Students and Their Computer Literacy: Evidence and Curriculum Implications
- Reference work entry
- First Online: 10 August 2018
- Cite this reference work entry
- John Ainley 5
Part of the book series: Springer International Handbooks of Education ((SIHE))
4853 Accesses
7 Citations
For a number of years, education authorities have responded to the importance of school students developing computer literacy by including it as part of the school curriculum, directly as a cross-curriculum capability, and by assessing the extent to which students are computer literate. Computer literacy and related concepts, such as ICT literacy, are defined so as to include both technological expertise and information literacy. Assessments of computer literacy, even though they vary, indicate that there are substantial variations in levels of computer literacy among students in the lower years of secondary school. In technologically developed countries, approximately one half of Year 8 students demonstrate proficiency, or advanced proficiency, in computer literacy, but up to 10% have very limited computer literacy. Assessments of computer literacy can also provide the basis for progression maps that could be used to inform curriculum development. Those progression maps will be more valuable if the frameworks on which they are based become more strongly integrated with each other. In addition, computer literacy appears to be influenced by student background, including familiarity with computers, as well as the emphases placed on it in classrooms and schools and the support provided by ICT in education systems. At present, there is less information about school and classroom influences on computer literacy than there is about student background influences. In the immediate future, the construct of computer literacy may need to accommodate increasingly to changes in software and hardware contexts in which it is manifested.
This is a preview of subscription content, log in via an institution to check access.
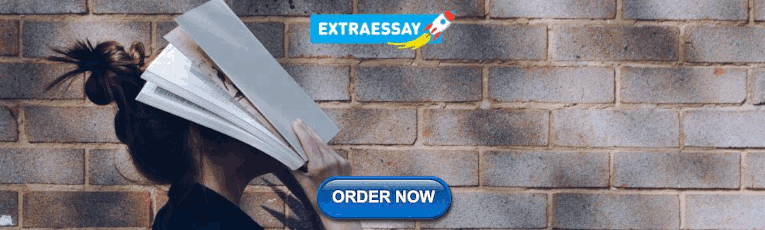
Access this chapter
Subscribe and save.
- Get 10 units per month
- Download Article/Chapter or eBook
- 1 Unit = 1 Article or 1 Chapter
- Cancel anytime
- Available as PDF
- Read on any device
- Instant download
- Own it forever
- Available as EPUB and PDF
- Durable hardcover edition
- Dispatched in 3 to 5 business days
- Free shipping worldwide - see info
Tax calculation will be finalised at checkout
Purchases are for personal use only
Institutional subscriptions
Similar content being viewed by others
The Influence of Information and Communication Technology Use on Students’ Information Literacy
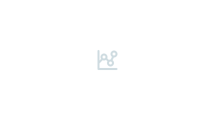
ICT Literacy: An Imperative of the Twenty-First Century
Aesaert, K., van Nijlen, D., Vanderlinde, R., & van Braak, J. (2014). Direct measures of digital information processing and communication skills in primary education: Using item response theory for the development and validation of an ICT competence scale. Computers & Education, 76 , 168–181. https://doi.org/10.1016/j.compedu.2014.03.013 .
Article Google Scholar
Aesaert, K., van Braak, J., van Nijlen, D., & Vanderlinde, R. (2015). Primary school pupils ICT competences: Extensive model and scale development. Computers & Education, 81 , 326–344. https://doi.org/10.1016/j.compedu.2014.10.021 .
Ainley, J., Schulz, W., Fraillon, J., & Gebhardt, E. (2016). Conceptualizing and measuring computer and information literacy in cross-national contexts. Applied Measurement in Education, 29 (4), 291–309. https://doi.org/10.1080/08957347.2016.1209205 .
Australian Council for Educational Research (ACER). (2016). A global measure of digital and ICT literacy skills. Background paper prepared for the 2016 Global Education Monitoring Report: Education for people and planet: Creating sustainable futures for all . Paris: UNESCO. ED/GEMR/MERT/2016/P1/4. http://unesdoc.unesco.org/images/0024/002455/245577e.pdf .
Australian Curriculum and Reporting Authority (ACARA). (2012). National Assessment Program – ICT literacy years 6 & 10 2011 report . Sydney: Australian Curriculum and Reporting Authority (ACARA). Available: http://www.nap.edu.au/_resources/NAP_ICTL_2011_Public_Report_Final.pdf .
Google Scholar
Australian Curriculum and Reporting Authority (ACARA). (2015). National Assessment Program – ICT literacy years 6 & 10 2014 report . Sydney: Australian Curriculum and Reporting Authority (ACARA). Available: http://www.nap.edu.au/_resources/D15_8761__NAP-ICT_2014_Public_Report_Final.pdf .
Australian Curriculum and Reporting Authority (ACARA). (2017). Information and communication technology (ICT) capability . Sydney: Australian Curriculum and Reporting Authority (ACARA). Available: http://v7-5.australiancurriculum.edu.au/GeneralCapabilities/Pdf/ICT .
Barr, D., Harrison, J., & Conery, L. (2011). Computational thinking: A digital age skill for everyone. Learning and Leading with Technology, 38 (6), 20–23.
Bennett, S., Maton, K., & Kervin, L. (2008). The “digital natives” debate: A critical review of the evidence. British Journal of Educational Technology, 39 (5), 775–786.
Binkley, M., Erstad, E., Herman, J., Raizen, S., Ripley, M., Miller-Ricci, M., et al. (2012). Defining 21st century skills. In P. Griffin, B. McGaw, & E. Care (Eds.), Assessment and teaching of 21st century skills . Dordrecht: Springer.
Catts, R., & Lau, J. (2008). Towards information literacy indicators . Paris: UNESCO.
Claro, M., Preiss, D., San Martin, E., Jara, I., Hinostraoza, J. E., Valenzuela, S., Cortes, F., & Nussbaum, M. (2012). Assessment of 21st century ICT skills in Chile: Test design and results from high school level students. Computers and Education, 59 , 1042–1053. https://doi.org/10.1016/j.compedu.2012.04.004 .
De Bortoli, L., Buckley, S., Underwood, C., O’Grady, E., & Gebhardt, E. (2014). ICILS 2013: Australian students’ readiness for study, work and life in the digital age . Melbourne: ACER. http://research.acer.edu.au/ict_literacy/6 .
Dexter, S. (2008). Leadership for IT in schools. In J. Voogt & G. Knezek (Eds.), International handbook of information technology in primary and secondary education (Vol. 2, pp. 543–554). New York: Springer.
Chapter Google Scholar
Educational Testing Service (ETS), International ICT Literacy Panel. (2002). Digital transformation – A framework for ICT literacy . Princeton: Educational Testing Service.
E-learning Nordic. (2006). E-learning Nordic 2006: The impact of ICT on education . Denmark: Ramboll Management.
European Commission. (2008). Digital Literacy European Commission working paper and recommendations from Digital Literacy High-Level Expert Group . Retrieved 21 Oct 2010 from http://www.ifap.ru/library/book386.pdf .
European Commission. (2010). Digital competence: Identification and European-wide validation of its key components for all levels of learners (DIGCOMP) . Retrieved 26 Nov 2012, from http://is.jrc.ec.europa.eu/pages/EAP/DIGCOMP.html .
European Commission, Joint Research Centre-IPTS on behalf of DG Education and culture. (2013). DIGCOMP: A framework for developing and understanding digital competence in Europe . Retrieved from http://ftp.jrc.es/EURdoc/JRC83167.pdf .
Ferrari, A. (2012). Digital competence in practice: An analysis of frameworks . Institute for Prospective Technological Studies. Retrieved 5 Feb 2013 from http://ipts.jrc.ec.europa.eu/publications/pub.cfm?id=5099 .
Fraillon, J., Schulz, W., & Ainley, J. (2013). International computer and information literacy study assessment framework . Amsterdam: International Association for the Evaluation of Educational Achievement (IEA). Retrieved from http://www.iea.nl/fileadmin/user_upload/Publications/Electronic_versions/ICILS_2013_Framework.pdf .
Fraillon, J., Ainley, J., Schulz, W., Friedman, T., & Gebhardt, E. (2014). Preparing for life in a digital age. The IEA international computer and literacy information study international report . Heidelberg: Springer Cham.
Griffin, P., McGaw, B., & Care, E. (2012). Assessment and teaching of 21st century skills . Dordrecht: Springer.
Book Google Scholar
Grover, S., & Pea, R. (2013). Computational thinking in K–12: A review of the state of the field. Educational Researcher, 42 (1), 38–43. https://doi.org/10.3102/0013189X12463051 .
Hatlevik, O. E., Ottestad, G., & Throndsen, I. (2015). Predictors of digital competence in 7th grade: A multilevel analysis. Journal of Computer Assisted Learning, 31 , 220–231.
Helsper, E., & Eynon, R. (2010). Digital natives: Where is the evidence? British Educational Research Journal, 36 (3), 503–520. https://doi.org/10.1080/01411920902989227 .
Hinostroza, J. E., Hepp, P., & Cox, C. (2008). Policies and practices on ICT in education in Chile: Enlaces. In T. Plomp, R. E. Anderson, N. Law, & A. Quale (Eds.), Cross-national policies and practices on information and communication technology in education (Revised ed.). Greenwich: Information Age Publishing.
Institute of Education Sciences, National Center for Education Statistics. (2012). National Assessment of Educational Progress (NAEP) technology and engineering literacy (TEL) assessment . Retrieved on 8 Feb 2013 from http://nces.ed.gov/nationsreportcard/tel/ .
Katz, I. R. (2007). Testing information literacy in digital environments: ETS’s iSkills assessment. Information Technology and Libraries, 26 (3), 3–12.
Kim, J., & Lee, W. (2013). Meanings of criteria and norms: Analyses and comparisons of ICT literacy competencies of middle school students. Computers & Education, 64 , 81–94. https://doi.org/10.1016/j.compedu.2012.12.018 .
Kim, H. S., Kil, H. J., & Shin, A. (2014). An analysis of variables affecting the ICT literacy level of Korean elementary school students. Computers & Education, 77 , 29–38. https://doi.org/10.1016/j.compedu.2014.04.009 .
Koutropoulos, A. (2011). Digital natives: Ten years after. MERLOT Journal of Online Learning and Teaching, 7 (4). Retrieved from http://jolt.merlot.org/vol7no4/koutropoulos_1211.htm .
Kozma, R. (Ed.). (2003). Technology, innovation, and educational change: A global perspective . Eugene: ISTE.
Lye, S. Y., & Koh, J. H. L. (2014). Review on teaching and learning of computational thinking through programming: What is next for K-12? Computers in Human Behavior, 41 , 51–61. https://doi.org/10.1016/j.chb.2014.09.012 .
Madaus, G., & O’Dwyer, L. M. (1999). A short history of performance assessment: Lessons learned. The Phi Delta Kappan, 89 (9), 688–695.
Markauskaite, L. (2006). Towards an integrated analytical framework of information and communications technology literacy: From intended to implemented and achieved dimensions. Information Research, 11 (3) paper 252. Available at http://InformationR.net/ir/11-3/paper252.html .
McDougall, A., Murnane, J. S., & Wills, S. (2014). The educational programming language logo: Its nature and its use in Australia. In A. Tatnall & B. Davey (Eds.), Reflections on the history of computers in education (IFIP advances in information and communication technology, Vol. 424). Berlin: Springer.
Ministerial Council on Education, Employment, Training and Youth Affairs (MCEETYA). (2005). National Assessment Program Information and Communication Technology literacy years 6 and 10 an assessment domain for ICT literacy . Carlton: Curriculum Corporation.
Ministerial Council on Education, Employment, Training and Youth Affairs (MCEETYA). (2007). National Assessment Program – ICT literacy years 6 & 10 2005 report . Carlton: Curriculum Corporation.
Ministerial Council on Education, Employment, Training and Youth Affairs (MCEETYA). (2008). Melbourne declaration on educational goals for young Australians . Carlton: Curriculum Corporation.
Moos, D., & Azevedo, R. (2009). Learning with computer-based learning environments: A literature review of computer self-efficacy. Review of Educational Research, 79 (2), 576–600.
National Center for Educational Statistics (NCES). (2016a). 2014 Abridged technology and engineering literacy framework for the 2014 National Assessment of educational progress . Washington, DC: National Assessment Governing Board.
National Center for Educational Statistics (NCES). (2016b). 2014 Nations Report Card: Technology & Engineering Literacy (TEL). https://www.nationsreportcard.gov/tel_2014/ .
Organisation for Economic Cooperation and Development (OECD). (2011). PISA 2009 results: Students on-line digital technologies and performance . Paris: OECD.
Peyton Jones, S. (2011). Computing at school: International comparisons . London: Microsoft Research.
Prensky, M. (2001). Digital natives, digital immigrants. On the Horizon, 9 (5), 1–6.
Qualifications and Curriculum Authority. (2007). About information and communication technology: Assessment guidance. Retrieved 7 Apr 2007 from http://www.qca.org.uk/7889.html .
Rohatgi, A., Scherer, R., & Hatlevik, O. (2016). The role of ICT self-efficacy for students’ ICT use and their achievement in a computer and information literacy test. Computers & Education, 102 , 103–116.
Sánchez, J., & Salinas, A. (2008). ICT & learning in Chilean schools: Lessons learned. Computers & Education, 51 (4), 1621–1633.
Selwyn, N. (2009). The digital native – myth and reality. Aslib Proceedings: New Information Perspectives, 61 (4), 364–379.
Senkbeil, M., Ihme, J. M., & Wittwer, J. (2013). The Test of Technological and Information Literacy (TILT) in the National Educational Panel Study: Development, empirical testing, and evidence for validity. Journal for Educational Research Online, 5 (2), 139–161.
Siddiq, F., Hatlevik, O. E., Olsen, R. V., Throndsen, I., & Scherer, R. (2016). Taking a future perspective by learning from the past - A systematic review of assessment instruments that aim to measure primary and secondary school students’ ICT literacy. Educational Research Review, 19 , 58–84.
Sturman, L., & Sizmur, J. (2011). International comparison of computing in schools . Slough: NFER.
United States Department of Education Office of Educational Technology. (2010). Transforming American education powered by technology: National Education Technology Plan 2010 . Washington, DC: United States Department of Education Office of Educational Technology.
Van Deursen, A. J., & Van Dijk, J. A. (2016). Modelling traditional literacy, internet skills and internet usage: An empirical study. Interacting with Computers, 28 (1), 13–26. https://doi.org/10.1093/iwc/wu027 .
Download references
Author information
Authors and affiliations.
Australian Council for Educational Research, Camberwell, Australia
John Ainley
You can also search for this author in PubMed Google Scholar
Corresponding author
Correspondence to John Ainley .
Editor information
Editors and affiliations.
University of Amsterdam, Amsterdam, The Netherlands
University of North Texas, Denton, Texas, USA
Gerald Knezek
Rhonda Christensen
University of Otago, Dunedin, New Zealand
Kwok-Wing Lai
Section Editor information
Department of Child Development and Education, University of Amsterdam, Amsterdam, The Netherlands
University of Oslo, Oslo, Norway
Rights and permissions
Reprints and permissions
Copyright information
© 2018 Springer International Publishing AG, part of Springer Nature
About this entry
Cite this entry.
Ainley, J. (2018). Students and Their Computer Literacy: Evidence and Curriculum Implications. In: Voogt, J., Knezek, G., Christensen, R., Lai, KW. (eds) Second Handbook of Information Technology in Primary and Secondary Education . Springer International Handbooks of Education. Springer, Cham. https://doi.org/10.1007/978-3-319-71054-9_4
Download citation
DOI : https://doi.org/10.1007/978-3-319-71054-9_4
Published : 10 August 2018
Publisher Name : Springer, Cham
Print ISBN : 978-3-319-71053-2
Online ISBN : 978-3-319-71054-9
eBook Packages : Education Reference Module Humanities and Social Sciences Reference Module Education
Share this entry
Anyone you share the following link with will be able to read this content:
Sorry, a shareable link is not currently available for this article.
Provided by the Springer Nature SharedIt content-sharing initiative
- Publish with us
Policies and ethics
- Find a journal
- Track your research
An IERI – International Educational Research Institute Journal
- Open access
- Published: 23 October 2020
The relationship between differences in students’ computer and information literacy and response times: an analysis of IEA-ICILS data
- Melanie Heldt 1 ,
- Corinna Massek 2 ,
- Kerstin Drossel 1 &
- Birgit Eickelmann 1
Large-scale Assessments in Education volume 8 , Article number: 12 ( 2020 ) Cite this article
4102 Accesses
3 Citations
14 Altmetric
Metrics details
Due to the increasing use of information and communication technology, computer-related skills are important for all students in order to participate in the digital age (Fraillon, J., Ainley, J., Schulz, W., Friedman, T. & Duckworth, D. ( 2019 ). Preparing for life in a digital world: IEA International Computer and Information Literacy Study 2018 International Report. Amsterdam: International Association for the Evaluation of Educational Achievement (IEA). Retrieved from https://www.iea.nl/sites/default/files/2019-11/ICILS%202019%20Digital%20final%2004112019.pdf ). Educational systems play a key role in the mediation of these skills (Eickelmann. Second Handbook of Information Technology in Primary and Secondary Education. Cham: Springer, 2018 ). However, previous studies have shown differences in students’ computer and information literacy (CIL). Although various approaches have been used to explain these differences, process data, such as response times, have never been taken into consideration. Based on data from the IEA-study ICILS 2013 of the Czech Republic, Denmark and Germany, this secondary analysis examines to what extent response times can be used as an explanatory approach for differences in CIL also within different groups of students according to student background characteristics (gender, socioeconomic background and immigrant background).
First, two processing profiles using a latent profile analysis (Oberski, D. ( 2016 ). Mixture Models: Latent Profile and Latent Class Analysis. In J. Robertson & M. Kaptein (Eds.), Modern Statistical Methods for HCI (pp. 275–287). Switzerland: Springer. https://doi.org/10.1007/978-3-319-26633-6 ) based on response times are determined—a fast and a slow processing profile. To detect how these profiles are related to students’ CIL, also in conjunction with students’ background characteristics (socioeconomic and immigrant background), descriptive statistics are used.
The results show that in the Czech Republic and Germany, students belonging to the fast processing profile have on average significantly higher CIL than students allocated to the slow processing profile. In Denmark, there are no significant differences. Concerning the student background characteristics in the Czech Republic, there are significant negative time-on-task effects for all groups except for students with an immigrant background and students with a high parental occupational status. There are no significant differences in Denmark. For Germany, a significant negative time-on-task effect can be found among girls. However, the other examined indicators for Germany are ambiguous.
Conclusions
The results show that process data can be used to explain differences in students’ CIL: In the Czech Republic and Germany, there is a correlation between response times and CIL (significant negative time-on-task effect). Further analysis should also consider other aspects of CIL (e.g. reading literacy). What becomes clear, however, is that when interpreting and explaining differences in competence, data should also be included that relates to the completion process during testing.
Introduction
Seeing how digitalization is becoming a more and more integral part of social and professional environments, competence in new technologies is becoming increasingly important for students (Fraillon et al. 2014 , 2019 ; Gerick et al. 2017 ; Gerick 2018 ). In this context, the acquisition of computer and information literacy (CIL) as an interdisciplinary key competence is of particular relevance (Eickelmann 2018 ). However, empirical findings show differences in CIL between different groups of students when students’ background characteristics (gender, socioeconomic status, immigrant background) are taken into account (summarizing Aesaert and van Braak 2018 ; Fraillon et al. 2014 , 2019 ). So far, predictors such as computer-based self-efficacy, computer use or computer experience have been used to explain these differences (Hatlevik et al. 2015 ; Luu and Freeman 2011 ; Punter et al. 2017 ). Besides determining these predictors of CIL, computer-based testing in the context of large-scale assessment studies also opens up possibilities to gather data on processing behaviour during testing—the so-called process data, such as response times, which can be used to model competencies and explain individual differences during testing (summarizing Goldhammer et al. 2017 ). Despite the potential to explain differences in competence among students, process data, such as response times, has never been used as an explanation regarding differences in students’ CIL. The present examination takes up this desideratum.
Following an overview of the theoretical classification, the state of research on differences in CIL and on the role of process data, a secondary analysis will be provided and include representative student data from the IEA-study ICILS 2013 (International Computer and Information Literacy Study; Fraillon et al. 2014 ) carried out in three Western European countries (the Czech Republic, Denmark and Germany) in which the students performed differently in CIL. This analysis is done to investigate the extent to which students’ response times during testing could be used as an explanation for differences in CIL and how CIL differs within different groups of students according to the response times. After the presentation of the results, they will be discussed and an outlook on future research will be given.
Theoretical background and state of research
Cil and student background characteristics—theoretical background.
The ICILS 2013 framework model can be used as a theoretical model to locate CIL and student background characteristics such as gender, socioeconomic background and immigrant background (Fraillon et al. 2014 ). This framework model distinguishes between the learning antecedents and learning processes with regard to the learning outcomes and thus, the computer and information literacy of the students (Fraillon et al. 2014 ). The present model, therefore, represents a classic input-process-output model. It further assumes that the features or predictors at the antecedent level (input) directly affect the learning processes (process). These learning processes, in turn, are assumed to correlate with students' CIL – the learning outcomes (output)—and thus, have an impact on competences and are influenced by competences (Fraillon et al. 2014 ). Figure 1 shows a graphic representation of the abovementioned model.
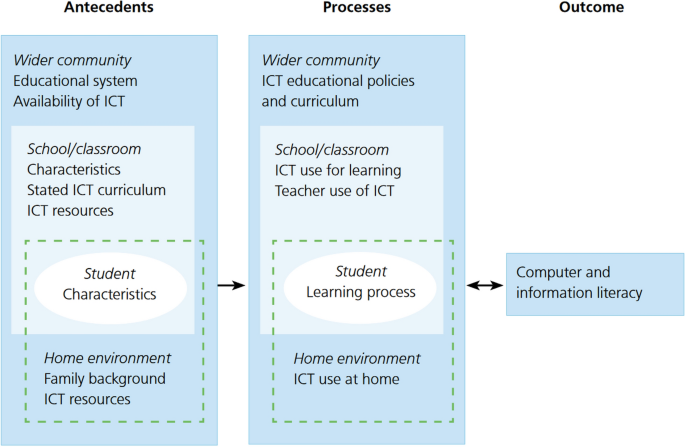
ICILS 2013 Framework Model (Fraillon et al. 2014 )
The described model is used for the present analysis in order to be able to locate the student background characteristics at an input level (Fraillon et al. 2014 ). At the same time, the student competences (CIL) can be located at the outcome level. What is unaccounted for in the model but relevant for the present research is what takes place at the process level within the framework of competence testing. For this reason, an additional model of theoretical localisation will be used in the course of this article.
CIL and student background characteristics—state of research
The empirical findings regarding gender differences in digital literacy are not clear. For example, some studies show a performance advantage in favour of boys (Goldhammer et al. 2013 ; Morris and Trushell 2014 ). Among others, the so-called COMPED study (Computer in Education Study), which was carried out internationally by the IEA at the beginning of the 1990s and determined the skills of fifth and eighth graders in dealing with new technologies by means of a competence test, can be listed here (Pelgrum et al. 1993 ). The students’ competences were determined by means of standardized paper-based tests, the contents of which were designed to learn about students’ application knowledge and knowledge about the use of computers (Pelgrum et al. 1993 ). The results showed that boys had on average a higher skill level than girls in all participating countries (Pelgrum et al. 1993 ).
In contrast to studies that show a performance advantage in favour of boys, like the COMPED study, other studies do not indicate any gender differences at all (e.g., Punter et al. 2017 ). For example, in a study in Norway, in which more than 4.000 seventh graders were tested using a web-based module to determine their ‘digital competence’, no differences were identified between girls and boys (Hatlevik and Christophersen 2013 ).
In turn, other studies found that female students had on average a higher skill level than boys (ACARA 2015 ; Aesaert and van Braak 2015 ; Fraillon et al. 2014 , 2019 ; Gebhardt et al. 2019 ; Thomson 2015 ). In Australia, for instance, it was observed that girls in sixth and tenth grade classes displayed a higher computer and information literacy than boys (ACARA 2015 , 2018 ). Similar results can also be found in the US. In 2013, the ‘technology literacy’ of 1.300 eighth graders in the US was tested by means of a web-based performance test. The study’s results showed the girls performed better than the boys (Hohlfeld et al. 2013 ). Furthermore, the gap between the average girls’ and boys’ achievement levels increased between the two cycles (ACARA 2015 , 2018 ). In a study of 378 sixth graders from 58 different elementary schools in Flanders, Aesaert and van Braak ( 2015 ), it was discovered, by means of a proficiency test on information and communication technology (ICT) skills, that girls have on average higher skills than boys. The ICILS 2013 and ICILS 2018 studies were also able to identify significantly higher levels of computer and information literacy skills for eighth-grade girls in comparison to the boys in all participating countries by using a computer-based proficiency test (Fraillon et al. 2014 , 2019 ). Through secondary analysis using the ICILS 2013 international database and subscales, Punter et al. ( 2017 ) were also able to support the hypothesis that the girls outperformed the boys in the overall results and discovered performance differences in that computer-related skills were more in favour of boys and information-related skills were more in favour of girls.
A potential reason for the hitherto ambiguous findings with regard to the connection between gender and digital literacy described above could thus be the use of different constructs with which the mentioned studies (e.g. PISA 2009, COMPED, ACARA, ICILS) assess the students’ ICT skills. An explanation for varying results between different countries could be the manifold ways in which different school systems foster ICT skills. In addition, a distinction must be made between self-assessed and actually measured competences (Hatlevik et al. 2017 ) as studies are often not based on valid competence assessment but on self-assessed skills.
Regarding the socioeconomic background of the students, empirical findings also point to differences. In comparison to gender, these differences are more consistent. Empirical evidence suggests that students from more privileged families display higher digital competencies than those from less privileged homes. For example, studies have identified a link between the socioeconomic background of students and their acquired competencies concerning the use of computers and the internet (Zhong 2011 ; Zillien and Hargittai 2009 ). Studies also show that students from less privileged families only possess basic skills in using new technologies (Aesaert and van Braak 2015 ; Fraillon et al. 2014 , 2019 ; Thomson, 2015 ). Furthermore, some reports point to a positive correlation between cultural capital and computer and information literacy (Fraillon et al. 2019 ; Hatlevik et al. 2015 ). Students whose parents have the highest occupational status have significantly higher digital competences than students whose parents have the lowest occupational status (Fraillon et al. 2019 ; Thomson 2015 ). The ACARA (Australian Curriculum Assessment and Reporting Authority) study shows similar findings for Australia. Among sixth and tenth graders whose parents have both a higher occupational status and level of education, higher competencies in using information and communication technologies have been identified in comparison to their classmates, whose parents have both a lower occupational status and level of education (ACARA 2018 ).
Similar findings exist concerning the immigrant background of students. In terms of access to and the use of computers, studies can be found that reveal no or only minor differences among students with an immigrant background (Bonfadelli et al. 2007 ; D'Haenens 2003 ); however, empirical findings show that students without an immigrant background have higher digital competences than those with an immigrant background (Fraillon et al. 2019 ; Luu and Freeman 2011 ). In addition to the parents’ country of origin, the language spoken at home can be another indicator for an immigrant background: Study findings suggest that the language spoken at home influences school performance (summarized by Fraillon et al. 2019 ). This is related to the fact that students from immigrant families often do not have sufficient knowledge of the language of instruction (Fraillon et al. 2019 ). A connection between the language spoken at home and CIL has also been found in ICILS 2013 and 2018 (Fraillon et al. 2014 , 2019 ). The findings of ICILS 2013 and 2018 for Germany also indicate that students whose families speak the test language in the home environment attain higher computer and information literacy than students who speak a different language at home (Fraillon et al. 2014 , 2019 ). In contrast, the results of the ACARA study for Australia show that sixth-grade students who speak a language other than the test language at home have significantly a higher skill level in using information and communication technologies. For the tenth graders, however, there is no significant difference (ACARA 2018 ). At the same time, tenth grade students who were born in Australia demonstrate a higher skill level than their classmates who were born abroad (ACARA 2018 ).
Predictors such as computer-based self-efficacy, computer experience and students’ computer use have often been taken into consideration in order to explain such differences in CIL (Hatlevik et al. 2018 ; Livingstone and Helpster 2010 ; Rohatgi et al. 2016 ).
The role of response times—theoretical background
In addition to the abovementioned predictors (e.g. computer-based self-efficacy, computer experience and computer use), computer-based testing in the context of large-scale assessment enables the determination of process data such as response times, which can describe individual behavioural differences during the process of task completion and thereby task success (summarizing Goldhammer et al. 2017 ). Although process data, such as response times (the time taken to complete a task), allows the use of personal differences in behaviour as an explanatory approach for competency modelling, it can also be used to explain performance differences in this context (ibid.).
Since the process data is not explicitly considered in the already presented ICILS model, another model according to Naumann ( 2012 ) is also used to theoretically embed the presented analysis. This model also represents a type of input-process-output model. In this framework model it is assumed that the completion process during testing (process), which is influenced by person-level characteristics and task-level characteristics (input), has a direct influence on the result of task completion (output) (Goldhammer et al. 2017 ; Naumann 2012 ). Figure 2 shows a graphic representation of the model.
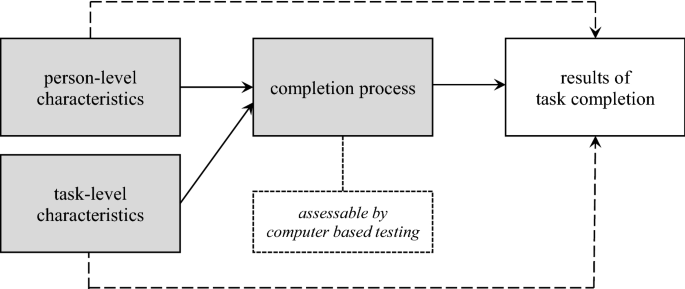
modified by Goldhammer ( 2013 )
Theoretical Model based on Naumann ( 2012 )
Against the background of the ICILS model described above, both theoretical models can be merged for the present work: at the input level, it is possible to locate the student background characteristics which, in the context of the present analysis, are assumed to have a direct influence on the level of the completion process in which the response times are located. From the level of the completion process, a direct influence on the results of task completion can be assumed. Here again, parallels to the ICILS model can be seen, from which, in turn, the reciprocal relationship between the process level and the output level can be adapted in the course of further analyses.
The role of response times—state of research
Empirical findings indicate correlations between response times and the processing success. These relationships can also be referred to as "time-on-task effects" where positive “time-on-task effects” (long response times with high processing success) and negative “time-on-task effects” (long response times with low processing success) can be distinguished (Goldhammer et al. 2014 , 2017 ; Naumann and Goldhammer 2017 ). With regard to problem-solving tasks in PIAAC (Programme for the International Assessment of Adult Competencies), Goldhammer et al. ( 2014 ) determined positive "time-on-task effects" as well as negative “time-on-task effects” (Goldhammer et al. 2014 ). In PIAAC, they used “a specific concept of problem solving […]; it refers to solving problems in technology-rich environments” (Goldhammer et al. 2014 , p. 10). The study by Stelter et al. ( 2015 ), for example, used data from the PIAAC study and built upon the research of Goldhammer et al. ( 2014 ). They analysed the specific part of the time spent on basic subtasks of PIAAC problem-solving tasks which could be solved through automated cognitive processing (Goldhammer et al. 2017 ; Stelter et al. 2015 ). The concept behind this study was that as soon as basic subtasks in problem-solving tasks were performed through automated processing, cognitive skills became available and therefore, benefited task processing and thus, the processing success (Goldhammer et al. 2017 ). As a result, negative “time-on-task effects” could be determined for problem-solving tasks. For reading tasks, there were correlations between response times as well as the results. Thus, positive as well as negative “time-on-task effects” could be determined during reading tasks (Goldhammer et al. 2014 ; Su 2017 ). Even within one study, different effects could be detected: firstly, a positive “time-on-task-effect” for slow digital readers in difficult tasks and tasks with high navigation requirements was identified. At the same time, a negative “time-on-task” effect could be detected for simple tasks with low navigation requirements (Naumann and Goldhammer 2017 ).
Research desideratum and research questions
Despite the abovementioned potential of process data, such as response times for analysing behaviour during testing to explain differences in competence, there is a lack of research on the extent to which response times can explain differences in students’ computer and information literacy (CIL).
Therefore, the present analysis focuses on the following research questions:
1. How do the response times relate to the CIL of students in Denmark, Germany and the Czech Republic?
2. How does CIL differ in terms of response times within different groups of students according to students’ background characteristics (gender, socioeconomic background and immigrant background)?
Data and methods
For the present secondary analysis, the representative student data of the Czech Republic (N = 3.066), Denmark (N = 1.767) and Germany (N = 2.225) from the IEA-study ICILS 2013 (Fraillon et al. 2015 ) is used. The uniqueness of the ICILS 2013 study is that students’ competencies in using information and communication technologies, or their computer and information literacy (CIL), could be assessed by means of computer-based performance tests for the first time (Fraillon et al. 2014 ). In addition to competence testing, the students took part in a written survey in which among others background information about the students such as gender, socioeconomic and immigrant background and other contextual information could be recorded. In addition to the framework concept which was based on the literacy concept, test instruments were developed for the survey to allow a computer-based determination of CIL.
Country selection
The country selection incorporated Western European countries in which student performance in CIL differed. As Germany was in the middle of the international field in terms of students’ CIL in ICILS 2013 ( M = 523 points, SE = 2.40), the Czech Republic has also been used as a reference country, since it was one of the top performers in the study ( M = 553 points, SE = 2.10). Students in Denmark ( M = 542 points, SE = 3.50), on the other hand, performed worse than students in the Czech Republic, but better than students in Germany.
Firstly, the so-called timing-items are used and represent the response times (in seconds) for the individual test tasks distributed over the four modules for each student. Student background characteristics (gender, socioeconomic background and immigrant background), collected through the questionnaires, are also utilised for the present analysis of the timing items. The gender is operationalized by the question 'Are you a girl or a boy?'.
Previous analyses of the ICILS 2013 data (e.g. Hatlevik et al. 2018 ) also show that the cultural capital determined by the number of books in the household can be used as an indicator of the socioeconomic background. For this reason, this indicator is also used in this paper (high cultural capital = more than 100 books; low cultural capital = 100 books or less). Furthermore, the occupation of the parents is operationalised in context of the International Socio-Economic Index of Occupational Status (ISEI; Ganzeboom et al. 1992 ). According to this indicator, low values suggest a low socio-economic background and high values a high socio-economic background. Therefore, the following groups are formed consistent with previous ICILS 2013 analyses (e.g. Fraillon et al. 2014 ): low parental occupational status (less than 40 points), medium parental occupational status (40 to 59 points) and high parental occupational status (60 points or more).
The immigrant background is, on the one hand, represented by the language spoken at home whereby, a distinction is made whether the test language is a language used in the home environment or another language. On the other hand, the immigrant background is represented by the parents’ country of birth. This has resulted in the following categories: no parent born abroad, one parent born abroad and both parents born abroad. An overview of the student background characteristics and the corresponding computer and information literacy distribution is shown in Table 1 .
In addition to the background characteristics and the timing-items, the five plausible values of the performance test which map CIL are used for further analysis. Furthermore, the student weight is included in the analysis.
The selected timing-items were also prepared for the further analysis with the so-called z-score standardization. Due to the nature of the data, direct comparability is not possible. The data must, therefore, first be prepared in such a way that the available response times for the respective tasks can be compared. To calculate the z-scores, the average of all values must be subtracted from each value before dividing it by the standard deviation. This calculation is depicted in the following formula (Mohamad and Usman 2013 , p. 3300):
By default, the variable that exists after the z-score standardization always has a mean value of 0 and a standard deviation of 1 (for example Mohamad and Usman 2013 ).
As a first step to make students’ response times more tangible and comparable, a latent profile analysis (LPA; Oberski 2016 ) using the software Mplus (Muthén and Muthén 2012 ) is carried out to identify possible processing profiles. The student weight is also used for the complete analysis to approximate the sample to the population and thus, to prevent possible distortions in the results (Jung and Carstens 2015 ). To answer the research questions, descriptive statistics are applied using the processing profiles to determine the extent to which differences in CIL can be explained by response times. In addition, descriptive statistics are used to measure how CIL varies in terms of response times within different groups of students due to student background characteristics. This is done using the IEA-IDB-Analyzer (Mirazchiyski 2015 ).
Results RQ1: processing profiles and CIL
Based on the parsimony principle, the interpretability, the mean class membership probabilities and the entropy value as criteria for evaluating the model quality (cf. Nylund et al. 2007 ; Tein et al. 2013 ), two profiles could be determined while profiles three to six are irrelevant given the figures related to the profile size and the associated interpretability shown in the table (cf. Table 2 ).
The first profile can be labelled as the "fast processing profile". This profile includes 81.07% of the students. The second profile, which can also be referred to as the "slow processing profile", only accounts for 18.93 percent of the students. While students in the first profile completed the tasks on average at a faster pace, the students in the second profile needed on average more time to complete the tasks. Exceptions are only found in so-called authoring tasks, also referred to as "big tasks"; each test module contains one (Fraillon et al. 2014 , 2019 ). In comparison to the other tasks (i.e. multiple choice) of the respective test module, an authoring task is a more complex task type as information products (i.e. a presentation) have to be created by the test participants (Fraillon et al. 2014 , 2019 ). The students who work on average at a faster pace need more time for these specific tasks. Students allocated to the slow processing profile, on the other hand, completed these tasks at a faster processing speed (cf. Figure 3 ). In the Czech Republic, most of the students can be fall into the first profile (71.32%), while 28.68% go in the second profile. In Denmark, 88.10% are part of the first profile, while 11.90% fit the second profile. In Germany, 82.51% of the students are in the first profile and 17.49% can be allocated to the second profile (cf. Fig. 3 ).
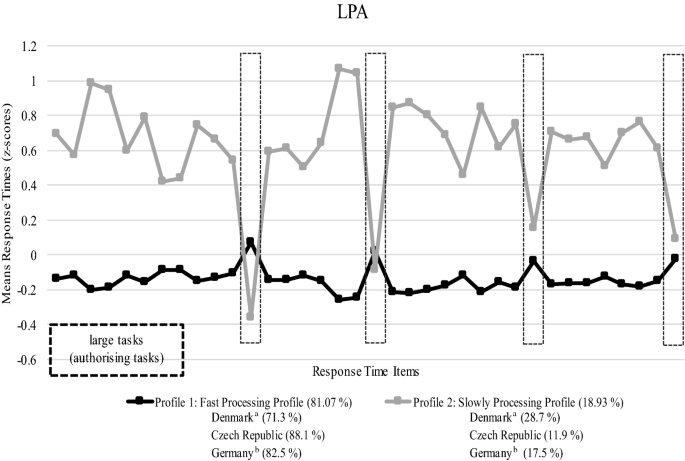
Latent Profile Analysis: Processing profiles. a Did not meet sample requirements. b “ Met guidelines for sampling participation rates only after replacement schools were included” ( Fraillon et al. 2014 , S. 112)
With regard to the first research question, the analysis shows that the Czech and German students belonging to the first profile have on average a significantly higher CIL than the students belonging to the second profile (Czech Republic profile 1: M = 558 points; profile 2: M = 541 points/Germany profile 1: M = 526 points; profile 2: M = 510 points / p < 0.05). In Denmark, there is no such significant difference between the students (profile 1: M = 542 points, /profile 2: M = 536 points). Response times can be used to explain students’ CIL in the Czech Republic and Germany. Therefore, we can speak of a so-called significant negative time-on-task effect (cf. Table 3 ).
Results RQ2: processing profiles and CIL regarding students’ background characteristics
Regarding the second research question, the following results are shown with regard to the student background characteristic of gender (cf. Table 4 ): In the Czech Republic as well as in Germany the girls assigned to the fast processing profile (Czech Republic: M = 565 points, 67.94%, Germany: M = 536 points, 80.91%) display significantly higher computer and information literacy than the girls from the slow processing profile (Czech Republic: M = 547 points, 32.06%, Germany: M = 517, 19.09%). In Denmark, there is no such significant difference among the girls (profile 1: M = 550 points, 87.23%; profile 2: M = 541 points, 12.77%). In the Czech Republic, a significantly higher computer and information literacy can be seen for the boys assigned to the fast-processing profile ( M = 552 points, 74.76%) in comparison to the boys allocated to the slow processing profile ( M = 533 points, 25.24%). A similar significant difference can neither be determined for the boys in Denmark nor in Germany (Denmark profile 1: M = 535 points, 88.94%; profile 2: M = 531 points, 11.06%/Germany profile 1: M = 518 points, 84.00%; profile 2: M = 503 points, 16.00%).
So-called negative time-on-task effects can be seen with regard to the socioeconomic background (cf. Table 5 ); in this case, the cultural capital (number of books in the household). In the Czech Republic, a negative time-on-task effect can be observed both for students with high cultural capital (profile 1: M = 576 points, 73.27%; profile 2: M = 560 points, 26.73%) as well as for students with low cultural capital (profile 1: M = 548 points, 70.00%; profile 2: M = 530 points, 30.00%). Such a significant negative time-on-task effect is also evident in Germany concerning students with high cultural capital (profile 1: M = 552 points, 82.43%; profile 2: M = 538 points, 17.57%), but not among high school students with low cultural capital (profile 1: M = 507 points, 83.13%; profile 2: M = 491 points, 16.87%). In Denmark, neither students with high cultural capital (profile 1: M = 564 points, 88.71%; profile 2: M = 561 points, 11.29%) nor students with low cultural capital (profile 1: M = 531 points, 88.16%; profile 2: M = 526 Points, 11.84%) show a significant correlation between their processing profile / processing time and their computer and information literacy (cf. Table 5 ).
Results concerning the parental occupation as a further indicator for socioeconomic background can be found in Table 6 . For the Czech Republic a significant negative time-on-task effect for students from families with a HISEI of less than 40 points is visible: The 68.08% of the students who belong to the fast profile have a higher computer and information literacy ( M = 540 points) in comparison to the 31.92% of students in the slow profile ( M = 519 points). There is also a significant negative time-on-task effect among students with a HISEI of 40 to 59 points (profile 1: M = 564 points, 72.43%; profile 2: 550 points, 27.57%). However, students with a HISEI of 60 points or more show no significant differences in the profiles. Furthermore, there are no significant differences regarding any of the HISEI categories in Denmark. Nonetheless, a positive though not significant time-on-task effect can be noted here for students with a HISEI of 60 points or more. The 88.91% of students belonging to the fast profile achieved an average of 562 points and thus, fewer points than the 11.09% students belonging to the slow profile (565 scale points). For Germany, a significant negative time-on-task effect can be identified for students with a HISEI less than 40 points (profile 1: M = 506 points, 79.54%; profile 2: M = 483 points, 20.46%).
With regard to the immigrant background, as determined by the language spoken at home, the following results are shown (cf. Table 7 ): In the Czech Republic it can be ascertained that the students without an immigrant background (the at-home spoken language is the same as the test language) who belong to the fast-paced processing profile have a higher computer and information literacy ( M = 559 points, 71.51%) than those who are allocated to the slow profile ( M = 542 points, 28.49%). For students with an immigrant background (the at-home spoken language differs from the test language), there is no significant difference in the Czech Republic (profile 1: M = 548 points, 64.19%; profile 2: M = 529 points, 35.81%). Likewise, in Denmark, there are no significant differences regarding the students with an immigrant background (profile 1: 502 points, 84.49%; profile 2: M = 495 points, 15.51%) and without an immigrant background (profile 1: M = 546 points, 88.7%; profile 2: M = 544 points, 11.3%). In Germany, as in the Czech Republic, only those students whose families use the test language in the home environment show a significant negative time-on-task effect (profile 1: M = 534 points, 83.16%; profile 2: M = 520 points, 16.84%). There was no significant difference for young people without an immigrant background (profile 1: M = 491 points, 80.01%; profile 2: M = 473 points, 19.99%).
In addition to the language spoken at home, the parents’ country of birth is included in the analysis regarding the immigrant background (cf. Table 8 ). The Czech Republic displays, as with the language spoken at home, a significant negative time-on-task effect for students without an immigrant background: 71.57% of the students whose parents were not born abroad belong to the fast profile ( M = 559) while the other 28.43% belong to the slow profile with lower CIL ( M = 542). For Denmark, there are no significant differences between the profiles, but a second not significant positive time-on-task effect can be identified: 13.32% of the students whose parents were both born abroad fall into to the slow profile. They achieve on average 507 points in CIL while the other 86.68% in the fast profile achieve 500 points. Here, however, the small number of students in the slow profile should be noted. For Germany, a significant negative time-on-task effect can be identified for students whose parents were both born abroad: In this group, 80.45% of the students can be allocated to the fast profile with an average computer and information literacy of 504 points; the remaining 19.55% are assigned to the slow profile and display fewer scale points ( M = 478) and therefore, a lower CIL.
Taking into account the student background characteristics of gender, socioeconomic background (determined here via cultural capital/number of books at home and HISEI) and the immigrant background (determined here via language use at home and parents’ country of birth), the summarized results regarding the second research question for the three countries are presented in Table 9 .
In the Czech Republic, there are significant differences in CIL for all groups except for students with an immigrant background (language used at home is different from the test language and students with one parent or both parents who were born abroad) and students with a high parental occupational status. Thus, there is a significant negative time-on-task effect for these groups. However, as shown in Table 9 there are no significant differences in the processing profiles and CIL for Denmark, even when students’ background characteristics are considered. For Germany, a significant negative time-on-task effect can be found for the girls. The other examined indicators for Germany are ambiguous: the students with a higher cultural capital show a significant negative time-on-task effect as do students with parents with a low occupational status. Additionally, significant negative time-on-task can be highlighted for the students who use the test language at home and those students whose parents were both born abroad. No significant differences in CIL can be found for the other groups.
Discussion and conclusions
The ICILS 2013 study identified differences in the CIL of students, particularly with regard to gender, socioeconomic background and immigrant background (Fraillon et al. 2014 ). The present analysis utilises the potential of process data to explain individual differences in competence tests and examines the relationship between CIL and response times on the basis of response times in IEA-ICILS 2013. For this purpose, two processing profiles (the fast processing profile and the slow processing profile) can be determined using a latent profile analysis. The students who belong to the fast profile finish the tasks on average at a fast pace and those who belong to the slow profile finish the tasks on average at a slow pace. The only exceptions are the so-called large tasks, in which the students who belong to the fast profile finish these tasks on average slower. A more intensive preoccupation with a task could be associated with greater care and thus, increase the probability of a correct answer. The students in the slow profile, on the other hand, finish these tasks faster on average, maybe because those students, who already know the answers, also give there a correct answer faster. This is a more complex task type in which information products (i.e. a presentation) have to be created by the test participants (Fraillon et al. 2014 , 2019 ). Thus, differences in response times with regard to task types in ICILS 2013 become apparent, which should be further analysed in the future.
On the basis of the profiles, it was found that the students from the Czech Republic and Germany who belong to the fast profile have significantly higher CIL than the students belonging to the slow profile and thus a so-called significant negative time-on-task effect (Goldhammer et al. 2014 , 2017 ; Naumann and Goldhammer 2017 ). Only in Denmark, there is no significant difference. In terms of student background characteristics, significant negative time-on-task effects can be observed in the Czech Republic for the majority of the groups (girls, boys, students with higher and lower cultural capital, students with low and medium occupational status and students without an immigrant status), except for students with an immigrant background and a high parental occupational status. While in Denmark no significant effects are noted, there are significant negative time-on-task effects in Germany for girls. The results regarding the socioeconomic and immigrant background in Germany meanwhile, are not as clear as there are significant negative time-on-task effects for students with high cultural capital, such results also applied to students with a low parental occupational status. Furthermore, significant negative time-on-task effects can be found for students who speak the same language as the test-language at home and students whose parents were both born abroad. Especially against the background of these results, it seems necessary to go into greater depth in further analyses and to look at the tasks and results along with the various indicators in smaller steps in order to thoroughly interpret the results described.
Against the background of these results, it can be discussed to what extent the response times can actually explain the differences in the students’ CIL along the student background characteristics gender, socioeconomic background and immigrant background. Although in the Czech Republic and Germany, there are clear correlations between CIL and the processing profiles, the extent to which this result can be used as the sole explanatory approach for the disparities described in the CIL can be questioned in association with student background characteristics. Future studies may also explore the degree to which CIL country-specific curricular requirements play a role.
Furthermore, the methodological approach should be discussed: importantly, the results for the large tasks make it clear that the latent profile analysis is only a first methodological approach in order to make the response times tangible. In further analyses, it therefore, seems logical to focus on the different types of tasks. Further methodological approaches are conceivable for subsequent analyses in this context, which have also been successfully used in previous investigations of response times regarding time-on-task effects (e.g. the Generalized Linear Mixed Model (GLMM) framework; Goldhammer et al. 2014 ). With regard to the selection of the profiles, further analyses must also check whether further profiles can be determined based on the quality criteria in a more fine-grained step-by-step evaluation by modules or task types. In addition, due to the quality criteria listed, which could also be applied to other profile solutions, it makes sense to carry out a different methodological approach, such as a cluster analysis, in order to empirically support the choice of the two-profile solution. Additionally, it would be viable to include the data from the second ICILS cycle, although it should be noted that only Denmark and Germany participated in the study again. Regarding the results of Denmark in comparison to the Czech Republic and Germany, it must be discussed why there are no significant differences between the two processing profiles, not even with regard to student background characteristics. One reason might be the small sample size resulting from the split of profiles and single background characteristics. However, when interpreting the results for the three countries, it should be noted that Denmark does not meet the sampling requirements, which may have affected the results. In the context of the present analyses, low-performing countries are not taken into account as indications should be generated as to how variations in good performance can be explained. Thus, for further analysis, in terms of country selection, it may be useful to select additional participating countries with a very low level of performance or other countries with a similar level of performance in order to improve comparability and determine how this relates to response times.
It seems sensible to include additional predictors in further analyses in order to explain the differences found between and across the countries. Particularly against the background of the abovementioned research, findings on the relationship between response times, reading tasks (Goldhammer et al. 2014 ; Su 2017 ) and other predictors can be used to explain differences in students’ CIL (Hatlevik et al. 2018 ; Livingstone and Helsper 2010 ; Rohatgi et al. 2016 ).
The results of these initial analysis based on the response times of the computer-based test modules clearly reveal that in order to explain and interpret differences in CIL, it makes sense to consider extra- and in-school-based conditions as well as use process data, which explains the behaviour during the test. In addition to a better understanding of how response times are related to CIL, particularly regarding differences between varying groups of students, the research presented in this article also offers potential insight for school practice. In the context of individual support and individualized learning processes, process data will increasingly play a role in the future (e.g. Wang et al. 2018 ), especially in the context of diagnostic measures. Thus, the further analysis of process data, such as response times, for future research in the context of school development processes is of particular relevance. The analysis of process data is also becoming increasingly important for the (further) development of competence testing within the framework of school performance studies. The time frame for the processing of tasks must be put into question as well as the task design.
Availability of data and materials
The data of ICILS 2013 are publicly available on the IEA website ( https://www.iea.nl/data-tools/repository/icils ).
Abbreviations
Australian Curriculum Assessment and Reporting Authority
- Computer and information literacy
Computer in Education
International Computer and Information Literacy Study
International Association for the Evaluation of Educational Achievement
Programme for the International Assessment of Adult Competencies
ACARA. (2015). National Assessment Program – ICT Literacy. Years 6 & 10. Report 2014. Sydney: ACARA. Retrieved from https://www.nap.edu.au/_resources/D15_8761__NAP-ICT_2014_Public_Report_Final.pdf
ACARA. (2018). NAP Sample Assessment ICT Literacy . Years 6 and 10. Sydney: ACARA. Retrieved from https://www.nap.edu.au/docs/defaultsource/default-document-library/2017napictlreport_final.pdf?sfvrsn=b5696d5e_2
Aesaert, K. & van Braak, J. (2018). Information and Communication Competences for Students. In J. Voogt; G. Knezek, R. Christensen & K.-W. Lai (Eds.), Second Handbook of Information Technology in Primary and Secondary Education (pp. 255–269). Cham: Springer. https://doi.org/10.1007/978-3-319-53803-7
Aesaert, K., & van Braak, J. (2015). Gender and socioeconomic related differences in performance based ICT competences. Computers & Education, 84, 8–25. https://doi.org/10.1016/j.compedu.2014.12.017
Article Google Scholar
Eickelmann, B. (2018). Section introduction: international policies on information and communication technology in primary and secondary schools. In J. Voogt, G. Knezek, R. Christensen & K.-W. Lai (Eds.), Second Handbook of Information Technology in Primary and Secondary Education . Cham: Springer. https://doi.org/10.1007/978-3-319-53803-7
Gerick, J., Eickelmann, B. & Bos, W. (2017). The international computer and information literacy study from a European perspective: introduction to the special issue. European Educational Research Journal , 16 (6), 707–715. https://doi.org/10.1177/1474904117735417
Bonfadelli, H., Bucher, P., & Piga, A. (2007). Use of old and new media in ethnic minority youth in Europe with a special emphasis on Switzerland. Communications, 32 (2), 141–170. https://doi.org/10.1515/COMMUN.2007.010
D’Haenens, L. (2003). ICT in multicultural society The Netherlands: A context for sound multiform media policy? Gazette, 65 (4–5), 401–421. https://doi.org/10.1177/0016549203654006
Fraillon, J., Ainley, J., Schulz, W., Friedman, T. & Duckworth, D. (2019). Preparing for life in a digital world: IEA International Computer and Information Literacy Study 2018 International Report. Amsterdam: International Association for the Evaluation of Educational Achievement (IEA). Retrieved from https://www.iea.nl/sites/default/files/2019-11/ICILS%202019%20Digital%20final%2004112019.pdf
Fraillon, J., Schulz, W., Friedman, T., Ainley, J., & Gebhardt, E. (2015). ICILS 2013 Technical Report . Amsterdam: IEA Secretariat. https://doi.org/10.15478/uuid:b9cdd888-6665-4e9f-a21e-61569845ed5b
Book Google Scholar
Fraillon, J., Ainley, J., Schulz, W., Friedman, T., & Gebhardt, E. (2014). Preparing for Life in a Digital Age. The IEA International Computer and Information Literacy Study International Report. Melbourne: Australian Council for Educational Research (ACER). https://doi.org/10.1007/978-3-319-14222-7
Ganzeboom, H. B. G., de Graaf, P. M., & Treiman, D. J. (1992). A standard international socio-economic index of occupational status. Social Science Research, 21 (1), 1–56.
Gebhardt, E., Thomson, S., Ainley, J., & Hillman, K. (2019). Gender differences in computer and information literacy. An In-depth Analysis of Data from ICILS. Cham: Springer. https://doi.org/10.1007/978-3-030-26203-7_1
Gerick, J. (2018). School Level Characteristics and Students’ CIL in Europe—a latent class analysis approach. Computers & Education, 120, 160–171. https://doi.org/10.1016/j.compedu.2018.01.013
Goldhammer, F. (2013). Prozessbezogene Verhaltensdaten für Rückmeldung in digitalen Lernumgebungen. [Process-related behavioral data for feedback in digital learning environments.] Deutsches Institut für Internationale Pädagogische Forschung (DIPF). Zentrum für internationale Bildungsvergleichsstudien (ZIB).
Goldhammer, F., Naumann, J., Rölke, H., Stelter, A. & Tóth, K. (2017). Relating Product Data to Process Data from Computer-Based Competency Assessment. In D. Leutner, J. Fleischer, J. Grünkorn & E. Klieme (Eds.), Competence Assessment in Education. Research, Models and Instruments (pp. 407–425). Heidelberg: Springer. https://doi.org/10.1007/978-3-319-50030-0
Goldhammer, F., Naumann, J., Stelter, A., Rölke, H., Tóth, K., & Klieme, E. (2014). The time-on-task effect in reading and problem solving is moderated by item difficulty and ability: Insights from computer-based large-scale assessment. Journal of Educational Psychology, 106, 608–626. https://doi.org/10.1037/a0034716
Goldhammer, F., Naumann, J., & Keßel, Y. (2013). Assessing individual differences in basic computer skills: Psychometric characteristics of an interactive performance measure. European Journal of Psychological Assessment, 29, 263–275. https://doi.org/10.1027/1015-5759/a000153
Hatlevik, O. E., Throndsen, I., Loi, M., & Gudmundsdottir, G. B. (2018). Students’ ICT self-efficacy and computer and information literacy: Determinants and relationships. Computers & Education, 118, 107–119. https://doi.org/10.1016/j.compedu.2017.11.011
Hatlevik, O. E., Scherer, R., & Christophersen, K.-A. (2017). Moving beyond the study of gender differences: An analysis of measurement invariance and differential item functioning of an ICT literacy scale. Computers & Education, 113, 280–293. https://doi.org/10.1016/j.compedu.2017.06.003
Hatlevik, O. E., Ottestad, G., & Throndsen, I. (2015). Predictors of digital competence in 7th grade: a multilevel analysis. Journal of Computer Assisted Learning, 31, 220–231. https://doi.org/10.1111/jcal.12065
Hatlevik, O. E., & Christophersen, K.-A. (2013). Digital competence at the beginning of upper secondary school: Identifying factors explaining digital inclusion. Computers & Education, 63, 240–247. https://doi.org/10.1016/j.compedu.2012.11.015
Hohlfeld, T. N., Ritzhaupt, A. D., & Barron, A. E. (2013). Are gender differences in perceived and demonstrated technology literacy significant? It depends on the model. Educational Technology Research and Development, 61 (4), 639–663. https://doi.org/10.1007/s11423-013-9304-7
Jung, M., & Carstens, R. (Eds.). (2015). ICILS 2013 User Guide for the International Database . Amsterdam: IEA. https://doi.org/10.15478/uuid:73a9f018-7b64-4299-affc-dc33fe57f3e1
Livingstone, S., & Helsper, E. (2010). Balancing opportunities and risks in teenagers’ use of the internet: the role of online skills and internet self-efficacy. New Media & Society, 12 (2), 309–329. https://doi.org/10.1177/1461444809342697
Luu, K., & Freeman, J. G. (2011). An analysis of the relationship between information and communication technology (ICT) and scientific literacy in Canada and Australia. Computers & Education, 56 (4), 1072–1082. https://doi.org/10.1016/j.compedu.2010.11.008
Mirazchiyski, P. (2015). Analyzing ICILS 2013 data using the IEA IDB Analyzer. In M. Jung & R. Carstens (Eds.), ICILS 2013 User Guide for the International Database (pp. 49–86). Amsterdam: IEA. https://doi.org/10.15478/uuid:73a9f018-7b64-4299-affc-dc33fe57f3e1
Mohamad, I. B., & Usman, D. (2013). Standardization and its effects on k-means clustering algorithm. Research Journal of Applied Science, Engineering and Technology, 6 (17), 3299–3303. https://doi.org/10.19026/rjaset.6.3638
Morris, D., & Trushell, J. (2014). Computer programming, ICT and gender in the classroom: a male-dominated domain or a female preserve? Research in teacher education, 4 (1), 4–9. Retrieved from https://www.uel.ac.uk/wwwmedia/microsites/riste/Article-1-David-Morris-and-John-Trushell.pdf
Muthén, L. K. & Muthén, B. O. (2012). Mplus Statistical Analysis With Latent Variables. User’s Guide . Seventh Edition ed. Los Angeles, CA: Muthén & Muthén. Retrieved from https://www.statmodel.com/download/usersguide/Mplus%20Users%20Guide%20v6.pdf
Naumann, J. (2012). Belastungen und Ressourcen beim Lernen aus Text und Hypertext. [Costs and resources in learning from text and hypertext] . (Habilitation thesis). Goethe Universität Frankfurt, Frankfurt, Germany. https://doi.org/10.13140/RG.2.2.34203.46888
Naumann, J., & Goldhammer, F. (2017). Time-on-task effects in digital reading are non-linear and moderated by persons’ skills and tasks’ demands. Learning and Individual Differences, 53, 1–16. https://doi.org/10.1016/j.lindif.2016.10.002
Nylund, K. L., Asparouhov, T., & Muthén, B. O. (2007). Deciding on the number of classes in latent class analysis and growth mixture modeling: A monte carlo simulation study. Structural Equation Modeling, 14 (4), 535–569. https://doi.org/10.1080/10705510701575396
Oberski, D. (2016). Mixture Models: Latent Profile and Latent Class Analysis. In J. Robertson & M. Kaptein (Eds.), Modern Statistical Methods for HCI (pp. 275–287). Switzerland: Springer. https://doi.org/10.1007/978-3-319-26633-6
Pelgrum, W. J., Reinen, I. A. M. J. & Plomp, T. (1993). Schools, teachers, students and computers: A cross-national perspecitve. IEA-Comped Study Stage 2 . Enschede: University of Twente. Retrieved from https://files.eric.ed.gov/fulltext/ED372734.pdf
Punter, R. A., Meelissen, M. R., & Glas, C. A. (2017). Gender differences in computer and information literacy: An exploration of the performances of girls and boys in ICILS 2013. European Educational Research Journal, 16 (6), 762–780. https://doi.org/10.1177/1474904116672468
Rohatgi, A., Scherer, R., & Hatlevik, O. E. (2016). The role of ICT self-efficacy for students’ ICT use and their achievement in a computer and information literacy test. Computers & Education, 102, 103–116. https://doi.org/10.1016/j.compedu.2016.08.001
Stelter, A., Goldhammer, F., Naumann, J., & Rölke, H. (2015). Die Automatisierung prozeduralen Wissens: Eine Analyse basierend auf Prozessdaten [The automation of procedural knowledge: An analysis based on process data]. In J. Stiller & C. Laschke (Eds.), Berlin-Brandenburger Beitrage zur Bildungsforschung 2015: Herausforderungen, Befunde und Perspektiven Interdisziplinärer Bildungsforschung (pp. 111–132). Frankfurt am Main: Lang. https://doi.org/10.3726/978-3-653-04961-9
Su, S. (2017). Incorporating Response Times in Item Response Theory Models of Reading Comprehension Fluency. University of Minnesota Digital Conservancy. Retrieved from https://search.proquest.com/docview/2013525225?accountid=13049
Tein, J.-Y., Coxe, S., & Cham, H. (2013). Statistical power to detect the correct number of classes in latent profile analysis. Structural Equation Modeling, 20 (4), 640–657. https://doi.org/10.1080/10705511.2013.824781
Thomson, S. (2015). Australian Students in a Digital World. Policy Insights, Issue 3 . Melbourne: ACER. Retrieved from https://research.acer.edu.au/cgi/viewcontent.cgi?article=1002&context=policyinsights
Wang, S., Zhang, S., Douglas, J. & Culpepper, S. (2018). Using Response Times to Assess Learning Progress: A Joint Model for Responses and Response Times. Measurement: Interdisciplinary Research and Perspectives, 16 (1), 45–58. https://doi.org/10.1080/15366367.2018.1435105
Zhong, Z.-J. (2011). From access to usage: The divide of self reported digital skills among adolescents. Computers and Education, 56 (3), 736–746. https://doi.org/10.1016/j.compedu.2010.10.016
Zillien, N., & Hargittai, E. (2009). Digital distinction: Status-specific types of internet usage. Social Science Quarterly, 90 (2), 274–291. https://doi.org/10.1111/j.1540-6237.2009.00617.x
Download references
Acknowledgements
Not applicable.
There was no funding for our research.
Author information
Authors and affiliations.
Institute for Educational Science, Paderborn University, Paderborn, Germany
Melanie Heldt, Kerstin Drossel & Birgit Eickelmann
Faculty of Linguistics and Literary Studies, Bielefeld University, Bielefeld, Germany
Corinna Massek
You can also search for this author in PubMed Google Scholar
Contributions
All authors contributed to the concept and design. They were jointly responsible for the elaboration and revision of the article. All authors read and approved the final manuscript.
Corresponding author
Correspondence to Melanie Heldt .
Ethics declarations
Ethics approval and consent to paricipate.
We rely on data from the ICILS 2013 study, which conforms to IEA ethical standards. The Australian Council for Educational Research (ACER) in Melbourne served as the international study center for ICILS, working in close cooperation with the IEA, and the national centers of participating countries.
Consent for publication
We provide our consent to publish this manuscript upon publication in the Springer open journal LSA.
Competing interests
The authors declare that they have no financial or non-financial competing interests.
Additional information
Publisher's note.
Springer Nature remains neutral with regard to jurisdictional claims in published maps and institutional affiliations.
Rights and permissions
Open Access This article is licensed under a Creative Commons Attribution 4.0 International License, which permits use, sharing, adaptation, distribution and reproduction in any medium or format, as long as you give appropriate credit to the original author(s) and the source, provide a link to the Creative Commons licence, and indicate if changes were made. The images or other third party material in this article are included in the article's Creative Commons licence, unless indicated otherwise in a credit line to the material. If material is not included in the article's Creative Commons licence and your intended use is not permitted by statutory regulation or exceeds the permitted use, you will need to obtain permission directly from the copyright holder. To view a copy of this licence, visit http://creativecommons.org/licenses/by/4.0/ .
Reprints and permissions
About this article
Cite this article.
Heldt, M., Massek, C., Drossel, K. et al. The relationship between differences in students’ computer and information literacy and response times: an analysis of IEA-ICILS data. Large-scale Assess Educ 8 , 12 (2020). https://doi.org/10.1186/s40536-020-00090-1
Download citation
Received : 17 December 2019
Accepted : 15 October 2020
Published : 23 October 2020
DOI : https://doi.org/10.1186/s40536-020-00090-1
Share this article
Anyone you share the following link with will be able to read this content:
Sorry, a shareable link is not currently available for this article.
Provided by the Springer Nature SharedIt content-sharing initiative
- Response times
- Student background characteristics
- Process data
- Research article
- Open access
- Published: 19 June 2006
Computer literacy and attitudes towards e-learning among first year medical students
- Thomas Michael Link 1 &
- Richard Marz 1
BMC Medical Education volume 6 , Article number: 34 ( 2006 ) Cite this article
62k Accesses
87 Citations
Metrics details
At the Medical University of Vienna, most information for students is available only online. In 2005, an e-learning project was initiated and there are plans to introduce a learning management system. In this study, we estimate the level of students' computer skills, the number of students having difficulty with e-learning, and the number of students opposed to e-learning.
The study was conducted in an introductory course on computer-based and web-based training (CBT/WBT). Students were asked to fill out a questionnaire online that covered a wide range of relevant attitudes and experiences.
While the great majority of students possess sufficient computer skills and acknowledge the advantages of interactive and multimedia-enhanced learning material, a small percentage lacks basic computer skills and/or is very skeptical about e-learning. There is also a consistently significant albeit weak gender difference in available computer infrastructure and Internet access. As for student attitudes toward e-learning, we found that age, computer use, and previous exposure to computers are more important than gender. A sizable number of students, 12% of the total, make little or no use of existing e-learning offerings.
Many students would benefit from a basic introduction to computers and to the relevant computer-based resources of the university. Given to the wide range of computer skills among students, a single computer course for all students would not be useful nor would it be accepted. Special measures should be taken to prevent students who lack computer skills from being disadvantaged or from developing computer-hostile attitudes.
Peer Review reports
Computer literacy has been a subject of educational research ever since personal computers were introduced to the classroom, either as teaching aids or as tools for self-study. In the 1980s, research on computer literacy focused on the question whether medical students were ready for the foreseeable omnipresence of computers in the future doctors' professional environments [ 1 – 4 ], i.e., whether they possessed the necessary computer skills [ 2 , 5 – 9 ]. The vision of a knowledge-based society saw future economic wealth dependent on people's abilities to deal with the growing information load and to adapt to an ever-changing working environment [ 10 – 13 ]. It was assumed that computers would become ubiquitous tools for managing medical knowledge [ 14 ]. In some medical schools, a privately owned computer was made a requirement for medical students [ 15 , 16 ].
E-Learning, in particular the use of learning management systems (LMSs), introduced a new aspect. Researchers [ 17 ] suggested that some students may lack the necessary skills to use web-based learning platforms effectively and are therefore handicapped. This issue is often discussed in the context of gender differences. The main concern is that female students are at a disadvantage due to different patterns of computer usage, e.g. a less dominant style of discussion in web-based communication [ 18 , 19 ]. These gender differences can be observed in students' computer-related behaviors but also in their attitudes towards computer-based and web-based training (CBT/WBT). In a Danish study, Dørup [ 9 ] reported that among first-year students, 46% of the men were in favor of replacing "traditional teaching with use of computers if possible" while only 22% women agreed with this statement.
In 2004, 80% of Austria's 20–29 year olds had Internet access and 75% of university and high school students used a computer daily [ 20 ]. We can thus assume that, in general, students entering university have good basic computer skills. Studies nevertheless demonstrate that there is a considerable difference in computer use according to students' disciplines. Middendorff [ 21 ] reports that German medical students spend an average of 8 hours per week at the computer (including private activities). This is the lowest value of all disciplines, what makes it difficult to draw conclusions about medical students' computer use from general surveys. Often the degree of "informational fluency" remains at a basic level and students tend to over-estimate their computer skills [ 22 ].
This study examines the level of computer literacy and patterns of computer usage of first-year medical students at the Medical University of Vienna. It was conducted in an introductory course for first-year students on CBT/WBT. The goal of the study was to determine the need for such introductory courses and to provide information that could be used to improve them. A secondary aim was to identify difficulties that may be encountered in implementing a university-wide LMS due to students' lack of computer literacy or low acceptance of e-learning. While multimedia learning programs have been praised for their educational superiority, actual use of these programs has sometimes failed to meet our expectations.
Since autumn 2003, we have required students to take an introductory course on CBT/WBT as a single 90-minute class session. This course is held for first-year students (about 1500 students took it in 2004 and 2005) and second-year students (about 600 students from 2003 to 2005) [ 23 ]. The course serves two main purposes:
To ensure a certain level of computer and information literacy, including online communication skills.
To acquaint students with computer and web-based learning materials.
In 2003 and 2004, students had to review web-based learning programs (e.g. [ 24 ]) and post their statements in a dedicated online forum. In the course for first-year students we used a student-developed platform [ 25 ]. In the course for second-year students, we used Manila [ 26 ] in 2003 and TikiWiki [ 27 ] in 2004 as a collaboration tool. In 2005, we switched to tools that were partly self-developed and less demanding with respect to the server load.
This paper reports on data from an online survey for the 2004 course for first-year students. Participation in the survey was voluntary and anonymous (though students were asked to give their student ID if they wanted to). The tutors were not able to determine who has or has not filled out the questionnaire. Using class time for students to fill out the questionnaire nevertheless ensured a high response rate of 79%.
A total of 1232 questionnaires were completed, 1160 of which remained in the data set after applying some filtering rules in order to eliminate records of uncertain origin. The gender breakdown of respondents was 61% female and 39% male. This corresponds exactly to the gender breakdown of the 1560 students entering the study module (61% female and 39% male). We thus conclude that our sample was representative of the 2004 cohort. Missing values due to non-responses are not included in tables or figures. Differences between the reported counts and the sample size (n = 1160) are thus due to missing responses.
Questionnaire
The questionnaire [ 28 ] (see Additional file 1 ) was designed to collect the following information:
Overall evaluation of the course
Attitudes towards e-learning as well as previous experiences and expectations about the use of CBT/WBT
Computer and Internet usage
Extent of students' private computer infrastructure
Basic demographic data.
In the following, we will focus on students' computer usage and private computer infrastructure as well as their attitudes toward e-learning.
Attitudes towards e-learning (understood as an umbrella concept for learning methods supported by information- and communication technologies (ICT) in general) were determined by the students' agreement or disagreement with several statements about the importance of ICT in medical education. These statements contained items like "Web-based learning programs are able to replace lectures" or "In medical teaching, there is no need for the use of Web-based programs." The students rated their agreement or disagreement on a bi-polar eight-point Likert scale. For the purpose of comparability with Dørup [ 9 ], we recoded their answers into dichotomous variables. As computer use and attitudes towards e-learning were measured on an ordinal scale, we accordingly used Spearman rho to describe the statistical relationship of these variables with other items. For other metric variables Pearson r was used.
Computer infrastructure
Almost all students (94%) have access to a privately owned PC they can use for their studies, which is either owned by the students themselves (74%) or shared with family members or roommates (20%). Only 5% rely primarily on public computer facilities (Table 1 ).
Student-owned PCs are on average 2.3 years old; 92% are newer than 5 years, 87% newer than 4 years. This corresponds to the life span of computers in companies or public administration offices. Only 3.2% of the students have a computer older than 6 years. Male students' PCs (mean ± SD: 2 ± 1.42 years) are newer than those owned by women (2.5 ± 2.05 years). The 95% confidence interval for the difference is 0.33..0.79 years.
Internet access
The great majority of students also have access to the Internet, though the quality of connectivity varies widely; 60% have access via ADSL, cable TV, or LAN (which, however, usually signifies the use of public facilities at the university or elsewhere); 37% have access using a telephone connection (modem or ISDN) (Table 2 ). The type of Internet access differs according to gender (Cramer V = 0.28, p = 0.001). Male students tend to have faster Internet access while older technologies (e.g. modem) are more common among women. The proportion of modem users is twice as high among women (33%) than among men (15%).
Computer use
Types of computer use.
Students are familiar with e-mail and the use of the Internet for information research; 94% of the students communicate via e-mail and 97% use the Internet for information research at least several times per month. While the use of word processors is very common (82% use such a program several times a month), students are less familiar with other program types (Table 3 ).
Very few medical students have experience in Web design or the creation of HTML documents (5% at least weekly) and thus make no use of the Internet for publishing or more sophisticated collaboration purposes. The frequencies of using communication technologies other than e-mail, e.g., chats (21%), forums and bulletin boards (13%), are also low.
One noteworthy detail is the proportion of students who use computers for organizing appointments, to do lists, or making notes: 28% use such a personal organizer software several times per week, which may point to the use of personal digital assistants (PDA) or smart cell phones.
Except for the categories "Word Processor" and "E-mail," male students use the computer significantly more often than women. The strength of this statistical relationship is weak. Spearman rho is highest for the categories "Web-design" (r s = 0.25, p = 0.001), "Games" (r s = 0.23, p = 0.001), "Forums" (r s = 0.21, p = 0.001), and "Spreadsheets" (r s = 0.20, p = 0.001).
Age when using a computer for the first time
Half of all students (50%) used a computer for the first time by the age of 11 (mean 11.2 ± 3.77 SD). By the time they entered university, i.e., before the age of 18, fully 96% of all students had begun to use computers. The average age when students began using computers for the first time is slightly lower for men (10.7 ± 3.40 years) than women (11.5 ± 3.96 years). The 95% confidence interval for this difference is 0.33..1.24 years.
Prior experiences and expectations
Half of the students (49%) report using a computer or Web-based learning program at least once per month. In order to determine how many students have little or no experience with e-learning, we consolidated answers to questions about four different kinds of e-learning programs (information retrieval, downloading scripts, LMS, and CBT/WBT) into one index. Because of the high response rates for "downloading learning material," we defined inexperienced users as those who answered "less often" or "never" to questions about at least three of these kinds of programs. Following this typology, 12% of the students are inexperienced, having used at most one kind of e-learning program at least once per term (Table 4 ).
The majority of students (66%) have already used a computer or Web-based dictionary like the Pschyrembel medical dictionary, which is one of the standard references used by Vienna medical students. Half of them (50%) have used an online image repository at least once and 42% have used some kind of online quiz to test their knowledge (Table 5 ). Other kinds of learning programs, such as those associated with a constructivist approach, are less well known among first-year Vienna students. The results given in Tables 4 and 5 relating to students' use of LMS are inconsistent. This inconsistency arises most likely from the students' lack of understanding of what a LMS is since very few lecturers use this kind of software to support their courses.
About 10% of the students have never used any of the above-mentioned kinds of e-learning programs and 4.4% do not regard any of them as helpful. Those who regard only two or fewer as helpful tend to prefer learning programs that have no "built-in" educational theory, such as encyclopedias (38%), image collections (23%), and quizzes (23%). The number of different kinds of programs that students have experience with and that they consider helpful correlates with Pearson r = 0.32 (p = 0.001) – the more kinds of programs they know, the more kinds they consider useful.
A majority of the students agree (median = 2, interquartile range = 3) that CBT/WBT should be offered as a supplement to lectures and seminars (Figure 1 ). On the other hand, most students disagree with the statement that e-learning should replace these traditional forms of teaching (median = 7, IQR = 4).
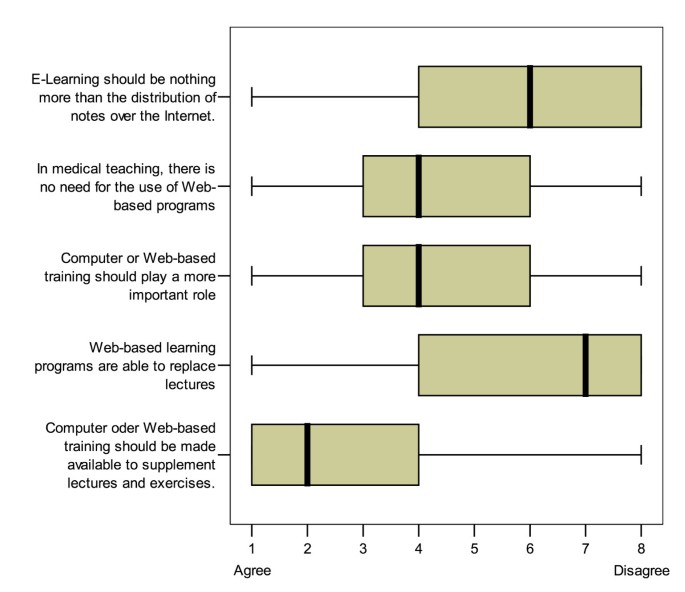
Students' agreement or disagreement with statements on the usefulness of e-learning . The x-axis represents the values of an 8-point bi-polar rating scale: 1 = strong agreement, 8 = strong disagreement. The boxes show the quartiles (25% of the distribution) and the median (50% cut).
Men (median = 6) tend to be slightly more in favor of replacing traditional lectures with CBT/WBT than women (median = 7). The strength of this effect is negligible (r s = 0.06, p = 0.041). After recoding to a dichotomous scale (1..4 = pro, 5..8 = contra), 28% of male and 25% of female students can be considered favoring the replacement of traditional teaching methods with e-learning. The gender difference is slightly bigger for the item "Computer or Web-based training should play a more important role" but still hardly noteworthy (r s = 0.16, p = 0.001). In general, the following variables have bigger effects on e-learning-related attitudes than gender per se:
Lack of experience with CBT/WBT
Productive computer and Internet use (e.g. spreadsheets, organizer, word processor, graphics, e-mail, Web design, and information research).
We consolidated statements 2 to 4 in Figure 1 into one index (Cronbach alpha = 0.65; inclusion of the items 1 and 5 leads to a slight decrease in reliability). In a regression model (Table 6 ) that includes the above 3 variables and gender (R 2 adj = 0.15, p = 0.001, SEE = 1.54), gender is not statistically significant (p = 0.41). When the stepwise regression method is used, gender is excluded from the final model.
Computer infrastructure and internet access
A sizable number of students still have Internet access only via dial-up connections using a modem. This mode of Internet access is slow and impedes the use of synchronous communication tools that require one to stay online for a long period of time. Even if the majority of students do have broadband access to the Internet, mandatory e-learning solutions cannot rely on synchronous online communication tools like chats and on extensive video material, e.g. recordings from lectures. Instead, preference should be given to asynchronous online communication tools and textual information along with videos. Asynchronous communication tools also have the advantage that teachers and students do not have to be online at the same time.
Computer use patterns
Only a small number of students have experience with Internet publishing and asynchronous communication tools like BBS or forums. Thus, most of our students are rather passive Internet users and miss out on numerous possibilities of virtual communities and Web-based publishing. The lack of experience with synchronous and asynchronous online communication, with the exception of e-mail, may cause problems when using the collaboration tools included in an LMS [ 29 ].
Attitudes towards e-learning
Most students agree that e-learning could serve as a supplement for lectures and seminars. However, about as many students disagree with the statement that e-learning could replace traditional ways of teaching. In the Danish context, Dørup [ 9 ] reported a slightly greater proportion of first-year medical students in favor of replacing traditional lectures with e-learning (47% men, 22% women). These higher levels of agreement could be explained by the different response scales used but also by the fact that Danish people in general are reported [ 30 ] to be more "digital literate" than Austrians – although this difference cannot be claimed for persons under 24 years of age [ 30 ].
The intensity of computer use and previous experience with CBT/WBT have the greatest effect on students' attitudes towards e-learning. The explanation for this could be a general discomfort with the technology that makes students who lack experience with ICT express themselves cautiously about its use in education [ 31 ]. It could also be explained by the relative novelty of e-learning and students' difficulties in integrating CBT/WBT into their way of learning [ 32 ].
Most students seem to acknowledge the range of possibilities of new media to enhance their learning experience although they consider CBT/WBT a supplement to rather than a replacement of other learning materials. However, there is also a group of students who are strictly opposed to CBT/WBT (4.4% of the first-year students do not value any of the kinds of programs mentioned above). More disturbing, 24% strongly agree (values 1 and 2 on an 8-point rating scale) with the statement that the Medical University of Vienna could do well without CBT/WBT. When introducing an online LMS or Web-based learning program, special care should be taken not to lose these students because of the choice of a certain learning technology.
In December 2005, we also held a few focus groups with teachers and students on a similar subject. In the course of these discussions it became clear how some characteristics of the new curriculum, especially the emphasis on the MCQ-based year-end examinations, impeded the use of CBT/WBT. In these discussions the students had doubts about the usability and efficiency of e-learning (with regard to costs, handling of ICT, but also learning efficiency) while they still acknowledged the possibilities of ICT support with respect to visualization, simulation, self-quizzing, and fast information retrieval from several sources such as encyclopedias or Web pages.
Gender differences
We were able to identify gender differences for all computer-related variables. In sum, men make more frequent use of computers and have access to better computer infrastructure and faster Internet connections. While this difference is quite consistent over several variables, the strength of the statistical relationship is weak and, with respect to students' attitudes towards e-learning, overshadowed by other variables (e.g. previous exposure to CBT/WBT) that are more important for predicting students' attitudes.
With respect to the implementation of an LMS, the most important difference between men and women is the relatively high number of women still using a slow dial-up connection to the Internet, which could impede the use of synchronous communication tools or multimedia-rich Web applications. Well planned use of e-learning and supportive measures should help to neutralize this difference. Although women have less experience with forums, Gunn [ 19 ] showed that these differences in online communication behavior do not necessarily result in worse examination outcomes.
E-Learning must be appropriate to students' level of computer expertise in order not to become a source of frustration. Courses to develop students' computer skills can improve this situation by influencing students' attitudes and capabilities. Our conclusions with respect to such introductory courses are twofold. Students certainly need some kind of formal introduction to the new ICT for learning purposes. But due to the wide range of previous experience and computer skills, there is no one-size-fits-all course design available. Such a course should either be split into several tracks according to students' different levels of computer literacy [ 33 ], or it should be held only for students with little or no computer experience.
There is, however, the danger that precisely those students who need this course the most will hesitate to attend it voluntarily. It is difficult to say how these students could be persuaded to take such a course despite their skepticism towards ICT and e-learning. One strategy would be to emphasize the practical value for solving everyday problems and obtaining useful information. Once they have learned how computers help them solve recurring problems, they will perhaps develop more computer-friendly attitudes. Another solution could be to make the course compulsory but to make the impact negligible for students with good ICT knowledge. This could be achieved with a Web-based entry test. Students who pass the test would be exempted from having to take the course.
When introducing a campus-wide LMS, one has to take into consideration that some students lack the necessary computer skills or infrastructure to participate effectively in online courses, and that others are strictly opposed to e-learning. Introducing a campus-wide e-learning solution thus poses not only technical and organizational challenges but also calls for a promotional strategy. In the future, we can expect more students to think of computers as standard tools for learning as schools make more use ICT in their classrooms. For example, an "avant-garde" of Vienna medical students already created an online forum [ 34 – 36 ] for informally exchanging information about courses as well as students authored learning materials.
Bresnitz EA, Stettin GD, Gabrielson IW: A survey of computer literacy among medical students. J Med Educ. 1986, 61: 410-2.
Google Scholar
Osman LM, Muir AL: Computer skills and attitudes to computer-aided learning among medical students. Med Educ. 1994, 28: 381-5.
Article Google Scholar
Koschmann T: Medical education and computer literacy: learning about, through, and with computers. Acad Med. 1995, 70: 818-21. 10.1097/00001888-199509000-00018.
McGowan JJ, Berner ES: Computer in medical education. International handbook of research in medical education. Edited by: Norman GR, van der Vleuten CPM, Newble DI. 2002, Dordrecht (Netherlands): Kluwer, 537-79.
Chapter Google Scholar
SEUSISS Project. Survey of European universities skills in ICT of students and staff. Final report. 2003, Edinburgh (United Kingdom): University of Edinburgh, [ http://www.intermedia.uib.no/seusiss/seusissreport.pdf ]
Asgari-Jirhandeh N, Haywood J: Computer awareness among medical students: a survey. Med Educ. 1997, 31 (3): 225-9.
Seago BL, Schlesinger JB, Hampton CL: Using a decade of data on medical student computer literacy for strategic planning. J Med Libr Assoc. 2002, 90 (2): 202-209. [ http://www.pubmedcentral.gov/articlerender.fcgi?tool=pubmed&pubmedid=11999178 ]
Hollander S: Assessing and enhancing medical students' computer skills: a two-year experience. Bull Med Libr Assoc. 1999, 87 (1): 67-73. [ http://www.pubmedcentral.gov/articlerender.fcgi?tool=pubmed&pubmedid=9934531 ]
Dørup J: Experience and attitudes towards information technology among first-year medical students in Denmark: longitudinal questionnaire survey. J Med Internet Res. 2004, 6 (1): e10-10.2196/jmir.6.1.e10. [ http://www.jmir.org/2004/1/e10/ ]
Bell D: The coming of the post-industrial society. 1999, New York: Basic Books, [1973]
Lyotard JF: The postmodern condition: a report on knowledge. 1994, Manchester: Manchester University Press, [1979]
Book Google Scholar
Virkus S: Information literacy in Europe: a literature review. Information Research. 2003, 8 (4): paper no. 159, [ http://informationr.net/ir/8-4/paper159.html ]
OECD: The knowledge-based economy. 1996, Paris (France): OECD, [ http://www.oecd.org/dataoecd/51/8/1913021.pdf ]
Faughnan JG, Elson R: Information technology and the clinical curriculum: some predictions and their implications for the class of 2003. Acad Med. 1998, 73 (7): 766-769. 10.1097/00001888-199807000-00013.
Mavis BE, Smith JM: Mandatory microcomputers: potential effects on medical school recruitment and admissions. Medical Education Online. 1997, 2: 5-[ http://www.med-ed-online.org/volume2.htm ]
Blue AV, Elam CL, Rubeck R, Nora LM: Implementing a requirement for computer ownership: One medical school's experience. Medical Education Online. 1997, 2-[ http://www.med-ed-online.org/t0000002.htm ]
Brüchner K: A longitudinal analysis of the development of learner-prerequisites for e-learning using a typology of computer use [in German]. Proceedings "med:u – e-learning in medical education", 7.-8.11.03 [in German]. 2003, Kiel (Germany): University of Kiel, 15-32.
Richter T, Naumann J, Horz H: Computer literacy, computer-related attitudes, and computer use among male and female students [in German]. People and computer. 1st interdisciplinary conference [in German]. Edited by: Oberquelle H, Oppermann R, Krause J Stuttgart. 2001, (Germany): B.G. Teubner, 71-80. [ http://mc.informatik.uni-hamburg.de/konferenzbaende/mc2001/V23.pdf ]
Gunn C: Dominant or different? Gender issues in computer supported learning. Journal of Asynchronous Learning Networks. 2003, 7 (1): 14-30. [ http://www.aln.org/publications/jaln/v7n1/pdf/v7n1_gunn.pdf ]
Integral. Austrian Internet Monitor – Internet development [in German]. Vienna (Austria): Integral. 2005
Middendorff E: Computer use and new media in higher education. Results from the 16th social survey of the German Students Association conducted by the HIS Higher Education-Information-System [in German]. 2002, Bonn (Germany): Bundesministerium für Bildung und Forschung
Gavriilidis K: No leap in quality ahead – empiric results concerning the integration and use of electronic information in higher education [in German]. Virtual campus: scenarios, strategies, studies [in German]. Edited by: Wagner E, Kindt M. 2001, Münster: Waxmann, 266-274.
Link TM, Marz R: Introduction to computer-based learning. Science and medicine. A textbook for the special studies module (SSM1) [in German]. Edited by: Trappl R. 2005, Vienna: Veritas, 147-162.
HemoSurf. [ http://www.aum.iawf.unibe.ch/vlz/BWL/HemoSurf/ ]
eMed. [ http://emed.mitsch.cc ]
Manila. [ http://manila.userland.com ]
TikiWiki. [ http://tikiwiki.org ]
1 st year students 2004 questionnaire. the orginal version in German http://www.meduniwien.ac.at/user/thomas.link/b2/index.php?review=04w , [ http://www.meduniwien.ac.at/user/thomas.link/b2_en/index.php?review=04w ]
Stokes CW, Cannavina C, Cannavina G: The state of readiness of student health professionals for web-based learning environments. Health Informatics Journal. 2004, 10 (3): 195-204. 10.1177/1460458204045434.
SIBIS – Statistical Indicators Benchmarking the Information Society: SIBIS pocket book 2002/03. Measuring the information society in the EU, the EU accession countries, Switzerland and the US. 2003, Bonn (Germany) empirica, [ http://www.sibis-eu.org/publications/pocketbook.htm ]
SPOT+: Students' perspective on technology. Survey report. 2004, [ http://www.spotplus.odl.org/downloads/Survey_report_final.pdf ]
Schanze S: The use of concept mapping in the med:u project – e-learning in medical education. A tool for structuring complex information and for testing learning performance [in German]. Evaluation of e-learning. Goals, methodological aspects, future [in German]. Edited by: Meister DM, Tergan S, Zentel P. 2004, Münster (Germany): Waxmann, 171-187.
Gibson KE, Silverberg M: A two-year experience teaching computer literacy to first-year medical students using skill-based cohorts. Bull Med Libr Assoc. 2000, 88 (2): 157-164.
MCW Portal. [ http://www.mcw-portal.com ]
Med-Forum. [ http://www.med-forum.at/ ]
Medizinstudium.at. [ http://www.medizinstudium.at ]
Pre-publication history
The pre-publication history for this paper can be accessed here: http://www.biomedcentral.com/1472-6920/6/34/prepub
Download references
Acknowledgements
We thank Thomas Benesch for statistical advice. We would also like to thank Jens Dørup, William Fulton, and Sean Marz for critically reading the manuscript and their helpful suggestions.
Author information
Authors and affiliations.
Core Unit for Medical Education, Medical University of Vienna, Vienna, Austria
Thomas Michael Link & Richard Marz
You can also search for this author in PubMed Google Scholar
Corresponding author
Correspondence to Thomas Michael Link .
Additional information
Competing interests.
The author(s) declare that they have no competing interests.
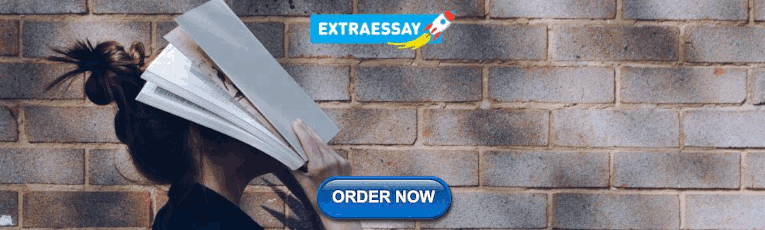
Authors' contributions
RM and TML planned and organized courses [ 23 ] to promote computer literacy among medical students.
TML was responsible for designing the study, implementing the online questionnaire, analyzing the data, writing the first draft, and proofreading the final draft.
RM was responsible for designing the course content, recruiting and training the tutors and supervising all aspects of the course. He revised the article extensively.
Both authors read and approved the final version.
Thomas Michael Link and Richard Marz contributed equally to this work.
Electronic supplementary material
12909_2006_133_moesm1_esm.htm.
Additional File 1: This is the HTML output of the English translation of the questionnaire we used. The HTML was originally generated by a PHP script. As this is only the HTML representation of the questionnaire, clicking on the "Submit" button has no effect. The file can be viewed in any Internet browser. (HTM 53 KB)
Authors’ original submitted files for images
Below are the links to the authors’ original submitted files for images.
Authors’ original file for figure 1
Authors’ original file for figure 2, authors’ original file for figure 3, rights and permissions.
This article is published under license to BioMed Central Ltd. This is an Open Access article distributed under the terms of the Creative Commons Attribution License ( http://creativecommons.org/licenses/by/2.0 ), which permits unrestricted use, distribution, and reproduction in any medium, provided the original work is properly cited.
Reprints and permissions
About this article
Cite this article.
Link, T.M., Marz, R. Computer literacy and attitudes towards e-learning among first year medical students. BMC Med Educ 6 , 34 (2006). https://doi.org/10.1186/1472-6920-6-34
Download citation
Received : 02 March 2006
Accepted : 19 June 2006
Published : 19 June 2006
DOI : https://doi.org/10.1186/1472-6920-6-34
Share this article
Anyone you share the following link with will be able to read this content:
Sorry, a shareable link is not currently available for this article.
Provided by the Springer Nature SharedIt content-sharing initiative
- Internet Access
- Computer Literacy
- Computer Skill
- Computer Infrastructure
- Basic Computer Skill
BMC Medical Education
ISSN: 1472-6920
- Submission enquiries: [email protected]
- General enquiries: [email protected]
- DOI: 10.32996/jweep.2021.3.6.4
- Corpus ID: 237740759
Students’ Computer Literacy and Academic Performance
- Alona Medalia Cadiz-Gabejan , Melinda Jr C. Takenaka
- Published in Journal of world Englishes… 30 June 2021
- Computer Science, Education
Tables from this paper
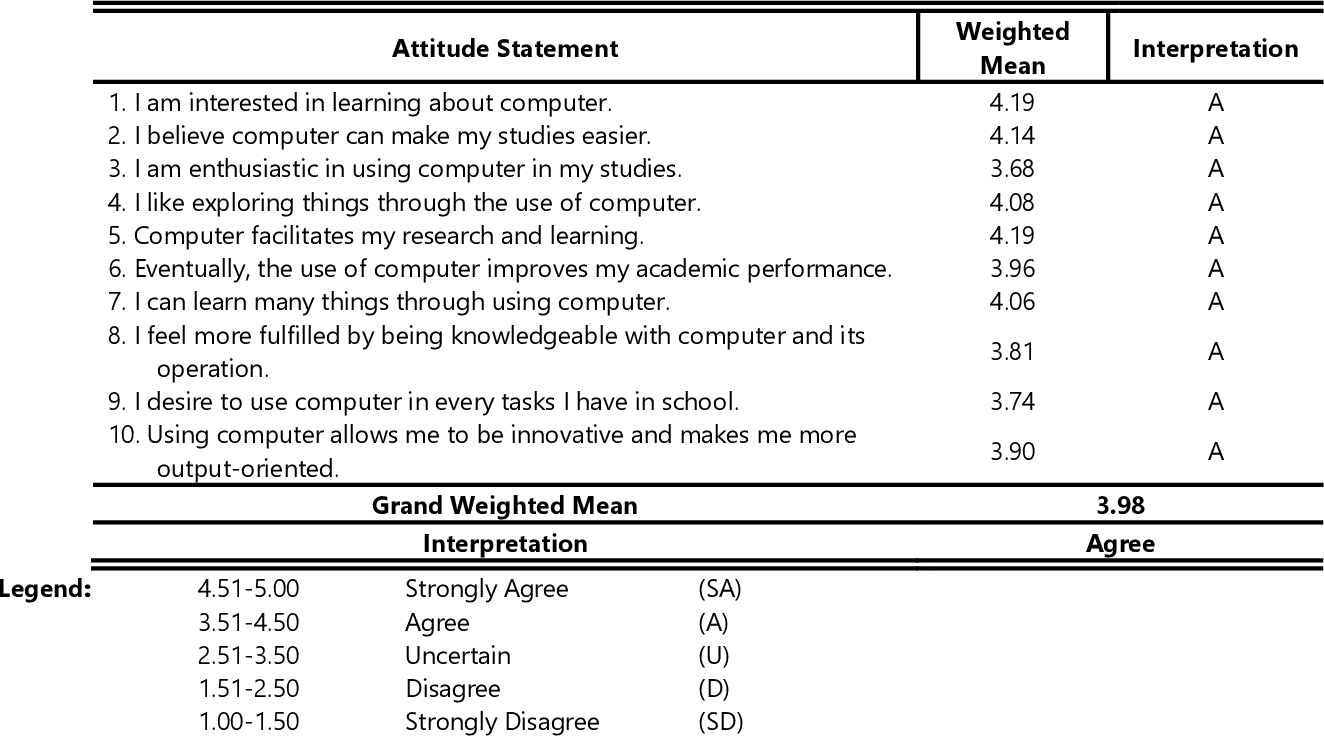
5 Citations
Teachers’ competency and students’ digital literacy in digital environment, enhancing computer literacy of grade 11 students through digital learning resource – project basics (beginners’ aid from school that imparts computer skills), non-ict students’ familiarity with basic computer concepts at the faculty of philosophy at the university of novi sad, navigating micro-credentials: an analysis of student needs, perceptions and skills development at a slovenian university, influence of computer literacy levels on tax compliance among harare central business district small and medium enterprises, 9 references, electronic literacy, reading skills and non-native speakers: issues for eap, the influence of information and communication technologies on students' academic performance, the use and effects of smartphones in higher education, the impact of computer literacy on students’ academic performance in senior secondary schools in esan west local government area, edo state, nigeria, related papers.
Showing 1 through 3 of 0 Related Papers
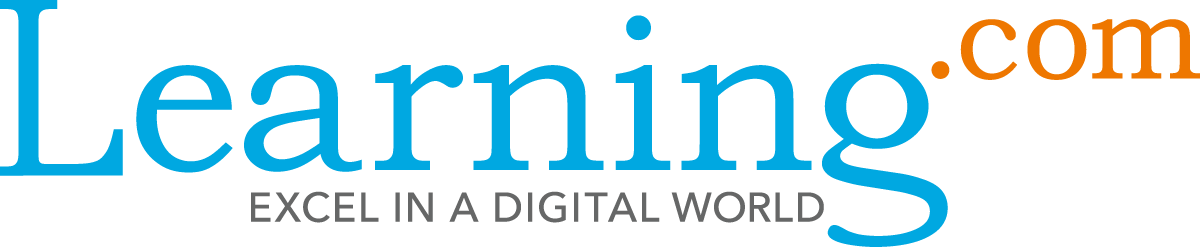
Basic Computer Skills for Elementary Students in 2024
by Lcom Team | Nov 28, 2023 | Blogs
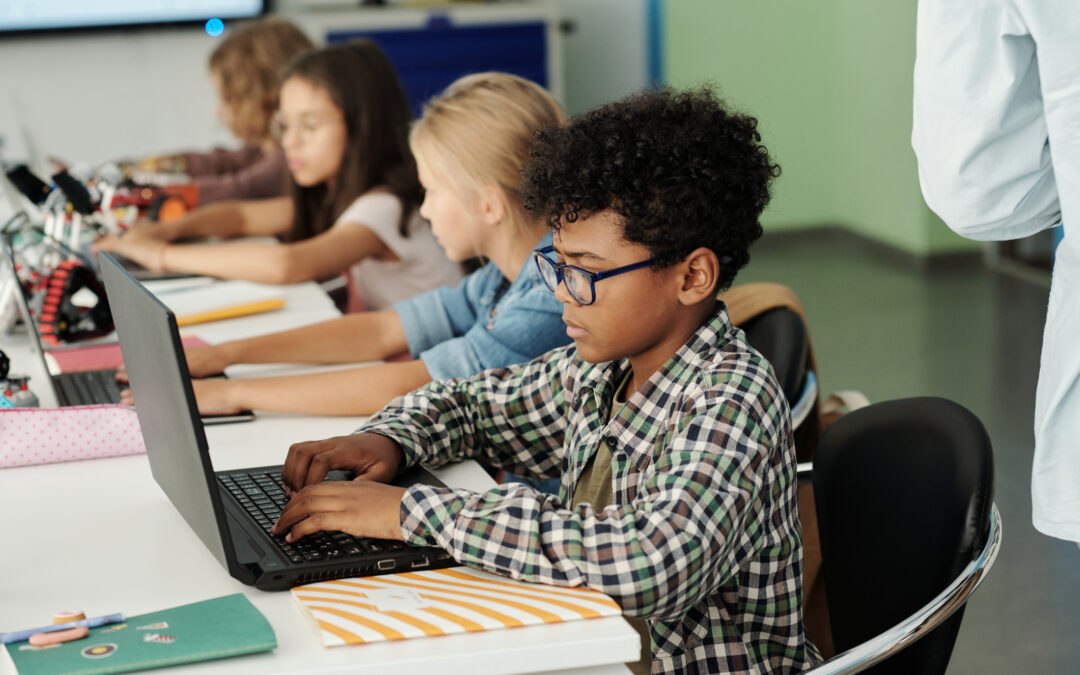
Share this article!
In this article, we discuss the basic computer skills that should be broadly considered in elementary technology curriculum as well as why these skills are important now and in the future, even as particular technologies continue to evolve.
What Basic Computer Skills do Elementary Students Need?
When designing curriculum for computer technology classes for elementary students, it can be difficult to discern what skills are best learned now versus in later grades, as well as what skills can transcend the rapid evolution of technology. Educators should consider that not only are they building a strong digital foundation for future-ready skills but are also helping to prevent the creation of bad or inefficient habits that would otherwise take additional effort to break and rewrite.
With these goals in mind, the following are basic computer skills elementary students should learn that help to set them on a productive and successful path for digital and future-ready skills.
Computer Navigation
One of the first computer skills an elementary student should learn is how to navigate a computer. This means being able to use basic hardware such as a mouse, keyboard, touchpad or touchscreen, as well as being able to find and access basic applications and files. While the technology itself may change immensely before current elementary students are using it in a career setting, the basic ability to navigate a technological device such as a computer, smartphone or tablet will likely be transferable to most future technologies.
Keyboarding
Keyboarding is another important skill for students , despite rapidly evolving technologies. This is because current applications in education, as well as careers, rely on manual keyboarding, with those who lack efficiency in this skill requiring extra time as well as mental energy to complete most keyboarding-based activities or tasks. While the basic skill of putting words into a digital format may eventually become antiquated, the skill remains essential for efficient and effective education, while also serving as a basis for code-based and numbers-based utilization.
Word Processing
Another basic computer skill elementary students should learn is word processing. This skill is used widely in education as well as professional settings as a form of communication, note-taking, composition and more. Being able to utilize word processing software is a college and career-ready skill integral to student success.
Spreadsheets
Elementary students should also learn reading, editing, and creating spreadsheets. Spreadsheets are a common form of data collection, presentation and manipulation, and are frequently used in a variety of careers as well as some education settings. The ability to read and write spreadsheets empowers students with skills they can later build upon as needed.
Digital Presentation
Students will encounter situations throughout their lives in which they are required to present information digitally. Whether putting together a presentation for a school class or a sales pitch to a potential client in a future career, digital presentations are a commonly required computer skill. Understanding the basics of digital presentation provides a foundation upon which elementary students can later grow presentation skills.
Online Communication
Online communication is another computer skill that is expected to evolve greatly while benefiting from a strong skills foundation. Today’s working adults utilize a wide range of platforms for communication, from email and chat tools to collaboration software and virtual meeting technology. While the technology may change as today’s elementary students become part of the workforce, the basic skills of navigating and utilizing these programs will remain relevant.
File Management
File management includes the ability to save, retrieve, backup and protect information. The concepts of file management will likely remain relatively the same, while the hardware and software to do so will likely continue to evolve. In the present, this skill is important for elementary students in educational applications and will later be an essential part of their personal and professional technology skills.
Troubleshooting
Younger generations typically have a reputation for being able to troubleshoot devices more effectively than some adults. While many of these skills may come from trial and error, a basic understanding of how to troubleshoot devices can strengthen a student’s ability to use technology more successfully in education and the future.
Digital Researching
Gone are the days of shelves of encyclopedias being considered a comprehensive resource for research needs. Interconnected communication has made digital research much more complete and accessible, though this comes with a different set of challenges. Elementary students should begin to understand how to complete digital research, validate the trustworthiness of resources, cite their sources and utilize information without plagiarizing or otherwise misusing content.
Online Safety
Arguably, one of the most important digital skills elementary students should learn is online safety . Digital threats evolve quickly, and it would be difficult for curriculum to evolve quickly enough to keep up with the latest threats, not to mention predicting threats the students may face in the future. Instead, students should learn the concepts of online safety, including keeping personally identifiable and sensitive information private, avoiding and managing cyberbullying, handling inappropriate or uncomfortable information, understanding the implications of their digital footprints, as well as recognizing technology’s effects on mental health and how to mitigate these risks.
Final Thoughts
Designing computer literacy curriculum for elementary students is an important responsibility because of its far-reaching effects. This makes it critical not only in building a foundation for future digital skills, but also in enabling student safety, equity, and success now and in future.
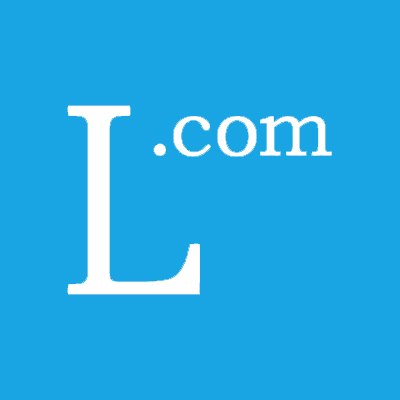
Learning.com Team
Staff Writers
Founded in 1999, Learning.com provides educators with solutions to prepare their students with critical digital skills. Our web-based curriculum for grades K-12 engages students as they learn keyboarding, online safety, applied productivity tools, computational thinking, coding and more.
Further Reading
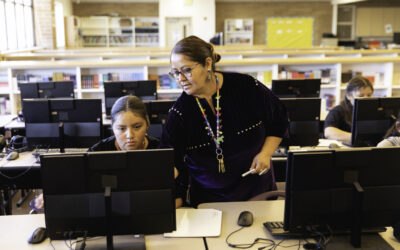
- CIPA Compliance Made Easy with Learning.com
by Rachel Minton | Jul 30, 2024
The Children’s Internet Protection Act (CIPA), enacted by Congress in 2000, addresses concerns about children’s access to harmful online content. It...
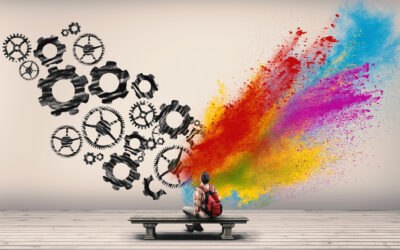
- Students as Logical Thinkers: How Computer Science Teaches Real-World Skills
by Lcom Team | Jul 23, 2024
There is little debate that computer science education has become an imperative curriculum for future-ready students. From modern professions and...
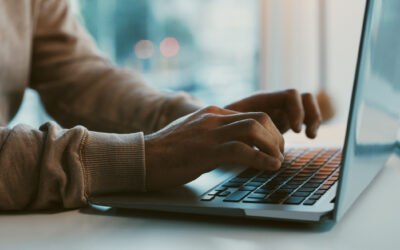
- The Digital Readiness Divide in Education
by Lcom Team | Jul 16, 2024
Following access and connectivity, the second digital divide refers to digital readiness, or the skillset that enables people to use technology as...
Quick Links
- Request More Info
Recent news & Articles
- How Were the TEKS Created & What is Its Significance?
- Pursuing Digital Equity Through Access & Opportunity – All Means All
Academia.edu no longer supports Internet Explorer.
To browse Academia.edu and the wider internet faster and more securely, please take a few seconds to upgrade your browser .
Enter the email address you signed up with and we'll email you a reset link.
- We're Hiring!
- Help Center
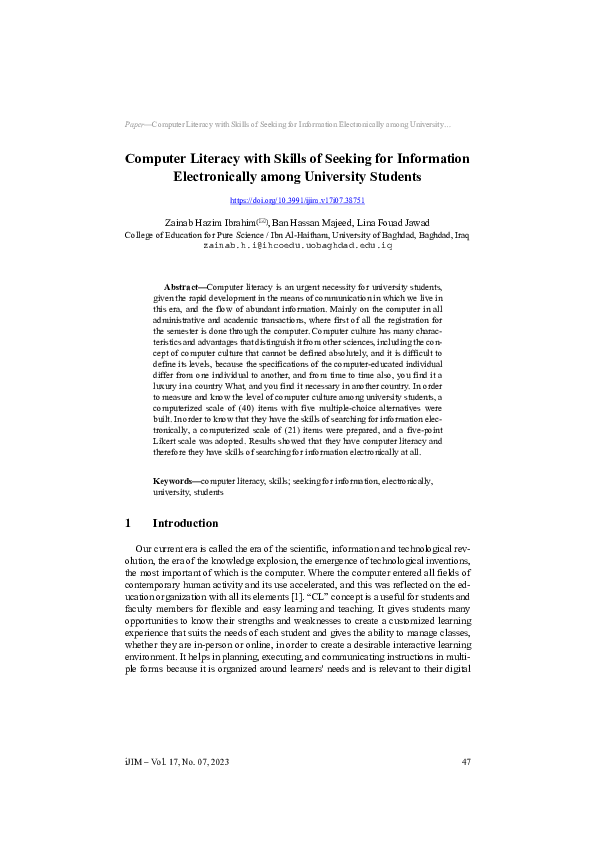
Computer Literacy with Skills of Seeking for Information Electronically among University Students

International Journal of Interactive Mobile Technologies (iJIM)
Computer literacy is an urgent necessity for university students, given the rapid development in the means of communication in which we live in this era, and the flow of abundant information. Mainly on the computer in all administrative and academic transactions, where first of all the registration for the semester is done through the computer. Computer culture has many characteristics and advantages that distinguish it from other sciences, including the concept of computer culture that cannot be defined absolutely, and it is difficult to define its levels, because the specifications of the computer-educated individual differ from one individual to another, and from time to time also, you find it a luxury in a country What, and you find it necessary in another country. In order to measure and know the level of computer culture among university students, a computerized scale of (40) items with five multiple-choice alternatives were built. In order to know that they have the skills of...
Related Papers
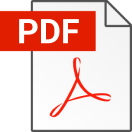
Mehmet güçlü
As technology advances, its impacts on people’s lives have become more visible. Therefore the dependency on technology has increased in time. Computers are of the most outstanding ones of the technological devices which become part of daily routines. To be able to make use of such a facility, recognizing the potential role of computers and being technologically literate are essential. Computer skills must be improved to become technologically proficient and to prevent the prospect negative situations in the career. In the recent years, education has started to be one of the fields which uses computer based technology intensively. Thus, having computer skills and using computer based technology effectively during the classes have started to become important aspects for teachers. In this study, it was tried to find out the computer skills of university students and their experience levels of using defined software.
Issues of Information Systems
Viktorija Florjancic
Atilla Bingöl
The purpose of this study is to identify the first-year university students’ skills in using computers and their attitudes towards computers and investigate them in terms of the variable of score type of their study fields or programs. To that end, “Scale for Skills in Using Computers and Attitudes towards Computers” consisting of 26 items and “Demographic Information Form” consisting of 7 items were applied online to the first-year students of Fırat University and 418 responses were assessed. According to the results obtained by data analysis, the first-year students of Fırat University have moderate-level perceptions on Skills in Using Computers and Attitudes towards Computers. While the students’ skills in using computers and their attitudes towards computers differ in terms of their study fields or programs, they don’t show a significant difference when analysed by the score averages of the perceptions of Scale for Skills in Using Computers and Attitudes towards Computers and the variable of score type of study field or program.
Turkish Online Journal of Educational Technology
Jacek Uziak
The aim of the study was to investigate access and use of computers and internet by students during their studies. The results are based on a survey conducted in 2009-2012 on groups of 320 to 405 students (each year) from two universities in eastern Poland. It was concluded that during the period under study access of students to computers and internet was at a relatively high level. In most of the years considered, there were statically significant differences in computer ownership and internet access between students from rural and urban areas. It was revealed that in students' opinion the application of ICT by lecturers in the courses' delivery did not change significantly since 2009. INTRODUCTION Computer and online use has increased significantly in the recent years and today it is a major component in modern society. University students all over the world use information communication technology in their studies. It is due not only to the advances in computer technolog...
Science Park Research Organization & Counselling
Nowadays; the usage of technology is not a privilege but an obligation. Technological developments influence structures and functions of educational institutions. It is also expected from the teachers that they integrate technology in their lessons in order to educate the individuals of information society. This research has covered 145(68 female, 78 male) students, studying in Near East University Faculty of Education. The Computer Skills Scale developed by Güçlü (2010) was used as a data collecting tool. Data were analysed using SPSS software program. In this study, students' computer skills were investigated; the variations in the relationships between computer skills and (a) gender, (b) family's net monthly income, (c) presence of computers at home, (d) presence of a computer laboratory at school and (e) parents' computer skills were examined. Frequency analysis, percentage and mean calculations were used. In addition, t-test and multi-variate analysis were used to look at the relationship between different variables. As a result of this study, a statistically significant relationship between computer skills of students who had a computer at home and computer skills of those who didn't have a computer at home were found.
Alma Selmani , European Scientific Journal ESJ
Technological developments that occurred in the late twentieth century and early twenty-first century, increased the use of technology in almost every aspect of human life and also its importance. The evolution of technology has meant that today anyone can use it at any time: everyone can have access to the Internet for different purposes. This thesis will focus on learning technology in higher education in Albania and specifically will use a survey on the use of technology by students of the Faculty of Economy during their studies. The main purpose of this work is to examine how much support there is and how students use technology in their learning process. The task has three main objectives: • To determine the attitudes and the degree of acceptance of technological change from students of faculty • To determine the extent of use of technologies during their studies, analyzing the types of technology frequently used by. • To determine how e-learning is recognized and how necessary is its usage by students's perspective. The methodology used is a qualitative analysis conducted through the distribution of questionnaires to students of faculty, as the main stakeholders of this research. Finally, from the analysis conducted it is concluded that students prefer technology; the most used technology is the laptop, less used is Instant Messaging and recognition of the concept of e-learning is at the average level, but still seen as a possibility to have a better study. Introduction Technology can be defined as the key word of this early twenty-first century. Technological developments that have occurred in the late twentieth century, where we can mention the invention of the airplane, telephone, computer and internet; and developments of the twenty one century, like:
Informacios Tarsadalom
Dubravka Valic Nedeljkovic
jozef bushati
SHS Web of Conferences
Loading Preview
Sorry, preview is currently unavailable. You can download the paper by clicking the button above.
RELATED TOPICS
- We're Hiring!
- Help Center
- Find new research papers in:
- Health Sciences
- Earth Sciences
- Cognitive Science
- Mathematics
- Computer Science
- Academia ©2024
Information
- Author Services
Initiatives
You are accessing a machine-readable page. In order to be human-readable, please install an RSS reader.
All articles published by MDPI are made immediately available worldwide under an open access license. No special permission is required to reuse all or part of the article published by MDPI, including figures and tables. For articles published under an open access Creative Common CC BY license, any part of the article may be reused without permission provided that the original article is clearly cited. For more information, please refer to https://www.mdpi.com/openaccess .
Feature papers represent the most advanced research with significant potential for high impact in the field. A Feature Paper should be a substantial original Article that involves several techniques or approaches, provides an outlook for future research directions and describes possible research applications.
Feature papers are submitted upon individual invitation or recommendation by the scientific editors and must receive positive feedback from the reviewers.
Editor’s Choice articles are based on recommendations by the scientific editors of MDPI journals from around the world. Editors select a small number of articles recently published in the journal that they believe will be particularly interesting to readers, or important in the respective research area. The aim is to provide a snapshot of some of the most exciting work published in the various research areas of the journal.
Original Submission Date Received: .
- Active Journals
- Find a Journal
- Proceedings Series
- For Authors
- For Reviewers
- For Editors
- For Librarians
- For Publishers
- For Societies
- For Conference Organizers
- Open Access Policy
- Institutional Open Access Program
- Special Issues Guidelines
- Editorial Process
- Research and Publication Ethics
- Article Processing Charges
- Testimonials
- Preprints.org
- SciProfiles
- Encyclopedia
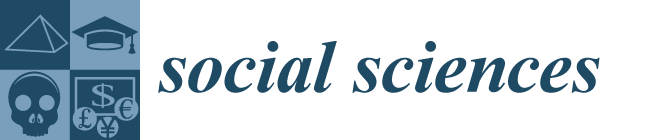
Article Menu
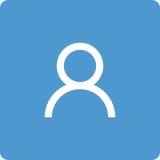
- Subscribe SciFeed
- Recommended Articles
- Author Biographies
- Google Scholar
- on Google Scholar
- Table of Contents
Find support for a specific problem in the support section of our website.
Please let us know what you think of our products and services.
Visit our dedicated information section to learn more about MDPI.
JSmol Viewer
Impacts of generative artificial intelligence in higher education: research trends and students’ perceptions.

1. Introduction
2. materials and methods.
- “Generative Artificial Intelligence” or “Generative AI” or “Gen AI”, AND;
- “Higher Education” or “University” or “College” or “Post-secondary”, AND;
- “Impact” or “Effect” or “Influence”.
- Q1— Does GenAI have more positive or negative effects on higher education? Options (to choose one): 1. It has more negative effects than positives; 2. It has more positive effects than negative; 3. There is a balance between positive and negative effects; 4. Don’t know.
- Q2— Identify the main positive effect of Gen AI in an academic context . Open-ended question.
- Q3— Identify the main negative effect of Gen AI in an academic context . Open-ended question.
3.1. Impacts of Gen AI in HE: Research Trends
3.1.1. he with gen ai, the key role that pedagogy must play, new ways to enhance the design and implementation of teaching and learning activities.
- Firstly, prompting in teaching should be prioritized as it plays a crucial role in developing students’ abilities. By providing appropriate prompts, educators can effectively guide students toward achieving their learning objectives.
- Secondly, configuring reverse prompting within the capabilities of Gen AI chatbots can greatly assist students in monitoring their learning progress. This feature empowers students to take ownership of their education and fosters a sense of responsibility.
- Furthermore, it is essential to embed digital literacy in all teaching and learning activities that aim to leverage the potential of the new Gen AI assistants. By equipping students with the necessary skills to navigate and critically evaluate digital resources, educators can ensure that they are prepared for the digital age.
The Student’s Role in the Learning Experience
The key teacher’s role in the teaching and learning experience, 3.1.2. assessment in gen ai/chatgpt times, the need for new assessment procedures, 3.1.3. new challenges to academic integrity policies, new meanings and frontiers of misconduct, personal data usurpation and cheating, 3.2. students’ perceptions about the impacts of gen ai in he.
- “It harms the learning process”: ▪ “What is generated by Gen AI has errors”; ▪ “Generates dependence and encourages laziness”; ▪ “Decreases active effort and involvement in the learning/critical thinking process”.
4. Discussion
- Training: providing training for both students and teachers on effectively using and integrating Gen AI technologies into teaching and learning practices.
- Ethical use and risk management: developing policies and guidelines for ethical use and risk management associated with Gen AI technologies.
- Incorporating AI without replacing humans: incorporating AI technologies as supplementary tools to assist teachers and students rather than replacements for human interaction.
- Continuously enhancing holistic competencies: encouraging the use of AI technologies to enhance specific skills, such as digital competence and time management, while ensuring that students continue to develop vital transferable skills.
- Fostering a transparent AI environment: promoting an environment in which students and teachers can openly discuss the benefits and concerns associated with using AI technologies.
- Data privacy and security: ensuring data privacy and security using AI technologies.
- The dynamics of technological support to align with the most suitable Gen AI resources;
- The training policy to ensure that teachers, students, and academic staff are properly trained to utilize the potential of Gen AI and its tools;
- Security and data protection policies;
- Quality and ethical action policies.
5. Conclusions
- Database constraints: the analysis is based on existing publications in SCOPUS and the Web of Science, potentially omitting relevant research from other sources.
- Inclusion criteria: due to the early stage of scientific production on this topic, all publications were included in the analysis, rather than focusing solely on articles from highly indexed journals and/or with a high number of citations as recommended by bibliometric and systematic review best practices.
- Dynamic landscape: the rate of publications on Gen AI has been rapidly increasing and diversifying in 2024, highlighting the need for ongoing analysis to track trends and changes in scientific thinking.
Author Contributions
Institutional review board statement, informed consent statement, data availability statement, conflicts of interest.
- Akakpo, Martin Gameli. 2023. Skilled for the Future: Information Literacy for AI Use by University Students in Africa and the Role of Librarians. Internet Reference Services Quarterly 28: 19–26. [ Google Scholar ] [ CrossRef ]
- AlAfnan, Mohammad Awad, Samira Dishari, Marina Jovic, and Koba Lomidze. 2023. ChatGPT as an Educational Tool: Opportunities, Challenges, and Recommendations for Communication, Business Writing, and Composition Courses. Journal of Artificial Intelligence and Technology 3: 60–68. [ Google Scholar ] [ CrossRef ]
- Almaraz-López, Cristina, Fernando Almaraz-Menéndez, and Carmen López-Esteban. 2023. Comparative Study of the Attitudes and Perceptions of University Students in Business Administration and Management and in Education toward Artificial Intelligence. Education Sciences 13: 609. [ Google Scholar ] [ CrossRef ]
- Al-Zahrani, Abdulrahman. 2023. The impact of generative AI tools on researchers and research: Implications for academia in higher education. Innovations in Education and Teaching International , 1–15. [ Google Scholar ] [ CrossRef ]
- Athilingam, Ponrathi, and Hong-Gu He. 2023. ChatGPT in nursing education: Opportunities and challenges. Teaching and Learning in Nursing 19: 97–101. [ Google Scholar ] [ CrossRef ]
- Álvarez-Álvarez, Carmen, and Samuel Falcon. 2023. Students’ preferences with university teaching practices: Analysis of testimonials with artificial intelligence. Educational Technology Research and Development 71: 1709–24. [ Google Scholar ] [ CrossRef ]
- Bannister, Peter, Elena Alcalde Peñalver, and Alexandra Santamaría Urbieta. 2023. Transnational higher education cultures and generative AI: A nominal group study for policy development in English medium instruction. Journal for Multicultural Education . ahead-of-print . [ Google Scholar ] [ CrossRef ]
- Bearman, Margaret, and Rola Ajjawi. 2023. Learning to work with the black box: Pedagogy for a world with artificial intelligence. British Journal of Educational Technology 54: 1160–73. [ Google Scholar ] [ CrossRef ]
- Boháček, Matyas. 2023. The Unseen A+ Student: Evaluating the Performance and Detectability of Large Language Models in the Classroom. CEUR Workshop Proceedings 3487: 89–100. Available online: https://openreview.net/pdf?id=9ZKJLYg5EQ (accessed on 7 January 2024).
- Chan, Cecilia Ka Yuk. 2023. A comprehensive AI policy education framework for university teaching and learning. International Journal of Educational Technology in Higher Education 20: 38. [ Google Scholar ] [ CrossRef ]
- Chan, Cecilia Ka Yuk, and Wenjie Hu. 2023. Students’ voices on generative AI: Perceptions, benefits, and challenges in higher education. International Journal of Educational Technology in Higher Education 20: 43. [ Google Scholar ] [ CrossRef ]
- Chan, Cecilia Ka Yuk, and Wenxin Zhou. 2023. An expectancy value theory (EVT) based instrument for measuring student perceptions of generative AI. Smart Learning Environments 10: 64. [ Google Scholar ] [ CrossRef ]
- Chang, Daniel H., Michael Pin-Chuan Lin, Shiva Hajian, and Quincy Q. Wang. 2023. Educational Design Principles of Using AI Chatbot That Supports Self-Regulated Learning in Education: Goal Setting, Feedback, and Personalization. Sustainability 15: 12921. [ Google Scholar ] [ CrossRef ]
- Chiu, Thomas. 2023. The impact of Generative AI (GenAI) on practices, policies and research direction in education: A case of ChatGPT and Midjourney. Interactive Learning Environments , 1–17. [ Google Scholar ] [ CrossRef ]
- Chun, John, and Katherine Elkins. 2023. The Crisis of Artificial Intelligence: A New Digital Humanities Curriculum for Human-Centred AI. International Journal of Humanities and Arts Computing 17: 147–67. [ Google Scholar ] [ CrossRef ]
- Cowling, Michael, Joseph Crawford, Kelly-Ann Allen, and Michael Wehmeyer. 2023. Using leadership to leverage ChatGPT and artificial intelligence for undergraduate and postgraduate research supervision. Australasian Journal of Educational Technology 39: 89–103. [ Google Scholar ] [ CrossRef ]
- Crawford, Joseph, Carmen Vallis, Jianhua Yang, Rachel Fitzgerald, Christine O’Dea, and Michael Cowling. 2023a. Editorial: Artificial Intelligence is Awesome, but Good Teaching Should Always Come First. Journal of University Teaching & Learning Practice 20: 01. [ Google Scholar ] [ CrossRef ]
- Crawford, Joseph, Michael Cowling, and Kelly-Ann Allen. 2023b. Leadership is needed for ethical ChatGPT: Character, assessment, and learning using artificial intelligence (AI). Journal of University Teaching & Learning Practice 20: 02. [ Google Scholar ] [ CrossRef ]
- Currie, Geoffrey. 2023a. A Conversation with ChatGPT. Journal of Nuclear Medicine Technology 51: 255–60. [ Google Scholar ] [ CrossRef ] [ PubMed ]
- Currie, Geoffrey. 2023b. GPT-4 in Nuclear Medicine Education: Does It Outperform GPT-3.5? Journal of Nuclear Medicine Technology 51: 314–17. [ Google Scholar ] [ CrossRef ] [ PubMed ]
- Currie, Geoffrey, and Kym Barry. 2023. ChatGPT in Nuclear Medicine Education. Journal of Nuclear Medicine Technology 51: 247–54. [ Google Scholar ] [ CrossRef ]
- Currie, Geoffrey, Clare Singh, Tarni Nelson, Caroline Nabasenja, Yazan Al-Hayek, and Kelly Spuur. 2023. ChatGPT in medical imaging higher education. Radiography 29: 792–99. [ Google Scholar ] [ CrossRef ]
- Dai, Yun, Ang Liu, and Cher P. Lim. 2023. Reconceptualizing Chatgpt and Generative AI as a Student-driven Innovation in Higher Education. Procedia CIRP Volume 119: 84–90. [ Google Scholar ] [ CrossRef ]
- Dogru, Tarik, Nathana Line, Lydia Hanks, Fulya Acikgoz, Je’Anna Abbott, Selim Bakir, Adiyukh Berbekova, Anil Bilgihan, Ali Iskender, Murat Kizildag, and et al. 2023. The implications of generative artificial intelligence in academic research and higher education in tourism and hospitality. Tourism Economics 30: 1083–94. [ Google Scholar ] [ CrossRef ]
- Duong, Cong Doanh, Trong Nghia Vu, and Thi Viet Nga Ngo. 2023. Applying a modified technology acceptance model to explain higher education students’ usage of ChatGPT: A serial multiple mediation model with knowledge sharing as a moderator. The International Journal of Management Education 21: 100883. [ Google Scholar ] [ CrossRef ]
- Eager, Bronwyn, and Ryan Brunton. 2023. Prompting Higher Education Towards AI-Augmented Teaching and Learning Practice. Journal of University Teaching & Learning Practice 20: 5. [ Google Scholar ] [ CrossRef ]
- Elkhodr, Mahmoud, Ergun Gide, Robert Wu, and Omar Darwish. 2023. ICT students’ perceptions towards ChatGPT: An experimental reflective lab analysis. STEM Education 3: 70–88. [ Google Scholar ] [ CrossRef ]
- Farrelly, Tom, and Nick Baker. 2023. Generative Artificial Intelligence: Implications and Considerations for Higher Education Practice. Education Sciences 13: 1109. [ Google Scholar ] [ CrossRef ]
- Farrokhnia, Mohammadreza, Seyyed Banihashem, Seyyed Kazem Banihashem, Omid Noroozi, and Arjen Wals. 2023. A SWOT analysis of ChatGPT: Implications for educational practice and research. Innovations in Education and Teaching International 61: 460–74. [ Google Scholar ] [ CrossRef ]
- Gong, Furong. 2023. The Impact of Generative AI like ChatGPT on Digital Literacy Education in University Libraries. Documentation, Information & Knowledge 40: 97–106, 156. [ Google Scholar ] [ CrossRef ]
- Han, Bingyi, Sadia Nawaz, George Buchanan, and Dana McKay. 2023. Ethical and Pedagogical Impacts of AI in Education. In Artificial Intelligence in Education . Edited by Ning Wang, Genaro Rebolledo-Mendez, Noboru Matsuda, Olga Santos and Vania Dimitrova. Lecture Notes in Computer Science. Cham: Springer, pp. 667–73. [ Google Scholar ] [ CrossRef ]
- Hassoulas, Athanasios, Ned Powell, Lindsay Roberts, Katja Umla-Runge, Laurence Gray, and Marcus J. Coffey. 2023. Investigating marker accuracy in differentiating between university scripts written by students and those produced using ChatGPT. Journal of Applied Learning and Teaching 6: 71–77. [ Google Scholar ] [ CrossRef ]
- Hernández-Leo, Davinia. 2023. ChatGPT and Generative AI in Higher Education: User-Centered Perspectives and Implications for Learning Analytics. CEUR Workshop Proceedings , 1–6. Available online: https://ceur-ws.org/Vol-3542/paper2.pdf (accessed on 7 January 2024).
- Hidayat-ur-Rehman, Imdadullah, and Yasser Ibrahim. 2023. Exploring factors influencing educators’ adoption of ChatGPT: A mixed method approach. Interactive Technology and Smart Education . ahead-of-print . [ Google Scholar ] [ CrossRef ]
- Ilieva, Galina, Tania Yankova, Stanislava Klisarova-Belcheva, Angel Dimitrov, Marin Bratkov, and Delian Angelov. 2023. Effects of Generative Chatbots in Higher Education. Information 14: 492. [ Google Scholar ] [ CrossRef ]
- Javaid, Mohd, Abid Haleem, Ravi Pratap Singh, Shahbaz Khan, and Haleem Ibrahim. 2023. Unlocking the opportunities through ChatGPT Tool towards ameliorating the education system. Bench Council Transactions on Benchmarks, Standards and Evaluations 3: 100115. [ Google Scholar ] [ CrossRef ]
- Kaplan-Rakowski, Regina, Kimberly Grotewold, Peggy Hartwick, and Kevin Papin. 2023. Generative AI and Teachers’ Perspectives on Its Implementation in Education. Journal of Interactive Learning Research 34: 313–38. Available online: https://www.learntechlib.org/primary/p/222363/ (accessed on 7 January 2024).
- Karunaratne, Thashmee, and Adenike Adesina. 2023. Is it the new Google: Impact of ChatGPT on Students’ Information Search Habits. Paper presented at the European Conference on e-Learning (ECEL 2023), Pretoria, South Africa, October 26–27; pp. 147–55. [ Google Scholar ] [ CrossRef ]
- Kelly, Andrew, Miriam Sullivan, and Katrina Strampel. 2023. Generative artificial intelligence: University student awareness, experience, and confidence in use across disciplines. Journal of University Teaching & Learning Practice 20: 12. [ Google Scholar ] [ CrossRef ]
- Kohnke, Lucas, Benjamin Luke Moorhouse, and Di Zou. 2023. Exploring generative artificial intelligence preparedness among university language instructors: A case study. Computers and Education: Artificial Intelligence 5: 100156. [ Google Scholar ] [ CrossRef ]
- Laker, Lauren, and Mark Sena. 2023. Accuracy and detection of student use of ChatGPT in business analytics courses. Issues in Information Systems 24: 153–63. [ Google Scholar ] [ CrossRef ]
- Lemke, Claudia, Kathrin Kirchner, Liadan Anandarajah, and Florian Herfurth. 2023. Exploring the Student Perspective: Assessing Technology Readiness and Acceptance for Adopting Large Language Models in Higher Education. Paper presented at the European Conference on e-Learning, (ECEL 2023), Pretoria, South Africa, October 26–27; pp. 156–64. [ Google Scholar ] [ CrossRef ]
- Limna, Pongsakorn, Tanpat Kraiwanit, Kris Jangjarat, and Prapasiri Klayklung. 2023a. The use of ChatGPT in the digital era: Perspectives on chatbot implementation. Journal of Applied Learning and Teaching 6: 64–74. [ Google Scholar ] [ CrossRef ]
- Limna, Pongsakorn, Tanpat Kraiwanit, Kris Jangjarat, and Yarnaphat Shaengchart. 2023b. Applying ChatGPT as a new business strategy: A great power comes with great responsibility [Special issue]. Corporate & Business Strategy Review 4: 218–26. [ Google Scholar ] [ CrossRef ]
- Lopezosa, Carlos, Carles Lluís Codina, Carles Pont-Sorribes, and Mari Vállez. 2023. Use of Generative Artificial Intelligence in the Training of Journalists: Challenges, Uses and Training Proposal. Profesional De La información Information Professional 32: 1–12. [ Google Scholar ] [ CrossRef ]
- Martineau, Kim. 2023. What Is Generative AI? IBM Research Blog . April 20. Available online: https://research.ibm.com/blog/what-is-generative-AI (accessed on 7 January 2024).
- Mondal, Himel, Shaikat Mondal, and Indrashis Podder. 2023. Using ChatGPT for Writing Articles for Patients’ Education for Dermatological Diseases: A Pilot Study. Indian Dermatology Online Journal 14: 482–86. [ Google Scholar ] [ CrossRef ]
- Moorhouse, Benjamin, Marie Alina Wan, and Yuwei Wan. 2023. Generative AI tools and assessment: Guidelines of the world’s top-ranking universities. Computers and Education Open 5: 100151. [ Google Scholar ] [ CrossRef ]
- Overono, Acacia L., and Annie Ditta. 2023. The Rise of Artificial Intelligence: A Clarion Call for Higher Education to Redefine Learning and Reimagine Assessment. College Teaching , 1–4. [ Google Scholar ] [ CrossRef ]
- Page, Matthew J., Joanne E. McKenzie, Patrick M. Bossuyt, Isabelle Boutron, Tammy C. Hoffmann, Cynthia D. Mulrow, Larissa Shamseer, Jennifer M. Tetzlaff, Elie A. Akl, Sue E. Brennan, and et al. 2021. The PRISMA 2020 statement: An updated guideline for reporting systematic reviews. BMJ 372: n71. [ Google Scholar ] [ CrossRef ]
- Pechenkina, Ekaterina. 2023. Artificial intelligence for good? Challenges and possibilities of AI in higher education from a data justice perspective. In Higher Education for Good: Teaching and Learning Futures . Edited by Laura Czerniewicz and Catherine Cronin. Cambridge, UK: Open Book Publishers, pp. 239–66. [ Google Scholar ] [ CrossRef ]
- Perkins, Mike, Jasper Roe, Darius Postma, James McGaughran, and Don Hickerson. 2023. Detection of GPT-4 Generated Text in Higher Education: Combining Academic Judgement and Software to Identify Generative AI Tool Misuse. Journal of Academic Ethics 22: 89–113. [ Google Scholar ] [ CrossRef ]
- Pitso, Teboho. 2023. Post-COVID-19 Higher Learning: Towards Telagogy, A Web-Based Learning Experience. IAFOR Journal of Education 11: 39–59. [ Google Scholar ] [ CrossRef ]
- Plata, Sterling, Maria Ana De Guzman, and Arthea Quesada. 2023. Emerging Research and Policy Themes on Academic Integrity in the Age of Chat GPT and Generative AI. Asian Journal of University Education 19: 743–58. [ Google Scholar ] [ CrossRef ]
- Rudolph, Jürgen, Samson Tan, and Shannon Tan. 2023a. War of the chatbots: Bard, Bing Chat, ChatGPT, Ernie and beyond. The new AI gold rush and its impact on higher education. Journal of Applied Learning and Teaching 6: 364–89. [ Google Scholar ] [ CrossRef ]
- Rudolph, Jürgen, Samson Tan, and Shannon Tan. 2023b. ChatGPT: Bullshit spewer or the end of traditional assessments in higher education? Journal of Applied Learning and Teaching 6: 342–63. [ Google Scholar ] [ CrossRef ]
- Ryall, Adelle, and Stephen Abblitt. 2023. “A Co-Pilot for Learning Design?”: Perspectives from Learning Designers on the Uses, Challenges, and Risks of Generative Artificial Intelligence in Higher Education. In People, Partnerships and Pedagogies. Proceedings ASCILITE 2023 . Edited by Thomas Cochrane, Vickel Narayan, Cheryl Brown, MacCallum Kathryn, Elisa Bone, Christopher Deneen, Robert Vanderburg and Brad Hurren. Christchurch: Te Pae Conference Center, pp. 525–30. [ Google Scholar ] [ CrossRef ]
- Santiago, Cereneo S., Steve I. Embang, Ricky B. Acanto, Kem Warren P. Ambojia, Maico Demi B. Aperocho, Benedicto B. Balilo, Erwin L. Cahapin, Marjohn Thomas N. Conlu, Samson M. Lausa, Ester Y. Laput, and et al. 2023. Utilization of Writing Assistance Tools in Research in Selected Higher Learning Institutions in the Philippines: A Text Mining Analysis. International Journal of Learning, Teaching and Educational Research 22: 259–84. [ Google Scholar ] [ CrossRef ]
- Solopova, Veronika, Eiad Rostom, Fritz Cremer, Adrian Gruszczynski, Sascha Witte, Chengming Zhang, Fernando Ramos López, Lea Plößl, Florian Hofmann, Ralf Romeike, and et al. 2023. PapagAI: Automated Feedback for Reflective Essays. In KI 2023: Advances in Artificial Intelligence. KI 2023 . Lecture Notes in Computer Science (Including Subseries Lecture Notes in Artificial Intelligence and Lecture Notes in Bioinformatics). Cham: Springer, vol. 14236, pp. 198–206. [ Google Scholar ] [ CrossRef ]
- Sridhar, Pragnya, Aidan Doyle, Arav Agarwal, Christopher Bogart, Jaromir Savelka, and Majd Sakr. 2023. Harnessing LLMs in Curricular Design: Using GPT-4 to Support Authoring of Learning Objectives. CEUR Workshop Proceedings 3487: 139–50. [ Google Scholar ]
- Sullivan, Miriam, Andrew Kelly, and Paul McLaughlan. 2023. ChatGPT in higher education: Considerations for academic integrity and student learning. Journal of Applied Learning and Teaching 6: 31–40. [ Google Scholar ] [ CrossRef ]
- Tominc, Polona, and Maja Rožman. 2023. Artificial Intelligence and Business Studies: Study Cycle Differences Regarding the Perceptions of the Key Future Competences. Education Sciences 13: 580. [ Google Scholar ] [ CrossRef ]
- van den Berg, Geesje, and Elize du Plessis. 2023. ChatGPT and Generative AI: Possibilities for Its Contribution to Lesson Planning, Critical Thinking and Openness in Teacher Education. Education Sciences 13: 998. [ Google Scholar ] [ CrossRef ]
- Walczak, Krzysztof, and Wojciech Cellary. 2023. Challenges for higher education in the era of widespread access to Generative AI. Economics and Business Review 9: 71–100. [ Google Scholar ] [ CrossRef ]
- Wang, Ting, Brady D. Lund, Agostino Marengo, Alessandro Pagano, Nishith Reddy Mannuru, Zoë A. Teel, and Jenny Pange. 2023. Exploring the Potential Impact of Artificial Intelligence (AI) on International Students in Higher Education: Generative AI, Chatbots, Analytics, and International Student Success. Applied Sciences 13: 6716. [ Google Scholar ] [ CrossRef ]
- Watermeyer, Richard, Lawrie Phipps, Donna Lanclos, and Cathryn Knight. 2023. Generative AI and the Automating of Academia. Postdigital Science and Education 6: 446–66. [ Google Scholar ] [ CrossRef ]
- Wolf, Leigh, Tom Farrelly, Orna Farrell, and Fiona Concannon. 2023. Reflections on a Collective Creative Experiment with GenAI: Exploring the Boundaries of What is Possible. Irish Journal of Technology Enhanced Learning 7: 1–7. [ Google Scholar ] [ CrossRef ]
- Yilmaz, Ramazan, and Fatma Gizem Karaoglan Yilmaz. 2023. The effect of generative artificial intelligence (AI)-based tool use on students’ computational thinking skills, programming self-efficacy and motivation. Computers and Education: Artificial Intelligence 4: 100147. [ Google Scholar ] [ CrossRef ]
- Zawiah, Mohammed, Fahmi Y. Al-Ashwal, Lobna Gharaibeh, Rana Abu Farha, Karem H. Alzoubi, Khawla Abu Hammour, Qutaiba A. Qasim, and Fahd Abrah. 2023. ChatGPT and Clinical Training: Perception, Concerns, and Practice of Pharm-D Students. Journal of Multidisciplinary Healthcare 16: 4099–110. [ Google Scholar ] [ CrossRef ]
Click here to enlarge figure
Selected Group of Students | Students Who Answered the Questionnaire | |||
---|---|---|---|---|
M | F | M | F | |
1st year | 59 | 5 | 34 | 2 |
2nd year | 36 | 5 | 29 | 4 |
1st year | 39 | 3 | 24 | 2 |
2nd year | 21 | 2 | 15 | 2 |
Country | N. | Country | N. | Country | N. | Country | N. |
---|---|---|---|---|---|---|---|
Australia | 16 | Italy | 2 | Egypt | 1 | South Korea | 1 |
United States | 7 | Saudi Arabia | 2 | Ghana | 1 | Sweden | 1 |
Singapore | 5 | South Africa | 2 | Greece | 1 | Turkey | 1 |
Hong Kong | 4 | Thailand | 2 | India | 1 | United Arab Emirates | 1 |
Spain | 4 | Viet Nam | 2 | Iraq | 1 | Yemen | 1 |
United Kingdom | 4 | Bulgaria | 1 | Jordan | 1 | ||
Canada | 3 | Chile | 1 | Malaysia | 1 | ||
Philippines | 3 | China | 1 | Mexico | 1 | ||
Germany | 2 | Czech Republic | 1 | New Zealand | 1 | ||
Ireland | 2 | Denmark | 1 | Poland | 1 |
Country | N. | Country | N. | Country | N. | Country | N. |
---|---|---|---|---|---|---|---|
Singapore | 271 | United States | 15 | India | 2 | Iraq | 0 |
Australia | 187 | Italy | 11 | Turkey | 2 | Jordan | 0 |
Hong Kong | 37 | United Kingdom | 6 | Denmark | 1 | Poland | 0 |
Thailand | 33 | Canada | 6 | Greece | 1 | United Arab Emirates | 0 |
Philippines | 31 | Ireland | 6 | Sweden | 1 | Yemen | 0 |
Viet Nam | 29 | Spain | 6 | Saudi Arabia | 1 | ||
Malaysia | 29 | South Africa | 6 | Bulgaria | 1 | ||
South Korea | 29 | Mexico | 3 | Czech Republic | 0 | ||
China | 17 | Chile | 3 | Egypt | 0 | ||
New Zealand | 17 | Germany | 2 | Ghana | 0 |
Categories | Subcategories | Nr. of Documents | References |
---|---|---|---|
HE with Gen AI | 15 | ( ); ( ); ( ); ( ); ( ); ( ); ( ); ( ); ( ); ( ); ( ); ( ); ( ); ( ); ( ); ( ); ( ); ( ). | |
15 | ( ); ( ); ( ); ( ); ( ); ( ); ( ); ( ); ( ); ( ); ( ); ( ); ( ); ( ); ( ); ( ). | ||
14 | ( ); ( ); ( ); ( ); ( ); ( ); ( ); ( ); ( ); ( ); ( ); ( ); ( ); ( ). | ||
8 | ( ); ( ); ( ); ( ); ( ); ( ); ( ); ( ). | ||
Assessment in Gen AI/ChatGPT times | 8 | ( ); ( ); ( ); ( ); ( ); ( ); ( ); ( ). | |
New challenges to academic integrity policies | 4 | ( ); ( ); ( ); ( ). |
Have You Tried Using a Gen AI Tool? | Nr. | % |
---|---|---|
Yes | 52 | 46.4% |
No | 60 | 53.6% |
Categories and Subcategories | % | Unit of Analysis (Some Examples) |
---|---|---|
1. Learning support: | ||
1.1. Helpful to solve doubts, to correct errors | 34.6% | |
1.2. Helpful for more autonomous and self-regulated learning | 19.2% | |
2. Helpful to carry out the academic assignments/individual or group activities | 17.3% | |
3. Facilitates research/search processes | ||
3.1. Reduces the time spent with research | 13.5% | |
3.2. Makes access to information easier | 9.6% | |
4. Reduction in teachers’ workload | 3.9% | |
5. Enables new teaching methods | 1.9% |
Categories and Subcategories | % | Unit of Analysis (Some Examples) |
---|---|---|
1. Harms the learning process: | ||
1.1. What is generated by Gen AI has errors | 13.5% | |
1.2. Generates dependence and encourages laziness | 15.4% | |
1.3. Decreases active effort and involvement in the learning/critical thinking process | 28.8% | |
2. Encourages plagiarism and incorrect assessment procedures | 17.3% | |
3. Reduces relationships with teachers and interpersonal relationships | 9.6% | |
4. No negative effect—as it will be necessary to have knowledge for its correct use | 7.7% | |
5. Don’t know | 7.7% |
The statements, opinions and data contained in all publications are solely those of the individual author(s) and contributor(s) and not of MDPI and/or the editor(s). MDPI and/or the editor(s) disclaim responsibility for any injury to people or property resulting from any ideas, methods, instructions or products referred to in the content. |
Share and Cite
Saúde, S.; Barros, J.P.; Almeida, I. Impacts of Generative Artificial Intelligence in Higher Education: Research Trends and Students’ Perceptions. Soc. Sci. 2024 , 13 , 410. https://doi.org/10.3390/socsci13080410
Saúde S, Barros JP, Almeida I. Impacts of Generative Artificial Intelligence in Higher Education: Research Trends and Students’ Perceptions. Social Sciences . 2024; 13(8):410. https://doi.org/10.3390/socsci13080410
Saúde, Sandra, João Paulo Barros, and Inês Almeida. 2024. "Impacts of Generative Artificial Intelligence in Higher Education: Research Trends and Students’ Perceptions" Social Sciences 13, no. 8: 410. https://doi.org/10.3390/socsci13080410
Article Metrics
Article access statistics, further information, mdpi initiatives, follow mdpi.
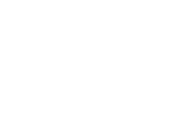
Subscribe to receive issue release notifications and newsletters from MDPI journals
Gender literacy through the making process: : A feminist pedagogy approach
New citation alert added.
This alert has been successfully added and will be sent to:
You will be notified whenever a record that you have chosen has been cited.
To manage your alert preferences, click on the button below.
New Citation Alert!
Please log in to your account
Information & Contributors
Bibliometrics & citations, view options, recommendations, intersections of gender and sexual minority status.
The present study refined existing bullying literature by examining differences in risk of three types of bullying victimization (offline only, cyberbullying only, and co-occurring victimization) for four different gender-sexual minority status groups ...
The Influence of Gender-Ethnic Intersectionality on Gender Stereotypes about IT Skills and Knowledge
One line of investigation in attempting to better understand the gender imbalance in the information technology (IT) field is to examine gender stereotypes about the skills and knowledge in the IT profession. A survey of 4046 university students in the ...
The development of gender differences in information and communication technology (ICT) literacy in middle adolescence
Information and communication technology (ICT) literacy represents an essential skill for adolescents to efficiently participate in a modern society. Previous research reported conflicting findings regarding gender differences in ICT ...
- A longitudinal studies with adolescents at ages 15 and 18 is presented.
Information
Published in.
Elsevier Science Publishers B. V.
Netherlands
Publication History
Author tags.
- Feminist pedagogy
- Design methods
- Genderless toys
- Research-article
Contributors
Other metrics, bibliometrics, article metrics.
- 0 Total Citations
- 0 Total Downloads
- Downloads (Last 12 months) 0
- Downloads (Last 6 weeks) 0
View options
Login options.
Check if you have access through your login credentials or your institution to get full access on this article.
Full Access
Share this publication link.
Copying failed.
Share on social media
Affiliations, export citations.
- Please download or close your previous search result export first before starting a new bulk export. Preview is not available. By clicking download, a status dialog will open to start the export process. The process may take a few minutes but once it finishes a file will be downloadable from your browser. You may continue to browse the DL while the export process is in progress. Download
- Download citation
- Copy citation
We are preparing your search results for download ...
We will inform you here when the file is ready.
Your file of search results citations is now ready.
Your search export query has expired. Please try again.
- Computer Science
- Computer and Society
- Digital Literacy
DIGITAL LITERACY OF STUDENTS AND ITS IMPROVEMENT AT THE UNIVERSITY
- Journal on Efficiency and Responsibility in Education and Science 7(2):26-32

- South-West University "Neofit Rilski"
Abstract and Figures

Discover the world's research
- 25+ million members
- 160+ million publication pages
- 2.3+ billion citations
- J Sci Educ Tech

- Astrid Ramadhanti

- Bello Sani Manzo
- Usman Abdulkadir Umar

- Dilva Hardila

- Internet High Educ

- COMPUT EDUC

- Peter Sloep

- Petter Karlström
- Recruit researchers
- Join for free
- Login Email Tip: Most researchers use their institutional email address as their ResearchGate login Password Forgot password? Keep me logged in Log in or Continue with Google Welcome back! Please log in. Email · Hint Tip: Most researchers use their institutional email address as their ResearchGate login Password Forgot password? Keep me logged in Log in or Continue with Google No account? Sign up
What Starts Here
, ph.d. program, master's programs, portfolio program in robotics, admissions & incoming students, current students, online programs & degrees, master's degrees, student experience, ut computer science lab announces way to make short-form content more accessible.
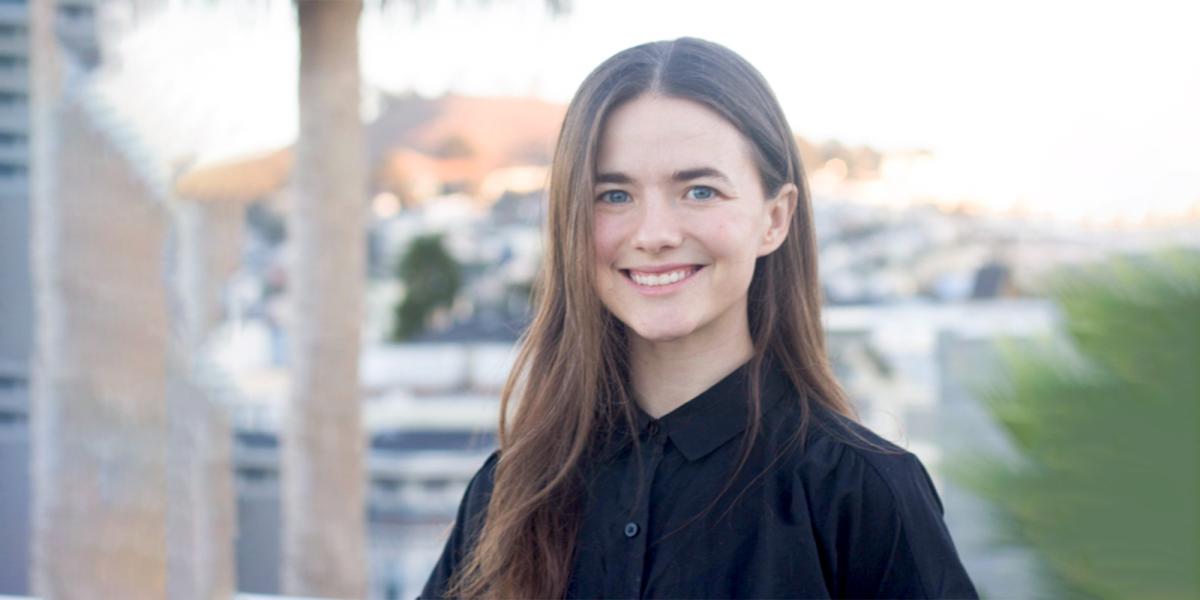
The UT computer science lab, with faculty member Amy Pavel and recent graduate Tess Van Daele at the forefront, has developed an AI system called ShortScribe to enhance accessibility for visually impaired users of short-form videos on platforms like TikTok and Instagram Reels. Pavel, an assistant computer science professor and co-author of the research paper, explained that the system utilizes AI technologies such as Optical Character Recognition, Automatic Speech Transmission, and GPT-4 to segment videos, transcribe speech, and create detailed audio descriptions. Van Daele, the first author of the research, hopes this work will inspire broader efforts to make digital content more accessible as new media formats emerge.
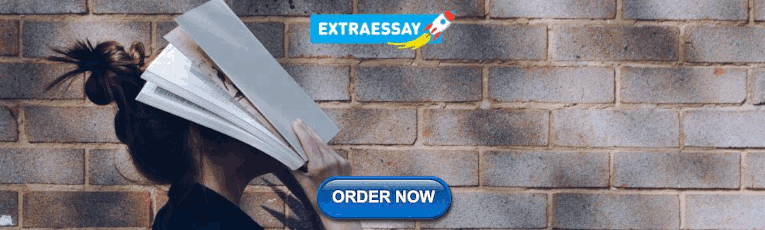
COMMENTS
2019-2020. This study determined t he level of computer literacy and its influence on the academic performance of Grade 10 students in. Silanga National High School under the District of ...
Computer literacy has been recognized for some time as important for life in modern society (Kozma 2003), and many countries have officially recognized the importance of developing computer literacy through schools.During the first decade of the twenty-first century, policy statements from many national education authorities asserted the importance of school students developing capabilities in ...
ICT Literacy in Grades 6 to 9. To address RQ1, we first considered the first-stage data of 6810 students and specified five models, which all included ICT literacy in Grade 6 (see Table 1).The associations between ICT literacy in Grade 6 and ICT literacy in Grade 9 ranged from β = 0.765 (SE = 0.020, p < .001) in M1 to β = 0.397 in M5 (SE = 0.030, p < .001).
Conceptualizing Computer Literacy The terms computer literacy, ICT literacy, and digital literacy have slightly different meanings but in practice are used interchangeably (Markauskaite 2006; Siddiq et al. 2016). This chapter refers to computer literacy but recognizes that the concept overlaps with other terms.
CL-AC1, Quinnipiac University. 275 Mt. Carmel Avenue. Hamden, CT 06518. [email protected]; [email protected]. ABSTRACT. Computing and technology departments often offer ...
Background Due to the increasing use of information and communication technology, computer-related skills are important for all students in order to participate in the digital age (Fraillon, J., Ainley, J., Schulz, W., Friedman, T. & Duckworth, D. (2019). Preparing for life in a digital world: IEA International Computer and Information Literacy Study 2018 International Report. Amsterdam ...
The results of the diagnostic analysis on students' computer literacy are presented in the article. The study involves students from Lithuanian universities and colleges (N=1004). Based on the study results attempts are made to identify and define the existing statistical types of students in connection with their attitudes towards a computer ...
Computer literacy is an urgent necessity for university students, given the rapid development in the means of communication in which we live in this era, and the flow of abundant information.
The International Computer and Information Literacy Study (ICILS 2013) provides, for the first time, information about students' computer and information literacy (CIL), as well as its acquisition, based on a computer-based test for students and background questionnaires. Among the 21 education systems that participated in ICILS 2013, there are 12 European countries, making secondary ...
1. Introduction. Over the last few decades, the concepts digital competence and digital literacy have been used more frequently and are increasingly discussed, particularly in policy documents and policy-related discussions related to "what kinds of skills and knowing people should have in a knowledge society, what to teach young people and how to do so" (Ilomäki, Paavola and Lakkala ...
2.2. Learning environments and motivations. The environment where students learn can affect their attitudes (Hsu and Huang, 2006).Studies have found that students achieve higher grades when they have a computer at home (Fairlie, 2012; Fairlie et al., 2010) and use it daily to facilitate their school work (Gu and Xu, 2019), suggesting that home computers improve educational outcomes and ...
ICT literacy, as a concept, integrates Internet literacy, Computer literacy and Information literacy. Here, this concept of ICT literacy will be adopted. The European Commission included in 2007 digital skills as one of the eight types of key competencies for lifelong learning, also known as the 21st century skills.
The learning assessments literature pro-vides a fruitful model for measuring computer assisted learning outcomes, while the computer literacy literature ex-amines some of the relevant student characteristics for measuring outcomes when computers are a component of the learning environment. The literature on the efficacy of CAL in the accounting ...
Results: The results showed that the 77.1% had personal computer. The total mean of students' computer literacy around six domains was 141.9±49.5 out of 240. The most familiarity with computers was the ability to it in internet (29.0±11.4) and the lowest was familiarity and using ability of hard ware (17.5±10.6).
Background At the Medical University of Vienna, most information for students is available only online. In 2005, an e-learning project was initiated and there are plans to introduce a learning management system. In this study, we estimate the level of students' computer skills, the number of students having difficulty with e-learning, and the number of students opposed to e-learning. Methods ...
computer literacy, which are required for educational purposes. Computer literacy appears to differ from context to context. The findings of previous research on computer literacy imply that the majority of students need training for the efficient use of computers and technology for educational purposes.
Abstract—Computer literacy is an urgent necessity for university students, given the rapid development in the means of communication in which we live in this era, and the flow of abundant ...
2014. 14. PDF. 1 Excerpt. The findings of the study revealed that the students needed to enhance the extent of their computer literacy in the areas of word processing, spreadsheet, presentation, and general computing, and the greater the extent, the higher their academic performance. This study determined the level of computer literacy and its ...
ASA Section on Survey Research Methods Computer Literacy of Adolescents in Grades 9 to 12: An Exploratory Study Christopher Sink Seattle Pacific University, School of Education Matthew Sink, Jonathan Stob, and Kevin Taniguchi Shoreline Christian School, WA Abstract This study conducted with 84 students in a small Christian high school examined gender differences in computer literacy skills.
Regarding AI literacy, students' cognitive literacy in AI ethics scored the highest (M = 5.740), while AI awareness literacy scored the lowest (M = 4.578). Students' attitudes toward GAI significantly and positively influenced their usage intention, with the combined TPB framework and AI literacy explaining 59.3% of the variance.
This study determined the level of computer literacy and its influence on the academic performance of junior high school students. Specifically, it probed into the students' attitude toward computers and sought answers to the following: the extent of students' computer literacy in terms of Word Processing, Spreadsheet, Presentation, and General Computing; their academic performance based ...
What is the extent of computer literacy of the student-respondents in terms of the following areas, namely: 2.1. Word Processing; 2.2. Spreadsheet; 2.3. Presentation; and .
Another basic computer skill elementary students should learn is word processing. This skill is used widely in education as well as professional settings as a form of communication, note-taking, composition and more. ... Designing computer literacy curriculum for elementary students is an important responsibility because of its far-reaching ...
Paper —Computer Literacy with Skills of Seeking for Information Electronically among University… skills. It provides the opportunity to understand, learn from each other and enhance the learning process [2]. 2 Computerized Literacy (CL) Literacy is the cumulative growth of the techniques, habits, and beliefs of a people. This cumulative growth is transmitted to the younger generation ...
In this paper, the effects of the rapid advancement of generative artificial intelligence (Gen AI) in higher education (HE) are discussed. A mixed exploratory research approach was employed to understand these impacts, combining analysis of current research trends and students' perceptions of the effects of Gen AI tools in academia. Through bibliometric analysis and systematic literature ...
This article aims at exploring how to support students' awareness on gender stereotypes and the development of gender literacy through the making process of interactive toys. I present a case study with 22 primary students (11-12 years) from a public primary school in Barcelona, Spain who were involved in a maker workshop series.
technologies (ICT) in education and training, this paper focuses on the key role of digital literacy and. the skills of students to use new technology that will have an increasing role in the ...
The UT computer science lab, with faculty member Amy Pavel and recent graduate Tess Van Daele at the forefront, has developed an AI system called ShortScribe to enhance accessibility for visually impaired users of short-form videos on platforms like TikTok and Instagram Reels. Pavel, an assistant computer science professor and co-author of the research paper, explained that the system utilizes ...