
An official website of the United States government
The .gov means it’s official. Federal government websites often end in .gov or .mil. Before sharing sensitive information, make sure you’re on a federal government site.
The site is secure. The https:// ensures that you are connecting to the official website and that any information you provide is encrypted and transmitted securely.
- Publications
- Account settings
Preview improvements coming to the PMC website in October 2024. Learn More or Try it out now .
- Advanced Search
- Journal List
- HHS Author Manuscripts

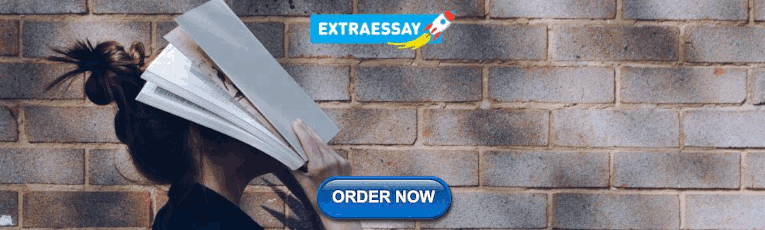
Delivery Timing and Cesarean Delivery Risk in Women with Mild Gestational Diabetes
To evaluate the relationship between gestational age (GA) and induction of labor (IOL) and the rate of cesarean delivery (CD) in women with mild gestational diabetes (GDM).
STUDY DESIGN
Secondary analysis of data from a multi-center RCT of mild GDM treatment. CD rate of women delivering at term (≥ 37 weeks) was evaluated using two complementary approaches: 1) IOL vs. spontaneous labor: women induced at each GA compared with those who spontaneously labored at the same GA, and 2) IOL vs . expectant management: women delivered after IOL at each GA compared with those delivering after spontaneous labor at the same GA or subsequently after spontaneous or induced labor (outcome at each week compared with expectant management at that week). Logistic regression adjusted for potential confounders.
The overall CD rate was 13%. When compared to 39 weeks (either IOL or spontaneous labor) as the referent, there was no significant difference in the CD rate in women delivered at 37, 38, or 40 weeks. However, IOL was associated with a 3-fold increase in CD rate at 41 weeks and beyond as compared with IOL at 39 weeks. Similarly, there was a 3-fold increase in CD rate in women who were induced when compared to those managed expectantly at 40 completed weeks.
CONCLUSIONS
Induction of labor in women with mild gestational diabetes mellitus (GDM) does not increase the rate of cesarean delivery prior to 40 weeks gestation.
INTRODUCTION
The cesarean delivery rate has continued to rise to a recent high of 32%, increasing more than 50% in the last decade 1 . Similarly, the rates of IOL have increased, now affecting 23% of all births 1 . Previous observational studies have suggested that induction predisposes women to CD. Specifically, when comparing women undergoing IOL to those experiencing spontaneous labor, an increased rate of CD has been observed. 2 , 3 However, when compared to expectant management, elective induction of labor at or beyond 41 weeks is associated with a decreased rate of cesarean section. 4 , 5 A recent Cochrane metanalysis suggests that elective induction of labor at term, when compared with expectant management, is associated with a decreased rate of cesarean section as well as other adverse perinatal outcomes. 6
The discrepancies in the findings of these are partially explained by differences in comparison groups. While initial studies compared induced with spontaneous labor, more recent studies have used an expectant management comparison group, which includes patients who spontaneously delivered as well as those who were induced at later gestational ages. This comparison is more appropriate in clinical decision-making since expectant management, not spontaneous labor, at a particular gestational age is the only alternative.
The MFMU Network randomized controlled trial (RCT) for the treatment of mild GDM demonstrated that treatment of mild gestational diabetes (GDM) is associated with a lower rate of cesarean delivery (26.9% vs. 33.8%, p = 0.02). 7 This reduced risk was apparent even after excluding cesarean indications such as malpresentation, placenta previa, oligohydramnios, and previous cesarean. A high proportion (27%) of patients underwent induction in each group.
The primary aim of this study was to compare the rate of CD by gestational age and by IOL vs. expectant management in women with GDM. As a secondary aim, we compared perinatal outcomes among the study groups.
METHODS AND MATERIALS
We performed a secondary analysis of a multicenter randomized trial evaluating the effect of treatment in women with mild GDM. 7 In the original trial, women at 24–30 weeks gestation with blood glucose levels of 135–199 mg/dL following a 50 gram glucose loading test were invited to enroll. Eligible women underwent a blinded 3-hour 100 gram oral glucose tolerance test. Mild GDM was defined as fasting glucose levels of less than 95 mg/dL and at least two abnormal timed measurements (>180 mg/dL at 1 hour, >155 mg/dL at 2 hours, and >140 mg/dL at 3 hours). Women with mild GDM were randomized to usual prenatal care or treatment with dietary modifications, glucose monitoring, and insulin, if indicated. Providers and patients were blinded to GDM diagnosis in the standard care group. The original study was approved by the institutional review boards of all participating clinical centers, and all enrolled women gave informed consent.
Women in both the treatment and standard care group were included in this secondary analysis if they delivered at term (≥37 weeks), underwent induced or spontaneous labor, and had cephalic presentations. Patients were excluded from analysis if they had an elective scheduled CD, had one or more previous CDs, had a non-cephalic presentation, or a major fetal anomaly. The primary outcome was CD. The CD rate was assessed by two approaches: 1) patients delivered at a specific GA (in completed weeks) and type of labor were compared to those who delivered at 39 weeks; 2) patients undergoing IOL at a specific GA were compared with those “expectantly managed”, which included patients who delivered at the same GA following spontaneous labor and patients who delivered at subsequent GA following spontaneous or induced labor. We examined several secondary outcomes. First, the composite perinatal outcome, included any one of the following: hypoglycemia, hyperbilirubinemia, RDS, and birth trauma which have been associated with GDM. We also assessed the frequency of admission of the infant to the neonatal intensive care unit (NICU), of birthweight >4000g, and of large-for-gestational age (LGA) infants, defined as birthweight > 90 th percentile. 8
Categorical variables were analyzed using the chi square or Fisher’s exact test. Continuous variables were analyzed using the Wilcoxon rank sum or the Kruskal-Wallis test. Study outcomes by completed GA week were calculated by type of labor for the two comparison methods. Multivariable logistic regression analysis was used to adjust for potential confounders for most outcomes including maternal age, race/ethnicity, pre-pregnancy body mass index (BMI), parity, smoking status, Cesarean delivery (for neonatal outcomes), infant sex (for neonatal outcomes), timing of dating ultrasound (trimester), SGA, and treatment group. No adjustments were made for the outcome of NICU admissions due to to small numbers. Adjusted odds ratios (OR) and 95% confidence intervals (CI) were estimated relative to the IOL group at 39 weeks in method 1 and the expectant management group at each GA in method 2.
Statistical analyses were conducted using SAS software (SAS Institute, Cary, NC). A nominal two-sided p-value < 0.05 was considered for statistical significance with no adjustments made for multiple comparisons.
Of the 958 women enrolled in the original RCT, 679 met inclusion and exclusion criteria for this secondary analysis. Baseline characteristics of the study population are shown in Table I . Reflective of the original study population, over half of the women were Hispanic, and the mean BMI at enrollment was approximately 30 (± 5.0) kg/m 2 . Race/ethnicity and GDM screen results differed by completed GA at delivery.
Characteristics of the study population *
The overall rate of CD was 13%. The indications for cesarean section were: failed induction (69.3%), non-reassuring fetal tracing (26.1%), cord prolapse (3.4%), and chorioamnionitis (1.2%). The crude and adjusted results for CD are presented in Table II . Of note, the results were adjusted for maternal age, ethnicity, BMI, parity, smoking, timing of dating ultrasound, SGA status, and assigned control vs. treatment group. The CD incidence increased from 10.3% to 22.7% as gestational age at delivery increased from 37 to 41 completed weeks. This pattern was primarily restricted to the IOL group. When comparing the rate of CD at each GA with 39 completed weeks as the referent (method 1), there was no significant difference in the adjusted CD delivery rate at 37, 38, and 40 completed weeks. However, at 41 weeks, IOL was associated with a 3-fold increase in CD as compared with IOL at 39 weeks. Likewise, when comparing women undergoing IOL vs. those expectantly managed (method 2), IOL was not associated with an increased adjusted rate of CD at 37, 38, or 39 completed weeks. At 40 completed weeks, IOL was associated with a 3-fold increased in CD rate. There was a similar increase in CD rate at 41 completed weeks and greater, but this association was not statistically significant. The CD risks when stratified by treatment group are shown in Tables IIIA and IIIB . In the treated group, based on small numbers, there was a trend toward a reduction in CD risk associated with IOL prior to 39 weeks the OR (95% CI) with IOL when compared to those expectantly managed was 1.97 (0.39, 9.95) at 40 and 3.94(0.10, 158.6) at ≥41 weeks ( Table IIIB ). However, these comparisons were not statistically significant. In the standard care group, the OR (95% CI) for IOL compared to the expectant management group was 3.07 (1.05, 8.96) at 40 weeks and 3.76 (0.69, 20.58) at ≥41 weeks; there were no increases at 37, 38 or 39 weeks ( Table IIIA ).
CD rates according to GA for IOL or spontaneous labor compared to 39 weeks or induction vs. expectant management.
CD rates according to GA for IOL or spontaneous labor compared to 39 weeks or induction vs. expectant management in the standard care group.
CD rates according to GA for IOL or spontaneous labor compared to 39 weeks or induction vs. expectant management in the treated group.
The frequency and adjusted odds of the composite perinatal outcome were not different in the IOL group when compared to either the spontaneous labor (method 1) or expectant management group (method 2) at all GAs ( Table IV ). There were no cases of stillbirth or neonatal deaths in this study. Furthermore, there were no significant differences in the unadjusted frequency of NICU admissions between the groups with the exception of an increase in NICU admissions in the IOL group at ≥ 41 weeks (there were no NICU admissions in the ≥ 41 week expectant management group; data not shown). There was a significant increase in the frequency of infants with birthweight > 4000g at ≥ 41 weeks when compared to 39 weeks (OR of 2.83 with 95% CI of 1.31–6.14) in all deliveries (data not shown). However, there were no significant differences in the frequency of either infants with birthweight > 4000g or LGA neonates in the IOL vs. expectant management groups at all gestational ages (data not shown).
Composite perinatal outcome * according to GA for induction or spontaneous labor compared to 39 weeks or induction vs. expectant management.
We found an increasing trend in cesarean delivery risk with increasing gestational age at term. When compared to expectant management, IOL in patients with mild GDM was not associated with an increase in CD rate until 40 weeks. When stratified for treatment group (standard care and treated groups), results (limited by numbers), were similar to the overall findings; the increase in CD risk from 40 weeks appeared to be more prominent among those who were not treated. Furthermore, we found no significant differences in perinatal outcomes between patients undergoing IOL compared to either those spontaneously laboring or those expectantly managed. No significant differences were also observed for NICU admissions, birthweight > 4000, or LGA neonates.
A recent study indicates that IOL at 39 in women with GDM confers a decrease in perinatal mortality. 9 Our results are mostly consistent with a previous cohort study of term deliveries that showed no difference in CD rate when IOL was compared to expectant management at all gestational ages. Similarly, a randomized controlled trial by Boulvain and colleagues showed no increase in CD rate in non-diabetic women undergoing IOL with suspected fetal macrosomia. 10
Our findings are in line with metanalyses that showed no increase in the CD rate in women undergoing elective IOL at term. 5 , 6 In our study, there were no differences in CD rates in women undergoing IOL vs. expectant management prior to 40 weeks. However, IOL was associated with an increase in CD rate from 40 weeks. As the population in the current study was restricted to women with mild GDM and included women undergoing both elective and indicated IOL, our findings could potentially be attributed to the higher risk of macrosomia, labor dystocia, and fetal heart rate abnormalities in gestational diabetics leading to higher CD rate with advancing gestation.
Previous studies have differed in the composition of the expectant management group. For example, Caughey et al. defined the expectant management group as women delivering, after either spontaneous or induced labor, during the week following the induction group. 11 This approach may favor the induction group because it excludes women who spontaneously labor in the same week from the expectant management group. In contrast, Glantz included women who delivered following spontaneous labor the same week as the induction group in the expectant management group. 12 In contrast to the current study, that analysis demonstrated a small but significant increased risk of CD associated with labor induction. 12 We chose to define the expectant management group as women who delivered at the same GA following spontaneous labor and patients who delivered at subsequent GA following spontaneous or induced labor. These differences may account for some of the variations in findings among these studies. Additionally, given the limitations of the precision of dating, some of these differences may be affected by variability in the assignment of gestational age.
Although we simulate IOL vs. expectant management, prospective management regarding timing of delivery was not a focus of the primary trial. Therefore, this secondary analysis does not fully capture prospective management intent. Additionally, while adjustment for confounders such as parity did not alter the results, we do not have data on other factors, such as cervical status, that may affect these findings. We also did not take into account the type of gestational diabetes given that few women required medication. Our population is made up of only women with mild GDM, limiting the generalizability of findings to all patients with GDM.
The overall CD rate in this selected population was relatively low at 13%. The CD rate in original trial was 30%, and the population selected for the current study excluded women with scheduled CDs, prior CDs, and non-cephalic presentations. It is not clear how a more liberal use of CD in this population would affect our findings. Over half of the patients in this study were Hispanic, reflective of the increased risk of GDM associated with Hispanic ethnicity. Finally, as this was a retrospective analysis of an existing trial, our sample size likely did not provide sufficient power to account for all possible differences in comparison groups.
As gestation progresses, particularly in pregnancies complicated by diabetes or other co-morbidities, the risks of adverse perinatal outcomes such as stillbirth, fetal heart rate abnormalities, macrosomia, and labor dystocia increases. 13 , 14 The current study was limited in that there were no stillbirths in this group, which is explained by the relatively small sample size of women with only mild GDM.
Taken together, these data support the hypothesis that a policy of induction prior to 40 weeks in women with GDM may not increase the rate of cesarean delivery. Considered collectively with the studies associating decreasing adverse pregnancy outcomes with increasing gestational age, our findings suggest that delivery prior to 40 weeks, perhaps as early as 38 weeks, may improve CD risk in women with mild GDM. This should be weighed against the impact on other important perinatal outcomes. Therefore, a large, randomized controlled trial is warranted to further evaluate the maternal and neonatal risks and benefits of IOL at term and, thus, to determine the optimal gestational age of delivery in women with GDM.
CLINICAL IMPLICATIONS
- Induction of labor, compared to expectant management, prior to 40 weeks does not increase cesarean delivery risk in women with mild gestational diabetes mellitus (GDM).
- Large randomized controlled trials are warranted to fully evaluate the maternal and neonatal risks associated with induction of labor and to determine the optimal gestational age of delivery in women with mild GDM.
Acknowledgments
The project described was supported by grants from the Eunice Kennedy Shriver National Institute of Child Health and Human Development (NICHD) [HD27915, HD34116, HD40485, HD34208, HD27869, HD40500, HD40560, HD34136, HD40544, HD27860, HD40545, HD53097, HD21410, HD27917, HD40512, HD53118, HD36801], General Clinical Research Centers Grant [M01-RR00034] and the National Center for Research Resources [UL1-RR024989, M01-RR00080, UL1-RR025764, C06-RR11234] and does not necessarily represent the official views of the NICHD or NIH.
The authors would like to thank Alan T. N. Tita, M.D., Ph.D. for guidance in study design, data analysis, and manuscript preparation; Dr. John C. Hauth for assistance in study design; Francee Johnson, R.N., B.S.N. and Jo-Ann Tillinghast, R.N., M.S.N. for protocol development and coordination between clinical research centers; Elizabeth Thom, Ph.D., for protocol development/data management and statistical analysis; and Marshall W. Carpenter, M.D., and Catherine Y. Spong, M.D. for protocol development and oversight.
In addition to the authors, other members of the Eunice Kennedy Shriver National Institute of Child Health and Human Development Maternal-Fetal Medicine Units Network are as follows:
University of Texas Southwestern Medical Center — K. Leveno, L. Moseley, J. Gold, D. Bradford, L. Fay, M. Garcia, F. Capellan
Columbia University — R. Wapner, M. Miodovnik, F. Malone, S. Bousleiman, H. Husami, V. Carmona, N. Fredericks, E. Gantioqui, B. Greenspan, M. Williams
University of Utah — K. Anderson (University of Utah Health Sciences Center), P. Ashby (University of Utah Health Sciences Center), S. McAllister (University of Utah Health Sciences Center), S. Quinn (LDS Hospital), A. Guzman (McKay-Dee Hospital), F. Castinella (LDS Hospital), J. Steiner (McKay-Dee Hospital), J. Parker (Utah Valley Regional Medical Center)
University of Alabama at Birmingham — J. Sheppard, J. Tisdale, A. Northen, D. Rouse, W. Andrews
Brown University — M. Carpenter, D. Catlow, D. Allard, M. Seebeck, J. Tillinghast
The Ohio State University — J. Iams, F. Johnson, C. Latimer, E. Weinandy, B. Maselli
University of North Carolina at Chapel Hill — K. Dorman, S. Brody, S. Timlin, J. Bernhardt
Drexel University — M. Hoffman, E. Guzman, M. Talucci, T. Grossman, C. Perez, L. Zeghibe, P. Tabangin
Case Western Reserve University-MetroHealth Medical Center — B. Mercer, B. Stetzer, C. Milluzzi, W. Dalton, S. Pichette
Wake Forest University Health Sciences — M. Swain, P. Meis, J. White
The University of Texas Health Science Center at Houston — L. Gilstrap, K. Cannon, J. Martinez, D. Dusek
University of Texas Medical Branch — J. Moss, J. Brandon, A. Jackson, G. Hankins, D. Sharp
University of Pittsburgh — M. Bickus, H. Birkland, M. Cotroneo, N. Cuddy
Wayne State University — G. Norman, P. Lockhart, S. Blackwell, L. Quast
Northwestern University — P. Simon, G. Mallett
Oregon Health & Science University — J. Tolosa, L. Davis, E. Lairson, C. Cromett, C. Naze, M. Blaser
The George Washington University Biostatistics Center — E. Thom, J. Zachary, B. Getachew, C. Cobb, L. Leuchtenburg, S. Gilbert
Eunice Kennedy Shriver National Institute of Child Health and Human Development — C. Spong, S. Tolivaisa, K. Howell
The MFMU Network Steering Committee Chair (University of Texas Medical Branch, Galveston, TX) — G.D. Anderson, M.D.
Disclosure: The authors report no conflict of interest.
Presented in part at the 32 st Annual Meeting of the Society for Maternal-Fetal Medicine in Dallas, Texas February 6–11, 2012.
Publisher's Disclaimer: This is a PDF file of an unedited manuscript that has been accepted for publication. As a service to our customers we are providing this early version of the manuscript. The manuscript will undergo copyediting, typesetting, and review of the resulting proof before it is published in its final citable form. Please note that during the production process errors may be discovered which could affect the content, and all legal disclaimers that apply to the journal pertain.
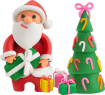
Get additional 3 months free and save upto 40%
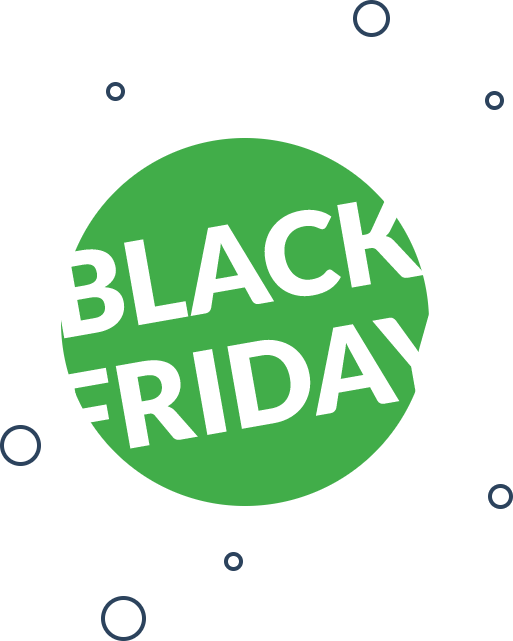
Don't miss out on our holiday offers
Get 25% off + additional 3 months free*, *for annual plans.
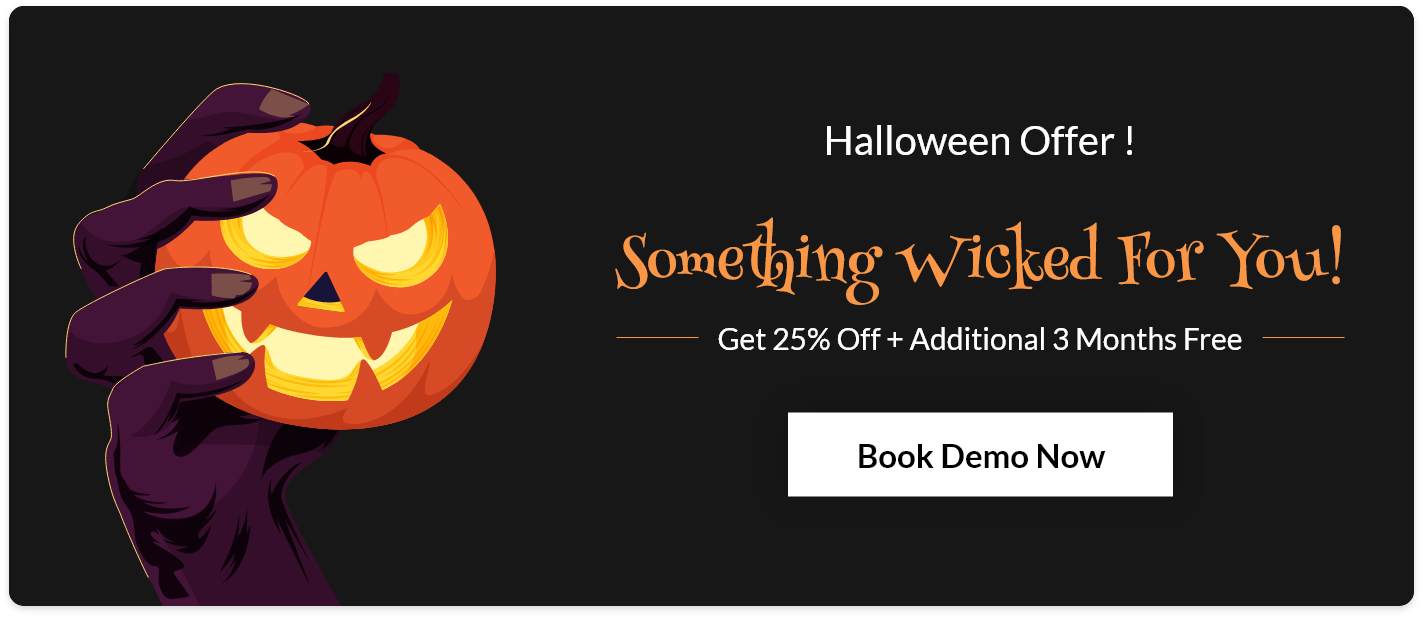
Top Ways in Which Data Analytics Is Transforming Delivery Management Business
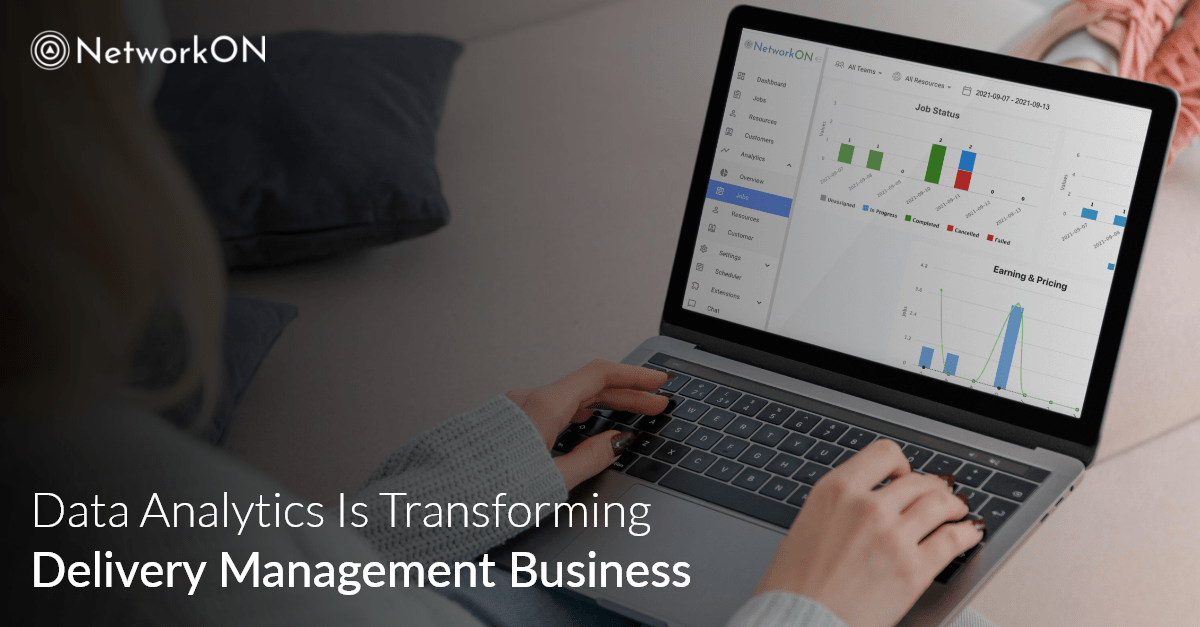
Data analytics is transforming every aspect of business across different industries, and a delivery management business is no exception. The dynamic nature of the supply chain business makes logistics the perfect use case for data.
A delivery management business consists of an intricate structure, and you can obtain valuable insights by leveraging data scattered across the business. This data helps solve a variety of business issues like streamlining operations, optimizing routes, and establishing more transparency throughout the supply chain.
Historically, the logistics industry has remained largely fragmented when it comes to processing complex data. But adopting data culture and putting effort into understanding advanced data analytics can be a boon for the logistics industry as a whole.
So, how does data analytics help? With the arrival of big data usage, businesses have transformed how they used to deal with analytics. Today, many logistics companies are using Shipping data analytics to anticipate peak functioning periods of their business, assess future risks, identify opportunities and manage potential supply disruptions proactively.
According to research by Global Trade , around 93% of shippers and 98% of third-party logistics companies believe that data analytics is crucial when making intelligent business decisions. In fact, 71% of them believe that data analytics adds greatly to the quality and performance of their business.
Logistics companies have been under immense pressure ever since the COVID-19 pandemic hit our lives. It has encouraged many companies to pair up their business operations with powerful delivery data analytics.
As life goes back to normal in the coming months, we can expect to see smarter and faster logistics networks, handling a multitude of challenges thrown at them with ease. However, they will continue to receive millions of daily orders globally and will only be able to sustain their business if they work with data.
How Data Analytics is Transforming Delivery Management Businesses
But all of the said changes cannot happen overnight. Logistics companies have spent a lot of time and money to optimize their network efficiencies. Setting up new infrastructure, upgrading their technology stack, and most importantly, implementing shipping analytics software to the logistics side of things.
Let’s find out how delivery analytics is helping logistics companies across the globe as they modernize their technology stack for present-day operations. Here are a few prominent areas that are being optimized with advanced data analytics:
- Managing Performance
It is crucial to understand and resolve inefficiencies across different metrics across the entire supply chain. Performance management teams can achieve actionable results using data insights at their disposal.
Data can help managers in monitoring the performance of their entire workforce in concrete numbers. It can also shed light on the reasons behind poor performance; these reasons often include poor routes, excessive resource utilization, improper docking schedules, and breakdowns in machinery, to name a few.
Once they know the reasons, managers can intervene quickly and improve the efficiency of in-house teams and other network partners.
- Improving Order Processing Capabilities
With efficient data integration into the operational processes, you can make space for additional orders in the system. As a result, you can cater to more orders and drive the demand for your services across the supply chain.
In a nutshell, delivery analytics improves the order processing capabilities of your organization, exposes any ongoing problems in the supply chain, and allows the concerned teams to fix the issues faster.
- Increasing Productivity
Data analytics can be beneficial for the company and its supply chain partners. Real-time data sharing with everyone is of prime importance and by enabling the same, you can increase the operational efficiency of your logistics business.
You can capture data from different sources like fluctuating customer demand, performance data of delivery partners, data on operational efficiency, and other external factors to improve transparency to all stakeholders.
It will also help you improve the overall performance of the operations teams and enable them to work towards increasing the efficiency of different processes.
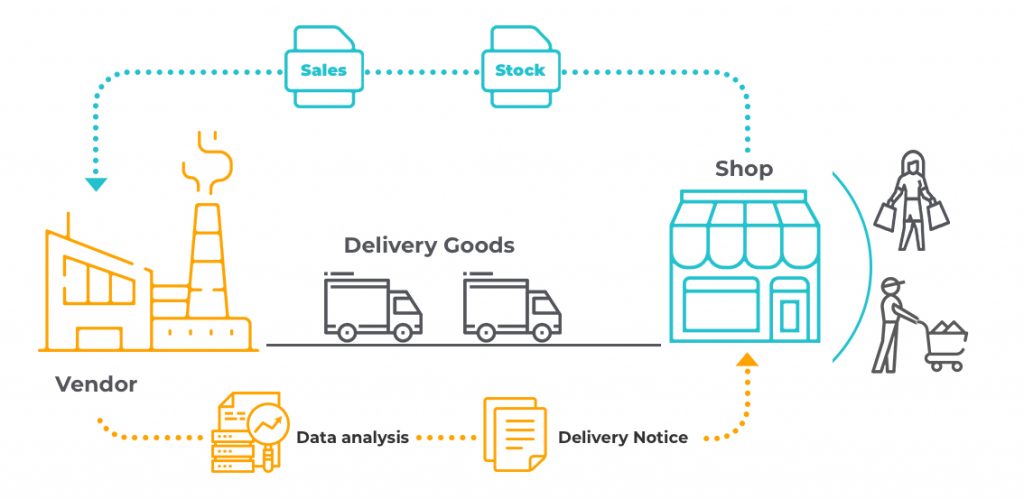
- Improving Forecasting
Monitoring the teams’ performance on an ongoing basis helps you identify inefficiencies in real-time. As soon as their performance data display any red flags or errors, you can jump into action and take the necessary measures to mitigate or eliminate them.
Some prominent errors include delays in picking procedures, lack of items in shipping, and errors in picking rates. Managers can utilize the information and intervene accordingly. They can also use predictive analytics to anticipate the demand for a product at a specific time based on historical data. They can also check different metrics and KPIs to determine any upcoming inventory shortages and reduce operational costs.
- Route Optimization
Implementing robust route optimization features like real-time GPS data, delivery partner schedules, road maintenance information, fleet location, and access to historical data, your delivery partners can access optimized routes at a specific time of delivery.
Logistics companies want to use data to improve their delivery speed and offer real-time visibility of orders to customers. On the other hand, customers expect that their orders will be delivered in time.
Hence, you need to analyze data to improve each aspect of your logistics operations to create lasting customer relationships.
- Digitization of Crucial Processes
Whether it is routing, pricing, or load shipment consolidation, advanced delivery analytics can help you optimize all these operations and more. Digitization also provides customers with a hassle-free experience and improves the visibility of your internal operations.
When automation is applied to the core of a business, it simplifies labor-intensive operations and makes the process more efficient.
Wrapping Up
The logistics industry is constantly evolving and is adapting to a new digital environment. The only way to use new technology properly is to have every form of data at your fingertips and use it to optimize different technologies in your stack.
Not sure how you can implement the aforementioned points into your logistics business? Why not invest in an AI-powered delivery management software that automates your business’ logistics side of things.
NetworkON offers outstanding features like AI-powered route optimization, digital proof of delivery, fleet visibility and management, geofencing, automatic order assignment, performance analytics, and access to various third-party integrations.
To know more about NetworkON and how it can create a significant impact on your business, write to us at [email protected] or visit our website . Read our blogs and learn more about the importance of delivery management for your business.
How Route Optimization Reduces Cost, Increases ROI, and Builds Brand Loyalty
Leave a Reply Cancel reply
Your email address will not be published. Required fields are marked *
Save my name, email, and website in this browser for the next time I comment.
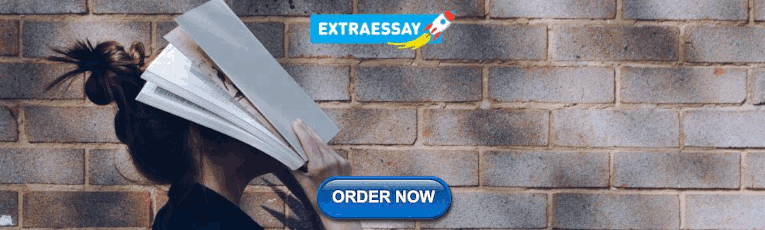
Popular Posts
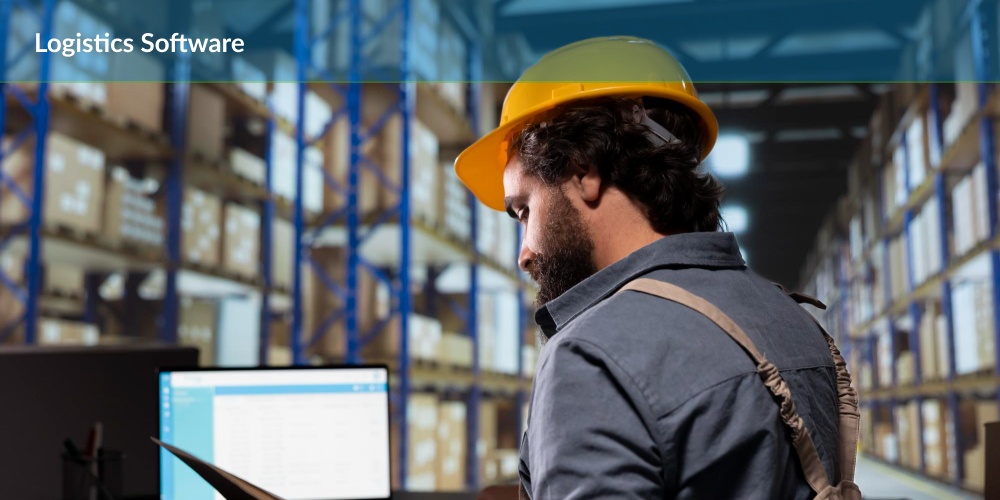
Revolutionizing Logistics Management with Cutting-Edge Software Solution
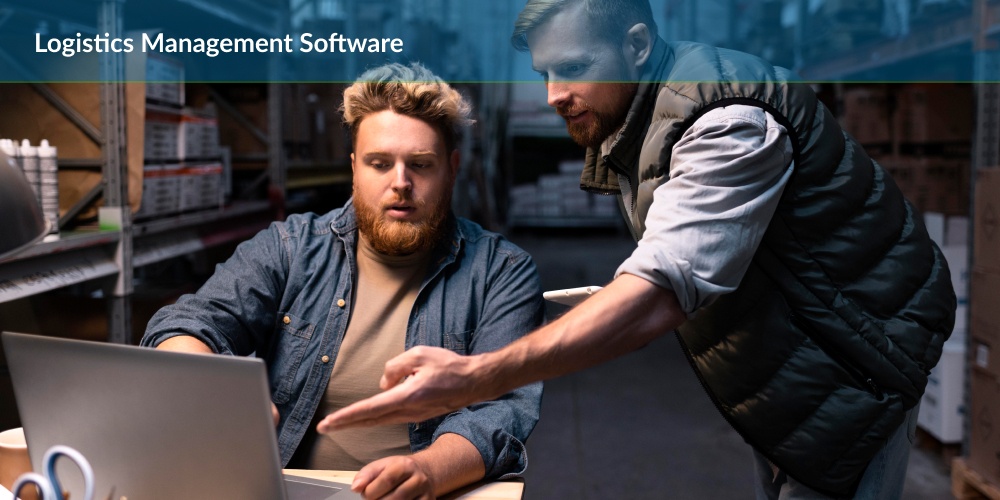
Streamline Your Supply Chain: The Power of Logistics Management Software
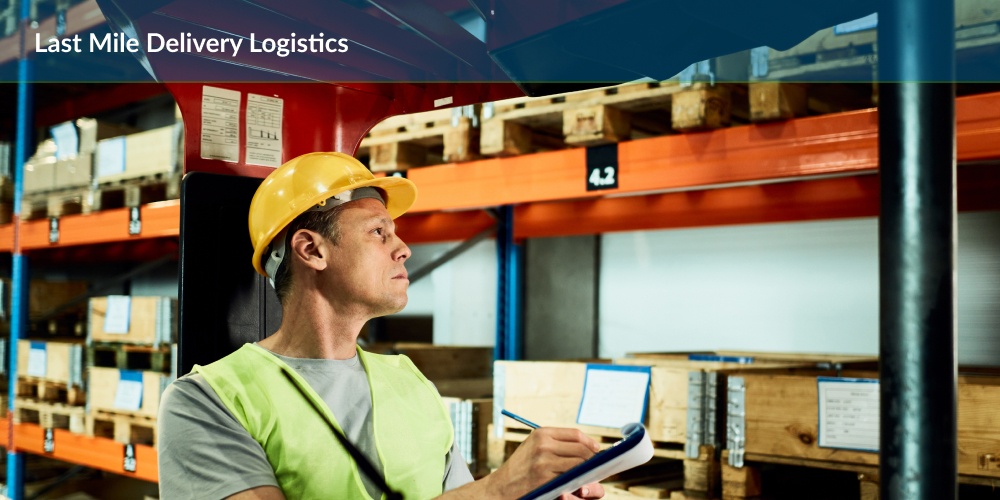
Determinants of Home Delivery among Mothers in Abobo District, Gambella Region, Ethiopia: A Case Control Study
Affiliations.
- 1 Gambella Regional Health Bureau, Gambella Region, Ethiopia.
- 2 Department of Epidemiology, Jimma University, Jimma, Oromia, Ethiopia.
- PMID: 33490230
- PMCID: PMC7787860
- DOI: 10.1155/2020/8856576
Introduction: Home delivery is one of the major reasons for high maternal mortality ratio in sub-Saharan Africa. Sub-Saharan Africa and South Asia together contribute over 85% of maternal deaths, of which, only half of deliveries are institutional. However, data are scarce on the availability of information with regard to the determinant factors for this high prevalence of home delivery in the study area.
Objective: This study is aimed at determining factors associated with home delivery, among mothers in Abobo Woreda, Gambella region, Southwest Ethiopia, 2019.
Methods: A case control study conducted from 12 March 2019 up to 2 April 2019 on 88 cases and 176 controls. Cases include mothers who gave birth at home and those mothers who gave birth at health facility in the last one year preceding the study included as controls. Data entry was made using Epi-Data version 3.1, and analysis was made using SPSS version 20. A binary logistic regression analysis was conducted to assess candidate variables and subsequently a multivariable regression to determine the statistical associations. Adjusted odds ratio (AOR) with 95% confidence interval (CI) was calculated to determine strength of association, and p value <0.05 was used to establish significant associations.
Results: No formal education (AOR: 5.07; 95% CI: 2.18-11.50), poor knowledge on obstetric complications (AOR: 3.83; 95% CI: 1.98-7.40), negative attitude towards delivery service (AOR: 3.25; 95% CI: 1.70-6.19), poor household wealth index (AOR: 4.55; 95% CI: 2.01-10.31), and no antenatal care visit (AOR: 3.29; 95% CI: 1.63-6.63) were found to be significantly associated with home delivery.
Conclusions: The findings do support that no formal education, poor knowledge on obstetric complications, negative attitude towards delivery service, poor household wealth index, and no antenatal care visit showed a significant association with home delivery.
Copyright © 2020 Asmelash Abera Mitiku et al.
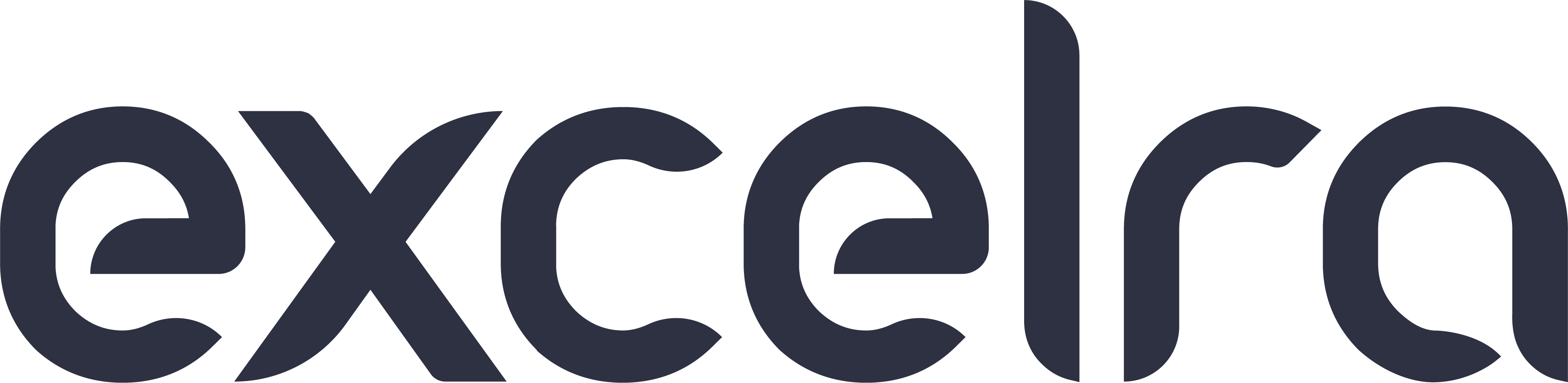
- Bioinformatics
- Biomarker Discovery
- Cheminformatics
- Clinical Pharmacology
- ELN and LIMS
- Curation services
- Clinical data services
- Semantics data services
- Data science
- Data visualization
- Product design and development
- Cloud enablement
- Data engineering
- ELN and LIMS consulting
- – For Pharma & Biotech
- – For AI/ML
- – For Academia
- Therapeutic Large Molecule Curation
- Custom Biomarker Knowledgebase
- Online Pipeline Platform (OP 2 )
- Single Cell Browser
- ImmunoRaptor Platform
- BioVisualizer
- Case studies
- Publications
- Whitepapers
- Leadership Team
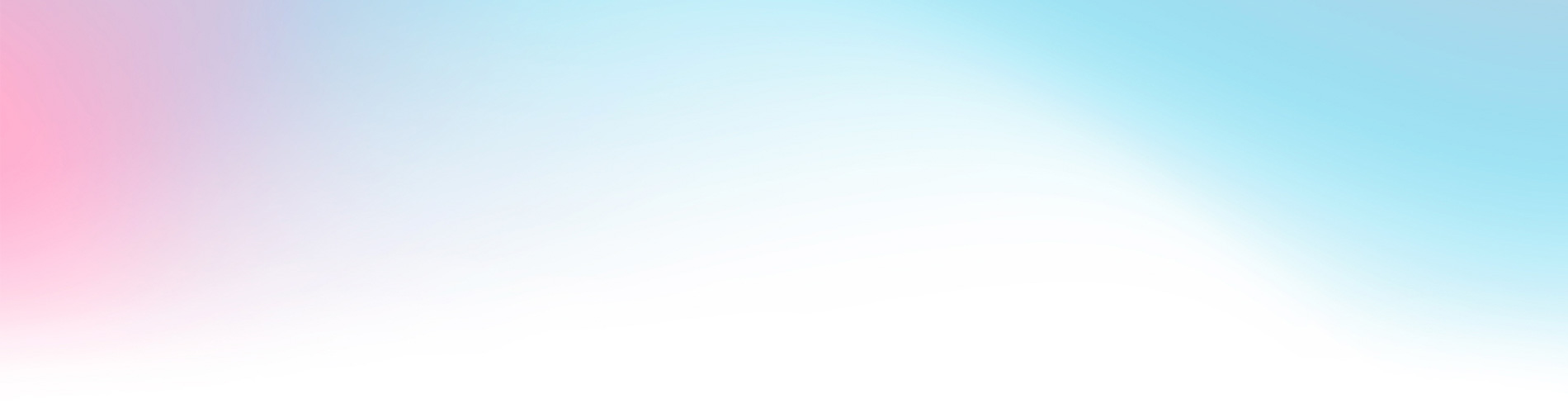
Biomarker Landscape Analysis: Unveiling Challenges and Opportunities
Authors: Sidharth Shankar Jha
Introduction
Biomarkers are indicators of normal physiological and pathogenic processes, and/or responses to therapeutic interventions. They are fundamentally required in drug development, facilitating response monitoring, dose optimization, and for identifying variations in drug response among patients. In general, biomarkers are of vast significance to biopharma, diagnostics, personalized medicine, and overall healthcare industry. Marketresearch.biz predicts that the biomarkers market valued at USD 58.6 billion in 2022 is expected to register a Compound Annual Growth Rate (CAGR) of 14.3% during the forecast period between 2023 to 2032. Biomarkers can be classified based on their nature, functional application. The BEST ( B iomarkers, E ndpoint S , and other T ools) resource categorizes biomarkers into seven types as summarized in Figure 1.
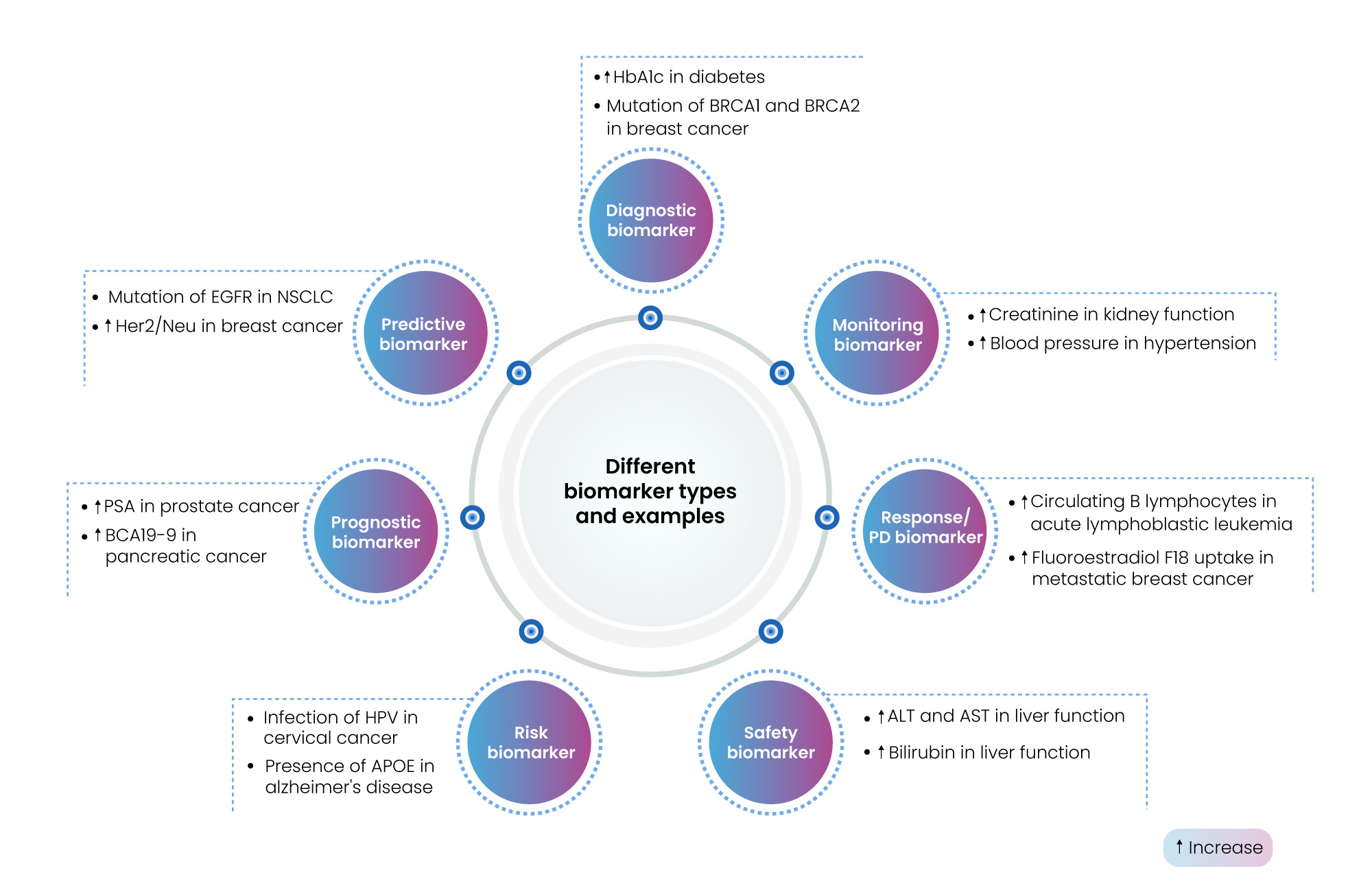
Figure 1: Different Biomarker types and examples
Biomarker landscape assessment is a comprehensive overview that identifies, analyses, and categorizes biomarkers in disease or drug development, focusing on their specificity, sensitivity, and utility. It is pivotal in healthcare and translational research. In the latter, the process validates biomarkers identified in preclinical studies for clinical relevance, aiding in the development of diagnostic tools, prognostic indicators, and safe and efficacious therapeutic interventions. Biomarkers play essential roles in various aspects of drug discovery and development like in validation of potential drug targets, and assessment of the mechanism of action (MoA). It plays a crucial role in clinical trials by facilitating patient stratification, endpoint selection, and treatment monitoring, thereby enhancing trial efficiency and success rates. The systematic assessment of the biomarker landscape is pivotal for the progression of personalized medicine. It involves identifying and understanding biomarkers linked to specific disease subtypes and therapeutic interventions. Moreover, biomarkers help to optimize patient outcomes while concurrently mitigating adverse effects.
Major challenges in biomarker landscape assessment:
Biomarker landscape assessment for a drug, a disease, or a target is associated with multiple challenges. Some of the major challenges are listed in Figure 2.
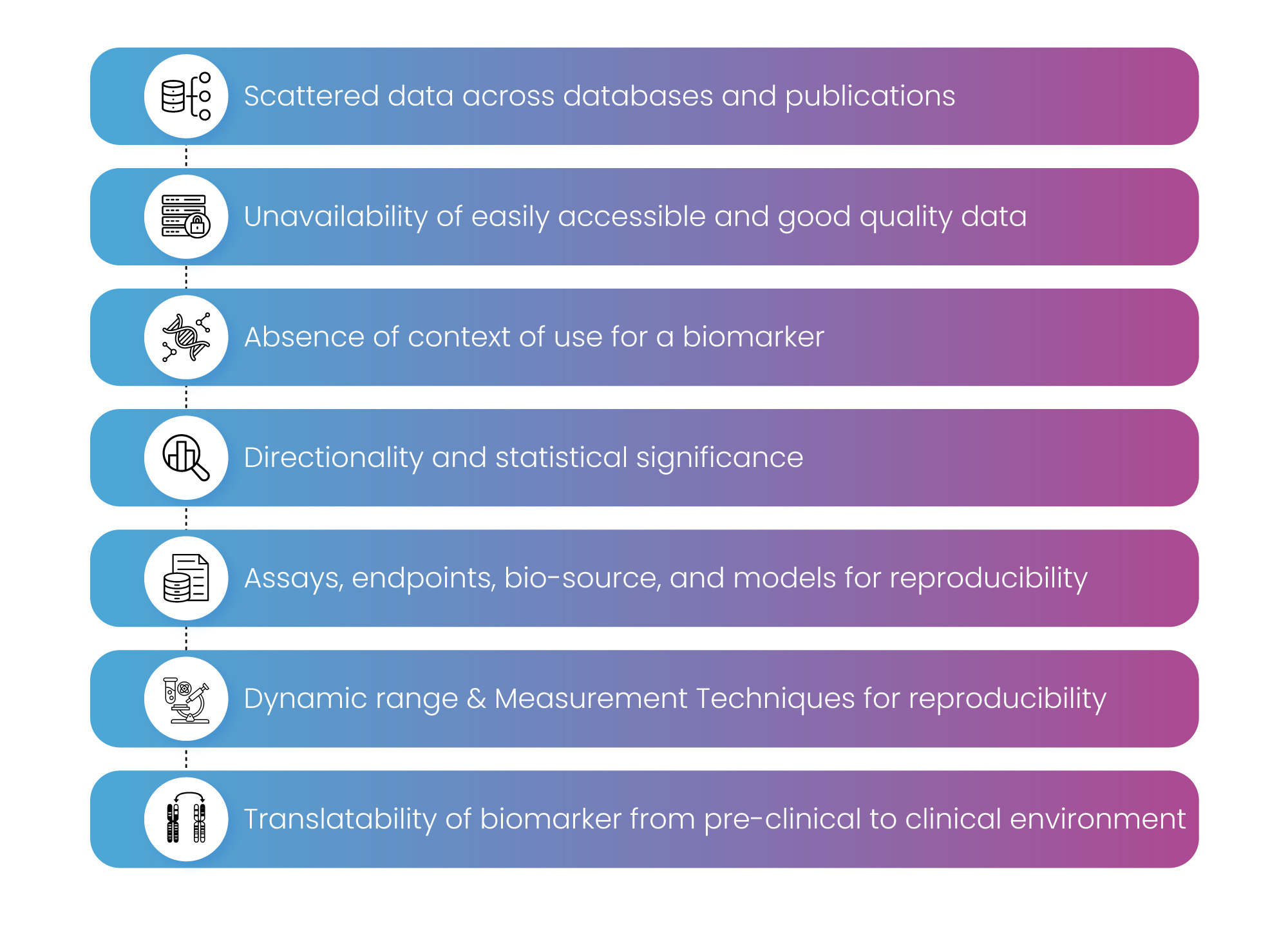
Figure 2: Major Challenges of Biomarker landscape assessment.
Excelra’s Approach to Biomarker Landscape assessment:
Excelra’s biomarker landscape assessment utilizes and integrates vital information from various systematic methodologies and resources, including proprietary databases, literature mining, clinical trial registries, computational analysis of data from high-throughput techniques, diagnostic imaging data, data analysis using statistical tools, and their application in building machine learning models for predicting and identifying suitable biomarker candidates as shown in Figure 3. The workflow helps interested stakeholders to make informed decisions pertaining to use of biomarkers in drug discovery and development programs.
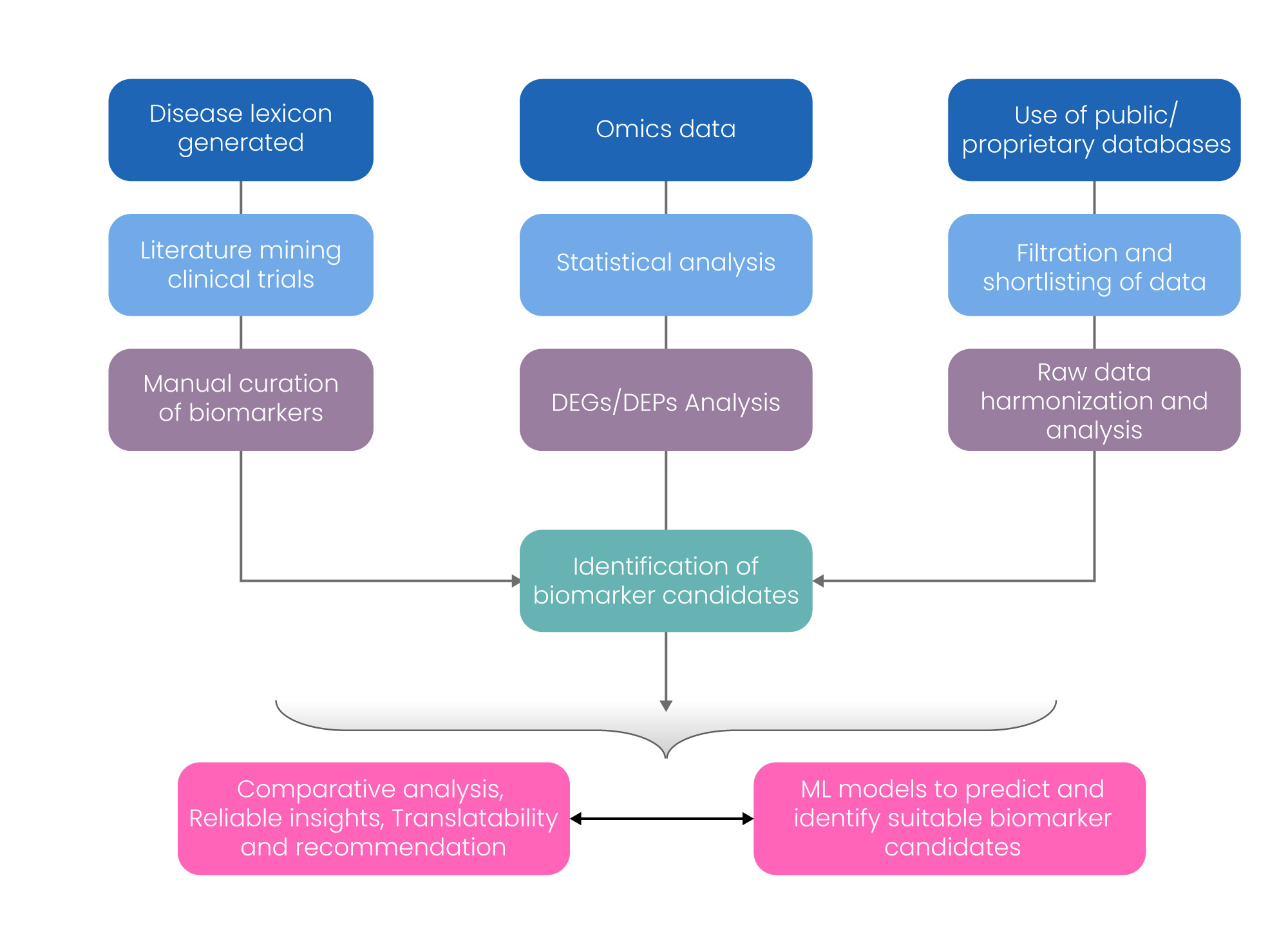
Figure 3: Excelra’s Approach of Biomarker Identification. DEGs- Differentially expressed Genes; DEPs- Differentially Expressed Proteins
Biomarker landscape assessment for Prostate cancer – a case study
Validation across diverse patient populations is crucial for clinical utility, while standardizing assay techniques and integrating biomarkers into guidelines is essential for widespread adoption. Addressing ethical and regulatory considerations is paramount. Our services offer a comprehensive profile, covering protein and gene aspects, leveraging a vast biomarker database to accelerate drug development for our partners. The landscape of prostate cancer biomarkers presents challenges, including the need for improved sensitivity and specificity for early detection and risk assessment (Figure 4).
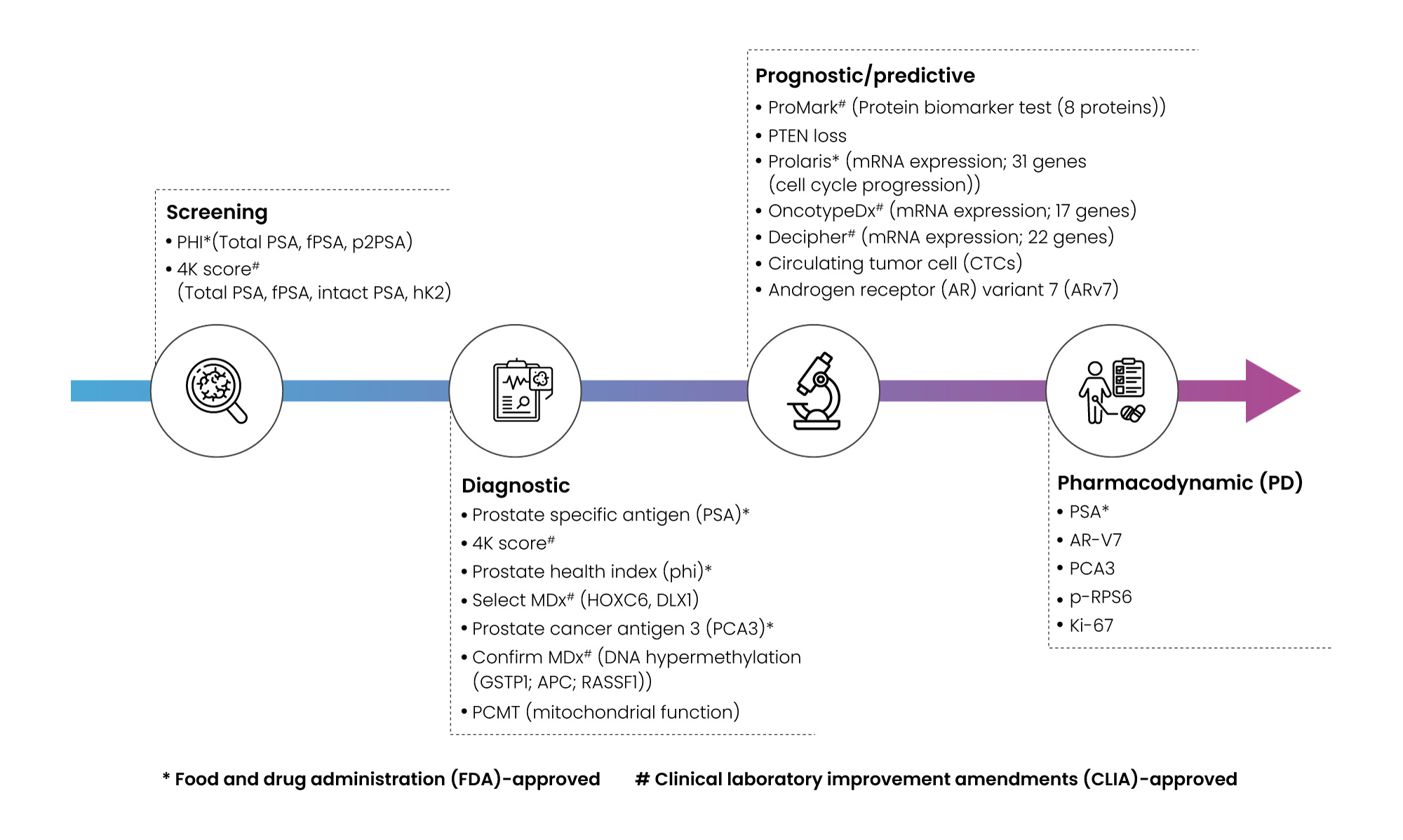
Figure 4: Prostate cancer biomarker landscape assessment.
Excelra’s USPs/Differentiators:
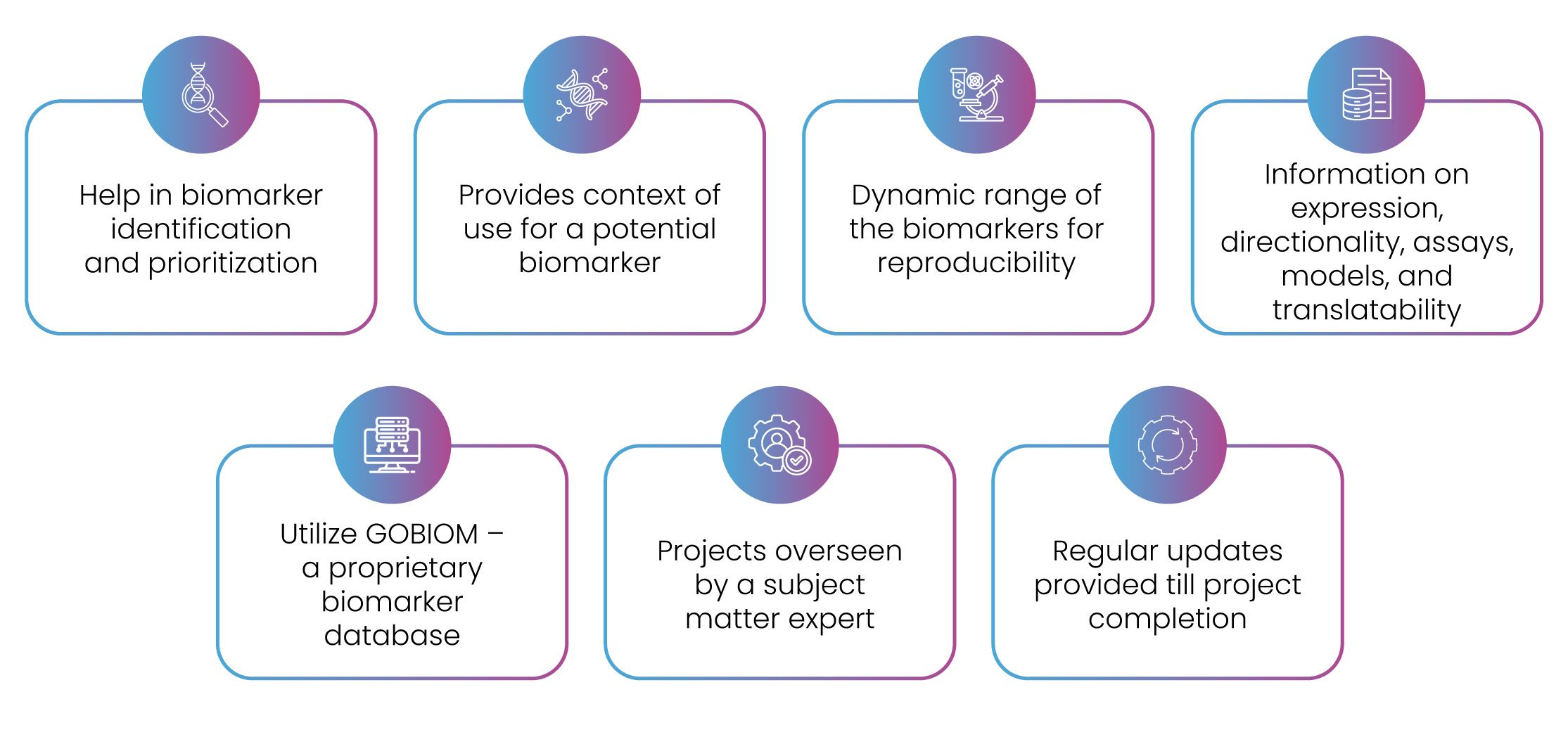
Figure 5: Excelra’s USP for biomarker landscape service.
Biomarkers play a crucial role in various aspects of healthcare, including disease diagnosis, prognosis, drug development, and monitoring therapeutic effects. Despite their immense potential, the conventional process of identifying biomarkers is often laborious and resource intensive. Both healthcare and pharmaceutical companies seek streamlined solutions to identify potential biomarkers and assess their translatability from pre-clinical models to clinical settings. Furthermore, the landscape of biomarker evaluation is undergoing a transformative shift with the integration of AI/ML models. These sophisticated algorithms enable the analysis of complex, high-throughput multi-omics, GWAS, and clinical data, revolutionizing biomarker assessment.
At Excelra, we offer tailored biomarker assessments designed to meet the unique requirements of our global Biotech and Pharma clients. Our comprehensive services cover specificity, sensitivity, and stability profiling (Figure 5). Leveraging our proprietary biomarker database and the expertise of our subject matter specialists, Excelra is well-positioned to support our partners in expediting their drug discovery and development endeavours.
That’s why you need more than just data. That’s why you need Excelra. Where data means more.
Recommended For You
Maximize your eln/lims investment with managed services for life sciences, lab informatics implementation and delivery, modernize your lab: migration, integrations, and custom app development.
We take the potential of data and extract insight, empowering life science companies on the journey to improve patient outcomes.
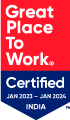
USEFUL LINKS
- About Excelra
OUR OFFICES
New Jersey – USA 200, Middlesex Essex Turnpike, Suite 204 Iseline, New Jersey, USA
Utrecht – Netherlands Melissekade 215, 3544 CW, Utrecht
Hyderabad – India NSL Arena, Uppal, Hyderabad – 500039
For sales & media enquiries: [email protected]
For career enquiries: [email protected]
Copyright © 2024 Excelra All Rights Reserved. | Privacy Policy | Terms & Conditions
Please fill the form
" * " indicates required fields
This will close in 0 seconds
Barriers to Healthcare Delivery in the U.S.: Case Studies Analysis Case Study
Americans in the US face multiple barriers to healthcare delivery related to cost, quality, and access. In the reviewed scenarios, we have Raymond, a 54-year-old skilled worker with no insurance, and Emmanuel, a 55-year-old office manager with insurance. They face different challenges related to their healthcare. Raymond’s main barriers include costs and access to proper healthcare. Since he is uninsured, he has to pay the exorbitant costs out of pocket (Beik et al., 2020). Prices prevent him from receiving regular and consistent healthcare. In addition, the prices for gas add to these ongoing expenditures, making the cost situation even worse.
In regards to accessibility, Raymond’s situation is worsened by being in a rural area. The nearest hospital is 30 miles away, which is the reason why gas prices are considered in the first place. Overall, these two factors combine together to create an unfavorable situation (Beik et al., 2020). Emmanuel’s situation is much different due to his having access to quality healthcare covered by insurance. There are no barriers present in his scenario – he has regular healthcare checks, receives referrals from his primary care providers, and his issues are covered by insurance.
Both case studies demonstrate how having insurance influences the decisions related to costs, quality, and access. In Raymond’s situation, had he been insured, he would have had funds to afford the gas to drive to the hospital and receive healthcare in a timely and appropriate manner. Thus, it is easy to see how insurance affects costs, which in turn affects accessibility, which influences the quality of care in a negative way (Bonewit-West & Hunt, 2019). Emmanuel does not have such problems – he lives in a big city, where access to care is readily available, and his insurance covers the majority of the costs (Bonewit-West & Hunt, 2019). Had he had no insurance, the situation might have been different, with him having to carry a greater burden due to managing his risks for Type 2 diabetes.
Potential health outcomes for each scenario are as follows: If nothing changes, Raymond’s situation is going to get worse. His lack of consistent care would result in increased hypertension and a greater likelihood of developing pulmonary embolisms and heart disease. Emmanuel, on the other hand, is at no risk of that and has a better chance of managing his diseases before they become chronic. Unless Raymond gets insurance, he will not have the opportunity to receive the care he needs.
Beik, J. I., & Pepper, J. (2020). Health insurance today-e-book . Elsevier Health Sciences.
Bonewit-West, K., & Hunt, S. (2019). Today’s medical assistant-e-book: Clinical & administrative procedures . Elsevier Health Sciences.
- Chicago (A-D)
- Chicago (N-B)
IvyPanda. (2024, May 6). Barriers to Healthcare Delivery in the U.S.: Case Studies Analysis. https://ivypanda.com/essays/barriers-to-healthcare-delivery-in-the-us-case-studies-analysis/
"Barriers to Healthcare Delivery in the U.S.: Case Studies Analysis." IvyPanda , 6 May 2024, ivypanda.com/essays/barriers-to-healthcare-delivery-in-the-us-case-studies-analysis/.
IvyPanda . (2024) 'Barriers to Healthcare Delivery in the U.S.: Case Studies Analysis'. 6 May.
IvyPanda . 2024. "Barriers to Healthcare Delivery in the U.S.: Case Studies Analysis." May 6, 2024. https://ivypanda.com/essays/barriers-to-healthcare-delivery-in-the-us-case-studies-analysis/.
1. IvyPanda . "Barriers to Healthcare Delivery in the U.S.: Case Studies Analysis." May 6, 2024. https://ivypanda.com/essays/barriers-to-healthcare-delivery-in-the-us-case-studies-analysis/.
Bibliography
IvyPanda . "Barriers to Healthcare Delivery in the U.S.: Case Studies Analysis." May 6, 2024. https://ivypanda.com/essays/barriers-to-healthcare-delivery-in-the-us-case-studies-analysis/.
- Garcinia Cambogia Weight‑Loss Supplementation
- Women's Gender Roles in American Literature
- Cognitive Behavioural and Emotion-Focused Therapies
- The Alcohol Use Disorder Treatment
- Developing a Healthy Lifestyle Campaign
- Discussion: Risk Factors of Cancer
- Medical Errors and Patient Care Quality
- Discussion: Human Papillomavirus (HPV)
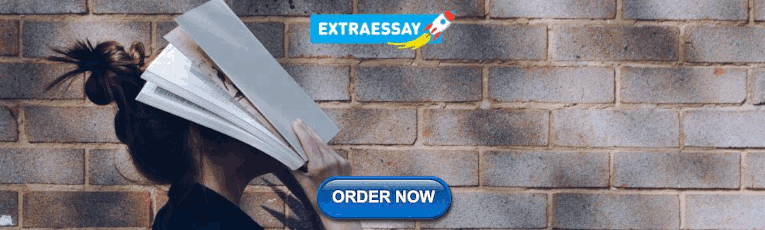
IMAGES
VIDEO
COMMENTS
View Case Study 3.29.docx from HITT 1301 at San Jacinto Community College. Case Study 3.29 Delivery Analysis Brooke Wagoner 1. Based on the national average of 32.8%, the cesarean rate in this graph
Statistics and Probability questions and answers. AHIMA Case 3.29- Delivery Analysis Opened: Tuesday, July 11, 2023, 12:00 AM Due: Monday, July 17, 2023, 11:59 PM Refer to AHIMA Case 3.29 in the case studies book for the needed graphs. The chief of obstetrics is asking for a six-year trend of vaginal versus cesarean section deliveries.
Study. Tasks. Homework help; Understand a topic; Writing & citations; Tools. Expert Q&A; Textbook Solutions; Math Solver ... 3.39 Delivery analysis Subdomain IIID.2 Analyze data to identify trends The chief of obstetrics is asking for a six year tnend of vaginal vensus cesanea seion delineries You supply the following graph Oakwood Memerial ...
Get instant study help. From your first assignment to your final exam, get the right tools designed to help you learn. 1. ^ Chegg survey fielded between 24 Sep and 12 Oct 2023 among US customers who used Chegg Study or Chegg Study Pack in Q2 2023 and Q3 2023. Respondent base (n=611) out of approximately 837,000 invitations.
Studies were all observational in nature. We identified no randomized controlled trials in the search strategy. These were published from 6 February 2020 to 12 June 2020. Forty-one were case report studies, 22 were case series studies, four cohort studies, and one case-control study (Table 1).
Formerly Health Services and Delivery Research (HS&DR) (ISSN: 2050-4357 (Online), ISSN: 2050-4349 (Print)) ... meta-analysis and case study. ... Being a manager, becoming a professional? A case study and interview-based exploration of the use of management knowledge across communities of practice in health-care organisations.
This suggests a need for further investigation and potential interventions to better manage and reduce the rate of C-section deliveries in order to align with national standards and improve patient outcomes. Answer to 3.29 Delivery analysis A Competency III.6DM Competency III.6 4 The...
37.5 × 5 days = 187.5 records per week. 187.5 × 52 weeks per year = 9,750 records per year. $32,136 / 9,750 = 3.296 = $3.30 per record. Exercise 9.8, page 189, question 5. The average work output per hour for coder 3 should be 2.46. Exercise 9.9, page 405, question 3d answers. The answer for coder A should be 20.98.
Case studies on transport policy, 9 (4) (2021), pp. 1737-1745. View PDF View article View in Scopus Google Scholar. ... Crowd-shipping services for last mile delivery: Analysis from American survey data. Transp. Res. Interdisciplinary Perspectives, 1 (2019), p. 100008. View PDF View article View in Scopus Google Scholar. Lewis, 2006.
STUDY DESIGN. Secondary analysis of data from a multi-center RCT of mild GDM treatment. CD rate of women delivering at term (≥ 37 weeks) was evaluated using two complementary approaches: 1) IOL vs. spontaneous labor: women induced at each GA compared with those who spontaneously labored at the same GA, and 2) IOL vs. expectant management: women delivered after IOL at each GA compared with ...
Rahul Saini September 17 , 2021. Data analytics is transforming every aspect of business across different industries, and a delivery management business is no exception. The dynamic nature of the supply chain business makes logistics the perfect use case for data. A delivery management business consists of an intricate structure, and you can ...
Books. HIM Case Studies, Second Edition. Dianna M. Foley. American Health Information Management Association, Nov 15, 2019 - Health & Fitness - 301 pages. "Collection of case studies, discussion questions, and assignments designed to give students practice applying their health information management (HIM) knowledge." --from back cover.
Corrections Calculating and Reporting Healthcare Statistics, Fifth Edition, Revised Reprint | AHIMA Product # AB120717 Chapter 3 Page 19, Changing a Percentage to a Fraction: The second equation should read, " 15% would be 15 x 1/100..." Chapter 8 Exercise 8.15, page 164, questions 6-10 The exercise should read "calculate the readmission rate for each position for the semiannual period."
Objective: This study is aimed at determining factors associated with home delivery, among mothers in Abobo Woreda, Gambella region, Southwest Ethiopia, 2019. Methods: A case control study conducted from 12 March 2019 up to 2 April 2019 on 88 cases and 176 controls. Cases include mothers who gave birth at home and those mothers who gave birth ...
The chief of obstetrics is asking for a six year trend of vaginal versus cesarean section deliveries You supply the following graph. Oakwood Memorial Births 600 ? 400 ...
Analysis: Case study of Latvia Aleksandrs Scedrovs, Karlis Mendzins, Aiga Barisa, Maksims Feofilovs ... • Last mile delivery (LMD) services, known to be as most expensive, least sustainable and inefficient delivery with longer delivery times in transportation of goods, yet the demand for e-commerce platforms has rapidly increased over last years
Question: Case Studies in Health Information Management Case Study 1: Mergers and Acquisitions Objectives Evaluate the centralization of health information management (HIM) services as required by the acquisition of one hospital by a larger hospital. Develop mission and vision statements for the new University Health Systems (UHS) HIM ...
Figure 3: Excelra's Approach of Biomarker Identification. DEGs- Differentially expressed Genes; DEPs- Differentially Expressed Proteins Biomarker landscape assessment for Prostate cancer - a case study Validation across diverse patient populations is crucial for clinical utility, while standardizing assay techniques and integrating biomarkers into guidelines is essential for widespread ...
We will write a custom essay on your topic a custom Case Study on Barriers to Healthcare Delivery in the U.S.: Case Studies Analysis. 808 writers online . Learn More . In regards to accessibility, Raymond's situation is worsened by being in a rural area. The nearest hospital is 30 miles away, which is the reason why gas prices are considered ...