Introduction to Correlation and Linear Regression Analysis
- First Online: 19 July 2019
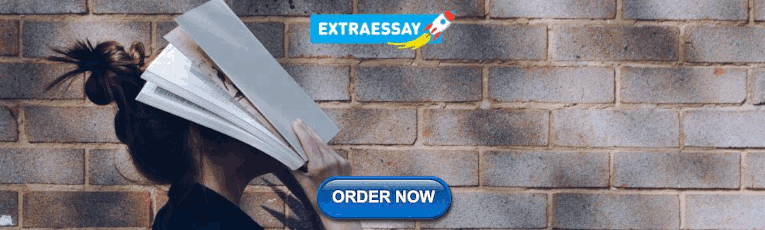
Cite this chapter
- Manoranjan Pal 3 &
- Premananda Bharati 4
2208 Accesses
11 Citations
3 Altmetric
This chapter gives some concepts of correlation and regression analysis. Correlation comes prior to regression analysis. It starts with the concept of simple correlation coefficient; which gives the degree of linear relationship between two variables. One should draw scatter diagram in order to judge whether there exists any linear relation between the two variables. The correlation coefficient is not only invariant under changes of unit of measurements but also unaffected by changes of origin for both variables. The value of the correlation coefficient always lies in-between –1 and +1. As the scatter points move closer to the straight line, it moves to –1 or +1 depending on whether the relation is negative or positive. The straight-line relation between the two variables can be found by Least Squares (LS) method. The goodness of fit of the linear regression can be measured by the square of the simple correlation coefficient. Multiple Linear Regression Model is an extension of Simple Linear Regression Model. In a multiple linear regression model, we have more than two independent variables. The goodness of fit in this case is measured by coefficient of determination which is the square of the multiple correlation coefficient.
This is a preview of subscription content, log in via an institution to check access.
Access this chapter
- Available as PDF
- Read on any device
- Instant download
- Own it forever
- Available as EPUB and PDF
- Compact, lightweight edition
- Dispatched in 3 to 5 business days
- Free shipping worldwide - see info
- Durable hardcover edition
Tax calculation will be finalised at checkout
Purchases are for personal use only
Institutional subscriptions
Center of Gravity is the \(\left( {\bar{x},\bar{y}} \right)\) point, where \(\bar{x}\) and \(\bar{y}\) are the arithmetic means of x values and y values, respectively.
If y causes x, then we just interchange the two symbols (i.e., x is denoted as y and y is denoted as x ) and y = a + bx and \(x = a^{{\prime }} + b^{{\prime }} y\) are equivalent.
For example, proportion of expenditure on food and proportion of expenditure on non-food always have perfect linear relation, sum of these two variables being 1, leading to the value of the correlation coefficient to be perfectly as 1.
If we are not specifically interested in the i th observation, we may simply write \(y = a + bx + e\) .
Strictly speaking, whether this transformation is valid depends on the nature of the error term. We have assumed that the errors are in a multiplicative form so that after transformation it is in the additive form.
The assumption of normal distribution is not needed for unbiased estimation of the regression coefficients. All we need is that e i has mean 0 for all i .
Author information
Authors and affiliations.
Economic Research Unit, Indian Statistical Institute, Kolkata, West Bengal, India
Manoranjan Pal
Biological Anthropology Unit, Indian Statistical Institute, Kolkata, West Bengal, India
Premananda Bharati
You can also search for this author in PubMed Google Scholar
Corresponding author
Correspondence to Manoranjan Pal .
Rights and permissions
Reprints and permissions
Copyright information
© 2019 Springer Nature Singapore Pte Ltd.
About this chapter
Pal, M., Bharati, P. (2019). Introduction to Correlation and Linear Regression Analysis. In: Applications of Regression Techniques. Springer, Singapore. https://doi.org/10.1007/978-981-13-9314-3_1
Download citation
DOI : https://doi.org/10.1007/978-981-13-9314-3_1
Published : 19 July 2019
Publisher Name : Springer, Singapore
Print ISBN : 978-981-13-9313-6
Online ISBN : 978-981-13-9314-3
eBook Packages : Mathematics and Statistics Mathematics and Statistics (R0)
Share this chapter
Anyone you share the following link with will be able to read this content:
Sorry, a shareable link is not currently available for this article.
Provided by the Springer Nature SharedIt content-sharing initiative
- Publish with us
Policies and ethics
- Find a journal
- Track your research

An official website of the United States government
The .gov means it’s official. Federal government websites often end in .gov or .mil. Before sharing sensitive information, make sure you’re on a federal government site.
The site is secure. The https:// ensures that you are connecting to the official website and that any information you provide is encrypted and transmitted securely.
- Publications
- Account settings
Preview improvements coming to the PMC website in October 2024. Learn More or Try it out now .
- Advanced Search
- Journal List
- Clin Kidney J
- v.14(11); 2021 Nov

Conducting correlation analysis: important limitations and pitfalls
Roemer j janse.
Department of Clinical Epidemiology, Leiden University Medical Center, Leiden, The Netherlands
Tiny Hoekstra
Department of Nephrology, Amsterdam Cardiovascular Sciences, Amsterdam UMC, Vrije Universiteit Amsterdam, Amsterdam, The Netherlands
Kitty J Jager
ERA-EDTA Registry, Department of Medical Informatics, Amsterdam Public Health Research Institute, Amsterdam UMC, University of Amsterdam, Amsterdam, The Netherlands
Carmine Zoccali
CNR-IFC, Center of Clinical Physiology, Clinical Epidemiology of Renal Diseases and Hypertension, Reggio Calabria, Italy
Giovanni Tripepi
Friedo w dekker, merel van diepen.
The correlation coefficient is a statistical measure often used in studies to show an association between variables or to look at the agreement between two methods. In this paper, we will discuss not only the basics of the correlation coefficient, such as its assumptions and how it is interpreted, but also important limitations when using the correlation coefficient, such as its assumption of a linear association and its sensitivity to the range of observations. We will also discuss why the coefficient is invalid when used to assess agreement of two methods aiming to measure a certain value, and discuss better alternatives, such as the intraclass coefficient and Bland–Altman’s limits of agreement. The concepts discussed in this paper are supported with examples from literature in the field of nephrology.
‘Correlation is not causation’: a saying not rarely uttered when a person infers causality from two variables occurring together, without them truly affecting each other. Yet, though causation may not always be understood correctly, correlation too is a concept in which mistakes are easily made. Nonetheless, the correlation coefficient has often been reported within the medical literature. It estimates the association between two variables (e.g. blood pressure and kidney function), or is used for the estimation of agreement between two methods of measurement that aim to measure the same variable (e.g. the Modification of Diet in Renal Disease (MDRD) formula and the Chronic Kidney Disease Epidemiology Collaboration (CKD-EPI) formula for estimating the glomerular filtration rate (eGFR)]. Despite the wide use of the correlation coefficient, limitations and pitfalls for both situations exist, of which one should be aware when drawing conclusions from correlation coefficients. In this paper, we aim to describe the correlation coefficient and its limitations, together with methods that can be applied to avoid these limitations.
The basics: the correlation coefficient
Fundamentals.
The correlation coefficient was described over a hundred years ago by Karl Pearson [ 1 ], taking inspiration from a similar idea of correlation from Sir Francis Galton, who developed linear regression and was the not-so-well-known half-cousin of Charles Darwin [ 2 ]. In short, the correlation coefficient, denoted with the Greek character rho ( ρ ) for the true (theoretical) population and r for a sample of the true population, aims to estimate the strength of the linear association between two variables. If we have variables X and Y that are plotted against each other in a scatter plot, the correlation coefficient indicates how well a straight line fits these data. The coefficient ranges from −1 to 1 and is dimensionless (i.e., it has no unit). Two correlations with r = −1 and r = 1 are shown in Figure 1A and B , respectively. The values of −1 and 1 indicate that all observations can be described perfectly using a straight line, which in turn means that if X is known, Y can be determined deterministically and vice versa. Here, the minus sign indicates an inverse association: if X increases, Y decreases. Nonetheless, real-world data are often not perfectly summarized using a straight line. In a scatterplot as shown in Figure 1C , the correlation coefficient represents how well a linear association fits the data.

Different shapes of data and their correlation coefficients. ( A ) Linear association with r = −1. ( B ) A linear association with r = 1. ( C ) A scatterplot through which a straight line could plausibly be drawn, with r = 0.50. ( D ) A sinusoidal association with r = 0. ( E ) A quadratic association with r = 0. ( F ) An exponential association with r = 0.50.
It is also possible to test the hypothesis of whether X and Y are correlated, which yields a P-value indicating the chance of finding the correlation coefficient’s observed value or any value indicating a higher degree of correlation, given that the two variables are not actually correlated. Though the correlation coefficient will not vary depending on sample size, the P-value yielded with the t -test will.
The value of the correlation coefficient is also not influenced by the units of measurement, but it is influenced by measurement error. If more error (also known as noise) is present in the variables X and Y , variability in X will be partially due to the error in X , and thus not solely explainable by Y . Moreover, the correlation coefficient is also sensitive to the range of observations, which we will discuss later in this paper.
An assumption of the Pearson correlation coefficient is that the joint distribution of the variables is normal. However, it has been shown that the correlation coefficient is quite robust with regard to this assumption, meaning that Pearson’s correlation coefficient may still be validly estimated in skewed distributions [ 3 ]. If desired, a non-parametric method is also available to estimate correlation; namely, the Spearman’s rank correlation coefficient. Instead of the actual values of observations, the Spearman’s correlation coefficient uses the rank of the observations when ordering observations from small to large, hence the ‘rank’ in its name [ 4 ]. This usage of the rank makes it robust against outliers [ 4 ].
Explained variance and interpretation
One may also translate the correlation coefficient into a measure of the explained variance (also known as R 2 ), by taking its square. The result can be interpreted as the proportion of statistical variability (i.e. variance) in one variable that can be explained by the other variable. In other words, to what degree can variable X be explained by Y and vice versa. For instance, as mentioned above, a correlation of −1 or +1 would both allow us to determine X from Y and vice versa without error, which is also shown in the coefficient of determination, which would be (−1) 2 or 1 2 = 1, indicating that 100% of variability in one variable can be explained by the other variable.
In some cases, the interpretation of the strength of correlation coefficient is based on rules of thumb, as is often the case with P-values (P-value <0.05 is statistically significant, P-value >0.05 is not statistically significant). However, such rules of thumb should not be used for correlations. Instead, the interpretation should always depend on context and purposes [ 5 ]. For instance, when studying the association of renin–angiotensin–system inhibitors (RASi) with blood pressure, patients with increased blood pressure may receive the perfect dosage of RASi until their blood pressure is exactly normal. Those with an already exactly normal blood pressure will not receive RASi. However, as the perfect dosage of RASi makes the blood pressure of the RASi users exactly normal, and thus equal to the blood pressure of the RASi non-users, no variation is left between users and non-users. Because of this, the correlation will be 0.
The linearity of correlation
An important limitation of the correlation coefficient is that it assumes a linear association. This also means that any linear transformation and any scale transformation of either variable X or Y , or both, will not affect the correlation coefficient. However, variables X and Y may also have a non-linear association, which could still yield a low correlation coefficient, as seen in Figure 1D and E , even though variables X and Y are clearly related. Nonetheless, the correlation coefficient will not always return 0 in case of a non-linear association, as portrayed in Figure 1F with an exponential correlation with r = 0.5. In short, a correlation coefficient is not a measure of the best-fitted line through the observations, but only the degree to which the observations lie on one straight line.
In general, before calculating a correlation coefficient, it is advised to inspect a scatterplot of the observations in order to assess whether the data could possibly be described with a linear association and whether calculating a correlation coefficient makes sense. For instance, the scatterplot in Figure 1C could plausibly fit a straight line, and a correlation coefficient would therefore be suitable to describe the association in the data.
The range of observations for correlation
An important pitfall of the correlation coefficient is that it is influenced by the range of observations. In Figure 2A , we illustrate hypothetical data with 50 observations, with r = 0.87. Included in the figure is an ellipse that shows the variance of the full observed data, and an ellipse that shows the variance of only the 25 lowest observations. If we subsequently analyse these 25 observations independently as shown in Figure 2B , we will see that the ellipse has shortened. If we determine the correlation coefficient for Figure 2B , we will also find a substantially lower correlation: r = 0.57.

The effect of the range of observations on the correlation coefficient, as shown with ellipses. ( A ) Set of 50 observations from hypothetical dataset X with r = 0.87, with an illustrative ellipse showing length and width of the whole dataset, and an ellipse showing only the first 25 observations. ( B ) Set of only the 25 lowest observations from hypothetical dataset X with r = 0.57, with an illustrative ellipse showing length and width.
The importance of the range of observations can further be illustrated using an example from a paper by Pierrat et al. [ 6 ] in which the correlation between the eGFR calculated using inulin clearance and eGFR calculated using the Cockcroft–Gault formula was studied both in adults and children. Children had a higher correlation coefficient than adults ( r = 0.81 versus r = 0.67), after which the authors mentioned: ‘The coefficients of correlation were even better […] in children than in adults.’ However, the range of observations in children was larger than the range of observations in adults, which in itself could explain the higher correlation coefficient observed in children. One can thus not simply conclude that the Cockcroft–Gault formula for eGFR correlates better with inulin in children than in adults. Because the range of the correlation influences the correlation coefficient, it is important to realize that correlation coefficients cannot be readily compared between groups or studies. Another consequence of this is that researchers could inflate the correlation coefficient by including additional low and high eGFR values.
The non-causality of correlation
Another important pitfall of the correlation coefficient is that it cannot be interpreted as causal. It is of course possible that there is a causal effect of one variable on the other, but there may also be other possible explanations that the correlation coefficient does not take into account. Take for example the phenomenon of confounding. We can study the association of prescribing angiotensin-converting enzyme (ACE)-inhibitors with a decline in kidney function. These two variables would be highly correlated, which may be due to the underlying factor albuminuria. A patient with albuminuria is more likely to receive ACE-inhibitors, but is also more likely to have a decline in kidney function. So ACE-inhibitors and a decline in kidney function are correlated not because of ACE-inhibitors causing a decline in kidney function, but because they have a shared underlying cause (also known as common cause) [ 7 ]. More reasons why associations may be biased exist, which are explained elsewhere [ 8 , 9 ].
It is however possible to adjust for such confounding effects, for example by using multivariable regression. Whereas a univariable (or ‘crude’) linear regression analysis is no different than calculating the correlation coefficient, a multivariable regression analysis allows one to adjust for possible confounder variables. Other factors need to be taken into account to estimate causal effects, but these are beyond the scope of this paper.
Agreement between methods
We have discussed the correlation coefficient and its limitations when studying the association between two variables. However, the correlation coefficient is also often incorrectly used to study the agreement between two methods that aim to estimate the same variable. Again, also here, the correlation coefficient is an invalid measure.
The correlation coefficient aims to represent to what degree a straight line fits the data. This is not the same as agreement between methods (i.e. whether X = Y ). If methods completely agree, all observations would fall on the line of equality (i.e. the line on which the observations would be situated if X and Y had equal values). Yet the correlation coefficient looks at the best-fitted straight line through the data, which is not per se the line of equality. As a result, any method that would consistently measure a twice as large value as the other method would still correlate perfectly with the other method. This is shown in Figure 3 , where the dashed line shows the line of equality, and the other lines portray different linear associations, all with perfect correlation, but no agreement between X and Y . These linear associations may portray a systematic difference, better known as bias, in one of the methods.

A set of linear associations, with the dashed line (- - -) showing the line of equality where X = Y . The equations and correlations for the other lines are shown as well, which shows that only a linear association is needed for r = 1, and not specifically agreement.
This limitation applies to all comparisons of methods, where it is studied whether methods can be used interchangeably, and it also applies to situations where two individuals measure a value and where the results are then compared (inter-observer variation or agreement; here the individuals can be seen as the ‘methods’), and to situations where it is studied whether one method measures consistently at two different time points (also known as repeatability). Fortunately, other methods exist to compare methods [ 10 , 11 ], of which one was proposed by Bland and Altman themselves [ 12 ].
Intraclass coefficient
One valid method to assess interchangeability is the intraclass coefficient (ICC), which is a generalization of Cohen’s κ , a measure for the assessment of intra- and interobserver agreement. The ICC shows the proportion of the variability in the new method that is due to the normal variability between individuals. The measure takes into account both the correlation and the systematic difference (i.e. bias), which makes it a measure of both the consistency and agreement of two methods. Nonetheless, like the correlation coefficient, it is influenced by the range of observations. However, an important advantage of the ICC is that it allows comparison between multiple variables or observers. Similar to the ICC is the concordance correlation coefficient (CCC), though it has been stated that the CCC yields values similar to the ICC [ 13 ]. Nonetheless, the CCC may also be found in the literature [ 14 ].
The 95% limits of agreement and the Bland–Altman plot
When they published their critique on the use of the correlation coefficient for the measurement of agreement, Bland and Altman also published an alternative method to measure agreement, which they called the limits of agreement (also referred to as a Bland–Altman plot) [ 12 ]. To illustrate the method of the limits of agreement, an artificial dataset was created using the MASS package (version 7.3-53) for R version 4.0.4 (R Corps, Vienna, Austria). Two sets of observations (two observations per person) were derived from a normal distribution with a mean ( µ ) of 120 and a randomly chosen standard deviation ( σ ) between 5 and 15. The mean of 120 was chosen with the aim to have the values resemble measurements of high eGFR, where the first set of observed eGFRs was hypothetically acquired using the MDRD formula, and the second set of observed eGFRs was hypothetically acquired using the CKD-EPI formula. The observations can be found in Table 1 .
Artificial data portraying hypothetically observed MDRD measurements and CKD-EPI measurements
The 95% limits of agreement can be easily calculated using the mean of the differences ( d ¯ ) and the standard deviation (SD) of the differences. The upper limit (UL) of the limits of agreement would then be UL = d ¯ + 1.96 * SD and the lower limit (LL) would be LL = d ¯ - 1.96 * SD . If we apply this to the data from Table 1 , we would find d ¯ = 0.32 and SD = 4.09. Subsequently, UL = 0.32 + 1.96 * 4.09 = 8.34 and LL = 0.32 − 1.96 * 4.09 = −7.70. Our limits of agreement are thus −7.70 to 8.34. We can now decide whether these limits of agreement are too broad. Imagine we decide that if we want to replace the MDRD formula with the CKD-EPI formula, we say that the difference may not be larger than 7 mL/min/1.73 m 2 . Thus, on the basis of these (hypothetical) data, the MDRD and CKD-EPI formulas cannot be used interchangeably in our case. It should also be noted that, as the limits of agreement are statistical parameters, they are also subject to uncertainty. The uncertainty can be determined by calculating 95% confidence intervals for the limits of agreement, on which Bland and Altman elaborate in their paper [ 12 ].
The limits of agreement are also subject to two assumptions: (i) the mean and SD of the differences should be constant over the range of observations and (ii) the differences are approximately normally distributed. To check these assumptions, two plots were proposed: the Bland–Altman plot, which is the differences plotted against the means of their measurements, and a histogram of the differences. If in the Bland–Altman plot the means and SDs of the differences appear to be equal along the x -axis, the first assumption is met. The histogram of the differences should follow the pattern of a normal distribution. We checked these assumptions by creating a Bland–Altman plot in Figure 4A and a histogram of the differences in Figure 4B . As often done, we also added the limits of agreement to the Bland–Altman plot, between which approximately 95% of datapoints are expected to be. In Figure 4A , we see that the mean of the differences appears to be equal along the x -axis; i.e., these datapoints could plausibly fit the horizontal line of the total mean across the whole x -axis. Nonetheless, the SD does not appear to be distributed equally: the means of the differences at the lower values of the x -axis are closer to the total mean (thus a lower SD) than the means of the differences at the middle values of the x -axis (thus a higher SD). Therefore, the first assumption is not met. Nonetheless, the second assumption is met, because our differences follow a normal distribution, as shown in Figure 4B . Our failure to meet the first assumption can be due to a number of reasons, for which Bland and Altman also proposed solutions [ 15 ]. For example, data may be skewed. However, in that case, log-transforming variables may be a solution [ 16 ].

Plots to check assumptions for the limits of agreement. ( A ) The Bland–Altman plot for the assumption that the mean and SD of the differences are constant over the range of observations. In our case, we see that the mean of the differences appears to be equal along the x -axis; i.e., these datapoints could plausibly fit the horizontal line of the total mean across the whole x -axis. Nonetheless, the SD does not appear to be distributed equally: the means of the differences at the lower values of the x -axis are closer to the total mean (thus a lower SD) than the means of the differences at the middle values of the x -axis (thus a higher SD). Therefore, the first assumption is not met. The limits of agreement and the mean are added as dashed (- - -) lines. ( B ) A histogram of the distribution of differences to ascertain the assumption of whether the differences are normally distributed. In our case, the observations follow a normal distribution and thus, the assumption is met.
It is often mistakenly thought that the Bland–Altman plot alone is the analysis to determine the agreement between methods, but the authors themselves spoke strongly against this [ 15 ]. We suggest that authors should both report the limits of agreement and show the Bland–Altman plot, to allow readers to assess for themselves whether they think the agreement is met.
The correlation coefficient is easy to calculate and provides a measure of the strength of linear association in the data. However, it also has important limitations and pitfalls, both when studying the association between two variables and when studying agreement between methods. These limitations and pitfalls should be taken into account when using and interpreting it. If necessary, researchers should look into alternatives to the correlation coefficient, such as regression analysis for causal research, and the ICC and the limits of agreement combined with a Bland–Altman plot when comparing methods.
CONFLICT OF INTEREST STATEMENT
None declared.
Contributor Information
Roemer J Janse, Department of Clinical Epidemiology, Leiden University Medical Center, Leiden, The Netherlands.
Tiny Hoekstra, Department of Nephrology, Amsterdam Cardiovascular Sciences, Amsterdam UMC, Vrije Universiteit Amsterdam, Amsterdam, The Netherlands.
Kitty J Jager, ERA-EDTA Registry, Department of Medical Informatics, Amsterdam Public Health Research Institute, Amsterdam UMC, University of Amsterdam, Amsterdam, The Netherlands.
Carmine Zoccali, CNR-IFC, Center of Clinical Physiology, Clinical Epidemiology of Renal Diseases and Hypertension, Reggio Calabria, Italy.
Giovanni Tripepi, CNR-IFC, Center of Clinical Physiology, Clinical Epidemiology of Renal Diseases and Hypertension, Reggio Calabria, Italy.
Friedo W Dekker, Department of Clinical Epidemiology, Leiden University Medical Center, Leiden, The Netherlands.
Merel van Diepen, Department of Clinical Epidemiology, Leiden University Medical Center, Leiden, The Netherlands.
- Privacy Policy

Home » Correlation Analysis – Types, Methods and Examples
Correlation Analysis – Types, Methods and Examples
Table of Contents

Correlation Analysis
Correlation analysis is a statistical method used to evaluate the strength and direction of the relationship between two or more variables . The correlation coefficient ranges from -1 to 1.
- A correlation coefficient of 1 indicates a perfect positive correlation. This means that as one variable increases, the other variable also increases.
- A correlation coefficient of -1 indicates a perfect negative correlation. This means that as one variable increases, the other variable decreases.
- A correlation coefficient of 0 means that there’s no linear relationship between the two variables.
Correlation Analysis Methodology
Conducting a correlation analysis involves a series of steps, as described below:
- Define the Problem : Identify the variables that you think might be related. The variables must be measurable on an interval or ratio scale. For example, if you’re interested in studying the relationship between the amount of time spent studying and exam scores, these would be your two variables.
- Data Collection : Collect data on the variables of interest. The data could be collected through various means such as surveys , observations , or experiments. It’s crucial to ensure that the data collected is accurate and reliable.
- Data Inspection : Check the data for any errors or anomalies such as outliers or missing values. Outliers can greatly affect the correlation coefficient, so it’s crucial to handle them appropriately.
- Choose the Appropriate Correlation Method : Select the correlation method that’s most appropriate for your data. If your data meets the assumptions for Pearson’s correlation (interval or ratio level, linear relationship, variables are normally distributed), use that. If your data is ordinal or doesn’t meet the assumptions for Pearson’s correlation, consider using Spearman’s rank correlation or Kendall’s Tau.
- Compute the Correlation Coefficient : Once you’ve selected the appropriate method, compute the correlation coefficient. This can be done using statistical software such as R, Python, or SPSS, or manually using the formulas.
- Interpret the Results : Interpret the correlation coefficient you obtained. If the correlation is close to 1 or -1, the variables are strongly correlated. If the correlation is close to 0, the variables have little to no linear relationship. Also consider the sign of the correlation coefficient: a positive sign indicates a positive relationship (as one variable increases, so does the other), while a negative sign indicates a negative relationship (as one variable increases, the other decreases).
- Check the Significance : It’s also important to test the statistical significance of the correlation. This typically involves performing a t-test. A small p-value (commonly less than 0.05) suggests that the observed correlation is statistically significant and not due to random chance.
- Report the Results : The final step is to report your findings. This should include the correlation coefficient, the significance level, and a discussion of what these findings mean in the context of your research question.
Types of Correlation Analysis
Types of Correlation Analysis are as follows:
Pearson Correlation
This is the most common type of correlation analysis. Pearson correlation measures the linear relationship between two continuous variables. It assumes that the variables are normally distributed and have equal variances. The correlation coefficient (r) ranges from -1 to +1, with -1 indicating a perfect negative linear relationship, +1 indicating a perfect positive linear relationship, and 0 indicating no linear relationship.
Spearman Rank Correlation
Spearman’s rank correlation is a non-parametric measure that assesses how well the relationship between two variables can be described using a monotonic function. In other words, it evaluates the degree to which, as one variable increases, the other variable tends to increase, without requiring that increase to be consistent.
Kendall’s Tau
Kendall’s Tau is another non-parametric correlation measure used to detect the strength of dependence between two variables. Kendall’s Tau is often used for variables measured on an ordinal scale (i.e., where values can be ranked).
Point-Biserial Correlation
This is used when you have one dichotomous and one continuous variable, and you want to test for correlations. It’s a special case of the Pearson correlation.
Phi Coefficient
This is used when both variables are dichotomous or binary (having two categories). It’s a measure of association for two binary variables.
Canonical Correlation
This measures the correlation between two multi-dimensional variables. Each variable is a combination of data sets, and the method finds the linear combination that maximizes the correlation between them.
Partial and Semi-Partial (Part) Correlations
These are used when the researcher wants to understand the relationship between two variables while controlling for the effect of one or more additional variables.
Cross-Correlation
Used mostly in time series data to measure the similarity of two series as a function of the displacement of one relative to the other.
Autocorrelation
This is the correlation of a signal with a delayed copy of itself as a function of delay. This is often used in time series analysis to help understand the trend in the data over time.
Correlation Analysis Formulas
There are several formulas for correlation analysis, each corresponding to a different type of correlation. Here are some of the most commonly used ones:
Pearson’s Correlation Coefficient (r)
Pearson’s correlation coefficient measures the linear relationship between two variables. The formula is:
r = Σ[(xi – Xmean)(yi – Ymean)] / sqrt[(Σ(xi – Xmean)²)(Σ(yi – Ymean)²)]
- xi and yi are the values of X and Y variables.
- Xmean and Ymean are the mean values of X and Y.
- Σ denotes the sum of the values.
Spearman’s Rank Correlation Coefficient (rs)
Spearman’s correlation coefficient measures the monotonic relationship between two variables. The formula is:
rs = 1 – (6Σd² / n(n² – 1))
- d is the difference between the ranks of corresponding variables.
- n is the number of observations.
Kendall’s Tau (τ)
Kendall’s Tau is a measure of rank correlation. The formula is:
τ = (nc – nd) / 0.5n(n-1)
- nc is the number of concordant pairs.
- nd is the number of discordant pairs.
This correlation is a special case of Pearson’s correlation, and so, it uses the same formula as Pearson’s correlation.
Phi coefficient is a measure of association for two binary variables. It’s equivalent to Pearson’s correlation in this specific case.
Partial Correlation
The formula for partial correlation is more complex and depends on the Pearson’s correlation coefficients between the variables.
For partial correlation between X and Y given Z:
rp(xy.z) = (rxy – rxz * ryz) / sqrt[(1 – rxz^2)(1 – ryz^2)]
- rxy, rxz, ryz are the Pearson’s correlation coefficients.
Correlation Analysis Examples
Here are a few examples of how correlation analysis could be applied in different contexts:
- Education : A researcher might want to determine if there’s a relationship between the amount of time students spend studying each week and their exam scores. The two variables would be “study time” and “exam scores”. If a positive correlation is found, it means that students who study more tend to score higher on exams.
- Healthcare : A healthcare researcher might be interested in understanding the relationship between age and cholesterol levels. If a positive correlation is found, it could mean that as people age, their cholesterol levels tend to increase.
- Economics : An economist may want to investigate if there’s a correlation between the unemployment rate and the rate of crime in a given city. If a positive correlation is found, it could suggest that as the unemployment rate increases, the crime rate also tends to increase.
- Marketing : A marketing analyst might want to analyze the correlation between advertising expenditure and sales revenue. A positive correlation would suggest that higher advertising spending is associated with higher sales revenue.
- Environmental Science : A scientist might be interested in whether there’s a relationship between the amount of CO2 emissions and average temperature increase. A positive correlation would indicate that higher CO2 emissions are associated with higher average temperatures.
Importance of Correlation Analysis
Correlation analysis plays a crucial role in many fields of study for several reasons:
- Understanding Relationships : Correlation analysis provides a statistical measure of the relationship between two or more variables. It helps in understanding how one variable may change in relation to another.
- Predicting Trends : When variables are correlated, changes in one can predict changes in another. This is particularly useful in fields like finance, weather forecasting, and technology, where forecasting trends is vital.
- Data Reduction : If two variables are highly correlated, they are conveying similar information, and you may decide to use only one of them in your analysis, reducing the dimensionality of your data.
- Testing Hypotheses : Correlation analysis can be used to test hypotheses about relationships between variables. For example, a researcher might want to test whether there’s a significant positive correlation between physical exercise and mental health.
- Determining Factors : It can help identify factors that are associated with certain behaviors or outcomes. For example, public health researchers might analyze correlations to identify risk factors for diseases.
- Model Building : Correlation is a fundamental concept in building multivariate statistical models, including regression models and structural equation models. These models often require an understanding of the inter-relationships (correlations) among multiple variables.
- Validity and Reliability Analysis : In psychometrics, correlation analysis is used to assess the validity and reliability of measurement instruments such as tests or surveys.
Applications of Correlation Analysis
Correlation analysis is used in many fields to understand and quantify the relationship between variables. Here are some of its key applications:
- Finance : In finance, correlation analysis is used to understand the relationship between different investment types or the risk and return of a portfolio. For example, if two stocks are positively correlated, they tend to move together; if they’re negatively correlated, they move in opposite directions.
- Economics : Economists use correlation analysis to understand the relationship between various economic indicators, such as GDP and unemployment rate, inflation rate and interest rates, or income and consumption patterns.
- Marketing : Correlation analysis can help marketers understand the relationship between advertising spend and sales, or the relationship between price changes and demand.
- Psychology : In psychology, correlation analysis can be used to understand the relationship between different psychological variables, such as the correlation between stress levels and sleep quality, or between self-esteem and academic performance.
- Medicine : In healthcare, correlation analysis can be used to understand the relationships between various health outcomes and potential predictors. For example, researchers might investigate the correlation between physical activity levels and heart disease, or between smoking and lung cancer.
- Environmental Science : Correlation analysis can be used to investigate the relationships between different environmental factors, such as the correlation between CO2 levels and average global temperature, or between pesticide use and biodiversity.
- Social Sciences : In fields like sociology and political science, correlation analysis can be used to investigate relationships between different social and political phenomena, such as the correlation between education levels and political participation, or between income inequality and social unrest.
Advantages and Disadvantages of Correlation Analysis
About the author.
Muhammad Hassan
Researcher, Academic Writer, Web developer
You may also like

Cluster Analysis – Types, Methods and Examples

Discriminant Analysis – Methods, Types and...

MANOVA (Multivariate Analysis of Variance) –...

Documentary Analysis – Methods, Applications and...

ANOVA (Analysis of variance) – Formulas, Types...

Graphical Methods – Types, Examples and Guide
- Open access
- Published: 27 May 2024
Association between gut microbiota and anxiety disorders: a bidirectional two-sample mendelian randomization study
- Jianbing Li 1 ,
- Changhe Fan 1 ,
- Jiaqi Wang 2 ,
- Bulang Tang 2 ,
- Jiafan Cao 2 ,
- Xianzhe Hu 2 ,
- Xuan Zhao 2 &
- Caiqin Feng 1
BMC Psychiatry volume 24 , Article number: 398 ( 2024 ) Cite this article
196 Accesses
4 Altmetric
Metrics details
There are many articles reporting that the component of intestinal microbiota implies a link to anxiety disorders (AD), and the brain-gut axis is also a hot topic in current research. However, the specific relevance between gut microbiota and AD is uncertain. We aimed to investigate causal relationship between gut microbiota and AD by using bidirectional Mendelian randomization (MR).
Genetic instrumental variable (IV) for the gut microbiota were obtained from a genome-wide association study (GWAS) involving 18,340 participants. Summary data for AD were derived from the GWAS and included 158,565 cases and 300,995 controls. We applied the inverse variance weighted (IVW) method as the main analysis. Cochran’s Q values was computed to evaluate the heterogeneity among IVs. Sensitivity analyses including intercept of MR-Egger method and MR-PRESSO analysis were used to test the horizontal pleiotropy.
We discovered 9 potential connections between bacterial traits on genus level and AD. Utilizing the IVW method, we identified 5 bacterial genera that exhibited a direct correlation with the risk of AD: genus Eubacteriumbrachygroup , genus Coprococcus3 , genus Enterorhabdus , genus Oxalobacter , genus Ruminiclostridium6 . Additionally, we found 4 bacterial genera that exhibited a negative association with AD: genus Blautia , genus Butyricicoccus , genus Erysipelotrichaceae-UCG003 and genus Parasutterella . The associations were confirmed by the sensitivity analyses.
Our study found a causal relation between parts of the gut microbiota and AD. Further randomized controlled trials are crucial to elucidate the positive effects of probiotics on AD and their particular protection systems.
Peer Review reports
Introduction
Anxiety disorders (AD), being the prevailing mental disorders, have a substantial impact on individuals and society alike [ 1 ]. The core features of AD contain indiscriminate anxiety and fear or elusion of persistent and debilitating threats, resulting in substantial medical costs and a burdensome morbidity burden [ 1 , 2 ]. As one of the most popular mental illnesses among young individuals, AD are also the earliest-onset mental disorders [ 3 ]. Amidst the COVID-19 pandemic, there has been a significant surge in the occurrence of AD among children, adolescents, and young adults globally [ 4 ]. First-line treatments for AD include medication and psychotherapy [ 5 ]. However, medication treatments carry certain side effects and risks, such as dependence, cognitive impairment, and an increased risk of heart disease [ 6 ]. The majority of individuals suffering from AD lack access to efficacious treatment options, leaving them vulnerable to relapse [ 7 , 8 ].
Many studies have shown that the occurrence of AD is related to changes in intestinal flora [ 9 , 10 ]. In social anxiety disorder (SAD), there was an increase in the relative abundance of Anaeromassillibacillus and Gordonibacter genera, whereas healthy controls exhibited an enrichment of Parasuterella [ 11 ]. Another article found a reduction in Eubacterium rectale and Fecalibacterium , as well as an increase in Escherichia , Shigella , Fusobacterium , and Ruminococcus in patients with generalized anxiety disorder (GAD) [ 12 ]. In addition, there are numerous documents demonstrating an association between the gut microbiota and mental illness, and the modulation of the gut microbiota on the gut-brain axis has garnered significant attention, such as an elevation of Enterobacteriaceae and Desulfovibrio , and a reduction of Faecalibacterium in patients with AD [ 10 , 13 , 14 , 15 , 16 , 17 ]. In the aforementioned section, it was observed that the evidence exhibits complexities and disparities, as well as some contradictory results, potentially stemming from various confounding factors among different studies.
The previous studies examining the connection between gut microbiota and AD have predominantly relied on cross-sectional designs, which limits the ability to establish a causal relationship between these associations. Therefore, unraveling the causal mechanisms behind gut microbiota-derived AD not only enhances our understanding of their pathogenesis but also provides valuable guidance for implementing microbiota-directed interventions in clinical settings to address AD. Previous Mendelian randomization (MR) studies have primarily focused on investigating the causal relationship between oral microbiota abundance and AD, or between gut microbiota and other psychiatric disorders. A systematic MR study specifically examining the causal relationship between gut microbiota and AD is still lacking in the current literature. In light of this, it is imperative to unravel the causal link between the gut microbiota and AD.
MR is a statistical approach that infers a causal relationship with exposure to a result. It leverages genetic variations linked to the exposure as a proxy for the exposure itself, enabling the assessment of the association between the exposure and the outcome [ 18 ]. Due to the highly effective findings of large-scale genome-wide association study (GWAS) at the gut microbiota and disease level, MR analysis has been abroad used in many scenarios, such as between the oral microbiome and AD, relations between genetically determined metabolites and anxiety symptoms [ 19 , 20 ]. However, there are no specific studies on the causal relationship between gut microbiota and AD. In this research, we applied a bidirectional two-sample MR method to investigate causal relationship between the gut microbiota and AD.
Materials and methods
The assumptions and study design of mr.
MR is a methodology employed to assess causal associations between variables. In order to ensure the validity of MR analysis, 3 fundamental assumptions must be met: (i) the instrumental variable (IV) exhibits a strong link to the exposure factor, (ii) the IV remains unaffected by potential confounding factors., and (iii) the IV influences the result factor solely via the exposure factor [ 21 ]. By applying strict selection criteria, appropriate SNPs were selected as IV for conducting MR analysis on two independent samples. The main aim was to examine the causal relationship between gut microbiota and AD. Furthermore, this study adhered to the guidelines outlined in the Strengthening the Reporting of Observational Studies in Epidemiology-Mendelian Randomization (STROBE-MR) framework [ 22 ] (Fig. 1 ).
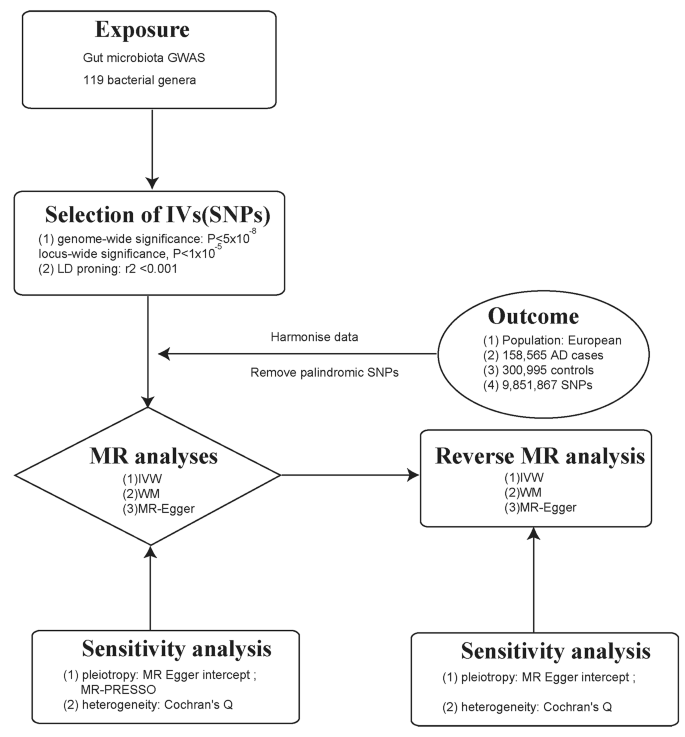
A flowchart illustrating the MR analysis process for the association between gut microbiota and AD
Data sources
The data on gut microbiota GWAS used in this study were obtained from an overall meta-analysis conducted by the MiBioGen consortium. The meta-analysis comprised a total of 18,340 individuals from 24 different groups. The alliance combines human whole-genome genotyping with fecal 16 S rRNA sequencing data to perform thorough research and analysis. The large-scale, multi-ethnic genome-wide meta-analysis provided valuable insights into the genetic influences on the gut microbiome composition [ 23 ]. The GWAS data on the gut microbiome can be integrated into MR studies to explore the causal relationship between genetic variations in the gut microbiome and phenotypic traits, providing valuable insights into the role of the microbiome in human health and disease.
As for the data on genetic variants linked to AD, they were sourced from the Medical Research Council Integrative Epidemiology Unit (MRC-IEU) consortium. The cases were defined as individuals who had sought medical attention for symptoms of nervousness, anxiety, or depression. The study population consisted of individuals of European descent, comprising both males and females, and the data were sourced from the year 2018. The dataset included a total of 158,565 cases and 300,995 controls. The diagnosis was based on self-report questionnaires. Detailed information regarding the data origins for this MR study can be found in Table 1 [ 24 , 25 ].
Selection of IV
The GWAS data of exposure contained a total of 5 taxonomic levels for 211 bacterial groups. The genus level is the smallest and most specific classification level. To accurately identify each pathogenic bacterial group, we focused our analysis only on the genus level, specifically examining 131 bacterial classifications. After excluding 12 unknown groups, a total of 119 bacterial genera were included in the study.
To fulfill the demands of MR studies, our initial step involved the SNPs that exhibited an intense association with the exposure factors. However, when employing a stringent threshold of ( P < 5 × 10 − 8 ), we obtained a limited number of IVs. Consequently, we adjusted the threshold to ( P < 1 × 10 − 5 ) to ensure the inclusion of more IVs, thereby enabling robust and reliable results. For the selection of IVs associated with AD in the reverse MR analysis, a heightened level of stringency was implemented by applying a P -value threshold of P < 5 × 10 − 8 .
We utilized the F-statistic to further evaluate the instrument strength. The F-statistic was determined using the formula: F = β 2 / SE 2 . This statistic provided an assessment of the overall instrument strength [ 26 ] (Fig. 2 ). An F-statistic exceeding 10 was considered indicative of an intense conjunction between the IV and the exposure. Besides P -value threshold, the F statistic in our analysis would provide additional information on the instrument strength beyond P -value.
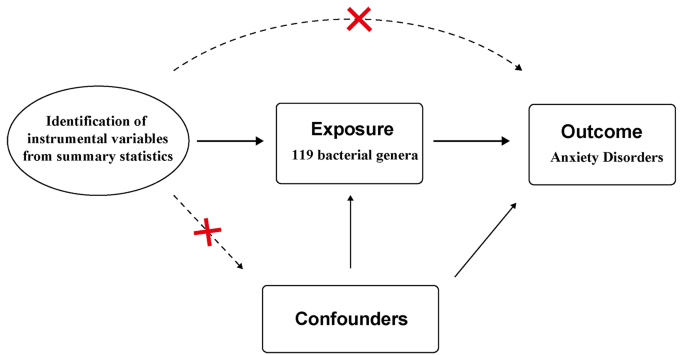
Assumptions in MR studies: a brief overview
Statistical analysis
The primary methodology employed in MR analysis is the inverse variance weighting (IVW) method. This approach utilizes a meta-analysis technique to combine the Wald estimates connected to individual single nucleotide polymorphisms (SNPs), providing comprehensive estimate of the collective impact of gut microbiota on AD. A crucial assumption in MR is the absence of horizontal pleiotropy, where the IV has a direct impact on the outcome variable solely through the exposure factor, without any influence from through alternative pathways. When this assumption is satisfied, the IVW method can provide estimates that are consistent and estimates [ 27 ]. In cases where a causal relationship ( P < 0.05) is established by the IVW method, two alternative approaches, namely MR-Egger and the weighted median approach, are utilized to supplement an enrich the IVW results. The MR-Egger method relaxes the assumption of a zero intercept, and it can estimate causal effects, even pleiotropy was presented in IVs. The intercept in the MR-Egger method can indicate the extent of horizontal pleiotropy [ 27 ]. These additional methods provide valuable insights and strengthen the overall analysis by considering potential biases and alternative causal pathways.
The weighted median method can return unbiased causal estimate when only 50% of SNPs are valid [ 28 ]. In this study, we employed a significance threshold of P < 0.05 to determine statistical significance, and the assessment of causality was expressed through odds ratios (OR) and 95% confidence intervals (CI). In instances where causal relationships were established, unidentified taxa were excluded, and additional sensitivity analyses were performed to guarantee the stability of the consequences. The false discovery rate (FDR) is utilized to control for multiple testing and reduce the likelihood of false positive findings. All of the aforementioned analyses were performed utilizing the TwoSampleMR package (version 0.5.7) in R (version 4.3.0), providing a robust and standardized approach to MR analysis.
According to the criteria for IV selection, a total of 1,531 SNPs were identified and selected as IV associated with gut microbiota. The F-statistics for these IVs all exceed 10, suggesting that the estimated coefficients are improbable to be influenced by the bias caused by weak instruments. Supplementary Tables 1 and 2 provides detailed information about the selected IVs. None of the SNPs were involved in more than one of the association results in Fig. 3 .
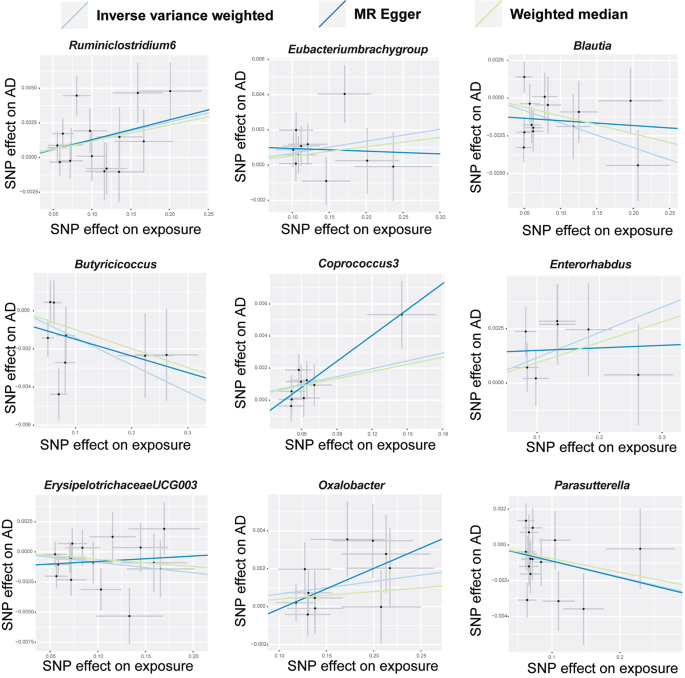
The scatter plots depict the causal relationship between gut microbiota and AD
The majority of gut microbiota showed no significant correlation with AD. However, using the IVW method, we identified 9 bacterial features that were significantly associated with the risk of AD on genus level (Supplementary Table 3 ). We used 3 methods, IVW, weighted median and MR-Egger, and defined P < 0.05 for IVW method screening as a positive result.
Among them, 4 bacterial genera are negatively correlated with AD, indicating that a higher genetically predicted a lower risk of for AD (Fig. 4 and Supplementary Table 4 ). They are: genus Blautia (OR = 0.9838, 95% CI, 0.9725–0.9952, P = 0.0056), genus Butyricicoccus (OR = 0.9859, 95% CI, 0.9739–0.9981, P = 0.0233), genus ErysipelotrichaceaeUCG003 (OR = 0.9914, 95% CI, 0.9833–0.9995, P = 0.0381) and genus Parasutterella (OR = 0.9911, 95% CI, 0.9823–0.9999, P = 0.0478). Supplementary Table 4 shows the completed data. In sensitivity analysis, MR-Egger, weighted median demonstrated consistent results, except for genus ErysipelotrichaceaeUCG003 , where the MR-Egger trend was in the contrary direction compared to IVW and weighted median.
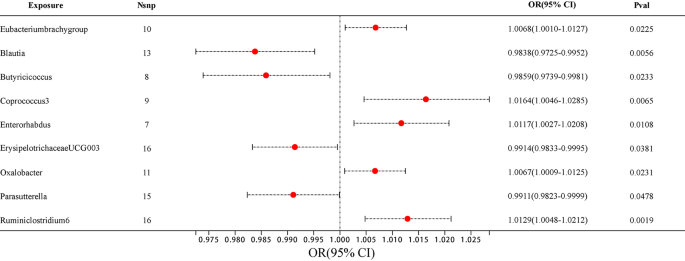
The forest plot illustrates the connections between 9 bacterial genus traits and the likelihood of developing AD
Another 5 bacterial genera showed a positive correlation with AD, genus Eubacteriumbrachygroup (OR = 1.0068, 95% CI, 1.0010–1.0127, P = 0.0225), genus Coprococcus3 (OR = 1.0164, 95% CI, 1.0046–1.0285, P = 0.0065), genus Enterorhabdus (OR = 1.0117, 95% CI, 1.0027–1.0208, P = 0.0108), genus Oxalobacter (OR = 1.0067, 95% CI, 1.0009–1.0125, P = 0.0231) and genus Ruminiclostridium6 (OR = 1.0129, 95% CI, 1.0048–1.0212, P = 0.0019) (Fig. 4 and Supplementary Table 4 ). In the MR-Egger method, the trends of genus Eubacteriumbrachygroup are different from those of the IVW and WM methods.
In horizontal pleiotropy analysis, we used the MR-Egger method and found P -value of the MR-intercept were all greater than 0.05. In addition, further MR PRESSO analysis was conducted, ruling out the existence of horizontal pleiotropy ( P > 0.05) (Supplementary Tables 5 and 6 ). To assess the heterogeneity of gut microbiome IVs, we employed Cochran’s Q test statistics, which revealed no heterogeneity among the gut microbiome IVs ( P > 0.05) (Supplementary Table 7 ).
Reverse MR analyses were conducted to examine the links between the 9 bacterial genera and AD. No significant statistical relationship was observed using the IVW method: genus Eubacteriumbrachygroup (OR = 1.4058, 95% CI, 0.4060–4.8674, P = 0.5909), genus Blautia (OR = 0.9453, 95% CI, 0.5572–1.6038, P = 0.8348), genus Butyricicoccus (OR = 0.9834, 95% CI, 0.5704–1.6952, P = 0.9518), genus Coprococcus3 (OR = 0.8886, 95% CI, 0.5040–1.5667, P = 0.6831), genus Enterorhabdus (OR = 1.0383, 95% CI, 0.4168–2.5868, P = 0.9356), genus ErysipelotrichaceaeUCG003 (OR = 0.6593, 95% CI, 0.3556–1.2221, P = 0.1858), genus Oxalobacter (OR = 1.2849, 95% CI, 0.4021–4.1051, P = 0.6724), genus Parasutterella (OR = 0.7245, 95% CI, 0.3713–1.4136, P = 0.3447), genus Ruminiclostridium6 (OR = 0.7095, 95% CI, 0.3825–1.3162, P = 0.2764) (Supplementary Tables 8 and 9 ).
In the context of this study, we used two-sample MR studies to discover the link between AD and gut microbiota. Among the 9 bacterial genus we found, 4 bacteria were negatively correlated with AD and may have a positive effect on AD, and the other 5 bacteria were positively correlated with the occurrence of AD and may promote the development of AD.
Blautia stercoris MRx0006 has been shown to alleviate social dysfunctions, monotonous behaviors, and anxiety-like behaviors relevant to autism disorders in a mouse model. MRx0006 administration at the microbial level, as observed by Paromita Sen et al., resulted in a reduction in the abundance of Alistipes putredinis, which likely underlie the observed increase in expressions of oxytocin, arginine vasopressin, and their receptors, ultimately leading to improved behavioral outcomes [ 29 ]. Butyricicoccus was also inversely associated with AD in a cross-sectional study, which is consistent with our findings [ 12 ]. Approximately 70% of individuals with autism spectrum disorder (ASD) exhibit comorbid symptoms of anxiety, and the findings from a published article confirming the decreased relative abundance of ErysipelotrichaceaeUCG003 in ASD patients further support our research results indicating a negative correlation between ErysipelotrichaceaeUCG003 and AD [ 30 ]. In a study examining SAD, the control group exhibited higher levels of the positive bacteria Parasutterella compared to the anxiety group. The term “psychobiotics” has been coined to refer to these microbes that are associated with improved mood [ 11 ]. However, in a study by Yi Zhang et al., a psychological stress model was established in C57BL/6J mice, followed by fecal microbiota transplantation using samples from stressed (S) and non-stressed (NS) mice. The results showed an increased abundance of Parasutterella in S mice and mechanistic analysis suggested its potential involvement in negative regulation of metabolism. Despite this controversial finding, our study utilized MR to reveal a negative association between Parasutterella and anxiety disorders. However, further experimental investigations are required to elucidate the underlying molecular mechanisms [ 31 ].
Five bacterial genera positively linked to anxiety may indicate that they exacerbate anxiety, but they were less reported. In a study in which consuming prebiotics altered the microbiota of healthy adults, the prebiotics reduced Eubacteriumbrachygroup but did not significantly change biomarkers of stress or mental health symptoms [ 32 ]. In previous studies on AD cases, it has been found that individuals with AD have lower levels of Coprococcus [ 33 ]. However, in our study, we observed an increasing trend in Coprococcus3 , despite belonging to the same genus. This suggests that even within the same genus, the impact of different genus may vary. In contrast to our findings, Enterorhabdus exhibited a declining pattern in a mouse model of anxiety and depression induced by social defeat [ 34 ]. This observation highlights the influence of various factors on alterations in gut microbiota, which may diverge across different species.
Nevertheless, it is crucial to acknowledge that our study has certain limitations. First, the results of this analysis are limited to European populations and may not be generalizable to other populations. Secondly, we observed that the adjusted P -values remained relatively large after multiple test adjustment. The reduced statistical power resulting from the limited sample size may also constrain our ability to detect significant associations between variables. Finally, proving the direct impact of sample types on the outcomes is challenging. However, the selection of sample types is often constrained by the availability of suitable genetic instruments and relevant data sources. The dataset we utilized does not provide specific information on the dietary habits of the individuals or their other medical conditions. Therefore, further examination and validation are needed in the future.
In summary, utilizing large-scale GWAS analysis, MR studies have disclosed a causal relationship between gut microbiota and AD. Among these, 4 bacterial genera exhibited a negative correlation, while 5 bacteria genera showed a positive correlation with AD. However, further exploration of the mechanisms linking gut microbiota to AD requires the establishment of larger GWAS databases. Several gut bacteria have been identified to reduce the occurrence of anxiety, offering promising prospects for the treatment and precaution of AD. Subsequent research should prioritize the exploration of the underlying mechanisms and the development of targeted interventions based on these findings.
Data availability
The raw data analyzed during the current study were available in public databases including IEU database(ukb-b-6991) and MiBioGen database(https://mibiogen.gcc.rug.nl). The code and data related to this study are available from the corresponding author upon reasonable request.
Abbreviations
- Anxiety disorders
- Mendelian randomization
Instrumental variable(s)
Genome-wide association study
Medical Research Council Integrative Epidemiology Unit
Inverse variance weighting
Social anxiety disorder
Generalized anxiety disorder
Strengthening the Reporting of Observational Studies in Epidemiology-Mendelian Randomization
Single nucleotide polymorphism(s)
Odds ratios
Confidence intervals
Autism spectrum disorder
Major depressive disorder
Penninx BW, Pine DS, Holmes EA, et al. Anxiety disorders[J] Lancet. 2021;397:914–27.
PubMed PubMed Central Google Scholar
Bandelow B, Michaelis S. Epidemiology of anxiety disorders in the 21st century[J]. Dialogues Clin Neurosci. 2015;17:327–35.
Article PubMed PubMed Central Google Scholar
Warner EN, Ammerman RT, Glauser TA, et al. Developmental epidemiology of pediatric anxiety disorders[J]. Child Adolesc Psychiatr Clin N Am. 2023;32:511–30.
Article PubMed Google Scholar
Fortuna LR, Brown IC, Lewis Woods GG, et al. The impact of COVID-19 on anxiety disorders in Youth: coping with stress, worry, and recovering from a Pandemic[J]. Child Adolesc Psychiatr Clin N Am. 2023;32:531–42.
Wehry AM, Beesdo-Baum K, Hennelly MM, et al. Assessment and treatment of anxiety disorders in children and adolescents[J]. Curr Psychiatry Rep. 2015;17:52.
Szuhany KL, Simon NM. Anxiety disorders: a review[J]. JAMA. 2022;328:2431–45.
Article CAS PubMed Google Scholar
Uher R. The global impact of anxiety disorders[J]. Lancet Psychiatry. 2023;10:239–40.
Scholten W, Ten Have M, Van Geel C, et al. Recurrence of anxiety disorders and its predictors in the general population[J]. Psychol Med. 2023;53:1334–42.
Yang B, Wei J, Ju P, et al. Effects of regulating intestinal microbiota on anxiety symptoms: a systematic review[J]. Gen Psychiatr. 2019;32:e100056.
Simpson CA, Diaz-Arteche C, Eliby D, et al. The gut microbiota in anxiety and depression - a systematic review[J]. Clin Psychol Rev. 2021;83:101943.
Butler MI, Bastiaanssen TFS, Long-Smith C, et al. The gut microbiome in social anxiety disorder: evidence of altered composition and function[J]. Translational Psychiatry. 2023;13:95.
Jiang HY, Zhang X, Yu ZH, et al. Altered gut microbiota profile in patients with generalized anxiety disorder[J]. J Psychiatr Res. 2018;104:130–6.
Socała K, Doboszewska U, Szopa A, et al. The role of microbiota-gut-brain axis in neuropsychiatric and neurological disorders[J]. Pharmacol Res. 2021;172:105840.
Nikolova VL, Smith MRB, Hall LJ, et al. Perturbations in gut microbiota composition in psychiatric disorders: a review and meta-analysis[J]. JAMA Psychiatry. 2021;78:1343–54.
Generoso JS, Giridharan VV, Lee J, et al. The role of the microbiota-gut-brain axis in neuropsychiatric disorders[J]. Braz J Psychiatry. 2021;43:293–305.
Mörkl S, Butler MI, Holl A, et al. Probiotics and the microbiota-gut-brain axis: focus on psychiatry[J]. Curr Nutr Rep. 2020;9:171–82.
Needham BD, Funabashi M, Adame MD, et al. A gut-derived metabolite alters brain activity and anxiety behaviour in mice[J]. Nature. 2022;602:647–53.
Article CAS PubMed PubMed Central Google Scholar
Emdin CA, Khera AV, Kathiresan S. Mendelian Randomization[J] JAMA. 2017;318:1925–6.
Li C, Chen Y, Wen Y, et al. A genetic association study reveals the relationship between the oral microbiome and anxiety and depression symptoms[J]. Front Psychiatry. 2022;13:960756.
Xiao G, He Q, Liu L, et al. Causality of genetically determined metabolites on anxiety disorders: a two-sample mendelian randomization study[J]. J Transl Med. 2022;20:475.
Xie L, Zhao H, Chen W. Relationship between gut microbiota and thyroid function: a two-sample mendelian randomization study[J]. Front Endocrinol (Lausanne). 2023;14:1240752.
Skrivankova VW, Richmond RC, Woolf BR, et al. Strengthening the reporting of Observational studies in Epidemiology using mendelian randomization: the STROBE-MR Statement[J]. JAMA. 2021;326:1614–21.
Kurilshikov A, Medina-Gomez C, Bacigalupe R, et al. Large-scale association analyses identify host factors influencing human gut microbiome composition[J]. Nat Genet. 2021;53:156–65.
Lyall DM, Inskip HM, Mackay D, et al. Low birth weight and features of neuroticism and mood disorder in 83 545 participants of the UK Biobank cohort[J]. BJPsych Open. 2016;2:38–44.
Smith DJ, Nicholl BI, Cullen B, et al. Prevalence and characteristics of probable major depression and bipolar disorder within UK biobank: cross-sectional study of 172,751 participants[J]. PLoS ONE. 2013;8:e75362.
Burgess S, Thompson SG. Avoiding bias from weak instruments in mendelian randomization studies[J]. Int J Epidemiol. 2011;40:755–64.
Burgess S, Butterworth A, Thompson SG. Mendelian randomization analysis with multiple genetic variants using summarized data[J]. Genet Epidemiol. 2013;37:658–65.
Bowden J, Davey Smith G, Haycock PC, et al. Consistent estimation in mendelian randomization with some Invalid instruments using a weighted median estimator[J]. Genet Epidemiol. 2016;40:304–14.
Sen P, Sherwin E, Sandhu K, et al. The live biotherapeutic Blautia stercoris MRx0006 attenuates social deficits, repetitive behaviour, and anxiety-like behaviour in a mouse model relevant to autism[J]. Brain Behav Immun. 2022;106:115–26.
Chen Y-C, Lin H-Y, Chien Y, et al. Altered gut microbiota correlates with behavioral problems but not gastrointestinal symptoms in individuals with autism[J]. Brain Behav Immun. 2022;106:161–78.
Zhang Y, Zhang J, Wu J, et al. Implications of gut microbiota dysbiosis and fecal metabolite changes in psychologically stressed mice[J]. Front Microbiol. 2023;14:1124454.
Mysonhimer AR, Cannavale CN, Bailey MA, et al. Prebiotic consumption alters Microbiota but not biological markers of stress and inflammation or mental health symptoms in healthy adults: a randomized, controlled, crossover trial[J]. J Nutr. 2023;153:1283–96.
Chen YH, Bai J, Wu D, et al. Association between fecal microbiota and generalized anxiety disorder: severity and early treatment response[J]. J Affect Disord. 2019;259:56–66.
Zou R, Tian P, Xu M, et al. Psychobiotics as a novel strategy for alleviating anxiety and depression[J]. J Funct Foods. 2021;86:104718.
Article Google Scholar
Download references
Acknowledgements
We express our gratitude to the hospital action teams, staff, and participants from the participating hospitals for their valuable support in data collection. Additionally, we extend our appreciation to our collaborators for their assistance throughout the process.
Program of Guangzhou Science and Technology Program Project (No. 202102010115) and Guangdong Yiyang Healthcare Charity Foundation (No. JZ2022001-3).
Author information
Authors and affiliations.
Department of Psychiatry, Guangdong Second Provincial General Hospital, Guangzhou, 510317, PR China
Jianbing Li, Changhe Fan & Caiqin Feng
School of Pharmacy, Guangdong Pharmaceutical University, Guangzhou, 510006, China
Jiaqi Wang, Bulang Tang, Jiafan Cao, Xianzhe Hu & Xuan Zhao
You can also search for this author in PubMed Google Scholar
Contributions
CQF designed the research framework. JBL is responsible for data and analysis methods determination as well as manuscript writing. CHF assisted in conducting the literature review. JQW was responsible for manuscript writing. BLT and JFC performed the data statistical analysis. XZH and XZ were responsible for critical revisions.
Corresponding author
Correspondence to Caiqin Feng .
Ethics declarations
Ethics approval and consent to participate.
Not applicable.
Consent for publication
Competing interests.
The authors declare no competing interests.
Additional information
Publisher’s note.
Springer Nature remains neutral with regard to jurisdictional claims in published maps and institutional affiliations.
Electronic supplementary material
Below is the link to the electronic supplementary material.
Supplementary Material 1
Supplementary material 2, supplementary material 3, supplementary material 4, supplementary material 5, supplementary material 6, supplementary material 7, supplementary material 8, supplementary material 9, rights and permissions.
Open Access This article is licensed under a Creative Commons Attribution 4.0 International License, which permits use, sharing, adaptation, distribution and reproduction in any medium or format, as long as you give appropriate credit to the original author(s) and the source, provide a link to the Creative Commons licence, and indicate if changes were made. The images or other third party material in this article are included in the article’s Creative Commons licence, unless indicated otherwise in a credit line to the material. If material is not included in the article’s Creative Commons licence and your intended use is not permitted by statutory regulation or exceeds the permitted use, you will need to obtain permission directly from the copyright holder. To view a copy of this licence, visit http://creativecommons.org/licenses/by/4.0/ . The Creative Commons Public Domain Dedication waiver ( http://creativecommons.org/publicdomain/zero/1.0/ ) applies to the data made available in this article, unless otherwise stated in a credit line to the data.
Reprints and permissions
About this article
Cite this article.
Li, J., Fan, C., Wang, J. et al. Association between gut microbiota and anxiety disorders: a bidirectional two-sample mendelian randomization study. BMC Psychiatry 24 , 398 (2024). https://doi.org/10.1186/s12888-024-05824-x
Download citation
Received : 23 December 2023
Accepted : 09 May 2024
Published : 27 May 2024
DOI : https://doi.org/10.1186/s12888-024-05824-x
Share this article
Anyone you share the following link with will be able to read this content:
Sorry, a shareable link is not currently available for this article.
Provided by the Springer Nature SharedIt content-sharing initiative
- Gut microbiota
- Single nucleotide polymorphism
BMC Psychiatry
ISSN: 1471-244X
- Submission enquiries: [email protected]
- General enquiries: [email protected]
Thank you for visiting nature.com. You are using a browser version with limited support for CSS. To obtain the best experience, we recommend you use a more up to date browser (or turn off compatibility mode in Internet Explorer). In the meantime, to ensure continued support, we are displaying the site without styles and JavaScript.
- View all journals
- My Account Login
- Explore content
- About the journal
- Publish with us
- Sign up for alerts
- Open access
- Published: 01 June 2024
Research of 2D-COS with metabolomics modifications through deep learning for traceability of wine
- Zhuo-Kang Wang 1 ,
- Hai-Cheng Wei 2 ,
- Jin-Hang Wang 1 ,
- Jing Zhao 3 &
Scientific Reports volume 14 , Article number: 12598 ( 2024 ) Cite this article
Metrics details
- Engineering
To tackle the difficulty of extracting features from one-dimensional spectral signals using traditional spectral analysis, a metabolomics analysis method is proposed to locate two-dimensional correlated spectral feature bands and combine it with deep learning classification for wine origin traceability. Metabolomics analysis was performed on 180 wine samples from 6 different wine regions using UPLC-Q-TOF-MS. Indole, Sulfacetamide, and caffeine were selected as the main differential components. By analyzing the molecular structure of these components and referring to the main functional groups on the infrared spectrum, characteristic band regions with wavelengths in the range of 1000–1400 nm and 1500–1800 nm were selected. Draw two-dimensional correlation spectra (2D-COS) separately, generate synchronous correlation spectra and asynchronous correlation spectra, establish convolutional neural network (CNN) classification models, and achieve the purpose of wine origin traceability. The experimental results demonstrate that combining two segments of two-dimensional characteristic spectra determined by metabolomics screening with convolutional neural networks yields optimal classification results. This validates the effectiveness of using metabolomics screening to determine spectral feature regions in tracing wine origin. This approach effectively removes irrelevant variables while retaining crucial chemical information, enhancing spectral resolution. This integrated approach strengthens the classification model's understanding of samples, significantly increasing accuracy.
Introduction
The geographical origin of wine has a significant impact on its sensory characteristics, chemical composition, and commercial value 1 . With the improvement of people's living standards and the surge in global wine sales, inferior wine with forged geographical labels has seriously threatened consumer health and industry development 2 , and wine origin traceability technology has gradually attracted public attention.
At present, the provenance traceability technology of wine mainly depends on stable nuclide technology, gas chromatography–mass spectrometry and high-performance liquid chromatography. For example, Sudol et al. used two-dimensional gas chromatography-time-of-flight mass spectrometry to analyze the volatile components of five white "Grillo" wines from Sicily, found the differential characteristic components that can characterize geographical labels, and carried out a traceability study on them 3 . Zhang et al. analyzed the Anthocyanidin derivatives of 234 red wines in different years by liquid chromatography-mass spectrometry, and classified the wines in different years and aging stages 4 . Wu et al. used Stable nuclide technology, element analysis and Chemometrics methods to screen six elements, such as Mg, Mn and Na, as important variables, to trace the origin of 240 wine samples from four different production regions in France within the regional and sub regional ranges 5 . Mattia Rapa et al. used ICP-MS technology to conduct multi-element screening analysis on soil and wine samples. They selected 10 elements, including Ni and Cs, from 45 elements as the main information variables for origin tracing. Combined with chemometric classification methods, they were able to accurately classify Piemonte and Sicily samples 6 .
However, in terms of large-scale origin testing of wine samples, the aforementioned methods encounter challenges such as labor-intensive sample preparation, expensive laboratory equipment, and specific experimental environment requirements. Spectral technology has been widely applied in wine origin identification, wine quality evaluation 7 , and various food research 8 , 9 due to its simplicity, high sensitivity, no need for sample pretreatment, and no need for experimental reagents. Lu et al. identified corresponding biomarkers by searching for Raman spectra of red wine, analyzed Raman spectra using PCA, and established a red wine origin recognition model by combining dimensionality reduction data with deep learning, achieving a more accurate classification of red wine origins 10 . Tana et al. used ultra-high performance liquid chromatography quadrupole time-of-flight mass spectrometry to identify characteristic substances in wine from different regions, screened out the characteristic bands of near-infrared spectroscopy of wine, and accurately divided wine samples from six regions 11 . Daniel et al. established a discriminative model for 64 white wine samples from Australia and New Zealand by spectral method, with an accuracy of 86% 12 .
Although the above method can quickly and conveniently achieve origin traceability by utilizing the spectral signal of wine, when using traditional spectral analysis methods to analyze spectral signals, there is a phenomenon of aliasing of signals from different components, making it difficult to extract useful information from the spectrum 13 , 14 . The proposal of two-dimensional correlation spectrum effectively makes up for the problem of poor resolution of traditional one-dimensional Spectral resolution 15 , 16 . In addition, because deep learning can improve the performance and accuracy of the model by automatically identifying the correlation of the original data without human intervention 17 , in other fields, deep learning is often used as an auxiliary method for the research of two-dimensional correlation spectral images. For example, Dong et al. combined the two-dimensional correlation spectrum of Lycium barbarum near-infrared hyperspectral image with convolutional neural network to establish the origin traceability model of Lycium barbarum origin, providing key technical support for the development of Lycium barbarum industry 18 . Liu et al. used two-dimensional correlation spectral images combined with residual convolutional neural network to effectively classify different origins and parts of Panax notoginseng, providing a feasible strategy for quality control of traditional chinese medicine 19 .
Using near-infrared two-dimensional correlation spectral images for wine origin traceability research can enhance spectral resolution. However, the large amount of irrelevant signals contained in the binary correlation spectra generated from the original spectral data can seriously affect detection accuracy. To address this issue, a method is proposed to screen spectral signal characteristic bands using metabolomics. Firstly, the main differential substances in wine samples from different regions are selected based on UPLC-Q-TOF-MS experimental results. Then, the characteristic bands of the wine's near-infrared spectrum are extracted. Finally, a generalized two-dimensional correlation spectral image analysis is performed on the two-dimensional correlation spectrum to establish a CNN origin classification model and achieve fast traceability detection of wine origin.
The Technology roadmap of this article is shown in Fig. 1 .
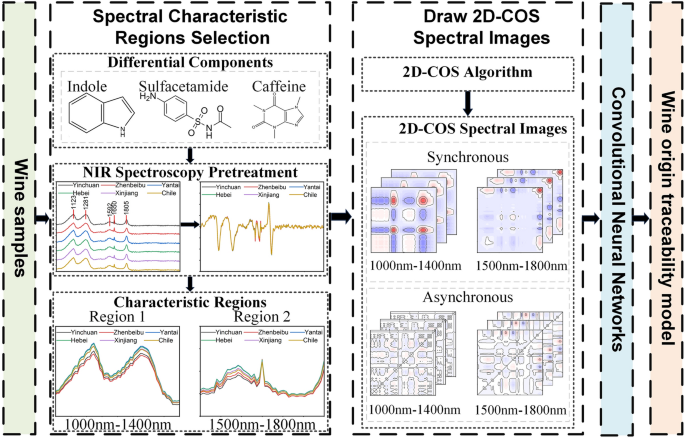
Technology roadmap.
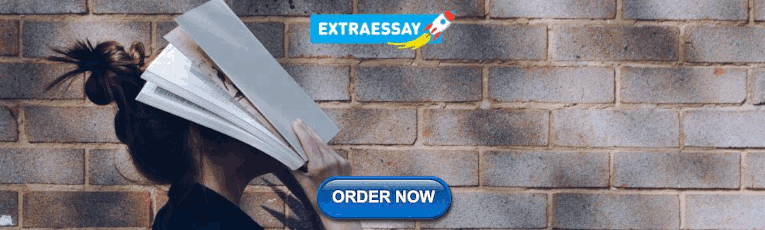
Materials and methods
Wine sample selection and near-infrared spectroscopy collection.
The samples used in the experiment were from six different regions, namely Yinchuan and Zhenbeibu in Ningxia, Changli in Hebei, Yantai in Shandong, Turpan in Xinjiang, and Limari Valley in Chile. 30 samples were collected from each production area, totaling 180 samples. In order to ensure the reliability of the experimental results, the wine samples used in the experiment were all finished wines made from Cabernet Sauvignon grapes through temperature controlled fermentation and aging in oak barrels.
The NIR test platform is composed of NIR2500 (Ideaoptics Instruments Co., Ltd., China), HL2000-12 halogen light source, RIB-600-NIR direct optical fiber,R4 color dish spectral measuring stand and Morpho software(Version 3.2 12.2, Available from http://www.ideaoptics.com ).
The experiment is carried out in a constant temperature and humidity environment. The wine samples are opened after 10 min’rest, placed in quartz cell, and the wine samples are collected using a near-infrared spectroscope. The wavelength range of the near-infrared spectrum collected by the wine samples ranged from 900 to 2500 nm, the wavelength resolution is 3.2 nm, the integration time is 1 ms–120 s, and the signal-to-noise ratio is 7500:1. The collection time for each spectral scan is 10 s. The total collection time for near-infrared spectroscopy of all samples is 50 min.
UPLC-Q-TOF-MS experimental method
The Q-TOF equipment used in the experiment is Agilent High Resolution Liquid Mass Spectrometry (HRLC-MS) system (Agilent Technologies, Santa Clara, CA, United States). The main components of wine are extracted and analyzed by MassHunter B.06.00 (Agilent Technologies, Inc. 2006–2019, Santa Clara, CA, United States) and Mass Profiler Professional 12.5 software (Agilent Technologies, Santa Clara, CA, United States) (Version 12.5, Available from www.agilent.com.cn ) to extract and analyze 130 main components of wine. Experimental reagents include distilled water, Ammonium formate (chromatographically pure) and methanol (chromatographically pure).
The experimental methods are as follows: Firstly, 1 mL sample is accurately measured in a 1.5 mL centrifugal tube, centrifuged at 4 °C for 10 min at 10,000 rpm, and passed through 0.22 μM Microporous filter, on-machine detection. The chromatographic column is Agilent Eclipse Plus C18 (3 × 150 mm, 1.8 μM). Column temperature: 40 °C; Automatic sampler temperature: 4 °C; Input: 2μL; Flow rate: 0.3 mL/min; Column balance time: 0.5 mL/min; Analysis time: 20 min. The mobile phase is 5 mmol/L ammonium formate aqueous phase and methanol phase.
In each data collection cycle, parent ions with an intensity greater than 5000 are screened. TOF-MS scan time is 150 ms, quality detection range is 50–1000 Da, collected in HighSensitivity mode.
Spectral data preprocessing
In addition to the feature data of the detected samples, the original near-infrared spectral data also contains many redundant variables and noise signals caused by external interference 20 . In order to eliminate interference and establish a highly reliable two-dimensional correlated infrared spectrum, MSC + S-G + FD was used to preprocess the original spectrum in the experiment to deduct the impact of instrument background or drift on the spectral signal, eliminate spectral differences caused by scattering effects caused by uneven particle sizes in the wine liquid during the spectral data collection process 21 , and reduce spectral signal noise and improve spectral signal to noise ratio.
Figure 2 a shows the original average near-infrared spectra of six wine samples from different regions in the range of 900–2500 nm. Except for five peaks at 1123 nm, 1281 nm, 1592 nm, 1650 nm, and 1805 nm with slight differences in absorbance values, the other bands are basically similar. Figure 2 b shows the average near-infrared spectra of 6 production areas after MSC + S-G + FD pretreatment.
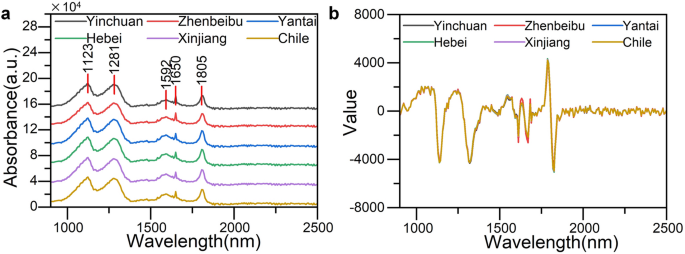
( a ) Original near-infrared spectra from six production areas, ( b) average near-infrared spectra after MSC + S-G + FD pretreatment.
2D-COS image acquisition
The acquisition of the generalized two-dimensional correlation infrared spectrum refers to the recording of the corresponding infrared spectrum (i.e. dynamic spectrum) of the sample under the disturbance state and the correlation analysis of a series of dynamic spectra when external disturbances (such as electrical, magnetic, thermal, mechanical, chemical, concentration and composition changes) are applied to the sample to be tested, and then the results are presented in a two-dimensional Contour line map or three-dimensional figure,the process of obtaining two-dimensional correlated infrared spectral images 17 .
Strictly speaking, dynamic spectrum refers to the result of subtracting a reference spectrum from the spectrum of a sample in different states due to interference. The dynamic spectrum with disturbances (electrical, magnetic, thermal, mechanical, chemical, concentration, and composition changes, etc.) can be recorded as Eq. ( 1 ):
\(x(v,t)\) represents the spectral intensity of the sample at variable \(v\) under disturbance \(t\) , while \(\overline{x}(v)\) represents the reference value of spectral intensity at variable \(v\) . In general, the average spectral intensity value at variable \(v\) in the sample spectrum obtained from the entire disturbance process (from \(t\) = \(T_{\min }\) to \(t\) = \(T_{\max }\) ) can be taken as \(\overline{x}(v)\) . Equation ( 2 ):
or a specific value can be selected as \(\overline{x}(v)\) , such as the spectral intensity value at variable \(v\) in the sample spectrum when \(t\) = \(T_{\min }\) or \(t\) = \(T_{\max }\) . At this point, the reference point is the initial or end state of the experiment. When the reference point is simply set to 0, the dynamic spectrum is the spectral intensity observed at the current moment.
Before calculating the generalized two-dimensional correlation spectrum, it is necessary to perform a Fourier transform on the dynamic spectrum \(y(v,t)\) , and the calculation of the generalized two-dimensional correlation spectrum obtained from cross correlation analysis is shown in Eq. ( 3 ):
The real part \(\Phi (v_{1} ,v_{2} )\) in Eq. ( 4 ) is called synchronous correlation spectrum, and the imaginary part \(\Psi (v_{1} ,v_{2} )\) is called asynchronous correlation spectrum.
The synchronous correlation spectrum represents the similarity change in spectral intensity between two variables as a function of disturbance. Asynchronous correlation spectra represent changes in spectral intensity with disturbance, differences in spectral intensity between two variables, or phase differences in spectral intensity between two variables.
In practical experiments, it is necessary to transform the integration formula under finite and discrete experimental values. Assuming that m data points are measured at equal intervals under disturbance \(t\) , the synchronous correlation spectrum at this time can be expressed as Eq. ( 5 ):
The calculation of asynchronous correlation spectra can be expressed as Eq. ( 6 ):
The \(M\) in Eq. ( 6 ) represents an n-th order Hilbert Noda matrix,and its expression is:
In the process of obtaining synchronous and asynchronous correlation spectral images of samples in the experiment, the average spectrum of the samples is first obtained, and then the characteristic band regions of the spectra are selected based on the Q-TOF results. The spectral data of each sample's characteristic region is compared with the average spectrum, and synchronous and asynchronous correlation spectral images of all samples are generated using time as the disturbance variable.
Establishing a deep learning model
The deep learning model used in the experiment is Convolutional Neural Networks (CNN). CNN, as a feedforward neural network with convolutional structure, extracts the features of input data through convolutional operations. As shown in Fig. 3 , the basic structure consists of an input layer, convolutional layer, pooling layer, fully connected layer, and output layer, with the structural characteristics of local area connection, weight sharing, and downsampling. Weight sharing and local area connectivity reduce the complexity of the network model and reduce the number of weights. The convolution calculation formula is:
\(m\) is the number of convolution layers and \(f()\) is the Activation function. Through calculation, the \(n\) feature map of layer \(m\) can be obtained, \(K\) represents the convolution kernel, and \(\hat{I}\) represents the input image set, representing the offset matrix corresponding to the \(n\) feature map of layer \(m\) .
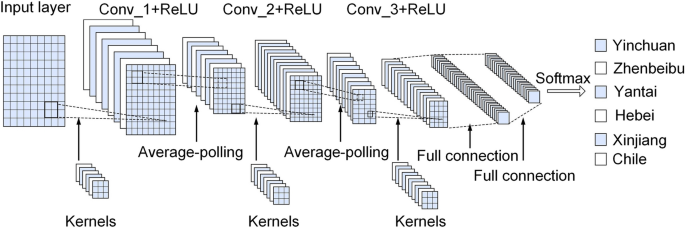
Structure diagram of Convolutional neural network model.
The structure of the CNN model is shown in Fig. 4 , the pooling layer in the structure can effectively reduce the size of the parameter matrix, improve the calculation speed and the robustness of the feature data. The activation function operates the output of the convolution layer nonlinearly to extract feature information. In this experiment, the linear rectification function (RELU) is selected as the activation function. Compared with other activation function, the sparsity of the linear rectification function can accelerate learning and simplify the model. After applying the linear rectification layer, the pooling layer performs parameter reduction to combine specific features of the convolutional layer, thereby avoiding overfitting and ensuring a stable convolutional process.
The experiment uses MATLAB 2020a to establish a CNN prediction model, uses the filtered near-infrared characteristic wavelength to construct a generalized 2D-COS image as the input, and the output layer has a neuron for regression of classification results.
Results and discussion
Uplc-q-tof-ms result analysis.
According to the Q-TOF experimental method described in Section “ UPLC-Q-TOF-MS experimental method ”, chemical composition analysis was conducted on wine samples from six production regions. The experimental results indicate that indole, sulfacetamide, and caffeine can be used as characteristic substances for tracing the origin of experimental samples. Three substances were t-tested using SPSS software (Version 19.0, Available from https://www.ibm.com/cn-zh/spss ), with p-values of 0.03, 0.02, and 0.05, respectively. The results indicate that all three substances can be used as characteristic components of the experimental wine sample. The main differential metabolites analysis results are shown in Table 1 .
To screen the characteristic wavelength range, the structural formulas of the three characteristic substances obtained from the experiment were analyzed. Indole is an aromatic heterocyclic organic compound, consisting of a six-membered benzene ring fused to a five-membered nitrogen-containing pyrrole ring 22 , 23 . Therefore, according to the IR functional group correlation table, this substance may exist within the range of 1000–1400 nm. Based on the analysis of the IR main functional group correlation table, it is known that acetamide may exist in the 1000–1400 nm range 24 . Caffeine is a biologically active alkaloid compound of methylxanthine, with a spectral range of 1500–1800 nm.
Therefore, the spectral range of 1000–1400 nm and 1500–1800 nm are the characteristic regions in the near-infrared spectrum. The near-infrared spectra of two characteristic bands are shown in Fig. 4 .
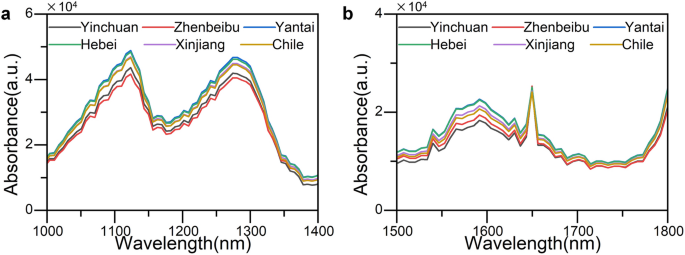
Characteristic regions ( a 1000–1400 nm, b 1500–1800 nm).
The 2D-COS spectra images analysis
Based on the Q-TOF experimental results, 720 two-dimensional correlation spectral images were drawn in two feature band ranges, with 180 synchronous correlation spectral images and 180 asynchronous correlation spectral images for each feature band region. The synchronous and asynchronous correlation spectral images of wine from different regions in the feature regions of 1000–1400 nm and 1500–1800 nm are shown in Fig. 5 A–D represent synchronous correlation spectra in the 1000–1400 nm wavelength range, synchronous correlation spectra in the 1500–1800 nm wavelength range, asynchronous correlation spectra in the 1000–1400 nm wavelength range, and asynchronous correlation spectra in the 1500–1800 nm wavelength range, respectively. YC, ZB, HB, YT, XJ, and CL represent regions of origin in Yinchuan, Zhenbeibu, Hebei, Yantai, Xinjiang, and Chile.
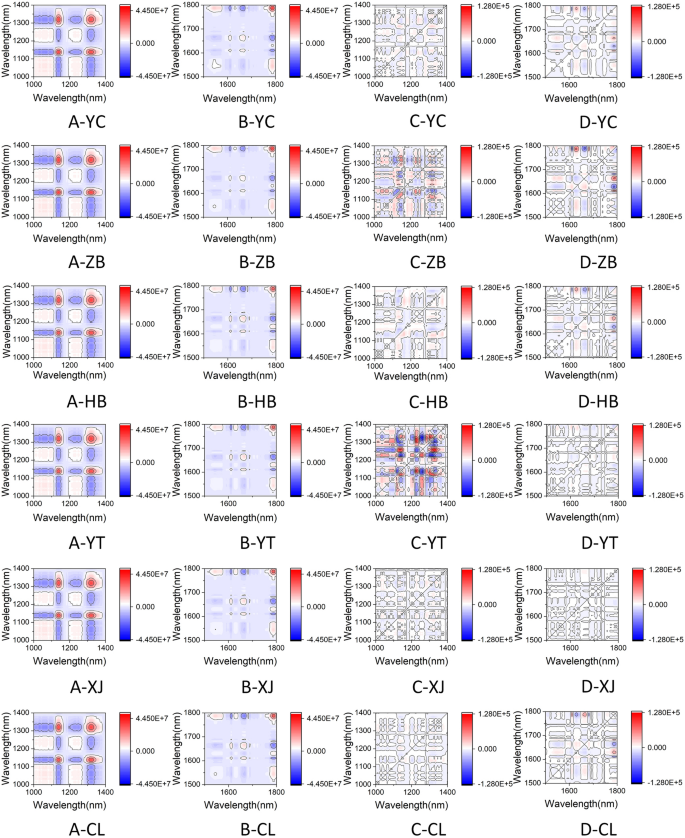
Six sources of two-dimensional correlation spectral images ( A : synchronous correlation spectrum in the 1000–1400 nm wavelength range; B : synchronous correlation spectrum in the 1500–1800 nm wavelength range; C : asynchronous correlation spectrum in the 1000–1400 nm wavelength range; D : asynchronous correlation spectrum in the 1500–1800 nm wavelength range. YC: Yinchuan, Ningxia; ZB: Zhenbeibu, Ningxia; HB: Changli, Hebei; YT: Yantai, Shandong; XJ: Turpan, Xinjiang; CL: Limari Valley, Chile).
For synchronous correlation spectra, the peak on the diagonal is called an automatic peak, which represents the similarity of spectral intensity changes with disturbance at a certain variable 25 , 26 . As shown in column 5A of Fig. 5 A, there are two obvious strong automatic peaks on the diagonal of the 1000–1400 nm synchronous correlation spectrum, located at 1139 nm and 1323 nm respectively. As shown in column 5B of Fig. 5 B, there is a clear strong automatic peak and multiple weak automatic peaks on the diagonal of the 1500–1800 nm synchronous correlation spectrum, with the strong automatic peak located at 1788 nm. It indicates that the spectral intensity changes of the samples at 1139 nm, 1323 nm, and 1788 nm are consistent, with the maximum similarity change, and the automatic peak has the maximum positive value. The peaks on the non diagonal lines of the synchronous correlation spectrum are called cross peaks, and their intensity can be positive or negative, indicating the positive or negative correlation of spectral intensity changes at the variable under disturbance. As shown in column 5A of Fig. 5 A, there is a positive strong cross peak at 1138 nm and 1320 nm, indicating a positive correlation in the spectral intensity change here. There are negative strong cross peaks at 1233 nm and 1320 nm, 1248 nm and 1320 nm, 1319 nm and 1090 nm, indicating a negative correlation change in spectral intensity.
The asynchronous correlation spectrum represents the difference in spectral intensity changes between two variables. There are no automatic peaks in the asynchronous correlation spectrum, and only cross peaks appear when the intensities of the two dynamic spectra undergo non phase (delayed or accelerated) changes. Generally speaking, asynchronous correlation spectra have higher resolution than synchronous correlation spectra, but due to complex cross peaks, the asynchronous correlation spectra of some complex mixtures are often difficult to interpret. From columns C and D, it is not difficult to see that wine samples from different regions within the same wavelength band have significant differences in asynchronous correlation spectra, making it difficult to accurately distinguish them based on vision. Therefore, it is necessary to further analyze using convolutional neural network models.
Analysis of CNN model results
Randomly divide the synchronous correlation spectral images and asynchronous correlation spectral images of each feature band from each production area into 2/3 training sets and 1/3 testing sets. Each time the dataset was randomly divided, out of a total of 720 two-dimensional spectral images of various types in each characteristic band of the six production areas, 480 were used as the training set and 240 were used as the test set. To avoid the randomness of single modeling results, the samples were randomly divided into 50 training and testing sets, and CNN origin traceability models for different varieties of wine were established. The average classification accuracy of the 50 sets was taken as the model accuracy.
To demonstrate that the classification performance of the model established by combining two-dimensional correlated spectral images with CNN is superior to that established by using one-dimensional near-infrared spectroscopy and traditional classification methods, two feature wavelengths were combined with LDA 27 , 28 , 29 algorithm and SVM 30 , 31 algorithm to establish a classification model. The near-infrared spectra of each feature band were still randomly divided into 2/3 training sets and 1/3 test sets 50 times for modeling, and take the average of 50 classification accuracies as the model accuracy.
The experimental results are shown in Table 2 . The accuracy of the CNN model test set established using asynchronous correlation spectral images drawn in two characteristic bands of 1000–1400 nm and 1500–1800 nm is 0.96 and 0.93, respectively. The results are superior to the LDA model and SVM model's 0.91, 0.83, 0.90 and 0.86, and their classification accuracy is basically consistent with the training set, indicating that the established model is stable and reliable. The CNN model established for synchronous correlation spectral images of wine has test set accuracy of 0.91 and 0.87 in the two feature bands of 1000–1400 nm and 1500–1800 nm. Compared to the classification model established for asynchronous correlation spectral images in the same feature band, the accuracy of the classification model is lower, but the classification results are still better than traditional LDA and SVM models.
The results indicate that the model established using asynchronous correlation spectral images has the strongest generalization ability and the highest classification accuracy, making it more suitable for establishing deep learning models based on image processing to identify different regions of wine. Due to the higher resolution of the preprocessed asynchronous correlation spectral image compared to the synchronous correlation spectrum, and the relatively complex non phase changes occurring in the cross peaks, the spectral resolution is more pronounced, and there are significant differences between samples of the same type, which is more conducive to distinguishing CNN models. The synchronous correlation spectrum essentially provides the same information as the one-dimensional spectrum. Although it can more clearly show the small peaks that were previously mixed in the one-dimensional spectrum when extended to two-dimensional, it has high consistency in phase changes, making the synchronous correlation spectrum images of different regions extremely similar and difficult to effectively distinguish. In addition, the accuracy of the three models based on the 1000–1400 nm feature region is higher than that based on the 1500–1800 nm feature region. This may be because the 1000–1400 nm feature band is determined by two different substances, and the number and types of molecular functional groups are more than the 1500–1800 nm feature band, providing more feature components in the infrared spectrum.
Summarizing the above experimental results, the synchronous correlation spectral images drawn based on two feature bands are more suitable for establishing deep learning models, which contain more information suitable for wine traceability. At the same time, the synchronous correlation spectral image drawn in the 1000–1400 nm feature area has higher accuracy, which can more effectively distinguish wine samples from different regions, thereby simplifying the model complexity. This method can effectively trace wine from different origins in cases of significant sample differences, providing a possible method for protecting wine geographical indications and improving the wine traceability system.
Conclusions
This experiment combines metabolomics analysis methods with near-infrared spectroscopy to locate the differential substances in wine from different regions based on UPLC-Q-TOF-MS analysis results. By analyzing the main chemical bonds of the differential substances, the corresponding spectra of the main infrared functional groups are searched, and the characteristic regions of near-infrared spectroscopy are screened. While effectively retaining more useful spectral information, irrelevant variables in the spectrum are removed as much as possible. In addition, the two-dimensional correlation spectral images drawn from the selected feature bands were combined with CNN to transform the complex one-dimensional spectral data analysis process into a relatively simple two-dimensional correlation spectral image processing problem, which was superior to traditional spectroscopy research and effectively avoided the common wave peak aliasing problem in near-infrared spectroscopy. For the collected wine samples from six different regions, this method has significant advantages in wine origin traceability and has high stability, providing a new method for wine origin traceability.
Data availability
The datasets generated and/or analyzed during the current study are not publicly available due to further investigation running on the same project for futuristic solutions but are available from the corresponding author on reasonable request.
Shen, X., Yuan, Y. & Nie, J. Research progress of stable isotopes in wine origin tracing. Sino-Overseas Grapevine Wine 6 , 60–65 (2020).
Google Scholar
Barbos, A. & Hartman, J. Reputational effects on third-party agents: A study of the market for fine and rare wines. J. Econ. Behav. Organ. 208 , 359–372 (2023).
Article Google Scholar
Sudol, P. E. et al. Untargeted profiling and differentiation of geographical variants of wine samples using headspace solid-phase microextraction flow-modulated comprehensive two-dimensional gas chromatography with the support of tile-based Fisher ratio analysis. J. Chromatogr. A 1662 , 462735 (2022).
Article CAS PubMed Google Scholar
Zhang, X.-K., Lan, Y.-B., Huang, Y., Zhao, X. & Duan, C.-Q. Targeted metabolomics of anthocyanin derivatives during prolonged wine aging: Evolution, color contribution and aging prediction. Food Chem. 339 , 127795 (2021).
Wu, H. et al. Origin verification of French red wines using isotope and elemental analyses coupled with chemometrics. Food Chem. 339 , 127760 (2021).
Article ADS CAS PubMed Google Scholar
Conti, M. E. et al. From land to glass: An integrated approach for quality and traceability assessment of top Italian wines. Food Control 158 , 110226 (2024).
Article CAS Google Scholar
Budziak-Wieczorek, I. et al. Evaluation of the quality of selected white and red wines produced from Moravia Region of Czech Republic using physicochemical analysis, FTIR infrared spectroscopy and chemometric techniques. Molecules 28 , 6326 (2023).
Article CAS PubMed PubMed Central Google Scholar
Schorn-García, D. et al. Assessment of variability sources in grape ripening parameters by using FTIR and multivariate modelling. Foods 12 , 962 (2023).
Article PubMed PubMed Central Google Scholar
Vladimír, M. et al. Chemometric approach to characterization of the selected grape seed oils based on their fatty acids composition and FTIR spectroscopy. Sci. Rep. 11 , 19256 (2021).
Article ADS PubMed PubMed Central Google Scholar
Lu, B. et al. Identification of Chinese red wine origins based on Raman spectroscopy and deep learning. Spectrochim. Acta Part A Mol. Biomol. Spectrosc. 291 , 122355 (2023).
Ta, N., Wei, H., Hu, Z., Cao, X. & Xiao, M. Wine component tracing method based on near infrared spectrum fusion machine learning. Front. Sustain. Food Syst. 7 , 1197508 (2023).
Cozzolino, D. The role of visible and infrared spectroscopy combined with chemometrics to measure phenolic compounds in grape and wine samples. Molecules 20 , 726–737 (2015).
Article PubMed Google Scholar
Dong, J.-E., Zuo, Z.-T., Zhang, J. & Wang, Y.-Z. Geographical discrimination of Boletus edulis using two dimensional correlation spectral or integrative two dimensional correlation spectral image with ResNet. Food Control 129 , 108132 (2021).
Liu, Z., Yang, S., Wang, Y. & Zhang, J. Discrimination of the fruits of Amomum tsao-ko according to geographical origin by 2DCOS image with RGB and Resnet image analysis techniques. Microchem. J. 169 , 106545 (2021).
Noda, I. Two-dimensional infrared (2D IR) spectroscopy: Theory and applications. Appl. Spectrosc. 44 , 550–561 (1990).
Article ADS CAS Google Scholar
Noda, I. Generalized two-dimensional correlation method applicable to infrared, Raman, and other types of spectroscopy. Appl. Spectrosc. 47 , 1329–1336 (1993).
Li, Y., Shen, Y., Yao, C.-L. & Guo, D.-A. Quality assessment of herbal medicines based on chemical fingerprints combined with chemometrics approach: A review. J. Pharm. Biomed. Anal. 185 , 113215 (2020).
Dong, F. et al. Identification of the proximate geographical origin of wolfberries by two-dimensional correlation spectroscopy combined with deep learning. Comput. Electron. Agric. 198 , 107027 (2022).
Liu, C., Xu, F., Zuo, Z. & Wang, Y. An identification method of herbal medicines superior to traditional spectroscopy: Two-dimensional correlation spectral images combined with deep learning. Vib. Spectrosc. 120 , 103380 (2022).
Nakandala, S., Zhang, Y. & Kumar, A. Cerebro: A data system for optimized deep learning model selection. Proc. VLDB Endowment 13 , 2159–2173 (2020).
Dhanoa, M., Lister, S., Sanderson, R. & Barnes, R. The link between multiplicative scatter correction (MSC) and standard normal variate (SNV) transformations of NIR spectra. J. Near Infrared Spectrosc. 2 , 43–47 (1994).
Arevalo-Villena, M., Bartowsky, E. J., Capone, D. & Sefton, M. A. Production of indole by wine-associated microorganisms under oenological conditions. Food Microbiol. 27 , 685–690 (2010).
Rosales, P. F., Bordin, G. S., Gower, A. E. & Moura, S. Indole alkaloids: 2012 until now, highlighting the new chemical structures and biological activities. Fitoterapia 143 , 104558 (2020).
Agbonkonkon, N. et al. Faster, reduced cost calibration method development methods for the analysis of fermentation product using near-infrared spectroscopy (NIRS). J. Ind. Microbiol. Biotechnol. 48 , kuab033 (2021).
Lefevre, T., Arseneault, K. & Pézolet, M. Study of protein aggregation using two-dimensional correlation infrared spectroscopy and spectral simulations. Biopolym.: Original Res. Biomol. 73 , 705–715 (2004).
Huang, H., Malkov, S., Coleman, M. & Painter, P. Application of two-dimensional correlation infrared spectroscopy to the study of miscible polymer blends. Macromolecules 36 , 8156–8163 (2003).
Arndt, M., Drees, A., Ahlers, C. & Fischer, M. Determination of the geographical origin of walnuts ( Juglans regia L) Using near-infrared spectroscopy and chemometrics. Foods 9 , 1860 (2020).
Yang, Y. et al. Use of near-infrared spectroscopy and chemometrics for fast discrimination of Sargassum fusiforme . J. Food Compos. Anal. 110 , 104537 (2022).
Raypah, M. E., Zhi, L. J., Loon, L. Z. & Omar, A. F. Near-infrared spectroscopy with chemometrics for identification and quantification of adulteration in high-quality stingless bee honey. Chemom. Intell. Lab. Syst. 224 , 104540 (2022).
Schütz, D., Riedl, J., Achten, E. & Fischer, M. Fourier-transform near-infrared spectroscopy as a fast screening tool for the verification of the geographical origin of grain maize ( Zea mays L.). Food Control 136 , 108892 (2022).
Onmankhong, J., Ma, T., Inagaki, T., Sirisomboon, P. & Tsuchikawa, S. Cognitive spectroscopy for the classification of rice varieties: A comparison of machine learning and deep learning approaches in analysing long-wave near-infrared hyperspectral images of brown and milled samples. Infrared Phys. Technol. 123 , 104100 (2022).
Download references
Acknowledgements
This research was funded by the Natural Science Foundation of Ningxia(2022AAC03244, 2022AAC03006), National Natural Science Foundation of China (No. 62361001); Leading Talent Project Plan of the State Ethnic Affairs Commission. Ningxia Technology Innovative Team of Advanced Intelligent Perception and Control, Leading talents in scientific and technological innovation of Ningxia; Graduate Student Innovation Project of North Minzu University (No.YCX23133);The Ningxia Autonomous Region Graduate Education Reform Project "Research on the Cultivation Model of Graduate Innovation Ability Based on Tutor Team Collaboration", (No.YJG202104); And Ningxia 2021 Industry University Collaborative Education Project "Construction and Exploration of the Four in One Practice Platform under the Background of New Engineering", (No.cxy2021017).
Author information
Authors and affiliations.
School of Electrical and Information Engineering, North Minzu University, No. 204 North Wenchang Street, Yinchuan, 750021, Ningxia, China
Zhuo-Kang Wang, Na Ta & Jin-Hang Wang
School of Medical Technology, North Minzu University, No. 204 North Wenchang Street, Yinchuan, 750021, Ningxia, China
Hai-Cheng Wei
School of Information Engineering, Ningxia University, Yinchuan, 750021, China
College of Biological Science and Engineering, North Minzu University, Yinchuan, 750021, Ningxia, China
You can also search for this author in PubMed Google Scholar
Contributions
All authors listed have made a substantial, direct, and intellectual contribution to the work and approved it for publication.
Corresponding author
Correspondence to Hai-Cheng Wei .
Ethics declarations
Competing interests.
The authors declare no competing interests.
Additional information
Publisher's note.
Springer Nature remains neutral with regard to jurisdictional claims in published maps and institutional affiliations.
Rights and permissions
Open Access This article is licensed under a Creative Commons Attribution 4.0 International License, which permits use, sharing, adaptation, distribution and reproduction in any medium or format, as long as you give appropriate credit to the original author(s) and the source, provide a link to the Creative Commons licence, and indicate if changes were made. The images or other third party material in this article are included in the article's Creative Commons licence, unless indicated otherwise in a credit line to the material. If material is not included in the article's Creative Commons licence and your intended use is not permitted by statutory regulation or exceeds the permitted use, you will need to obtain permission directly from the copyright holder. To view a copy of this licence, visit http://creativecommons.org/licenses/by/4.0/ .
Reprints and permissions
About this article
Cite this article.
Wang, ZK., Ta, N., Wei, HC. et al. Research of 2D-COS with metabolomics modifications through deep learning for traceability of wine. Sci Rep 14 , 12598 (2024). https://doi.org/10.1038/s41598-024-63280-9
Download citation
Received : 26 October 2023
Accepted : 27 May 2024
Published : 01 June 2024
DOI : https://doi.org/10.1038/s41598-024-63280-9
Share this article
Anyone you share the following link with will be able to read this content:
Sorry, a shareable link is not currently available for this article.
Provided by the Springer Nature SharedIt content-sharing initiative
- Traceability of wine origin
- Convolutional neural network
- Metabolomics
By submitting a comment you agree to abide by our Terms and Community Guidelines . If you find something abusive or that does not comply with our terms or guidelines please flag it as inappropriate.
Quick links
- Explore articles by subject
- Guide to authors
- Editorial policies
Sign up for the Nature Briefing newsletter — what matters in science, free to your inbox daily.

- Open access
- Published: 29 May 2024
Correlation between the triglyceride-glucose index and left ventricular global longitudinal strain in patients with chronic heart failure: a cross-sectional study
- Shuai Zhang 1 , 2 , 3 na1 ,
- Yan Liu 1 , 2 , 3 na1 ,
- Fangfang Liu 1 , 2 , 3 ,
- Qing Ye 1 , 2 , 3 ,
- Dachuan Guo 1 , 2 , 3 ,
- Panpan Xu 1 , 2 , 3 ,
- Tianhao Wei 1 , 2 , 3 ,
- Cheng Zhang 1 , 2 , 3 &
- Huixia Lu 1 , 2 , 3
Cardiovascular Diabetology volume 23 , Article number: 182 ( 2024 ) Cite this article
10 Accesses
Metrics details
Left ventricular global longitudinal strain (GLS) holds greater diagnostic and prognostic value than left ventricular ejection fraction (LVEF) in the heart failure (HF) patients. The triglyceride-glucose (TyG) index serves as a reliable surrogate for insulin resistance (IR) and is strongly associated with several adverse cardiovascular events. However, there remains a research gap concerning the correlation between the TyG index and GLS among patients with chronic heart failure (CHF).
427 CHF patients were included in the final analysis. Patient demographic information, along with laboratory tests such as blood glucose, lipids profiles, and echocardiographic data were collected. The TyG index was calculated as Ln [fasting triglyceride (TG) (mg/dL) × fasting plasma glucose (FPG) (mg/dL)/2].
Among CHF patients, GLS was notably lower in the higher TyG index group compared to the lower TyG index group. Following adjustment for confounding factors, GLS demonstrated gradual decrease with increasing TyG index, regardless of the LVEF level and CHF classification.
Elevated TyG index may be independently associated with more severe clinical left ventricular dysfunction in patients with CHF.
Introduction
Heart failure (HF) is a major global medical challenge with an increasing prevalence and poor prognosis [ 1 , 2 ]. Traditionally, HF has been broadly categorized according to the left ventricular ejection fraction (LVEF) into three groups: heart failure with reduced ejection fraction (HFrEF, LVEF is ≤ 40%), heart failure with mildly-reduced ejection fraction (HFmrEF, LVEF 41–49%), and heart failure with preserved ejection fraction (HFpEF, LVEF ≥ 50%), each exhibiting varying degrees of systolic and diastolic dysfunction [ 3 , 4 ]. While LVEF, typically assessed via echocardiography, remains pivotal for HF diagnosis, characterization, prognosis, patient triage, and treatment selection [ 5 , 6 ], However, this parameter is constrained not only by technical limitations but also by pathophysiological factors, including situations where the ratio of stroke volume to left ventricular (LV) cavity size remains unchanged [ 7 ]. Moreover, LVEF fails to differentiate between healthy hearts and HFpEF patients and inadequately reflects actual cardiac function [ 8 , 9 ]. Strain analysis, emerging as a promising tool for evaluating cardiac contractility and myocardial deformation, offers a more comprehensive characterization of patients [ 10 ]. A growing number of studies have demonstrated that strain provides better predictor of prognostic value in HF patients compared to LVEF [ 5 , 11 , 12 , 13 ].
Insulin resistance (IR), indictive of metabolic disorders and systemic inflammation, is an independent and significant risk factor for HF [ 14 , 15 , 16 ]. However, the gold standard method for measuring IR, the hyperinsulinemic-euglycemic clamp (HIEC), is time-consuming and invasive [ 17 , 18 ], limiting its clinical applicability. The triglyceride-glucose (TyG) index has recently been regarded as a simpler, cost-effective, and reliable surrogate marker of IR, demonstrating high concordance with the HIEC [ 19 , 20 , 21 ]. Previous studies have found that the TyG index may play an essential role in the impairment of left ventricular structure and function [ 22 , 23 ] and is associated with the development of HF and poor prognosis [ 24 , 25 , 26 , 27 ]. Na et al. reported an independent association between the higher TyG index and reduced GLS in patients with coronary artery disease (CAD) [ 28 ].
Although several recent studies have established a link between the TyG index and left ventricular function, no study has explored the relationship between the TyG index and GLS in CHF. Thus, we investigated the relationship between the TyG index and GLS in CHF patients and, for the first time, examining its relevance across different HF groups.
Study population
The study adhered to the principles outlined in the Declaration of Helsinki and was approved by the Ethics Review Committee of Qilu Hospital of Shandong University.
This retrospective study spanned from September 2020 to December 2023 and involved 573 consecutive patients presenting with CHF at Qilu Hospital of Shandong University. Among them, 49 patients were excluded due to poor image quality, 32 patients lacked fasting glucose and triglycerides, 26 patients were younger than 18 years old, and 39 patients had malignant neoplasms. Ultimately, 427 patients with CHF were included in the final analysis, comprising 175 HFrEF, 92 HFmrEF, and 160 HFpEF (Fig. 1 ).
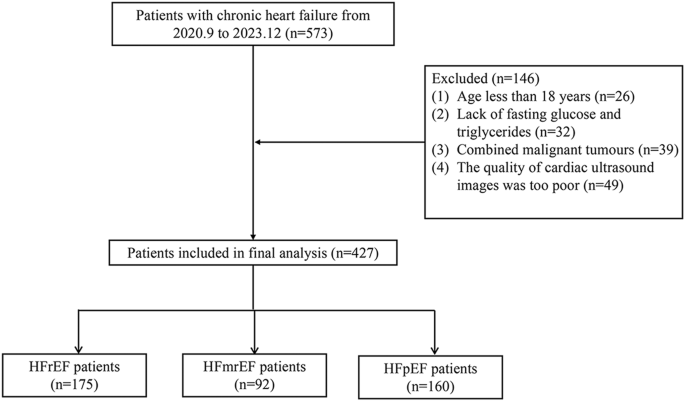
Flow diagram of patient selection
Echocardiographic measurement
Echocardiographic images were acquired by an experienced echocardiogram physician. Adhering to the 2016 guidelines provided by the American Society of Echocardiography and the European Society of Cardiovascular Imaging, participants were applied to the anterior chest area and connected to a three-lead ECG and we instructed all participants to hold their breath during image acquisition to ensure high-quality images [ 29 ]. The left ventricular end systolic volume (LVESV), left ventricular end diastolic volume (LVEDV), left ventricular end diastolic dimension (LVDd), left ventricular end systolic dimension (LVDs), left ventricular posterior wall thickness (LVPWT), interventricular septal thickness (IVST), LVEF and GLS was acquired by GEVividE95 color ultrasound diagnostic instrument (GE of USA) with an M5s probe and a frequency \(1.5 \sim 3.6\) MHz.
Data collection and definitions
Data on demographic characteristics, medical history, personal history, and medication usage were collected through an electronic medical record system. Venous blood samples were drawn after overnight fasting to measure levels of N-terminal pro-brain natriuretic peptide (NT-proBNP), fasting plasma glucose (FPG), serum creatinine (SCr), and lipid profile. CHF was defined according to the 2021 ESC Guidelines for the diagnosis and treatment of acute and chronic heart failure [ 30 ]. Body mass index (BMI) was calculated as weight (kg) divided by the square of height (m 2 ). Hypertension was defined as systolic blood pressure ≥ 140 mmHg and/or diastolic blood pressure ≥ 90 mmHg, or the use of antihypertensive medication. Diabetes mellitus (DM) was defined as an FPG level ≥ 7.0 mmol/L or 2 h plasma glucose level ≥ 11.1 mmol/L following an oral glucose tolerance test (OGTT), or the use of oral hypoglycemic agents or insulin [ 31 ]. Hyperlipidemia was diagnosed with and ICD-10 code E78 along with lipid-lowering medication, or a total serum cholesterol ≥ 240 mg/dL) [ 32 ]. CAD was confirmed by the presence of at least one major coronary artery with ≥ 50% stenosis as evaluated by coronary angiography (CAG), including the left anterior descending, left circumflex, and right coronary arteries [ 33 ]. The diagnosis of obstructive sleep apnea (OSA) relied on an AHI ≥ 5/h, accompanied by suggestive symptoms according to the International Classification of Sleep Disorders (Third Edition) criteria [ 34 ]. Patients with a self-reported diagnosis of hypertension, DM, hyperlipidemia, CAD, or OSA, substantiated by corresponding medical records, were also identified as having these conditions. The estimated glomerular filtration rate (eGFR) was calculated using the following equations: eGFR (mL/min/1.73 m 2 ) = 175 × SCr (mg/dL) −1.234 × age (year) −0.179 × 0.79 (in the case of women) [ 35 ]. Chronic kidney disease (CKD) was defined as an eGFR < 60 mL/min/1.73 m 2 . The TyG index was determined by the formula: Ln [fasting triglyceride (TG) (mg/dL) × FPG (mg/dL)/2] [ 21 ]. Reduced GLS is now defined as GLS < 11.2%, which represents the value of GLS less than the median in HF. Left ventricular mass (LVM, g) = 0.8 [1.04 (LVDd + LVPWT + LVST) 3 − (LVDd) 3 ] + 0.6. Body surface area (BSA, m 2 ) = 0.0061*height (cm) + 0.0128*weight (kg) − 0.1529. Left ventricular mass index (LVMi, g/m 2 ) = LVM (g)/BAS(m 2 ).
Statistical analysis
Statistical analysis was performed using SPSS version 25.0 (SPSS, Chicago, IL, United States), R software version 4.2.0 (R Foundation for Statistical Computing, Vienna, Austria), and GraphPad PRISM version 10.0 (GraphPad Software—San Diego, CA, USA). Initially, we assessed the baseline characteristics of the overall enrolled population and categorized them according to the tertile of the TyG index. Continuous variables were presented as mean ± standard deviation (SD) or median (interquartile range) and comparisons were made using Student’s t-test or Mann–Whitney U-test as appropriate. Categorical variables were expressed as counts and percentages and analyzed using the chi-square or Fisher exact tests. We used Pearson or Spearman correlation analysis to evaluate the association between the TyG index and cardiovascular risk factors in CHF, HFrEF, HFmrEF, and HFpEF. To mitigated bias from multicollinearity, we calculated the variance inflation factor (VIF) of the variables in the model (Additional file 1 : Table S1). We did not find evidence of collinearity in the models, given the VIF of < 10. The association between the TyG index and GLS in CHF and its three subtypes was assessed using multivariable linear regression. Three models were constructed following adjusting for potential confounders: model 1 adjusted for age and gender; model 2 further adjusted for BMI, hypertension, CAD, DM, hyperlipidemia, OSA, smoking, and drinking; and model 3 additionally adjusted for LVEF, NT-proBNP, total cholesterol (TC), low-density lipoprotein cholesterol (LDL-C), high-density lipoprotein cholesterol (HDL-C), eGFR, E/e’, and LVMi. Furthermore, the TyG index was progressively included as both a continuous and categorical variable in multivariable logistic regression. To further explore the relationship between the TyG index and GLS, we employed restricted cubic spline curve (RCS) analysis. Subgroup analysis was conducted based on gender, hypertension, hyperlipidemia, DM, CAD, and BMI to assess potential variations in the association between the TyG index and GLS, with interaction P-values calculated accordingly. Finally, sensitivity analysis was performed by excluding patients with a history of lipid-lowering or glucose-lowering drug use, a history of sodium-glucose cotransporter-2 inhibitors (SGLT-2i) usage, and those with DM. Statistical significance was set at P-values less than 0.05.
427 CHF patients were included in this study with a mean age of 48.37 ± 14.50 years, and 271 (63.5%) were males. As shown in Table 1 , the patients were stratified into 3 groups according to the tertiles of the TyG index (tertile 1: n = 143, TyG index < 8.38; tertile 2: n = 141, 8.38 ≤ TyG index < 8.82; and tertile 3: n = 143, TyG index ≥ 8.82). As illustrated in Table 1 , higher baseline TyG index was associated with increased prevalence of DM and hyperlipidemia as well as lower LVEF and GLS, and a higher ratio of males and patients with a history of coronary artery bypass grafting (CABG). Statistical significance was also found in other parameters such as E/e’, FPG, TC, LDL-C, HDL-C, TG, and hypoglycemic drug usage (all P-values < 0.05). No significant difference was observed in the other indicators (Table 1 ). Further comparisons of baseline characteristics of HFrEF, HFmrEF, and HFpEF was showed in Additional file 1 : Table S2.
In CHF patients, there was a significant reduction in GLS with increasing TyG index, as depicted in Fig. 2 and Fig. 3 . We plotted histograms and scatter plots in HFrEF, HFmrEF, and HFpEF and obtained similar results in HFrEF, HFmrEF, and HFpEF patients (Additional file 1 : Figures S1, S2). Pearson or Spearman correlation analysis revealed significant positive correlations between the TyG index and TC and LDL-C (all P-values < 0.05) and negative association with HDL-C, LVEF, and GLS (all P-values < 0.05) (Table 2 ), with GLS demonstrating the strongest negative correlation (r = − 0.365, P < 0.001). Additional correlation analysis for the three HF types (HFrEF, HFmrEF, and HFpEF) are detailed in Additional file 1 : Table S3.
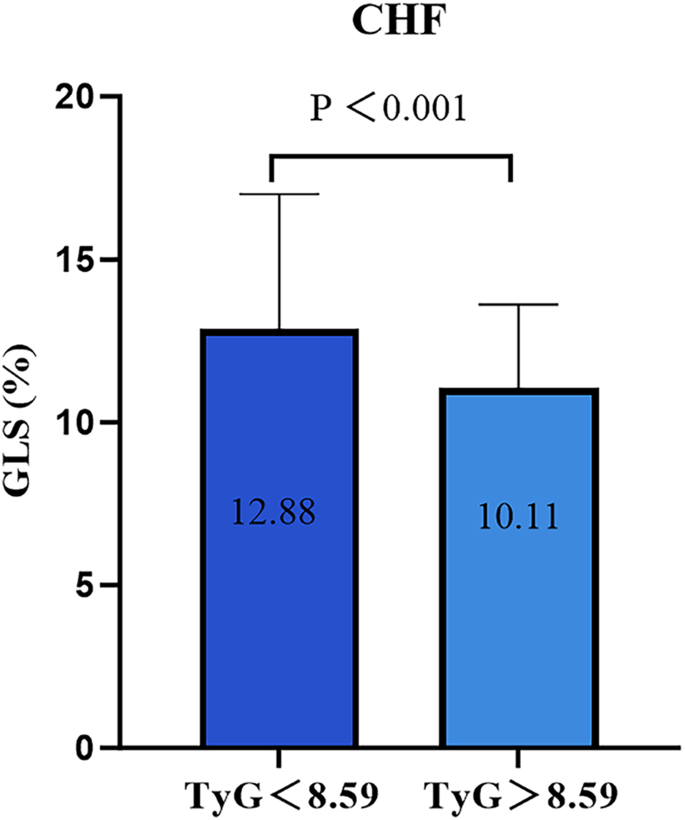
Comparison of GLS of high TyG index group with low TyG index. TyG index triglyceride-glucose index, GLS global longitudinal strain
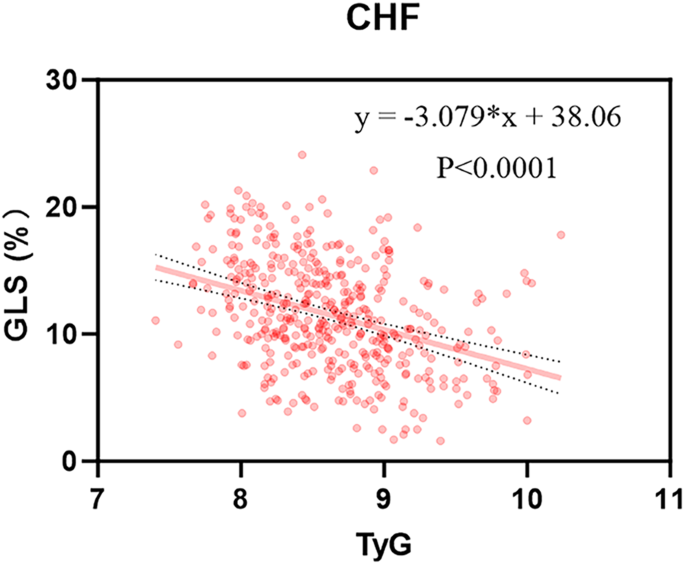
Scatterplot of GLS and TyG index for CHF. TyG index triglyceride-glucose index GLS global longitudinal strain, CHF chronic heart failure
Multivariable linear regression models were constructed to explore the independent association between the TyG index and GLS in CHF (Table 3 ). The β coefficients (95%CI) for the association between a 1-unit increase in the TyG index and decrease in GLS were documented as − 2.94 (− 3.70 to − 2.18), − 3.06 (− 3.84 to − 2.28), and − 1.99 (− 2.55 to − 1.44) in models 1, 2, and 3, respectively. In addition, when the TyG index was included as a categorical variable in the regression model, a significant linear trend with GLS was observed with a P-value < 0.05. In model 3, GLS for the highest tertile of TyG index decreased by 2.38 units [β (95% CI) − 2.38 (− 3.08 to − 1.68), P < 0.001], compared to the lowest tertile.
Multivariable logistic regression analysis revealed the TyG index as a risk factor for reduced GLS (Table 4 ). The risk of reduced GLS in CHF patients increased by 244%, 299%, and 337% with a 1-unit increase in the TyG index in models 1, 2, and 3, respectively. Similarly, compared to tertile 1, the risk of reduced GLS in tertile 3 surged by 306%, 398%, and 549% in models 1, 2, and 3, respectively. Similarly, we utilized multivariable linear and logistic regression analysis to investigated whether the TyG index is independently correlated with GLS in HFrEF, HFmrEF, and HFmrEF, with results being provided in Additional file 1 : Tables S4, S5. We evaluated the continuous relationship between the TyG index and GLS using RCS based on a multivariable logistic regression model (Fig. 4 ). Following adjustments for traditional factors, the TyG index demonstrated a gradual increase, accompanied by a corresponding rise in the OR of the lower GLS. Furthermore, we explored the relationship between the TyG index and GLS in the three types of HF using RCS (Additional file 1 : Figure S3). To validate the relationship between the TyG index and GLS, we conducted additional sensitivity analyses. Specifically, we excluded three groups of individuals from the analysis: group 1: those with DM; group 2: those with a history of lipid-lowering or hypoglycemic usage; group 3: those with a history of SGLT-2i usage (Table 5 ). The correlation between the TyG index and GLS was also present in three groups, which may indicate that the correlation is independent of medication and DM. We performed corresponding sensitivity analyses in different HF types (Additional file 1 : Table S6).
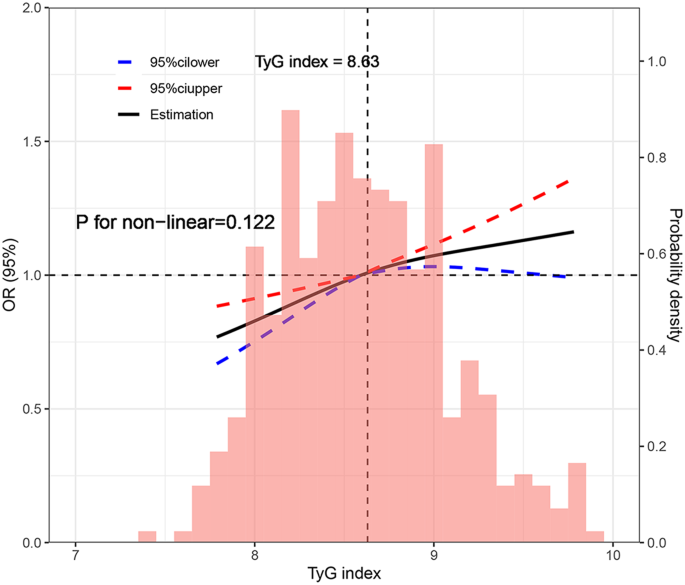
Restricted cubic spline plot between the TyG index and reduced GLS. Reduced GLS: GLS < 11.2%. Adjusted for age, gender, BMI, hypertension, CAD, DM, hyperlipidemia, OSA, smoking, drinking, LVEF, NT-proBNP, TC, LDL-C, HDL-C, eGFR, E/e’, LVMi. TyG index triglyceride-glucose index, GLS global longitudinal strain BMI body mass index, CAD coronary artery disease, DM diabetes mellitus, OSA obstructive sleep apnea, LVEF left ventricular ejection fraction, NT-proBNP N-terminal pro-brain natriuretic peptide, TC total cholesterol, LDL-C low-density lipoprotein cholesterol, HDL-C , high-density lipoprotein cholesterol, eGFR estimated glomerular filtration rate, E/e ’ the ratio of early diastolic mitral inflow velocity to septal mitral annulus tissue relaxation velocity in early diastole, LVMi left ventricular mass index
The association between the TyG index and GLS for CHF was examined in subgroup analyses, and the calculated interaction P values are shown in Fig. 5 . No significant interaction was found. Across various groups, the TyG index exhibited statistical significance in predicting reduced GLS, suggesting stability and consistency in the TyG index and GLS relationship. Additionally, subgroup analyses were conducted in HFrEF, HFmrEF, and HFpEF (Additional file 1 : Table S7).
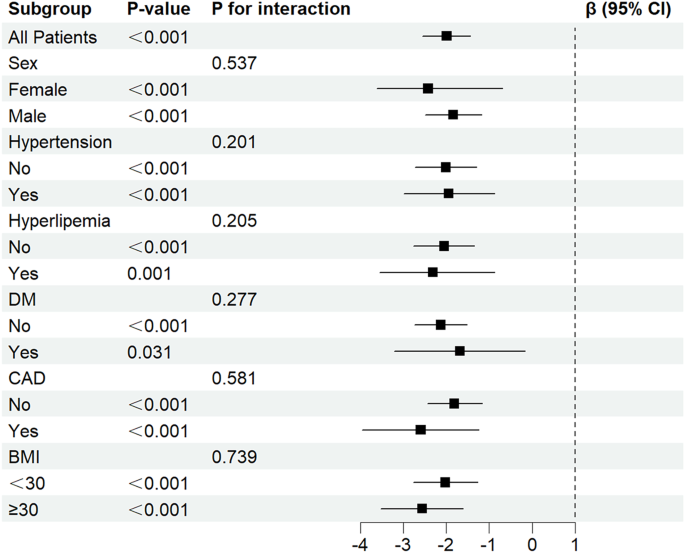
Subgroup analysis of the correlation between TyG index and GLS in patients with CHF. T yG index triglyceride-glucose index GLS global longitudinal strain, CHF chronic heart failure, DM diabetes mellitus, CAD coronary artery disease, BMI body mass index
In the present study, we investigated the relationship between the TyG index and GLS in CHF, and further explored this association across different subgroups. After multivariable linear and logistic regression, the TyG index emerged as an independent indicator of reduced GLS in CHF. Elevated TyG index remained independently associated with reduced GLS in all three groups of patients with HFrEF, HFmrEF and HFpEF. This suggests that an elevated TyG index may be independently associated with more severe left ventricular insufficiency in CHF, regardless of specific classifications of CHF and the levels of LVEF.
A growing number of studies have suggested that GLS outperforms LVEF as a prognostic marker in HF patients [ 5 , 11 , 12 , 13 , 36 ]. A previous study demonstrated GLS’s super prognostic value for mortality compared to LVEF in patients with acute heart failure (AHF) [ 36 ]. GLS is associated with cardiac death and all-cause mortality in patients with CHF, independent of clinical features and cardiac structure and function [ 12 ]. Similarly, GLS has independently predicted poor long-term prognosis of HFrEF [ 11 ], and HFpEF [ 5 ].
Moreover, the Atherosclerosis Risk in the community study have highlighted the association between hemoglobin A1c (HbA1c) levels and GLS, indicating a nearly linear relationship [ 37 ]. A study analyzing the Framingham Heart Study found a significant association between IR and hypertriglyceridemia and GLS [ 38 ]. Previous study found that while DM itself may not be directly associated with GLS, elevated HbA1c levels have been independently associated with GLS, suggesting a potential direct impact of glucose metabolism on myocardial function [ 12 ]. Li et al. reported that IR is a critical component of metabolic syndrome (MetS), accounting for > 90% of the association between MetS and HF risk [ 39 ].
Previous studies have shown that IR is prevalent in patients with HF, and precedes the development of HF [ 40 ]. IR is also an indicator of HF and heart function deterioration [ 41 ]. IR contributes to adverse cardiac remodeling and dysfunction, thereby increasing cardiovascular risk by inducing glucose and lipid metabolism imbalances and triggering oxidative stress and inflammatory responses, endothelial dysfunction, and ectopic lipid accumulation [ 14 , 15 , 42 , 43 , 44 ]. The TyG index, serving as a reliable surrogate for IR, exhibit a robust association with homeostasis model assessment of HIEC [ 19 , 20 , 21 ].
Most previous studies investigating the impact of elevated TyG index on cardiac function have predominantly focused on non-HF patients and long-term adverse events in various populations. Recently, several cohort studies have confirmed that the TyG index can play a predictive role in the development of HF [ 25 , 27 , 45 ]. Chen et al. identified a significant association between higher TyG index and subclinical LV systolic dysfunction in T2DM patients with LVEF ≥ 50% [ 23 ]. Similarly, Huang et al. showed that elevated baseline and long-term TyG index levels were significantly linked to an increased risk of adverse LV remodeling, LV dysfunction and an increased risk of HF in a US community population without HF and CAD [ 22 ]. Additionally, a cross-sectional study confirmed an independent association between elevated TyG index and reduced GLS in patients with CAD, suggesting a potential association between the TyG index and subclinical left ventricular dysfunction in CAD patients [ 28 ].
SGLT-2 inhibitors could modify myocardial metabolism and have a favorable effect on LV function [ 46 , 47 , 48 ]. Recognizing the interplay between LV remodeling, diastolic function, and LVGLS in prediabetes and diabetes [ 38 , 49 ]. we conducted a sensitivity analysis. This involved excluding patients using SGLT-2i and those with DM, which showed no change in the correlation between the TyG index and GLS. In different subgroups, elevated TyG index was statistically associated with significant reductions in GLS, suggesting stability and consistency of the relationship in patients with CHF.
The TyG index is an easy-to-measure parameter that predicts the incidence of HF in populations ranging from those without DM and CAD to those with DM and CAD [ 22 , 26 , 50 ] and has demonstrated diagnostic ability in distinguishing HFpEF patients from non-HFpEF individuals [ 51 ]. Meanwhile, previous studies have shown that the TyG index is associated with a poor long-term prognosis in HF [ 25 , 27 , 45 ]. The TyG index can therefore be considered as an additional tool in the routine clinical assessment of individuals at risk of HF. In resource-limited countries and settings, heart failure clinics could benefit from incorporating the TyG into their routine assessment. [ 52 ]. Given its ease of measurement, the TyG index can help in stratifying HF patient risk, ultimately enabling healthcare providers to provide more personalized care and tailored advice to patients.
This study revealed that higher TyG index levels were associated with an increased risk of left ventricular insufficiency in CHF and all three types of HF despite adjusting for confounding factors (e.g. age, sex, smoking, hypertension, and diabetes). We also found that the TyG index was associated with a reduced strain of HF in non-DM patients and among those excluding glucose- lowering and lipid-lowering medications, suggesting the stability of the relationship between the TyG index and reduced GLS. By using a novel IR alternative index to the TyG index, our study underscores the important role of IR in strain reduction in selected patients with HF, providing new insights into the pathogenesis of left ventricular insufficiency across the three types of HF.
Several limitations should be acknowledged in this study. (1) We acknowledged the limitations associated with the single-center, cross-sectional design of the study. This design may introduce bias, and we recognize the inability to assess whether the high TyG index group is more susceptible to subsequent adverse cardiovascular events compared to the low TyG index group. (2) While our study demonstrates relatively robust correlations between the TyG index and GLS in CHF across multiple multivariable models and subgroup analyses, we recognize the uncertainty regarding the utility of applying thees results to daily clinical practice. Further research is needed to determine the clinical implications of these findings. (3) We acknowledge the limitation posed by the different cutoff values for the TyG index among HF subgroups, which may restrict the generalization of the results to other populations. Understanding the variability of TyG index cutoffs across different HF subgroups is important for accurately interpreting and applying our findings in diverse clinical contexts. (4) We only analyzed patient GLS data and did not include assessments of left ventricular global circumferential strain or global radial strain, which could provide additional insights into cardiac function. (5) The use of a 2D scatter tracking technique for ultrasound image acquisition may be limited by acoustic window conditions, potentially impacting image quality and the analysis of hypoechoic regions. (6) Finally, we recognize the need for additional research to explore the relationship between the TyG index and GLS under the treatment of CHF. Investigating how the TyG index and GLS evolve in response to HF treatment could provide valuable insights into their clinical significance and potential therapeutic implications.
In summary, our findings suggested that a high TyG index in CHF patients may be independently associated with clinically more pronounced LV dysfunction. Therefore, monitoring TyG index levels could be crucial to mitigate subsequent adverse outcomes.
Availability of data and materials
The datasets used and/or analyzed during the current study are available from the corresponding author on reasonable request.
Abbreviations
- Global longitudinal strain
Left ventricular ejection fraction
Left ventricular
Heart failure
- Triglyceride-glucose index
Insulin resistance
- Chronic heart failure
Fasting plasma glucose
Triglyceride
Heart failure with reduced ejection fraction
Heart failure with mildly-reduced ejection fraction
Heart failure with preserved ejection fraction
Hyperinsulinemic-euglycemic clamp
Coronary artery disease
Left ventricular end systolic volume
Left ventricular end diastolic volume
Left ventricular end diastolic dimension
Left ventricular end systolic dimension
Left ventricular posterior wall thickness
Interventricular septal thickness
N-terminal pro-brain natriuretic peptide
Serum creatinine
Body mass index
Diabetes mellitus
Oral glucose tolerance test
Coronary angiography
Obstructive sleep apnea
Chronic kidney disease
Estimated glomerular filtration rate
Left ventricular mass
Body surface area
Left ventricular mass index
Total cholesterol
Low-density lipoprotein cholesterol
High-density lipoprotein cholesterol
Restricted cubic spline
Acute heart failure
Hemoglobin A1c
McDonagh TA, Metra M, Adamo M, Gardner RS, Baumbach A, Böhm M, Burri H, Butler J, Čelutkienė J, Chioncel O, et al. ESC Guidelines for the diagnosis and treatment of acute and chronic heart failure: developed by the task force for the diagnosis and treatment of acute and chronic heart failure of the European society of cardiology (ESC). With the special contribution of the heart failure association (HFA) of the ESC. Eur J Heart Fail. 2022;24(1):4–131.
Article PubMed Google Scholar
Shah KS, Xu H, Matsouaka RA, Bhatt DL, Heidenreich PA, Hernandez AF, Devore AD, Yancy CW, Fonarow GC. Heart failure with preserved, borderline, and reduced ejection fraction: 5-year outcomes. J Am Coll Cardiol. 2017;70(20):2476–86.
Yancy CW, Jessup M, Bozkurt B, Butler J, Casey DE Jr, Colvin MM, Drazner MH, Filippatos GS, Fonarow GC, Givertz MM, et al. 2017 ACC/AHA/HFSA focused update of the 2013 ACCF/AHA guideline for the management of heart failure: a report of the american college of cardiology/american heart association task force on clinical practice guidelines and the heart failure society of America. Circulation. 2017;136(6):e137–61.
Ponikowski P, Voors AA, Anker SD, Bueno H, Cleland JGF, Coats AJS, Falk V, González-Juanatey JR, Harjola VP, Jankowska EA, et al. 2016 ESC Guidelines for the diagnosis and treatment of acute and chronic heart failure: the task force for the diagnosis and treatment of acute and chronic heart failure of the European society of cardiology (ESC)Developed with the special contribution of the heart failure association (hFA) of the ESC. Eur Heart J. 2016;37(27):2129–200.
Brann A, Miller J, Eshraghian E, Park JJ, Greenberg B. Global longitudinal strain predicts clinical outcomes in patients with heart failure with preserved ejection fraction. Eur J Heart Fail. 2023;25(10):1755–65.
Curtis JP, Sokol SI, Wang Y, Rathore SS, Ko DT, Jadbabaie F, Portnay EL, Marshalko SJ, Radford MJ, Krumholz HM. The association of left ventricular ejection fraction, mortality, and cause of death in stable outpatients with heart failure. J Am Coll Cardiol. 2003;42(4):736–42.
Potter E, Marwick TH. Assessment of left ventricular function by echocardiography: the case for routinely adding global longitudinal strain to ejection fraction. JACC Cardiovasc Imaging. 2018;11(2 Pt 1):260–74.
Cikes M, Solomon SD. Beyond ejection fraction: an integrative approach for assessment of cardiac structure and function in heart failure. Eur Heart J. 2016;37(21):1642–50.
Hashemi D, Motzkus L, Blum M, Kraft R, Tanacli R, Tahirovic E, Doeblin P, Zieschang V, Zamani SM, Kelm M, et al. Myocardial deformation assessed among heart failure entities by cardiovascular magnetic resonance imaging. ESC Heart Fail. 2021;8(2):890–7.
Article PubMed PubMed Central Google Scholar
Marwick TH, Shah SJ, Thomas JD. myocardial strain in the assessment of patients with heart failure: a review. JAMA Cardiol. 2019;4(3):287–94.
Sengeløv M, Jørgensen PG, Jensen JS, Bruun NE, Olsen FJ, Fritz-Hansen T, Nochioka K, Biering-Sørensen T. Global longitudinal strain is a superior predictor of all-cause mortality in heart failure with reduced ejection fraction. JACC Cardiovasc Imaging. 2015;8(12):1351–9.
Tröbs SO, Prochaska JH, Schwuchow-Thonke S, Schulz A, Müller F, Heidorn MW, Göbel S, Diestelmeier S, Lerma Monteverde J, Lackner KJ, et al. Association of global longitudinal strain with clinical status and mortality in patients with chronic heart failure. JAMA Cardiol. 2021;6(4):448–56.
Stanton T, Leano R, Marwick TH. Prediction of all-cause mortality from global longitudinal speckle strain: comparison with ejection fraction and wall motion scoring. Circ Cardiovasc Imaging. 2009;2(5):356–64.
Zheng L, Li B, Lin S, Chen L, Li H. Role and mechanism of cardiac insulin resistance in occurrence of heart failure caused by myocardial hypertrophy. Aging (Albany NY). 2019;11(16):6584–90.
Article CAS PubMed Google Scholar
Wamil M, Coleman RL, Adler AI, McMurray JJV, Holman RR. Increased risk of incident heart failure and death is associated with insulin resistance in people with newly diagnosed type 2 diabetes: UKPDS 89. Diabetes Care. 2021;44(8):1877–84.
Erqou S, Adler AI, Challa AA, Fonarow GC, Echouffo-Tcheugui JB. Insulin resistance and incident heart failure: a meta-analysis. Eur J Heart Fail. 2022;24(6):1139–41.
Rudvik A, Månsson M. Evaluation of surrogate measures of insulin sensitivity - correlation with gold standard is not enough. BMC Med Res Methodol. 2018;18(1):64.
Antuna-Puente B, Disse E, Rabasa-Lhoret R, Laville M, Capeau J, Bastard JP. How can we measure insulin sensitivity/resistance? Diabetes Metab. 2011;37(3):179–88.
Abbasi F, Reaven GM. Comparison of two methods using plasma triglyceride concentration as a surrogate estimate of insulin action in nondiabetic subjects: triglycerides × glucose versus triglyceride/high-density lipoprotein cholesterol. Metabolism. 2011;60(12):1673–6.
Vasques AC, Novaes FS, de Oliveira Mda S, Souza JR, Yamanaka A, Pareja JC, Tambascia MA, Saad MJ, Geloneze B. TyG index performs better than HOMA in a Brazilian population: a hyperglycemic clamp validated study. Diabetes Res Clin Pract. 2011;93(3):e98–100.
Guerrero-Romero F, Simental-Mendía LE, González-Ortiz M, Martínez-Abundis E, Ramos-Zavala MG, Hernández-González SO, Jacques-Camarena O, Rodríguez-Morán M. The product of triglycerides and glucose, a simple measure of insulin sensitivity comparison with the euglycemic-hyperinsulinemic clamp. J Clin Endocrinol Metab. 2010;95(7):3347–51.
Huang R, Lin Y, Ye X, Zhong X, Xie P, Li M, Zhuang X, Liao X. Triglyceride-glucose index in the development of heart failure and left ventricular dysfunction: analysis of the ARIC study. Eur J Prev Cardiol. 2022;29(11):1531–41.
Chen Y, Fu J, Wang Y, Zhang Y, Shi M, Wang C, Li M, Wang L, Liu X, Ta S, et al. Association between triglyceride glucose index and subclinical left ventricular systolic dysfunction in patients with type 2 diabetes. Lipids Health Dis. 2023;22(1):35.
Article CAS PubMed PubMed Central Google Scholar
Li X, Chan JSK, Guan B, Peng S, Wu X, Lu X, Zhou J, Hui JMH, Lee YHA, Satti DI, et al. Triglyceride-glucose index and the risk of heart failure: evidence from two large cohorts and a mendelian randomization analysis. Cardiovasc Diabetol. 2022;21(1):229.
Huang R, Wang Z, Chen J, Bao X, Xu N, Guo S, Gu R, Wang W, Wei Z, Wang L. Prognostic value of triglyceride glucose (TyG) index in patients with acute decompensated heart failure. Cardiovasc Diabetol. 2022;21(1):88.
Xu L, Wu M, Chen S, Yang Y, Wang Y, Wu S, Tian Y. Triglyceride-glucose index associates with incident heart failure: a cohort study. Diabetes Metab. 2022;48(6).
Zhou Y, Wang C, Che H, Cheng L, Zhu D, Rao C, Zhong Q, Li Z, Wang X, Wu Z, et al. Association between the triglyceride-glucose index and the risk of mortality among patients with chronic heart failure: results from a retrospective cohort study in China. Cardiovasc Diabetol. 2023;22(1):171.
Na L, Cui W, Li X, Chang J, Xue X. Association between the triglyceride-glucose index and left ventricular global longitudinal strain in patients with coronary heart disease in Jilin Province, China: a cross-sectional study. Cardiovasc Diabetol. 2023;22(1):321.
Lang RM, Badano LP, Mor-Avi V, Afilalo J, Armstrong A, Ernande L, Flachskampf FA, Foster E, Goldstein SA, Kuznetsova T, et al. Recommendations for cardiac chamber quantification by echocardiography in adults: an update from the American society of echocardiography and the european association of cardiovascular imaging. Eur Heart J Cardiovasc Imaging. 2015;16(3):233–70.
McDonagh TA, Metra M, Adamo M, Gardner RS, Baumbach A, Böhm M, Burri H, Butler J, Čelutkienė J, Chioncel O, et al. 2021 ESC Guidelines for the diagnosis and treatment of acute and chronic heart failure. Eur Heart J. 2021;42(36):3599–726.
ElSayed NA, Aleppo G, Aroda VR, Bannuru RR, Brown FM, Bruemmer D, Collins BS, Hilliard ME, Isaacs D, Johnson EL, et al. 2 classification and diagnosis of diabetes: standards of care in diabetes-2023. Diabetes Care. 2023;46(Suppl 1):S19-s40.
Hong S, Han K, Park CY. The triglyceride glucose index is a simple and low-cost marker associated with atherosclerotic cardiovascular disease: a population-based study. BMC Med. 2020;18(1):361.
Wang X, Xu W, Song Q, Zhao Z, Meng X, Xia C, Xie Y, Yang C, Jin P, Wang F. Association between the triglyceride-glucose index and severity of coronary artery disease. Cardiovasc Diabetol. 2022;21(1):168.
Bikov A, Frent SM, Meszaros M, Kunos L, Mathioudakis AG, Negru AG, Gaita L, Mihaicuta S. Triglyceride-glucose index in non-diabetic, non-obese patients with obstructive sleep apnoea. J Clin Med. 2021;10(9):1932.
Ma YC, Zuo L, Chen JH, Luo Q, Yu XQ, Li Y, Xu JS, Huang SM, Wang LN, Huang W, et al. Modified glomerular filtration rate estimating equation for Chinese patients with chronic kidney disease. J Am Soc Nephrol. 2006;17(10):2937–44.
Park JJ, Park JB, Park JH, Cho GY. Global longitudinal strain to predict mortality in patients with acute heart failure. J Am Coll Cardiol. 2018;71(18):1947–57.
Skali H, Shah A, Gupta DK, Cheng S, Claggett B, Liu J, Bello N, Aguilar D, Vardeny O, Matsushita K, et al. Cardiac structure and function across the glycemic spectrum in elderly men and women free of prevalent heart disease: the atherosclerosis risk in the community study. Circ Heart Fail. 2015;8(3):448–54.
Ho JE, McCabe EL, Wang TJ, Larson MG, Levy D, Tsao C, Aragam J, Mitchell GF, Benjamin EJ, Vasan RS, et al. Cardiometabolic traits and systolic mechanics in the community. Circ Heart Fail. 2017. https://doi.org/10.1161/CIRCHEARTFAILURE.116.003536 .
Li C, Ford ES, McGuire LC, Mokdad AH. Association of metabolic syndrome and insulin resistance with congestive heart failure: findings from the third national health and nutrition examination survey. J Epidemiol Community Health. 2007;61(1):67–73.
Witteles RM, Fowler MB. Insulin-resistant cardiomyopathy clinical evidence, mechanisms, and treatment options. J Am Coll Cardiol. 2008;51(2):93–102.
Cai X, Liu X, Sun L, He Y, Zheng S, Zhang Y, Huang Y. Prediabetes and the risk of heart failure: a meta-analysis. Diabetes Obes Metab. 2021;23(8):1746–53.
Nishida K, Otsu K. Inflammation and metabolic cardiomyopathy. Cardiovasc Res. 2017;113(4):389–98.
Kolwicz SC Jr, Purohit S, Tian R. Cardiac metabolism and its interactions with contraction, growth, and survival of cardiomyocytes. Circ Res. 2013;113(5):603–16.
Samuelsson AM, Bollano E, Mobini R, Larsson BM, Omerovic E, Fu M, Waagstein F, Holmäng A. Hyperinsulinemia: effect on cardiac mass/function, angiotensin II receptor expression, and insulin signaling pathways. Am J Physiol Heart Circ Physiol. 2006;291(2):H787-796.
Zhou Q, Yang J, Tang H, Guo Z, Dong W, Wang Y, Meng X, Zhang K, Wang W, Shao C, et al. High triglyceride-glucose (TyG) index is associated with poor prognosis of heart failure with preserved ejection fraction. Cardiovasc Diabetol. 2023;22(1):263.
Tanaka H, Soga F, Tatsumi K, Mochizuki Y, Sano H, Toki H, Matsumoto K, Shite J, Takaoka H, Doi T, et al. Positive effect of dapagliflozin on left ventricular longitudinal function for type 2 diabetic mellitus patients with chronic heart failure. Cardiovasc Diabetol. 2020;19(1):6.
Nesti L, Pugliese NR, Sciuto P, Trico D, Dardano A, Baldi S, Pinnola S, Fabiani I, Di Bello V, Natali A. Effect of empagliflozin on left ventricular contractility and peak oxygen uptake in subjects with type 2 diabetes without heart disease: results of the EMPA-HEART trial. Cardiovasc Diabetol. 2022;21(1):181.
Palmiero G, Cesaro A, Galiero R, Loffredo G, Caturano A, Vetrano E, Rinaldi L, Salvatore T, Ruggiero R, Rosaria Di Palo M, et al. Impact of gliflozins on cardiac remodeling in patients with type 2 diabetes mellitus & reduced ejection fraction heart failure: a pilot prospective study. GLISCAR study Diabetes Res Clin Pract. 2023;2023(200).
Article Google Scholar
Cheng S, McCabe EL, Larson MG, Merz AA, Osypiuk E, Lehman BT, Stantchev P, Aragam J, Solomon SD, Benjamin EJ, et al. Distinct aspects of left ventricular mechanical function are differentially associated with cardiovascular outcomes and all-cause mortality in the community. J Am Heart Assoc. 2015;4(10).
Tai S, Fu L, Zhang N, Zhou Y, Xing Z, Wang Y. Impact of baseline and trajectory of triglyceride-glucose index on cardiovascular outcomes in patients With type 2 diabetes mellitus. Front Endocrinol. 2022;13.
Liao LP, Yang Y, Wu Y, Li W. Correlation analysis of the triglyceride glucose index and heart failure with preserved ejection fraction in essential hypertensive patients. Clin Cardiol. 2022;45(9):936–42.
Khalaji A, Behnoush AH, Khanmohammadi S, Ghanbari Mardasi K, Sharifkashani S, Sahebkar A, Vinciguerra C, Cannavo A. Triglyceride-glucose index and heart failure: a systematic review and meta-analysis. Cardiovasc Diabetol. 2023;22(1):244.
Download references
Acknowledgements
We are grateful to all the subjects who participated in the study.
This work was supported by the National Key Research and Development Program of China (No.2021YFF0501403 and No.2021YFF0501404).
Author information
Shuai Zhang and Yan Liu have contributed equally to this work.
Authors and Affiliations
State Key Laboratory for Innovation and Transformation of Luobing Theory, Jinan, China
Shuai Zhang, Yan Liu, Fangfang Liu, Qing Ye, Dachuan Guo, Panpan Xu, Tianhao Wei, Cheng Zhang & Huixia Lu
Key Laboratory of Cardiovascular Remodeling and Function Research, Chinese Ministry of Education, Chinese National Health Commission and Chinese Academy of Medical Sciences, Jinan, China
Department of Cardiology, Qilu Hospital of Shandong University, Jinan, China
You can also search for this author in PubMed Google Scholar
Contributions
SZ and YL drafted and revised the manuscript and contributed to the conception and design of this article. XP, QY, and FL contributed to the collection of cases and the organization of the database. SZ, TW and DG were responsible for the statistical analysis of the data. CZ, YL and HL interpreted the results. All authors read and approved the final manuscript.
Corresponding authors
Correspondence to Cheng Zhang or Huixia Lu .
Ethics declarations
Ethics approval and consent to participate.
This study adhered to the principles outlined in the Declaration of Helsinki and was approved by the Ethics Review Committee of Qilu Hospital of Shandong University.
Consent for publication
Not applicable.
Competing interests
The authors declare that they have no competing interests.
Additional information
Publisher's note.
Springer Nature remains neutral with regard to jurisdictional claims in published maps and institutional affiliations.
Supplementary Information
Below is the link to the electronic supplementary material.
12933_2024_2259_MOESM1_ESM.docx
Supplementary material 1. Figure S1 Comparison of GLS between high TyG index group and control group in three heart failure types groups. Figure S2 Scatterplot of GLS versus TyG index for three heart failure types. Figure S3 Restricted cubic spline plot between the TyG index level and reduced GLS for three heart failure types. Table S1 Co-linearity analysis between covariates. Table S2 Baseline characteristics of the study population according to the heart failure group. Table S3 Correlation between TyG index and cardiovascular risk factors in three heart failure types. Table S4 multivariable variable linear regression in three heart failure types. Table S5 Multivariable logistic regression analysis in three heart failure types. Table S6 Sensitivity analysis: exclusion of patient with DM, hypoglycemic or lipid-lowering drug use and SGLT-2i use in three heart failure types. Table S7 Subgroup analysis in three heart failure types.
Rights and permissions
Open Access This article is licensed under a Creative Commons Attribution 4.0 International License, which permits use, sharing, adaptation, distribution and reproduction in any medium or format, as long as you give appropriate credit to the original author(s) and the source, provide a link to the Creative Commons licence, and indicate if changes were made. The images or other third party material in this article are included in the article’s Creative Commons licence, unless indicated otherwise in a credit line to the material. If material is not included in the article’s Creative Commons licence and your intended use is not permitted by statutory regulation or exceeds the permitted use, you will need to obtain permission directly from the copyright holder. To view a copy of this licence, visit http://creativecommons.org/licenses/by/4.0/ . The Creative Commons Public Domain Dedication waiver ( http://creativecommons.org/publicdomain/zero/1.0/ ) applies to the data made available in this article, unless otherwise stated in a credit line to the data.
Reprints and permissions
About this article
Cite this article.
Zhang, S., Liu, Y., Liu, F. et al. Correlation between the triglyceride-glucose index and left ventricular global longitudinal strain in patients with chronic heart failure: a cross-sectional study. Cardiovasc Diabetol 23 , 182 (2024). https://doi.org/10.1186/s12933-024-02259-2
Download citation
Received : 25 March 2024
Accepted : 01 May 2024
Published : 29 May 2024
DOI : https://doi.org/10.1186/s12933-024-02259-2
Share this article
Anyone you share the following link with will be able to read this content:
Sorry, a shareable link is not currently available for this article.
Provided by the Springer Nature SharedIt content-sharing initiative
Cardiovascular Diabetology
ISSN: 1475-2840
- Submission enquiries: [email protected]
This paper is in the following e-collection/theme issue:
Published on 31.5.2024 in Vol 26 (2024)
Evaluation and Comparison of Academic Impact and Disruptive Innovation Level of Medical Journals: Bibliometric Analysis and Disruptive Evaluation
Authors of this article:

Original Paper
- Yuyan Jiang 1 , MPhil ;
- Xue-li Liu 1, 2 , BM ;
- Zixuan Zhang 1 , MPhil ;
- Xinru Yang 1 , MPhil
1 Henan Research Center for Science Journals, Xinxiang Medical University, Xinxiang, China
2 Faculty of Humanities & Social Sciences, Xinxiang Medical University, Xinxiang, China
Corresponding Author:
Xue-li Liu, BM
Faculty of Humanities & Social Sciences
Xinxiang Medical University
Library and Information Building, 2nd Fl.
No. 601, Jinsui Avenue, Hongqi District
Xinxiang, 450003
Phone: 86 1 383 736 0965
Email: [email protected]
Background: As an important platform for researchers to present their academic findings, medical journals have a close relationship between their evaluation orientation and the value orientation of their published research results. However, the differences between the academic impact and level of disruptive innovation of medical journals have not been examined by any study yet.
Objective: This study aims to compare the relationships and differences between the academic impact, disruptive innovation levels, and peer review results of medical journals and published research papers. We also analyzed the similarities and differences in the impact evaluations, disruptive innovations, and peer reviews for different types of medical research papers and the underlying reasons.
Methods: The general and internal medicine Science Citation Index Expanded (SCIE) journals in 2018 were chosen as the study object to explore the differences in the academic impact and level of disruptive innovation of medical journals based on the OpenCitations Index of PubMed open PMID-to-PMID citations (POCI) and H1Connect databases, respectively, and we compared them with the results of peer review.
Results: First, the correlation coefficients of the Journal Disruption Index (JDI) with the Journal Cumulative Citation for 5 years (JCC 5 ), Journal Impact Factor (JIF), and Journal Citation Indicator (JCI) were 0.677, 0.585, and 0.621, respectively. The correlation coefficient of the absolute disruption index (Dz) with the Cumulative Citation for 5 years (CC 5 ) was 0.635. However, the average difference in the disruptive innovation and academic influence rankings of journals reached 20 places (about 17.5%). The average difference in the disruptive innovation and influence rankings of research papers reached about 2700 places (about 17.7%). The differences reflect the essential difference between the two evaluation systems. Second, the top 7 journals selected based on JDI, JCC 5 , JIF, and JCI were the same, and all of them were H-journals. Although 8 (8/15, 53%), 96 (96/150, 64%), and 880 (880/1500, 58.67%) of the top 0.1%, top 1%, and top 10% papers selected based on Dz and CC 5 , respectively, were the same. Third, research papers with the “changes clinical practice” tag showed only moderate innovation (4.96) and impact (241.67) levels but had high levels of peer-reviewed recognition (6.00) and attention (2.83).
Conclusions: The results of the study show that research evaluation based on innovative indicators is detached from the traditional impact evaluation system. The 3 evaluation systems (impact evaluation, disruptive innovation evaluation, and peer review) only have high consistency for authoritative journals and top papers. Neither a single impact indicator nor an innovative indicator can directly reflect the impact of medical research for clinical practice. How to establish an integrated, comprehensive, scientific, and reasonable journal evaluation system to improve the existing evaluation system of medical journals still needs further research.
Introduction
Scientific and technical journals play a crucial role in showcasing research findings, and the value orientation of their published results is closely intertwined with their evaluation orientation. However, since Garfield [ 1 ] put forward the idea that “citation analysis can be used as an evaluation tool for journals” in 1972, the evaluation system of journals based on academic impact has become mainstream. However, relying too much on impact indicators for evaluation may hurt academic research and discipline progress.
On the one hand, some scholars have long pointed out that ranking journals according to their impact factors is noncomprehensive and may lead to misleading conclusions [ 2 , 3 ]. Meanwhile, many academic journals and publishers have engaged in strategic self-citation, leading to an overinflated journal impact factor (JIF) [ 4 ]. Some editorial behaviors to enhance the JIF clearly violate academic norms [ 5 ]. Some scholars are overciting each other’s work to enhance their academic impact [ 6 ]. External contingencies can have a devastating effect on citation indicators [ 7 ]. Scientists themselves also present a mixed attitude toward impact factors [ 8 ].
On the other hand, despite all the benefits of increased academic impact to journals, there is a nonnegligible problem in the evaluation of journals that citation indicators essentially characterize the impact of journals rather than their disruptive innovation [ 9 ]. Relevant studies have confirmed that the level of disruptive innovation of scientific research is getting increasingly lower [ 10 ] and the progress in various disciplines is slowing [ 11 ]. This is often overlooked against the background of impact-only evaluations. Therefore, despite the urgent need for disruptive innovations in science [ 12 ], impact-based journal rankings have made it more difficult to accept novel results [ 13 ], replacing the “taste of science” with the “taste of publication” [ 14 ] in the actual environment.
The evaluation of academic journals is about not only the journals themselves [ 15 ] but also the wide use of the evaluation results in academic review, promotion, and tenure decisions [ 16 ]. Meanwhile, the quality and results of medical research are directly related to human health and life and have a direct impact on human health and well-being. Therefore, the general and internal medicine journals indexed in Science Citation Index Expanded (SCIE) in 2018 were chosen as the study object. The OpenCitations Index of PubMed open PMID-to-PMID citations (POCI) and H1 Connect databases were selected as the sources for citation relationship data and peer review data. We investigated the connections and contrasts between the academic impact, disruptive innovation level, and results of peer review for medical journals and published research papers. We also analyzed the similarities and differences as well as the fundamental causes of the varying evaluation results in terms of impact evaluation, disruptive innovation, and peer review for various types of medical research papers. We aimed to provide a reference for the correct understanding of the innovation level of the results published by journals; the scientific and reasonable evaluation of medical journals; and the construction of a scientific, objective, and fair academic evaluation system.
Research Object
Because there is basically no disruptive innovation in the review literature, this paper only focuses on the general and internal medicine journals indexed in SCIE in 2018 and the research papers involved. In addition, considering the computational efficiency, accuracy, and difficulty of data acquisition, research papers for which citation relationships in the aforementioned journals were not included in POCI and the journals with too few references (less than 10) were excluded. Finally, 114 journals were retained at the journal level, and 15,206 research papers were retained at the paper level.
Data Resource
The data acquired in this study included journal information, literature information, citation relationship data, and peer review data. The data were obtained through the Journal Citation Reports (JCR), Web of Science (WoS), POCI, and H1 Connect databases.
Of these databases, POCI is a Resource Description Framework (RDF) data set containing details of all the citations from publications bearing PMIDs to other PMID-identified publications harvested from the National Institutes of Health Open Citations Collection. POCI covers more than 29 million bibliographic resources and more than 717 million citation links (as of the last update in January 2023). Citations in POCI are not considered simple links but as data entities. This means that it is permissible to assign descriptive attributes to each citation, such as the date of creation of the citation, its time span, and its type [ 17 ].
H1 Connect (formerly Faculty Opinions), the world’s most authoritative peer review database in the biomedical field, incorporates the combined efforts of more than 8000 international authoritative experts from around the globe and is a knowledge discovery tool used to evaluate published research. H1 Connect’s reviewers are authoritative experts in the life sciences and medical fields. They provide commentary, opinions, and validation of key papers in their own field. The quality and rigor of the reviewers mean that researchers can be assured of the quality of the papers they recommend, and H1 Connect brings these recommendations together to recommend high-quality research to a wider audience. H1 Connect’s experts typically evaluate the “high level” of research literature in the field within 2 months of publication, with over 90% of recommendations made within 6 months of publication.
Data Acquisition and Processing
The data acquired for this study consisted of 3 parts. The specific steps for data acquisition and processing were (1) data acquisition and (2) data processing.
Data Acquisition
The steps taken to acquire the data included the following: log in to JCR; select Medicine, General & Internal in the “Browse categories” page; select JCR Year=2018 and Citation Indexes=SCIE in the filters; export the result to XLS format; according to the acquired journal title, select the publication year as 2018 and the literature type as Article to search the WoS core database; and export the full record of the journal literature in XLS format. Finally, we downloaded the related H1 Connect literature data and POCI data according to the list of journals.
Data Processing
To process the data, we undertook the following steps: use Navicat to import the full record of research papers into the local SQLite database, process the downloaded POCI data, extract the PMID numbers of all the focus papers from the full record, retrieve the references and citations of the focus papers as well as the citations of the references of the focus papers in the local database transformed based on the POCI data, and establish the relevant data tables for the subsequent calculations.
Evaluation Indicators
Innovation indicators.
Some researchers have observed, at an early stage, that some technological innovations complete and improve current technologies without replacing them while others outright eliminate technologies that were used in the past. However, for a long time, scholars did not analyze and explain the essence of this phenomenon. It was not until 1986 that Tushman and Anderson [ 18 ] summarized the phenomenon as follows: There are 2 types of major technological shifts that disrupt or enhance the capabilities of existing firms in an industry. However, Christensen [ 19 ], a professor at Harvard Business School in the United States, argued that disruptive innovations are new technologies that replace existing mainstream technologies in unexpected ways. Building on these views, Funk and Owen-Smith [ 20 ] provided deeper and more insightful insights. They argued that the dichotomy between disruptive and augmentative technologies lacks nuance and that the impact of new technologies on the status quo is a matter of degree rather than absolute impact.
In this regard, Govindarajan and Kopalle [ 21 ] also pointed out that disruptive innovation lacks reliable and valid measurement standards. Therefore, Funk and Owen-Smith [ 20 ] created the consolidation-disruption (CD) index, which aims to quantify the degree of technological change brought about by new patents. The index drew the attention of Wu et al [ 22 ], who analogized the basic principle of the CD index to measure disruption by calculating the citation substitution of the focus paper in the citation network and who were the first to apply the evaluation of disruptive innovation to the world of bibliometrics.
As an important carrier of academic results, it is important to evaluate papers quantitatively, rationally, and efficiently in terms of their innovation [ 23 ]. The disruption (D) index has received widespread attention after it was proposed by Wu et al [ 22 ]. A subset of scholars then explored disruptive papers in specific subject areas, including scientometrics [ 24 ], craniofacial surgery [ 25 ], pediatric surgery [ 26 ], synthetic biology [ 27 ], energy security [ 28 ], colorectal surgery [ 29 ], otolaryngology [ 30 ], military trauma [ 31 ], breast cancer [ 32 ], radiology [ 33 ], ophthalmology [ 34 ], plastic surgery [ 35 ], urology [ 36 ], and general surgery [ 37 ], based on the D index. Park et al [ 10 ] also analyzed the annual dynamics of the disruption level of papers and patents across the subject areas.
Another group of scholars conducted in-depth research on the index itself: Bornmann et al [ 38 ] explored the convergent validity of the index and the variants that may enhance the effectiveness of the measure and tested the validity of the D index based on the literature in the field of physics [ 39 ]. Ruan et al [ 40 ] provided an in-depth reflection on the limitations of the application of the D index as a measure of scientific and technological progress. Liu et al [ 41 , 42 ] empirically investigated the stabilization time window of the D index in different subject areas and addressed the mathematical inconsistency of the traditional D index and proposed an absolute disruption index (Dz; as in Equation 2) [ 43 ].
This series of studies has made it possible to evaluate the disruption of research papers based on the D index, which has gradually matured. On this basis, Jiang and Liu [ 44 ] proposed the Journal Disruption Index (JDI) to evaluate the disruptive innovation level of journals (as in Equation 3) and validated the evaluation effect of this indicator based on Chinese SCIE journals [ 45 ].
In Equations 1, 2, and 3, N F refers to the literature that only cites the focus paper (FP), N B refers to the literature that cites both the focus paper and at least one reference (R) of the focus paper, and N R refers to the literature that only cites at least one reference (R) of the focus paper but not the focus paper. n is the number of “Article” type pieces of literature contained in the journal, and Dz i is the Dz of the i th article in the journal.
In this study, Dz and JDI were chosen to evaluate the disruption of the selected studies at the literature and journal levels, respectively. Bornmann and Tekles [ 46 ] argued that 3 years is necessary regardless of the measurement for that discipline. Considering that, in the determination of the stabilization time window of the D index for each discipline conducted by Liu et al [ 42 ], the stabilization time window for clinical medicine is 4 years after publication. Therefore, in the process of calculating Dz in this paper, the citation time window of the focus papers was set to 2018 to 2022 to ensure the validity of the results. In addition, because the JDI value was too small, we multiplied the JDI by 1000 in all subsequent presentations.
Peer Review Indicators
The peer review indicators selected for this study included the peer review score (PScore), weighted peer review stars (PStar_w), and weighted peer review evaluation times (PTime_w). In this case, the weighted indicators refer to the weighting of ratings and number of evaluations by the number of evaluators when an evaluation was completed by more than one reviewer.
The advantage of using peer review indicators is that it can make up for the lag and one-sidedness of relying solely on the citations among the literature to assess the quality of the literature. It also corrects the shortcomings of the traditional JIF to judge the quality of the literature. Compared with a single impact indicator, it is more scientific.
Impact Indicators
The impact indicators selected for this study included the Cumulative Citation for 5 years (CC 5 ), JIF, Journal Citation Indicator (JCI), and Journal Cumulative Citation for 5 years (JCC 5 ). Among these, JIF is the total number of citations for scholarly articles published in the journal in the past 2 years divided by the total number of citable articles. JCI is the average Category Normalized Citation Impact (CNCI) of the citable literature published in the specific journal in the previous 3 years. The JCC 5 is obtained by dividing the sum of the citation frequencies recorded in the POCI repository of the corresponding research papers (focus papers) of the selected journals for the years 2018 to 2022 by the number of research papers published by the journal (as in Equation 4).
In Equation 4, a i C t represents the number of citations of the i th research paper of the journal in year t, and n is the number of research papers published by the journal.
Evaluation and Correlation Analyses of Journals Under Different Evaluation Perspectives
Analysis of differences in academic influence and level of disruptive innovation of journals.
The academic impact and level of disruptive innovation of the selected 114 journals, the results of the correlation analyses of the indicators, and the differences in the rankings are shown in Table 1 , Table 2 , and Figure 1 , respectively. From these, we can see that (1) journals that are at the top of the ranking of impact indicators are usually also ranked at the top in the ranking of disruptive innovation, (2) journals at the bottom of the impact rankings are usually at the bottom of the innovation rankings, and (3) there is little difference in the ranking results of journals under different impact indicators, but there is a big difference in the ranking results of influence and disruptive innovation. Although there are moderate correlations between the JDI of journals and the 3 influence indicators of JIF, JCI, and JCC 5 , the average difference between the disruptive innovation ranking and academic influence ranking of the journals included in the study reached 20 places.
a JCC 5 : Journal Cumulative Citation for 5 years.
b JDI: Journal Disruption Index.
c JIF: Journal Impact Factor.
d JCI: Journal Citation Indicator.
a JDI: Journal Disruption Index.
b JCC 5 : Journal Cumulative Citation for 5 years.
e Not applicable.

Analysis of the Differences Between the Journals’ Impact and Disruption and the Results of Peer Review
In order to better analyze the differences between the academic impact and level of disruptive innovation with peer-reviewed results of journals in the field of general and internal medicine, papers indexed in H1 Connect were referred to as “H-papers,” and the source journals of “H-papers” are referred to as “H-journals.” The evaluation indicators, ranking of H-journals, and the percentage of H-papers are shown in Table 3 and Table 4 . We can see that (1) the top 7 journals in terms of both academic impact and disruptive innovation are all H-journals, (2) the average impact ranking of H-journals is higher than the average innovation ranking, and (3) some journals with low impact and innovation also became H-journals.
a JPScore: journal peer review score.
b JPTime_w: weighted journal peer review evaluation time.
c JFPStar_w: weighted journal peer review star.
b JIF: Journal Impact Factor.
c JCI: Journal Citation Indicator.
Evaluation and Correlation Analyses of Papers Under Different Evaluation Perspectives
Analysis of differences in academic impact and level of disruptive innovation of papers.
Ideally, if an article is accepted by a specific journal, it is because its overall quality is similar to other papers previously published in that journal [ 47 ]. However, journal-level indicators are, at best, only moderately suggestive of the quality of an article [ 48 ], which makes indicators that measure specific articles more popular [ 49 ]. Therefore, in this study, research papers in the field of general and internal medicine were also evaluated in terms of their academic impact and level of disruptive innovation, and the results are shown in Figure 2 . From the results, we can see that research papers that rank high in the impact ranking usually also rank high in the disruptive innovation ranking. There were 8 (8/15, 53%), 96 (96/150, 64%), and 880 (880/1500, 58.67%) of the Top 0.1%, Top 1%, and Top 10% papers, respectively, selected based on the Dz and CC 5 that were the same. Second, the level of disruptive innovation of research papers with the same level of impact varied greatly, and the impact level of research papers with the same level of disruptive innovation also varied greatly. Third, despite the high correlation ( r =0.635, P <.001) between the Dz and CC 5 of the selected research papers, the average difference between their innovation and impact rankings reached about 2700. Fourth, the actual analysis results showed no correlation between the innovation of the selected research paper and the number of references, which indicates that the Dz index is basically unaffected by the difference in the number of references in the actual evaluation process ( r =0.006, P =.43).

Analysis of Differences Between Papers’ Impact and Disruption and the Results of Peer Review
In order to better analyze the differences between the academic impact, level of disruptive innovation, and peer review results of journal research papers in the field of general and internal medicine, the differences between the academic impact and disruptive innovation level and the peer review results of H-papers were analyzed. The specific results are shown in Table 5 and Figure 3 . From the results, we see that there were 8 (8/15, 53%), 65 (65/150, 43.3%), and 187 (187/1500, 12.47%) H-papers among the top 0.1%, top 1%, and top 10% papers, respectively, selected based on Dz. There were 5 (5/15, 33%), 74 (74/150, 49.3%), and 220 (220/1500, 14.67%) H-papers among the top 0.1%, top 1%, and top 10% papers, respectively, selected based on CC 5 . Second, there was a significant positive correlation between the peer review indicators, disruptive innovation indicators, and academic impact indicators of H-papers, reflecting the consistency between the quantitative evaluation and peer review at the overall level. Third, the average impact ranking of H-papers was 865 (top 5.68%), and the average disruptive innovation ranking was 1726 (top 11.35%), which means that the average impact ranking of H-papers was higher than the average disruptive innovation ranking. Fourth, some papers with low academic impact and disruptive innovation level also became H-papers. Fifth, compared with the CC 5 , Dz has a minor correlation advantage with PTime_w and PStar_w.
a CC 5 : Cumulative Citation for 5 years.
b Dz: absolute disruption index.
c PTime_w: weighted peer review evaluation times.
d PStar_w: weighted peer review stars.
e PScore: peer review score.
f Not applicable.

In addition to rating and commenting on the included research papers, different labels are added by the reviewers of articles in H1 Connect according to their different internal characteristics. The relevant definitions are shown in Table 6 (refer to Du et al [ 50 ]). We categorized and counted the 257 H-papers (in this study, if a paper had more than 1 tag, it was counted separately in the calculation of each tag), and the results are shown in Table 7 . From this, we can see that (1) research papers with the tags in the “Novel Drug Target,” “Technical Advance,” and “New Finding” categories had a high academic impact and a high disruptive innovation level; (2) research papers with the “Changes Clinical Practice” tag showed only a moderate academic impact and disruptive innovation level but had high levels of peer-reviewed recognition and attention; (3) experts showed higher recognition and concern for research papers with tags of “Negative/Null Result,” “Controversial,” “Refutation,” and “Interesting Hypothesis,” but their academic impact and disruptive innovation level were lower than others.
c PScore: peer review score.
e PTime_w: weighted peer review evaluation times.
Principal Findings
Evaluation of disruptive innovation is detached from the traditional evaluation system.
From these research results, large differences were seen between the innovation and impact rankings of the journals and research papers included in the study. This is also consistent with the findings of Guo and Zhou [ 51 ]. This phenomenon reflects the essential difference between the 2 different evaluation systems. It also proves that the evaluation of the disruptive innovation of research papers and journals based on Dz and JDI is not consistent with the traditional evaluation system of impact.
The essence of disruptive evaluation is to measure the innovation from the substitution level of knowledge structure. This evaluation method brings new ideas to the field of scientific research evaluation, helps relevant institutions and scholars remove the constraints of the traditional evaluation system, and helps to establish a value orientation of encouraging innovation for scientific research and scientific and technological journals, so as to promote the benign development of the academic ecology.
The 3 Evaluation Systems Only Have High Consensus on the Top Object
Although, given the consistency of scientific evaluation, there will be some uniformity in the level of disruptive innovation, level of academic impact, and peer review results of journals as well as research papers.
However, from the research results presented in this paper, we can see that (1) the top 7 journals in terms of both academic impact and disruptive innovation were all H-journals, (2) more than one-half of the top papers selected based on Dz and CC 5 were the same, (3) the average H-papers were ranked at the top in terms of impact and innovation, and (4) the results of the different evaluation systems only had high consensus on the authoritative journals and top papers in the field.
These findings are also consistent with those of Goman [ 52 ] and Chi et al [ 53 ]. A fundamental reason for this phenomenon is that the purposes of the 3 evaluation systems are inherently different. Therefore, in the actual evaluation process, the 3 kinds of indicators are not interchangeable, and the combination of the 3 evaluation systems may be a feasible solution to establish a comprehensive, scientific, and reasonable journal evaluation system.
A Single Indicator Cannot Accurately Reflect the Impact of Medical Research on Clinical Practice Alone
From the aforementioned findings, we can see that research papers of the “Novel Drug Target,” “ Technical Advance,” and “New Finding” types have high academic impact and high levels of innovation, which is in line with their classification definitions. This is also consistent with the findings of Du et al [ 50 ], Thelwall et al [ 54 ], and Jiang and Liu [ 55 ]. Second, research papers of the “Changes Clinical Practice” type showed only moderate levels of innovation and impact but had high levels of peer-reviewed recognition and attention. This reflects the difficulty of evaluating the impact of a particular academic paper on clinical practice, whether the evaluation system is based on academic impact or level of disruptive innovation. Third, peer-reviewed experts show higher recognition and concern for research papers of the types “Negative/Null Result,” “Controversial,” “Refutation,” and “Interesting Hypothesis,” but the level of impact and disruptive innovation of these papers are lower. This partly reflects the current academic community’s excessive focus on positive results; deliberate avoidance of negative results; and overall lack of support for debatable, falsified, and other types of research.
Several scholars have recently suggested that the evaluation system of medical journals should be redesigned for contemporary clinical impact and utility [ 56 ]. In this regard, Thelwall and Maflahi [ 57 ] advocated that the references of a guideline are an important basis for studying clinical value. However, Traylor and Herrmann-Lingen [ 58 ] found only a weak correlation between the number of citations to individual journals in the guidelines and their respective JIF. Therefore, the JIF is not a suitable tool for assessing the clinical relevance of medical research. The results of this study similarly found that research papers of the “Changes Clinical Practice” type showed only moderate levels of disruptive innovation and academic impact, but such research papers received higher recognition and attention from peer review experts. Therefore, combining quantitative evaluation with peer review may be a feasible way to measure the impact of medical research on clinical practice.
Limitations
However, this study also has the following limitations. First, papers in the medical field have a preference for citing review articles [ 59 ], which has a certain impact on the evaluation of the disruptive innovation of research papers. Second, the scoring mechanism provided to its reviewers by H1 Connect has a low differentiation degree and cannot perfectly distinguish the differences in quality between papers yet. In addition, H1 Connect has too few evaluators. Brezis and Birukou [ 60 ] illustrated that, if the number of reviewers is increased to about 10, the correlation between the results and the quality of the paper will be significantly improved. However, it is difficult to seek so many high-quality experts who are willing to accept open peer review in the high pressure environment of “Publish or Perish” [ 61 ]. Third, since the citation data sources used in this study are all based on PubMed data, this study also suffers from the problem of missing references and citations that are not labeled with a PMID, which affects the accuracy of the evaluation results to a certain extent. In future studies, we will obtain more accurate measurement results by jointly using multiple sources of citation data.
Conclusions
In this study, the general and internal medicine journals indexed in SCIE in 2018 were chosen as the study object. The POCI and H1 Connect databases were selected as sources of citation relationship data and peer review data. We investigated the connections and contrasts between the academic impact, level of disruptive innovation, and results of peer review for medical journals and published research papers. We also analyzed the similarities and differences as well as the fundamental causes of the varying evaluation results in terms of impact evaluation, disruptive innovation, and peer review for various types of medical research papers.
The results of this study show that the evaluation of scientific research based on the innovation index is detached from the traditional impact evaluation system, the 3 evaluation systems only have high consistency for authoritative journals and top papers, and neither the single impact index nor the innovation index can directly reflect the impact of medical research on clinical practice.
In addition, with the increasing importance of replicative science, the accuracy of statistical reports, evidential value of reported data, and replicability of given experimental results [ 62 ] should also be included in the examination of journal quality. How to establish a comprehensive, all-encompassing, scientific, and reasonable journal evaluation system needs to be further investigated.
Acknowledgments
XL was supported by the National Social Science Foundation of China (23BTQ085), Major Project of Basic Research on Philosophy and Social Sciences in Henan Universities (2024-JCZD-23).
Data Availability
All citation data can be obtained from [ 63 ].
Authors' Contributions
XL conceptualized the study, reviewed and edited the manuscript, and supervised the study. YJ was responsible for the methodology, provided the software and other resources, performed the formal analysis, and wrote the original manuscript draft. YJ and XL acquired funding. YJ, XY, and ZZ curated the data. XY and ZZ also contributed to the study investigation.
Conflicts of Interest
None declared.
- Garfield E. Citation analysis as a tool in journal evaluation. Science. Nov 03, 1972;178(4060):471-479. [ CrossRef ] [ Medline ]
- Hansson S. Impact factor as a misleading tool in evaluation of medical journals. Lancet. Sep 30, 1995;346(8979):906. [ CrossRef ] [ Medline ]
- Metze K, Borges da Silva FA. Ranking of journals by journal impact factors is not exact and may provoke misleading conclusions. J Clin Pathol. May 11, 2022;75(10):649-650. [ CrossRef ] [ Medline ]
- Siler K, Larivière V. Who games metrics and rankings? Institutional niches and journal impact factor inflation. Research Policy. Dec 2022;51(10):104608. [ CrossRef ]
- Martin BR. Editors’ JIF-boosting stratagems – Which are appropriate and which not? Research Policy. Feb 2016;45(1):1-7. [ CrossRef ]
- Zaidi SJA, Taqi M. Citation cartels in medical and dental journals. J Coll Physicians Surg Pak. Jun 01, 2023;33(6):700-701. [ CrossRef ] [ Medline ]
- Fassin Y. Research on Covid-19: a disruptive phenomenon for bibliometrics. Scientometrics. May 07, 2021;126(6):5305-5319. [ FREE Full text ] [ CrossRef ] [ Medline ]
- Buela-Casal G, Zych I. What do the scientists think about the impact factor? Scientometrics. Feb 22, 2012;92(2):281-292. [ CrossRef ]
- Thelwall M, Kousha K, Stuart E, Makita M, Abdoli M, Wilson P, et al. In which fields are citations indicators of research quality? Asso for Info Science & Tech. May 04, 2023;74(8):941-953. [ CrossRef ]
- Park M, Leahey E, Funk R. Papers and patents are becoming less disruptive over time. Nature. Jan 2023;613(7942):138-144. [ CrossRef ] [ Medline ]
- Chu JSG, Evans JA. Slowed canonical progress in large fields of science. Proc Natl Acad Sci U S A. Oct 12, 2021;118(41):1. [ FREE Full text ] [ CrossRef ] [ Medline ]
- Schattner U, Shatner M. Science desperately needs disruptive innovation. Qeios. Jun 07, 2023:1. [ CrossRef ]
- Liang Z, Mao J, Li G. Bias against scientific novelty: A prepublication perspective. Asso for Info Science & Tech. Nov 19, 2022;74(1):99-114. [ CrossRef ]
- Osterloh M, Frey BS. Ranking games. Eval Rev. Aug 04, 2014;39(1):102-129. [ CrossRef ]
- Kulczycki E, Huang Y, Zuccala AA, Engels TCE, Ferrara A, Guns R, et al. Uses of the Journal Impact Factor in national journal rankings in China and Europe. Asso for Info Science & Tech. Aug 13, 2022;73(12):1741-1754. [ CrossRef ]
- McKiernan E, Schimanski L, Muñoz Nieves C, Matthias L, Niles M, Alperin J. Use of the Journal Impact Factor in academic review, promotion, and tenure evaluations. Elife. Jul 31, 2019;8:8. [ FREE Full text ] [ CrossRef ] [ Medline ]
- Heibi I, Peroni S, Shotton D. Software review: COCI, the OpenCitations Index of Crossref open DOI-to-DOI citations. Scientometrics. Sep 14, 2019;121(2):1213-1228. [ CrossRef ]
- Tushman ML, Anderson P. Technological discontinuities and organizational environments. Administrative Science Quarterly. Sep 1986;31(3):439. [ CrossRef ]
- Christensen CM. The Innovator's Dilemma: When New Technologies Cause Great Firms to Fail (Management of Innovation and Change). Boston, MA. Harvard Business Review Press; 1997.
- Funk RJ, Owen-Smith J. A dynamic network measure of technological change. Management Science. Mar 2017;63(3):791-817. [ CrossRef ]
- Govindarajan V, Kopalle PK. Disruptiveness of innovations: measurement and an assessment of reliability and validity. Strategic Management Journal. Dec 22, 2005;27(2):189-199. [ CrossRef ]
- Wu L, Wang D, Evans JA. Large teams develop and small teams disrupt science and technology. Nature. Feb 13, 2019;566(7744):378-382. [ CrossRef ] [ Medline ]
- Kostoff RN, Boylan R, Simons GR. Disruptive technology roadmaps. Technological Forecasting and Social Change. Jan 2004;71(1-2):141-159. [ CrossRef ]
- Bornmann L, Devarakonda S, Tekles A, Chacko G. Disruptive papers published in Scientometrics: meaningful results by using an improved variant of the disruption index originally proposed by Wu, Wang, and Evans (2019). Scientometrics. Mar 14, 2020;123(2):1149-1155. [ CrossRef ]
- Horen S, Hansdorfer M, Kronshtal R, Dorafshar A, Becerra A. The most disruptive publications in craniofacial surgery (1954-2014). J Craniofac Surg. Oct 01, 2021;32(7):2426-2430. [ CrossRef ] [ Medline ]
- Sullivan GA, Skertich NJ, Gulack BC, Becerra AZ, Shah AN. Shifting paradigms: The top 100 most disruptive papers in core pediatric surgery journals. J Pediatr Surg. Aug 2021;56(8):1263-1274. [ CrossRef ] [ Medline ]
- Meyer C, Nakamura Y, Rasor BJ, Karim AS, Jewett MC, Tan C. Analysis of the innovation trend in cell-free synthetic biology. Life (Basel). Jun 11, 2021;11(6):551. [ FREE Full text ] [ CrossRef ] [ Medline ]
- Jiang Y, Liu X. A bibliometric analysis and disruptive innovation evaluation for the field of energy security. Sustainability. Jan 05, 2023;15(2):969. [ CrossRef ]
- Becerra A, Grimes C, Grunvald M, Underhill J, Bhama A, Govekar H, et al. A new bibliometric index: the top 100 most disruptive and developmental publications in colorectal surgery journals. Dis Colon Rectum. Mar 01, 2022;65(3):429-443. [ CrossRef ] [ Medline ]
- Sheth AH, Hengartner A, Abdou H, Salehi PP, Becerra AZ, Lerner MZ. Disruption index in otolaryngology: uncovering a bibliometric history of a rapidly evolving field. Laryngoscope. Feb 19, 2024;134(2):629-636. [ CrossRef ] [ Medline ]
- Dilday J, Gallagher S, Bram R, Williams E, Grigorian A, Matsushima K, et al. Citation versus disruption in the military: Analysis of the top disruptive military trauma research publications. J Trauma Acute Care Surg. May 15, 2023;95(2S):S157-S169. [ CrossRef ]
- Grunvald M, Williams M, Rao R, O’Donoghue C, Becerra A. 100 disruptive publications in breast cancer research. Asian Pac J Cancer Prev. Aug 01, 2021;22(8):2385-2389. [ CrossRef ]
- Abu-Omar A, Kennedy P, Yakub M, Robbins J, Yassin A, Verma N, et al. Extra credit for disruption: trend of disruption in radiology academic journals. Clin Radiol. Dec 2022;77(12):893-901. [ CrossRef ] [ Medline ]
- Patel PA, Javed Ali M. Characterizing innovation in science through the disruption index. Semin Ophthalmol. Aug 18, 2022;37(6):790-791. [ CrossRef ] [ Medline ]
- Neubauer DC, Blum JD, Labou SG, Heskett KM, Calvo RY, Reid CM, et al. Using the disruptive score to identify publications that changed plastic surgery practice. Ann Plast Surg. 2022;88(4):S385-S390. [ CrossRef ]
- Khusid JA, Gupta M, Sadiq AS, Atallah WM, Becerra AZ. Changing the status quo: the 100 most-disruptive papers in urology? Urology. Jul 2021;153:56-68. [ CrossRef ] [ Medline ]
- Williams MD, Grunvald MW, Skertich NJ, Hayden DM, O'Donoghue C, Torquati A, et al. Disruption in general surgery: Randomized controlled trials and changing paradigms. Surgery. Dec 2021;170(6):1862-1866. [ CrossRef ] [ Medline ]
- Bornmann L, Devarakonda S, Tekles A, Chacko G. Are disruption index indicators convergently valid? The comparison of several indicator variants with assessments by peers. Quantitative Science Studies. Aug 2020;1(3):1242-1259. [ CrossRef ]
- Bu Y, Waltman L, Huang Y. A multidimensional framework for characterizing the citation impact of scientific publications. Quantitative Science Studies. 2021;2(1):155-183. [ CrossRef ]
- Ruan X, Lyu D, Gong K, Cheng Y, Li J. Rethinking the disruption index as a measure of scientific and technological advances. Technological Forecasting and Social Change. Nov 2021;172:121071. [ CrossRef ]
- Liu X, Shen Z, Liao Y, Yang L. The research about the improved disruption index and its influencing factors. Library and Information Service. 2020;64(24):84-91. [ CrossRef ]
- Liu X, Shen Z, Liao Y, Zhu M, Yang L. Research on the stable time window of disruption index. Library and Information Service. 2021;65(18):49-57. [ CrossRef ]
- Liu X, Liao Y, Zhu M. A preliminary study on the application of disruption index to scientific research evaluation. Information Studies: Theory & Application. 2021;44(12):34-40. [ CrossRef ]
- Jiang Y, Liu X. A construction and empirical research of the journal disruption index based on open citation data. Scientometrics. May 18, 2023;128(7):3935-3958. [ FREE Full text ] [ CrossRef ] [ Medline ]
- Jiang Y, Wang L, Liu X. Innovative evaluation of Chinese SCIE-indexed scientific journals: An empirical study based on the disruption index. Chinese Journal of Scientific and Technical Periodicals. 2023;34(8):1060-1068. [ CrossRef ]
- Bornmann L, Tekles A. Disruption index depends on length of citation window. EPI. Mar 25, 2019;28(2):1. [ CrossRef ]
- Migheli M, Ramello GB. The unbearable lightness of scientometric indices. Manage Decis Econ. Nov 04, 2021;42(8):1933-1944. [ CrossRef ]
- Thelwall M, Kousha K, Makita M, Abdoli M, Stuart E, Wilson P, et al. In which fields do higher impact journals publish higher quality articles? Scientometrics. May 18, 2023;128(7):3915-3933. [ CrossRef ]
- Iyengar KP, Vaishya R. Article-level metrics: A new approach to quantify reach and impact of published research. J Orthop. Jun 2023;40:83-86. [ CrossRef ] [ Medline ]
- Du J, Tang X, Wu Y. The effects of research level and article type on the differences between citation metrics and 1000 recommendations. Asso for Info Science & Tech. Jun 2015;67(12):3008-3021. [ CrossRef ]
- Guo L, Zhou Q. Research on characteristics of disruptive papers and their correlation with traditional bibliometric indicators. Journal of Intelligence. 2021;40(1):183-207. [ CrossRef ]
- Gorman DM, Huber C. Ranking of addiction journals in eight widely used impact metrics. J Behav Addict. Jul 13, 2022;11(2):348-360. [ FREE Full text ] [ CrossRef ] [ Medline ]
- Chi P, Ding J, Leng F. Characteristics of the technology impact of breakthrough papers in biology and medicine. Journal of the China Society for Scientific and Technical Information. 2022;41(7):675. [ CrossRef ]
- Thelwall M, Kousha K, Abdoli M. Is medical research informing professional practice more highly cited? Evidence from AHFS DI Essentials in drugs.com. Scientometrics. Feb 21, 2017;112(1):509-527. [ CrossRef ]
- Jiang Y, Liu X. The relationship between absolute disruption index, peer review index and CNCI: a study based on virology papers. Library and Information Service. 2023;67(3):96-105. [ CrossRef ]
- Klein-Fedyshin M, Ketchum A. PubMed's core clinical journals filter: redesigned for contemporary clinical impact and utility. J Med Libr Assoc. Jul 10, 2023;111(3):665-676. [ FREE Full text ] [ CrossRef ] [ Medline ]
- Thelwall M, Maflahi N. Guideline references and academic citations as evidence of the clinical value of health research. Asso for Info Science & Tech. Mar 17, 2015;67(4):960-966. [ CrossRef ]
- Traylor C, Herrmann-Lingen C. Does the journal impact factor reflect the impact of German medical guideline contributions? Scientometrics. Feb 03, 2023;128(3):1951-1962. [ CrossRef ]
- Marks MS, Marsh MC, Schroer TA, Stevens TH. An alarming trend within the biological/biomedical research literature toward the citation of review articles rather than the primary research papers. Traffic. Jan 19, 2013;14(1):1-1. [ FREE Full text ] [ CrossRef ] [ Medline ]
- Brezis ES, Birukou A. Arbitrariness in the peer review process. Scientometrics. Feb 03, 2020;123(1):393-411. [ CrossRef ]
- Jiang Y, Liu X. Open peer review: Mode, technology, problems, and countermeasures. Chinese Journal of Scientific and Technical Periodicals. 2022;33(9):1196-1205. [ CrossRef ]
- Dougherty MR, Horne Z. Citation counts and journal impact factors do not capture some indicators of research quality in the behavioural and brain sciences. R Soc Open Sci. Aug 17, 2022;9(8):220334. [ FREE Full text ] [ CrossRef ] [ Medline ]
- COCI CSV dataset of all the citation data. Figshare. Feb 07, 2023. URL: https://figshare.com/articles/dataset/Crossref_Open_Citation_Index_CSV_dataset_of_all_the_citation_data/6741422?file=37483791 [accessed 2024-05-24]
Abbreviations
Edited by S Ma; submitted 04.12.23; peer-reviewed by O Beiki, C Oo; comments to author 28.02.24; revised version received 16.04.24; accepted 29.04.24; published 31.05.24.
©Yuyan Jiang, Xue-li Liu, Zixuan Zhang, Xinru Yang. Originally published in the Journal of Medical Internet Research (https://www.jmir.org), 31.05.2024.
This is an open-access article distributed under the terms of the Creative Commons Attribution License (https://creativecommons.org/licenses/by/4.0/), which permits unrestricted use, distribution, and reproduction in any medium, provided the original work, first published in the Journal of Medical Internet Research, is properly cited. The complete bibliographic information, a link to the original publication on https://www.jmir.org/, as well as this copyright and license information must be included.
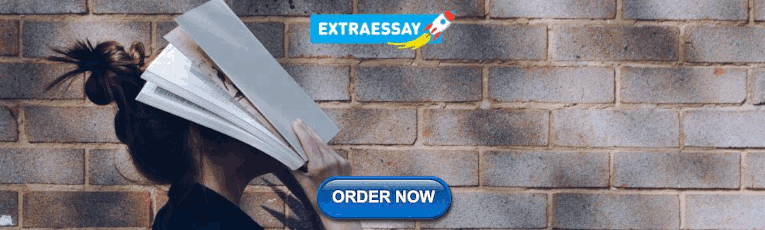
IMAGES
VIDEO
COMMENTS
Correlation is a measure of a monotonic association. between 2 variables. A monotonic relationship between 2. variables is a one in which either (1) as the value of 1 vari. able increases, so does ...
correlational designs. We begin this chapter with an introduction to the research design that was illustrated here: the survey research design. 8.1 An Overview of Survey Designs A nonexperimental research design used to describe an individual or a group by having participants complete a survey or questionnaire is called the survey research design.
This chapter reviews several of the more common applications of correlation statistics. It examines the Pearson product-moment correlation (r) and basic regression analysis. Although these are not the only statistical tools for analyzing the relationships among variables, they are among the most frequently used analytical techniques in the ...
Research Question Is there a correlation between how long it takes for a student to complete an exam and the grade on that exam? ... Notice that in correlation analysis, you can mix apples and oranges; time is a measure of minutes, whereas grade is a measure of academic performance. The only constraints
gression, lends itself to answering different research questions. Using different perspectives on regression will show us the generality of the technique, which will help us solve new types of data analysis problems that we may encounter in our research. 1.Describing bivariate data. The bivariate normal distribution generalizes the normal ...
1. Correlations. In many areas of empirical science, one of the first steps to assess observed data is to identify and. interpret patterns in these data. This may inductively lead to the ...
Revised on June 22, 2023. A correlational research design investigates relationships between variables without the researcher controlling or manipulating any of them. A correlation reflects the strength and/or direction of the relationship between two (or more) variables. The direction of a correlation can be either positive or negative.
Proper Interpretation of Correlation. Correlational analyses have been reported as one of the most common analytic techniques in research at the beginning of the 21 st century, particularly in health and epidemiological research. 15 Thus effective and proper interpretation is critical to understanding the literature.
Its goal is to be lightweight, easy to use, and allows for the computation of many diferent kinds of correlations, such as: Pearson's correlation: This is the most common correlation method. It corresponds to the covariance of the two variables normalized (i.e., divided) by the product of their standard deviations. cov(x; y) rxy = SDx SDy.
The decision about how significant the results of a correlation analysis are needs to be informed by the purpose of the analysis. For example, if the correlation analysis is used as a reliability check of a questionnaire or for inter-rater reliability then the r coefficient needs to be very high r≥.80. If the analysis examines the possible ...
Calculation of sample sizes for analysis of correlations has been explored in an earlier edition of this periodical. 10. Correlation coefficients have inferential properties and, in scientific texts, should preferably be expressed with their 95% confidence intervals and significance (p-value), for example: ρ = 0.76 (95%CI 0.61-0.91), p < 0.01.
Correlation is a fundamental tool for multivariate data analysis. Most multivariate statistical methods use correlation as a basis for data analytics. Machine learning methods are also impacted by correlations in data. With todays' big data, the role of correlation becomes increasingly important. Although the basic concept of correlation is ...
Principles of Correlation Analysis. N. Gogtay, U. Thatte. Published in Journal of Association of… 1 March 2017. Medicine. TLDR. This article discusses how to establish a relationship or an association between two variables that can be "measured", and explores several possible re lat ionships between these binary variables and understood ...
Step 1: Collect the data, and create a table from your data. Step 2: Rank the data: Ranking is achieved by giving the ranking '1' to the biggest number in a column, '2' to the second biggest value and so on. The smallest value in the column will get the lowest ranking. This should be done for both sets of measurements.
Correlation comes prior to regression analysis. It starts with the concept of simple correlation coefficient; which gives the degree of linear relationship between two variables. One should draw scatter diagram in order to judge whether there exists any linear relation between the two variables. The correlation coefficient is not only invariant ...
A simple correlation analysis represents measures the degree of closeness between two related. variables. The correlation coefficient (r or R) as a measure provid es information about closeness ...
The correlation coefficient is easy to calculate and provides a measure of the strength of linear association in the data. However, it also has important limitations and pitfalls, both when studying the association between two variables and when studying agreement between methods. These limitations and pitfalls should be taken into account when ...
Correlation analysis is a statistic technique which aims to find relationship with correlation degree between two variables (Zawawi, 2012: 46). The researcher choose correlational research because in this study there are two variables, vocabulary mastery and reading comprehension that the purpose is to ... quantitative method, with the analysis ...
Correlation analysis is a statistical method used to evaluate the strength and direction of the relationship between two or more variables. The correlation coefficient ranges from -1 to 1. A correlation coefficient of 1 indicates a perfect positive correlation. This means that as one variable increases, the other variable also increases.
school climate. This research utilized a quantitative research design and a correlational analysis. I have worked in the education field for over nine years, and my interest in this topic was enhanced when my school incorporated restorative practices to manage student behaviors and improve our school climate.
correlation coefficient are. For example, for n =5, r =0.878 means that there is only a 5% chance of getting a result of 0.878 or greater if there is no correlation between the variables. Such a value, therefore, indicates the likely existence of a relationship between the variables. (no.of pairs) n r 3 0.997 4 0.950 5 0.878 6 0.811 7 0.755 8 0.707
Correlation is a real number that gives us an idea. of the degree of association between two vari-. ables. At a basic level, it is also known as " bivar-. iate "statistic. Thus, correlation is ...
The assumptions and study design of MR. MR is a methodology employed to assess causal associations between variables. In order to ensure the validity of MR analysis, 3 fundamental assumptions must be met: (i) the instrumental variable (IV) exhibits a strong link to the exposure factor, (ii) the IV remains unaffected by potential confounding factors., and (iii) the IV influences the result ...
2D-COS image acquisition. The acquisition of the generalized two-dimensional correlation infrared spectrum refers to the recording of the corresponding infrared spectrum (i.e. dynamic spectrum) of ...
However, there remains a research gap concerning the correlation between the TyG index and GLS among patients with chronic heart failure (CHF). 427 CHF patients were included in the final analysis. Patient demographic information, along with laboratory tests such as blood glucose, lipids profiles, and echocardiographic data were collected.
For the purposes of this research, a descriptive analysis was conducted along with Pearson's correlation and multiple linear regression analysis [9]. The regression analysis via SPSS has helped in ...
Background/Objectives: The general condition of implantology patients is crucial when considering the long- and short-term survival of dental implants. The aim of the research was to evaluate the correlation between the new corticalization index (CI) and patients' condition, and its impact on marginal bone loss (MBL) leading to implant failure, using only radiographic (RTG) images on a pixel ...
Background: As an important platform for researchers to present their academic findings, medical journals have a close relationship between their evaluation orientation and the value orientation of their published research results. However, the differences between the academic impact and level of disruptive innovation of medical journals have not been examined by any study yet.