assess the health recommender system, it will be necessary to consider 3 main aspects. First of all, we should understand the health domain. In this aspect, answering these two questions is very important: 1) which one of following items is recommended (nutrition, medicine, sport)? In addition, 2) who is it recommended to (the patient, the doctor, the nurse, policy maker)?
Another aspect that should be considered is the understanding of technical specifications and data analysis methods used in health recommendation system. The third aspect that needs to be addressed in the analysis of health recommendation system is the understanding of the methods and techniques of evaluating recommender systems. Besides, this paper for the first time reviewed the technical specifications of NRS.
The items in the results have been discussed in the following:
Type of recommendation systems
Hybrid recommender systems (HRS) are the mostly used recommender type in nutrition recommendation systems ( Figure 2 ). As these systems use a combination of other systems type, they could compensate disadvantages of one type of recommendation system by another type. Moreover, hybrid types are applied in order to increase the recommender’s performance [ 21 , 25 ].
AI or intelligent techniques applied in recommendation systems
In NRS, rule-based techniques are frequently used ( Figure 3 ). The reason behind that might be the simplicity, transparency, and consistency of rule-based techniques with nutrition knowledge. Ontology techniques also have been used for the same reasons to show the knowledge and concepts of relations in a clear manner.
Cold start, which is one of the classic problems in recommendation systems, happens when a new user uses the system with no preferences [ 54 ]. Although this situation seems to not occur frequently in NRS, recommendation of collaborative filtering, which is content-based, is made by [ 41 ] to overcome this issue.
Type of system’s platform and their structure
Owing to the penetration of smartphones, their processing, connectivity capabilities and their accessibility [ 55 , 56 ], mobile applications had a growth in the medical field [ 56 ]. As it has been shown that mobile apps have potential effects on health behavior change [ 57 ] and chronic disease management [ 58 ], they have been used in the most of reviewed systems for NRS as shown in Figure 4 .
In addition, web technology, due to its cross-platform specificity leading into the use of multiple clients with different hardware and operating system, is popular for these recommendation systems.
This is the first systematically review of the recommendation systems in the nutrition field with focus on characteristics and types. The study offers some important insights into the condition of recommendation systems in the nutrition field with focus on technical aspects. This study can help to inform specialists in the nutrition informatics domain, which was necessary to design and develop NRS.
However, there are many mobile applications in the nutrition field useing “recommendation system” technology which have not been mentioned in the design methodology [ 57 ]. This study concentrates only on research, which explicitly discusses the use of the recommendation system.
In this paper, we systematically reviewed the recommender systems in nutrition scope with a focus on characteristics and types. The results of this investigation show that hybrid recommender systems (HRS) are mostly used recommender type in NRS. Many AI or intelligent techniques applied in recommendation systems, which are one of the most frequented techniques, are systems based on rules. Furthermore, the frequented platform applied in NRS was a mobile application. If NRS is properly designed, implemented, and finally evaluated, it can be used as an effective tool to improve nutrition and promote a healthy lifestyle.
Acknowledgement
The authors would like to gratefully acknowledge the contribution department of health information management and department of community nutrition in Tehran University of Medical Sciences. This paper was developed as part of a Ph.D. thesis that is funded and supported jointly by Tehran University of Medical Sciences and Shiraz University of Medical Sciences.
Conflict of Interest: The authors declare that there is no conflict of interest regarding the publication of this manuscript.
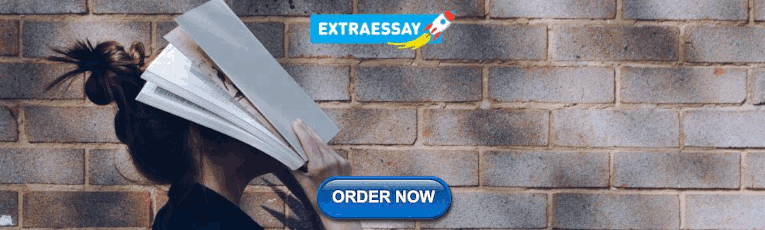
Hybrid Diet Recommender System Using Machine Learning Technique
- Conference paper
- First Online: 25 May 2023
- Cite this conference paper
- N. Vignesh 15 ,
- S. Bhuvaneswari 15 ,
- Ketan Kotecha 16 &
- V. Subramaniyaswamy ORCID: orcid.org/0000-0001-5328-7672 15
Part of the book series: Lecture Notes in Networks and Systems ((LNNS,volume 647))
Included in the following conference series:
- International Conference on Hybrid Intelligent Systems
511 Accesses
Obesity is a dangerous epidemic worldwide and is the root cause of many diseases. It is difficult for people to have the same diet with an optimized calorie intake as it becomes monotonous and boring. It will be much better if a dynamic diet can be generated depending upon the calories burnt by a person and their current Body Mass Index (BMI). The active diet planner could provide a person with some change regarding the food consumed and, at the same time, regulate the calorie intake depending upon the user’s requirements. Previously proposed models are either focused only on one aspect of the nutritional information of food or on presenting a diet for a specific issue which is presently facing by user. The proposed system utilizes more balanced approach that focuses on most of the nutritional features of food, and can recommend different foods to a user depending on their BMI. The fat, carbohydrate, calorie, and protein content of food and the BMI of the user are considered while preparing the diet chart. K-means clustering is used to cluster food of similar nutritional content, and a random forest classifier is then used to build the model to recommend a diet for the user. The result of the system cannot be compared with a standard metric. Still, some of the factors that influence the performance of the diet recommender system include the truthfulness of the user while providing information to the design and the accuracy at which the parameters for the model had been set. The advantage of the system comes from the fact that the user has more options to choose from within their suitable range.
This is a preview of subscription content, log in via an institution to check access.
Access this chapter
- Available as PDF
- Read on any device
- Instant download
- Own it forever
- Available as EPUB and PDF
- Compact, lightweight edition
- Dispatched in 3 to 5 business days
- Free shipping worldwide - see info
Tax calculation will be finalised at checkout
Purchases are for personal use only
Institutional subscriptions
World Health Organization (WHO): Fact Sheet, 312 (2011)
Google Scholar
World Health Organization-Benefits of healthy diet https://www.who.int/initiatives/behealthy/healthy
Toledo, R.Y., Alzahrani, A.A., Martinez, L.: A food recommender system considers nutritional information and user preferences. IEEE Access 7 , 96695–96711 (2019)
Article Google Scholar
Shrimal, M., Khavnekar, M., Thorat, S., Deone, J.: Nutriflow: a diet recommendation system (2021). SSRN 3866863
Iwendi, C., Khan, S., Anajemba, J.H., Bashir, A.K., Noor, F.: Realizing an efficient IoMT-assisted patient diet recommendation system through a machine learning model. IEEE Access 8 , 28462–28474 (2020)
Vasireddy, P.: An autonomous diet recommendation bot using intelligent automation. In: 2020 4th International Conference on Intelligent Computing and Control Systems (ICICCS), pp. 449–454. IEEE (May 2020)
Chavan, P., Thoms, B., Isaacs, J.: A recommender system for healthy food choices: building a hybrid model for recipe recommendations using big data sets. In: Proceedings of the 54th Hawaii International Conference on System Sciences, p. 3774 (January 2021)
Tabassum, N., Rehman, A., Hamid, M., Saleem, M., Malik, S., Alyas, T.: Intelligent nutrition diet recommender system for diabetic patients. Intell. Autom. Soft Comput. 30 (1), 319–335 (2021)
Manoharan, S.: Patient diet recommendation system using K clique and deep learning classifiers. J. Artif. Intell. 2 (02), 121–130 (2020)
Kim, J.-H., Lee, J.-H., Park, J.-S., Lee, Y.-H., Rim, K.-W.: Design of diet recommendation system for healthcare service based on user information. In: 2009Fourth International Conference on Computer Sciences and Convergence Information Technology, pp. 516–518 (2009). https://doi.org/10.1109/ICCIT.2009.293
Geetha, M., Saravanakumar, C., Ravikumar, K., &Muthulakshmi, V.: Human body analysis and diet recommendation system using machine learning techniques (2021)
Hsiao, J.H., Chang, H.: SmartDiet: a personal diet consultant for healthy meal planning. In: 2010 IEEE 23rd International Symposium on Computer-Based Medical Systems (CBMS), pp. 421–425. IEEE (October 2010)
Princy, J., Senith, S., Kirubaraj, A.A., Vijaykumar, P.: A personalized food recommender system for women considering nutritional information. Int. J. Pharm. Res. 13 (2) (2021)
Agapito, G., Calabrese, B., Guzzi, P.H., Cannataro, M., Simeoni, M., Caré, I., Pujia, A., et al.: DIETOS: a recommender system for adaptive diet monitoring and personalized food suggestion. In 2016 IEEE 12th International Conference on Wireless and Mobile Computing, Networking and Communications (WiMob), pp. 1–8. IEEE (October 2016)
Padmapritha, T., Subathra, B., Ozyetkin, M.M., Srinivasan, S., Bekirogulu, K., Kesavadev, J., Sanal, G., et al.: Smart artificial pancreas with diet recommender system for elderly diabetes. IFAC-PapersOnLine 53 (2), 16366–16371 (2020)
Ghosh, P., Bhattacharjee, D., Nasipuri, M.: Dynamic diet planner: a personal diet recommender system based on daily activity and physical condition. IRBM 42 (6), 442–456 (2021)
Chavan, S.V., Sambare, S.S., Joshi, A.: Diet recommendationsare based on prakriti and season using fuzzy ontology and type-2 fuzzy logic. In: 2016 International Conference on Computing Communication Control and Automation (ICCUBEA), pp. 1–6. IEEE (August 2016)
Pawar, R., Lardkhan, S., Jani, S., Lakhi, K.: NutriCure: a disease-based food recommender system. Int. J. Innov. Sci. Res. Technol. 6 , 2456–2165
Hernandez-Ocana, B., Chavez-Bosquez, O., Hernandez-Torruco, J., Canul-Reich, J., Pozos-Parra, P.: Bacterial foraging optimization algorithm for menu planning. IEEE Access 6 , 8619–8629 (2018)
Download references
Acknowledgments
The authors gratefully acknowledge the Science and Engineering Research Board (SERB), Department of Science & Technology, India, for the financial support through the Mathematical Research Impact Centric Support (MATRICS) scheme (MTR/2019/000542). The authors also acknowledge SASTRA Deemed University, Thanjavur, for extending infrastructural support to carry out this research.
Author information
Authors and affiliations.
School of Computing, SASTRA Deemed University, Thanjavur, 613401, India
N. Vignesh, S. Bhuvaneswari & V. Subramaniyaswamy
Symbiosis Centre for Applied Artificial Intelligence, Symbiosis International (Deemed University), Pune, India
Ketan Kotecha
You can also search for this author in PubMed Google Scholar
Corresponding author
Correspondence to V. Subramaniyaswamy .
Editor information
Editors and affiliations.
Faculty of Computing and Data Science, FLAME University, Pune, Maharashtra, India
Ajith Abraham
National University of Kaohsiung, Kaohsiung, Taiwan
Tzung-Pei Hong
Symbiosis International University, Pune, India
University of Jinan, Jinan, China
Scientific Network for Innovation and Research Excellence, Machine Intelligence Research Labs, Mala, Kerala, India
Pooja Manghirmalani Mishra
Scientific Network for Innovation and Research Excellence, Machine Intelligence Research Labs, Auburn, WA, USA
Niketa Gandhi
Rights and permissions
Reprints and permissions
Copyright information
© 2023 The Author(s), under exclusive license to Springer Nature Switzerland AG
About this paper
Cite this paper.
Vignesh, N., Bhuvaneswari, S., Kotecha, K., Subramaniyaswamy, V. (2023). Hybrid Diet Recommender System Using Machine Learning Technique. In: Abraham, A., Hong, TP., Kotecha, K., Ma, K., Manghirmalani Mishra, P., Gandhi, N. (eds) Hybrid Intelligent Systems. HIS 2022. Lecture Notes in Networks and Systems, vol 647. Springer, Cham. https://doi.org/10.1007/978-3-031-27409-1_10
Download citation
DOI : https://doi.org/10.1007/978-3-031-27409-1_10
Published : 25 May 2023
Publisher Name : Springer, Cham
Print ISBN : 978-3-031-27408-4
Online ISBN : 978-3-031-27409-1
eBook Packages : Intelligent Technologies and Robotics Intelligent Technologies and Robotics (R0)
Share this paper
Anyone you share the following link with will be able to read this content:
Sorry, a shareable link is not currently available for this article.
Provided by the Springer Nature SharedIt content-sharing initiative
- Publish with us
Policies and ethics
- Find a journal
- Track your research
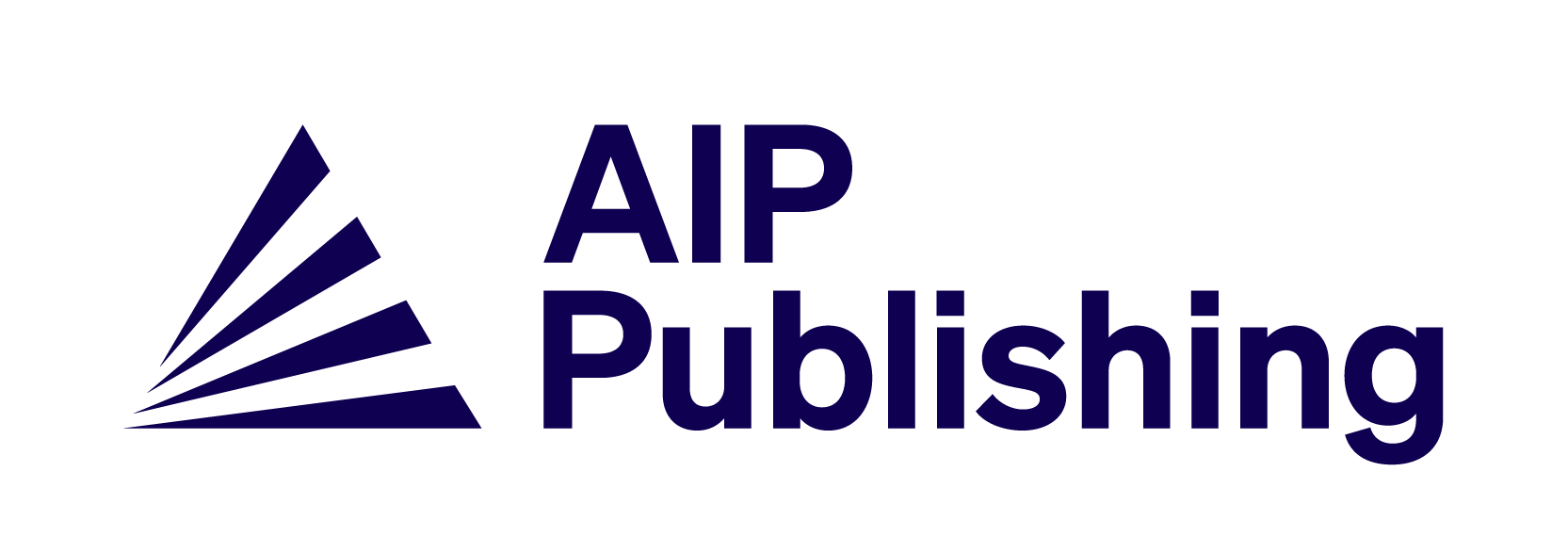
Yourcare: A diet and fitness recommendation system using machine learning algorithms
- Article contents
- Figures & tables
- Supplementary Data
- Peer Review
- Reprints and Permissions
- Cite Icon Cite
- Search Site
Sarathchandran Hemaraju , Parvathi Manoj Kaloor , Krishnamoorthy Arasu; Yourcare: A diet and fitness recommendation system using machine learning algorithms. AIP Conf. Proc. 4 May 2023; 2655 (1): 020011. https://doi.org/10.1063/5.0134382
Download citation file:
- Ris (Zotero)
- Reference Manager
The advancement in technology is gaining an increased significance in the world today. The advancement of machine learning has given rise to several automation systems and technologies like intelligent gaming, automotive cars, environmental solutions health care, etc. The paper focuses on the development of machine learning algorithms for a productive and functional health care system. The algorithms focused are employed in creating a system for recommending diet and fitness plans. One such algorithm in which we approach is the K-Means Clustering algorithm. This technique provides the diet and exercise routine with to the dataset trained. Therefore, our approach has helped in building the system in specialized way. Moreover, the system’s preciseness and accuracy are compared with different other machine learning algorithms for a better understanding of the model.
Sign in via your Institution
Citing articles via, publish with us - request a quote.
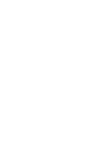
Sign up for alerts
- Online ISSN 1551-7616
- Print ISSN 0094-243X
- For Researchers
- For Librarians
- For Advertisers
- Our Publishing Partners
- Physics Today
- Conference Proceedings
- Special Topics
pubs.aip.org
- Privacy Policy
- Terms of Use
Connect with AIP Publishing
This feature is available to subscribers only.
Sign In or Create an Account
INSIGHTS FROM MACHINE-LEARNED DIET SUCCESS PREDICTION
Affiliation.
- 1 Qatar Computing Research Institute, Doha, Qatar, [email protected].
- PMID: 26776216
To support people trying to lose weight and stay healthy, more and more fitness apps have sprung up including the ability to track both calories intake and expenditure. Users of such apps are part of a wider "quantified self" movement and many opt-in to publicly share their logged data. In this paper, we use public food diaries of more than 4,000 long-term active MyFitnessPal users to study the characteristics of a (un-)successful diet. Concretely, we train a machine learning model to predict repeatedly being over or under self-set daily calories goals and then look at which features contribute to the model's prediction. Our findings include both expected results, such as the token "mcdonalds" or the category "dessert" being indicative for being over the calories goal, but also less obvious ones such as the difference between pork and poultry concerning dieting success, or the use of the "quick added calories" functionality being indicative of over-shooting calorie-wise. This study also hints at the feasibility of using such data for more in-depth data mining, e.g., looking at the interaction between consumed foods such as mixing protein- and carbohydrate-rich foods. To the best of our knowledge, this is the first systematic study of public food diaries.
Publication types
- Research Support, Non-U.S. Gov't
- Cluster Analysis
- Computational Biology / methods
- Computational Biology / statistics & numerical data
- Data Mining / methods
- Data Mining / statistics & numerical data
- Diet Records*
- Diet, Reducing*
- Energy Intake
- Energy Metabolism
- Machine Learning*
- Mobile Applications*
- Weight Reduction Programs / statistics & numerical data*
Research Article
Human Body Analysis and Diet Recommendation System using Machine Learning Techniques
- @INPROCEEDINGS{10.4108/eai.16-5-2020.2304203, author={M. Geetha and C. Saravanakumar and K. Ravikumar and V. Muthulakshmi}, title={Human Body Analysis and Diet Recommendation System using Machine Learning Techniques}, proceedings={Proceedings of the First International Conference on Advanced Scientific Innovation in Science, Engineering and Technology, ICASISET 2020, 16-17 May 2020, Chennai, India}, publisher={EAI}, proceedings_a={ICASISET}, year={2021}, month={1}, keywords={machine learning deep learning recommendation systems healthcare data mining}, doi={10.4108/eai.16-5-2020.2304203} }
- M. Geetha C. Saravanakumar K. Ravikumar V. Muthulakshmi Year: 2021 Human Body Analysis and Diet Recommendation System using Machine Learning Techniques ICASISET EAI DOI: 10.4108/eai.16-5-2020.2304203
- 1: Professor, Department of Computer Science and Engineering, School of Computing, SRM Institute of Science and Technology, Vadapalani, Chennai, India
- 2: Associate Professor, Department of Information Technology, St.Joseph’s Institute of Technology, OMR, Chennai - 600119, India
- 3: Associate Professor, Department of Computer Science and Engineering, Rrase College of Engineering, Chennai - 600119, India
- 4: Associate Professor, Department of Information Technology St. Joseph’s College of Engineering, OMR, Chennai 600 119, India
Nowadays the human faces the problem in maintaining the health condition in proper level. The problem is occurred due to excess consumption of the food which leads to obesity and also causes health issues. Mismanagement of the human health system is monitored using automated system which provides the report to the person. Traditional health monitoring model lacks in the accuracy of the report so it is improved by implementing intelligent diet control system. This provides the human choose and consumed proper food based on their health condition and extend the life time. The main objective of the proposed system analyses the body and provides the diet report to the person accurately. Exiting techniques are only support the person based on the current activity which leads the reliability problem. The proposed model uses the machine learning approach which analyzes the body of the human with pre medical history and predict the future health condition over the year. It provides the diet recommendation systems by considering the current and past food consumption record and recommend proper diet report with more reliable manner.
e-Health Monitoring System with Diet and Fitness Recommendation using Machine Learning
Ieee account.
- Change Username/Password
- Update Address
Purchase Details
- Payment Options
- Order History
- View Purchased Documents
Profile Information
- Communications Preferences
- Profession and Education
- Technical Interests
- US & Canada: +1 800 678 4333
- Worldwide: +1 732 981 0060
- Contact & Support
- About IEEE Xplore
- Accessibility
- Terms of Use
- Nondiscrimination Policy
- Privacy & Opting Out of Cookies
A not-for-profit organization, IEEE is the world's largest technical professional organization dedicated to advancing technology for the benefit of humanity. © Copyright 2024 IEEE - All rights reserved. Use of this web site signifies your agreement to the terms and conditions.
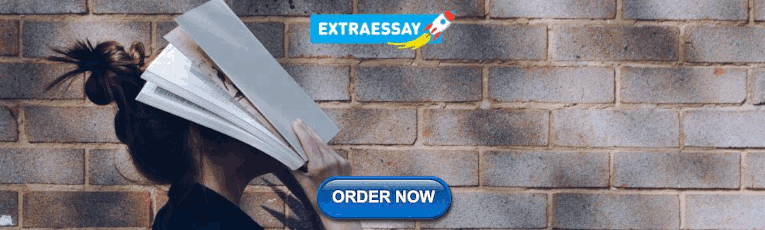
IMAGES
VIDEO
COMMENTS
The Research and Design of Recommendation System for Nutritional Combo Li and Yang 2016 China- Hybrid recommender systems (HRS) ... Design of Diet Recommendation System for Healthcare Service Based on User Information Kim et al. ... state-of-the-art and future perspectives. Machine Learning for Health Informatics: Springer; 2016. p. 391-414 ...
suggestions to balance a diet, nutrition aspects that are crucial for making food recommendations and creating a diet that is balanced are disregarded. IV. PROPOSED SYSTEM The system operates in a machine learning environment where it analyses user data to determine the best diet to follow. We separated the dataset into three groups: 1. Lunch ...
In food diet communication domain, images convey important information to capture users' attention beyond the traditional ingredient content, making it crucial to influence user-decision about the relevancy of a given diet. By using a deep learning-based image clustering method, this paper proposes an Explainable Food Recommendation system that ...
In today's culture, many people suffer from a range of ailments and illnesses. It's not always simple to recommend a diet right away. The majority of individuals are frantically trying to reduce weight, gain weight, or keep their health in check. Time has also become a potential stumbling block. The study relies on a database that has the exact amounts of a variety of nutrients. As a ...
The System operates within a Machine Learning Environment, processing user data to generate recommended diet plans. The dataset is divided into three categories: 1. Lunch Data 2. Breakfast Data 3. Dinner Data Utilizing machine learning algorithms, the system aims to deliver personalized diet recommendations tailored to individual user needs and ...
E-Health Monitoring System with Diet and Fitness Recommendation using Machine Learning : 2021: India: USDA Food Composition: Patients of Diabetes, Blood Pressure or Thyroid: Diet and Exercise: Collaborative: Online: Enhanced predictive learning approaches for tweaked diet proposal system in health care : 2020: India: Depending on the objective
It provides a variety of useful tools for statistical modelling and machine learning, including dimensional reduction, regression, clustering, and classification, through a standardized Python interface. This library is primarily written in Python and is based on NumPy, SciPy, and Matplotlib. The architecture of disease-based diet prediction ...
Abstract. Development of a recommendation system with help of Machine learning techniques to promote healthy eating habits and easy access for user for a tailored diet recommendation which can ...
The food recommendation system generates a diet for the user to help them reduce, gain, or maintain their current Body Mass Index. The system considers the current BMI of the user and recommends a diet depending on the function needed [ 11 ]. Fig. 1. Overview architecture of the system to recommend diet using BMI.
Machine Learning (ML) for generating healthy diet recommendations. The proposed system is known as Hybrid Recommender System (HRS). It involves a hybrid approach with Natural Language Processing (NLP) and machine learning. An algorithm named Intelligent Recommender for Healthy Diet (IR-HD) is proposed to analyze data and provide healthy diet
Index Terms - diet recommendation system, machine learning, diet plan. 1. INTRODUCTION. The recommending systems for the patients diets tracks the complete health conditions of the patients in a personalized manner and recommends the suitable and the variety of the food items that the patients can have. The dieting recommending system (DRS ...
Diet Recommendation System Using Machine Learning. Yuvraj Chibber, Dushyant Betala, Srividhya. A suggested system recommends a diet for you based on your physical characteristics and your end aim to help you achieve and maintain a healthy weight, lower your chance of developing chronic diseases, and improve your general health when combined ...
Therefore, we suggest a diet and exercise recommendation system that offers a list of foods and exercise suggestions based on user inputs like height, weight, and age used to determine their BMI. Along with other pertinent information including proteins, fats, calcium, iron, potassium, sodium, sugar, vitamin D, carbohydrates, and fibre, various ...
The paper focuses on the development of machine learning algorithms for a productive and functional health care system. The algorithms focused are employed in creating a system for recommending diet and fitness plans. One such algorithm in which we approach is the K-Means Clustering algorithm.
The Diet Recommendation System leverages user inputs such as medical data and the option of vegetarian or non-vegetarian meals from the two categories above to predict food items and includes a comparative review of the advantages and disadvantages of machine learning methods. In today's culture, many people suffer from a range of ailments and illnesses.
An IoT-Based Framework for Personalized Health Assessment and Recommendations Using Machine Learning. A framework is presented that assesses an individual's existing health conditions, enabling people to evaluate their well-being conveniently without the need for a doctor's consultation, and employs a two-stage classifier system to recommend ...
To. address this issue, we present a cloud based food recommendation system, called Diet-Right, for dietary recom mendations based on users' pathological reports. The model uses ant colony. a ...
cholesterol, blood pressure, and other factors. In this paper, we propose a diet recommendation system based on the user's physics details and ailments. This research includes different machine learning and deep learning techniques. The system learns from its training data, which includes daily calorie intake
We assist users by providing food recommendations based on their personal tastes using Collaborative Filtering and Fuzzy logic for creating a recommender framework. Users can monitor their steps as part of an operation or workout using the Android-based application's Step Counter or Pedometer.
In this paper, we use public food diaries of more than 4,000 long-term active MyFitnessPal users to study the characteristics of a (un-)successful diet. Concretely, we train a machine learning model to predict repeatedly being over or under self-set daily calories goals and then look at which features contribute to the model's prediction.
The main objective of the proposed system analyses the body and provides the diet report to the person accurately. Exiting techniques are only support the person based on the current activity which leads the reliability problem. The proposed model uses the machine learning approach which analyzes the body of the human with pre medical history ...
Index Terms - diet recommendation system, machine learning, diet plan. INTRODUCTION. The recommending systems for the patients diets tracks the complete health conditions of the patients in a personalized manner and recommends the suitable and the variety of the food items that the patients can have. The dieting recommending system (DRS) is ...
Health Monitoring, 2. Diet & Exercise Recommendation. In the Health Monitoring module, the system would suggest follow-up sessions until the reports come normal. For the Diet and Exercise Recommendation module, the algorithm that is used is a Decision tree for classification. To be precise, C4.5 is used to give recommendations of diet and exercise.