- Open access
- Published: 03 November 2021
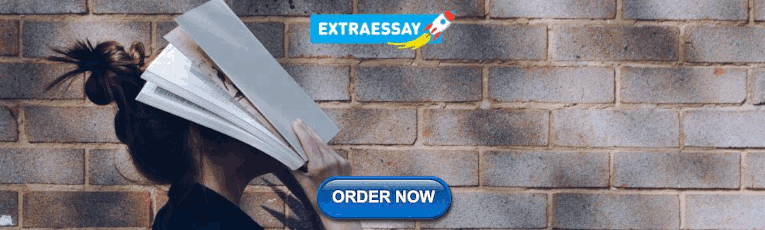
The association between obesity and quality of life: a retrospective analysis of a large-scale population-based cohort study
- J. Stephenson 1 ,
- C. M. Smith 1 ,
- B. Kearns 2 ,
- A. Haywood 2 &
- P. Bissell 1
BMC Public Health volume 21 , Article number: 1990 ( 2021 ) Cite this article
15k Accesses
60 Citations
63 Altmetric
Metrics details
The relationship between obesity and health-related quality of life (HRQoL) may be confounded by factors such as multimorbidity. The aim of the study was to explore this relationship, controlling for long-term conditions and other health, lifestyle and demographic factors in a general adult population. There was specific interest in the impact of high weight status, measured by body mass index (BMI) levels (obesity, morbid obesity) compared with individuals of normal weight.
Health, lifestyle and demographic data were collected from 64,631 individuals aged 16 years and over registered in the Yorkshire Health Study; a long-term cohort study. Data were collected in 2 waves: from patients attending GP surgeries in the South Yorkshire region; and using online recruitment across the entire Yorkshire and Humber area. Univariable and multivariable regression methods were utilised to identify factors associated with HRQoL as measured by the EQ-5D summary score. Long-term conditions were tested as both covariates and mediating factors on the causal pathway between obesity and HRQoL.
Increasing levels of obesity are associated with reduced HRQoL, although this difference is negligible between those of normal weight and those who are overweight. Individuals with obesity and morbid obesity score 4.9 and 11.3 percentage points less on the EQ-5D summary scale respectively than those of normal weight. Concurrent physical, and particularly mental health-related long-term conditions are substantively related to HRQoL: those with 3 or more reported mental or physical health conditions score 29.8 and 14.6 percentage points less on the EQ-5D summary scale respectively than those with fewer conditions. Long-term conditions can be conceptualised as lying on the causal path between obesity and HRQoL, but there is weak evidence for a partial mediating relationship only.
Conclusions
To conclude, in agreement with the established literature we have found a clear inverse relationship between increasing weight status and decreasing HRQoL and confirmed the mediating role of long-term conditions in the reduction of HRQoL in people with obesity. Nevertheless, a high BMI remains independently related to HRQoL, suggesting that ‘healthy people with obesity’ may be in transition to an unhealthy future.
Peer Review reports
Health-related quality of life (HRQoL) is a broad subjective concept that encompasses both physical and mental health, which are themselves in complex relationships with other external factors such as health, socio-economic status, the environment and other factors [ 1 ]. Obesity is a condition of ‘abnormal or excessive fat accumulation that may impair health’, defined by the WHO [ 2 ] as a body mass index (BMI) greater than 30 kg/m 2 , with a BMI of more than 40 kg/m 2 defined as morbid obesity. The aetiology of obesity is complex and multifaceted, stemming from biological, behavioural and environmental causes [ 3 ].
Worldwide obesity has tripled since 1975, and in 2016, 1.9 billion adults (39% of the worldwide adult population) were considered to be overweight: i.e. have a BMI in the range 25 kg/m 2 ≤ BMI < 30 kg/m 2 ; and 650 million (13% of the worldwide population) were considered to have obesity: i.e. have a BMI in the range BMI ≤ 30 kg/m 2 [ 2 ]. In England in 2018, 63% of adults were classified as being overweight or having obesity, with 2 and 4% of men and women respectively being defined as having morbid obesity: i.e. have a BMI in the range BMI ≤ 40 kg/m 2 [ 4 ]. It has been predicted that by 2050 Britain could be a mainly obese society [ 3 ]. Connelly reported a noticeable increase in the proportion of the United Kingdom population at very high risk of chronic disease due to their weight [ 4 ]. Physical associations include long-term health conditions such as Type 2 diabetes, hypertension, dyslipidaemia, coronary artery disease, stroke, various cancers, reduced reproductive function, osteoarthritis, liver and gall bladder disease, chronic pain and adverse respiratory effects [ 3 , 5 , 6 ]. The proportion of individuals reporting long-term conditions (LTCs) has been shown to increase linearly with increasing BMI, and to be independently related to BMI, after adjusting for age and gender [ 7 ]. Similarly, the number of reported LTCs increases with BMI, with 25 and 42% of individuals with moderate and morbid obesity respectively reporting 3 or more LTCs, compared with 12% of normal weight individuals. In addition to physical disease, obesity is also associated with mental health conditions: sleep disorders, anxiety, depression low self-esteem, motivational disorders, eating disorders, impaired body image [ 1 , 8 , 9 , 10 ] and serious psychiatric disorders [ 10 , 11 ].
Obesity is associated with physical, mental and economic consequences. The economic consequences of obesity are substantial and increasing [ 12 ]. In the UK alone it is estimated that by 2050 the societal and business costs of obesity will reach £49.9billion per year [ 3 ]. These costs have been categorised by Seidall [ 13 ] as direct costs from treating obesity and its related diseases; societal costs arising from loss of work due to increased absence, physical limitations, lower life expectancy and unemployment benefits; and personal costs stemming e.g. from stigmatisation and discrimination leading to lower incomes and higher healthcare costs. Physical and mental long-term conditions can impact both on each other and Health Related Quality of Life [ 6 , 14 , 15 , 16 ], and the relationship between obesity and HRQoL can be both mediated and confounded by the presence of comorbidities [ 17 , 18 ] and other effects such as medication [ 11 ] and polypharmacy [ 19 ].
The Yorkshire Health Study (YHS) is an observational cohort study of health and lifestyle in Yorkshire and the Humber [ 20 , 21 ] supported by NIHR CLAHRC (Collaboration for Leadership in Applied Health Research and Care). Adults (aged 16 and over) residing in the in the Yorkshire and Humber region of England are eligible to enter.
The data, from 70,836 adults, was collected in two waves: the first 27,813 were recruited via GP surgeries in South Yorkshire between 2010 and 2012; the second wave of data collection, from 2013 to 2015 utilised online recruitment and the National Clinical Research Network to recruit 43,023 participants. The majority of participants, whether recruited in Waves 1 or 2, completed one survey only. It is well established that there is an inverse relationship between QoL and obesity [ 12 , 17 , 22 , 23 , 24 ]. There are many research studies that demonstrate improved quality of life following both dietary and surgical weight loss [ 25 , 26 , 27 ].
The aim of this study was to utilise a large, contemporary cohort from the UK to explore the relationships between obesity and HRQoL, controlling for LTCs and other health, lifestyle and demographic factors in a general adult population; considering specifically the impact of high levels of BMI (obesity and morbid obesity) in comparison to BMI levels corresponding to individuals of normal weight.
Personal (age, gender, academic history, employment status, socio-economic status, quality of life), health (history of diabetes, physical and mental long-term conditions, frequency of visits to health care professionals, frequency of visits to hospital, days off work due to sickness) and lifestyle (smoking status, weekly levels of walking and exercise) data were collected from participants who responded to either Wave 1 and/or the full version of the questionnaire administered in Wave 2 of the YHS.
HRQoL, as measured by the EQ-5D summary index (measured on a scale from 0 to 1, with higher values representing higher QoL, and derived from scores on individual EQ-5D domains of mobility, self-care, activities, pain and anxiety), was considered to be the outcome measure in the current investigation. The key predictor variable was weight status, measured using BMI, categorised for the purposes of the current investigation as Normal weight (18 kg/m 2 ≤ BMI < 25 kg/m 2 ); Overweight (25 kg/m 2 ≤ BMI < 30 kg/m 2 ), Obese (30 kg/m 2 ≤ BMI < 40 kg/m 2 ), and Morbidly obese (BMI ≥ 40 kg/m 2 ). This variable was collected in both waves of the survey. Individuals with BMI less than 18 kg/m 2 were not included in the analysis, as BMIs in this range may be indicative of illness or eating disorder. An investigation into the relationship between QoL and BMI using the first wave only of the YHS [ 17 ] revealed the relationship to be monotonic and approximately linear in individuals with BMI values of 18 kg/m 2 or more: inclusion of underweight individuals’ results in a curvilinear effect.
Additionally, a number of variables, also collected in one or both waves of the survey, were collected and examined for potential inclusion as covariates in the analysis (Table 1 ). The first mentioned category of the categorical variables above was considered to be the reference category in all cases.
In addition to modelling the LTC variables as covariates in a multiple regression model, these variables were assessed for their effect as mediating variables on the causal pathway between BMI and QoL; in the light of findings by Doll et al. [ 7 ] that the proportion of individuals reporting LTCs, and the number of reported LTCs are significantly predicted by BMI in controlled models.
Physical exercise (including activities such as swimming, playing football, cycling and aerobics) and walking time (including walking to work, to shops and leisure walking) in the week preceding data collection were estimated using the mid-point of options presented as ranges of times (none; 0–1 h per week; 2–3 h per week etc.) offered to respondents as response categories.
The data set was checked before analysis for errors. Any values outside of theoretical or plausible ranges were deleted or replaced with a limiting value as appropriate, with limits for inclusion of BMI values obtained using guidelines. The extent and nature of data missingness was investigated. Missing values were assessed for nature of missingness using Little’s test for data missing completely at random (MCAR) and separate variance t -tests and cross-tabulations. Data missing at random (MAR) was inferred if the MCAR test was statistically significant but missingness could be predicted from variables other than the outcome variable from separate variance t -tests and cross-tabulations. Following verification of missing data on key variables to be MCAR or MAR, complete case analysis was used with respect to both the key predictor variable (weight status as measured by BMI category) and the outcome measure (EQ-5D score) with no imputation conducted on these variables. Controlling variables with more than 5% missing values on remaining cases were dropped from further analysis. Controlling variables with less than 5% missing values that could be shown or inferred to be MCAR or MAR were imputed using expectation maximisation.
The data were summarised descriptively, by weight status (BMI category) and as a full cohort. A series of simple (univariable) regression models were conducted on valid cases, with imputation where necessary and appropriate, considering both the key variable of weight status and each controlling variable in turn as predictors. Controlling variables showing some substantive relationship with the outcome measure were carried forward for inclusion in a subsequent main effects multiple linear regression analysis alongside weight status. Included variables were assessed for collinearity and regression assumptions for the final multiple model were checked post-estimation using residual plots.
Model transferability was assessed by cross-validation. A regression equation was constructed based on a random 80% of cases with model coefficients used to obtain predicted values on the remaining validation sample. The correlation between predicted and actual values in the validation sample was then compared with the corresponding statistic for the main sample; with low or no reductions representing good model transferability.
Ethical approval for the YHS was granted by the NHS Research Ethics Committee (09/H1306/97).
Valid data were collected on 64,631 individuals. Data checking revealed a small proportion of certain variables with implausible or impossible data values. These were investigated on an individual basis and deleted or amended where necessary.
Calculated BMI values of the cleaned data set ranged from 8.32 to 85.9 kg/m 2 ; with a mean value of 26.7 kg/m 2 (SD 5.50 kg/m 2 ). The BMI ranges and corresponding frequencies associated with each original and merged category are summarised in Table 2 .
A summary of participant characteristics (by weight status) before imputation and variable deletion is summarised in Table 3 ; with data based on respondents from whom a valid weight status could be deduced.
While most differences across groups were statistically significant at the 5% significance level, reflecting the large sample size, few substantive differences across groups were observed. Uni-variable tests of significance revealed low effect sizes (measured by the ϕ and partial-η 2 statistics) of less than 5% for most reported variables in the table above. However, some cross-group differences of non-negligible magnitude were observed with respect to gender, diabetes status and academic qualifications. A higher proportion of women than men were in the group with morbid obesity; however, overall mean male BMI (26.9 kg/m 2 ; SD 4.83 kg/m 2 ) was higher than the mean female BMI (26.6 kg/m 2 ; SD 5.84 kg/m 2 ). The proportion of those in the Normal weight group who were qualified to degree level or above was, at 12.1%, more than double that of those in the group with obesity (5.5%) and over 3 times that of those in the group with morbid obesity (3.5%). The proportion of those in the Normal weight group who suffered from 3 or more long-term mental health-related conditions was, at 6.7%, less than half that of those in the group with obesity (15.7%) and less than a third that of those in the group with morbid obesity (24.5%).
Little’s test for MCAR using all quantitative variables with complete or near-complete cases revealed no evidence that missing EQ-5D scores were not MCAR ( p = 0.408). Separate variance t-tests revealed no evidence that missing weight statuses were not MAR. The variables corresponding to diabetes status, employment status, IMD, exercise levels, alcohol consumption and days off work due to sickness were not carried forward for consideration due to excessive proportions of missing values on these variables.
P -values, parameter estimates, associated confidence intervals, and effect sizes (using the partial-η 2 statistic) from a series of univariable regression analyses conducted the outcome measure of EQ-5D score on an imputed data set including the key predictor variable and all controlling variables with complete or near-complete set of cases as identified in Table 3 above, are summarised in Table 4 .
A mediation analysis revealed that both of the variables modelling mental or physical health-related LTCs exhibited some mediating effect on the relationship between weight status and HRQoL. All paths in the mediation models considering weight status as a predictor, and the mental or physical health-related LTCs in turn as mediators were significant. Path coefficients for weight status were revealed to be − 0.010 in a univariable regression of QoL on weight status; − 0.007 in a model including the variable modelling mental health LTCs and − 0.007 in a model including the variable modelling physical health LTCs. Hence while conditions for partial mediation were met, the conditions were full mediation were not met. The substantive mediating effect was low and weight status continued to significantly predict the outcome in the presence of the mediating variable. Hence analysis proceeded with LTCs being modelled as a controlling covariate.
The simple regression models suggested that age, presence/absence of long-term conditions, level of contact with health professions in last 3 months, number of hours per week spent walking, and number of hospital outpatient visits in previous 3 months should be included alongside a weight status category in a multiple model. As strong evidence for statistical significance was expected in most cases due to the size of the data set, assessments for inclusion were made primarily on the basis of effect sizes, with an associated partial-η 2 statistic of about 0.025 or more considered to indicate grounds for inclusion of a particular variable. As the predictor variable of key contextual interest, this did not apply to any of the weight status categories. Model parameters from this multiple model are summarised in Table 5 .
The R 2 and adjusted-R 2 statistics for this model were both 0.390; representing a moderately good fit to the data. No evidence for collinearity was revealed, with variance inflation factors all within tolerable limits. Analysis of residuals revealed no clear evidence for violations of regression assumptions, with normally distributed standardised residuals which exhibited no clear pattern when plotted against standardised predicted values. The model showed very good cross-validation properties, with negligible loss in correlation computed from the validation sample fitted values against predictions from the training sample model coefficients.
Hence controlling for other categorical factors and covariates, compared to individuals in the Normal weight category; HRQoL was essentially the same in individuals in the Overweight category; slightly lower (4.9 percentage points less on the EQ-5D summary index) in individuals in the Obese category and lower (11.3 percentage points less on the EQ-5D summary index) in individuals in the Morbidly obese category. Hence the effect of morbid obesity, compared to normal weight, has approximately the same impact as 3 or more physical long-term conditions or an increase in age of about 55 years. Amongst the controlling variables, those with the greatest substantive effect on QoL were mental and physical health-related LTCs: those with 3 or more mental health conditions scored 29.8 percentage points less on the EQ-5D summary index than those with 2 or fewer conditions; and those with 3 or more physical health conditions scored 14.6 percentage points less on the EQ-5D summary index than those with 2 or fewer conditions. Higher quality of life was also reported by younger people, by those who saw health professionals more infrequently and spent less time visiting hospital as an outpatient, and by those who spent more time walking.
Key findings
The analysis has revealed a clear relationship indicating lower levels of QoL with weight status defined by categories of increasing BMI in individuals with BMIs in the range of 18 kg/m 2 and above. This monotonic decrease in QoL, recorded in groups categorised by increasing BMI, is consistent with both the findings relating to the individual EQ-5D items in the analysis by Kearns et al. [ 17 ] of the first wave of the YHS data, and the wider literature [ 12 , 23 ]. The effect on QoL of weight status category is substantial, particularly for those in the highest BMI category. This reduction in QoL as a result of increasing BMI is greater than that found linked to cancer, myocardial infarction and diabetes, and similar to having schizophrenia, heart failure or kidney failure (Sullivan 2001). However, the EQ-5D summary index is a highly negatively skewed measure, with about one third of our respondents scoring the maximum value of 1.00 and over half of respondents scoring 0.84 or more.
Comparing the estimates and magnitudes thereof of the weight status variables in the simple and multiple models reveals that the effect of weight status is smaller in the multiple (controlled) model. The variables corresponding to mental and physical health-related LTCs in the multiple model appear to be of greater effect on QoL than weight status itself. This may be due to a proportion of the residual variance ascribed to weight status in the simple model being ascribed to other variables in the multiple model; specifically, LTCs, which are already known to be related to weight status from the descriptive analysis and is reflected in the 2007 Sach analysis of BMI and quality of life. It may also reflect the status of obesity as a risk factor for many LTCs [ 3 , 5 , 6 , 7 , 8 ]. However, there are no changes of direction of association of parameter coefficients or substantial changes in parameter estimates or inferences of significance between the models. Further work considering the impact of specific individual conditions may be beneficial.
The mediation analysis reveals that the presence of mental or physical health-related LTCs has a limited partial mediating effect on the underlying relationship between weight status and QoL. In the current analysis, LTCs are analysed as controlling factors. Nonetheless, LTCs can alternatively be conceptualised as lying on the causal path between BMI and QoL [ 1 , 10 , 17 ]; although the direct link between BMI and QoL is stronger and more intuitive. Further model-testing work is needed to establish the existence of, and direction of associations between other constructs represented in the YHS.
The unique contribution of BMI to QoL is consistent with Scottish data [ 18 ] which found an independent relationship between obesity and Quality of Life. This is in contrast to the ‘Healthy Obesity’ hypothesis and may represent a subset of the population ‘in transition’ to unhealthy obesity [ 28 ] via metabolic syndrome, not measured in our study.
The largest unique effect in the multiple model was the presence of 3 or more mental health LTCs. This may be an artefact of the data, explained by a presumed higher likelihood of MH LTCs being related in our sample, compared to the ‘independence’ of the physical domains of LTC. The second biggest effect is degree of contact with a health professional, which we presume is acting as a proxy measure for general health.
Strengths and limitations
The strengths of the YHS are its large sample size which allows for an exploration of detailed obesity categories, comprehensive examination of a wide range of variables, and the use of EQ-5D which measures HRQoL using public preferences.
Most measures captured by the YHS are self-reported and may not be completely reliable; particularly those requiring accurate recall, such as activity levels or levels of contact with healthcare professionals over an extended period of time; or the ability of respondents to distinguish between, for example, hospital visits as an out-patient or day case. The key predictor of BMI requires accurate self-reporting of both height and body weight in appropriate units. In addition, self-reported height and weight are respectively over and underestimated in both men and women (Niedhammer 2000, Spencer 2002, Taylor 2006). In the current study, analysis was restricted to variables which were derived from items elicited in both waves of the questionnaire.
The fit of the multiple regression model to the data, though of moderately high magnitude, may have been constrained in magnitude by uncertainties in the integrity of certain measures and the limited availability of variables for which an acceptable proportion of valid cases were available. Nonetheless, a moderately good fit was obtained and cross-validation procedures revealed that model portability is good; it should be expected that the model will perform equally well on samples other than that from which parameter coefficients were derived.
Implications for future work
This study has demonstrated that further work is needed to establish the existence of, and direction of associations; for example, it seems plausible that not only can factors such as BMI and exercise impact on quality of life (as was assumed in this analysis), but also that variables such as exercise level and BMI are correlated with a plausible association in either direction. A number of models are required to be tested for model fit using, for example, a confirmatory factor analysis approach in order to ensure that an optimal series of relationships are tested.
To conclude, in agreement with the established literature we have found a clear inverse relationship between increasing weight status and decreasing QoL, using a large regional cohort study. We have investigated the influence of other demographic, lifestyle and health related domains on this relationship and confirmed the mediating role of LTCs in the reduction of QoL in people with obesity. Nevertheless, a high weight status remains independently related to QoL, suggesting that the ‘healthy obese’ may be in transition to an unhealthy future.
Availability of data and materials
Anonymised data and details regarding using the resource for recruiting participants to studies can be gathered by contacting Professor Elizabeth Goyder ( [email protected] ). Multi-disciplinary collaboration is strongly encouraged.
Abbreviations
Body mass index
Health-related quality of life
- Quality of life
- Long-term conditions
Taylor VH, Forhan M, Vigod SN, McIntyre RS, Morrison KM. The impact of obesity on quality of life. Best Pract Res Clin Endocrinol Metab. 2013;27(2):139–46. https://doi.org/10.1016/j.beem.2013.04.004 .
Article PubMed Google Scholar
World Health Organization. Obesity and overweight. 2018. Accessed 10 June 2020 http://www.who.int/mediacentre/factsheets/fs311/en/ .
Butland B, Jebb S, Kopelman P, McPherson K, Thomas S, Mardell J, et al. Foresight. Tackling obesities: future choices—project report. London: Government Office for Science; 2007. http://www.foresight.gov.uk .
Google Scholar
Conolly A, Craig S. Health survey for England 2018 - overweight and obesity in adults and children. Leeds: NHS Digital, NHS; 2019.
Chu DT, Nguyet NT, Dinh TC, Lien NV, Nguyen KH, Ngoc VT, et al. An update on physical health and economic consequences of overweight and obesity. Diab Metab Syndr. 2018;12(6):1095–100. https://doi.org/10.1016/j.dsx.2018.05.004 .
Article Google Scholar
Okifuji A, Hare BD. The association between chronic pain and obesity. J Pain Res. 2015;8:399. https://doi.org/10.2147/JPR.S55598 .
Article PubMed PubMed Central Google Scholar
Doll HA, Petersen SE, Stewart-Brown SL. Obesity and physical and emotional well-being: associations between body mass index, chronic illness, and the physical and mental components of the SF-36 questionnaire. Obes Res. 2000;8(2):160–70. https://doi.org/10.1038/oby.2000.17 .
Article CAS PubMed Google Scholar
Chu DT, Nguyet NT, Nga VT, Lien NV, Vo DD, Lien N, et al. An update on obesity: mental consequences and psychological interventions. Diab Metab Syndr. 2019;13(1):155–60. https://doi.org/10.1016/j.dsx.2018.07.015 .
Scott KM, Bruffaerts R, Simon GE, Alonso J, Angermeyer M, De Girolamo G, et al. Obesity and mental disorders in the general population: results from the world mental health surveys. Int J Obes. 2008;32(1):192–200. https://doi.org/10.1038/sj.ijo.0803701 .
Article CAS Google Scholar
Simon GE, Von Korff M, Saunders K, Miglioretti DL, Crane PK, Van Belle G, et al. Association between obesity and psychiatric disorders in the US adult population. Arch Gen Psychiatry. 2006;63(7):824–30. https://doi.org/10.1001/archpsyc.63.7.824 .
Holt RI, Peveler RC. Obesity, serious mental illness and antipsychotic drugs. Diabetes Obes Metab. 2009;11(7):665–79. https://doi.org/10.1111/j.1463-1326.2009.01038.x .
Dixon JB. The effect of obesity on health outcomes. Mol Cell Endocrinol. 2010;316(2):104–8. https://doi.org/10.1016/j.mce.2009.07.008 .
Seidell JC. Societal and personal costs of obesity. Exper Clin Endocrinol Diab. 1998;106(S 02):7–10.
Kearns B, Rafia R, Leaviss J, Preston L, Brazier JE, Palmer S, et al. The cost-effectiveness of changes to the care pathway used to identify depression and provide treatment amongst people with diabetes in England: a model-based economic evaluation. BMC Health Serv Res. 2017;17(1):78. https://doi.org/10.1186/s12913-017-2003-z .
Naylor C, Parsonage M, McDaid D, Knapp M, Fossey M, Galea A: Long-term conditions and mental health. The cost of co-morbidities. The King’s Fund and Centre for Mental Health; 2012. http://www.kingsfund.org.uk/sites/files/kf/field/field_publication_file/long-term-conditions-mental-health-costcomorbidities-naylor-feb12.pdf .
Wu M, Brazier JE, Kearns B, Relton C, Smith C, Cooper CL. Examining the impact of 11 long-standing health conditions on health-related quality of life using the EQ-5D in a general population sample. Eur J Health Econ. 2015;16(2):141–51. https://doi.org/10.1007/s10198-013-0559-z .
Kearns B, Ara R, Young T, Relton C. Association between body mass index and health-related quality of life, and the impact of self-reported long-term conditions–cross-sectional study from the South Yorkshire cohort dataset. BMC Public Health. 2013;13(1):1009. https://doi.org/10.1186/1471-2458-13-1009 .
Ul-Haq Z, Mackay DF, Fenwick E, Pell JP. Impact of metabolic comorbidity on the association between body mass index and health-related quality of life: a Scotland-wide cross-sectional study of 5,608 participants. BMC Public Health. 2012;12(1):143. https://doi.org/10.1186/1471-2458-12-143 .
Silveira EA, Dalastra L, Pagotto V. Polypharmacy, chronic diseases and nutritional markers in community-dwelling older. Revista Brasileira de Epidemiologia. 2014;17(4):818–29. https://doi.org/10.1590/1809-4503201400040002 .
Green MA, Little E, Cooper R, Relton C, Strong M. Investigation of social, demographic and health variations in the usage of prescribed and over-the-counter medicines within a large cohort (South Yorkshire, UK). BMJ Open. 2016;6(9):e012038. https://doi.org/10.1136/bmjopen-2016-012038 .
Stephenson J, Smith CM, Goyder EC, et al. Cohort profile update: the Yorkshire health study. Int J Epidemiology. (in press).
Jia H, Lubetkin EI. The impact of obesity on health-related quality-of-life in the general adult US population. J Public Health. 2005;27(2):156–64. https://doi.org/10.1093/pubmed/fdi025 .
Kolotkin RL, Andersen JR. A systematic review of reviews: exploring the relationship between obesity, weight loss and health-related quality of life. Clin Obes. 2017;7(5):273–89. https://doi.org/10.1111/cob.12203 .
Article CAS PubMed PubMed Central Google Scholar
Sach TH, Barton GR, Doherty M, Muir KR, Jenkinson C, Avery AJ. The relationship between body mass index and health-related quality of life: comparing the EQ-5D, EuroQol VAS and SF-6D. Int J Obes. 2007;31(1):189–96. https://doi.org/10.1038/sj.ijo.0803365 .
Carson TL, Hidalgo B, Ard JD, Affuso O. Dietary interventions and quality of life: a systematic review of the literature. J Nutr Educ Behav. 2014;46(2):90–101. https://doi.org/10.1016/j.jneb.2013.09.005 .
Dixon JB, Dixon ME, O’Brien PE. Quality of life after lap-band placement: influence of time, weight loss, and comorbidities. Obes Res. 2001;9(11):713–21. https://doi.org/10.1038/oby.2001.96 .
Hachem A, Brennan L. Quality of life outcomes of bariatric surgery: a systematic review. Obes Surg. 2016;26(2):395–409. https://doi.org/10.1007/s11695-015-1940-z .
Smith GI, Mittendorfer B, Klein S. Metabolically healthy obesity: facts and fantasies. J Clin Investig. 2019;129(10):3978–89. https://doi.org/10.1172/JCI129186 .
Relton C, Bissell P, Smith C, Blackbrun J, Cooper CL, Nicholl J, et al. South Yorkshire cohort: a ‘cohort trials facility’ study of health and weight - protocol for the recruitment phase. BMC Public Health. 2011;11(1):640. https://doi.org/10.1186/1471-2458-11-640 .
Download references
Acknowledgements
This report is independent research funded by the National Institute for Health Research (NIHR) Collaborations for Leadership in Applied Health Research and Care (CLAHRC) Yorkshire and Humber. The views expressed in this publication are those of the authors and not necessarily those of the Yorkshire Health Study Management Team or Steering Committee, National Institute for Health Research or the Department of Health and Social Care.
This work was supported by the National Institute for Health Research (NIHR) Collaborations for Leadership in Applied Health Research and Care (CLAHRC) Yorkshire and Humber (NIHR200166). Analysis was supported by the Universities of Huddersfield and Sheffield.
Author information
Authors and affiliations.
School of Human & Health Sciences, University of Huddersfield, Queensgate, Huddersfield, HD1 3DH, UK
J. Stephenson, C. M. Smith & P. Bissell
School of Health and Related Research, University of Sheffield, Sheffield, UK
B. Kearns & A. Haywood
You can also search for this author in PubMed Google Scholar
Contributions
PB, AH, CS and BK had the original concept. JS and CS designed the work. All authors agreed the methodology. JS performed the statistical analyses. All authors interpreted the results. CS and JS drafted the manuscript. All authors fed back comments. All authors read and approved the final manuscript.
Corresponding author
Correspondence to J. Stephenson .
Ethics declarations
Ethics approval and consent to participate.
The protocol for the South Yorkshire Cohort (the early name for the Yorkshire Health Study) was approved by the NHS Research Ethics Committee on 27 April 2010 (09/H1306/97). All methods were carried out in accordance with relevant guidelines and regulations. Informed consent was obtained from all participants based on the principle of ‘patient-centred informed consent’ i.e. where patient information and consent aim to replicate that of real world routine healthcare rather than conform to the needs of standard trial designs. Therefore all cohort patients consented to provide observational data at the outset, be contacted again, and for their information to be used to look at the benefit of healthcare treatments; however, consent to “try” a particular intervention in the future was sought only from those offered that intervention. This method of obtaining consent replicates the ‘patient-centred’ information and consent procedures that exist in routine health care, where clinicians provide patients with the information they need, at the time they need it. The consent procedure is described fully in the South Yorkshire Cohort Protocol [ 29 ]. Research on human data was performed in accordance with the Declaration of Helsinki.
Consent for publication
Not applicable.
Competing interests
The authors declare that they have no competing interests.
Additional information
Publisher’s note.
Springer Nature remains neutral with regard to jurisdictional claims in published maps and institutional affiliations.
Rights and permissions
Open Access This article is licensed under a Creative Commons Attribution 4.0 International License, which permits use, sharing, adaptation, distribution and reproduction in any medium or format, as long as you give appropriate credit to the original author(s) and the source, provide a link to the Creative Commons licence, and indicate if changes were made. The images or other third party material in this article are included in the article's Creative Commons licence, unless indicated otherwise in a credit line to the material. If material is not included in the article's Creative Commons licence and your intended use is not permitted by statutory regulation or exceeds the permitted use, you will need to obtain permission directly from the copyright holder. To view a copy of this licence, visit http://creativecommons.org/licenses/by/4.0/ . The Creative Commons Public Domain Dedication waiver ( http://creativecommons.org/publicdomain/zero/1.0/ ) applies to the data made available in this article, unless otherwise stated in a credit line to the data.
Reprints and permissions
About this article
Cite this article.
Stephenson, J., Smith, C.M., Kearns, B. et al. The association between obesity and quality of life: a retrospective analysis of a large-scale population-based cohort study. BMC Public Health 21 , 1990 (2021). https://doi.org/10.1186/s12889-021-12009-8
Download citation
Received : 08 March 2021
Accepted : 21 September 2021
Published : 03 November 2021
DOI : https://doi.org/10.1186/s12889-021-12009-8
Share this article
Anyone you share the following link with will be able to read this content:
Sorry, a shareable link is not currently available for this article.
Provided by the Springer Nature SharedIt content-sharing initiative
BMC Public Health
ISSN: 1471-2458
- General enquiries: [email protected]
Thank you for visiting nature.com. You are using a browser version with limited support for CSS. To obtain the best experience, we recommend you use a more up to date browser (or turn off compatibility mode in Internet Explorer). In the meantime, to ensure continued support, we are displaying the site without styles and JavaScript.
- View all journals
- Explore content
- About the journal
- Publish with us
- Sign up for alerts
- Review Article
- Published: 27 February 2019
Obesity: global epidemiology and pathogenesis
- Matthias Blüher 1
Nature Reviews Endocrinology volume 15 , pages 288–298 ( 2019 ) Cite this article
56k Accesses
2510 Citations
984 Altmetric
Metrics details
- Epidemiology
- Health policy
- Pathogenesis
The prevalence of obesity has increased worldwide in the past ~50 years, reaching pandemic levels. Obesity represents a major health challenge because it substantially increases the risk of diseases such as type 2 diabetes mellitus, fatty liver disease, hypertension, myocardial infarction, stroke, dementia, osteoarthritis, obstructive sleep apnoea and several cancers, thereby contributing to a decline in both quality of life and life expectancy. Obesity is also associated with unemployment, social disadvantages and reduced socio-economic productivity, thus increasingly creating an economic burden. Thus far, obesity prevention and treatment strategies — both at the individual and population level — have not been successful in the long term. Lifestyle and behavioural interventions aimed at reducing calorie intake and increasing energy expenditure have limited effectiveness because complex and persistent hormonal, metabolic and neurochemical adaptations defend against weight loss and promote weight regain. Reducing the obesity burden requires approaches that combine individual interventions with changes in the environment and society. Therefore, a better understanding of the remarkable regional differences in obesity prevalence and trends might help to identify societal causes of obesity and provide guidance on which are the most promising intervention strategies.
Obesity prevalence has increased in pandemic dimensions over the past 50 years.
Obesity is a disease that can cause premature disability and death by increasing the risk of cardiometabolic diseases, osteoarthritis, dementia, depression and some types of cancers.
Obesity prevention and treatments frequently fail in the long term (for example, behavioural interventions aiming at reducing energy intake and increasing energy expenditure) or are not available or suitable (bariatric surgery) for the majority of people affected.
Although obesity prevalence increased in every single country in the world, regional differences exist in both obesity prevalence and trends; understanding the drivers of these regional differences might help to provide guidance for the most promising intervention strategies.
Changes in the global food system together with increased sedentary behaviour seem to be the main drivers of the obesity pandemic.
The major challenge is to translate our knowledge of the main causes of increased obesity prevalence into effective actions; such actions might include policy changes that facilitate individual choices for foods that have reduced fat, sugar and salt content.
This is a preview of subscription content, access via your institution
Access options
Access Nature and 54 other Nature Portfolio journals
Get Nature+, our best-value online-access subscription
24,99 € / 30 days
cancel any time
Subscribe to this journal
Receive 12 print issues and online access
195,33 € per year
only 16,28 € per issue
Buy this article
- Purchase on Springer Link
- Instant access to full article PDF
Prices may be subject to local taxes which are calculated during checkout
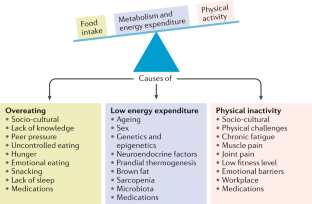
Similar content being viewed by others
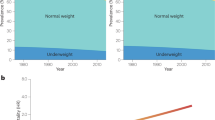
Obesity and the risk of cardiometabolic diseases
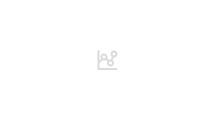
Obesity: a 100 year perspective
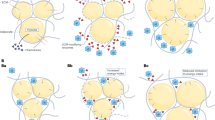
Obesity-induced and weight-loss-induced physiological factors affecting weight regain
World Health Organization. Noncommunicable diseases progress monitor, 2017. WHO https://www.who.int/nmh/publications/ncd-progress-monitor-2017/en/ (2017).
Fontaine, K. R., Redden, D. T., Wang, C., Westfall, A. O. & Allison, D. B. Years of life lost due to obesity. JAMA 289 , 187–193 (2003).
PubMed Google Scholar
Berrington de Gonzalez, A. et al. Body-mass index and mortality among 1.46 million white adults. N. Engl. J. Med. 363 , 2211–2219 (2010).
CAS PubMed Google Scholar
Prospective Studies Collaboration. Body-mass index and cause-specific mortality in 900000 adults: collaborative analyses of 57 prospective studies. Lancet 373 , 1083–1096 (2009).
PubMed Central Google Scholar
Woolf, A. D. & Pfleger, B. Burden of major musculoskeletal conditions. Bull. World Health Organ. 81 , 646–656 (2003).
PubMed PubMed Central Google Scholar
Bray, G. A. et al. Obesity: a chronic relapsing progressive disease process. A position statement of the World Obesity Federation. Obes. Rev. 18 , 715–723 (2017).
World Health Organization. Obesity and overweight. WHO https://www.who.int/mediacentre/factsheets/fs311/en/ (2016).
World Health Organization. Political declaration of the high-level meeting of the general assembly on the prevention and control of non-communicable diseases. WHO https://www.who.int/nmh/events/un_ncd_summit2011/political_declaration_en.pdf (2012).
Franco, M. et al. Population-wide weight loss and regain in relation to diabetes burden and cardiovascular mortality in Cuba 1980-2010: repeated cross sectional surveys and ecological comparison of secular trends. BMJ 346 , f1515 (2013).
Swinburn, B. A. et al. The global obesity pandemic: shaped by global drivers and local environments. Lancet 378 , 804–814 (2011).
Yanovski, J. A. Obesity: Trends in underweight and obesity — scale of the problem. Nat. Rev. Endocrinol. 14 , 5–6 (2018).
Heymsfield, S. B. & Wadden, T. A. Mechanisms, pathophysiology, and management of obesity. N. Engl. J. Med. 376 , 254–266 (2017).
Murray, S., Tulloch, A., Gold, M. S. & Avena, N. M. Hormonal and neural mechanisms of food reward, eating behaviour and obesity. Nat. Rev. Endocrinol. 10 , 540–552 (2014).
Farooqi, I. S. Defining the neural basis of appetite and obesity: from genes to behaviour. Clin. Med. 14 , 286–289 (2014).
Google Scholar
Anand, B. K. & Brobeck, J. R. Hypothalamic control of food intake in rats and cats. Yale J. Biol. Med. 24 , 123–140 (1951).
CAS PubMed PubMed Central Google Scholar
Zhang, Y. et al. Positional cloning of the mouse obese gene and its human homologue. Nature 372 , 425–432 (1994).
Coleman, D. L. & Hummel, K. P. Effects of parabiosis of normal with genetically diabetic mice. Am. J. Physiol. 217 , 1298–1304 (1969).
Farooqi, I. S. & O’Rahilly, S. 20 years of leptin: human disorders of leptin action. J. Endocrinol. 223 , T63–T70 (2014).
Börjeson, M. The aetiology of obesity in children. A study of 101 twin pairs. Acta Paediatr. Scand. 65 , 279–287 (1976).
Stunkard, A. J., Harris, J. R., Pedersen, N. L. & McClearn, G. E. The body-mass index of twins who have been reared apart. N. Engl. J. Med. 322 , 1483–1487 (1990).
Montague, C. T. et al. Congenital leptin deficiency is associated with severe early-onset obesity in humans. Nature 387 , 903–908 (1997).
Farooqi, I. S. et al. Effects of recombinant leptin therapy in a child with congenital leptin deficiency. N. Engl. J. Med. 341 , 879–884 (1999).
Clément, K. et al. A mutation in the human leptin receptor gene causes obesity and pituitary dysfunction. Nature 392 , 398–401 (1998).
Farooqi, I. S. et al. Dominant and recessive inheritance of morbid obesity associated with melanocortin 4 receptor deficiency. J. Clin. Invest. 106 , 271–279 (2000).
Krude, H. et al. Severe early-onset obesity, adrenal insufficiency and red hair pigmentation caused by POMC mutations in humans. Nat. Genet. 19 , 155–157 (1998).
Hebebrand, J., Volckmar, A. L., Knoll, N. & Hinney, A. Chipping away the ‘missing heritability’: GIANT steps forward in the molecular elucidation of obesity - but still lots to go. Obes. Facts 3 , 294–303 (2010).
Speliotes, E. K. et al. Association analyses of 249,796 individuals reveal 18 new loci associated with body mass index. Nat. Genet. 42 , 937–948 (2010).
Sharma, A. M. & Padwal, R. Obesity is a sign - over-eating is a symptom: an aetiological framework for the assessment and management of obesity. Obes. Rev. 11 , 362–370 (2010).
Berthoud, H. R., Münzberg, H. & Morrison, C. D. Blaming the brain for obesity: integration of hedonic and homeostatic mechanisms. Gastroenterology 152 , 1728–1738 (2017).
Government Office for Science. Foresight. Tackling obesities: future choices – project report. GOV.UK https://assets.publishing.service.gov.uk/government/uploads/system/uploads/attachment_data/file/287937/07-1184x-tackling-obesities-future-choices-report.pdf (2007).
World Health Organization. International Statistical Classification of Diseases and Related Health Problems 10th revision. WHO http://apps.who.int/classifications/icd10/browse/2010/en (2010).
Hebebrand, J. et al. A proposal of the European Association for the Study of Obesity to improve the ICD-11 diagnostic criteria for obesity based on the three dimensions. Obes. Facts 10 , 284–307 (2017).
Ramos Salas, X. et al. Addressing weight bias and discrimination: moving beyond raising awareness to creating change. Obes. Rev. 18 , 1323–1335 (2017).
Sharma, A. M. et al. Conceptualizing obesity as a chronic disease: an interview with Dr. Arya Sharma. Adapt. Phys. Activ Q. 35 , 285–292 (2018).
Hebebrand, J. et al. “Eating addiction”, rather than “food addiction”, better captures addictive-like eating behavior. Neurosci. Biobehav. Rev. 47 , 295–306 (2014).
Phelan, S. M. et al. Impact of weight bias and stigma on quality of care and outcomes for patients with obesity. Obes. Rev. 16 , 319–326 (2015).
Kushner, R. F. et al. Obesity coverage on medical licensing examinations in the United States. What is being tested? Teach Learn. Med. 29 , 123–128 (2017).
NCD Risk Factor Collaboration (NCD-RisC). Worldwide trends in body-mass index, underweight, overweight, and obesity from 1975 to 2016: a pooled analysis of 2416 population-based measurement studies in 128.9 million children, adolescents, and adults. Lancet 390 , 2627–2642 (2017).
NCD Risk Factor Collaboration (NCD-RisC). Trends in adult body-mass index in 200 countries from 1975 to 2014: a pooled analysis of 1698 population-based measurement studies with 19.2 million participants. Lancet 387 , 1377–1396 (2016).
Organisation for Economic Co-operation and Development. Obesity update 2017. OECD https://www.oecd.org/els/health-systems/Obesity-Update-2017.pdf (2017).
Geserick, M. et al. BMI acceleration in early childhood and risk of sustained obesity. N. Engl. J. Med. 379 , 1303–1312 (2018).
Ezzati, M. & Riboli, E. Behavioral and dietary risk factors for noncommunicable diseases. N. Engl. J. Med. 369 , 954–964 (2013).
Kleinert, S. & Horton, R. Rethinking and reframing obesity. Lancet 385 , 2326–2328 (2015).
Roberto, C. A. et al. Patchy progress on obesity prevention: emerging examples, entrenched barriers, and new thinking. Lancet 385 , 2400–2409 (2015).
Lundborg, P., Nystedt, P. & Lindgren, B. Getting ready for the marriage market? The association between divorce risks and investments in attractive body mass among married Europeans. J. Biosoc. Sci. 39 , 531–544 (2007).
McCabe, M. P. et al. Socio-cultural agents and their impact on body image and body change strategies among adolescents in Fiji, Tonga, Tongans in New Zealand and Australia. Obes. Rev. 12 , 61–67 (2011).
Hayashi, F., Takimoto, H., Yoshita, K. & Yoshiike, N. Perceived body size and desire for thinness of young Japanese women: a population-based survey. Br. J. Nutr. 96 , 1154–1162 (2006).
Hardin, J., McLennan, A. K. & Brewis, A. Body size, body norms and some unintended consequences of obesity intervention in the Pacific islands. Ann. Hum. Biol. 45 , 285–294 (2018).
Monteiro, C. A., Conde, W. L. & Popkin, B. M. Income-specific trends in obesity in Brazil: 1975–2003. Am. J. Public Health 97 , 1808–1812 (2007).
Mariapun, J., Ng, C. W. & Hairi, N. N. The gradual shift of overweight, obesity, and abdominal obesity towards the poor in a multi-ethnic developing country: findings from the Malaysian National Health and Morbidity Surveys. J. Epidemiol. 28 , 279–286 (2018).
Gebrie, A., Alebel, A., Zegeye, A., Tesfaye, B. & Ferede, A. Prevalence and associated factors of overweight/ obesity among children and adolescents in Ethiopia: a systematic review and meta-analysis. BMC Obes. 5 , 19 (2018).
Rokholm, B., Baker, J. L. & Sørensen, T. I. The levelling off of the obesity epidemic since the year 1999 — a review of evidence and perspectives. Obes. Rev. 11 , 835–846 (2010).
Hauner, H. et al. Overweight, obesity and high waist circumference: regional differences in prevalence in primary medical care. Dtsch. Arztebl. Int. 105 , 827–833 (2008).
Myers, C. A. et al. Regional disparities in obesity prevalence in the United States: a spatial regime analysis. Obesity 23 , 481–487 (2015).
Wilkinson, R. G. & Pickett, K. The Spirit Level: Why More Equal Societies Almost Always Do Better 89–102 (Bloomsbury Press London, 2009).
Sarget, M. Why inequality is fatal. Nature 458 , 1109–1110 (2009).
Plachta-Danielzik, S. et al. Determinants of the prevalence and incidence of overweight in children and adolescents. Public Health Nutr. 13 , 1870–1881 (2010).
Bell, A. C., Ge, K. & Popkin, B. M. The road to obesity or the path to prevention: motorized transportation and obesity in China. Obes. Res. 10 , 277–283 (2002).
Ludwig, J. et al. Neighborhoods, obesity, and diabetes — a randomized social experiment. N. Engl. J. Med. 365 , 1509–1519 (2011).
Beyerlein, A., Kusian, D., Ziegler, A. G., Schaffrath-Rosario, A. & von Kries, R. Classification tree analyses reveal limited potential for early targeted prevention against childhood overweight. Obesity 22 , 512–517 (2014).
Reilly, J. J. et al. Early life risk factors for obesity in childhood: cohort study. BMJ 330 , 1357 (2005).
Kopelman, P. G. Obesity as a medical problem. Nature 404 , 635–643 (2000).
CAS Google Scholar
Bouchard, C. et al. The response to long-term overfeeding in identical twins. N. Engl. J. Med. 322 , 1477–1482 (1990).
Sadeghirad, B., Duhaney, T., Motaghipisheh, S., Campbell, N. R. & Johnston, B. C. Influence of unhealthy food and beverage marketing on children’s dietary intake and preference: a systematic review and meta-analysis of randomized trials. Obes. Rev. 17 , 945–959 (2016).
Gilbert-Diamond, D. et al. Television food advertisement exposure and FTO rs9939609 genotype in relation to excess consumption in children. Int. J. Obes. 41 , 23–29 (2017).
Frayling, T. M. et al. A common variant in the FTO gene is associated with body mass index and predisposes to childhood and adult obesity. Science 316 , 889–894 (2007).
Loos, R. J. F. & Yeo, G. S. H. The bigger picture of FTO-the first GWAS-identified obesity gene. Nat. Rev. Endocrinol. 10 , 51–61 (2014).
Wardle, J. et al. Obesity associated genetic variation in FTO is associated with diminished satiety. J. Clin. Endocrinol. Metab. 93 , 3640–3643 (2008).
Tanofsky-Kraff, M. et al. The FTO gene rs9939609 obesity-risk allele and loss of control over eating. Am. J. Clin. Nutr. 90 , 1483–1488 (2009).
Hess, M. E. et al. The fat mass and obesity associated gene (Fto) regulates activity of the dopaminergic midbrain circuitry. Nat. Neurosci. 16 , 1042–1048 (2013).
Fredriksson, R. et al. The obesity gene, FTO, is of ancient origin, up-regulated during food deprivation and expressed in neurons of feeding-related nuclei of the brain. Endocrinology 149 , 2062–2071 (2008).
Cohen, D. A. Neurophysiological pathways to obesity: below awareness and beyond individual control. Diabetes 57 , 1768–1773 (2008).
Richard, D. Cognitive and autonomic determinants of energy homeostasis in obesity. Nat. Rev. Endocrinol. 11 , 489–501 (2015).
Clemmensen, C. et al. Gut-brain cross-talk in metabolic control. Cell 168 , 758–774 (2017).
Timper, K. & Brüning, J. C. Hypothalamic circuits regulating appetite and energy homeostasis: pathways to obesity. Dis. Model. Mech. 10 , 679–689 (2017).
Kim, K. S., Seeley, R. J. & Sandoval, D. A. Signalling from the periphery to the brain that regulates energy homeostasis. Nat. Rev. Neurosci. 19 , 185–196 (2018).
Cutler, D. M., Glaeser, E. L. & Shapiro, J. M. Why have Americans become more obese? J. Econ. Perspect. 17 , 93–118 (2003).
Löffler, A. et al. Effects of psychological eating behaviour domains on the association between socio-economic status and BMI. Public Health Nutr. 20 , 2706–2712 (2017).
Chan, R. S. & Woo, J. Prevention of overweight and obesity: how effective is the current public health approach. Int. J. Environ. Res. Public Health 7 , 765–783 (2010).
Hsueh, W. C. et al. Analysis of type 2 diabetes and obesity genetic variants in Mexican Pima Indians: marked allelic differentiation among Amerindians at HLA. Ann. Hum. Genet. 82 , 287–299 (2018).
Schulz, L. O. et al. Effects of traditional and western environments on prevalence of type 2 diabetes in Pima Indians in Mexico and the US. Diabetes Care 29 , 1866–1871 (2006).
Rotimi, C. N. et al. Distribution of anthropometric variables and the prevalence of obesity in populations of west African origin: the International Collaborative Study on Hypertension in Blacks (ICSHIB). Obes. Res. 3 , 95–105 (1995).
Durazo-Arvizu, R. A. et al. Rapid increases in obesity in Jamaica, compared to Nigeria and the United States. BMC Public Health 8 , 133 (2008).
Hu, F. B., Li, T. Y., Colditz, G. A., Willett, W. C. & Manson, J. E. Television watching and other sedentary behaviors in relation to risk of obesity and type 2 diabetes mellitus in women. JAMA 289 , 1785–1791 (2003).
Rissanen, A. M., Heliövaara, M., Knekt, P., Reunanen, A. & Aromaa, A. Determinants of weight gain and overweight in adult Finns. Eur. J. Clin. Nutr. 45 , 419–430 (1991).
Zimmet, P. Z., Arblaster, M. & Thoma, K. The effect of westernization on native populations. Studies on a Micronesian community with a high diabetes prevalence. Aust. NZ J. Med. 8 , 141–146 (1978).
Ulijaszek, S. J. Increasing body size among adult Cook Islanders between 1966 and 1996. Ann. Hum. Biol. 28 , 363–373 (2001).
Snowdon, W. & Thow, A. M. Trade policy and obesity prevention: challenges and innovation in the Pacific Islands. Obes. Rev. 14 , 150–158 (2013).
McLennan, A. K. & Ulijaszek, S. J. Obesity emergence in the Pacific islands: why understanding colonial history and social change is important. Public Health Nutr. 18 , 1499–1505 (2015).
Becker, A. E., Gilman, S. E. & Burwell, R. A. Changes in prevalence of overweight and in body image among Fijian women between 1989 and 1998. Obes. Res. 13 , 110–117 (2005).
Swinburn, B., Sacks, G. & Ravussin, E. Increased food energy supply is more than sufficient to explain the US epidemic of obesity. Am. J. Clin. Nutr. 90 , 1453–1456 (2009).
Swinburn, B. A. et al. Estimating the changes in energy flux that characterize the rise in obesity prevalence. Am. J. Clin. Nutr. 89 , 1723–1728 (2009).
US Department of Agriculture. Food availability (per capita) data system. USDA https://www.ers.usda.gov/data-products/food-availability-per-capita-data-system/ (updated 29 Oct 2018).
Carden, T. J. & Carr, T. P. Food availability of glucose and fat, but not fructose, increased in the U.S. between 1970 and 2009: analysis of the USDA food availability data system. Nutr. J. 12 , 130 (2013).
Hall, K. D., Guo, J., Dore, M. & Chow, C. C. The progressive increase of food waste in America and its environmental impact. PLOS ONE 4 , e7940 (2009).
Scarborough, P. et al. Increased energy intake entirely accounts for increase in body weight in women but not in men in the UK between 1986 and 2000. Br. J. Nutr. 105 , 1399–1404 (2011).
McGinnis, J. M. & Nestle, M. The Surgeon General’s report on nutrition and health: policy implications and implementation strategies. Am. J. Clin. Nutr. 49 , 23–28 (1989).
Krebs-Smith, S. M., Reedy, J. & Bosire, C. Healthfulness of the U.S. food supply: little improvement despite decades of dietary guidance. Am. J. Prev. Med. 38 , 472–477 (2010).
Malik, V. S., Popkin, B. M., Bray, G. A., Després, J. P. & Hu, F. B. Sugar-sweetened beverages, obesity, type 2 diabetes mellitus, and cardiovascular disease risk. Circulation 121 , 1356–1364 (2010).
Schulze, M. B. et al. Sugar-sweetened beverages, weight gain, and incidence of type 2 diabetes in young and middle-aged women. JAMA 292 , 927–934 (2004).
Mozaffarian, D., Hao, T., Rimm, E. B., Willett, W. C. & Hu, F. B. Changes in diet and lifestyle and long-term weight gain in women and men. N. Engl. J. Med. 364 , 2392–2404 (2011).
Malik, V. S. & Hu, F. B. Sugar-sweetened beverages and health: where does the evidence stand? Am. J. Clin. Nutr. 94 , 1161–1162 (2011).
Qi, Q. et al. Sugar-sweetened beverages and genetic risk of obesity. N. Engl. J. Med. 367 , 1387–1396 (2012).
Heiker, J. T. et al. Identification of genetic loci associated with different responses to high-fat diet-induced obesity in C57BL/6N and C57BL/6J substrains. Physiol. Genomics 46 , 377–384 (2014).
Wahlqvist, M. L. et al. Early-life influences on obesity: from preconception to adolescence. Ann. NY Acad. Sci. 1347 , 1–28 (2015).
Rohde, K. et al. Genetics and epigenetics in obesity. Metabolism . https://doi.org/10.1016/j.metabol.2018.10.007 (2018).
Article PubMed Google Scholar
Panzeri, I. & Pospisilik, J. A. Epigenetic control of variation and stochasticity in metabolic disease. Mol. Metab. 14 , 26–38 (2018).
Ruiz-Hernandez, A. et al. Environmental chemicals and DNA methylation in adults: a systematic review of the epidemiologic evidence. Clin. Epigenet. 7 , 55 (2015).
Quarta, C., Schneider, R. & Tschöp, M. H. Epigenetic ON/OFF switches for obesity. Cell 164 , 341–342 (2016).
Dalgaard, K. et al. Trim28 haploinsufficiency triggers bi-stable epigenetic obesity. Cell 164 , 353–364 (2015).
Michaelides, M. et al. Striatal Rgs4 regulates feeding and susceptibility to diet-induced obesity. Mol. Psychiatry . https://doi.org/10.1038/s41380-018-0120-7 (2018).
Article PubMed PubMed Central Google Scholar
Weihrauch-Blüher, S. et al. Current guidelines for obesity prevention in childhood and adolescence. Obes. Facts 11 , 263–276 (2018).
Nakamura, R. et al. Evaluating the 2014 sugar-sweetened beverage tax in Chile: An observational study in urban areas. PLOS Med. 15 , e1002596 (2018).
Colchero, M. A., Molina, M. & Guerrero-López, C. M. After Mexico implemented a tax, purchases of sugar-sweetened beverages decreased and water increased: difference by place of residence, household composition, and income level. J. Nutr. 147 , 1552–1557 (2017).
Brownell, K. D. & Warner, K. E. The perils of ignoring history: Big Tobacco played dirty and millions died. How similar is Big Food? Milbank Q. 87 , 259–294 (2009).
Mialon, M., Swinburn, B., Allender, S. & Sacks, G. ‘Maximising shareholder value’: a detailed insight into the corporate political activity of the Australian food industry. Aust. NZ J. Public Health 41 , 165–171 (2017).
Peeters, A. Obesity and the future of food policies that promote healthy diets. Nat. Rev. Endocrinol. 14 , 430–437 (2018).
Hawkes, C., Jewell, J. & Allen, K. A food policy package for healthy diets and the prevention of obesity and diet-related non-communicable diseases: the NOURISHING framework. Obes. Rev. 14 (Suppl. 2), 159–168 (2013).
World Health Organisation. Global database on the Implementation of Nutrition Action (GINA). WHO https://www.who.int/nutrition/gina/en/ (2012).
Popkin, B., Monteiro, C. & Swinburn, B. Overview: Bellagio Conference on program and policy options for preventing obesity in the low- and middle-income countries. Obes. Rev. 14 (Suppl. 2), 1–8 (2013).
Download references
Reviewer information
Nature Reviews Endocrinology thanks G. Bray, A. Sharma and H. Toplak for their contribution to the peer review of this work.
Author information
Authors and affiliations.
Department of Medicine, University of Leipzig, Leipzig, Germany
Matthias Blüher
You can also search for this author in PubMed Google Scholar
Corresponding author
Correspondence to Matthias Blüher .
Ethics declarations
Competing interests.
The author declares no competing interests.
Additional information
Publisher’s note.
Springer Nature remains neutral with regard to jurisdictional claims in published maps and institutional affiliations.
Rights and permissions
Reprints and permissions
About this article
Cite this article.
Blüher, M. Obesity: global epidemiology and pathogenesis. Nat Rev Endocrinol 15 , 288–298 (2019). https://doi.org/10.1038/s41574-019-0176-8
Download citation
Published : 27 February 2019
Issue Date : May 2019
DOI : https://doi.org/10.1038/s41574-019-0176-8
Share this article
Anyone you share the following link with will be able to read this content:
Sorry, a shareable link is not currently available for this article.
Provided by the Springer Nature SharedIt content-sharing initiative
This article is cited by
Body composition, lifestyle, and depression: a prospective study in the uk biobank.
BMC Public Health (2024)
Physical activity, gestational weight gain in obese patients with early gestational diabetes and the perinatal outcome – a randomised–controlled trial
- Lukasz Adamczak
- Urszula Mantaj
- Ewa Wender-Ozegowska
BMC Pregnancy and Childbirth (2024)
Effects of N-acetylcysteine on the expressions of UCP1 and factors related to thyroid function in visceral adipose tissue of obese adults: a randomized, double-blind clinical trial
- Mohammad Hassan Sohouli
- Ghazaleh Eslamian
Genes & Nutrition (2024)
Effect of COVID-19 Pandemic Lockdowns on Body Mass Index of Primary School Children from Different Socioeconomic Backgrounds
- Ludwig Piesch
- Robert Stojan
- Till Utesch
Sports Medicine - Open (2024)
Prevalence and risk factors of obesity among undergraduate student population in Ghana: an evaluation study of body composition indices
- Christian Obirikorang
- Evans Asamoah Adu
- Lois Balmer
Quick links
- Explore articles by subject
- Guide to authors
- Editorial policies
Sign up for the Nature Briefing newsletter — what matters in science, free to your inbox daily.

- - Google Chrome
Intended for healthcare professionals
- Access provided by Google Indexer
- My email alerts
- BMA member login
- Username * Password * Forgot your log in details? Need to activate BMA Member Log In Log in via OpenAthens Log in via your institution

Search form
- Advanced search
- Search responses
- Search blogs
- Effectiveness of...
Effectiveness of weight management interventions for adults delivered in primary care: systematic review and meta-analysis of randomised controlled trials
- Related content
- Peer review
- Claire D Madigan , senior research associate 1 ,
- Henrietta E Graham , doctoral candidate 1 ,
- Elizabeth Sturgiss , NHMRC investigator 2 ,
- Victoria E Kettle , research associate 1 ,
- Kajal Gokal , senior research associate 1 ,
- Greg Biddle , research associate 1 ,
- Gemma M J Taylor , reader 3 ,
- Amanda J Daley , professor of behavioural medicine 1
- 1 Centre for Lifestyle Medicine and Behaviour (CLiMB), The School of Sport, Exercise and Health Sciences, Loughborough University, Loughborough LE11 3TU, UK
- 2 School of Primary and Allied Health Care, Monash University, Melbourne, Australia
- 3 Department of Psychology, Addiction and Mental Health Group, University of Bath, Bath, UK
- Correspondence to: C D Madigan c.madigan{at}lboro.ac.uk (or @claire_wm and @lboroclimb on Twitter)
- Accepted 26 April 2022
Objective To examine the effectiveness of behavioural weight management interventions for adults with obesity delivered in primary care.
Design Systematic review and meta-analysis of randomised controlled trials.
Eligibility criteria for selection of studies Randomised controlled trials of behavioural weight management interventions for adults with a body mass index ≥25 delivered in primary care compared with no treatment, attention control, or minimal intervention and weight change at ≥12 months follow-up.
Data sources Trials from a previous systematic review were extracted and the search completed using the Cochrane Central Register of Controlled Trials, Medline, PubMed, and PsychINFO from 1 January 2018 to 19 August 2021.
Data extraction and synthesis Two reviewers independently identified eligible studies, extracted data, and assessed risk of bias using the Cochrane risk of bias tool. Meta-analyses were conducted with random effects models, and a pooled mean difference for both weight (kg) and waist circumference (cm) were calculated.
Main outcome measures Primary outcome was weight change from baseline to 12 months. Secondary outcome was weight change from baseline to ≥24 months. Change in waist circumference was assessed at 12 months.
Results 34 trials were included: 14 were additional, from a previous review. 27 trials (n=8000) were included in the primary outcome of weight change at 12 month follow-up. The mean difference between the intervention and comparator groups at 12 months was −2.3 kg (95% confidence interval −3.0 to −1.6 kg, I 2 =88%, P<0.001), favouring the intervention group. At ≥24 months (13 trials, n=5011) the mean difference in weight change was −1.8 kg (−2.8 to −0.8 kg, I 2 =88%, P<0.001) favouring the intervention. The mean difference in waist circumference (18 trials, n=5288) was −2.5 cm (−3.2 to −1.8 cm, I 2 =69%, P<0.001) in favour of the intervention at 12 months.
Conclusions Behavioural weight management interventions for adults with obesity delivered in primary care are effective for weight loss and could be offered to members of the public.
Systematic review registration PROSPERO CRD42021275529.
Introduction
Obesity is associated with an increased risk of diseases such as cancer, type 2 diabetes, and heart disease, leading to early mortality. 1 2 3 More recently, obesity is a risk factor for worse outcomes with covid-19. 4 5 Because of this increased risk, health agencies and governments worldwide are focused on finding effective ways to help people lose weight. 6
Primary care is an ideal setting for delivering weight management services, and international guidelines recommend that doctors should opportunistically screen and encourage patients to lose weight. 7 8 On average, most people consult a primary care doctor four times yearly, providing opportunities for weight management interventions. 9 10 A systematic review of randomised controlled trials by LeBlanc et al identified behavioural interventions that could potentially be delivered in primary care, or involved referral of patients by primary care professionals, were effective for weight loss at 12-18 months follow-up (−2.4 kg, 95% confidence interval −2.9 to−1.9 kg). 11 However, this review included trials with interventions that the review authors considered directly transferrable to primary care, but not all interventions involved primary care practitioners. The review included interventions that were entirely delivered by university research employees, meaning implementation of these interventions might differ if offered in primary care, as has been the case in other implementation research of weight management interventions, where effects were smaller. 12 As many similar trials have been published after this review, an updated review would be useful to guide health policy.
We examined the effectiveness of weight loss interventions delivered in primary care on measures of body composition (weight and waist circumference). We also identified characteristics of effective weight management programmes for policy makers to consider.
This systematic review was registered on PROSPERO and is reported according to the preferred reporting items for systematic reviews and meta-analyses (PRISMA) statement. 13 14
Eligibility criteria
We considered studies to be eligible for inclusion if they were randomised controlled trials, comprised adult participants (≥18 years), and evaluated behavioural weight management interventions delivered in primary care that focused on weight loss. A primary care setting was broadly defined as the first point of contact with the healthcare system, providing accessible, continued, comprehensive, and coordinated care, focused on long term health. 15 Delivery in primary care was defined as the majority of the intervention being delivered by medical and non-medical clinicians within the primary care setting. Table 1 lists the inclusion and exclusion criteria.
Study inclusion and exclusion criteria
- View inline
We extracted studies from the systematic review by LeBlanc et al that met our inclusion criteria. 11 We also searched the exclusions in this review because the researchers excluded interventions specifically for diabetes management, low quality trials, and only included studies from an Organisation for Economic Co-operation and Development country, limiting the scope of the findings.
We searched for studies in the Cochrane Central Register of Controlled Trials, Medline, PubMed, and PsychINFO from 1 January 2018 to 19 August 2021 (see supplementary file 1). Reference lists of previous reviews 16 17 18 19 20 21 and included trials were hand searched.
Data extraction
Results were uploaded to Covidence, 22 a software platform used for screening, and duplicates removed. Two independent reviewers screened study titles, abstracts, and full texts. Disagreements were discussed and resolved by a third reviewer. All decisions were recorded in Covidence, and reviewers were blinded to each other’s decisions. Covidence calculates proportionate agreement as a measure of inter-rater reliability, and data are reported separately by title or abstract screening and full text screening. One reviewer extracted data on study characteristics (see supplementary table 1) and two authors independently extracted data on weight outcomes. We contacted the authors of four included trials (from the updated search) for further information. 23 24 25 26
Outcomes, summary measures, and synthesis of results
The primary outcome was weight change from baseline to 12 months. Secondary outcomes were weight change from baseline to ≥24 months and from baseline to last follow-up (to include as many trials as possible), and waist circumference from baseline to 12 months. Supplementary file 2 details the prespecified subgroup analysis that we were unable to complete. The prespecified subgroup analyses that could be completed were type of healthcare professional who delivered the intervention, country, intensity of the intervention, and risk of bias rating.
Healthcare professional delivering intervention —From the data we were able to compare subgroups by type of healthcare professional: nurses, 24 26 27 28 general practitioners, 23 29 30 31 and non-medical practitioners (eg, health coaches). 32 33 34 35 36 37 38 39 Some of the interventions delivered by non-medical practitioners were supported, but not predominantly delivered, by GPs. Other interventions were delivered by a combination of several different practitioners—for example, it was not possible to determine whether a nurse or dietitian delivered the intervention. In the subgroup analysis of practitioner delivery, we refer to this group as “other.”
Country —We explored the effectiveness of interventions by country. Only countries with three or more trials were included in subgroup analyses (United Kingdom, United States, and Spain).
Intensity of interventions —As the median number of contacts was 12, we categorised intervention groups according to whether ≤11 or ≥12 contacts were required.
Risk of bias rating —Studies were classified as being at low, unclear, and high risk of bias. Risk of bias was explored as a potential influence on the results.
Meta-analyses
Meta-analyses were conducted using Review Manager 5.4. 40 As we expected the treatment effects to differ because of the diversity of intervention components and comparator conditions, we used random effects models. A pooled mean difference was calculated for each analysis, and variance in heterogeneity between studies was compared using the I 2 and τ 2 statistics. We generated funnel plots to evaluate small study effects. If more than two intervention groups existed, we divided the number of participants in the comparator group by the number of intervention groups and analysed each individually. Nine trials were cluster randomised controlled trials. The trials had adjusted their results for clustering, or adjustment had been made in the previous systematic review by LeBlanc et al. 11 One trial did not report change in weight by group. 26 We calculated the mean weight change and standard deviation using a standard formula, which imputes a correlation for the baseline and follow-up weights. 41 42 In a non-prespecified analysis, we conducted univariate and multivariable metaregression (in Stata) using a random effects model to examine the association between number of sessions and type of interventionalist on study effect estimates.
Risk of bias
Two authors independently assessed the risk of bias using the Cochrane risk of bias tool v2. 43 For incomplete outcome data we defined a high risk of bias as ≥20% attrition. Disagreements were resolved by discussion or consultation with a third author.
Patient and public involvement
The study idea was discussed with patients and members of the public. They were not, however, included in discussions about the design or conduct of the study.
The search identified 11 609 unique study titles or abstracts after duplicates were removed ( fig 1 ). After screening, 97 full text articles were assessed for eligibility. The proportionate agreement ranged from 0.94 to 1.0 for screening of titles or abstracts and was 0.84 for full text screening. Fourteen new trials met the inclusion criteria. Twenty one studies from the review by LeBlanc et al met our eligibility criteria and one study from another systematic review was considered eligible and included. 44 Some studies had follow-up studies (ie, two publications) that were found in both the second and the first search; hence the total number of trials was 34 and not 36. Of the 34 trials, 27 (n=8000 participants) were included in the primary outcome meta-analysis of weight change from baseline to 12 months, 13 (n=5011) in the secondary outcome from baseline to ≥24 months, and 30 (n=8938) in the secondary outcome for weight change from baseline to last follow-up. Baseline weight was accounted for in 18 of these trials, but in 14 24 26 29 30 31 32 44 45 46 47 48 49 50 51 it was unclear or the trials did not consider baseline weight. Eighteen trials (n=5288) were included in the analysis of change in waist circumference at 12 months.

Studies included in systematic review of effectiveness of behavioural weight management interventions in primary care. *Studies were merged in Covidence if they were from same trial
- Download figure
- Open in new tab
- Download powerpoint
Study characteristics
Included trials (see supplementary table 1) were individual randomised controlled trials (n=25) 24 25 26 27 28 29 32 33 34 35 38 39 41 44 45 46 47 50 51 52 53 54 55 56 59 or cluster randomised controlled trials (n=9). 23 30 31 36 37 48 49 57 58 Most were conducted in the US (n=14), 29 30 31 32 33 34 35 36 37 45 48 51 54 55 UK (n=7), 27 28 38 41 47 57 58 and Spain (n=4). 25 44 46 49 The median number of participants was 276 (range 50-864).
Four trials included only women (average 65.9% of women). 31 48 51 59 The mean BMI at baseline was 35.2 (SD 4.2) and mean age was 48 (SD 9.7) years. The interventions lasted between one session (with participants subsequently following the programme unassisted for three months) and several sessions over three years (median 12 months). The follow-up period ranged from 12 months to three years (median 12 months). Most trials excluded participants who had lost weight in the past six months and were taking drugs that affected weight.
Meta-analysis
Overall, 27 trials were included in the primary meta-analysis of weight change from baseline to 12 months. Three trials could not be included in the primary analysis as data on weight were only available at two and three years and not 12 months follow-up, but we included these trials in the secondary analyses of last follow-up and ≥24 months follow-up. 26 44 50 Four trials could not be included in the meta-analysis as they did not present data in a way that could be synthesised (ie, measures of dispersion). 25 52 53 58 The mean difference was −2.3 kg (95% confidence interval −3.0 to −1.6 kg, I 2 =88%, τ 2 =3.38; P<0.001) in favour of the intervention group ( fig 2 ). We found no evidence of publication bias (see supplementary fig 1). Absolute weight change was −3.7 (SD 6.1) kg in the intervention group and −1.4 (SD 5.5) kg in the comparator group.

Mean difference in weight at 12 months by weight management programme in primary care (intervention) or no treatment, different content, or minimal intervention (control). SD=standard deviation
Supplementary file 2 provides a summary of the main subgroup analyses.
Weight change
The mean difference in weight change at the last follow-up was −1.9 kg (95% confidence interval −2.5 to −1.3 kg, I 2 =81%, τ 2 =2.15; P<0.001). Absolute weight change was −3.2 (SD 6.4) kg in the intervention group and −1.2 (SD 6.0) kg in the comparator group (see supplementary figs 2 and 3).
At the 24 month follow-up the mean difference in weight change was −1.8 kg (−2.8 to −0.8 kg, I 2 =88%, τ 2 =3.13; P<0.001) (see supplementary fig 4). As the weight change data did not differ between the last follow-up and ≥24 months, we used the weight data from the last follow-up in subgroup analyses.
In subgroup analyses of type of interventionalist, differences were significant (P=0.005) between non-medical practitioners, GPs, nurses, and other people who delivered interventions (see supplementary fig 2).
Participants who had ≥12 contacts during interventions lost significantly more weight than those with fewer contacts (see supplementary fig 6). The association remained after adjustment for type of interventionalist.
Waist circumference
The mean difference in waist circumference was −2.5 cm (95% confidence interval −3.2 to −1.8 cm, I 2 =69%, τ 2 =1.73; P<0.001) in favour of the intervention at 12 months ( fig 3 ). Absolute changes were −3.7 cm (SD 7.8 cm) in the intervention group and −1.3 cm (SD 7.3) in the comparator group.

Mean difference in waist circumference at 12 months. SD=standard deviation
Risk of bias was considered to be low in nine trials, 24 33 34 35 39 41 47 55 56 unclear in 12 trials, 25 27 28 29 32 45 46 50 51 52 54 59 and high in 13 trials 23 26 30 31 36 37 38 44 48 49 53 57 58 ( fig 4 ). No significant (P=0.65) differences were found in subgroup analyses according to level of risk of bias from baseline to 12 months (see supplementary fig 7).

Risk of bias in included studies
Worldwide, governments are trying to find the most effective services to help people lose weight to improve the health of populations. We found weight management interventions delivered by primary care practitioners result in effective weight loss and reduction in waist circumference and these interventions should be considered part of the services offered to help people manage their weight. A greater number of contacts between patients and healthcare professionals led to more weight loss, and interventions should be designed to include at least 12 contacts (face-to-face or by telephone, or both). Evidence suggests that interventions delivered by non-medical practitioners were as effective as those delivered by GPs (both showed statistically significant weight loss). It is also possible that more contacts were made with non-medical interventionalists, which might partially explain this result, although the metaregression analysis suggested the effect remained after adjustment for type of interventionalist. Because most comparator groups had fewer contacts than intervention groups, it is not known whether the effects of the interventions are related to contact with interventionalists or to the content of the intervention itself.
Although we did not determine the costs of the programme, it is likely that interventions delivered by non-medical practitioners would be cheaper than GP and nurse led programmes. 41 Most of the interventions delivered by non-medical practitioners involved endorsement and supervision from GPs (ie, a recommendation or checking in to see how patients were progressing), and these should be considered when implementing these types of weight management interventions in primary care settings. Our findings suggest that a combination of practitioners would be most effective because GPs might not have the time for 12 consultations to support weight management.
Although the 2.3 kg greater weight loss in the intervention group may seem modest, just 2-5% in weight loss is associated with improvements in systolic blood pressure and glucose and triglyceride levels. 60 The confidence intervals suggest a potential range of weight loss and that these interventions might not provide as much benefit to those with a higher BMI. Patients might not find an average weight loss of 3.7 kg attractive, as many would prefer to lose more weight; explaining to patients the benefits of small weight losses to health would be important.
Strengths and limitations of this review
Our conclusions are based on a large sample of about 8000 participants, and 12 of these trials were published since 2018. It was occasionally difficult to distinguish who delivered the interventions and how they were implemented. We therefore made some assumptions at the screening stage about whether the interventionalists were primary care practitioners or if most of the interventions were delivered in primary care. These discussions were resolved by consensus. All included trials measured weight, and we excluded those that used self-reported data. Dropout rates are important in weight management interventions as those who do less well are less likely to be followed-up. We found that participants in trials with an attrition rate of 20% or more lost less weight and we are confident that those with high attrition rates have not inflated the results. Trials were mainly conducted in socially economic developed countries, so our findings might not be applicable to all countries. The meta-analyses showed statistically significant heterogeneity, and our prespecified subgroups analysis explained some, but not all, of the variance.
Comparison with other studies
The mean difference of −2.3 kg in favour of the intervention group at 12 months is similar to the findings in the review by LeBlanc et al, who reported a reduction of −2.4 kg in participants who received a weight management intervention in a range of settings, including primary care, universities, and the community. 11 61 This is important because the review by LeBlanc et al included interventions that were not exclusively conducted in primary care or by primary care practitioners. Trials conducted in university or hospital settings are not typically representative of primary care populations and are often more intensive than trials conducted in primary care as a result of less constraints on time. Thus, our review provides encouraging findings for the implementation of weight management interventions delivered in primary care. The findings are of a similar magnitude to those found in a trial by Ahern et al that tested primary care referral to a commercial programme, with a difference of −2.7 kg (95% confidence interval −3.9 to −1.5 kg) reported at 12 month follow-up. 62 The trial by Ahern et al also found a difference in waist circumference of −4.1 cm (95% confidence interval −5.5 to −2.3 cm) in favour of the intervention group at 12 months. Our finding was smaller at −2.5 cm (95% confidence interval −3.2 to −1.8 cm). Some evidence suggests clinical benefits from a reduction of 3 cm in waist circumference, particularly in decreased glucose levels, and the intervention groups showed a 3.7 cm absolute change in waist circumference. 63
Policy implications and conclusions
Weight management interventions delivered in primary care are effective and should be part of services offered to members of the public to help them manage weight. As about 39% of the world’s population is living with obesity, helping people to manage their weight is an enormous task. 64 Primary care offers good reach into the community as the first point of contact in the healthcare system and the remit to provide whole person care across the life course. 65 When developing weight management interventions, it is important to reflect on resource availability within primary care settings to ensure patients’ needs can be met within existing healthcare systems. 66
We did not examine the equity of interventions, but primary care interventions may offer an additional service and potentially help those who would not attend a programme delivered outside of primary care. Interventions should consist of 12 or more contacts, and these findings are based on a mixture of telephone and face-to-face sessions. Previous evidence suggests that GPs find it difficult to raise the issue of weight with patients and are pessimistic about the success of weight loss interventions. 67 Therefore, interventions should be implemented with appropriate training for primary care practitioners so that they feel confident about helping patients to manage their weight. 68
Unanswered questions and future research
A range of effective interventions are available in primary care settings to help people manage their weight, but we found substantial heterogeneity. It was beyond the scope of this systematic review to examine the specific components of the interventions that may be associated with greater weight loss, but this could be investigated by future research. We do not know whether these interventions are universally suitable and will decrease or increase health inequalities. As the data are most likely collected in trials, an individual patient meta-analysis is now needed to explore characteristics or factors that might explain the variance. Most of the interventions excluded people prescribed drugs that affect weight gain, such as antipsychotics, glucocorticoids, and some antidepressants. This population might benefit from help with managing their weight owing to the side effects of these drug classes on weight gain, although we do not know whether the weight management interventions we investigated would be effective in this population. 69
What is already known on this topic
Referral by primary care to behavioural weight management programmes is effective, but the effectiveness of weight management interventions delivered by primary care is not known
Systematic reviews have provided evidence for weight management interventions, but the latest review of primary care delivered interventions was published in 2014
Factors such as intensity and delivery mechanisms have not been investigated and could influence the effectiveness of weight management interventions delivered by primary care
What this study adds
Weight management interventions delivered by primary care are effective and can help patients to better manage their weight
At least 12 contacts (telephone or face to face) are needed to deliver weight management programmes in primary care
Some evidence suggests that weight loss after weight management interventions delivered by non-medical practitioners in primary care (often endorsed and supervised by doctors) is similar to that delivered by clinician led programmes
Ethics statements
Ethical approval.
Not required.
Data availability statement
Additional data are available in the supplementary files.
Contributors: CDM and AJD conceived the study, with support from ES. CDM conducted the search with support from HEG. CDM, AJD, ES, HEG, KG, GB, and VEK completed the screening and full text identification. CDM and VEK completed the risk of bias assessment. CDM extracted data for the primary outcome and study characteristics. HEJ, GB, and KG extracted primary outcome data. CDM completed the analysis in RevMan, and GMJT completed the metaregression analysis in Stata. CDM drafted the paper with AJD. All authors provided comments on the paper. CDM acts as guarantor. The corresponding author attests that all listed authors meet authorship criteria and that no others meeting the criteria have been omitted.
Funding: AJD is supported by a National Institute for Health and Care Research (NIHR) research professorship award. This research was supported by the NIHR Leicester Biomedical Research Centre. The views expressed are those of the authors and not necessarily those of the NHS, the NIHR, or the Department of Health and Social Care. ES’s salary is supported by an investigator grant (National Health and Medical Research Council, Australia). GT is supported by a Cancer Research UK fellowship. The funders had no role in considering the study design or in the collection, analysis, interpretation of data, writing of the report, or decision to submit the article for publication.
Competing interests: All authors have completed the ICMJE uniform disclosure form at www.icmje.org/disclosure-of-interest/ and declare: This research was supported by the National Institute for Health and Care Research Leicester Biomedical Research Centre; no financial relationships with any organisations that might have an interest in the submitted work in the previous three years, no other relationships or activities that could appear to have influenced the submitted work.
The lead author (CDM) affirms that the manuscript is an honest, accurate, and transparent account of the study being reported, and that no important aspects of the study have been omitted.
Dissemination to participants and related patient and public communities: We plan to disseminate these research findings to a wider community through press releases, featuring on the Centre for Lifestyle Medicine and Behaviour website ( www.lboro.ac.uk/research/climb/ ) via our policy networks, through social media platforms, and presentation at conferences.
Provenance and peer review: Not commissioned; externally peer reviewed.
This is an Open Access article distributed in accordance with the terms of the Creative Commons Attribution (CC BY 4.0) license, which permits others to distribute, remix, adapt and build upon this work, for commercial use, provided the original work is properly cited. See: http://creativecommons.org/licenses/by/4.0/ .
- Renehan AG ,
- Heller RF ,
- Bansback N ,
- Birmingham CL ,
- Abdullah A ,
- Peeters A ,
- de Courten M ,
- Stoelwinder J
- Aghili SMM ,
- Ebrahimpur M ,
- Arjmand B ,
- KETLAK Study Group
- ↵ Department of Health and Social Care. New specialised support to help those living with obesity to lose weight UK2021. www.gov.uk/government/news/new-specialised-support-to-help-those-living-with-obesity-to-lose-weight [accessed 08/02/2021].
- U.S. Preventive Services Task Force
- ↵ National Institute for Health and Care Excellence. Maintaining a Healthy Weight and Preventing Excess Weight Gain in Children and Adults. Cost Effectiveness Considerations from a Population Modelling Viewpoint. 2014, NICE. www.nice.org.uk/guidance/ng7/evidence/evidence-review-2-qualitative-evidence-review-of-the-most-acceptable-ways-to-communicate-information-about-individually-modifiable-behaviours-to-help-maintain-a-healthy-weight-or-prevent-excess-weigh-8733713.
- ↵ The Health Foundation. Use of primary care during the COVID-19 pandemic. 17/09/2020: The Health Foundation, 2020.
- ↵ Australian Bureau of Statistics. Patient Experiences in Australia: Summary of Findings, 2017-18. 2019 ed. Canberra, Australia, 2018. www.abs.gov.au/AUSSTATS/[email protected]/Lookup/4839.0Main+Features12017-18?OpenDocument.
- LeBlanc ES ,
- Patnode CD ,
- Webber EM ,
- Redmond N ,
- Rushkin M ,
- O’Connor EA
- Damschroder LJ ,
- Liberati A ,
- Tetzlaff J ,
- Altman DG ,
- PRISMA Group
- McKenzie JE ,
- Bossuyt PM ,
- ↵ WHO. Main terminology: World Health Organization; 2004. www.euro.who.int/en/health-topics/Health-systems/primary-health-care/main-terminology [accessed 09.12.21].
- Aceves-Martins M ,
- Robertson C ,
- REBALANCE team
- Glasziou P ,
- Isenring E ,
- Chisholm A ,
- Wakayama LN ,
- Kettle VE ,
- Madigan CD ,
- ↵ Covidence [program]. Melbourne, 2021.
- Welzel FD ,
- Carrington MJ ,
- Fernández-Ruiz VE ,
- Ramos-Morcillo AJ ,
- Solé-Agustí M ,
- Paniagua-Urbano JA ,
- Armero-Barranco D
- Bräutigam-Ewe M ,
- Hildingh C ,
- Yardley L ,
- Christian JG ,
- Bessesen DH ,
- Christian KK ,
- Goldstein MG ,
- Martin PD ,
- Dutton GR ,
- Horswell RL ,
- Brantley PJ
- Wadden TA ,
- Rogers MA ,
- Berkowitz RI ,
- Kumanyika SK ,
- Morales KH ,
- Allison KC ,
- Rozenblum R ,
- De La Cruz BA ,
- Katzmarzyk PT ,
- Martin CK ,
- Newton RL Jr . ,
- Nanchahal K ,
- Holdsworth E ,
- ↵ RevMan [program]. 5.4 version: Copenhagen, 2014.
- Sterne JAC ,
- Savović J ,
- Gomez-Huelgas R ,
- Jansen-Chaparro S ,
- Baca-Osorio AJ ,
- Mancera-Romero J ,
- Tinahones FJ ,
- Bernal-López MR
- Delahanty LM ,
- Tárraga Marcos ML ,
- Panisello Royo JM ,
- Carbayo Herencia JA ,
- Beeken RJ ,
- Leurent B ,
- Vickerstaff V ,
- Hagobian T ,
- Brannen A ,
- Rodriguez-Cristobal JJ ,
- Alonso-Villaverde C ,
- Panisello JM ,
- Conroy MB ,
- Spadaro KC ,
- Takasawa N ,
- Mashiyama Y ,
- Pritchard DA ,
- Hyndman J ,
- Jarjoura D ,
- Smucker W ,
- Baughman K ,
- Bennett GG ,
- Steinberg D ,
- Zaghloul H ,
- Chagoury O ,
- Leslie WS ,
- Barnes AC ,
- Summerbell CD ,
- Greenwood DC ,
- Huseinovic E ,
- Leu Agelii M ,
- Hellebö Johansson E ,
- Winkvist A ,
- Look AHEAD Research Group
- LeBlanc EL ,
- Wheeler GM ,
- Aveyard P ,
- de Koning L ,
- Chiuve SE ,
- Willett WC ,
- ↵ World Health Organization. Obesity and Overweight, 2021, www.who.int/news-room/fact-sheets/detail/obesity-and-overweight
- Starfield B ,
- Sturgiss E ,
- Dewhurst A ,
- Devereux-Fitzgerald A ,
- Haesler E ,
- van Weel C ,
- Gulliford MC
- Fassbender JE ,
- Sarwer DB ,
- Brekke HK ,
- Introduction
- Conclusions
- Article Information
Adjusted for age, sex, race and ethnicity, Townsend Deprivation Index, years of education, smoking status, alcohol intake, healthy diet score, physical activity, depression, anxiety, eating disorder, hypertension, high cholesterol, diabetes, metformin use, glucocorticoid use, and hemoglobin A 1c level.
eFigure 1. Flowchart of Participant Enrollment
eFigure 2. Directed Acyclic Graph (DAG) Indicating the Associations Among Exposures, Outcomes, and Included Covariates In The Analyses
eFigure 3. Cumulative Hazard of All-Cause Mortality Among Participants With Obesity Compared With Participants Without Obesity With Different Social Isolation Index and Loneliness Index
eFigure 4. Joint Association of Social Isolation and Obesity With All-Cause Mortality via Multivariable Model
eFigure 5. Cumulative Hazard of All-Cause and Cause-Specific Mortality for Loneliness Index Among 84 920 Participants With Obesity Compared With 84 920 Participants Without Obesity
eFigure 6. Relative Importance of Risk Factors for Mortality in 93 357 People With Obesity
eTable 1. Evaluation of Social Isolation and Loneliness in the UK Biobank
eTable 2. Assessment of Healthy Diet Score in the UK Biobank
eTable 3. Self-Reported and ICD-10 Codes for Prevalent Diseases in This Study
eTable 4. Summary of Medications for Metformin Use and Glucocorticoid Use
eTable 5. Numbers and Percentages of Participants With Missing Covariates
eTable 6. Associations of Social Isolation and Loneliness With Risk for Cancer-Related and CVD-Related Mortality in 93 357 People With Obesity
eTable 7. Pairwise Comparisons for Associations of Social Isolation and Loneliness With Risk for All-Cause Mortality in 93 357 People With Obesity
eTable 8. Pairwise Comparisons for Associations of Social Isolation and Loneliness With Risk for All-Cause Mortality in 305 615 People Without Obesity
eTable 9. Association of Individual Components of Social Isolation and Loneliness With Risk of All-Cause Mortality and Cause-Specific Mortality in 93 357 People With Obesity via Multivariable Model
eTable 10. Associations of Social Isolation and Loneliness With Risk for Cancer-Related and CVD-Related Mortality in 84 920 People With Obesity Compared With 84 920 Matched Control Participants via Multivariable Model
eTable 11. Associations of Social Isolation and Loneliness With Risk for All-Cause Mortality in 84 920 People With Different Obesity Classes Compared With 84 920 Matched Control Participants via Multivariable Model
eTable 12. Association of Individual Components of Social Isolation and Loneliness With Risk of All-Cause Mortality and Cause-Specific Mortality in 84 920 People With Obesity Compared With 84 920 Matched Control Participants via Multivariable Model
eTable 13. Associations of Social Isolation and Loneliness With Risk of All-Cause Mortality and Cause-Specific Mortality in 93 037 People With Obesity via Multivariable Model After Excluding Participants Who Died During the First 2 Years of Follow-Up
eTable 14. Associations of Social Isolation and Loneliness With Risk of All-Cause Mortality and Cause-Specific Mortality in 84 920 People With Obesity Compared With 84 920 Matched Control Participants Multivariable Model After Excluding Participants Who Died During the First 2 Years of Follow-Up
eTable 15. Associations of Social Isolation and Loneliness With Risk of All-Cause Mortality and Cause-Specific Mortality in 93 357 People With Obesity via Multivariable Model With All Missing Covariate Data Imputed Using Multiple Imputation
eTable 16. Associations of Social Isolation and Loneliness With Risk of All-Cause Mortality and Cause-Specific Mortality in 84 920 People With Obesity Compared With 84 920 Matched Control Participants via Multivariable Model With All Missing Covariate Data Imputed Using Multiple Imputation
Data Sharing Statement
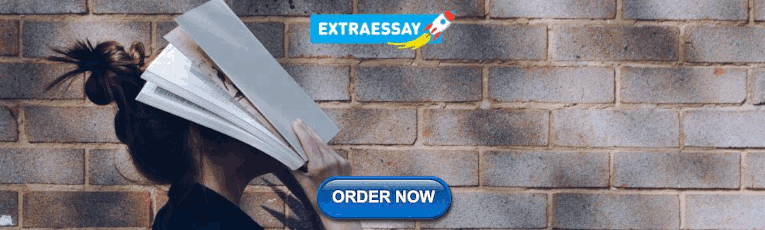
See More About
Sign up for emails based on your interests, select your interests.
Customize your JAMA Network experience by selecting one or more topics from the list below.
- Academic Medicine
- Acid Base, Electrolytes, Fluids
- Allergy and Clinical Immunology
- American Indian or Alaska Natives
- Anesthesiology
- Anticoagulation
- Art and Images in Psychiatry
- Artificial Intelligence
- Assisted Reproduction
- Bleeding and Transfusion
- Caring for the Critically Ill Patient
- Challenges in Clinical Electrocardiography
- Climate and Health
- Climate Change
- Clinical Challenge
- Clinical Decision Support
- Clinical Implications of Basic Neuroscience
- Clinical Pharmacy and Pharmacology
- Complementary and Alternative Medicine
- Consensus Statements
- Coronavirus (COVID-19)
- Critical Care Medicine
- Cultural Competency
- Dental Medicine
- Dermatology
- Diabetes and Endocrinology
- Diagnostic Test Interpretation
- Drug Development
- Electronic Health Records
- Emergency Medicine
- End of Life, Hospice, Palliative Care
- Environmental Health
- Equity, Diversity, and Inclusion
- Facial Plastic Surgery
- Gastroenterology and Hepatology
- Genetics and Genomics
- Genomics and Precision Health
- Global Health
- Guide to Statistics and Methods
- Hair Disorders
- Health Care Delivery Models
- Health Care Economics, Insurance, Payment
- Health Care Quality
- Health Care Reform
- Health Care Safety
- Health Care Workforce
- Health Disparities
- Health Inequities
- Health Policy
- Health Systems Science
- History of Medicine
- Hypertension
- Images in Neurology
- Implementation Science
- Infectious Diseases
- Innovations in Health Care Delivery
- JAMA Infographic
- Law and Medicine
- Leading Change
- Less is More
- LGBTQIA Medicine
- Lifestyle Behaviors
- Medical Coding
- Medical Devices and Equipment
- Medical Education
- Medical Education and Training
- Medical Journals and Publishing
- Mobile Health and Telemedicine
- Narrative Medicine
- Neuroscience and Psychiatry
- Notable Notes
- Nutrition, Obesity, Exercise
- Obstetrics and Gynecology
- Occupational Health
- Ophthalmology
- Orthopedics
- Otolaryngology
- Pain Medicine
- Palliative Care
- Pathology and Laboratory Medicine
- Patient Care
- Patient Information
- Performance Improvement
- Performance Measures
- Perioperative Care and Consultation
- Pharmacoeconomics
- Pharmacoepidemiology
- Pharmacogenetics
- Pharmacy and Clinical Pharmacology
- Physical Medicine and Rehabilitation
- Physical Therapy
- Physician Leadership
- Population Health
- Primary Care
- Professional Well-being
- Professionalism
- Psychiatry and Behavioral Health
- Public Health
- Pulmonary Medicine
- Regulatory Agencies
- Reproductive Health
- Research, Methods, Statistics
- Resuscitation
- Rheumatology
- Risk Management
- Scientific Discovery and the Future of Medicine
- Shared Decision Making and Communication
- Sleep Medicine
- Sports Medicine
- Stem Cell Transplantation
- Substance Use and Addiction Medicine
- Surgical Innovation
- Surgical Pearls
- Teachable Moment
- Technology and Finance
- The Art of JAMA
- The Arts and Medicine
- The Rational Clinical Examination
- Tobacco and e-Cigarettes
- Translational Medicine
- Trauma and Injury
- Treatment Adherence
- Ultrasonography
- Users' Guide to the Medical Literature
- Vaccination
- Venous Thromboembolism
- Veterans Health
- Women's Health
- Workflow and Process
- Wound Care, Infection, Healing
Get the latest research based on your areas of interest.
Others also liked.
- Download PDF
- X Facebook More LinkedIn
Zhou J , Tang R , Wang X , Li X , Heianza Y , Qi L. Improvement of Social Isolation and Loneliness and Excess Mortality Risk in People With Obesity. JAMA Netw Open. 2024;7(1):e2352824. doi:10.1001/jamanetworkopen.2023.52824
Manage citations:
© 2024
- Permissions
Improvement of Social Isolation and Loneliness and Excess Mortality Risk in People With Obesity
- 1 Department of Epidemiology, Tulane University School of Public Health and Tropical Medicine, New Orleans, Louisiana
- 2 Department of Orthopedics, The Second Xiangya Hospital of Central South University, Changsha, China
- 3 Department of Nutrition, Harvard T.H. Chan School of Public Health, Boston, Massachusetts
Question Could the excess risk of mortality related to obesity be attenuated through the improvement of social isolation and loneliness?
Findings In this cohort study of 398 972 UK Biobank participants, as the index of social isolation and loneliness went from highest to lowest, the risk of all-cause mortality decreased by 36% and 9%, respectively, in people with obesity compared with people without obesity. Social isolation ranked higher than loneliness, depression, anxiety, and lifestyle-related risk factors for estimating the risk of mortality.
Meaning These findings support the improvement of social isolation and loneliness in people with obesity to decrease obesity-related excess risk of mortality.
Importance Individuals with obesity experience markedly higher levels of social isolation and loneliness than those without obesity, but little is known about whether improvement of social isolation or loneliness might attenuate obesity-related excess risk of mortality.
Objective To investigate whether improvement of social isolation or loneliness is associated with lower obesity-related excess risk of mortality.
Design, Setting, and Participants This cohort study included individuals without cancer or cardiovascular disease (CVD) at baseline from the UK Biobank with follow-up beginning in March 2006 and ending in November 2021.
Main Outcomes and Measures All-cause, cancer-related, and CVD-related mortality were estimated.
Results A total of 398 972 participants were included in this study (mean [SD] age, 55.85 [8.08] years; 220 469 [55.26%] women; 13 734 [3.44%] Asian, 14 179 [3.55%] multiracial, and 363 685 [91.16%] White participants). Overall, 93 357 (23.40%) had obesity, and 305 615 (76.60%) did not. During a median (IQR) follow-up of 12.73 (12.01-13.43) years, a total of 22 872 incident deaths were recorded. Compared with participants with obesity with an index of 2 or greater for social isolation, the multivariable adjusted hazard ratios (HRs) for all-cause mortality were 0.85 (95% CI, 0.79-0.91) and 0.74 (95% CI, 0.69-0.80) for participants with obesity and a social isolation index of 1 and 0, respectively ( P for trend < .001); compared with participants with obesity and an index of 2 for loneliness, the HRs and 0.97 (95% CI, 0.89-1.06) and 0.86 (95% CI, 0.79-0.94) for participants with obesity and a loneliness index of 1 and 0, respectively ( P for trend < .001). As the index of social isolation and loneliness went from highest to lowest, the HR for all-cause mortality decreased by 36% and 9%, respectively, in people with obesity compared with people without obesity using the multivariable model. Social isolation was ranked higher than loneliness, depression, anxiety, and lifestyle-related risk factors including alcohol, physical activity, and healthy diet for estimating the risks of all-cause mortality, cancer-related mortality, and CVD-related mortality.
Conclusions and Relevance In this cohort study of UK Biobank participants, a lower index of social isolation or loneliness was associated with a decreased risk of all-cause mortality among people with obesity, and improvement of social isolation and loneliness attenuated obesity-related excess risk of all-cause mortality.
Obesity is a pervasive and escalating global concern. In high-income countries, approximately 30% of the general population is classified as having obesity. 1 Obesity has been consistently related to excess risks of all-cause mortality and mortality due to cardiovascular disease (CVD) and cancer in various populations. 2
Efforts to tackle the issues of social isolation and loneliness have been simmering for decades. 3 The US Surgeon General has highlighted an urgent need to confront the public health crisis posed by loneliness. 4 In our recent work, 5 we observed a significant association between loneliness and risk of CVD in patients with diabetes. Mounting evidence shows that people with obesity encounter markedly higher levels of social isolation and loneliness than those without obesity. 6 - 8 Social isolation and loneliness are crucial aspects of social determinants of health that represent distinct components of social contact. 9 , 10 Social isolation refers to the amount of social interaction observed in behavior, while loneliness typically pertains to emotional experiences linked to social relationships’ quality. 11 , 12 Previous studies on the general population have found that social isolation 13 , 14 and loneliness 15 , 16 were significantly associated with elevated risks of mortality. We hypothesized that improvement of isolation and loneliness would be associated with a reduction of obesity-related excess risk of mortality. To our knowledge, no prospective study has yet addressed such a hypothesis.
In this study, our aim was to examine the associations of improvement of social isolation and loneliness with the risk of mortality in individuals with obesity or without obesity. We also compared people with obesity with matched control participants without obesity to investigate whether the obesity-related excess risk of mortality could be attenuated or eliminated through improving social isolation and loneliness indexes. Additionally, we sought to compare the significance of social isolation and loneliness against lifestyle-related risk factors for mortality.
This cohort study followed the Strengthening the Reporting of Observational Studies in Epidemiology ( STROBE ) reporting guideline. The UK Biobank study was approved by the National Health and Social Care Information Management Board and the North West Multicenter Research Ethics Committee and the institutional review board of Tulane University. All participants provided written informed consent at recruitment to the study. 17
The UK Biobank is a large-scale prospective population-based cohort study with more than 500 000 participants aged 40 to 70 years between 2006 and 2010. 17 The obesity and nonobesity groups were defined as body mass index (BMI; calculated as weight in kilograms divided by height in meters squared) of 30 or greater and less than 30, respectively. 18 In this cohort study, we included 93 357 people with obesity and 305 615 people without obesity. A total of 84 920 people with obesity were then matched for age, sex, and assessment center with 1 control participant without obesity randomly, and 84 920 matched control participants were enrolled in this study (eFigure 1 in Supplement 1 ).
The social isolation and loneliness indexes were constructed using self-reported questionnaires in UK Biobank 12 , 19 (eTable 1 in Supplement 1 ). The social isolation and loneliness index were assessed via 3 and 2 questions, respectively. Given the relatively small number of participants in with social isolation index scores of 3, participants with an index of 3 were combined into index of 2 or greater group. We calculated the total indexes of social isolation and loneliness by summing the individual indexes of the 3 and 2 corresponding indicators, respectively, with a range of 0 to 2 or greater and 0 to 2. Detailed information of scoring methods for social isolation and loneliness are indicated in eTable 1 in Supplement 1 .
The primary results of this study included mortality from all causes, cancer, and CVD. The end date for follow-up was determined as the date of the baseline to death or the censoring date (November 27, 2021), whichever occurred first. The results were categorized according to the International Statistical Classification of Diseases and Related Health Problems, Tenth Revision (ICD-10) . For the current study, we analyzed mortality for all-cause, cancer (codes C00 to C97), and CVD (codes I00 to I99). 20 , 21
Age, sex, race and ethnicity, Townsend Deprivation Index, education years, smoking status, alcohol intake, and hemoglobin A 1c (HbA 1c ) level were obtained directly from UK Biobank. The healthy diet score (eTable 2 in Supplement 1 ) was created according to our previous studies. 22 , 23 We classified participants into 2 groups based on their total moderate physical activity minutes per week, following global recommendations for physical activity and health. 24 One minute of vigorous physical activity was considered equivalent to 2 minutes of moderate physical activity. The 2 groups were defined as follows: less than 150 or 150 or more minutes per week. Depression, anxiety, and eating disorders were defined as having a self-reported history or being diagnosed using ICD-10 codes 25 (eTable 3 in Supplement 1 ). We defined hypertension as having a self-reported history of hypertension, a systolic blood pressure equal to or greater than 140 mm Hg, a diastolic blood pressure equal to or greater than 90 mm Hg, taking antihypertensive medications, or being diagnosed using ICD-10 codes. High cholesterol was defined as having a self-reported history of high cholesterol, taking cholesterol-lowering medications, or being diagnosed using ICD-10 codes. Diabetes was defined as having a self-reported history of diabetes, using insulin, or being diagnosed using ICD-10 codes (eTable 3 in Supplement 1 ). Metformin use and glucocorticoid use were defined as self-reported drug use 26 (eTable 4 in Supplement 1 ). The details of these variables can be found on the UK Biobank website. 27
Continuous variables were presented as mean and SD and categorical variables were presented as counts with percentages. We used Fisher exact tests or Wilcoxon rank-sum tests to examine participant characteristics according to whether participants had obesity. We used Cox regression models to observe the association of social isolation and loneliness with risk of all-cause mortality, cancer-related mortality, and CVD-related mortality in people with obesity. The validity of the proportional hazards assumption was assessed by utilizing Schoenfeld residuals and Kaplan-Meier methods, and all evaluations satisfied the predetermined criteria. The group with the highest index was set as the reference group. The basic model was adjusted for age (years) and sex (male or female). The multivariable model was further adjusted for race and ethnicity (Asian, Black, Chinese, multiracial, White, or other [not further defined in UK Biobank]), Townsend Deprivation Index (continuous), years of education(<15, 15-19 or ≥20 years), smoking status (never, previous, or current smoking), alcohol intake (<3 or ≥3 times/wk), healthy diet score (<3 or ≥3), physical activity (≥150-min/wk or <150-min/wk), depression (yes or no), anxiety (yes or no), eating disorders (yes or no), hypertension (yes or no), high cholesterol (yes or no), diabetes (yes or no), metformin use (yes or no), glucocorticoid use (yes or no) and HbA 1c level (continuous). Then we used the same Cox models when comparing people with obesity with matched control participants. We coded the missing data of categorical covariates and continuous variables using a missing indicator category and mean values respectively. The numbers and percentages of participants with missing covariates are shown in eTable 5 in Supplement 1 . eFigure 2 in Supplement 1 is a directed acyclic graph explaining the associations between the exposures, the outcome, and the covariates.
We further categorized the social isolation status as social isolation (social isolation index ≥2) and non–social isolation (social isolation index <2). 19 , 28 , 29 Similarly, the loneliness status was split into 2 groups: loneliness (loneliness index of 2) and nonloneliness (loneliness index <2). 19 , 28 , 29 To calculate the contribution of social isolation, loneliness, and covariates to the explained relative risk derived from the multivariable model, we used the coxphERR function 30 from Rstudio version 4.1.2 (R Project for Statistical Computing) to observe the relative importance of risk factors in people with obesity.
Two sensitivity analyses were conducted to explore the stability of the results. Participants who died during the first 2 years of follow-up were excluded. Then, all missing covariate data were inputted using chained equations. All results were expressed as the hazard ratio (HR) and 95% CI. SAS version 9.4 (SAS Institute) was used to perform the statistical analysis, and we considered a 2-sided P < .05 as indicating statistically significant differences.
Of the 398 972 included participants (mean [SD] age, 55.85 [8.08] years; 220 469 [55.26%] women; 13 734 [3.44%] Asian, 14 179 [3.55%] multiracial, and 363 685 [91.16%] White participants), 93 357 participants (23.40%) had obesity, and 305 615 (76.60%) did not. Among 93 357 people with obesity, 45 673 (48.92%), 37 881 (40.58%), and 9803 (10.50%) participants were defined as having a social isolation index of 0, 1, and 2 or greater, respectively. The corresponding percentage was 59 342 (63.56%), 26 627 (28.52%), and 7388 (7.91%) for participants with obesity and loneliness index of 0, 1, and 2. The prevalence of social isolation and loneliness in people with obesity was significantly higher than that in people without obesity ( P < .001) ( Table 1 ).
During a median (IQR) follow-up of 12.73 (12.01-13.43) years, a total of 22 872 incident deaths were recorded, including 11 442 cancer-related deaths, 4372 CVD-related deaths, and 7058 other deaths. The unadjusted model and basic model adjusted for age and sex indicated that lower index of social isolation was significantly associated with lower risks of all-cause mortality in people with obesity ( Table 2 ). In the multivariable adjusted model, compared with participants with obesity with the highest social isolation index (≥2), HRs for all-cause mortality were 0.85 (95% CI, 0.79- 0.91) and 0.74 (95% CI, 0.69-0.80) for participants with obesity and a social isolation index of 1 and 0, respectively ( P for trend < .001) ( Table 2 ). Similar results were observed for cancer-related and CVD-related mortality (eTable 5 and eTable 6 in Supplement 1 ). For loneliness, compared with participants with obesity with the highest loneliness index (2), HRs for all-cause mortality were 0.97 (95% CI, 0.89-1.06) and 0.86 (95% CI, 0.79-0.94) for participants with obesity and a loneliness index of 1 and 0, respectively ( P for trend < .001) ( Table 2 ). Similar results were observed in people without obesity ( Table 3 ). Pairwise comparisons appear in eTable 7 and eTable 8 in Supplement 1 . We found that the association of social isolation with all-cause mortality was stronger in people without obesity ( P for interaction = .003). However, we observed that the cumulative risk of all-cause mortality across the 3 groups of social isolation in participants with obesity was still higher than that of participants without obesity and the same social isolation or loneliness index (eFigure 3 in Supplement 1 ). Additionally, we found the association of obesity with all-cause mortality was strengthened by social isolation index (eFigure 4 in Supplement 1 ).
Furthermore, the associations of individual social isolation and loneliness indicators with risk of mortality were analyzed. Almost all the individual indicators of social isolation and loneliness were significantly associated with all-cause mortality and CVD-related mortality in people with obesity (eTable 9 in Supplement 1 ).
The adjusted cumulative hazard curves for the probability of mortality among participants without and with obesity with social isolation or loneliness index of 0, 1, or 2 or greater are presented in the Figure and eFigure 5 in Supplement 1 . To estimate the extent to which the obesity-related risk of mortality could be attenuated through controlling the social isolation and loneliness index, we evaluated the risk of mortality according to the degree of social isolation and loneliness among people with obesity vs matched control participants. Compared with people without obesity, the risks of all-cause mortality among people with obesity constantly decreased with decreasing levels of social isolation and loneliness ( Table 4 ). The findings were consistent in the unadjusted model and multivariable adjusted model. As the index of social isolation and loneliness went from highest to lowest, the HR for all-cause mortality decreased by 36% and 9% in people with obesity compared to people without obesity using multivariable model. Similar results were observed for CVD-related mortality (eTable 10 in Supplement 1 ). Additionally, we found a similar pattern for all-cause mortality among those with more severe obesity classes (eTable 11 in Supplement 1 ). Moreover, the risks of all-cause mortality and CVD-related mortality among people with obesity constantly decreased with the individual indicator of social isolation and loneliness control (eTable 12 in Supplement 1 ).
Relative importance analysis indicated that social isolation ranked fourth, fourth, third, and eighth in relative strength for risk of all-cause mortality, cancer-related mortality, CVD-related mortality, and other mortality, respectively, while loneliness was in fourteenth place for risk of all-cause mortality in participants with obesity. Social isolation was ranked higher than loneliness, depression, anxiety, and lifestyle-related risk factors including alcohol, physical activity, and healthy diet (eFigure 6 in Supplement 1 ).
The associations of isolation and loneliness with risk of mortality did not change significantly when we excluded participants who died during the first 2 years of follow-up (eTables 13 and 14 in Supplement 1 ). Additionally, when all the missing covariates were inputted using multiple imputation, the results remained stable (eTables 15 and 16 in Supplement 1 ).
In this prospective cohort study with a median follow-up of 12.73 years, we found that improvement of social isolation and loneliness were associated with lower risks of all-cause mortality. Additionally, the obesity-related excess risks of all-cause mortality decreased with decreased indexes of social isolation and loneliness. Furthermore, social isolation was ranked as the fourth strongest factor, while loneliness was ranked the fourteenth, in risk of all-cause mortality compared with other lifestyle-related risk factors.
For the first time to our knowledge, our study found significant associations of social isolation and loneliness with all-cause mortality in people with obesity. Our findings are supported by several previous studies conducted in the general populations, in which positive correlations of social isolation and loneliness with mortality were observed. 31 , 32 A cohort study from Finland showed that social isolation was related to a 26% increased risk of all-cause mortality in the general population when separately adjusting for socioeconomic factors, biological factors, depressive symptoms, cognitive performance, health-related behaviors, and self-rated health. 12 Another study using data from the Swedish Panel Study of Living Conditions of the Oldest Old (SWEOLD) Study indicated that social isolation and loneliness were associated with an increased all-cause mortality risk. 16
Obesity, social isolation, and loneliness are all associated with many health issues. 33 , 34 A lack of social support may exacerbate the health-risk behaviors of people with obesity including smoking, inactivity, and unhealthy diets and might also neglect health-protective behaviors, such as adherence to medical recommendations. 35 Moreover, those who live alone or lack social contacts may be at a heightened risk of death if they develop acute symptoms because they might not have a strong network of confidantes to urge them to seek medical attention. 36 Addressing social isolation and loneliness in individuals with obesity may potentially help improve unhealthy lifestyles, provide better psychological support, and encourage people at high risk to seek medical assistance when necessary.
It is not surprising that the protective association between improvement of social isolation and all-cause mortality was stronger in people without obesity than those with obesity. The reason why the association between social isolation and mortality is relatively weak among people with obesity may be partly because obesity introduces various biological complications that can increase mortality risk, such as cardiovascular problems, type 2 diabetes, and chronic inflammation. These health issues closely related to obesity might mask or override the protective effects of improvement of social isolation on mortality in the obese population, making the association less pronounced compared with individuals without obesity. Importantly, our findings emphasize that more intensive interventions are needed to improve social isolation in people with obesity than people without obesity to lower the risk of mortality.
We observed that social isolation was significantly associated with CVD-related mortality in people with obesity, which may be attributed to the following reasons. CVDs are the leading cause of death globally, 37 with relatively high incidence and mortality rates. The association of social isolation with CVD-related mortality is pronounced due to a larger population affected. Additionally, social connections and social support play an important role in heart health. Social interaction and support may reduce stress, promote healthy behaviors, provide emotional support, 38 and reduce the risk of CVD through positive social engagement. 39 Social isolation may weaken these factors, leading to an increased risk of CVD. Additionally, we compared the relative importance of social isolation and loneliness to depression, anxiety, the Townsend Index, and lifestyle-related risk factors in risk of mortality among people with obesity and found that social isolation ranked higher than loneliness, depression, anxiety, and lifestyle-related risk factors, including alcohol, physical activity, and healthy diet, which indicated that social isolation played a significant role in estimating the risk of mortality.
Our study indicated that social isolation was more strongly associated with mortality than loneliness, consistent with a previous study in a general population. 12 Social isolation and loneliness are distinct factors that correlate differently with health outcomes and mortality. Social isolation measures the scarcity of contact with others and related health resources, while loneliness reflects a sense of detachment potentially linked to emotional states like depression. 12 Individuals can experience loneliness even when married or living with others. 40 Our results suggest that improvement of social isolation may provide more benefits for reducing risks of all-cause mortality and CVD mortality than loneliness in people with obesity.
In addition, we analyzed associations of social isolation and loneliness improvement with obesity-related excess mortality, by comparing with matched individuals without obesity. Interestingly, we found that obesity-related excess risk of all-cause mortality and CVD-related mortality could be attenuated by controlling social isolation or loneliness. The risk of all-cause mortality among people with obesity constantly decreased with decreasing levels of social isolation or loneliness. Improvement of social isolation in people with obesity could provide more benefits for reducing obesity-related risk of mortality than loneliness.
This study has several strengths, including a prospective study design, a large sample size of people with obesity with data on social isolation and loneliness, and comprehensive information on covariates. However, there are also several limitations to consider. First, no data of duration of loneliness or social isolation and stability can be obtained from the UK Biobank cohort. Second, we did not adjust for cognitive function due to a limited number of participants completing the test, which warrants future research. Third, the study sample included a relatively low percentage of non-White European participants, and generalization of our findings to other racial and ethnic groups requires further investigation. Fourth, the social isolation and loneliness indexes were constructed from simple questions, which might not fully capture the complex social networking and interaction phenomenon. Fifth, the observational nature of the study limited causal inference. Sixth, UK Biobank participants may have healthier behaviors, potentially limiting the generalizability of findings. However, a representative population may not be necessary for assessing exposure-disease associations.
In this cohort study of UK Biobank participants, we found that improvement in social isolation and loneliness were associated with a lower risk of all-cause mortality in people with obesity. Social isolation was more important to the estimation of risk of all-cause mortality than loneliness, depression, anxiety, and lifestyle-related risk factors, including alcohol, physical activity, and healthy diet. Importantly, our results indicated that control of social isolation and loneliness might attenuate the obesity-related excess risk of all-cause mortality. Our findings lend support to social isolation and loneliness control to decrease the risk of all-cause mortality in people with obesity.
Accepted for Publication: December 1, 2023.
Published: January 22, 2024. doi:10.1001/jamanetworkopen.2023.52824
Open Access: This is an open access article distributed under the terms of the CC-BY License . © 2024 Zhou J et al. JAMA Network Open .
Corresponding Author: Lu Qi, MD, PhD, Department of Epidemiology, Tulane University School of Public Health and Tropical Medicine, 1440 Canal St, Ste 1724, New Orleans, LA 70112 ( [email protected] ).
Author Contributions: Dr Qi had full access to all of the data in the study and takes responsibility for the integrity of the data and the accuracy of the data analysis.
Concept and design: Zhou, Wang, Qi.
Acquisition, analysis, or interpretation of data: Zhou, Tang, Li, Heianza.
Drafting of the manuscript: Zhou, Qi.
Critical review of the manuscript for important intellectual content: All authors.
Statistical analysis: Zhou, Tang, Wang, Qi.
Obtained funding: Zhou, Qi.
Administrative, technical, or material support: Zhou, Qi.
Supervision: Zhou, Qi.
Conflict of Interest Disclosures: None reported.
Funding/Support: The study was supported by grants HL071981, HL034594, and HL126024 from the National Heart, Lung, and Blood Institute; grants DK115679, DK091718, DK100383, and DK078616 from the National Institute of Diabetes and Digestive and Kidney Diseases; and grant 2P20GM109036-06A1 (Sub-Project ID 7233) from the National Institute of General Medical Sciences.
Role of the Funder/Sponsor: The funders had no role in the design and conduct of the study; collection, management, analysis, and interpretation of the data; preparation, review, or approval of the manuscript; and decision to submit the manuscript for publication.
Data Sharing Statement: See Supplement 2 .
- Register for email alerts with links to free full-text articles
- Access PDFs of free articles
- Manage your interests
- Save searches and receive search alerts

An official website of the United States government
The .gov means it’s official. Federal government websites often end in .gov or .mil. Before sharing sensitive information, make sure you’re on a federal government site.
The site is secure. The https:// ensures that you are connecting to the official website and that any information you provide is encrypted and transmitted securely.
- Publications
- Account settings
Preview improvements coming to the PMC website in October 2024. Learn More or Try it out now .
- Advanced Search
- Journal List

Forecasting the prevalence of overweight and obesity in India to 2040
Shammi Luhar
1 Department of Population Health, London School of Hygiene & Tropical Medicine, London, England, United Kingdom
2 Department of Public Health and Primary Care, University of Cambridge, Cambridge, England, United Kingdom
Ian M. Timæus
3 Centre for Actuarial Research, University of Cape Town, Cape Town, South Africa
Rebecca Jones
4 Laney Graduate School, Emory University, Atlanta, Georgia, United States of America
Solveig Cunningham
5 Hubert Department of Global Health, Emory University, Atlanta, Georgia, United States of America
Shivani A. Patel
Sanjay kinra, lynda clarke, rein houben.
6 Department of Infectious Disease Epidemiology, London School of Hygiene & Tropical Medicine, London, England, United Kingdom
Associated Data
The National Family Health Survey data used to support the findings of this study have been deposited in the Measure DHS repository (available at: https://www.dhsprogram.com/data/available-datasets.cfm ). The Study on global AGEing and adult health data have been deposited in the WHO Multi-Country Studies Data Archive (available at http://apps.who.int/healthinfo/systems/surveydata/index.php/catalog/sage ). Both of the above data sets are available to download for researchers who satisfy the criteria to access confidential data. The publicly available Sample Registration System data have been deposited in the Office of the Registrar General & Census Commissioner repository (available at: http://censusindia.gov.in/vital_statistics/SRS_Based/SRS_Based.html ).
In India, the prevalence of overweight and obesity has increased rapidly in recent decades. Given the association between overweight and obesity with many non-communicable diseases, forecasts of the future prevalence of overweight and obesity can help inform policy in a country where around one sixth of the world’s population resides.
We used a system of multi-state life tables to forecast overweight and obesity prevalence among Indians aged 20–69 years by age, sex and urban/rural residence to 2040. We estimated the incidence and initial prevalence of overweight using nationally representative data from the National Family Health Surveys 3 and 4, and the Study on global AGEing and adult health, waves 0 and 1. We forecasted future mortality, using the Lee-Carter model fitted life tables reported by the Sample Registration System, and adjusted the mortality rates for Body Mass Index using relative risks from the literature.
The prevalence of overweight will more than double among Indian adults aged 20–69 years between 2010 and 2040, while the prevalence of obesity will triple. Specifically, the prevalence of overweight and obesity will reach 30.5% (27.4%-34.4%) and 9.5% (5.4%-13.3%) among men, and 27.4% (24.5%-30.6%) and 13.9% (10.1%-16.9%) among women, respectively, by 2040. The largest increases in the prevalence of overweight and obesity between 2010 and 2040 is expected to be in older ages, and we found a larger relative increase in overweight and obesity in rural areas compared to urban areas. The largest relative increase in overweight and obesity prevalence was forecast to occur at older age groups.
The overall prevalence of overweight and obesity is expected to increase considerably in India by 2040, with substantial increases particularly among rural residents and older Indians. Detailed predictions of excess weight are crucial in estimating future non-communicable disease burdens and their economic impact.
Approximately 39% of the global adult population were classified as overweight (Body Mass Index (BMI) 25.0–29.9 kg/m 2 ) or obese (BMI > 29.9kg/m 2 ) in 2014; a doubling since 1975[ 1 ]. Whereas the prevalence of obesity was 6.4% among women and 3.2% among men in 1975, it had risen to 14.9% and 10.8%, respectively by 2014[ 1 ]. In developing countries like India, the increasing prevalence of overweight and obesity has coincided with the demographic and epidemiological transitions, in which mortality and fertility have declined, and lifestyle-related diseases have become more common[ 2 – 4 ].
The prevalence of overweight and obesity in India is increasing faster than the world average. For instance, the prevalence of overweight increased from 8.4% to 15.5% among women between 1998 and 2015, and the prevalence of obesity increased from 2.2% to 5.1% over the same period[ 5 – 7 ]. This fast-paced growth has been accompanied by notable increases in the burden of non-communicable diseases (NCDs). Whereas in 1990 the number of life years lost to disability (DALYs) attributable to communicable, maternal, neonatal and nutritional disorders exceeded that attributable to NCDs in virtually all of India’s states, currently the opposite is true[ 3 ]. Given the extent of the increase in prevalence of overweight and obesity, and its relationships with NCDs[ 8 ], reliably predicting its future prevalence has become increasingly important.
Despite this, few studies have attempted to estimate future trends in overweight and obesity in India. One study that reports on global trends estimated that 27.8% of all Indians would be overweight, and 5.0% obese, by 2030[ 9 ]. Another study estimated that around 20% of rural Indian adults will be either overweight or obese by 2030[ 10 ]. However, these previous studies have merely extrapolated previous trends in prevalence without accounting for a changing population at risk of becoming overweight or obese which declines as the proportion of the population classified as overweight or obese increases.
Simulation models offer a more sophisticated alternative to the extrapolation of secular trends and may produce more accurate forecasts. For example, as an internally logical system, the population at-risk of becoming overweight or obese is regularly updated at each forecasted time interval. Such models therefore allow the incorporation of the impact on future prevalence of past increases in the incidence of overweight or obesity[ 11 ]. Additionally, the logical framework enables the estimation of potential impacts of policy decisions, directed at the incidence of overweight and obesity[ 11 , 12 ], and identification of at-risk subpopulations[ 11 , 13 , 14 ]. This analysis brings together nationally-representative data from a range of publicly available sources in a dynamic simulation model to forecast the future prevalence of overweight and obesity in India to 2040 among adults aged 20–69 years.
- National Family and Health Survey (NFHS) . The nationally-representative NFHS collects health and demographic data among women aged 15–49 years and men aged 15–54 years. NFHS 3 (2005–06) interviewed 124,385 and 74,369 adult women and men respectively, and NFHS 4 (2015–16) contains data on 625,000 adult women and 93,065 adult men[ 6 , 7 ].
- The Study on global AGEing and adult health (SAGE) . SAGE Waves 0 (2002–04) and 1 (2007–10) contain longitudinal health and demographic data on people aged 50 or more years from six states which are believed to be nationally-representative [ 15 ]. Wave 0 collected health information on 2559 adults aged 50 or more years, whereas Wave 1 collected data on approximately 3000 men and 3000 women aged 50 or more years.
- Sample Registration System (SRS) . The SRS reports sex- and residence-specific abridged life tables by five-year age groups for each state for every year between 1997 and 2015[ 16 – 21 ]. The SRS dually records deaths using representative samples from across the country[ 22 ].
- United Nations World Population Prospects 2019 and World Urbanization Prospects 2018 . The 2019 round of the World Population Prospects includes population projections and estimates by the Population Division of the Department of Economic and Social Affairs of the United Nations Secretariat[ 23 ]. The Division uses the cohort-component method for each country and major geographical region to produce population projections under a number of different future fertility scenarios. Separate urban and rural projections that are consistent with the national projections are reported by the UN World Urbanization Prospects 2018[ 24 ].
Model inputs
From these data sources, we extracted the following model inputs for the age, sex and residence-specific forecasts of overweight prevalence in India:
Age-specific prevalence of overweight and obesity
We estimated the prevalence of overweight and obesity among individuals aged 20–49 using the BMI variable in NFHS-3 and NFHS-4, whereby individuals with a BMI>24.9kg/m 2 and <30.0kg/m 2 were classified as overweight, and those with a BMI>29.9kg/m 2 were classified as obese following the World Health Organization’s (WHO) recommendations[ 8 ]. Pregnant women (5.2% of women in NFHS 3 and 4.4% in NFHS 4) were excluded from our sample as their pregnancy could give misleadingly high BMI scores. We used survey weights to calculate age-specific prevalence accounting for the complex survey design and based the baseline (2010) age-specific prevalence on the mid-point prevalence of the two surveys. Among individuals aged 50–69, we used the BMI variable, and the same cut-offs, in SAGE wave 0 and 1 to estimate the overall age-specific prevalence and applied the overall relative risk of overweight and obesity among urban and rural residents to obtain residence-specific prevalence estimates. The prevalence estimates from the data are included in the S2 File .
Age-specific incidence of overweight and obesity and age-specific rates of urbanization
We used the changing prevalence of overweight and obesity between 2005 and 2015 among the population aged 20–49 years to estimate the age-specific incidence of overweight among the underweight/normal weight population, and incidence of obesity among overweight individuals in our baseline year of 2010. We used the iterative intracohort interpolation procedure[ 25 ] whereby the observed changes in overweight status to specific cohorts are translated into age-specific rates for the inter-survey interval (a more detailed explanation is presented in S1 File ). The age-specific rates were estimated separately by sex, residency (urban and rural) and age (20–24, 25–29, 30–34, 35–39, 40–44, 45–49 years).
Age-specific rates of urbanization were also calculated by the same method, using the age-specific proportions of the population in urban and rural areas in the two NFHS surveys.
For those aged 50–69, we calculated the incidence of overweight and obesity using longitudinal data from SAGE waves 0 and 1 for men and women separately by dividing the number of incident cases by the person-years of exposure. As information on the exact time an incident case occurred was not available, incident cases were assumed to have occurred at the halfway-point between the two waves. We calculated an overall incidence rate among men and women separately, and indirectly standardized the rates using the age-distribution of obesity incidence from a study in the United States[ 26 ] that used data from the Behavioral Risk Factor Surveillance System in order to obtain net rates for the following age groups: 50–54, 55–59, 60–64, and 65–69 years ( S1 File ).
We fitted a spline to smooth the age-specific incidence rates across the lifespan and used age-specific incidence by the five-year age groups in the final analysis.
We incorporated the potential for individuals to transition from overweight and obesity to lower BMI groups by modelling gross, rather than net incidence rates at all ages. Remission refers to reverse transitions, whereby the simulated population is able to transition from a state of ‘Obese’ to ‘Overweight’, and ‘Overweight’ to ‘Not Overweight/Obese’. We used rates of remission that allowed our model with gross rates to closely match the measured age-specific prevalence in 2015 from NFHS-4. To estimate remission in older ages, we applied an odds ratio of remission in older ages (50+ years), relative to younger ages. A prospective study in rural India carried out between 2008 and 2017[ 27 ] found an elevated odds of remission from higher to lower BMIs of 1.74 and 2.12 among older aged men and women, relative to younger counterparts.
Current and future age-, sex- and urban/rural residence- specific mortality rates
We converted conditional mortality probabilities reported by the SRS to age-specific mortality rates from 1997 to 2013 using standard demographic procedures[ 28 ] and used these rates to forecast future mortality to 2040 using the Lee-Carter method[ 29 , 30 ]. In brief, the Lee-Carter method summarizes a series of sets of age-specific mortality rates for successive periods of time by its average age-schedule, age-specific deviations from the average age-schedule, and the trend in the overall level of mortality over time. The forecast is contingent on the extrapolation of this latter parameter ( S1 File ).
Relative risk of dying for those overweight or obese compared with those who are not
We adjusted the forecasted mortality rates to account for differential mortality between overweight and obese individuals and those who are in lower weight categories. Relative risks of dying, based on BMI group, were adopted from the findings reported in a study that examined the association between BMI and mortality in Mumbai[ 31 ]. This study reported relative risks of dying for those who are overweight excluding obesity (OW), relative to normal (N) weight, those who are obese (OB) relative to normal weight, and those who are underweight (UW) relative to normal weight. The authors report risk ratios, along with confidence intervals, for men and women aged 35–59 and 60 or more, separately. As the study did not calculate risk ratios for individuals aged 20–34, we assumed that the relative risk of dying at 35–59 also prevailed at these ages. We obtained separate relative risks of dying for those who are overweight and obese relative to new reference categories of ‘not overweight’ and ‘not obese’ using a basic algebraic approach ( S1 File ), and subsequently used these relative risks to calculate BMI specific rates of mortality using the population level mortality rate. Below we present an example of the calculation of obesity-specific mortality rates.
Where δ x , t O B refers to the prevalence of obesity in age group a at time t , and R a is the relative risk of dying among obese adults relative to non-obese counterparts aged a.
Age-, sex- and urban/rural residence-specific population in 2010
Estimates of the 2010 urban and rural population were taken from the World Urbanization Prospects[ 24 ] and disaggregated using the average age-group and sex structure of urban and rural populations separately, which we obtained from the NFHS-3 and NFHS-4[ 6 , 7 ].
Population aged 20–24 entering the simulation at every interval
The new entrants aged 20–24 that join the urban and rural populations in each time interval were estimated using the projected population aged 20–24 from the medium fertility projection scenario by the United Nations’ World Population Prospects[ 23 ] and split into the projected proportions of the population in urban and rural areas from the World Urbanization Prospects[ 24 ].
We estimated the future prevalence of overweight and obesity through 2040 using an age-stratified simulation model based on a system of multi-state lifetables[ 32 ], that moved individuals through mutually-exclusive health states depending on our estimated transition rates as they age. The model operated in discrete time, estimating the prevalence of overweight and obesity separately among men and women in urban and rural areas separately, at five-yearly intervals between 2010 and 2040. The system of multi-state lifetables is shown in Fig 1 .

Most epidemiological studies apply transition probabilities to the population at risk of a transition at the beginning of a time period to determine the distribution of the population across health states in a succeeding time period, without taking account of a changing population at risk within a time interval. This is due to individuals being able to enter and re-enter a particular BMI group within a time interval. In order to sufficiently account for this, we employed a multi-state lifetable system first developed by Schoen and Nelson (1974) who addressed questions about flows in and out of marriage in the UK and USA. Rather than work with transition probabilities derived from the rates, this approach to forecasting health states uses the rates directly. A detailed description of this method is included in S1 File .
Assumptions
Firstly, between 2005 and 2015 the pace of increase in prevalence of overweight and obesity is faster in rural populations than in urban areas. As the prevalence of overweight and obesity among the 20–24 population is not determined within the model, we assumed that the rate of increase in this age group observed from the overweight and obesity prevalence in the NFHS data decreased and converged towards a 0% increase by 2040, so as not to overinflate our estimates. Additionally, our baseline forecasts, assumed that the empirically estimated overweight and obesity incidence for each demographic group for the baseline year (2010) applied throughout the forecasting period. This assumption provides a clear and easily interpretable counterfactual scenario against which to compare other scenarios whereby incidence is allowed to vary over the forecast period. For simplicity, we assumed that there is no migration in and out of India. Finally, it was assumed that the rate of urbanisation measured between 2005 and 2015 prevailed throughout the forecast period.
Uncertainty analyses
To obtain uncertainty bounds for our estimates we simultaneously selected random prevalence, incidence and mortality rates from the distributions that informed their uncertainty. We repeated the simulation 5000 times and we reported the median estimate as the final point estimate, whilst the range of estimates for each population subgroup informed our uncertainty bounds. The analysis was conducted in R version 3.5.1.
Sensitivity analyses
The future incidence of overweight may continue to increase due to economic development creating an increasingly obesogenic environment. To explore the implications of this potential trend, we included additional scenarios. Scenario 1 involved examining the effect on future prevalence the incidence parameter increasing at a constant annual rate of 1%. In Scenario 2, we examined the effect on future overweight and obesity of the urbanization rate being set at its upper confidence bound throughout the forecast period. Finally, Scenario 3 examined the extent to which the total prevalence of overweight and obesity prevalence would change if no further urbanization were to take place to 2040. Although unrealistic, this provides an understanding as to the extent to which the future increase in prevalence is driven by future urbanization.
We also performed additional analysis using the South Asian BMI cut-offs values. Some advocate the use of these BMI cut-offs due to a stronger positive association between BMI and body fat observed in South Asians compared to White Caucasians, and consequently an elevated disease risk at lower BMI levels[ 33 , 34 ]. Under this assumption, a BMI between 23.0 kg/m 2 and 27.5 kg/m 2 was used to define individuals who are overweight, and a BMI greater than 27.5 kg/m 2 was used to define obesity[ 35 ].
Ethics statement
The analysis of secondary data was approved by the London School of Hygiene & Tropical Medicine’s Research Ethics Committee (ref: 16190).
Nationally, our model estimates that the prevalence of overweight among women will increase from 14.7% (13.7%-15.5%) to 27.4% (24.5%-30.6%) between 2010 and 2040, whereas the prevalence of obesity is forecasted to increase from 4.4% (4.0%-4.9%) to 14.0% (10.5%-16.9%) over the same period ( Fig 2 ). Among men, the prevalence of overweight and obesity is forecasted to increase from 12.6% (11.6%-13.7%) and 2.4% (2.1%-2.8%) in 2010 to 30.5% (27.4%-34.4%) and 9.5% (5.4%-13.3%), respectively, by 2040 ( Fig 2 ).

The prevalence of overweight and obesity is forecasted to remain higher in urban areas, compared with rural areas, reaching 32.3% (27.8%-37.1%) and 19.7% (14.0%-24.5%), respectively among urban women and 37.1% (31.6%-43.8%) and 11.4% (5.7%-16.8%), respectively, among urban men by 2040. However, the relative increase will be larger in rural areas, where the baseline model forecasts that the prevalence of obesity among women will be 4 times higher in 2040 than in 2010 in rural areas, compared to a 2.2 times higher prevalence of obesity in urban areas over the same period.
The model also predicts larger increases in the prevalence of overweight and obesity in older age groups. Using the broad age groups in Tables Tables1 1 and and2, 2 , we find that, for example, among men, the prevalence of overweight in urban areas among 55-69-year-olds is predicted to almost quadruple from 10.8% (8.4%-13.6%) to 38.5% (31.3%-48.0%) between 2010 and 2040, whereas the prevalence of overweight in rural areas is predicted to increase from 4.7% (3.5%-5.9%) to 30.5% (23.6–37.0%). On the other hand, our model predicts that younger age groups in our model will experience the smallest absolute increase in the overweight (Figs (Figs3 3 and and4 4 ).

Under the assumption of a 1% annual increase in incidence of overweight and obesity from 2015, we expect the national prevalence of overweight to increase to 29.9% (26.7%-33.7%) by 2040 among women and to 33.1% (29.4%-37.3%) over the same period for men ( Fig 5 ). Over the same period, we expect the national prevalence of obesity to increase to 16.9% (11.9%-21.3%) among women and 12.3% (7.8%-17.0%) among men. Under the high urbanization scenario, we find that the future national prevalence of overweight between 2010 and 2040 will increase to 28.4% (25.5%-31.8%) among women, compared to 27.0% (23.7%-30.5%) under an assumption of no further urbanization. The high urbanization scenario for men finds a 1.4% higher percentage point prevalence of overweight among men in 2040, compared to the scenario of no further urbanization. The prevalence of obesity in 2040 does not vary notably between these scenarios ( Fig 5 ).

Overall, we predict that the prevalence of overweight will increase approximately double among Indian adults aged 20–69 years between 2010 and 2040, whilst the prevalence of obesity is expected to increase approximately three-fold over the same period. Specifically, amongst men, we predict that the prevalence of overweight and obesity respectively will reach around 30% and 10%, whilst 27% and 14% of women are expected to be overweight and obese, respectively, by 2040. Our model additionally predicts an ageing distribution of overweight and obesity, with the largest relative increases in prevalence observed among the 55-69-year age group (in this age group the prevalence of obesity among women is predicted to increase almost 6-fold in rural areas and almost 5-fold in urban areas over the forecast period). Whilst prevalence of overweight and obesity is expected to be higher in urban areas throughout the forecast period, we predict larger relative increases in their prevalence in rural areas.
Our forecasting model has a number of limitations. Firstly, we determine the future prevalence of the new cohorts of 20-24-year individuals outside of the model, where we applied a declining rate of increase in prevalence, so as to not grossly inflate future prevalence in this age group to unrealistic levels. Studies have documented increasing overweight prevalence among young adults in India, especially among men and high socioeconomic status individuals[ 36 ].
Secondly, we used standard global BMI thresholds over which there is some controversy. Some researchers advocate for using lower BMI thresholds for South Asians[ 35 ] due to a higher percentage of body fat among South Asians compared to Caucasians of the same BMI[ 33 , 34 ]. Some research has documented a nearly 10–15% higher prevalence of overweight among individuals with Asian heritage if Asian-specific cut-offs are used[ 34 ]. Others have found no higher risk of mortality among obese Asians compared to obese non-Asians, and advocate for global consistency in the definition of overweight and obesity[ 9 , 37 , 38 ]. We opted to use global cut-offs for this reason and in order to facilitate direct comparison of the predictions with similar forecasting studies in Western countries[ 39 – 41 ]. We sought to remedy this limitation by performing sensitivity analysis using South-Asian BMI cut-offs, and identified potential underestimation of our results ( S2 File ). For instance, among urban men, we identified a potential underestimation of the 2040 obesity prevalence of around 20 percentage points, suggesting that using global cut-offs may underestimate the future overall public health challenge related to excess weight in India.
Thirdly, our assumption of no migration in and out of India may slightly bias our findings if individuals leaving India to elsewhere are more likely to be overweight or obese than individuals who remain or enter. Any bias attributable to our assumption of zero migration in an out of India is however likely to be negligible as the number of annual net migrants (minus 2.5 million in 2017 according to the World Bank[ 42 ]) currently represents less than 1% of the total population[ 23 ].
Finally, it would have been desirable to have accommodated the changing socioeconomic patterning of overweight and obesity in India in our forecasts. Studies have shown that rural and lower SEP Indians are at increasing risk of overweight and obesity, with significant variation in this patterning sub-nationally[ 43 , 44 ]. However, due to the uncertainty in how these socioeconomic patterning trends will evolve throughout the forecasting period, the inclusion of socioeconomic status in our model has the potential to, at best, make the predictions marginally more accurate, and at worst, considerably more uncertain, and consequently, we opted for a relatively parsimonious model.
Despite these limitations, our study has a number of strengths. Firstly, we have quantified the future prevalence of overweight and obesity in India using the most recent nationally-representative publicly available data. Our model is able to reflect the changing demographic profile of India in future estimates of the prevalence of overweight and obesity and, in addition, to incorporate future rates of urbanization. Additionally, it models the future age- and sex-specific prevalence of overweight as a function of past and current age- and sex-specific incidence and mortality; reflecting the real-life lag between demographic changes, changes in incidence and mortality and their effect on the overall prevalence at various ages in the future. Unlike previous studies predicting future prevalence of overweight and obesity in India[ 9 , 10 ], we forecasted prevalence for age-stratified subgroups as well as generating aggregated forecasts, putting emphasis on demographic groups that are expected to experience particularly high increases in prevalence.
Few studies have attempted to forecast the future prevalence of overweight in India. One study from 2005 predicted that the prevalence of overweight among Indian adults, assuming a continuation of past trends, will increase to 27.8% by 2030, whist the prevalence of obesity is predicted to reach 5.0%[ 9 ]. The overweight estimations closely resemble our predictions for men and are slightly above what we predict for women. However, our model predicts a considerably higher prevalence of obesity by 2030, with 11.5% of women and 7.4% of men predicted to be obese by 2030.
Another study, focusing on rural India estimated that the prevalence of overweight will approach 20% among men and just exceed 20% among women aged 18 and over by 2030[ 10 ]. We, on the other hand, expect 29.1% of men and 29.9% of women to be either overweight or obese by 2030. The discrepancy between these two separate findings may indeed be due to the different methodologies adopted but is more likely explained the fact that our study included older age groups among whom overweight and obesity prevalence is expected to increase most substantially by 2030.
The differences between our results and previous forecasts of the prevalence of overweight in India may also be explained by our attempts to take into account some of the heterogeneity in the incidence of overweight an obesity and mortality sub-nationally, estimating urban and rural outcomes separately for men and women. Also, instead of making a priori assumptions about the future prevalence of overweight and obesity, for instance a linearly increasing prevalence rate, we model future prevalence as a function of a continuously updated ‘population at-risk’. Although we expect our baseline results to be relatively conservative, as we fix age-specific incidence rates over the forecast period, we expect them to be more accurate than previous attempts. This is due to our use of the most up-to-date data, and the fundamental differences in modelling approaches.
The ageing age distribution of overweight prevalence is likely to be driven by a cohort effect. Previous research has reported a peak in the prevalence of overweight in the 40–49 age group in 2005 in India, whereas in more economically developed countries, the prevalence in the same year peaks in the 60–69 age group[ 9 ]. Our finding of an older age distribution of both overweight and obesity prevalence in 2040, compared with 2010, may be associated with India’s increasing resemblance to higher-income countries in terms of overall prevalence of overweight and economic development. When we tested our forecasts holding future mortality rates at the 2010 level, future prevalence did not notably differ from the forecasts in which future mortality was allowed to decline. Consequently, previous and continuing increases in longevity are not likely to be an important driver of this ageing age distribution of overweight.
We confirmed that our model predictions were very similar to the 2015 age-specific prevalence estimates reported by the NFHS. Another way we assessed the ability of our model to accurately predict future overweight was to compare our output with collected data on overweight prevalence from a data source that was not used in the parameterization of our model. The National Nutrition Monitoring Bureau (NNMB) reports that in 2017 the prevalence of overweight in urban areas was 34% among men. In our model, the prevalence of overweight and obesity combined among urban men is 35%, and the NNMB estimate falls comfortably within our uncertainty bound of 29.5%–40.3%. The NNMB also reports a point estimate of 44.0% prevalence among urban women in 2017, falling within our uncertainty bound of 34.7%– 45.8%, although our point estimate is lower, at 40.4%. We would expect the interval around their estimate to considerably overlap with ours, however this interval was unavailable. Although NNMB estimates fall comfortably within our uncertainty bound, differences between point estimates can derive from a number of sources. Firstly, different sampling frames are used in the surveys, whereby the NNMB in urban areas selects a sampling frame from under half (16) of Indian states they believe to accurately reflect national trends[ 45 ]. Additionally, the NNMB included individuals aged 70 years or more, the majority of whom are likely to be urban women due to their higher life expectancy[ 21 ].
We have found that the prevalence of obesity in 2040 is expected to be lower than levels that are currently observed in some of the world’s most industrialized economies, implying that India could be susceptible to considerable further increases in obesity prevalence beyond 2040. For instance, a recent survey has found that using the same BMI cut-offs as in our study, 40.4% and 35.0% of women and men in the US, respectively were classified as obese in 2013–14[ 46 ], whereas we find that in urban India, a relatively obesogenic environment, 19% of women and 11% of men are likely to be obese by 2040, however, this is one of our most conservative estimates, assuming a constant rate of incidence over the forecast period. Nevertheless, a 1% annual increase in incidence, corresponding to a 35% overall increase in incidence over the forecast period, only leads to a 5 percentage point higher prevalence in combined overweight and obesity by 2040, suggesting that much of the future forecasted prevalence will be determined by the changing demographic profile and background BMI trends of India. Attempts to reduce the forecasted prevalence in 2040 may aim to target a reduction in overweight and obesity incidence, starting among children and adolescents yet to pass through the 20-69-year-old population.
The future task of tackling the increasing disease burden associated with the tripling of obesity prevalence will be particularly challenging in India, given its already high burden of infectious diseases[ 3 ], and given that it is soon expected to have the largest population in the world [ 23 ]. Obesity is the main risk factor for a range of NCDs, including diabetes. A meta-analysis of prospective cohort studies found a 7.19 times higher risk of diabetes among obese individuals compared to normal weight individuals[ 47 ]. Given that people with diabetes are at a high risk of diabetes related complications, including long-term vascular complications affecting the kidneys, heart, and nerves[ 48 ], addressing the growing obesity prevalence and ageing pattern of prevalence, is of great urgency. The demand for medical services to tackle the increasing burden of overweight/obesity related diseases is also likely to increase substantially into the near future. Potential interventions include preventative measures such as screening for diabetes among high risk overweight/obese individuals to increase the proportion of people with diabetes that are diagnosed[ 49 ]. Further efforts may also wish to improve the provision of already established initiatives, particularly the National Programme for Prevention and Control of Cancer, Diabetes, Cardiovascular Disease and Stroke (NPCDCS), that in-part aims to reduce out of pocket expenditure on diabetes healthcare and promote behavioural and lifestyle improvements that reduce the risk of such diseases[ 50 ].
Although the overall prevalence of overweight is expected to be higher in urban areas, our baseline scenario suggests that in urban India future overweight prevalence may begin to plateau during the forecasting period if incidence remains at 2010 levels over the forecast period, while rural areas will continue to experience an increasing prevalence. On the other hand, our model has predicted an almost linear growth in the prevalence of obesity in both urban and rural areas. Irrespective of future incidence or urbanization rate however, our results suggest that a considerably larger proportion of the population in both urban and rural areas will be either overweight or obese by 2040 compared to 2010, driven by the ageing of overweight and obese younger people and increasing prevalence of overweight and obesity in younger ages.
Close monitoring of these populations may be warranted, and interventions to reduce the overall growth in prevalence way wish to target these populations, particularly among populations susceptible to becoming obese for whom the risk of NCDs is substantially higher[ 51 ]. Additionally, health policy planners may wish to pay particular attention to individuals at younger ages to avoid early onset of overweight and the accumulation of overweight prevalence in older age groups.
Given the considerable heterogeneity in customs, diet and economic development between India’s states, these forecasts are likely to mask subnational variation. In future work, an examination of how these forecasts may differ at the state level may be particularly useful for health policy planning as the constitution of India devolves the deliverance of health and nutrition policy to the state level[ 52 ].
Our model is simple enough to apply to other developing countries with similarly limited data, and its flexibility can be demonstrated by appropriately adjusting the transition rates[ 53 ]. Our predictions can also provide the basis of future modelling studies aiming to quantify both monetary costs and future disease burden associated with excess weight in India[ 54 – 58 ].
Our model predicts a considerable increase and an ageing cohort pattern in overweight and obesity across India to 2040, which could have serious implications for future levels of obesity-related diseases, such as diabetes. Initiatives, such as the Integrated National Health Mission[ 59 ], which aims to raise overall population health, may wish to use these forecasts to target sub-populations in which the prevalence of excess weight is likely to be highest in the future. Our findings can be extended to quantify the impact of reductions in the incidence of overweight and obesity among certain subgroups and ages. This information may be crucial in estimating the future burden of NCDs, as well as their economic impact.
Supporting information
This file contains a detailed description of the estimation of model parameters and the procedure followed in the forecasts.
This file contains prevalence of overweight and obesity estimated in the data sets, forecasts by five-year age groups, a table comparing results of forecasting studies in India, and forecasts of overweight and obesity using South Asian BMI cut-offs.
Funding Statement
This work was supported by the Economic and Social Research Council ( https://esrc.ukri.org ) [grant number ES/J500021/1] and the funding was received by SL. The funders had no role in study design, data collection and analysis, decision to publish, or preparation of the manuscript.
Data Availability
- PLoS One. 2020; 15(2): e0229438.
Decision Letter 0
13 Aug 2019
PONE-D-19-17017
Forecasting the Prevalence of Overweight and Obesity in India to 2040
Dear Mr Luhar,
Thank you for submitting your manuscript to PLOS ONE. After careful consideration, we feel that it has merit but does not fully meet PLOS ONE’s publication criteria as it currently stands. Therefore, we invite you to submit a revised version of the manuscript that addresses the points raised during the review process.
We would appreciate receiving your revised manuscript by Sep 27 2019 11:59PM. When you are ready to submit your revision, log on to https://www.editorialmanager.com/pone/ and select the 'Submissions Needing Revision' folder to locate your manuscript file.
If you would like to make changes to your financial disclosure, please include your updated statement in your cover letter.
To enhance the reproducibility of your results, we recommend that if applicable you deposit your laboratory protocols in protocols.io, where a protocol can be assigned its own identifier (DOI) such that it can be cited independently in the future. For instructions see: http://journals.plos.org/plosone/s/submission-guidelines#loc-laboratory-protocols
Please include the following items when submitting your revised manuscript:
- A rebuttal letter that responds to each point raised by the academic editor and reviewer(s). This letter should be uploaded as separate file and labeled 'Response to Reviewers'.
- A marked-up copy of your manuscript that highlights changes made to the original version. This file should be uploaded as separate file and labeled 'Revised Manuscript with Track Changes'.
- An unmarked version of your revised paper without tracked changes. This file should be uploaded as separate file and labeled 'Manuscript'.
Please note while forming your response, if your article is accepted, you may have the opportunity to make the peer review history publicly available. The record will include editor decision letters (with reviews) and your responses to reviewer comments. If eligible, we will contact you to opt in or out.
We look forward to receiving your revised manuscript.
Kind regards,
William Joe
Academic Editor
Journal Requirements:
1. When submitting your revision, we need you to address these additional requirements.
Please ensure that your manuscript meets PLOS ONE's style requirements, including those for file naming. The PLOS ONE style templates can be found at
http://www.journals.plos.org/plosone/s/file?id=wjVg/PLOSOne_formatting_sample_main_body.pdf and http://www.journals.plos.org/plosone/s/file?id=ba62/PLOSOne_formatting_sample_title_authors_affiliations.pdf
2. Please include captions for your Supporting Information files at the end of your manuscript, and update any in-text citations to match accordingly. Please see our Supporting Information guidelines for more information: http://journals.plos.org/plosone/s/supporting-information .
3. Please upload a copy of Figure 6, to which you refer in your text on page 12. If the figure is no longer to be included as part of the submission please remove all reference to it within the text.
[Note: HTML markup is below. Please do not edit.]
Reviewers' comments:
Reviewer's Responses to Questions
Comments to the Author
1. Is the manuscript technically sound, and do the data support the conclusions?
The manuscript must describe a technically sound piece of scientific research with data that supports the conclusions. Experiments must have been conducted rigorously, with appropriate controls, replication, and sample sizes. The conclusions must be drawn appropriately based on the data presented.
Reviewer #1: Yes
Reviewer #2: Yes
2. Has the statistical analysis been performed appropriately and rigorously?
3. Have the authors made all data underlying the findings in their manuscript fully available?
The PLOS Data policy requires authors to make all data underlying the findings described in their manuscript fully available without restriction, with rare exception (please refer to the Data Availability Statement in the manuscript PDF file). The data should be provided as part of the manuscript or its supporting information, or deposited to a public repository. For example, in addition to summary statistics, the data points behind means, medians and variance measures should be available. If there are restrictions on publicly sharing data—e.g. participant privacy or use of data from a third party—those must be specified.
Reviewer #1: No
4. Is the manuscript presented in an intelligible fashion and written in standard English?
PLOS ONE does not copyedit accepted manuscripts, so the language in submitted articles must be clear, correct, and unambiguous. Any typographical or grammatical errors should be corrected at revision, so please note any specific errors here.
5. Review Comments to the Author
Please use the space provided to explain your answers to the questions above. You may also include additional comments for the author, including concerns about dual publication, research ethics, or publication ethics. (Please upload your review as an attachment if it exceeds 20,000 characters)
Reviewer #1: In this paper the author has used a system of multi-state life tables to forecast overweight and obesityprevalence among Indians aged 20-69 years by age, sex and urban/rural residence to2040. The author has improved over the existing methodologies employed for similar projection studies by incorporating the change in future risk of becoming overweight by accounting for change in projected population as obesity and overweight increases over time. They report estimates which are close to those provided by NFHS and NNMB, implying that their results are robust. The paper can prove useful for policy makers in the area of health since prevalence of overweight and obesity is associated with numerous non-communicable diseases. However, more information about the values of the inputs used for the study is desirable.
1) The descriptive analyses related with prevalence of obesity for the years considered (From NFHS data and SAGE) for projection exercise can be included before presenting the results. Moreover, results can be presented for 5 year age groups as existing burden is higher for 40+ age groups.
2) A table on comparison of results from projection exercise with that of previous studies for India, their methodology and limitations can be included. The estimated burden can be presented for all the studies with the author’s calculation.
3) A look at the NFHS-4 data reveals that the prevalence of obesity is higher among the upper wealth quintiles. Therefore, projecting the burden separately by wealth quintiles might be a better idea for both rural and urban areas. The risk factor being adjusted for calculating obesity will vary across these groups. The results might change.
4) There is too much variation in BMI levels across States. The percentage of men and women who are overweight is surprisingly low in the most populated States such as Uttar Pradesh and Bihar. Given the dietary habits and pace of urbanization, it might not be desirable to project overall burden with assumptions that urbanization will grow at a stagnant rate. Moreover, use of low BMI thresholds for Asia can be one of the scenarios for the sensitivity analysis.
Reviewer #2: This is a well-written paper (apart from minor typos) and the methods used seem to be reasonable to answer the research question. I have some minor comments:
- Regarding the paragraph on remission it is not clear to me what you mean by this. Please explain what is exactly meant with rates of remission.
- I do not understand the meaningfulness of the comparison of future prevalence rates in India to that of the US (page 19), as obesity rates in the US are already higher also at the baseline. Please explain why you are making this comparison.
-Increases in oweight/obesity do not only result in higher prevalence rates of associated diseases but also in the need for medical care and facilities, which is in the short run more important, as it seems unrealistic with regard to past trends that the trends in overweight and obesity can be reversed in the short term. This could be better elaborated in the discussion.
6. PLOS authors have the option to publish the peer review history of their article ( what does this mean? ). If published, this will include your full peer review and any attached files.
If you choose “no”, your identity will remain anonymous but your review may still be made public.
Do you want your identity to be public for this peer review? For information about this choice, including consent withdrawal, please see our Privacy Policy .
Reviewer #2: No
[NOTE: If reviewer comments were submitted as an attachment file, they will be attached to this email and accessible via the submission site. Please log into your account, locate the manuscript record, and check for the action link "View Attachments". If this link does not appear, there are no attachment files to be viewed.]
While revising your submission, please upload your figure files to the Preflight Analysis and Conversion Engine (PACE) digital diagnostic tool, https://pacev2.apexcovantage.com/ . PACE helps ensure that figures meet PLOS requirements. To use PACE, you must first register as a user. Registration is free. Then, login and navigate to the UPLOAD tab, where you will find detailed instructions on how to use the tool. If you encounter any issues or have any questions when using PACE, please email us at gro.solp@serugif . Please note that Supporting Information files do not need this step.
Submitted filename: PONE-D-19-17017_review_cw.pdf
Author response to Decision Letter 0
Dear Editors,
We thank the reviewers for the comments given on the manuscript titled “Forecasting the Prevalence of Overweight and Obesity in India to 2040” We have edited the manuscript in Track Changes to address their concerns. Below we provide detailed responses to how we have addressed each of the comments. Additionally, the manuscript has been edited to match the journal’s preferred style.
We believe that the manuscript is now suitable for publication in PLoS One.
Best wishes,
On behalf of all authors
1) The descriptive analyses related with prevalence of obesity for the years considered (From NFHS data and SAGE) for projection exercise can be included before presenting the results. Moreover, results can be presented for 5-year age groups as existing burden is higher for 40+ age groups.
We agree with the reviewer on this point and have reported the prevalence of overweight and obesity from the data sets in S2 Files. We chose to include this here rather than in the manuscript so as to not clutter the paper. In the section describing the input parameters, we have included the following on page 6 so that readers know they can refer to the Supporting Material for this information:
“The prevalence estimates from the data are included in the S2 File”.
We agree with the reviewer. We have included a table in the S2 File that compares the results of the other forecasts of overweight and obesity in India, along with their limitations.
Wealth quintiles reported in survey data is a relative measure, and consequently, the definition of what it means to be in each quintile is likely to vary considerably over time. This, therefore, makes its inclusion in the model inappropriate in the author’s opinion.
The inclusion of a comparative wealth index may be a solution to the issue of comparability over time. However, the association of wealth with overweight and obesity is both complicated, extremely variable by various population subgroups, and has constantly changed over the past two decades. Additionally, the proportion of the population in each quintile of a comparative wealth index is likely to change considerably over time. We refer you to the article below that describes the trends in the association of socioeconomic position with overweight and obesity, and the extent of this variation in these trends sub-nationally[1]. Its inclusion, we believe, would add many extra layers of uncertainty that would detract from the straightforward and relatively elegant model we sought to build.
1. Luhar, S., Mallinson, P.A.C., Clarke, L. and Kinra, S., 2019. Do trends in the prevalence of overweight by socio-economic position differ between India’s most and least economically developed states?. BMC Public Health, 19(1), p.783.
4) There is too much variation in BMI levels across States. The percentage of men and women who are overweight is surprisingly low in the most populated States such as Uttar Pradesh and Bihar. Given the dietary habits and pace of urbanization, it might not be desirable to project overall burden with assumptions that urbanization will grow at a stagnant rate.
We take this opportunity to clarify our use of the term urbanization in the context of this study. In this study, we used urbanization to refer to an individual’s propensity to migrate from a rural area to an urban area, and thus do not assume that urbanization will grow at a stagnant rate.
As urbanization is commonly defined as an increase in the proportion of the total population living in urban areas, assuming that the individual propensity to migrate to urban areas will remain constant over time implies that the growth in the urban population, whilst substantial, will increase at a variable rate. With this assumption, in states with a lower baseline prevalence of overweight/obesity and lower proportion urban, the rate at which the urban population will continue to grow will be faster than in states that have a higher proportion urban at baseline.
Moreover, use of low BMI thresholds for Asia can be one of the scenarios for the sensitivity analysis.
We agree with the reviewer’s comments that including sensitivity using the BMI cut-off values specific to South Asian populations are desirable. This sensitivity analysis is included in the S2 File, and is also referred to in the text on page 11:
“We also performed additional analysis using the South Asian BMI cut-offs values. Some advocate the use of these BMI cut-offs due to a stronger positive association between BMI and body fat observed in South Asians compared to White Caucasians, and consequently an elevated disease risk at lower BMI levels[2,3]. Under this assumption, a BMI between 23.00 kg/m2 and 27.49 kg/m2 was used to define individuals who are overweight, and a BMI greater than 27.50 kg/m2 was used to define obesity[4].”
We have also included the following on page 17, regarding the limitation of using global-cut-offs:
“We sought to remedy this limitation by performing sensitivity analysis using South-Asian BMI cut-offs, and identified potential underestimation of our results (refer to S2 File). For instance, among urban men, we identified a potential underestimation of the 2040 obesity prevalence of nearly 20 percentage points, suggesting that using global cut-offs may underestimate the future overall public health challenge related to excess weight in India.”
1) Regarding the paragraph on remission it is not clear to me what you mean by this. Please explain what is exactly meant with rates of remission.
We agree with the reviewer that this point could benefit from further clarification. In the text we have included the following on page 7:
“Remission refers to reverse transitions, whereby the simulated population is able to transition from a state of ‘Obese’ to ‘Overweight’, and ‘Overweight’ to ‘Not Overweight/Obese.”
2) I do not understand the meaningfulness of the comparison of future prevalence rates in India to that of the US (page 19), as obesity rates in the US are already higher also at the baseline. Please explain why you are making this comparison.
The way in which I have phrased this is misleading due to my use of the word baseline (which refers to the baseline scenario and not the baseline year). I wanted to convey that even by 2040, India’s prevalence of obesity will not reach the prevalence levels that are currently observed in the United States or other industrialized countries, allowing potential for further increases in future prevalence in India. The sentence has been since altered to the following:
“Our study has found that the prevalence of obesity in 2040 is expected to be lower than levels that are currently observed in some of the world’s most industrialized economies, implying potential for considerable further increases beyond 2040.”
3) Increases in overweight/obesity do not only result in higher prevalence rates of associated diseases but also in the need for medical care and facilities, which is in the short run more important, as it seems unrealistic with regard to past trends that the trends in overweight and obesity can be reversed in the short term. This could be better elaborated in the discussion.
We agree with the reviewer and have included the following in the discussion to elaborate on this point:
“The demand for medical services to tackle the increasing burden of overweight/obesity related diseases is also likely to increase substantially into the near future. Potential interventions include preventative measures such as screening for diabetes among high risk overweight/obese individuals to increase the proportion of people with diabetes that are diagnosed[5]. Further efforts may also wish to improve the provision of already established initiatives, particularly the National Programme for Prevention and Control of Cancer, Diabetes, Cardiovascular Disease and Stroke (NPCDCS), that in-part aims to reduce out of pocket expenditure on diabetes healthcare and promote behavioural and lifestyle improvements that reduce the risk of such diseases[6].”
1. Luhar, S., Alice, P., Mallinson, C., Clarke, L. & Kinra, S. Do trends in the prevalence of overweight by socio-economic position differ between India ’ s most and least economically developed states ? 1–12 (2019).
2. Misra, A. Ethnic-Specific Criteria for Classification of Body Mass Index: A Perspective for Asian Indians and American Diabetes Association Position Statement. Diabetes Technol. Ther. 17, 667–71 (2015).
3. Stegenga, H., Haines, A., Jones, K. & Professor, J. W. Identification, assessment, and management of overweight and obesity: Summary of updated NICE guidance. BMJ 349, g6608 (2014).
4. Nishida, C. et al. Appropriate body-mass index for Asian populations and its implications for policy and intervention strategies. Lancet 363, 157 (2004).
5. Basu, S. et al. The Health System and Population Health Implications of Large-Scale Diabetes Screening in India: A Microsimulation Model of Alternative Approaches. PLoS Med. 12, 1–21 (2015).
6. Ministry of Health and Family Welfare, G. of I. National Program for Prevention and Control of Cancer, Diabetes, CVD and Stroke( NPCDCS). Directorate General of Health Services Available at: https://dghs.gov.in/content/1363_3_NationalProgrammePreventionControl.aspx . (Accessed: 14th August 2019)
Submitted filename: PLoS One Response to Reviewers.docx
Decision Letter 1
PONE-D-19-17017R1
Kindly note that one of the reviewer has suggested revisions to incorporate the vast heterogeneity of India by incorporating features such as urbanization and socioeconomic differentials in the forecasting approach. The authors are requested to incorporate these concerns in the revision or in the authors' reply to the comments.
We would appreciate receiving your revised manuscript by Nov 16 2019 11:59PM. When you are ready to submit your revision, log on to https://www.editorialmanager.com/pone/ and select the 'Submissions Needing Revision' folder to locate your manuscript file.
1. If the authors have adequately addressed your comments raised in a previous round of review and you feel that this manuscript is now acceptable for publication, you may indicate that here to bypass the “Comments to the Author” section, enter your conflict of interest statement in the “Confidential to Editor” section, and submit your "Accept" recommendation.
Reviewer #1: (No Response)
Reviewer #2: All comments have been addressed
2. Is the manuscript technically sound, and do the data support the conclusions?
3. Has the statistical analysis been performed appropriately and rigorously?
4. Have the authors made all data underlying the findings in their manuscript fully available?
5. Is the manuscript presented in an intelligible fashion and written in standard English?
6. Review Comments to the Author
Reviewer #1: I still do not agreee with the assumptions that have been made with respect to urbanization and wealth. Also, the process to arrive the burden is not clear.
Reviewer #2: The points raised in my first review have adequately been addressed. The authors have clarified what they mean with remission. The comparison to the US overweight and obesity rates makes sense now. However the last sentence regarding future potential of obesity beyond 2040 is still a bit misleading. I would suggest not to call it "potential" but e.g. "vulnerability" or "susceptibility". The discussion section has also been elaborated.
7. PLOS authors have the option to publish the peer review history of their article ( what does this mean? ). If published, this will include your full peer review and any attached files.
Author response to Decision Letter 1
13 Nov 2019
Reviewer #1: I still do not agree with the assumptions that have been made with respect to urbanization and wealth.
We thank the reviewer for their comment and agree with the reviewer that forecasting the future prevalence of overweight and obesity by separate wealth quintiles would be ideal, however we opted against doing this for the following reasons:
Firstly, there are inherent inadequacies of a wealth index that is commonly used to capture socioeconomic position in cross sectional surveys in low- and middle-income countries. A wealth index is designed as a relative measure of wealth, rather than an absolute measure. Therefore, the index used to calculate quintiles is specific to the setting and the time period in which it is collected1. Consequently, it cannot be compared over time, and thus cannot be used in the forecasting of overweight and obesity over the specified forecast period.
Additionally, the changing nature of the association of overweight and obesity with socioeconomic position makes it very difficult to predict in the future which would be set externally to the model. Whilst it is true that survey data shows a higher prevalence of overweight and obesity in higher wealth quintiles, we are unable to firstly determine the proportion of the population that is expected to be in pre-specified wealth quintiles over the forecasting period (2010-2040), and secondly, it is not possible to ascertain what the exact association between overweight/obesity and socioeconomic position will be in the future. A number of systematic reviews2–5, in addition to recent studies in India6–8, have documented and provided evidence that in low and middle income countries, like India, the socioeconomic patterning of overweight and obesity changes considerably over time. The higher prevalence initially observed among high socioeconomic status groups will turn negative along with continued economic development. Due to the likely uncertain nature and considerable subnational heterogeneity in the future socioeconomic patterning of overweight and obesity throughout the forecasting period, we strongly believe that its inclusion in the model would be inappropriate and what results would come out of the model would be uninterpretable due to escalating uncertainty in the data and projections of SES distribution.
We have also included the following on page 18 under the limitations section:
“Finally, it would have been desirable to have accommodated the changing socioeconomic patterning of overweight and obesity in India in our forecasts. Studies have shown that rural and lower SEP Indians are at increasing risk of overweight and obesity, with significant variation in this patterning sub-nationally[43],[44]. However, due to the uncertainty in how these socioeconomic patterning trends will evolve throughout the forecasting period, the inclusion of socioeconomic status in our model has the potential to, at best, make the predictions marginally more accurate, and at worst, considerably more uncertain, and consequently, we opted for a relatively parsimonious model.”
1. Rutstein SO, Johnson K. DHS Comparative reports No.6. The DHS Wealth Index. Calverton, Maryland, USA; 2004.
2. Dinsa GD, Goryakin Y, Fumagalli E, Suhrcke M. Obesity and socioeconomic status in developing countries: A systematic review. Obes Rev. 2012;13(11):1067–79.
3. McLaren L. Socioeconomic status and obesity. Epidemiol Rev. 2007;29(1):29–48.
4. McLaren L. Socioeconomic status and obesity. Epidemiol Rev. 2007;29(1):29–48.
5. Monteiro CA, Moura EC, Conde WL, Popkin BM. Public Health Reviews Socioeconomic status and obesity in adult populations of developing countries : a review. Public Health Rev. 2004;82(12):940–6.
6. Luhar S, Mallinson PAC, Clarke L, Kinra S. Trends in the socioeconomic patterning of overweight/obesity in India: A repeated cross-sectional study using nationally representative data. BMJ Open. 2018;8(10):e023935.
7. Luhar S, Alice P, Mallinson C, Clarke L, Kinra S. Do trends in the prevalence of overweight by socio-economic position differ between India ’ s most and least economically developed states ? 2019;1–12.
8. Sengupta A, Angeli F, Syamala TS, Dagnelie PC, Schayck CP van. Overweight and obesity prevalence among Indian women by place of residence and socio-economic status: Contrasting patterns from “underweight states” and “overweight states” of India. Soc Sci Med. 2015;138:161–9.
In regards urbanisation, this study does not make any assumption that urbanisation (the proportion of the population living in urban areas) will continue at a stagnant rate. In the paper, we use the term ‘urbanisation’ to refer to any one individual’s propensity to migrate to an urban area, not the population level of urbanisation, which is commonly defined as an increase in the proportion of the population residing in urban areas.
The highly populated and low overweight/obesity states such as Uttar Pradesh and Bihar also have relatively lower proportion of the population in urban areas. In these states, with comparatively lower levels of overweight and obesity, the increase in the proportion of the population residing in urban areas will be relatively fast compared in these low overweight/obesity prevalence areas compared to high overweight/obesity prevalence areas, addressing the reviewer’s initial concerns.
Reviewer #1: Also, the process to arrive the burden is not clear.
We agree with the reviewer that the calculation of the burden was not clear. We have clarified this in the S2 File in a table footnote under the ‘Table of Comparison’ tab. Specifically, we included the following:
“For consistency across the different studies, we calculated the burden using the United Nations World Population Prospects (2017) population projections, and United Nations World Urbanization Prospects (2018), and applying the proportions identified in the studies”
Reviewer #2: The points raised in my first review have adequately been addressed. The authors have clarified what they mean with remission. The comparison to the US overweight and obesity rates makes sense now. However, the last sentence regarding future potential of obesity beyond 2040 is still a bit misleading. I would suggest not to call it "potential" but e.g. "vulnerability" or "susceptibility". The discussion section has also been elaborated.
We thank the reviewer for clarifying that we have adequayely addressed what was meant by remission. We agree with the reviewer’s additional concerns and have amended the sentence in the manuscript.
The original sentence:
“Our study has found that the prevalence of obesity in 2040 is expected to be lower than levels that are currently observed in some of the world’s most industrialized economies, implying the potential for considerable further increases beyond 2040.”
Has been changed to:
“We have found that the prevalence of obesity in 2040 is expected to be lower than levels that are currently observed in some of the world’s most industrialized economies, implying that India could be susceptible to considerable further increases in obesity prevalence beyond 2040.”
Submitted filename: Response_to_Reviewers_Shammi .docx
Decision Letter 2
15 Jan 2020
PONE-D-19-17017R2
We would appreciate receiving your revised manuscript by Feb 29 2020 11:59PM. When you are ready to submit your revision, log on to https://www.editorialmanager.com/pone/ and select the 'Submissions Needing Revision' folder to locate your manuscript file.
Reviewer #1: All comments have been addressed
Reviewer #1: It would have been desirable to include a number of socio-economic determinants such as marital status, job status, income status, smoking, alcohol consumption, sleep duration, psychological factors, dietary intake, and fertility rate which in literature are identified as key determinants of overweight/obesity. But it is also true that it can be very challenging to forecast using these parameters which keep changing. However, the study can be a good starting point for those who want to do further research in this area. At the outset, margin of error should be expected since the values are being projected for such a long period of time. Also, the paper uses the WPP 2017 revision available on the population projection from the UN population projection. Since, the new version (2019) of the WPP and World Urbanization Prospects data is available, I leave it up to the authors to decide whether they want to update the analyses since it can be time consuming and the broad inference might not change.
Author response to Decision Letter 2
27 Jan 2020
Response: We thank the reviewer for the feedback on our study and agree that the study can be a good starting point for those who want to do further research in this area. We also thank the reviewer for the opportunity to update our analyses with newly published data. We have since re-run the models with the new data and the changes have been included in the manuscript, graphs, tables and the supplementary information.
Decision Letter 3
PONE-D-19-17017R3
Dear Dr. Luhar,
We are pleased to inform you that your manuscript has been judged scientifically suitable for publication and will be formally accepted for publication once it complies with all outstanding technical requirements.
Within one week, you will receive an e-mail containing information on the amendments required prior to publication. When all required modifications have been addressed, you will receive a formal acceptance letter and your manuscript will proceed to our production department and be scheduled for publication.
Shortly after the formal acceptance letter is sent, an invoice for payment will follow. To ensure an efficient production and billing process, please log into Editorial Manager at https://www.editorialmanager.com/pone/ , click the "Update My Information" link at the top of the page, and update your user information. If you have any billing related questions, please contact our Author Billing department directly at gro.solp@gnillibrohtua .
If your institution or institutions have a press office, please notify them about your upcoming paper to enable them to help maximize its impact. If they will be preparing press materials for this manuscript, you must inform our press team as soon as possible and no later than 48 hours after receiving the formal acceptance. Your manuscript will remain under strict press embargo until 2 pm Eastern Time on the date of publication. For more information, please contact gro.solp@sserpeno .
With kind regards,
Additional Editor Comments (optional):
Acceptance letter
11 Feb 2020
Dear Dr. Luhar:
I am pleased to inform you that your manuscript has been deemed suitable for publication in PLOS ONE. Congratulations! Your manuscript is now with our production department.
If your institution or institutions have a press office, please notify them about your upcoming paper at this point, to enable them to help maximize its impact. If they will be preparing press materials for this manuscript, please inform our press team within the next 48 hours. Your manuscript will remain under strict press embargo until 2 pm Eastern Time on the date of publication. For more information please contact gro.solp@sserpeno .
For any other questions or concerns, please email gro.solp@enosolp .
Thank you for submitting your work to PLOS ONE.
PLOS ONE Editorial Office Staff
on behalf of
Dr. William Joe
- Alzheimer's disease & dementia
- Arthritis & Rheumatism
- Attention deficit disorders
- Autism spectrum disorders
- Biomedical technology
- Diseases, Conditions, Syndromes
- Endocrinology & Metabolism
- Gastroenterology
- Gerontology & Geriatrics
- Health informatics
- Inflammatory disorders
- Medical economics
- Medical research
- Medications
- Neuroscience
- Obstetrics & gynaecology
- Oncology & Cancer
- Ophthalmology
- Overweight & Obesity
- Parkinson's & Movement disorders
- Psychology & Psychiatry
- Radiology & Imaging
- Sleep disorders
- Sports medicine & Kinesiology
- Vaccination
- Breast cancer
- Cardiovascular disease
- Chronic obstructive pulmonary disease
- Colon cancer
- Coronary artery disease
- Heart attack
- Heart disease
- High blood pressure
- Kidney disease
- Lung cancer
- Multiple sclerosis
- Myocardial infarction
- Ovarian cancer
- Post traumatic stress disorder
- Rheumatoid arthritis
- Schizophrenia
- Skin cancer
- Type 2 diabetes
- Full List »
share this!
June 1, 2024
This article has been reviewed according to Science X's editorial process and policies . Editors have highlighted the following attributes while ensuring the content's credibility:
fact-checked
trusted source
Study shows risk for heart attack and stroke increases in people with obesity for a decade or more
by The Endocrine Society
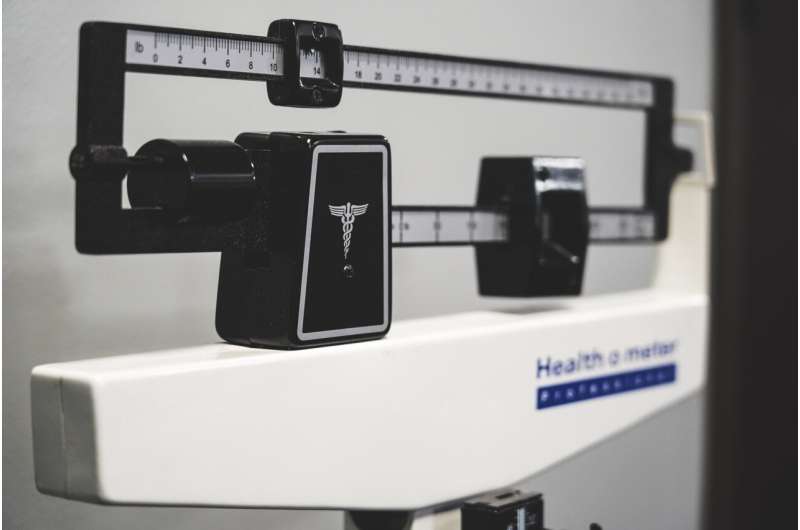
People under age 50 have a greater risk for heart attack or stroke if they've lived with obesity for 10 years, according to industry-sponsored research being presented Saturday at ENDO 2024 , the Endocrine Society's annual meeting in Boston, Mass.
"It is well established that people who have excess weight at any point in time have a greater risk of heart attacks and strokes. What was not known was whether it matters for how long someone has been exposed to excess weight ," said Alexander Turchin, M.D., M.S., Director of Quality at the Division of Endocrinology at Brigham & Women's Hospital and associate professor of medicine at Harvard Medical School in Boston.
Turchin and his team, including researchers from Eli Lilly, conducted a comprehensive study using data from the Nurses' Health Study (NHS) and Health Professionals Follow-Up Study (HPFS). They specifically focused on patients who had a body-mass index (BMI) greater than 25 kg/m 2 at least once over a 10-year period (1990–1999) to understand how their weight impacted their risk for heart attack or stroke over the next two decades (2000–2020).
The researchers analyzed data from 109,259 women and 27,239 men who had an average age of 48.6 years and a BMI of 27.2 kg/m 2 in 1990. Of those, 6,862 had atherosclerotic cardiovascular disease , 3,587 had type 2 diabetes, and 65,101 had a history of smoking. At follow-up in 2020, the data revealed 12,048 cardiovascular events.
"We found that among women younger than 50 and men younger than 65, having obesity over a 10-year period was associated with a 25–60% increase in the risk of heart attack and stroke—and was more important than their weight at a single point in time in 1990," Turchin said.
However, obesity in women older than 50 and men older than 65 was not associated with an increased risk for heart attack and stroke .
These findings are important for clinicians who see younger people living with obesity, as they show that the sooner someone is treated, the better.
"Viewed as a 'glass half full,' these findings mean that obesity at any given point in time does not 'seal' one's fate," Turchin said. "If obesity is treated in a timely fashion, its complications can be prevented."
Explore further
Feedback to editors

Cancer patients often do better with less intensive treatment, new research finds
16 hours ago

Study sheds new light on the contribution of dopamine to reinforcement learning
23 hours ago

Almost 1 in 3 Americans know someone who's died from a drug overdose
Jun 1, 2024

Antibody discovery promises new hope in influenza B battle, paves way for universal vaccine

Eye-tracking techniques could help primary care providers diagnose autism sooner, more accurately
May 31, 2024

This self-powered sensor could make MRIs more efficient

Not eating can hinder weight loss, study in fruit flies suggests

Neuroscience research suggests ketones can enhance cognitive function and protect brain networks

New research finds antidepressants may help deliver other drugs into the brain

Mediterranean diet tied to one-fifth lower risk of death in women
Related stories.

Study finds one in five patients at high risk of cardiovascular disease refuse statin therapy
Feb 28, 2023

Patient-provider discussions about bariatric surgery play pivotal role in weight loss outcomes
Jun 10, 2021

Semaglutide reduces cardiovascular events by 20% in overweight or obese adults who don't have diabetes: Trial
Nov 13, 2023

Wegovy lowered users' heart risks in new trial
Aug 8, 2023

Monthly lifestyle counseling tied to better outcomes for patients with diabetes
Aug 1, 2019

Weight-reduction surgery for severely obese adults may prevent second heart attack, death
Oct 26, 2020
Recommended for you

Cardiomyocytes study discovers new way to regenerate damaged heart cells

Cause of common type of heart failure may be different for women and men
May 30, 2024

Mummies study finds heart disease plagued the ancients, too

Study finds older adults hospitalized for heart failure had high risk of kidney complications
May 29, 2024

Study shows orange peel extract may improve heart health
May 28, 2024
Let us know if there is a problem with our content
Use this form if you have come across a typo, inaccuracy or would like to send an edit request for the content on this page. For general inquiries, please use our contact form . For general feedback, use the public comments section below (please adhere to guidelines ).
Please select the most appropriate category to facilitate processing of your request
Thank you for taking time to provide your feedback to the editors.
Your feedback is important to us. However, we do not guarantee individual replies due to the high volume of messages.
E-mail the story
Your email address is used only to let the recipient know who sent the email. Neither your address nor the recipient's address will be used for any other purpose. The information you enter will appear in your e-mail message and is not retained by Medical Xpress in any form.
Newsletter sign up
Get weekly and/or daily updates delivered to your inbox. You can unsubscribe at any time and we'll never share your details to third parties.
More information Privacy policy
Donate and enjoy an ad-free experience
We keep our content available to everyone. Consider supporting Science X's mission by getting a premium account.
E-mail newsletter
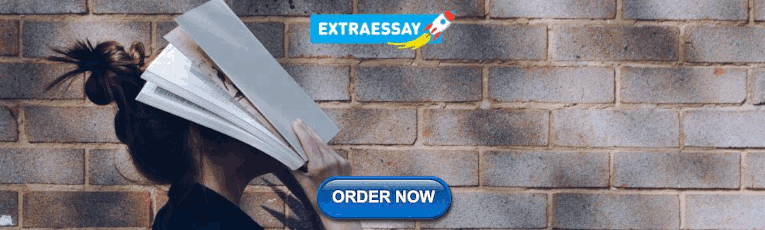
IMAGES
VIDEO
COMMENTS
The psychological factors associated with overweight and obesity 1 UNDERSTANDING THE PSYCHOLOGICAL FACTORS ASSOCIATED WITH OVERWEIGHT AND OBESITY ESME BANTING A thesis submitted in partial fulfilment of the requirements of the degree of Doctor of Clinical Psychology, validated by the University of Oxford (Trinity Term) July 2017 Word Count: 20,324
The estimated obesity-related health care costs are as high as $147. billion per year. Despite the alarming health risks and increasing health care costs, the. rates of screening and counseling for obesity in the primary care setting are merely 30%. This project outlines weight-management counseling strategies.
Overweightness and obesity are also well-documented as increasing the risk of diabetes, particularly type 2 diabetes mellitus [103]. de Oliveira et al. [104] confirmed that diabetes occurs with high incidence in obese patients. aTbares2017health [105] indicated that diabetes followed obesity in 2015 as the second leading cause of BMI related ...
The relationship between obesity and health-related quality of life (HRQoL) may be confounded by factors such as multimorbidity. The aim of the study was to explore this relationship, controlling for long-term conditions and other health, lifestyle and demographic factors in a general adult population. There was specific interest in the impact of high weight status, measured by body mass index ...
Dissertation Advisor: Dr. Edward Giovannucci Donghoon Lee Predicted Lean Body Mass and Fat Mass: Novel Insights into Obesity, Chronic Disease, and Mortality Research ... independent dataset and using obesity-related biomarkers, suggesting their potential application in epidemiological studies. Using the developed anthropometric equations ...
carbohydrate is a crucial factor in the obesity epidemic. 18 Soft drinks, alcoholic beverages and fast food tend to be calorie rich. In Britain, there has been a signi cant rise in the amount of ...
Abstract. Obesity is a multi-factorial disease and its prevention and management require knowledge of the complex interactions underlying it and adopting a whole system approach that addresses obesogenic environments within country specific contexts. The pathophysiology behind obesity involves a myriad of genetic, epigenetic, physiological, and ...
Obesity negatively impacts health-related quality of life, with greater degrees of obesity associated with greater impairments.[100,101,102] While most individuals often report significant difficulties with physical and occupational functioning, many also experience problems with social functioning, such as social withdrawal and social isolation.
The obesity epidemic is one of the most significant public health problems facing the United States (US). Reducing current weight in adults and preventing further weight gain among those already overweight and obese provides a critical opportunity to curb the rising burden of obesity-related morbidity and mortality. As a result, many jurisdictions
Obesity and overweight have become national health concerns and have ... This dissertation is dedicated to my dad who was a life-long educator, servant of ... which supported Hong Liu to present her research work related to this study at professional conferences.
Obesity prevalence among children is >30% in the Cook Islands, Nauru and Palau, with a notable increase over the past few decades. Worldwide prevalence of obesity increased at an alarming rate in ...
Environmental Factors on Obesity Comparative Analysis of Mexico and the United States Andrea Robina Galatas Supervisor: Prof. Dr. Adnan Kisa Master Thesis as part of the European Master's in Health ... (Del Parigi, A., 2010). The association between visceral fat and other obesity-related diseases was shown to be independent of the amount of ...
reality, there is also an economic strain. The aggregate cost of obesity to society is $150 billion every year and this figure is predicted to double in a decade (Orszag, 2010). As this daunting truth looms over our heads, we are able to take comfort in recognizing that obesity and other weight-related conditions are largely preventable.
Introduction. Obesity is associated with an increased risk of diseases such as cancer, type 2 diabetes, and heart disease, leading to early mortality.1 2 3 More recently, obesity is a risk factor for worse outcomes with covid-19.4 5 Because of this increased risk, health agencies and governments worldwide are focused on finding effective ways to help people lose weight.6
Review Summary. As far as possible a comprehensive and systematic ap- proach has been taken in this review of the methodology employed in qualitative studies of adults' experiences of obesity. However, the diverse range and variable quality of studies has necessitated a more exploratory and open review method.
Obesity in Men Because health complications can be reduced if weight is properly managed, researchers have attempted to identify specific populations that are at risk for developing obesity. Although obesity is a problem for both sexes, statistics show that men are more likely to be overweight or obese (Flegal et al., 2010).
This Dissertation is brought to you for free and open access by the Walden Dissertations and Doctoral Studies ... Obesity-related chronic diseases and opportunity cost significantly impact individuals and communities. Current prevention policies focus on diet and exercise without demonstrating the benefits of stress coping
The management of obesity before a woman conceives is one of the most effective efforts a woman can take in decreasing her risk of obesity-related complications during pregnancy. Evidence supports the idea that maternal obesity influences maternal and fetal outcomes, leading to maternal and fetal morbidities. Physicians acknowledge the
The undersigned certify that they have read and hereby recommend for acceptance by the University of Dar es Salaam a dissertation entitled: Prevalence and Implications of Overweight and Obesity in Children's health and Learning Behaviour, in fulfillment of the requirements for the degree of Masters of Arts (Education) of the
To facilitate a more comprehensive evaluation of obesity-related complex multimorbidity, we analysed individual-level data from two Finnish cohort studies and the UK Biobank, examined obesity as a risk factor for incident cases of 78 predefined diseases, determined associations between obesity-related diseases, and characterised resulting ...
Key Points. Question Could the excess risk of mortality related to obesity be attenuated through the improvement of social isolation and loneliness?. Findings In this cohort study of 398 972 UK Biobank participants, as the index of social isolation and loneliness went from highest to lowest, the risk of all-cause mortality decreased by 36% and 9%, respectively, in people with obesity compared ...
1) The descriptive analyses related with prevalence of obesity for the years considered (From NFHS data and SAGE) for projection exercise can be included before presenting the results. Moreover, results can be presented for 5 year age groups as existing burden is higher for 40+ age groups.
Obesity in children is defined as a BMI-z score above the 95th percentile (Ogden C. L., Carroll, Curtin, Lamb, & Flegal, 2010). The increasing prevalence of childhood obesity worldwide is considered a major global public health problem. While many factors contribute to the development of obesity, an imbalance between
A study published in JAMA found that among 5053 people who underwent bariatric surgery, 2.9% developed an obesity-related cancer over 10 years compared with 4.9% in the nonsurgery group.
Gains in knowledge about the causes of obesity and how to treat it often aren't getting to the people who need them, according to a new science report. ... the need for future research and policy changes to improve current patient care models and ensure equitable access to obesity-related care for people in underrepresented groups."
There were a total of 4,726 subjects, all of whom either had obesity (a body mass index, or BMI, of greater than 30), or overweight (a BMI of at least 27) along with an obesity-related medical ...
For women under 50 and men under 65 with obesity for 10 years or more, the researchers reported that: There was a 25% to 60% increase in the risk of heart attack and stroke. For women older than ...
At follow-up in 2020, the data revealed 12,048 cardiovascular events. "We found that among women younger than 50 and men younger than 65, having obesity over a 10-year period was associated with a ...
Study finds BMI, despite flaws, is useful estimate of body fat in children. T he body mass index has long been slammed as a blunt instrument for evaluating health, even more so with new obesity ...
Anti-obesity medication Zepbound (tirzepatide) delivers significant weight loss even in people with multiple obesity-related medical conditions, a new study found. The research challenges the ...