- See us on facebook
- See us on twitter
- See us on youtube
- See us on linkedin
- See us on instagram
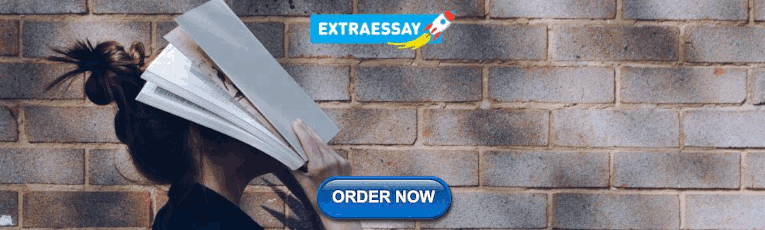
Human body temperature has decreased in United States, study finds
Stanford researchers have determined that average human body temperature in the United States has decreased since the 1800s.
January 7, 2020 - By Jack J. Lee
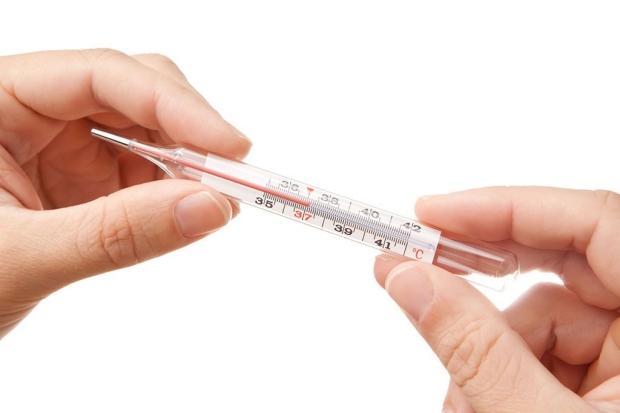
Modern studies have called the "normal" human temperature of 37 degrees Celsius (or 98.6 degrees Fahrenheit) into question, suggesting that it’s too high. Dinga/Shutterstock.com
Since the 19 th century, the average human body temperature in the United States has dropped, according to researchers at the Stanford University School of Medicine .
“Our temperature’s not what people think it is,” said Julie Parsonnet , MD, professor of medicine and of health research and policy. “What everybody grew up learning, which is that our normal temperature is 98.6, is wrong.”
That standard of 98.6 degrees Fahrenheit was made famous by German physician Carl Reinhold August Wunderlich, who published the figure in a book in 1868. Modern studies, however, have called that number into question, suggesting that it’s too high. A recent study, for example, found the average temperature of 25,000 British patients to be 97.9 F.
In a study published today in eLife , Parsonnet and her colleagues explore body temperature trends and conclude that temperature changes since the time of Wunderlich reflect a true historical pattern, rather than measurement errors or biases. Parsonnet, who holds the George DeForest Barnett Professorship, is the senior author. Myroslava Protsiv, a former Stanford research scientist who is now at the Karolinska Institute, is the lead author.
The researchers propose that the decrease in body temperature is the result of changes in our environment over the past 200 years, which have in turn driven physiological changes.
Digging into the past
Parsonnet and her colleagues analyzed temperatures from three datasets covering distinct historical periods. The earliest set, compiled from military service records, medical records and pension records from Union Army veterans of the Civil War, captures data between 1862 and 1930 and includes people born in the early 1800s. A set from the U.S. National Health and Nutrition Examination Survey I contains data from 1971 to 1975. Finally, the Stanford Translational Research Integrated Database Environment comprises data from adult patients who visited Stanford Health Care between 2007 and 2017.
The researchers used the 677,423 temperature measurements from these datasets to develop a linear model that interpolated temperature over time. The model confirmed body temperature trends that were known from previous studies, including increased body temperature in younger people, in women, in larger bodies and at later times of the day.
The researchers determined that the body temperature of men born in the early to mid-1990s is on average 1.06 F lower than that of men born in the early 1800s. Similarly, they determined that the body temperature of women born in the early to mid-1990s is on average 0.58 F lower than that of women born in the 1890s. These calculations correspond to a decrease in body temperature of 0.05 F every decade.
As part of the study, the authors investigated the possibility that the decrease could simply reflect improvements in thermometer technology; thermometers used today are far more accurate than those used two centuries ago. “In the 19 th century, thermometry was just beginning,” Parsonnet said.
To assess whether temperatures truly decreased, the researchers checked for body temperature trends within each dataset; for each historical group, they expected that measurements would be taken with similar thermometers. Within the veterans dataset, they observed a similar decrease for each decade, consistent with observations made using the combined data.
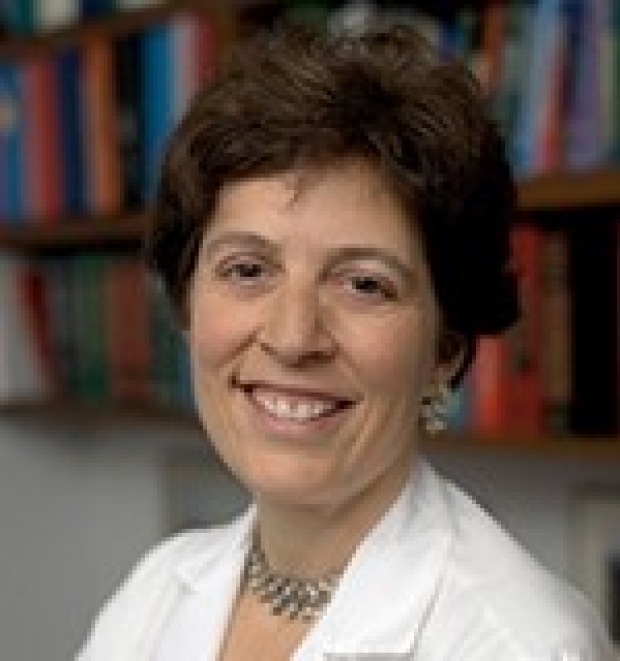
Julie Parsonnet
While the authors are confident of a cooling trend, the strong influences of age, time of day, and genders on body temperature preclude an updated definition of “average body temperature” to cover all Americans today.
Potential causes of colder body temperature
The decrease in average body temperature in the United States could be explained by a reduction in metabolic rate, or the amount of energy being used. The authors hypothesize that this reduction may be due to a population-wide decline in inflammation: “Inflammation produces all sorts of proteins and cytokines that rev up your metabolism and raise your temperature,” Parsonnet said. Public health has improved dramatically in the past 200 years due to advances in medical treatments, better hygiene, greater availability of food and improved standards of living. The authors also hypothesize that comfortable lives at constant ambient temperature contribute to a lower metabolic rate. Homes in the 19 th century had irregular heating and no cooling; today, central heating and air conditioning are commonplace. A more constant environment removes a need to expend energy to maintain a constant body temperature.
“Physiologically, we’re just different from what we were in the past,” Parsonnet said. “The environment that we’re living in has changed, including the temperature in our homes, our contact with microorganisms and the food that we have access to. All these things mean that although we think of human beings as if we’re monomorphic and have been the same for all of human evolution, we’re not the same. We’re actually changing physiologically.”
Other Stanford co-authors of the study are senior research scientist Catherine Ley, PhD; postdoctoral scholar Joanna Lankester, PhD; and Trevor Hastie, PhD, professor of statistics and of biomedical data sciences.
The research was supported by the National Institutes of Health (grants P01AG10120 and UL1TR003142).
Stanford’s departments of Medicine and of Health Research and Policy also supported the work.
- Jack J. Lee Jack J. Lee is a freelance writer.
About Stanford Medicine
Stanford Medicine is an integrated academic health system comprising the Stanford School of Medicine and adult and pediatric health care delivery systems. Together, they harness the full potential of biomedicine through collaborative research, education and clinical care for patients. For more information, please visit med.stanford.edu .
Hope amid crisis
Psychiatry’s new frontiers
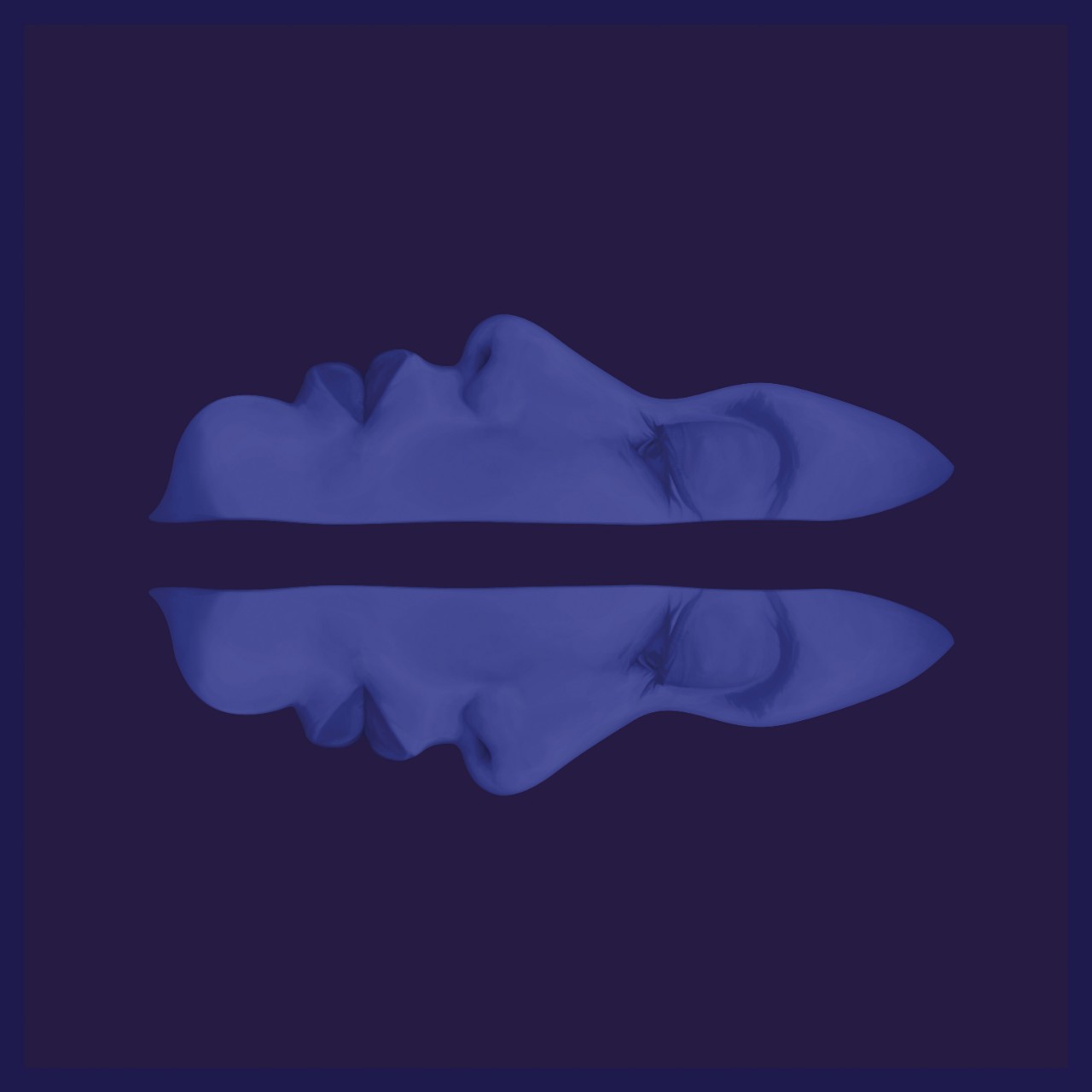
- - Google Chrome
Intended for healthcare professionals
- My email alerts
- BMA member login
- Username * Password * Forgot your log in details? Need to activate BMA Member Log In Log in via OpenAthens Log in via your institution

Search form
- Advanced search
- Search responses
- Search blogs
- Individual differences...
Individual differences in normal body temperature: longitudinal big data analysis of patient records
- Related content
- Peer review
- Ziad Obermeyer , assistant professor 1 2 ,
- Jasmeet K Samra , research analyst 1 ,
- Sendhil Mullainathan , professor 3
- 1 Department of Emergency Medicine, Brigham and Women’s Hospital, Boston, MA, USA
- 2 Department of Emergency Medicine and Health Care Policy, Harvard Medical School, Boston, MA, USA
- 3 Department of Economics, Harvard University, Boston, MA, USA
- Correspondence to: Z Obermeyer zobermeyer{at}bwh.harvard.edu
- Accepted 23 November 2017
Objective To estimate individual level body temperature and to correlate it with other measures of physiology and health.
Design Observational cohort study.
Setting Outpatient clinics of a large academic hospital, 2009-14.
Participants 35 488 patients who neither received a diagnosis for infections nor were prescribed antibiotics, in whom temperature was expected to be within normal limits.
Main outcome measures Baseline temperatures at individual level, estimated using random effects regression and controlling for ambient conditions at the time of measurement, body site, and time factors. Baseline temperatures were correlated with demographics, medical comorbidities, vital signs, and subsequent one year mortality.
Results In a diverse cohort of 35 488 patients (mean age 52.9 years, 64% women, 41% non-white race) with 243 506 temperature measurements, mean temperature was 36.6°C (95% range 35.7-37.3°C, 99% range 35.3-37.7°C). Several demographic factors were linked to individual level temperature, with older people the coolest (–0.021°C for every decade, P<0.001) and African-American women the hottest (versus white men: 0.052°C, P<0.001). Several comorbidities were linked to lower temperature (eg, hypothyroidism: –0.013°C, P=0.01) or higher temperature (eg, cancer: 0.020, P<0.001), as were physiological measurements (eg, body mass index: 0.002 per m/kg 2 , P<0.001). Overall, measured factors collectively explained only 8.2% of individual temperature variation. Despite this, unexplained temperature variation was a significant predictor of subsequent mortality: controlling for all measured factors, an increase of 0.149°C (1 SD of individual temperature in the data) was linked to 8.4% higher one year mortality (P=0.014).
Conclusions Individuals’ baseline temperatures showed meaningful variation that was not due solely to measurement error or environmental factors. Baseline temperatures correlated with demographics, comorbid conditions, and physiology, but these factors explained only a small part of individual temperature variation. Unexplained variation in baseline temperature, however, strongly predicted mortality.
Introduction
Have you ever felt cold, or warm, in a room where everyone else felt comfortable? This common experience about room temperature has some interesting lessons for body temperature and how we measure it. To know how warm or cold someone feels, we would not look at room temperature alone. Married couples sitting next to each other in the same room routinely disagree about whether to turn the heat up or down. Individuals have different baseline propensities to feel hot or cold—at any given absolute room temperature.
Yet doctors have forgotten this lesson when measuring core body temperature. We would not use absolute room temperature to infer perceived warmth, but we do use absolute body temperature to infer fever.
In medical school, students are taught that humans have a core body temperature as a species, not as individuals. When clinicians take patients’ temperatures in the clinic or hospital, they compare the measurements with the population average. Deviations from this single number help in the diagnosis of acute pathological states, from infections to thyroid disorders.
Why should someone’s physiological state be compared with an absolute standard temperature? Body temperature deviations, after all, can have their roots in individual physiology, such as age 1 2 and circadian, 3 metabolic, 4 and ovulatory cycles. 5 These factors vary dramatically across individuals, raising the possibility that individuals have baseline temperatures that differ systematically from the population average. The same temperature that is normal for one person might be dangerously high for another.
Historically, the use of a population average was partly a data problem: estimating each individual’s baseline body temperature would have been challenging. Any credible effort would need to tease apart individual baseline temperatures from other sources of variation in measured temperatures: ambient conditions when temperature is taken, 6 differences in technique, 7 8 and random error due to intrinsic variability in the measurement process itself. 8 9 Distinguishing signal from noise under these conditions would require large amounts of data: both large numbers of patients and multiple temperature measurements for each patient.
The modern electronic health record stores rich physiological data, including temperature, on large numbers of patients, and many have noted its potential to generate new clinical knowledge. 10 11 We used these data to deal with random errors in individual temperature measurements, by applying statistical techniques that find signal and minimize noise across multiple temperature measurements, taken in a variety of outpatient settings and for a large number of patients. To deal with the many factors known to affect temperature measurements, we used rich contextual data to control for conditions at the time of measurement: ambient temperature, humidity, time of day, date, and body site of measurement. This allowed us to estimate stable baseline temperatures for every patient in our large and diverse sample, as opposed to population averages. We then explored links between individual temperature and a range of other variables: demographics; physiological measures, including vital signs; and mortality.
Individual differences in body temperature might be meaningful in two ways. Firstly, their very existence could open new insights into human physiology and links between body temperature, metabolism, and longevity. 12 13 14 Secondly, individualized “precision temperatures” could allow doctors to tailor testing and treatment decisions to patients’ physiology. At a minimum, they might change one familiar part of the doctor-patient conversation: the eye-rolling that sometimes ensues when patients report that a given temperature, although normal, is “high for me.”
Until recently, large scale databases of temperature measurements were scarce: with some notable exceptions, 15 16 most studies of body temperature in humans date from 1950 or before 17 and have important limitations. Temperatures were measured at varying times of day and in different seasons, using unspecified instruments. 17 Details regarding enrollment procedures were often absent, but—particularly in older studies—there was little apparent concern about achieving a diverse sample of patients for race, sex, and comorbid conditions. 17 Lack of longitudinal data meant that temperature measurements could not be correlated to subsequent outcomes. 15 16 17 Perhaps most importantly, albeit with some exceptions, 15 18 sample sizes were small—often in the 10s to 100s of patients. 16 17
We used a dataset of electronic health records from a large US based academic hospital. The dataset was assembled in two steps: firstly, we identified patients to be included in the cohort—those with one or more visits to the hospital’s emergency and outpatient departments during 2010-12; and, secondly, we obtained data on each of these patient’s outpatient visits from 2009-14, consisting of visits to clinics during which a temperature was measured (Welch Allyn SureTemp Plus digital thermometers were present in most examination rooms).
Since we were interested in individual estimates of normal body temperature in adults, we focused on routine visits during which temperature was expected to be within normal limits. We did not include emergency department visits, during which acute physiological disturbances may affect measured temperatures. We excluded patients aged less than 18 years and those seen on weekends or outside business hours (7 am-6 pm), to avoid selecting patients seen for non-routine problems; those with implausible recorded temperatures (<32°C and >45°C; 0.04% of the total sample); and those visiting clinics for infections, to remove the effect of infection related disturbances in body temperature. We thus excluded visits with ICD-9 (international classification of diseases, ninth revision) codes for infectious diseases, and visits with antibiotics prescribed in the week after the visit (see supplement eTable 1 for cohort construction). Our final sample accounted for roughly 3-5% of the hospital’s annual load of outpatient visits.
Statistical analysis
Estimating individual baseline temperatures.
In the included sample of routine outpatient visits, we used ordinary least squares regression to model measured visit level temperature as a function of external conditions (ie, ambient temperature and dew point, drawn from National Oceanographic and Atmospheric Administration data 19 ), body site (eg, oral, axillary), and time of measurement (hour, day, month, and year).
To estimate baseline temperatures, we modeled individual patients’ deviations from the population mean, controlling for the selected factors. We used fixed effects to ensure that individual temperature effects were approximately normally distributed (see supplement eFigure 1), then we re-estimated the model using random effects. We chose random effects because even in the presence of measurement error they are consistent and efficient estimators of variance. 20 21 These steps allowed us to estimate individual temperature effects as well as features of the population level distribution, such as the variance of individual effects. Using individual level effects necessarily restricted the sample to patients with at least two measured temperatures over the study period. We omitted time invariant attributes of patients (eg, sex, race) since these cannot be estimated alongside individual level effects. Standard errors were clustered at patient level.
We correlated the resulting individual temperature random effects with other variables of interest in the electronic health record, in three groups: demographics, including age, sex, and race; comorbidities, defined using ICD-9 codes over the year before visits, following usual practice 22 ; and physiological measurements (pulse, systolic and diastolic blood pressure, body mass index), using average values over the period spanning from first to last included visit date for each patient—this mirrored the period over which individual level effects were estimated in our dataset. Since we were exploring a range of correlations, we adjusted P values for multiple hypothesis testing using the Holm sequential procedure. 23 Alternative methods of adjustment 24 25 are provided in supplement eTable 3 (results were substantively unchanged).
Relation between individual temperature effects and mortality
Finally, we explored the relation between individual temperature and mortality, using linkage to state social security data. To accurately calculate one year mortality, we addressed a source of bias in these longitudinal cohort data: patients were sampled and included in the cohort because of encounters over 2010-12, up until which they were necessarily alive. Likewise, patients were followed until their last temperature measurement, as late as 2014, at which time they were also alive by construction. Since our primary interest was the correlation between mortality and routine temperature measurements, we calculated mortality in the year after each patient’s last temperature measurement, excluding those whose 2010-12 sampling event occurred after the last temperature measurement (16% of the sample). We then estimated the relation between one year mortality and individual temperature effects by logistic regression, controlling for demographics, comorbidities, and physiological measures. As the hospital is a referral center serving patients from the local community and further away—who require specialized care for complex, serious illnesses with high mortality—we also controlled for log distance between patients’ home zip code and hospital zip code.
Statistical packages
All analyses were performed in STATA (version 14.0) and R (version 3.2.3; Foundation for Statistical Computing).
Patient involvement
No patients were involved in setting the research question or the outcome measures, nor were they involved in developing plans for design or implementation of the study. No patients were asked to advise on interpretation or writing up of results. There are no plans to disseminate the results of the research to study participants or the relevant patient community.
Of 374 306 patients with temperature measurements at outpatient visits, we excluded 130 800 (largely because of infection diagnoses or antibiotic prescriptions; see supplement eTable 1), leaving 243 506 visits (18% of all outpatient visits meeting the other inclusion criteria). Table 1 shows demographics, physiological measurements, comorbidities, and one year mortality. The mean age at time of the visit was 52.9 years, 64% of patients were women, and 41% were of non-white race (including 16% black or African-American patients, 17% Hispanic patients). The most common primary diagnoses at included outpatient visits were osteoarthritis (5.9%), back pain (4.9%), and routine evaluation and examination (4.5%). The clinics most commonly visited were orthopedics (10%) and internal medicine (hospital based clinic: 7.8%, community clinic: 5.7%). One year mortality in this sample of patients receiving care at a tertiary referral hospital was 6.2%, considerably higher than in the general population (<1% in a similar age range).
Demographics, physiological measurements, comorbidities, and one year mortality for full sample. Values are numbers (percentages) unless stated otherwise
- View inline
The mean measured temperature was 36.6°C (95% confidence interval 36.6°C to 36.6°C). Each patient had a median 5 (interquartile range 3-9) temperature measurements over a median of 2.1 (0.8-3.8) years, and 19% had more than 10 measurements.
Summary statistics on temperature
Mean 36.6°C (95% range 35.7-37.3°C; 99% range 35.3-37.7°C)
Measurements at different sites (versus oral): temporal: –0.03°C; tympanic: –0.06°C; axillary: –0.26°C
Figure 1 shows the correlation of temporal and environmental factors to measured body temperature, estimated by using random effects regression. Temperature measurements were largely oral (88.2% oral, 3.5% temporal, 3.0% tympanic, 0.1% axillary, 5.2% not recorded), and temporal, tympanic, and axillary temperatures were significantly lower than oral temperatures (by –0.03°C, –0.06°C, and –0.27°C, respectively; all P<0.001). We observed diurnal variation in temperature by hour, with a peak at 4 pm (0.03°C v 12 pm, P<0.001). Higher ambient temperature and dew point were both linked to higher body temperature. Month effects worked to offset these effects—that is, at the same ambient temperature and dew point, summer months were linked to lower body temperature and winter months to higher body temperature. On a median temperature day in our dataset (12.2°C), body temperature was on average 0.08°C lower in July than in February, presumably reflecting the effect of compensatory physiological mechanisms (eg, evaporative cooling, vasoconstriction) by season. Supplement eTable 2 presents the full coefficients from the model.
Relation of temporal and environmental factors to measured body temperature. Coefficients estimated by random effects regression are shown for ambient temperature, dew point, hour, and month, compared with reference categories: median temperature 10th (12.2°C), median dew point 10th (4.7˚C), 12 pm, and April, respectively
- Download figure
- Open in new tab
- Download powerpoint
The standard deviation for random effects from this model, denoted as individual baseline temperatures, was 0.15, with 95% range (ie, 2.5–97.5th centile range) 0.60°C (–0.33-0.27°C). In comparison, the standard deviation for raw measured body temperature was 0.42, with 95% range 1.67°C (after subtracting the mean, for comparability: –0.93-0.74°C).
Table 2 shows demographics, vital signs, and comorbidities by fifth of individual baseline temperature, along with regression coefficients of baseline temperatures on the variable. Baseline temperatures declined with age (–0.02°C every decade, P<0.001). African-American women had the highest temperature (0.052°C higher than white men, P<0.001). Baseline temperature also varied significantly as a function of comorbid conditions. Cancer was linked to higher temperature (0.02°C, P<0.001), whereas hypothyroidism was linked to lower temperature (–0.01°C, P=0.01; the relation was linear for mean thyroid stimulating hormone level over the span of the data (see supplement eFigure 2A). The total number of comorbidities was not statistically significantly linked to baseline temperature over and above all individual included comorbidities.
Coefficients from regression of individual temperature effect on demographics, comorbidities, and physiological measurements in 20 718 participants
Table 2 shows the relation between individual baseline temperatures and average physiological measurements over the study period. Controlling for demographic factors and comorbidities, higher temperatures were linked to increased body mass index (0.002°C per m/kg 2 , P<0.001). Higher temperature was also linked to higher pulse (4.0×10 −5 °C per beats per minute, P=0.17) and increased diastolic blood pressure (1.2×10 −4 °C per mm Hg, P=0.01). Figure 2 shows mean vital signs over the study period and their relation to baseline temperatures by sex.
Physiological measurements and relation to temperature effects, by sex. Dots represent centiles of individual temperature effect and vertical bar 95% confidence intervals
Despite the many statistically significant relations identified between individual baseline temperatures and demographics, comorbidities, and physiological measures, these factors collectively accounted for only 8.2% of variation (adjusted R 2 ) in temperature. Residual variation was greater for women than for men (residual sum of squares: 283 v 170), raising the possibility that at least part of the residual difference was driven by hormonal cycles (not measured). However, most variation in baseline temperatures remained unexplained by commonly measured health variables in both sexes.
Table 3 shows the relation between individual baseline temperature and mortality. Controlling for age, sex, race, vital signs, and comorbidities, a 1°C increase in temperature translated into 3.5% higher mortality (P = 0.014). For example, a 1 SD increase in temperature (0.15°C) would translate into a 0.52% absolute increase in one year mortality. Compared with a mean mortality of 6.2% in our sample, this represented an 8.4% relative increase in mortality risk.
Regression of one year mortality on individual temperature, controlling for demographics, comorbidities, and physiological measures in 15 821 participants
To our knowledge, this is the first attempt to demonstrate meaningful variation in individual body temperature, separately from random measurement error and the influence of external factors, and to correlate them to a range of patient factors and outcomes in a diverse patient population.
These results illustrate a way in which “big data” can serve to generate new medical knowledge. Here we used these data not to answer a causal question (eg, to discover side effects of drugs) or to predict an outcome (eg, to create an early warning system for a given condition), but to discover previously unsuspected and potentially important patterns in human physiology. It is unlikely that any one practitioner could have noticed these patterns, despite their fairly large magnitude connections with mortality. Rather, large datasets and statistical methods are needed to bring out these patterns from the empirical record.
Our most noteworthy result was the connection between temperature and mortality. This fits with a larger body of research showing that reduction in body temperature increases longevity and delays aging in a range of (ectothermic) experimental models, including Drosophila and Caenorhabditis elegans , 26 as well as transgenic (homeothermic) mice engineered to have lower temperatures. 12 This observation raises a set of questions that may be worth answering through further research. What is the biological basis for an individual’s baseline temperature? And how might these factors teach us more about subtle but important physiological patterns that might lead to good or poor outcomes? Two sets of results from our analysis were suggestive along these lines.
Firstly, individual temperatures were highly correlated with measured patient characteristics, particularly those related to metabolism and obesity. These differences may have their roots in obvious thermodynamic factors: bodies with larger mass dissipate heat less rapidly, leading to higher temperatures. Fat (which is correlated with mass) could also act as an insulator, independent of mass, leading to higher heat retention in people with more fat. Other explanations, however, are also plausible. It is well known that caloric restriction through fasting leads to down-regulation of temperature, presumably to conserve energy 27 ; indeed, reduced temperature is now considered a biomarker for caloric restriction. 13 14 Although there are few trials of caloric supplementation, the existing literature suggests that individuals vary widely in their ability to dissipate excess energy from overfeeding. 28 Given the strong links between resting metabolic rate and body temperature, 4 it is possible that higher resting body temperature could be a response serving to dissipate excess energy from caloric intake. We found that a raised temperature correlated with both body mass index and activation of the sympathetic nervous system (ie, increased pulse rate and diastolic blood pressure). Thus temperature could be another variable in the cluster of traits linking obesity and activation of the sympathetic nervous system. 29 30 31 Interestingly, while our temperature effects were estimated over longer periods, some small studies have found that hypothermia is linked to increased mortality for acute events (eg, in hip fractures), 32 potentially implying a different physiology in short term versus long term temperature regulation.
Secondly, we found a large correlation between individual baseline temperature and mortality that was not explained by measured patient characteristics. What factors might this temperature variation be picking up on that confer an 8.4% mortality disadvantage? It is tempting to speculate. We did identify a correlation between diagnosed cancers and temperature. This has been noted in the literature previously, either because of the direct metabolic demands of the cancer itself, 33 or because of the body’s immune response. 34 Subclinical infections or rheumatological diseases could exert a similar effect. If higher temperature reflected undiagnosed cancers or other illnesses, this would generate the correlation we observed between the unexplained component of temperature variation and subsequent mortality from these same illnesses. Another potential explanation is that higher temperature reflected a pro-inflammatory milieu; however, we found no clear connection between individual temperature and the inflammatory marker reactive protein (see supplement eFigure 2b). Ultimately, further study is required. We could imagine studies that estimated individual temperature effects using similar methods, then subjected those with higher temperatures to additional diagnostic studies to identify undiagnosed illnesses.
The finding that measured temperature was, other things being equal, lower in hot months and higher in cold months may reflect engagement of well known compensatory adaptations (eg, plasma volume, evaporative cooling, vasoconstriction, shivering) to temperatures experienced over longer periods, as opposed to the short term direct effects of higher or lower temperature. 35 Related recent work has shown, for example, that drinking warm beverages on warm days results in heat loss from increased sweat output. 36
Finally, our estimate of population mean temperature differed from other studies—for example, it was lower than in a sample of primarily young, healthy participants, 16 and higher than in a population of older adults seen in healthcare settings. 15 One potential advantage of our approach is that it adjusted for environmental and temporal factors at the time of measurement, which was not possible in other studies. Although this might enhance the generalizability of our estimate, it would be difficult for any one study of core temperature to ensure that estimates are valid for an entire species. However, it may help with clinical decision making for populations seeking healthcare in similar settings.
Limitations of this study
Our study had several limitations. We considered patients at one academic center and measured temperature using similar equipment, which can have correlated errors in measurement. Our methods were designed to estimate robust deviations in individual temperature separately from measurement error but might not generalize to other equipment, although we would guess that stringent Food and Drug Administration standards for clinical electronic thermometers offer some guarantees that the relative magnitude of effects should be similar. Our data came from one climate zone. Although we controlled for the substantial variation in environmental conditions within this zone, temperature and compensatory mechanisms may vary across climactic zones. We excluded patients with infection and those prescribed antibiotics, but it is possible that some patients had infections that were undiagnosed and untreated by their physicians. However, since this would have to be a consistent finding over multiple visits for the same patients, and since most infections are by contrast transient, this is unlikely to have affected our estimated correlation between individual baseline temperature and mortality. We sampled patients based on visits to a hospital emergency department and clinics. This resulted in an ethnically and medically diverse sample over multiple years of data, but it also selected a sicker set of patients, with a higher comorbidity burden and mortality than the general population. An advantage is that our sample was representative of the population of patients using healthcare today.
Conclusions
We found that individuals have body temperature baselines that correlate with a range of demographic factors, comorbid conditions, and physiological measurements. Since the unexplained variation in temperature is large and correlates with mortality, it may be an interesting and important area for further study.
What is already known on this topic
A long tradition of research on human core body temperature, starting in the 19th century, has focused on establishing average temperature in a population
Temperature is known to be influenced by many factors that differ widely across patients (eg, age and circadian, metabolic, and ovulatory cycles) raising the possibility that individual baseline body temperatures might vary systematically
What this study adds
Individual baseline temperatures are correlated with specific demographic factors, with older people the coldest and African-American women the hottest
Particular medical conditions were also statistically significantly linked to lower or higher temperature, as were physiological measurements, but these factors explained only 8.2% of variation in individual baseline temperatures
The remaining unexplained variation was a large and significant predictor of subsequent mortality, nearly 8.4% higher mortality for a 1 SD increase in temperature
Acknowledgments
We thank Philip Mackowiak for comments on an early draft of this manuscript.
Contributors: ZO and SM designed the study and wrote the manuscript. ZO obtained funding. JKS and ZO analyzed the data. All authors had full access to all of the data (including statistical reports and tables) in the study and can take responsibility for the integrity of the data and the accuracy of the data analysis. ZO acts as guarantor.
Funding: This study was supported by a grant from the office of the director of the National Institutes of Health (DP5 OD012161) to ZO. This research was independent from funders. The funder had no role in the study design; in the collection, analysis, and interpretation of data; in the writing of the report; or in the decision to submit the article for publication.
Competing interests: All authors have completed the ICMJE uniform disclosure form at www.icmje.org/coi_disclosure.pdf and declare: no financial relationships with any organizations that might have an interest in the submitted work in the previous three years; no other relationships or activities that could appear to have influenced the submitted work.
Ethical approval: This study was approved by the institutional review boards of Partners HealthCare, the parent organization of the hospital where the research was conducted.
Data sharing: No additional data available.
Transparency: The lead author (ZO) affirms that the manuscript is an honest, accurate, and transparent account of the study being reported; that no important aspects of the study have been omitted; and that any discrepancies from the study as planned have been explained.
This is an Open Access article distributed in accordance with the Creative Commons Attribution Non Commercial (CC BY-NC 4.0) license, which permits others to distribute, remix, adapt, build upon this work non-commercially, and license their derivative works on different terms, provided the original work is properly cited and the use is non-commercial. See: http://creativecommons.org/licenses/by-nc/4.0/ .
- Castle SC ,
- Norman DC ,
- Yoshikawa TT
- Refinetti R ,
- Landsberg L ,
- Leonard WR ,
- Linsenmeier RA ,
- Moghissi KS ,
- Halonen JI ,
- Zanobetti A ,
- Sparrow D ,
- Vokonas PS ,
- Sund-Levander M ,
- Forsberg C ,
- Burnham RS ,
- McKinley RS ,
- Murdoch TB ,
- Sanchez-Alavez M ,
- Winsky-Sommerer R ,
- Ingram DK ,
- Heilbronn LK ,
- de Jonge L ,
- Frisard MI ,
- Pennington CALERIE Team
- Mackowiak PA ,
- Wasserman SS ,
- Horvath SM ,
- Menduke H ,
- ↵ Wunderlich CA. On the temperature in diseases: a manual of medical thermometry . New Sydenham Society; 1871. https://books.google.com/books?hl=en&lr=&id=3-wHAAAAIAAJ&oi=fnd&pg=PA19&dq=wunderlich+temperature&ots=9atUlZjAlx&sig=qsNSqTYmhYcZHG-zvNaB-8l-Cmk .
- ↵ National Oceanographic and Atmospheric Administration. NCEP Meteorological Assimilation Data Ingest System (MADIS). https://madis.ncep.noaa.gov/ . Accessed September 18, 2017.
- Gardiner JC ,
- ↵ Wooldridge JM. Econometric analysis of cross section and panel data . MIT press; 2010. https://books.google.com/books?hl=en&lr=&id=hSs3AgAAQBAJ&oi=fnd&pg=PP1&dq=econometrics+wooldridge&ots=VXLTsxYXQr&sig=fVNIOkwnd6Z_LROIp8nfTGXk1rA .
- Schneeweiss S
- Benjamini Y ,
- Bouchard C ,
- Tremblay A ,
- Després J-P ,
- Seravalle G ,
- Cattaneo BM ,
- Troisi RJ ,
- Parker DR ,
- Landsberg L
- Scherrer U ,
- Vollenweider P ,
- Jéquier E ,
- Farrier AJ ,
- Venkatesan M ,
- Lawson RN ,
- Chughtai MS
- Repasky EA ,
- Dewhirst MW
- Kleeman CR ,
- Henschel A ,
- Hegnauer AH
- Lesperance NC ,
- Agency for Healthcare Research and Quality

An official website of the United States government
The .gov means it’s official. Federal government websites often end in .gov or .mil. Before sharing sensitive information, make sure you’re on a federal government site.
The site is secure. The https:// ensures that you are connecting to the official website and that any information you provide is encrypted and transmitted securely.
- Publications
- Account settings
- My Bibliography
- Collections
- Citation manager
Save citation to file
Email citation, add to collections.
- Create a new collection
- Add to an existing collection
Add to My Bibliography
Your saved search, create a file for external citation management software, your rss feed.
- Search in PubMed
- Search in NLM Catalog
- Add to Search
Normal Body Temperature: A Systematic Review
Affiliations.
- 1 State University of New York Upstate Medical University, Syracuse, NY, USA.
- 2 Department of Internal Medicine, Icahn School of Medicine at Mount Sinai, New York, NY.
- 3 Division of Infectious Diseases, Icahn School of Medicine at Mount Sinai, New York, NY.
- 4 Icahn School of Medicine at Mount Sinai, New York, NY.
- PMID: 30976605
- PMCID: PMC6456186
- DOI: 10.1093/ofid/ofz032
PubMed was searched from 1935 to December 2017 with a variety of search phrases among article titles. The references of the identified manuscripts were then manually searched. The inclusion criteria were as follows: (1) the paper presented data on measured normal body temperature of healthy human subjects ages 18 and older, (2) a prospective design was used, and (3) the paper was written in or translated into the English language. Thirty-six articles met the inclusion criteria. This comprised 9227 measurement sites from 7636 subjects. The calculated ranges (mean ± 2 standard deviations) were 36.32-37.76 (rectal), 35.76-37.52 (tympanic), 35.61-37.61 (urine), 35.73-37.41 (oral), and 35.01-36.93 (axillary). Older adults (age ≥60) had lower temperature than younger adults (age <60) by 0.23°C, on average. There was only insignificant gender difference. Compared with the currently established reference point for normothermia of 36.8°C, our means are slightly lower but the difference likely has no physiological importance. We conclude that the most important patient factors remain site of measurement and patient's age.
Keywords: body temperature; fever; hypothermia; normothermia.
PubMed Disclaimer
Literature search results and the…
Literature search results and the determinants of normothermia. (A) Number of studies and…
Similar articles
- Folic acid supplementation and malaria susceptibility and severity among people taking antifolate antimalarial drugs in endemic areas. Crider K, Williams J, Qi YP, Gutman J, Yeung L, Mai C, Finkelstain J, Mehta S, Pons-Duran C, Menéndez C, Moraleda C, Rogers L, Daniels K, Green P. Crider K, et al. Cochrane Database Syst Rev. 2022 Feb 1;2(2022):CD014217. doi: 10.1002/14651858.CD014217. Cochrane Database Syst Rev. 2022. PMID: 36321557 Free PMC article.
- Comprehensive Analysis of Temperature in Hospitalized Patients. Geneva II, Cuzzo B, Fazili T, Javaid W. Geneva II, et al. Am J Med Sci. 2019 Aug;358(2):134-142. doi: 10.1016/j.amjms.2019.05.011. Epub 2019 Jun 1. Am J Med Sci. 2019. PMID: 31331451
- Comparison of rectal and aural core body temperature thermometry in hyperthermic, exercising individuals: a meta-analysis. Huggins R, Glaviano N, Negishi N, Casa DJ, Hertel J. Huggins R, et al. J Athl Train. 2012 May-Jun;47(3):329-38. doi: 10.4085/1062-6050-47.3.09. J Athl Train. 2012. PMID: 22892415 Free PMC article.
- A systematic review of body temperature variations in older people. Lu SH, Leasure AR, Dai YT. Lu SH, et al. J Clin Nurs. 2010 Jan;19(1-2):4-16. doi: 10.1111/j.1365-2702.2009.02945.x. Epub 2009 Nov 3. J Clin Nurs. 2010. PMID: 19886869 Review.
- Normal oral, rectal, tympanic and axillary body temperature in adult men and women: a systematic literature review. Sund-Levander M, Forsberg C, Wahren LK. Sund-Levander M, et al. Scand J Caring Sci. 2002 Jun;16(2):122-8. doi: 10.1046/j.1471-6712.2002.00069.x. Scand J Caring Sci. 2002. PMID: 12000664 Review.
- Home Monitoring for Fever: An Inexpensive Screening Method to Prevent Household Spread of COVID-19. Kim J, Threadcraft MA, Xue W, Yue S, Wenzel RP, Southwick FS. Kim J, et al. J Biotechnol Biomed. 2023;6(3):392-400. doi: 10.26502/jbb.2642-91280101. Epub 2023 Sep 27. J Biotechnol Biomed. 2023. PMID: 38745997 Free PMC article.
- A Pilot Study on the Safety and Adequacy of a Novel Ecofriendly Hemodialysis Prescription-Green Nephrology. Solomon D, Arumugam V, Sakthirajan R, Lamech TM, Dineshkumar T, Vathsalyan P, Senthilkumaran G, Krishna R, Shaji S, Gopalakrishnan N. Solomon D, et al. Kidney Int Rep. 2024 Feb 13;9(5):1496-1503. doi: 10.1016/j.ekir.2024.02.014. eCollection 2024 May. Kidney Int Rep. 2024. PMID: 38707836 Free PMC article.
- [Fever in rheumatological diseases]. Nies JF, Krusche M. Nies JF, et al. Z Rheumatol. 2024 Jun;83(5):341-353. doi: 10.1007/s00393-024-01505-y. Epub 2024 Apr 18. Z Rheumatol. 2024. PMID: 38634905 Review. German.
- Deciphering the relationship between temperature and immunity. Maloney E, Duffy D. Maloney E, et al. Discov Immunol. 2024 Jan 30;3(1):kyae001. doi: 10.1093/discim/kyae001. eCollection 2024. Discov Immunol. 2024. PMID: 38567294 Free PMC article. Review.
- Characterization of Plastic Scintillator Detector for In Vivo Dosimetry in Gynecologic Brachytherapy. Herreros A, Pérez-Calatayud J, Ballester F, Abellana R, Neves J, Melo J, Moutinho L, Tarrats-Rosell J, Serrano-Rueda S, Tagliaferri L, Placidi E, Rovirosa A. Herreros A, et al. J Pers Med. 2024 Mar 20;14(3):321. doi: 10.3390/jpm14030321. J Pers Med. 2024. PMID: 38541063 Free PMC article.
- Kluger MJ, Kozak W, Conn CA, et al. . Role of fever in disease. Ann N Y Acad Sci 1998; 856:224–33. - PubMed
- Boeck B.[ Diagnose im alten mesopotamien. Ueberlegungen zu graenzen und moeglichkeiten der interpretation keilschriftlicher diagnostischer texte.] Orientalistische Literaturzeitung 2009; 104:382–98.
- Geller MJ. West meets East: early Greek and Babylonian diagnosis. In: Horstmanshoff FJ, Stol M, eds. Magic and Rationality in Acient Near Eastern and Graeco-Roman Medicine. Leiden-Boston: Brill; 2004. - PubMed
- Heessel N.[Babylonisch-assyrische Diagnostik.] Muenster: Ugarit Verlag; 2000.
- Heessel N. Reading and interpreting medical cuneiform texts-methods and problems. Le Journal des Medecines Cuneiformed 2004; 3:6–7.
Publication types
- Search in MeSH
Related information
- Cited in Books
LinkOut - more resources
Full text sources.
- Europe PubMed Central
- PubMed Central
- Silverchair Information Systems
Miscellaneous
- NCI CPTAC Assay Portal
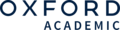
- Citation Manager
NCBI Literature Resources
MeSH PMC Bookshelf Disclaimer
The PubMed wordmark and PubMed logo are registered trademarks of the U.S. Department of Health and Human Services (HHS). Unauthorized use of these marks is strictly prohibited.
What’s Really the Average Human Body Temperature?
Long thought to be 98.6 degrees Fahrenheit, the measurement is highly personal and varies depending on time of day, among other factors, new research finds
/https://tf-cmsv2-smithsonianmag-media.s3.amazonaws.com/accounts/headshot/SarahKuta.png)
Daily Correspondent
:focal(3118x2078:3119x2079)/https://tf-cmsv2-smithsonianmag-media.s3.amazonaws.com/filer_public/55/57/5557e687-8141-4511-af48-4b0f9920e1f3/pexels-rdne-stock-project-8363035.jpg)
For decades, 98.6 degrees Fahrenheit has been the widely accepted “normal” average temperature for the human body.
But new research adds to the growing body of evidence that humans actually run a bit cooler. In addition, the study suggests there’s no such thing as a “normal” body temperature, because readings vary greatly depending on a variety of factors, from the person’s age to the time of day.
Researchers described these and other findings last month in JAMA Internal Medicine .
Scientists analyzed the oral temperature measurements of more than 126,000 adults seen at Stanford Health Care between 2008 and 2017. They also compiled information about each patient, such as their height, weight, sex and age, and made note of the time of day each temperature was taken.
They found the average human body temperature to be around 97.9 degrees, nearly a degree lower than 98.6. But are humans actually getting cooler? Maybe, but maybe not.
The belief in the 98.6 degree average dates back to 1868, when German physician Carl Wunderlich took more than a million temperature measurements from 25,000 people.
But a lot has changed in the world of scientific and medical research over the last 150 years, so holding up today’s studies against Wunderlich’s may not necessarily be an apples-to-apples comparison.
“Statistics were not in common use then, much less computers,” Philip Mackowiak , a physician at the University of Maryland, told National Geographic ’s Brian Gutierrez last year. “So how he could have processed a million data points and come up with the results that he did, it’s just impossible to imagine.”
Where on the body Wunderlich took the patients’ temperatures may also have something to do with the difference. For instance, oral temperatures tend to be lower than rectal temperatures.
In addition, 98.6 degrees was simply the number that stuck in the public consciousness when, in reality, Wunderlich reported a range of temperatures. He also noted that measurements varied depending on the time of day, as well as patients’ age and sex.
“Instead of thinking about a distribution in temperatures, which is what the initial study showed, we’ve taken a mean of 98.6 F and used it as a cutoff value,” says Catherine Ley , an epidemiologist at Stanford University who co-authored the new study, in a statement . “We’ve used an average value to create a false dichotomy of what’s normal and what’s not.”
It is also possible that average body temperatures have dropped in recent decades because, by and large, humans are getting healthier. Thanks to advances in medicine and dentistry, patients are dealing with less inflammation than they likely were 150 years ago, reports the New York Times ’ Dana G. Smith.
The new study also finds variations in temperature depending on patient characteristics and time of day. For example, women tend to run hotter than men. Older people tend to have lower temperatures than younger people. And, among all demographics, temperatures tend to be lower in the morning and higher in the afternoon. (If you’re curious about what your temperature might be throughout the day, the team also built an online personalized temperature range calculator using their findings.)
An elevated temperature, or fever, suggests the body might be fending on some sort of infection—and that means the immune system is doing its job. Fevers are “actually not necessarily something we have to eliminate at all times," says Angela Mattke , a pediatrician at the Mayo Clinic, to CBS News ’ Aliza Chasan.
But, since there’s so much variability in body temperature, a high reading for one person could be normal for someone else. Taken together, these and other recent findings suggest doctors should rethink the temperature benchmarks they use to determine when a patient is healthy and when something might be wrong. Individualized temperature evaluations and personalized fever thresholds might be more useful than comparing everyone to the average, the researchers say. For example, a doctor might consider how a patient’s current temperature compares to their historical readings.
“Most people, including many doctors, still think that everyone’s normal temperature is 98.6,” says study co-author Julie Parsonnet , also an epidemiologist at Stanford, in a statement. “In fact what’s normal depends on the person and the situation.”
Get the latest stories in your inbox every weekday.
/https://tf-cmsv2-smithsonianmag-media.s3.amazonaws.com/accounts/headshot/SarahKuta.png)
Sarah Kuta | READ MORE
Sarah Kuta is a writer and editor based in Longmont, Colorado. She covers history, science, travel, food and beverage, sustainability, economics and other topics.
January 17, 2020
Are Human Body Temperatures Cooling Down?
A new study finds that they have dropped, on average, over the past century and a half
By Karen Weintraub
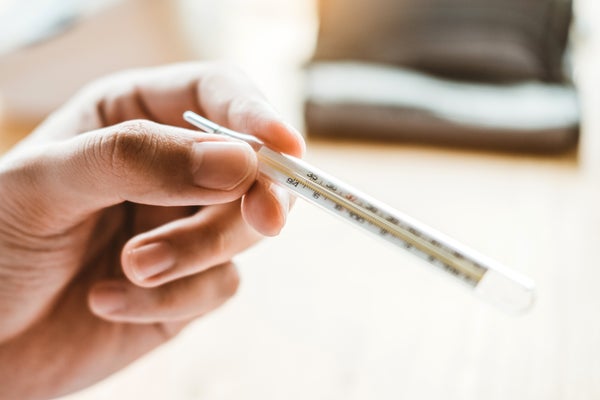
Sarinya Pinngam Getty Images
It is one of those facts of life that we learn early and don’t forget: normal body temperature is 98.6 degrees Fahrenheit. But a new study in eLife argues that that number is outdated.
The figure was probably accurate in 1851, when German doctor Carl Reinhold August Wunderlich found it to be the average armpit temperature of 25,000 patients. Times have changed, though, according to the recent paper : the average American now seems to run more than a degree F lower.
Stanford University researchers looked at data from Civil War soldiers and veterans and two more recent cohorts to confirm that body temperatures among American men averaged around 98.6 degrees F back then but have steadily fallen over time and that temperatures among women have fallen as well. Their data find an average for men and women of 97.5 degrees F.
On supporting science journalism
If you're enjoying this article, consider supporting our award-winning journalism by subscribing . By purchasing a subscription you are helping to ensure the future of impactful stories about the discoveries and ideas shaping our world today.
The study suggests that in the process of altering our surroundings, we have also altered ourselves, says senior author Julie Parsonnet of Stanford. “We’ve changed in height, weight—and we’re colder,” she says. “I don’t really know what [the new measurements] mean in terms of health, but they’re telling us something. They’re telling us that we are changing and that what we’ve done in the last 150 years has made us change in ways we haven’t before.”
The researchers did not determine the cause of the apparent temperature drop, but Parsonnet thinks it could be a combination of factors, including warmer clothing, indoor temperature controls, a more sedentary way of life and—perhaps most significantly—a decline in infectious diseases. She notes that people today are much less likely to have infections such as tuberculosis, syphilis or gum disease.
In places like the U.S., people also spend more time in what scientists call the thermoneutral zone—an environment of climate-controlled temperatures that make it unnecessary to rev up the metabolic system to stay warm or to cool off, she says. That perpetually 72-degree-F office may feel cold to some, but it does not stress out the human body the way it would to spend the night in a 40-degree-F cave. It is unclear whether those who live closer to the way people did in the 1800s—with more infection or less climate control—have higher body temperatures.
Research on the Tsimané, indigenous people who live in lowland Bolivia, suggests that infections can boost average body temperature. A 2016 paper showed that infections accounted for about 10 percent of resting metabolism in that population and that lower metabolism was associated with slightly lower body temperature, says Michael Gurven, an anthropologist at the University of California, Santa Barbara, who conducted that study but was not involved in the new one. Yet even in healthy members of the Tsimané population, temperatures appear to have dropped between 2004 and 2018, he adds—a phenomenon he plans to investigate further.
Parsonnet says she suspects it might be healthier to have a lower metabolism and body temperature. And she hopes to explore that connection more in the future.
For the eLife study, she and her colleagues compared temperatures from three different data sets: a total of 83,900 measurements from the Union Army Veterans of the Civil War (UAVCW) cohort, collected between 1862 and 1930; 15,301 measurements from the National Health and Nutrition Examination Survey I (NHANES I), collected between 1971 and 1975; and 578,222 measurements from the Stanford Translational Research Integrated Database Environment (STRIDE), collected between 2007 and 2017. Figures for women were not available from the earliest data set but were collected from the two later cohorts, and the research showed that body temperature for men and women decreased steadily across the time periods.
Philip Mackowiak, an emeritus professor of medicine at the University of Maryland School of Medicine, who was not involved in the new study, says data from as far back as the Civil War is inherently suspect. “That’s not to say that what [the new study] found is not valid. It could be, but you just don’t know,” he says, because there are so many variables that could not be controlled for in the data set, such as whether soldiers and veterans were healthy when tested, where the thermometer was placed and what kind of instrument was used.
Even Wunderlich’s established 1851 result is questionable, Mackowiak says, because although he had a large database of patients, it is hard to know whether he measured temperature consistently or how he analyzed such a volume of information long before the invention of computers. And “the body is composed of a whole host of temperatures,” Mackowiak adds. The liver is the hottest part, and the surface of the skin is the coldest. Plus, he says, “there’s no ‘normal’ temperature; there’s a range of temperatures ,” with people running hotter later in the day than they do in the morning. Women also have higher temperatures on average than men, in part because they rise with ovulation.
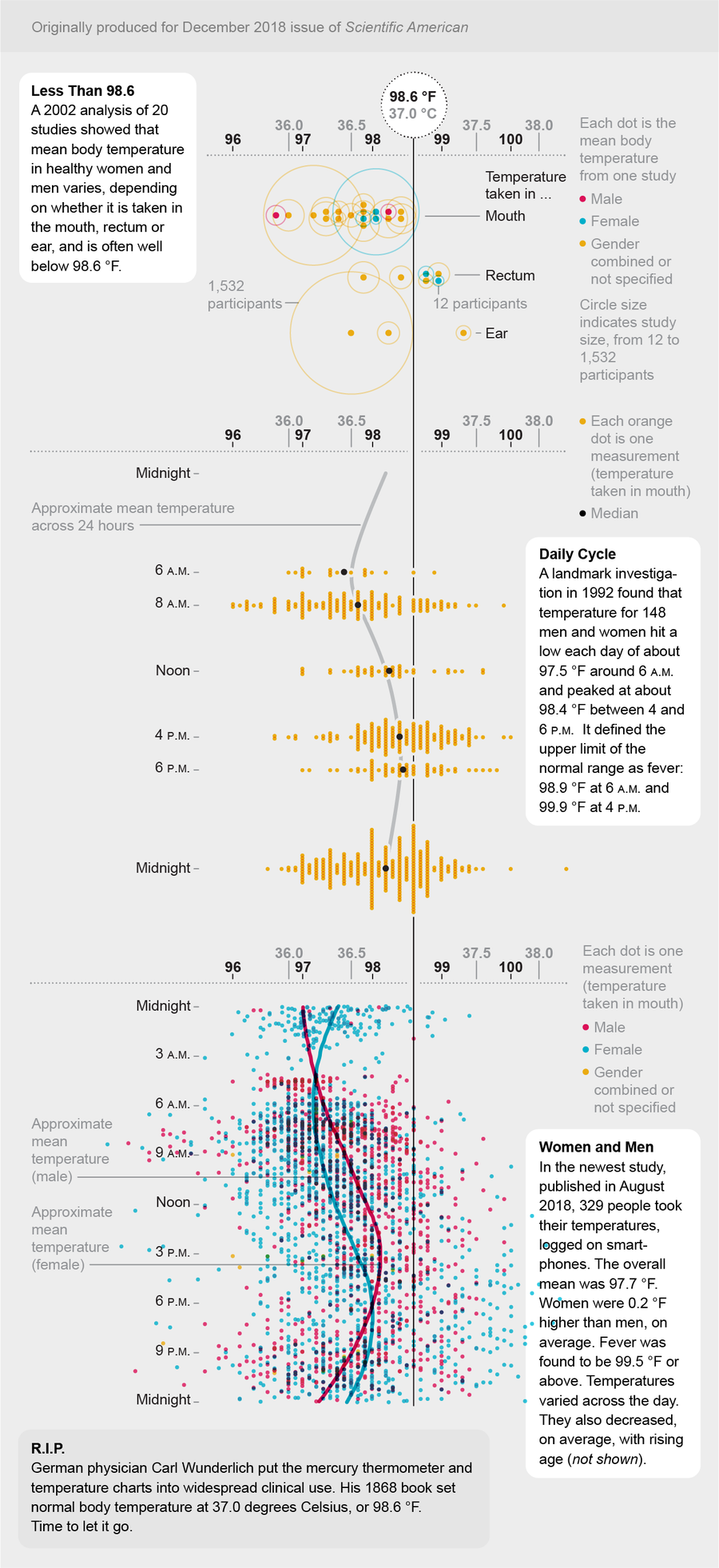
Credit: Nadieh Bremer; Sources: “Normal Oral, Rectal, Tympanic and Axillary Body Temperature in Adult Men and Women: A Systematic Literature Review,” by Märtha Sundlevander et al., in Scandinavian Journal of Caring Sciences , Vol. 16, No. 2; June 2002; “A Critical Appraisal of 98.6 °F, the Upper Limit of the Normal Body Temperature, and Other Legacies of Carl Reinhold August Wunderlich,” by Philip A. Mackowiak et al., in JAMA , Vol. 268, No. 12; September 23–30, 1992; “Using Smartphone Crowdsourcing to Redefine Normal and Febrile Temperatures in Adults: Results from the Feverprints Study,” by Jonathan S. Hausmann et al., in Journal of General Internal Medicine . Published online August 13, 2018
Parsonnet agrees that the Civil War data set has some limitations, such as where caregivers took the temperatures and whether they were careful or simply filled in 98.6 degrees F because that’s what they knew normal temperature was supposed to be. Those concerns were tempered, she says, by the fact that she and her team found a similar annual drop in temperature between the 1970s cohort and the current one. The effect was still present when examined by the soldiers’ and veterans’ year of birth rather than when the temperature was obtained, suggesting that the type of thermometer or the caregiver’s attitude could not explain the change. And within the data set, the researchers found the expected variation by age, weight and height, suggesting that the values were not random.
Even with the data’s limitations, the findings are compelling, according to Frank Rühli, founding chair and director of the Institute of Evolutionary Medicine at the University of Zurich, who says he reviewed the paper for eLife but was not involved in the research. “Human body temperature data going back that far—roughly 150 years—is very interesting,” he says. “It allows us to see short-term alterations of physiological traits in humans, which is quite rare.”
All the experts agree on one thing: a fever is still a fever . Lowering the average for normal body temperature does not mean that the standard for a fever—generally considered more than 100 degrees F for adults—should be changed, Mackowiak says. “Temperature can be helpful in determining whether or not you’re ill and, based on its level, how ill you might be,” he says. For patients, a bacterial infection plus a lower than normal temperature could be an even more ominous sign than a higher than normal one, he says. Following a rise or fall in temperature can also indicate whether you are getting better or how you are responding to medication, he adds, though “how you feel is the most important thing.”
The new study probably should not change the definition of fever, Rühli says. “But the variety of what is looked at as being normal should probably be adjusted.”
- First Aid & Emergencies
What Is a Normal Body Temperature?

What Is Body Temperature?
Your body's temperature comes from your body doing the work that keeps you alive, with most of your body's heat coming from your heart, brain, liver, and muscles.. Most of the time, your temperature will stay around the same level – though body temperature can change, depending on your activity level and time of day, among other things. When your body puts out a lot more or a lot less heat than usual, it’s trying to tell you there’s a problem.
Different Ways to Measure Body Temperature
There are several ways to measure body temperature. The best way may depend on the situation and whose body temperature is being measured.
Rectal temperature. This is the most accurate method for taking the temperature of babies and infants, especially those that are younger than 3 months old. Make sure to use a digital rectal thermometer with a short probe.
Oral temperature. You can also use a thermometer in your mouth to measure temperature. Make sure to clamp your lips together tightly and breathe through your nose.
Tympanic temperature. This is when you use a digital thermometer pointed into your ear. These thermometers are quick and easy to use, but they may not be as accurate as other methods.
Forehead temperature. You can use an electronic digital thermometer on your forehead to measure your temperature. You can also use another type of thermometer, called a noncontact infrared thermometer. Don’t use plastic strip thermometers that change color when you press them against your skin, because these are the least accurate method of measuring temperature.
Armpit temperature. You can also use any digital thermometer to take the temperature in your armpit if you don’t want a thermometer in your mouth, rectum, ear, or on your forehead. This is also called an axillary temperature.
What Are Normal Body Temperature Ranges?
Not everyone’s “normal” body temperature is the same. Yours could be a whole degree different from someone else’s. Your temperature doesn’t stay the same all day, and it will vary throughout your lifetime, too.
A German doctor in the 19th century set the standard at 98.6 F, but more recent studies say the baseline for most people may be decreasing.
Normal range of body temperature based on age
For a typical adult, body temperature can be anywhere from 97 F to 99 F. Adults over the age of 60 tend to have a lower body temperature, compared to younger adults. Babies and children have a wider range: 95.9 F to 99.5 F (if measured with an oral thermometer) or 97.9 F to 100.4 F (if measured by a rectal thermometer).
What is the normal forehead temperature for adults?
Your temperature reading may also change based on where on your body you measure it. Underarm readings can be a degree lower than what you’d find from your mouth . Rectal temperatures usually are up to a degree higher than mouth readings. If you are using a digital or noncontact infrared thermometer on your forehead, it can be slightly lower than mouth readings. But a normal temperature using a noncontact infrared thermometer will still be in the range of 97 F to 99 F for adults.
A body temperature higher than your normal range is a fever. It’s hypothermia when the body temperature dips too low. Both need to be watched.
Fever (High Body Temperature)
How high is too high when it comes to your temperature? Anything above 100.4 F is considered a fever. You may feel terrible, but on the whole, a fever isn’t bad for you. It’s a sign your body is doing what it should when germs invade. It’s fighting them off.
Fever in adults
There are different levels of severity of fevers in adults. These include:
- Low-grade fevers: 99.1 to 100.4 F
- Moderate-grade fevers: 100.6 to 102.2 F
- High-grade fevers: 102.4 to 105.8 F
If you're an adult with a fever of over 104 F, you should call your doctor.
If you have a fever, you might have other symptoms besides just feeling warm. These can include:
- Achy muscles or chest pain
- Hot, dry skin
- Nausea or vomiting
- A flushed face
- No interest in eating
Many fevers will go away within a few days. But you should call a doctor if you have any of these symptoms with a fever:
- Confusion or feeling sleepy or dull
- Loss of consciousness
- Trouble breathing
- Painful or burning urination
- Severe pain anywhere on your body
- A purple-spotted rash
Fever in children
Fevers in children can be tricky, and children may not be able to show that they feel uncomfortable. Signs of a fever in children can include:
- Looking red or flushed
- Feeling hot
- Feeling sweaty
- Losing their appetite
- Acting sleepy or cranky
- Complaining of a headache or body aches
Like in adults, in many cases a child’s fever can be managed at home. But you should contact your child’s pediatrician if your child is:
- Under 3 months old and has a rectal temperature of 100.4 F or higher
- Between 3 months and 3 years old and has a rectal temperature over 102 F
- Older than 3 years and has an oral temperature above 103 F
- Between 3 and 6 months old and – along with a fever – is fussier or more uncomfortable than usual, or doesn’t seem alert
- Sick enough for you to be concerned, regardless of what the thermometer says, or has a fever for longer than 3 days
How can you bring down a fever?
If you have had a high fever for 3 days or more, it is time to contact your doctor for help.
But if you have a moderate or low fever and are uncomfortable, there are some things you can try at home to bring down a fever.
- Take an anti-fever medicine, like acetaminophen or ibuprofen. Do not give a child or teenager with a fever aspirin, because it has been linked to a rare swelling in the liver and brain called Reye’s syndrome.
- Take a lukewarm bath or shower.
- Apply damp washcloths to the forehead and wrists.
- Drink fluids.
- Get a lot of rest.
Hypothermia (Low Body Temperature)
If your body loses too much heat, it can be very serious, even fatal. Hypothermia is when your body temperature goes below 95 F, and your body is losing heat faster than it can produce heat. You might think of hypothermia as something that only happens when you’re exposed to extremely cold weather for a long time. But it happens indoors, too. A below-normal body temperature can also be a sign that you’re sick.
Hypothermia in adults
Hypothermia is a special concern for the elderly. Older adults can struggle to keep their body temperature in a normal range if they’re somewhere with intense air conditioning or there’s not enough heat.
Other things can also make you more likely to get hypothermia. They include:
- Alcohol or drug use
- Hypothyroidism (an underactive thyroid )
- Sepsis (an overwhelming infection)
- Severe burns
- Parkinson’s disease
- Nerve damage
- Malnutrition
- Medicines such as antidepressants , antipsychotics, or sedatives
- Exposure to cold weather or cold water
Whether you are indoors or outdoors, the signs of hypothermia are similar. Hypothermia causes mental confusion, so someone who is hypothermic may not be aware that they need help. Symptoms of hypothermia can include:
- Shivering (this may stop at extremely low body temperature)
- Exhaustion or feeling tired
- Slurred speech
- Clumsiness or lack of coordination
- Pale and cold skin
- Slowed breathing or heart rate
- Memory loss
Hypothermia in children
Hypothermia is also particularly concerning for children. Babies may not be good at controlling their temperature. They can lose heat quickly. It’s important to keep them warm. A temperature below 97 F is considered too low for babies.
Symptoms of hypothermia in babies include:
- cold skin that looks flushed or bright red
How to treat hypothermia at home
Hypothermia is a life-threatening condition. If you suspect that you or someone that you’re with has hypothermia, you should call 911 right away or go to the emergency room.
While you’re waiting for emergency services to arrive, there are some things that you can do at home to help someone start getting warm. These include:
- Gently move the person into a warm room if you're outside.
- Remove any wet clothing the person is wearing, and replace it with dry clothing.
- If you have an electric blanket, use it to warm their chest, neck, and groin.
- You can also use skin-to-skin contact to warm someone with body heat while under loose blankets or sheets.
- If the person is alert, give them a warm beverage to drink. Do not give them any alcohol or caffeine.
- If the person is unconscious and not breathing, perform CPR until emergency services arrive. Keep trying to warm them while performing CPR.
Is 96 F a normal temperature for a kid?
If you’re measuring with an oral thermometer, the normal range for children’s temperatures is 95.9 F to 99.5 F. So 96 F would be within the normal range for a child. But for an infant, 96 F is too low. If your baby is under a year old and has a temperature below 97 F, call your pediatrician.
Things That Affect Normal Body Temperature
A lot of things can change your normal body temperature, making it higher or lower than 98.6 F. These include:
- How active you are
- What time of day it is
- The weather
- If you have any medical conditions
- What you’ve eaten or had to drink
- Where you are in your menstrual cycle
- What body part or method you're using to measure the temperature (U nderarm readings can be a degree lower than mouth readings, and rectal temperatures are usually up to a degree higher than ones taken in the mouth .)
Doctors used to think that the “normal” body temperature was 98.6 F, but we now know that there is a range of normal body temperatures for adults and children. Things including activity level, time of day, weather, age, and more can also impact your body temperature. A normal temperature for adults is in the range of 97 F to 99 F, and for children it is 95.9 F to 99.5 F. Higher temperatures than these are fevers and are caused by the body fighting off an infection. Lower temperatures can lead to hypothermia, a dangerous emergency condition.
Normal Body Temperature FAQs
What is the normal tympanic temperature range for adults? A normal tympanic (in the ear) temperature range for adults is between 95.7 F and 100 F.
What is normal toddler temperature? The normal temperature for a toddler between the ages of 1 and 3 is 95.9 F to 99.5 F, if you’re taking their temperature with an oral thermometer.
What is the normal body temperature for a newborn? The normal body temperature for a newborn is above 97 F and below 100.4 F. If your newborn falls outside of that range, take them to see a doctor right away.
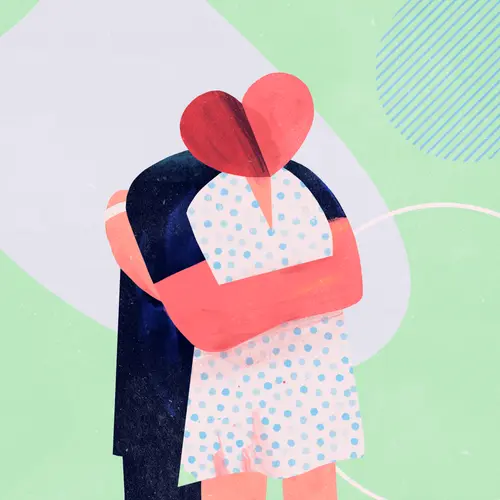
Top doctors in ,
Find more top doctors on, related links.
- First Aid News
- First Aid Reference
- First Aid Slideshows
- First Aid Videos
- First Aid Find a Doctor
- Find a Hospital
- First Aid A-Z
- How to Do CPR
- Common First Aid Conditions
- Symptom Checker
- More Related Topics
There's a Strange Link Between Depression And Body Temperature, Study Finds
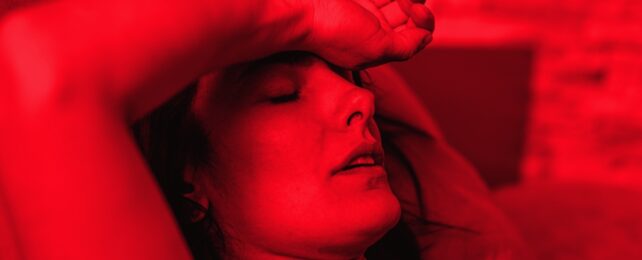
To better treat and prevent depression , we need to understand more about the brains and bodies in which it occurs.
Curiously, a handful of studies have identified links between depressive symptoms and body temperature, yet their small sample sizes have left too much room for doubt.
In a more recent study published in February, researchers led by a team from the University of California San Francisco (UCSF) analyzed data from 20,880 individuals collected over seven months, confirming that those with depression tend to have higher body temperatures.
As thorough as the study is – involving participants from 106 countries – it's not enough to show that a higher body temperature is causing depression , or indeed that depression is leading to the warming up of the body.
However, it does suggest that there's a connection here worth investigating. If something as simple as keeping cool could help tackle the symptoms of depression, then that has the potential to help millions of people around the world.
"To our knowledge, this is the largest study to date to examine the association between body temperature – assessed using both self-report methods and wearable sensors – and depressive symptoms in a geographically broad sample," said UCSF psychiatrist Ashley Mason when the results were published.
There could be a number of reasons for the link, the researchers think. It might be that depression is tied to metabolic processes that generate extra heat perhaps, or tied to cooling biological functions that aren't operating properly.
Or there might be a common shared cause, such as mental stress or inflammation that impacts both body temperature and depressive symptoms separately.
That's something future studies could look into. For now, we know that depression is a complex and multi-faceted condition, most likely with lots of different triggers , and body temperature could be playing a role.
Previous research has found that hot tubs and saunas can lessen the symptoms of depression, albeit in small sample groups. It's possible that the self-cooling this triggers, through sweating, is having a mental effect too.
"Ironically, heating people up actually can lead to rebound body temperature lowering that lasts longer than simply cooling people down directly, as through an ice bath," said Mason.
"What if we can track the body temperature of people with depression to time heat-based treatments well?"
The study data showed that as self-reported depression symptoms became more severe, body temperature averages got higher. There was also some association between higher depression scores and lower daily temperature fluctuations, but not to a statistically significant level.
With around 5 percent of people around the world thought to be living with depression, efforts to understand and effectively treat it are now more urgent than ever. Each new discovery brings more hope in tackling the problem.
"Given the climbing rates of depression in the United States, we're excited by the possibilities of a new avenue for treatment," said Mason.
The research has been published in Scientific Reports .
An earlier version of this article was published in February 2024.
- Mental Health
- Social Psychology
- Cognitive Science
- Psychopharmacology
- Neuroscience
Surprising link observed between body temperature and depression
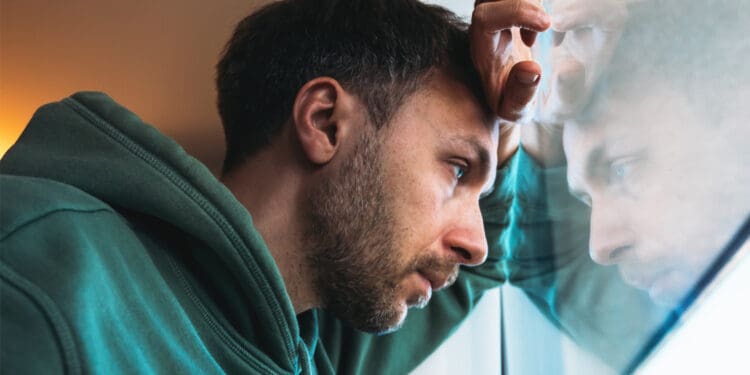
(Photo credit: Adobe Stock)
In a groundbreaking study that draws attention to the intersection between physiology and mental health, researchers have uncovered a link between depression and higher body temperatures. This discovery, published in Scientific Reports , not only deepens our understanding of depression but also hints at innovative treatments that might involve regulating body temperature to alleviate symptoms of the disorder.
The motivation behind this study was rooted in the alarming rise of depression rates globally and the pressing need for new treatment avenues. Depression, particularly major depressive disorder (MDD), has seen a surge in prevalence across various demographics, notably among youth and young adults in the United States.
This rise coincides with increased antidepressant usage, despite the limitations of these medications in terms of efficacy. Identifying physiological signatures unique to individuals with MDD could pave the way for developing targeted treatments, especially for those within a biologically homogeneous subgroup.
For their study, the researchers harnessed data from 20,880 individuals from the TemPredict Study, a prospective, worldwide cohort study initially designed to identify the onset of COVID-19 using physiological metrics collected by the Oura Ring, a wearable device. The TemPredict Study spanned several months, during which participants provided daily self-reported body temperature readings and completed monthly surveys assessing depression severity.
“To our knowledge, this is the largest study to date to examine the association between body temperature, assessed using both self-report methods and wearable sensors, and depressive symptoms in a geographically broad sample,” said Ashley Mason, an associate professor of psychiatry at UC San Francisco Weill Institute for Neurosciences and the study’s lead author. “Given the climbing rates of depression in the U.S., we’re excited by the possibilities of a new avenue for treatment.”
The use of the Oura Ring added a layer of precision and continuity to the data collection process. This wearable device, worn on the finger, measures distal body temperature every minute, providing a continuous stream of data on the participants’ thermal state. This allowed the researchers to analyze not just static temperature readings, but also the dynamic changes in temperature throughout the day and night.
The researchers found that higher levels of depressive symptoms were consistently associated with higher body temperatures. This correlation was observed across both self-reported data and minute-level temperature data collected by the Oura Ring. This pattern suggests a robust relationship between elevated body temperature and the presence of depressive symptoms, reinforcing the hypothesis that thermoregulatory dysregulation may play a role in depression.
“Though there are studies from decades ago documenting a correlation between depression and body temperature, those studies were small, often with 10-20 people in them,” Mason said. “This study that we have just published shows this correlation in a much larger sample, and will hopefully inspire more work into the mechanisms that underpin this correlation.”
Moreover, the analysis of wearable sensor data revealed nuanced details about the thermoregulatory patterns associated with depression. The study identified smaller differences between awake and asleep distal body temperatures in individuals with more severe depressive symptoms. This finding indicates that depression may affect the body’s ability to regulate temperature across different states of consciousness, potentially impacting the natural cooling processes that facilitate sleep onset and quality.
The consistency of these temperature elevations, particularly during periods that are crucial for thermoregulatory cooling, underscores the potential significance of body temperature as a physiological marker of depression.
Interestingly, the study also explored the diurnal amplitude of distal body temperature, which refers to the variation in temperature between daytime and nighttime. Participants with depression showed lower diurnal temperature amplitudes, suggesting a blunted circadian rhythm in body temperature. This aligns with previous research indicating circadian rhythm disturbances in depression and adds further evidence to the complex relationship between sleep, thermoregulation, and mood disorders.
The researchers noted the potential for decreased ability to induce thermoregulatory cooling or increased metabolic heat production, or a combination of both, as mechanisms underlying the observed temperature elevations in individuals with depression.
But the findings do not establish a causal relationship between body temperature and depression. The study was unable to determine whether elevated body temperatures contribute to the development or exacerbation of depressive symptoms, or if depression leads to an increase in body temperature due to altered metabolic or thermoregulatory processes.
Future research directions include exploring the underlying biological mechanisms of this association, examining the potential of temperature-based interventions for depression, and investigating the impact of controlled body temperature modulation on depressive symptoms.
“The link is particularly fascinating because there are data showing that when people recover from their depression – regardless of how they got better – their temperature tends to regularize,” Mason explained. “Then we have newer data suggesting that temperature-based interventions may reduce depression symptoms.”
“For example, data have shown that using heat-based treatments, in particular infrared sauna, cause acute increases in body temperature. These increases in body temperature engage the body’s self-cooling mechanisms (think, sweating) and can lead to subsequent decreases in body temperature (we sweat, we cool ourselves down).”
“And one study showed that decreases in a person’s body temperature in the days after a single heat treatment correlated with decreases in their depression symptoms over that same time period,” Mason continued. “So what’s exciting here is that the link might operate in multiple ways – what’s new is that we might be able to intervene directly on body temperature to address depression symptoms.”
“We are actively studying heat treatments, in particular sauna treatments, as a body-based intervention for depression symptoms here at UCSF in the heart of San Francisco. We have an ongoing trial right now for individuals with clinical depression where we are pairing sauna treatments with cognitive behavioral therapy for depression. Interested parties can learn more here: https://www.sealab.ucsf.edu/heatbedstudy .”
The study, “ Elevated body temperature is associated with depressive symptoms: results from the TemPredict Study ,” was authored by Ashley E. Mason, Patrick Kasl, Severine Soltani, Abigail Green, Wendy Hartogensis, Stephan Dilchert, Anoushka Chowdhary, Leena S. Pandya, Chelsea J. Siwik, Simmie L. Foster, Maren Nyer, Christopher A. Lowry, Charles L. Raison, Frederick M. Hecht, and Benjamin L. Smarr.
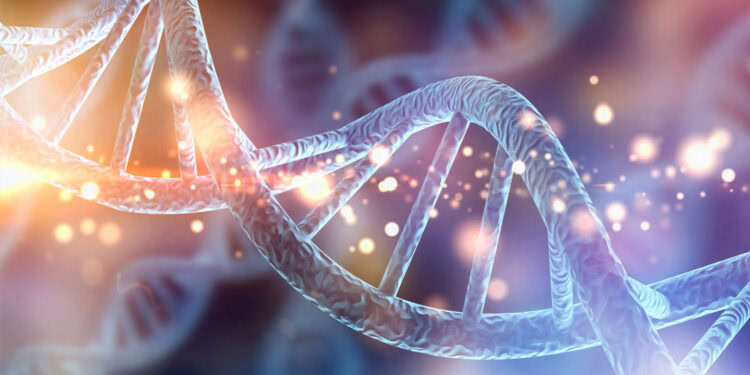
Genetic clues to depression revealed in large study
A study of over 14,000 people found that those with higher genetic risks for mental disorders reported more exposure to stressful events, challenging the simple distinction between reactive and endogenous depression.
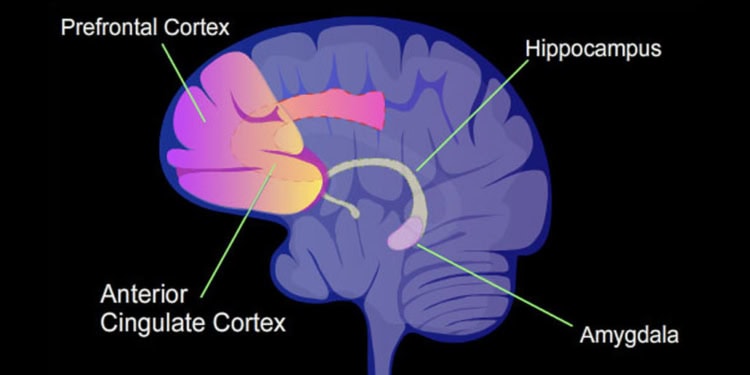
Amygdala response can predict treatment outcomes in difficult-to-treat depression
New research has found that lower amygdala activation to sad versus happy faces predicts poorer treatment outcomes in difficult-to-treat major depressive disorder, suggesting the brain's positive emotional processing is key to recovery.
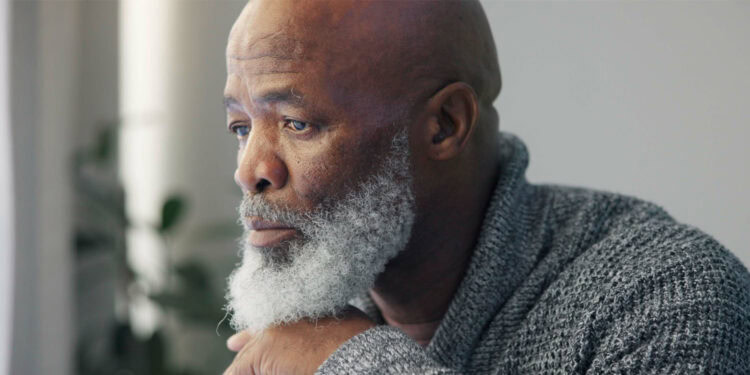
Bidirectional link found between depression and memory decline in older adults
A new study has found that depressive symptoms are linked to subsequent memory decline and poor memory is linked to subsequent increases in depressive symptoms in older adults.
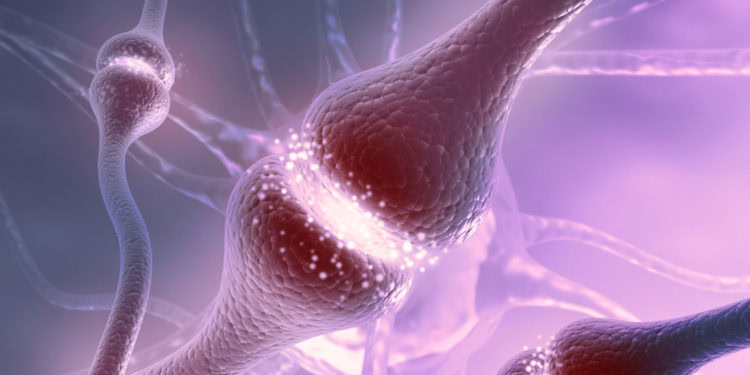
Intriguing connection found between serotonin and fertility
Serotonin neurons sense glucose and regulate reproductive hormones, maintaining fertility, according to a new study published in Scientific Reports.
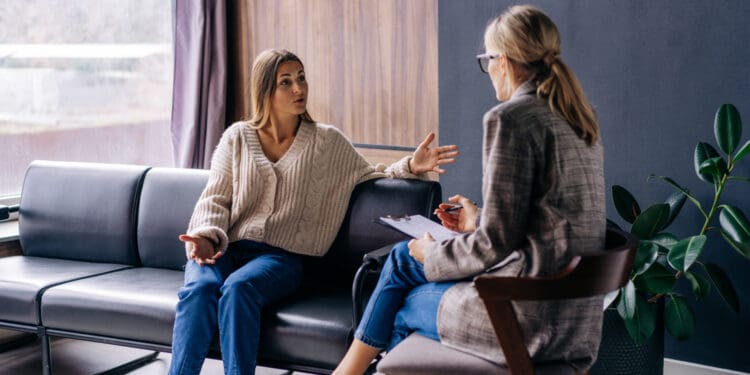
Psychotherapy frequency more crucial than session count, study suggests
Increasing the frequency of psychotherapy sessions significantly improves depression treatment outcomes, while the number of sessions has little impact, according to a recent study in the Journal of Affective Disorders.
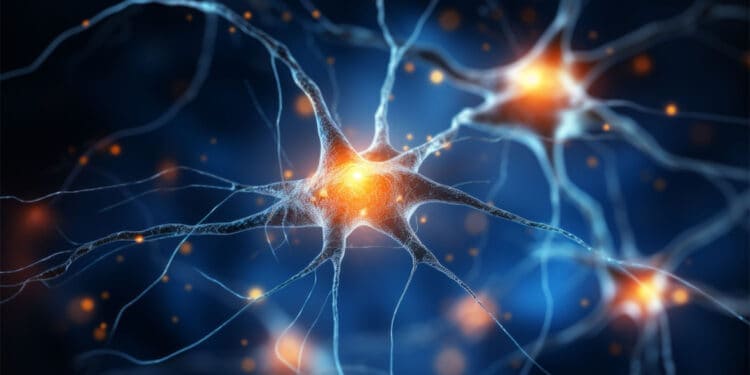
Researchers identify brain abnormalities in treatment-resistant depression
Researchers found that individuals with treatment-resistant depression exhibit heightened amygdala responses to negative emotions and reduced responses to positive ones, suggesting distinct brain mechanisms that could inform new, targeted treatments.
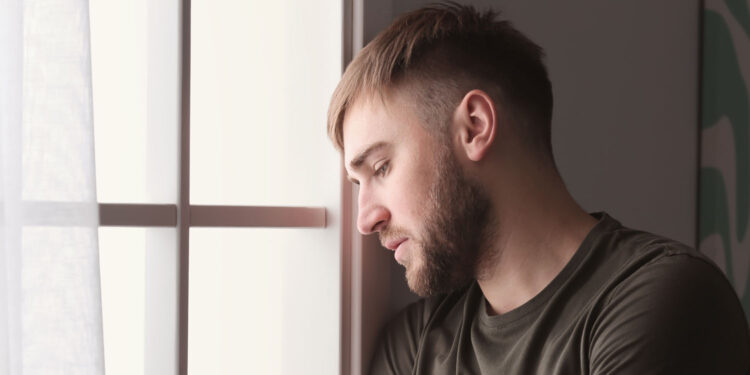
Fantasy proneness linked to greater sense of meaning in depressed individuals
New research suggests that for people with depression, engaging in vivid mental fantasies can help them find a sense of meaning in life. This offers an alternative way to create purpose when traditional sources of meaning, like relationships or work, are less effective.
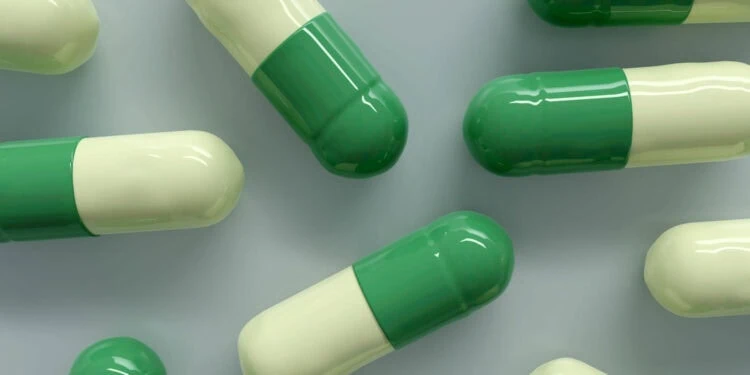
New study claims antidepressant withdrawal is less common than thought. But there’s a big problem
A review confirms antidepressant withdrawal exists but is less common and severe than thought. However, it likely underestimates symptoms in long-term users, who are most at risk.
STAY CONNECTED
Affective polarization: unpacking the emotional divide in politics, researchers shed light on the neurobiological mechanism behind mdma’s empathy-enhancing effects, individuals who were abused as children tend to have worse marital relationships as adults, service dogs appear to enhance mental health and social functioning for veterans with ptsd, video games help teens cope with academic stress, study suggests, dna methylation patterns reveal faster aging in children with mental health issues, why would shoppers prefer chatbots to humans new study pinpoints a key factor.
- Privacy policy
- Terms and Conditions
Welcome Back!
Login to your account below
Remember Me
Retrieve your password
Please enter your username or email address to reset your password.
Add New Playlist
- Select Visibility - Public Private
- Cognitive Science Research
- Mental Health Research
- Social Psychology Research
- Drug Research
- Relationship Research
- About PsyPost
- Privacy Policy
Information
- Author Services
Initiatives
You are accessing a machine-readable page. In order to be human-readable, please install an RSS reader.
All articles published by MDPI are made immediately available worldwide under an open access license. No special permission is required to reuse all or part of the article published by MDPI, including figures and tables. For articles published under an open access Creative Common CC BY license, any part of the article may be reused without permission provided that the original article is clearly cited. For more information, please refer to https://www.mdpi.com/openaccess .
Feature papers represent the most advanced research with significant potential for high impact in the field. A Feature Paper should be a substantial original Article that involves several techniques or approaches, provides an outlook for future research directions and describes possible research applications.
Feature papers are submitted upon individual invitation or recommendation by the scientific editors and must receive positive feedback from the reviewers.
Editor’s Choice articles are based on recommendations by the scientific editors of MDPI journals from around the world. Editors select a small number of articles recently published in the journal that they believe will be particularly interesting to readers, or important in the respective research area. The aim is to provide a snapshot of some of the most exciting work published in the various research areas of the journal.
Original Submission Date Received: .
- Active Journals
- Find a Journal
- Proceedings Series
- For Authors
- For Reviewers
- For Editors
- For Librarians
- For Publishers
- For Societies
- For Conference Organizers
- Open Access Policy
- Institutional Open Access Program
- Special Issues Guidelines
- Editorial Process
- Research and Publication Ethics
- Article Processing Charges
- Testimonials
- Preprints.org
- SciProfiles
- Encyclopedia
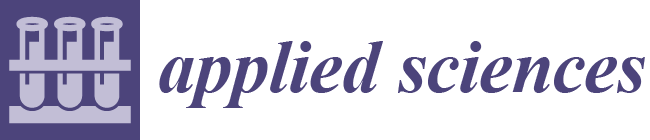
Article Menu
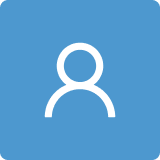
- Subscribe SciFeed
- Recommended Articles
- Author Biographies
- Google Scholar
- on Google Scholar
- Table of Contents
Find support for a specific problem in the support section of our website.
Please let us know what you think of our products and services.
Visit our dedicated information section to learn more about MDPI.
JSmol Viewer
Daily variation of body temperature: an analysis of influencing physiological conditions.
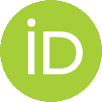
Share and Cite
Arrigo, F.; Arfuso, F.; Faggio, C.; Piccione, G. Daily Variation of Body Temperature: An Analysis of Influencing Physiological Conditions. Appl. Sci. 2024 , 14 , 5413. https://doi.org/10.3390/app14135413
Arrigo F, Arfuso F, Faggio C, Piccione G. Daily Variation of Body Temperature: An Analysis of Influencing Physiological Conditions. Applied Sciences . 2024; 14(13):5413. https://doi.org/10.3390/app14135413
Arrigo, Federica, Francesca Arfuso, Caterina Faggio, and Giuseppe Piccione. 2024. "Daily Variation of Body Temperature: An Analysis of Influencing Physiological Conditions" Applied Sciences 14, no. 13: 5413. https://doi.org/10.3390/app14135413
Article Metrics
Article access statistics, further information, mdpi initiatives, follow mdpi.
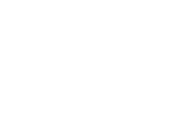
Subscribe to receive issue release notifications and newsletters from MDPI journals
Extreme heat is a global killer — and worse for our health than previously thought, new research shows
Heat records are being smashed, global death toll for 2024 is already staggering.
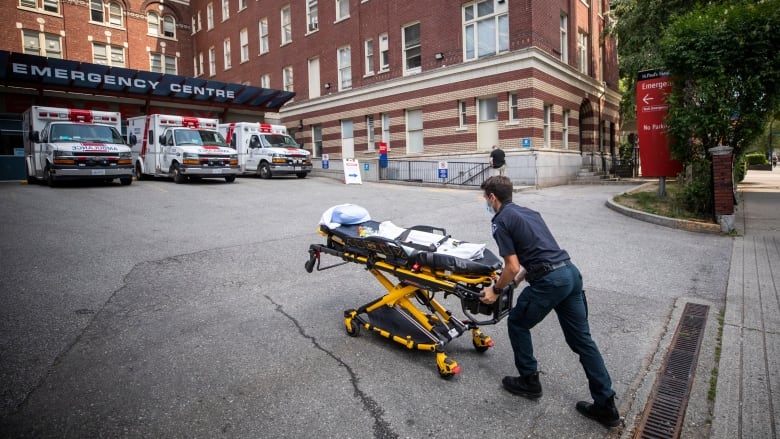
Social Sharing
This story is part of CBC Health's Second Opinion, a weekly analysis of health and medical science news emailed to subscribers on Saturday mornings. If you haven't subscribed yet, you can do that by clicking here .
More than 1,000 people died as temperatures hit nearly 52 C during this year's hajj in Saudi Arabia. Around 2,300 cases of heatstroke and dehydration were reported during Mexico's recent stretch of extreme heat. Eight known deaths occured in just 72 hours amid India's longest heatwave on record.
We're only halfway through 2024, yet the global death toll from surging temperatures has been staggering, and a clearer picture is now emerging of extreme heat as one of the deadly emergencies facing regions around the world.
It's no secret, of course, that high heat can kill you. Doctors have long warned that rising temperatures lead to conditions like heatstroke — a life-threatening medical emergency where your body simply can't stop overheating — or exacerbate underlying illnesses like diabetes, asthma, or cardiovascular disease.
What's new is just how many people are dying as global temperatures continue to rise, including across Canada and the northern U.S., where high heat previously wasn't a top-of-mind concern.
More than 100 Canadian heat records were smashed on Wednesday alone, all while emerging research reveals the toll of high heat on the human body is far worse than previously thought.
"Most [Canadians] would probably take a look at these headlines and think, oh, it doesn't really concern me," said Toby Mündel, a Canada Research Chair in Extreme Human Environments and a professor of kinesiology at Brock University in St. Catharines, Ont.
"Not only is it going to happen to you, but it's something that you can probably prepare for," Mündel said.
Extreme heat days, deaths both rising
A report last month by leading climate scientists examined 76 extreme heat waves across 90 countries over a 12-month period starting in May 2023.
In that period, 6.3 billion people — roughly 78 per cent of the population — experienced at least 31 days of extreme heat that were "made at least two times more likely due to human-caused climate change," the report said.
Andrew Pershing, vice-president of climate science at the U.S.-based Climate Central, one of the organizations behind the study, has been tracking the heat wave that blanketed a large part of the U.S. and eastern Canada over the past week.
- Heat waves linked to higher mortality rates, especially in cities with more renters
- Second Opinion Pregnant people, children face 'dire' health consequences from climate change, WHO warns
"This whole region is experiencing at least one day that's at Level 2 or Level 3, meaning it's twice as likely or three times as likely because of climate change," he said.
Such events, he said, "really signal how unusual this heat is, how there's a really strong tie to carbon pollution in the atmosphere."
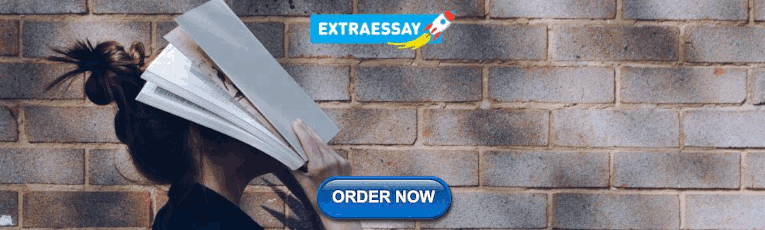
Vulnerable populations, renters at higher risk
These catastrophic climate shifts are spiking hospitalizations and hitting vulnerable populations hardest, causing higher death tolls among marginalized communities and certain ethnic groups.
One national study from Statistics Canada looking at deaths related to extreme heat events — which are defined differently city to city — found that between 2000 and 2020, roughly 670 more deaths than usual were recorded in the country's 12 largest cities during periods of extreme heat that lasted two days or longer, the research team said .
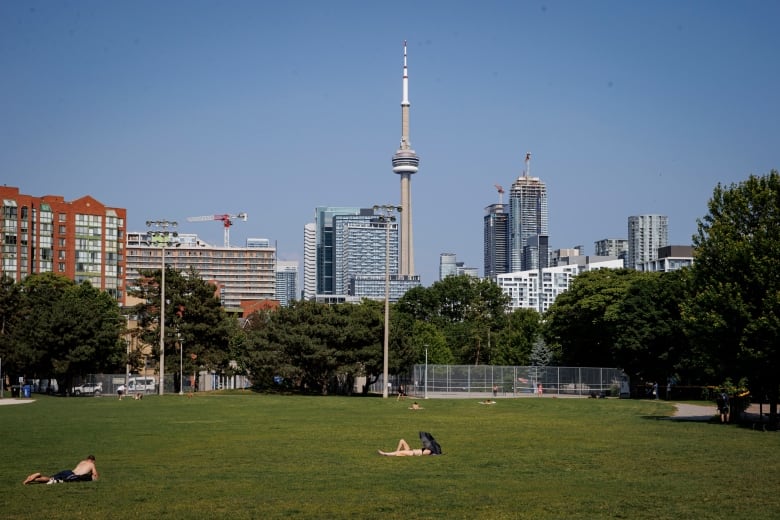
Cities where extreme heat events are less common saw the greatest spike in deaths, as did cities with more renters, which are less likely to have air conditioning.
South of the border, this year's heat-related death report for New York City offered another granular look at who actually dies during periods of high heat.
Black individuals have death rates two times higher than people who are white, the report noted, due to economic, health care and housing divides stemming from "past and current structural racism." The vast majority of people dying also have underlying health issues, with nearly nine in 10 heat deaths striking people with one or more chronic conditions.
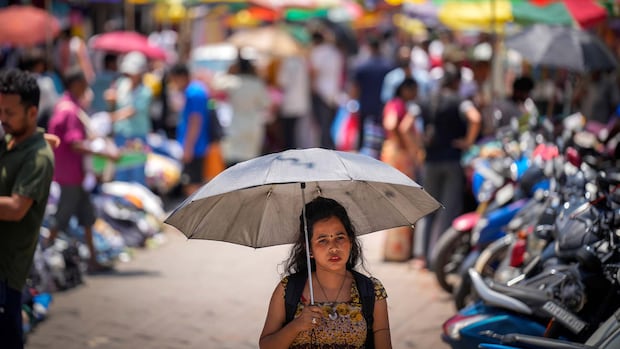
Delhi records all-time record temperature of 52.9 C
And the most important risk factor for heat-related deaths? Not having air conditioning, the New York team found. Among people exposed to heat in their own homes, zero deaths occurred between 2013 and 2022 among those with a working, operational AC, while every other death was among people who didn't have access to a functioning AC unit.
Those findings echoed data from the sweltering 2021 heat dome in B.C., with researchers noting several striking trends among the province's more than 600 heat-related deaths : many of the victims had chronic health conditions, the vast majority died in their homes and most of those at-home deaths were concentrated in lower-income neighbourhoods.
Serious health impacts
Heat waves have been linked to serious health effects, including higher suicide rates in the U.S. and Mexico, as well as higher rates of pre-term births in socioeconomically disadvantaged groups . Even the normal reaction of sweating can drive up the risk of heart attacks and strokes.
"We know that when we sweat a lot, our blood becomes thicker," explains Dr. Matthew Bennett, a cardiologist and chair of the Canadian Cardiovascular Society's planetary committee.
"With that increased concentration, there is a higher likelihood of clotting."
- 4 things to know about the 'oppressive' heat wave in Ontario, Quebec and Atlantic Canada
- Environment Canada says it can now rapidly link high-heat weather events to climate change
New Canadian research, led by Daniel Gagnon at the Montreal Heart Institute, looked into what happens in the heart when body temperatures rise. By heating up participants slowly in a modified bodysuit, Gagnon scanned their hearts to see what was going on.
Gagnon saw "the amount of blood that goes to the heart increases substantially during heat exposure" and that it took as little as half a degree to get healthy hearts working "60 to 70 per cent" of maximum capacity.
In some participants with coronary artery disease — blockages of the main pathways into the heart — raising the body's temperature led to ischemia, which Gagnon describes as a "mismatch" between the oxygen the heart needs versus what the blood vessels can supply. This can lead to arrhythmia, which is a known trigger of heart attacks.
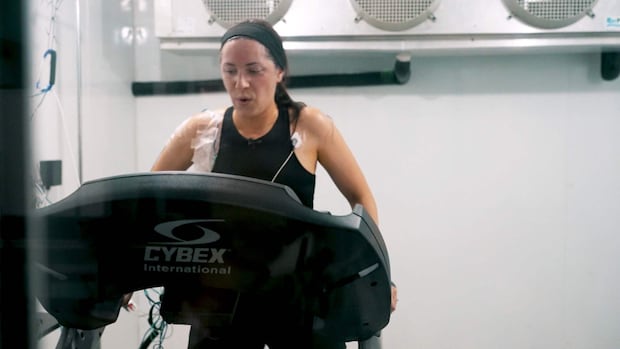
A 20-minute run in 40 C. What happens to the body in extreme heat
Heat can also cause the body to develop edemas (swelling), rashes, cramps and more dangerous conditions like dizziness and vomiting. Worse still, doctors say symptoms can present as other illnesses.
"Heat stroke, for example, can look a lot like severe infections," explained Dr. Anna Gunz, an intensive care pediatrician with Children's Hospital at London Health Sciences Centre. The latter is far more common and therefore more likely to be chosen by a doctor as a diagnosis.
As climate change increases the frequency of these heat events, experts are calling for more awareness in the medical community of heat-related health issues, along with further studies to understand who's most at risk.
- Study suggests hundreds of Quebec deaths each year are related to heat
- Temperatures soar to nearly 50 C across India in ongoing heat wave
Recent research does suggest there are unique health impacts on various groups. One Harvard team found heat increases the number of kids who visit emergency departments for any reason in the summer months, while another team studying pregnancy impacts recently identified links between higher temperatures and impaired fetal growth .
System-level solutions needed
What's crucial going forward is for countries to develop a multi-pronged approach to keep various populations safe in hot weather, according to Dr. Caleb Dresser, an assistant professor of emergency medicine at Harvard Medical School and director of healthcare solutions for the university's C-CHANGE centre for climate, health, and the global environment.
"Sometimes it's individual-level, like knocking on your neighbour's door to see how they're doing in the heat," he said. "Sometimes it's system-level action… like research to know when heat turns dangerous, what populations are at risk."
And, he added, those efforts need to be coupled with large-scale policy decisions, including aggressive reductions in fossil fuel use and greenhouse gas emissions, along with investments to ensure air conditioning and cooling centres become the norm.
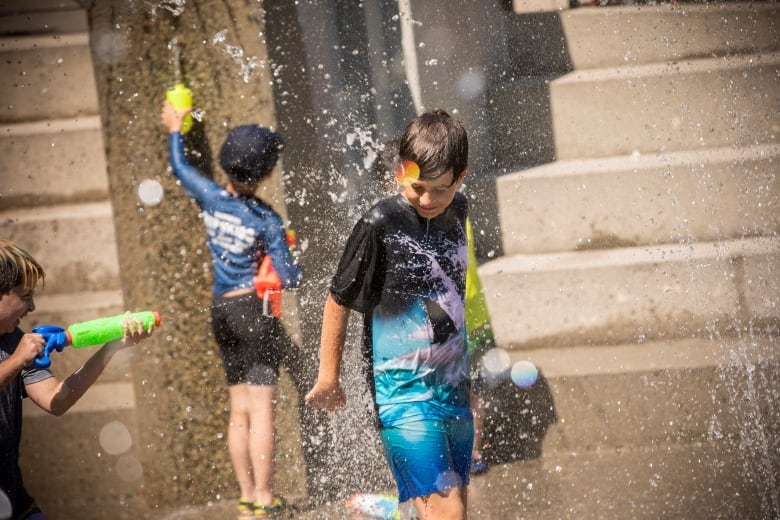
On that front, there's a growing movement in Toronto and elsewhere to legislate a maximum temperature in apartments, to ensure renters have access to a cool space.
Pershing, from U.S.-based Climate Central said unlike other extreme weather events made more common by climate change, the dangerous effects of heat are preventable.
"We know how to keep people cool," he said. "And so it really is like a resource problem. It's a system problem."
Related Stories
- Extreme heat days are overheating schools more often — and experts say it needs our attention
Add some “good” to your morning and evening.
A vital dose of the week's news in health and medicine, from CBC Health. Delivered to your inbox every Saturday morning.
This site is protected by reCAPTCHA and the Google Privacy Policy and Google Terms of Service apply.
Advertisement
How Heat Affects the Brain
High temperatures can make us miserable. Research shows they also make us aggressive, impulsive and dull.
- Share full article

By Dana G. Smith
- June 19, 2024
In July 2016, a heat wave hit Boston, with daytime temperatures averaging 92 degrees for five days in a row. Some local university students who were staying in town for the summer got lucky and were living in dorms with central air-conditioning. Other students, not so much — they were stuck in older dorms without A.C.
Jose Guillermo Cedeño Laurent, a Harvard researcher at the time, decided to take advantage of this natural experiment to see how heat, and especially heat at night, affected the young adults’ cognitive performance . He had 44 students perform math and self-control tests five days before the temperature rose, every day during the heat wave, and two days after.
“Many of us think that we are immune to heat,” said Dr. Cedeño, now an assistant professor of environmental and occupational health and justice at Rutgers University. “So something that I wanted to test was whether that was really true.”
It turns out even young, healthy college students are affected by high temperatures. During the hottest days, the students in the un-air-conditioned dorms, where nighttime temperatures averaged 79 degrees, performed significantly worse on the tests they took every morning than the students with A.C., whose rooms stayed a pleasant 71 degrees.
A heat wave is once again blanketing the Northeast, South and Midwest . High temperatures can have an alarming effect on our bodies , raising the risk for heart attacks, heatstroke and death, particularly among older adults and people with chronic diseases . But heat also takes a toll on our brains, impairing cognition and making us irritable, impulsive and aggressive.
How heat hurts our cognition
Numerous studies in lab settings have produced similar results to Dr. Cedeño’s research, with scores on cognitive tests falling as scientists raised the temperature in the room. One investigation found that just a four-degree increase — which participants described as still feeling comfortable — led to a 10 percent average drop in performance across tests of memory, reaction time and executive functioning.
We are having trouble retrieving the article content.
Please enable JavaScript in your browser settings.
Thank you for your patience while we verify access. If you are in Reader mode please exit and log into your Times account, or subscribe for all of The Times.
Thank you for your patience while we verify access.
Already a subscriber? Log in .
Want all of The Times? Subscribe .

Issue in Review: The Impact of Rising Temperatures on Human Health
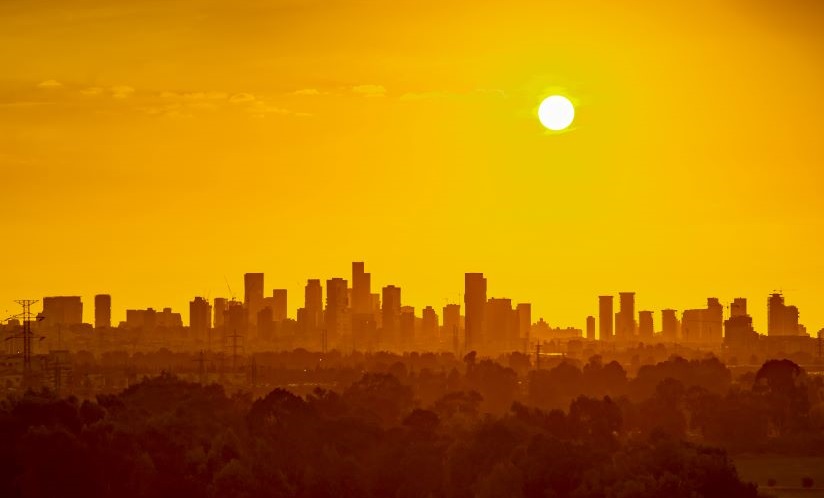
By Sarah Timbie
Summer 2024 is kicking off with an intense heat wave in the U.S. Extremely high temperatures were felt across the South, Midwest, and Northeast last weekend and into the following week, with some areas reaching 100 degrees. On Tuesday morning, June 18, more than 76 million people across the United States were put under heat alerts. Moving into the weekend, the country will continue to heat up, with record-breaking temperatures expected for areas of the Northeast.
The frequency and severity of heat waves in the U.S. have increased from an average of 2.2 heat waves lasting around three days each in the 1960s to six per season lasting four days each in the 2010s and 2020s. More than 2,300 people died from the effects of excessive heat in summer 2023, and the toll of 2024 is likely to be even worse. This past May has been the twelfth consecutive record-warm month, as global surface temperatures have been the warmest since the earliest recordings in 1850.
With increased temperatures threatening the health of millions of Americans, it is essential to be aware of the health risks heat poses and who is most vulnerable to their effects. Below, find some recent Rollins research about heat's impacts on health.
Heat Waves Increase Risk of Early-Term Birth
As heat waves become more common and severe, birthing parents are at a higher risk of experiencing preterm and early-term labor. A recent study , co-led by researchers at Rollins, analyzed 53 million births in 50 of the most populous U.S. metropolitan areas from 1993-2017. They found that the rates of preterm and early-term births increased during periods of abnormally high heat. Preterm and early-term births are leading causes of infant mortality and long-term health issues. As heat waves continue this summer, pregnant individuals may benefit from increased attention to keeping cool.
Temperature Variability Increases Risk of Cardiovascular Events
Climate change is causing increased frequency and intensity of heat waves and more dramatic temperature changes. This variation can occur within a single day or over the course of a few days. A Rollins-led study published earlier this year highlights the association between increased variation in temperature over three-day periods and cardiovascular health events, showing the link between heart health and temperature shifts. Stroke and peripheral vascular disease in particular were highly connected to increased temperature variability. Increased risk of negative cardiovascular health outcomes due to temperature fluctuation is of highest concern during October and March through May, and for populations older than 65 years of age.
As Temperatures Warm, Dementia-Related Hospital Visits Rise
Alzheimer's disease and dementia affect the lives of millions of Americans and their families. Recent research led by Rollins faculty has revealed a possible link between warmer temperatures and an increased risk of negative health outcomes related to Alzheimer’s disease and dementia. While the specific mechanism behind this link is mostly unknown, the results of this study show that people living with Alzheimer's disease or dementia represent a vulnerable population in the context of global warming and could benefit from additional protection from periods of increased heat.
Warmer Outdoor Temperatures Increase the Risk of Snakebites in Georgia
According to the World Health Organization, snakebites are considered a high priority health concern, but research on the environmental risk factors involved is lacking. A Rollins study from last year found a link between a one degree increase in daily temperature highs and an increase in emergency department visits for snakebites from 2014 to 2020. While only a handful of Georgia’s are venomous, the increase in frequency and severity of heat waves may call for keeping a more active eye out for snakes when spending time outside.
Associated Topics:
- Environmental Health
- Extreme Heat
- Rollins News
- In the Media
- Rollins Experts
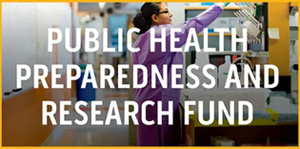
Thank you for visiting nature.com. You are using a browser version with limited support for CSS. To obtain the best experience, we recommend you use a more up to date browser (or turn off compatibility mode in Internet Explorer). In the meantime, to ensure continued support, we are displaying the site without styles and JavaScript.
- View all journals
- Explore content
- About the journal
- Publish with us
- Sign up for alerts
- Open access
- Published: 24 June 2024
Establishment and risk factor assessment of the abnormal body temperature probability prediction model (ABTP) for dairy cattle
- Tsair-Fwu Lee 1 , 2 , 3 , 4 , 5 ,
- Chien-Liang Chiu 1 ,
- Yen-Hsien Liu 1 ,
- Chu-Ho Chang 1 ,
- Jen-Chung Shao 1 , 2 ,
- Shih-Sian Guo 1 , 2 ,
- Yi-Lun Liao 1 ,
- Chia-Hui Chen 1 ,
- Chin-Dar Tseng 1 , 2 ,
- Pei-Ju Chao 1 , 2 &
- Shen-Hao Lee 1 , 2 , 6
Scientific Reports volume 14 , Article number: 14557 ( 2024 ) Cite this article
111 Accesses
Metrics details
- Engineering
- Health care
- Mathematics and computing
- Risk factors
The study aims to develop an abnormal body temperature probability (ABTP) model for dairy cattle, utilizing environmental and physiological data. This model is designed to enhance the management of heat stress impacts, providing an early warning system for farm managers to improve dairy cattle welfare and farm productivity in response to climate change. The study employs the Least Absolute Shrinkage and Selection Operator (LASSO) algorithm to analyze environmental and physiological data from 320 dairy cattle, identifying key factors influencing body temperature anomalies. This method supports the development of various models, including the Lyman Kutcher-Burman (LKB), Logistic, Schultheiss, and Poisson models, which are evaluated for their ability to predict abnormal body temperatures in dairy cattle effectively. The study successfully validated multiple models to predict abnormal body temperatures in dairy cattle, with a focus on the temperature-humidity index (THI) as a critical determinant. These models, including LKB, Logistic, Schultheiss, and Poisson, demonstrated high accuracy, as measured by the AUC and other performance metrics such as the Brier score and Hosmer–Lemeshow (HL) test. The results highlight the robustness of the models in capturing the nuances of heat stress impacts on dairy cattle. The research develops innovative models for managing heat stress in dairy cattle, effectively enhancing detection and intervention strategies. By integrating advanced technologies and novel predictive models, the study offers effective measures for early detection and management of abnormal body temperatures, improving cattle welfare and farm productivity in changing climatic conditions. This approach highlights the importance of using multiple models to accurately predict and address heat stress in livestock, making significant contributions to enhancing farm management practices.
Similar content being viewed by others
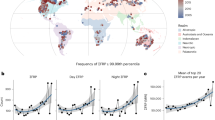
Increasing frequency and intensity of the most extreme wildfires on Earth
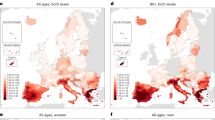
Heat-related mortality in Europe during the summer of 2022
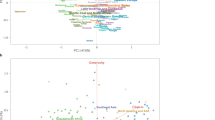
Principal component analysis
Introduction.
This study focuses on the impact of climate change-induced high temperature and humidity conditions on the survival challenges of lactating dairy cows, particularly how these conditions affect milk production. It investigates the effects of heat stress in subtropical climates, such as in the Southeastern United States and Taiwan, where it persists for half a year, causing significant health stress on dairy cows and considerable economic losses to the livestock industry 1 . To facilitate early detection and management of heat stress, Jaddoa et al.’s study 2 employed efficient and non-invasive infrared thermography to specifically monitor the temperature around a cow’s eye socket. This was done in conjunction with an automated body temperature monitoring platform developed by Guo et al. 3 . The study aims to develop an abnormal body temperature probability (ABTP) model for dairy cattle, providing a powerful tool for farm managers to protect cattle from environmental stress.
Existing methods for monitoring and managing heat stress in dairy cattle traditionally involve environmental modifications, like providing shade and ventilation, and behavioral observations, such as monitoring for signs of heat stress 4 , 5 . However, these methods have limitations in their accuracy and timeliness. They often rely on subjective assessments and may not detect early stages of heat stress 6 , 7 , 8 . In contrast, technological advancements like infrared thermography offer more precise, non-invasive, and real-time monitoring of cattle body temperature, allowing for earlier intervention 8 . These new methods represent a significant improvement in both detecting and managing heat stress, enhancing the welfare of dairy cattle and the overall efficiency of dairy farming operations.
In this study, we aim to develop a dairy cattle body temperature ABTP to assist farm managers in early identification and effective management of heat stress effects on dairy cattle due to high-temperature and high-humidity environments. This is crucial for enhancing the welfare and milk yield of dairy cattle. To achieve this, the research team utilized statistical methods such as the least absolute shrinkage and selection operator (LASSO) to analyze risk factors affecting body temperature anomalies 9 , 10 . LASSO is a widely used technique for prediction and variable selection, handles datasets with numerous variables by compressing and selecting the most predictive ones to enhance model accuracy. Additionally, biological growth models like Logistic, Schultheiss, and Lyman Kutcher Burman (LKB) model, which simulate growth processes and cellular characteristics 11 , 12 were employed to accurately predict dairy cows’ biological responses. These models help distinguish between low and high-risk assessment intervals, offering a new scientific approach to health management under heat stress, contributing to the sustainable development of livestock farming and animal welfare.
The study aims to develop a model capable of accurately predicting and providing early warnings for abnormal body temperatures in dairy cows. This model assists farm managers in better adapting to and addressing challenges brought by climate change. The research process is detailed in Fig. 1 , with further descriptions provided below.
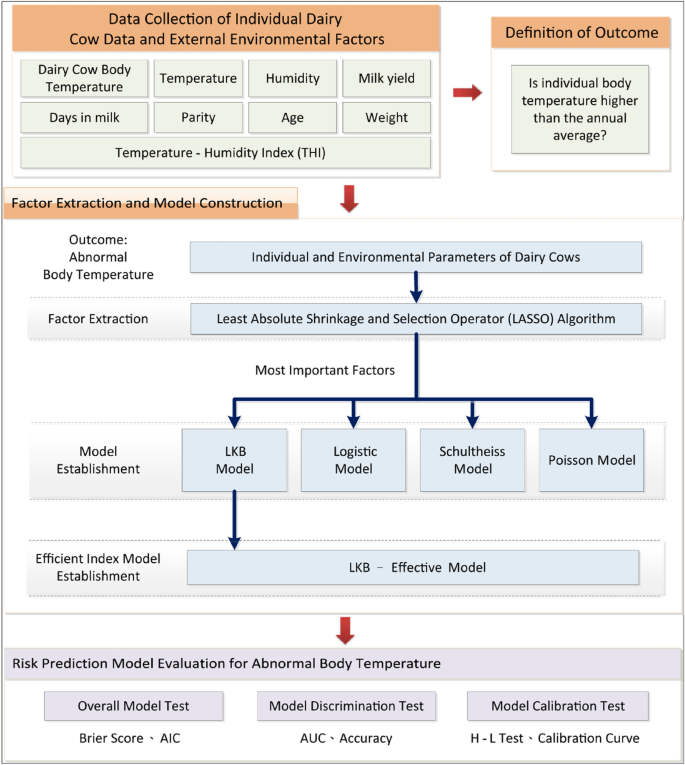
Diagram of the research process. LASSO: least absolute shrinkage and selection operator; LKB: Lyman Kutcher-Burman; AIC: Akaike’s entropic information criterion; AUC: Area Under Curve; H–L Test: Hosmer–Lemeshow test.
Dairy cattle body temperature and environmental data analysis
From July 2019 to April 2020, we collected data from 320 Holstein–Friesian cattle at a research dairy farm operated by the Council of Agriculture’s Animal Husbandry Research Institute 13 . The dataset comprehensively included individual cattle data such as body temperature, environmental temperature, humidity, age, days in milk, parity, body weight, and milk yield, all of which are meticulously detailed in Table 1 . This data was gathered using advanced tools such as the LWIR infrared thermal imager (A615 model, FLIR Systems Inc., MA, USA), known for its precision of temperature measurement within a margin of ± 2%. This instrumentation was rigorously calibrated using the SR800 blackbody furnace (CI Systems Inc, Israel) to ensure accurate temperature recordings. This methodological rigor supports the reliability of our data collection process, addressing concerns about the adequacy of our data gathering techniques.
In this study, methods were conducted according to the guidelines and regulations of our country. Since the experimental design involves capturing images through surveillance without direct contact, there’s no need for approval by an institutional review board for animal participants. Moreover, all methods adhere to the ARRIVE guidelines, ensuring transparency and repeatability.
The study utilized these data to determine if an individual cow’s body temperature deviated from the annual average, indicating potential anomalies. Factor extraction and model construction were subsequently undertaken using the LASSO algorithm to identify critical factors influencing body temperature anomalies. We developed various models, including LKB, Logistic, Schultheiss, and Poisson, to assess the risks associated with abnormal temperatures. The final stage of our research involved evaluating the predictive performance of these models using statistical measures such as Brier Score, Akaike Information Criterion (AIC), Area under the ROC curve (AUC), accuracy, Hosmer–Lemeshow (HL) test, and calibration curves. This extensive process aimed to establish an early-warning system to manage the impacts of climate change on dairy cattle health effectively.
Throughout the research process, rigorous quality control measures were implemented at each stage to ensure the reliability and accuracy of the findings 14 . During the data collection phase, temperature and humidity sensors underwent regular calibration to maintain their precision. In the factor extraction and model construction phase, the LASSO algorithm’s settings were fine-tuned to prevent overfitting. For model evaluation, cross-validation techniques were used to assess the models’ performance across various data subsets. Use a predefined threshold for performance metrics like AUC, Brier Score, and HL test to ensure consistency. This multi-layered approach to quality assurance underpins the robustness of our ABTP models.
In this study, a specific temperature-humidity index (THI) designed for dairy cows was utilized, which is a comprehensive indicator considering the effects of air temperature and humidity on heat stress. THI is crucial for assessing climate adaptability and is essential in analyzing the sensitivity of animals and humans to changes in temperature and humidity. The THI conversion formula (Eq. 1 ) 15 used in the study is as follows:
where T represents the temperature parameter in degrees Celsius (°C), and RH represents the humidity parameter, expressed as a percentage (%).
Application of LASSO and ABTP for dairy cattle
LASSO is effective in handling complex scenarios with multiple variables. This method compresses regression coefficients and ranks the importance of each factor. LASSO uses constraints to reduce some coefficients to zero, thereby identifying the most critical variables. The mathematical formula (Eq. 2 ) for LASSO provides a detailed approach for this selection and compression process.
where ‘d’ represents the number of selected factors, and ‘t’ is the coefficient for the constraint term.
Sigmoid curves are primarily used to describe the relationship between quantitative parameters and symptom prediction. Four different growth models were utilized to forecast the impact of specific variable parameters on the probability of abnormal body temperature. These models are: (1) LKB Model, (2) Logistic Model, (3) Schultheiss Model, and (4) Poisson Model. The mathematical formulas for these four models are detailed in Eqs. ( 3 )–( 6 ).
where ‘n’ refers to the important factor parameters selected by LASSO, ‘Tn 50 ’ is the quantitative parameter corresponding to a 50% probability of abnormal body temperature, and ‘m’ and ‘γ’ are parameters for the curve’s slope.
To optimize parameters in the dairy cattle abnormal body temperature probability prediction models, Maximum Likelihood Estimation (MLE) was employed to ensure the best fit with actual data. This involved comprehensive searching and step-by-step calculations, primarily aimed at minimizing residuals by analyzing sample data. The method is based on the Log-Likelihood estimation formula (Eq. ( 7 )).
where yi represents the observed data points, which can be 0 or 1, indicating the presence or absence of a characteristic or outcome. The pi denotes the predicted probability of the occurrence of the characteristic or outcome as predicted by the model for each data point i . This formula is used to measure the fit of the model to the observed data.
Effective index model (LKB eff ) for abnormal body temperature
The LKB eff model was originally proposed by Lyman et al. in 1985 for the field of radiation therapy, centers around a 50% probability threshold. It integrates radiation dosage calculations per unit volume and quantifies tolerance dosages for three types of complications 11 . In our study, we applied this effective index model to investigate the correlation between dairy cattle’s abnormal body temperature and key factors selected using the LASSO method. A curve response formula was utilized to determine the critical parameters causing a 50% probability of abnormal body temperature in the dataset. The detailed formula can be found in Eq. ( 8 ).
where ‘temp’ represents the dairy cattle body temperature parameter, where c > 0 indicates the relationship of change between body temperature and key factors.
In the LKB ABTP model, quantitative parameters ni are standardized, and their calculated ratios are applied to the corresponding effective temperature parameters \({Temp}_{eff}^{\left(i\right)}\) . If these quantified key factors equal the standard reference value, then \({Temp}_{i}\) will be equivalent to the effective temperature parameter \({Temp}_{eff}^{\left(i\right)}\) . The formula for effective temperature is detailed in Eq. ( 9 ). This approach involves normalizing each quantitative parameter ni and using these normalized values to calculate the corresponding effective temperature indices \({Temp}_{eff}^{\left(i\right)}\) .
When applying the aforementioned method to the LKB model 16 , 17 , relevant variables are substituted into Eq. ( 3 ), resulting in Eq. ( 10 ).
Figure 2 showcases the ABTP model from multiple perspectives. Part (a) identifies significant factors influencing the model. Part (b) offers insights into dairy cattle body temperature, while part (c) demonstrates the ABTP eff model at 50% and 20% probability levels. This composite view provides a nuanced understanding of how various factors interplay and influence study outcomes. To illustrate this interplay, consider how temperature and humidity combine into the THI, significantly impacting dairy cattle’s heat stress levels. As THI increases, so does the risk of heat stress, which our models can quantitatively assess. For example, consider the interaction between the THI and physiological responses of dairy cattle. As THI rises, indicating higher combined effects of temperature and humidity, it leads to an increase in the cattle’s body temperature. This elevated body temperature can trigger a series of stress responses such as reduced feed intake, lowered milk production, and increased susceptibility to health issues like heat exhaustion. These responses are quantitatively analyzed using our ABTP models, which incorporate both environmental THI and physiological data to assess the risk of heat stress. The model effectively predicts the likelihood of heat stress impacts, allowing for proactive management strategies to mitigate these effects. This demonstrates the complex interactions between climatic conditions and cattle health, underscoring the relevance of our integrated modeling approach in predicting and managing heat stress.
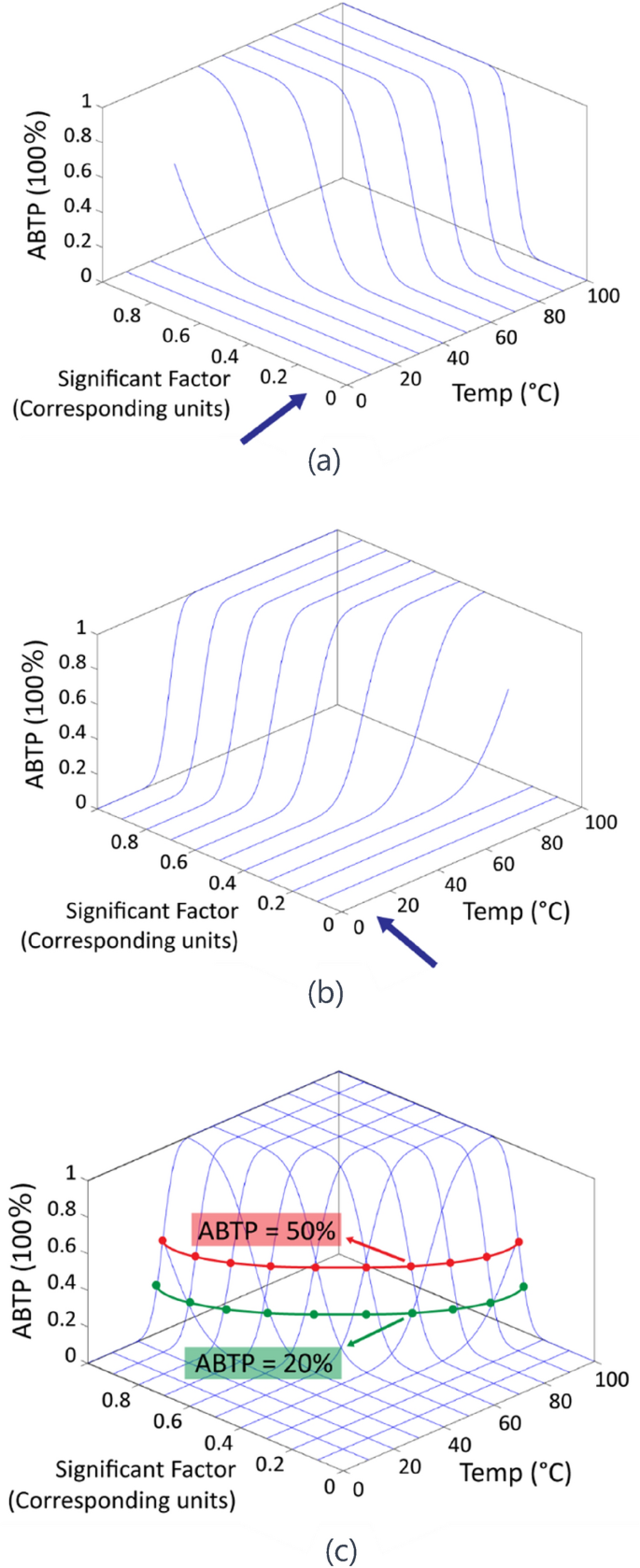
( a ) Significant Factors from the Perspective of the ABTP Model; ( b ) Dairy Cattle Body Temperature Perspective; ( c ) ABTP eff 50% and 20% Illustration. ABTP: Abnormal Body Temperature Probability, THI: Temperature-Humidity Index. The "two distinct three-dimensional models" refer to models that incorporate three dimensions of data: temperature, humidity (both contributing to the THI), and the physiological parameters of the dairy cattle like body temperature. These dimensions are crucial for evaluating the environmental and physiological factors that influence heat stress.
Additionally, the study employs unique three-dimensional models (shown in Fig. 2 a,b) to illustrate the data transformation process based on predefined formulas. By using the LASSO method to filter key variables, the ABTP—n eff and ABTP—temp eff models display curves associated with a 50% occurrence probability, forming a transverse arc curve that represents the effective temperature model trajectory. This enables rapid calculation of body temperature and key factors at different probabilities of temperature anomalies 11 .
In a subsequent phase, these 3D models are merged into a comprehensive three-factor model, depicted in Fig. 2 c, which integrates multiple dimensions to enhance data analysis, focusing on displaying the 50% and 20% probability scenarios in the ABTP eff model.
Evaluation method for the dairy cattle ABTP
Area under the roc curve (auc) and accuracy.
The performance evaluation of the ABTP prediction model includes testing for AUC and accuracy. The model’s predictive capability is assessed by comparing predicted probabilities with the actual occurrence of anomalies. In this study, samples with predicted probabilities exceeding 50% are classified as abnormal body temperature cases. The results allow for examining if samples conform to the model’s predictions, and the corresponding accuracy parameters are calculated 18 .
AIC model evaluation criterion (Akaike’s entropic information criterion)
AIC is a tool for evaluating statistical models, allowing the assessment and selection of the best model based on fundamental properties and parameter impacts. It’s calculated using log-likelihood estimates to evaluate differences between models 11 , 19 .
ΔAIC is computed by subtracting the AIC of the best-performing model (the one with the lowest AIC) from the AICs of the other models. In this analysis, the LKB model was used as the reference or baseline model.
Brier score
An indicator for evaluating the overall predictive performance of a model, where a smaller predictive error indicates better model fit 20 .
Calibration curve
Used to assess the consistency between actual observations and expected outcomes 21 . The ideal curve shows a 45-degree line, indicating higher accuracy of the predictive model.
Hosmer–Lemeshow test
Analyzes the similarity between model predictions and actual observations 22 . This test assesses the goodness of fit of a model. A result above 0.05 indicates that the model’s predicted values are not significantly different from the observed values, suggesting a good fit.
Ethical approval and consent to participate
This study integrates non-contact methods using surveillance footage for image capture, avoiding direct interaction and thus not involving animal subjects in a manner requiring Institutional Review Board approval. All methods were conducted following national guidelines and regulations to ensure transparency and reproducibility, adhering to the ARRIVE guidelines.
Results of factor selection using least absolute shrinkage and selection operator (LASSO)
In this study, LASSO was used to converge coefficients and rank them in order of importance as follows: (1) Temperature-Humidity Index (THI), (2) Temperature, (3) Parity, (4) Days in Milk, (5) Humidity, (6) Body Weight, (7) Age, and (8) Milk Yield. The convergence of LASSO coefficients is depicted in Fig. 3 a, while Fig. 3 b shows the number of factors selected as significant by the lassoglm method. The THI was identified as the most significant factor influencing abnormal body temperatures in dairy cattle, which was subsequently used as a quantitative parameter in the ABTP model. Analysis with the lassoglm equation confirmed THI as the key factor selected for predicting abnormal temperatures.
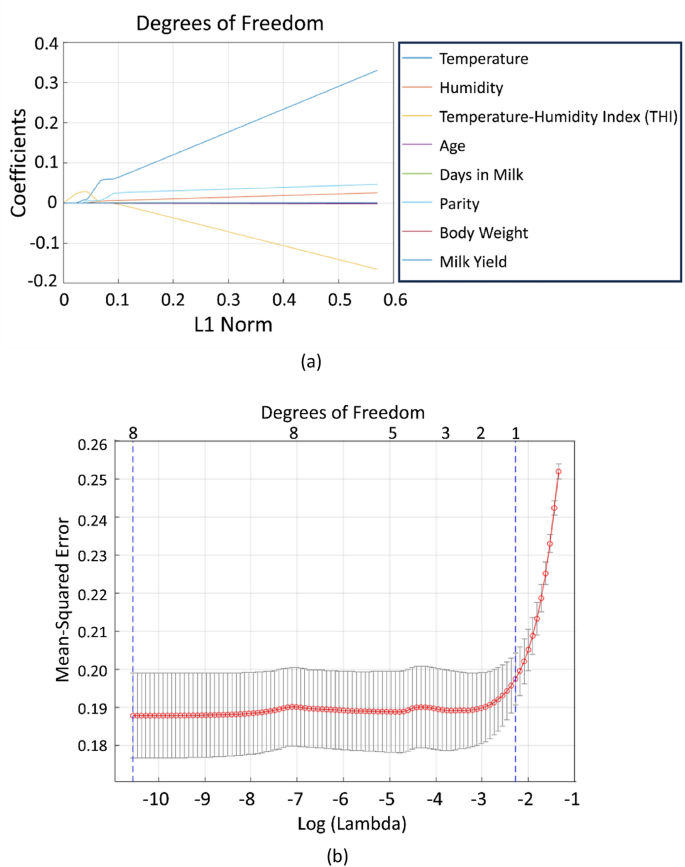
( a ) The convergence of LASSO coefficients ( b ) the selected number of factors from the lassoglm. LASSO: least absolute shrinkage and selection operator;
Figure 4 a illustrates the establishment and results of the LKB, Logistic, Schultheiss, and Poisson ABTP models, with TT50 values displayed at the bottom right of the graph. These visualizations provide an intuitive understanding of each model’s performance and characteristics, facilitating a comparison of their effectiveness. They lay the groundwork for further exploration and utilization of these models in research. Additionally, Fig. 4 b, which plots the relationship between the THI and the body temperature of dairy cattle across 320 data entries, demonstrates that as THI increases, so does the average body temperature of the cattle. TT50 and TT20 are defined as the THI values at which there is a 50% and 20% probability, respectively, of encountering abnormal body temperatures in dairy cattle.

( a ) Four Establishment of ABTP prediction models (LKB, Logistic, Schultheiss, and Poisson) ( b ) THI and dairy cattle body temperature relationship. ABTP: Abnormal body temperature probability, LKB: Lyman Kutcher-Burman, THI: Temperature-Humidity Index, \(\text{THI}=\left(1.8\times \text{T}+32\right)-(0.55-0.0055\times \text{RH})\times (1.8\times \text{T}-26)\) Where T represents the temperature parameter in degrees Celsius (°C), and RH represents the humidity parameter, expressed as a percentage (%). TT 50 represents the Temperature-Humidity Index at which there is a 50% probability of abnormal body temperature occurrence. The slope parameters for the four models are as follows: LKB: m, Logistic: γ50, Schultheiss: k, Poisson: γ.
Establishment and evaluation of the effective index model for abnormal body temperature
In the study, the ABTP—THI eff model was crafted utilizing the LKB ABTP model’s framework to pinpoint THI values associated with 20% and 50% probabilities of abnormal body temperature predictions. Key findings indicated that a THI value of 65 corresponds with a 20% probability of ABTP, while a THI of 75 aligns with a 50% ABTP. A critical body temperature parameter of 38.32 °C was identified.
Table 2 presents the performance evaluations of four ABTP models: LKB, Logistic, Schultheiss, and Poisson. These evaluations, including predictive power, accuracy, AIC, Brier score, HL-test, and calibration curves are essential for comparing the models’ effectiveness in predicting abnormal body temperatures in dairy cattle and provide important data for further research and application. Our results suggest that all models show similar AUC, accuracy, and calibration curve values, indicating comparable predictive abilities. Variations in AIC and Brier Score may suggest differences in model fit and prediction error, while the HL-Test results across models do not show significant differences, indicating all models have an acceptable fit.
As Fig. 5 illustrates, the study identified an abnormal body temperature threshold for dairy cattle at 38.32 °C using the ABTP eff model. Taking into account the precision and error margin of the thermal imaging device, the study defined normal body temperature as below 38.32 °C, body temperatures between 38.32 and 39.09 °C as warranting observation, and temperatures at or above 39.09 °C as abnormal, necessitating immediate notification. Furthermore, an environmental temperature-humidity alert is issued if the THI exceeds 75. The ABTP eff chart is thus divided into two color blocks, with the colored area providing a reference for farm management to control cattle body temperature and THI.

ABTP eff model usage instructions. ABTP: Abnormal body temperature probability, THI: Temperature-Humidity Index. TT 50 and TT 20 denote the Temperature-Humidity Indices corresponding to a 50% and 20% probability, respectively, of abnormal body temperature in dairy cattle.
The research presents significant findings regarding the ABTP model for dairy cattle, emphasizing the utility of the THI in predicting and managing heat stress. The study successfully integrates LASSO and biological growth models, such as LKB, Logistic, Schultheiss, and Poisson, to enhance the detection and management of heat-related risks. This provides a robust predictive framework that not only increases the accuracy of identifying heat stress but also offers actionable insights for improving farm management practices and cattle welfare.
The highlights of this study include: (1) Integration of infrared thermography and automated monitoring systems for real-time heat stress assessment in dairy cattle. (2) Application of the LASSO method to pinpoint significant predictors influencing body temperature. (3) Creation of the ABTP model, validated through statistical metrics like AUC and accuracy, to predict thermal stress effects. (4) Identification of the THI as a key environmental determinant. (5) Implementation of the ABTP model enhances farm management practices and animal welfare by providing early warning systems for heat stress management.
Our results indicate that all models demonstrate similar performance in terms of AUC, accuracy, and calibration curves, suggesting comparable predictive abilities for each. While variations in the AIC and Brier Score among the models highlight slight differences in model fit and prediction error, these differences are not substantial enough to decisively favor one model over another. Additionally, the HL Test results do not reveal significant discrepancies across the models, confirming that each has an acceptable fit for practical application. This comprehensive evaluation aids farm managers and researchers in selecting the appropriate model based on specific operational contexts, enhancing the utility of our findings in real-world scenarios where a balance between accuracy, model complexity, and computational efficiency is crucial.
A comparative analysis of the different ABTP models developed in this study. Each model—LKB, Logistic, Schultheiss, and Poisson—was designed with specific features that cater to diverse environmental and physiological variables: LKB Model : This model showed robust performance in environments with lower variability in temperature and humidity, which makes it particularly suitable for stable, temperate climates. Its strength lies in consistent predictions under uniform conditions 23 . Logistic Model : Proven to be highly effective in capturing non-linear relationships, this model excels in areas experiencing sudden changes in environmental conditions 24 . Its flexibility makes it ideal for regions with fluctuating weather patterns. Schultheiss Model : Best suited for regions with high humidity variations, this model offers reliable predictions in subtropical and tropical climates. Its sensitivity to humidity changes provides accurate forecasts, crucial for managing heat stress in such environments. Poisson Model : With its capability to handle large datasets with sparse extreme heat stress events, this model is valuable for large-scale dairy operations 25 . It ensures that predictions remain reliable even with infrequent occurrences of high heat stress levels.
The originality of this study lies in its interdisciplinary approach, combining advanced infrared thermal imaging technology, automated dairy cattle body temperature monitoring systems, and sophisticated statistical models. This comprehensive method brings innovation to the field of heat stress research. The integration of these technologies not only enhances the early detection of dairy cattle in heat stress conditions but also offers new strategies for sustainable livestock farming. In the context of global climate change, high temperatures and humidity have become major challenges in agriculture, particularly in livestock farming. These environmental changes affect animal physiology and agricultural productivity and profitability 26 . Thus, developing models that can accurately monitor and predict abnormal body temperatures in dairy cattle is crucial for improving farm management efficiency and animal welfare.
The ABTP prediction model, developed through LASSO analysis, identified key factors affecting dairy cattle body temperature, prioritizing THI 27 . This composite index of temperature and humidity renders individual factors like temperature more significant. Analyzing 320 data entries, the study confirmed THI’s correlation with body temperature. The model pinpointed the critical TT50 value, averaging 74.96, ranging from 74.44 to 75.29, indicating rising cattle body temperature with increasing THI.
Research findings similar to ours, such as those by Liu et al. 28 and Xue 29 , emphasize the impact of the Temperature-Humidity Index (THI) on dairy cattle. These studies show that high THI levels significantly influence milk yield and physiological responses like body temperature and food intake. They also highlight that THI alone does not fully capture the physiological changes in cattle under heat stress, suggesting the need for an integrated analysis of THI with physiological parameters to better understand the dynamics of milk production.
Xue’s findings indicate a complex, non-linear relationship between THI and rectal temperature, with significant increases observed as THI rises above 72. Complementary research from Taiwan’s Council of Agriculture confirmed a consistent correlation between orbital and rectal temperatures, suggesting a sharp increase in these temperatures when THI exceeds 70, emphasizing the importance of setting appropriate THI thresholds.
Peng et al.’s research corroborates these findings, revealing a high correlation between various body temperatures and environmental THI 30 . This establishes the reliability of measuring temperatures at multiple body locations under different THI conditions, highlighting a gap in precise quantitative data linking THI to body temperature changes, thus leading to the development of the LKB THIeff model to further refine our understanding.
According to data from Taiwan’s Central Weather Bureau, temperatures in spring, summer, and autumn seasons, excluding winter, often exceed 20 °C, approaching 30 °C 31 . Using the THI index, these seasons, except for winter, can easily reach heat stress thresholds. This indicates that dairy cattle may experience varying degrees of heat stress outside of winter. The study suggests that managing environmental temperature and humidity is crucial for farm management, as prolonged standing due to heat can lead to hoof diseases and discomfort in cattle. The THI index, considering seasonal variations in temperature and humidity, serves as an effective reference throughout the year, with a THI above 75 acting as a warning indicator for potential abnormal rises in cattle body temperature.
This research incorporates "Smart Dairy Farming Technology: Comprehensive Health Monitoring of Dairy Cattle via Infrared Temperature Sensing" from the Hsinchu Branch of the Livestock Research Institute, Council of Agriculture, Executive Yuan, as a fundamental reference for confirming dairy cattle body temperatures 32 . Infrared thermal imaging tests on the farms revealed an average eye-socket temperature of 38.1 °C in cattle. Based on the ABTP eff model, abnormal body temperature is defined as 38.32 °C, considering the thermal imager’s ± 2% accuracy and error margin. Normal temperatures are classified as below 38.32 °C, temperatures ranging from 38.32 to 39.09 °C require close observation, and temperatures exceeding 39.09 °C are deemed abnormal, necessitating immediate action.
In parallel, the study analyzed dairy cattle body temperature and environmental factors, confirming the THI as a crucial determinant of abnormal body temperatures. It established four predictive ABTP models—LKB, Logistic, Schultheiss, and Poisson—with an average TT 50 value of 74.96 (ranging from 74.44 to 75.29), which corresponds to a THI of 75, marking a significant risk threshold. This THI level serves as a quantitative alert indicator for a 50% risk of abnormal body temperature. Furthermore, surpassing a THI of 75 triggers environmental heat stress warnings, advocating for proactive measures such as enhanced ventilation or cooling sprays to mitigate high temperatures’ impact and ensure cattle welfare. This integrative approach aids in establishing comprehensive early warning parameters and a predictive framework that enhances cattle management practices under varying climatic conditions.
This study on heat stress management in dairy cattle through the ABTP models provides valuable insights but has certain limitations. The primary constraints include the limited sample size of 320 Holstein–Friesian cattle from a single farm, potentially limiting the generalizability of findings across different breeds and environments. Seasonal variations and their impacts were not thoroughly analyzed, which could affect model accuracy seasonally. The reliance on the THI as a primary predictor does not capture all environmental factors influencing body temperature. Additionally, measurement errors with an accuracy margin of ± 2% and the potential for statistical overfitting or underfitting with the LASSO method could affect the precision and reliability of the findings. Practical implementation challenges such as the need for additional resources and training for effective on-farm application also pose constraints.
To address these limitations, future research could involve expanding the sample size, enhancing environmental monitoring, and testing the models under varied conditions to improve their robustness and applicability. A significant expansion plan includes conducting field tests in various tropical regions, which would allow us to confirm the models’ effectiveness across a broader range of environmental conditions. This step is crucial for their practical application in global agricultural practices, ensuring the models are adaptable and effective in different climatic challenges faced by dairy farms worldwide.
This research introduces significant advancements in dairy cattle management under climate change by integrating innovative technologies and creating the novel ABTP model. The study developed multiple models, such as LKB, Logistic, Schultheiss, and Poisson, utilizing the THI to predict abnormal body temperatures and setting a critical temperature threshold of 38.32 °C. This approach not only advances scientific understanding but also provides practical tools for enhancing farm productivity and animal welfare, significantly contributing to agricultural practices and responsive health management in changing environmental conditions. Our findings offer practical solutions and methodologies that are directly applicable to improving the management of dairy cattle under changing climatic conditions, thereby filling a gap in current research and practice.
Data availability
The datasets used and/or analyzed during the current study available from the corresponding author on reasonable request.
St-Pierre, N., Cobanov, B. & Schnitkey, G. Economic losses from heat stress by US livestock industries. J. Dairy Sci. 86 , E52–E77 (2003).
Article Google Scholar
Jaddoa, M.A., Al-Jumaily, A., Gonzalez, L., Cuthbertson, H. Automatic eyes localization in thermal images for temperature measurement in cattle. in 2017 12th International Conference on Intelligent Systems and Knowledge Engineering (ISKE): 2017 : IEEE; 2017: 1–6.
Guo, S.-S. et al. Development of an automated body temperature detection platform for face recognition in cattle with YOLO V3-tiny deep learning and infrared thermal imaging. Appl. Sci. 12 (8), 4036 (2022).
Article CAS Google Scholar
Herbut, P., Angrecka, S. & Walczak, J. Environmental parameters to assessing of heat stress in dairy cattle—A review. Int. J. Biometeorol. 62 , 2089–2097 (2018).
Article ADS PubMed PubMed Central Google Scholar
Islam, M. A. et al. Automated monitoring of cattle heat stress and its mitigation. Front. Animal Sci. 2 , 60 (2021).
Sejian, V., Bhatta, R., Gaughan, J., Dunshea, F. & Lacetera, N. Adaptation of animals to heat stress. Animal 12 (s2), s431–s444 (2018).
Article CAS PubMed Google Scholar
Polsky, L. & von Keyserlingk, M. A. Invited review: Effects of heat stress on dairy cattle welfare. J. Dairy Sci. 100 (11), 8645–8657 (2017).
Lees, A. M. et al. The impact of heat load on cattle. Animals 9 (6), 322 (2019).
Article PubMed PubMed Central Google Scholar
Frizzarin, M. et al. Predicting cow milk quality traits from routinely available milk spectra using statistical machine learning methods. J. Dairy Sci. 104 (7), 7438–7447 (2021).
Kong, C. et al. LASSO-based NTCP model for radiation-induced temporal lobe injury developing after intensity-modulated radiotherapy of nasopharyngeal carcinoma. Sci. Rep. 6 (1), 1–8 (2016).
Google Scholar
Guo, S.-S. Comparison of dose-response characteristics of five normal tissue complication probability models through outcomes of radiation pneumonitis in breast cancer patients. Master’s Thesis in the Department of electronic engineering, National Kaohsiung University of Applied Sciences 2015.
Lee, T.-F. et al. Normal tissue complication probability modeling for cochlea constraints to avoid causing tinnitus after head-and-neck intensity-modulated radiation therapy. Radiat. Oncol. 10 , 1–8 (2015).
Article ADS Google Scholar
Sie, S.-J. Analysis of the influence of dairy cattle temperature and environmental parameters on milk yield for automated thermal imaging monitoring platform. Master’s Thesis in the Department of electronic engineering, National Kaohsiung University of Science and Technology 2021.
Westgard, J. O. Internal quality control: Planning and implementation strategies. Ann. Clin. Biochem. 40 (6), 593–611 (2003).
Article PubMed Google Scholar
Bohmanova, J., Misztal, I. & Cole, J. Temperature-humidity indices as indicators of milk production losses due to heat stress. J. Dairy Sci. 90 (4), 1947–1956 (2007).
Lyman, J. T. Complication probability as assessed from dose-volume histograms. Radiat. Res. 104 (2s), S13–S19 (1985).
Luxton, G., Keall, P. J. & King, C. R. A new formula for normal tissue complication probability (NTCP) as a function of equivalent uniform dose (EUD). Phys. Med. Biol. 53 (1), 23–36 (2008).
Congalton, R. G. A review of assessing the accuracy of classifications of remotely sensed data. Remote Sensing Environ. 37 (1), 35–46 (1991).
Bozdogan, H. Model selection and Akaike’s information criterion (AIC): The general theory and its analytical extensions. Psychometrika 52 (3), 345–370 (1987).
Article MathSciNet Google Scholar
Steyerberg, E. W. et al. Assessing the performance of prediction models: A framework for some traditional and novel measures. Epidemiology (Cambridge, Mass) 21 (1), 128 (2010).
Steyerberg, E. W. et al. Internal validation of predictive models: Efficiency of some procedures for logistic regression analysis. J. Clin. Epidemiol. 54 (8), 774–781 (2001).
Miladinovic, B. et al. External validation of a web-based prognostic tool for predicting survival for patients in hospice care. J. Palliative Care 29 (3), 140–146 (2013).
Stewart, R. D. & Li, X. A. BGRT: Biologically guided radiation therapy—The future is fast approaching!. Med. Phys. 34 (10), 3739–3751 (2007).
Bloom, J. Z. Tourist market segmentation with linear and non-linear techniques. Tourism Manag. 25 (6), 723–733 (2004).
Greenland, S., Mansournia, M.A., Altman, D.G. Sparse data bias: A problem hiding in plain sight. BMJ . 352 (2016).
Morash, A. J. & Alter, K. Effects of environmental and farm stress on abalone physiology: Perspectives for abalone aquaculture in the face of global climate change. Rev. Aquac. 8 (4), 342–368 (2016).
Gaughan, J., Mader, T. L., Holt, S. & Lisle, A. A new heat load index for feedlot cattle. J. Animal Sci. 86 (1), 226–234 (2008).
Liu, J., Li, L., Chen, X., Lu, Y. & Wang, D. Effects of heat stress on body temperature, milk production, and reproduction in dairy cows: A novel idea for monitoring and evaluation of heat stress—A review. Asian-Aust. J. Animal Sci. 32 (9), 1332 (2019).
Xue, B., Wang, Z., Li, S., Wang, L. & Wang, Z. Temperature-humidity index on performance of cows. China Anim. Husb. Vet. Med. 37 , 153–157 (2010).
MathSciNet CAS Google Scholar
Peng, D. et al. Infrared thermography measured body surface temperature and its relationship with rectal temperature in dairy cows under different temperature-humidity indexes. Int. J. Biometeorol. 63 (3), 327–336 (2019).
Article ADS CAS PubMed Google Scholar
Liu, W.-Y., Huang, Y.-H. & Hsieh, C.-M. The impacts of different climate change scenarios on visits toward the National Forest Park in Taiwan. Forests 11 (11), 1203 (2020).
Chen, Y.-H., Chen, Y.-H., Hsiao, F.-J. Smart dairy farming technology: Comprehensive health monitoring of dairy cattle via infrared temperature sensing. Scitechvista . (2017).
Download references
Acknowledgements
This study was supported financially by Grant from the Ministry of Science and Technology, Executive Yuan, Taiwan, ROC (111-2221-E-992-016-MY2).
Author information
Authors and affiliations.
Department of Electronic Engineering, National Kaohsiung University of Science and Technology, Kaohsiung, 80778, Taiwan
Tsair-Fwu Lee, Chien-Liang Chiu, Yen-Hsien Liu, Chu-Ho Chang, Jen-Chung Shao, Shih-Sian Guo, Yi-Lun Liao, Chia-Hui Chen, Chin-Dar Tseng, Pei-Ju Chao & Shen-Hao Lee
Medical Physics and Informatics Laboratory of Electronic Engineering, National Kaohsiung University of Science and Technology, Kaohsiung, 80778, Taiwan
Tsair-Fwu Lee, Jen-Chung Shao, Shih-Sian Guo, Chin-Dar Tseng, Pei-Ju Chao & Shen-Hao Lee
Department of Medical Imaging and Radiological Sciences, Kaohsiung Medical University, Kaohsiung, 80708, Taiwan
Tsair-Fwu Lee
PhD Program in Biomedical Engineering, Kaohsiung Medical University, Kaohsiung, 80708, Taiwan
School of Dentistry, College of Dental Medicine, Kaohsiung Medical University, Kaohsiung, 80708, Taiwan
Department of Radiation Oncology, Linkou Chang Gung Memorial Hospital and Chang Gung University College of Medicine, Linkou, Taiwan
Shen-Hao Lee
You can also search for this author in PubMed Google Scholar
Contributions
T-F L: Conceived and designed the analysis; collected the data; contributed data or analysis tools; performed the analysis; wrote the paper. C-L C: Contributed to the writing and editing of the paper. Y-H L: Analyzed and interpreted the data; contributed to the writing of the paper. C-H C: Contributed data or analysis tools; assisted in data interpretation. J-C S: Collected the data; contributed to the writing of the paper. S–S G: Assisted in the design of the analysis; contributed to the writing and revision of the paper; contributed to data analysis and interpretation. Y-L L: Assisted in the analysis and interpretation of data. C-H C: Assisted in the analysis and interpretation of data. C-D T: Participated in data collection and analysis; provided technical support for data management. P-J C: Provided expertise in machine learning algorithms; Participated in data collection; contributed to the preparation and revision of the manuscript. S–H L: Guided the analysis and interpretation of data; critically revised the manuscript for important intellectual content.
Corresponding authors
Correspondence to Pei-Ju Chao or Shen-Hao Lee .
Ethics declarations
Competing interests.
The authors declare no competing interests.
Additional information
Publisher's note.
Springer Nature remains neutral with regard to jurisdictional claims in published maps and institutional affiliations.
Rights and permissions
Open Access This article is licensed under a Creative Commons Attribution 4.0 International License, which permits use, sharing, adaptation, distribution and reproduction in any medium or format, as long as you give appropriate credit to the original author(s) and the source, provide a link to the Creative Commons licence, and indicate if changes were made. The images or other third party material in this article are included in the article's Creative Commons licence, unless indicated otherwise in a credit line to the material. If material is not included in the article's Creative Commons licence and your intended use is not permitted by statutory regulation or exceeds the permitted use, you will need to obtain permission directly from the copyright holder. To view a copy of this licence, visit http://creativecommons.org/licenses/by/4.0/ .
Reprints and permissions
About this article
Cite this article.
Lee, TF., Chiu, CL., Liu, YH. et al. Establishment and risk factor assessment of the abnormal body temperature probability prediction model (ABTP) for dairy cattle. Sci Rep 14 , 14557 (2024). https://doi.org/10.1038/s41598-024-65419-0
Download citation
Received : 15 February 2024
Accepted : 20 June 2024
Published : 24 June 2024
DOI : https://doi.org/10.1038/s41598-024-65419-0
Share this article
Anyone you share the following link with will be able to read this content:
Sorry, a shareable link is not currently available for this article.
Provided by the Springer Nature SharedIt content-sharing initiative
- Dairy cattle
- Least absolute shrinkage and selection operator (LASSO)
- Abnormal body temperature probability (ABTP)
- Temperature-humidity index (THI)
By submitting a comment you agree to abide by our Terms and Community Guidelines . If you find something abusive or that does not comply with our terms or guidelines please flag it as inappropriate.
Quick links
- Explore articles by subject
- Guide to authors
- Editorial policies
Sign up for the Nature Briefing: AI and Robotics newsletter — what matters in AI and robotics research, free to your inbox weekly.


Separating the genetic and environmental drivers of body temperature during the development of endothermy in an altricial bird
- Find this author on Google Scholar
- Find this author on PubMed
- Search for this author on this site
- ORCID record for Lucy A Winder
- For correspondence: [email protected]
- Info/History
- Supplementary material
- Preview PDF
When altricial birds hatch, they are unable to regulate their own temperature, but by the time they fledge they are thermally independent. Early-life conditions have been shown to be an important factor contributing to an individual's performance in adult life. However, it is currently unknown to what extent body temperature during endothermy development is driven by genetic variation or by the early environment. We use thermal images of cross-fostered house sparrows (Passer domesticus) throughout the nestling period to separate genetic and environmental drivers of body temperature. Our results show small heritability of body temperature. We further found that there are effects from the natal environment which carry over into the late nestling stage. A correlation between early to the mid-nestling period was explained by the natal environment and during this period body temperature and growth follow independent developmental trajectories. Furthermore, higher body temperature is under viability selection independent of body mass. We, therefore, demonstrate the natal environment influences future offspring phenotype in a novel measure; body temperature. Our study provides the first study into the environmental and genetic drivers of body temperature variation in a wild bird, furthering our understanding of how an individual's traits evolve.
Competing Interest Statement
The authors have declared no competing interest.
View the discussion thread.
Supplementary Material
Thank you for your interest in spreading the word about bioRxiv.
NOTE: Your email address is requested solely to identify you as the sender of this article.

Citation Manager Formats
- EndNote (tagged)
- EndNote 8 (xml)
- RefWorks Tagged
- Ref Manager
- Tweet Widget
- Facebook Like
- Google Plus One
Subject Area
- Evolutionary Biology
- Animal Behavior and Cognition (5409)
- Biochemistry (12201)
- Bioengineering (9136)
- Bioinformatics (30113)
- Biophysics (15481)
- Cancer Biology (12571)
- Cell Biology (18061)
- Clinical Trials (138)
- Developmental Biology (9746)
- Ecology (14601)
- Epidemiology (2067)
- Evolutionary Biology (18758)
- Genetics (12539)
- Genomics (17202)
- Immunology (12298)
- Microbiology (29014)
- Molecular Biology (12034)
- Neuroscience (63164)
- Paleontology (463)
- Pathology (1935)
- Pharmacology and Toxicology (3366)
- Physiology (5184)
- Plant Biology (10797)
- Scientific Communication and Education (1709)
- Synthetic Biology (3004)
- Systems Biology (7534)
- Zoology (1692)
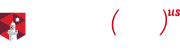
Hot spot 'saunas' a new lifeline for endangered frog populations
Macquarie University researchers have used heat to develop a simple and effective way to help endangered frogs avoid the catastrophic impact of a pandemic sweeping multiple species.
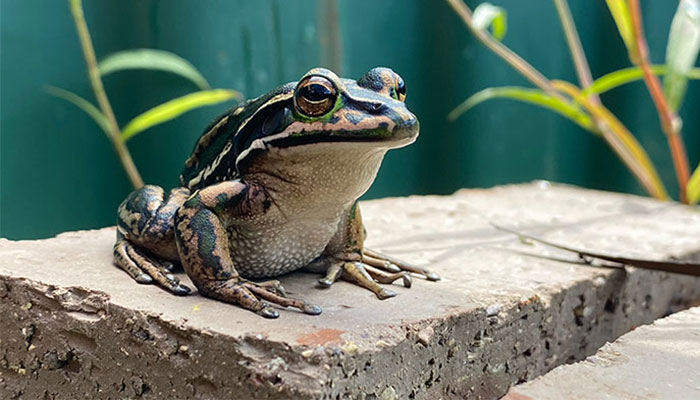
Under threat: Green and golden bell frogs are vulnerable to a deadly fungal infection that has seen their numbers plummet in Australia. Photo: Dr Athony Waddle
In collaboration with the University of Melbourne, researchers targetted the fungal disease chytridiomycosis , which has already driven at least six species of amphibians to extinction in Australia, and threatens dozens more worldwide.
The findings, published in the journal Nature , offer a potential lifeline for rapidly declining populations such as the green and golden bell frog ( Litoria aurea ), which has disappeared from more than 90 per cent of its native range in Australia since the disease arrived from the Korean Peninsula in 1978.
Dr Anthony Waddle, a Schmidt Science Fellow at Macquarie University's Applied BioSciences and lead author of the study, says very few interventions address the impact of the international spread of the disease-causing chytridiomycosis fungus ( Batrachochytrium dendrobatidis or Bd ).
“In the 25 years since chytridiomycosis was identified as a major cause of the global collapse of amphibian populations, our results are the first to provide a simple, inexpensive and widely applicable strategy to buffer frogs against this disease,” Dr Waddle says.
In these simple little hot spots, frogs can go and heat up their bodies to a temperature that destroys the infections.
Chytridiomycosis typically establishes itself permanently once it spreads to a new environment, and has caused greater damage to global biodiversity than any other recorded disease or invasive species.
Of chytridiomycosis-affected species worldwide, 90 have become extinct or are presumed extinct in the wild. Another 124 species have declined in number by more than 90 per cent.
Senior author Professor Rick Shine, from Macquarie University’s School of Natural Sciences, says this study has demonstrated a simple intervention which can easily scale up, potentially helping reduce the impact of the deadly pandemic.
“Chytrid isn’t going away, but our behavioural ecology intervention can help endangered amphibians co-exist with chytridiomycosis in their ecosystems,” Professor Shine says.
Mini med spa for frogs
The research team found providing artificial 'hot spot' shelters allows frogs to quickly "bake off" infections.
The team built small shelters from readily available materials, such as bricks and PVC greenhouses, so frogs could regulate their body temperature and live in conditions that suppress the growth of the fungal pathogen.
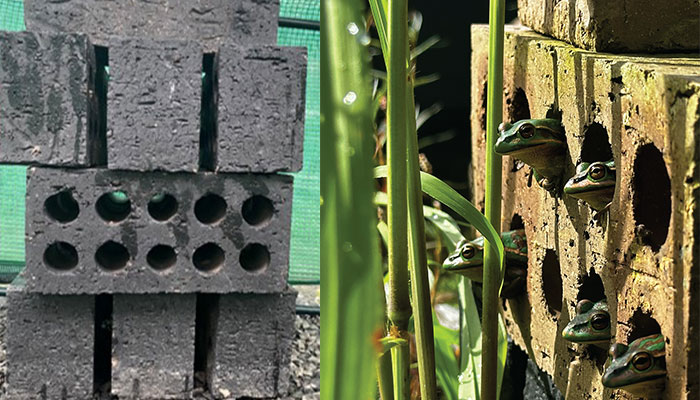
Green and golden bell frogs naturally prefer temperatures around 29 degrees Celsius, which is much higher than the chytridiomycosis fungus can tolerate, Dr Waddle says.
The research found when frogs shifted to hot spot shelters, infections were reduced significantly, and frogs that cured their infections with heat were then immune to subsequent infections.
“The whole thing is like a mini med spa for frogs,” says Dr Waddle.
“In these simple little hotspots, frogs can go and heat up their bodies to a temperature that destroys the infections.
“As with many human diseases, such as influenza, chytridiomycosis is seasonal. Winter is a particularly vulnerable time for frogs, given there are few opportunities to heat themselves up.
“By making hot spots available to frogs in winter, we empower them to cure their infections, or not even get sick at all.”
Attractive habitats
A critical factor in the project was creating habitats that frogs would actively seek out and use, Dr Waddle says.
“Getting the mix of shade and heat right is really important, because there’s little point having hotspots available in nature if the frogs don't use them,” Dr Waddle says.
The simple prototypes – a pile of bricks inside a greenhouse – proved to be a secure and attractive place for frogs to gather.
We’ve shown that it works; now we’re putting it into one of the most vulnerable populations where we expect to see immediate impact.
To test the effectiveness of the hot spot shelters in a more realistic setting, the team conducted a 15-week research project using the outdoor experiment technique known as 'mesocosms', which are designed to mimic the frogs' natural habitat.
Inside thermal shelters, the maximum daily temperature was about 4.5 degrees Celsius higher in shelters exposed to direct sunlight compared to those that were shaded.
The results showed that frogs infected with chytridiomycosis shake off the infection faster when they can warm up in unshaded shelters, compared to shaded ones.
Survivor populations
The study also showed frogs who survive an infection can develop a form of acquired immunity, making them more resistant to future infections.
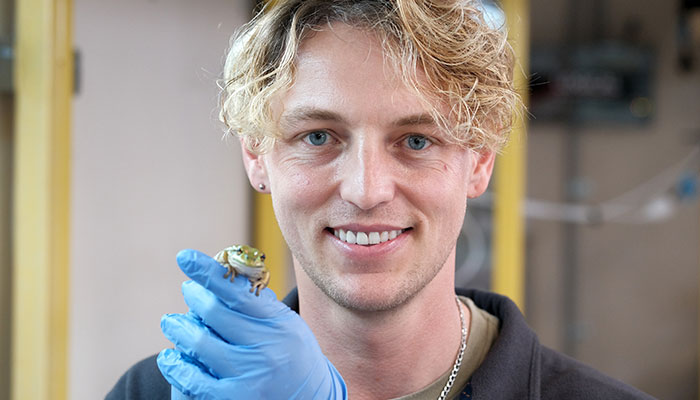
Innovators: Dr Anthony Waddle, pictured above, led a team of researchers who are the first to provide a simple, and widely applicable strategy for frogs to fight off the deadly chytridiomycosis fungal infection. Photo: Yorick Lambreghts
When re-exposed to the fungus, these "pathogen-experienced" frogs had a much higher survival rate (86 per cent) compared with frogs which hadn’t been previously infected (22 per cent). They also exhibited lower infection levels overall.
“Lowering mortality rates and boosting their immunity to chytridiomycosis is the key to protecting amphibians from this disease, which is now endemic around the world,” says Dr Waddle.
Chytridiomycosis is native to the Korean Peninsula but has devastated frogs outside this area because they have no natural protection.
Dr Waddle says these simple hot spot shelters are easy to reproduce, and the strategy can easily be scaled up with community involvement.
“Our study used green and golden bell frogs, which used to be very common across eastern Australia; they lived in letterboxes and backyards and really adapted to human settlements, before chytridiomycosis came and hammered the population,” Dr Waddle says.
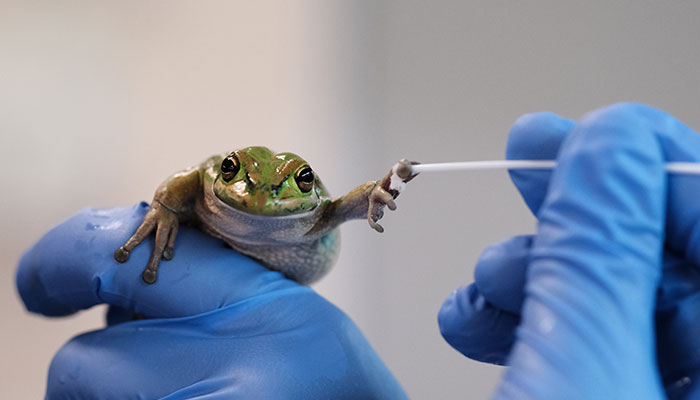
Swab: Dr Waddle's results showed frogs who survived a chytridiomycosis infection can also develop a form of acquired immunity, making them more resistant to future infections. Photo: Yorick Lambreghts
The research team is now working on expanding the study and implementing the hotspot shelters in one of the largest and most vulnerable populations of green and golden bell frogs, at Sydney Olympic Park.
“We’ve shown that it works; now we’re putting it into one of the most vulnerable populations where we expect to see immediate impact,” says Dr Waddle.
Dr Anthony Waddle is a a Schmidt Science Fellow at Macquarie University's Applied BioSciences in the Faculty of Science and Engineering.
Recommended Reading
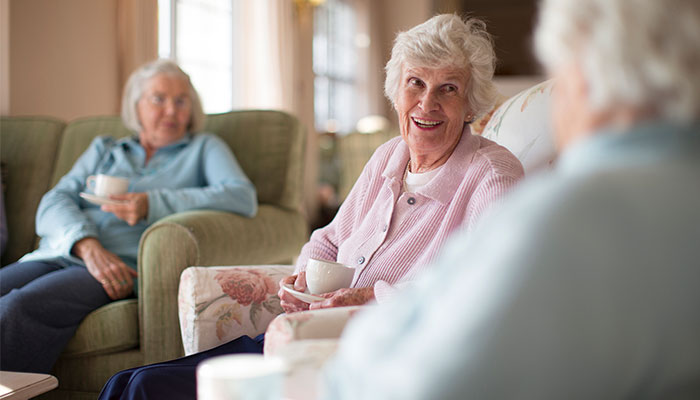

An official website of the United States government
The .gov means it’s official. Federal government websites often end in .gov or .mil. Before sharing sensitive information, make sure you’re on a federal government site.
The site is secure. The https:// ensures that you are connecting to the official website and that any information you provide is encrypted and transmitted securely.
- Publications
- Account settings
Preview improvements coming to the PMC website in October 2024. Learn More or Try it out now .
- Advanced Search
- Journal List
- Front Neurosci
The Temperature Dependence of Sleep
Edward c. harding.
1 Department of Life Sciences, Imperial College London, London, United Kingdom
Nicholas P. Franks
2 Centre for Neurotechnology, Imperial College London, London, United Kingdom
3 UK Dementia Research Institute, Imperial College London, London, United Kingdom
William Wisden
Mammals have evolved a range of behavioural and neurological mechanisms that coordinate cycles of thermoregulation and sleep. Whether diurnal or nocturnal, sleep onset and a reduction in core temperature occur together. Non-rapid eye movement (NREM) sleep episodes are also accompanied by core and brain cooling. Thermoregulatory behaviours, like nest building and curling up, accompany this circadian temperature decline in preparation for sleeping. This could be a matter of simply comfort as animals seek warmth to compensate for lower temperatures. However, in both humans and other mammals, direct skin warming can shorten sleep-latency and promote NREM sleep. We discuss the evidence that body cooling and sleep are more fundamentally connected and that thermoregulatory behaviours, prior to sleep, form warm microclimates that accelerate NREM directly through neuronal circuits. Paradoxically, this warmth might also induce vasodilation and body cooling. In this way, warmth seeking and nesting behaviour might enhance the circadian cycle by activating specific circuits that link NREM initiation to body cooling. We suggest that these circuits explain why NREM onset is most likely when core temperature is at its steepest rate of decline and why transitions to NREM are accompanied by a decrease in brain temperature. This connection may have implications for energy homeostasis and the function of sleep.
Introduction
In all mammals, sleep appears to be indispensable and coincides with a conserved circadian temperature rhythm. When our core and brain temperatures are in rapid decline we are most likely to choose to sleep, and if we dissociate from this cycle of body cooling we experience insomnia ( Hayward, 1968 ; Campbell and Broughton, 1994 ; Lack et al., 2008 ). Here, we review the evidence that thermoregulatory mechanisms are fundamental to sleep and consider the neuronal circuits that connect these two physiologies. These circuits use the presence of warm microclimates to gate sleep and may enhance circadian body cooling as our first non-rapid eye movement (NREM) bout approaches. The same neurons directly link NREM initiation to body cooling and may explain why transitions from wakefulness to NREM sleep, across the sleep cycle, are immediately followed by a decrease in brain temperature, whilst transitions back to REM or WAKE are accompanied by rewarming ( Alföldi et al., 1990 ; Landolt et al., 1995 ). The partitioning of brain cooling during NREM sleep and the coordination of the circadian core temperature rhythm are important for effective sleep.
This may have particular consequences for energy homeostasis and could open a window on sleep function.
Preparation for Sleep is a Thermoregulatory Behaviour
Mammals have a range of thermoregulatory behaviours that allow adaptation to environmental temperature fluctuations throughout the day, but these are most visible in the preparations for sleep ( Peever, 2018 ). These behaviours include warmth and shelter seeking, nest building, curling up and huddling (see Figure 1A ). Mice that are inactive or sleeping are much more likely to do so in contact with nesting material ( Gaskill et al., 2011 ). As small rodents they demonstrate surprisingly sophisticated thermal adaptations. As environmental temperature decreases, nest quality rises to compensate and results in measurable improvements in insulation ( Gaskill et al., 2013a ). When they can, mice engage in huddling with group members ( Gaskill et al., 2011 , 2012 ; Gordon et al., 2014 ). They also have a clear thermal preference during the sleep phase (lights on), choosing warmer environments approaching thermoneutrality (27–30°C) and minimising energy expenditure ( Gordon et al., 1998 ; Gaskill et al., 2012 ). These behaviours align the circadian temperature decline with the light and dark cycle and sleep onset. An example of the circadian core temperature cycle over several days can be seen in Figure 1B . The decline in core temperature intersects the light–dark cycle and changes over a range of about 2°C at the transition from the active phase of the mice (lights off) to the sleep phase (lights on) ( Figure 1C,D ).

Sleep preparation is a thermoregulatory behaviour (A) shows typical nesting behaviour in four species. Mouse nesting ( Mus musculus , C57Bl6/J), house cat ( Felis catus ) curling up, nest building in the chimpanzee ( Pan troglodytes verus ) and bedding ( Homo sapiens ). (B) Example of circadian temperature cycle over 6 days in a male C57Bl6/J mouse. (C) Average of transitions from the same mouse over 16 consecutive days 2 h before and after the light change. (D) Minimum temperature ( n = 21) during light phase compared to minimum ( n = 21) and maximum temperature ( n = 16) in the dark phase, plotted as change from zero for a group of male C57Bl6/J mice. Data shown in (B–D) is from (Harding et al., unpublished). All images used with permission or copyright clearance. The nesting chimpanzee photo credit: Kathelijne Koops. The nesting cat photo credit: Isobel Harding, the sleeping human is available under CC0-1.0 universal and the nesting mouse is adapted from Deacon (2006) .
Ambient temperature is a critical determinant of energy expenditure, and failure to carry out thermal defence behaviours has consequences for energy homeostasis ( Yu et al., 2018 ). Fur removal in Siberian hamsters, for example, increases food consumption by almost a quarter; whereas, in cold conditions, group huddling or the provision of nesting material can reduce food consumption by 15–20%, respectively ( Kauffman et al., 2003 ; Batavia et al., 2010 ). Similarly, provision of nesting material at temperatures below thermoneutrality increases breeding efficiency giving larger litters, higher pup weight and reduced pup mortality ( Gaskill et al., 2013b ).
Thermoregulatory behaviour prior to sleeping is a core part of maintaining energy balance, at least in smaller mammals, where the consequence of thermal inefficiency is an increased need for food. In larger mammals, however, the drive towards thermal preparation for sleep is no weaker. Chimpanzees and other primates select their arboreal sleeping sites ( Figure 1A ), at least partly, on thermal characteristics, and during colder weather even adjust their nest sites to be more insulating ( Koops et al., 2012 ; Samson and Hunt, 2012 ; Stewart et al., 2018 ). In addition, humans actively regulate temperature during sleep by unconsciously increasing their exposed surface area as ambient temperatures rise. In optimal room temperatures, approximately 19–21°C, we attempt to establish skin microclimates between 31 and 35°C and deviation from this range has a negative influence on sleep ( Figure 2A ) ( Muzet et al., 1984 ; Okamoto-Mizuno et al., 2003 ; Raymann et al., 2005 ). A key factor in using microclimates is that, at least in humans, it cannot be replaced by ambient warming at the same temperature, perhaps because it disrupts the self-adjustment required over the course of the night ( Muzet et al., 1984 ; Raymann et al., 2008 ).

Thermoregulation is important for human sleep. (A) Humans use bedding to form warm microclimates during sleep. These activate central hypothalamic mechanisms to induce sleep and peripheral vasodilation. (B) Distal-to-proximal gradient and core temperature decline predict sleep onset (adapted from Krauchi et al., 2000 ).
In summary, thermoregulatory behaviours prior to sleep are conserved across mammalian species suggesting they are not just a matter of comfort and may have a more functional role in sleep initiation and maintenance.
The “Warm Bath Effect”
In humans, immersion in hot water prior to, but not immediately before, the sleep period decreases sleep latency and increases sleep depth. This is the known as the ‘Warm Bath Effect’ ( Horne and Reid, 1985 ; Parmeggiani, 1987 ; Bunnell et al., 1988 ; Shapiro et al., 1989 ; Jordan et al., 1990 ; Dorsey et al., 1999 ). In fact, warming for up to 4 h, between 1 and 8 h before to going to bed, increases slow wave sleep (SWS), increases NREM consolidation and decreases REM sleep. This effect embodies a key connection between temperature and sleep. Warming, at the right time, is causatively associated with sleep initiation. However, sleep initiation occurs within the decline of circadian temperature and NREM is associated with further reductions in the temperature of both core and the brain ( Alföldi et al., 1990 ; Landolt et al., 1995 ; Kräuchi and Wirz-Justice, 2001 ). Many sleep studies have attempted to reconcile this counter-intuitive relationship to explain two conditions: How warming might initiate sleep and be compatible with body cooling, and how we might encounter this warming under ‘everyday’ conditions.
Optimal ambient temperatures, in combination with bedding, appear to be crucial for efficient sleep onset in humans ( Haskell et al., 1981 ; Okamoto-Mizuno et al., 2003 ; Raymann et al., 2008 ). Responses to external temperature also appear to be important as the extent of vasodilation, particularly in the hands and feet (distal-skin), is a good predictor of sleep initiation ( Krauchi et al., 1999 ). This vasodilation is usually considered part of the circadian temperature decline and is observed up to 2 h prior to the start of the first sleep episode, during the wake phase ( Krauchi et al., 2000 ). As core temperature falls it coincides with a decrease in self-assessed alertness ( Czeisler et al., 1980 ; van den Heuvel et al., 1998 ). In experiments where participants ‘self-selected’ their bed time, subjects were most likely to select a moment when the body temperature was at its maximum rate of decline ( Campbell and Broughton, 1994 ). As sleep approaches, core temperature and heart rate drop, and their steepest decline intersects ‘lights-off’ and sleep onset ( Figure 2B ). At this point the proximal-to-distal temperature gradient is as much as 1.5°C, but as core temperature falls the gradient reduces to about 0.5°C; a new cooler set-point is reached just after sleep onset. The lowest core temperature is observed about 2 h after ‘lights-off’ and sleep onset in Homo sapiens ( Krauchi et al., 2000 ). Under natural conditions, increased circulating melatonin also coincides with declining core temperature prior to sleep onset ( Krauchi et al., 1997 ; Krauchi et al., 2006 ; Logan and McClung, 2019 ).
Studying the temperature dependence of sleep in people has always been confounded by our ability to manipulate our environment and escape daily fluctuations in light and temperature. To get around this, Yetish et al. (2015) looked at sleep in three geographically distinct pre-industrial societies. They found that sleep onset coincided most strongly with a reduction in environmental temperature. Sleep was most often initiated after dark and the entire sleep period took place as ambient temperature was declining. Awakening also occurred before dawn, as ambient temperature reached its lowest point, and coincided with vasoconstriction, as measured by finger temperature ( Yetish et al., 2015 ). A change in temperature in the fingers is a good measure of change in blood flow, and so it seems likely that these subjects started sleep in a state of vasodilation that was progressively replaced by vasoconstriction until waking ( Rubinstein and Sessler, 1990 ; van Marken Lichtenbelt et al., 2006 ). A similar result was also observed by Han et al. (2018) , under sleep-laboratory conditions, with high numbers of skin-temperature sensors distributed across the body. These indicated progressive vasodilation from sleep onset to waking. However, this was mostly represented in the torso, and the hands and feet were not recorded ( Han et al., 2018 ).
The circadian cycle and the onset of the first NREM episode are strongly linked. If sleep onset is postponed by sleep deprivation, then the circadian temperature rhythm is disrupted. Similarly, a delay in core temperature decline of more than 2 h, is observed in patients with delayed sleep phase disorders (DSPDs) ( Ozaki et al., 1996 ; van den Heuvel et al., 1998 ; Watanabe et al., 2003 ). Disruption of the peripheral vasodilatory response is sufficient to disrupt sleep. For instance, those with difficulty in peripheral vasodilation (vasospastic disorders) have longer sleep latencies than healthy controls ( Pache et al., 2001 ). Narcoleptic patients also have a strongly altered proximal-to-distal skin temperature gradient during daytime waking ( Fronczek et al., 2006 ). But manipulating the proximal-to-distal relationship can change sleep propensity. Warming of the core (proximal-skin) by less than 1°C, easily within the range encountered within the circadian day, is sufficient to shorten sleep latency ( Raymann et al., 2005 ). Temperature manipulation can also selectively and predictably alter vigilance states in patients with narcolepsy ( Fronczek et al., 2008a , b ). Additional work in the clinic has shown that neonates are three times more likely to fall asleep within 30 min, if their distal-to-proximal skin gradient is greater than 2.5°C ( Abe and Kodama, 2015 ). Distal vasodilation and higher foot temperature in preterm neonates is also correlated with shorter wake bouts ( Barcat et al., 2017 ).
Understanding how warmth might be encountered on a daily basis to precipitate these changes that initiate sleep and vasodilation is crucial. But it seems that the ‘warm bath effect’ is more subtle than previously thought. Raymann et al. (2008) have extended the warming paradigm with the aid of a custom-made ‘thermosuit’ for the manipulation of skin temperature. Small changes in skin temperature of only 0.4°C (in the 31–35 range), can shorten sleep latencies without altering core temperature. They can even encourage deeper sleep in more challenging patient groups, such as elderly insomniacs ( Raymann et al., 2008 ). This latter group was particularly susceptible to this thermal management, supporting the hypothesis that sleep difficulties in the elderly relate to deficits in normal thermoregulation ( Raymann and Van Someren, 2008 ).
In summary, humans and other mammals show thermoregulatory behaviour in preparation for sleep, including curling up, using bedding and nest building. This may generate a microclimate of warmth around the skin that enables entry into sleep while facilitating vasodilation in the ‘distal’ hands and feet. This vasodilation may prepare the ‘proximal’ core for the cooler and inactive phase of the circadian cycle. This warming persists through the night to maintain a sleep-permissive state that also permits selective vasodilation in NREM and constriction in REM and wake. It does so whilst maximising thermal efficiency of the core. The reasons that body cooling and sleep onset coincide are not clear. Body and brain cooling per se has not been shown to initiate NREM but is instead a consequence of vasodilation. We might expect that an upstream mechanism in the brain coordinates both NREM onset and vasodilation and in the next section we discuss how this might function ( Van Someren, 2000 ).
Neuronal Control of Thermogenesis and its Influence on Sleep
Sleep is a fundamental physiological process that is widely believed to be essential for life but its vital function has yet to be identified. The neuronal circuits that control sleep need to integrate information from at least two distinct inputs. According to current thinking, these are known as Process C and Process S, the circadian and the homeostatic input, respectively, and are part of the two-process model ( Borbély, 1982 ). Transitions from wake to NREM and REM sleep are carried out by neurons that respond to cues from the homeostatic drive, that tracks the time spent awake, as well as more salient cues from the circadian zeitgeber, via the suprachiasmatic nucleus (SCN). The homeostatic process tracks the duration of the waking period and dissipates this load during sleep. However, as we have seen, sleep onset is also gated by other inputs: ambient temperature, as well as levels of satiety, mating opportunities and the need to escape predators all determine the appropriate moment for NREM onset ( Borbély, 1982 ; Borbély et al., 2016 ; Eban-Rothschild et al., 2017 ; Logan and McClung, 2019 ). Neurons that influence sleep are widely distributed throughout the brain. This may allow the integration of behavioural and autonomic inputs onto the classical homeostatic and circadian sleep drive. For example, inhibition of ventral tegmental area (VTA) dopamine neurons promotes both nesting behaviour and sleep initiation ( Eban-Rothschild et al., 2016 ). While the homeostatic drive promotes sleep following prolonged wakefulness, the circadian, the behavioural and the autonomic inputs are permissive conditions for sleep onset (see Figure 3 ). These four inputs work together to gate sleep.

Sensory and homeostatic inputs that gate sleep. Sleep onset is determined by four competing inputs: the homeostatic drive to sleep and three permissive conditions that relate to sleep timing, the behavioural input, the circadian input and the autonomic input. Endocrine inputs are also a key part of each category. Ghrelin and leptin are important for sensing of hunger/satiety, respectively, while melatonin is a key component of the circadian rhythm. Adenosine and NO may form part of the homeostatic input. (Top - Factors promoting wakefulness) Circadian cues are permissive for wake and homeostatic pressure to sleep is low. Behavioural factors also promote wakefulness and autonomic inputs are not permissive for sleep. Wake promoting nuclei drive cortical and thalamic excitability, whilst inhibiting sleep-prompting areas such as PO and vPAG. Behavioural needs of food and reproduction overcome those of sleep and thermal comfort. Behavioural inputs are also wake-promoting and may integrate this information in the VTA. Hormonal inputs, such as ghrelin, are detected in the ARC and are sleep-permissive. Autonomic signals, such as ambient temperature, are relayed via the spinal cord and pass through the LPb to the PO for integration. Circuits detecting environmental warmth are not active, vasoconstriction dominates and BAT is active. AgRP neurons signal hunger and inhibit sleep. (Bottom – Factors promoting NREM sleep) Circadian cues are now permissive for sleep and homeostatic pressure to sleep is high. Behavioural factors also promote sleep and autonomic inputs are permissive for sleep. On seeking shelter and warmth and having eaten, sleep is permitted. Autonomic signals, such as ambient temperature, are relayed via the spine and pass through the LPb to the PO for integration. NOS1-glutamate neurons are activated by skin warmth and initiate both NREM and body cooling. Activation of vasodilatory and BAT downregulation circuits is via NOS1 projections to LPO GABAergic neurons or via direct projections to DMH and rRPA/RVLM. Behavioural inputs are now sleep promoting and may integrate this information in the VTA. Hormonal inputs, such as leptin, are detected in the ARC and are sleep permissive. POMC neurons detect satiety and are permissive for sleep. NO, nitric oxide; NOS1, nitric oxide synthase-1; PO, preoptic area; LPO, lateral preoptic area; vPAG, ventral periaqueductal grey; TMN, tuberomammillary nucleus; VTA, ventral tegmental area; ARC, arcuate nucleus; LPb, lateral parabrachial; LC, locus coeruleus; DR, dorsal raphe; BAT, brown adipose tissue; AgRP, agouti-related peptide; DMH, dorsal medial hypothalamus; rRPA, rostral raphe pallidus; RVLM, rostral ventrolateral medulla; POMC, pro-opiomelanocortin ( Leshan et al., 2012 ; Eban-Rothschild et al., 2016 ; Weber and Dan, 2016 ; Yu et al., 2016 ; Goldstein et al., 2018 ; Harding et al., 2018 ; Yu et al., 2019 ).
Although sleep onset and the regulation of sleep transitions may involve multiple nuclei in the brain, one area has been historically associated with NREM onset. The preoptic hypothalamus (PO) is a key site for NREM initiation but is also considered an integrator for thermoregulatory information, including cold and warm-defence ( Szymusiak et al., 2007 ). It consists primarily of the median (MnPO), the medial (MPO) and lateral (LPO) areas that are associated with a large array of functions from sleep to parental behaviour.
Preoptic circuits have been proposed as the mechanistic connection between whole body warming and sleep induction ( Morairty et al., 1993 ). The simplest version of this idea is that warming induces activity in sleep-promoting neurons. Indeed, warm stimuli are well known to increase activity in the PO (e.g., as seen by c-FOS expression) ( Scammell et al., 1993 ; Gong et al., 2000 ). Consistent with this idea, lesions in the PO of the cat disrupt both warm-defence behaviour and reduce total sleep ( Szymusiak et al., 1991 ). Only significant warming of these cats was able to rescue normal sleep amounts, possibly through compensation or mechanisms outside the PO ( Szymusiak and McGinty, 1986 ). In rats, PO lesions alter thermal preference behaviour which subsequently converges on warmer temperatures (∼30°C) that promote sleep recovery ( Ray et al., 2005 ). In crucial experiments, using a ‘thermode’ implanted into the PO, warming, but not cooling, increases delta power in the EEG ( Roberts and Robinson, 1969 ; Glotzbach and Heller, 1976 ; McGinty et al., 1994 ). To characterise preoptic neurons in this role Alam et al. (1995) repeated this protocol using an implanted microdrive and recorded the properties of preoptic neurons. Remarkably, 21% were thermosensitive and these could be further divided into two groups – cold-sensitive neurons (CSNs) and warm-sensitive neurons (WSNs). About 60% of WSN also increased their activity during NREM ( Alam et al., 1995 ). During warming in the rat brain, they could inhibit important arousal nuclei including dorsal raphe and posterior hypothalamic neurons ( Krilowicz et al., 1994 ; Guzmán-Marín et al., 2000 ; Steininger et al., 2001 ). In a detailed analysis of MnPO neurons by Suntsova et al. (2002) , more than 75% demonstrated properties that may facilitate NREM sleep induction. This included a gradual increase in firing into, and peaking during, NREM sleep and, unexpectedly, even higher firing rates during REM sleep ( Suntsova et al., 2002 ). Mapping of neuronal projections using retrograde and anterograde tracers has confirmed that the MnPO sends dense innervations to wake-promoting regions and is well placed to influence wake-to-sleep transitions by modulation of the lateral preoptic, lateral hypothalamus and dorsal raphe ( Uschakov et al., 2007 ). Lastly, some MnPO neurons express c-FOS in response to sleep deprivation and may also send projections to the LPO ( Chou et al., 2002 ; Zhang et al., 2015 ).
WSNs can directly sense brain temperature and are proposed to be modulated by pyrogens such as prostaglandin E2 ( Scammell et al., 1996 ; Lazarus et al., 2007 ). A population of glutamatergic neurons in the midline PO express the transient receptor potential member 2 (TRPM2) channel, enabling direct warm-sensing of local brain temperature. These could function to carry out heat defence but can also modulate the response to fever ( Song et al., 2016 ).
With the exception of fever, it is unclear if skin warming could induce an increase in brain temperature that could be sensed by WSNs ( Tan et al., 2016 ; Siemens and Kamm, 2018 ). Instead, a synaptic pathway is more likely. Neurons that receive afferent temperature information, but are not direct temperature ‘sensors’, have been distinguished by the term ‘warm-activated’ neurons ( Tan and Knight, 2018 ). The MnPO and MPO hypothalamus receives sensory afferents conveying thermal information from the skin ( Hammel, 1968 ; Boulant and Gonzalez, 1977 ; Morrison and Nakamura, 2011 ) ( Figure 4 ). Sensory neurons relay ambient temperature information via the spinal cord to glutamatergic relay neurons, and on to sub-regions of the lateral parabrachial nucleus (LPb). The LPb may also receive information from other parts of the body such as the viscera, and then pass these signals onto the MnPO and MPO regions ( Nakamura and Morrison, 2008 , 2010 ). At the first point of integration, the glutamatergic neurons conveying excessive warmth synapse at glutamatergic neurons in the MnPO, whose output initiates cooling by promoting vasodilation and switching off brown fat thermogenesis ( Morrison and Nakamura, 2011 ) ( Figure 4 ). What are these neurons in the PO that respond to warming? Recent work using GCaMP6 photometry has shown that these neurons can response to external warm challenges between 30 and 40°C and RNA sequencing has identified them as expressing pituitary adenylate cyclase-activating polypeptide (PACAP) and brain-derived neurotrophic factor (BDNF) ( Tan et al., 2016 ). These neurons are predominantly GABAeric and can induce hypothermia when activated. They function, at least in part, by inhibiting dorsal medial hypothalamus (DMH) glutamatergic neurons that stimulate BAT thermogenesis ( Tan et al., 2016 ). A further population of GABAergic neurons, that act through the DMH, have also been discovered in the nearby ventral LPO ( Zhao et al., 2017 ).

Signal integration in the preoptic hypothalamus. Warmth on the skin stimulates sensory inputs, through the LPb, to preoptic nitrergic-glutamatergic neurons that initiate simultaneous NREM and body cooling. This maybe through activation of separate GABAergic neurons for sleep and hypothermia in the MPO and LPO, but they may also activate galinergic-GABAergic neurons to initiate sleep and body cooling. The synaptic role of NO is unknown in these circuits but potential sites are labelled. NREM is initiated by inhibition of arousal nuclei, including the TMN and the LH. Others are likely to be involved. Body cooling is facilitated by activation of DMH and inhibition of rRPA neurons to induce vasodilation and downregulation of BAT thermogenesis. Inputs to the lateral parabrachial and the preoptic area are modulated by AgRP neuron-mediate inhibition from the arcuate. These detect hunger and put a break on NREM. Satiety induces activation of POMC neurons, that also express TRPV1, are permissive for NREM and induce local inhibition of AgRP neurons. Nitrergic-glutamate neurons may respond to leptin through the leptin Rb, as do AgRP and POMC neurons. They, or a separate local population, may also respond to changes in brain temperature through the TRPM2 ion channel. NO, nitric oxide; NOS1, nitric oxide synthase-1; PO, preoptic area; LPO, lateral preoptic area; vPAG, ventral periaqueductal grey; TMN, tuberomammillary nucleus; ARC, arcuate nucleus; LPb, lateral parabrachial; BAT, brown adipose tissue; AgRP, agouti-related peptide; POMC, pro-opiomelanocortin; DMH, dorsal medial hypothalamus; rRPA, rostral raphe pallidus; RVLM, rostral ventrolateral medulla; TRPM2, transient receptor potential cation channel; TRPV1, transient receptor potential cation channel vallinoid-1; GAL, Galanin ( Leshan et al., 2012 ; Weber and Dan, 2016 ; Yu et al., 2016 ; Goldstein et al., 2018 ; Harding et al., 2018 ; Jeong et al., 2018 ; Tan and Knight, 2018 ; Yu et al., 2019 ).
The PO is highly diverse region with many overlapping populations, but only some of these neurons have been functionally characterised ( Moffitt et al., 2018 ). For example, GABAergic-galanin neurons are associated with both sleep and parental behaviour, but populations of galanin-glutamate neurons also exist ( Sherin et al., 1998 ; Wu et al., 2014 ; Moffitt et al., 2018 ). PACAP/BDNF, TRPM2-glutamate and nitrergic-glutamate neurons are associated with warm-defence and fever but many other subpopulations exist ( Song et al., 2016 ; Tan et al., 2016 ; Harding et al., 2018 ). Whilst the latter is also associated with sleep induction, populations of GABAergic-nitrergic neurons have been found but are uncharacterised ( Harding et al., 2018 ; Moffitt et al., 2018 ). Given extensive diversity in PO neuronal subtypes ( Moffitt et al., 2018 ), methods such as c-FOS-dependent activity tagging, that allow functional dissection of specific circuits from the surrounding milieu, are particularly important ( Zhang et al., 2015 ). The PO area, including both MPO and LPO responds to recovery sleep, the sleep following sleep deprivation, by expressing c-FOS. The same regions are excited by the, α 2A -adrenergic agonist and sedative, dexmedetomidine (DEX) ( Zhang et al., 2015 ). To understand whether these physiologies shared the same circuitry Zhang et al. (2015) used c-FOS-dependent activity-tagging to separate the neurons activated by recovery sleep or DEX from other PO neurons that respond to a variety of environmental and homeostatic stimuli. These neurons expressed an excitatory hM 3 d q DREADD receptor such that, when these mice were given clozapine N -oxide, only this unique ensemble was activated. This resulted in consolidated NREM sleep, consistent with recovery sleep or sedation. However, PO ensembles, tagged by either recovery sleep or DEX, also induced hypothermia ( Zhang et al., 2015 ). In fact, essentially all sedatives and general anaesthetics used clinically induce core-to-peripheral heat redistribution from vasodilation and, without warming, hypothermia ( Díaz and Becker, 2010 ; Sessler, 2016 ). This suggests that core PO circuitry could link natural sleep induction, the induction of body cooling and the mechanisms of sedative class drugs.
We hypothesised that thermoregulatory circuits themselves might have an important role in facilitating sleep. This would also explain the propensity of either external or direct PO warming to induce NREM sleep. We again used activity-tagging, but this time labelled only those preoptic ensembles that received warm sensory information. Reactivation of these ‘warm-tagged’ neurons produced simultaneous NREM and body cooling ( Harding et al., 2018 ). These neurons expressed a mixture of cell type markers including the vesicular glutamate transporter 2 (VGLUT2), glutamate decarboxylase (GAD67), and nitric oxide synthase 1 (NOS1). When activity-tagging was repeated in NOS1-CRE mice, these also experienced simultaneous NREM and body cooling. However, when it was repeated in vGAT-CRE mice only NREM and a little body cooling was observed. As these NOS1 neurons express VGLUT2, our data suggest a distinct nitrergic-glutamatergic circuit for linking thermal sensory information to NREM-onset that may reside upstream of a GABAergic sleep ‘switch’ ( Harding et al., 2018 ). In this circuitry, external warmth is a permissive state for NREM initiation. Without this sensory input NREM onset is inhibited. We think this is consistent with data showing that external warming promotes sleep in humans and animals, whilst also providing a possible mechanism for why mammals seek nesting sites: to produce microclimates of skin warmth that permit sleep. We don’t yet know if NOS1 neurons utilise nitric oxide (NO) in synaptic transmission. However, NO is implicated in modulating arousal in other areas of the brain ( Gerashchenko et al., 2008 ; Kalinchuk et al., 2010 ; Cespuglio et al., 2012 ; Morairty et al., 2013 ; Yu et al., 2019 ).
What is downstream of the MnPO/MPO nitrergic-glutamate neurons? The local preoptic area contains multiple populations of galanin neurons both excitatory and inhibitory ( Moffitt et al., 2018 ). Recently, experiments have demonstrated that activating galanin neurons in the ventrolateral preoptic area (VLPO) can induce both NREM and hypothermia ( Kroeger et al., 2018 ). Similarly, activation of galanin neurons in LPO can also induce NREM and hypothermia ( Ma et al., 2019 ). The latter has parallels to the activation of GABAergic neurons activity-tagged during recovery sleep ( Zhang et al., 2015 ). As the MnPO is known to send projections to both LPO and VLPO we have hypothesised that GABAergic-galanin neurons may be targets for nitrergic-glutamate neurons ( Uschakov et al., 2007 ). In VLPO, activation of galanin neurons using DREADD receptors facilitated more NREM sleep when the mice were closer to thermoneutrality (29°C) and when hypothermia was blunted by warming at 36°C. Thermoneutrality appears to allow optimum recovery of REM sleep, compared to either the ambient (22°C) or warmed (36°C) conditions ( Kroeger et al., 2018 ). This is consistent with the idea of a narrow temperature range for optimised REM sleep ( Czeisler et al., 1980 ; Szymusiak and Satinoff, 1981 ). Galanin neurons in LPO appear to be necessary for the activation of homeostatic mechanisms that trigger recovery sleep. Deletion of these neurons using caspase expression ablates rebound delta power following sleep deprivation ( Ma et al., 2019 ). More data is needed to confirm if LPO-galanin are genuine targets of nitrergic-glutamate neurons. The latter may also have other long-range projections.
Thermogenesis Links Sleep to Energy Homeostasis
Thermoregulation, in particular thermal inefficiency, impacts energy homeostasis and changes feeding requirements. This is an additional homeostatic drive that adds its own ‘pressure’ to modulate sleep networks ( Figure 3 ). Following a meal, adipocytes secrete the hormone leptin. This hormone is indicative of excess energy intake and discourages feeding. Leptin works through well-established pathways in the arcuate hypothalamic nucleus, where it inhibits NPY expressing AgRP neurons ( Williams et al., 2009 ). However, there are also leptin receptors elsewhere in the CNS, including in the PO hypothalamus. PO glutamatergic neurons expressing the leptin receptor (leptinRb) are excited (they express c-FOS) when ambient temperature rises ( Yu et al., 2016 ). This results in a reduced energy expenditure, through inhibited thermogenesis, and a decrease in food consumption ( Zhang et al., 2011 ; Yu et al., 2016 ). Neurons that coexpress NOS1 and leptinRb have been identified in other parts of the hypothalamus and these can also inhibit thermogenesis ( Leshan et al., 2012 ). Hence, it seems likely that there is some overlap between preoptic glutamatergic-leptinRb neurons and the NOS1 populations identified in Harding et al. (2018) . Similarly, many BDNF/PACAP in the PO express VGLUT2 and a subpopulation of these neurons that express c-FOS in response to a warm stimulus has recently been shown to coexpress the leptinRb suggesting yet further overlap between these populations ( Moffitt et al., 2018 ). As well as energy regulation, leptin signalling appears to have a more direct role in sleep. Systemic administration of recombinant leptin in food-deprived mice increases both NREM and REM sleep durations, while mice deficient in leptin (ob/ob) have fragmented sleep as well as lower average core temperature ( Sinton et al., 1999 ; Laposky et al., 2006 ). A key remaining question is whether NOS1 neurons that initiate NREM sleep and body cooling provide a wider link between sleep and energy homeostasis ( Harding et al., 2018 ). These circuits are summarised in Figure 4 .
Recent data have provided a new insight into how energy balance might influence sleep. Goldstein et al. (2018) have directly assessed the impact of AgRP/POMC neuronal activity in the arcuate nucleus on the drive to sleep. AgRP neurons can detect energy intake and are considered ‘hunger sensors’, inhibited by both circulating leptin and insulin. POMC oppose the action of AgRP neurons and are activated by leptin ( Cowley et al., 2001 ). AgRP activity promotes food seeking behaviour, even at the expense of sleep. But, if mice are food deprived, inhibition of these neurons rescues sleeping behaviour at the expense of eating ( Goldstein et al., 2018 ). As thermal inefficiency results in increased feeding, we would expect circuitry that links thermal sensation to appetite control. Consistent with this idea, Jeong et al. (2018) have shown that POMC neurons express the transient receptor potential vanilloid-1 (TRPV1) channel. Optogenetic activation of these neurons produces feeding inhibition ( Jeong et al., 2018 ). Although this study did not assess sleep, activation of POMC neurons by Goldstein et al. (2018) could rescue sleep in animals deprived of food ( Goldstein et al., 2018 ). This may be because POMC neurons densely innervate sleep-promoting areas including the PO and may inhibit local GABAergic interneurons ( Elias et al., 1999 ; Wang et al., 2015 ; Weber and Dan, 2016 ). POMC neurons also inhibit AgRP neurons which project to several sleep-promoting regions including the PO, the ventral periaqueductal grey (vPAG) and parabrachial nucleus (Pb) ( Betley et al., 2013 ; Wang et al., 2015 ; Weber and Dan, 2016 ; Weber et al., 2018 ) (see Figure 4 ). Arcuate NPY neurons that normally stimulate eating also downregulate BAT thermogenesis and so may have similar roles in thermoregulatory connections to sleep ( Shi et al., 2013 ). These autonomic signals are interpreted as strong behavioural drives, for instance to find food.
In summary, it seems likely that there is significant overlap between neuronal populations that regulate sleep onset, thermogenesis and energy homeostasis. Sleep onset may be controlled, in part, by integrating these sensory inputs, including ambient temperature and energy status. It is less clear why gating sleep with these inputs would be beneficial.
Sleep Deprivation Disrupts Thermoregulation and Energy Balance
Sleep architecture is highly dependent on thermal factors, but the consequence of total sleep loss is a radical alteration of thermoregulation and energy balance. In rats, chronic total-sleep deprivation and selective REM deprivation, using the disk-over-water method for many days, leads to profound physiological effects and eventually death ( Everson et al., 1989 ). In the early stages, an increase in metabolic function was observed in these rats, including core body temperature, and with it an increase in food consumption. However, the temperature rise was quickly reversed and the rats progressively developed hypothermia. They also moved to warmer parts of a temperature gradient as their sleep deprivation deepened ( Prete et al., 1991 ). This may be an energy conservation strategy, reducing thermal load, increasing appetite and simultaneously cooling the body ( Rechtschaffen and Bergmann, 1995 ). Similar strategies are seen in torpid animals when food is scarce ( Ruf and Geiser, 2015 ). In the sleep-deprived animals this strategy ultimately failed, as the rats rapidly lost weight ( Bergmann et al., 1989 ; Everson et al., 1989 ; Rechtschaffen and Bergmann, 1995 ). Sleep deprivation appears to either increase the metabolic requirements of the animal, or by other means facilitates excessive heat loss, perhaps through over activation of NREM-initiating circuits that induce vasodilation.
One mechanism by which mammals, and small rodents in particular, generate heat is through brown adipose tissue thermogenesis (BAT). This is also a key mechanism in regulating energy homeostasis. Uncoupling protein 1 (UCP-1) is a key component of thermogenesis in brown adipose tissue (BAT). It decouples the electron transport chain from the ATP-synthase, facilitating heat production through proton gradient dissipation, without ATP production, and compensatory metabolism ( Cannon and Nedergaard, 2004 ). UCP-1 knockout mice have weakened homeostatic rebound following sleep deprivation. They also show a blunted sleep induction effect of warmer temperatures observed in control mice ( Szentirmai and Kapas, 2014 ). Similarly, the β3-adrenergic receptor agonists, which activate BAT thermogenesis, usually induce sleep in control mice but this response is ablated in mice with chemical deafferentation of the BAT ( Szentirmai and Kapás, 2017 ). These data suggest that UCP-1 mediated BAT thermogenesis is helpful in both recovery sleep (sleep following sleep deprivation) and NREM sleep induction. UCP-1 may also have a role facilitating NREM sleep during systemic inflammation ( Szentirmai and Kapas, 2018 ). The heat generated by these mechanisms could activate the sensory receptors in the skin and so trigger NREM sleep ( Harding et al., 2018 ).
Torpor and Hibernation: Too Cold to Sleep?
The cooperation of body cooling and NREM sleep suggest that energy homeostasis is an important factor for sleep, but it is natural to ask if there is any link to more extreme states ( Figure 5 ). When the need to save energy is sufficiently high many mammals sacrifice sleep to adopt an alternative thermoregulatory strategy of daily torpor or seasonal hibernation ( Ruf and Geiser, 2015 ). Daily torpor is a state of hypothermia triggered by food scarcity. Mammals that use daily torpor, such as the Djungarian hamsters ( Phodopus sungorus ), typically drop their core temperature to 15–20°C for many hours, but, in many species, daily torpor can range between 10°C or as high as 30°C ( Ruf and Geiser, 2015 ) ( Figure 5 ).

State transitions at different core temperatures. Sleep, anaesthesia and torpor sit on a continuum of decreasing core temperature that directly influences EEG power. On average NREM bouts are cooler than those of wake whilst brain temperature during REM is warmer. A representative example of NREM EEG at approximately 37°C is shown in green. Sedatives and anaesthetics induces delta oscillations in the EEG but also hypothermia. Dexmedetomidine (DEX; 100 μg/kg IP) induces sustained sedation but the power of the delta oscillations is suppressed. The example is shown in blue, 2 h after injection with core temperature at approximately 26°C. If the same dose of DEX is given to animal in a warm chamber then the power of the delta oscillations recovers. The example is shown in red 2 h after injection with a core temperature at 34°C. Some mammals use daily torpor to save energy during times of food scarcity. On average these are between 15 and 20°C but can range between 10 and 30°C. At approximately 21°C states of torpor may generate a sleep debt that results in recovery sleep on rewarming to 37°C. Artificial hypothermia, sometimes known as synthetic torpor, can be induced by 5-AMP (0.5 g/kg IP). This also induces delta oscillations that are suppressed by hypothermia. The example is shown in blue, 1.5 h after injection with core temperature at approximately 23°C. Below approximately 10°C the EEG is isoelectric and no oscillation can be discerned. Hibernators have periods have interbout euthermia with normal EEG power and wake-NREM and wake-REM transitions are detected. Example species are labelled with the temperature that they have been observed in for either daily torpor or hibernation. This reflects ambient environmental conditions important for EEG measurements but is not a strict hierarchy. EEG examples are from (Harding et al., unpublished) except for the hibernation example which is adapted from Frerichs et al. (1994) . IP, intraperitoneal; 5-AMP, adenosine monophosphate. Djungarian hamster ( Phodopus sungorus ), Golden-mantled ground squirrel ( Callospermophilus lateralis ), Fat-tailed dwarf lemur ( Cheirogaleus medius ), Arctic ground squirrel ( Urocitellus parryii ). Data adapted from Frerichs et al. (1994) ; Ruf and Geiser (2015) , and Vyazovskiy et al. (2017) .
A range of mammals from ground squirrels to brown bears also use annual hibernation strategies for winter survival and reproduction ( Carey et al., 2003 ; Ruf and Geiser, 2015 ). In hibernators core temperatures typically fall between 0 and 10°C and are maintained for weeks or months. Exposed ambient temperatures can fall below 0°C and core temperatures are maintained only a few degrees higher at 1% of euthermic (normal temperature) metabolic rates ( Carey et al., 2003 ). In extreme cases, such as the arctic ground squirrel ( Urocitellus parryii ), abdominal and peripheral temperatures can be stable at around -2°C whilst head and neck are just above 0°C ( Barnes, 1989 ; Boyer and Barnes, 1999 ). Only at body temperatures around 0°C do metabolic rates rise to defend the animal from freezing, suggesting an extremely low temperature set point ( Buck and Barnes, 2000 ) ( Figure 5 ).
Animals in either daily torpor or hibernation enter a state of inactivity or quiescence, but the power of the EEG signal observed in these animals scales with temperature: the lower the body temperature, the lower the power of the EEG ( Deboer, 1998 ). At a core temperatures of approximately 22°C or above, the frequency component remains within the delta band of 1–4 Hz and can be classified as sleep, although the EEG power is much reduced ( Walker et al., 1981 ; Daan et al., 1991 ). However, at lower temperatures this is not the case. If core and brain temperature is sufficiently low, then the EEG power falls below the threshold for attribution of sleep states ( Figure 5 ). At brain temperatures between 10°C and 25°C reduced-power delta oscillations can still be identified in the EEG signal, whereas below about 10°C the signal is isoelectric ( Walker et al., 1977 ). It is not clear to what extent the power of these oscillations is important for natural sleep function. In dwarf lemurs ( Cheirogaleus medius ), for example, hibernating at low ambient temperatures of only 5°C, EEG recordings are isoelectric and evidence of NREM or REM sleep are absent. In this case, only on rewarming was sleep (REM) observed ( Krystal et al., 2013 ). It has been suggested that torpor states may be a form of sleep deprivation. For instance, the recovery of Djungarian hamsters from daily torpor, with core and brain temperatures of around 23°C, results in a period of recovery sleep with an increased power in the delta band ( Deboer, 1998 ). Similarly, this sleep could be deferred by sleep deprivation suggesting the accumulation of a sleep debt during torpor ( Deboer and Tobler, 1994 ; Palchykova et al., 2002 ). While this sleep debt, as measured by delta power, accumulates during torpor it does so almost three times slower at brain temperature below 27°C, compared with time awake ( Deboer and Tobler, 2003 ). However, comparisons of recovery sleep EEG, following sleep deprivation or torpor, revealed differences in cortical network activity suggesting that torpor is not entirely equivalent to either sleep deprivation or natural sleep ( Vyazovskiy et al., 2017 ). Thus, a critical temperature may exist below which sleep function is impaired.
To understand the relationship between sleep and temperature in hibernators, researchers have compared animals that hibernate at different ambient temperatures. Animals that hibernate at low temperatures, such as the arctic ground squirrels ( Urocitellus parryii ), briefly warm up to levels comparable to waking (36–37°C). These are periods of interbout euthermia ( Boyer and Barnes, 1999 ; Carey et al., 2003 ). In these periods of warming, squirrels transition from wake to NREM and then REM sleep before returning to hibernation ( Daan et al., 1991 ). Hibernation of the golden-mantled ground squirrel ( Callospermophilus lateralis ) under warmer laboratory conditions (22°C) produced continuous NREM sleep ( Walker et al., 1981 ). During hibernation at colder temperatures, of 11°C ambient, the minimum brain temperature, not the hibernation bout length, was the best predictor of rebound delta power during subsequent interbout euthermia. The same authors observed that, at this temperature (11°C), the euthermic (36–37°C) period following hibernation consisted of almost 70–80% NREM sleep, whereas animals hibernating at 21°C spent only 40% of their euthermic period in NREM ( Larkin and Heller, 1996 ). This indicates that the temperature at which hibernation takes places influences the degree to which sleep debt accumulates (see Figure 5 ). Of course, there are variations between species. When European ground squirrels enter a euthermic period, following hibernation at 5.5°C, the time spent in NREM sleep is proportional to the hibernation bout length, not temperature per se ( Strijkstra and Daan, 1997 ). Collectively, these data suggest that the restorative component of sleep is temperature-dependent.
The same temperature dependence of sleep is seen in hibernating primates. When dwarf lemurs ( Cheirogaleus medius ) choose a hibernaculum at warmer temperatures, their EEG resembles NREM and REM sleep and the episodes of euthermia disappear ( Dausmann et al., 2004 ; Krystal et al., 2013 ). In other species of lemur ( C. crossleyi and C. sibreei ) that occupy a cooler environmental niche, sleep is consistently absent during the torpor phase, but returns during periods of interbout euthermia ( Blanco et al., 2016 ). Black bears ( Ursus americanus ), which always hibernate at warmer temperatures of 32–34°C, and actively defend this temperature set point, also do not show the inter-bout arousals seen in smaller mammals ( Tøien et al., 2011 ). Like the dwarf lemurs, these higher temperatures appear to allow brown bears to spend large amount of time in NREM sleep ( Tøien et al., 2015 ). The defending of lower temperature set points in larger mammals has remarkable parallels with people under anaesthesia. In people given either the sedative DEX or the anaesthetic propofol, shivering thresholds reduce to between 32 and 34°C, respectively ( Matsukawa et al., 1995 ; Talke et al., 1997 ; Sessler, 2016 ). Hence, hibernation may be too cold to facilitate sleep and episodes of interbout euthermia, lasting 12–24 h, may allow sleep processes to be recovered ( Carey et al., 2003 ).
The neuronal circuitry that induces torpor and/or hibernation is not known. However, it is possible that it uses components of the natural sleep–wake circuitry. For example, NOS1-glutamate neurons in PO that induce NREM sleep and sustained hypothermia ( Harding et al., 2018 ), could in colder climates, have a role in torpor or hibernation induction, but with different behavioural and environmental triggers.
In summary, NREM sleep in a state of mild body cooling may be the preferred biological condition, but clearly in extreme environments, winter survival or times of food scarcity the restorative effects of sleep are, at least in part, sacrificed for energy conservation. As sleep can only be maintained at higher temperatures, it is energetically more expensive than torpor or hibernation. At these colder brain and core temperatures, sleep debts accumulate almost three times slower than during waking ( Deboer and Tobler, 2003 ).
Why Link NREM Sleep and Body Cooling?
To recap, sleep in rodents is associated with temperature-cycling: wake to NREM sleep transitions coincide with a cooler body and brain facilitated by tail vasodilation. Indeed, effective thermoregulation and nesting behaviour produce warm microclimates that have a role in stimulating NREM sleep and body cooling. We have suggested that PO neurons both receive warm thermal information from the skin and simultaneously coordinate NREM sleep initiation and body cooling ( Harding et al., 2018 ). Transitions to wakefulness or REM sleep are accompanied by vasoconstriction and brain warming ( Alföldi et al., 1990 ; Imeri and Opp, 2009 ). The absolute change in brain temperature at each NREM transition is small, about 0.2–0.4°C, but may reach larger values, comparable with the total diurnal variation in temperature (approximately 2°C), during extended bouts of sleep. In humans, core temperature reliably falls about 2 h prior to sleep onset and the first NREM episode is more likely to occur at the steepest point of temperature decline. Brain temperature appears to do the same ( Landolt et al., 1995 ). This rate of decline may be highest when PO circuitry is maximally activated, facilitating NREM sleep to the greatest extent. Other sensory inputs, such as satiety, are also permissive for sleep and their inputs are integrated to determine the precise moment of NREM onset. These temperature changes may have a direct role in the restorative functions of sleep.
One of the first hypothesis regarding the lower temperatures coinciding with NREM sleep was that it existed specifically to cool the brain ( McGinty and Szymusiak, 1990 ). It was proposed that a lower brain temperature would reduce cerebral metabolism, conserve energy and assist other functions from immune regulation to circadian coordination ( McGinty and Szymusiak, 1990 ). Conservation of energy for sleep in its entirety has also been proposed ( Berger and Phillips, 1995 ). However, we have seen that when mammals of all sizes prioritise conservation of energy, extremes of hypometabolism in torpor and hibernation are selected at the expense of sleep ( Ruf and Geiser, 2015 ). This suggests that energy conservation alone is not the primary function of sleep. Indeed, estimates of energy use over 24 h put the cost of sleep as high as 85–95% of the metabolic cost of waking ( Jung et al., 2011 ; Abreu-Vieira et al., 2015 ; Hibi et al., 2017 ).
It is feasible that reduced temperatures have a more direct function in the brain. At temperatures of 20°C or less, during which sleep debt is accumulated, morphological changes have been observed in dendritic spines ( Peretti et al., 2015 ). Hibernators can undergo synaptic remodelling while cold, as do animals in artificial torpor induced by 5′-adenosine monophosphate ( Popov and Bocharova, 1992 ; Magariños et al., 2006 ; Popov et al., 2007 ). In the latter condition, the total number of synapses is reduced (GM). The presence of these process may explain why sleep, as a restorative process, is inhibited at lower temperatures. Large changes in gene expression are also observed both in the brain and across the body in hibernators ( Williams et al., 2005 ). Colder temperatures, particularly in the brain, can induce expression of so called ‘cold-shock’ proteins including cold-inducible RNA binding protein (CIRP) and RNA-binding motif protein 3 (RBM3) ( Morf et al., 2012 ; Peretti et al., 2015 ; Hoekstra et al., 2019 ). Body and brain cooling during natural sleep are small, both from the reduction in diurnal core temperature and reductions in brain temperature at each NREM transition, but recent data suggest they are sufficient to increase CIRP expression and so influence the expression of other genes, including the circadian genes Period and Clock ( Morf et al., 2012 ; Hoekstra et al., 2019 ). This is important as cortical temperature changes are heavily influenced by the sleep–wake transitions and entry into NREM sleep can then influence clock gene expression to drive further transcriptional changes. In mice without CIRP, sleep deprivation results in 50% less REM sleep, illustrating the strength of this mechanism ( Hoekstra et al., 2019 ). This also provides one possible mechanism that the brain may keep track of the time spent in NREM sleep.
The extensive neuronal inter-connections that cross-regulate energy use, sleep induction and body temperature (see Figure 3 ) hint that the function of sleep plays an important role in energy homeostasis. The temperature-dependence of sleep debt accumulation, which is slowed at cooler temperatures, suggests that this debt is inherently a metabolic processes. Lastly, the synchronised changes in brain temperature during sleep may coordinate gene expression important for the functions of sleep, whilst contributing to a mechanism that measures the time spent sleeping.
Author Contributions
EH wrote the manuscript and designed the figures. All authors have discussed and edited the manuscript.
Conflict of Interest Statement
The authors declare that the research was conducted in the absence of any commercial or financial relationships that could be construed as a potential conflict of interest.
Acknowledgments
All images are used with permission or copyright clearance. The sleeping human ( Figure 1A ) is available under CC0-1.0 universal. We are grateful to Kathelijne Koops for the nesting chimpanzee picture, Isobel Harding for the picture of a sleeping cat, and to Ália dos Santos for proofreading the manuscript.
Funding. This work was funded by the UK Dementia Research Institute, which receives its funding from UK DRI, funded by the UK Medical Research Council, Alzheimer’s Society, and Alzheimer’s Research UK (NF and WW) and also by the Wellcome Trust (107839/Z/15/Z to NF and 107841/Z/15/Z to WW).
- Abe N., Kodama H. (2015). Distal-proximal skin temperature gradient prior to sleep onset in infants for clinical use. Pediatr. Int. 57 227–233. 10.1111/ped.12473 [ PubMed ] [ CrossRef ] [ Google Scholar ]
- Abreu-Vieira G., Xiao C., Gavrilova O., Reitman M. L. (2015). Integration of body temperature into the analysis of energy expenditure in the mouse. Mol. Metab. 4 461–470. 10.1016/j.molmet.2015.03.001 [ PMC free article ] [ PubMed ] [ CrossRef ] [ Google Scholar ]
- Alam M. N., McGinty D., Szymusiak R. (1995). Neuronal discharge of preoptic/anterior hypothalamic thermosensitive neurons: relation to NREM sleep. Am. J. Physiol. 269(5 Pt 2) R1240–R1249. 10.1152/ajpregu.1995.269.5.R1240 [ PubMed ] [ CrossRef ] [ Google Scholar ]
- Alföldi P., Rubicsek G., Cserni G., Obál F. J. P. A. (1990). Brain and core temperatures and peripheral vasomotion during sleep and wakefulness at various ambient temperatures in the rat. Pflügers Archiv. 417 336–341. 10.1007/bf00371001 [ PubMed ] [ CrossRef ] [ Google Scholar ]
- Barcat L., Decima P., Bodin E., Delanaud S., Stephan-Blanchard E., Leke A., et al. (2017). Distal skin vasodilation promotes rapid sleep onset in preterm neonates. J. Sleep Res. 26 572–577. 10.1111/jsr.12514 [ PubMed ] [ CrossRef ] [ Google Scholar ]
- Barnes B. M. (1989). Freeze avoidance in a mammal: body temperatures below 0 degree C in an Arctic hibernator. Science 244 1593–1595. 10.1126/science.2740905 [ PubMed ] [ CrossRef ] [ Google Scholar ]
- Batavia M., Matsushima A., Eboigboden O., Zucker I. (2010). Influence of pelage insulation and ambient temperature on energy intake and growth of juvenile Siberian hamsters. Physiol. Behav. 101 376–380. 10.1016/j.physbeh.2010.07.001 [ PubMed ] [ CrossRef ] [ Google Scholar ]
- Berger R. J., Phillips N. H. (1995). Energy conservation and sleep. Behav. Brain Res. 69 65–73. 10.1016/0166-4328(95)00002-B [ PubMed ] [ CrossRef ] [ Google Scholar ]
- Bergmann B. M., Everson C. A., Kushida C. A., Fang V. S., Leitch C. A., Schoeller D. A., et al. (1989). Sleep deprivation in the rat: V. Energy use and mediation. Sleep 12 31–41. 10.1093/sleep/12.1.31 [ PubMed ] [ CrossRef ] [ Google Scholar ]
- Betley J. N., Cao Zhen Fang H., Ritola, Kimberly D., Sternson, Scott M. (2013). Parallel, redundant circuit organization for homeostatic control of feeding behavior. Cell 155 1337–1350. 10.1016/j.cell.2013.11.002 [ PMC free article ] [ PubMed ] [ CrossRef ] [ Google Scholar ]
- Blanco M. B., Dausmann K. H., Faherty S. L., Klopfer P., Krystal A. D., Schopler R., et al. (2016). Hibernation in a primate: Does sleep occur? R. Soc. Open Sci. 3 : 160282 . 10.1098/rsos.160282 [ PMC free article ] [ PubMed ] [ CrossRef ] [ Google Scholar ]
- Borbély A. A. (1982). A two process model of sleep regulation. Hum. Neurobiol. 1 195–204. [ PubMed ] [ Google Scholar ]
- Borbély A. A., Daan S., Wirz-Justice A., Deboer T. (2016). The two-process model of sleep regulation: a reappraisal. J. Sleep Res. 25 131–143. 10.1111/jsr.12371 [ PubMed ] [ CrossRef ] [ Google Scholar ]
- Boulant J. A., Gonzalez R. R. (1977). The effect of skin temperature on the hypothalamic control of heat loss and heat production. Brain Res. 120 367–372. 10.1016/0006-8993(77)90916-7 [ PubMed ] [ CrossRef ] [ Google Scholar ]
- Boyer B. B., Barnes B. M. (1999). Molecular and Metabolic Aspects of Mammalian Hibernation: Expression of the hibernation phenotype results from the coordinated regulation of multiple physiological and molecular events during preparation for and entry into torpor. Bioscience 49 713–724. 10.2307/1313595 [ CrossRef ] [ Google Scholar ]
- Buck C. L., Barnes B. M. (2000). Effects of ambient temperature on metabolic rate, respiratory quotient, and torpor in an arctic hibernator. Am. J. Physiol. Regul. Integr. Comp. Physiol. 279 R255–R262. 10.1152/ajpregu.2000.279.1.R255 [ PubMed ] [ CrossRef ] [ Google Scholar ]
- Bunnell D. E., Agnew J. A., Horvath S. M., Jopson L., Wills M. (1988). Passive body heating and sleep: influence of proximity to sleep. Sleep 11 210–219. 10.1093/sleep/11.2.210 [ PubMed ] [ CrossRef ] [ Google Scholar ]
- Campbell S. S., Broughton R. J. (1994). Rapid decline in body temperature before sleep: fluffing the physiological pillow? Chronobiol. Int. 11 126–131. 10.3109/07420529409055899 [ PubMed ] [ CrossRef ] [ Google Scholar ]
- Cannon B., Nedergaard J. (2004). Brown adipose tissue: function and physiological significance. Physiol. Rev. 84 277–359. 10.1152/physrev.00015.2003 [ PubMed ] [ CrossRef ] [ Google Scholar ]
- Carey H. V., Andrews M. T., Martin S. L. (2003). Mammalian hibernation: cellular and molecular responses to depressed metabolism and low temperature. Physiol. Rev. 83 1153–1181. 10.1152/physrev.00008.2003 [ PubMed ] [ CrossRef ] [ Google Scholar ]
- Cespuglio R., Amrouni D., Meiller A., Buguet A., Gautier-Sauvigné S. (2012). Nitric oxide in the regulation of the sleep-wake states. Sleep Med. Rev. 16 265–279. 10.1016/j.smrv.2012.01.006 [ PubMed ] [ CrossRef ] [ Google Scholar ]
- Chou T. C., Bjorkum A. A., Gaus S. E., Lu J., Scammell T. E., Saper C. B. (2002). Afferents to the ventrolateral preoptic nucleus. J. Neurosci. 22 977–990. 10.1523/JNEUROSCI.22-03-00977.2002 [ PMC free article ] [ PubMed ] [ CrossRef ] [ Google Scholar ]
- Cowley M. A., Smart J. L., Rubinstein M., Cerdan M. G., Diano S., Horvath T. L., et al. (2001). Leptin activates anorexigenic POMC neurons through a neural network in the arcuate nucleus. Nature 411 480–484. 10.1038/35078085 [ PubMed ] [ CrossRef ] [ Google Scholar ]
- Czeisler C. A., Zimmerman J. C., Ronda J. M., Moore-Ede M. C., Weitzman E. D. (1980). Timing of REM sleep is coupled to the circadian rhythm of body temperature in man. Sleep 2 329–346. 10.1093/sleep/2.3.329 [ PubMed ] [ CrossRef ] [ Google Scholar ]
- Daan S., Barnes B. M., Strijkstra A. M. (1991). Warming up for sleep? Ground squirrels sleep during arousals from hibernation. Neurosci. Lett. 128 265–268. 10.1016/0304-3940(91)90276-Y [ PubMed ] [ CrossRef ] [ Google Scholar ]
- Dausmann K. H., Glos J., Ganzhorn J. U., Heldmaier G. (2004). Physiology: hibernation in a tropical primate. Nature 429 825–826. 10.1038/429825a [ PubMed ] [ CrossRef ] [ Google Scholar ]
- Deacon R. M. J. (2006). Assessing nest building in mice. Nat. Protoc. 1 1117–1119. 10.1038/nprot.2006.170 [ PubMed ] [ CrossRef ] [ Google Scholar ]
- Deboer T. (1998). Brain temperature dependent changes in the electroencephalogram power spectrum of humans and animals. J. Sleep Res. 7 254–262. 10.1046/j.1365-2869.1998.00125.x [ PubMed ] [ CrossRef ] [ Google Scholar ]
- Deboer T., Tobler I. (1994). Sleep EEG after daily torpor in the Djungarian hamster: similarity to the effects of sleep deprivation. Neurosci. Lett. 166 35–38. 10.1016/0304-3940(94)90834-6 [ PubMed ] [ CrossRef ] [ Google Scholar ]
- Deboer T., Tobler I. (2003). Sleep regulation in the Djungarian hamster: comparison of the dynamics leading to the slow-wave activity increase after sleep deprivation and daily torpor. Sleep 26 567–572. 10.1093/sleep/26.5.567 [ PubMed ] [ CrossRef ] [ Google Scholar ]
- Díaz M., Becker D. E. (2010). Thermoregulation: physiological and clinical considerations during sedation and general anesthesia. Anesth. Prog. 57 25–32. 10.2344/0003-3006-57.1.25 [ PMC free article ] [ PubMed ] [ CrossRef ] [ Google Scholar ]
- Dorsey C. M., Teicher M. H., Cohen-Zion M., Stefanovic L., Satlin A., Tartarini W., et al. (1999). Core body temperature and sleep of older female insomniacs before and after passive body heating. Sleep 22 891–898. 10.1093/sleep/22.7.891 [ PubMed ] [ CrossRef ] [ Google Scholar ]
- Eban-Rothschild A., Giardino W. J., de Lecea L. (2017). To sleep or not to sleep: neuronal and ecological insights. Curr. Opin. Neurobiol. 44 132–138. 10.1016/j.conb.2017.04.010 [ PMC free article ] [ PubMed ] [ CrossRef ] [ Google Scholar ]
- Eban-Rothschild A., Rothschild G., Giardino W. J., Jones J. R., de Lecea L. (2016). VTA dopaminergic neurons regulate ethologically relevant sleep–wake behaviors. Nat. Neurosci. 19 1356 . 10.1038/nn.4377 [ PMC free article ] [ PubMed ] [ CrossRef ] [ Google Scholar ]
- Elias C. F., Aschkenasi C., Lee C., Kelly J., Ahima R. S., Bjorbæk C., et al. (1999). Leptin Differentially Regulates NPY and POMC Neurons Projecting to the Lateral Hypothalamic Area. Neuron 23 775–786. 10.1016/S0896-6273(01)80035-0 [ PubMed ] [ CrossRef ] [ Google Scholar ]
- Everson C. A., Bergmann B. M., Rechtschaffen A. (1989). Sleep deprivation in the rat: III. Total sleep deprivation. Sleep 12 13–21. 10.1093/sleep/12.1.13 [ PubMed ] [ CrossRef ] [ Google Scholar ]
- Frerichs K. U., Kennedy C., Sokoloff L., Hallenbeck J. M. (1994). Local cerebral blood flow during hibernation, a model of natural tolerance to “cerebral ischemia”. J. Cereb. Blood Flow Metab. 14 193–205. 10.1038/jcbfm.1994.26 [ PubMed ] [ CrossRef ] [ Google Scholar ]
- Fronczek R., Overeem S., Lammers G. J., van Dijk J. G., Van Someren E. J. (2006). Altered skin-temperature regulation in narcolepsy relates to sleep propensity. Sleep 29 1444–1449. 10.1093/sleep/29.11.1444 [ PubMed ] [ CrossRef ] [ Google Scholar ]
- Fronczek R., Raymann R. J., Overeem S., Romeijn N., van Dijk J. G., Lammers G. J., et al. (2008a). Manipulation of skin temperature improves nocturnal sleep in narcolepsy. J. Neurol. Neurosurg. Psychiatry 79 1354–1357. 10.1136/jnnp.2008.143610 [ PubMed ] [ CrossRef ] [ Google Scholar ]
- Fronczek R., Raymann R. J., Romeijn N., Overeem S., Fischer M., van Dijk J. G., et al. (2008b). Manipulation of core body and skin temperature improves vigilance and maintenance of wakefulness in narcolepsy. Sleep 31 233–240. [ PMC free article ] [ PubMed ] [ Google Scholar ]
- Gaskill B., Rohr S. A., Pajor E. A., Lucas J., Garner J. (2011). Working with what you’ve got: changes in thermal preference and behavior in mice with or without nesting material. J. Thermal Biol. 36 193–199. 10.1016/j.jtherbio.2011.02.004 [ CrossRef ] [ Google Scholar ]
- Gaskill B. N., Gordon C. J., Pajor E. A., Lucas J. R., Davis J. K., Garner J. P. (2012). Heat or insulation: behavioral titration of mouse preference for warmth or access to a nest. PLoS One 7 : e32799 . 10.1371/journal.pone.0032799 [ PMC free article ] [ PubMed ] [ CrossRef ] [ Google Scholar ]
- Gaskill B. N., Gordon C. J., Pajor E. A., Lucas J. R., Davis J. K., Garner J. P. (2013a). Impact of nesting material on mouse body temperature and physiology. Physiol. Behav. 11 87–95. 10.1016/j.physbeh.2012.12.018 [ PubMed ] [ CrossRef ] [ Google Scholar ]
- Gaskill B. N., Pritchett-Corning K. R., Gordon C. J., Pajor E. A., Lucas J. R., Davis J. K., et al. (2013b). Energy reallocation to breeding performance through improved nest building in laboratory mice. PLoS One 8 : e74153 . 10.1371/journal.pone.0074153 [ PMC free article ] [ PubMed ] [ CrossRef ] [ Google Scholar ]
- Gerashchenko D., Wisor J. P., Burns D., Reh R. K., Shiromani P. J., Sakurai T., et al. (2008). Identification of a population of sleep-active cerebral cortex neurons. Proc. Natl. Acad. Sci. U.S.A. 105 10227–10232. 10.1073/pnas.0803125105 [ PMC free article ] [ PubMed ] [ CrossRef ] [ Google Scholar ]
- Glotzbach S. F., Heller H. C. (1976). Central nervous regulation of body temperature during sleep. Science 194 537–539. 10.1126/science.973138 [ PubMed ] [ CrossRef ] [ Google Scholar ]
- Goldstein N., Levine B. J., Loy K. A., Duke W. L., Meyerson O. S., Jamnik A. A., et al. (2018). Hypothalamic neurons that regulate feeding can influence sleep/wake states based on homeostatic need. Curr. Biol. 28 3736–3747.e3. 10.1016/j.cub.2018.09.055 [ PMC free article ] [ PubMed ] [ CrossRef ] [ Google Scholar ]
- Gong H., Szymusiak R., King J., Steininger T., McGinty D. (2000). Sleep-related c-Fos protein expression in the preoptic hypothalamus: effects of ambient warming. Am. J. Physiol. Regul. Integr. Comp. Physiol. 279 R2079–R2088. 10.1152/ajpregu.2000.279.6.R2079 [ PubMed ] [ CrossRef ] [ Google Scholar ]
- Gordon C. J., Aydin C., Repasky E. A., Kokolus K. M., Dheyongera G., Johnstone A. F. (2014). Behaviorally mediated, warm adaptation: a physiological strategy when mice behaviorally thermoregulate. J. Therm. Biol. 44 41–46. 10.1016/j.jtherbio.2014.06.006 [ PubMed ] [ CrossRef ] [ Google Scholar ]
- Gordon C. J., Becker P., Ali J. S. (1998). Behavioral thermoregulatory responses of single- and group-housed mice. Physiol. Behav. 65 255–262. 10.1016/S0031-9384(98)00148-6 [ PubMed ] [ CrossRef ] [ Google Scholar ]
- Guzmán-Marín R., Alam M. N., Szymusiak R., Drucker-Colín R., Gong H., McGinty D. (2000). Discharge modulation of rat dorsal raphe neurons during sleep and waking: effects of preoptic/basal forebrain warming. Brain Res. 875 23–34. 10.1016/S0006-8993(00)02561-0 [ PubMed ] [ CrossRef ] [ Google Scholar ]
- Hammel H. T. (1968). Regulation of internal body temperature. Annu. Rev. Physiol. 30 641–710. 10.1146/annurev.ph.30.030168.003233 [ PubMed ] [ CrossRef ] [ Google Scholar ]
- Han S., Kim J., Won S. M., Ma Y., Kang D., Xie Z., et al. (2018). Battery-free, wireless sensors for full-body pressure and temperature mapping. Sci. Transl. Med. 10 : eaan4950 . 10.1126/scitranslmed.aan4950 [ PMC free article ] [ PubMed ] [ CrossRef ] [ Google Scholar ]
- Harding E. C., Yu X., Miao A., Andrews N., Ma Y., Ye Z., et al. (2018). A neuronal hub binding sleep initiation and body cooling in response to a warm external stimulus. Curr. Biol. 28 2263–2273.e2. 10.1016/j.cub.2018.05.054 [ PMC free article ] [ PubMed ] [ CrossRef ] [ Google Scholar ]
- Haskell E. H., Palca J. W., Walker J. M., Berger R. J., Heller H. C. (1981). The effects of high and low ambient temperatures on human sleep stages. Electroencephalogr. Clin. Neurophysiol. 51 494–501. 10.1016/0013-4694(81)90226-1 [ PubMed ] [ CrossRef ] [ Google Scholar ]
- Hayward J. N. (1968). Brain temperature regulation during sleep and arousal in the dog. Exp. Neurol. 21 201–212. 10.1016/0014-4886(68)90138-6 [ PubMed ] [ CrossRef ] [ Google Scholar ]
- Hibi M., Kubota C., Mizuno T., Aritake S., Mitsui Y., Katashima M., et al. (2017). Effect of shortened sleep on energy expenditure, core body temperature, and appetite: a human randomised crossover trial. Sci. Rep. 7 : 39640 . 10.1038/srep39640 [ PMC free article ] [ PubMed ] [ CrossRef ] [ Google Scholar ]
- Hoekstra M. M. B., Emmenegger Y., Hubbard J., Franken P. (2019). Cold-inducible RNA-binding protein (CIRBP) adjusts clock-gene expression and REM-sleep recovery following sleep deprivation. Elife 8 : e43400 . 10.7554/eLife.43400 [ PMC free article ] [ PubMed ] [ CrossRef ] [ Google Scholar ]
- Horne J. A., Reid A. J. (1985). Night-time sleep EEG changes following body heating in a warm bath. Electroencephalogr. Clin. Neurophysiol. 60 154–157. 10.1016/0013-4694(85)90022-7 [ PubMed ] [ CrossRef ] [ Google Scholar ]
- Imeri L., Opp M. R. (2009). How (and why) the immune system makes us sleep. Nat. Rev. Neurosci. 10 199–210. 10.1038/nrn2576 [ PMC free article ] [ PubMed ] [ CrossRef ] [ Google Scholar ]
- Jeong J. H., Lee D. K., Liu S. M., Chua SC, Jr, Schwartz G. J., Jo Y. H. (2018). Activation of temperature-sensitive TRPV1-like receptors in ARC POMC neurons reduces food intake. PLoS Biol. 16 : e2004399 . 10.1371/journal.pbio.2004399 [ PMC free article ] [ PubMed ] [ CrossRef ] [ Google Scholar ]
- Jordan J., Montgomery I., Trinder J. (1990). The effect of afternoon body heating on body temperature and slow wave sleep. Psychophysiology 27 560–566. 10.1111/j.1469-8986.1990.tb01976.x [ PubMed ] [ CrossRef ] [ Google Scholar ]
- Jung C. M., Melanson E. L., Frydendall E. J., Perreault L., Eckel R. H., Wright K. P. (2011). Energy expenditure during sleep, sleep deprivation and sleep following sleep deprivation in adult humans. J. Physiol. 589(Pt 1) 235–244. 10.1113/jphysiol.2010.197517 [ PMC free article ] [ PubMed ] [ CrossRef ] [ Google Scholar ]
- Kalinchuk A. V., McCarley R. W., Porkka-Heiskanen T., Basheer R. (2010). Sleep deprivation triggers inducible nitric oxide-dependent nitric oxide production in wake-active basal forebrain neurons. J. Neurosci. 30 13254–13264. 10.1523/jneurosci.0014-10.2010 [ PMC free article ] [ PubMed ] [ CrossRef ] [ Google Scholar ]
- Kauffman A. S., Paul M. J., Butler M. P., Zucker I. (2003). Huddling, locomotor, and nest-building behaviors of furred and furless Siberian hamsters. Physiol. Behav. 79 247–256. 10.1016/S0031-9384(03)00115-X [ PubMed ] [ CrossRef ] [ Google Scholar ]
- Koops K., McGrew W. C., de Vries H., Matsuzawa T. (2012). Nest-Building by Chimpanzees (Pan troglodytes verus) at Seringbara, Nimba Mountains: Antipredation, Thermoregulation, and Antivector Hypotheses. Int. J. Primatol. 33 356–380. 10.1007/s10764-012-9585-4 [ CrossRef ] [ Google Scholar ]
- Krauchi K., Cajochen C., Pache M., Flammer J., Wirz-Justice A. (2006). Thermoregulatory effects of melatonin in relation to sleepiness. Chronobiol. Int. 23 475–484. 10.1080/07420520500545854 [ PubMed ] [ CrossRef ] [ Google Scholar ]
- Krauchi K., Cajochen C., Werth E., Wirz-Justice A. (1999). Warm feet promote the rapid onset of sleep. Nature 401 36–37. 10.1038/43366 [ PubMed ] [ CrossRef ] [ Google Scholar ]
- Krauchi K., Cajochen C., Werth E., Wirz-Justice A. (2000). Functional link between distal vasodilation and sleep-onset latency? Am. J. Physiol. Regul. Integr. Comp. Physiol. 278 R741–R748. 10.1152/ajpregu.2000.278.3.R741 [ PubMed ] [ CrossRef ] [ Google Scholar ]
- Krauchi K., Cajochen C., Wirz-Justice A. (1997). A relationship between heat loss and sleepiness: effects of postural change and melatonin administration. J. Appl. Physiol. 83 134–139. 10.1152/jappl.1997.83.1.134 [ PubMed ] [ CrossRef ] [ Google Scholar ]
- Kräuchi K., Wirz-Justice A. (2001). Circadian clues to sleep onset mechanisms. Neuropsychopharmacology 25 : S92 . 10.1016/S0893-133X(01)00315-3 [ PubMed ] [ CrossRef ] [ Google Scholar ]
- Krilowicz B. L., Szymusiak R., McGinty D. (1994). Regulation of posterior lateral hypothalamic arousal related neuronal discharge by preoptic anterior hypothalamic warming. Brain Res. 668 30–38. 10.1016/0006-8993(94)90507-X [ PubMed ] [ CrossRef ] [ Google Scholar ]
- Kroeger D., Absi G., Gagliardi C., Bandaru S. S., Madara J. C., Ferrari L. L., et al. (2018). Galanin neurons in the ventrolateral preoptic area promote sleep and heat loss in mice. Nat. Commun. 9 : 4129 . 10.1038/s41467-018-06590-7 [ PMC free article ] [ PubMed ] [ CrossRef ] [ Google Scholar ]
- Krystal A. D., Schopler B., Kobbe S., Williams C., Rakatondrainibe H., Yoder A. D., et al. (2013). The relationship of sleep with temperature and metabolic rate in a hibernating primate. PLoS One 8 : e69914 . 10.1371/journal.pone.0069914 [ PMC free article ] [ PubMed ] [ CrossRef ] [ Google Scholar ]
- Lack L. C., Gradisar M., Van Someren E. J. W., Wright H. R., Lushington K. (2008). The relationship between insomnia and body temperatures. Sleep Med. Rev. 12 307–317. 10.1016/j.smrv.2008.02.003 [ PubMed ] [ CrossRef ] [ Google Scholar ]
- Landolt H. P., Moser S., Wieser H. G., Borbely A. A., Dijk D. J. (1995). Intracranial temperature across 24-hour sleep-wake cycles in humans. Neuroreport 6 913–917. 10.1097/00001756-199504190-00022 [ PubMed ] [ CrossRef ] [ Google Scholar ]
- Laposky A. D., Shelton J., Bass J., Dugovic C., Perrino N., Turek F. W. (2006). Altered sleep regulation in leptin-deficient mice. Am. J. Physiol. Regul. Integr. Comp. Physiol. 290 R894–R903. 10.1152/ajpregu.00304.2005 [ PubMed ] [ CrossRef ] [ Google Scholar ]
- Larkin J. E., Heller H. C. (1996). Temperature sensitivity of sleep homeostasis during hibernation in the golden-mantled ground squirrel. Am. J. Physiol. Regul. Integr. Comp. Physiol. 270 R777–R784. 10.1152/ajpregu.1996.270.4.R777 [ PubMed ] [ CrossRef ] [ Google Scholar ]
- Lazarus M., Yoshida K., Coppari R., Bass C. E., Mochizuki T., Lowell B. B., et al. (2007). EP3 prostaglandin receptors in the median preoptic nucleus are critical for fever responses. Nat. Neurosci. 10 1131–1133. 10.1038/nn1949 [ PubMed ] [ CrossRef ] [ Google Scholar ]
- Leshan R. L., Greenwald-Yarnell M., Patterson C. M., Gonzalez I. E., Myers M. G., Jr. (2012). Leptin action through hypothalamic nitric oxide synthase-1–expressing neurons controls energy balance. Nat. Med. 18 820–823. 10.1038/nm.2724 [ PMC free article ] [ PubMed ] [ CrossRef ] [ Google Scholar ]
- Logan R. W., McClung C. A. (2019). Rhythms of life: circadian disruption and brain disorders across the lifespan. Nat. Rev. Neurosci. 20 49–65. 10.1038/s41583-018-0088-y [ PMC free article ] [ PubMed ] [ CrossRef ] [ Google Scholar ]
- Ma Y., Miracca G., Yu X., Harding E. C., Miao A., Yustos R., et al. (2019). Galanin neurons in the hypothalamus link sleep homeostasis, body temperature and actions of the alpha2 adrenergic agonist dexmedetomidine. bioRxiv 10.1101/565747 [ CrossRef ] [ Google Scholar ]
- Magariños A. M., McEwen B. S., Saboureau M., Pevet P. (2006). Rapid and reversible changes in intrahippocampal connectivity during the course of hibernation in European hamsters. Proc. Natl. Acad. Sci. U.S.A. 103 18775–18780. 10.1073/pnas.0608785103 [ PMC free article ] [ PubMed ] [ CrossRef ] [ Google Scholar ]
- Matsukawa T., Kurz A., Sessler D. I., Bjorksten A. R., Merrifield B., Cheng C. (1995). Propofol linearly reduces the vasoconstriction and shivering thresholds. Anesthesiology 82 1169–1180. 10.1097/00000542-199505000-00012 [ PubMed ] [ CrossRef ] [ Google Scholar ]
- McGinty D., Szymusiak R. (1990). Keeping cool: a hypothesis about the mechanisms and functions of slow-wave sleep. Trends Neurosci. 13 480–487. 10.1016/0166-2236(90)90081-K [ PubMed ] [ CrossRef ] [ Google Scholar ]
- McGinty D., Szymusiak R., Thomson D. (1994). Preoptic/anterior hypothalamic warming increases EEG delta frequency activity within non-rapid eye movement sleep. Brain Res. 667 273–277. 10.1016/0006-8993(94)91506-7 [ PubMed ] [ CrossRef ] [ Google Scholar ]
- Moffitt J. R., Bambah-Mukku D., Eichhorn S. W., Vaughn E., Shekhar K., Perez J. D., et al. (2018). Molecular, spatial and functional single-cell profiling of the hypothalamic preoptic region. Science 362 : eaau5324 . 10.1126/science.aau5324 [ PMC free article ] [ PubMed ] [ CrossRef ] [ Google Scholar ]
- Morairty S. R., Dittrich L., Pasumarthi R. K., Valladao D., Heiss J. E., Gerashchenko D., et al. (2013). A role for cortical nNOS/NK1 neurons in coupling homeostatic sleep drive to EEG slow wave activity. Proc. Natl. Acad. Sci. U.S.A. 110 20272–20277. 10.1073/pnas.1314762110 [ PMC free article ] [ PubMed ] [ CrossRef ] [ Google Scholar ]
- Morairty S. R., Szymusiak R., Thomson D., McGinty D. J. (1993). Selective increases in non-rapid eye movement sleep following whole body heating in rats. Brain Res. 617 10–16. 10.1016/0006-8993(93)90606-N [ PubMed ] [ CrossRef ] [ Google Scholar ]
- Morf J., Rey G., Schneider K., Stratmann M., Fujita J., Naef F., et al. (2012). Cold-Inducible RNA-binding protein modulates circadian gene expression posttranscriptionally. Science 338 : 379 . 10.1126/science.1217726 [ PubMed ] [ CrossRef ] [ Google Scholar ]
- Morrison S. F., Nakamura K. (2011). Central neural pathways for thermoregulation. Front. Biosci. 16 74–104. 10.2741/3677 [ PMC free article ] [ PubMed ] [ CrossRef ] [ Google Scholar ]
- Muzet A., Libert J. P., Candas V. (1984). Ambient temperature and human sleep. Experientia 40 425–429. 10.1007/BF01952376 [ PubMed ] [ CrossRef ] [ Google Scholar ]
- Nakamura K., Morrison S. F. (2008). A thermosensory pathway that controls body temperature. Nat. Neurosci. 11 62–71. 10.1038/nn2027 [ PMC free article ] [ PubMed ] [ CrossRef ] [ Google Scholar ]
- Nakamura K., Morrison S. F. (2010). A thermosensory pathway mediating heat-defense responses. Proc. Natl. Acad. Sci. U.S.A. 107 8848–8853. 10.1073/pnas.0913358107 [ PMC free article ] [ PubMed ] [ CrossRef ] [ Google Scholar ]
- Okamoto-Mizuno K., Nagai Y., Iizuka S. (2003). The effect of ambient temperature change on the covered area of the body during sleep. J. Home Econ. Jpn. 54 1025–1030. 10.11428/jhej1987.54.1025 [ CrossRef ] [ Google Scholar ]
- Ozaki S., Uchiyama M., Shirakawa S., Okawa M. (1996). Prolonged interval from body temperature nadir to sleep offset in patients with delayed sleep phase syndrome. Sleep 19 36–40. [ PubMed ] [ Google Scholar ]
- Pache M., Krauchi K., Cajochen C., Wirz-Justice A., Dubler B., Flammer J., et al. (2001). Cold feet and prolonged sleep-onset latency in vasospastic syndrome. Lancet 358 125–126. 10.1016/s0140-6736(01)05344-2 [ PubMed ] [ CrossRef ] [ Google Scholar ]
- Palchykova S., Deboer T., Tobler I. (2002). Selective sleep deprivation after daily torpor in the Djungarian hamster. J. Sleep Res. 11 313–319. 10.1046/j.1365-2869.2002.00310.x [ PubMed ] [ CrossRef ] [ Google Scholar ]
- Parmeggiani P. L. (1987). Interaction between sleep and thermoregulation: an aspect of the control of behavioral states. Sleep 10 426–435. 10.1093/sleep/10.5.426 [ PubMed ] [ CrossRef ] [ Google Scholar ]
- Peever J. (2018). Neuroscience: a “Skin Warming” circuit that promotes sleep and body cooling. Curr. Biol. 28 R800–R802. 10.1016/j.cub.2018.06.043 [ PubMed ] [ CrossRef ] [ Google Scholar ]
- Peretti D., Bastide A., Radford H., Verity N., Molloy C., Martin M. G., et al. (2015). RBM3 mediates structural plasticity and protective effects of cooling in neurodegeneration. Nature 518 236–239. 10.1038/nature14142 [ PMC free article ] [ PubMed ] [ CrossRef ] [ Google Scholar ]
- Popov V. I., Bocharova L. S. (1992). Hibernation-induced structural changes in synaptic contacts between mossy fibres and hippocampal pyramidal neurons. Neuroscience 48 53–62. 10.1016/0306-4522(92)90337-2 [ PubMed ] [ CrossRef ] [ Google Scholar ]
- Popov V. I., Medvedev N. I., Patrushev I. V., Ignat’ev D. A., Morenkov E. D., Stewart M. G. (2007). Reversible reduction in dendritic spines in CA1 of rat and ground squirrel subjected to hypothermia-normothermia in vivo: a three-dimensional electron microscope study. Neuroscience 149 549–560. 10.1016/j.neuroscience.2007.07.059 [ PubMed ] [ CrossRef ] [ Google Scholar ]
- Prete F. R., Bergmann B. M., Holtzman P., Obermeyer W., Rechtschaffen A. (1991). Sleep deprivation in the rat: XII. Effect on ambient temperature choice. Sleep 14 109–115. 10.1093/sleep/14.2.109 [ PubMed ] [ CrossRef ] [ Google Scholar ]
- Ray B., Mallick H. N., Kumar V. M. (2005). Changes in sleep–wakefulness in the medial preoptic area lesioned rats: role of thermal preference. Behav. Brain Res. 158 43–52. 10.1016/j.bbr.2004.08.006 [ PubMed ] [ CrossRef ] [ Google Scholar ]
- Raymann R. J., Swaab D. F., Van Someren E. J. (2005). Cutaneous warming promotes sleep onset. Am. J. Physiol. Regul. Integr. Comp. Physiol. 288 R1589–R1597. 10.1152/ajpregu.00492.2004 [ PubMed ] [ CrossRef ] [ Google Scholar ]
- Raymann R. J., Swaab D. F., Van Someren E. J. (2008). Skin deep: enhanced sleep depth by cutaneous temperature manipulation. Brain 131(Pt 2) 500–513. 10.1093/brain/awm315 [ PubMed ] [ CrossRef ] [ Google Scholar ]
- Raymann R. J. E. M., Van Someren E. J. W. (2008). Diminished capability to recognize the optimal temperature for sleep initiation may contribute to poor sleep in elderly people. Sleep 31 1301–1309. [ PMC free article ] [ PubMed ] [ Google Scholar ]
- Rechtschaffen A., Bergmann B. M. (1995). Sleep deprivation in the rat by the disk-over-water method. Behav. Brain Res. 69 55–63. 10.1016/0166-4328(95)00020-T [ PubMed ] [ CrossRef ] [ Google Scholar ]
- Roberts W. W., Robinson T. C. L. (1969). Relaxation and sleep induced by warming of preoptic region and anterior hypothalamus in cats. Exp. Neurol. 25 282–294. 10.1016/0014-4886(69)90051-X [ PubMed ] [ CrossRef ] [ Google Scholar ]
- Rubinstein E. H., Sessler D. I. (1990). Skin-surface temperature gradients correlate with fingertip blood flow in humans. Anesthesiology 73 541–545. 10.1097/00000542-199009000-00027 [ PubMed ] [ CrossRef ] [ Google Scholar ]
- Ruf T., Geiser F. (2015). Daily torpor and hibernation in birds and mammals. Biol. Rev. Camb. Philos. Soc. 90 891–926. 10.1111/brv.12137 [ PMC free article ] [ PubMed ] [ CrossRef ] [ Google Scholar ]
- Samson D. R., Hunt K. D. (2012). A thermodynamic comparison of arboreal and terrestrial sleeping sites for dry-habitat chimpanzees (Pan troglodytes schweinfurthii) at the Toro-Semliki Wildlife Reserve. Uganda. Am. J. Primatol. 74 811–818. 10.1002/ajp.22031 [ PubMed ] [ CrossRef ] [ Google Scholar ]
- Scammell T. E., Elmquist J. K., Griffin J. D., Saper C. B. (1996). Ventromedial preoptic prostaglandin E2 activates fever-producing autonomic pathways. J. Neurosci. 16 6246–6254. 10.1523/JNEUROSCI.16-19-06246.1996 [ PMC free article ] [ PubMed ] [ CrossRef ] [ Google Scholar ]
- Scammell T. E., Price K. J., Sagar S. M. (1993). Hyperthermia induces c-fos expression in the preoptic area. Brain Res. 618 303–307. 10.1016/0006-8993(93)91280-6 [ PubMed ] [ CrossRef ] [ Google Scholar ]
- Sessler D. I. (2016). Perioperative thermoregulation and heat balance. Lancet 387 2655–2664. 10.1016/S0140-6736(15)00981-2 [ PubMed ] [ CrossRef ] [ Google Scholar ]
- Shapiro C. M., Allan M., Driver H., Mitchell D. (1989). Thermal load alters sleep. Biol. Psychiatry 26 736–740. 10.1016/0006-3223(89)90110-8 [ PubMed ] [ CrossRef ] [ Google Scholar ]
- Sherin J. E., Elmquist J. K., Torrealba F., Saper C. B. (1998). Innervation of histaminergic tuberomammillary neurons by GABAergic and galaninergic neurons in the ventrolateral preoptic nucleus of the rat. J. Neurosci. 18 4705–4721. 10.1523/JNEUROSCI.18-12-04705.1998 [ PMC free article ] [ PubMed ] [ CrossRef ] [ Google Scholar ]
- Shi Y. C., Lau J., Lin Z., Zhang H., Zhai L., Sperk G., et al. (2013). Arcuate NPY controls sympathetic output and BAT function via a relay of tyrosine hydroxylase neurons in the PVN. Cell Metab. 17 236–248. 10.1016/j.cmet.2013.01.006 [ PubMed ] [ CrossRef ] [ Google Scholar ]
- Siemens J., Kamm G. B. (2018). Cellular populations and thermosensing mechanisms of the hypothalamic thermoregulatory center. Pflügers Archiv. 470 809–822. 10.1007/s00424-017-2101-0 [ PubMed ] [ CrossRef ] [ Google Scholar ]
- Sinton C. M., Fitch T. E., Gershenfeld H. K. (1999). The effects of leptin on REM sleep and slow wave deltain rats are reversed by food deprivation. J. Sleep Res. 8 197–203. 10.1046/j.1365-2869.1999.00158.x [ PubMed ] [ CrossRef ] [ Google Scholar ]
- Song K., Wang H., Kamm G. B., Pohle J., de Castro Reis F., Heppenstall P., et al. (2016). The TRPM2 channel is a hypothalamic heat sensor that limits fever and can drive hypothermia. Science 353 1393–1398. 10.1126/science.aaf7537 [ PMC free article ] [ PubMed ] [ CrossRef ] [ Google Scholar ]
- Steininger T. L., Gong H., McGinty D., Szymusiak R. (2001). Subregional organization of preoptic area/anterior hypothalamic projections to arousal-related monoaminergic cell groups. J. Comp. Neurol. 429 638–653. 10.1002/1096-9861(20010122)429:4<638::AID-CNE10>3.0.CO;2-Y [ PubMed ] [ CrossRef ] [ Google Scholar ]
- Stewart F. A., Piel A. K., Azkarate J. C., Pruetz J. D. (2018). Savanna chimpanzees adjust sleeping nest architecture in response to local weather conditions. Am. J. Phys. Anthropol. 166 549–562. 10.1002/ajpa.23461 [ PubMed ] [ CrossRef ] [ Google Scholar ]
- Strijkstra A., Daan S. (1997). Sleep during arousal episodes as a function of prior torpor duration in hibernating European ground squirrels. J. Sleep Res. 6 36–43. 10.1046/j.1365-2869.1997.00024.x [ PubMed ] [ CrossRef ] [ Google Scholar ]
- Suntsova N., Szymusiak R., Alam M. N., Guzman-Marin R., McGinty D. (2002). Sleep-waking discharge patterns of median preoptic nucleus neurons in rats. J. Physiol. 543(Pt 2) 665–677. 10.1113/jphysiol.2002.023085 [ PMC free article ] [ PubMed ] [ CrossRef ] [ Google Scholar ]
- Szentirmai E., Kapas L. (2014). Intact brown adipose tissue thermogenesis is required for restorative sleep responses after sleep loss. Eur. J. Neurosci. 39 984–998. 10.1111/ejn.12463 [ PubMed ] [ CrossRef ] [ Google Scholar ]
- Szentirmai E., Kapas L. (2018). Brown adipose tissue plays a central role in systemic inflammation-induced sleep responses. PLoS One 13 : e0197409 . 10.1371/journal.pone.0197409 [ PMC free article ] [ PubMed ] [ CrossRef ] [ Google Scholar ]
- Szentirmai ,É, Kapás L. (2017). The role of the brown adipose tissue in β3-adrenergic receptor activation-induced sleep, metabolic and feeding responses. Sci. Rep. 7 : 958 . 10.1038/s41598-017-01047-1 [ PMC free article ] [ PubMed ] [ CrossRef ] [ Google Scholar ]
- Szymusiak R., Danowski J., McGinty D. (1991). Exposure to heat restores sleep in cats with preoptic/anterior hypothalamic cell loss. Brain Res. 541 134–138. 10.1016/0006-8993(91)91086-G [ PubMed ] [ CrossRef ] [ Google Scholar ]
- Szymusiak R., Gvilia I., McGinty D. (2007). Hypothalamic control of sleep. Sleep Med. 8 291–301. 10.1016/j.sleep.2007.03.013 [ PubMed ] [ CrossRef ] [ Google Scholar ]
- Szymusiak R., McGinty D. (1986). Sleep-related neuronal discharge in the basal forebrain of cats. Brain Res. 370 82–92. 10.1016/0006-8993(86)91107-8 [ PubMed ] [ CrossRef ] [ Google Scholar ]
- Szymusiak R., Satinoff E. (1981). Maximal REM sleep time defines a narrower thermoneutral zone than does minimal metabolic rate. Physiol. Behav. 26 687–690. 10.1016/0031-9384(81)90145-1 [ PubMed ] [ CrossRef ] [ Google Scholar ]
- Talke P., Tayefeh F., Sessler D. I., Jeffrey R., Noursalehi M., Richardson C. (1997). Dexmedetomidine does not alter the sweating threshold, but comparably and linearly decreases the vasoconstriction and shivering thresholds. Anesthesiology 87 835–841. 10.1097/00000542-199710000-00017 [ PubMed ] [ CrossRef ] [ Google Scholar ]
- Tan C. L., Cooke E. K., Leib D. E., Lin Y. C., Daly G. E., Zimmerman C. A., et al. (2016). Warm-sensitive neurons that control body temperature. Cell 167 47–59.e15. 10.1016/j.cell.2016.08.028 [ PMC free article ] [ PubMed ] [ CrossRef ] [ Google Scholar ]
- Tan C. L., Knight Z. A. (2018). Regulation of body temperature by the nervous system. Neuron 98 31–48. 10.1016/j.neuron.2018.02.022 [ PMC free article ] [ PubMed ] [ CrossRef ] [ Google Scholar ]
- Tøien Ø, Blake J., Barnes B. M. (2015). Thermoregulation and energetics in hibernating black bears: metabolic rate and the mystery of multi-day body temperature cycles. J. Comp. Physiol. B 185 447–461. 10.1007/s00360-015-0891-y [ PubMed ] [ CrossRef ] [ Google Scholar ]
- Tøien Ø, Blake J., Edgar D. M., Grahn D. A., Heller H. C., Barnes B. M. (2011). Hibernation in black bears: independence of metabolic suppression from body temperature. Science 331 : 906 . 10.1126/science.1199435 [ PubMed ] [ CrossRef ] [ Google Scholar ]
- Uschakov A., Gong H., McGinty D., Szymusiak R. (2007). Efferent projections from the median preoptic nucleus to sleep- and arousal-regulatory nuclei in the rat brain. Neuroscience 150 104–120. 10.1016/j.neuroscience.2007.05.055 [ PMC free article ] [ PubMed ] [ CrossRef ] [ Google Scholar ]
- van den Heuvel C. J., Noone J. T., Lushington K., Dawson D. (1998). Changes in sleepiness and body temperature precede nocturnal sleep onset: evidence from a polysomnographic study in young men. J. Sleep Res. 7 159–166. 10.1046/j.1365-2869.1998.00112.x [ PubMed ] [ CrossRef ] [ Google Scholar ]
- van Marken Lichtenbelt W. D., Daanen H. A. M., Wouters L., Fronczek R., Raymann R. J. E. M., Severens N. M. W., et al. (2006). Evaluation of wireless determination of skin temperature using iButtons. Physiol. Behav. 88 489–497. 10.1016/j.physbeh.2006.04.026 [ PubMed ] [ CrossRef ] [ Google Scholar ]
- Van Someren E. J. (2000). More than a marker: interaction between the circadian regulation of temperature and sleep, age-related changes, and treatment possibilities. Chronobiol. Int. 17 313–354. 10.1081/CBI-100101050 [ PubMed ] [ CrossRef ] [ Google Scholar ]
- Vyazovskiy V. V., Palchykova S., Achermann P., Tobler I., Deboer T. (2017). Different effects of sleep deprivation and torpor on EEG slow-wave characteristics in djungarian hamsters. Cereb. Cortex 27 950–961. 10.1093/cercor/bhx020 [ PMC free article ] [ PubMed ] [ CrossRef ] [ Google Scholar ]
- Walker J. M., Glotzbach S. F., Berger R. J., Heller H. C. (1977). Sleep and hibernation in ground squirrels (Citellus spp): electrophysiological observations. Am. J. Physiol. Regul. Integr. Comp. Physiol. 233 R213–R221. 10.1152/ajpregu.1977.233.5.R213 [ PubMed ] [ CrossRef ] [ Google Scholar ]
- Walker J. M., Haskell E. H., Berger R. J., Heller H. C. (1981). Hibernation at moderate temperatures: a continuation of slow wave sleep. Experientia 37 726–728. 10.1007/BF01967947 [ PubMed ] [ CrossRef ] [ Google Scholar ]
- Wang D., He X., Zhao Z., Feng Q., Lin R., Sun Y., et al. (2015). Whole-brain mapping of the direct inputs and axonal projections of POMC and AgRP neurons. Front. Neuroanat. 9 : 40 . 10.3389/fnana.2015.00040 [ PMC free article ] [ PubMed ] [ CrossRef ] [ Google Scholar ]
- Watanabe T., Kajimura N., Kato M., Sekimoto M., Nakajima T., Hori T., et al. (2003). Sleep and circadian rhythm disturbances in patients with delayed sleep phase syndrome. Sleep 26 657–661. 10.1093/sleep/26.6.657 [ PubMed ] [ CrossRef ] [ Google Scholar ]
- Weber F., Dan Y. (2016). Circuit-based interrogation of sleep control. Nature 538 51–59. 10.1038/nature19773 [ PubMed ] [ CrossRef ] [ Google Scholar ]
- Weber F., Hoang Do J. P., Chung S., Beier K. T., Bikov M., Saffari Doost M., et al. (2018). Regulation of REM and Non-REM Sleep by Periaqueductal GABAergic Neurons. Nat. Commun. 9 : 354 . 10.1038/s41467-017-02765-w [ PMC free article ] [ PubMed ] [ CrossRef ] [ Google Scholar ]
- Williams D. R., Epperson L. E., Li W., Hughes M. A., Taylor R., Rogers J., et al. (2005). Seasonally hibernating phenotype assessed through transcript screening. Physiol. Genomics 24 13–22. 10.1152/physiolgenomics.00301.2004 [ PubMed ] [ CrossRef ] [ Google Scholar ]
- Williams K. W., Scott M. M., Elmquist J. K. (2009). From observation to experimentation: leptin action in the mediobasal hypothalamus. Am. J. Clin. Nutr. 89 985S–990S. 10.3945/ajcn.2008.26788D [ PMC free article ] [ PubMed ] [ CrossRef ] [ Google Scholar ]
- Wu Z., Autry A. E., Bergan J. F., Watabe-Uchida M., Dulac C. G. (2014). Galanin neurons in the medial preoptic area govern parental behaviour. Nature 509 325–330. 10.1038/nature13307 [ PMC free article ] [ PubMed ] [ CrossRef ] [ Google Scholar ]
- Yetish G., Kaplan H., Gurven M., Wood B., Pontzer H., Manger P. R., et al. (2015). Natural sleep and its seasonal variations in three pre-industrial societies. Curr. Biol. 25 2862–2868. 10.1016/j.cub.2015.09.046 [ PMC free article ] [ PubMed ] [ CrossRef ] [ Google Scholar ]
- Yu S., Cheng H., François M., Qualls-Creekmore E., Huesing C., He Y., et al. (2018). Preoptic leptin signaling modulates energy balance independent of body temperature regulation. Elife 7 : e33505 . 10.7554/eLife.33505 [ PMC free article ] [ PubMed ] [ CrossRef ] [ Google Scholar ]
- Yu S., Qualls-Creekmore E., Rezai-Zadeh K., Jiang Y., Berthoud H.-R., Morrison C. D., et al. (2016). Glutamatergic preoptic area neurons that express leptin receptors drive temperature-dependent body weight homeostasis. J. Neurosci. 36 5034–5046. 10.1523/JNEUROSCI.0213-16.2016 [ PMC free article ] [ PubMed ] [ CrossRef ] [ Google Scholar ]
- Yu X., Li W., Ma Y., Tossell K., Harris J. J., Harding E. C., et al. (2019). GABA and glutamate neurons in the VTA regulate sleep and wakefulness. Nat. Neurosci. 22 106–119. 10.1038/s41593-018-0288-9 [ PMC free article ] [ PubMed ] [ CrossRef ] [ Google Scholar ]
- Zhang Y., Kerman I. A., Laque A., Nguyen P., Faouzi M., Louis G. W., et al. (2011). Leptin-receptor-expressing neurons in the dorsomedial hypothalamus and median preoptic area regulate sympathetic brown adipose tissue circuits. J. Neurosci. 31 1873–1884. 10.1523/jneurosci.3223-10.2011 [ PMC free article ] [ PubMed ] [ CrossRef ] [ Google Scholar ]
- Zhang Z., Ferretti V., Guntan I., Moro A., Steinberg E. A., Ye Z., et al. (2015). Neuronal ensembles sufficient for recovery sleep and the sedative actions of alpha2 adrenergic agonists. Nat. Neurosci. 18 553–561. 10.1038/nn.3957 [ PMC free article ] [ PubMed ] [ CrossRef ] [ Google Scholar ]
- Zhao Z.-D., Yang W. Z., Gao C., Fu X., Zhang W., Zhou Q., et al. (2017). A hypothalamic circuit that controls body temperature. Proc. Natl. Acad. Sci. U.S.A. 114 : 2042 . 10.1073/pnas.1616255114 [ PMC free article ] [ PubMed ] [ CrossRef ] [ Google Scholar ]
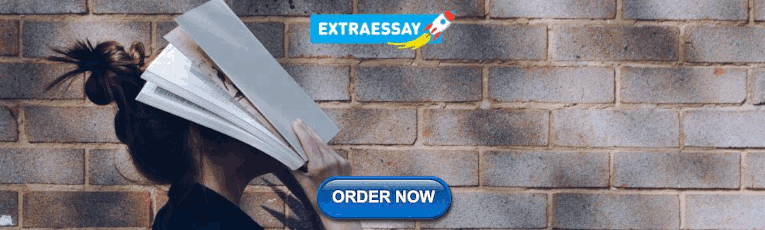
IMAGES
VIDEO
COMMENTS
Furthermore, research had shown that body temperature is a nonlinear function of several variables such as age, state of health, gender, environmental temperature, time of the diurnal cycle, among many others [9, 10]. To make the best use of the currently available literature, we reviewed and herein present an analysis of previously published ...
A body temperature drop. In recent years, Parsonnet's team has found that the average body temperature in the U.S. has dropped from 98.6 F by about 0.05 F every decade since the 19th century, likely due to better health and living conditions that reduce inflammation. They found that today's normal body temperature hovers closer to 97.9 F.
Since the 19 th century, the average human body temperature in the United States has dropped, according to researchers at the Stanford University School of Medicine. "Our temperature's not what people think it is," said Julie Parsonnet, MD, professor of medicine and of health research and policy."What everybody grew up learning, which is that our normal temperature is 98.6, is wrong."
Feed-forward and feedback regulation of body temperature. Body temperature is not a single value but varies depending on where it is measured. In studies of thermoregulation, it is common to divide the body into two compartments: (1) the external shell, which includes the skin and largely fluctuates in temperature along with the environment, and (2) the internal core, which includes the ...
Body temperature is a physiological response controlled by a thermoregulation set point programmed by the hypothalamic thermoreceptors (set point) and conditioned by the body's precise thermoregulatory systems. ... The research therefore confirms the validity of temperature measurement as a basic screening test for the detection of COVID-19. W.
A long tradition of research on human core body temperature, starting in the 19th century, has focused on establishing average temperature in a population. Temperature is known to be influenced by many factors that differ widely across patients (eg, age and circadian, metabolic, and ovulatory cycles) raising the possibility that individual ...
The inclusion criteria were as follows: (1) the paper presented data on measured normal body temperature of healthy human subjects ages 18 and older, (2) a prospective design was used, and (3) the paper was written in or translated into the English language. Thirty-six articles met the inclusion criteria. This comprised 9227 measurement sites ...
Core body temperature is one of the four key vital signs, which is regularly assessed by healthcare settings, alongside respiration rate, blood pressure and heart rate 1.In an in-patient setting ...
Most people probably grew up being told a body's normal temperature was 98.6 degrees Fahrenheit (or 37 degrees Celsius). That widely accepted number originated from a study done in the mid-1800s ...
Pexels. For decades, 98.6 degrees Fahrenheit has been the widely accepted "normal" average temperature for the human body. But new research adds to the growing body of evidence that humans ...
It is one of those facts of life that we learn early and don't forget: normal body temperature is 98.6 degrees Fahrenheit. But a new study in eLife argues that that number is outdated. The ...
The human body maintains a temperature of about 98.6°F (37°C) using various physical processes. These include sweating to lower the body temperature, shivering to raise it, and narrowing or ...
Normal human body temperature (normothermia, euthermia) is the typical temperature range found in humans. The normal human body temperature range is typically stated as 36.5-37.5 °C (97.7-99.5 °F). ... Several research papers show that its accuracy is similar to the invasive methods. Internal ...
considerable effort has been devoted to understanding the relationship between body temperature and human performance (2, 4, 12, 24, 34,48).Kleitman originally proposed that body temperature was an underlying mechanism regulating performance."Assuming that the effect of temperature indicates that we are dealing with a chemical phenomenon, there are two interpretations of the relationship ...
The body temperature data also showed a trend toward higher depression scores in people whose temperatures had less fluctuation throughout a 24-hour period, but this finding didn't reach significance. The findings shed light on how a novel depression treatment method might work, said Ashley Mason, PhD, the study's lead author and associate ...
Normal range of body temperature based on age. For a typical adult, body temperature can be anywhere from 97 F to 99 F. Adults over the age of 60 tend to have a lower body temperature, compared to ...
A healthy individual will have a core body temperature of 37 +/- 0.5°C (98.6 +/- 0.9°F), the temperature range needed for the body's metabolic processes to function correctly.[1] ... Research has demonstrated a considerable delay in rectal temperature results, especially during rapid temperature changes, and many things can alter a rectal ...
Researchers analyzed data from more than 20,000 international participants who wore a device that measures body temperature, and also self-reported their body temperatures and depression symptoms daily. The seven-month study began in early 2020 and included data from 106 countries.
"To our knowledge, this is the largest study to date to examine the association between body temperature - assessed using both self-report methods and wearable sensors ... Previous research has found that hot tubs and saunas can lessen the symptoms of depression, albeit in small sample groups. It's possible that the self-cooling this triggers ...
The researchers found that higher levels of depressive symptoms were consistently associated with higher body temperatures. This correlation was observed across both self-reported data and minute-level temperature data collected by the Oura Ring. This pattern suggests a robust relationship between elevated body temperature and the presence of ...
Abstract: The evaluation of thermoregulation in homeothermic animals is important for their health assessment. Body temperature is influenced by the circadian rhythm, which, through certain signals, is regulated by the suprachiasmatic nucleus. Temperature is collected by various methods; to reduce the invasiveness of rectal temperature sampling, considered the most accurate, infrared ...
More than 100 Canadian heat records were smashed on Wednesday alone, all while emerging research reveals the toll of high heat on the human body is far worse than previously thought.
High temperatures can make us miserable. Research shows they also make us aggressive, impulsive and dull. By Dana G. Smith In July 2016, a heat wave hit Boston, with daytime temperatures averaging ...
Although surface body temperature can be tolerated in a broad range and reach close to 0 °C in an extreme condition, the core body temperature is still maintained at the rational physiological set point around 37.0 °C by the thermoregulatory mechanisms. ... This study was supported in part by the Competitive Research Fund 2018-P-14 of the ...
According to the World Health Organization, snakebites are considered a high priority health concern, but research on the environmental risk factors involved is lacking. A Rollins study from last year found a link between a one degree increase in daily temperature highs and an increase in emergency department visits for snakebites from 2014 to ...
Dairy cattle body temperature and environmental data analysis. From July 2019 to April 2020, we collected data from 320 Holstein-Friesian cattle at a research dairy farm operated by the Council ...
When altricial birds hatch, they are unable to regulate their own temperature, but by the time they fledge they are thermally independent. Early-life conditions have been shown to be an important factor contributing to an individual's performance in adult life. However, it is currently unknown to what extent body temperature during endothermy development is driven by genetic variation or by ...
1. Introduction. Exertional heat-related illness (HRI) is increasing in incidence as global temperatures rise, with at least 9000 student athletes and 2000 military members affected in the US each year [1,2,3,4].The spectrum of illness of HRI ranges from heat-induced muscle cramps to life-threatening heat stroke, and a rising core body temperature (CBT) during exercise directly leads to HRI ...
The research team found providing artificial 'hot spot' shelters allows frogs to quickly "bake off" infections. The team built small shelters from readily available materials, such as bricks and PVC greenhouses, so frogs could regulate their body temperature and live in conditions that suppress the growth of the fungal pathogen.
The authors declare that the research was conducted in the absence of any commercial or financial relationships that could be construed as a potential conflict of interest. ... Core body temperature and sleep of older female insomniacs before and after passive body heating. Sleep 22 891-898. 10.1093/sleep/22.7.891 [Google ...