Psychological Experimental Design
- Living reference work entry
- First Online: 15 February 2024
- Cite this living reference work entry
- Zhang Houcan 2 &
- He Dongjun 3
11 Accesses
Psychological experimental design refers to the experimental design and methodological approaches devised by researchers before conducting an experiment based on the research objectives. It can be broadly or narrowly defined. Broadly, psychological experimental design refers to the general procedure of scientific research, including problem formulation, hypothesis development, selection of variables, manipulation, and control, as well as statistical analysis of results and paper writing, among other series of activities. Narrowly, psychological experimental design refers to the specific experimental plan or model that researchers develop for arranging variables and procedures, along with the related statistical analysis. The main components of psychological experimental design include how to reasonably arrange the experimental procedures and how to perform statistical analysis on the experimental data. The main steps can be summarized as follows: (1) formulate hypotheses based on...
This is a preview of subscription content, log in via an institution to check access.
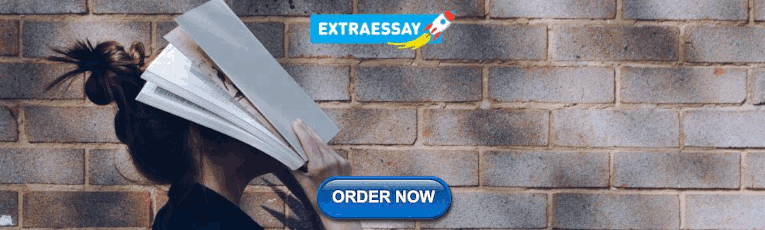
Access this chapter
Institutional subscriptions
Further Reading
Kantowitz BH, Roediger HL, Elmes DG (2015) Experimental psychology, 10th edn. Cengage Learning, Boston
Google Scholar
Zhang X-M, Hua S (2014) Experimental psychology. Beijing Normal University Publishing Group, Beijing
Download references
Author information
Authors and affiliations.
Faculty of Psychology, Beijing Normal University, Beijing, China
Zhang Houcan
School of Psychology, Chengdu Medical University, Chengdu, China
You can also search for this author in PubMed Google Scholar
Corresponding author
Correspondence to He Dongjun .
Rights and permissions
Reprints and permissions
Copyright information
© 2024 Encyclopedia of China Publishing House
About this entry
Cite this entry.
Houcan, Z., Dongjun, H. (2024). Psychological Experimental Design. In: The ECPH Encyclopedia of Psychology. Springer, Singapore. https://doi.org/10.1007/978-981-99-6000-2_490-1
Download citation
DOI : https://doi.org/10.1007/978-981-99-6000-2_490-1
Received : 04 January 2024
Accepted : 05 January 2024
Published : 15 February 2024
Publisher Name : Springer, Singapore
Print ISBN : 978-981-99-6000-2
Online ISBN : 978-981-99-6000-2
eBook Packages : Springer Reference Behavioral Science and Psychology Reference Module Humanities and Social Sciences Reference Module Business, Economics and Social Sciences
- Publish with us
Policies and ethics
- Find a journal
- Track your research
- Bipolar Disorder
- Therapy Center
- When To See a Therapist
- Types of Therapy
- Best Online Therapy
- Best Couples Therapy
- Best Family Therapy
- Managing Stress
- Sleep and Dreaming
- Understanding Emotions
- Self-Improvement
- Healthy Relationships
- Student Resources
- Personality Types
- Guided Meditations
- Verywell Mind Insights
- 2024 Verywell Mind 25
- Mental Health in the Classroom
- Editorial Process
- Meet Our Review Board
- Crisis Support
How Does Experimental Psychology Study Behavior?
Purpose, methods, and history
Kendra Cherry, MS, is a psychosocial rehabilitation specialist, psychology educator, and author of the "Everything Psychology Book."
:max_bytes(150000):strip_icc():format(webp)/IMG_9791-89504ab694d54b66bbd72cb84ffb860e.jpg)
Sean is a fact-checker and researcher with experience in sociology, field research, and data analytics.
:max_bytes(150000):strip_icc():format(webp)/Sean-Blackburn-1000-a8b2229366944421bc4b2f2ba26a1003.jpg)
- Why It Matters
What factors influence people's behaviors and thoughts? Experimental psychology utilizes scientific methods to answer these questions by researching the mind and behavior. Experimental psychologists conduct experiments to learn more about why people do certain things.
Overview of Experimental Psychology
Why do people do the things they do? What factors influence how personality develops? And how do our behaviors and experiences shape our character?
These are just a few of the questions that psychologists explore, and experimental methods allow researchers to create and empirically test hypotheses. By studying such questions, researchers can also develop theories that enable them to describe, explain, predict, and even change human behaviors.
For example, researchers might utilize experimental methods to investigate why people engage in unhealthy behaviors. By learning more about the underlying reasons why these behaviors occur, researchers can then search for effective ways to help people avoid such actions or replace unhealthy choices with more beneficial ones.
Why Experimental Psychology Matters
While students are often required to take experimental psychology courses during undergraduate and graduate school , think about this subject as a methodology rather than a singular area within psychology. People in many subfields of psychology use these techniques to conduct research on everything from childhood development to social issues.
Experimental psychology is important because the findings play a vital role in our understanding of the human mind and behavior.
By better understanding exactly what makes people tick, psychologists and other mental health professionals can explore new approaches to treating psychological distress and mental illness. These are often topics of experimental psychology research.
Experimental Psychology Methods
So how exactly do researchers investigate the human mind and behavior? Because the mind is so complex, it seems like a challenging task to explore the many factors that contribute to how we think, act, and feel.
Experimental psychologists use a variety of different research methods and tools to investigate human behavior. Methods in the experimental psychology category include experiments, case studies, correlational research, and naturalistic observations.
Experiments
Experimentation remains the primary standard in psychological research. In some cases, psychologists can perform experiments to determine if there is a cause-and-effect relationship between different variables.
The basics of conducting a psychology experiment involve:
- Randomly assigning participants to groups
- Operationally defining variables
- Developing a hypothesis
- Manipulating independent variables
- Measuring dependent variables
One experimental psychology research example would be to perform a study to look at whether sleep deprivation impairs performance on a driving test. The experimenter could control other variables that might influence the outcome, varying the amount of sleep participants get the night before.
All of the participants would then take the same driving test via a simulator or on a controlled course. By analyzing the results, researchers can determine if changes in the independent variable (amount of sleep) led to differences in the dependent variable (performance on a driving test).
Case Studies
Case studies allow researchers to study an individual or group of people in great depth. When performing a case study, the researcher collects every single piece of data possible, often observing the person or group over a period of time and in a variety of situations. They also collect detailed information about their subject's background—including family history, education, work, and social life—is also collected.
Such studies are often performed in instances where experimentation is not possible. For example, a scientist might conduct a case study when the person of interest has had a unique or rare experience that could not be replicated in a lab.
Correlational Research
Correlational studies are an experimental psychology method that makes it possible for researchers to look at relationships between different variables. For example, a psychologist might note that as one variable increases, another tends to decrease.
While such studies can look at relationships, they cannot be used to imply causal relationships. The golden rule is that correlation does not equal causation.
Naturalistic Observations
Naturalistic observation gives researchers the opportunity to watch people in their natural environments. This experimental psychology method can be particularly useful in cases where the investigators believe that a lab setting might have an undue influence on participant behaviors.
What Experimental Psychologists Do
Experimental psychologists work in a wide variety of settings, including colleges, universities, research centers, government, and private businesses. Some of these professionals teach experimental methods to students while others conduct research on cognitive processes, animal behavior, neuroscience, personality, and other subject areas.
Those who work in academic settings often teach psychology courses in addition to performing research and publishing their findings in professional journals. Other experimental psychologists work with businesses to discover ways to make employees more productive or to create a safer workplace—a specialty area known as human factors psychology .
Experimental Psychology Research Examples
Some topics that might be explored in experimental psychology research include how music affects motivation, the impact social media has on mental health , and whether a certain color changes one's thoughts or perceptions.
History of Experimental Psychology
To understand how experimental psychology got where it is today, it can be helpful to look at how it originated. Psychology is a relatively young discipline, emerging in the late 1800s. While it started as part of philosophy and biology, it officially became its own field of study when early psychologist Wilhelm Wundt founded the first laboratory devoted to the study of experimental psychology.
Some of the important events that helped shape the field of experimental psychology include:
- 1874 - Wilhelm Wundt published the first experimental psychology textbook, "Grundzüge der physiologischen Psychologie" ("Principles of Physiological Psychology").
- 1875 - William James opened a psychology lab in the United States. The lab was created for the purpose of class demonstrations rather than to perform original experimental research.
- 1879 - The first experimental psychology lab was founded in Leipzig, Germany. Modern experimental psychology dates back to the establishment of the very first psychology lab by pioneering psychologist Wilhelm Wundt during the late nineteenth century.
- 1883 - G. Stanley Hall opened the first experimental psychology lab in the United States at John Hopkins University.
- 1885 - Herman Ebbinghaus published his famous "Über das Gedächtnis" ("On Memory"), which was later translated to English as "Memory: A Contribution to Experimental Psychology." In the work, Ebbinghaus described learning and memory experiments that he conducted on himself.
- 1887 - George Truball Ladd published his textbook "Elements of Physiological Psychology," the first American book to include a significant amount of information on experimental psychology.
- 1887 - James McKeen Cattell established the world's third experimental psychology lab at the University of Pennsylvania.
- 1890 - William James published his classic textbook, "The Principles of Psychology."
- 1891 - Mary Whiton Calkins established an experimental psychology lab at Wellesley College, becoming the first woman to form a psychology lab.
- 1893 - G. Stanley Hall founded the American Psychological Association , the largest professional and scientific organization of psychologists in the United States.
- 1920 - John B. Watson and Rosalie Rayner conducted their now-famous Little Albert Experiment , in which they demonstrated that emotional reactions could be classically conditioned in people.
- 1929 - Edwin Boring's book "A History of Experimental Psychology" was published. Boring was an influential experimental psychologist who was devoted to the use of experimental methods in psychology research.
- 1955 - Lee Cronbach published "Construct Validity in Psychological Tests," which popularized the use of construct validity in psychological studies.
- 1958 - Harry Harlow published "The Nature of Love," which described his experiments with rhesus monkeys on attachment and love.
- 1961 - Albert Bandura conducted his famous Bobo doll experiment, which demonstrated the effects of observation on aggressive behavior.
Experimental Psychology Uses
While experimental psychology is sometimes thought of as a separate branch or subfield of psychology, experimental methods are widely used throughout all areas of psychology.
- Developmental psychologists use experimental methods to study how people grow through childhood and over the course of a lifetime.
- Social psychologists use experimental techniques to study how people are influenced by groups.
- Health psychologists rely on experimentation and research to better understand the factors that contribute to wellness and disease.
A Word From Verywell
The experimental method in psychology helps us learn more about how people think and why they behave the way they do. Experimental psychologists can research a variety of topics using many different experimental methods. Each one contributes to what we know about the mind and human behavior.
Shaughnessy JJ, Zechmeister EB, Zechmeister JS. Research Methods in Psychology . McGraw-Hill.
Heale R, Twycross A. What is a case study? . Evid Based Nurs. 2018;21(1):7-8. doi:10.1136/eb-2017-102845
Chiang IA, Jhangiani RS, Price PC. Correlational research . In: Research Methods in Psychology, 2nd Canadian edition. BCcampus Open Education.
Pierce T. Naturalistic observation . Radford University.
Kantowitz BH, Roediger HL, Elmes DG. Experimental Psychology . Cengage Learning.
Weiner IB, Healy AF, Proctor RW. Handbook of Psychology: Volume 4, Experimental Psychology . John Wiley & Sons.
By Kendra Cherry, MSEd Kendra Cherry, MS, is a psychosocial rehabilitation specialist, psychology educator, and author of the "Everything Psychology Book."
5.2 Experimental Design
Learning objectives.
- Explain the difference between between-subjects and within-subjects experiments, list some of the pros and cons of each approach, and decide which approach to use to answer a particular research question.
- Define random assignment, distinguish it from random sampling, explain its purpose in experimental research, and use some simple strategies to implement it
- Define several types of carryover effect, give examples of each, and explain how counterbalancing helps to deal with them.
In this section, we look at some different ways to design an experiment. The primary distinction we will make is between approaches in which each participant experiences one level of the independent variable and approaches in which each participant experiences all levels of the independent variable. The former are called between-subjects experiments and the latter are called within-subjects experiments.
Between-Subjects Experiments
In a between-subjects experiment , each participant is tested in only one condition. For example, a researcher with a sample of 100 university students might assign half of them to write about a traumatic event and the other half write about a neutral event. Or a researcher with a sample of 60 people with severe agoraphobia (fear of open spaces) might assign 20 of them to receive each of three different treatments for that disorder. It is essential in a between-subjects experiment that the researcher assigns participants to conditions so that the different groups are, on average, highly similar to each other. Those in a trauma condition and a neutral condition, for example, should include a similar proportion of men and women, and they should have similar average intelligence quotients (IQs), similar average levels of motivation, similar average numbers of health problems, and so on. This matching is a matter of controlling these extraneous participant variables across conditions so that they do not become confounding variables.
Random Assignment
The primary way that researchers accomplish this kind of control of extraneous variables across conditions is called random assignment , which means using a random process to decide which participants are tested in which conditions. Do not confuse random assignment with random sampling. Random sampling is a method for selecting a sample from a population, and it is rarely used in psychological research. Random assignment is a method for assigning participants in a sample to the different conditions, and it is an important element of all experimental research in psychology and other fields too.
In its strictest sense, random assignment should meet two criteria. One is that each participant has an equal chance of being assigned to each condition (e.g., a 50% chance of being assigned to each of two conditions). The second is that each participant is assigned to a condition independently of other participants. Thus one way to assign participants to two conditions would be to flip a coin for each one. If the coin lands heads, the participant is assigned to Condition A, and if it lands tails, the participant is assigned to Condition B. For three conditions, one could use a computer to generate a random integer from 1 to 3 for each participant. If the integer is 1, the participant is assigned to Condition A; if it is 2, the participant is assigned to Condition B; and if it is 3, the participant is assigned to Condition C. In practice, a full sequence of conditions—one for each participant expected to be in the experiment—is usually created ahead of time, and each new participant is assigned to the next condition in the sequence as he or she is tested. When the procedure is computerized, the computer program often handles the random assignment.
One problem with coin flipping and other strict procedures for random assignment is that they are likely to result in unequal sample sizes in the different conditions. Unequal sample sizes are generally not a serious problem, and you should never throw away data you have already collected to achieve equal sample sizes. However, for a fixed number of participants, it is statistically most efficient to divide them into equal-sized groups. It is standard practice, therefore, to use a kind of modified random assignment that keeps the number of participants in each group as similar as possible. One approach is block randomization . In block randomization, all the conditions occur once in the sequence before any of them is repeated. Then they all occur again before any of them is repeated again. Within each of these “blocks,” the conditions occur in a random order. Again, the sequence of conditions is usually generated before any participants are tested, and each new participant is assigned to the next condition in the sequence. Table 5.2 shows such a sequence for assigning nine participants to three conditions. The Research Randomizer website ( http://www.randomizer.org ) will generate block randomization sequences for any number of participants and conditions. Again, when the procedure is computerized, the computer program often handles the block randomization.
Random assignment is not guaranteed to control all extraneous variables across conditions. The process is random, so it is always possible that just by chance, the participants in one condition might turn out to be substantially older, less tired, more motivated, or less depressed on average than the participants in another condition. However, there are some reasons that this possibility is not a major concern. One is that random assignment works better than one might expect, especially for large samples. Another is that the inferential statistics that researchers use to decide whether a difference between groups reflects a difference in the population takes the “fallibility” of random assignment into account. Yet another reason is that even if random assignment does result in a confounding variable and therefore produces misleading results, this confound is likely to be detected when the experiment is replicated. The upshot is that random assignment to conditions—although not infallible in terms of controlling extraneous variables—is always considered a strength of a research design.
Matched Groups
An alternative to simple random assignment of participants to conditions is the use of a matched-groups design . Using this design, participants in the various conditions are matched on the dependent variable or on some extraneous variable(s) prior the manipulation of the independent variable. This guarantees that these variables will not be confounded across the experimental conditions. For instance, if we want to determine whether expressive writing affects people’s health then we could start by measuring various health-related variables in our prospective research participants. We could then use that information to rank-order participants according to how healthy or unhealthy they are. Next, the two healthiest participants would be randomly assigned to complete different conditions (one would be randomly assigned to the traumatic experiences writing condition and the other to the neutral writing condition). The next two healthiest participants would then be randomly assigned to complete different conditions, and so on until the two least healthy participants. This method would ensure that participants in the traumatic experiences writing condition are matched to participants in the neutral writing condition with respect to health at the beginning of the study. If at the end of the experiment, a difference in health was detected across the two conditions, then we would know that it is due to the writing manipulation and not to pre-existing differences in health.
Within-Subjects Experiments
In a within-subjects experiment , each participant is tested under all conditions. Consider an experiment on the effect of a defendant’s physical attractiveness on judgments of his guilt. Again, in a between-subjects experiment, one group of participants would be shown an attractive defendant and asked to judge his guilt, and another group of participants would be shown an unattractive defendant and asked to judge his guilt. In a within-subjects experiment, however, the same group of participants would judge the guilt of both an attractive and an unattractive defendant.
The primary advantage of this approach is that it provides maximum control of extraneous participant variables. Participants in all conditions have the same mean IQ, same socioeconomic status, same number of siblings, and so on—because they are the very same people. Within-subjects experiments also make it possible to use statistical procedures that remove the effect of these extraneous participant variables on the dependent variable and therefore make the data less “noisy” and the effect of the independent variable easier to detect. We will look more closely at this idea later in the book . However, not all experiments can use a within-subjects design nor would it be desirable to do so.
One disadvantage of within-subjects experiments is that they make it easier for participants to guess the hypothesis. For example, a participant who is asked to judge the guilt of an attractive defendant and then is asked to judge the guilt of an unattractive defendant is likely to guess that the hypothesis is that defendant attractiveness affects judgments of guilt. This knowledge could lead the participant to judge the unattractive defendant more harshly because he thinks this is what he is expected to do. Or it could make participants judge the two defendants similarly in an effort to be “fair.”
Carryover Effects and Counterbalancing
The primary disadvantage of within-subjects designs is that they can result in order effects. An order effect occurs when participants’ responses in the various conditions are affected by the order of conditions to which they were exposed. One type of order effect is a carryover effect. A carryover effect is an effect of being tested in one condition on participants’ behavior in later conditions. One type of carryover effect is a practice effect , where participants perform a task better in later conditions because they have had a chance to practice it. Another type is a fatigue effect , where participants perform a task worse in later conditions because they become tired or bored. Being tested in one condition can also change how participants perceive stimuli or interpret their task in later conditions. This type of effect is called a context effect (or contrast effect) . For example, an average-looking defendant might be judged more harshly when participants have just judged an attractive defendant than when they have just judged an unattractive defendant. Within-subjects experiments also make it easier for participants to guess the hypothesis. For example, a participant who is asked to judge the guilt of an attractive defendant and then is asked to judge the guilt of an unattractive defendant is likely to guess that the hypothesis is that defendant attractiveness affects judgments of guilt.
Carryover effects can be interesting in their own right. (Does the attractiveness of one person depend on the attractiveness of other people that we have seen recently?) But when they are not the focus of the research, carryover effects can be problematic. Imagine, for example, that participants judge the guilt of an attractive defendant and then judge the guilt of an unattractive defendant. If they judge the unattractive defendant more harshly, this might be because of his unattractiveness. But it could be instead that they judge him more harshly because they are becoming bored or tired. In other words, the order of the conditions is a confounding variable. The attractive condition is always the first condition and the unattractive condition the second. Thus any difference between the conditions in terms of the dependent variable could be caused by the order of the conditions and not the independent variable itself.
There is a solution to the problem of order effects, however, that can be used in many situations. It is counterbalancing , which means testing different participants in different orders. The best method of counterbalancing is complete counterbalancing in which an equal number of participants complete each possible order of conditions. For example, half of the participants would be tested in the attractive defendant condition followed by the unattractive defendant condition, and others half would be tested in the unattractive condition followed by the attractive condition. With three conditions, there would be six different orders (ABC, ACB, BAC, BCA, CAB, and CBA), so some participants would be tested in each of the six orders. With four conditions, there would be 24 different orders; with five conditions there would be 120 possible orders. With counterbalancing, participants are assigned to orders randomly, using the techniques we have already discussed. Thus, random assignment plays an important role in within-subjects designs just as in between-subjects designs. Here, instead of randomly assigning to conditions, they are randomly assigned to different orders of conditions. In fact, it can safely be said that if a study does not involve random assignment in one form or another, it is not an experiment.
A more efficient way of counterbalancing is through a Latin square design which randomizes through having equal rows and columns. For example, if you have four treatments, you must have four versions. Like a Sudoku puzzle, no treatment can repeat in a row or column. For four versions of four treatments, the Latin square design would look like:
You can see in the diagram above that the square has been constructed to ensure that each condition appears at each ordinal position (A appears first once, second once, third once, and fourth once) and each condition preceded and follows each other condition one time. A Latin square for an experiment with 6 conditions would by 6 x 6 in dimension, one for an experiment with 8 conditions would be 8 x 8 in dimension, and so on. So while complete counterbalancing of 6 conditions would require 720 orders, a Latin square would only require 6 orders.
Finally, when the number of conditions is large experiments can use random counterbalancing in which the order of the conditions is randomly determined for each participant. Using this technique every possible order of conditions is determined and then one of these orders is randomly selected for each participant. This is not as powerful a technique as complete counterbalancing or partial counterbalancing using a Latin squares design. Use of random counterbalancing will result in more random error, but if order effects are likely to be small and the number of conditions is large, this is an option available to researchers.
There are two ways to think about what counterbalancing accomplishes. One is that it controls the order of conditions so that it is no longer a confounding variable. Instead of the attractive condition always being first and the unattractive condition always being second, the attractive condition comes first for some participants and second for others. Likewise, the unattractive condition comes first for some participants and second for others. Thus any overall difference in the dependent variable between the two conditions cannot have been caused by the order of conditions. A second way to think about what counterbalancing accomplishes is that if there are carryover effects, it makes it possible to detect them. One can analyze the data separately for each order to see whether it had an effect.
When 9 Is “Larger” Than 221
Researcher Michael Birnbaum has argued that the lack of context provided by between-subjects designs is often a bigger problem than the context effects created by within-subjects designs. To demonstrate this problem, he asked participants to rate two numbers on how large they were on a scale of 1-to-10 where 1 was “very very small” and 10 was “very very large”. One group of participants were asked to rate the number 9 and another group was asked to rate the number 221 (Birnbaum, 1999) [1] . Participants in this between-subjects design gave the number 9 a mean rating of 5.13 and the number 221 a mean rating of 3.10. In other words, they rated 9 as larger than 221! According to Birnbaum, this difference is because participants spontaneously compared 9 with other one-digit numbers (in which case it is relatively large) and compared 221 with other three-digit numbers (in which case it is relatively small).
Simultaneous Within-Subjects Designs
So far, we have discussed an approach to within-subjects designs in which participants are tested in one condition at a time. There is another approach, however, that is often used when participants make multiple responses in each condition. Imagine, for example, that participants judge the guilt of 10 attractive defendants and 10 unattractive defendants. Instead of having people make judgments about all 10 defendants of one type followed by all 10 defendants of the other type, the researcher could present all 20 defendants in a sequence that mixed the two types. The researcher could then compute each participant’s mean rating for each type of defendant. Or imagine an experiment designed to see whether people with social anxiety disorder remember negative adjectives (e.g., “stupid,” “incompetent”) better than positive ones (e.g., “happy,” “productive”). The researcher could have participants study a single list that includes both kinds of words and then have them try to recall as many words as possible. The researcher could then count the number of each type of word that was recalled.
Between-Subjects or Within-Subjects?
Almost every experiment can be conducted using either a between-subjects design or a within-subjects design. This possibility means that researchers must choose between the two approaches based on their relative merits for the particular situation.
Between-subjects experiments have the advantage of being conceptually simpler and requiring less testing time per participant. They also avoid carryover effects without the need for counterbalancing. Within-subjects experiments have the advantage of controlling extraneous participant variables, which generally reduces noise in the data and makes it easier to detect a relationship between the independent and dependent variables.
A good rule of thumb, then, is that if it is possible to conduct a within-subjects experiment (with proper counterbalancing) in the time that is available per participant—and you have no serious concerns about carryover effects—this design is probably the best option. If a within-subjects design would be difficult or impossible to carry out, then you should consider a between-subjects design instead. For example, if you were testing participants in a doctor’s waiting room or shoppers in line at a grocery store, you might not have enough time to test each participant in all conditions and therefore would opt for a between-subjects design. Or imagine you were trying to reduce people’s level of prejudice by having them interact with someone of another race. A within-subjects design with counterbalancing would require testing some participants in the treatment condition first and then in a control condition. But if the treatment works and reduces people’s level of prejudice, then they would no longer be suitable for testing in the control condition. This difficulty is true for many designs that involve a treatment meant to produce long-term change in participants’ behavior (e.g., studies testing the effectiveness of psychotherapy). Clearly, a between-subjects design would be necessary here.
Remember also that using one type of design does not preclude using the other type in a different study. There is no reason that a researcher could not use both a between-subjects design and a within-subjects design to answer the same research question. In fact, professional researchers often take exactly this type of mixed methods approach.
Key Takeaways
- Experiments can be conducted using either between-subjects or within-subjects designs. Deciding which to use in a particular situation requires careful consideration of the pros and cons of each approach.
- Random assignment to conditions in between-subjects experiments or counterbalancing of orders of conditions in within-subjects experiments is a fundamental element of experimental research. The purpose of these techniques is to control extraneous variables so that they do not become confounding variables.
- You want to test the relative effectiveness of two training programs for running a marathon.
- Using photographs of people as stimuli, you want to see if smiling people are perceived as more intelligent than people who are not smiling.
- In a field experiment, you want to see if the way a panhandler is dressed (neatly vs. sloppily) affects whether or not passersby give him any money.
- You want to see if concrete nouns (e.g., dog ) are recalled better than abstract nouns (e.g., truth).
- Birnbaum, M.H. (1999). How to show that 9>221: Collect judgments in a between-subjects design. Psychological Methods, 4 (3), 243-249. ↵

Share This Book
- Increase Font Size
- - Google Chrome
Intended for healthcare professionals
- Access provided by Google Indexer
- My email alerts
- BMA member login
- Username * Password * Forgot your log in details? Need to activate BMA Member Log In Log in via OpenAthens Log in via your institution

Search form
- Advanced search
- Search responses
- Search blogs
- Beauty sleep:...
Beauty sleep: experimental study on the perceived health and attractiveness of sleep deprived people
- Related content
- Peer review
- John Axelsson , researcher 1 2 ,
- Tina Sundelin , research assistant and MSc student 2 ,
- Michael Ingre , statistician and PhD student 3 ,
- Eus J W Van Someren , researcher 4 ,
- Andreas Olsson , researcher 2 ,
- Mats Lekander , researcher 1 3
- 1 Osher Center for Integrative Medicine, Department of Clinical Neuroscience, Karolinska Institutet, 17177 Stockholm, Sweden
- 2 Division for Psychology, Department of Clinical Neuroscience, Karolinska Institutet
- 3 Stress Research Institute, Stockholm University, Stockholm
- 4 Netherlands Institute for Neuroscience, an Institute of the Royal Netherlands Academy of Arts and Sciences, and VU Medical Center, Amsterdam, Netherlands
- Correspondence to: J Axelsson john.axelsson{at}ki.se
- Accepted 22 October 2010
Objective To investigate whether sleep deprived people are perceived as less healthy, less attractive, and more tired than after a normal night’s sleep.
Design Experimental study.
Setting Sleep laboratory in Stockholm, Sweden.
Participants 23 healthy, sleep deprived adults (age 18-31) who were photographed and 65 untrained observers (age 18-61) who rated the photographs.
Intervention Participants were photographed after a normal night’s sleep (eight hours) and after sleep deprivation (31 hours of wakefulness after a night of reduced sleep). The photographs were presented in a randomised order and rated by untrained observers.
Main outcome measure Difference in observer ratings of perceived health, attractiveness, and tiredness between sleep deprived and well rested participants using a visual analogue scale (100 mm).
Results Sleep deprived people were rated as less healthy (visual analogue scale scores, mean 63 (SE 2) v 68 (SE 2), P<0.001), more tired (53 (SE 3) v 44 (SE 3), P<0.001), and less attractive (38 (SE 2) v 40 (SE 2), P<0.001) than after a normal night’s sleep. The decrease in rated health was associated with ratings of increased tiredness and decreased attractiveness.
Conclusion Our findings show that sleep deprived people appear less healthy, less attractive, and more tired compared with when they are well rested. This suggests that humans are sensitive to sleep related facial cues, with potential implications for social and clinical judgments and behaviour. Studies are warranted for understanding how these effects may affect clinical decision making and can add knowledge with direct implications in a medical context.
Introduction
The recognition [of the case] depends in great measure on the accurate and rapid appreciation of small points in which the diseased differs from the healthy state Joseph Bell (1837-1911)
Good clinical judgment is an important skill in medical practice. This is well illustrated in the quote by Joseph Bell, 1 who demonstrated impressive observational and deductive skills. Bell was one of Sir Arthur Conan Doyle’s teachers and served as a model for the fictitious detective Sherlock Holmes. 2 Generally, human judgment involves complex processes, whereby ingrained, often less consciously deliberated responses from perceptual cues are mixed with semantic calculations to affect decision making. 3 Thus all social interactions, including diagnosis in clinical practice, are influenced by reflexive as well as reflective processes in human cognition and communication.
Sleep is an essential homeostatic process with well established effects on an individual’s physiological, cognitive, and behavioural functionality 4 5 6 7 and long term health, 8 but with only anecdotal support of a role in social perception, such as that underlying judgments of attractiveness and health. As illustrated by the common expression “beauty sleep,” an individual’s sleep history may play an integral part in the perception and judgments of his or her attractiveness and health. To date, the concept of beauty sleep has lacked scientific support, but the biological importance of sleep may have favoured a sensitivity to perceive sleep related cues in others. It seems warranted to explore such sensitivity, as sleep disorders and disturbed sleep are increasingly common in today’s 24 hour society and often coexist with some of the most common health problems, such as hypertension 9 10 and inflammatory conditions. 11
To describe the relation between sleep deprivation and perceived health and attractiveness we asked untrained observers to rate the faces of people who had been photographed after a normal night’s sleep and after a night of sleep deprivation. We chose facial photographs as the human face is the primary source of information in social communication. 12 A perceiver’s response to facial cues, signalling the bearer’s emotional state, intentions, and potential mate value, serves to guide actions in social contexts and may ultimately promote survival. 13 14 15 We hypothesised that untrained observers would perceive sleep deprived people as more tired, less healthy, and less attractive compared with after a normal night’s sleep.
Using an experimental design we photographed the faces of 23 adults (mean age 23, range 18-31 years, 11 women) between 14.00 and 15.00 under two conditions in a balanced design: after a normal night’s sleep (at least eight hours of sleep between 23.00-07.00 and seven hours of wakefulness) and after sleep deprivation (sleep 02.00-07.00 and 31 hours of wakefulness). We advertised for participants at four universities in the Stockholm area. Twenty of 44 potentially eligible people were excluded. Reasons for exclusion were reported sleep disturbances, abnormal sleep requirements (for example, sleep need out of the 7-9 hour range), health problems, or availability on study days (the main reason). We also excluded smokers and those who had consumed alcohol within two days of the protocol. One woman failed to participate in both conditions. Overall, we enrolled 12 women and 12 men.
The participants slept in their own homes. Sleep times were confirmed with sleep diaries and text messages. The sleep diaries (Karolinska sleep diary) included information on sleep latency, quality, duration, and sleepiness. Participants sent a text message to the research assistant by mobile phone (SMS) at bedtime and when they got up on the night before sleep deprivation. They had been instructed not to nap. During the normal sleep condition the participants’ mean duration of sleep, estimated from sleep diaries, was 8.45 (SE 0.20) hours. The sleep deprivation condition started with a restriction of sleep to five hours in bed; the participants sent text messages (SMS) when they went to sleep and when they woke up. The mean duration of sleep during this night, estimated from sleep diaries and text messages, was 5.06 (SE 0.04) hours. For the following night of total sleep deprivation, the participants were monitored in the sleep laboratory at all times. Thus, for the sleep deprivation condition, participants came to the laboratory at 22.00 (after 15 hours of wakefulness) to be monitored, and stayed awake for a further 16 hours. We therefore did not observe the participants during the first 15 hours of wakefulness, when they had had a slightly restricted sleep, but had good control over the last 16 hours of wakefulness when sleepiness increased in magnitude. For the sleep condition, participants came to the laboratory at 12.00 (after five hours of wakefulness). They were kept indoors two hours before being photographed to avoid the effects of exposure to sunlight and the weather. We had a series of five or six photographs (resolution 3872×2592 pixels) taken in a well lit room, with a constant white balance (×900l; colour temperature 4200 K, Nikon D80; Nikon, Tokyo). The white balance was differently set during the two days of the study and affected seven photographs (four taken during sleep deprivation and three during a normal night’s sleep). Removing these participants from the analyses did not affect the results. The distance from camera to head was fixed, as was the focal length, within 14 mm (between 44 and 58 mm). To ensure a fixed surface area of each face on the photograph, the focal length was adapted to the head size of each participant.
For the photo shoot, participants wore no makeup, had their hair loose (combed backwards if long), underwent similar cleaning or shaving procedures for both conditions, and were instructed to “sit with a straight back and look straight into the camera with a neutral, relaxed facial expression.” Although the photographer was not blinded to the sleep conditions, she followed a highly standardised procedure during each photo shoot, including minimal interaction with the participants. A blinded rater chose the most typical photograph from each series of photographs. This process resulted in 46 photographs; two (one from each sleep condition) of each of the 23 participants. This part of the study took place between June and September 2007.
In October 2007 the photographs were presented at a fixed interval of six seconds in a randomised order to 65 observers (mainly students at the Karolinska Institute, mean age 30 (range 18-61) years, 40 women), who were unaware of the conditions of the study. They rated the faces for attractiveness (very unattractive to very attractive), health (very sick to very healthy), and tiredness (not at all tired to very tired) on a 100 mm visual analogue scale. After every 23 photographs a brief intermission was allowed, including a working memory task lasting 23 seconds to prevent the faces being memorised. To ensure that the observers were not primed to tiredness when rating health and attractiveness they rated the photographs for attractiveness and health in the first two sessions and tiredness in the last. To avoid the influence of possible order effects we presented the photographs in a balanced order between conditions for each session.
Statistical analyses
Data were analysed using multilevel mixed effects linear regression, with two crossed independent random effects accounting for random variation between observers and participants using the xtmixed procedure in Stata 9.2. We present the effect of condition as a percentage of change from the baseline condition as the reference using the absolute value in millimetres (rated on the visual analogue scale). No data were missing in the analyses.
Sixty five observers rated each of the 46 photographs for attractiveness, health, and tiredness: 138 ratings by each observer and 2990 ratings for each of the three factors rated. When sleep deprived, people were rated as less healthy (visual analogue scale scores, mean 63 (SE 2) v 68 (SE 2)), more tired (53 (SE 3) v 44 (SE 3)), and less attractive (38 (SE 2) v 40 (SE 2); P<0.001 for all) than after a normal night’s sleep (table 1 ⇓ ). Compared with the normal sleep condition, perceptions of health and attractiveness in the sleep deprived condition decreased on average by 6% and 4% and tiredness increased by 19%.
Multilevel mixed effects regression on effect of how sleep deprived people are perceived with respect to attractiveness, health, and tiredness
- View inline
A 10 mm increase in tiredness was associated with a −3.0 mm change in health, a 10 mm increase in health increased attractiveness by 2.4 mm, and a 10 mm increase in tiredness reduced attractiveness by 1.2 mm (table 2 ⇓ ). These findings were also presented as correlation, suggesting that faces with perceived attractiveness are positively associated with perceived health (r=0.42, fig 1 ⇓ ) and negatively with perceived tiredness (r=−0.28, fig 1). In addition, the average decrease (for each face) in attractiveness as a result of deprived sleep was associated with changes in tiredness (−0.53, n=23, P=0.03) and in health (0.50, n=23, P=0.01). Moreover, a strong negative association was found between the respective perceptions of tiredness and health (r=−0.54, fig 1). Figure 2 ⇓ shows an example of observer rated faces.
Associations between health, tiredness, and attractiveness
Fig 1 Relations between health, tiredness, and attractiveness of 46 photographs (two each of 23 participants) rated by 65 observers on 100 mm visual analogue scales, with variation between observers removed using empirical Bayes’ estimates
- Download figure
- Open in new tab
- Download powerpoint
Fig 2 Participant after a normal night’s sleep (left) and after sleep deprivation (right). Faces were presented in a counterbalanced order
To evaluate the mediation effects of sleep loss on attractiveness and health, tiredness was added to the models presented in table 1 following recommendations. 16 The effect of sleep loss was significantly mediated by tiredness on both health (P<0.001) and attractiveness (P<0.001). When tiredness was added to the model (table 1) with an estimated coefficient of −2.9 (SE 0.1; P<0.001) the independent effect of sleep loss on health decreased from −4.2 to −1.8 (SE 0.5; P<0.001). The effect of sleep loss on attractiveness decreased from −1.6 (table 1) to −0.62 (SE 0.4; P=0.133), with tiredness estimated at −1.1 (SE 0.1; P<0.001). The same approach applied to the model of attractiveness and health (table 2), with a decrease in the association from 2.4 to 2.1 (SE 0.1; P<0.001) with tiredness estimated at −0.56 (SE 0.1; P<0.001).
Sleep deprived people are perceived as less attractive, less healthy, and more tired compared with when they are well rested. Apparent tiredness was strongly related to looking less healthy and less attractive, which was also supported by the mediating analyses, indicating that a large part of the found effects and relations on appearing healthy and attractive were mediated by looking tired. The fact that untrained observers detected the effects of sleep loss in others not only provides evidence for a perceptual ability not previously subjected to experimental control, but also supports the notion that sleep history gives rise to socially relevant signals that provide information about the bearer. The adaptiveness of an ability to detect sleep related facial cues resonates well with other research, showing that small deviations from the average sleep duration in the long term are associated with an increased risk of health problems and with a decreased longevity. 8 17 Indeed, even a few hours of sleep deprivation inflict an array of physiological changes, including neural, endocrinological, immunological, and cellular functioning, that if sustained are relevant for long term health. 7 18 19 20 Here, we show that such physiological changes are paralleled by detectable facial changes.
These results are related to photographs taken in an artificial setting and presented to the observers for only six seconds. It is likely that the effects reported here would be larger in real life person to person situations, when overt behaviour and interactions add further information. Blink interval and blink duration are known to be indicators of sleepiness, 21 and trained observers are able to evaluate reliably the drowsiness of drivers by watching their videotaped faces. 22 In addition, a few of the people were perceived as healthier, less tired, and more attractive during the sleep deprived condition. It remains to be evaluated in follow-up research whether this is due to random error noise in judgments, or associated with specific characteristics of observers or the sleep deprived people they judge. Nevertheless, we believe that the present findings can be generalised to a wide variety of settings, but further studies will have to investigate the impact on clinical studies and other social situations.
Importantly, our findings suggest a prominent role of sleep history in several domains of interpersonal perception and judgment, in which sleep history has previously not been considered of importance, such as in clinical judgment. In addition, because attractiveness motivates sexual behaviour, collaboration, and superior treatment, 13 sleep loss may have consequences in other social contexts. For example, it has been proposed that facial cues perceived as attractive are signals of good health and that this recognition has been selected evolutionarily to guide choice of mate and successful transmission of genes. 13 The fact that good sleep supports a healthy look and poor sleep the reverse may be of particular relevance in the medical setting, where health estimates are an essential part. It is possible that people with sleep disturbances, clinical or otherwise, would be judged as more unhealthy, whereas those who have had an unusually good night’s sleep may be perceived as rather healthy. Compared with the sleep deprivation used in the present investigation, further studies are needed to investigate the effects of less drastic acute reductions of sleep as well as long term clinical effects.
Conclusions
People are capable of detecting sleep loss related facial cues, and these cues modify judgments of another’s health and attractiveness. These conclusions agree well with existing models describing a link between sleep and good health, 18 23 as well as a link between attractiveness and health. 13 Future studies should focus on the relevance of these facial cues in clinical settings. These could investigate whether clinicians are better than the average population at detecting sleep or health related facial cues, and whether patients with a clinical diagnosis exhibit more tiredness and are less healthy looking than healthy people. Perhaps the more successful doctors are those who pick up on these details and act accordingly.
Taken together, our results provide important insights into judgments about health and attractiveness that are reminiscent of the anecdotal wisdom harboured in Bell’s words, and in the colloquial notion of “beauty sleep.”
What is already known on this topic
Short or disturbed sleep and fatigue constitute major risk factors for health and safety
Complaints of short or disturbed sleep are common among patients seeking healthcare
The human face is the main source of information for social signalling
What this study adds
The facial cues of sleep deprived people are sufficient for others to judge them as more tired, less healthy, and less attractive, lending the first scientific support to the concept of “beauty sleep”
By affecting doctors’ general perception of health, the sleep history of a patient may affect clinical decisions and diagnostic precision
Cite this as: BMJ 2010;341:c6614
We thank B Karshikoff for support with data acquisition and M Ingvar for comments on an earlier draft of the manuscript, both without compensation and working at the Department for Clinical Neuroscience, Karolinska Institutet, Sweden.
Contributors: JA designed the data collection, supervised and monitored data collection, wrote the statistical analysis plan, carried out the statistical analyses, obtained funding, drafted and revised the manuscript, and is guarantor. TS designed and carried out the data collection, cleaned the data, drafted, revised the manuscript, and had final approval of the manuscript. JA and TS contributed equally to the work. MI wrote the statistical analysis plan, carried out the statistical analyses, drafted the manuscript, and critically revised the manuscript. EJWVS provided statistical advice, advised on data handling, and critically revised the manuscript. AO provided advice on the methods and critically revised the manuscript. ML provided administrative support, drafted the manuscript, and critically revised the manuscript. All authors approved the final version of the manuscript.
Funding: This study was funded by the Swedish Society for Medical Research, Rut and Arvid Wolff’s Memory Fund, and the Osher Center for Integrative Medicine.
Competing interests: All authors have completed the Unified Competing Interest form at www.icmje.org/coi_disclosure.pdf (available on request from the corresponding author) and declare: no support from any company for the submitted work; no financial relationships with any companies that might have an interest in the submitted work in the previous 3 years; no other relationships or activities that could appear to have influenced the submitted work.
Ethical approval: This study was approved by the Karolinska Institutet’s ethical committee. Participants were compensated for their participation.
Participant consent: Participant’s consent obtained.
Data sharing: Statistical code and dataset of ratings are available from the corresponding author at john.axelsson{at}ki.se .
This is an open-access article distributed under the terms of the Creative Commons Attribution Non-commercial License, which permits use, distribution, and reproduction in any medium, provided the original work is properly cited, the use is non commercial and is otherwise in compliance with the license. See: http://creativecommons.org/licenses/by-nc/2.0/ and http://creativecommons.org/licenses/by-nc/2.0/legalcode .
- ↵ Deten A, Volz HC, Clamors S, Leiblein S, Briest W, Marx G, et al. Hematopoietic stem cells do not repair the infarcted mouse heart. Cardiovasc Res 2005 ; 65 : 52 -63. OpenUrl Abstract / FREE Full Text
- ↵ Doyle AC. The case-book of Sherlock Holmes: selected stories. Wordsworth, 1993.
- ↵ Lieberman MD, Gaunt R, Gilbert DT, Trope Y. Reflection and reflexion: a social cognitive neuroscience approach to attributional inference. Adv Exp Soc Psychol 2002 ; 34 : 199 -249. OpenUrl CrossRef
- ↵ Drummond SPA, Brown GG, Gillin JC, Stricker JL, Wong EC, Buxton RB. Altered brain response to verbal learning following sleep deprivation. Nature 2000 ; 403 : 655 -7. OpenUrl CrossRef PubMed
- ↵ Harrison Y, Horne JA. The impact of sleep deprivation on decision making: a review. J Exp Psychol Appl 2000 ; 6 : 236 -49. OpenUrl CrossRef PubMed Web of Science
- ↵ Huber R, Ghilardi MF, Massimini M, Tononi G. Local sleep and learning. Nature 2004 ; 430 : 78 -81. OpenUrl CrossRef PubMed Web of Science
- ↵ Spiegel K, Leproult R, Van Cauter E. Impact of sleep debt on metabolic and endocrine function. Lancet 1999 ; 354 : 1435 -9. OpenUrl CrossRef PubMed Web of Science
- ↵ Kripke DF, Garfinkel L, Wingard DL, Klauber MR, Marler MR. Mortality associated with sleep duration and insomnia. Arch Gen Psychiatry 2002 ; 59 : 131 -6. OpenUrl CrossRef PubMed Web of Science
- ↵ Olson LG, Ambrogetti A. Waking up to sleep disorders. Br J Hosp Med (Lond) 2006 ; 67 : 118 , 20. OpenUrl PubMed
- ↵ Rajaratnam SM, Arendt J. Health in a 24-h society. Lancet 2001 ; 358 : 999 -1005. OpenUrl CrossRef PubMed Web of Science
- ↵ Ranjbaran Z, Keefer L, Stepanski E, Farhadi A, Keshavarzian A. The relevance of sleep abnormalities to chronic inflammatory conditions. Inflamm Res 2007 ; 56 : 51 -7. OpenUrl CrossRef PubMed Web of Science
- ↵ Haxby JV, Hoffman EA, Gobbini MI. The distributed human neural system for face perception. Trends Cogn Sci 2000 ; 4 : 223 -33. OpenUrl CrossRef PubMed Web of Science
- ↵ Rhodes G. The evolutionary psychology of facial beauty. Annu Rev Psychol 2006 ; 57 : 199 -226. OpenUrl CrossRef PubMed Web of Science
- ↵ Todorov A, Mandisodza AN, Goren A, Hall CC. Inferences of competence from faces predict election outcomes. Science 2005 ; 308 : 1623 -6. OpenUrl Abstract / FREE Full Text
- ↵ Willis J, Todorov A. First impressions: making up your mind after a 100-ms exposure to a face. Psychol Sci 2006 ; 17 : 592 -8. OpenUrl Abstract / FREE Full Text
- ↵ Krull JL, MacKinnon DP. Multilevel modeling of individual and group level mediated effects. Multivariate Behav Res 2001 ; 36 : 249 -77. OpenUrl CrossRef Web of Science
- ↵ Ayas NT, White DP, Manson JE, Stampfer MJ, Speizer FE, Malhotra A, et al. A prospective study of sleep duration and coronary heart disease in women. Arch Intern Med 2003 ; 163 : 205 -9. OpenUrl CrossRef PubMed Web of Science
- ↵ Bryant PA, Trinder J, Curtis N. Sick and tired: does sleep have a vital role in the immune system. Nat Rev Immunol 2004 ; 4 : 457 -67. OpenUrl CrossRef PubMed Web of Science
- ↵ Cirelli C. Cellular consequences of sleep deprivation in the brain. Sleep Med Rev 2006 ; 10 : 307 -21. OpenUrl CrossRef PubMed Web of Science
- ↵ Irwin MR, Wang M, Campomayor CO, Collado-Hidalgo A, Cole S. Sleep deprivation and activation of morning levels of cellular and genomic markers of inflammation. Arch Intern Med 2006 ; 166 : 1756 -62. OpenUrl CrossRef PubMed Web of Science
- ↵ Schleicher R, Galley N, Briest S, Galley L. Blinks and saccades as indicators of fatigue in sleepiness warnings: looking tired? Ergonomics 2008 ; 51 : 982 -1010. OpenUrl CrossRef PubMed Web of Science
- ↵ Wierwille WW, Ellsworth LA. Evaluation of driver drowsiness by trained raters. Accid Anal Prev 1994 ; 26 : 571 -81. OpenUrl CrossRef PubMed Web of Science
- ↵ Horne J. Why we sleep—the functions of sleep in humans and other mammals. Oxford University Press, 1988.
- The 25 Most Influential Psychological Experiments in History

While each year thousands and thousands of studies are completed in the many specialty areas of psychology, there are a handful that, over the years, have had a lasting impact in the psychological community as a whole. Some of these were dutifully conducted, keeping within the confines of ethical and practical guidelines. Others pushed the boundaries of human behavior during their psychological experiments and created controversies that still linger to this day. And still others were not designed to be true psychological experiments, but ended up as beacons to the psychological community in proving or disproving theories.
This is a list of the 25 most influential psychological experiments still being taught to psychology students of today.
1. A Class Divided
Study conducted by: jane elliott.
Study Conducted in 1968 in an Iowa classroom

Experiment Details: Jane Elliott’s famous experiment was inspired by the assassination of Dr. Martin Luther King Jr. and the inspirational life that he led. The third grade teacher developed an exercise, or better yet, a psychological experiment, to help her Caucasian students understand the effects of racism and prejudice.
Elliott divided her class into two separate groups: blue-eyed students and brown-eyed students. On the first day, she labeled the blue-eyed group as the superior group and from that point forward they had extra privileges, leaving the brown-eyed children to represent the minority group. She discouraged the groups from interacting and singled out individual students to stress the negative characteristics of the children in the minority group. What this exercise showed was that the children’s behavior changed almost instantaneously. The group of blue-eyed students performed better academically and even began bullying their brown-eyed classmates. The brown-eyed group experienced lower self-confidence and worse academic performance. The next day, she reversed the roles of the two groups and the blue-eyed students became the minority group.
At the end of the experiment, the children were so relieved that they were reported to have embraced one another and agreed that people should not be judged based on outward appearances. This exercise has since been repeated many times with similar outcomes.
For more information click here
2. Asch Conformity Study
Study conducted by: dr. solomon asch.
Study Conducted in 1951 at Swarthmore College

Experiment Details: Dr. Solomon Asch conducted a groundbreaking study that was designed to evaluate a person’s likelihood to conform to a standard when there is pressure to do so.
A group of participants were shown pictures with lines of various lengths and were then asked a simple question: Which line is longest? The tricky part of this study was that in each group only one person was a true participant. The others were actors with a script. Most of the actors were instructed to give the wrong answer. Strangely, the one true participant almost always agreed with the majority, even though they knew they were giving the wrong answer.
The results of this study are important when we study social interactions among individuals in groups. This study is a famous example of the temptation many of us experience to conform to a standard during group situations and it showed that people often care more about being the same as others than they do about being right. It is still recognized as one of the most influential psychological experiments for understanding human behavior.
3. Bobo Doll Experiment
Study conducted by: dr. alburt bandura.
Study Conducted between 1961-1963 at Stanford University

In his groundbreaking study he separated participants into three groups:
- one was exposed to a video of an adult showing aggressive behavior towards a Bobo doll
- another was exposed to video of a passive adult playing with the Bobo doll
- the third formed a control group
Children watched their assigned video and then were sent to a room with the same doll they had seen in the video (with the exception of those in the control group). What the researcher found was that children exposed to the aggressive model were more likely to exhibit aggressive behavior towards the doll themselves. The other groups showed little imitative aggressive behavior. For those children exposed to the aggressive model, the number of derivative physical aggressions shown by the boys was 38.2 and 12.7 for the girls.
The study also showed that boys exhibited more aggression when exposed to aggressive male models than boys exposed to aggressive female models. When exposed to aggressive male models, the number of aggressive instances exhibited by boys averaged 104. This is compared to 48.4 aggressive instances exhibited by boys who were exposed to aggressive female models.
While the results for the girls show similar findings, the results were less drastic. When exposed to aggressive female models, the number of aggressive instances exhibited by girls averaged 57.7. This is compared to 36.3 aggressive instances exhibited by girls who were exposed to aggressive male models. The results concerning gender differences strongly supported Bandura’s secondary prediction that children will be more strongly influenced by same-sex models. The Bobo Doll Experiment showed a groundbreaking way to study human behavior and it’s influences.
4. Car Crash Experiment
Study conducted by: elizabeth loftus and john palmer.
Study Conducted in 1974 at The University of California in Irvine
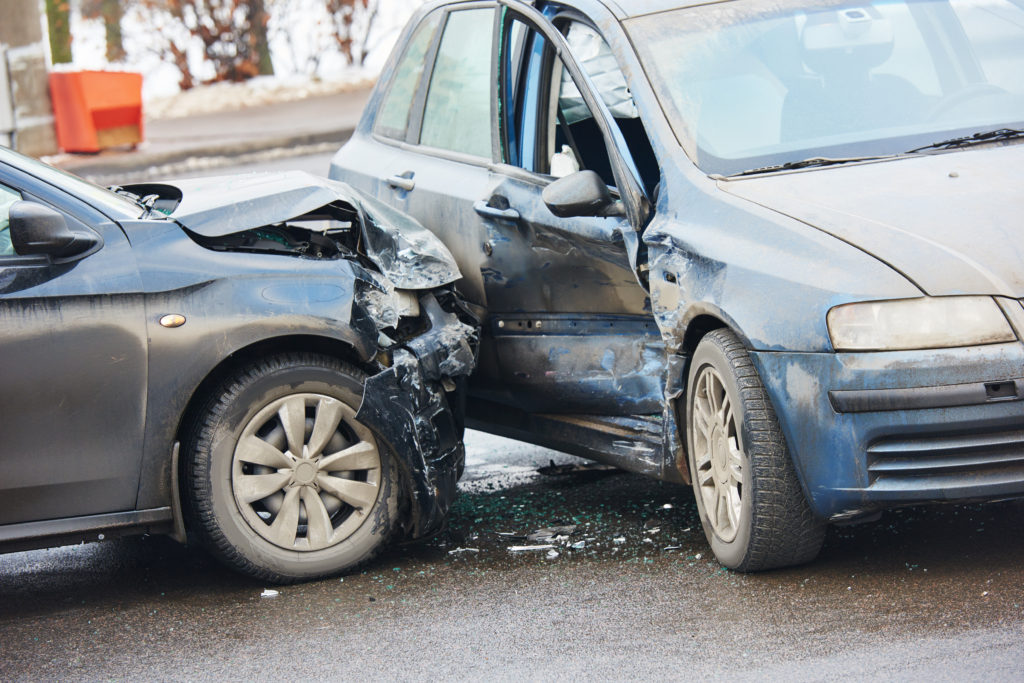
The participants watched slides of a car accident and were asked to describe what had happened as if they were eyewitnesses to the scene. The participants were put into two groups and each group was questioned using different wording such as “how fast was the car driving at the time of impact?” versus “how fast was the car going when it smashed into the other car?” The experimenters found that the use of different verbs affected the participants’ memories of the accident, showing that memory can be easily distorted.
This research suggests that memory can be easily manipulated by questioning technique. This means that information gathered after the event can merge with original memory causing incorrect recall or reconstructive memory. The addition of false details to a memory of an event is now referred to as confabulation. This concept has very important implications for the questions used in police interviews of eyewitnesses.
5. Cognitive Dissonance Experiment
Study conducted by: leon festinger and james carlsmith.
Study Conducted in 1957 at Stanford University
Experiment Details: The concept of cognitive dissonance refers to a situation involving conflicting:
This conflict produces an inherent feeling of discomfort leading to a change in one of the attitudes, beliefs or behaviors to minimize or eliminate the discomfort and restore balance.
Cognitive dissonance was first investigated by Leon Festinger, after an observational study of a cult that believed that the earth was going to be destroyed by a flood. Out of this study was born an intriguing experiment conducted by Festinger and Carlsmith where participants were asked to perform a series of dull tasks (such as turning pegs in a peg board for an hour). Participant’s initial attitudes toward this task were highly negative.
They were then paid either $1 or $20 to tell a participant waiting in the lobby that the tasks were really interesting. Almost all of the participants agreed to walk into the waiting room and persuade the next participant that the boring experiment would be fun. When the participants were later asked to evaluate the experiment, the participants who were paid only $1 rated the tedious task as more fun and enjoyable than the participants who were paid $20 to lie.
Being paid only $1 is not sufficient incentive for lying and so those who were paid $1 experienced dissonance. They could only overcome that cognitive dissonance by coming to believe that the tasks really were interesting and enjoyable. Being paid $20 provides a reason for turning pegs and there is therefore no dissonance.
6. Fantz’s Looking Chamber
Study conducted by: robert l. fantz.
Study Conducted in 1961 at the University of Illinois
Experiment Details: The study conducted by Robert L. Fantz is among the simplest, yet most important in the field of infant development and vision. In 1961, when this experiment was conducted, there very few ways to study what was going on in the mind of an infant. Fantz realized that the best way was to simply watch the actions and reactions of infants. He understood the fundamental factor that if there is something of interest near humans, they generally look at it.
To test this concept, Fantz set up a display board with two pictures attached. On one was a bulls-eye. On the other was the sketch of a human face. This board was hung in a chamber where a baby could lie safely underneath and see both images. Then, from behind the board, invisible to the baby, he peeked through a hole to watch what the baby looked at. This study showed that a two-month old baby looked twice as much at the human face as it did at the bulls-eye. This suggests that human babies have some powers of pattern and form selection. Before this experiment it was thought that babies looked out onto a chaotic world of which they could make little sense.
7. Hawthorne Effect
Study conducted by: henry a. landsberger.
Study Conducted in 1955 at Hawthorne Works in Chicago, Illinois

Landsberger performed the study by analyzing data from experiments conducted between 1924 and 1932, by Elton Mayo, at the Hawthorne Works near Chicago. The company had commissioned studies to evaluate whether the level of light in a building changed the productivity of the workers. What Mayo found was that the level of light made no difference in productivity. The workers increased their output whenever the amount of light was switched from a low level to a high level, or vice versa.
The researchers noticed a tendency that the workers’ level of efficiency increased when any variable was manipulated. The study showed that the output changed simply because the workers were aware that they were under observation. The conclusion was that the workers felt important because they were pleased to be singled out. They increased productivity as a result. Being singled out was the factor dictating increased productivity, not the changing lighting levels, or any of the other factors that they experimented upon.
The Hawthorne Effect has become one of the hardest inbuilt biases to eliminate or factor into the design of any experiment in psychology and beyond.
8. Kitty Genovese Case
Study conducted by: new york police force.
Study Conducted in 1964 in New York City
Experiment Details: The murder case of Kitty Genovese was never intended to be a psychological experiment, however it ended up having serious implications for the field.
According to a New York Times article, almost 40 neighbors witnessed Kitty Genovese being savagely attacked and murdered in Queens, New York in 1964. Not one neighbor called the police for help. Some reports state that the attacker briefly left the scene and later returned to “finish off” his victim. It was later uncovered that many of these facts were exaggerated. (There were more likely only a dozen witnesses and records show that some calls to police were made).
What this case later become famous for is the “Bystander Effect,” which states that the more bystanders that are present in a social situation, the less likely it is that anyone will step in and help. This effect has led to changes in medicine, psychology and many other areas. One famous example is the way CPR is taught to new learners. All students in CPR courses learn that they must assign one bystander the job of alerting authorities which minimizes the chances of no one calling for assistance.
9. Learned Helplessness Experiment
Study conducted by: martin seligman.
Study Conducted in 1967 at the University of Pennsylvania

Seligman’s experiment involved the ringing of a bell and then the administration of a light shock to a dog. After a number of pairings, the dog reacted to the shock even before it happened. As soon as the dog heard the bell, he reacted as though he’d already been shocked.
During the course of this study something unexpected happened. Each dog was placed in a large crate that was divided down the middle with a low fence. The dog could see and jump over the fence easily. The floor on one side of the fence was electrified, but not on the other side of the fence. Seligman placed each dog on the electrified side and administered a light shock. He expected the dog to jump to the non-shocking side of the fence. In an unexpected turn, the dogs simply laid down.
The hypothesis was that as the dogs learned from the first part of the experiment that there was nothing they could do to avoid the shocks, they gave up in the second part of the experiment. To prove this hypothesis the experimenters brought in a new set of animals and found that dogs with no history in the experiment would jump over the fence.
This condition was described as learned helplessness. A human or animal does not attempt to get out of a negative situation because the past has taught them that they are helpless.
10. Little Albert Experiment
Study conducted by: john b. watson and rosalie rayner.
Study Conducted in 1920 at Johns Hopkins University
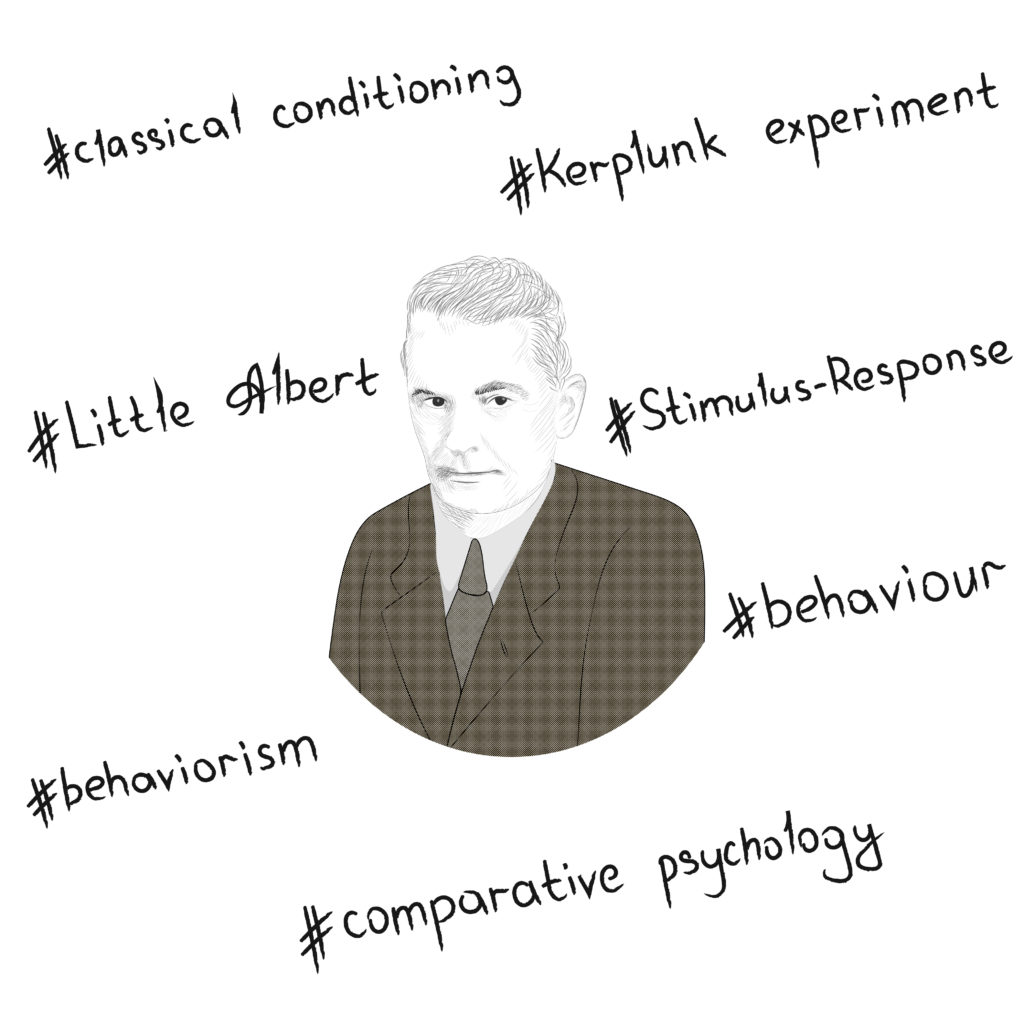
The experiment began by placing a white rat in front of the infant, who initially had no fear of the animal. Watson then produced a loud sound by striking a steel bar with a hammer every time little Albert was presented with the rat. After several pairings (the noise and the presentation of the white rat), the boy began to cry and exhibit signs of fear every time the rat appeared in the room. Watson also created similar conditioned reflexes with other common animals and objects (rabbits, Santa beard, etc.) until Albert feared them all.
This study proved that classical conditioning works on humans. One of its most important implications is that adult fears are often connected to early childhood experiences.
11. Magical Number Seven
Study conducted by: george a. miller.
Study Conducted in 1956 at Princeton University
Experiment Details: Frequently referred to as “ Miller’s Law,” the Magical Number Seven experiment purports that the number of objects an average human can hold in working memory is 7 ± 2. This means that the human memory capacity typically includes strings of words or concepts ranging from 5-9. This information on the limits to the capacity for processing information became one of the most highly cited papers in psychology.
The Magical Number Seven Experiment was published in 1956 by cognitive psychologist George A. Miller of Princeton University’s Department of Psychology in Psychological Review . In the article, Miller discussed a concurrence between the limits of one-dimensional absolute judgment and the limits of short-term memory.
In a one-dimensional absolute-judgment task, a person is presented with a number of stimuli that vary on one dimension (such as 10 different tones varying only in pitch). The person responds to each stimulus with a corresponding response (learned before).
Performance is almost perfect up to five or six different stimuli but declines as the number of different stimuli is increased. This means that a human’s maximum performance on one-dimensional absolute judgment can be described as an information store with the maximum capacity of approximately 2 to 3 bits of information There is the ability to distinguish between four and eight alternatives.
12. Pavlov’s Dog Experiment
Study conducted by: ivan pavlov.
Study Conducted in the 1890s at the Military Medical Academy in St. Petersburg, Russia

Pavlov began with the simple idea that there are some things that a dog does not need to learn. He observed that dogs do not learn to salivate when they see food. This reflex is “hard wired” into the dog. This is an unconditioned response (a stimulus-response connection that required no learning).
Pavlov outlined that there are unconditioned responses in the animal by presenting a dog with a bowl of food and then measuring its salivary secretions. In the experiment, Pavlov used a bell as his neutral stimulus. Whenever he gave food to his dogs, he also rang a bell. After a number of repeats of this procedure, he tried the bell on its own. What he found was that the bell on its own now caused an increase in salivation. The dog had learned to associate the bell and the food. This learning created a new behavior. The dog salivated when he heard the bell. Because this response was learned (or conditioned), it is called a conditioned response. The neutral stimulus has become a conditioned stimulus.
This theory came to be known as classical conditioning.
13. Robbers Cave Experiment
Study conducted by: muzafer and carolyn sherif.
Study Conducted in 1954 at the University of Oklahoma
Experiment Details: This experiment, which studied group conflict, is considered by most to be outside the lines of what is considered ethically sound.
In 1954 researchers at the University of Oklahoma assigned 22 eleven- and twelve-year-old boys from similar backgrounds into two groups. The two groups were taken to separate areas of a summer camp facility where they were able to bond as social units. The groups were housed in separate cabins and neither group knew of the other’s existence for an entire week. The boys bonded with their cabin mates during that time. Once the two groups were allowed to have contact, they showed definite signs of prejudice and hostility toward each other even though they had only been given a very short time to develop their social group. To increase the conflict between the groups, the experimenters had them compete against each other in a series of activities. This created even more hostility and eventually the groups refused to eat in the same room. The final phase of the experiment involved turning the rival groups into friends. The fun activities the experimenters had planned like shooting firecrackers and watching movies did not initially work, so they created teamwork exercises where the two groups were forced to collaborate. At the end of the experiment, the boys decided to ride the same bus home, demonstrating that conflict can be resolved and prejudice overcome through cooperation.
Many critics have compared this study to Golding’s Lord of the Flies novel as a classic example of prejudice and conflict resolution.
14. Ross’ False Consensus Effect Study
Study conducted by: lee ross.
Study Conducted in 1977 at Stanford University
Experiment Details: In 1977, a social psychology professor at Stanford University named Lee Ross conducted an experiment that, in lay terms, focuses on how people can incorrectly conclude that others think the same way they do, or form a “false consensus” about the beliefs and preferences of others. Ross conducted the study in order to outline how the “false consensus effect” functions in humans.
Featured Programs
In the first part of the study, participants were asked to read about situations in which a conflict occurred and then were told two alternative ways of responding to the situation. They were asked to do three things:
- Guess which option other people would choose
- Say which option they themselves would choose
- Describe the attributes of the person who would likely choose each of the two options
What the study showed was that most of the subjects believed that other people would do the same as them, regardless of which of the two responses they actually chose themselves. This phenomenon is referred to as the false consensus effect, where an individual thinks that other people think the same way they do when they may not. The second observation coming from this important study is that when participants were asked to describe the attributes of the people who will likely make the choice opposite of their own, they made bold and sometimes negative predictions about the personalities of those who did not share their choice.
15. The Schacter and Singer Experiment on Emotion
Study conducted by: stanley schachter and jerome e. singer.
Study Conducted in 1962 at Columbia University
Experiment Details: In 1962 Schachter and Singer conducted a ground breaking experiment to prove their theory of emotion.
In the study, a group of 184 male participants were injected with epinephrine, a hormone that induces arousal including increased heartbeat, trembling, and rapid breathing. The research participants were told that they were being injected with a new medication to test their eyesight. The first group of participants was informed the possible side effects that the injection might cause while the second group of participants were not. The participants were then placed in a room with someone they thought was another participant, but was actually a confederate in the experiment. The confederate acted in one of two ways: euphoric or angry. Participants who had not been informed about the effects of the injection were more likely to feel either happier or angrier than those who had been informed.
What Schachter and Singer were trying to understand was the ways in which cognition or thoughts influence human emotion. Their study illustrates the importance of how people interpret their physiological states, which form an important component of your emotions. Though their cognitive theory of emotional arousal dominated the field for two decades, it has been criticized for two main reasons: the size of the effect seen in the experiment was not that significant and other researchers had difficulties repeating the experiment.
16. Selective Attention / Invisible Gorilla Experiment
Study conducted by: daniel simons and christopher chabris.
Study Conducted in 1999 at Harvard University
Experiment Details: In 1999 Simons and Chabris conducted their famous awareness test at Harvard University.
Participants in the study were asked to watch a video and count how many passes occurred between basketball players on the white team. The video moves at a moderate pace and keeping track of the passes is a relatively easy task. What most people fail to notice amidst their counting is that in the middle of the test, a man in a gorilla suit walked onto the court and stood in the center before walking off-screen.
The study found that the majority of the subjects did not notice the gorilla at all, proving that humans often overestimate their ability to effectively multi-task. What the study set out to prove is that when people are asked to attend to one task, they focus so strongly on that element that they may miss other important details.
17. Stanford Prison Study
Study conducted by philip zimbardo.
Study Conducted in 1971 at Stanford University

The Stanford Prison Experiment was designed to study behavior of “normal” individuals when assigned a role of prisoner or guard. College students were recruited to participate. They were assigned roles of “guard” or “inmate.” Zimbardo played the role of the warden. The basement of the psychology building was the set of the prison. Great care was taken to make it look and feel as realistic as possible.
The prison guards were told to run a prison for two weeks. They were told not to physically harm any of the inmates during the study. After a few days, the prison guards became very abusive verbally towards the inmates. Many of the prisoners became submissive to those in authority roles. The Stanford Prison Experiment inevitably had to be cancelled because some of the participants displayed troubling signs of breaking down mentally.
Although the experiment was conducted very unethically, many psychologists believe that the findings showed how much human behavior is situational. People will conform to certain roles if the conditions are right. The Stanford Prison Experiment remains one of the most famous psychology experiments of all time.
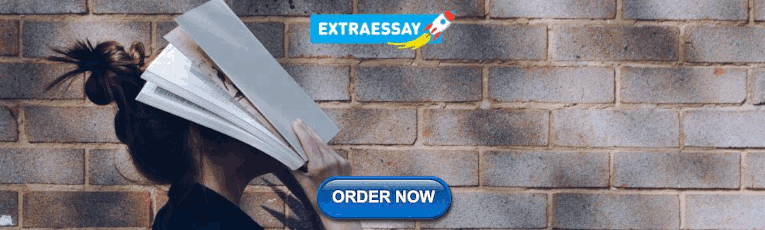
18. Stanley Milgram Experiment
Study conducted by stanley milgram.
Study Conducted in 1961 at Stanford University
Experiment Details: This 1961 study was conducted by Yale University psychologist Stanley Milgram. It was designed to measure people’s willingness to obey authority figures when instructed to perform acts that conflicted with their morals. The study was based on the premise that humans will inherently take direction from authority figures from very early in life.
Participants were told they were participating in a study on memory. They were asked to watch another person (an actor) do a memory test. They were instructed to press a button that gave an electric shock each time the person got a wrong answer. (The actor did not actually receive the shocks, but pretended they did).
Participants were told to play the role of “teacher” and administer electric shocks to “the learner,” every time they answered a question incorrectly. The experimenters asked the participants to keep increasing the shocks. Most of them obeyed even though the individual completing the memory test appeared to be in great pain. Despite these protests, many participants continued the experiment when the authority figure urged them to. They increased the voltage after each wrong answer until some eventually administered what would be lethal electric shocks.
This experiment showed that humans are conditioned to obey authority and will usually do so even if it goes against their natural morals or common sense.
19. Surrogate Mother Experiment
Study conducted by: harry harlow.
Study Conducted from 1957-1963 at the University of Wisconsin
Experiment Details: In a series of controversial experiments during the late 1950s and early 1960s, Harry Harlow studied the importance of a mother’s love for healthy childhood development.
In order to do this he separated infant rhesus monkeys from their mothers a few hours after birth and left them to be raised by two “surrogate mothers.” One of the surrogates was made of wire with an attached bottle for food. The other was made of soft terrycloth but lacked food. The researcher found that the baby monkeys spent much more time with the cloth mother than the wire mother, thereby proving that affection plays a greater role than sustenance when it comes to childhood development. They also found that the monkeys that spent more time cuddling the soft mother grew up to healthier.
This experiment showed that love, as demonstrated by physical body contact, is a more important aspect of the parent-child bond than the provision of basic needs. These findings also had implications in the attachment between fathers and their infants when the mother is the source of nourishment.
20. The Good Samaritan Experiment
Study conducted by: john darley and daniel batson.
Study Conducted in 1973 at The Princeton Theological Seminary (Researchers were from Princeton University)
Experiment Details: In 1973, an experiment was created by John Darley and Daniel Batson, to investigate the potential causes that underlie altruistic behavior. The researchers set out three hypotheses they wanted to test:
- People thinking about religion and higher principles would be no more inclined to show helping behavior than laymen.
- People in a rush would be much less likely to show helping behavior.
- People who are religious for personal gain would be less likely to help than people who are religious because they want to gain some spiritual and personal insights into the meaning of life.
Student participants were given some religious teaching and instruction. They were then were told to travel from one building to the next. Between the two buildings was a man lying injured and appearing to be in dire need of assistance. The first variable being tested was the degree of urgency impressed upon the subjects, with some being told not to rush and others being informed that speed was of the essence.
The results of the experiment were intriguing, with the haste of the subject proving to be the overriding factor. When the subject was in no hurry, nearly two-thirds of people stopped to lend assistance. When the subject was in a rush, this dropped to one in ten.
People who were on the way to deliver a speech about helping others were nearly twice as likely to help as those delivering other sermons,. This showed that the thoughts of the individual were a factor in determining helping behavior. Religious beliefs did not appear to make much difference on the results. Being religious for personal gain, or as part of a spiritual quest, did not appear to make much of an impact on the amount of helping behavior shown.
21. The Halo Effect Experiment
Study conducted by: richard e. nisbett and timothy decamp wilson.
Study Conducted in 1977 at the University of Michigan
Experiment Details: The Halo Effect states that people generally assume that people who are physically attractive are more likely to:
- be intelligent
- be friendly
- display good judgment
To prove their theory, Nisbett and DeCamp Wilson created a study to prove that people have little awareness of the nature of the Halo Effect. They’re not aware that it influences:
- their personal judgments
- the production of a more complex social behavior
In the experiment, college students were the research participants. They were asked to evaluate a psychology instructor as they view him in a videotaped interview. The students were randomly assigned to one of two groups. Each group was shown one of two different interviews with the same instructor. The instructor is a native French-speaking Belgian who spoke English with a noticeable accent. In the first video, the instructor presented himself as someone:
- respectful of his students’ intelligence and motives
- flexible in his approach to teaching
- enthusiastic about his subject matter
In the second interview, he presented himself as much more unlikable. He was cold and distrustful toward the students and was quite rigid in his teaching style.
After watching the videos, the subjects were asked to rate the lecturer on:
- physical appearance
His mannerisms and accent were kept the same in both versions of videos. The subjects were asked to rate the professor on an 8-point scale ranging from “like extremely” to “dislike extremely.” Subjects were also told that the researchers were interested in knowing “how much their liking for the teacher influenced the ratings they just made.” Other subjects were asked to identify how much the characteristics they just rated influenced their liking of the teacher.
After responding to the questionnaire, the respondents were puzzled about their reactions to the videotapes and to the questionnaire items. The students had no idea why they gave one lecturer higher ratings. Most said that how much they liked the lecturer had not affected their evaluation of his individual characteristics at all.
The interesting thing about this study is that people can understand the phenomenon, but they are unaware when it is occurring. Without realizing it, humans make judgments. Even when it is pointed out, they may still deny that it is a product of the halo effect phenomenon.
22. The Marshmallow Test
Study conducted by: walter mischel.
Study Conducted in 1972 at Stanford University

In his 1972 Marshmallow Experiment, children ages four to six were taken into a room where a marshmallow was placed in front of them on a table. Before leaving each of the children alone in the room, the experimenter informed them that they would receive a second marshmallow if the first one was still on the table after they returned in 15 minutes. The examiner recorded how long each child resisted eating the marshmallow and noted whether it correlated with the child’s success in adulthood. A small number of the 600 children ate the marshmallow immediately and one-third delayed gratification long enough to receive the second marshmallow.
In follow-up studies, Mischel found that those who deferred gratification were significantly more competent and received higher SAT scores than their peers. This characteristic likely remains with a person for life. While this study seems simplistic, the findings outline some of the foundational differences in individual traits that can predict success.
23. The Monster Study
Study conducted by: wendell johnson.
Study Conducted in 1939 at the University of Iowa
Experiment Details: The Monster Study received this negative title due to the unethical methods that were used to determine the effects of positive and negative speech therapy on children.
Wendell Johnson of the University of Iowa selected 22 orphaned children, some with stutters and some without. The children were in two groups. The group of children with stutters was placed in positive speech therapy, where they were praised for their fluency. The non-stutterers were placed in negative speech therapy, where they were disparaged for every mistake in grammar that they made.
As a result of the experiment, some of the children who received negative speech therapy suffered psychological effects and retained speech problems for the rest of their lives. They were examples of the significance of positive reinforcement in education.
The initial goal of the study was to investigate positive and negative speech therapy. However, the implication spanned much further into methods of teaching for young children.
24. Violinist at the Metro Experiment
Study conducted by: staff at the washington post.
Study Conducted in 2007 at a Washington D.C. Metro Train Station

During the study, pedestrians rushed by without realizing that the musician playing at the entrance to the metro stop was Grammy-winning musician, Joshua Bell. Two days before playing in the subway, he sold out at a theater in Boston where the seats average $100. He played one of the most intricate pieces ever written with a violin worth 3.5 million dollars. In the 45 minutes the musician played his violin, only 6 people stopped and stayed for a while. Around 20 gave him money, but continued to walk their normal pace. He collected $32.
The study and the subsequent article organized by the Washington Post was part of a social experiment looking at:
- the priorities of people
Gene Weingarten wrote about the social experiment: “In a banal setting at an inconvenient time, would beauty transcend?” Later he won a Pulitzer Prize for his story. Some of the questions the article addresses are:
- Do we perceive beauty?
- Do we stop to appreciate it?
- Do we recognize the talent in an unexpected context?
As it turns out, many of us are not nearly as perceptive to our environment as we might like to think.
25. Visual Cliff Experiment
Study conducted by: eleanor gibson and richard walk.
Study Conducted in 1959 at Cornell University
Experiment Details: In 1959, psychologists Eleanor Gibson and Richard Walk set out to study depth perception in infants. They wanted to know if depth perception is a learned behavior or if it is something that we are born with. To study this, Gibson and Walk conducted the visual cliff experiment.
They studied 36 infants between the ages of six and 14 months, all of whom could crawl. The infants were placed one at a time on a visual cliff. A visual cliff was created using a large glass table that was raised about a foot off the floor. Half of the glass table had a checker pattern underneath in order to create the appearance of a ‘shallow side.’
In order to create a ‘deep side,’ a checker pattern was created on the floor; this side is the visual cliff. The placement of the checker pattern on the floor creates the illusion of a sudden drop-off. Researchers placed a foot-wide centerboard between the shallow side and the deep side. Gibson and Walk found the following:
- Nine of the infants did not move off the centerboard.
- All of the 27 infants who did move crossed into the shallow side when their mothers called them from the shallow side.
- Three of the infants crawled off the visual cliff toward their mother when called from the deep side.
- When called from the deep side, the remaining 24 children either crawled to the shallow side or cried because they could not cross the visual cliff and make it to their mother.
What this study helped demonstrate is that depth perception is likely an inborn train in humans.
Among these experiments and psychological tests, we see boundaries pushed and theories taking on a life of their own. It is through the endless stream of psychological experimentation that we can see simple hypotheses become guiding theories for those in this field. The greater field of psychology became a formal field of experimental study in 1879, when Wilhelm Wundt established the first laboratory dedicated solely to psychological research in Leipzig, Germany. Wundt was the first person to refer to himself as a psychologist. Since 1879, psychology has grown into a massive collection of:
- methods of practice
It’s also a specialty area in the field of healthcare. None of this would have been possible without these and many other important psychological experiments that have stood the test of time.
- 20 Most Unethical Experiments in Psychology
- What Careers are in Experimental Psychology?
- 10 Things to Know About the Psychology of Psychotherapy
About Education: Psychology
Explorable.com
Mental Floss.com
About the Author
After earning a Bachelor of Arts in Psychology from Rutgers University and then a Master of Science in Clinical and Forensic Psychology from Drexel University, Kristen began a career as a therapist at two prisons in Philadelphia. At the same time she volunteered as a rape crisis counselor, also in Philadelphia. After a few years in the field she accepted a teaching position at a local college where she currently teaches online psychology courses. Kristen began writing in college and still enjoys her work as a writer, editor, professor and mother.
- 5 Best Online Ph.D. Marriage and Family Counseling Programs
- Top 5 Online Doctorate in Educational Psychology
- 5 Best Online Ph.D. in Industrial and Organizational Psychology Programs
- Top 10 Online Master’s in Forensic Psychology
- 10 Most Affordable Counseling Psychology Online Programs
- 10 Most Affordable Online Industrial Organizational Psychology Programs
- 10 Most Affordable Online Developmental Psychology Online Programs
- 15 Most Affordable Online Sport Psychology Programs
- 10 Most Affordable School Psychology Online Degree Programs
- Top 50 Online Psychology Master’s Degree Programs
- Top 25 Online Master’s in Educational Psychology
- Top 25 Online Master’s in Industrial/Organizational Psychology
- Top 10 Most Affordable Online Master’s in Clinical Psychology Degree Programs
- Top 6 Most Affordable Online PhD/PsyD Programs in Clinical Psychology
- 50 Great Small Colleges for a Bachelor’s in Psychology
- 50 Most Innovative University Psychology Departments
- The 30 Most Influential Cognitive Psychologists Alive Today
- Top 30 Affordable Online Psychology Degree Programs
- 30 Most Influential Neuroscientists
- Top 40 Websites for Psychology Students and Professionals
- Top 30 Psychology Blogs
- 25 Celebrities With Animal Phobias
- Your Phobias Illustrated (Infographic)
- 15 Inspiring TED Talks on Overcoming Challenges
- 10 Fascinating Facts About the Psychology of Color
- 15 Scariest Mental Disorders of All Time
- 15 Things to Know About Mental Disorders in Animals
- 13 Most Deranged Serial Killers of All Time

Site Information
- About Online Psychology Degree Guide

An official website of the United States government
The .gov means it’s official. Federal government websites often end in .gov or .mil. Before sharing sensitive information, make sure you’re on a federal government site.
The site is secure. The https:// ensures that you are connecting to the official website and that any information you provide is encrypted and transmitted securely.
- Publications
- Account settings
Preview improvements coming to the PMC website in October 2024. Learn More or Try it out now .
- Advanced Search
- Journal List
- J Athl Train
- v.45(1); Jan-Feb 2010
Study/Experimental/Research Design: Much More Than Statistics
Kenneth l. knight.
Brigham Young University, Provo, UT
The purpose of study, experimental, or research design in scientific manuscripts has changed significantly over the years. It has evolved from an explanation of the design of the experiment (ie, data gathering or acquisition) to an explanation of the statistical analysis. This practice makes “Methods” sections hard to read and understand.
To clarify the difference between study design and statistical analysis, to show the advantages of a properly written study design on article comprehension, and to encourage authors to correctly describe study designs.
Description:
The role of study design is explored from the introduction of the concept by Fisher through modern-day scientists and the AMA Manual of Style . At one time, when experiments were simpler, the study design and statistical design were identical or very similar. With the complex research that is common today, which often includes manipulating variables to create new variables and the multiple (and different) analyses of a single data set, data collection is very different than statistical design. Thus, both a study design and a statistical design are necessary.
Advantages:
Scientific manuscripts will be much easier to read and comprehend. A proper experimental design serves as a road map to the study methods, helping readers to understand more clearly how the data were obtained and, therefore, assisting them in properly analyzing the results.
Study, experimental, or research design is the backbone of good research. It directs the experiment by orchestrating data collection, defines the statistical analysis of the resultant data, and guides the interpretation of the results. When properly described in the written report of the experiment, it serves as a road map to readers, 1 helping them negotiate the “Methods” section, and, thus, it improves the clarity of communication between authors and readers.
A growing trend is to equate study design with only the statistical analysis of the data. The design statement typically is placed at the end of the “Methods” section as a subsection called “Experimental Design” or as part of a subsection called “Data Analysis.” This placement, however, equates experimental design and statistical analysis, minimizing the effect of experimental design on the planning and reporting of an experiment. This linkage is inappropriate, because some of the elements of the study design that should be described at the beginning of the “Methods” section are instead placed in the “Statistical Analysis” section or, worse, are absent from the manuscript entirely.
Have you ever interrupted your reading of the “Methods” to sketch out the variables in the margins of the paper as you attempt to understand how they all fit together? Or have you jumped back and forth from the early paragraphs of the “Methods” section to the “Statistics” section to try to understand which variables were collected and when? These efforts would be unnecessary if a road map at the beginning of the “Methods” section outlined how the independent variables were related, which dependent variables were measured, and when they were measured. When they were measured is especially important if the variables used in the statistical analysis were a subset of the measured variables or were computed from measured variables (such as change scores).
The purpose of this Communications article is to clarify the purpose and placement of study design elements in an experimental manuscript. Adopting these ideas may improve your science and surely will enhance the communication of that science. These ideas will make experimental manuscripts easier to read and understand and, therefore, will allow them to become part of readers' clinical decision making.
WHAT IS A STUDY (OR EXPERIMENTAL OR RESEARCH) DESIGN?
The terms study design, experimental design, and research design are often thought to be synonymous and are sometimes used interchangeably in a single paper. Avoid doing so. Use the term that is preferred by the style manual of the journal for which you are writing. Study design is the preferred term in the AMA Manual of Style , 2 so I will use it here.
A study design is the architecture of an experimental study 3 and a description of how the study was conducted, 4 including all elements of how the data were obtained. 5 The study design should be the first subsection of the “Methods” section in an experimental manuscript (see the Table ). “Statistical Design” or, preferably, “Statistical Analysis” or “Data Analysis” should be the last subsection of the “Methods” section.
Table. Elements of a “Methods” Section

The “Study Design” subsection describes how the variables and participants interacted. It begins with a general statement of how the study was conducted (eg, crossover trials, parallel, or observational study). 2 The second element, which usually begins with the second sentence, details the number of independent variables or factors, the levels of each variable, and their names. A shorthand way of doing so is with a statement such as “A 2 × 4 × 8 factorial guided data collection.” This tells us that there were 3 independent variables (factors), with 2 levels of the first factor, 4 levels of the second factor, and 8 levels of the third factor. Following is a sentence that names the levels of each factor: for example, “The independent variables were sex (male or female), training program (eg, walking, running, weight lifting, or plyometrics), and time (2, 4, 6, 8, 10, 15, 20, or 30 weeks).” Such an approach clearly outlines for readers how the various procedures fit into the overall structure and, therefore, enhances their understanding of how the data were collected. Thus, the design statement is a road map of the methods.
The dependent (or measurement or outcome) variables are then named. Details of how they were measured are not given at this point in the manuscript but are explained later in the “Instruments” and “Procedures” subsections.
Next is a paragraph detailing who the participants were and how they were selected, placed into groups, and assigned to a particular treatment order, if the experiment was a repeated-measures design. And although not a part of the design per se, a statement about obtaining written informed consent from participants and institutional review board approval is usually included in this subsection.
The nuts and bolts of the “Methods” section follow, including such things as equipment, materials, protocols, etc. These are beyond the scope of this commentary, however, and so will not be discussed.
The last part of the “Methods” section and last part of the “Study Design” section is the “Data Analysis” subsection. It begins with an explanation of any data manipulation, such as how data were combined or how new variables (eg, ratios or differences between collected variables) were calculated. Next, readers are told of the statistical measures used to analyze the data, such as a mixed 2 × 4 × 8 analysis of variance (ANOVA) with 2 between-groups factors (sex and training program) and 1 within-groups factor (time of measurement). Researchers should state and reference the statistical package and procedure(s) within the package used to compute the statistics. (Various statistical packages perform analyses slightly differently, so it is important to know the package and specific procedure used.) This detail allows readers to judge the appropriateness of the statistical measures and the conclusions drawn from the data.
STATISTICAL DESIGN VERSUS STATISTICAL ANALYSIS
Avoid using the term statistical design . Statistical methods are only part of the overall design. The term gives too much emphasis to the statistics, which are important, but only one of many tools used in interpreting data and only part of the study design:
The most important issues in biostatistics are not expressed with statistical procedures. The issues are inherently scientific, rather than purely statistical, and relate to the architectural design of the research, not the numbers with which the data are cited and interpreted. 6
Stated another way, “The justification for the analysis lies not in the data collected but in the manner in which the data were collected.” 3 “Without the solid foundation of a good design, the edifice of statistical analysis is unsafe.” 7 (pp4–5)
The intertwining of study design and statistical analysis may have been caused (unintentionally) by R.A. Fisher, “… a genius who almost single-handedly created the foundations for modern statistical science.” 8 Most research did not involve statistics until Fisher invented the concepts and procedures of ANOVA (in 1921) 9 , 10 and experimental design (in 1935). 11 His books became standard references for scientists in many disciplines. As a result, many ANOVA books were titled Experimental Design (see, for example, Edwards 12 ), and ANOVA courses taught in psychology and education departments included the words experimental design in their course titles.
Before the widespread use of computers to analyze data, designs were much simpler, and often there was little difference between study design and statistical analysis. So combining the 2 elements did not cause serious problems. This is no longer true, however, for 3 reasons: (1) Research studies are becoming more complex, with multiple independent and dependent variables. The procedures sections of these complex studies can be difficult to understand if your only reference point is the statistical analysis and design. (2) Dependent variables are frequently measured at different times. (3) How the data were collected is often not directly correlated with the statistical design.
For example, assume the goal is to determine the strength gain in novice and experienced athletes as a result of 3 strength training programs. Rate of change in strength is not a measurable variable; rather, it is calculated from strength measurements taken at various time intervals during the training. So the study design would be a 2 × 2 × 3 factorial with independent variables of time (pretest or posttest), experience (novice or advanced), and training (isokinetic, isotonic, or isometric) and a dependent variable of strength. The statistical design , however, would be a 2 × 3 factorial with independent variables of experience (novice or advanced) and training (isokinetic, isotonic, or isometric) and a dependent variable of strength gain. Note that data were collected according to a 3-factor design but were analyzed according to a 2-factor design and that the dependent variables were different. So a single design statement, usually a statistical design statement, would not communicate which data were collected or how. Readers would be left to figure out on their own how the data were collected.
MULTIVARIATE RESEARCH AND THE NEED FOR STUDY DESIGNS
With the advent of electronic data gathering and computerized data handling and analysis, research projects have increased in complexity. Many projects involve multiple dependent variables measured at different times, and, therefore, multiple design statements may be needed for both data collection and statistical analysis. Consider, for example, a study of the effects of heat and cold on neural inhibition. The variables of H max and M max are measured 3 times each: before, immediately after, and 30 minutes after a 20-minute treatment with heat or cold. Muscle temperature might be measured each minute before, during, and after the treatment. Although the minute-by-minute data are important for graphing temperature fluctuations during the procedure, only 3 temperatures (time 0, time 20, and time 50) are used for statistical analysis. A single dependent variable H max :M max ratio is computed to illustrate neural inhibition. Again, a single statistical design statement would tell little about how the data were obtained. And in this example, separate design statements would be needed for temperature measurement and H max :M max measurements.
As stated earlier, drawing conclusions from the data depends more on how the data were measured than on how they were analyzed. 3 , 6 , 7 , 13 So a single study design statement (or multiple such statements) at the beginning of the “Methods” section acts as a road map to the study and, thus, increases scientists' and readers' comprehension of how the experiment was conducted (ie, how the data were collected). Appropriate study design statements also increase the accuracy of conclusions drawn from the study.
CONCLUSIONS
The goal of scientific writing, or any writing, for that matter, is to communicate information. Including 2 design statements or subsections in scientific papers—one to explain how the data were collected and another to explain how they were statistically analyzed—will improve the clarity of communication and bring praise from readers. To summarize:
- Purge from your thoughts and vocabulary the idea that experimental design and statistical design are synonymous.
- Study or experimental design plays a much broader role than simply defining and directing the statistical analysis of an experiment.
- A properly written study design serves as a road map to the “Methods” section of an experiment and, therefore, improves communication with the reader.
- Study design should include a description of the type of design used, each factor (and each level) involved in the experiment, and the time at which each measurement was made.
- Clarify when the variables involved in data collection and data analysis are different, such as when data analysis involves only a subset of a collected variable or a resultant variable from the mathematical manipulation of 2 or more collected variables.
Acknowledgments
Thanks to Thomas A. Cappaert, PhD, ATC, CSCS, CSE, for suggesting the link between R.A. Fisher and the melding of the concepts of research design and statistics.
Click through the PLOS taxonomy to find articles in your field.
For more information about PLOS Subject Areas, click here .
Loading metrics
Open Access
Peer-reviewed
Research Article
Experimental induction of state rumination: A study evaluating the efficacy of goal-cueing task in different experimental settings
Roles Conceptualization, Formal analysis, Investigation, Project administration, Writing – original draft
* E-mail: [email protected]
Affiliation Department of Clinical Psychology and Neuropsychology, Institute for Psychology, Johannes Gutenberg-University Mainz, Mainz, Germany

Roles Writing – review & editing
Affiliations Department of Clinical Psychology and Neuropsychology, Institute for Psychology, Johannes Gutenberg-University Mainz, Mainz, Germany, Leibniz Institute for Resilience Research (LIR), Mainz, Germany
Roles Conceptualization, Writing – review & editing
- Alena Michel-Kröhler,
- Michèle Wessa,
- Stefan Berti
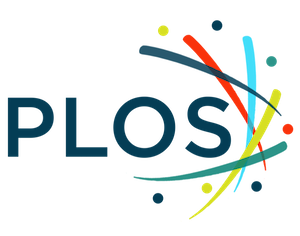
- Published: November 22, 2023
- https://doi.org/10.1371/journal.pone.0288450
- Reader Comments
Based on previous studies, the present four experiments (total N = 468) aimed at investigating the effectivity of rumination induction in different experimental settings. We were particularly interested in rumination in the context of individual goal achievement and tested whether an instruction that referred to unresolved goals had a direct observable effect on state rumination. For this purpose, participants were asked to identify, evaluate, and focus on a personally relevant goal that was previously unresolved and still bothered them. In Experiment 1a to 1c, we compared three different modifications of the unresolved condition with shortened instructions with the elaborated unresolved condition and an additional control condition that did not refer to goals. In general, the results were mixed, but basically confirmed the effectiveness of the method used. Finally, in Experiment 2, we compared the two most promising versions of the unresolved condition and, by adding a goal-related control condition, we examined which control condition was best suited to maximize effects related to state rumination in future research. Results of various mixed ANOVAs demonstrated that a shortened version (in terms of shortened audio instructions) of the unresolved condition could be used as well as the original unresolved condition to induce reliable state rumination. The significance of the effects obtained with this method for real-life applications as well as approaches for future research are discussed.
Citation: Michel-Kröhler A, Wessa M, Berti S (2023) Experimental induction of state rumination: A study evaluating the efficacy of goal-cueing task in different experimental settings. PLoS ONE 18(11): e0288450. https://doi.org/10.1371/journal.pone.0288450
Editor: Ricky Siu Wong, University of Hertfordshire, UNITED KINGDOM
Received: October 17, 2022; Accepted: June 27, 2023; Published: November 22, 2023
Copyright: © 2023 Michel-Kröhler et al. This is an open access article distributed under the terms of the Creative Commons Attribution License , which permits unrestricted use, distribution, and reproduction in any medium, provided the original author and source are credited.
Data Availability: The data and materials for all experiments are available at https://osf.io/h7zng/?view_only=e3cd29d81b9a4fdeb1a3210d80e69a88 .
Funding: This work was partly supported (Experiment 1c and Experiment 2) by the Deutsche Forschungsgemeinschaft (DFG, German Research Foundation; https://www.dfg.de/ ) awarded to AMK – 454608048 The funders had no role in study design, data collection and analysis, decision to publish, or preparation of the manuscript.
Competing interests: The authors have declared that no competing interests exist.
Introduction
Rumination, defined as repetitive, intrusive thoughts, can affect different cognitive processes, and has the potential to impair performance, psychological wellbeing, or mental health [ 1 , 2 ]. Despite increasing research in this area, specific mechanisms underlying these negative effects are not completely understood. One way to gain detailed insight into the effects of rumination as well as the mechanisms that cause these effects, is to induce ruminative thoughts within an experimental design so that a direct measurement of the potential effects of rumination on selected outcome variables is possible. However, to the best of our knowledge evaluation of rumination induction tasks outside of a clinical context is lacking. Here, we report results of four experiments in which we aimed at evaluating the effectivity of a procedure to induce rumination (namely, the goal-cueing task [ 3 ]) in different experimental settings, with the goal of enabling its application in and outside the laboratory. In contrast to other approaches, we focus on a non-clinical setting because the overall aim of the study is to establish an effective procedure for rumination induction within a broad range of rumination research. In the following, we will first describe the theoretical background of the goal-cueing task to motivate the application of this specific procedure. Second, we will give an overview of the four experiments performed within this study.
Relevance of goals in our daily lives and the Goal-Progress Theory
Goals structure our daily actions and serve as the main motivation for pursuing our careers, our studies, our leisure activities such as sports, and various other activities in our private lives. In doing so, we try to pursue various goals from different areas at the same time ("providing a good presentation", "keeping physically fit", "being a good friend", etc.) [ 4 ]. However, what happens when we fail to achieve a goal that we really want to achieve, or when we continue to hold on to a goal even though we seem not capable of achieving it [ 5 ]? A discrepancy occurs that implies dissatisfaction with the current state and a desire to achieve a goal or specific outcome [ 6 ]. As a result, negative thoughts and feelings can arise that revolve around the failed goal achievement, and if persistent and recurring, can lead to rumination [ 5 ].
The Goal Progress Theory (GPT) by Martin and Tesser [ 4 ] provides a detailed account of goal discrepancies as a central mechanism of rumination. GPT states that people’s thoughts and actions are guided by individual, conscious or unconscious goals, which thus play an important role in the self-regulation of behavior [ 7 ]. In addition, a lack of progress (more or less) toward an individual goal is likely to trigger rumination, which persists until the discrepancy is resolved either by restoring goal progress or by moving away from the goal [ 4 ]. It is worth noting that rumination does not necessarily have to be dysfunctional. It can also be adaptive and functional in certain circumstances to highlight unresolved issues and then address them as progress is made in reducing the discrepancy [ 1 , 8 ]. However, rumination often does not help to reduce goal discrepancy or to disengage from the goal even when there is no prospect of success. This is especially the case when individuals are unable to reprioritize or identify alternative ways to achieve the goal [ 4 ]. Thus, rumination can be seen mainly as an unsuccessful problem-solving attempt that is unhelpful and causes a persistent goal discrepancy [ 1 , 4 ]. Nevertheless, individuals continue to ruminate in some situations, believing that this gives them an advantage or brings them closer to their goal [ 9 – 11 ]. Therefore, first, it is important to understand the thoughts associated with the individual goals and how they affect individuals’ well-being and daily performance. Second, it is advantageous if individuals adopt a proactive attitude after setbacks or failures and sustain capabilities that contribute to the improvement of the situation instead of remaining in the situation that has occurred [ 12 – 14 ].
To date, some studies have linked problematic goal attainment with rumination: For instance, results of an experience-sampling study [ 15 ] revealed that low goal success and high goal importance was associated with high levels of negative affect, and that this interaction was marginally significant for ruminative self-focus. In addition, Huffziger and colleagues [ 16 , 17 ] demonstrated that induced rumination in an everyday life application immediately deteriorated mood-related valence and calmness and was linked to stronger reductions in positive mood. Moreover, Krys [ 18 ] showed in a two-week diary study that rumination exerted a negative indirect effect on subjective problem solving via perceived stress and negative mood. In contrast, results of another study [ 8 ] demonstrated that goal-directed rumination has positive effects on academic performance if negative effects of psychological distress were simultaneously accounted for. However, goal-directed rumination per se was not related to academic performance [ 8 ]. Finally, one study from the context of sports [ 19 ] showed that athletes who fulfilled their goals at the end of a competitive season had lower rumination scores compared with athletes who did not. Overall, the results of the studies indicate that rumination can play a relevant role in different contexts during the individual goal achievement process. Moreover, these findings also suggest that goals, more precisely, the perceived goal discrepancy, can serve as a trigger for rumination especially if they are related to failure.
Rumination and the goal-cueing task
Rumination and its consequences have attracted increasing theoretical and empirical interest in the past 30 years [ 20 , 21 ]. In this research process, rumination has been examined both as a habitual tendency toward repetitive and passive self-focus in response to depressed mood [ 1 , 22 ] and as a temporary cognitive response that is highly dependent on situational cues [ 4 , 20 , 23 , 24 ]. In this context, numerous scales have been developed to capture rumination as a trait (for an overview see Krys [ 25 ]), including the Rumination-Reflection Questionnaire (RRQ; [ 26 ]) and the Perseverative Thinking Questionnaire (PTQ; [ 27 ]). In contrast, there is no “gold standard” for measuring state rumination [ 23 ]. Numerous studies have used various state rumination measures with different psychometric properties and levels of validity, among which the Brief State Rumination Inventory (BSRI) can be considered the most established [ 23 ]. In addition, however, various experimental approaches have been developed with the aim of examining ruminative thoughts within an experimental study so that a direct measurement of the potential effects of rumination on selected outcome variables is possible [e.g., 28–31]. An effective possibility of inducing rumination is the response manipulation task, which was designed to influence the contents of participants’ thoughts by forcing them to focus their attention on emotion-, symptom- and self-focused thoughts (cf. [ 31 ], e.g.: “think about how active/passive you feel” or “think about what your feelings might mean”). The response manipulation task is well established, but limits rumination to a specific subset of thoughts related to mental health. A potential alternative to induce ruminative thoughts, which might be more common in everyday life, is the so-called goal-cueing task by Roberts and colleagues [ 3 ]. This task does not restrict or pre-define the area of ruminative thoughts by cueing specific feelings and personal attributes related to rumination. Instead, it focuses on concerns or problems during the individual goal achievement process. In this context, participants are free to choose the content so that a broader range of thoughts can be addressed, which occur more naturally in everyday situations. Ruminating can refer, for example, to a certain negative event and its influence on how the person has felt in recent weeks. It can also be thoughts about the fact that the comparison to other people in relation to a personal important aspect is not positive or one has the feeling to disappoint a person.
The goal-cueing task developed by Roberts and colleagues [ 3 , 32 ] consists of three steps, namely (1) identification of a problem in the individual goal achievement process, (2) evaluation of the identified problem, and (3) 10-minute goal focus period, which is predicted to elicit rumination. In addition, the task consists of two conditions, a resolved and an unresolved goal condition. In the unresolved goal condition, participants are instructed to identify an ongoing and unresolved concern in their goal achievement process that repeatedly comes into their mind and caused them to feel negative or stressed during the previous week. In contrast, participants in the resolved goal condition are asked to identify a concern in their goal achievement process that had previously troubled them, but that had since been resolved. Thus, the two conditions of the goal-cueing task were designed to directly contrast the impact of self-focus on resolved and unresolved goals, thereby manipulating self-discrepancy and rumination [ 33 ]. However, there are only a few studies applying this procedure and systematic evaluation is still lacking. Following the original study by Roberts and colleagues [ 3 ], Lanning [ 33 ] applied the procedure in the context of research on the influence on general memory, and Edwards [ 34 ] investigated whether an approach or avoidance framing influences rumination cued by unresolved goals. Kornacka and colleagues [ 28 ] used the unresolved condition of the goal-cueing task to activate rumination in their participants before investigating the experimental induction of abstract versus concrete repetitive negative thoughts and their influence on emotional reactivity and attentional disengagement. Roberts et al. [ 32 ] found that cueing an unresolved goal triggered more spontaneous rumination compared to cueing a resolved goal. Importantly, in the studies by Roberts et al. [ 3 , 32 ] and Edwards [ 34 ], state rumination was not assessed in a direct way (i.e., by means of a validated questionnaire. In contrast, state rumination was measured with probes of which one probe represented the identified concern (i.e., the resolved or unresolved problem) as index of state rumination (for more details regarding probe approach see also Kane et al. [ 35 ]). Further, Roberts et al. [ 3 , 32 ] (see also Edwards [ 34 ]) applied an additional outcome measure (SART: Sustained Attention to response task [ 36 ]) to test potential effects of rumination on performance in an unrelated cognitive task, assessing attentional lapses due to minimal demands on control processes. Results were mixed, as condition differences in task performance were found in one study [ 3 ]), while no differences were obtained in two other studies [ 32 , 34 ].
In summary, one of the advantages of the goal-cueing task is that it deals with unresolved goals in the individual goal achievement process, and because people consciously and unconsciously pursue multiple goals daily, the likelihood is very high that discrepancies will be perceived, which can lead to rumination. On the one hand, this suggests that the individual goal achievement process can be considered a reliable indicator of rumination. On the other hand, the reference to goals creates a more natural setting (compared to the response task), which produces more personally relevant reasons to ruminate [ 15 ]). Finally, referring to individual goals may increase participants’ commitment to an experiment as well as personal relevance compared to clinical settings. Overall, the goal-cueing task is promising in its application and theoretically justified, but direct evidence for inducing state rumination is lacking.
Overview of Experiments 1a-c
The overall goal of the following three experiments is to examine the effectiveness of the goal-cueing task in different experimental settings. For this, we used different measures of state rumination covering different facets of rumination: First, we applied the BSRI, to capture the momentary occurrence of thoughts that focus one’s attention on one’s distress along with its possible causes and implications [ 23 ]. Second, we used a single rumination item according to Koval et al. [ 37 ], to measure general state rumination and third, we utilized the index of ruminative self-focus by Moberly and Watkins [ 14 ], which involves the focus on feelings as well as the focus on problems that matches discrepancy-based accounts.
Moreover, following the GPT and findings of previous studies [ 3 , 32 ], we focused exclusively on unresolved goals in the individual goal-achievement process to induce state rumination in participants and evaluate to what extent this condition can be modified for application in laboratory or field research. The effectivity of these modifications (i.e., shortenings of the elaborated unresolved condition to different degrees) is compared with the elaborated unresolved condition according to Roberts et al. [ 3 ] and with an additional neutral control condition in Experiments 1b-c. Specifically, we were interested in whether the use of the goal-cueing task had a direct observable effect on state rumination as assessed by different measures. In addition, we tested whether the effectivity of the goal-cueing task in inducing state rumination remains stable when the experimental condition is shortened. Therefore, in Experiment 1a, we compared the elaborated unresolved condition (i.e., 3 steps of the goal-cueing task) with an experimental condition in which step 3 (goal focus period) was omitted. Based on the results, in Experiment 1b we further shortened the experimental condition (including only step 1 of the goal-cueing task) and compared it to the elaborated unresolved condition and to a neutral control condition that was not related to personal goals. In Experiment 1c, we then reduced the goal focus period of the elaborated unresolved condition from 10 min to 5 min and compared this again with the shortened experimental condition from Experiment 1a (i.e., step 3 –goal focus period was omitted) and the neutral control condition already mentioned. We assessed further variables, using the same approach, to account for emotional processes in addition to cognitive processes (for more details see Material & Measures for each study).
Shortening the elaborated unresolved condition would have the advantage of (1) reducing the mental stress on participants, (2) allowing for application in a variety of contexts including performance contexts (in sports, in the academic or medical domain), where time economy plays an important role, and (3) future application in the field or in experimental ambulatory assessment studies.
Experiment 1a
In the first experiment, we examined the efficacy of a shortened unresolved condition (referred to as experimental condition 2; EC2) compared to the elaborated unresolved condition (referred to as experimental condition 1; EC1) to provide information for further application. Therefore, we modified the unresolved condition so that we only applied the first two steps of the goal-cueing task (goal identification and goal evaluation) and checked whether different modifications in the unresolved condition led to same outcomes in terms of state-rumination.
Participants.
In our Experiment 1a, 164 participants (female: n = 101; male: n = 61) completed an online session. The mean age was 28.68 ( SD = 13.11). Most of the participants were students with different subjects (n = 94), followed by 34 employers and 12 officers. Twenty-four of the participants had other employment relationships. Table 1 presents an overview of sample characteristics separated by condition. Moreover, participants in the two conditions did not significantly differ in their level of trait rumination, t (161.57) = -0.54, p = .59, d = 0.08.
- PPT PowerPoint slide
- PNG larger image
- TIFF original image
https://doi.org/10.1371/journal.pone.0288450.t001
Participants were invited to participate in the experiments via institutes’ student mailing list, student contacts, and notices on the bulletin board. The experiment consisted of two parts that both were completed online at home due to the ongoing pandemic at the time of experiment. First, participants completed an initial online survey and then completed the experimental part second.
The initial survey was conducted using SoSci Survey [ 38 ] and comprised biographical and sociodemographic questions as well as different personality questionnaires (see below for detailed descriptions of the utilized questionnaires). The questionnaire data was also the basis for a stratified randomization of the participants. In detail, we first grouped participants according to their level of trait rumination (measured with the Perseverative Thinking Questionnaire; PTQ, [ 27 ]) and their gender, and second randomly assigned them to one of the two experimental conditions (or to a neutral control condition in Experiment 1b and 1c). We thus ensured that the participants in the different conditions did not differ significantly from each other in these characteristics at the group level.
The study protocol was approved by the local Review Board of the Institute for Psychology of the Johannes Gutenberg-University Mainz and was conducted according to the guidelines of the Declaration of Helsinki. Participants were informed about the nature and the procedure of the experiment and gave consent before completing the questionnaires. Participation in this experiment was voluntary. Psychology students received course credits for participating. This procedure also corresponds to that of the following two experiments, unless otherwise stated.
Advantage and disadvantage of applying an online experiment in our study.
On the one hand, an online experiment has some advantages, such as facilitation regarding sample size, flexibility of data collection, and time economy. On the other hand, the main disadvantages of online experiments include the difficulty to control the situation in which participants complete the task and thus the standardization of data collection, as well as the more difficult protection of the participants from stress exposure [ 39 ]. Therefore, we took a few steps to increase the quality of the implementation of the online experiments and to ensure the protection of participants from negative emotional reactions or stress.
First, even though in previous experiments in our department, there were no negative emotional reactions of the participants after the experimental setting, potential negative reactions cannot be detected in the online experiments due to the lack of interaction. Consequently, before participants had the possibility to complete the initial survey, we conducted a screening in advance to exclude vulnerable individuals from the experiment. Exclusion criteria were an experienced traumatic event in the past, an actual diagnosed mental disorder, or a current psychotherapeutic treatment. In addition, we provided our contact details on several occasions, enabling participants to contact us if they felt unwell or found themselves in a bad emotional state.
Second, to increase the understanding of important information and the procedure of the experiment, as well as to reduce the number of confounding variables, participants received detailed instructions on how to conduct the experiment prior to their participation. We instructed the participants to find a quiet place for the duration of the experiment where they would be undisturbed and not distracted by friends or family, the radio, television, or their smartphone. Participants were advised to switch off their smartphone or put it in airplane mode and find a place where they could be themselves and did not have to leave for 60 minutes.
Third, to get an idea about the quality of performance in the online experiment, we asked participants at the end of the experiment (1) how focused they were, (2) how seriously they tried to implement the instructions on how to perform the experiment, and (3) how well they succeeded. Participants answered these three questions on a 5-point scale ranging from ‘1’ ( not at all ) to ‘5’ ( very ). Participants indicated that their concentration was 3.79 ( SD = 1.00), on average they tried to follow the instructions seriously with 4.51 ( SD = 0.70), and on average they succeeded with a mean of 4.06 ( SD = 0.93) on the 5-point scale. Furthermore, participants in the two conditions showed no significant differences in the three quality measures (all p wilcox ‘s > .05).
Measures & material.
We used the German versions of the following questionnaires. Unless otherwise stated, these questionnaires were used in all four experiments. Means, standard deviations, 95% confidence intervals as well as Cronbach’s alpha for the present sample are summarized in Table 1 .
Perseverative thinking . The Perseverative Thinking Questionnaire (PTQ; [ 27 ]) is a content-independent self-report questionnaire of repetitive negative thoughts. The PTQ consists of 15 items (e.g., “Thoughts come to my mind without me wanting them to”) and is rated on a 5-point scale, ranging from ‘0’ ( never ) to ‘4’ ( almost always ). Here, we report the general PTQ score. Cronbach’s alpha for the entire PTQ is α = 0.95 for the original study [ 27 ].
Brooding and reflection . Huffziger and Kühner [ 40 ] validated the 10-item short version of the Response Styles Questionnaire (RSQ; original English version: Treynor et al. [ 41 ]; long version: Nolen-Hoeksema [ 42 ]) with the facets Reflection and Brooding. In the RSQ, it is assumed that brooding describes dysfunctional ruminating about an unattained goal (e.g., “What am I doing to deserve this?"), while reflection describes a more goal- and solution-oriented self-reflection (e.g., “I write down what I am thinking and analyze it.”). Each scale comprises five items. Participants rated all 10 items on a 4-point Likert Scale ranging from ‘1’ ( almost never ) to ‘4’ ( almost always ). Cronbach’s α for the original study is .60 for brooding and .73 for reflection [ 40 ].
Self-efficacy . We used the General Self-Efficacy Scale (GSE; Hinz et al. [ 43 ]; English version: Schwarzer & Jerusalem [ 44 ]) to assess participants’ general sense of perceived self-efficacy, for instance in relation to coping with everyday life or after experiencing all kinds of stressful life events. The GSE comprises 10 items (e.g., “I can always manage to solve difficult problems if I try hard enough.”) and is answered on a 4-point scale (1 = not at all true , 2 = hardly true , 3 = moderately true , 4 = exactly true ). Cronbach’s α in the original study is .92 [ 43 ].
State rumination . We tested state rumination with two measures: First, we assessed the 8-item Brief State Rumination Inventory (BSRI, Marchetti et al. [ 23 ] to capture the current level of repetitive negative thinking at the time of answering. The BSRI was designed to capture maladaptive state rumination defined as “the momentary occurrence of thoughts that focus one’s attention on one’s distress along with its possible causes and implications ([ 23 ], p.2). All eight statements of the BSRI (e.g., “Right now, it is hard for me to shut off negative thoughts about myself”) were answered on an 11-point scale ranging from ‘0’ ( not at all ) to ‘10’ ( very ). We have translated the items into German for the purpose of our experiments. However, the items in the translated version are not validated. Cronbach’s α of the original study is .89 and .91 for before and after an experimental manipulation [ 23 ]. Second, we used a single item (“To which extent did you ruminate over something?”, see also Koval et al. [ 37 ]) to assess general state rumination (hereinafter referred to as general rumination rating), which was also rated on an 11-point scale ranging from ‘0’ ( not at all ) to ‘10’ ( very ).
Perceived strain . In addition, participants complete the same 11-point scale on how much they felt burdened by the problem.
It should be noted that there is a risk that the use of single-item scales may not adequately capture the construct. Nevertheless, the use of such single-item scales is also recommended [ 45 ] because they are “easier and take less time to complete, may be less expensive, may contain more face validity, and may be more flexible than multiple-item scales” [ 46 , p. 77].
Mood . We assessed the Multidimensional Mood Questionnaire (MDMQ; Wilhelm & Schoebi [ 47 ]) to measure three basic dimensions of mood–valence, energetic arousal, and calmness. The MDMQ consists of six items and is a bipolar measure that comprises three pairs of adjectives rated on a 7-point scale describing opposite end points of different mood dimensions (e.g., energetic arousal: tired vs. awake, full of energy vs. without energy). Cronbach’s α of the three scales ranged from .73 to .89 in the original study [ 47 ].
Goal-cueing task. The goal-cueing task by Roberts et al. [ 3 ] can be divided into three steps, i.e., identification, evaluation and focusing period of a personal relevant goal. In the EC1 (i.e., unresolved goal condition), participants are instructed to identify an ongoing unresolved goal that repeatedly troubled them, causing them to feel sad, negative, or stressed during the previous week. Participants are also provided with appropriate examples of problems. Then, participants briefly outline their problem in 5 to 10 sentences. In step 2 (evaluation), participants indicate the extent to which the unresolved hamper their individual goal achievement process at two points in time, the present time, and the time when it was at its worst. Further, participants indicate how important the goal is, how much the problem in the individual goal achievement process exemplifies more general problems, how long the problem exists and how much time they spend thinking about the problem last week. Step 3 consists of a 10-minute goal-focused period, where participants work through a pre-recoded script delivered over headphones, which guide them thorough focusing on the identified unresolved concern. Example instructions are “Think about what is important about this difficulty in terms of your personal goals”, or “Focus on the aspects of the difficulty that repeatedly come to mind”. Based on findings of Klinger et al. [ 48 , p. 3] showing that cues may take many forms, for example, “a word, an image, or a smell that is associated with an ongoing goal pursuit (including cues related to failure to achieve a goal)”, we extended the original goal-focusing period to increase the induction of state rumination. We then added seven new instructions at the beginning of the goal focus period that trigger all five senses of the participants (e.g., “What did the situation look like in concrete terms?… What did you see? Remember all the sounds you perceived in the situation?…Try to remember every detail of your surroundings.”) to increase the probability of finding a trigger for spontaneous state rumination. We also shortened the intervals between the instructions so that the total time of 10 minutes was not exceeded.
Sustained Attention to Response Task (SART) . The SART [ 36 ] is a simple go/no-go paradigm in which neutral words (e.g., “father”, “shirt”, or “green”) are presented in white text on a black background in the center of the screen. Each word appeared individually on screen for 300ms followed by a 900ms mask (see Fig 1 ). The participants’ task was to respond to words in lowercase letters as quickly as possible by pressing the space bar (go-trials, e.g., “flower”). When a word appeared in upper case, participants were required to withhold their response (no-go-trial, e.g., “CHURCH”). The SART comprises in its shortened version two blocks of 450 trials each, consisting of 45 words repeated ten times in a different order. Within each set of 45 words, five uppercase words appeared randomly among 40 lowercase words. After 450 trials (i.e., one block), we allowed the participants to take a break, where they could determine the length of the break themselves up to a maximum of 3 minutes. The SART took approximately 30 minutes to complete.
(A) Overview of the procedure of Experiment 1a. After participants completed the initial survey, they were assigned to the different conditions and given access information for the experimental session. The experimental session started with a baseline survey, in which the current levels of state rumination, mood, and perceived strain were collected. Subsequently, the goal-cueing task, with its three steps–step1: Goal identification, step 2: Goal evaluation and, step 3: Goal focus period) was carried out. The manipulation check, which included the same items as the baseline survey followed. To get familiar with the task, participants played 18 practice trials before they started with the first bock of the SART (for Experiments 1c and 2, the practice trials were conducted at the beginning of the experimental session before the baseline survey). Participants played two blocks with self-determined breaks, in which the current level of state rumination, mood, and perceived strain were collected. We also performed the same query after 60% of no-go trials in every block and after the SART ended. In addition, five post questions concerning the current evaluation of the identified goal followed. Finally, the debriefing took place in which the participants are fully informed about the objectives of the experiment. (B) shows a sequence of the SART, consisting of a go-trial (lowercase word) and a no-go trial (uppercase word), which are displayed 300ms each. In between appeared a mask for 900ms.
https://doi.org/10.1371/journal.pone.0288450.g001
Post evaluation of the identified problem . After completing the experimental part, participants indicated (1) how difficult it was to stop thinking about the concern, (2) to what extent their focus was mainly on negative aspects, or (3) on bad feelings, (4) to what extent thinking about the problem made it seem worse and (5) made them feel worse (questions were adapted from Mosewich et al. [ 49 ]). Participants rated all questions on a 5-point scale ranging from ‘1’ ( not at all ) to ‘5’ ( very ).
Data analyses.
Collection of experimental data from all four experiments was carried out with the Inquisit Webplayer [ 50 ] and data preparation and all statistical analyses were performed with the software RStudio [ 51 ].
Statistical tests . To analyze the effects of our goal-cueing task on state-rumination, mood and perceived strain, we applied single mixed analyses of variance (ANOVAs) using the ezANOVA-function (“ez”-package; [ 52 ]) with time (pre vs. post goal-cueing task) and condition (unresolved vs. shortened unresolved [vs. control]) as a factor. Beforehand, we checked the requirements for the application (normal distribution and homogeneity of variances). We conducted a Shapiro-Wilk Test for testing the assumption of normality ( p > .05) and a Levene’s Test for testing the homogeneity of variance ( p > .05). Given the data we collected to test the effectiveness of the experimental setting in different contexts, we were also able to conduct an exploratory analysis related to gender differences to provide further information to researchers and practitioners. For this purpose, we included gender as an additional factor in our ANOVA. Since this analysis was not the focus of our research question, we did not formulate a specific hypothesis in this regard. However, previous studies highlighted the tendency of women to show more rumination as opposed to men [ 41 , 53 , 54 ]. The results of the exploratory analyses are summarized in S1 Table .
We additionally applied single mixed ANOVAs with time (four time points during the SART) and condition (unresolved vs. modified unresolved goal) as factors to investigate whether the goal-cueing task also led to changes of state rumination, mood, and perceived strain during the SART. Before that, we checked whether the data fulfilled the requirements. In case of violation of sphericity, we used Mauchly’s Test ( p < .05) and applied a Greenhouse-Geisser correction for ANOVAs. Since these analyses were not the focus of our experiments, but we are pleased to provide the information for further research purposes, we report the results in S2 and S3 Tables.
To compare the SART task performance across participants in different conditions, we examined the errors of commission (i.e., incorrect responses: key presses in no-go trials) as well as the mean reaction times (mean RTs) for correct go-trials. According to Cheyne et al. [ 55 ], mean RTs were calculated for all response latencies over 200ms. Reaction times less than 100ms were coded as anticipatory and reaction times between 100ms and 200ms were coded as ambiguous. We then applied mixed ANOVAs with time (SART: Block 1 vs. Block 2) and condition (e.g., unresolved vs. shortened unresolved [vs. control]) as factors.
Regarding participants’ characterization of problems in the individual goal achievement process and their evaluation of the identified problem after the experimental setting, we analyzed mean differences between conditions with independent t -tests. In case of non-parametric distribution, we reported the significance of Wilcoxon signed-rank test as robust alternative for independent t -tests ( p wilcox ; Field et al. [ 56 ]).
Effect sizes . We report the effect size of mean differences between conditions with Cohen’s d [ 57 ] with the following criteria: d = 0.20, d = 0.50 and d = 0.80 for small, medium, and large effects (see also Fritz et al. [ 58 ] for interpretation). In case of non-parametric distribution, we report the significance of Wilcoxon signed-rank test with corresponding robust effect size (r). The interpretation values for r are: 0.10 to < 0.30 for a small effect, 0.30 to < 0.50 for a moderate effect and ≥ 0.50 for a large effect [ 58 ]. For the ANOVAs, we report partial eta squared (ηp2) as a measure of effect with the following criteria for small, medium, and large effect: 0.01, 0.06, and > 0.14 [ 59 , 60 ].
Characterization of problems in the individual goal achievement process.
Considering the results of the characterization of the identified problems, there were no differences between the participants in both conditions. That is, there were no differences in terms of how the unresolved goal hampered participants individual goal achievement process at the present time and at the time when it was at its worst, how important the goal was, how much the problem in the individual goal achievement process exemplified more general problems, how long the problem existed and how much time they spent thinking about the problem last week. The upper part of Table 2 summarizes the descriptive statistics and the results of the independent t -tests for participants’ goal-evaluations for Experiments 1a-c.
https://doi.org/10.1371/journal.pone.0288450.t002
Effects of goal-cueing task on state rumination, mood and perceived strain.
Table 4 summarizes the results of the respective F -statistic for each mixed ANOVA separated by main effects.
We found no significant difference between the conditions in our experimental variables except for perceived strain, F (1,162) = 4.13, p = .04, ηp 2 = .02, indicating higher values for participant in the EC1 compared to participants in the EC2. Furthermore, time effects were obtained in all variables used except for energetic arousal, F (1,162) < 1. Participants’ valence and calmness levels decreased significantly after the goal-cueing task, whereas levels of state rumination and perceived strain increased.
In addition, there was a significant interaction effect for the general rumination rating, and energetic arousal. Participants’ general state rumination was lower before than after the goal-cueing task in both experimental conditions (EC1: p bonf < .001; EC2: p bonf < .01) with significantly different scores between the two conditions after the goal-cueing task ( p bonf = .04). Regarding participants’ energetic arousal, post-hoc test revealed no significant differences.
Errors of commission and mean RT during the SART.
There are no differences in SART performance between participants in either condition (see Table 5 ).
Evaluation of the identified problem/concern after the experimental setting.
To retrospectively assess the impact of the identified problem on various aspects and to examine respective potential differences between conditions, participants answered five different questions. Results indicated that participants in the EC1 had significantly more difficulty stopping to think about the problem than participants in the EC2 ( p wilcox < .01, r = .24). There were no differences in the other goal evaluation questions. Table 2 summarizes mean values and standard deviations, as well as the respective test statistic for each experiment.
Summary of the results
The results confirm a successful induction of state rumination in that values of both state rumination measures increase from before to after the goal-cueing task. Interestingly, an additional interaction effect shows that the values after the goal-cueing task are significantly higher in the EC1 than in EC2. However, this applies only for the general rumination rating and the effect can be considered small. In terms of mood, there was essentially a decrease over time (except for energetic arousal) and perceived strain showed an inverse effect. In addition, there were no differences in performance on the SART and no differences in problem characterization. Furthermore, regarding post-evaluation, participants of EC1 reported more difficulties to stop thinking about the problem (which is a definitional component of rumination) than participants of EC2.
Discussion Experiment 1a
Shortening the instructions of the unresolved condition (i.e., omitting step 3 –goal focus period) did not in principle affect the results and led to similar outcomes in terms of state-rumination on both measures compared to the elaborated unresolved condition. This suggests that both experimental conditions can be used equally to induce state rumination in the participants. However, the experiment is limited by the absence of a control condition. To make a valid statement regarding the effectivity of the two experimental conditions, they must be compared with a control condition in a next step.
In general, the shortened version would have the advantage of saving time and reducing mental stress for the participants. This would be beneficial for future application in laboratory and in field studies. To this end, it would be necessary to verify whether the difference between the two ECs in terms of participants’ perceived strain disappears because it is due to the different duration of the conditions (and thus to a difference in intensity), or whether other causes underlie the difference in strain perception.
Experiment 1b
Results from Experiment 1a suggested that the identification of a problem in the individual goal achievement and its evaluation is sufficient to produce state rumination. The aim of the second experiment was now to compare the experimental condition (i.e., the elaborated unresolved condition) with a further shortened experimental condition (i.e., omitting step 2 –goal evaluation and step 3 –goal focus period) to examine whether the effects remain stable with respect to state rumination. To better interpret potential effects, we also added a neutral control condition [i.e., NCC], which does not refer to individual goals.
Overall, forty psychology students were recruited from the Johannes Gutenberg-University Mainz for the online experiment. However, we excluded one participant who reported not making a serious effort to follow the implementation instructions from further data analysis (see also Evaluation of the quality of the online experiment ). Thus, 39 participants (female: n = 27; male: n = 12) were included in the following analysis. At the time of the data collection, 20 students were enrolled in the bachelor and 19 in the master program. The mean age was 26.20 ( SD = 6.79), and participants received course credits for participating. The lower part of Table 1 presents an overview of sample characteristics separated by condition. Moreover, participants in the three conditions did not significantly differ in their level of trait rumination, F (2,36) = 0.02, p = .97, ηp 2 < .01.
Evaluation of the quality of the online experiment.
Participants rated the quality of their performance, indicating that their concentration was 3.67 ( SD = 0.84), that they made an average effort of 4.54 ( SD = 0.68) to follow the instructions seriously, and that they succeeded with 4.05 ( SD = 1.00). Furthermore, participants in the three conditions did not differ significantly on these three quality measures (all F ’s [ 2 , 36 ] < 1).
Goal-cueing task . The EC1 remained the same as in the previous experiment. EC2 was a shortened version of the original unresolved condition, in which participants identified only a problem in their individual goal achievement process (step 1), whereas participants in the NCC were given a neutral writing task that did not relate to personal goal but had the same extent. Participants were asked to describe what they did from the morning until the time of the experiment [ 61 , 62 ] instead of identifying a problem of their individual goal achievement process.
The characterization of the identified problem refers solely to EC1, since this part was not included in EC2. Table 2 shows mean values and standard deviations for comparison with the other experiments.
Effects of goal-cueing task on state rumination, mood, and perceived strain.
Mean values and standard deviations of the relevant experimental variables are presented in Table 3 and the results of the respective F -statistic for each mixed ANOVA are summarized in Table 4 .
https://doi.org/10.1371/journal.pone.0288450.t003
https://doi.org/10.1371/journal.pone.0288450.t004
State rumination . With respect to the state ruminations measures, a significant interaction effect for the general ruminations rating was observed in addition to significant time and condition effects. Post-hoc tests indicated a significant difference before and after the goal-cueing task for EC2 ( p bonf = .04). Furthermore, both experimental conditions differ significantly in their general state rumination after the goal-cueing task from the NCC (EC1: p bonf < .001; EC2: p bonf = .001). Finally, we found no differences between the two experimental conditions after the goal-cueing task and no differences before the goal-cueing task for all conditions.
Perceived strain . A similar pattern of results emerged for the perceived strain, where participants in NCC reported significant lower perceived strain after the goal-cueing task than the EC1 and the EC2 (both ECs: p bonf < .001). Differences in perceived strain before and after the goal-cueing task were evident only for both ECs (EC1: p bonf < .01; EC2: p bonf = .04). There were no significant differences between conditions before the goal-cueing task.
Mood . For mood, there were no significant main effects for either condition or time. However, significant interactions were found for energetic arousal and valence, although these related only to differences in valence before and after the goal-cueing task in the EC1 ( p bonf = .02) and to differences after the goal-cueing task between EC1 and NCC ( p bonf < .01).
With regard to participants SART performance, results revealed a single significant effect for the factor time, F (1,36) = 5.70, p = .02, ηp 2 = .14, indicating that more errors of commission were made as time went on (see Table 5 ).
https://doi.org/10.1371/journal.pone.0288450.t005
No differences were found between the EC1 and the EC2 regarding the evaluation of the identified problems after the experiment.
Summary of the results.
The results of the mixed ANOVAs showed a significant interaction effect for the general rumination rating, in that both ECs were significantly different from the NCC after the goal-cueing task. Post-hoc analyses also showed a significant change in the general rumination rating from before to after the goal-cueing task for EC2. The same pattern of results applies to the participants’ perceived strain. Here, in addition to the change in EC2 over time in the goal-cueing task, an increase in perceived strain in EC1 was also noted. With respect to the BSRI, the interaction effect was again absent. In terms of mood, the only notable effect is the significant interaction between condition and time in the valence scores, which increased significantly in EC1 after the goal-cueing task and differed significantly from NCC after the goal-cueing task. The results of the SART performance analysis showed that errors of commission increased over time regardless of conditions. Finally, the goal characteristic of EC1 is comparable to the values from Experiment 1 and there are no differences between ECs in terms of the post evaluation.
Discussion Experiment 1b
The main aim of Experiment 1b was to further shorten the experimental condition (i.e., original unresolved condition) for future application and to examine whether the effects remain stable with respect to state rumination. We also added a neutral control condition to better interpret potential effects. In detail, we maintained the EC1 from the previous experiment and compared it to two further conditions: EC2 consisted of a shortened version of the elaborated unresolved condition and included only step 1 of the goal-cueing task while NCC was not related to a personal goal. As expected, significant condition and time effects were obtained using both measures of state rumination, namely the BSRI and the general rumination rating. With respect to the latter, there was a significant condition x time interaction that, in addition to significant differences in state rumination before and after the goal-cueing task for both experimental conditions, also significantly differentiated the EC1 and EC2 from the NCC after the goal-cueing task. At this point, however, it must be noted that even though the conditions before the goal-cueing task did not differ significantly in their level of state rumination, the EC1 showed relatively high values. This could be due, among other things, to the fact that participants are already told in the instructions immediately before the experimental part that they must identify a personal problem, which could already trigger initial ruminative processes. However, the question of why the elevated values were found exclusively in the EC1 remained unanswered.
As part of the development process of the goal-cueing task, we only aimed to test whether further shortening would tend to result in comparable state rumination, so Experiment 1b is more of an exploratory approach. Further, Experiment 1b is also severely limited by the small sample size and the general homogeneous sample of psychology students. During college, reflecting on successes and failures in achieving important personal goals is a commonplace process [ 63 ]. Especially psychology students represent a target group with experience in self-regulation or at least a good knowledge of it. Thus, it cannot be ruled out that theoretical and practical knowledge of self-regulation influenced the effects of the goal-cueing task. Hence, only cautious recommendations can be made to further shorten the experimental condition for future laboratory and field applications. In addition, it must be considered that while further shortening may save time in an experimental setting, valuable information related to individual goals is lost.
Experiment 1c
Since EC1 has so far achieved the strongest effects in Experiments 1a and 1b, but this is the "least favorable" condition due to its length and the loss of information when reducing the experimental condition to only one step of the goal-cueing task seems too large for some application contexts, we reduced the goal focus period (step 3) of the elaborated unresolved condition from 10 min to 5 min in Experiment 1c. We then compared these again with the shortened experimental condition from Study 1 (omitting step 3 –goal focus period) and the neutral control condition. In addition, we applied a new measure of state rumination, namely the ruminative self-focus index according to Moberly and Watkins [ 15 ], which captures participants’ focus on their own feelings and problems at the time of the prompt.
Sixty participants completed the experiment. One participant who reported not making a serious effort to follow the implementation instructions was excluded from further data analysis (see also Evaluation of the quality of the online experiment ). Thus, 59 participants (female: n = 41, male: n = 18, M age = 24.76, SD age = 5.37) were included in the following analyses. Participants received 12 Euro as compensation for their participation in the experiment. Table 1 presents an overview of sample characteristics separated by both experimental (i.e., EC1 and EC2) and the neutral control condition (i.e., NCC). Participants in three conditions did not significantly differ in their level of trait rumination, F (2,56) = 0.04, p = .96, ηp 2 < .01.
Regarding the quality of performing the online experiment, participants indicated that their concentration was 3.65 ( SD = 0.94), that they made an average effort of 4.59 ( SD = 0.65) to follow the instructions seriously, and that they succeeded with 4.08 ( SD = 0.95). Participants in the three conditions did not differ significantly on the three quality measures (concentration & seriousness: F (2,56) < 1; success: F (2,56) = 1.49, p = .23, ηp 2 = .05).
Ruminative Self-focus . In addition to the BSRI, we now measure state rumination with the momentary ruminative self-focus index from Moberly and Watkins [ 15 ], which includes two items assessing (1) to which extent participants were focused on their feelings and (2) to which extent they were focused on their problems. Both items were rated on an 11-point scale ranging from ‘0’ ( not at all ) to ‘10’ ( very ).
Goal-cueing task . The goal focus period of the original unresolved condition was now shortened von 10 to 5 minutes (EC1). The shortening essentially referred to the removal of the seven instructions at the beginning of the audio script that were intended to trigger the participants’ five senses. EC2 corresponds to EC2 from Experiment 1a (i.e., step3 –goal focus period was omitted, and the neutral control condition (NCC) remained the same as in Experiment 1b.
There were no differences between the two experimental conditions in terms of their characterization of the identified problem (see Table 2 ).
Table 3 presents mean values and standard deviations of the relevant experimental variables. Table 4 summarizes the results of the respective F -statistic for each mixed ANOVA.
Ruminative self-focus . With respect to the ruminative self-focus of participants, a significant interaction effect was found in addition to significant time and condition effects. Post-hoc tests indicated that ruminative self-focus significantly increased from before to after the goal-cueing task ( p bonf < .001) in the EC1. There were no differences for the NCC ( p bonf > .99) and no differences for EC2 ( p bonf = .08). In addition, the two experimental conditions showed no significant differences in ruminative self-focus after the goal-cueing task ( p bonf > .99); however, both differed significantly from the NCC (EC1: p bonf < .001; EC2: p bonf < .01).
BSRI . Regarding the results of the BSRI, a significant time effect as well as a significant time x condition interaction was obtained. Subsequent post-hoc analyses showed that the NCC significantly differed in their BSRI scores from the EC1 ( p bonf = .01) but not from the EC2 ( p bonf = .18) after the goal-cueing task. Moreover, we obtained no other significant differences between conditions.
Perceived strain . For the main effects, the pattern of results was the same as for the BSRI. Post-hoc analysis showed that perceived strain significantly differed from before to after the goal-cueing task in the EC1 ( p bonf < .001) and EC2 ( p bonf < .01) but not in the NCC ( p bonf > .99). In addition, the two experimental conditions did not significantly differ in their perceived strain after the task ( p bonf > .99); however, both differed significantly from the NCC (EC1: p bonf < .001; EC2: p bonf < .001).
Mood . We found no significant effects for either condition or time or its interaction in the mood measures.
Regarding participants SART performance, results revealed a significant effect for the factor time, F (1,57) = 4.41, p = .04, ηp 2 = .08 for errors of commission, indicating that more errors of commission were made as time went on (see Table 5 ).
No differences were found between the EC1 and the EC2 regarding the evaluation of the identified problems after the experiment (see Table 2 ).
The results confirm a successful induction of state rumination, which is first expressed by a significant interaction with a large effect on both rumination measures. Both measures differ significantly from the NCC after the goal-cueing task, although for EC2 this only applies to ruminative self-focus. The same pattern of results was found for perceived strain. No significant effects were observed regarding mood. There were also no significant differences in terms of goal characteristic and post evaluation. Finally, the results of the SART analysis, showed a similar result as in Experiment 1b: regardless of the condition, more errors of commission were made over time.
Discussion Experiment 1c
Since EC1 has so far produced the strongest effects in Experiments 1a and 1b but is the "least favorable" condition due to its length, the third experiment aimed to test the effectivity of the elaborated unresolved condition with a reduced goal focus period (5 min). This reduced EC1 was then compared again with EC2 from Experiment 1a (i.e., omitted step 3 –goal focus period) and NCC (i.e., not related to personal goals) from Experiment 1b. In addition, we added a new measure of state rumination, namely the rumination self-focus index according to Moberly and Watkins [ 15 ], which captures participants’ focus on their own feelings and problems.
The results underscore the findings from the previous two experiments, in the sense that now significant interaction effects were obtained for both ruminations measures, confirming the effectivity in inducing state rumination as well as the clear distinction from a neutral control condition unrelated to personal goals. With respect to SART performance, a further time effect for errors of commission was shown in addition to Experiment 1b, indicating an increase in errors of commission for all conditions. Why no other significant main effects were obtained in the SART task in Experiments 1a-c is discussed in the general discussion.
General discussion and limitations Experiments 1a-c
In our three experiments, we focused on an experimental condition (i.e., goal-cueing task) to induce state rumination. In this task, participants were asked to identify, evaluate, and focus on a personally relevant goal that was previously unresolved and still bothering them. We further tested modifications of the task (i.e., shortenings of the elaborated unresolved condition to different degrees) and tested the effectivity of these modifications compared to the elaborated unresolved condition. The results of these three experiments demonstrate that state rumination can be induced by the application of the goal-cueing task. However, some shortcomings of the general approach of these three studies must be considered before a final conclusion can be drawn.
One limitation is that the three experiments obtained mixed results regarding the state rumination measures. While the overall pattern of results supports the assumption that the goal-cueing task can elicit rumination in an experimental context, the question remains whether all three versions are correspondingly effective. One reason for the mixed results could be that the three experiments have very different sample sizes, which leads to a potential power problem especially in Experiments 1b and 1c. To test this, we calculated post-hoc power analyses for all three experiments, which showed low power for Experiments 1a and 1b and sufficient power for Experiment 1c. In detail, results of post-hoc power analyses in terms of the strongest interaction effects were as follows: Experiment 1a: N = 164, α = .03, η p 2 = .03 ( f = .17), power = .52; Experiment 1b: N = 39, α = .03, η p 2 = .17 ( f = .45), power = .55; Experiment 1c: N = 59, α = .001, η p 2 = .33 ( f = .77), power = .91). Another drawback of our approach is the type of state rumination measures we used. To conduct our experiments, we relied on partially unvalidated measures such as our translated version of the BSRI. Although this means that the results should be interpreted with caution, this fact points to a general problem of limited measures of state rumination (especially in the German-speaking area). Complementing this, Marchetti et al. [ 23 ] highlighted that even available measures are often still characterized by unknown and/or unreplicated psychometric properties. To further validate the efficacy of the goal-cueing task, additional state measures should be considered that capture additional facets of rumination, such as repetitive quality, degree of abstractness, or uncontrollability of rumination processes. Thus, for future research, a 4-item measure from Rosenkranz et al. [ 64 ] or Krys [ 18 ] seems promising for capturing more facets of rumination.
Considering the results from the first three experiments (especially those from Experiment 1c), the EC1 with the reduced goal focus period (5 minutes) seems to be a reliable induction of rumination. The shortening of the goal-cueing task also has the advantage of avoiding confusion between abstract and concrete processing style of rumination. While in Experiments 1a and 1b the intention was to enhance the induction of rumination by adding items that involved the use of all senses, participants may also have been more focused on the direct, specific, and contextualized experience of an event through this procedure, which would correspond to a more adaptive, concrete processing style of rumination [ 1 , 28 ]. However, this is in contrast to the more abstract processing style, which refers to repetitive and difficult to control dwelling on one or more negative topics, which can be considered a more maladaptive processing style that has classically been defined as rumination [ 1 , 28 ]. Consequently, different processing styles and their mixture can have different effects on affect, mood, and emotion regulation [ 1 , 2 , 28 , 29 , 65 ]. Although the results of Experiments 1a and 1b pointed in the desired direction regarding state rumination, the instructions should be clearly assigned to a processing style in the future to increase the effects of the goal-cueing task. Furthermore, the use of a neutral control condition can also be seen as limiting. In Roberts’ original study [ 3 ], the control condition also related to a goal. This allows the comparison of variables according to similar induction conditions with the emphasis on the goal discrepancy to be activated in the unresolved goal condition but not in the resolved goal condition. However, the reflection of an achieved goal can initiate the processing of currently pursued, unachieved goals [ 66 ]. Consequently, information associated with unachieved goals may have higher availability, be prioritized accordingly, and thus automatically attract attention [ 67 ]. Therefore, to draw more precise conclusions about which conditions in the goal-cueing task should be used in further research, it is necessary to compare the two control conditions (resolved goal condition vs . neutral control condition) with each other as well as the effectivity of the shortened experimental condition compared to the original unresolved condition from Roberts and colleagues [ 3 ].
Experiment 2
Experiment 2 aimed to address some of the limitations observed in Experiments 1a-c, namely, the lack of comparison between the original goal-related control condition of Roberts et al. [ 3 ] and the neutral control condition previously used in Experiment 1b and 1c. Further we aimed at comparing our experimental condition 1 (i.e., shortened audio instructions; 5 min), with the original unresolved experimental condition of Roberts et al. [ 3 ] (see for a detailed description Measures and Material section Experiment 1a). In detail, we compared the experimental condition 1 (i.e., shortened unresolved condition; EC1) and the neutral control condition (i.e., neutral control condition not related to a goal; NCC) from Experiment 1c with the original experimental condition from Roberts et al. [ 3 , i.e., original unresolved condition; EC2] as well as the original resolved control condition from Roberts et al. [ 3 , i.e., goal-related control condition; GRCC]. Using four 4 (EC1, EC2, GRCC, NCC) x 2 (before the goal-cueing task, after the goal-cueing task) mixed ANOVAs, we examined the effectiveness of the goal-cueing task with different state ruminations measures. For this purpose, in addition to the already applied measures (BSRI, ruminative self-focus index, and general rumination rating) we used a new measure to capture participant’s momentary repetitive negative thinking [ 64 ]. Although we criticized the use of the BSRI in Experiments 1a-c, we applied it again in Experiment 2 to be consistent with our previous experiments and to be able to directly compare results. Moreover, we assessed the same additional variables (MDMQ and perceived strain) to account for emotional processes in addition to cognitive processes, and again used the SART as an additional outcome measure.
We performed an a priori power analysis (G*Power 3.1.) [ 68 ] for Experiment 2 to determine an appropriate sample size. To obtain a mean interaction effect ( f = 0.25) with an alpha of α = .05 and a power of .80 using a mixed ANOVA with time (induction: pre vs. post) and condition (EG1 vs. EG2 vs. GRCC vs. NCC) as factors, a total sample size of N = 184 was recommended (n = 46 per condition).
Overall, 214 participants completed the experiment. However, we excluded two participants who reported not making a serious effort to follow the implementation instructions and further seven participants, who stated that they had already participated in a previous study on the same topic. Accordingly, the final sample consisted of 205 participants (female: n = 142; male: n = 61; divers: n = 2). The mean age was 24.28 ( SD = 5.70). Most of the participants were students with different subjects (n = 172), followed by 21 employers. The remaining 12 participants were either pupils, trainees, civil servants, self-employed, job seekers or other. Table 6 presents an overview of sample characteristics separated by condition. Moreover, participants in the four conditions did not significantly differ in their level of trait rumination, F (1,203) = 0.07, p = .79, ƞp 2 < .01.
https://doi.org/10.1371/journal.pone.0288450.t006
Participants rated the quality of their performance, indicating that their concentration was 3.48 ( SD = 1.01), that they made an average effort of 4.46 ( SD = 0.68) to follow the instructions seriously, and that they succeeded with 4.02 ( SD = 0.92). In terms of concentration, there were no differences between participants in the four conditions, F (3,201) = 1.61, p = .19, ƞp 2 = .02. In contrast, GRCC ( M = 4.25, SD = 0.72) differed significantly from NCC ( M = 4.68, SD = 0.55; p bonf < .01) in how seriously they tried to perform the experiment, F (3,201) = 4.14, p = .01, ƞp 2 = .06. In addition, there was also a significant difference between NCC ( M = 4.32, SD = 0.85) and GRCC ( M = 3.83, SD = 0.89; p bonf = .03) in terms of how well they succeeded in implementing the instructions, F (3,201) = 3.45, p = .02, ƞp 2 = .05.
Participants were invited to participate in the experiment via institutes’ student mailing list, via the respective study offices of the study programs as well as notices on the bulletin board of the Johannes Gutenberg-University Mainz, and personal contacts. Participation in this experiment was voluntary and participants received 12 Euro as compensation (psychology students could alternatively receive course credit for their participation). Otherwise, the procedure is the same as in the Experiments 1a-c.
Here we report only the measures that we added compared to Experiments 1a-c.
State Rumination . In addition to the already used BSRI, ruminative self-focus index, and the general rumination rating, we applied a measure to capture momentary repetitive negative thinking (MRNT, i.e., process-related scale) [ 64 ]. The scale comprises four items (e.g., “Thoughts come to my mind without me wanting them to”.), each of which focused on a core characteristic of rumination–repetitiveness, intrusiveness, uncontrollability, and impairment. Participants answer each statement on a 7-point scale ranging from ‘1’ ( not at all ) to 7 ( very ). Just like the BSRI, we collected the MRNT at four points in time.
Goal-cueing task . EC1 and NCC remained the same as in Experiment 1c. EC2 and GRCC referred to the original conditions of Roberts and colleagues [ 3 , for a detailed description of EC2 see Measures & Materials section of Experiment 1a]. Participants in the GRCC were instructed to identify a problem from the past that has since been resolved, has not come to mind in the past, and no longer makes them feel bad, sad, down, or stressed. Like EC2, participants were asked to briefly outline the identified problem in writing, answer some questions about it, and follow a 10-minute audio instruction (for example: "Think about why solving this problem will make progress toward your personal goals; for detailed information see Roberts et al. [ 3 ]).
We performed the same analysis as in Experiments 1c. The only difference is that we used four conditions in the group comparisons. In case of a significant two-way interaction, we applied a simple main effect analysis (one-way model) of the condition variable at each level of the time variable and reported adjusted p -values according to Bonferroni. If the simple main effect was significant, we ran multiple pairwise comparisons to determine which conditions were different. In addition, differences in terms of goal characteristics and post evaluation were calculated using an ANOVA rather than independent t -tests due to the three conditions being compared. Moreover, we also computed the same exploratory analyses in terms of the influence of gender as well as the change in the state measures during the SART. The results of these analyses are summarized in S4 to S6 Tables.
Results indicated that there were significant differences between the conditions in five of six goal-related questions. There was no significant effect of condition on participants’ evaluation of how long the problem exists. The upper part of Table 7 summarizes the descriptive statistics and the results of the F -tests for participants’ goal-evaluations. Post-hoc tests showed that both ECs differed significantly from GRCC in terms of trouble at the time of the experiment and time spent on the problem (both p bonf < .001).
https://doi.org/10.1371/journal.pone.0288450.t007
In addition, only the EC1 significantly differed from the GRCC in terms of goal importance ( p bonf < .01), how much the goal related to general concerns ( p bonf = .04), and how much the problem had bothered them at its worst ( p bonf = .01). Thus, the goals identified in the two ECs did not differ in subjective evaluations of their nature or severity, but participants in the ECs (especially in the EC1) reported that the problem was one that was bothering them more than participants in the GRCC.
Four two-factorial mixed effects ANOVAs with the between group factor condition (4 levels) and the repeated measure factor time (2 levels: pre vs. post goal-cueing task) were computed to examine the effects of the goal-cueing task on state rumination, mood, and perceived strain. Table 8 presents the mean values and standard deviations of the relevant experimental variables. With regard to state rumination, results indicated that there were significant interaction effects and significant condition effects for all used measures. For time, however, only two of four state rumination measures showed a significant effect.
https://doi.org/10.1371/journal.pone.0288450.t008
BSRI . There was a significant main effect of condition, F (3,201) = 3.86, p = .01, ηp 2 = .05, and a significant main effect of time, F (1,201) = 11.22, p = .001, ηp 2 = .05 which were qualified by a significant interaction between time and condition, F (3,201) = 8.94, p < .001, ηp 2 = .12. Subsequent pairwise comparisons between condition levels showed that the simple main effect of condition was not significant for the time prior the goal-cueing task ( p bonf = .67) but for the time after to the goal-cueing task ( p bonf < .001). Pairwise comparison between post goal-cueing task and each condition level showed that the mean BSRI score was significantly different in EC1 vs. NCC ( p bonf = .01), in EC2 vs. NCC ( p bonf < .001) and in CC vs. NCC ( p bonf = .02).
Momentary repetitive negative thinking . With regard to the results of MRNT, a significant condition effect was obtained, F (3,201) = 4.75, p < .01, ηp 2 = .07, as well as a significant time x condition interaction, F (3,201) = 11.67, < .001, ηp 2 = .15. The effect for time was not significant, F (1,201) = 1.35, p = .25, ηp 2 = .01. Considering the Bonferroni-adjusted p -value of the simple main effect analysis, the effect for condition at the time before the goal-cueing task was not significant ( p bonf = .44) compared to the time after the goal-cueing task ( p bonf < .001). Pairwise comparison showed that the MRNT was significantly different in EC1 vs. NCC ( p bonf < .001), in EC2 vs. NCC ( p bonf < .001), as well as in EC1 vs. GRCC ( p bonf = .01) and in EC2 vs. GRCC ( p bonf = .01).
Ruminative self-focus . There was a significant main effect of condition, F (3,201) = 16.54, p < .001, ηp 2 = .20, and a significant main effect of time, F (1,201) = 7.46, p < .01, ηp 2 = .03, which were qualified by a significant interaction between time and condition, F (3,201) = 29.96, p < .001, ηp 2 = .31. Subsequent pairwise comparisons between condition levels showed that the simple main effect of condition was not significant for the time prior the goal-cueing task ( p bonf = .87) but for the time after to the goal-cueing task ( p bonf < .001). Pairwise comparison between post goal-cueing task and each condition level showed that ruminative focus differed in EC1 vs. NCC ( p bonf < .001), in EC2 vs. NCC ( p bonf < .001) and in GRCC vs. NCC ( p bonf < .001).
General rumination rating . Regarding the general state rumination rating, a significant effect of condition, F (3,201) = 9.88, p < .001, ηp 2 = .13, as well as of the interaction between time and condition, F (3,201) = 13.82, p < .001, ηp 2 = .17 was obtained. The effect for time was not significant, F (1,201) < 1. Results of the subsequent simple main effect analysis showed that the effect for condition at the time before the goal-cueing task was not significant ( p bonf = .73) but was significant at the time after the goal-cueing task ( p bonf < .001). Pairwise comparison showed that the general rumination rating was different in participants from EC1 vs. NCC ( p bonf < .001), in EC2 vs. NCC ( p bonf < .001) and in GRCC vs. NCC ( p bonf < .001). There were also significant differences between EC1 vs. GRCC ( p bonf < .001) and EC2 vs. GRCC ( p bonf = .01).
Mood . We conducted three more 4 × 2 ANOVAs to also examine the effects of the goal-cueing task on mood assessed by the MDMQ. Regarding the energetic arousal, there was a significant effect for condition, F (3,201) = 4.02, p < .01, ηp 2 = .05, and for the time x condition interaction, F (3,201) = 4.61, p < .01, ηp 2 = .06, as well as a tendency to significance for time, F (1,201) = 3.77, p = .05, ηp 2 = .02. Subsequent pairwise comparisons between condition levels showed that the simple main effect of condition was significant for the time prior the goal-cueing task ( p bonf < .01) but not for the time after to the goal-cueing task ( p bonf = .07). Pairwise comparison between pre goal-cueing task and each condition level showed that energetic arousal differed in EC1 vs. EC2 ( p bonf = .001) as well as in EC1 and GRCC ( p bonf = .03). With regard to valence, we found a significant effect of condition, F (3,201) = 3.09, p = .03, ηp 2 = .04, and a significant main effect of time, F (1,201) = 20.69, p < .001, ηp 2 = .09 which were qualified by a significant interaction between time and condition, F (3,201) = 11.36, p < .001, ηp 2 = .14. Subsequent main effect analysis of the condition variable revealed no significant differences before the goal-cueing task ( p bonf = .70), but significant differences after the goal-cueing task ( p bonf < .001). Pairwise comparison showed that valence was different in participants from EC1 vs. NCC ( p bonf < .001), in EC2 vs. NCC ( p bonf < .01), and EC1 vs. GRCC ( p bonf = .03). Finally, results of the 4 x 2 ANOVA for calmness indicated a significant effect for condition, F (3,201) = 3.04, p = .03, ηp 2 = .04, and for the time x condition interaction, F (3,201) = 6.77, p < .001, ηp 2 = .09, but not for time, F (1,201) = 2.70, p = .11, ηp 2 = .01. Subsequent pairwise comparisons between condition levels showed that the simple main effect of condition was not significant for the time prior the goal-cueing task ( p bonf = .99) but for the time after to the goal-cueing task ( p bonf < .001). Pairwise comparison showed that calmness values were different in participants from EC1 vs. NCC ( p bonf = .001), and in EC2 vs. NCC ( p bonf < .001).
Perceived strain . Last, a 4 × 2 mixed ANOVA was applied to investigate the effect of the goal-cueing task on the perceived strain of the participants. There was a significant effect of condition, F (3,201) = 11.68, p < .001, ηp 2 = .15, and a significant effect of the interaction between time and condition, F (3,201) = 18.14, p < .001, ηp 2 = .21. The effect for time was not significant, F (1,201) < 1. Subsequent pairwise comparisons between condition levels showed that the simple main effect of condition was not significant for the time prior the goal-cueing task ( p bonf = .99) but for the time after to the goal-cueing task ( p bonf < .001). Pairwise comparison between post goal-cueing task and each condition level showed that perceived strain differed in EC1 vs. NCC ( p bonf < .001), in EC2 vs. NCC ( p bonf <. 001), as well as in EC1 vs. GRCC ( p bonf < .001) and in EC2 vs. GRCC ( p bonf < .001).
Table 9 illustrates the percentage error rates and mean RTs for each condition during each block of the SART and the respective results of the F -statistic. Regarding participants errors of commission, results revealed a significant effect for condition, F (3,187) = 3.30, p = .02, ηp 2 = .05, and for time, F (1,187) = 7.36, p < .01, ηp 2 = .04, but not for the interaction between condition x time, F (3,187) = 2.04, p = .11, ηp 2 = .03. With regard to the mean RT for correct go-trials, there was significant effect for time, F (1,187) = 6.26, p = .01, ηp 2 = .03, but no significant effects for condition as well as for the condition x time interaction, both F ’s(3,187) < 1, indicating slower mean RTs as time went on.
https://doi.org/10.1371/journal.pone.0288450.t009
No differences were found between the EC1, EC1 and the GRCC regarding the evaluation of the identified problems after the experiment (see Table 7 ). We also asked participants in both control conditions if they were thinking about a current problem that was bothering them during the experiment. This question was answered in the affirmative by 40 participants from GRCC (75.5%) and 31 participants from NCC (58.5%).
Discussion Experiment 2
Experiment 2 had two aims. First, we aimed at testing which control condition (goal-related vs . neutral) is best suited to maximize the effects of the goal-cueing task in terms of state rumination in future research. Second, we wanted to examine whether the shortened unresolved condition is as effective in inducing state rumination as the original unresolved condition by Roberts et al. [ 3 ]. For this purpose, we applied different rumination measures, namely the BSRI, the ruminative self-focus index, a general rumination rating, and a momentary repetitive negative thinking measure and performed four two-factorial mixed effects ANOVAs. Moreover, we assessed further variables (MDMQ and perceived strain), using the same approach, to account for emotional processes in addition to cognitive processes and again used the SART as an additional outcome measure.
Results of the mixed ANOVA indicated a successful induction of rumination, which is expressed by significant interaction effects (time x condition) on all four state rumination measures. Subsequent pairwise comparisons between condition levels showed that the simple main effect of condition was not significant for the time prior the goal-cueing task but was significant for the time after the goal-cueing task. This was consistent for all applied state rumination measures. In detail, participants in the two experimental conditions reported significantly higher scores on all four state ruminations measures after the goal-cueing task (except for the BSRI for the EC1, which showed only a trend effect with p bonf = .06) compared to participants from the neutral control condition. However, this did not apply to the goal-related control condition (i.e., original resolved condition by Roberts et al. [ 3 ]). Here, only the examination of the general rumination rating showed a significant difference to EC1 after the goal-cueing task. In addition, the GRCC showed higher state ruminations scores on two of four measures (ruminative self-focus, general ruminations rating) compared to the NCC. This could also be partly because 75% of the participants in the GRCC also thought about a current problem while conducting the experiment. According to Shah [ 67 ], reflecting on a solved problem during the goal-focusing period may have resulted in initiating the processing of currently pursued, unachieved goals. Consequently, it cannot be assumed that the goal discrepancy is activated only in the unresolved goal condition and not in the resolved goal condition. Whether these results ultimately also had an influence on the quality of the execution of the experiment, however, remains open. Here, minor differences between the two control conditions ( M Δ < 0.50) emerged in the sense that the goal-related control condition reported lower values for seriousness and successful execution. To summarize, in terms of state rumination, the results of Experiment 2 showed that the two ECs can be used equally well to induce state rumination in participants, which means that depending on the experimental design and the time available, either the original experimental condition or the shortened experimental condition can be applied. To maximize the effects in terms of state rumination, the NCC seems to be more suitable due to the better differentiation in the state measures.
In terms of mood, valence in EC2 was found to decrease during the goal-cueing task, and participants in both ECs reported significantly lower valence scores thereafter compared to the NCC. It seems unsurprising that participants who are asked to address an unresolved issue that has made them sad, depressed, or down in the recent past feel more uncomfortable than someone who is asked to be as objective as possible about their past daily routine. In addition, the NCC participants showed higher calmness values after the goal-cueing task compared to both ECs and the effect of energetic arousal refers to the difference in pre induction values between EC1 and GRCC and the two ECs. With this one exception, no further significant differences were found between the GRCC and the two ECs. Accordingly, it can be assumed that the mood in both ECs is similar, in some cases slightly more "negative" compared to the NCC, but not compared to the GRCC. The latter is consistent with Roberts et al. [ 3 ], who showed that participants in the unresolved and resolved condition did not differ with respect to sadness, tension, and self-focus. However, in the subsequent study [ 32 ], the two conditions were found to have different effects on participants’ negative affect (measured with the Positive Affect and Negative Affect Schedule [ 69 ], in the sense that negative affect increased during the goal-cueing task in the unresolved condition and differed significantly from the resolved condition thereafter. So far, however, it seems difficult to draw conclusions about the change in mood during the goal-cueing task, as either mood or affect were assessed with different measures in the studies.
In terms of performance in the SART, participants’ reaction times worsened regardless of condition and more errors were made as time went on. A condition effect indicates that the EC1 in principle has a higher error rate in the SART compared to the other conditions. However, due to the lack of interaction effects, no significant differences can be found with respect to the goal-cueing task. Why no other significant main effects were obtained in the SART is discussed in the general discussion.
Finally, we would like to address a potential limitation of this study: To examine the effectiveness of the goal-cueing task and its directly observable effect on state rumination, we used different state rumination measures to capture rumination with focus on different aspects (e.g., core characteristics of rumination, self-focused attention, attention to one’s distress and its possible causes and effects, general state of rumination), and to figure out which measure would be most appropriate for future studies. However, it cannot be ruled out that these in some way influenced each other. Therefore, the number of measurement variables for rumination should be reduced in future studies to avoid potential interference.
General discussion
The aim of the four experiments of the present study was to evaluate the effectivity of the goal-cueing task procedure [ 3 ] to induce state rumination on an individual level in different experimental settings. For this, we used different measures of state rumination covering different facets of rumination, namely the BSRI [ 23 ], an index of ruminative self-focus [ 15 ], a general rumination rating [ 37 ], and an EMA measure of momentary repetitive negative thinking [ 64 ]. Specifically, we were interested in whether the use of the goal-cueing task had a direct observable effect on state rumination as assessed by these different state rumination measures.
Since we have already discussed the results of each of the individual experiments above, we would only like to give a brief overview of the main results and discuss overarching aspects in this section. Experiment 1a showed that shortening the instructions of the unresolved condition (i.e., omitting step 3 –goal focus period) did not in principle affect the results. However, the significance is limited because a control condition was missing to relate the effects found. In Experiment 1b, we compared the EC from the previous experiment with two further conditions: another shortened experimental condition (included only step 1 of the goal-cueing task) and a neutral control condition that was not related to a personal goal. Here, for the first time, we found a significant interaction between time and condition with respect to the general rumination rating. However, Experiment 1b was severely limited by the small sample size and the general homogeneous sample of psychology students. Experiment 1c picked up the shortened experimental condition from Experiment 1a and compared it with another shortened experimental condition (reduced goal-focus period to 5 min) and the neutral control condition. By shortening the goal focus period, previously existing concerns about the processing style of rumination during the goal-cueing task could be eliminated. Significant interaction effects on both state rumination measures underlined the assumption of an effective form of rumination induction. Finally, Experiment 2 filled the gap, which became evident by the limitations and open questions of the previous experiments: 1) the comparison of the experimental condition with the shortened goal focus period and the neutral control condition from Experiment 1c with the original experimental condition from Roberts et al. [ 3 , i.e., original unresolved condition] as well as the original resolved control condition from Roberts et al. [ 3 , i.e., goal-related control condition]. Results from Experiment 2 confirmed a successful induction of rumination through the shortened experimental condition, which is expressed by significant interaction effects on all four state rumination measures.
Regarding the performance in the SART, apart from a few time effects (and an additional condition effect in Experiment 2); there were no relevant rumination-related effects. Null results between conditions regarding the SART may be attributed to three different reasons: First, consistent with the findings of Roberts et al. [ 32 ], the absence of condition effects could indicate that participants in the conditions did not differ in their attentional control capabilities. This is in line with a study by Schmitz-Hübsch and Schindler [ 70 ] who could not replicate the postulated relationship between performance on the SART in two different versions and self-reported everyday errors. Further, a study by Edwards [ 34 ] did not find differences in SART performance between a goal-avoidance and a goal-approach condition following the goal-cueing task. Second, participants in the unresolved condition, due to the perceived goal discrepancy, might have used more resources, such as attention, effort, or energy to solve the current discrepancy accordingly [ 71 – 73 ], and thus obtained similar results in the SART as the participants in the control conditions. Roberts et al. [ 3 ] even indicated that participants who focused on unresolved goals were slower and more accurate on the SART compared to participants in the resolved condition and speculated that this might be a compensatory strategy of slowing responses to reduce the risk of errors. Accordingly, the SART could also be seen as a welcome distraction to switch from a self-referential focus to an external focus. Third, Huffziger and colleagues [ 16 ] suggested that consequences of induced rumination might be stronger when inductions occur in natural contexts because it produces more personal-relevant reasons to ruminate about, which may consequently influence individuals’ actions and behaviors. Following the three reasons mentioned above, the use of the SART should be reconsidered in future studies depending on the research question.
Furthermore, in our own as well as previous studies [ 3 , 32 , 34 ], participants defined a personally relevant goal but were then confronted with a task that was unrelated to the defined goal and thus did not seem goal enhancing or particularly personally relevant. Accordingly, the question remains open how the goal manipulation affects the performance of personally relevant tasks such as they may occur in everyday life, for example perceived discrepancy or problems at work [ 74 ], an exam at university [ 8 ], or competitions in sports [ 75 ]. Future studies could therefore combine the goal-cueing task with a more relevant task in the laboratory or directly transfer the goal-cueing task into everyday life by means of an ambulatory assessment approach (see for example Huffziger et al. [ 16 ]). For this, the different modifications of our experimental conditions could provide first interesting starting points.
Finally, successful, and valid induction of rumination could be particularly useful in explaining causal relationships between rumination and individual goal achievement (e.g., what types of goals are more likely to trigger rumination that potentially affect daily performance and impact well-being, are they avoidance or approach goals, well versus poorly defined goals, or externally motivated goals versus internally motivated goals). Moreover, as a tool, this induction form can support further research in uncovering the mechanisms underlying rumination that influence specific mental processes (e.g., testing further hypotheses from the H-EX-A-GO-N model [ 1 ]). Building on the findings, future research could develop specific cognitive interventions (e.g., adapting cognitive-behavioral therapy for rumination for a nonclinical context [see: 24]) and effective problem-solving strategies through which rumination could be resolved and prevented in the future.
Limitations
A general limitation was that Experiments 1a-c took place during pandemic lockdown and therefore were performed as online experiments (Experiment 2 was then also conducted as an online experiment to be consistent with the previous experiments), making it much more difficult to control conditions and potential confounding variables compared to conducting the experiments in the laboratory [ 39 ]. However, we took some steps to increase the quality of the implementation of the online experiments (compare Advantage and disadvantage of applying an online experiment in our study section Experiment 1a). In addition, we asked the participants for their honest evaluation of their performance quality and the results suggested that the implementation and the environment was appropriate for participation in the experiments. In addition, existing comparative studies between laboratory and online experiments showed that the results agree surprisingly well [ 76 , 77 ].
In conclusion, the current experiments add to the research field of rumination and goal achievement by confirming experimental evidence for the correlation of rumination and individual goal achievement in the face of unresolved goals. Ultimately, these findings contribute to our understanding of potential triggers for rumination in non-clinical samples. Thus, the goal-cueing task allows researchers to test causal links between rumination and factors that are thought to lead to ruminative thinking. In addition, the results of the four experiments provide hints as to how the paradigm can be modified to be applied in other experimental settings in and outside the laboratory. In the future, the effects potentially achieved with this paradigm could also be significant in real life. For example, it would be valuable to examine the relationship between the dynamics of momentary rumination and day-to-day performance, as well as performance in specific contexts (e.g., work, sport, academics etc.).
Supporting information
S1 table. results of the multivariate analysis with group, gender and measure time as well as their respective interaction as factors and different rumination measures as dependent variables for experiments 1a-c..
https://doi.org/10.1371/journal.pone.0288450.s001
S2 Table. Test statistics for variables during the SART and corresponding effect sizes (ηp 2 ) of the respective mixed ANOVAs separated by effects for Experiments 1a-c.
https://doi.org/10.1371/journal.pone.0288450.s002
S3 Table. Mean values and standard deviations of relevant experimental variables assessed during the SART and separated by condition and time for Experiments 1a-c.
https://doi.org/10.1371/journal.pone.0288450.s003
S4 Table. Results of the multivariate analysis with group, gender and measure time as well as their respective interaction as factors and different rumination measures as dependent variables for Experiment 2.
https://doi.org/10.1371/journal.pone.0288450.s004
S5 Table. Test statistics for variables during the SART and corresponding effect sizes (ηp 2 ) of the respective mixed ANOVAs separated by effects for Experiment 2.
https://doi.org/10.1371/journal.pone.0288450.s005
S6 Table. Mean values and standard deviations of relevant experimental variables assessed during the SART and separated by condition and time for Experiments 2.
https://doi.org/10.1371/journal.pone.0288450.s006
Acknowledgments
We would like to thank Laura Huber and Jannis Tauchert for their help in collecting the data as well as all the volunteers who participated in our experiments. We would also like to thank Sandra Schönfelder for her advice on modifying the audio instructions.
- View Article
- PubMed/NCBI
- Google Scholar
- 4. Martin LL, Tesser A. Some ruminative thoughts. In: Wyer RS Jr., editor. Ruminative Thoughts. Advances in Social Cognition. Psychology Press; 1996. pp. 1–47. https://doi.org/10.4324/9780203763513
- 5. Martin LL, Tesser A. Extending the Goal Progress Theory of RuminationGoal Reevaluation and Growth. In Sanna LJ, Chang EC, editors. Judgments Over Time: The Interplay of Thoughts, Feelings, and Behaviors. Oxford University Press. 2006 Mar 30;145–62. https://doi.org/10.1093/acprof:oso/9780195177664.003.0009
- 9. Wimmer T. Positive und negative Metakognitionen über die Rumination und ihre differentiellen Effekte auf die kognitive Flexibilität bei dysphorisch/depressiven Frauen [Positive and negative metacognitions about rumination and their differential effects on cognitive flexibility in dysphoric/depressed women; dissertation]. Westfälischen Wilhelms-University, Münster; 2005. Available at https://miami.uni-muenster.de/Record/2ff91faf-5c87-47c5-9dc2-aaac555a0962 .
- 12. Kuhl J. Motivation und Persönlichkeit: Interaktionen psychischer Systeme [motivation and personality: Interactions of psychological systems]. Göttingen: Hogrefe; 2001. German.
- 25. Krys S. Intrusive and repetitive thoughts: Investigating the construct of rumination [dissertation]. Kiel University; 2019.Available at https://macau.uni-kiel.de/servlets/MCRFileNodeServlet/dissertation_derivate_00008314/Dissertation_Sabrina_Krys.pdf .
- 33. Lanning LER. Effect of Goal Discrepancy Rumination on Overgeneral Memory [dissertation]. University of Exeter: 2015. Available at http://hdl.handle.net/10871/20203 .
- 34. Edwards LC. Does approach vs. avoidance framing influence rumination cued by unresolved goals? [dissertation]. University of Exeter: 2017. Available at: https://ore.exeter.ac.uk/repository/bitstream/handle/10871/29758/EdwardsL.pdf?sequence=1&isAllowed=y .
- 44. Schwarzer R, Jerusalem M. Generalized Self-Efficacy scale. In: Weinman J, Wright S, Johnston M, editors. Measures in health psychology: A user’s portfolio. Causal and control beliefs. Windsor, UK: 1995. p. 35–37.
- 48. Klinger E, Koster EHW, Marchetti I. Spontaneous Thought and Goal Pursuit. From functions such as planning to dysfunctions such as rumination. In: Christoff K, Fox KCR, editors. Oxford Handbooks Online. Oxford University Press. 2018 Apr 5; 215–247. https://doi.org/10.1093/oxfordhb/9780190464745.013.24
- 50. Free Inquisit 5 Player app. version 5 [software]. Seattle, WA: Millisecond Software.
- 51. R Core Team. R: A language and environment for statistical computing. R Foundation for Statistical Computing, Vienna: 2018. Available from: https://www.R-project.org/ .
- 56. Field A, Miles J, Field Z. Discovering Statistics Using R. Sage Publication Ltd; 2012.
- 70. Schmitz-Hübsch M, Schindler S. Alltagsfehler und Sustained Attention- eine Replikation zum SART [Everyday errors and sustained attention- a replication of the SART]. [Unpublished undergraduate theses]. Technical University Dresden, 2008. German.
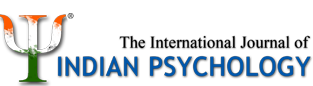
The International Journal of Indian Psychȯlogy
Effectiveness of Mindfulness Meditation in Controlling Examination Anxiety of Secondary School Students
| Published: May 31, 2024

This quasi-experimental study aims to find out the effectiveness of mindfulness meditation on controlling examination anxiety in secondary school students of Kerala. Pretest-posttest control group design was employed wherein four intact divisions of ninth grade students were taken randomly as one control group (n = 39) and three experimental groups (n = 116). Pre-testing and post-testing of examination anxiety was done by using the Examination Anxiety Scale for Secondary School Students developed by the investigators. The treatment groups were exposed to eight 90 minutes sessions of Brief Mindfulness-Based Stress Reduction Programme. One-way ANCOVA was performed to compare the control group and experimental group with respect to their post-test scores of examination anxiety after partialling out the effect of pre-test scores. The analysis revealed that mindfulness meditation is effective in reducing examination anxiety of secondary school students significantly. While the mindfulness treatment was equally effective for both the gender groups, significant difference was found to exists among students in different levels of academic achievement regarding the success of mindfulness meditation in alleviating examination anxiety.
Examination anxiety , Mindfulness meditation , Brief Mindfulness-Based Stress Reduction Programme , Secondary school students

This is an Open Access Research distributed under the terms of the Creative Commons Attribution License (www.creativecommons.org/licenses/by/2.0), which permits unrestricted use, distribution, and reproduction in any Medium, provided the original work is properly cited.
© 2024, Jithesh, T.V., Renjith Kumar, P. & Arjunan, N.K.
Received: May 20, 2024; Revision Received: May 27, 2024; Accepted: May 31, 2024
Arjunan N. K. @ [email protected]

Article Overview
Published in Volume 12, Issue 2, April-June, 2024
Low Self Esteem: What Does it Mean to Lack Self-Esteem?
Saul Mcleod, PhD
Editor-in-Chief for Simply Psychology
BSc (Hons) Psychology, MRes, PhD, University of Manchester
Saul Mcleod, PhD., is a qualified psychology teacher with over 18 years of experience in further and higher education. He has been published in peer-reviewed journals, including the Journal of Clinical Psychology.
Learn about our Editorial Process
Olivia Guy-Evans, MSc
Associate Editor for Simply Psychology
BSc (Hons) Psychology, MSc Psychology of Education
Olivia Guy-Evans is a writer and associate editor for Simply Psychology. She has previously worked in healthcare and educational sectors.
On This Page:
Self-esteem should be viewed as a continuum and can be high, medium, or low, and it is often quantified as a number in empirical research.
When considering self-esteem, it is important to note that both high and low levels can be emotionally and socially harmful to the individual. Indeed it is thought an optimum level of self-esteem lies in the middle of the continuum. Individuals operating within this range are thought to be more socially dominant within relationships.
Empirical Research
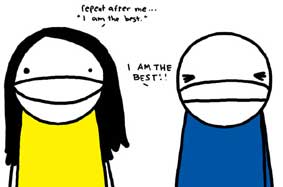
Research has shown key differences between individuals with high and low self-esteem. For example, people with high self-esteem focus on growth and improvement, whereas people with low self-esteem focus on not making mistakes in life.
Low self-esteem has been shown to be correlated with several negative outcomes, such as depression (Silverstone & Salsali, 2003).
Rosenberg and Owen (2001) offer the following description of low self-esteem people based on empirical research. People with low self-esteem are more troubled by failure and tend to exaggerate events as being negative.
For example, they often interpret non critical comments as critical. They are more likely to experience social anxiety and low levels of interpersonal confidence.
This in turn makes social interaction with others difficult as they feel awkward, shy, conspicuous, and unable to adequately express themselves when interacting with others (p. 409). Furthermore, low self-esteem individuals tend to be pessimistic towards people and groups within society.
Research has also shown that low self-esteem has to linked to an increased risk of teenage pregnancy.
Guindon (2002) asked school counsellors to list five characteristics that best describe students with low self-esteem. Over 1000 words were used and the most common are listed below:
- Withdrawn/shy/quiet
- Underachieving
- Negative (attitude)
- Socially inept
- Angry/hostile
- Unmotivated
- Dependent/follower
- Poor self-image
- Non-risk-taker
- Lacks self-confidence
- Poor communication
Low Self-Esteem in Children
It should be noted that, on average, self-esteem during childhood is found to be relatively high. However, there are individual differences, and some children are unfortunate to experience feelings of low self-esteem.
Low self-esteem in children tends to be related to physical punishment and the withholding of love and affection by parents. Carl Rogers would describe this as conditional positive regard, whereby individuals only receive positive attention from significant others (such as parents) when they act in a certain way. This reinforces to the child that they are only a person of value when they act a certain way (e.g., achieving A grades on a test).
Children with low self-esteem rely on coping strategies that are counterproductive such as bullying, quitting, cheating, avoiding, etc. Although all children will display some of these behaviors at times, low self-esteem is strongly indicated when these behaviors appear with regularity.
Socially children with low self-esteem can be withdrawn or shy and find it difficult to have fun. Although they may have a wide circle of friends, they are more likely to yield to group pressure and more vulnerable to bullying. At school, they avoid trying new things (for fear of failure) and will give up easily.
Low Self-Esteem in Teenagers
Self-esteem continues to decline during adolescence (particularly for girls). Researchers have explained this decline to body image and other problems associated with puberty.
Although boys and girls report similar levels of self-esteem during childhood, a gender gap emerges by adolescence in that adolescent boys have higher self-esteem than adolescent girls (Robins et al., 2002).
Girls with low self-esteem appear to be more vulnerable to perceptions of the ideal body image perpetuated in western media (through methods such as airbrushing models on magazine covers).
Abraham, T. (1988). Toward a Self-Evaluation Maintenance Model of Social behavior. In L. Berkowitz (Ed), Advances in Experimental Social Psychology (pp. 181–227).Academic Press.
Coopersmith, S. (1967). The Antecedents of Self-esteem . Freeman.
Harter, S. 1993. Causes and Consequences of Low Self-esteem in Children and Adolescents. In Baumeister, R.F. (Ed.) Self-Esteem: The Puzzle of Low Self-regard (pp. 87-116).
Mruk, C. (1995). Self-Esteem: Research, Theory, and Practice . Springer.
Guindon, M. H. (2002). Toward Accountability in the Use of the Self‐Esteem Construct. Journal of Counseling & Development, 80(2) , 204-214.
Robins, R.W., Trzesniewski, K.H., Tracy, J.L., Gosling, S.D., & Potter, J. (2002). Global self-esteem across the lifespan. Psychology and Aging , 17, 423-434.
Rosenberg, M. (1976). Beyond Self-Esteem: The Neglected Issues in Self-concept Research . Paper presented at the annual meetings of the ASA.
Rosenberg, M. (1979). Conceiving the Self . Basic Books.
Rosenberg, M., & Owens, T.J. (2001). Low self-esteem people: A collective portrait. In T.J. Owens. S. Stryker, & N. Goodmanm (Eds.), Extending self-esteem theory and research (pp. 400-436). New York: Cambridge University Press.
Silverstone, P. H., & Salsali, M. (2003). Low self-esteem and psychiatric patients: Part I–The relationship between low self-esteem and psychiatric diagnosis. Annals of General Psychiatry, 2(1) , 2.
Viktor, G. (1982). The Self-Concept. Annual Review of Sociology , 8:1–33.
Viktor, G., & Schwalbe, M.L. (1983). Beyond the Looking-glass Self: Social Structure and Efficacy-Based Self-Esteem. Social Psychology Quarterly , 46:77–88.

Related Articles
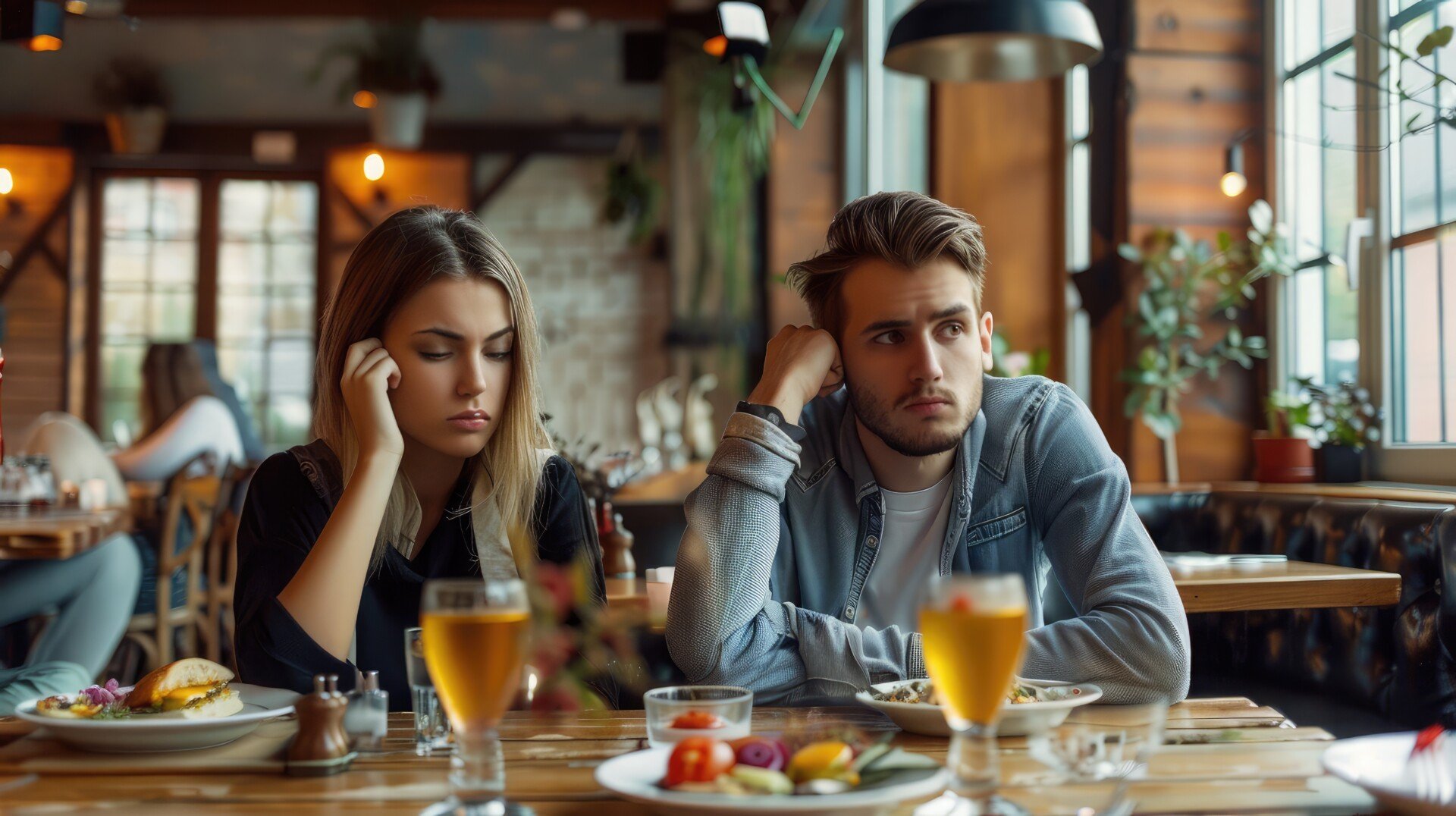
Dating Tips , Relationships
My Boyfriend Is Boring: What Should I Do?
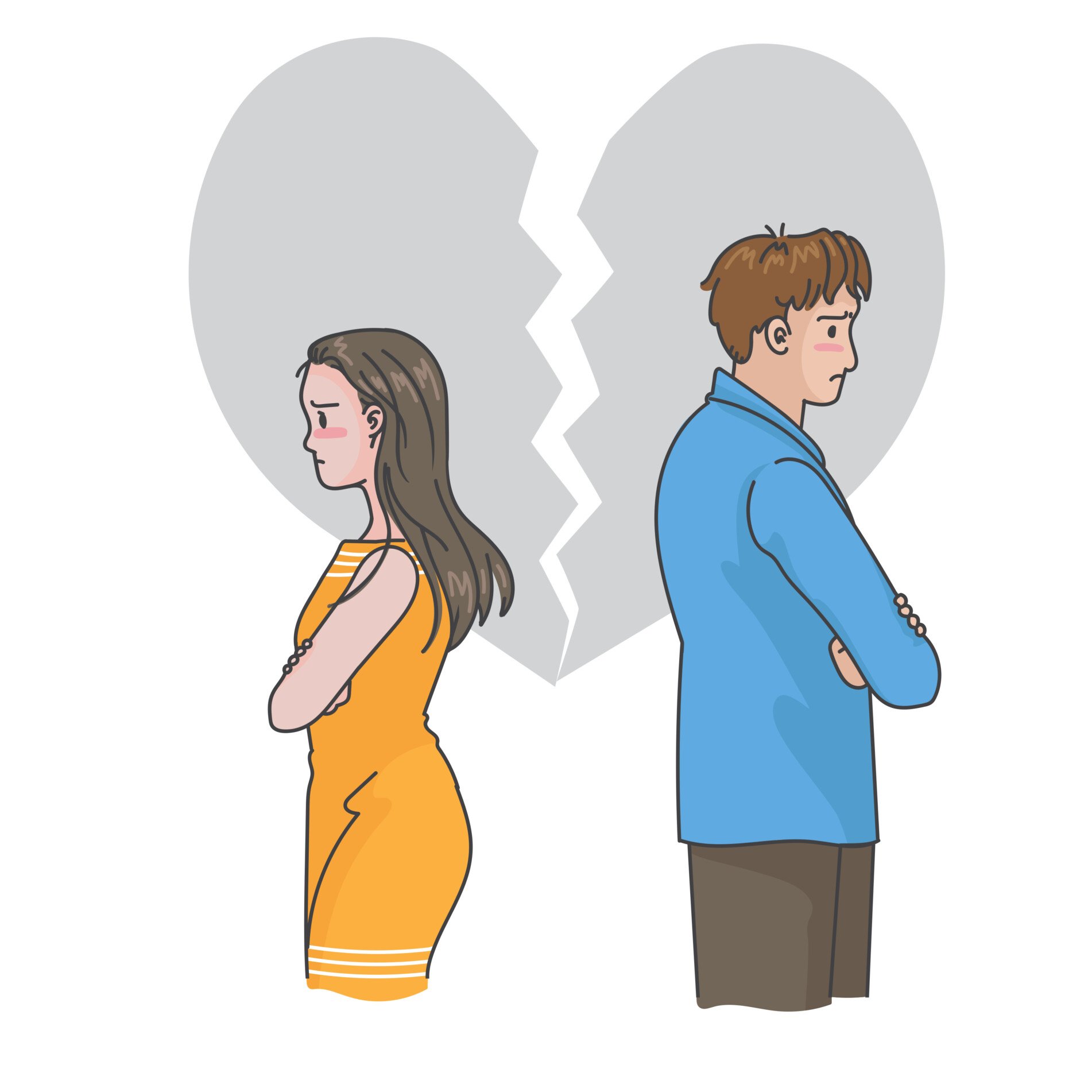
Why Do Relationships Fail?
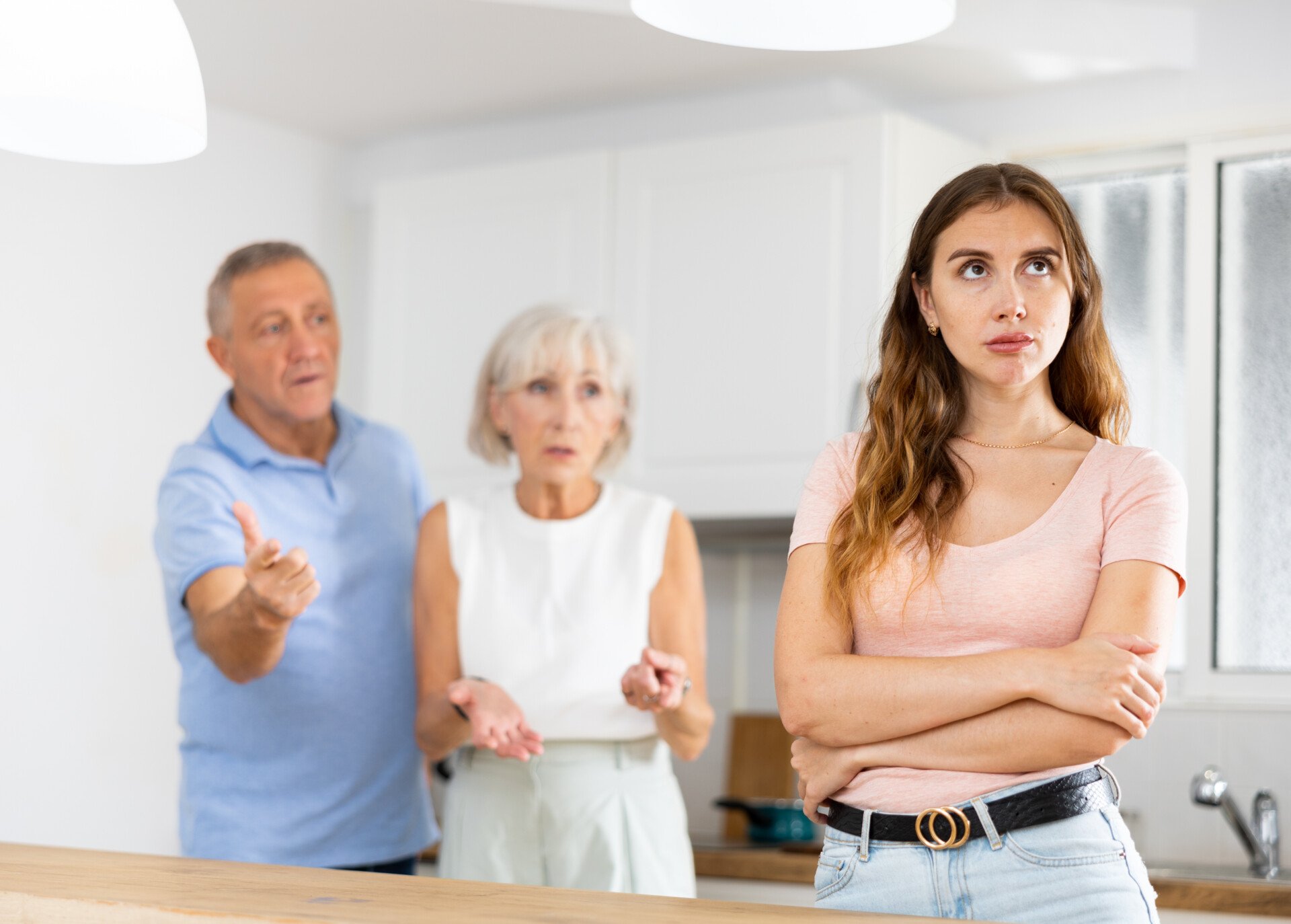
Relationships
Boundaries with Adult Children
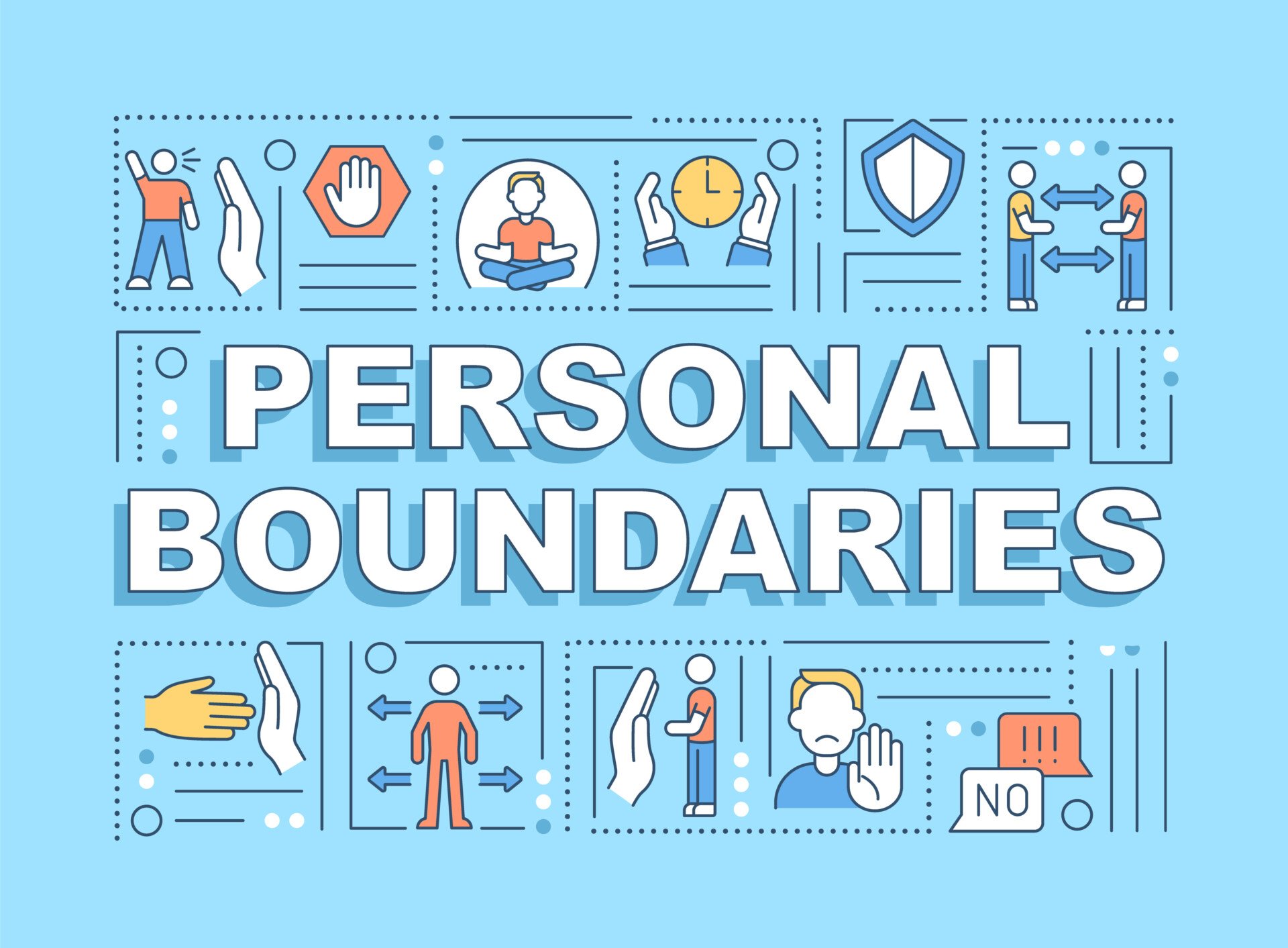
Boundaries vs Expectations
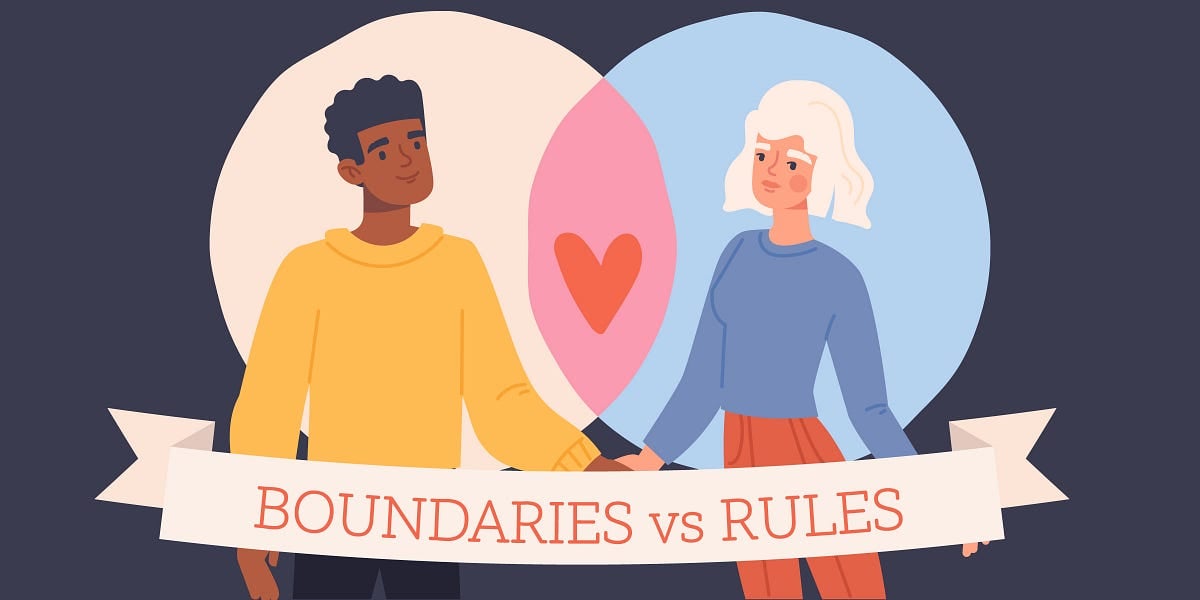
Boundaries vs Rules
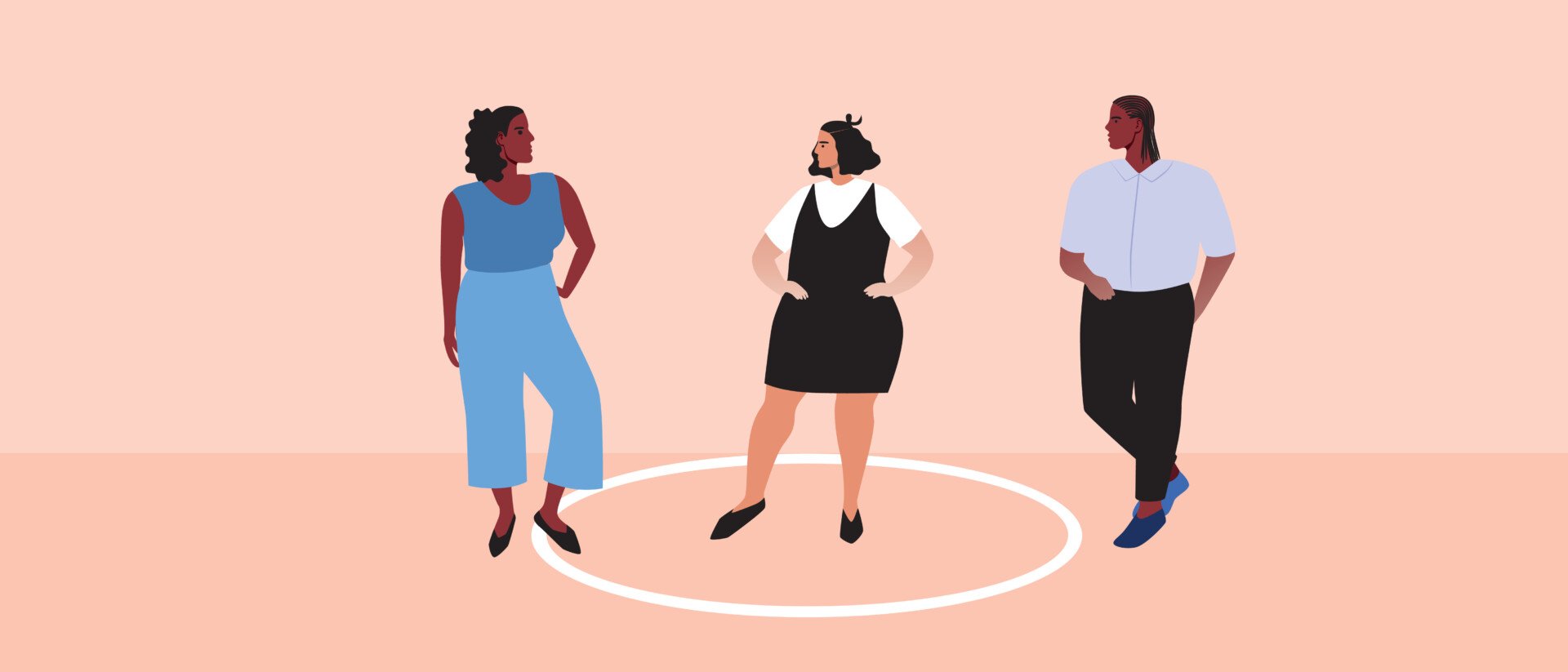
How to Set Boundaries with Parents
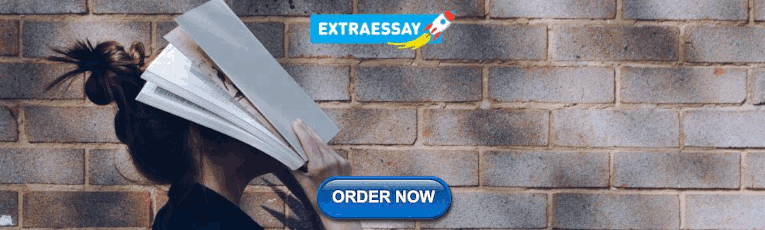
IMAGES
VIDEO
COMMENTS
The experimental method involves manipulating one variable to determine if this causes changes in another variable. This method relies on controlled research methods and random assignment of study subjects to test a hypothesis. For example, researchers may want to learn how different visual patterns may impact our perception.
1. Lab Experiment. A laboratory experiment in psychology is a research method in which the experimenter manipulates one or more independent variables and measures the effects on the dependent variable under controlled conditions. A laboratory experiment is conducted under highly controlled conditions (not necessarily a laboratory) where ...
Journal of Experimental Psychology: General publishes Registered Reports (RRs). Registered Reports require a two-step review process. The first step is the submission of the registration manuscript. This is a partial manuscript that includes hypotheses, rationale for the study, experimental design, and methods.
The Journal of Experimental Psychology: Learning, Memory, and Cognition ® publishes original experimental and theoretical research on human cognition, with a special emphasis on learning, memory, language, and higher cognition.. The journal publishes impactful articles of any length, including literature reviews, meta-analyses, replications, theoretical notes, and commentaries on previously ...
The Journal of Experimental Psychology: Applied ® publishes original empirical investigations in experimental psychology that bridge practical problems and psychological theory.. Review articles may be considered for publication if they contribute significantly to important topics within applied experimental psychology, but the primary focus is on original experimental investigations ...
Experimental psychology refers to work done by those who apply experimental methods to psychological study and the underlying processes. Experimental psychologists employ human participants and animal subjects to study a great many topics, including (among others) sensation, perception, memory, cognition, learning, motivation, emotion; developmental processes, social psychology, and the neural ...
The main components of psychological experimental design include how to reasonably arrange the experimental procedures and how to perform statistical analysis on the experimental data. The main steps can be summarized as follows: (1) formulate hypotheses based on research objectives; (2) develop methods and procedures to test the hypotheses ...
The aim of psychology is to understand the human mind and behavior. In contemporary psychology, the method of choice to accomplish this incredibly complex endeavor is the experiment. This dominance has shaped the whole discipline from the self-concept as an empirical science and its very epistemological and theoretical foundations, via research ...
Introduction. The past decade has been a bruising one for experimental psychology. The publication of a paper by Simmons, Nelson, and Simonsohn (2011) entitled "False-positive psychology" drew attention to problems with the way in which research was often conducted in our field, which meant that many results could not be trusted. Simmons et al. focused on "undisclosed flexibility in data ...
These areas include the study of sensation and perception, learning and memory, motivation, and biological psychology. There are experimental branches in many other areas, however, including child psychology, clinical psychology, educational psychology, and social psychology. Usually the experimental psychologist deals with normal, intact ...
Experimental psychology is not the only theme that uses experimental research and focuses on the traditional core topics of psychology ... (3.91%), as the third most popular method. Mixed-methods research studies (0.98%) occurred across most themes, whereas multi-method research was indicated in only one study and amounted to 0.10% of the ...
Olivia Guy-Evans, MSc. Research methods in psychology are systematic procedures used to observe, describe, predict, and explain behavior and mental processes. They include experiments, surveys, case studies, and naturalistic observations, ensuring data collection is objective and reliable to understand and explain psychological phenomena.
Three types of experimental designs are commonly used: 1. Independent Measures. Independent measures design, also known as between-groups, is an experimental design where different participants are used in each condition of the independent variable. This means that each condition of the experiment includes a different group of participants.
1874 - Wilhelm Wundt published the first experimental psychology textbook, "Grundzüge der physiologischen Psychologie" ("Principles of Physiological Psychology").; 1875 - William James opened a psychology lab in the United States. The lab was created for the purpose of class demonstrations rather than to perform original experimental research. 1879 - The first experimental psychology lab was ...
Experimental research serves as a fundamental scientific method aimed at unraveling. cause-and-effect relationships between variables across various disciplines. This. paper delineates the key ...
Random assignment is a method for assigning participants in a sample to the different conditions, and it is an important element of all experimental research in psychology and other fields too. In its strictest sense, random assignment should meet two criteria. One is that each participant has an equal chance of being assigned to each condition ...
Attention to Emotion. Attention is biased toward negative emotional expressions. Read previous issues of PeePs. Date created: 2014. Particularly Exciting Experiments in Psychology™ (PeePs) is a free summary of ongoing research trends common to six APA journals that focus on experimental psychology.
Objective To investigate whether sleep deprived people are perceived as less healthy, less attractive, and more tired than after a normal night's sleep. Design Experimental study. Setting Sleep laboratory in Stockholm, Sweden. Participants 23 healthy, sleep deprived adults (age 18-31) who were photographed and 65 untrained observers (age 18-61) who rated the photographs. Intervention ...
3. Bobo Doll Experiment Study Conducted by: Dr. Alburt Bandura. Study Conducted between 1961-1963 at Stanford University . Experiment Details: During the early 1960s a great debate began regarding the ways in which genetics, environmental factors, and social learning shaped a child's development. This debate still lingers and is commonly referred to as the Nature vs. Nurture Debate.
Study, experimental, or research design is the backbone of good research. It directs the experiment by orchestrating data collection, defines the statistical analysis of the resultant data, and guides the interpretation of the results. When properly described in the written report of the experiment, it serves as a road map to readers, 1 helping ...
Based on previous studies, the present four experiments (total N = 468) aimed at investigating the effectivity of rumination induction in different experimental settings. We were particularly interested in rumination in the context of individual goal achievement and tested whether an instruction that referred to unresolved goals had a direct observable effect on state rumination.
Experimental psychologists are interested in exploring theoretical questions, often by creating a hypothesis and then setting out to prove or disprove it through experimentation. They study a wide range of behavioral topics among humans and animals, including sensation, perception, attention, memory, cognition and emotion.
The International Journal of Indian Psychȯlogy(ISSN 2348-5396) is an interdisciplinary, peer-reviewed, academic journal that examines the intersection of Psychology, Social sciences, Education, and Home science with IJIP. IJIP is an international electronic journal published in quarterly. All peer-reviewed articles must meet rigorous standards and can represent a broad range of substantive ...
Research has shown key differences between individuals with high and low self-esteem. For example, people with high self-esteem focus on growth and improvement, whereas people with low self-esteem focus on not making mistakes in life. Low self-esteem has been shown to be correlated with several negative outcomes, such as depression (Silverstone ...
Experimental Psychology Assignment (PSY406) In a university, students of BS (Psychology) were divided into two groups for the same course. One group was given the traditional handbook to prepare for the course. The other group was given an interactive textbook having research findings and experiments included in it. After the course ended, final term exams' marks of both groups were compared ...
from Journal of Experimental Psychology: Human Perception and Performance. July 10, 2017. Paw Preference and Temperament in Cats. from Journal of Comparative Psychology. June 29, 2017. Executive Function and Emotion Processing in Attention-Deficit / Hyperactivity and Bipolar Disorders. from Behavioral Neuroscience.
Journal of Experimental Psychology: General. ®. The Role of Political Devotion in Sharing Partisan Misinformation and Resistance to Fact-Checking (PDF, 3.3MB) June 2023. by Clara Pretus, Camila Servin-Barthet, Elizabeth A. Harris, William J. Brady, Oscar Vilarroya, and Jay J. Van Bavel. The Secret to Happiness: Feeling Good or Feeling Right?