Systematic literature review on identifying influencers in social networks
- Published: 30 June 2023
- Volume 56 , pages 567–660, ( 2023 )
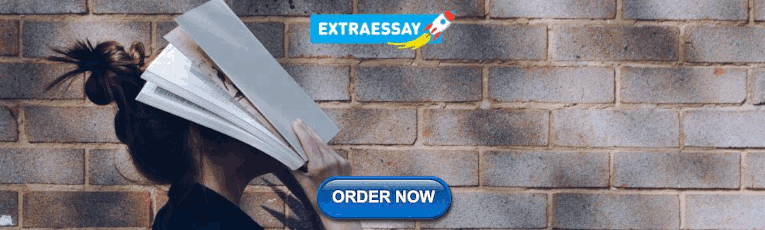
Cite this article
- Seyed Farid Seyfosadat 1 &
- Reza Ravanmehr 1
1209 Accesses
Explore all metrics
Considering the ever-increasing size and complexity of social networks, developing methods to extract meaningful knowledge and information from users’ vast amounts of data is crucial. Identifying influencers on social networks is one of the essential investigations on these networks and has many applications in marketing, advertising, sociology, behavior analysis, and security issues. In recent years, many studies have been conducted on analyzing and identifying influencers on social networks. Therefore, in this article, a Systematic Literature Review (SLR) has been performed on previous studies about the methods of identifying influencers. To this end, we review the definitions of influencers, the datasets used for evaluation purposes, the methods of identifying influencers, and the evaluation techniques. Furthermore, the quality assessment of the recently published papers also has been performed in different aspects to find whether research about identifying influencers has progressed. Finally, trends and opportunities for future studies about influencers’ identification are presented. The result of this SLR shows that the quantity and quality of articles in the field of identifying influencers in social networks are growing and progressive, which shows this field is a dynamic and active area of research.
This is a preview of subscription content, log in via an institution to check access.
Access this article
Price includes VAT (Russian Federation)
Instant access to the full article PDF.
Rent this article via DeepDyve
Institutional subscriptions
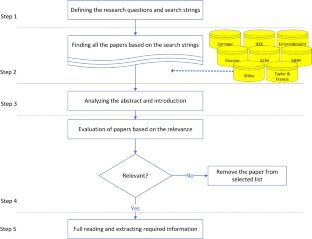
Similar content being viewed by others
How to Measure Influence in Social Networks?
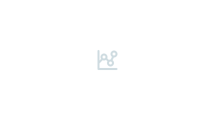
Social Influence Study in Online Networks: A Three-Level Review
Social Influence Analysis in Online Social Networks for Viral Marketing: A Survey
Abd Al-Azim NAR, Gharib TF, Afify Y, Hamdy M (2020) Influence propagation: Interest groups and node ranking models. Physica A: Statistical Mech and its Appl 553:124247
Google Scholar
Agarwal S, Mehta S (2020) Effective influence estimation in twitter using temporal, profile, structural and interaction characteristics. Inf Process Manage 57(6):102321
Aghaee Z, Kianian S (2020) Influence maximization algorithm based on reducing search space in the social networks. SN Appl Sci 2(12):1–14
Ahajjam S, El Haddad M, Badir H (2018) A new scalable leader-community detection approach for community detection in social networks. Soc Net 54:41–49
Alassani, R. and Göretz, J. Product Placements by Micro and Macro Influencers on Instagram . in Social Computing and Social Media. Communication and Social Communities . 2019. Cham: Springer International Publishing.
Al-garadi MA, Varathan KD, Ravana SD (2017) Identification of influential spreaders in online social networks using interaction weighted K-core decomposition method. Physica A 468:278–288
Al-Garadi MA, Varathan KD, Ravana SD, Ahmed E, Mujtaba G, Khan MUS, Khan SU (2018) Analysis of online social network connections for identification of influential users: survey and open research issues. ACM Computing Surveys (CSUR) 51(1):1–37
Al-Oraiqat AM, Ulichev OS, Meleshko YV, AlRawashdeh HS, Smirnov OO, Polishchuk LI (2022) Modeling strategies for information influence dissemination in social networks. J Ambient Intell Humaniz Comput 13(5):2463–2477
Alp, Z.Z. and Öğüdücü, Ş.G. Influential user detection on Twitter: analyzing effect of focus rate . in 2016 IEEE/ACM International Conference on Advances in Social Networks Analysis and Mining (ASONAM) . 2016. IEEE.
Althbiti, A. and Ma, X. Social Network Influencers’ Data Augmenting Recommender Systems . in 2020 International Conference on Computational Science and Computational Intelligence (CSCI) . 2020. IEEE.
Amati, G., Angelini, S., Gambosi, G., Rossi, G., and Vocca, P. The Analysis of Influential Users Evolution in Microblogging Social Networks . in International Conference on Smart Objects and Technologies for Social Good . 2017. Springer.
Anger, I. and Kittl, C., Measuring influence on Twitter , in Proceedings of the 11th International Conference on Knowledge Management and Knowledge Technologies . 2011, Association for Computing Machinery: Graz, Austria. p. Article 31.
Arora A, Bansal S, Kandpal C, Aswani R, Dwivedi Y (2019) Measuring social media influencer index-insights from Facebook, Twitter and Instagram. J Retail Consum Serv 49:86–101
Arularasan A, Suresh A, Seerangan K (2019) Identification and classification of best spreader in the domain of interest over the social networks. Clust Comput 22(2):4035–4045
Azaouzi M, Mnasri W, Ben Romdhane L (2021) New trends in influence maximization models. Comput Sci Rev 40:100393
MATH Google Scholar
Bahutair M, Al Aghbari Z, Kamel I (2022) NodeRank: finding influential nodes in social networks based on interests. J Supercomput 78(2):2098–2124
Bakshy, E., Hofman, J.M., Mason, W.A., and Watts, D.J., Everyone's an influencer: quantifying influence on twitter , in Proceedings of the fourth ACM international conference on Web search and data mining . 2011, Association for Computing Machinery: Hong Kong, China. p. 65–74.
Banerjee S, Jenamani M, Pratihar DK (2020) A survey on influence maximization in a social network. Knowl Inf Syst 62(9):3417–3455
Barta S, Belanche D, Fernández A, Flavián M (2023) Influencer marketing on TikTok: the effectiveness of humor and followers’ hedonic experience. J Retail Consum Serv 70:103149
Bawack, R.E. and Bonhoure, E. Influencer is the New Recommender: Insights for Enhancing Social Recommender Systems . in Conference on e-Business, e-Services and e-Society . 2021. Springer.
Bhowmick AK, Gueuning M, Delvenne J-C, Lambiotte R, Mitra B (2019) Temporal sequence of retweets help to detect influential nodes in social networks. IEEE Trans Comput Soc Syst 6(3):441–455
Bonifazi G, Cauteruccio F, Corradini E, Marchetti M, Pierini A, Terracina G, Ursino D, Virgili L (2022a) An approach to detect backbones of information diffusers among different communities of a social platform. Data Knowl Eng 140:102048
Bonifazi G, Corradini E, Ursino D, Virgili L (2022b) Defining user spectra to classify Ethereum users based on their behavior. Journal of Big Data 9(1):37
Bonnevie E, Rosenberg SD, Kummeth C, Goldbarg J, Wartella E, Smyser J (2020a) Using social media influencers to increase knowledge and positive attitudes toward the flu vaccine. PLoS ONE 15(10):e0240828
Bonnevie, E., Smith, S., Kummeth, C., Goldbarg, J., and Smyser, J., Using Social Media Influencers to Deliver Positive Information About the Flu Vaccine: Findings from a Multi-Year Qualitative Study. Available at SSRN 3697432, 2020b.
Britt BC, Britt RK, Anderson J, Fahrenwald N, Harming S (2022) Finding the invisible leader: when a priori opinion leader identification is impossible. Commun Rep 35(1):38–52
Bu Z, Li H-J, Zhang C, Cao J, Li A, Shi Y (2020) Graph K-means based on leader identification, dynamic game, and opinion dynamics. IEEE Trans Knowl Data Eng 32(7):1348–1361
Chen Y, Qu Q, Ying Y, Li H, Shen J (2020) Semantics-aware influence maximization in social networks. Inf Sci 513:442–464
MathSciNet MATH Google Scholar
Chen J, Kou G, Wang H, Zhao Y (2021) Influence identification of opinion leaders in social networks: an agent-based simulation on competing advertisements. Information Fusion 76:227–242
Chen CY-H, Härdle WK, Klochkov Y (2022) SONIC: social network analysis with influencers and communities. Journal of Econometrics 228(2):177–220
Chen, Y.-C., Cheng, J.-Y., and Hsu, H.-H. A cluster-based opinion leader discovery in social network . in 2016 conference on technologies and applications of artificial intelligence (TAAI) . 2016. IEEE.
Conti M, Gathani J, Tricomi PP (2022) Virtual Influencers in Online Social Media. IEEE Commun Mag 60(8):86–91
Cortis K, Davis B (2021) Over a decade of social opinion mining: a systematic review. Artif Intell Rev 54(7):4873–4965
Dang-Pham D, Kautz K, Hoang A-P, Pittayachawan S (2022) Identifying information security opinion leaders in organizations: Insights from the theory of social power bases and social network analysis. Comput Secur 112:102505
De Veirman M, Cauberghe V, Hudders L (2017) Marketing through Instagram influencers: the impact of number of followers and product divergence on brand attitude. Int J Advert 36(5):798–828
Dong C, Xu G, Meng L, Yang P (2022) CPR-TOPSIS: A novel algorithm for finding influential nodes in complex networks based on communication probability and relative entropy. Physica A 603:127797
Eliacik AB, Erdogan N (2018) Influential user weighted sentiment analysis on topic based microblogging community. Expert Syst Appl 92:403–418
Featherstone JD, Barnett GA, Ruiz JB, Zhuang Y, Millam BJ (2020) Exploring childhood anti-vaccine and pro-vaccine communities on twitter–a perspective from influential users. Online Soc Net Media 20:100105
Gao P, Hung J, Xu Y (2020) A k-core decomposition-based opinion leaders identifying method and clustering-based consensus model for large-scale group decision making. Comput Ind Eng 150:106842
Geyser, W. The State of Influencer Marketing 2023: Benchmark Report . 2023; Available from: https://influencermarketinghub.com/influencer-marketing-benchmark-report/ .
Girgin BA (2021) Ranking influencers of social networks by semantic kernels and sentiment information. Expert Syst Appl 171:114599
Haenlein, M., Anadol, E., Farnsworth, T., Hugo, H., Hunichen, J., and Welte, D., 2020 Navigating the New Era of Influencer Marketing: How to be Successful on Instagram, TikTok, & Co. California Management Review. 63 (1): 5–25.
Hajarathaiah K, Enduri MK, Anamalamudi S (2022) Efficient algorithm for finding the influential nodes using local relative change of average shortest path. Physica A 591:126708
Han J, Chen Q, Jin X, Xu W, Yang W, Kumar S, Zhao L, Sundaram H, Kumar R (2021) FITNet: identifying fashion influencers on Twitter. Proc of the ACM Hum-Comput Interact. https://doi.org/10.1145/3449227
Article Google Scholar
Harrigan P, Daly TM, Coussement K, Lee JA, Soutar GN, Evers U (2021) Identifying influencers on social media. Int J Inf Manage 56:102246
Hasson, S.T. and Akeel, E. Influence maximization problem approach to model social networks . in 2019 International Conference on Advanced Science and Engineering (ICOASE) . 2019. IEEE.
Hou L (2022) Network versus content: the effectiveness in identifying opinion leaders in an online social network with empirical evaluation. Physica A 592:126879
Hou, X., Lei, C.-U., and Kwok, Y.-K. OP-DCI: A riskless K-means clustering for influential user identification in MOOC forum . in 2017 16th IEEE International Conference on Machine Learning and Applications (ICMLA) . 2017. IEEE.
Huang C-C, Liang W-Y, Chen P-A, Chan Y-C (2020a) Identification of opinion leaders and followers—a case study of green energy and low carbons. Appl Sci 10(23):8416
Huang X, Chen D, Wang D, Ren T (2020b) Identifying influencers in social networks. Entropy 22(4):450
MathSciNet Google Scholar
Huang X, Chen D, Wang D, Ren T (2020c) MINE: identifying top-k vital nodes in complex networks via maximum influential neighbors expansion. Mathematics 8(9):1449
Huynh T, Nguyen H, Zelinka I, Dinh D, Pham XH (2020) Detecting the influencer on social networks using passion point and measures of information propagation. Sustainability 12(7):3064
Huynh, T., Zelinka, I., Pham, X.H., and Nguyen, H.D. Some measures to detect the influencer on social network based on information propagation . in Proceedings of the 9th International Conference on Web Intelligence, Mining and Semantics . 2019.
Ibrahim, R.A., Hefny, H.A., and Hassanien, A.E. Group impact: local influence maximization in social networks . in International Conference on Advanced Intelligent Systems and Informatics . 2016. Springer.
Israfilova, E., Arslan, A., Yildirim, N., and Kaya, T., Influencer identification system design using machine learning techniques. Advances in Intelligent Systems and Computing, 1197 AISC, 1092–1099 . 2021.
Jain L (2022) An entropy-based method to control COVID-19 rumors in online social networks using opinion leaders. Technol Soc 70:102048
Jain L, Katarya R (2019) Discover opinion leader in online social network using firefly algorithm. Expert Syst Appl 122:1–15
Jain S, Sinha A (2020) Identification of influential users on Twitter: a novel weighted correlated influence measure for covid-19. Chaos, Solitons Fractals 139:110037
Jain L, Katarya R, Sachdeva S (2020) Opinion leader detection using whale optimization algorithm in online social network. Expert Syst Appl 142:113016
Javadpour Boroujeni R, Soleimani S (2022) The role of influential nodes and their influence domain in community detection: an approximate method for maximizing modularity. Exp Syst with Appl 202:117452
Jin SV, Muqaddam A, Ryu E (2019) Instafamous and social media influencer marketing. Mark Intell Plan 37(5):567–579
Kaur, S., Saxena, R., and Bhatnagar, V. Leveraging hierarchy and community structure for determining influencers in networks . in International Conference on Big Data Analytics and Knowledge Discovery . 2017. Springer.
Kazemzadeh F, Safaei AA, Mirzarezaee M (2022) Influence maximization in social networks using effective community detection. Physica A 598:127314
Kim, S., Jiang, J.-Y., and Wang, W., Discovering Undisclosed Paid Partnership on Social Media via Aspect-Attentive Sponsored Post Learning , in Proceedings of the 14th ACM International Conference on Web Search and Data Mining . 2021, Association for Computing Machinery: Virtual Event, Israel. p. 319–327.
Kitchenham B, Pearl Brereton O, Budgen D, Turner M, Bailey J, Linkman S (2009) Systematic literature reviews in software engineering – a systematic literature review. Inf Softw Technol 51(1):7–15
Kuikka V (2018) Influence spreading model used to analyse social networks and detect sub-communities. Comput Soc Net 5(1):1–39
Kumar, S., Gupta, A., and Khatri, I., CSR: A community based spreaders ranking algorithm for influence maximization in social networks. World Wide Web, 2022.
Kumar S, Panda B (2020) Identifying influential nodes in social networks: neighborhood coreness based voting approach. Physica A 553:124215
Ladhari R, Massa E, Skandrani H (2020) YouTube vloggers’ popularity and influence: the roles of homophily, emotional attachment, and expertise. J Retail Consum Serv 54:102027
Li S, Xiao F (2021) The identification of crucial spreaders in complex networks by effective gravity model. Inf Sci 578:725–749
Li C, Bai J, Zhang L, Tang H, Luo Y (2019) Opinion community detection and opinion leader detection based on text information and network topology in cloud environment. Inf Sci 504:61–83
Li X, Sun C, Zia MA (2020) Social influence based community detection in event-based social networks. Inf Process Manage 57(6):102353
Li H, Shang Q, Deng Y (2021) A generalized gravity model for influential spreaders identification in complex networks. Chaos, Solitons Fractals 143:110456
Li, H., Huang, S., and Sun, G. An opinion leader perceptual model based on PageRank algorithm . in 2015 International Conference on Behavioral, Economic and Socio-cultural Computing (BESC) . 2015. IEEE.
Li, Y., Fan, J., Zhang, D., and Tan, K.-L. 2017 Discovering your selling points: Personalized social influential tags exploration. In Proceedings of the 2017 ACM International Conference on Management of Data..
Liao, X., Zheng, D., Wu, Y., and Cao, X. Should We Trust Influencers on Social Networks? On Instagram Sponsored Post Analysis . in 2021 International Conference on Computer Communications and Networks (ICCCN) . 2021.
Lin L-F, Li Y-M (2021) An efficient approach to identify social disseminators for timely information diffusion. Inf Sci 544:78–96
Litterio AM, Nantes EA, Larrosa JM, Gómez LJ (2017) Marketing and social networks: a criterion for detecting opinion leaders. European J Manag and Business Economics. https://doi.org/10.1108/EJMBE-10-2017-020
Liu Y, Fan Z-P, You T-H, Zhang W-Y (2018) Large group decision-making (LGDM) with the participators from multiple subgroups of stakeholders: a method considering both the collective evaluation and the fairness of the alternative. Comput Ind Eng 122:262–272
Liu X-C, Zhu X-Z, Tian H, Zhang Z-P, Wang W (2019) Identifying localized influential spreaders of information spreading. Physica A 519:92–97
Liu X, Ye S, Fiumara G, Meo PD (2022) Influential spreaders identification in complex networks with TOPSIS and K-shell decomposition. IEEE Trans Comput Soc Syst. https://doi.org/10.1109/TCSS.2022.3148778
Lotf JJ, Azgomi MA, Dishabi MRE (2022) An improved influence maximization method for social networks based on genetic algorithm. Physica A 586:126480
Ma N, Liu Y, Chi Y (2018) Influencer discovery algorithm in a multi-relational network. Physica A 510:415–425
Ma T, Liu Q, Cao J, Tian Y, Al-Dhelaan A, Al-Rodhaan M (2020) LGIEM: global and local node influence based community detection. Futur Gener Comput Syst 105:533–546
Mao C, Xiao W (2018) A comprehensive algorithm for evaluating node influences in social networks based on preference analysis and random walk. Complexity. https://doi.org/10.1155/2018/1528341
Mao Y, Zhou L, Xiong N (2021) TPS: a topological potential scheme to predict influential network nodes for intelligent communication in social networks. IEEE Trans on Net Sci and Eng 8(1):529–540
Martins P, Martins FA (2021) Launcher nodes for detecting efficient influencers in social networks. Online Soc Net and Media 25:100157
Mehta Y, Majumder N, Gelbukh A, Cambria E (2020) Recent trends in deep learning based personality detection. Artif Intell Rev 53(4):2313–2339
Meng L, Xu G, Yang P, Tu D (2022) A novel potential edge weight method for identifying influential nodes in complex networks based on neighborhood and position. J Comput Sci 60:101591
Mittal D, Suthar P, Patil M, Pranaya P, Rana DP, Tidke B (2020) Social network influencer rank recommender using diverse features from topical graph. Procedia Comput Sci 167:1861–1871
Mnasri W, Azaouzi M, Romdhane LB (2021) Parallel social behavior-based algorithm for identification of influential users in social network. Appl Intell. https://doi.org/10.1007/s10489-021-02203-x
Article MATH Google Scholar
Mohamed A, Najafabadi MK, Wah YB, Zaman EAK, Maskat R (2020) The state of the art and taxonomy of big data analytics: view from new big data framework. Artif Intell Rev 53(2):989–1037
Moher D, Liberati A, Tetzlaff J, Altman DG (2009) Preferred reporting items for systematic reviews and meta-analyses: the PRISMA statement. BMJ 339:b2535
Monti D, Rizzo G, Morisio M (2021) A systematic literature review of multicriteria recommender systems. Artif Intell Rev 54(1):427–468
Moraes L, Cordeiro R (2019) Detecting influencers in very large social networks of games . In: Proceedings of the 21st international conference on enterprise information systems, ICEIS, pp 93–103
More, J.S. and Lingam, C. A scalable data mining model for social media influencer identification . In International Conference on Smart Trends for Information Technology and Computer Communications . 2016. Springer.
More JS, Lingam C (2019) A SI model for social media influencer maximization. Appl Comput and Inform 15(2):102–108
Mussiraliyeva, S., Baispay, G., Ospanov, R., Medetbek, Z., and Shalabayev, K. Graphical Visualization of the Connections of Involved Users and Identifying Influential Spreaders in a Social Network . in 2022 9th International Conference on Electrical and Electronics Engineering (ICEEE) . 2022.
Namtirtha A, Dutta B, Dutta A (2022) Semi-global triangular centrality measure for identifying the influential spreaders from undirected complex networks. Expert Syst Appl 206:117791
Neves A, Vieira R, Mourao F, Rocha L (2015) Quantifying complementarity among strategies for influencers’ detection on Twitter. Procedia Computer Sci 51:2435–2444
Ou Y, Guo Q, Xing J-L, Liu J-G (2022) Identification of spreading influence nodes via multi-level structural attributes based on the graph convolutional network. Expert Syst Appl 203:117515
Oueslati W, Arrami S, Dhouioui Z, Massaabi M (2021) Opinion leaders’ detection in dynamic social networks. Concurrency and Comput: Practice and Exp 33(1):e5692
Page MJ, McKenzie JE, Bossuyt PM, Boutron I, Hoffmann TC, Mulrow CD, Shamseer L, Tetzlaff JM, Akl EA, Brennan SE, Chou R, Glanville J, Grimshaw JM, Hróbjartsson A, Lalu MM, Li T, Loder EW, Mayo-Wilson E, McDonald S, McGuinness LA, Stewart LA, Thomas J, Tricco AC, Welch VA, Whiting P, Moher D (2021) The PRISMA 2020 statement: an updated guideline for reporting systematic reviews. BMJ 372:n71
Pajo S, Verhaegen P-A, Vandevenne D, Duflou JR (2015) Fast lead user identification framework. Procedia Eng 131:1140–1145
Panagopoulos G, Malliaros F, Vazirgiannis M (2020) Multi-task learning for influence estimation and maximization. IEEE Trans Knowledge and Data Eng 34(9):4398–4409
Peng S, Zhou Y, Cao L, Yu S, Niu J, Jia W (2018) Influence analysis in social networks: a survey. J Netw Comput Appl 106:17–32
Pöyry E, Reinikainen H, Luoma-Aho V (2022) The role of social media influencers in public health communication: case COVID-19 pandemic. Int J Strateg Commun 16(3):469–484
Prado-Romero, M.A., Oliva, A.F., and Hernández, L.G. Identifying twitter users influence and open mindedness using anomaly detection . in International Workshop on Artificial Intelligence and Pattern Recognition . 2018. Springer.
Qing, L., Wei, W., Yi, H., and Qian, Z. Analyzing the influential people in Sina Weibo dataset . in 2013 IEEE Global Communications Conference (GLOBECOM) . 2013.
Rakoczy, M.E., Bouzeghoub, A., Gancarski, A.L., and Wegrzyn-Wolska, K. In the search of quality influence on a small scale–micro-influencers discovery . in OTM Confederated International Conferences" On the Move to Meaningful Internet Systems” 2018. Springer.
Rehman AU, Jiang A, Rehman A, Paul A, Sadiq MT (2020) Identification and role of opinion leaders in information diffusion for online discussion network. J Ambient Intell and Humanized Comput. https://doi.org/10.1007/s12652-019-01623-5
Rios SA, Aguilera F, Nuñez-Gonzalez JD, Graña M (2019) Semantically enhanced network analysis for influencer identification in online social networks. Neurocomputing 326:71–81
Riquelme F, Gonzalez-Cantergiani P, Hans D, Villarroel R, Munoz R (2019) Identifying opinion leaders on social networks through milestones definition. IEEE Access 7:75670–75677
Röchert D, Cargnino M, Neubaum G (2022) Two sides of the same leader: an agent-based model to analyze the effect of ambivalent opinion leaders in social networks. J Comput Soc Sci. https://doi.org/10.1007/s42001-022-00161-z
Rodríguez-Vidal J, Gonzalo J, Plaza L, Sánchez HA (2019) Automatic detection of influencers in social networks: authority versus domain signals. J Am Soc Inf Sci 70(7):675–684
Rosenthal S, Mckeown K (2017) Detecting influencers in multiple online genres. ACM Trans on Internet Technol (TOIT) 17(2):1–22
Ruas PHB, Machado AD, Silva MC, Meireles MR, Cardoso AMP, Zárate LE, Nobre CN (2019) Identification and characterisation of Facebook user profiles considering interaction aspects. Behav Inform Technol 38(8):858–872
Rui X, Meng F, Wang Z, Yuan G (2019) A reversed node ranking approach for influence maximization in social networks. Appl Intell 49(7):2684–2698
Rui X, Yang X, Fan J, Wang Z (2020) A neighbour scale fixed approach for influence maximization in social networks. Computing 102(2):427–449
Ruiz, J., Featherstone, J.D., and Barnett, G.A. Identifying Vaccine Hesitant Communities on Twitter and their Geolocations: A Network Approach . In Proceedings of the 54th Hawaii international conference on system sciences . 2021.
Samanta S, Dubey VK, Sarkar B (2021) Measure of influences in social networks. Appl Soft Comput 99:106858
Samir AM, Rady S, Gharib TF (2021) LKG: A fast scalable community-based approach for influence maximization problem in social networks. Physica A 582:126258
Sardana, N., Thakur, T., Mehrotra, M., and Tejwani, D. Nature Inspired Algorithm towards Influence Maximization in Social Networks . in 2022 1st International Conference on Informatics (ICI) . 2022.
Sarkar R, Barman D, Chowdhury N (2018) To detect the influencers in a dynamic co-authorship network using heat-diffusion model. Advanced Computational and Communication Paradigms. Springer, pp 259–268
Saxena B, Kumar P (2019) A node activity and connectivity-based model for influence maximization in social networks. Soc Netw Anal Min 9(1):1–16
Segev, N., Avigdor, N., and Avigdor, E., Measuring Influence on Instagram: A Network-Oblivious Approach, in The 41st International ACM SIGIR Conference on Research & Development in Information Retrieval. 2018, Association for Computing Machinery: Ann Arbor, MI, USA. p. 1009–1012
Senevirathna C, Gunaratne C, Rand W, Jayalath C, Garibay I (2021) Influence cascades: entropy-based characterization of behavioral influence patterns in social media. Entropy 23(2):160
Shang Q, Deng Y, Cheong KH (2021) Identifying influential nodes in complex networks: effective distance gravity model. Inf Sci 577:162–179
Sheikhahmadi A, Nematbakhsh MA, Zareie A (2017) Identification of influential users by neighbors in online social networks. Physica A 486:517–534
Shetty RD, Bhattacharjee S, Dutta A, Namtirtha A (2022) GSI: an influential node detection approach in heterogeneous network using covid-19 as use case. IEEE Trans Comput Soc Syst. https://doi.org/10.1109/TCSS.2022.3180177
Shi L, Wu Y, Liu L, Sun X, Jiang L (2018) Event detection and identification of influential spreaders in social media data streams. Big Data Mining and Analytics 1(1):34–46
Shinde, M. and Girase, S. Identification of topic-specific Opinion Leader using SPEAR algorithm in Online Knowledge communities . in 2016 International Conference on Computing, Analytics and Security Trends (CAST) . 2016. IEEE.
ŞİMŞEK A, Resul K (2018) Using swarm intelligence algorithms to detect influential individuals for influence maximization in social networks. Exp Syst with Appl 114:224–236
Singh AK, Kailasam L (2021) Link prediction-based influence maximization in online social networks. Neurocomputing 453:151–163
Ssanyu JN, Kiguba R, Olum R, Kiguli J, Kitutu FE (2022) Using community influencer groups to address COVID-19 misinformation and vaccine hesitancy in Uganda: a protocol for a prospective quasi-experimental study. BMJ Open 12(8):e057994
Statista. Number of Instagram users worldwide from 2020a to 2025 . 2023; Available from: https://www.statista.com/statistics/183585/instagram-number-of-global-users/ .
Statista. Number of TikTok users worldwide from 2020b to 2025 . 2023; Available from: https://www.statista.com/statistics/1327116/number-of-global-tiktok-users/ .
Statista. Number of internet and social media users worldwide as of January 2023. Available from: https://www.statista.com/statistics/617136/digital-population-worldwide/ .
Tafesse W, Wood BP (2021) Followers’ engagement with instagram influencers: the role of influencers’ content and engagement strategy. J Retail Consum Serv 58:102303
Tang J, Zhang R, Wang P, Zhao Z, Fan L, Liu X (2020) A discrete shuffled frog-leaping algorithm to identify influential nodes for influence maximization in social networks. Knowl-Based Syst 187:104833
Tantisuwankul, J., Manaskasemsak, B., and Rungsawang, A., Identifying Influencers in Thai Internet Forum based on Topic-oriented Gravity Model, in 2020 4th International Conference on Computer Science and Artificial Intelligence. 2020, Association for Computing Machinery: Zhuhai, China. p. 271–277.
Tsapatsoulis, N., Anastasopoulou, V., and Ntalianis, K. The central community of Twitter ego-networks as a means for fake influencer detection . in 2019 IEEE Intl Conf on Dependable, Autonomic and Secure Computing, Intl Conf on Pervasive Intelligence and Computing, Intl Conf on Cloud and Big Data Computing, Intl Conf on Cyber Science and Technology Congress (DASC/PiCom/CBDCom/CyberSciTech) . 2019. IEEE.
Vassio, L., Garetto, M., Chiasserini, C., and Leonardi, E., Temporal dynamics of posts and user engagement of influencers on Facebook and Instagram , in Proceedings of the 2021 IEEE/ACM International Conference on Advances in Social Networks Analysis and Mining . 2022, Association for Computing Machinery: Virtual Event, Netherlands. p. 129–133.
Vega-Oliveros DA, da Fontoura Costa L, Rodrigues FA (2020) Influence maximization by rumor spreading on correlated networks through community identification. Commun Nonlinear Sci Numer Simul 83:105094
Vikatos P, Gryllos P, Makris C (2020) Marketing campaign targeting using bridge extraction in multiplex social network. Artif Intell Rev 53(1):703–724
Wang N, Sun Q, Zhou Y, Shen S (2016a) A study on influential user identification in online social networks. Chin J Electron 25(3):467–473
Wang D, Yan J, Chen D, Fang B, Huang X (2020) Rna: A reject neighbors algorithm for influence maximization in complex networks. Mathematics 8(8):1313
Wang S, Gan T, Liu Y, Zhang L, Wu J, Nie L (2022) Discover micro-influencers for brands via better understanding. IEEE Trans Multimedia. https://doi.org/10.1109/TMM.2021.3087038
Wang S, Gan T, Liu Y, Wu J, Cheng Y, Nie L (2022) Micro-influencer recommendation by multi-perspective account representation learning. IEEE Trans on Multimedia. https://doi.org/10.1109/TMM.2022.3151029
Wang F, Sun Z, Gan Q, Fan A, Shi H, Hu H (2022c) Influential node identification by aggregating local structure information. Physica A 593:126885
Wang, C., Du, Y.J., and Tang, M.W. Opinion leader mining algorithm in microblog platform based on topic similarity . in 2016b 2nd IEEE International Conference on Computer and Communications (ICCC) . 2016b. IEEE.
Wei J, Meng F (2021) How opinion distortion appears in super-influencer dominated social network. Futur Gener Comput Syst 115:542–552
Wen T, Pelusi D, Deng Y (2020) Vital spreaders identification in complex networks with multi-local dimension. Knowl-Based Syst 195:105717
Wendy, B. and Faina, D., THE FUTURE OF INFLUENCER MARKETING IN THE DIGITAL AGE OF VIRTUAL INFLUENCERS. Global Fashion Management Conference, 2019: p. 604–607.
Wibisono, A.I. and Ruldeviyani, Y. Detecting Social Media Influencers of Airline Services through Social Network Analysis on Twitter: A Case Study of the Indonesian Airline Industry . in 2021 3rd East Indonesia Conference on Computer and Information Technology (EIConCIT) . 2021. IEEE.
Wu J, Shen J, Zhou B, Zhang X, Huang B (2019) General link prediction with influential node identification. Physica A 523:996–1007
Xiao M, Wang R, Chan-Olmsted S (2018) Factors affecting YouTube influencer marketing credibility: a heuristic-systematic model. J Media Bus Stud 15(3):188–213
Xu S, Wang P, Zhang C (2019) Identification of influential spreaders in bipartite networks: a singular value decomposition approach. Physica A 513:297–306
Yang X, Xiao F (2021) An improved gravity model to identify influential nodes in complex networks based on k-shell method. Knowl-Based Syst 227:107198
Yang L, Qiao Y, Liu Z, Ma J, Li X (2018) Identifying opinion leader nodes in online social networks with a new closeness evaluation algorithm. Soft Comput 22(2):453–464
Yu E-Y, Wang Y-P, Fu Y, Chen D-B, Xie M (2020a) Identifying critical nodes in complex networks via graph convolutional networks. Knowl-Based Syst 198:105893
Yu E, Fu Y, Tang Q, Zhao J-Y, Chen D-B (2020b) A re-ranking algorithm for identifying influential nodes in complex networks. IEEE Access 8:211281–211290
Yusuf, A.M., Saputro, M.R.G., and Maharani, W. Identifying Influencers On Twitter For Covid-19 Education And Vaccination Using Social Network Analysis . In 2021 International Conference on Software Engineering & Computer Systems and 4th International Conference on Computational Science and Information Management (ICSECS-ICOCSIM) . 2021.
Zarei, K., Ibosiola, D., Farahbakhsh, R., Gilani, Z., Garimella, K., Crespi, N., and Tyson, G. Characterising and Detecting Sponsored Influencer Posts on Instagram . in 2020 IEEE/ACM International Conference on Advances in Social Networks Analysis and Mining (ASONAM) . 2020.
Zareie A, Sheikhahmadi A, Jalili M (2019) Influential node ranking in social networks based on neighborhood diversity. Futur Gener Comput Syst 94:120–129
Zareie A, Sheikhahmadi A, Jalili M (2020) Identification of influential users in social network using gray wolf optimization algorithm. Expert Syst Appl 142:112971
Zatwarnicka-Madura B, Nowacki R, Wojciechowska I (2022) Influencer marketing as a tool in modern communication—possibilities of use in green energy promotion amongst poland’s generation Z. Energies. https://doi.org/10.3390/en15186570
Zhang B, Zhang L, Mu C, Zhao Q, Song Q, Hong X (2019b) A most influential node group discovery method for influence maximization in social networks: a trust-based perspective. Data Knowl Eng 121:71–87
Zhang B, Bai Y, Zhang Q, Lian J, Li M (2020) An opinion-leader mining method in social networks with a phased-clustering perspective. IEEE Access 8:31539–31550
Zhang Z, Li X, Gan C (2021) Identifying influential nodes in social networks via community structure and influence distribution difference. Digital Commun and Net 7(1):131–139
Zhang, Z., Zhao, W., Yang, J., Paris, C., and Nepal, S. Learning influence probabilities and modelling influence diffusion in twitter . in Companion Proceedings of The 2019a World Wide Web Conference . 2019a.
Zhao Y, Kou G, Peng Y, Chen Y (2018) Understanding influence power of opinion leaders in e-commerce networks: an opinion dynamics theory perspective. Inf Sci 426:131–147
Zhao Q, Yang J, Wang S, Li M, Zhang W (2019) High-value user identification based on topic weight. IEEE Access 7:175917–175928
Zhao G, Jia P, Zhou A, Zhang B (2020) InfGCN: Identifying influential nodes in complex networks with graph convolutional networks. Neurocomputing 414:18–26
Zhao Z, Shi L, Li T, Shao J, Cheng Y (2022) Opinion dynamics of social networks with Intermittent-Influence Leaders. IEEE Trans on Comput Soc Syst. https://doi.org/10.1109/TCSS.2022.3145044
Zheng, C., Zhang, Q., Young, S., and Wang, W. On-demand Influencer Discovery on Social Media . In Proceedings of the 29th ACM International Conference on Information & Knowledge Management . 2020.
Zhou Z, Tian B (2019) Research on community detection of online social network members based on the sparse subspace clustering approach. Future Internet 11(12):254
Zhou F, Lü L, Mariani MS (2019) Fast influencers in complex networks. Commun Nonlinear Sci Numer Simul 74:69–83
Zhu J, Liu Y, Yin X (2017) A new structure-hole-based algorithm for influence maximization in large online social networks. IEEE Access 5:23405–23412
Zhuang Y-B, Li Z-H, Zhuang Y-J (2021) Identification of influencers in online social networks: measuring influence considering multidimensional factors exploration. Heliyon 7(4):e06472
Ziarani RJ, Ravanmehr R (2021) Serendipity in recommender systems: a systematic literature review. J Comput Sci Technol 36(2):375–396
Download references
Author information
Authors and affiliations.
Department of Computer Engineering, Central Tehran Branch, Islamic Azad University, Tehran, Iran
Seyed Farid Seyfosadat & Reza Ravanmehr
You can also search for this author in PubMed Google Scholar
Contributions
Seyed Farid Seyfosadat: Concept, Design, Methodology, Evaluation. Reza Ravanmehr: Concept, Verification, Validation, Editing.
Corresponding author
Correspondence to Reza Ravanmehr .
Ethics declarations
Competing interests.
The authors declare no competing interests.
Additional information
Publisher's note.
Springer Nature remains neutral with regard to jurisdictional claims in published maps and institutional affiliations.
Rights and permissions
Springer Nature or its licensor (e.g. a society or other partner) holds exclusive rights to this article under a publishing agreement with the author(s) or other rightsholder(s); author self-archiving of the accepted manuscript version of this article is solely governed by the terms of such publishing agreement and applicable law.
Reprints and permissions
About this article
Seyfosadat, S.F., Ravanmehr, R. Systematic literature review on identifying influencers in social networks. Artif Intell Rev 56 (Suppl 1), 567–660 (2023). https://doi.org/10.1007/s10462-023-10515-2
Download citation
Published : 30 June 2023
Issue Date : October 2023
DOI : https://doi.org/10.1007/s10462-023-10515-2
Share this article
Anyone you share the following link with will be able to read this content:
Sorry, a shareable link is not currently available for this article.
Provided by the Springer Nature SharedIt content-sharing initiative
- Systematic literature review
- Social network
- Opinion leader
- Quality assessment
- Performance analysis
- Find a journal
- Publish with us
- Track your research

An official website of the United States government
The .gov means it’s official. Federal government websites often end in .gov or .mil. Before sharing sensitive information, make sure you’re on a federal government site.
The site is secure. The https:// ensures that you are connecting to the official website and that any information you provide is encrypted and transmitted securely.
- Publications
- Account settings
Preview improvements coming to the PMC website in October 2024. Learn More or Try it out now .
- Advanced Search
- Journal List
- Entropy (Basel)

Identifying Influencers in Social Networks
Social network analysis is a multidisciplinary research covering informatics, mathematics, sociology, management, psychology, etc. In the last decade, the development of online social media has provided individuals with a fascinating platform of sharing knowledge and interests. The emergence of various social networks has greatly enriched our daily life, and simultaneously, it brings a challenging task to identify influencers among multiple social networks. The key problem lies in the various interactions among individuals and huge data scale. Aiming at solving the problem, this paper employs a general multilayer network model to represent the multiple social networks, and then proposes the node influence indicator merely based on the local neighboring information. Extensive experiments on 21 real-world datasets are conducted to verify the performance of the proposed method, which shows superiority to the competitors. It is of remarkable significance in revealing the evolutions in social networks and we hope this work will shed light for more and more forthcoming researchers to further explore the uncharted part of this promising field.
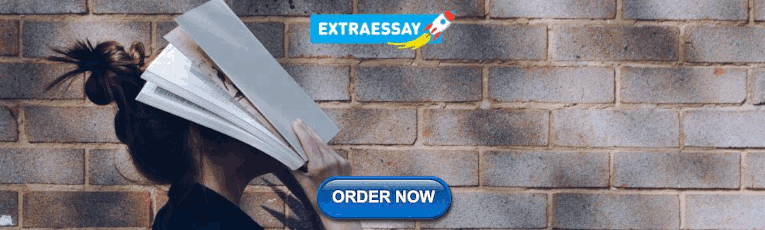
1. Introduction
The research of network science is experiencing a blossom in the last decade, which provides profound implications in very different fields, from finance to social and biological networks [ 1 ]. Considering the enormous data scale, most studies merely focus on a small group of influential nodes rather than the whole network. Take social networks for instance, influential nodes are those that have the most spreading ability, or playing a predominant role in the network evolution. Notably, a popular star in online social media may remarkably accelerate the spreading of rumors, and a few super spreaders [ 2 ] could largely expand the epidemic prevalence of a disease (e.g., COVID-19) [ 3 ]. The research of influencer identification is beneficial to understanding and controlling the spreading dynamics in social networks with diverse applications such as epidemiology, collective dynamics and viral marketing [ 4 , 5 ].
Nowadays, individuals interact with each other in more complicated patterns than ever. It is a challenging task to identify influencers in social networks for the various kinds of interactions. As we have known, the graph model is widely utilized to represent social networks, however, it is incapable of dealing with the multiple social links. For example, people use Facebook or WeChat to keep communication with family members or friends, use Twitter to post news, use LinkedIn to search for jobs, and use TikTok to create and share short videos [ 6 ]. It is easy to represent each social scenario via a graph model separately, in spite of they are belonging to the same group of individuals. The neglect of the multiple relationships between social actors may lead to an incorrect result of the most versatile users [ 7 ]. With the proposal of multilayer networks [ 8 , 9 ], we are able to encode the various interactions, which is of great importance and necessity of identifying influencers in multiple social networks.
In this paper, we design a novel node centrality measure for monolayer network, and then apply it to multilayer networks to identify influencers in multiple social networks. This method is solely based on the local knowledge of a network’s topology in order to be fast and scalable due to the huge size of networks, and thus suitable for both real-time applications and offline mining.
The rest of this paper is organized as follows. Section 2 introduces the related works on influencers identification in monolayer network and multilayer networks. Section 3 presents the mathematical model and the method for detecting influencers. Section 4 exhibits the experiments and analysis, including comparison experiments on twenty-one real-world datasets, which verifies the feasibility and veracity of the proposed method. Section 5 summarizes the whole paper and provides concluding remarks.
2. Related Works
The initial research on influencers identification may date back to the study of node centrality, which means to measure how “central” a focal node is [ 10 ]. A plethora of methods for influencers identification are proposed in the past 40 years, which can be mainly classified into centrality measures, link topological ranking measures, entropy measures, and node embedding measures [ 11 , 12 ]. Some of these measures take only the local information into account, while others even employ machine learning methods. Nowadays, it has been one of the most popular research topics and yielded a variety of applications [ 7 ] such as identifying essential proteins and potential drug targets for the survival of the cell [ 13 ], controlling the outbreak of epidemics [ 14 ], preventing catastrophic outages in power grids [ 15 ], driving the network toward a desired state [ 16 ], improving transport capacity [ 17 ], promoting cooperation in evolutionary games [ 18 ], etc. This paper investigates the problem of identifying influencers in social networks, by introducing a family of centrality-like measures and gives a brief comparison in Table 1 .
Classical node centrality metrics comparison.
Degree Centrality (DC) [ 19 ] is the simplest centrality measure, which merely counts how many social connections (i.e., the number of neighbors) a focal node has, defined as
where N is the total number of nodes, a i j is the weight of edge ( i , j ) if i is connected to j , and 0 otherwise. The degree centrality is simple and merely considers the local structure around a focal node [ 20 ]. However, this method is probably mistaken for the negligence of global information, i.e., a node might be in a central position to reach others quickly although it is not holding a large number of neighbors [ 21 ]. Thus, Betweenness Centrality (BC) [ 22 ] is proposed to assess the degree to which a node lies on the shortest path between two other nodes, defined as
where g s t is the total number of shortest paths, g s t ( i ) is the shortest path between s and t that pass through node i . The betweenness centrality considers global information and can be applied to networks with disconnected components. However, there is a great proportion of nodes that do not lie on the shortest path between any two other nodes, thereby the computational result receives the same score of 0. Besides, high computational complexity is also a limitation of applying for large-scale networks. Analogously, Closeness Centrality (CC) [ 23 ] is proposed to represent the inverse sum of shortest distances to all other nodes from a focal node, defined as
where N is the total number of nodes, d i j is the shortest path length from node i to node j . The closeness centrality is capable of measuring the core position of a focal node via the utilization of global shortest path length, while it suffers from the lack of applicability to networks with disconnected components, e.g., if two nodes that belong to different components do not have a finite distance between them, it will be unavailable. Besides, it is also criticized by high computational complexity.
Eigenvector Centrality [ 24 ] (EC) is a positive multiple of the sum of adjacent centralities. Relative scores are assigned to all nodes in a network based on an assumption that connections to high-scoring nodes contribute more to the score of the node than connections to low-scoring nodes, defined as
where k i depicts the eigenvalue of adjacency matrix A , x = k 1 A x depicts the eigenvector stable state of interactions with eigvenvalue k 1 − 1 . This measure considers the number of neighbors and the centrality of neighbors simultaneously, however, it is incapable of dealing with non-cyclical graphs. In 1998, Brin and Page developed the PageRank algorithm [ 25 ], which is the fundamental search engine mechanism of Google. PageRank (PR) is a positive multiple of the sum of adjacent centralities, defined as
where N depicts the total number of nodes, ∑ i = 1 N P R 0 ( i ) = 0 , k j o u t is the number of edges from node j point to i . Likewise, this method is efficient but also criticized by non-convergence in cyclical structures. As we have known, the clustering coefficient [ 26 , 27 ] is a measure of the degree to which nodes in a graph tend to cluster together, defined as
It is widely considered that a node with a higher clustering coefficient may benefit forming communities and enhancing local information spreading. However, Chen et al. expressed contrary views that the local clustering has negative impacts on information spreading. They proposed a ClusterRank algorithm for ranking nodes in large-scale directed networks and verified its superiority to PageRank and LeaderRank [ 28 ]. Therefore, the effect of clustering coefficient on information spreading is uncertain, which may benefit local information spreading but prohibit global (especially directional network) information spreading. In 2016, Ma et al. proposed a gravity centrality [ 29 ] (GR) by considering the interactions comes from the neighbors within three steps, defined as
where k s ( i ) and k s ( j ) are the k -shell index of i and j , respectively. ψ i is the neighborhood set whose distance to node i is less than or equal to 3, d i j is the shortest path length between i and j . These methods consider semi-local knowledge of a focal node, i.e., the neighboring nodes within three steps, which are successful in many real-world datasets, such as Jazz [ 30 ], NS [ 31 ] and USAir network [ 32 ], etc. However, they are also with high computational complexity by globally calculating k -shell. In 2019, Li et al. improved the gravity centrality and proposed a Local-Gravity centrality (LGR) [ 33 ] by replacing k -shell computing and merely considering the neighbors within R steps, defined as
where k i and k j are the degrees of i and j , respectively, d i j is the shortest path length between i and j . This method had been extremely successful in a variety of real-world datasets, however, the parameter R requires the calculating of network diameter, which is also a time-consuming process.
The above-mentioned centrality measures have been utilized to rank nodes’ spreading abilities in monolayer networks. The ranking of nodes in multilayer networks is a more challenging task and is still an open issue. The information propagation process over multiple social networks is more complicated, and conventional models are incapable without any modifications. Zhuang and Yaǧan [ 36 ] proposed a clustered multilayer network model, where all constituent layers are random networks with high clustering to simulate the information propagation process in multiple social networks. Likewise, Basaras et al. [ 37 ] proposed an improved susceptible–infected–recovered (SIR) model with information propagation probability parameters (i.e., λ i i for intralayer connections and λ i j for interlayer connections). Most of the recent endeavors concentrated on the multiplex networks, (e.g., clustering coefficient in multiplex networks [ 38 ]), where all layers share the identical set of nodes but may have multiple types of interactions. Rahmede et al. proposed a MultiRank algorithm [ 39 ] for the weighted ranking of nodes and layers in large multiplex networks. The basic idea is to assign more centrality to nodes that are linked to central nodes in highly influential layers. The layers are more influential if highly central nodes are active in them. Wang et al. proposed a tensor decomposition method (i.e., EDCPTD centrality) [ 7 ], which utilize the fourth-order tensor to represent multilayer networks and identify essential nodes based on CANDECOMP/PARAFAC (CP) tensor decomposition. They also exhibited the superiority to traditional solutions by comparing the performance of the proposed method with the aggregated monolayer networks. In a word, it is of great significance in identifying influencers in multiplex networks. Our purpose in this work is to devise a measure that can accurately detect influential nodes in a general multilayer network.
3. Modeling and Methods
3.1. network modeling.
The problem of finding influential nodes is described as extracting a small set of nodes that can bring the greatest influence on the network dynamics. With a given network model G = ( V , E ) , where V = { v 1 , v 2 , … , v n } is the node set and E = { ( v i , v j ) } , ( v i , v j ∈ V ) is the edge set. The identification of influential nodes is to pick a minimum of nodes as the initial seeds, which can achieve the maximum influenced scope, described as
where A is the initially infected nodes, σ ( A ) denotes the final influenced node set. This problem is simplified as top- k influencers identification by additional setting | A | = k , which has recently attracted great research interests [ 40 , 41 , 42 ]. A variety of real-world social networks are, in fact, interconnected by different types of interactions between nodes, forming what is known as multilayer networks. In this paper, we employ a multilayer network model [ 9 ], which can represent nodes sharing links in different layers. The multilayer network model is defined as
where G = { G α ; α ∈ { 1 , … , L } } is a family of (directed or undirected, weighted or unweighted) graphs G α = ( V α , E α ) , which represents layers of M and C depicts the interactions between nodes of any two different layer, given by
The corresponding supra-adjacency matrix can be represented as
where A 1 , A 2 , … , A L are the adjacency matrix of layer 1 , 2 , … , L , respectively. N is the total number of the nodes, which can be calculated by N = ∑ 1 ≤ l ≤ L | V l | . The non-diagonal block I α β represents the inter-layer edges of layer α and layer β . Thus, the interlayer edges can be represented as
Take the 9/11 terrorists network [ 43 ] for instance, the edges are classified into three categories (i.e., layers) according to the observed interactions which are plotted in Figure 1 .

Network of 9/11 terrorists. ( a ) The monolayer network representation, the size of a node represents its degree; ( b ) The 9/11 terrorists’ interactions represented by a multilayer network model, where L 1 presents confirmed close contact, L 2 layer shows various recorded interactions, L 3 contains potential or planed or unconfirmed interactions; ( c ) The super-adjacency matrix representation.
3.2. Methods
We employ the susceptible–infected–recovered (SIR) spreading model [ 44 ] as the influence analysis model. It has three possible states:
- Susceptible (S) state, where a node is vulnerable to infection.
- Infectious (I) state, where a node tries to infect its susceptible neighbors.
- Recovered (R) state, where a node has recovered (or isolated) and can no longer infect others.
In a network, if two nodes are connected then they are considered to have “contact”. If one node is “infected”, and the other is susceptible, then with a certain probability the latter may become infected through contact [ 45 ]. A node is considered to be recovered if it is isolated or immune to the disease. In detail, to check the spreading influence of one given node, we set this node as an infected node and the other nodes are susceptible nodes. At each time step, each infected node can infect its susceptible neighbors with infection probability β , and then it recovered from the diseases with probability γ , the differential equations are shown in Figure 2 . For simplicity, we set γ = 1 . The process of the SIR model is plotted in Figure 3 and Figure 4 with the famous Krackhardt’s Kite network [ 46 ].

The illustration of parameters in the susceptible–infected–recovered (SIR) model and corresponding differential equations.

The process of SIR model on Krackhardt’s Kite network. In panel ( a ), all the nodes are in Susceptible state; while we select one node to be infected, and the neighbors will be infected soon, as shown in panel ( b ); Finally, the network will reach a stable state, i.e., the number of recovered nodes will reach a maximum, as shown in panel ( c ).

The varying susceptible, infectious and recovered nodes with the increasing of iterations.
In this paper, we define the node influence (INF, for short) as the energy derived from the neighbors, given as
where R is the truncation radius, Γ ( i ) is the set of neighbors of node i , d i j is the shortest path length between node i and node j , k j is the degree of node j , w i j is the weight of edge e i j . For unweighted networks, w i j = 1 . Analogously, we apply the proposed INF measure to multilayer networks (represented as I N F R M ) by the following modifications
where R is the truncation radius, Γ α ( i ) is the set of neighbors of node i at layer α , k j α is the degree of node j at layer α , d i j is the shortest path length between node i and node j . For simplicity, we choose R = 1 , thus d i j = 1 if node i and node j is connected through an intralayer edge or interlayer edge, and 0 otherwise.
To explain the effect, we take the above-mentioned Krackhardt’s Kite network (as plotted in Figure 5 ) and the 9/11 terrorists network (as plotted in Figure 1 ) for examples. The nodes centralities in Krackhardt’s Kite network are shown in Table 2 .

The network structure of Krackhardt’s Kite network.
Classic node centralities comparison of kite network. The maximum centralities are marked in bold.
As shown in Table 2 , Node 4 is considered to be the most important node under the Degree, Katz and the proposed INF measure, while Node 8 has greater Betweenness, Node 6 and node 7 has greater Closeness or (Eigenvector). Thus, the node list (i.e., [4, 6, 7, 8]) is considered to be the influencers. Furthermore, to evaluate the nodes’ influence, we set each node as the initially infected and recorded the final recovered nodes, respectively. This process is repeated for 10,000 times and the results are shown in Table 3 .
Averaging recovered nodes and iterations times of each node as initially infected spreaders under 10,000 times SIR stimulations with parameters setting β = 0.35 , γ = 1.
As shown in Table 3 , Node 4 (i.e., Diane), which is considered to be more influential under Degree, Katz, and INF centrality, shows more recovered nodes (i.e., 5.3182) after 10,000 times SIR stimulations. This experiment is available at https://neusncp.com/api/sir . Analogously, we conduct experiments on the three-layer 9/11 terrorists network. Particularly, we set the infected probability between intralayer edges as β and the probability between interlayer edges as β M = w i j β . The experimental results are plotted in Figure 6 .

The comparison of recovered nodes in 9/11 terrorists network by initially setting every node infected separately. The recovered nodes and the corresponding INF values are normalized, which are marked in cyan and red, respectively. The comparison of the nodes in three layers are plotted in facet L 1 , L 2 and L 3 .
By conducting SIR simulations on the three-layer 9/11 terrorists network, we can obtain the influential nodes of each layer by calculating the number of finally recovered nodes. Afterward, we sort the nodes by the averaging recovered nodes, and compare the order with the results computed from the proposed INF indicator. It is shown in Figure 6 that the compared values (i.e., recovered nodes and INF) are in the same tendency, which verifies the feasibility of the proposed INF measure. Notably, several influential nodes, such as “Essid Sami Ben Khemais”, “Mohamed Atta”, and “Marwan Al-Shehhi” are also in the central position of the network, as shown in Figure 1 a.
The experimental results on the two sample networks show the feasibility of the proposed measure on monolayer and multilayer networks, respectively. Experiments on more real-world networks will be given in Section 4 .
3.3. Complexity Analysis
Suppose m and n are the numbers of edges and nodes, respectively, L is the number of layers, the average degree of nodes is d , R is the truncation radius (commonly setting as R = 1 ). The complexity of INF for monolayer network is O ( n + d R ) . As for multilayer networks, the computational complexity is O ( n + L d R ) , where L is also a small positive integer. Thus, the time complexity is acceptable as O ( n + L d ) . Overall, the proposed measure considers more neighboring information than the degree centrality and has a lower computational complexity than betweenness centrality and closeness centrality (i.e., O ( n m + n 2 log n ) ).
4. Experiments and Discussion
The experimental environment was with Intel(R) Core (TM) i5-7200U CPU @ 2.50 GHz (4 CPUs), 2.7 GHz, the memory was 8 GB DDR3. The operating system was Windows 10 64 bit, the programming language was Python 3.7.1, and the relevant libs were NetworkX 2.2 and Multinetx. The goal of the experiments was to compare the performance of the proposed INF measure with competitive indicators.
4.1. Experimental Datasets
In this paper, 21 real-world datasets were employed to verify the performance of the proposed method, which were classified into two groups. The first group covered 12 monolayer networks, which comprised four social networks (i.e., Club, Dolphins, 911 and Lesmis), three biological networks (i.e., Escherichia, C.elegans and DMLC), collaboration networks (i.e., Jazz and NS), a communication network (i.e., Eron), a power network (i.e., Power) and a transport network (i.e., USAir), as shown in Table 4 .
Statistics of 12 real-world monolayer networks.
Note: | V | and | E | denotes the number of nodes and edges, respectively. < k > is the average degree; < d > is the average shortest path length; | C | is the average clustering index; < r > is the assortativity coefficient; | H | is the degree heterogeneity and β c represents the epidemic threshold of the SIR model. Club contains the friendships between the 34 members of a karate club at a US university. Dolphins dataset is a animals social network. 911 represents a monolayer terrorist network of September 11 attacks. Lesmis is the coappearance network of characters in the novel Les Miserables. Escherichia represetns transcriptional regulation networks in cells orchestrate gene expression, where nodes are operons, and each edge is directed from an operon that encodes a transcription factor to an operon that it directly regulates (an operon is one or more genes transcribed on the same mRNA). Eron is a email network collected from Eron company. Jazz lists the collaboration patterns of jazz musicians. USAir is an undirected weighted network as obtained by considering the 500 US airports with the largest amount of traffic from publicly available data. Nodes represent US airports and edges represent air travel connections among them. NS represents coauthorships between 379 scientists whose research centers on the properties of networks of one kind or another. C.elegans represents the edges of the metabolic network of C.elegans. DMLC represents the inferred Links by small/medium-scale rotein-protein interactions (collected from protein-protein interaction data bases). Power is a power grid of the western United States.
The second group covered nine multilayer networks, which comprised six social networks (i.e., Padgett, Krackhardt, Vickers, Kapferer, Lazega and CS-Aarhus), two transport networks (i.e., LondonTransport and EUAirTransportation) and a biological network (i.e., humanHIV), as shown in Table 5 . Data availability: http://www.neusncp.com/user/file?id=12&code=data .
Statistics of nine real-world multilayer networks.
Note: | L | denotes the number of layers; | V | and | E | are the total number of nodes and edges, respectively; | E A | and | E C | denote the number of intralayer edges and interlayer edges, respectively. < k > is the average degree; < d > is the average shortest path length; | C | is the average clustering index; Padgett consists of 2 layers (marriage alliances and business relationships) describing florentine families in the Renaissance; Krackhardt consists of 3 kinds of relationships (Advice, Friendship and “Reports to”) between managers of a high-tech company; Vickers is collected by Vickers from 29 seventh grade students in a school in Victoria, Australia. Students are asked to nominate their classmates on a number of three kinds of relations; Kapferer exhibits interactions in a tailor shop in Zambia (then Northern Rhodesia) over a period of ten months, where layers represent two different types of interaction, recorded at two different times (seven months apart) over a period of one month; Lazega consists of three kinds of interactions (Co-work, Friendship and Advice) between partners and associates of a corporate law partnership; humanHIV1 represents the multiplex genetic and protein interactions network of the human HIV type 1; CS-Aarhus consists of five kinds of online and offline relationships (Facebook, Leisure, Work, Co-authorship, Lunch) between the employees of Computer Science department at Aarhus; LondonTransport is collected from the official website of Transport for London ( https://www.tfl.gov.uk/ ). Nodes are train stations in London and edges encode existing routes between stations; EUAirTransportation is composed by thirty-seven different layers each one corresponding to a different airline operating in Europe.
4.2. Performance Comparison
To verify the performance of the proposed node influence in networks, this paper carries out a comparison experiment on the above-mentioned datasets: The nodes were removed by a certain indicator in descending order, and the number of subgraphs was recorded. This process repeated until there were not any nodes left. The varying tendency of the subgraphs’ number exhibited the influence of a focal centrality. The experimental results are shown in Figure 7 .

The varying subgraphs of 12 monolayer networks with the removal of the most influential nodes repeatedly with each centrality indicator.
As shown in Figure 7 , with the nodes removing, the number of subgraphs was increasing and reached a maximum when the network was totally broken up, i.e., there were no edges at this moment. Afterward, the number of subgraphs (i.e., the number of nodes) was decreasing and finally reached zero when all the nodes were removed. The maximum numbers of subgraphs were obtained by the proposed INF measure on all the datasets except C.Elegans. However, the result of C.Elegans obtained by INF was very close to the best situation of BC, which suggests the feasibility of the proposed INF measure.
We applied the SIR model to compare the rankings of influences calculated by each indicator among the above-mentioned networks. Initially, one node was set as “infected” state to infect its neighbors with probability β . Afterward, the infected nodes were recovered and never be infected again with probability γ . This spreading process repeated until there were no more infected nodes in the network. The influence of any node i can be estimated by
where N R is the number of recovered nodes after the spreading process, and N is the total number of nodes in the network. For simplicity, we set γ = 1 and the epidemic threshold was
After having obtained the standard nodes’ influence sequence via SIR model simulations, we employed the Kendall’s Tau coefficient [ 65 ] to compare the performance of each indicator. The Kendall’s Tau coefficient is an index measuring the correlation strength between two sequences. Suppose given the standard sequence X = ( x 1 , x 2 , … , x N ) , and we obtained the computational sequence Y = ( y 1 , y 2 , … , y N ) by a certain indicator. Any pair of two-tuples ( x i , y i ) and ( x j , y j ) ( x ≠ j ) are concordant if both x i > x j and y i > y j or x i < x j and y i < y j . Meanwhile, they are considered as discordant, if x i > x j and y i < y j or x i < x j and y i > y j . If x i = x j or y i = y j , pairs are neither concordant nor discordant. Therefore, Kendall’s Tau coefficient is defined as
where N c and N d indicate the number of concordant and discordant pairs, respectively. The range of τ is [ − 1 , 1 ] . Table 6 shows the computational Tau results with the comparison of standard sequence from SIR model simulations.
The indicators’ accuracies measured by the Kendall’s Tau ( τ ).
Note: Given a network, the parameters of SIR model are given with the transmission probability β = 0.35 and recovering probability μ = 1 for simplicity. To obtain the standard ranking of nodes’ influences, we conducted 1000 independent simulations, in each process every node is selected once as the infect seed once. The best perfromed indicator for each network is emphasized by bold.
As shown in Table 6 , the proposed measure outperformed the competitors in most cases, even in the Escherichia network, the computed Tau result of INF (0.0692) was close to that of CC (0.0971). Thus, it was also competitive in this network.
If setting the limitation of identifying k influencers, we conducted experiments on the real-world datasets with top- k nodes by computational centrality nodes and compared the recovered nodes (i.e., the final number of nodes with recovered states). To compare the varying parameter k with the obtained τ , we conducted experiments on the above-mentioned datasets and set the ratio of β / β c , as shown in Figure 8 .

The algorithms’ performance comparison for varying k (ranging from 0 to 20 percent of total nodes), measured by the recovered nodes. The betweenness centrality and the proposed INF measure are very competitive than the others.
As shown in Figure 8 , the proposed node influence method is quite competitive in most of the datasets, although second to the performance of betweenness indicator in DMLC and Jazz datasets.
Analogously, we conducted experiments on the nine multilayer networks by removing nodes with maximum centralities; the results are plotted in Figure 9 .

The computational time comparison of different indicators. The accumulation of running time on the 12 real-world datasets has exhibited that the proposed INF measure is much more efficient than the competitors.
To compare the time complexity of the proposed INF measure with classic methods, the runtime of 21 networks are recorded and shown in Figure 10 .

The runtime comparison of each indicator.
As shown in Figure 10 , the runtime accumulated from either group indicated that the proposed INF measure was efficient, which was close to that of DC and superior to BC, CC and LGR.
4.3. Discussion
Influencers identification is a fundamental issue with wide applications in different fields of reality, such as epidemic control, information diffusion, viral marketing, etc. Currently, degree centrality [ 19 ] is the simplest method, which considers nodes with larger degrees are more influential. However, for the lack of global information, a node lying in a “bridge” position might be neglected for holding a small degree. The betweenness [ 22 ] and closeness [ 23 ] centrality consider global information, but they are holding a high complexity, which are not suitable for applications in large-scale networks. Local gravity is a balanced method, however, the determination of parameter R requires computing network diameter, which is also time-consuming. Thus, a novel node influence measure is proposed in this paper, which merely considers the local neighboring information of a focal node with the complexity of O ( n + L d ) . Experimental results on 21 real-world datasets indicate the feasibility of the proposed measure.
Firstly, the experiments of counting subgraphs with removing influential nodes show that the capability of the proposed INF measure. By removing the nodes according to the INF indicator, the networks are more easily broken up, as shown in Figure 7 and Figure 9 . Secondly, we apply the SIR model to evaluate the node influence, which suggested the proposed INF measure is competitive to other indicators in most cases. Although inferior to BC on Jazz and DMLC networks, it is also competitive. By analyzing the structures of these two networks, we find that the nodes of Jazz network are densely connected (i.e., the average degree of 27.6970) and most of the nodes are holding the same number of neighbors (approximately 28 neighbors), which brings difficulties to identify which node is more influential. On the contrary, there is only one node (i.e., Node 2) holding a large number of neighbors (i.e., 439 neighbors) and the others only holding few neighbors (approximately four neighbors) in DMLC network, which is also difficult to identify influencers. Overall, the proposed method outperforms the other indicators in most cases. Finally, we compare the running time of each indicator on the 21 real-world datasets. Experimental results show the efficiency of the proposed measure.
5. Conclusions
Aiming at solving the problem of identifying influencers in social networks, this paper proposes a novel node influence indicator. This method merely considers the local neighboring information in order to be fast and suitable for applications in large-scale networks. Extensive experiments on 21 real-world datasets are conducted, and the experimental results show that the proposed method outperforms competitors. Afterwards, the time complexity is compared, and we verify the efficiency of the proposed indicator. Overall, the proposed node influence indicator is capable of identifying influencers in social networks. The contribution of this work is likely to benefit many real-world social applications, such as promoting network evolutions, preventing the spreading of rumors, etc.
As part of future works, the influencers in dynamic networks can be further studied by applying the proposed INF measure into a multilayer network model with numerous ordinal layers. The node’s influence can be calculated by accumulating the local neighbors across all the layers. Besides, the effect of layers needs to be taken into consideration. In a word, we hope the findings in this work will help to improve the researches in this promising field.
Acknowledgments
We would like to thank the anonymous reviewers for their careful reading and useful comments that helped us to improve the final version of this paper.
Author Contributions
X.H. designed the method and wrote the original draft; D.C. revised the manuscript; T.R. and D.W. checked the manuscript and made some modifications. All authors have read and agreed to the published version of the manuscript.
This work was partially supported by Liaoning Natural Science Foundation under Grant No. 20170540320, the Doctoral Scientific Research Foundation of Liaoning Province under Grant No. 20170520358, the National Natural Science Foundation of China under Grant No. 61473073, and the Fundamental Research Funds for the Central Universities under Grant Nos. N161702001, N2017010 and N172410005-2.
Conflicts of Interest
The authors declare that there are no conflict of interest regarding the publication of this paper.
Identifying Influencers in Social Networks
Affiliation.
- 1 Software College, Northeastern University, Shenyang 110169, China.
- PMID: 33286224
- PMCID: PMC7516930
- DOI: 10.3390/e22040450
Social network analysis is a multidisciplinary research covering informatics, mathematics, sociology, management, psychology, etc. In the last decade, the development of online social media has provided individuals with a fascinating platform of sharing knowledge and interests. The emergence of various social networks has greatly enriched our daily life, and simultaneously, it brings a challenging task to identify influencers among multiple social networks. The key problem lies in the various interactions among individuals and huge data scale. Aiming at solving the problem, this paper employs a general multilayer network model to represent the multiple social networks, and then proposes the node influence indicator merely based on the local neighboring information. Extensive experiments on 21 real-world datasets are conducted to verify the performance of the proposed method, which shows superiority to the competitors. It is of remarkable significance in revealing the evolutions in social networks and we hope this work will shed light for more and more forthcoming researchers to further explore the uncharted part of this promising field.
Keywords: complex network; multilayer network; node influence; social network analysis.
Grants and funding
- No.20170540320/Liaoning Natural Science Foundation
- No. 20170520358/the Doctoral Scientific Research Foundation of Liaoning Province
- No. 61473073/National Natural Science Foundation of China
- Nos. N161702001, N2017010 and N172410005-2/the Fundamental Research Funds for the Central Universities
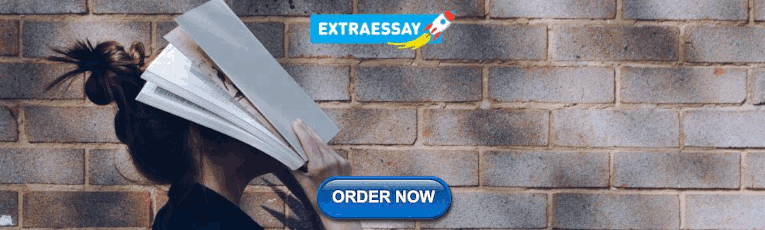
IMAGES
VIDEO
COMMENTS
Considering the ever-increasing size and complexity of social networks, developing methods to extract meaningful knowledge and information from users' vast amounts of data is crucial. Identifying influencers on social networks is one of the essential investigations on these networks and has many applications in marketing, advertising, sociology, behavior analysis, and security issues. In ...
Identifying influencers on social networks is one of the essential investigations on these networks and has many applications in marketing, advertising, sociology, behavior analysis, and security issues. ... Monti D Rizzo G Morisio M A systematic literature review of multicriteria recommender systems Artif Intell Rev 2021 54 1 427 468 Google ...
Systematic literature review on identifying influencers in social networks. June 2023. Artificial Intelligence Review 56 (Suppl 1):1-94. DOI: 10.1007/s10462-023-10515-2. Authors: Seyed Farid ...
In recent years, many studies have been conducted on analyzing and identifying influencers on social networks. Therefore, in this article, a Systematic Literature Review (SLR) has been performed on previous studies about the methods of identifying influencers. To this end, we review the definitions of influencers, the datasets used for ...
Identifying influencers, of which market mavens are a key type, is a fundamental task for marketers. • We have used a range of social media-based metrics to identify market mavens in a dataset combining over half a million Tweets with self-report survey data. • Mavens have more followers, post more often, and use hashtags more often than ...
Discussion. Influencers identification is a fundamental issue with wide applications in different fields of reality, such as epidemic control, information diffusion, viral marketing, etc. Currently, degree centrality [ 19] is the simplest method, which considers nodes with larger degrees are more influential.
Data Profiling and Machine Learning to Identify Influencers from Social Media Platforms. J. ICT Stand. This research aims to find the best machine learning model for characterizing influencers on social media networks by applying the PageRank algorithm to profile influential nodes throughout the social media network graph. Expand.
1. Introduction. With the integration and development of technologies and social networking services, Online Social Networks (OSNs) is becoming the decisive dissemination platform of information, knowledge, technology and other resources [1].However, due to the scale-free property of social networks [2], the diffusion in OSNs hinges on a specific set of users, called influencers, which allows ...
Abstract. Individuals influence each other through social interactions and marketers aim to leverage this interpersonal influence to attract new customers. It still remains a challenge to identify those customers in a social network that have the most influence on their social connections. A common approach to the influence maximization problem ...
This paper employs a general multilayer network model to represent the multiple social networks, and then proposes the node influence indicator merely based on the local neighboring information, which shows superiority to the competitors. Social network analysis is a multidisciplinary research covering informatics, mathematics, sociology, management, psychology, etc. In the last decade, the ...
The emergence of various social networks has greatly enriched our daily life, and simultaneously, it brings a challenging task to identify influencers among multiple social networks. The key problem lies in the various interactions among individuals and huge data scale. Aiming at solving the problem, this paper employs a general multilayer ...
ABSTRACT. In recent years, social networks have become very popular and an integral part of everyday life. People express their feelings and experiences in this virtual environment and become aware of others' opinions and interests. Among them, influential users play an important role in disseminating information on social networks.
Social network analysis is a multidisciplinary research covering informatics, mathematics, sociology, management, psychology, etc. In the last decade, the development of online social media has provided individuals with a fascinating platform of sharing knowledge and interests. The emergence of various social networks has greatly enriched our daily life, and simultaneously, it brings a ...
The research of influencer identification is beneficial to understanding and controlling the spreading. dynamics in social networks with diverse applications such as epidemiology, collective ...
Influence propagation in social networks is a subject of growing interest. A relevant issue in those networks involves the identification of key influencers. These players have an important role on viral marketing strategies and message propagation, including political propaganda and fake news. ... Systematic literature review on identifying ...
In response, this paper is the first to consolidate the present state of research on IM within social media settings. More specifically, a systematic review of relevant studies published in peer-reviewed academic journals across diverse fields was conducted in order to identify key themes and dominant concepts.
Social media influencers are considered a type of endorser who influence and shape. up audience attitudes through blogs, tweets, posts, and the use of other social media. platforms such as ...
posed so far, this study aims to provide an approach to identify the influencers by combining the high-level features extracted from images, regardless of the network structure information and only based on the content of a user's posts. On the other hand, as a popular image-sharing social network, Instagram is
Identifying opinion leaders in social networks Various methods have been suggested in the literature for detection of opinion leaders and the flow of influence. These methods can be grouped into five categories: 1) diffusion-based approaches, 2) graph-based approaches, 3) statistical and stochastic approaches, 4) PageRank-based approaches, and ...
Systematic literature review on identifying influencers in social networks ... X. Social Network Influencers' Data Augmenting Recommender Systems. in 2020 International Conference on Computational Science and Computational Intelligence (CSCI). 2020. ... G., Angelini, S., Gambosi, G., Rossi, G., and Vocca, P. The Analysis of Influential Users ...