Thank you for visiting nature.com. You are using a browser version with limited support for CSS. To obtain the best experience, we recommend you use a more up to date browser (or turn off compatibility mode in Internet Explorer). In the meantime, to ensure continued support, we are displaying the site without styles and JavaScript.
- View all journals
- My Account Login
- Explore content
- About the journal
- Publish with us
- Sign up for alerts
- Open access
- Published: 19 February 2020
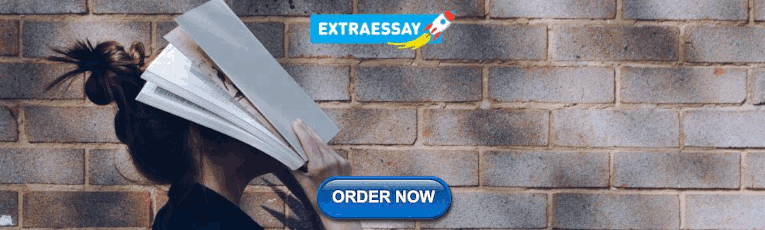
Air pollution control strategies directly limiting national health damages in the US
- Yang Ou ORCID: orcid.org/0000-0002-1889-6218 1 , 2 nAff5 ,
- J. Jason West ORCID: orcid.org/0000-0001-5652-4987 1 ,
- Steven J. Smith ORCID: orcid.org/0000-0003-3248-5607 3 ,
- Christopher G. Nolte ORCID: orcid.org/0000-0001-5224-9965 4 &
- Daniel H. Loughlin ORCID: orcid.org/0000-0002-5102-3507 4
Nature Communications volume 11 , Article number: 957 ( 2020 ) Cite this article
18k Accesses
55 Citations
157 Altmetric
Metrics details
- Energy modelling
- Environmental impact
Exposure to fine particulate matter (PM 2.5 ) from fuel combustion significantly contributes to global and US mortality. Traditional control strategies typically reduce emissions for specific air pollutants and sectors to maintain pollutant concentrations below standards. Here we directly set national PM 2.5 mortality cost reduction targets within a global human-earth system model with US state-level energy systems, in scenarios to 2050, to identify endogenously the control actions, sectors, and locations that most cost-effectively reduce PM 2.5 mortality. We show that substantial health benefits can be cost-effectively achieved by electrifying sources with high primary PM 2.5 emission intensities, including industrial coal, building biomass, and industrial liquids. More stringent PM 2.5 reduction targets expedite the phaseout of high emission intensity sources, leading to larger declines in major pollutant emissions, but very limited co-benefits in reducing CO 2 emissions. Control strategies limiting health damages achieve the greatest emission reductions in the East North Central and Middle Atlantic states.
Similar content being viewed by others
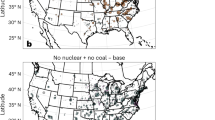
Nuclear power generation phase-outs redistribute US air quality and climate-related mortality risk
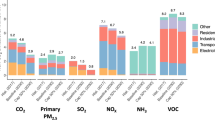
Air quality related equity implications of U.S. decarbonization policy
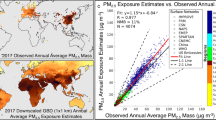
Source sector and fuel contributions to ambient PM2.5 and attributable mortality across multiple spatial scales
Introduction.
Air pollution control in the USA is focused on compliance with emission limits and meeting air quality standards. Over recent decades, US air quality regulations have greatly reduced emissions from traditional polluting sources, such as sulfur dioxide (SO 2 ) and nitrogen oxides (NO x ) from coal-fired power plants and on-road transportation. These pollutants, as well as directly emitted PM 2.5 , contribute to ambient PM 2.5 levels. As a result of these emission reductions, the national average annual PM 2.5 concentration has declined by 42% from 2000 to 2015 1 , and the corresponding PM 2.5 mortality burden has also decreased significantly 2 , 3 . Despite decreasing PM 2.5 concentrations, recent epidemiological evidence indicates there are still significant adverse health effects associated with PM 2.5 exposure at approximately current US concentration levels 4 , 5 .
A primary goal of air pollution control is to protect human health. Air pollution health risks can be most cost-effectively addressed through strategies that simultaneously consider multiple pollutants from multiple source sectors, as well as factors such as long-term population and economic growth. Analyses of the impacts of proposed regulations 6 , 7 , 8 , 9 typically follow an emission-impact paradigm, in which a first step is to identify emission-reduction targets that meet air pollutant concentration standards. Next, control strategies are devised to achieve the emision reduction targets. Candidate strategies are then evaluated using air quality and health impact modeling to estimate the benefits of the proposed actions. However, since the emission-reduction target determination and air quality and health modeling are decoupled, the complexity and time requirements of these models limit the ability to explore more than a handful of control strategies. In addition, there is no guarantee that the proposed control strategy or the specific combination of control measures is the most cost-effective pathway. Alternatively, air quality management studies 10 , 11 , 12 , 13 , 14 , 15 , 16 , 17 have explored the development of multi-pollutant control strategies using an optimization framework. While these studies in general confirm that integrated planning across multiple pollutants is more cost-effective than individual mitigation actions, their focus generally has been on a relatively small spatial scale 12 , 13 , 15 , 16 or small number of pollutants 14 over a short period 10 , 12 , 13 , 17 , due to the complexity of their optimization algorithms required to address exposure-outcome nonlinearties.
To our knowledge, this is the first study to model the most cost-effective actions to directly achieve a specified reduction in national health damages of air pollution. In contrast with traditional strategies based on absolute or relative emission reductions for specific air pollutants or sectors, this paper focuses on PM 2.5 -related mortality costs (hereinafter referred to as PMMC), which account for over 90% of the total monetized PM 2.5 health impact 18 . We investigate which sectors, technologies, and states can most cost-effectively reduce emissions to achieve various levels of PMMC reduction targets. This is accomplished by integrating a representation of the monetized PM 2.5 attributable mortality of inorganic air pollutant emissions 19 , 20 into a version of the Global Change Assessment Model with state-level representation of the US energy system (GCAM-USA) 21 , 22 , which provides a platform that allows a national mortality reduction target to be applied. PMMC is modeled in GCAM-USA by multiplying a pollutant-, source category-, and state-specific PMMC coefficient ($ Tg −1 ) with the corresponding pollutant emissions (Tg) for each technology. Within this framework, we impose PMMC reduction targets, and GCAM-USA finds how to meet those targets cost-effectively while meeting energy demands.
Our results suggest that even under a scenario with limited transitions from coal toward renewable energy, substantial health benefits can still be achieved cost-effectively by using electricity to replace sources with high primary PM 2.5 emission intensities, including industrial coal, building biomass, and industrial liquids. This finding also holds under a scenario with a more aggressive transition toward renewable energy.
Reference scenarios
We define a reference scenario (BASE REF) that includes updated baseline assumptions about technology trajectories harmonized with the 2018 Energy Information Administration (EIA) Annual Energy Outlook (AEO) 23 , as well as an alternative reference scenario (HR REF) with much higher shares of wind and solar generation (e.g., 48% versus 25% in 2050), intended to reflect a continuation of recent, rapid growth in renewables. For both, major air pollutant and CO 2 emission regulations currently in place are represented 24 , assuming no additional future regulations (“Methods”). Electric sector coal use in HR REF decreases by 51% from 2015 to 2050, compared with the 8% increase in BASE REF (Supplementary Fig. 1 ). Projected emissions of major air pollutants are also considerably lower in HR REF (Supplementary Fig. 2 ). In 2050, SO 2 emissions decrease by 47% and 21% from 2015 in HR REF and BASE REF, correspondingly. Primary PM 2.5 emissions decrease by 65% and 27% from 2015 in HR REF and BASE REF, correspondingly. These additional pollutant emission reductions lead to a much lower baseline PMMC projection.
Relative to BASE REF, five additional scenarios (US10–US50) apply increasingly stringent national PMMC reduction targets of 10% (US10) to 50% (US50) reductions in 2050 relative to BASE REF in 2050. Similarly, a 50% PMMC reduction target is applied to HR REF (HR US50). We focus on the BASE scenarios in this study because the coal and renewable assumptions under BASE REF pose greater challenges for reducing PMMC (“Methods”). For simplicity, REF and US10–US50 throughout the rest of the study refer to scenarios based on BASE REF. HR REF and HR US50 are used to evaluate the robustness of the major findings using BASE.
Under REF, PMMC in the US declines in the near-term, but then starts to level off around 2035 (Supplementary Fig. 3 ). This decline is driven by both socioeconomic and energy factors 21 . Decreased energy intensity (energy use per unit GDP), more stringent emission controls, and decreased baseline mortality rates contribute to lower PMMC. Countervailing factors, such as growing population, higher income levels (which increase willingness-to-pay), and higher future energy demands, contribute to the leveling off of PMMC after 2035.
Effects of 50% PMMC reductions
For both BASE and HR scenarios, reducing PMMC by 50% leads to very little change in national total energy use in 2050, relative to REF (Fig. 1a, b ). The PMMC reduction in US50 is achieved mainly by substitution of industrial coal, building biomass, and industrial liquids with electricity (Fig. 1b ). Compared with REF, electric sector energy input for US50 is 4.2% higher in 2050, primarily from natural gas and clean energy, such as wind and solar. Total energy use in the industry and building sectors decreases 5.2% and 1.1% on an end-use equivalent basis, respectively, while PMMC from these sectors is reduced by 71% and 65%, respectively (Fig. 1e ). The decrease in industrial coal, building biomass, and industrial liquids in US50 is equivalent to only 2% of the total energy consumption in 2050, but contributes 87% of the total PMMC reductions relative to REF. Here, we call these high-emission-intensity sources because of their disproportionately high contributions to PMMC.
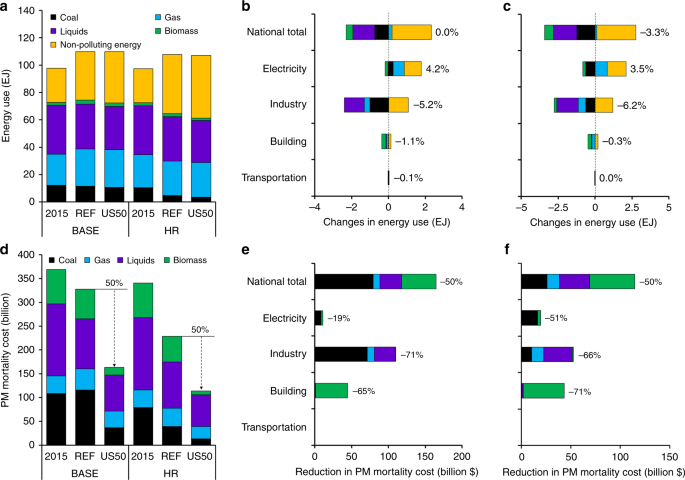
National total energy use (EJ) by fuel ( a ), sectoral changes in energy use (EJ) by fuel for BASE US50 relative to BASE REF ( b ) and HR US50 relative to HR REF ( c ); national total PM 2.5 mortality costs (billion 2018$) by fuel ( d ), and sectoral changes in PM 2.5 mortality costs (billion $) by fuel for US50 reductions under BASE ( e ), and HR scenarios ( f ). With the exception of the bars labeled 2015, all values are for 2050. Changes in the transportation sector are negligible. The nonpolluting energy category includes geothermal, hydropower, wind, and solar power in the electricity sector, and hydrogen and electricity in end-use sectors. Source data are provided as a Source Data file.
Under the HR US50 (Fig. 1c , f ), the decrease in these high-emission-intensity sources is also equivalent to 2% of the total energy consumption in 2050 but still contributes to 70% of the PMMC redutions, with additional contributions from decreased electric coal use. Electric coal use is further reduced for the following two reasons. First, wind and solar costs are much lower in HR US50 than BASE US50, making it more cost-effective to displace coal generation. Second, the HR US50 mortality reduction target, which is relative to the HR 2050 reference case value, is more stringent than the BASE US50 target because the HR REF already has a lower baseline PMMC compared to BASE REF (Fig. 1 ).
Overall and sectoral reductions of PMMC in US50 are dominated by reductions in primary PM 2.5 emissions (Supplementary Fig. 2 ). Electricity generation is not a major sector for achieving PMMC reductions in these scenarios, because existing regulations incorporated in REF already reduce NO x , SO 2 , and primary PM 2.5 emissions from the electric power sector by 2050, particularly from coal-fired power plants 24 , 25 . US50 also has a negligible effect on the transportation sector, because emission standards for new on-road vehicles result in a much lower polluting fleet by 2050. In addition, these modeling results suggest that large-scale vehicle electrification is less cost-competitive for reducing PM 2.5 compared with actions available in other sectors under the assumptions in REF, which considers recent market trends of battery vehicle costs (Supplementary Table 1 ) and fuel economy requirements (Supplementary Table 2 ; “Methods”).
This synergy of clean power and electrified end-use technologies is the key to achieve substantial multisector PMMC reductions. In US50, 86% of the additional electric sector energy input in 2050 relative to REF is through nonpolluting energy sources (such as wind and solar) or gas. Compared with REF, electricity generation from wind and solar is 15% (0.8 EJ) larger in 2050, while generation from oil and biomass decreases 18% (0.06 EJ) (Supplementary Table 3 ). This structural change in the electric sector lowers pollutant emissions. For end-use sectors, replacing coal, liquids, and gas with electricity achieves significant emission reductions for industrial SO 2 (−62%) and NO x (−34%), accounting for over 90% of the total reductions in SO 2 and NO x in US50 in 2050 (Supplementary Fig. 2 ). Electrified end-use technologies can also promote energy efficiency. For example, electric heat pumps for residential heating are cleaner and more efficient than traditional combustion-based technologies for space heating, which explains the slightly reduced building energy use in US50.
Effects of PMMC reduction stringency
The choice of which pollutants to reduce from which sectors is determined endogenously by both their respective dollar-per-ton mortality impact, the cost of control options, and alternative technologies. Compared with REF, US50 leads to 51% and 32% reductions of the primary PM 2.5 and SO 2 emissions in 2050, respectively, but reduces NO x emissions by only 11% (Fig. 2 ). The primary PM 2.5 and SO 2 reductions in 2030 and 2035 under US50 are already close to the corresponding reductions achieved by REF in 2050. Further decreasing emissions of primary PM 2.5 and SO 2 more cost-effectively achieves PMMC reductions than decreasing NO x emissions for two reasons. First, NO x has the lowest $ ton −1 PMMC coefficient (Supplementary Table 4 ), making NO x reduction less cost-effective as a means of reducing PMMC. Second, remaining PM 2.5 and SO 2 emissions are largely from the same high-emission-intensity sources (Supplementary Figs. 4 – 6 ) that can be reduced simultaneously and cost-effectively by technology switching. In contrast, the largest remaining source of NO x emissions is liquid fuels in the transportation sector, which is more expensive to replace.
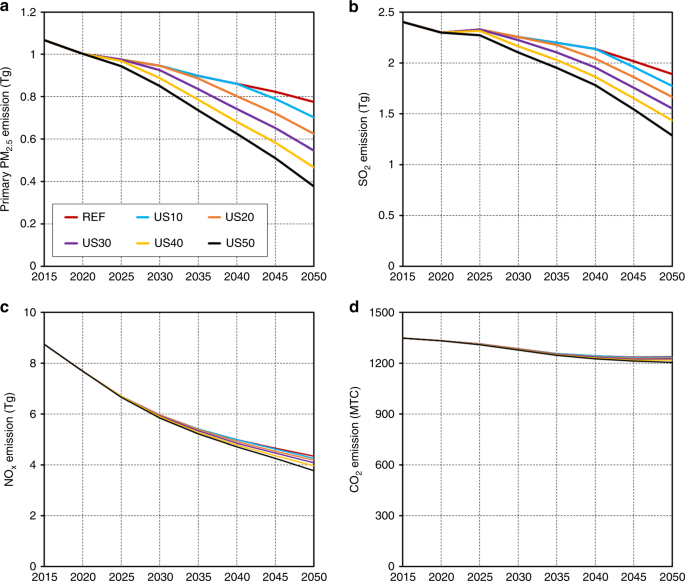
Compared with REF, more stringent PM 2.5 -related mortality costs (PMMC) reduction targets lead to greater reductions of the primary PM 2.5 ( a ) and SO 2 ( b ) emissions in 2050, respectively, but minor reductions of NO x ( c ) and CO 2 emissions ( d ). National emissions include anthropogenic sources from electricity, industry, transportation, and buildings sectors in continental US. Emissions from AK and HI are not included. Source data are provided as a Source Data file.
While CO 2 reductions are a co-benefit from reducing PMMC (Fig. 2d ), this co-benefit is minor because the high-emission-intensity sources affected by PMMC reduction targets are minor contributors to energy consumption in the industry and building sectors (Supplementary Figs. 7 and 8 ), as well as relatively small sources of CO 2 . Thus, while many studies have found substantial air quality co-benefits of CO 2 reductions 22 , 26 , 27 , our findings suggest that emission reductions driven solely by air pollution health improvement targets do not necessarily result in large CO 2 emission reductions under the current US energy structure (see “Discussion”). This finding is valid under both BASE and HR energy trajectories (Supplementary Fig. 9 ).
More stringent PMMC reductions promote more rapid electrification and technology replacement as early as 2020, which is the first modeling period with PMMC constraints. Compared with REF, US10 does not see greater electricity production until 2035, while US50 promotes greater electrification from 2020 (Fig. 3a ). In addition, more stringent PMMC reductions also expedite the retirement of high-emission-intensity sources in end-use sectors. For example, US10 does not have additional reductions in building biomass until 2035 relative to REF, while US50 reduces building biomass immediately in 2020 (Fig. 3b ). More stringent PMMC reductions also lower the average PM 2.5 emission intensity for the same source (Fig. 3c ). For example, the 2050 average PM 2.5 emission intensity for building biomass in US50 is 48% lower than that in REF, reflecting the greater efficiency and lower emission intensities of new building biomass units 28 . This result suggests that when phasing out high-emission-intensity sources in end-use sectors, the most cost-effective approach is to start with the older vintages.
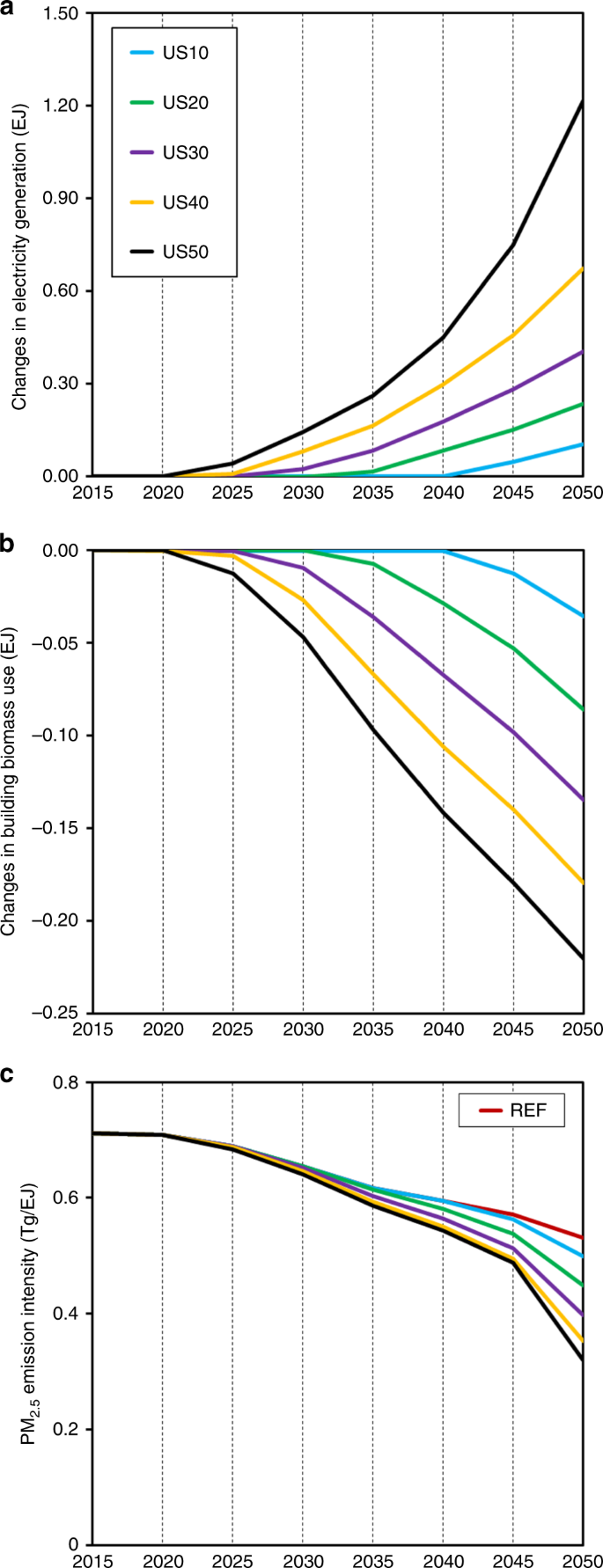
Changes relative to REF for each modeling year for electricity generation (EJ) ( a ) and building biomass use (EJ) ( b ), and average PM 2.5 emission intensity (Tg EJ −1 energy input) of building biomass ( c ). More stringent PM 2.5 -related mortality costs (PMMC) reductions expedite electrification and the retirement of high- emission-intensity sources in end-use sectors. Emission intensity is also found to decrease for other major polluting sources, such as industrial coal use (Supplementary Fig. 10 ). Source data are provided as a Source Data file.
Regional PMMC reductions and actions
The PMMC of state-level emissions depend in part on the magnitude and species of pollutant emissions and the population exposure within or near the emitting state. The distribution of PMMC in 2015 is mainly affected by the distribution of traditional polluting sources (such as coal-fired electric utilities) and population density, with the highest PMMC in OH and PA (Fig. 4a ). In REF at 2050, PMMC declines in the East North Central states, including OH, and in the Middle Atlantic states, including PA (Fig. 4b ). However, despite an overall national reduction, PMMC are higher in 2050 for a number of Southern states (such as TX and FL), largely driven by population growth and increasing contributions from historically less-controlled sources in the industry sector 21 .
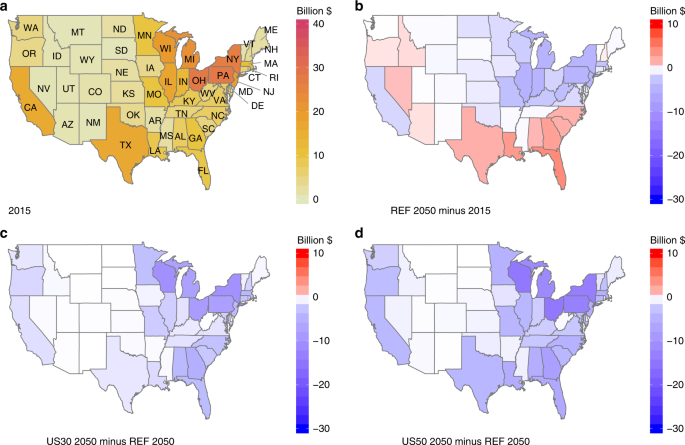
Estimates of state-level PM 2.5 mortality costs (2018$ billion) in REF for 2015 ( a ), and absolute changes ($ billion) in REF by 2050 ( b ). Changes in PMMC relative to REF for US30 in 2050 ( c ) and US50 in 2050 ( d ). In REF, a number of states will face challenges in reducing their PMMC from 2015 to 2050. When applying national reduction targets, all states will have lower PMMC relative to 2015. This is meaningful for long-term air quality management, especially among states with high existing PMMC (such as PA and OH) and states with increasing PMMC in REF (such as TX and FL). Source data are provided as a Source Data file.
When national PMMC reduction targets are applied, the greatest PMMC reductions are obtained in the East North Central and Middle Atlantic states (Fig. 4c, d ; Supplementary Fig. 11 ), which tend to have greater populations and larger emissions. Specifically, OH, PA, NY, MI, and WI have the greatest PMMC reductions in 2050 under both US30 and US50, suggesting lower costs for these states to replace high-emission-intensity sources with clean energy. All states have lower PMMC compared with REF, despite the challenges from increasing population. However, the reduction fraction varies widely across states. For example, under US50, the PMMC in MT and ND decreases by <5%, while WI and VT reduce their PMMC by >80% (Supplementary Fig. 12 ). The relative reductions in 2050 for some highly populated states such as TX and CA are also much less than the national target of 50% under US50.
The spatial pattern of PMMC reductions in Fig. 4 can be further explained by considering the state-level distribution of high-emission-intensity sources, particularly industrial coal, building sector biomass, and industrial liquids (Fig. 5 ). PA and OH together account for 31% of the national total industrial coal use in 2015, while growth of industrial coal is a major contributor to the increased PMMC at 2050 in most southeastern states 21 . Building biomass is broadly used in the US, with the highest absolute utilization in CA, which also has the largest reduction of building biomass use in US50 at 2050. US50 prevents the increased use of building biomass in the New England states seen in 2050 under REF, which is a major contributor to regional PMMC in the future. Industrial liquids are predominantly used in TX in 2015, accounting for 32% of the national total usage. TX also has the highest growth in industrial liquids use to 2050, together with slight increases among many southeastern and western states. Under US50, TX has the largest decrease in industrial liquids compared with REF in 2050, together with broad reductions in industrial liquid use for the eastern states and the west coast. Reducing these high-emission-intensity sources is accompanied by greater electricity generation in the states where those sources are located (Supplementary Fig. 13 ). The energy consumption changes for high-emission-intensity sources in 2030 have a similar geographic pattern for US30 and US50, although the magnitudes are smaller than the changes in 2050 (Supplementary Fig. 14 ).
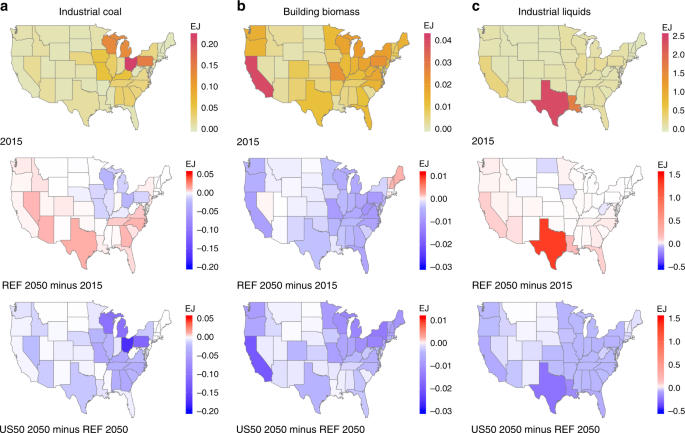
The first row shows 2015 estimates of energy consumption in three high-emission-intensity sources—industrial coal ( a ), building biomass ( b ), and industrial liquids ( c ) for continental US states. The second row shows energy consumption changes for high-emission-intensity sources in 2050 compared with 2015, and the third row shows energy consumption changes in US50 relative to REF in 2050, as actions of reducing PMMC. Blue indicates reduced energy use in high-emission-intensity sources, and red indicates increased energy use in high-emission-intensity sources. Source data are provided as a Source Data file.
Cost-effectiveness of PMMC reductions
From US10 to US50, the marginal benefits in 2050 for every 10% PMMC reduction are the same (~$33 billion, Fig. 6a ), as defined by the PMMC reduction targets, while the estimated marginal costs increase by a factor of 50, from $0.3 billion in REF-US10 to $14.7 billion in US40–US50, as the most cost-effective control options are exhausted first (Fig. 6b ). For all PMMC reduction scenarios considered, including US50, the benefits of reduced PMMC exceed the control costs. Even in US50, the marginal PM benefit of avoided mortality in 2050 is still over twice the marginal policy cost, while the national total health benefit in US50 is nearly seven times the corresponding policy cost.
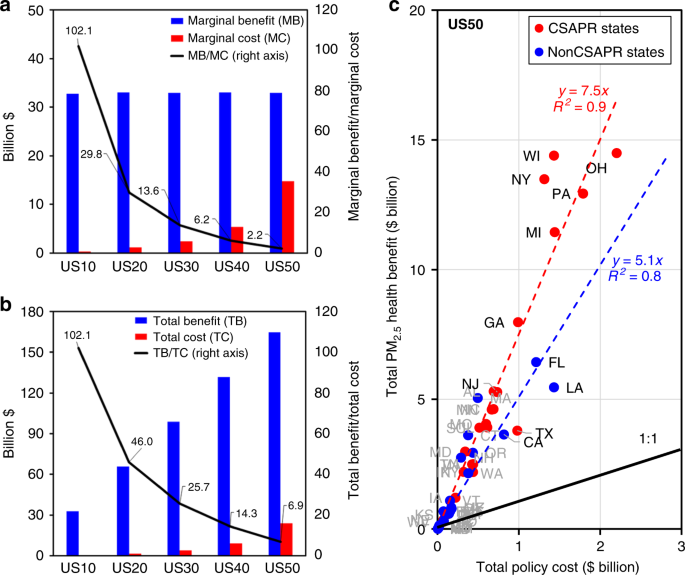
Marginal PM 2.5 health benefit and marginal policy cost ( a ), total PM 2.5 health benefit and total policy cost ( b ) under PM 2.5 mortality cost reductions in 2050, and state-level estimates of total PM 2.5 health benefit and policy cost in US50 in 2050 ( c ). Source data are provided as a Source Data file.
US50 is also cost-effective for all states (Fig. 6c ). In general, it is more cost-effective to reduce PMMC among the Cross-State Air Pollution Rule (CSAPR) states (simple linear regression coefficient, β = 7.5, N = 23) compared with non-CSAPR states ( β = 5.1, N = 26). CSAPR states are those identified in the CSAPR as having emissions that significantly contribute to downwind air pollution 29 . Note that the state-level PMMC coefficients represent the mortality impact of emissions from that state on both the population within that state and downwind of that state. The 23 CSAPR states together account for 60% of the total US population in 2015, while under US50 they account for 76% of the total PM health benefits and 71% of the total policy cost in 2050. Emission reductions in OH lead to the highest health benefits of $14.5 billion in 2050, as well as the highest policy cost of $2.2 billion. PMMC reductions among CSAPR states are achieved through emission reductions beyond the electric sector NO x and SO 2 emission caps that are mandated under CSAPR, which are fully phased in by 2030 under REF. State-level policy costs and health benefits of US50 and HR US50 are reported in Supplementary Tables 5 and 6 , respectively. Notably, HR US50 is also cost-effective for all states.
Current US air pollution control is driven by compliance with air pollutant concentration standards and achieved through emission reductions from individual sources. Here we demonstrate a health-driven approach, in which PM 2.5 -related mortality costs are directly constrained in the future. We simulate how national PMMC reduction targets could be achieved within a global human–earth systems model with US state-level energy system representations, allowing the model to identify the states and actions that can most cost-effectively decrease future PMMC.
Our state-level results demonstrate the importance of devising state-specific strategies for improving air quality and protecting human health. Here, state-level actions are implicitly coordinated to represent the most cost-effective pathway to reach the specified national PMMC mitigation target. In this context, both the cost-effectiveness and the PMMC per capita can be very different across states (Supplementary Tables 5 – 7 ), and the relative reductions for some states can be much less than the national targets. Therefore, this approach may not achieve environmental equity across states, and may not be consistent with the goals of individual states. An extension of this work could explore cost-effective actions to achieve greater PMMC reductions in each state driven by state-specific targets.
We find limited co-benefits of CO 2 emission reductions under even stringent PMMC reductions up to US50. Similar findings are also shown in China’s Clean Air Action, which successfully reduced major air pollutants from 2010 to 2017, but as accompanied by 16% increases in CO 2 emissions 30 . Another study found that wealthier cities in North America have reduced their PM 2.5 concentrations and mortality burdens considerably in recent years, while their CO 2 emissions have not declined in parallel 31 . This largely one-way co-benefit pathway is because of differences in the major actions for controlling CO 2 versus other pollutant emissions 16 . The most cost-effective CO 2 controls involve fuel switching or efficiency improvements 32 , 33 , both of which also reduce air pollutants. In contrast, the most cost-effective air pollutant control strategies in the US do not necessarily cause major changes in the general energy structure (Supplementary Figs. 7 and 8 ). Rather, they impose end-of-pipe controls or replace high-emission-intensity sources only, which have a limited effect on CO 2 emissions. Here, we find that most PMMC reductions can be achieved by reducing only a small portion of high-emission-intensity fuel use including industrial coal, industrial liquids, and building biomass, but the overall fuel mix does not change dramatically.
This work produces novel insights into potential architectures for multi-pollutant control strategies that are explicitly driven by PMMC reduction targets. Since GCAM-USA is a global modeling framework, the general approach demonstrated here can be extended with health impact representations for other countries or regions. Considering the differences in energy structures, domestic policy and geopolitical issues among different regions, the specific solutions may be very different. For example, China can obtain substantial air quality health benefits from large-scale fleet electrification, especially in populous megacities 34 . India’s ambient air quality standard is achievable by mitigating household emission sources and rural electrification 35 . This methodology can also be extended with additional environmental and health endpoints, supporting a wide range of single and multi-objective outcome-driven policy analyses.
The Global Change Assessment Model (GCAM) ( http://jgcri.github.io/gcam-doc/ ) is a global human–earth system model designed for long-term, integrated assessment of the economy, energy, agriculture, land-use, water, and climate 36 , 37 . GCAM-USA builds upon GCAM by disaggregating the USA region to represent state-level energy supply and demand markets, nested within a global framework with 32 regions 38 , 39 , 40 . State-level economic activities are driven by exogenous assumptions regarding population, GDP, and labor productivity, which determine energy and service demands for each state. GCAM-USA simulates the operation of markets for energy goods and services from 2010 to 2100 in 5-year increments. Supply and demand are balanced in each modeling period by solving for a set of equilibrium prices for all agriculture, energy, emission, and policy-related markets. As a result, model solutions represent the most cost-effective combinations of technologies satisfying the energy and service demands for the current modeling period. Market shares of competing technologies and fuels are determined via logit functions, apportioning market share as a function of cost-effectiveness. Note that the resulting choices of technologies over time are not necessarily the economically optimal solution across the entire modeling period, because the dynamic recursive approach in GCAM simulates how the system evolves under imperfect foresight, in contrast to intertemporal optimization models. GCAM-USA has been employed for many US-specific policy analyses, addressing environmental implications of low-carbon pathways 22 , 39 , 41 and water use for power generation 42 .
GCAM-USA estimates air pollutant emissions as the product of an economic activity (energy input or service output of a specific technology) and the corresponding emission coefficient. In our previous work 21 , 22 , 24 , we have attempted to harmonize emission coefficients with EPA’s National Emission Inventory (NEI) and its projections, and have included representations of important existing national and regional air quality, climate, and energy policies. These state-level updates allow a more a realistic reference scenario that serves as the baseline for examining additional PM 2.5 mortality cost reductions. Supplementary Tables 1 and 2 briefly summarize how energy use and air pollutant controls are modified in GCAM-USA for this study, and Supplementary Tables 8 – 10 include PM 2.5 , NO x , and SO 2 emission factors from 2015 to 2050, applied for all states. More detailed documentation and evaluation of GCAM-USA emissions can be found in our previous work 24 .
Marginal abatement curves (MACs) in GCAM-USA
GCAM-USA includes marginal abatement curves (MACs) for electric sector NO x and SO 2 for existing coal-fired plants 24 , 43 . For existing power plants, the selective catalytic reduction (SCR) is the major control measure for NO x , and the flue gas desulfurization (FGD) is the major control measure for SO 2 . The costs of adding these controls are the same for all states (Supplementary Tables 11 and 12 ). In GCAM-USA, MACs can only be triggered when the corresponding emission markets are set, so we have applied MACs for both pollutants and their corresponding PMMCs. For CSAPR states, the emission markets are set by the emission caps of NO x and SO 2 for existing coal-fired power plants. This flexibility of adding additional emission controls through the MAC partially explains why CSAPR states can most cost-effectively reduce PMMC associated with their emissions.
In this work, we further develop MACs for NO x , SO 2 , and PM 2.5 emissions from coal, gas, and refined liquid sources in industrial sector. First, we examine existing industrial sources in the 2011 NEI to estimate the fraction of emissions to which controls could be applied, and then we combine these fractions with the corresponding control cost derived from the Control Strategy Tool 44 . For sources with different control options, an average control cost is applied to represent a one-step control. Supplementary Tables 13 – 21 show the resulting MACs of PM 2.5 , NO x , and SO 2 for industrial coal, gas, and refined liquid sources. Note that MACs are only applied to controllable sources. For states that do not have certain emissions from some source categories, or are already fully controlled, MACs are not available. These MACs allow electric and industry sectors to respond to policy targets by explicitly considering the application of end-of-pipe controls in addition to fuel switching and adopting more efficient technologies (Supplementary Table 22 ).
Although GCAM-USA is technology-rich in most sectors, the current version of GCAM-USA does not have technology-level representation of industrial fuel use, or explicit representation of high-efficiency wood stoves for residential heating. Adding these technological details in the future can more accurately quantify the technology turnover of high-polluting sources identified in this paper, as well as more explicit representation of technology-specific polices, such as the Maximum Achievable Control Technology (MACT) standard for industrial boiler 45 and state-specific residential wood stove emission standards.
State-level PMMC in GCAM-USA
The Estimating Air pollution Social Impact Using Regression (EASIUR) model 19 , 20 is a reduced-form model derived from regression of outputs of a chemical transport model. It has estimated the PM 2.5 -attributable deaths within the US per tonne of inorganic air pollutant emissions (primary PM 2.5 , SO 2 , and NO x ) from each county in 2005. The concentration-response relation used for health impact estimation in EASIUR is derived from Krewski et al. 46 , and $8.6 M (in 2010 USD) is used for the value of a statistical life (VSL) 47 .
These county-level death-per-tonne estimates were aggregated to the state level for the electricity, industry, transportation, and building sectors in 2005, based on the emission-weighted sum for each sector, and then adjusted for each future time period modeled in GCAM-USA (2010 to 2050 in 5-year increments) to account for future changes in population exposure, baseline mortality rates, and VSL 19 (Supplementary Table 23 ). The state-level mortality costs presented here represent the impact of emissions from that state on the population within that state and all downwind states. State-level PMMC coefficients for all sectors vary widely across states (Supplementary Table 4 ). Our previous research 21 , 22 demonstrated that integrating PMMC coefficients into GCAM-USA provides an efficient and rapid approximation of PM 2.5 mortality impacts, allowing it to be used for evaluating large numbers of candidate management strategies to support decision-making.
The following caveats should be noted for the PMMC coefficients adopted in this study. First, PMMC coefficients consider only PM 2.5 -related mortality impacts on adults from emissions of primary PM 2.5 and inorganic precursors, ignoring other emissions that lead to PM 2.5 pollution. Primary organic PM 2.5 is treated as inert in EASIUR, and secondary organic aerosol (SOA) is neglected because of the rapidly evolving understanding and representation of SOA formation in chemical transport models 20 . Anthropogenic emissions generally increase SOA through both volatile organic compounds (VOC) emissions and by enhancing the production of SOA from biogenic sources 48 , 49 . Therefore, the present analysis most likely underestimates the total mortality cost of PM 2.5 pollution, and omits the effects on SOA from other precursors such as NO x and effects of VOC emissions. The morbidity effects of PM 2.5 and the health effects of other pollutants such as ozone are not included, which would require the development of state-specific impact coefficients. For PM 2.5 mortality, EASIUR assumes a log-linear exposure-response function and equal toxicity of all PM 2.5 , while nonlinearities may exist at low levels of exposure, and particles from different emission sources may have different toxicities 50 , 51 . A systematic quantification of these uncertainties in the chemical transport models used to develop EASIUR and similar reduced-form models is beyond the scope of this study.
Second, the use of PMMC coefficients assumes a linear relationship between changes in emissions and deaths. Although linearity may diminish for large changes in multiple pollutant emissions over a long-term 52 , 53 , estimates of PMMC coefficients are generally considered valid within a factor of two, even over fairly large changes in baseline emissions 20 . Third, the PMMC coefficients adopted in this paper are derived based on 2005 emissions and meteorology 19 , future work should seek to use PMMC coefficients determined specifically for future-year emissions and demographics, considering also potential changes in climate and consequent changes in PM 2.5 54 . Fourth, while we use state-level PMMC coefficients which allow us to capture projected large-scale population shifts within the US, finer scale shifts, such as changes in urbanization and aging, are also expected to occur 55 . The detailed process of incorporating EASIUR estimates into GCAM-USA and relevant discussion can be found in our previous work 21 .
REF is developed to include air pollutant emission reductions and plausible energy changes from on the books regulations and policies including New Source Performance Standards 29 , Tier 3 mobile vehicle fuel and emission standards 56 , Cross-State Air Pollution Rule (CSAPR) 57 (Supplementary Tables 24 and 25 ), and the Regional Greenhouse Gas Initiative 58 . We also include the Corporate Average Vehicle Efficiency (CAFE) standards 59 (Supplementary Table 26 ), which affect air pollutant emissions through their impact on technology choices. Some state-specific requirements are considered. For example, we assume no new coal generation in California to reflect its SB-100 requirement 60 . We have discussed the role of these existing regulations in future pollutant emissions and PMMC in REF previously 22 .
We consider two reference energy trajectories, BASE REF and HR REF. In BASE REF, key economic activity parameters such as electricity generation and projections of coal use in electric and industry sectors have been harmonized with the 2018 AEO 23 at national or regional levels 24 .
In HR REF, capital costs of wind and solar power generation technologies are further modified such that the scenario results in greater renewable electricity penetration and more rapid electricity coal retirement (Supplementary Table 27 ). Alternative wind capital cost assumptions are developed using the Department of Energy 2016 Wind Technologies Market Report created by Lawrence Berkley National Laboratory (LBNL) 61 . Alternative solar PV capital cost assumptions are developed using the 2015 Utility-Scale Solar Report by LBNL 62 . To create an alternative capital cost trajectory, we averaged the lowest-cost projections across each wind and PV class, and calculated the percent change relative to the 2010 starting point 22 . In addition, we also modified the share weight assumptions of electricity coal use in GCAM-USA. Share weight is a calibration parameter used to reflect non-modeled factors that affect the competition among technologies. In most markets, share weights for 2010 are derived by comparing historic market penetrations versus model-predicted penetrations. For future years, the calibrated share weights transition incrementally to 1, which indicates no specific preference for any particular technology. To model technology shifting and state-specific fuel preference, share weights can be tuned based on expert judgement. In this work, the share weight of the conventional pulverized coal electricity production technology has been reduced from 0.35 to 0.03 in 2010, and kept constant in future modeling years to reflect a substantially reduced preference for coal-fired electric power plants in this scenario.
PMMC reduction targets (US10–US50) are assumed to be linearly applied beginning in 2020, in 5-year increments, to reach their designated percentage reduction (10–50%) relative to REF in 2050. These stylized scenarios are not intended to represent a realistic policy prescription, but are instead meant to show illustrative pathways that represent a continuation, to varying degrees, of the near-term decrease in mortality projected in REF and HR REF under current regulations.
Representation of PMMC reduction targets in GCAM-USA
Similar to pollutant emissions, PMMC are represented as byproducts associated with each economic activity in GCAM-USA. By setting reductions on the national PMMC, GCAM-USA creates a national PMMC market and places a shadow price on PMMC 63 , which then impacts both technology choices and potential addition of end-of-pipe emission controls via the MACs. Existing and future technologies with emissions causing high PM 2.5 levels will be assigned a higher PMMC cost, providing an incentive to reduce PMMC by reducing use of these technologies, including the early retirement of existing equipment. The equilibrium price of PMMC for each modeling period reflects the iterative process of simultaneously solving all national and regional markets, including the US national PMMC market to meet the specified level of reduction.
We define the cost-effectiveness of a PMMC reduction scenario as the ratio of avoided mortality to the required control cost. This metric expands the traditional emission-impact sensitivity paradigm (avoided mortality per ton of emission reduction) to an energy-emission-impact framework (avoided mortality per dollar of control cost invested), illustrated in Eq. ( 1 )
where ΔM represents the avoided mortality, ΔC represents the required control cost, and ΔE represents the avoided air pollutant emissions. Although many studies have separately investigated the ΔM/ΔE component 53 , 55 , 64 and the ΔE/ΔC component 65 , 66 , the states and control actions that most cost-effectively reduce PMMC are identified in this study as those with the highest ΔM/ΔC.
The total PM 2.5 health benefit of a given PMMC reduction scenario in a given year is defined as the avoided PMMC relative to REF. The corresponding marginal PM 2.5 health benefit is estimated as the avoided PMMC relative to the last 10% PMMC reduction scenario, in a specific future year. The total policy cost of a given PMMC reduction scenario in a given year is estimated as the area below the marginal abatement curve (MAC) of PMMC 66 , 67 (Supplementary Fig. 15 ). The corresponding marginal policy cost is estimated as the difference between the policy costs of the current scenario and the next-lowest PMMC reduction scenario for the same modeling year.
The estimated policy cost in this paper represents the system-wide cost from all PMMC mitigation efforts, which is used as a general metric to better characterize the overall cost-effectiveness of PMMC reductions, neglecting feedback on the macroeconomy and population distribution. Evaluation of the cost-effectiveness of more detailed actions such as specific air pollutant control measures, energy efficiency and fuel switching require a bottom-up approach with detailed representation of technology-level controls 65 , 66 .
Reporting summary
Further information on research design is available in the Nature Research Reporting Summary linked to this article.
Data availability
Data used to perform this study can be found in the Supplementary Information and Supplementary Data. The source data underlying all figures are provided in the Source Data file. GCAM-USA is publicly available ( https://github.com/JGCRI/gcam-core/releases ). The additional GCAM-USA input files required to perform this study are available from the corresponding author upon request.
Code availability
Code used in GCAM and R for this work is available from the corresponding author upon request.
U.S. Environmental Protection Agency (EPA). Our nation’s air: 2019. https://gispub.epa.gov/air/trendsreport/2019/#home (2019).
Zhang, Y. et al. Long-term trends in the ambient PM 2.5 - and O 3 -related mortality burdens in the United States under emission reductions from 1990 to 2010. Atmos. Chem. Phys. 18 , 15003–15016 (2018).
Article ADS CAS PubMed PubMed Central Google Scholar
Fann, N., Kim, S., Olives, C. & Sheppard, L. Estimated changes in life expectancy and adult mortality resulting from declining PM 2.5 exposures in the contiguous United States: 1980–2010. Environ. Health Perspect. 125 , 097003 (2017).
Article PubMed PubMed Central Google Scholar
Pope, C. et al. Mortality risk and fine particulate air pollution in a large, representative cohort of U.S. adults. Environ. Health Perspect. 127 , 077007 (2019).
Article PubMed Central Google Scholar
Bennett, J. et al. Particulate matter air pollution and national and county life expectancy loss in the USA: a spatiotemporal analysis. PLoS Med. 16 , e1002856 (2019).
U.S. EPA. Regulatory impact analysis of the cross-state air pollution rule (CSAPR) update for the 2008 national ambient air quality standards for ground-level ozone. https://www3.epa.gov/ttn/ecas/docs/ria/transport_ria_final-csapr-update_2016-09.pdf (2016).
U.S. EPA. Regulatory impact analysis: control of air pollution from motor vehicles: tier 3 motor vehicle emission and fuel standards final rule. https://nepis.epa.gov/Exe/ZyPDF.cgi/P100ISWM.PDF?Dockey=P100ISWM.PDF (2014).
U.S. EPA. Regulatory impact analysis: national emissions standards for hazardous air pollutants for industrial, commercial, and institutional boilers and process heaters. http://www.epa.gov/ttn/ecas/regdata/RIAs/boilersriafinal110221_psg.pdf (2011).
U.S. EPA. Regulatory impact analysis: final mercury and air toxics standards. https://www3.epa.gov/ttnecas1/regdata/RIAs/matsriafinal.pdf (2011).
Peng, W. et al. Managing China’s coal power plants to address multiple environmental objectives. Nat. Sustain. 1 , 693–701 (2018).
Article Google Scholar
Brown, K., Henze, D. & Milford, J. How accounting for climate and health impacts of emissions could change the US energy system. Energy Policy 102 , 396–405 (2017).
Fann, N. et al. Maximizing health benefits and minimizing inequality: incorporating local‐scale data in the design and evaluation of air quality policies. Risk Anal. 31 , 908–922 (2011).
Article PubMed Google Scholar
Wesson, K., Fann, N., Morris, M., Fox, T. & Hubbell, B. A multi–pollutant, risk–based approach to air quality management: case study for Detroit. Atmos. Pollut. Res. 1 , 296–304 (2010).
Article CAS Google Scholar
Macpherson, A. J., Simon, H., Langdon, R. & Misenheimer, D. A mixed integer programming model for National Ambient Air Quality Standards (NAAQS) attainment strategy analysis. Environ. Model. Softw. 91 , 13–27 (2017).
Liao, K. J. & Hou, X. Optimization of multipollutant air quality management strategies: a case study for five cities in the United States. J. Air Waste Manag. Assoc. 65 , 732–742 (2015).
Article CAS PubMed Google Scholar
West, J. J., Osnaya, P., Laguna, I., Martínez, J. & Fernández, A. Co-control of urban air pollutants and greenhouse gases in Mexico City. Environ. Sci. Technol. 38 , 3474–3481 (2004).
Article ADS CAS PubMed Google Scholar
Amann, M. et al. Cost-effective control of air quality and greenhouse gases in Europe: modeling and policy applications. Environ. Model. Softw. 26 , 1489–1501 (2011).
Martenies, S. E., Wilkins, D. & Batterman, S. A. Health impact metrics for air pollution management strategies. Environ. Int. 85 , 84–95 (2015).
Heo, J., Adams, P. J. & Gao, H. O. Reduced-form modeling of public health impacts of inorganic PM 2.5 and precursor emissions. Atmos. Environ. 137 , 80–89 (2016).
Article ADS CAS Google Scholar
Heo, J., Adams, P. J. & Gao, H. O. Public health costs of primary PM 2.5 and inorganic PM 2.5 precursor emissions in the United States. Environ. Sci. Technol. 50 , 6061–6070 (2016).
Ou, Y., Smith, S. J., West, J. J., Nolte, C. G. & Loughlin, D. H. State-level drivers of future fine particulate matter mortality in the United States. Environ. Res. Lett. 14 , 124071 (2019).
Article ADS Google Scholar
Ou, Y. et al. Estimating environmental co-benefits of US low-carbon pathways using an integrated assessment model with state-level resolution. Appl. Energy 216 , 482–493 (2018).
U.S. Energy Information Administration (EIA). Annual energy outlook 2018. https://www.eia.gov/outlooks/archive/aeo18/pdf/AEO2018.pdf (2018).
Shi, W. et al. Projecting state-level air pollutant emissions using an integrated assessment model: GCAM-USA. Appl. Energy 208 , 511–521 (2017).
Article CAS PubMed PubMed Central Google Scholar
Cole, W., Frazier, W., Donohoo-Vallett, P., Mai, T., & Das, P. Standard Scenarios Report: A U.S. Electricity Sector Outlook. https://www.nrel.gov/docs/fy19osti/71913.pdf (2018).
Chang, K. M. et al. Ancillary health effects of climate mitigation scenarios as drivers of policy uptake: a review of air quality, transportation and diet co-benefits modeling studies. Environ. Res. Lett. 12 , 113001 (2017).
West, J. J. et al. Co-benefits of mitigating global greenhouse gas emissions for future air quality and human health. Nat. Clim. Change 3 , 885–889 (2013).
U.S. EPA. Regulatory actions for residential wood heaters. https://www.epa.gov/residential-wood-heaters/regulatory-actions-residential-wood-heaters (2015).
Federal Register. Standards of performance for new and existing stationary sources: electric utility steam generating units; final rule. 40 CRF parts 60, 72 and 75. Environmental protection agency, Vol. 70, No. 95. https://www.govinfo.gov/content/pkg/FR-2005-05-18/pdf/05-8447.pdf (2005).
Zheng, B. et al. Trends in China’s anthropogenic emissions since 2010 as the consequence of clean air actions. Atmos. Chem. Phys. 18 , 14095–14111 (2018).
Anenberg, S. C. et al. Particulate matter-attributable mortality and relationships with carbon dioxide in 250 urban areas worldwide. Sci. Rep. 9 , 11552 (2019).
Grubler, A. et al. A low energy demand scenario for meeting the 1.5 °C target and sustainable development goals without negative emission technologies. Nat. Energy 3 , 515–527 (2018).
Clarke, L. E. et al. Technology and US emissions reductions goals: results of the EMF 24 modeling exercise. Energy J . 35 , 9–32 (2014).
Liang, X. et al. Air quality and health benefits from fleet electrification in China. Nat. Sustain. 2 , 962–971 (2019).
Chowdhury, S. et al. Indian annual ambient air quality standard is achievable by completely mitigating emissions from household sources. Proc. Natl Acad. Sci. USA 116 , 10711–10716 (2019).
Calvin, K. V. et al. GCAM v5.1: Representing the linkages between energy, water, land, climate, and economic systems. Geosci. Model Dev. 12 , 677–698 (2019).
Joint Global Change Research Institute (JGCRI). GCAM v5.2 documentation: GCAM model overview. http://jgcri.github.io/gcam-doc/overview.html (2019).
Zhou, Y. et al. Modeling the effect of climate change on US state-level buildings energy demands in an integrated assessment framework. Appl . Energy 113 , 1077–1088 (2014).
Google Scholar
Iyer, G. et al. GCAM-USA Analysis of US Electric Power Sector Transitions (US Department of Energy, 2017).
JGCRI. GCAM v5.2 documentation: GCAM-USA, http://jgcri.github.io/gcam-doc/gcam-usa.html (2020).
Hodson, E. L. et al. US energy sector impacts of technology innovation, fuel price, and electric sector CO 2 policy: results from the EMF 32 model intercomparison study. Energy Econ. 73 , 352–370 (2018).
Liu, L., Hejazi, M., Iyer, G. & Forman, B. A. Implications of water constraints on electricity capacity expansion in the United States. Nat. Sustain. 2 , 206 (2019).
U.S. EPA. Documentation for EPA base case v.5.13: using the integrated planning model. https://www.epa.gov/sites/production/files/2015-07/documents/documentation_for_epa_base_case_v.5.13_using_the_integrated_planning_model.pdf (2013).
U.S. EPA. Control strategy tool (CoST) control development documentation. https://www3.epa.gov/ttn/ecas/docs/CoST_DevelopmentDoc_02-23-2016.pdf (2016).
Federal Register. National emission standards for hazardous air pollutants for major sources: industrial, commercial, and institutional boilers and process heaters; final rule. 40 CFR Parts 63, Vol 78, No. 21, Environmental Protection Agency. https://www.govinfo.gov/content/pkg/FR-2013-01-31/pdf/2012-31646.pdf (2013).
Krewski, D. et al. Extended follow-up and spatial analysis of the American Cancer Society study linking particulate air pollution and mortality. Res. Rep. Health Eff. Inst. 140 , 115–136 (2009).
U.S. EPA. Guidelines for preparing economic analyses. national center for environmental economics, office of policy. https://www.epa.gov/environmental-economics/guidelines-preparing-economic-analyses (2010).
Riva, M. et al. Increasing isoprene epoxydiol-to-inorganic sulfate aerosol (IEPOX: Sulfinorg) ratio results in extensive conversion of inorganic sulfate to organosulfur forms: implications for aerosol physicochemical properties. Environ. Sci. Technol. 53 , 8682–8694 (2019).
Jimenez, J. L. et al. Evolution of organic aerosols in the atmosphere. Science 326 , 1525–1529 (2009).
Thurston, G. D. et al. Ambient particulate matter air pollution exposure and mortality in the NIH-AARP diet and health cohort. Environ. Health Perspect. 124 , 484–490 (2016).
Burnett, R. et al. Global estimates of mortality associated with long-term exposure to outdoor fine particulate matter. Proc. Natl Acad. Sci. USA 115 , 9592–9597 (2018).
Zhao, B. et al. Nonlinear relationships between air pollutant emissions and PM 2.5 -related health impacts in the Beijing-Tianjin-Hebei region. Sci. Total Environ. 661 , 375–385 (2019).
Lee, C. J. et al. Response of global particulate-matter-related mortality to changes in local precursor emissions. Environ. Sci. Technol. 49 , 4335–4344 (2015).
Fiore, A. M., Naik, V. & Leibensperger, E. M. Air quality and climate connections. J. Air Waste Manag. Assoc. 65 , 645–685 (2015).
Goodkind, A. L., Tessum, C. W., Coggins, J. S., Hill, J. D. & Marshall, J. D. Fine-scale damage estimates of particulate matter air pollution reveal opportunities for location-specific mitigation of emissions. Proc. Natl Acad. Sci. USA 116 , 8775–8780 (2019).
Federal Register. Control of air pollution from motor vehicles: Tier 3 motor vehicle emission and fuel standards; final rule. 40 CFR Parts 79, 80, 85, et al. Part II Environmental Protection Agency, Vol. 79, No. 81, https://www.govinfo.gov/content/pkg/FR-2014-04-28/pdf/2014-06954.pdf (2014).
U.S. EPA. Overview of the cross-state air pollution rule (CSAPR): CSAPR framework. https://www.epa.gov/csapr/overview-cross-state-air-pollution-rule-csapr#affected (2018).
The Regional Greenhouse Gas Initiative. The regional greenhouse gas initiative: an initiative of the New England and Mid-Atlantic states of the U.S. https://www.rggi.org/program-overview-and-design/elements (2019).
Federal Register. 2017 and later model year light-duty vehicle greenhouse gas emissions and corporate average fuel economy standards; final rule. 40 CFR Parts 85, 86, and 600. Part II Environmental Protection Agency, Vol. 77, No. 199, https://www.govinfo.gov/content/pkg/FR-2012-10-15/pdf/2012-21972.pdf (2012).
Senate Bill No. 100 (SB-100), California Renewables Portfolio Standard Program: emissions of greenhouse gases. 2017-2018. https://leginfo.legislature.ca.gov/faces/billPdf.xhtml?bill_id=201720180SB100&version=20170SB10087CHP (2018).
Wiser, R. & Bolinger, M. 2016 Wind Technologies Market Report. U.S. Department of Energy. Prepared by the Lawrence Berkeley National Laboratory (LBNL). DOE/GO-102917-5033. https://www.energy.gov/sites/prod/files/2017/10/f37/2016_Wind_Technologies_Market_Report_101317.pdf (2017).
Bolinger, M. & Seel, J. Utility-Scale Solar 2015—An empirical analysis of project cost, performance, and pricing trends in the United States. U.S. Department of Energy SunShot. Prepared by the Lawrence Berkeley National Laboratory (LBNL). LBNL-1006037. https://eta-publications.lbl.gov/sites/default/files/lbnl-1006037_report.pdf (2016).
JGCRI. GCAM v5.1 documentation: policies and costs. http://jgcri.github.io/gcam-doc/policies.html (2019).
Fann, N., Baker, K. R. & Fulcher, C. M. Characterizing the PM 2.5 -related health benefits of emission reductions for 17 industrial, area and mobile emission sectors across the US. Environ. Int. 49 , 141–151 (2012).
Loughlin, D. H., Macpherson, A. J., Kaufman, K. R. & Keaveny, B. N. Marginal abatement cost curve for nitrogen oxides incorporating controls, renewable electricity, energy efficiency, and fuel switching. J. Air Waste Manag. Assoc. 67 , 1115–1125 (2017).
Xing, J. et al. Least-cost control strategy optimization for air quality attainment of Beijing–Tianjin–Hebei region in China. J. Environ. Manag. 245 , 95–104 (2019).
Calvin, K. V. et al. The EU20-20-20 energy policy as a model for global climate mitigation. Clim. Policy 14 , 581–598 (2014).
Download references
Acknowledgements
Yang Ou was supported by the Research Participation Program at the Center for Environmental Measurement and Modeling, U.S. Environmental Protection Agency, administered by the Oak Ridge Institute for Science and Education (ORISE). The views expressed in this article are those of the authors, and do not necessarily represent the views or policies of the U.S. Environmental Protection Agency.
Author information
Present address: Joint Global Change Research Institute, Pacific Northwest National Laboratory, 5825 University Research Court, College Park, MD, 20740, USA
Authors and Affiliations
Department of Environmental Sciences and Engineering, University of North Carolina at Chapel Hill, Chapel Hill, NC, 27599, USA
Yang Ou & J. Jason West
ORISE Participant at the U.S. Environmental Protection Agency, 109 T.W. Alexander Drive, Research Triangle Park, NC, 27711, USA
Joint Global Change Research Institute, Pacific Northwest National Laboratory, 5825 University Research Court, College Park, MD, 20740, USA
Steven J. Smith
Center for Environmental Measurement and Modeling, U.S. Environmental Protection Agency, 109 T.W. Alexander Drive, Research Triangle Park, NC, 27711, USA
Christopher G. Nolte & Daniel H. Loughlin
You can also search for this author in PubMed Google Scholar
Contributions
Y.O., J.J.W., D.L., and C.N. designed the study. Y.O. performed the research. S.J.S. provided guidance on GCAM modeling. Y.O. wrote the initial paper and all authors contributed to subsequent revisions.
Corresponding author
Correspondence to Daniel H. Loughlin .
Ethics declarations
Competing interests.
The authors declare no competing interests.
Additional information
Peer review information Nature Communications thanks Philip Landrigan and the other, anonymous, reviewer(s) for their contribution to the peer review of this work. Peer reviewer reports are available.
Publisher’s note Springer Nature remains neutral with regard to jurisdictional claims in published maps and institutional affiliations.
Supplementary information
Supplementary information, peer review file, reporting summary, source data, rights and permissions.
Open Access This article is licensed under a Creative Commons Attribution 4.0 International License, which permits use, sharing, adaptation, distribution and reproduction in any medium or format, as long as you give appropriate credit to the original author(s) and the source, provide a link to the Creative Commons license, and indicate if changes were made. The images or other third party material in this article are included in the article’s Creative Commons license, unless indicated otherwise in a credit line to the material. If material is not included in the article’s Creative Commons license and your intended use is not permitted by statutory regulation or exceeds the permitted use, you will need to obtain permission directly from the copyright holder. To view a copy of this license, visit http://creativecommons.org/licenses/by/4.0/ .
Reprints and permissions
About this article
Cite this article.
Ou, Y., West, J.J., Smith, S.J. et al. Air pollution control strategies directly limiting national health damages in the US. Nat Commun 11 , 957 (2020). https://doi.org/10.1038/s41467-020-14783-2
Download citation
Received : 14 November 2019
Accepted : 04 February 2020
Published : 19 February 2020
DOI : https://doi.org/10.1038/s41467-020-14783-2
Share this article
Anyone you share the following link with will be able to read this content:
Sorry, a shareable link is not currently available for this article.
Provided by the Springer Nature SharedIt content-sharing initiative
This article is cited by
Risk assessment for extreme air pollution events using vine copula.
- Mohd Sabri Ismail
- Nurulkamal Masseran
Stochastic Environmental Research and Risk Assessment (2024)
Diabetes and climate change: current evidence and implications for people with diabetes, clinicians and policy stakeholders
- Jacqueline M. Ratter-Rieck
- Michael Roden
- Christian Herder
Diabetologia (2023)
Synergistic strategies for urban passenger transport pollution control and CO2 reduction based on the sunk cost effect
Environment, Development and Sustainability (2023)
Physio-chemical modeling of the NOx-O3 photochemical cycle and the air pollutants’ reactive dispersion around an isolated building
- Xisheng Lin
Building Simulation (2023)
Differential persistence of neutralizing antibody against SARS-CoV-2 in post immunized Bangladeshi population
- Md. Mosfequr Rahman
- Ariful Haque
Scientific Reports (2022)
By submitting a comment you agree to abide by our Terms and Community Guidelines . If you find something abusive or that does not comply with our terms or guidelines please flag it as inappropriate.
Quick links
- Explore articles by subject
- Guide to authors
- Editorial policies
Sign up for the Nature Briefing: Anthropocene newsletter — what matters in anthropocene research, free to your inbox weekly.

Editor’s choice
Announcements
Tackling air pollution and climate change together – an impact case study
Recognizing the value of SEI York’s work integrating air pollution and climate change mitigation , the University of York’s Environment and Geography Department included it as an impact case study in its REF2021 submission. Read about this work, which helped the department become ranked as one of the best of its kind for research impact.
Photo: Jonathan Jobs / Getty Images
SEI has a partnership with the University of York and SEI’s York centre sits within the University’s Environment and Geography Department (EGD). This work by SEI York’s air pollution team was submitted by the department as one of three impact case studies for the 2021 Research Excellence Framework (REF) exercise and contributed to the department being ranked second for impact in Earth Systems and Environmental Sciences in the Times Higher Education REF 2021 ranking.
SEI is proud to contribute to the University of York’s continuing reputation for research with impact that makes a positive difference to communities around the world.

Air pollution and climate change are closely linked. The sources of health-damaging air pollutants and climate-warming greenhouse gases are often the same, including transport, industry, households, agriculture and waste. In addition, a subset of pollutants, short-lived climate pollutants (SLCPs), directly contribute to both issues. SLCPs include black carbon (or soot), which contributes to particulate matter air pollution and warms the atmosphere. Methane is the second largest contributor to climate change behind carbon dioxide, and contributes to the formation of tropospheric ozone, a toxic air pollutant.
These two links mean that there is a substantial opportunity to design plans, strategies and policies which achieve global climate change goals and benefits for local air pollution and public health simultaneously. As part of the Paris Agreement of 2015, governments from over 190 countries have developed Nationally Determined Contributions (NDCs) – climate action plans outlining each country’s commitment to dealing with climate change.
Understanding the extent of the impact of SLCPs, and knowing how to reduce them, is key to helping governments formulate research-driven climate plans and put them into practice, bringing us all closer to reaching the international climate change targets. Studying the global impact of SLCPs also provides insights into the impact these air pollutants have on human health.
The research
Since 2012 SEI York has worked at the forefront of research into SLCPs. Working with organizations including the Climate and Clean Air Coalition ( CCAC ), United Nations Environment Programme ( UNEP ) United Nations Development Programme ( UNDP ), and NDC Partnership , SEI York researchers have supported approximately 25 countries to increase their capacity to reduce SLCPs.
As part of this work, SEI extended the existing planning tool LEAP (Low Emissions Analysis Platform system) to assess the impacts on human health and climate change mitigation strategies.
SEI has delivered bespoke training to government officials, academics and NGOs in partner countries on the development of integrated air pollution and climate change mitigation assessments. Training and supporting local organizations to undertake such assessments demonstrates, often for the first time, the extent of health issues caused by exposure to air pollution, as well as the impact of SLCPs on climate change. It also helps identify possible solutions to reduce these impacts. SEI supports the integration of these assessments into national planning processes, so that the assessment can inform decision making and target setting.
SEI York has also produced significant assessments of the global impacts of air pollutants on premature births and annual asthma emergency room visits. Following the 2015 revelations that car manufacturers had been using software which deliberately misled regulatory testing, SEI York were the first to publish a study to quantify the effects of hidden excess nitrogen oxide emissions from diesel vehicles.
The outcome
SEI York’s research has supported multiple policy and planning processes in partner countries. In advance of the COP26 UN Climate Conference in November 2021, 17 countries were supported by SEI York to submit more ambitious climate change plans that included actions on SLCPs. Ten countries, with support from SEI York, have also developed National Action Plans to reduce SLCPs, which have been endorsed at a Ministerial or Cabinet level.
In addition to these impacts on national government policy and practice, research by SEI York has provided robust evidence to back calls for strengthening global and regional climate responses. One project alone was cited in 94 policy documents from around the world, including by the UN’s Intergovernmental Panel on Climate Change ( IPCC ) and the World Health Organization ( WHO ).
Featured researchers
Reader / Research Leader
Senior Research Fellow
Research Associate
Read more about SEI’s work on air pollution in our feature for the International Day of Clean Air for blue skies 2022 .
- Visit the University of York webpage
Related projects and tools
Initiative / This initiative enables integrated national planning for Nationally Determined Contributions and Sustainable Development Goals.
2020 - 2024 / About Planning and modelling , Pollution and Sustainable Development Goals
Initiative / This initiative focuses on integrated analysis and planning for the mitigation of air pollution and greenhouse gases, including short-lived climate pollutants.
2015 - 2019 / About Cities , Climate policy , Food and agriculture , Fossil fuels , Household energy , Mitigation , Planning and modelling , Pollution , Renewables , Short lived climate pollutants and Transport
Project / On behalf of CCAC, UNEP is cooperating with SEI to support the Ministry of Environment and Forestry of Kenya under SNAP.
2021 / About Cities , Pollution and Short lived climate pollutants
Project / A scientifically robust and policy-relevant framework to identify and assess priority measures that maximize the multiple-benefits for air quality and climate.
2019 - 2023 / About Cities , Food and agriculture , Household energy , Pollution , Short lived climate pollutants and Transport
Tool / The Low Emissions Analysis Platform (LEAP) is a powerful, versatile system for energy planning and climate change mitigation assessment.
About Adaptation , Mitigation , Planning and modelling and Pollution
You might also be interested in
Feature / The AUC, UNEP, SEI and CCAC are supporting researchers and African country representatives to evaluate the state of air pollution and the climate in Africa.
26 April 2022 / About Pollution and Short lived climate pollutants
Journal article / In this journal article, researchers from SEI York discuss climate change mitigation and sustainable development planning in Nigeria.
16 September 2021 / About Cities , Pollution and Public policy
Feature / SEI researchers trained energy sector practitioners on how to use LEAP in Islamabad, Pakistan, as part of the nation's effort to tackle its energy crisis.
1 September 2022 / About Business , Energy access , Fossil fuels , Planning and modelling , Public policy and Renewables
Design and development by Soapbox .
Groundbreaking air pollution study marks 30 years
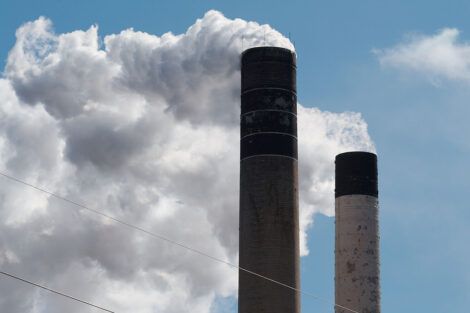
January 4, 2024 – The Harvard Six Cities Study , which had a profound impact on efforts to curb air pollution in the U.S., turned 30 in December. The study found that fine particulate pollution was linked with mortality at much lower levels than previously thought.
A December 29 article in The Guardian quoted Douglas Dockery , John L. Loeb and Frances Lehman Loeb Professor of Environmental Epidemiology, Emeritus, and lead author of the study, about how the study was designed and what it found.
Harvard researchers followed more than 8,000 adults from six U.S. cities with different concentrations of air pollution. They sent a postcard annually to each participant, asking if the named person had died. They also collected air pollution data from each city.
Even after considering a range of factors that could contribute to mortality—such as age, diet, occupation, and smoking history—the researchers found there was a 26% difference in survival between the participants in the least-polluted cities and the most-polluted. Pollution from fine particulate matter, known as PM2.5, was the most dangerous to health, the study found.
“We showed that long-term exposure to air pollution had much more significant effects on mortality risk than previously believed,” Dockery said. “It changed the dynamics of the debate.”
In spite of pushback from various industries that relied on fossil fuels, the study’s findings were confirmed by many subsequent studies, and have led to stricter air pollution guidelines that are estimated to save many thousands of lives each year.
Read The Guardian article: Lasting legacy of the Six Cities study into harms of air pollution
Major Harvard Chan studies concur: Air pollution boosts U.S. death rates (Harvard Chan School news)
Photo: Dori
- Architecture and Design
- Asian and Pacific Studies
- Business and Economics
- Classical and Ancient Near Eastern Studies
- Computer Sciences
- Cultural Studies
- Engineering
- General Interest
- Geosciences
- Industrial Chemistry
- Islamic and Middle Eastern Studies
- Jewish Studies
- Library and Information Science, Book Studies
- Life Sciences
- Linguistics and Semiotics
- Literary Studies
- Materials Sciences
- Mathematics
- Social Sciences
- Sports and Recreation
- Theology and Religion
- Publish your article
- The role of authors
- Promoting your article
- Abstracting & indexing
- Publishing Ethics
- Why publish with De Gruyter
- How to publish with De Gruyter
- Our book series
- Our subject areas
- Your digital product at De Gruyter
- Contribute to our reference works
- Product information
- Tools & resources
- Product Information
- Promotional Materials
- Orders and Inquiries
- FAQ for Library Suppliers and Book Sellers
- Repository Policy
- Free access policy
- Open Access agreements
- Database portals
- For Authors
- Customer service
- People + Culture
- Journal Management
- How to join us
- Working at De Gruyter
- Mission & Vision
- De Gruyter Foundation
- De Gruyter Ebound
- Our Responsibility
- Partner publishers

Your purchase has been completed. Your documents are now available to view.
Reduction of transport-related air pollution. A case study based on the impact of the COVID-19 pandemic on the level of NO x emissions in the city of Krakow
This article describes the impact of limiting human mobility related to the COVID-19 pandemic on the level of air pollution related to transport. The city of Krakow and the emission level of nitrogen oxides (NO x ) were used as a case study. This article describes the air quality monitoring system in Krakow and the measurement results from the measurement station at Krasinskiego Avenue. The average values of the pollution level in April 2018–2020 were compared. For the selected range of data, a significance test was performed, which resulted in no grounds for rejecting the hypothesis of the equality of the mean levels of nitrogen oxides concentrations in the spring. The analysis takes into account the average monthly temperatures in the discussed years.
1 Introduction
At the beginning of March 2020, the first case of infection with the SARS-CoV-2 virus was recorded in Poland. The following days and months brought new realities of social life that all people and the entire Polish economy had to face. Due to the COVID-19 pandemic, schools have been closed, border controls have been reintroduced and international flights have been suspended. Lockdown has started. April 2020 was special – the largest restrictions during the COVID-19 pandemic were maintained throughout the month, under the slogan “stay at home,” and Poles had to come to terms with spending Easter in a limited group. Schools and universities, shopping centers, cultural and sports centers were closed. Employees of many companies worked remotely. These limitations resulted from the introduced regulations [ 1 ]. The lockdown affected all areas of the economy and caused a significant reduction in the mobility of society. On the streets of cities, the traffic of vehicles and the number of people decreased significantly.
Air pollution is caused by industrial plants, transport, and heating devices used in households [ 2 , 3 , 4 , 5 ]. Among the pollutants emitted to the atmosphere, nitrogen oxides (NO x ) can be indicated as characteristic of transport and conventional propulsion sources. This is due to the combustion process in the cylinder chamber. The conditions of vehicle operation in urban areas [ 6 , 7 , 8 ] and relatively frequent engine starts (compared to the operation of vehicles outside urban agglomerations) can be indicated as favoring the occurrence of this pollution. The mobility limitation related to the COVID-19 pandemic in its initial phase (“hard lockdown”) in Poland was a good time to investigate the impact of transport on air pollution. In scientific publications [ 9 , 10 , 11 , 12 , 13 , 14 , 15 ], the impact of pandemic restrictions on noise reduction has been observed. It seems logical that if the intensity of traffic decreased (due to lockdown) then NO x emissions will decrease as well. The aim of the study was to analyze numerical data for a city, whose location favors the accumulation of pollution. The study [ 16 ] analyzed the effect of the Olympic-year policy package on the level of air pollution. Local air pollution has been reduced by enforcing traffic restrictions. This conclusion [ 16 ] concerned a city with a large area and large number of inhabitants. This article covers a city much smaller than Beijing. The city of Krakow was selected as the case study. An important aspect of the analysis carried out in this study is the adoption of such a methodology as to reduce the error associated with emissions related to the use of heating devices in households.
Krakow is one of the largest cities in Poland (approximately 760,000 inhabitants) and is located in the southern part of the country. Due to its geographic location and topography, it is particularly exposed to smog. The city is located at an altitude of 187 m above sea level, up to 384 m above sea level and is located in the Vistula valley – the largest river in Poland. On the north and south sides of the city, there are elevations of the terrain, which impede air circulation and ventilation of pollutants. In Krakow, about 120 days a year are known as “windless” days, and the wind speed often does not exceed 2 m/s [ 17 ]. Krakow is an important administrative, scientific, business, and cultural center. The city has a well-developed air quality monitoring infrastructure, and the solutions introduced to reduce pollutant emissions (especially from households) allow Krakow to be defined as the Polish leader in this field. For many years, the city has been reimbursing some of the costs related to the replacement of coal-fired stoves, conducts educational campaigns, introduces appropriate law, and develops low-emission public transport. In order to reduce the error related to emissions from heating appliances in households, the analysis compared the results for one selected month, taking into account the weather conditions influencing the demand for thermal power.
2 Transport-related air pollution
It is estimated that up to 30% of Europeans living in urban areas are exposed to air pollution that exceeds allowable concentrations and EU quality standards [ 18 , 19 ]. The air condition and visibility in smog conditions on one of the streets of Krakow are shown in Figure 1 . The picture shown in Figure 1 was taken at night to show the pollution level clearly. There is no doubt that actions related to the reduction of pollutant emissions are needed. In the field of transport, such measures are, for example, replacing the public transport fleet with electric and hybrid vehicles, introducing discounts for low-emission car owners, and reducing road traffic in city centers to a minimum – while promoting and favoring “active” mobility. Public transport also uses means of transport where the emission of pollutants depends on the type of fuel [ 20 , 21 , 22 ].

Dietla street in Krakow, during the smog. Source: own materials.
The largest share in the emission related to transport is [ 2 , 18 , 23 ] carbon dioxide (CO 2 ), carbon monoxide (CO), nitrogen oxides (NO x ), non-metallic volatile organic compounds and solid particles. The pollutants, the significant emitter of which is transport, also include methane (CH 2 ), nitrous oxide (N 2 O), sulfur dioxide (SO 2 ) and lead (Pb). The combustion of liquid fuels in motor vehicles may also affect the formation of ground-level ozone, and moving vehicles cause emissions with abrasion of tires, brakes, and road surfaces. There is also “secondary dusting,” consisting in the lifting of dust already existing on the road [ 18 , 24 ]. This article focuses primarily on the analysis of changes in the concentration of nitrogen oxides (NO x ) in the air. This pollutant component is typical of emissions related to the operation of internal combustion engines and at the same time has a small share in the pollution related to energy and industrial activities.
3 Analysis of data from the air quality monitoring system
The monitoring of air quality in Krakow is carried out by the Provincial Inspectorate for Environmental Protection. In April 2020, eight air pollution measurement stations were located in the city [ 25 ]. The concentration of nitrogen oxides (NO x ) was measured in four of them. For the analysis in this article, the station on Krasinskiego Avenue was selected ( Figure 2 ). It is a station located on one of the main communication routes in Krakow. Krasinskiego Avenue is a north–south transit artery along the so-called Alley of the Three Hangers. For this communication section (as for others located in Krakow), there were no available data on the number of vehicles on the road. On the other hand, there are data on the general traffic intensity in terms of its changes during the lockdown and for the entire city [ 23 ]. The measuring station located on AlejaKrasinskiego collects data on air pollution related to road traffic, which makes it possible to observe and analyze any changes in transport emissions.
![case study related to air pollution Figure 2
Location of Krasinskiego Avenue within the city of Krakow [26].](https://www.degruyter.com/document/doi/10.1515/eng-2021-0077/asset/graphic/j_eng-2021-0077_fig_002.jpg)
Location of Krasinskiego Avenue within the city of Krakow [ 26 ].
Measurements of average daily concentrations of nitrogen oxides in a selected station in April 2018–2020 were analyzed. Figure 3 presents their results, comparing the maximum and minimum levels for the following days. The lowest level of the average daily concentration of nitrogen oxides was recorded on April 13, 2020. It had a value of 28 µg/m 3 , the highest level was recorded on April 4, 2018. It had a value of 296 µg/m 3 . Then, the measurement results from the entire analyzed period were compared and the 20 lowest and 20 highest daily mean values were selected from them. Their total number in individual years is presented in Table 1 . For 2020, there are no events among the 20 highest average daily concentrations, as well as a large share of days with the lowest concentration (75% of them occurred in April 2020). The difference is much clearer when you compare 10 days with the best air quality. There are eight of them for 2020, and one for 2018 and 2019. Fluctuations in the level of emissions and the achieved minimum values on individual days in April, in the compared years, may be related to non-working days and holidays. It can be seen, for example, that on selected days of 2019, the level of pollutant emissions was lower than the level of emissions registered on the same days in April 2020. The period of Easter in April 2019 fell on the 21st and 22nd of the month. The traffic and communication needs of the inhabitants at that time were small. Regardless of these circumstances, it can be seen that the absolute drops in the pollution value on the selected days of April 2018 or 2019 are higher than the absolute increases in pollution on the selected days of April 2020.
![case study related to air pollution Figure 3
Average daily concentration of NOx in the air in Krakow on Krasinskiego Avenue in April 2018–2020. Source: own study based on data from ref. [25].](https://www.degruyter.com/document/doi/10.1515/eng-2021-0077/asset/graphic/j_eng-2021-0077_fig_003.jpg)
Average daily concentration of NO x in the air in Krakow on Krasinskiego Avenue in April 2018–2020. Source: own study based on data from ref. [ 25 ].
Summary of 20 days with the lowest and highest average daily concentration of NO x in the air in Krakow (on Krasinskiego Avenue), broken down by year
Source: Own study based on data from ref. [ 25 ].
Figure 4 compares the average monthly and average daily minimum and maximum value of NO x concentration for April in the analyzed years. In any event, the lowest results were recorded in 2020. The highest average monthly value and the maximum average-daily emission were recorded in 2018. In the case of the days with the lowest value of the average-daily NO x emission, 2019 was the least favorable. It should be noted that the maximum average-daily emission value in April 2020 (165 µg/m 3 ) is lower than the monthly average for 2018 (181 µg/m 3 ) and 2019 (171 µg/m 3 ).
![case study related to air pollution Figure 4
Comparison of average values, minimum and maximum of average-daily concentration of NOx in the air in Krakow at Krasinskiego Avenue in April 2018–2020. Source: own study based on data from ref. [25].](https://www.degruyter.com/document/doi/10.1515/eng-2021-0077/asset/graphic/j_eng-2021-0077_fig_004.jpg)
Comparison of average values, minimum and maximum of average-daily concentration of NO x in the air in Krakow at Krasinskiego Avenue in April 2018–2020. Source: own study based on data from ref. [ 25 ].
4 Comparison of the average level of pollutants
between 2018 and 2019,
between 2018 and 2020,
between 2019 and 2020.
the average for spring 2018 is higher than that for spring 2019,
the average for spring 2018 is higher than that for spring 2020,
the average for spring 2019 is higher than that for spring 2020.
Then, a statistical analysis was performed based on formula (1) [ 30 ]. The calculated Z value is a statistic that allows you to determine whether it is possible to reject the null hypothesis in favor of an alternative hypothesis (the Z value will be in the critical area) or to find that there are no grounds for rejecting it (the Z value will be within the acceptability area of the null hypothesis).
where: X ¯ n – arithmetic mean of the sample, S n – standard deviation of the sample, n – sample size.
For the significance level of 0.01, the critical area was determined < z α ; +∞, where z α = 2.33 [ 30 ].
Comparing the averages calculated for 2018 and 2019, the value Z 18,19 = 0.73 was obtained. Due to the fact that Z was not in the critical area, at the significance level of 0.01, there was no basis to reject the hypothesis of the equality of the average levels of nitrogen oxides concentration in spring 2018 and 2019. When comparing the averages calculated for 2018 and 2020, the highest result was obtained for statistics Z 18,20 = 7.77. Its value was in the critical area. Therefore, it was found that with a 99% probability one can reject the null hypothesis of equality of the averages from 2018 and 2020 in favor of the alternative hypothesis that the average concentration of NO x in Krakow in spring 2018 is higher than that in 2020. Compared to averages calculated for 2019 and 2020, the statistics result was Z 19,20 = 6.24. It falls in the critical area and it is slightly lower than that in the case of the analysis conducted for 2018 and 2020 ( Z 18,20 ). Therefore, at a significance level of 0.01, the hypothesis that the mean concentration of NO x in the spring in 2019 and 2020 can be rejected in favor of the alternative hypothesis such that the mean for 2019 is higher. The results of all significance tests are summarized in Table 2 .
Summary of significance test results
The condition of the air quality, apart from other factors, is also influenced – directly and indirectly – by weather conditions, in particular the movements of air masses, humidity, and temperature [ 23 ]. For temperature, its indirect relationship with the level of pollutants emitted is best visible in the case of the so-called low emissions during the heating season: the lower the temperature, the greater the demand for heating houses, therefore the combustion in household stoves and the amount of harmful substances released into the atmosphere increases. A similar relationship may occur between the temperature and the level of emissions from transport, which may be related to the comfort of travel. The lower the temperature, higher humidity, and more rainfall, theoretically people should choose to drive their own heated car than to walk, cycle, or use public transport and wait at stops. Increased road traffic may mean more transport pollution.
Bearing in mind the above, in order to complete the observations and analyzes, the average monthly temperatures in Krakow in the selected period of time (April 2018–2020) were analyzed, as well as the sum of precipitation and the number of days with rain. Their values are presented in Table 3 .
Average temperature, rainfall, and number of rainfall days in Krakow in April 2018–2020
Source: own study based on data from ref. [ 4 , 5 , 27 , 28 ].
Taking into account the values of average temperatures in April 2018–2020, theoretically the best conditions for using public transport and means of transport – other than motor vehicles – were April 2018. The least favorable in this respect was April 2020. Taking into account the sum of rainfall and the number of days when it occurred, April 2020 should be considered the most favorable in this regard, and the least April 2019. The analysis of the NO x emission level in the analyzed period shows no relationship between air pollution and the average monthly temperature in the whole analyzed period. Much more complex is the impact of rainfall and the inhabitant’s behavior. While the sum of rainfall in 2020 was the smallest – which in a sense could explain the reduction of NO x emissions – it is worth paying attention to the comparison of 2018 and 2019. In April 2019, the average daily amount of rainfall (on the days on which it was recorded) was much higher than that in April 2018. Despite this – as shown by the statistical analysis – the average concentrations of NO x in the air were at a similar level ( Table 2 ). This prompts us to conduct further, extended research taking this aspect into account. The lowest average temperature value recorded for the analyzed period in April 2020 indicates that the demand for heating power in households was not lower than in 2018–2019. Thus, the NO x emission level at that time was not lower. The actual reduction in its level can be associated mainly with the lockdown and the resulting limitation of the mobility of inhabitants. The weather conditions in April 2020 were not conducive to reduce the level of transport pollution, but it decreased compared to previous years.
5 Recapitulation
According to data for the entire city, which were made available by the Department of the Municipal Traffic Engineer of the City of Krakow, during the lockdown in spring 2020, road traffic in the city decreased by 30% to even 70% [ 23 ]. The authors did not obtain more precise data on the traffic volume in selected points in Krakow, e.g. on Krasinskiego Avenue – where the level of air pollution was analyzed. The reduction in the number of vehicles on the road was clearly noticeable and was reflected in the amount of transport pollutants emitted into the atmosphere. The performed statistical analysis showed a decrease in the mean values of the concentration of nitrogen oxides during the restrictions related to the COVID pandemic. April 2018 and 2019 did not differ significantly in relation to the average level of NO x concentration in the air. However, this cannot be said for 2020, when the average concentration of this pollutant was significantly lower than that in spring 2018 and 2019. In April 2020, the highest number of days with the cleanest air and the least with the most polluted air were registered in Krakow. This state of affairs was not related to the air temperature and the reduction of pollutant emissions from households, as April 2020 was the coldest in the analyzed period. Were it not for the limitations related to the COVID-19 pandemic, the inhabitants of Krakow would use private cars more often than public transport, bicycles, or other personal transport devices. There is a close relationship between air quality and the size of traffic flows. This confirms the desired direction of activities consisting in the development of low-emission or zero-emission means of transport. It is also appropriate to restrict passenger car traffic and to replace them with public transport or active mobility. It is worth mentioning here that Polish cities (with a population of over 100,000) have relatively new tools to combat transport pollution. These are clean transport zones. They make it possible to designate areas that can only be entered by vehicles powered by electricity, hydrogen, or natural gas [ 29 ]. So far, only Krakow in the Kazimierz districts, as well as the Old Town, has used this option ( Figure 5 ).

Marking of the clean transport zone in Krakow.
The need for further research on the impact of the size of road traffic flows on air pollution, both locally and globally, should be emphasized. The period of the COVID-19 pandemic should become a valuable lesson for the future and a knowledge base for further studies and implementations. In particular, units responsible for the organization of road traffic and the development of public transport should show interest in this regard.
Conflict of interest: The authors declare that they have no known conflict of interest or personal relationships that could have appeared to influence the work reported in this article.
[1] Ustawa z dnia 2 marca 2020 r. o szczególnych rozwiązaniach związanych z zapobieganiem, przeciwdziałaniem i zwalczaniem COVID-19, innych chorób zakaźnych oraz wywołanych nimi sytuacji kryzysowych Act of 2020, Pub. L. No. 374. Poland; 2020 Mar 2. Search in Google Scholar
[2] Plan zrównoważonego rozwoju publicznego transportu zbiorowego dla gminy Kraków i gmin sąsiadujących, z którymi gmina Kraków zawarła porozumienie w zakresie organizacji publicznego transportu zbiorowego – Krakow City Council Resolution No. LXXX/1220/13. Poland; 2013 Aug 28. Search in Google Scholar
[3] Kowal S. Krakowska Tarcza dla Mobilności, Mobilność w Krakowie 2020 [Internet]; 2020 Apr [cited 2020 Oct 23]. Available from: http://mobilnykrakow.pl/krakowska-tarcza-dla-mobilnosci Search in Google Scholar
[4] “Mark” Ekstremalnie ciepły kwiecień Post [Internet]. Poland: “Mark”; 2018 May – [cited 2020 Nov 6]. Available from: https://meteoprognoza.pl/2018/05/03/ekstremalnie-cieply-kwiecien/ Search in Google Scholar
[5] “Mark” Kwiecień – sucho, słonecznie, ale bez znacznych skoków temperatury Post [Internet]. Poland: “Mark”; 2020 May 3 – [cited 2020 Nov. 6]. Available from: https://meteoprognoza.pl/2020/05/03/kwiecien-sucho-slonecznie-ale-bez-znacznych-skokow-temperatury/ Search in Google Scholar
[6] Pielecha J, Skobiej K, Kurtyka K. Testing and evaluation of cold-start emissions from a gasoline in RDE test at two diffrent ambient temperatures. Open Eng. 2021;11:425–34. 10.1515/eng-2021-0047 Search in Google Scholar
[7] Ambrozik A, Ambrozik T, Łagowski P. Fuel impact on emissions of harmful components of the exhaut gas from the CI engine during cold start-up. Maint Reliab. 2015;17(1):95–9. 10.17531/ein.2015.1.13 Search in Google Scholar
[8] Reiter MS, Kockelman KM. The problems of cold starts: a closer look at mobile source emissions levels. Transp Res Part D Transp Environ. 2016 Mar;43:123–32. 10.1016/j.trd.2015.12.012 Search in Google Scholar
[9] Kalawapudi K, Singh T, Vijay R, Goyal N, Kumar R. Effects of COVID-19 pandemic on festival celebrations and noise pollution levels. Noise Mapp. 2021;8:89–93. 10.1515/noise-2021-0006 Search in Google Scholar
[10] Zambon G, Confalonieri CH, Angelini F, Benocci R. Effects of COVID-19 outbreak on the sound environment of the city of Milan, Italy. Noise Mapp. 2021;8:116–28. 10.1515/noise-2021-0009 Search in Google Scholar
[11] Gevu N, Carvalho B, Fagerlande GC, Niemeyer M, Medeiros Cortes M, Torres JCB. Rio de Janeiro noise mapping during the COVID-19 pandemic period. Noise Mapp. 2021;8:162–71. 10.1515/noise-2021-0012 Search in Google Scholar
[12] Aletta F, Brinchi S, Carrese S, Gemma A, Guttari C, Mannini L, et al. Analysing urban traflc volumes and mapping noise emissions in Rome (Italy) in the context of containment measures for the COVID-19 disease. Noise Mapp. 2020;7:114–22. 10.1515/noise-2020-0010 Search in Google Scholar
[13] Baratalucci CH, Borchi F, Carfagni M. Noise monitoring in Monza (Italy) during COVID-19 pandemic by means of the smart network of sensors developed in the LIFE MONZA project. Noise Mapp. 2020;7:199–211. 10.1515/noise-2020-0017 Search in Google Scholar
[14] Yildirim Y, Arefi M. Noise complaints during a pandemic: a longitudinal analysis. Noise Mapp. 2021;8:108–15. 10.1515/noise-2021-0008 Search in Google Scholar
[15] Asdrubali F, Brambilla G. Noise mapping special issue: the noise climate at the time of SARS-CoV-2 Virus/COVID-19 Disease. Noise Mapp. 2021;8:204–6. 10.1515/noise-2021-0015 Search in Google Scholar
[16] Liu Z, Kong H. New evidence of the effect of Beijing’s driving restriction and other Olympic-year policies on air pollution. BE J Econ Anal Policy. 2021;21(1):241–72. 10.1515/bejeap-2019-0295 Search in Google Scholar
[17] Dlaczego w Krakowie jest smog? Szybka odpowiedź [Internet]; 2019 Nov 12 [cited 2021 May 8]. Available from: https://krakow.dlawas.info/zdrowie/dlaczego-w-krakowie-jest-smog-szybka-odpowiedz/ Search in Google Scholar
[18] Sówka I. Transport drogowy jako źródło zanieczyszczenia powietrza w miastach. Czysta Energia. 2017;1–2:24–8. Poland. Search in Google Scholar
[19] World Health Organization. Health and environment in Europe: progress assessment. Copenhagen, Denmark: WHO; 2010. Search in Google Scholar
[20] Gis M, Pielecha J, Gis W. Exhaust emissions of buses LNG and Diesel in RDE tests. Open Eng. 2021;11:356–64. 10.1515/eng-2021-0038 Search in Google Scholar
[21] Wyszomirski O, Hebel K, Grzelec K. Zarządzanie zbiorowym transportem miejskim w warunkach polityki zrównoważonej mobilności. Gdańsk: Wydawnictwo Uniwersytetu Gdańskiego; 2020. Poland. Search in Google Scholar
[22] Zaprawa K, Filipowicz P, Filipowicz J. Emisja zanieczyszczeń spalinowych przez autobusy komunikacji miejskiej. Autobusy – Technika, Eksploatacja, Systemy Transportowe. 2017;9:52–5. Poland. Search in Google Scholar
[23] Mikulski M. Efektywność bezpłatnego transportu miejskiego w walce z zanieczyszczeniem powietrza. Przegląd Komunikacyjny. 2020;6–8:9–13. Search in Google Scholar
[24] Wojtal R. Zanieczyszczenie powietrza w miastach w aspekcie ruchu samochodowego. Transport Miejski i Regionalny. 2018;1:12–7. Poland. Search in Google Scholar
[25] System monitoringu jakości powietrza Wojewódzkiego Inspektoratu Ochrony Środowiska w Krakowie [internet] [cited: 2020 Nov 6]. Available from: http://monitoring.krakow.pios.gov.pl/dane-pomiarowe/automatyczne/ Search in Google Scholar
[26] [map][Internet] [cited 2020-11-06]. Available from: www.google.pl/maps Search in Google Scholar
[27] “Mark” Bardzo ciepło w kwietniu, ale i sucho. Post [Internet] Poland: “Mark”; 2019 May 12 [cited 2020 Nov 6]. Available from: https://meteoprognoza.pl/2019/05/12/bardzo-cieplo-w-kwietniu-ale-i-sucho/ Search in Google Scholar
[28] Archive database – rainfall and rainfall days [Internet] [cited 2021 May 8]. Available from: https://weatheronline.pl/ Search in Google Scholar
[29] Ustawa z dnia 11 stycznia 2018 r. o elektromobilności i paliwach alternatywnych. Act of 2018, Pub. L. No 317; 2018 Jan 11. Poland. Search in Google Scholar
[30] Wasilewska E. Statystyka matematyczna w praktyce. Warszawa: Difin; 2015. p. 225–7. Poland. Search in Google Scholar
© 2021 Maciej Mikulski et al ., published by De Gruyter
This work is licensed under the Creative Commons Attribution 4.0 International License.
- X / Twitter
Supplementary Materials
Please login or register with De Gruyter to order this product.
Journal and Issue
Articles in the same issue.
- Open supplemental data
- Reference Manager
- Simple TEXT file
People also looked at
Original research article, the use of tree barks to monitor traffic related air pollution: a case study in são paulo–brazil.
- 1 Departamento de Patologia, Faculdade de Medicina, Universidade de São Paulo, São Paulo, Brazil
- 2 Departamento de Estatistica, Instituto de Matemática e Estatística, Universidade de São Paulo, São Paulo, Brazil
- 3 Departamento de Geografia, Faculdade de Filosofia, Letras e Ciências Humanas, Universidade de São Paulo, São Paulo, Brazil
- 4 Instituto de Estudos Avançados, Universidade de São Paulo, São Paulo, Brazil
- 5 Instituto de Pesquisas Energéticas e Nucleares, Comissão Nacional de Energia Nuclear, São Paulo, Brazil
- 6 Instituto de Biociências, Universidade de São Paulo, São Paulo, Brazil
The analysis of chemical elements in the barks of trees is an alternative procedure to access spatial heterogeneity of traffic related air pollution. However, the role of tree species in the characterization of the variability of airborne pollution is poorly known. We present an observational study conducted in São Paulo, Brazil, based on the analysis of 498 trees from three common species: Tipuana tipu, Poincianella pluviosa , and Ligustrum sp . We considered ANCOVA models to compare the concentrations of Al, Fe, Zn, Cu, Mn, Ba, and S in the bark (periderm) of trees located close to streets with different levels of traffic intensity controlling for the extension of nearby green areas. The expected trend of increasing elemental concentration in the bark of trees located near streets with greater traffic intensity or close to smaller green areas was only fully evidenced by T. tipu . For instance, the concentrations of Zn, Fe, Al, and Ba increase by 200, 350, 230, and 280% respectively, for trees of this species located near arterial streets when compared to those observed near local streets. On the other hand, the concentrations of Zn, Fe, Al, and Ba are reduced by 41, 45, 50, and 30%, respectively, for trees located near green areas. For P. pluviosa , the capacity to suggest an association between the tree bark concentration of chemical elements with increasing levels of air pollution and presence of green areas was only fully observed for Zn and Cu. For Ligustrum sp., weaker and sometimes non-expected associations between bark concentrations of the chemical elements and either street classification or green area extension were observed. Our results indicate that the choice of species is a key element in the use of tree barks as a biomonitoring tool in urban landscapes. Species like T. tipu , with rough and highly porous bark, are the most appropriate for such purpose.
Introduction
Airborne pollution is a current problem in highly populated megacities such as São Paulo, Brazil, one of the largest in the world ( World Urbanization Prospects, 2017 ). It has about 12 million inhabitants ( IBGE, 2017 ) and a fleet of 8 million vehicles ( DETRAN-State Department of Traffic of São Paulo, 2017 ) corresponding to almost 1 car for each 1.5 inhabitants. Currently, its citizens are exposed to an average annual concentration of 21.7 μg/m 3 of PM 2.5 ( Cheng et al., 2016 ), mostly generated by vehicular traffic ( Andrade et al., 2012 ). The characterization of spatial variability of airborne pollutant concentrations and source apportionment is a topic of major importance for studies designed to quantify their adverse effects on human health ( Pope et al., 2002 ; Mauad et al., 2008 ; Pereira Filho et al., 2008 ; Baccarelli, 2009 ; Zanobetti et al., 2009 ; de Brito et al., 2010 ; Santos et al., 2016 ). The environmental heterogeneity observed within cities, including non-uniform distribution of traffic intensity and extension of green areas ( Janhäll, 2015 ), demands high-resolution measurements of airborne pollution. Accessing the variability of air pollution in such complex urban environments requires a dense network of measuring devices, which are usually scarce in developing countries. In addition, many of the available monitoring sites only provide measurements of particulate matter concentration with no indication of the associated chemical composition.
As an alternative, biomonitoring methods are already used in many countries ( Falla et al., 2000 ) to address variability of air pollution in large geographical areas (e.g., Kuik and Wolterbeek, 1994 ; Böhm et al., 1998 ). Tree barks have been recently used to evaluate a fine spatial distribution of chemical elements concentration ( Guéguen et al., 2012 ; Ejidike and Onianwa, 2015 ), which increases with the levels of traffic intensity ( Moreira et al., 2016 ). Such biomonitoring results may have an accuracy of 100 m ( Carneiro et al., 2011 ). However, the complexity of air pollution in cities depends not only on its sources, but also on the filtering properties of green areas. Although generally neglected in biomonitoring studies, the presence and the size of green spaces are also important to understand and adequately characterize the dispersion and the concentration of air pollution. Several studies suggest that local air pollution is reduced by the presence of trees ( Vailshery et al., 2013 ; Nowak et al., 2014 ; Janhäll, 2015 ; Jeanjean et al., 2016 ). Several factors are involved in the filtering and dispersion of air pollution by the presence of trees ( Selmi et al., 2016 ). The main interaction is the dry deposition which is the mechanism by which vegetation removes air pollutants from the troposphere in non-precipitation periods. It depends on the combination of pollutants (e.g., gaseous/particles), surfaces (e.g., size; roughness; chemical nature), and microclimate (e.g., wind speed and direction; temperature; solar radiation; air turbulence; Selmi et al., 2016 ).
Despite the recent advances stemming from tree bark studies ( Catinon et al., 2009 ; Perelman et al., 2010 ; Guéguen et al., 2012 ; Moreira et al., 2016 ), identification of tree species with better capacity to record the fine spatial variability of air pollution is still poorly known and constitutes an important research topic. Bark morphology is subject to a high degree of interspecific variability ( Yunus et al., 1990 ; Junikka, 1994 ) with potential to influence the species ability to record airborne element concentrations ( Szczepaniak and Biziuk, 2003 ). This issue is of special concern because some species might better suit the biomonitoring role than others.
The objective of this observational study is to compare different trees species via the concentrations of chemical elements in their barks with respect to their potential to discriminate traffic generated air pollution, controlling for the presence of green areas. In particular, we focus our attention on Al, Fe, and Cu, that are related to re-suspension of road dust, on Ba, Cu, and Zn, that are related to brake and tire wear and on Mn and S, that are related to fossil fuel emissions as indicated in Liu et al. (2007) , de Almeida Albuquerque et al. (2012) , and Hetem and Andrade (2016) . These specific emission sources were also identified in São Paulo in the study conducted by Moreira et al. (2016) . We considered three tree species, Poincianella pluviosa (DC.) L.P. Queiroz (former Caesalpinia pluviosa ), Tipuana tipu (Benth.) Kuntze, and Ligustrum sp. L., to quantify their abilities to record the fine spatial variability of air pollution. These species were chosen because they are widely distributed in São Paulo and have different external bark morphologies.
Study Site and Species
Samples of tree barks of the three selected species were collected in a large region of São Paulo (Figure 1 ), which includes areas under the influence of no green spaces to large urban parks. São Paulo has a tropical to subtropical climate with a well-defined dry season from June to August. Mean temperature is 20.7°C, and annual precipitation is 1,545 mm. Overall, the main wind direction is southeast with an average speed of 2.6 m/s (refer to the Supplementary Material for a climate diagram and wind rose, Figure S1). This heterogeneous region includes residential and commercial areas, but no industrial activities. The automotive fleet in São Paulo is composed by cars (78.9%), motorcycles (16.3%), trucks (1.6%) and buses (2.7%) ( Companhia de Engenharia de Tráfego, 2017a ).
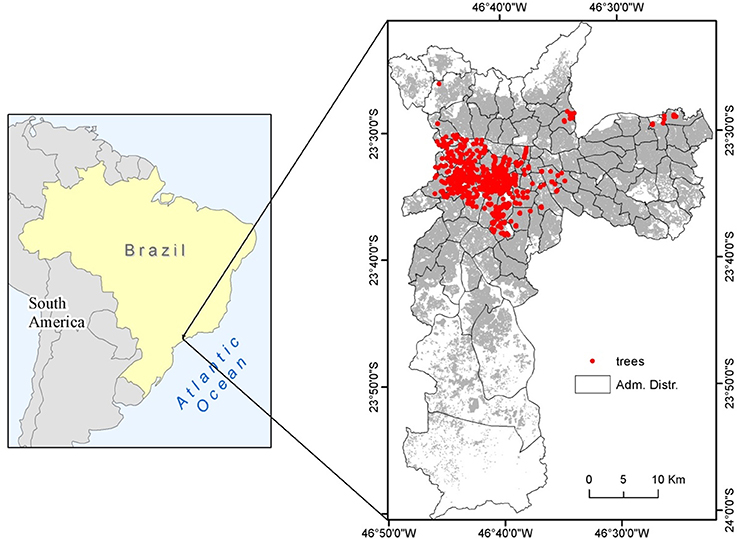
Figure 1 . Location of all sampled trees and districts in the study area (São Paulo, Brazil).
The distribution of the sampled trees is a consequence of the distinct density and heterogeneity of their location in each district of São Paulo; this explains the low number of trees selected in the eastern district. Both street trees and trees growing in parks were sampled in the vicinity of streets with different traffic intensities classified according to the city traffic authority ( Companhia de Engenharia de Tráfego, 2017b ). This classification is based on street characteristics and on the importance of the traffic flow and consists of the following four categories in decreasing order of traffic intensity: Express, Arterial, Collector or Local. Given the existence of few trees located in the vicinity of Express streets, we combined the Express and Arterial categories. We also reclassified the trees originally placed near local streets into two groups: Local I, if their distances to streets with higher traffic intensity were < 100 m and Local II, otherwise. We also classified the sampled trees according to the spatial proportion of green areas as: Inexistent/Very small (0–20% of green area cover), Small (20–40%), Medium (40–60%), and Dominant/Large (>60%) ( Tarifa and Azevedo, 2001 ).
The sampled trees belong to the three most common tree species in São Paulo Tipuana tipu is an exotic species from Bolivia that was extensively planted in the middle of the twentieth century in São Paulo. It is also present in other Brazilian cities, as well as in other countries from South America ( Breuste, 2013 ; Mazza et al., 2013 ; Teixeira, 2014 ), North America ( Legumes, 2017 ), Africa ( Kuruneri-Chitepo and Shackleton, 2011 ), Europe ( Sashua-Bar et al., 2010 ; Soares et al., 2011 ), and Oceania (check the Supplementary Material for the map of species distribution based on herbaria records, GBIF, 2018 , Figures S2–S4). These trees are deciduous and they reach large sizes in São Paulo, with diameter at breast height (DBH) close to one meter and height of 20 m. Ligustrum sp. is also an exotic tree species from Asia, that is largely available in cities from different continents. These trees are usually smaller, with DBH up to 50 cm and height up to 15 m in São Paulo. Ligustrun sp. trees are evergreen in São Paulo. The only native species is Poincinella pluviosa , reaching DBH values up to 60 cm and height of up to 20 m.
Morphological Characterization of the Species Bark
In order to support the discussion of the role of tree species in the results of biomonitoring studies using tree barks, we qualitatively characterized the external morphology of the studied species. Since the external bark morphology is a rather conservative characteristic ( Junikka, 1994 ), we only evaluated major differences in bark macroscopic rugosity and microscopic cellular characteristics, not accounting for the effects of bark plasticity on the results. For this purpose, we selected similarly sized individuals of each species, with diameter at breast height (DBH) of about 30 cm, from which we collected 5 × 5 cm samples of the bark. These samples were analyzed by two different methods to qualitatively describe their macroscopic and microscopic bark characteristics. Rugosity was evaluated as the variation in surface depth. We used a stereomicroscope (Leica M205 FA) to create optical slices of the bark external surface and compute their depth profile (LAS software V 4.8) in three transects. These profiles represent the amplitude of variability of the surface depth. Then, we obtained a 5 × 5 mm sample to analyse the cellular characteristics of the bark surface using a scanning electron microscope (SEM, Zeiss Sigma VP). Before running the analysis, all samples were coated with gold to enhance contrast in the SEM images.
Bark Sampling and Preparation
The sample consisted of 498 trees (including 158 from Moreira et al., 2016 ) of which 193 trees were of the species T. tipu (38.9%), 228 trees were of the species P. pluviosa (45.6%) and 77 trees were of the species Ligustrum sp. (15.5%). Sampling occurred in the years of 2012, 2013, and 2014. The spatial distribution of the sampled trees according to species as well as to the different São Paulo districts is displayed in Figure 2 . Sampling was conducted in the dry seasons (from June to August, when monthly precipitation is lower than 50 mm) in order to avoid rain washing and sample degradation by fungi. All tree barks were obtained with titanium tools to avoid contamination. From each selected tree, a portion of 8 × 8 cm of the periderm was collected from each quadrant of the trunk according to the cardinal points at a height of 1.5–2.0 m from the topsoil and these portions were pooled for analysis. The harvesting of bark in four sectors is a conservative approach to have a representative sample independently of the possible existence of a predominant wind direction or of other external factors that may create variations of environmental exposure along the trunk surface. Samples were placed in paper bags and stored in a cabinet under low humidity conditions until the analysis could be conducted. Information regarding tree geographical coordinates was also recorded.
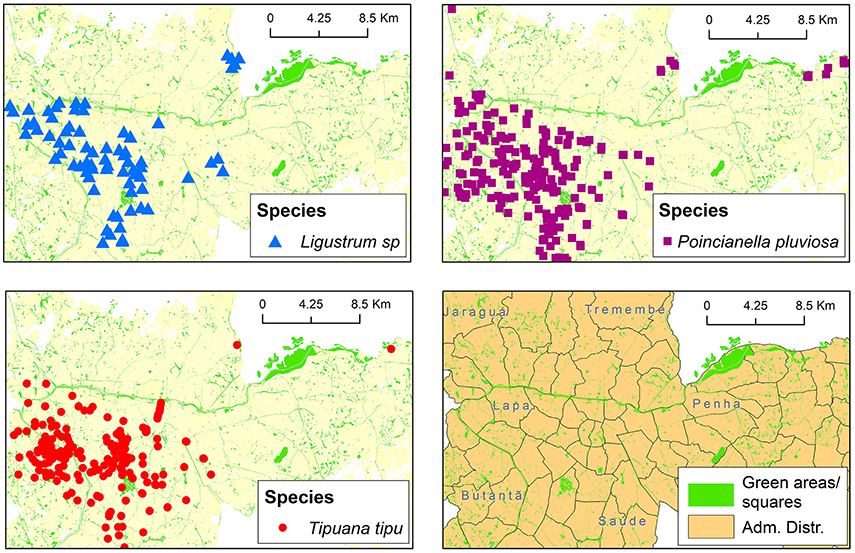
Figure 2 . Location of the sampled trees by species in São Paulo and the presence of green areas in the study site.
The collected tree barks were first cleaned using a soft nylon dental brush to eliminate external materials such as dead insects, dust and lichens. The samples were obtained by grating the outermost 3 mm of the bark with a titanium tool ( Wolterbeek and Bode, 1995 ). Each sample was then ground to powder using a vibratory micro-mill with agate mortar ( Fritsch Pulverisette 0, Fritsch GmbH, Idar-Oberstein, DE). The powder was compressed by applying a pressure of 3.9 ton/cm 2 to make a double layer pellet composed of 0.5 g of tree bark powder and 1 g of boric acid (H 3 BO 3 , p.a. ACS reagent).
Elemental Analysis
The bark samples were submitted to an energy dispersive X-ray fluorescence spectrometer-EDXRF (EDX 700-HS, Shimadzu Corp., Kyoto, Japan). The pellets were irradiated with X-rays (source). The emitted fluorescent X-rays (secondary) have a unique wavelength and energy that characterizes each element contained in the sample. As the fluorescent X-ray intensity is a function of the concentration, quantitative analysis is performed by measuring the amount of X-rays of each element ( Van Grieken and Markowicz, 2002 ). This technique was chosen due to its multi-element capability, sensitivity, easy sample preparation without sample digestion, accuracy of the results and analysis cost. The concentrations of Al, Fe, Zn, Cu, Mn, Ba, and S were recorded for having well-described sources in São Paulo ( Moreira et al., 2016 ). These elements may be considered as proxies of the complex mixture generated by vehicular emissions. Although other elements were also measured via the EDXRF, their values were close to the limits of detection. The measurement parameters were: time (240 s), target (Rh, 5–50 kV _ 1–1,000 A) and Si (Li) detector in a vacuum atmosphere. The calibration curves were fitted via linear regression methods using specific parameters of the equipment to correct the matrix effects. A certified reference material [Peach Leaves #1547, National Institute of Standards and Technology (NIST), Gaithersburg, MD, USA] was also analyzed using the same procedure for quality control purposes (Table S1).
Statistical Analysis
The distribution of elemental concentration was described via boxplots constructed by species, street classification and green area extension, as well as via tables with descriptive statistics. The association between element concentration in the tree barks and air pollution as measured via street classification, controlling for species, green area extension, diameter at breast height and sampling campaign was assessed by analysis of covariance (ANCOVA) models ( Neter et al., 2005 ). Therefore, differences in the size of the trees and sampling campaign are accounted for with this model. An interaction term between species and street classification was included in the model to verify if the three species record different patterns of air pollution in their barks. Because residual analyses suggested coarse deviations from the usual Gaussian model assumptions, the data were reanalysed considering the logarithm of element concentration as response.
Non-significant (for α = 0.05) variables and interactions between variables were dropped from the initial model and predicted concentrations of the chemical elements for different street classifications by species and extension of green areas were computed via the final model equation. Bootstrap estimators of prediction standard errors ( Efron, 1982 ) were considered. The model predicted values were used to compare the mean concentrations of the elements across streets with different traffic intensities for each species, controlling by the other factors. Detailed results are given for Zn in the main text because of space limitations, but all other results are presented in the Supplementary Material (Tables S3.1–S3.7).
The three studied species have different external bark morphologies (Figure 3 ). Our qualitative analysis shows that Tipuana tipu has a relatively deeply furrowed bark consisting of a loose tissue where cells have their lumen exposed to the atmosphere. Not only the outermost layer of the cork has its lumen open to the outside environment, but it seems that this is also true for the lumen of few inner layers of cork cells. Neither P. pluviosa nor Ligustrum sp. have a striped bark like the former species, but they have different porosity characteristics. The analyzed portion of the bark of P. pluviosa is slightly more compact when compared to that of T. tipu . The cork cells of P. pluviosa are stacked and only the lumen of the outermost cells is exposed to the atmosphere. Cells of the bark of Ligustrum sp. are even more compactly arranged and their lumen is rarely exposed to the environment.
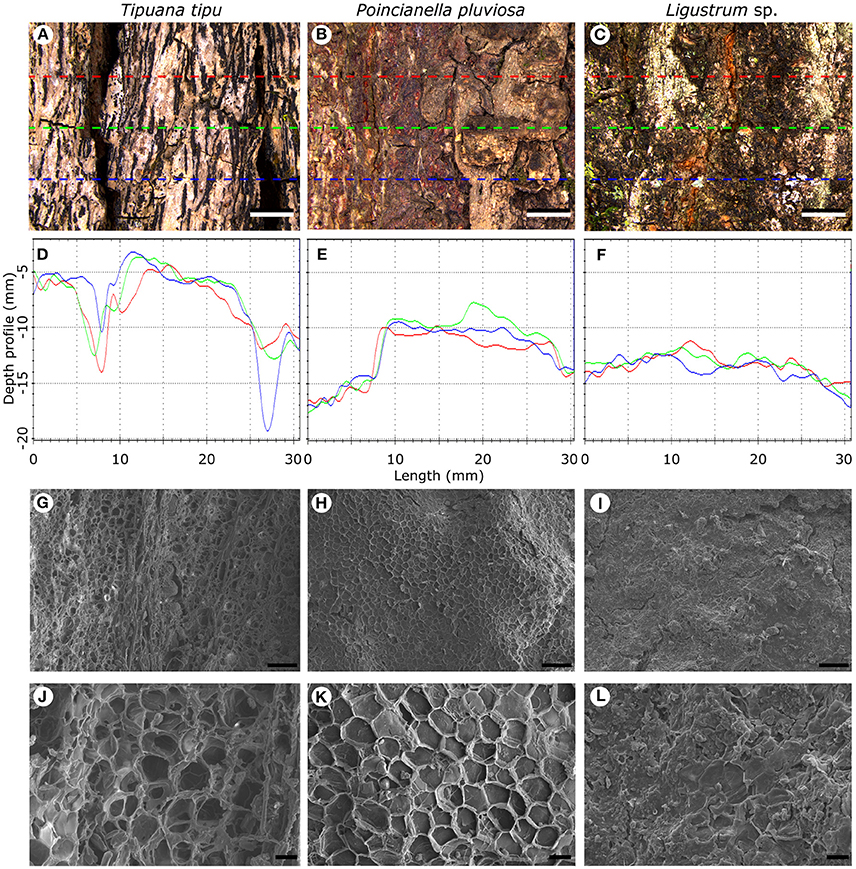
Figure 3 . External bark morphology of Tipuana tipu, Poincianella pluviosa and Ligustrum sp. including a macroscopic view of the bark surface ( A–C , 5 mm) and the respective depth profiles of surface height variability ( D–F ; line colors represent the transects highlighted as dashed lines in the macroscopic images); a microscopic view of the surface porosity in lower ( G–I , 100 μm) and higher magnification ( J–L , 20 μm).
In general, the observed median concentration of Al, Fe, Zn, Cu, Mn, and Ba in the barks of T. tipu increased with their proximity to streets with higher traffic intensity, from Local II to Arterial (Figure 4 ). Only S did not show such a trend. In the barks of P. pluviosa , a similar pattern was observed for the median concentrations of Zn, Cu, and Ba. Ligustrum sp. was the only species for which the trends in the median tree bark concentrations were not as consistent as the trends observed for the other two species. For T. tipu , the median elemental concentrations of all elements but S tend to increase as the extension of nearby green areas extensions decrease from Dominant/Large to Very Small/Inexistent (Figure 5 ). For P. pluviosa the same pattern was observed for the median concentrations of all elements but S, Mn, and Ba. Such a trend was not detected for any element in the barks of Ligustrum sp.
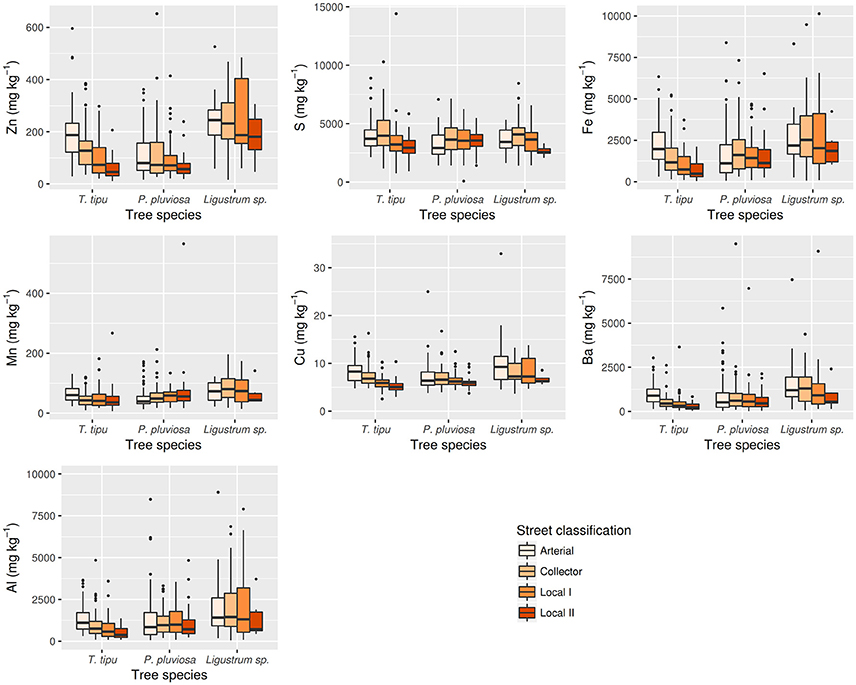
Figure 4 . Boxplots of elemental concentration on tree barks of Tipuana tipu, Poincianella pluviosa and Ligustrum sp. by different street categories: Arterial, Collector, Local I, and Local II.
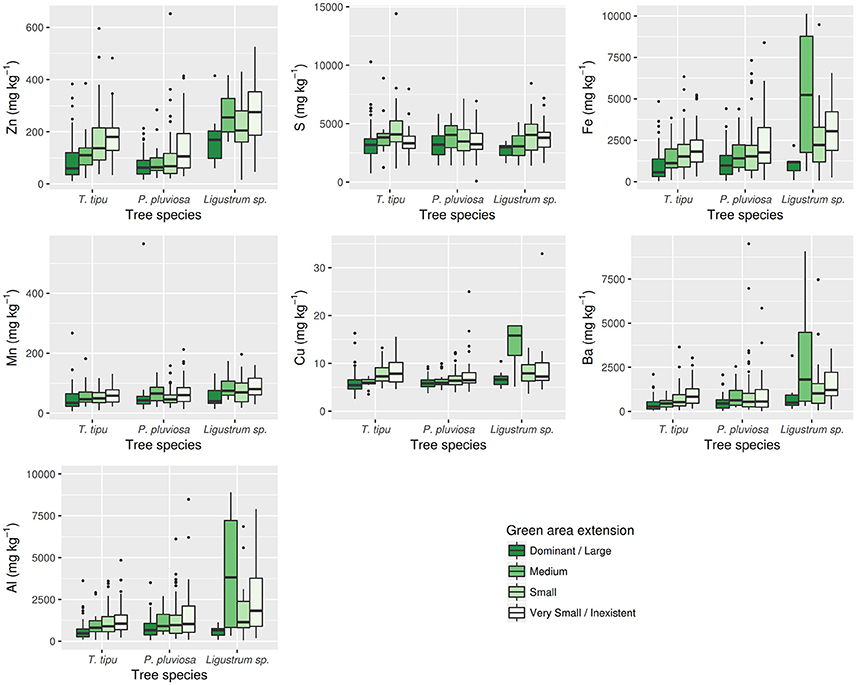
Figure 5 . Boxplots of elemental concentration on tree barks of Tipuana tipu, Poincianella pluviosa , and Ligustrum sp. by different green areas extension: Dominant/Large, Medium, Small, Very Small/Inexistent.
Details on the distribution of the observed Zn concentration in T. tipu tree barks taking into account both street classification and the extension of green areas are shown in Table 1 . Overall, the average and median values of Zn concentration decrease for trees located near Arterial to Local II categories, within each green area extension category. The highest values of the concentration of Zn are found in trees located near Arterial or Collector streets with Small to Inexistent green areas. On the other hand, the smallest values are found in the bark of trees located near Local II streets with Large to Dominant green areas. But these results do not take into account the possible role of other factors like tree size, differences of sampling campaigns, and driving conditions including speed and intensity of breaks use. These factors, however, were taken into account in the ANCOVA model to precisely define the variation of the elemental concentration attributed to traffic intensity and green area extension. The P -values corresponding to significance tests for the main effects and interaction terms of the ANCOVA models are displayed in Table 2 . The interaction between species and street classification is significant for all elements. On the other hand, the effect of the sampling campaigns is significant for all elements but Zn, and DBH is only associated with the concentration of Cu, Mn and Ba. This result, along with an analysis of the boxplots, suggest different patterns of association between the element concentration and traffic intensity for the three species. In particular, T. tipu stands out as the species with the highest sensibility to changes in traffic intensity, followed by P. pluviosa . Ligustrum sp. is less sensible to changes in traffic intensity.
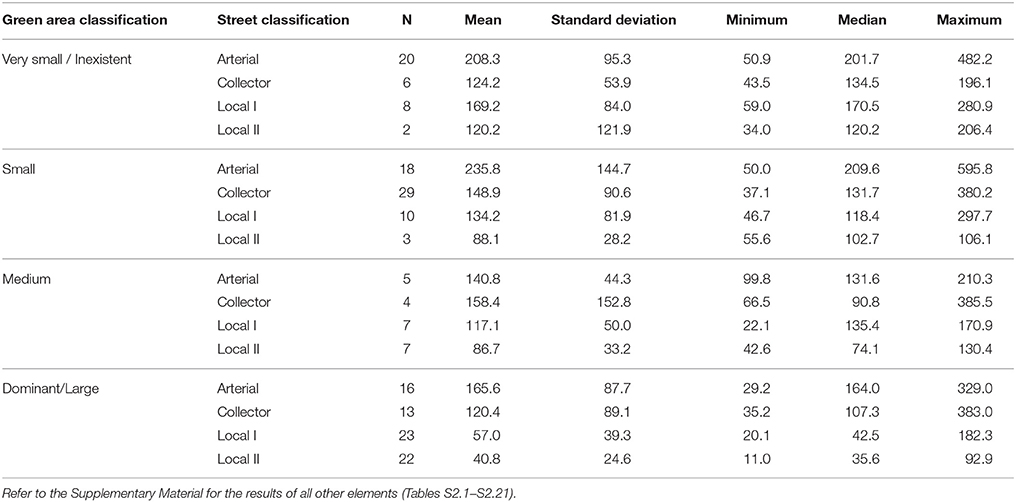
Table 1 . Descriptive statistics of Zn concentrations (mg kg −1 ) in the barks of Tipuana tipu according to green areas size and street classification.
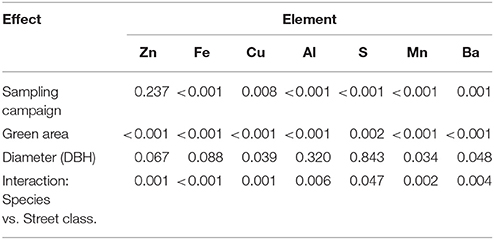
Table 2 . P values for effects in analysis of covariance models.
The predicted mean concentrations of Zn from the ANCOVA models are shown in Table 3 (the predicted mean concentrations of Fe, Cu, S, Al, Mn, and Ba are available in the Tables S3.1–S3.6). These predicted means (adjusted for the mean DBH) were computed from the final model containing only significant effects. The results presented in Table 3 clearly show that the expected trend of decreasing mean element concentrations from streets with heavy traffic to local streets is more evident for T. tipu , corroborating the previous conclusions. In the bark of this species, the concentration of Zn increased by 200% from Local II to Arterial streets. On the other hand, it decreased by 41% from inexistent to large green areas. This trend is still present for P. pluviosa , in which the concentrations of Zn increased by 37% from Local II to Arterial streets, and decreased by 41% from inexistent to large green areas. Although the concentrations of Zn increased by 40% from Local II to Arterial streets, and decreases by 41% from inexistent to large green areas for Ligustrum sp., the prediction error is higher for this species.
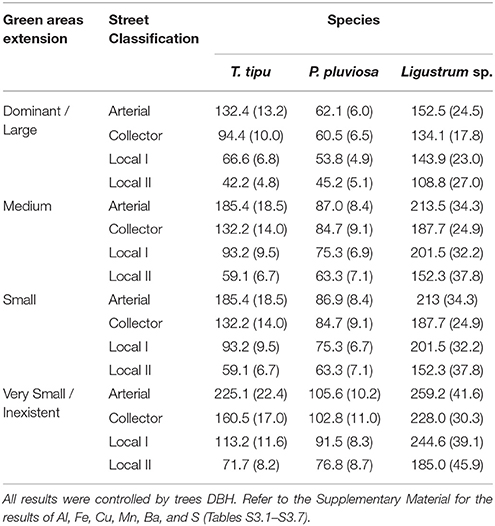
Table 3 . Predicted Zn concentration means and standard errors (mg kg −1 ) based on the ANCOVA models.
The concentration of Fe is also strongly dependent on traffic intensity and green area extension as evidenced by T. tipu . In the bark of this species, the concentration of Fe increased by 350% from Local II to Arterial streets. On the other hand, it decreased by 45% from inexistent to large green areas. Aluminum is another element that showed relevant differences evidenced by the bark of T. tipu . The concentration of Al increased by 230% from Local II to Arterial streets, while it decreased by 50% from inexistent to large green areas. Such differences were also found for Ba, that increases by 280% from Local II to Arterial streets, while it decreases by 38% from inexistent to large green areas. Differences for Cu, Mn, and S are less evident, especially when analyzed in the barks of P. pluviosa and Ligustrum sp.
Most urban agglomerations with more than 10 million inhabitants are currently found in developing countries ( United Nations, 2012 ). These regions have high levels of urban air pollution ( Baklanov et al., 2016 ) concentrating more than 80% of related deaths ( Baklanov et al., 2016 ; WHO, 2017 ). In general, their urban landscapes are highly complex and the evaluation of the effect of air pollution on health outcomes must consider their inherent heterogeneity. Biomonitoring methods based on tree barks have been considered as an alternative and/or as a complement to field measurements ( Falla et al., 2000 ) and to satellite image data ( Van Donkelaar et al., 2016 ), which are highly cost restrictive specially for developing countries ( Molina et al., 2004 ). We evaluate the role of tree species in low cost passive biomonitoring techniques designed to provide a higher spatial resolution of exposure to chemical elements composition required by environmental epidemiology ( Pope et al., 2009 ; Bravo et al., 2015 ).
In this context, a higher element concentration is expected in trees located close to streets with high traffic intensity. This is especially true in São Paulo where most of the air pollution is generated by traffic ( Moreira et al., 2016 ). Indeed, this is exactly the trend observed in the elemental concentration in the tree barks of this study. This trend is more evident for trees of the species T. tipu , as well as for few elements measured in P. pluviosa . Ligustrum sp., on the other hand, stands out as a species that is not reliable for biomonitoring studies based on tree barks. Element concentration in the barks of trees of this species does not discriminate between streets with different traffic intensities. These expected trends, which are usually higher in the barks of trees located in the vicinity of Arterial streets, were not observed for S in any species. Sulfur captured by tree barks in this study is mainly particulate and has a stronger interaction with tree surface ( Yli-Pelkonen et al., 2017 ). The gaseous phase (SO 2 ) is relatively negligible in São Paulo ( Miranda et al., 2012 ). This odd trend is probably a result of the higher dispersion capability of S which is subject to long-range transportation ( Masri et al., 2015 ).
In addition to traffic intensity, the presence of different extensions of green areas is also associated with the concentration of elements in tree barks. Indeed, air pollution is considerably lower in areas under the influence of vegetation, not only because of the filtering properties of leaves ( Bolund and Hunhammar, 1999 ), but also because of the passive deposition on the surface of the plant body, aerodynamic dispersion properties of tree structure ( Janhäll, 2015 ; Jeanjean et al., 2016 ) and higher wind speed and lower temperature ( Kassomenos et al., 2014 ) in open green areas ( Oliveira et al., 2011 ). Based on these properties, a decreasing element concentration in the tree barks along a gradient of increasing influence of green areas is expected. Once more, this is the trend observed with the barks of T. tipu for all studied elements but Mn and S. A similar result was found in the barks of P. pluviosa but not in the barks of Ligustrum sp. In fact, this species showed a rather unexpected result, with a higher concentration of elements in trees under the influence of medium sized green areas.
Our results point toward the importance of the choice of the appropriate species for biomonitoring studies based on tree barks. The biodiversity available in an urban environment like the one in São Paulo adds a complication to the problem of choosing the most appropriate species for this type of study ( Falla et al., 2000 ). This choice must be based on the combination of spatial coverage and ability to distinguish between different levels of local air pollution. Geographic distribution is probably the main reason used for choosing a species ( Böhm et al., 1998 ; Pacheco et al., 2002 ; Minganti et al., 2015 ). However, the effect of the interspecific morphology of barks on the results, although important ( Sawidis et al., 2011 ), is generally poorly considered (e.g., Oliva and Mingorance, 2006 ) especially in studies whose goal is a fine characterization of urban air pollution.
Some species may not show differences in element concentrations where they actually exist or, even worse, may generate misleading results. The different abilities to detect spatial variability of air pollution seems to depend on the bark external morphology. In our study, the species specific bark morphologies ranged from a highly porous and rough bark on T. tipu to a rather smooth bark with low porosity on Ligustrum sp., while P. pluviosa shows intermediate bark characteristics. This gradient of qualitative features seems to be in accordance with the results of the ANCOVA model. However, a quantitative evaluation of the association between bark morphology and the ability to absorb the chemical elements under investigation was not the objective of the present study, and it is an interesting topic for further research. The lack of statistical significance of the results for Ligustrum sp. could be attributed to the smaller sample size, but there is no evidence that the results are biased by it. Providentially, T. tipu is an abundant tree species not only in cities of Brazil, but also in other cities including those in a few developing countries ( Sashua-Bar et al., 2010 ; Kuruneri-Chitepo and Shackleton, 2011 ; Soares et al., 2011 ; Breuste, 2013 ; Mazza et al., 2013 ; Teixeira, 2014 ; Legumes, 2017 ). If local conditions do not influence the favorable characteristics of the external bark of this species, it may be used in biomonitoring studies in such localities. Similarly, to T. tipu , some Pinus spp. are usually regarded as good biomonitoring species because of their favorable bark characteristics ( Oliva and Mingorance, 2006 ; Sawidis et al., 2011 ). These interspecific differences are clearly relevant when studying finer gradients of air pollution ( Ejidike and Onianwa, 2015 ) like in our study.
Large-scale estimation or reconstruction of air pollution usually relies on remote sensing or land-use regression techniques. In both cases, the use of local data obtained from automatic stations and/or passive monitoring is required to validate and improve the simulations ( Van Donkelaar et al., 2016 ). Air pollution data obtained from the analysis of element concentration in the bark of trees can be used as additional observational data in this context ( Wolterbeek, 2002 ), either with a complementary role or even replacing traditional methods. The use of tree barks for biomonitoring purposes has few reported limitations. Despite the role of tree species, tree barks do not provide the temporal resolution required for acute exposure risk ( Marć et al., 2015 ). Nonetheless, tree barks may be more suitable for studying long-term exposures ( Catinon et al., 2009 ) usually related to chronic diseases. The specification of standard procedures ( Marć et al., 2015 ) to account for the many sources of data noise, in addition to the choice of species, should be object of future research.
Conclusions
Tree barks are reliable biomonitors and this is the first study showing that they can be used to distinguish both traffic generated pollution and the filtering properties of green areas. Our results suggest that biomonitoring studies based on tree barks are strongly dependent on species. The choice of species with a rough and highly porous bark favors the discrimination of different levels of air pollution accounting for the complex landscape found within cities. Out of the studied species, T. tipu stands out as a reliable one for such studies. Fortunately, this species occurs in different cities around the world where they can be used in biomonitoring programs. The use of tree barks may complement or, whenever needed, replace local measurements used in studies based on satellite images or land-use regression.
Author Contributions
TM, LA-L, GdS, CS, PdA, LB, JMS, PS, MS, and GL conceived the study. TM and LA-L sampled the trees and analyzed the chemical composition of the barks. GL analyzed the external bark morphology; GdS, CS, PdA, LB, and JMS analyzed the data. TM, LA-L, GdS, CS, PdA, LB, JMS, PS, MS, and GL contributed to the interpretation of the results. TM, LA-L, GdS, CS, PdA, LB, JMS, PS, MS, and GL wrote the manuscript.
Authors thank the São Paulo Research Foundation (FAPESP 13/21728-2) and Coordination for the Improvement of Higher Education Personnel (CAPES), from Brazil for financial support. The author TM is grateful for a fellowship from the Coordination for the Improvement of Higher Education Personnel (CAPES-1078178). LA-L is grateful for a fellowship from the São Paulo Research Foundation (FAPESP 2014/19201-9). GL is grateful for a fellowship from the São Paulo Research Foundation (FAPESP 2015/25511-3). JMS had partial financial support from Conselho Nacional de Desenvolvimento Científico e Tecnológico (CNPq-304126/2015). Authors also thank the valuable contribution of the reviewers.
Conflict of Interest Statement
The authors declare that the research was conducted in the absence of any commercial or financial relationships that could be construed as a potential conflict of interest.
Acknowledgments
The authors thank SVMA-SP for providing the permissions for sampling trees bark in the city.
Supplementary Material
The Supplementary Material for this article can be found online at: https://www.frontiersin.org/articles/10.3389/fenvs.2018.00072/full#supplementary-material
Andrade, M. F., Miranda, R. M., Fornaro, A., Kerr, A., Oyama, B., Andrade, P. A., et al. (2012). Vehicle emission and PM 2.5 mass concentration in six Brazilian cities. air quality Atmos. Hlth . 5, 79–88. doi: 10.1007/s11869-010-0104-5
CrossRef Full Text
Baccarelli, A. (2009). Breathe deeply into your genes genetic variants and air pollution effects . Am. J. Resp. Crit. Care 1796, 431–432. doi: 10.1164/rccm.200812-1808ED
Baklanov, A., Molina, L. T., and Gauss, M. (2016). Megacities, air quality and climate. Atmos. Environ. 126, 235–249. doi: 10.1016/j.atmosenv.2015.11.059
CrossRef Full Text | Google Scholar
Böhm, P., Wolterbeek, H., Verburg, T., and Musilek, L. (1998). The use of tree bark for environmental pollution monitoring in the Czech Republic. Environ. Pollut. 1022, 243–250. doi: 10.1016/S0269-7491(98)00082-7
Bolund, P., and Hunhammar, S. (1999). Ecosystem services in urban areas. Ecol. Econ. 292, 293–301. doi: 10.1016/S0921-8009(99)00013-0
Bravo, M. A., Son, J., de Freitas, C. U., Gouveia, N., and Bell, M. L. (2015). Air pollution and mortality in São Paulo, Brazil: effects of multiple pollutants and analysis of susceptible populations. J. Expo. Sci. Env. Epid . 262, 150–161. doi: 10.1038/jes.2014.90
Breuste, J. H. (2013) Investigations of the urban street tree forest of Mendonza, Argentina. Urban Ecosyst. 16, 801–818. doi: 10.1007/s11252-012-0255-2.
Carneiro, M. F. H., Ribeiro, F. Q., Fernandes-Filho, F. N., Lobo, D. J. A., Barbosa, F., Rhoden, C. R., et al. (2011). Pollen abortion rates, nitrogen dioxide by passive diffusive tubes and bioaccumulation in tree barks are effective in the characterization of air pollution. Environ. Exp. Bot. 722, 272–277. doi: 10.1016/j.envexpbot.2011.04.001
Catinon, M., Ayrault, S., Clocchiatti, R., Boudouma, O., Asta, J., Tissut, M., et al. (2009). The anthropogenic atmospheric elements fraction: a new interpretation of elemental deposits on tree barks. Atmos. Environ. 435, 1124–1130. doi: 10.1016/j.atmosenv.2008.11.004
Cheng, Z., Luo, L., Wang, S., Wang, Y., Sharma, S., Shimadera, H., et al. (2016). Status and characteristics of ambient PM 2.5 pollution in global megacities. Environ. Int. 89, 212–221. doi: 10.1016/j.envint.2016.02.003
Companhia de Engenharia de Tráfego (2017a). Pesquisa de Monitoração Damobilidade. Mobilidade no Sistema Viário Principal. São Paulo . Available online at: http://www.cetsp.com.br/media/499255/2015.pdf (Accessed November and December, 2017).
Companhia de Engenharia de Tráfego (2017b). Companhia de Engenharia de Tráfego . Available online at: http://www.cetsp.com.br/sobre-a-cet/relatorios-corporativos.aspx (Accessed December 13, 2017).
de Almeida Albuquerque, T. T., de Fátima Andrade, M., and Ynoue, R. Y. (2012). Characterization of atmospheric aerosols in the city of São Paulo, Brazil: comparisons between polluted and unpolluted periods. Environ. Monit. Assess. 184, 969. doi: 10.1007/s10661-011-2013-y
de Brito, J. M., Belotti, L., Toledo, A. C., Antonangelo, L., Silva, F. S., Alvim, D. S., et al. (2010). Acute cardiovascular and inflammatory toxicity induced by inhalation of diesel and biodiesel exhaust particles. Toxicol. Sci. 1161, 67–78. doi: 10.1093/toxsci/kfq107
DETRAN-State Department of Traffic of São Paulo (2017). Frota De Veí culos Em SP-Por Tipo De Ve í culo . Available online at: https://www.detran.sp.gov.br/wps/wcm/connect/portaldetran/detran/detran/estatisticastransito/sa-frotaveiculos/d28760f7-8f21-429f-b039-0547c8c46ed1 (Accessed January 13, 2017).
Efron, B. (1982). The Jackknife, the Bootstrap and Other Resampling , 38th Edn. Philadelphia, PA: Society For Industrial And Applied Mathematics.
Ejidike, I. P., and Onianwa, P. C. (2015). Assessment of trace metals concentration in tree barks as indicator of atmospheric pollution within Ibadan City, South-West, Nigeria. J. Anal. Methods Chem . 2015:243601. doi: 10.1155/2015/243601
Falla, J., Laval-Gilly, P., Henryon, M., Morlot, D., and Ferard, J. F. (2000). Biological air quality monitoring: a review. Environ. Monit. Assess. 643, 627–644. doi: 10.1023/A:1006385924945
GBIF (2018). GBIF.org. GBIF Occurrence Download . Available online at: https://doi.org/10.15468/dl.7hk9m6
Guéguen, F., Stille, P., Geagea, M. L., and Boutin, R. (2012). Atmospheric pollution in an urban environment by tree bark biomonitoring–(Pt) I: trace element analysis. Chemosphere 8610, 1013–1019. doi: 10.1016/j.chemosphere.2011.11.040
Hetem, I. G., and Andrade, M. F. (2016). Characterization of fine particulate matter emitted from the resuspension of road and pavement dust in the metropolitan area of São Paulo, Brazil. Atmos. Basel. 7:31. doi: 10.3390/atmos7030031
IBGE (2017). IBGE Cidades São Paulo, São Paulo. Available online at: https://cidades.ibge.gov.br/brasil/sp/sao-paulo/panorama (Accessed March 4, 2017).
Janhäll, S. (2015). Review on urban vegetation and particle air pollution–deposition and dispersion. Atmos. Environ. 105, 130–137. doi: 10.1016/j.atmosenv.2015.01.052
Jeanjean, A. P. R., Monks, P. S., and Leigh, R. J. (2016). Modelling the effectiveness of urban trees and grass on PM 2.5 reduction via dispersion and deposition at a city scale. Atmos. Environ. 147, 1–10. doi: 10.1016/j.atmosenv.2016.09.033
Junikka, L. (1994). Survey of English macroscopic bark terminology. IAWA J. , 151, 3–45. doi: 10.1163/22941932-90001338
Kassomenos, P. A., Vardoulakis, S., Chaloulakou, A., Paschalidou, A. K., Grivas, G., Borge, R., et al. (2014). Study of PM 10 and PM 2.5 levels in three European cities: analysis of intra and inter urban variations. Atmos. Environ. 87, 153–163. doi: 10.1016/j.atmosenv.2014.01.004
Kuik, P., and Wolterbeek, H. T. H. (1994). Factor analysis of trace-element data from tree-bark samples in the Netherlands. Environ. Monit. Assess. 32, 207–226. doi: 10.1007/BF00546277
PubMed Abstract | CrossRef Full Text | Google Scholar
Kuruneri-Chitepo, C., and Shackleton, C. M. (2011) The distribution, abundance composition of street trees in selected towns of the Eastern Cape, South Africa. Urban For. Urban Green. 10, 247–254. doi: 10.1016/j.ufug.2011.06.001
Legumes (2017). Version 10.38 20 July 2010-Scientific Names: Tipuana tipu (Benth.) Kuntze . Available online at: http://www.legumes-online.net/ildis/aweb/td006/td_01183.htm (Accessed December 13, 2017).
Liu, Y., Franklin, M., Kahn, R., and Koutrakis, P. (2007). Using aerosol optical thickness to predict ground-level PM 2.5 concentrations in the St. Louis area: a comparison between MISR and MODIS. REMOTE Sens Environ. 107, 33–44. doi: 10.1016/j.rse.2006.05.022
Marć, M., Tobiszewski, M., Zabiegała, B., de la Guardia, M., and Namieśnik, J. (2015). Current air quality analytics and monitoring: a review. Anal. Chim. Acta 853, 116–126. doi: 10.1016/j.aca.2014.10.018
Masri, S., Kang, C. M., and Koutrakis, P. (2015). Composition and sources of fine and coarse particles collected during 2002–2010 in Boston, MA. J. Air Waste Mgt. 653, 287–297. doi: 10.1080/10962247.2014.982307
Mauad, T., Rivero, D. H. R. F., de Oliveira, R. C., de Faria Coimbra Lichtenfels, A. J., Guimaraes, E. T., de Andre, P. A., et al. (2008). Chronic exposure to ambient levels of urban particles affects mouse lung development. Am. J. Resp. Crit. Care 1787, 721–728. doi: 10.1164/rccm.200803-436OC
Mazza, M., Refojo, N., Bosco-Borgeat, M. E., Taverna, C. G., Trovero, A. C., Rog,é, A., et al. (2013). Cryptococcus gattii in urban trees from cities in North-eastern Argentina. Mycoses 56, 645–650. doi: 10.1111/myc.12084
Minganti, V., Drava, G., De Pellegrini, R., Anselmo, M., Modenesi, P., Malaspina, P., et al. (2015). The bark of holm oak Quercus ilex , L. for airborne Cr VI monitoring . Chemosphere 119, 1361–1364. doi: 10.1016/j.chemosphere.2014.02.026
Miranda, R. M., Andrade, M. F., Fornaro, A., Astolfo, R., Andrade, P. A., and Saldiva, P. (2012). Urban air pollution: a representation survey of PM 2.5 mass concentrations in six Brazilian cities. Air Qual. Atmos. Health 5, 63–77. doi: 10.1007/s11869-010-0124-1
Molina, L. T., Molina, M. J., Slott, R. S., Kolb, C. E., Gbor, P. K., Meng, F., et al. (2004). Air quality in selected megacities. J. Air Waste Manag. Assoc. 54, 1–73. doi: 10.1080/10473289.2004.10471015
Moreira, T. C. L., de Oliveira, R. C., Amato, L. F. L., Kang, C. M., Saldiva, P. H. N., and Saiki, M. (2016). Intra-urban biomonitoring: source apportionment using tree barks to identify air pollution sources. Environ. Int. 91, 271–275. doi: 10.1016/j.envint.2016.03.005
Neter, J., Kutner, M. H., Nachtsheim, C. J., and Li, W. (2005). Applied Linear Statistical Models . 5th Edn. Chicago, IL: Irwin.
Nowak, D. J., Hirabayashi, S., Bodine, A., and Greenfield, E. (2014). Tree and forest effects on air quality and human health in the United States. Environ. Pollut. 193, 119–129. doi: 10.1016/j.envpol.2014.05.028
Oliva, S. R., and Mingorance, M. D. (2006). Assessment of airborne heavy metal pollution by aboveground plant parts. Chemosphere 652, 177–182. doi: 10.1016/j.chemosphere.2006.03.003
Oliveira, S., Andrade, H., and Vaz, T. (2011). The cooling effect of green spaces as a contribution to the mitigation of urban heat: a case study in Lisbon. Build Environ. 46, 2186–2194. doi: 10.1016/j.buildenv.2011.04.034
Pacheco, A. M. G., Barros, L. I. C., Freitas, M. C., Reis, M. A., Hipólito, C., and Oliveira, O. R. (2002). An evaluation of olive-tree bark for the biological monitoring of airborne trace-elements at ground level. Environ. Pollut. 1201, 79–86. doi: 10.1016/S0269-7491(02)00130-6
Pereira Filho, M. A., Pereira, L. A. A., Arbex, F. F., Arbex, M., Conceição, G. M., Santos, U. P., et al. (2008). Effect of air pollution on diabetes and cardiovascular diseases in São Paulo, Brazil. Braz. J. Med. Biol. Res. 416, 526–532. doi: 10.1590/S0100-879X2008005000020
Perelman, P., Faggi, A., Castro, M., and Carretero, E. M. (2010). Pollution trends using bark of Morus alba in the cities of Buenos Aires and Mendoza Argentina. Rev. Arvore 343, 505–511. doi: 10.1590/S0100-67622010000300014
Pope, C. A. III., Burnett, R. T., Thun, M. J., Calle, E. E., Krewski, D., Ito, K., et al. (2002). Lung cancer, cardiopulmonary mortality, and long-term exposure to fine particulate air pollution. Jama , 2879, 1132–1141. doi: 10.1001/jama.287.9.1132
Pope, C. A. III., Ezzati, M., and Dockery, D. W. (2009). Fine-particulate air pollution and life expectancy in the United States. New Engl J. Med . 3604, 376–386. doi: 10.1056/NEJMsa0805646
Santos, U. P., Garcia, M. L. S. B., Braga, A. L. F., Pereira, L. A. A., Lin, C. A., de André, P. A., et al. (2016). Association between traffic air pollution and reduced forced vital capacity: a study using personal monitors for outdoor workers. PLoS ONE 1110:e0163225. doi: 10.1371/journal.pone.0163225
Sashua-Bar, L., Potchter, O., Bitan, A., Boltansky, D., and Yaakov, Y. (2010). Microclimate modelling of street tree species effects within the varied urban morphology in the Mediterranean city of Tal Avivi, Israel. Int. J. Climatol. 30, 44–47. doi: 10.1002/joc.1869
Sawidis, T., Breuste, J., Mitrovic, M., Pavlovic, P., and Tsigaridas, K. (2011). Trees as bioindicator of heavy metal pollution in three European cities. Environ. Pollut. 15912, 3560–3570. doi: 10.1016/j.envpol.2011.08.008
Selmi, W., Weber, C., Rivière, E., Blond, N., Mehdi, L., and Nowak, D. (2016). Air pollution removal by trees in public green spaces in Strasbourg city, France. Urban For. Urban Green. 17, 192–201. doi: 10.1016/j.ufug.2016.04.010
Soares, A. L., Rego, F. C., McPherson, E. G., Simpson, J. R., Peper, P. J., and Xiao, Q. (2011). Benefits and costs of street trees in Lisbon, Portugal Urban For. Urban Green . 10, 69–70. doi: 10.1016/j.ufug.2010.12.001
Szczepaniak, K., and Biziuk, M. (2003). Aspects of the biomonitoring studies using mosses and lichens as indicators of metal pollution. Environ. Res. 93, 221–230. doi: 10.1016/S0013-9351(03)00141-5
Tarifa, J., and Azevedo, T. (2001). Os Climas na Cidade de São Paulo .: Universidade de São Paulo, Pró-Reitoria de Cultura e Extensão Universitária (São Paulo).
Teixeira, E. (2014). Evaluación de la estructura y comportamiento del arbolado urbano en Motevideo , Ph.D Thesis, Universidade de la República, Facultad de Agronomia, Montivideo, Uruguay.
United Nations (2012). Department of Economic and Social Affairs . Population Division. World Urbanization Prospects: The 2011 Revision. United Nations.
Vailshery, L. S., Jaganmohan, M., and Nagendra, H. (2013). Effect of street trees on microclimate and air pollution in a tropical city. Urban For. Urban Green . 123, 408–415. doi: 10.1016/j.ufug.2013.03.002
Van Donkelaar, A., Martin, R. V., Brauer, M., Hsu, N. C., Kahn, R. A., Levy, R. C., et al. (2016). Global estimates of fine particulate matter using a combined geophysical-statistical method with information from satellites, models, and monitors. Environ. Sci. Technol. 507, 3762–3772. doi: 10.1021/acs.est.5b05833
Van Grieken, R. E., and Markowicz, A. A. (2002). Handbook of X-ray Spectrometry, 2nd Edn. Revised And Expanded. (New York, NY; Basel: Marcel Dekker).
Google Scholar
WHO (2017). 7 Million Premature Deaths Annually Linked to Air Pollution. Available online at: http://www.who.int/mediacentre/news/releases/2014/air-pollution/en/ (Accessed January 4, 2017).
Wolterbeek, B. (2002). Biomonitoring of trace element air pollution: principles, possibilities and perspectives. Environ. Pollut. 1201, 11–21. doi: 10.1016/S0269-7491(02)00124-0
Wolterbeek, H. T., and Bode, P. (1995). Strategies in sampling and sample handling in the context of large-scale plat biomonitoring surveys of trace element air pollution. Sci. Total Environ. 176, 33-43. doi: 10.1016/0048-9697(95)04828-6
World Urbanization Prospects (2017). World Urbanization Prospects-Population Division-United Nations . Available online at: http://esa.un.org/unpd/wup/Documentation/final-report.htm (Accessed June 13, 2017).
Yli-Pelkonen, V., Setälä, H., and Viippola, V. (2017). Urban forests near roads do not reduce gaseous air pollutant concentrations but have an impact on particles levels. Landscape and Urban Plan . 158, 39–47. doi: 10.1016/j.landurbplan.2016.09.014
Yunus, M., Yunus, D., and Iqbal, M. (1990). Systematic bark morphology of some tropical trees. Bot. J. Linn. Soc. 1034, 367–377. doi: 10.1111/j.1095-8339.1990.tb00196.x
Zanobetti, A., Franklin, M., Koutrakis, P., and Schwartz, J. (2009). Fine particulate air pollution and its components in association with cause-specific emergency admissions. Environ. Health 81, 1–12. doi: 10.1186/1476-069X-8-58
Keywords: bark morphology, biomonitoring, EDXRF, green areas, tree species
Citation: Moreira TCL, Amato-Lourenço LF, da Silva GT, Saldiva de André CD, de André PA, Barrozo LV, Singer JM, Saldiva PHN, Saiki M and Locosselli GM (2018) The Use of Tree Barks to Monitor Traffic Related Air Pollution: A Case Study in São Paulo–Brazil. Front. Environ. Sci . 6:72. doi: 10.3389/fenvs.2018.00072
Received: 13 February 2018; Accepted: 15 June 2018; Published: 13 July 2018.
Reviewed by:
Copyright © 2018 Moreira, Amato-Lourenço, da Silva, Saldiva de André, de André, Barrozo, Singer, Saldiva, Saiki and Locosselli. This is an open-access article distributed under the terms of the Creative Commons Attribution License (CC BY) . The use, distribution or reproduction in other forums is permitted, provided the original author(s) and the copyright owner(s) are credited and that the original publication in this journal is cited, in accordance with accepted academic practice. No use, distribution or reproduction is permitted which does not comply with these terms.
*Correspondence: Tiana C. L. Moreira, [email protected]
† These authors have contributed equally to this work.

An official website of the United States government
The .gov means it’s official. Federal government websites often end in .gov or .mil. Before sharing sensitive information, make sure you’re on a federal government site.
The site is secure. The https:// ensures that you are connecting to the official website and that any information you provide is encrypted and transmitted securely.
- Publications
- Account settings
Preview improvements coming to the PMC website in October 2024. Learn More or Try it out now .
- Advanced Search
- Journal List
- Int J Environ Res Public Health

Impact of Air Pollution on Asthma Outcomes
Angelica i. tiotiu.
1 Department of Pulmonology, University Hospital of Nancy, 54395 Nancy, France
2 Development of Adaptation and Disadvantage, Cardiorespiratory Regulations and Motor Control (EA 3450 DevAH), University of Lorraine, 54395 Nancy, France
Plamena Novakova
3 Clinic of Clinical Allergy, Medical University, 1000 Sofia, Bulgaria; moc.oohay@anemalpn
Denislava Nedeva
4 Medical University Sofia, 1000 Sofia, Bulgaria; [email protected]
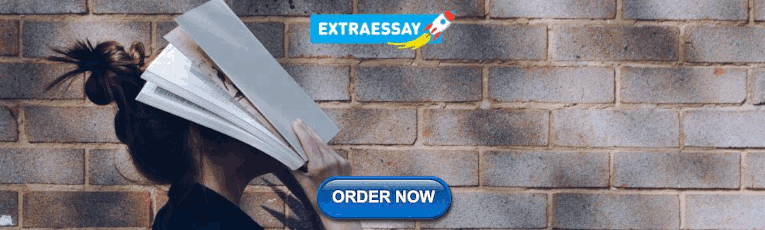
Herberto Jose Chong-Neto
5 Division of Allergy and Immunology, Department of Pediatrics, Federal University of Paraná, Curitiba 80000-000, Brazil; [email protected]
Silviya Novakova
6 Allergy Unit, Internal Consulting Department, University Hospital “St. George”, 4000 Plovdiv, Bulgaria; moc.oohay@66avokavon
Paschalis Steiropoulos
7 Department of Respiratory Medicine, Medical School, Democritus University of Thrace, University General Hospital Dragana, 68100 Alexandroupolis, Greece; moc.oohay@soluoporiets
Krzysztof Kowal
8 Department of Allergology and Internal Medicine, Medical University of Bialystok, 15-037 Bialystok, Poland; lp.ude.bmu@dmklawok
Asthma is a chronic respiratory disease characterized by variable airflow obstruction, bronchial hyperresponsiveness, and airway inflammation. Evidence suggests that air pollution has a negative impact on asthma outcomes in both adult and pediatric populations. The aim of this review is to summarize the current knowledge on the effect of various outdoor and indoor pollutants on asthma outcomes, their burden on its management, as well as to highlight the measures that could result in improved asthma outcomes. Traffic-related air pollution, nitrogen dioxide and second-hand smoking (SHS) exposures represent significant risk factors for asthma development in children. Nevertheless, a causal relation between air pollution and development of adult asthma is not clearly established. Exposure to outdoor pollutants can induce asthma symptoms, exacerbations and decreases in lung function. Active tobacco smoking is associated with poorer asthma control, while exposure to SHS increases the risk of asthma exacerbations, respiratory symptoms and healthcare utilization. Other indoor pollutants such as heating sources and molds can also negatively impact the course of asthma. Global measures, that aim to reduce exposure to air pollutants, are highly needed in order to improve the outcomes and management of adult and pediatric asthma in addition to the existing guidelines.
1. Introduction
Air pollution can be defined as the presence in the air of substances harmful to humans and is associated with a high risk for premature deaths due to cardio-vascular diseases (e.g., ischaemic heart disease and strokes), chronic obstructive pulmonary disease, asthma, lower respiratory infections and lung cancer [ 1 , 2 ]. People living in developing and overpopulated countries disproportionately experience the burden of outdoor (ambient) air pollution with 91% of the 4.2 million premature deaths in 2016 occurring in low- and middle-income countries of the South-East Asia, Central Africa and Western Pacific regions where exposure is higher [ 1 , 3 ]. The quality of air has been improving in the developed countries, however air pollution is steadily rising in the developing ones [ 4 ]. In order to quantify air pollution, standards of air quality for different pollutants were developed by the World Health Organization (WHO). The data from WHO indicate that 9 out of 10 people breathe air containing high levels of pollutants. More than 80% of people living in urban areas, where air pollution is monitored, are exposed to air pollutant levels that exceed WHO guideline limits. In addition, approximately 3 billion people are exposed to high levels of indoor (household) air pollution due to the use of biomass, kerosene fuels and coal for cooking and the heating of their homes, inducing a high prevalence of respiratory disorders [ 5 ].
Although there are many natural sources of air pollution such as volcanos or wildfires, it was the industrial revolution that made air pollution a real global problem [ 1 ]. Ambient air pollution affects the quality of indoor air and vice versa. According to the particle size, pollutants can be categorized as gaseous and particulate matter (PM) [ 6 ]. The main gaseous pollutants include inorganic components such as nitrogen dioxide (NO 2 ), sulphur dioxide (SO 2 ), ozone (O 3 ), carbon monoxide (CO), carbon dioxide (CO 2 ) and heavy metals such as lead or chromium (Pb or Cr), as well as volatile organic compounds (VOCs) including polycyclic aromatic hydrocarbons (PAHs). Some of them, for example NO 2 or SO 2 , are directly produced by different pollution sources while others, i.e., O 3 are formed by the interaction of nitric oxides and VOCs with the sunlight. The pollutants with the greatest impact on humans health are PM, which are commonly used as a measure of air quality [ 6 , 7 ]. Traffic-related air pollution (TRAP), a complex mixture rich in PM, exerts a particularly deleterious effect on the function of the respiratory system [ 5 ].
Asthma is a chronic inflammatory airway disease characterized by respiratory symptoms such as wheeze, dyspnoea, cough and chest tightness asssociated with variable expiratory airflow limitation. The prevalence of asthma is estimated at between 1 and 18% of the population in different countries. Evidence suggests that 13% of global incidence of asthma in children could be attributable to TRAP and data showed that air pollution has a negative impact on asthma outcomes in both adult and pediatric populations [ 8 ].
The aim of this review is to summarize the recent data about the effects of various outdoor and indoor pollutants on asthma development, symptoms, exacerbations/hospitalisations, severity, lung function and medication use, as well as to highlight the possible measures that could reduce their impact on asthma outcomes. A better knowledge of the negative impact of air pollution on asthma outcomes could help physicians (e.g., general practitioners, pulmonologists, allergologists, pediatrics, gynecologists, and emergency doctors) to improve their daily practice by adding in the interrogatory specific questions on a possible recent exposure worsening the respiratory symptoms, to educate the patients about how they could minimise the exposure and manage their asthma by an action plan. At the same time, the global awareness of air pollution effects on asthma should stimulate public health authorities and governments to take more efficient measures to limit the exposure to air pollutants.
2. Search Strategy, Data Sources and Selection Criteria
This review examines the literature linking air pollution and asthma across PubMed and Medline databases from January 1, 2010 and June 30, 2020. The search terms used were: “air pollution”, “outdoor air pollutants”, “indoor air pollutants”, “environmental risk factors”, “ambient sources of pollution”, “household sources of pollution”, “smoking”, and “preventive measures to reduce air pollution” associated with “asthma”. We prioritized cross-sectional and observational studies, followed by meta-analyses, systematic reviews, and general reviews. A preference was given to more recent articles published the last five years in order to have the most up-to-date evidence. Results were limited to publications in English.
3. Air Pollution and Risk of Asthma
The effect of air pollution on the development of asthma has been studied for many years. Increasing evidence indicates that both outdoor and indoor air pollution contributes to asthma development. Numerous cross-sectional studies provided evidence for an association between poor air quality and the incidence of asthma [ 9 , 10 , 11 , 12 , 13 ]. One of those studies, conducted in an urban population, demonstrated that the association between asthma morbidity and air pollutions was stronger in children than in adolescents and adults [ 10 ]. The important role of increased exposure to TRAP, particularly to its components PM 2.5 , PM 10 , NO 2 and black carbon, in asthma development was demonstrated in a recent meta-analysis of 41 publications [ 14 ]. Those observations are supported by longitudinal studies evaluating the relationship between early childhood exposure to ambient air pollution and future asthma incidence. A meta-analysis of published birth cohort studies reported significant associations between long-term exposure to black carbon and PM 2.5 and the risk of asthma in childhood up to 12 years of age [ 13 ]. The interaction between air pollution exposure in early life and asthma development was demonstrated in a prospective study on the cohort Prevention and Incidence of Asthma and Mite Allergy (PIAMA). Both early and recent exposures to PM 2.5 , PM 10 or NO 2 , especially TRAP, were associated with a higher incidence of asthma until age of 20 years [ 15 ]. Another large population-based birth cohort study found a positive association between perinatal exposure to air pollution and asthma incidence during preschool years [ 16 ]. A recently published birth cohort study including 184,604 children born between 2004 and 2011 in Taiwan demonstrated that both prenatal and postnatal exposures to air pollutants, in particular PM 2.5 , were associated with later development of asthma [ 17 ]. The role of prenatal exposure to air pollutants in childhood asthma development was also shown in two independent meta-analyses [ 18 , 19 ]. The long-term effects of air pollution on asthma have been summarized in an American Thoracic Society Workshop Report, which indicates that the available evidence indicates that long-term exposure to air pollution was a cause of childhood asthma, but the evidence for a similar determinant role for adult asthma remained insufficient [ 20 ]. Some studies also provided evidence for positive associations between indoor air pollution, mainly due to cooking with polluting fuels, and asthma development in children. In a meta-analysis of 41 studies, a positive association between gas cooking, exposure to NO 2 , and childhood asthma or wheeze was found [ 21 ].
Second-hand smoking (SHS) was also reported to play an important role in asthma development. However, it is plausible that gene–environment interaction is also important for the effects of air pollution on asthma development. It has been shown recently that exposure to PM 10 and maternal smoking was associated with a higher susceptibility for infants with an adverse genetic predisposition to asthma that also depended on the infant’s ancestry [ 22 ]. Genetic traits that affect the risk of asthma due to SHS were also demonstrated in American children participants in the Cincinnati Childhood Allergy and Air Pollution Study. Variation in the N-Acetyltransferase 1 (NAT1) gene modified asthma risk in children exposed to SHS [ 23 ]. Systematic reviews and meta-analyses have demonstrated that maternal smoking during pregnancy is a risk factor of wheezing and asthma in children, especially in the first years of life [ 24 , 25 ]. The mechanisms behind the adverse health effects of maternal smoking during pregnancy are still not entirely clear, but epigenetics most likely plays a role [ 26 ]. Associations between deoxyribonucleic acid (DNA) methylation at loci previously linked to in utero tobacco smoke exposure and asthma-related outcomes were observed [ 27 ]. Grandmothers smoking during pregnancy with the mother, increases the risk for asthma in the grandchild, independently of the mother’s smoking status, suggesting a transgenerational impact of prenatal tobacco smoke exposure on asthma development [ 28 , 29 ]. Prenatal paternal smoking exposure was also associated with childhood asthma development at 6 years of age, presumably mediated by an IgE-independent mechanism. Prenatal paternal smoking led to epigenetic modifications in certain genes as such as LIM Domain Only 2 (LMO 2 ) and interleukin-10 (IL-10) via cytosine-phosphate-guanine (CpG) methylation, and these modifications are correlated to childhood asthma development [ 30 ].
Postnatal exposure to maternal and paternal smoking is also associated with wheezing in infants and pre-school children, while the data for school-aged children and adolescents are contradictory [ 24 ]. One of the limitations related to the investigation of the effect of postnatal exposure is the fact that most of the parents smoke during both the prenatal and postnatal periods, and studies on solely postnatal exposure lack consistency. A recent study showed that fathers’ smoking before the age of 15 of their children increased the risk of asthma without nasal allergies in their offspring, suggesting an effect of paternal pre-adolescent environment on the next generation [ 29 ].
Less data is available for maternal smoking and adult onset asthma. A recent study found that gestational tobacco smoke exposure is associated with new asthma diagnoses in adult offspring between 31 and 46 years, thus indicating the long-term effect of smoking. The association was accentuated in offspring who reported at age 31 as having past respiratory problems (wheeze) [ 31 ]. In addition, a reduction in forced expiratory volume in the one second (FEV 1 )/forced vital capacity (FVC) ratio was observed at age 31 years in the offspring with gestational smoke exposure [ 29 ]. Several longitudinal studies showed a positive association between active and passive smoking and the incidence of asthma in adults [ 32 , 33 , 34 , 35 , 36 , 37 , 38 , 39 , 40 ]. Women seem to be more susceptible to the effect of tobacco smoking than men [ 34 , 40 ]. Some of the studies suggested a stronger association between smoking and onset of asthma for non-atopics [ 35 , 37 ]. Other trials did not find any relation between smoking and newly onset asthma in the adult population [ 41 , 42 , 43 ]. A possible explanation of the inconsistencies between the results could be the different definitions of asthma that were used, self-reported questionnaires and evaluation, changes in tobacco smoking habits during the follow-up period and the ‘healthy smoker effect’ (reduces smoking initiation or favors smoking cessation among people more susceptible to the noxious effects of smoking) [ 44 ]. Current evidence is suggestive but not sufficient for a causal relationship between smoking and the incidence of asthma in adults and future research is needed in this domain.
4. Outdoor Air Pollution
The composition of outdoor air pollution is complex and dynamic. It changes from season to season, and is influenced by human activity and meteorological events [ 45 ]. Outdoor air pollutions include both primary pollutants emitted directly into the atmosphere and secondary pollutants formed in the air from chemical transformation of the primary. These chemical reactions depend on temperature and therefore can be influenced by global climate warming. Accumulated evidence suggested that air pollution cannot only aggravate asthma symptoms but might cause new-onset asthma as well. Several mechanisms have been identified and implicated. The respiratory mucosa formed by the airway epithelium represents the first contact between air pollutants and the respiratory system, functioning as a mechanical and immunologic barrier. Airway epithelial cells are connected by tight junctions and secrete mucus, host defense peptides and antioxidants, and express innate immune receptors, which could be activated by inhaled foreign substances and pathogens [ 45 ]. Under conditions of air pollution exposure, the defenses of the airway epithelium are compromised by the disruption of epithelial integrity, uptake of particles, activation of Toll-like and Nucleotid-binding Oligomerization Domain (NOD-receptors), epithelial growth factor receptor and induction of oxidative stress. Activation of these receptors results in (NF)-kB (nuclear factor Kappa B) activation, leading to pro-inflammatory cytokine expression [ 46 ]. Oxidative stress is one of the biological mechanisms proposed to partly explain the association between outdoor air pollution and asthma. Neutrophils attracted into the airways after exposure to certain pollutions produce reactive oxygen species (ROS) that induce epithelial cell inflammation, airway hyperreactivity (AHR) and lung injury. Pollutants can act directly by the production of free ROS and diffusion from the airway surface, or indirectly by inducing inflammation. Ozone (O 3 ) exposure causes ROS production and changes in the expression of claudins, the major components of tight junctions, thus leading to tight junction barrier permeability and AHR [ 47 ].
Importantly, pollutants (e.g., O 3 , SO 2 ) can act as adjuvants and affect the production of some cytokines (e.g., thymic stromal lymphopoetine) in airway epithelial cells, which promote T-helper 2 (Th2) phenotypic differentiation and IgE production. There is evidence for the stimulation of T-helper 17 (Th17) responses as well. Furthermore, repeated exposure to O 3 induces group 2 innate lymphoid cells (ILC2)-mediated airway type 2 immunity and the nonatopic asthma phenotype [ 20 ]. Numerous studies have repeatedly demonstrated epidemiological links between air pollution and increased respiratory tract infections in patients of all ages, which are considered the cause of asthma exacerbations. Changes in receptor expression for pathogens, antiviral mechanisms, or host defense peptide biology could be responsible. Oxidative stress could affect intercellular adhesion molecule-1 (ICAM-1) responses to rhinoviruses in epithelial cells of the respiratory mucosa. Coexposure of airway epithelial cells to rhinoviruses and NO 2 appears to induce a synergistic upregulation of ICAM-1, which could exaggerate the pathogen response. Available data indicated that oxidant pollutants (NO 2 or O 3 ) could amplify the generation of proinflammatory cytokines by rhinovirus-infected cells in epithelial cells of the respiratory mucosa. Oxidative stress has also been linked to a reduction in corticosteroid (CS) responsiveness in asthma patients, an important observation from a practical point of view [ 48 ].
One predisposing factor that contributes to the injury of airways by air pollutants might be atopy. Conversely, air pollutants could increase the risk of sensitization and the responses to inhaled allergen in asthma patients. Such a potential enhancing effect has been studied and demonstrated for O 3 , NO 2 , SO 2 . The mechanisms that could explain the enhanced sensitisation to aeroallergens by air pollutants include the higher deposition of allergen in the airways due to carriage by particles, an increased epithelial permeability due to oxidative stress, a greater antigenicity of proteins, and a possible direct adjuvant effect [ 49 ]. Apparently, the responses to air pollutants are diverse and individual. Genetic variations affect the function and susceptibility of epithelial cells. Specific polymorphisms in antioxidant enzyme genes, such as the glutathione-S-transferase family, especially Glutathione S-Transferase Pi 1 (GSTP1), are associated with differences in susceptibility to the adverse effects of pollutants and can modify the risk of asthmatic responses. Adults and children with Glutathione S-Transferase Mu 1 (GSTM1) null genotypes have reduced glutathione-S-transferase enzyme activity and are at increased risk of developing asthma when exposed to O 3 . The association of tumor necrosis factor (TNF) polymorphisms with asthma and differences in susceptibility to the adverse effects of pollutants has been demonstrated. TNF polymorphisms, thought to affect the expression of pro-inflammatory cytokines, seem to influence the response of the lungs to O 3 , and the risk of developing asthma [ 50 ]. Results from a recent genome-wide interaction study identified gene–NO 2 interactions on asthma and indicated that gene–environment interactions are important for asthma development [ 51 ]. Mucin gene variants contribute to air pollutant responses in asthmatic patients. Their role in air pollution-induced mucin production has been demonstrated [ 52 ].
The impact of air pollution on asthma could by modified by other individual factors like obesity, as reported in most studies. A large cross-sectional study found that the effect of NO 2 and SO 2 on asthma was significantly greater in overweight or obese children. Similarly, the exposure to O 3 is associated with a poorer lung function for obese adults when compared to people with a normal weight [ 53 ].
In the troposphere, O 3 is a secondary pollutant generated through a chemical reaction between oxides of nitrogen and Volatile Organic Compounds (VOCs) released by natural sources or following anthropogenic activities in the presence of sunlight. Other involved elements are CO and methane [ 54 ]. The combustion of fossil fuels, emissions from industrial facilities and electric utilities, gasoline vapors, motor vehicle exhaust and chemical solvents are among the main sources of O 3 precursors. Anthropogenic emissions were responsible for 37% of O 3 impacts in 2015 globally [ 55 ]. Due to its low water-solubility, O 3 is not effectively removed by the upper respiratory tract and has the capacity to penetrate deeply into the lungs [ 7 ].
It is well established that inhaled O 3 first interacts with antioxidants in the airway epithelial cells. Surfactant protein D in particular has been shown to modulate the response to O 3 and appears to have important genetic variability that influences personal susceptibility [ 56 ]. When the dose of O 3 in the respiratory tract exceeds the protective capacity of antioxidants, adverse health effects are likely to occur. The oxidative stress induced by the secondary oxidation results in airway inflammation, AHR, and decrements in lung function in asthmatic adults. As a highly reactive gaseous pollutant, O 3 exerts inflammatory effects on the respiratory system. The oxygen radicals evoke oxidative stress and airway inflammation, more pronounced among allergic subjects [ 57 ].
Personal short-term exposure to O 3 increases the risk of current asthma with persistent evidence that it could directly cause asthma exacerbation [ 58 , 59 ]. Increased rates of asthma hospital admissions and emergency department visits following days of elevated ambient O 3 concentrations have been reported in some epidemiology studies. The consequences of short-term O 3 exposure have been evaluated in meta-analysis of 47 eligible studies published recently, which confirmed the association between O 3 exposure and asthma exacerbations measured as emergency room visits or hospitalizations. The association was significant during the warm season and in the areas where ambient O 3 concentrations were higher [ 60 ]. As estimated recently, 9–23 million annual asthma emergency room visits globally in 2015 could be attributable to O 3 , representing 8–20% of the annual number of global visits [ 53 ]. Children appeared to be more susceptible to O 3 . This may be due to children’s higher breathing rate, narrower airways, lungs and immune system still being in development, and more frequent outdoor activities. Results from time series analysis of asthma hospital admissions and daily 8-h maximum O 3 concentrations established significant relationships for all ages with the highest risk for children [ 61 ].
Strong evidence of a relationship between long-term O 3 exposure and respiratory morbidity is provided by studies focused on asthma development in children and on increased respiratory symptoms in asthmatics. It was demonstrated that exposure to O 3 in early life was significantly and positively associated with a detrimental effect on the lung function development in children, larger in boys. Nevertheless, no clear and consistent findings have been reported for the long-term effects on lung function [ 54 ]. Prenatal exposure to O 3 has not been associated with subsequent childhood asthma [ 18 ]. O 3 might be an important risk factor affecting the progression of asthma to chronic obstructive pulmonary disease (COPD), defining the asthma–COPD overlap (ACO) syndrome. It was established that individuals with asthma exposed to higher levels of O 3 had greater odds of developing ACO [ 62 ]. Long-term O 3 exposure is significantly associated with the risk of death, especially for cardiovascular and respiratory diseases. A study compared daily O 3 concentrations to the daily number of deaths in an urban European population during 3-years. An increase in O 3 concentration was observed during the warm period of the year, and was associated with an increase in the daily number of deaths (0.33%), notably respiratory deaths (1.13%). No effect was observed during wintertime [ 7 ].
4.2. Nitrogen Dioxide
NO 2 is a traffic-related pollutant, as it is emitted from automobile motor engines. Transportation can contribute up to 80% of ambient NO 2 , so it is a convenient marker of primary pollutant. NO 2 is an irritant of the respiratory system, which penetrates deep into the lung, inducing coughing, wheezing, dyspnea, bronchospasm, and even pulmonary edema when inhaled at high levels. It seems that concentrations of over 0.2 parts per billion (ppb) produce these adverse effects in humans, while concentrations higher than 2.0 ppb affect T-lymphocytes, particularly the CD8+ cells and natural killer (NK) cells involved in different immune responses [ 7 ]. It can augment the degree of allergic airway inflammation and prolong allergen-induced AHR. Compared with its direct effects on the airways, NO 2 might play a more prominent role as a sensitizing agent to inhaled allergen. Exposure to 0.4 ppm NO 2 for 4 h enhances both immediate- and late-phase responses to inhaled allergen, and can activate NF-κB, and develop allergen sensitization. High exposure to NO 2 during the first year of life was associated with increased risk of sensitization to pollens at age 4 years [ 63 ]. Meta-analysis provided evidence for association between NO 2 exposure during pregnancy and differential offspring DNA methylation in mitochondria-related genes. Exposure to NO 2 was also linked to differential methylation as well as the expression of genes involved in antioxidant defense pathways [ 64 ].
NO 2 is associated with significant morbidity in asthmatic individuals and might be a cause of incident asthma. Consistent with other studies that found associations between prior pollution exposure and future asthma risk, a recent study revealed that the odds of future asthma diagnosis for children exposed to a high concentration of NO 2 in early life were 1.25 times greater than those for children exposed to a low concentration of NO 2 [ 65 ]. A recent estimation on the basis of data from 194 countries concluded that, each year, 4.0 million (95% CI 1.8–5.2) new cases of paediatric asthma might be attributable to NO 2 pollution, accounting for 13% (5.8–16) of global incidence. This contribution exceeded 20% of new asthma cases. The European analysis subset reported in the same paper estimated that 17% of the burden in Western Europe, 14% in Central Europe and 17% in Eastern Europe was attributable to NO 2 . About 92% of paediatric asthma incidence attributable to NO 2 exposure occurred in areas with annual average NO 2 concentrations lower than the WHO guideline of 21 ppb [ 66 ]. The longest longitudinal study of the health of school-aged children in Canada with >17 years of follow-up found that exposure to total oxidants (O 3 and NO 2 ) at birth increased the risk of developing asthma by 17% [ 67 ]. A recent meta-analysis, using observational data from five European birth cohorts, found no evidence suggesting that long-term air pollution levels including NO 2 were associated with the prevalence of current pediatric asthma up to age eight years [ 68 ]. Compliance with the NO 2 WHO air quality guidelines was estimated to prevent 2434 (0.4% of total cases) incident childhood asthma cases per year across eighteen European countries [ 11 ].
Studies in children and adults have identified associations between even low-levels of NO 2 and symptoms of asthma, reduced lung function, and exacerbation of asthma. Data from several cross-sectional studies and from a meta-analysis of published studies evaluated the association between air pollution and lung function in children. NO 2 exposure was correlated with an increase of fractional exhaled nitric oxide (FeNO) and a delayed increase in both FEV 1 and FVC [ 69 , 70 ]. Even during pregnancy, NO 2 exposure could impair lung function in early life [ 71 ]. A systematic review showed a significant association between NO 2 exposure and moderate/severe asthma exacerbations in children and adults (OR: 1.024; 95% CI [1.005, 1.043]) [ 72 ].
4.3. Carbon Monoxide and Carbon Dioxide
CO and CO 2 are produced by fossil fuel when combustion is incomplete. Higher temperatures and amounts of CO 2 in the atmosphere are major factors that have been linked to an increased duration of pollen seasons, quantity of pollen produced by plants, and possibly allergenicity of pollen. It has been demonstrated that birch pollen extracts from trees grown in warmer temperatures had stronger IgE binding intensity. Enhanced ragweed pollen production as a function of increasing CO 2 levels has also been established. The growth of Alternaria species can become more abundant and produce more allergens in an enhanced CO 2 environment. These changes could affect allergic asthma. A potential link with thunderstorm-related asthma epidemics could be suspected [ 73 ].
Evidence suggests an association between exposure to CO and moderate or severe asthma exacerbations in adults (OR: 1.045; 95% CI: [1.005, 1.086]), but the link was not confirmed in children. Significant associations were observed between decreasing death rates of asthma and lower CO levels [ 72 ].
4.4. Sulfur Dioxide
SO 2 is released primarily from the combustion of sulfur-containing coal and oil. People prone to allergies, especially allergic asthma, can be extremely sensitive to inhaled SO 2 . The major health problems associated with SO 2 are bronchitis, mucus production, and bronchospasm. It is an irritant that penetrates deep into the lung where is converted into bisulfite and interacts with sensory receptors, causing bronchoconstriction [ 5 ]. In response to SO 2 , asthmatic subjects experience increased symptoms and a greater decrease in lung function at lower concentrations compared with non-asthmatics, who are often unresponsive at concentrations of less than 5 ppm. Considerable individual variations in the spirometric response to inhaled SO 2 have been noticed, suggesting a potential genetic link. Children with a particular polymorphism in the TNF-α gene had more significant reductions in lung function after SO 2 exposure [ 74 , 75 ].
A significant association between SO 2 and both asthma prevalence and current symptoms among children, especially in those with atopy, has already been established. Decreased lung function and increased hospital admissions with enhanced SO 2 exposure have been documented in pediatric populations. Even the low-dose SO 2 exposure is associated with a decline in lung function (FEV 1 and FVC) among the general population [ 76 ]. A systematic review showed a significant relationship between SO 2 and moderate/severe asthma exacerbations in children aged 0 to 18 years (OR: 1.047; 95% CI: [1.009, 1.086]) but not in adults [ 72 ].
4.5. Particulate Matter
PM is a complex heterogeneous mixture of dirt, soot, smoke and liquid droplets from both natural and man-made sources. PM ambient air pollution is responsible for approximately 0.8 million premature deaths per year and 6.4 million years of life lost [ 77 ]. It was also estimated that PM 2.5 was responsible for around 16 million incident cases of childhood asthma every year. Although particles are detected in many organs, the respiratory system is usually the first line of entry into the body. PM penetrates deeply into the lungs and increases the frequency and severity of asthma attacks, exacerbating bronchitis and other lung diseases [ 55 ].
Sources of PM could be natural or anthropogenic. The former include wind-blown dust, sea salt, volcanic ash, pollens, fungal spores, soil particles, the products of forest fires and the oxidation of biogenic reactive gases. Anthropogenic emissions of PM derive from industrial processes, construction work, mining, cigarette smoking, fossil fuel combustion and wood stove burning. The main sources of PM in the urban areas are road traffic as well as the burning of fossil fuels in power stations [ 74 , 78 ].
Depending on the way it is released into the environment, PM could be primary or secondary. Primary particles are introduced into the atmosphere directly from their sources (road transport, combustion as well as the land and sea through soils carried by the wind), whereas secondary PM is a product of chemical reactions among different primary particulates. The difference between these two types of PM is the length of stay in the atmosphere—it takes more time for the secondary PM to be formed and therefore its persistence is prolonged [ 78 ].
The size of PM is of vital importance since it determines, to a great extent, its impact on respiratory health and the penetration degree in the human respiratory system. According to its diameter, PM could be divided into three categories: coarse PM 10 (from 2.5 to 10 μm), fine PM 2.5 (from 0.1 to 2.5 μm), ultrafine PM 0.1 (UFPs) (less than 0.1 μm). Coarse PM deposits primarily in the nasopharynx or primary bronchi; fine PM in the alveoli and terminal bronchioles; UFPs cross cell membranes and interact directly with cellular structures. The greatest number of particles fall into the ultrafine size range. UFPs have a detrimental effect on human health because their small size allows the greatest lung penetration and passage across the air–blood barrier [ 6 , 7 , 78 ].
Inhaled PM has the capacity to elicit lung oxidative stress as well as to interact with different components of the immune system and enhance allergic inflammatory response. What is more, not only do the particles infiltrate the circulatory system through layers of alveolar obstruction, but they can also absorb many airborne toxic substances on their surface, such as heavy metals, PAHs and organic/inorganic ions. It has been suggested that PM induces oxidative stress through several different mechanisms. Firstly, the redox cycle of some components of the particle’s surface, like iron or quinones, leads to the formation of ROS, hydrogen peroxide and the damaging hydroxyl radical in the lungs. Secondly, bacterial endotoxins associated with the particle surface can trigger inflammation. The particle surface itself has also been found to cause oxidative stress in vivo but this effect is not well defined yet [ 78 ]. PM enhances airway inflammation by interacting with the innate and adaptive immune system. It was suggested that PM activates neutrophils and eosinophils through increased levels of proinflammatory cytokines. PM induces antigen-presenting cell-mediated inflammatory responses as well as an imbalance of Th cells with an increase in Th2 and Th17 cells and downregulation of T-helper 1 (Th1). Exposure to PM could also lead to apoptosis and autophagy in lung epithelial cells in asthma. What is more, UFPs cross cell membranes and directly interact with cellular structures. UFPs escape the mucociliary clearance and the ingestion by alveolar macrophage scavenging [ 79 ]. A study of Mills et al. demonstrated that UFPs were detected in the blood immediately after inhalation and remained in the lungs for up to 6h after installation. Therefore, UFPs can induce severe eosinophilic inflammation, alveolar macrophage chemotaxis and epithelial damage in asthma [ 80 ].
The respiratory health effects of short-term exposure to air pollution include worsening of asthma symptoms, school absences, emergency department visits, hospitalizations and decreased lung function. Despite the limited available data, there is growing evidence about a possible impact of long-term outdoor air pollution exposures and asthma incidence. The American Thoracic Society Workshop Report revealed a strong correlation between childhood asthma and long-term air pollution exposure, especially to TRAP. PM 2.5 was found to induce airway remodeling and an increase in the incidence/severity of asthma-like phenotypes [ 20 ]. Particulate pollution might be a risk factor for the progression of asthma to ACO. The Canadian Community Health Survey found that asthmatics exposed to higher levels of PM 2.5 had nearly three-fold greater odds of ACO [ 62 ]. It was also demonstrated that PM increases the rate of emergency room visits due to asthma exacerbations in both adults and children. A study of Anenberg et al. concluded that 5–10 million annual asthma emergency room visits globally (4–9%) could be attributable to PM 2.5 [ 55 ]. A significant correlation was found between increased asthma emergency room visits in adults and high PM 2.5 concentrations, especially in the warm seasons [ 81 ]. It was also demonstrated that PM 10 was a statistically significant risk factor for a 2% increase in the number of asthma-related emergency visits in children [ 82 ]. A recent meta-analysis revealed that UFP exposure increased the number of asthma exacerbations, subsequent emergency room visits and hospital admissions in children [ 83 ]. In contrast, the exposure to PM attributable to landscape fire smoke (PM LFS ) seems to be associated with a higher risk for emergency department visits for the elderly compared to children. A meta-analysis showed that emergency department attendance increases with a 10μg/m 3 PM 2.5LFS of 15% for elderly (95% CI [1.1–1.2]) vs 4% (95% CI [1–1.08]) for children [ 84 ]. The risk is greatest on the day of exposure to PM LFS (an increase in emergence department attendances for asthma by 1.96% [95% CI: 0.02, 3.94]) and for women 20 years and older (5.08% 95% CI [1.76, 8.51]). [ 85 , 86 ].
There is growing evidence that PM exposure could be associated with impaired asthma control. A study found a correlation between poor asthma control, elevated PM 2.5 and pollen severity in a pediatric population [ 87 ]. A cohort study of 32 asthmatic adult patients revealed that a 10 mg/m 3 increase in PM 10 personal exposure was associated with an increase in Saint George Respiratory Questionnaire scores and a decrease in Asthma Control Test scores [ 88 ].
PM exposure might be an important risk factor for lung function deterioration in both children and adults with asthma. An association between the fall of the FEV 1 /FVC ratio and air pollution was found in a cohort study by Yu et al. Acute exposure to PM 10 in non-smoking adults with refractory asthma correlated with a 0.4% drop in the FEV 1 /FVC ratio in the spring [ 89 ]. A longitudinal analysis showed that an increase in PM 10 concentration was associated with increased peak expiratory flow (PEF) variability of >20% and a decrease in the mean PEF among 64 adults with asthma [ 90 ]. It was also found that an increase of 10 μg/m 3 in 24-h mean PM was associated with a drop of 3 L/min in PEF in children hospitalized for severe asthma exacerbations [ 91 ].
4.6. Outdoor Air Pollution and Asthma Outcomes
Many studies have demonstrated so far a clear association between short-term exposure to outdoor air pollutants and different asthma outcomes including asthma control [ 87 ], lung function [ 92 ], consumption of asthma medications [ 93 , 94 ], outpatient visits [ 95 , 96 ], asthma exacerbations [ 97 , 98 ], emergency room visits [ 99 ], hospitalizations [ 100 , 101 ], length of stay in the hospital [ 102 ] and deaths [ 2 ]. Several air pollutants have been implicated in the loss of asthma control. It seems that TRAP plays a particular role in this process because associations of asthma outcomes with outdoor air pollution were enhanced among subjects living in homes with high TRAP [ 9 ]. A study found that TRAP was associated with increased risk of hospitalizations due to asthma exacerbations in a population of 0–14 years of age children in California [ 100 ].
On the other hand, some air pollutants are associated with asthma morbidity independent of their source. In one study, exposure to outdoor PM 2.5 was significantly associated with an increased number of emergency room visits due to asthma and the effect was independent of the source of PM 2.5 [ 99 ]. An analysis of 3520 cases of acute asthma exacerbation indicated a positive association with the concentration of PM 2.5 in the outdoor air and the association remained significant after adjusting for gaseous co-pollutants [ 97 ]. Several meta-analyses have been performed in order to single out those components responsible for asthma exacerbations. A meta-analysis of 26 studies conducted worldwide found a 4.8% increase in the risk of asthma-associated emergency department visits and admissions among children exposed to short-term increases in PM 2.5 of 10 μg/m 3 , with greater effects in Europe and North America than in Asia [ 103 ]. In children, the association between NO 2 , SO 2 , and PM 2.5 exposures and asthma exacerbations, as well between all outdoor pollutants and hospital admissions, were confirmed by two meta-analyses [ 72 , 104 ].
The effect of outdoor air pollution may change with time within the same population. In a longitudinal study, it was demonstrated that each 6.8 μg/m 3 increase in PM 2.5 on the same day was associated with 0.4% (0.0%, 0.8%), 0.3% (−0.2%, 0.7%), and 2.7% (1.9%, 3.5%) increases in the rate of asthma emergency department visits in the 2005–2007, 2008–2013, and 2014–2016 periods, suggesting that the toxicity from PM 2.5 increased with the time [ 105 ].
Although the relation between exposure to outdoor air pollution and exacerbations of childhood asthma has been well documented, there is less evidence on exposure to indoor air pollution from incomplete combustion of polluting fuels. However, in a recent study, coexposure to elevated concentrations of indoor and outdoor pollutants was synergistically associated with increased emergency room visits for asthma [ 106 ].
It has been estimated that the combined effects of outdoor and indoor air pollution are responsible for approximately seven million premature deaths every year, mainly due to respiratory and cardiovascular diseases. Annually, around 500,000 deaths of children under 5 years of age and 50,000 deaths of children aged 5–15 years were attributable to air pollution. The burden of disease attributable to air pollution is not evenly distributed is greater in low- and middle-income countries than in high-income countries [ 107 ].
Table 1 shows the legal concentrations of outdoor air pollutants according to WHO guidelines and summarizes their negative impact on asthma outcomes.
Effects of outdoor air pollutants on asthma outcomes if legal concentrations are exceeded.
4.7. Outdoor Air Pollution and Asthma Management
Several risk reduction measures have been recommended. These include personal strategies, community and government interventions, as well as the use of effect modifiers, which could reduce the risk factors [ 108 ]. Patients’ education to minimise their exposure to air pollutants represents an important step in asthma management. Several measures could be beneficial, like the use of close-fitting N95 facemasks when air pollution levels are high, shifting from motorised to active travel (e.g., cycling, walking), selecting low-traffic routes or those with open spaces, driving with windows closed, maintaining car air filtration systems and internal circulation, and being informed of local air pollution levels [ 108 ]. For that purpose, alerts on the occurrence of peaks of pollution, freely available online for the general population, could be helpful. This exclusion of outdoor activities during the period of poor air quality could be added to the asthma action plan. Moreover, peak pollution levels could be concomitant with exposure to seasonal aeroallergens, with an additive negative impact on asthma outcomes [ 109 ]. It was also suggested that patients with asthma ought to live at least 300 m from major roadways to reduce the impact of pollutant exposure on their asthma [ 49 ]. Community-level interventions such as urban planning of “smart” cities with more green space at distance from major traffic arteries and industrial areas, as well as the development of walking and cycling paths separated from motorised streets, may reduce respiratory morbidity [ 108 ]. Governments must monitor air pollution, inform the population about the risks when air pollution levels are high and take measures in controlling the release of PM, like considering alternative fuels such as gas, fuel-cleaning options such as coal washing as well as alternative production processes and technologies [ 49 ]. For example, an European pediatric study showed that compliance with the WHO air quality guidelines for PM 2.5 could prevent 11% of all incident asthma cases, while the minimum air pollution levels for NO 2 (1.5 µg·m −3 ) and PM 2.5 (0.4 µg·m −3 ) were estimated to prevent 23% and 33% of incident cases, respectively [ 11 ].
The treatment of asthma exacerbations related to air pollution is not different from the usual clinical practice. All asthmatic patients must have a controller asthma treatment as recommended by current guidelines [ 8 ]. Inhaled corticosteroids (ICSs), the first choice treatment as a controller of asthma, proved to be beneficial in decreasing adverse responses to pollutant exposures [ 46 ]. Dietary supplements such as carotenoids, vitamin D and vitamin E are suggested to protect against airway inflammation and damage induced by pollutants that can trigger asthma initiation. Vitamin C, curcumin, choline and omega-3 fatty acids may also play a role [ 46 , 110 ]. Previous data showed that dietary intake of fruits and vegetables (e.g., Mediterranean diet) was associated with a better lung function, particularly among children exposed to O 3 [ 46 ]. However, this protective effect of dietary antioxidant intake seems more evident in children with low levels of outdoor air pollution exposure, but may be insufficient for the children exposed to higher amounts of air pollutants [ 111 ].
Most of enumerated measures to reduce the impact of air pollutants on asthma outcomes are easier to apply in developed countries with adapted economical resources than in low- or middle-income countries. Poor countries often lack the technology and resources to fight pollution because their economies are still growing, so their citizens are more at risk of respiratory and cardiovascular diseases related to high levels of air pollution. Energy production is one of the most polluting activites because much of this energy comes from coal. While developed countries are more likely to invest in cleaner fuel sources, and technologies that limit emissions, governments of developing nations just want to ensure energy for their citizens at competitive and accessible prices. Even the monitoring of air pollution is sometimes difficult in developing countries; it must be encouraged, and the use of mobile devices is a less expensive solution [ 1 , 5 ]. In addition, evidence suggests that asthma is underdiagnosed and undertreated in low-income countries [ 112 ]. However, access to proper diagnosis and treatment with controller medications for asthma (e.g., ICSs) is feasible and cost-effective even in low-resource settings by reducing symptoms, health care utilization, mortality and improving quality of life [ 112 ]. Actions by cities and national governments are needed in developing counties to minimize the impact of air pollution on their populations’ health. Monitoring of air quality, education for health, development of healthcare systems, active and public transport infrastructure, use of better methods of energy production (e.g., renewable energy sources) and technologies to reduce emissions are efficient measures that improve air quality and consequently life expectancy and worker productivity. It is important for developing nations to find a balance between economic growth and air quality to protect the health and standard of living of their citizens [ 50 , 113 ].
The effects of various air pollutants on asthma outcomes and their socio-economic impact are represented in Figure 1 .

Burden of air pollutants on asthma outcomes and socio-economic impact.
5. Indoor Air Pollution
5.1. tobacco smoke.
Tobacco smoking is the inhalation of smoke produced during the burning of tobacco leaves. Currently, more than 1.1 billion people are smokers and, additionally, another part of population is exposed to SHS. Tobacco smoke is a complex and dynamic mixture containing more than 7000 chemicals, of which at least 250 are known to be harmful and at least 69 are known to cause cancer [ 114 ]. Mainstream smoke is the aerosol drawn and inhaled directly by a smoker from a cigarette, cigar, or pipe, while sidestream smoke is the aerosol emitted in the surrounding air from a smoldering tobacco product [ 115 ]. Sidestream smoke is the main part of the SHS. The other main contributor to SHS is the exhaled portion of mainstream smoke. SHS is also known as passive smoking or environmental tobacco smoke. The aerosol of mainstream smoke is complex and consists of vapor and particulate phase. The main component of the vapor phase is the CO, but it also contains acetaldehyde, formaldehyde, acrolein, nitrogen oxides and CO 2 . Tar and nicotine form the particulate part of the mainstream smoke aerosol [ 116 ]. Tobacco smoking increases the risk of developing cardiovascular disease, stroke, COPD, lung cancer and other cancers [ 117 ]. It has an impact on asthma at various levels and it is a well known modifiable risk factor for symptom control and exacerbation. The prevalence of smoking among asthma patients is comparable to the general population (around 20%) [ 118 ].
Airway inflammation in asthmatic smokers differs from asthmatic non-smokers with higher total sputum cell counts, predominance of activated macrophages and neutrophils in sputum, airways, and lung parenchyma as in early COPD [ 119 , 120 ]. Previous data showed that smokers with asthma have higher sputum matrix metalloproteinase (MMP)-12 concentrations compared to non-smokers and the levels are inversely associated with lung function and positively related to sputum neutrophil counts [ 121 ]. This neutral endopeptidase is primarily responsible for the degradation of extracellular matrix components during the remodelling processes essential for normal tissue growth and repair. The excessive activity of MMPs and the impaired balance between them and their regulators, tissue inhibitors of metalloproteinases (TIMPs), have been implicated in the tissue-destructive processes associated with chronic lung diseases, including COPD and asthma [ 121 ]. In the same line, another study found reduced sputum MMP-9 activity/TIMP ratios in smokers with asthma compared with never-smokers. Low sputum ratios in asthmatic smokers were associated with persistent airflow obstruction and a reduced CT airway lumen area, which may indicate that an imbalance of MMP-9 and TIMPs contributes to structural changes to the airways in this group [ 121 ]. These results suggest that the persistent exposure to cigarette smoke drives additive or synergistic inflammatory and remodelling responses in the asthmatic airways [ 122 ]. In addition, the number of CD83+ mature DCs and B lymphocyte cells in bronchial biopsies are significantly lower in asthmatic smokers in comparison with never-smokers with asthma, which could explain the higher number of lower respiratory tract infections in the group of smokers [ 123 ]. The rhinovirus respiratory infection is an etiologic factor for severe asthma exacerbations necessitating hospitalization, and after adjustment for baseline asthma severity, rhinovirus-positive patients were more likely to be current smokers [ 124 ].
Asthmatic smokers are less sensitive to the therapeutic effects of inhaled and oral CSs in short- to medium term administration [ 125 , 126 , 127 , 128 , 129 , 130 , 131 , 132 , 133 , 134 , 135 , 136 , 137 , 138 , 139 ]. The impact of smoking in long-term CSs treatment still needs to be investigated, but the data collected showed that impairment in response to smoking was present, even after one year of treatment with ICSs, overruling the suggestion that the insensitivity could be improved with prolonged treatment [ 130 ]. Two main mechanisms explain the CSs insensitivity in smokers with asthma. The first one is the decrease in histone deacetylase-2 (HDAC-2) activity among smokers with increased inflammatory gene expression [ 131 , 132 , 133 , 134 ]. This is the consequence of oxidative stress. There are high levels of nitric oxide in tobacco smoke generating peroxynitrite, which leads to the inactivation of HDAC-2 via nitration and ubiquitination [ 135 , 136 ]. The second mechanism involves glucocorticoid receptor (GR). CSs act through the GR that has two alternative splicing isoforms, the GRα and GRβ. GRα is the classic and the functional isoform, through which the effects of CSs are mediated, while the overexpression of GRβ inhibits the action of the ligand-activated GRα [ 137 , 138 , 139 ]. The ratio of GRα/GRβ isoforms is reduced in peripheral blood mononuclear cells from current-smokers compared with non-smokers, including patients with asthma, which could lead to a lower CS [ 140 ]. These mechanisms could partially explain the poorer asthma outcomes in smokers with asthma.
Smoking patients with asthma form a separate phenotype that requires better understanding of underlying disease mechanisms and specific management.
5.2. Wood-Burning and Unflued Gas Heaters, Cooking Behaviors (Using Wood or Coal), Molds
Biomass combustion is known to be an important contributor to indoor air pollution in developing countries with resultant adverse health effects [ 141 ]. Residential heating devices can be a large contributor to ambient PM, notably in rural communities; the majority of these heating sources are old and inefficient, resulting in high levels of PM emissions [ 142 ]. The Consumer Product Safety Commission estimates that there are nearly 9 million wood stoves currently in use in the US [ 143 ]. Estimates of the contribution of wood-burning to ambient air quality can vary widely [ 144 ], but wood smoke accounts for 80–90% of the PM concentrations in communities with a high proportion of wood-burning households [ 145 ]. In the European Union, it is estimated that domestic woodstoves will be the dominant source of ambient PM 2.5 , accounting for 38% of all emissions by 2020 [ 146 ]. Follow-up of cohorts or panels of asthmatic patients have demonstrated that increases in levels of PM 10 and PM 2.5 in the indoor environment are associated with increases in severe asthma attacks, respiratory symptoms, asthma medication use, and hospital emergency department visits [ 147 , 148 ]. PM exposures have been shown to result in annual lung function growth deficits that are greater than those attributed to SHS exposure in children [ 149 ].
Unflued gas heaters (UFGHs) are a major source of NO 2 , nitrous acid and CO indoors, and they can also emit formaldehyde and produce water vapour [ 150 , 151 ]. Exposure to gas appliances or indoor NO 2 has been associated with a worsening of asthma symptoms in children and adults. A positive association was found between indoor NO 2 exposure and asthma exacerbations [ 152 ]. The effects of UFGHs exposure was studied in seventy-one patients with >55 years of age with mild to moderate asthma. A significant increase in respiratory symptoms was shown (wheeze and dyspnoea) when the people used UFGHs compared with days without exposure. In addition, there were significant increases in the average odds of reported wheeze and dyspnoea per hour of UFGH use. Small but significant reductions in morning to evening PEF and FEV 1 were observed on the days when UFGH was used compared with days when other/no heating was used [ 153 ].
Cooking has been also extensively studied as a source of indoor air pollution. Most people from low- or middle-income countries use, in their homes, biomass fuel (wood, animal dung and crop residues) or coal to cook, producing high levels of indoor pollution (e.g., CO, PM). Using coal and wood as cooking fuels were identified as risk factors of asthma in child and adult populations [ 154 , 155 ].
Indoor sources of molds may also be a risk factor for asthma [ 156 , 157 ]. If more studies showed a causal relationship between indoor mould exposure and the development/exacerbations of asthma in children, a limited level of evidence was found in the adult asthma population [ 157 ]. Several species, such as Aspergillus fumigatus and versicolor, Penicillium spp or Cladosporium sphaerospermum, herbarum and cladosporioides, display a more pronounced indoor tropism [ 158 ]. Exposure to increased daily levels of basidiospores and ascospores in the first 3 months of life were associated with increased odds of wheezing among children under 24 months in a cohort study in California [ 159 ]. Fungal components are biologically active and contribute to asthma development and the severity by IgE- and non-IgE-mediated mechanisms [ 156 ]. Fungal sensitization is associated with earlier onset of asthma and demonstrates a dose-dependent relationship of symptom severity and duration [ 160 ]. The senzitization to Aspergillus fumigatus and Penicillium spp was linked to an increased risk of severe asthma [ 158 ]. Alternaria alternata sensitization is a predictor of epidemic asthma in patients with seasonal asthma and is likely to be an important factor in thunderstorm-related asthma [ 161 ]. These data suggest that indoor mold exposure can initiate asthma and influence asthma outcomes.
5.3. Indoor Air Pollution and Asthma Outcomes
The asthmatics who are active smokers have increased morbidity and mortality, more severe symptoms, difficulties in controlling asthma, higher rates of exacerbations, worse quality of life, and an increased number of life-threatening asthma attacks [ 162 ]. This group of patients have more frequent unscheduled doctor visits and hospital admission, thus leading to the utilization of more health care resources [ 8 , 163 , 164 , 165 ]. The risk of death from asthma is increased in asthmatic patients with a smoking history of more than 20 pack-years [ 166 ]. In a cohort of 147 cases of near-fatal asthma attacks, smoking was associated with a higher in-hospital and post-hospitalization mortality [ 167 ]. Several population-based surveys demonstrate that smoking is strongly associated with poorer asthma control and this seems to be dose-dependent [ 168 ].
Exposure to SHS impacts asthma control and severity in both adults and children. A systematic review and meta-analysis has shown a nearly two-fold increase in the risk of hospitalization for asthma exacerbations in children with asthma and SHS exposure [ 169 ]. Adolescents with asthma exposed to SHS were at increased risk of having a dry cough at night, wheeze and sleep disturbance due to asthma symptoms. Dyspnea and exercise limitations were also more frequent among this population. The risk of having Emergency department/urgent care visits was two times higher in asthmatic adolescents exposed to SHS compared with unexposed adolescents. The group of adolescents with asthma were also 1.5 times more likely to use a rescue medication, nebulizer treatment, or other controlling medication and over 3.5 times more likely to have had an asthma attack that required the use of an oral or injected CS [ 170 ]. A recent study found that SHS in children aggravated the severity of asthma by affecting the balance of Treg/Th17 cells with a higher percentage of Th17 cells, while the percentage of Treg cells was reduced. SHS significantly reduced the levels of FoxP3 and tumor growth factor-β, which were associated with Treg cells, and increased the levels of interleukin-17A and interleukin-23, which were associated with Th17 cells [ 171 ]. Adults with asthma who are exposed to SHS have poor symptom control, worse quality of life, lower lung function and greater healthcare utilization [ 172 ].
Asthma itself is associated with a decline in lung function over time [ 173 ] and this process is more rapid in asthmatic smokers, compared with asthmatic non-smokers [ 174 , 175 , 176 , 177 ]. A smoking history of ≥10 pack-years seems to be a significant predictor factor of accelerated loss of lung function [ 176 ]. Regular/former smoking reduces lung function levels with a dose–response pattern (daily smoking rate and cumulative smoking) by affecting both larger and smaller airways [ 177 ].
Smoking is a predictive factor for ACO development, a term introduced to describe patients who have features of both asthma and COPD [ 8 ]. More than 25% of asthma patients with a smoking history of at least 10 pack-years have ACO [ 178 ]. It is important to actively look for features of ACO in asthmatic smokers because these patients have more frequent exacerbations, a poor quality of life, a more rapid decline in lung function, and high mortality, compared with asthma or COPD alone [ 8 , 179 ].
Exposure to high levels of PM from wood-burning or NO 2 from UFGHs is associated with more symptoms of asthma, a high rate of exacerbations and has a negative impact on lung function [ 147 , 148 , 152 ]. These effects seem more evident in children and older people with asthma [ 147 , 148 , 153 ]. Children exposed to biomass smoke from cooking have more frequent symptoms of severe asthma [ 180 ] and the risk of asthma-related symptoms is greater for males [ 180 ], while those exposed to NO 2 from cooking with natural gas have a higher risk of asthma exacerbations [ 21 ]. A large cross-sectional study performed in China showed that adults using coal for cooking have a higher risk for asthma symptoms than those without such exposure, and they have more asthma symptoms and poorer lung function in winter than in summer [ 181 ].
Several data sources suggested an association between the sensitization to Aspergillus fumigatus or Penicillium spp. and severe asthma [ 158 , 160 ]. A study perfomed in Mexico City including asthmatic patients found an association between the exposure to some molds, particularly Aspergillus fumigatus, Aureobasidium pullulans, Stachybotrys chartarum, Alternaria alternata, Cladosporium cladosporioides, Cladosporium herbarum, and Epicoccum nigrum, and uncontrolled asthma in males, but not in female patients, suggesting a possible gender susceptibility [ 182 ].
The effects of indoor air pollution on asthma are summarized in Table 2 .
Effects of indoor pollution on asthma according to their sources.
SHS: second-hand smoking.
A large cross-sectional Brazilian study including adult asthmatic patients evaluated the impact of smoking, indoor air pollution, and dual exposure on asthma outcomes. Exposure to indoor air pollution was associated with poorer asthma control, a higher proportion of severe asthma, and worsening of lung function in exposed vs. unexposed individuals. These effects were more important for the double-exposure. Exposure to indoor air pollution and double-exposure were predictive factors for uncontrolled and severe asthma in multivariate analysis [ 183 ].
All these findings suggest that indoor pollutants play a negative role on asthma outcomes, but, more importantly, an additive effect if they are associated.
5.4. Indoor Air Pollution and Asthma Management
The management of asthmatics exposed to indoor pollutants must include preventive measures to reduce/avoid exposure, such as smoking cessation, efficient household ventilation, use of clean fuels (e.g., methane, liquid petroleum gas, electricity, solar cookers), portable air cleaners, and pharmacologic interventions to optimize asthma treatment.
Smoking cessation must be encouraged in all possible ways to reduce the exposure of people with asthma. Smoking cessation in patients with asthma leads to better symptom control, less use of rescue medication, improved asthma quality of life score, lung function and AHR [ 184 , 185 ]. However, former-smokers have an accelerated FEV 1 decline for decades after smoking cessation compared to never-smokers, but less important than for current-smokers, suggesting a lasting and progressive lung damage induced by tobacco smoke [ 186 ]. Counseling and first-line medications for smoking cessation (nicotine replacement therapy, bupropion and varenicline) significantly increase quitting rates along with increasing the chance of preventing relapses [ 187 ]. E-cigarettes could be a valid option for asthmatic patients who cannot quit smoking by other methods. A study showed significant improvements in lung function, asthma control and AHR for asthmatic patients who chose this method for smoking cessation [ 188 ]; however, e-cigarettes might generate respiratory toxicants such as acrolein, formaldehyde, and acetaldehyde [ 189 ]. A study found that current e-cigarette use is associated with 39% higher odds of self-reported asthma compared to never e-cigarette users [ 190 ]. Therefore, the recommendation of e-cigarettes as a smoking cessation tool must be balanced against the short- and long-term safety of these products.
In general, it could be suggested that the treatment of asthmatic smokers should follow the international guidelines for asthma treatment [ 8 ], but this could be challenging due to the aforementioned. Although the data shows that asthmatic smokers have decreased sensitivity to ICSs, there are studies demonstrating that long-term ICS treatment may reduce the decline in lung function in smokers with asthma, with a greather benefit for people who have smoked <5 pack-years [ 191 , 192 ]. The combination therapy with ICS and long-acting beta-agonist (LABA) is probably the preferable option, in asthmatic smokers, to increasing the dose of ICS, due to the relative insensitivity and the potential adverse effects of the latter [ 128 , 193 , 194 ]. One of the most significant changes in asthma management was the announcement of the Global Initiative for Asthma (GINA) 2019 recommendations regarding the use of as-required ICS/LABA as rescue medication in symptomatic mild or moderate asthma. Trials investigating this rescue medication option are lacking in asthmatic smokers; it seems reasonable that current or former-smokers should not be excluded from this new GINA recommendation [ 195 ]. Montelukast, a leukotriene receptor antagonist, represents another therapeutic option as a controller of asthma. A study showed a significant benefit of the montelukast treatment (10 mg/day) on asthma control over 6 months compared to placebo in asthmatic patients actively smoking cigarettes. This effect is comparable to the administration of 250 μg of fluticasone propionate twice daily. However, the patients with a smoking history of more than 11 pack-years experienced better benefits with montelukast than with fluticasone. The explanation of these findings is that the more intensive exposure to tobacco smoke induces an increased synthesis of leukotrienes [ 196 ]. The effect of tiotropium, an inhaled long-acting muscarinic antagonist, is comparable in current-smokers and non-smokers with asthma treated by ICSs plus a second controller, with a significant improvement of symptoms and lung function in both groups [ 197 ]. Preliminary findings suggest that biologic therapies, such as omalizumab, mepolizumab, and dupilumab, improve clinical outcomes in smokers with asthma or ACO but current data is limited [ 198 ]. The group of asthmatic smokers forms a distinct asthma phenotype with worse outcomes, altered airway inflammation and changes in the response to pharmacological treatment. Smoking cessation interventions must start as early as possible. More studies investigating the effect of pharmacological treatments in this group of asthma patients are required.
Several studies showed the positive impact of the comprehensive statewide smoke-free indoor air laws on SHS exposure and the improvement of asthma outcomes through a reduction in prevalence, respiratory symptoms and exacerbations/hospitalizations [ 199 , 200 , 201 ]. The respect of smoke-free indoor environments and public areas is beneficial, and smoke-free indoor air laws should be enforced in all states. Several studies of children with asthma in urban environments have targeted the indoor environment to improve health outcomes. Improving household ventilation by opening windows or doors, using chimneys, hoods, or exhaust fans decreased asthma symptoms in children [ 202 , 203 ]. The intervention strategies (e.g., education by a health coach, remediation of the exposure by air cleaners) consistently demonstrated declines in asthma-related symptoms and significant improvements in peak expiratory flow [ 108 ]. However, all of these studies were conducted in urban environments, and the nature of these exposures likely differ from that of exposures in rural environments [ 204 , 205 , 206 ]. A study showed that installing non-polluting, more effective heating (with lower levels of indoor exposure to NO 2 ) in the homes of children with asthma significantly reduced respiratory symptoms, days off school, healthcare utilisation, and visits to a pharmacist [ 207 ]. Similarly, the replacement of UFGHs with high NO 2 emission in the schools by flue gas or electric heaters reduced asthma symptoms in children during 12 weeks following the intervention [ 208 ]. In contrast, household-level interventions, such as improved-technology wood-burning appliances or air-filtration devices did not affect quality-of-life measures among children with asthma and chronic exposure to wood-smoke [ 209 ]. If the avoidance of wood-smoke or UFGHs emission is not possible, several general cautionary measures could be made in practice.
A study found that the removal of indoor molds significantly improved asthma symptoms and reduced medication use at 6 and 12 months compared with the group without this intervention [ 210 ]. The advice of a Medical Indoor Environment Counselor could be also useful to improve the quality of the indoor environment [ 211 ]. This is summarized in the following Table 3 .
Recommendations to minimise indoor air pollution from heating sources.
UFGHs: Unflued gas heaters.
These measures could reduce the exposure but, unfortunately, the complete avoidance of the pollutants is impossible, so the asthma management plan and the controller medication use are mandatory for all asthmatics, as recommended by current guidelines [ 8 ].
Unfortunately, if most of these measures to reduce indoor pollution are feasible and already promoted in developed countries, their application in low- or middle-income countries is more difficult because of limited financial resources. If the use of air cleaners, low-polluting sources for cooking and heating, or personal devices to monitor indoor air pollution are less accessible for people who live in developing countries, government measures promoting health, such as smoking cessation programs and avoidance of SHS, education of people to improve household ventilation by opening windows or doors (at least during cooking periods) and mold removal, are cost-effective methods that could reduce the negative impact of indoor air pollution on asthma, and are feasible worldwide, independent of socioeconomic status.
6. Conclusions
Indoor and outdoor pollution represents a major public health threat with a negative impact on asthma outcomes. Current data showed that not only is the TRAP a risk factor for asthma development in children, but so are the NO 2 and SHS exposures. A causal relation between air pollution and the development of adult asthma is not yet clearly established. The exposure to ourdoor pollutants (O 3 , NO 2 , SO 2, CO, PM) could induce asthma symptoms, exacerbations and hospitalizations. The effects are dose and duration-dependent. A decrease in lung function was more frequently reported for O 3, NO 2 , SO 2 and PM. Active tobacco smoking is associated with poorer asthma control, more frequent exacerbations/hospitalizations, accelerated decline of lung function and a lower response to CS. Exposure to SHS increases the risk of asthma exacerbations, respiratory symptoms, healthcare utilization, and poor lung function. High-level exposures to indoor pollutants (e.g., PM from wood-burning or NO 2 from UFGHs) could induce more symptoms of asthma and higher rates of exacerbation, and have a negative impact on lung function. The sensitization to Aspergillus fumigatus and Penicillium spp seems to be associated with more severe asthma. These negative effects are more evident in children and older people with asthma. Asthma management according to current guidelines could reduce these effects but global measures are mandatory to minimize the exposure to indoor/outdoor pollutants and to improve asthma outcomes. Limited data exists for the effects of dual exposures (e.g., tobacco smoking and other air pollutants or associations between outdoor and indoor air pollutants) on asthma outcomes. Future research is needed on double or multiple exposures, as well as in the identification of a pattern of respiratory disease that increases susceptibility to air pollution.
Acknowledgments
The authors would like to thank the Interasma European Scientific network (INES).
Author Contributions
A.I.T. Conceptualization, writing—original draft preparation, review and editing, visualization, supervision; P.N., D.N., H.J.C.-N., S.N. Writing—original draft preparation, P.S., K.K. Writing—original draft preparation, review and editing. All authors have read and agreed to the published version of the manuscript.
This research received no external funding.
Conflicts of Interest
The authors declare no conflict of interest.
Advertisement
Air pollution prediction with machine learning: a case study of Indian cities
- Original Paper
- Published: 15 May 2022
- Volume 20 , pages 5333–5348, ( 2023 )
Cite this article
- K. Kumar ORCID: orcid.org/0000-0002-1884-0548 1 &
- B. P. Pande ORCID: orcid.org/0000-0001-9085-6731 2
33k Accesses
58 Citations
3 Altmetric
Explore all metrics
The survival of mankind cannot be imagined without air. Consistent developments in almost all realms of modern human society affected the health of the air adversely. Daily industrial, transport, and domestic activities are stirring hazardous pollutants in our environment. Monitoring and predicting air quality have become essentially important in this era, especially in developing countries like India. In contrast to the traditional methods, the prediction technologies based on machine learning techniques are proved to be the most efficient tools to study such modern hazards. The present work investigates six years of air pollution data from 23 Indian cities for air quality analysis and prediction. The dataset is well preprocessed and key features are selected through the correlation analysis. An exploratory data analysis is exercised to develop insights into various hidden patterns in the dataset and pollutants directly affecting the air quality index are identified. A significant fall in almost all pollutants is observed in the pandemic year, 2020. The data imbalance problem is solved with a resampling technique and five machine learning models are employed to predict air quality. The results of these models are compared with the standard metrics. The Gaussian Naive Bayes model achieves the highest accuracy while the Support Vector Machine model exhibits the lowest accuracy. The performances of these models are evaluated and compared through established performance parameters. The XGBoost model performed the best among the other models and gets the highest linearity between the predicted and actual data.
Similar content being viewed by others
Air Quality Prediction Using Machine Learning Methods: A Case Study of Bjelave Neighborhood, Sarajevo, BiH
Predictive Analysis of Air Pollutants Using Machine Learning
Prediction of Air Quality Using Machine Learning
Avoid common mistakes on your manuscript.
Introduction
Energy consumption and its consequences are inevitable in modern age human activities. The anthropogenic sources of air pollution include emissions from industrial plants; automobiles; planes; burning of straw, coal, and kerosene; aerosol cans, etc. Various dangerous pollutants like CO, CO 2 , Particulate Matter (PM), NO 2 , SO 2 , O 3 , NH 3 , Pb, etc. are being released into our environment every day. Chemicals and particles constituting air pollution affect the health of humans, animals, and even plants. Air pollution can cause a multitude of serious diseases in humans, from bronchitis to heart disease, from pneumonia to lung cancer, etc. Poor air conditions lead to other contemporary environmental issues like global warming, acid rain, reduced visibility, smog, aerosol formation, climate change, and premature deaths. Scientists have realized that air pollution bears the potential to affect historical monuments adversely (Rogers 2019 ). Vehicle emissions, atmospheric releases of power plants and factories, agriculture exhausts, etc. are responsible for increased greenhouse gases. The greenhouse gases adversely affect climate conditions and consequently, the growth of plants (Fahad et al. 2021a ). Emissions of inorganic carbons and greenhouse gases also affect plant-soil interactions (Fahad et al. 2021b ). Climatic fluctuations not only affect humans and animals but agricultural factors and productivity are also greatly influenced (Sönmez et al. 2021 ). Economic losses are the allied consequences too. The Air Quality Index (AQI) , an assessment parameter is related to public health directly. A higher level of AQI indicates more dangerous exposure for the human population. Therefore, the urge to predict the AQI in advance motivated the scientists to monitor and model air quality. Monitoring and predicting AQI, especially in urban areas has become a vital and challenging task with increasing motor and industrial developments. Mostly, the air quality-based studies and research works target the developing countries, although the concentration of the most deadly pollutant like PM 2.5 is found to be in multiple folds in developing countries (Rybarczyk and Zalakeviciute 2021 ). A few researchers endeavored to undertake the study of air quality prediction for Indian cities. After going through the available literature, a strong need had been felt to fill this gap by attempting analysis and prediction of AQI for India.
Various models have been exercised in the literature to predict AQI, like statistical, deterministic, physical, and Machine Learning (ML) models. The traditional techniques based on probability, and statistics are very complex and less efficient. The ML-based AQI prediction models have been proved to be more reliable and consistent. Advanced technologies and sensors made data collection easy and precise. The accurate and reliable predictions through such huge environmental data require rigorous analysis which only ML algorithms can deal with efficiently. Al-Jamimi et al. ( 2018 ) thoroughly discussed the importance of supervised ML algorithms for applied environment protection issues. The present work investigates six years of air pollution data of the Indian cities and analyzes twelve air pollutants and AQI. The dataset is preprocessed and cleaned first, then methods of data visualization are applied to develop better insights and to investigate hidden patterns and trends. This work exploits the essence of correlation coefficient with ML models which has been exercised by very few scholars in the literature (Alade et al. 2019a ). The data imbalance is identified and addressed with a resampling technique. Five popular ML models are exercised in context with this resampling technique. Their performances are then compared through standard metrics. These metrics are utilized by many scholars of the realm (see Table 1 ) and some other authors of ML applications like Ayturan et al. ( 2020 ), Alade et al. ( 2019b ), Al-Jamimi et al ( 2019 ), and Al-Jamimi and Saleh ( 2019 ), etc.
Section 2 presents the literature survey with a comparative analysis of the literary works in the realm of air quality prediction with ML. Section 3 describes the dataset being studied, preprocessing, and feature selection techniques applied. Section 4 deals with observing hidden patterns in the dataset through data visualisation. Section 5 is dedicated to the experimental design, analysis of seasonal trends, empirical results, and discussions. The final section concludes the present work.
Date: 17 February 2022.
Place: Qadian, Punjab and Pithoragarh, Uttarakhand, India.
A brief literature review
Gopalakrishnan ( 2021 ) combined Google’s Street view data and ML to predict air quality at different places in Oakland city, California. He targeted the places where the data were unavailable. The author developed a web application to predict air quality for any location in the city neighborhoods. Sanjeev ( 2021 ) studied a dataset that included the concentration of pollutants and meteorological factors. The author analyzed and predicted the air quality and claimed that the Random Forest (RF) classifier performed the best as it is less prone to over-fitting.
Castelli et al. ( 2020 ) endeavored to forecast air quality in California in terms of pollutants and particulate levels through the Support Vector Regression (SVR) ML algorithm. The authors claimed to develop a novel method to model hourly atmospheric pollution. Doreswamy et al. ( 2020 ) investigated ML predictive models for forecasting PM concentration in the air. The authors studied six years of air quality monitoring data in Taiwan and applied existing models. They claimed that predicted values and actual values were very close to each other. Liang et al. ( 2020 ) studied the performances of six ML classifiers to predict the AQI of Taiwan based on 11 years of data. The authors reported that Adaptive Boosting (AdaBoost) and Stacking Ensemble are most suitable for air quality prediction but the forecasting performance varies over different geographical regions. Madan et al. ( 2020 ) compared twenty different literary works over pollutants studied, ML algorithms applied, and their respective performances. The authors found that many works incorporated meteorological data such as humidity, wind speed, and temperature to predict pollution levels more accurately. They found that the Neural Network (NN) and boosting models outperformed the other eminent ML algorithms. Madhuri et al. ( 2020 ) mentioned that wind speed, wind direction, humidity, and temperature played a significant role in the concentration of air pollutants. The authors employed supervised ML techniques to predict the AQI and found that the RF algorithm exhibited the least classification errors. Monisri et al. ( 2020 ) collected air pollution data from various sources and endeavored to develop a mixed model for predicting air quality. The authors claimed that the proposed model aims to help people in small towns to analyze and predict air quality. Nahar et al. ( 2020 ) developed a model to predict AQI based on ML classifiers. Their authors studied the data collected over the tenure of 28 months by the ministry of environment, Jordan, and identified the concentrations of pollutants. Their proposed model detected the most contaminated areas with satisfying accuracy. Patil et al. ( 2020 ) presented some literary works on various ML techniques for AQI modeling and forecasting. The authors found that Artificial Neural Network (ANN) , Linear Regression (LR), and Logistic Regression (LogR) models were exploited by most of the scholars for AQI prediction.
Bhalgat et al. ( 2019 ) applied the ML technique to predict the concentration of SO 2 in the environment of Maharashtra, India. The authors concluded that being highly polluted, some cities of this Indian province require grave attention. The authors mentioned that their model was not capable of exhibiting expected outputs. Mahalingam et al. ( 2019 ) developed a model to predict the AQI of smart cities and tested it in Delhi, India. The authors reported that the medium Gaussian Support Vector Machine (SVM) exhibited maximum accuracy. The authors claim that their model can be used in other smart cities too. Soundari et al. ( 2019 ) developed a model based on NNs to predict the AQI of India. The authors claimed that their proposed model could predict the AQI of the whole county, of any province, or of any geographical region when the past data on concentration of pollutants were available.
Sweileh et al. ( 2018 ) came up with a very interesting study about the analysis of global peer-reviewed literature about air pollution and respiratory health. The authors extracted 3635 documents from the Scopus database published between 1990 and 2017. They observed that there was a substantial increase in publications from 2007 to 2017. The authors reported active countries, institutions, journals, authors, international collaborations in the realm and concluded that research works on air pollution and respiratory health had been receiving a lot of attention. They suggested securing public opinions about mitigation of outdoor air pollution and investment in green technologies. Zhu et al. ( 2018 ) refined the problem of AQI prediction as a multi-task learning problem. The authors utilized large-scale optimization techniques and endeavored to reduce the number of parameters. Based on their empirical results, they claimed that the proposed model exhibited better results than existing regression models.
Bellinger et al. ( 2017 ) carried out a detailed literature analysis on the application of ML and data mining methods toward air pollution epidemiology. The authors found that the researchers from Europe, China, and the USA were very active in this realm and the following classifiers had been widely applied: Decision Tree (DT) , SVMs , K-means clustering, and the APRIORI algorithm. Rybarczyk and Zalakeviciute ( 2017 ) endeavored to develop a model that correlated traffic density with air pollution. The author mentioned that such traffic data collection was economical, and integrating it with meteorological features boosted accuracy. The authors found that the hybrid model performed the best and accuracy based on morning time data was the highest.
Table 1 shown below presents a concise and comparative analysis of the literary works in the realm of AQI prediction.
It has been observed that research works in air quality analysis and prediction for Indian cities acquired lesser attention from scholars. In spite of the fact that out of the ten most polluted cities in the world, nine cities are Indian (Deshpande 2021 ), very few researchers investigated AQI prediction from the Indian perspective. The present work endeavors to fill this gap by studying 5 years of substantial air pollution data from twenty-three Indian cities. The current study is an earnest attempt to contribute to the literature with novel ideas of data visualizations, exploiting correlation coefficient-based statistical outliers for analytics, and comparison of five key ML models over standard performance metrics.
Material and methods
Some Indian cities fall in the array of the most polluted cities in the world, and the threat of air pollution is being raised day by day. Poor air quality in India is now considered a significant health challenge and a major obstacle to economic growth. According to a new study released jointly by a UK-based non-profit management firm, Dalberg Advisors and Industrial Development Corporation , air pollution in India caused annual losses of up to Rs 7 lakh crore ($95 billion) (Dalberg 2019 ). The main pollutant emissions in India are due to the energy production industry, vehicle traffic on roads, soil and road dust, waste incineration, power plants, open waste burning, etc. The present research investigates air pollution data extracted from the Central Pollution Control Board (CPCB) , India. Footnote 1 This dataset possesses observations from January 2015 to July 2020 and it is comprised of 12 features with 29,531 instances from 23 different Indian cities. Table 2 presented below provides brief descriptive statistics of the pollutants/particles and AQI from this dataset.
Analysis of some major air pollutants such as PM 2.5 , PM 10 , NO 2 , CO, SO 2 , O 3, etc. and prediction of AQI are the essence of the current work. The methodological steps of the adopted process are presented in the following figure (Fig. 1 ).
Data preprocessing
Quality of data is the first and most important prerequisite for effective visualization and creation of efficient ML models. The preprocessing steps help in reducing the noise present in the data which eventually increases the processing speed and generalization capability of ML algorithms. Outliers and missing data are the two most common errors in data extraction and monitoring applications. The data preprocessing step performs various operations on data such as filling out not-a-number (NAN) data, removing or changing outlier data, etc. Figure 2 shown below presents a view of the missing values in each feature of the dataset. Observe that among all other features, Xylene has the most missing values and CO has the least missing values. A large number of missing values may be existing due to a variety of factors, such as a station that can sense data but does not possess a device to record it.
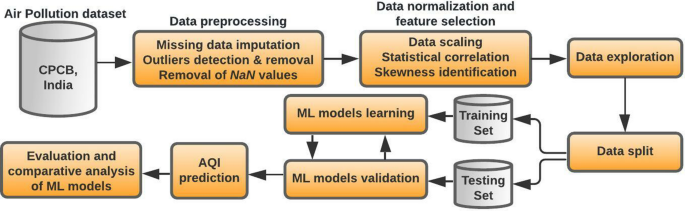
Flowchart of the proposed model
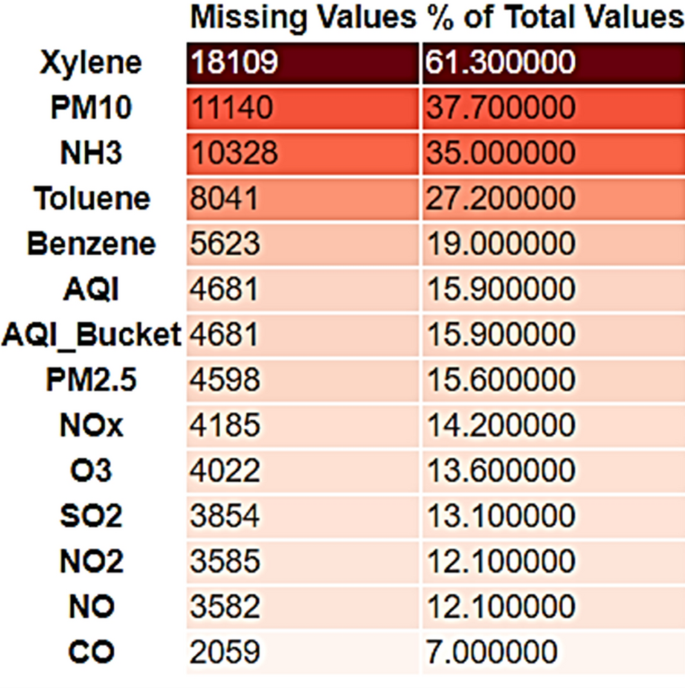
Missing values of the features and their percentages
All the missing values are filled with the median values against each feature to solve the missing data problem. Next, a normalisation process has been applied to standardize the data, ensuring that the significance of variables is unaffected by their ranges or units. The data normalisation process helps to bring different data attributes into a similar scale of measurement. This process plays a vital role in the stable training of ML models and boosts performance. The datatypes of all the variables are also examined during normalisation. For example, the dataset is collected from different monitoring stations which deal with different representations of dates. Thus, the date ‘Monday, May 17, 2021’ may be represented as ‘17/5/2021’ or as ‘17–05-2021’ etc. Such date feature has been normalised through the datetime Python library.
Feature selection
The CPCB dataset under study involves a specific parameter viz, AQI and government agencies use this parameter to alert people about the quality of the air and also practice forecasting it. According to the National Ambient Air Quality Standards , there are six AQI categories: good (0–50), satisfactory (51–100), moderate (101–200), poor (201–300), very poor (301–400), and severe (401–500). Scholars in the realm suggest that reducing input variables lowers the computational cost of modeling and enhances prediction performance. A correlation-based feature selection method has been exploited in the present work to determine the optimal number of input variables (pollutants) when developing a predictive model. Statistical correlation-based feature selection algorithms compute correlations between every pair of the input variable and the target variable. The variables possessing the strongest correlation with the target variable are then filtered for further study. Since many ML algorithms are sensitive to outliers, any feature in the input dataset which does not follow the general trend of that data must be found. For the present dataset, a correlation-based statistical outliers detection method has been applied to identify the outliers. To select significant features, the correlation analysis of the AQI feature has been exercised with features of other pollutants. Figure 3 , shown below clearly reveals that pollutants PM 10 , PM 2.5 , CO, NO 2 , SO 2 , NO X, and NO are generally responsible for the AQI to attain higher values. These pollutants are correlated with AQI based on the correlation values above the threshold of 0.4.
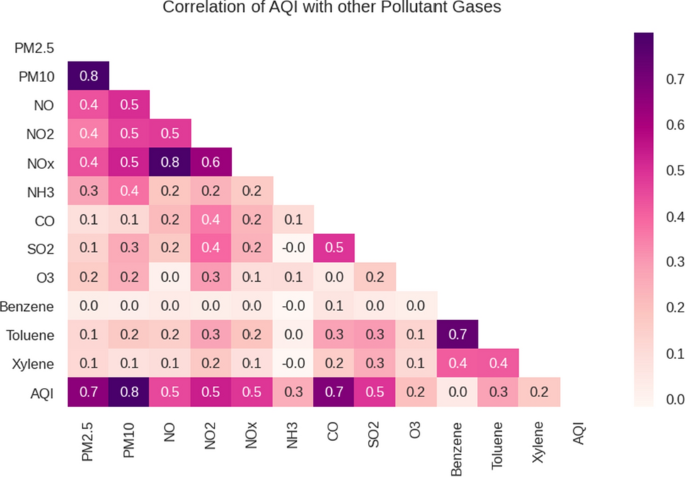
Correlation heatmap of AQI with other pollutants (Threshold: 0.4)
Table 3 given below shows the exact correlation values of each pollutant of the dataset with AQI.
Many ML models function better when data have a normal distribution and underperform when data have a skewed distribution. Therefore, it is necessary to identify the skewness being present in the features and to perform some transformations and mappings which convert the skewed distribution into a normal distribution. Figure 4 , given below shows that the features of Benzene , Toluene , CO, and Xylene are highly skewed. To make these skewed features more normal, the logarithmic transformations have been used to reduce the impact of outliers by normalising magnitude differences.
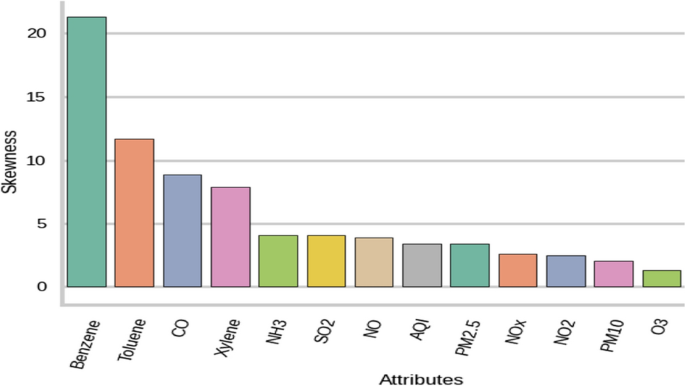
Skewness present in dataset features
- Exploratory data analysis
This section of the present study deals with data exploration and analysis for finding various hidden patterns present in the dataset. Exploratory data analysis is the first step in data analytics which is performed before applying any ML model. Under this, the following important things are being analyzed: (a) exploring statuses and trends of air pollutants over the past six years i.e. from 2015 to 2020; (b) exploring the distribution of pollutants in the air along with top-six polluted cities with their average AQI values; and (c) estimating top four pollutants which are directly involved in increasing the AQI values.
Exploring the trends of air pollutants over the last six years
India has become one of the few countries having the most severe air pollution resulting from rapid industrialization and booming urbanization over the last several years. Air pollution is among grave public health and environmental issues, and the Health Effects Institute (HEI) ranks it among the top five global risk factors for mortality (IHME 2019 ). According to the HEI research, the emission of PM was the third leading cause of death in 2017, and this rate was highest in India. Based on the emissions of PM 2.5 and other pollutants, the World Health Organization (WHO) ranked India as the fifth most polluted country (Gurjar, 2021 ). The trends of various pollutants from 2015 to 2020 are observed and shown in the figure below (Fig. 5 ). Observe that except for O 3 and Benzene , all other pollutants exhibited a significant fall in 2020. The year 2020 witnessed the most strict lockdown in the history of mankind and ceased industrial, automobile, and aviation activities in India and the world served as some ambrosia for the ailing environment and air.
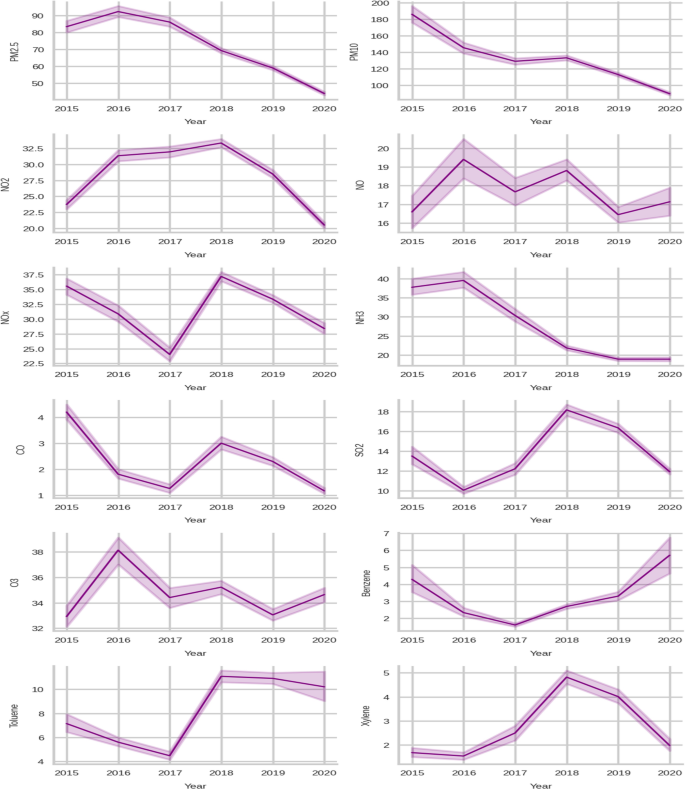
Intensities of various pollutants from 2015 to 2020
Figure 6 shown below depicts the average AQI values over the aforementioned tenure for the six most polluted cities in India.
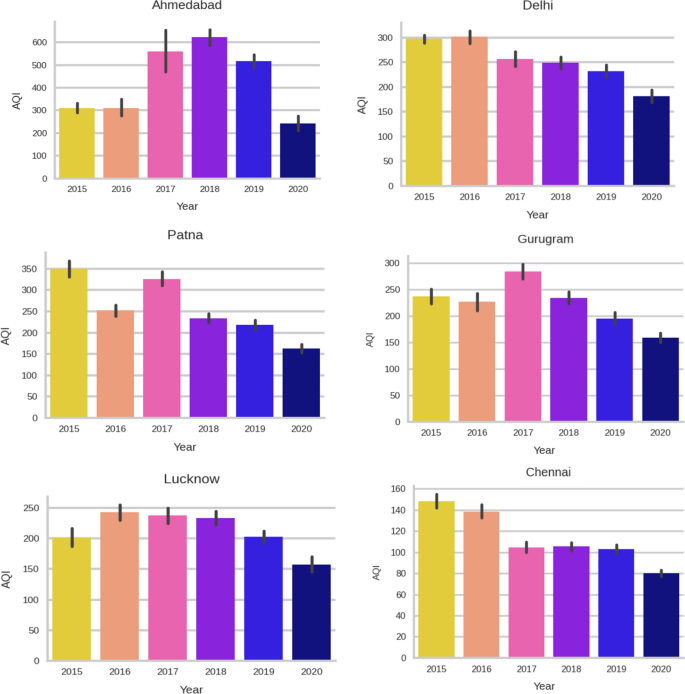
The six most polluted Indian cities with their average AQI values from 2015 to 2020
Pollutants that are directly involved in increasing AQI values
The correlation values between different pollutants and AQI have been exercised and the pollutants for which this correlation value is greater than the threshold of 0.5, i.e. the correlation is strongly positive have been identified. Figure 7 shown below depicts the concentration of four such pollutants in various cities in India.
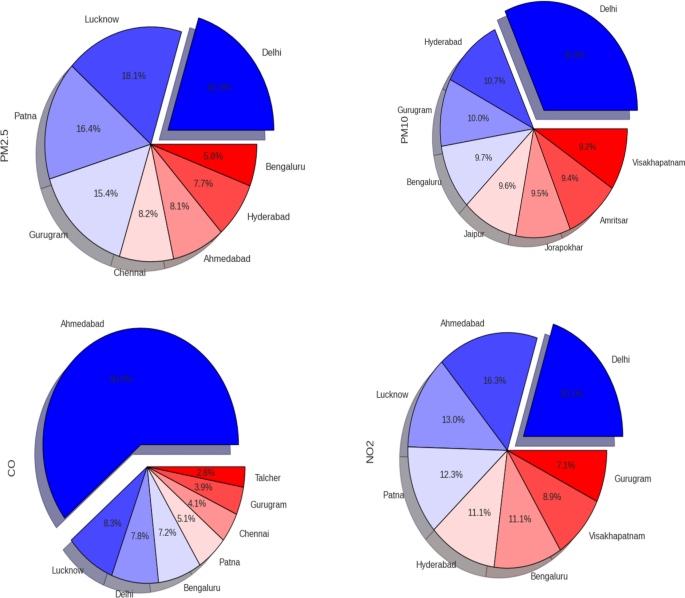
Pollutants governing AQI directly
Results and discussion
This section deals with the experimental design and empirical analysis for predicting AQI values through the pollutants present in the air. The air pollution dataset is split into training (75%) and testing (25%) subsets before evaluating ML models. The Google Colab Pro cloud platform with Intel(R) Xeon(R) CPU @ 2.30 GHz, Tesla P100-PCIE-16 GB, 12.8 GB RAM, and 180 GB of disc space has been utilized for executing Python scripts. The Python libraries like Scikit-learn , NumPy , Pandas , Seaborn , etc. are exploited for various data processing tasks. Next, the dataset is explored with the motive to find the overall value of the AQI with respect to those pollutants which have a significant role in raising the AQI value. In Fig. 8 shown below, a timeline graph of AQI is depicted over some particular pollutants which are directly responsible for higher values of AQI. From Fig. 8 , it is clear that each pollutant grows and drops year after year, and their values do not remain constant every year. PM 2.5 and PM 10 have seasonal effects, with higher pollution levels in the winter than in the summer. After 2018, the level of SO 2 began to rise, but the level of O 3 stayed unchanged from 2018 to 2020. The same trend can be seen in BTX Footnote 2 levels as well. Except for CO, practically every pollutant has exhibited seasonal variations.
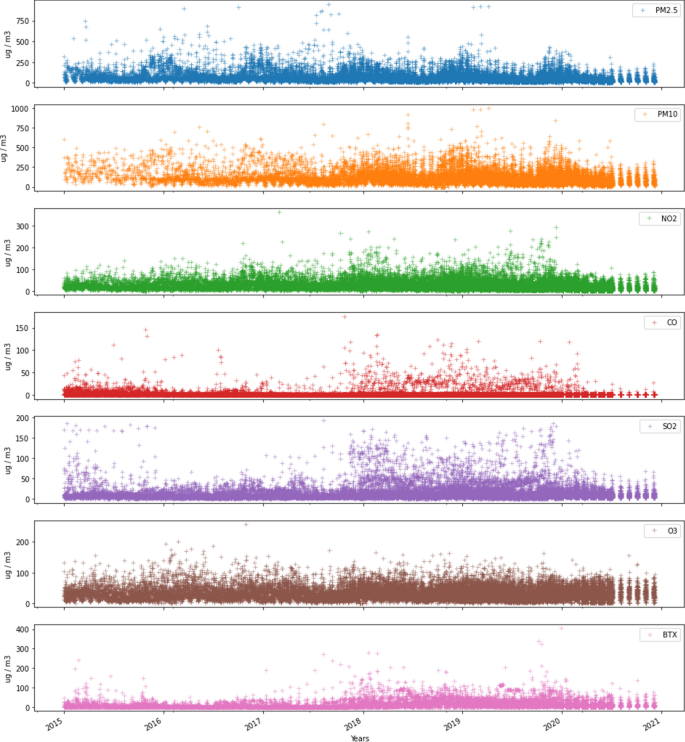
Timeline graph of AQI with respect to specific pollutants
To examine the seasonality of the data thoroughly, Box plot visualizations are employed. Box plots categorise data into different periods by grouping the entire information in years and months. Figure 9 presents the Box plots of various pollutants over time, both annually and monthly. Notice that pollution levels in India decrease between June and August. It may be the consequence of the inception of the Monsoon in the Indian subcontinent during this tenure. BTX levels exhibit a significant drop between March and April, a modest rise from May to September, and a sharp surge from October to December. The median values for 2020 are lower than those for previous years, indicating that pollution may have decreased substantially in 2020. Strict lockdown ceased human and industrial activities in India during the COVID-19 pandemic are the obvious reasons for this observed phenomenon.
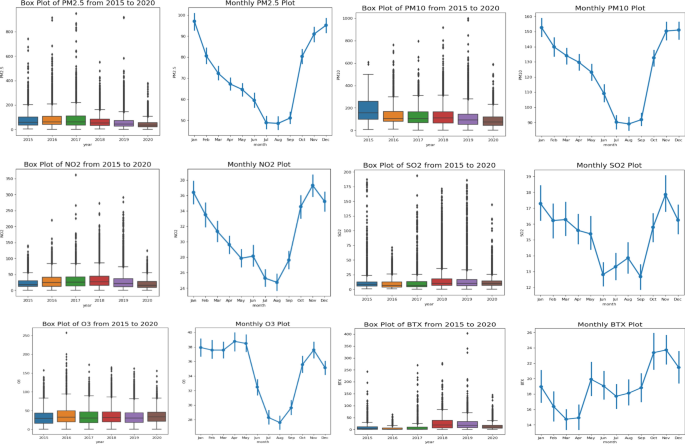
Variation analysis of pollutants through Box plots
Next, the detailed development of ML-based AQI prediction models is discussed. Finally, the performance of the AQI forecasting models is evaluated. The target attribute, AQI_Bucket has some missing values which result in the unequal splitting of the classes. Many ML models ignore this imbalanced datasets problem which may lead to poor classification and prediction performances. To overcome this data imbalance problem, the SMOTE (Synthetic Minority Oversampling Technique) has been applied. In this technique, the algorithm synthesizes new elements for minority classes rather than creating copies of already existing elements. It functions by randomly choosing a point from the minority class and computing the k-nearest neighbor distances for the selected point. The newly created synthetic points are added between the chosen point and its neighbors. To implement SMOTE for class imbalance, we have used an imbalanced-learn Python library in the SMOTE class. Now, five popular ML models, KNN , Gaussian Naive Bayes (GNB) , SVM , RF , and XGBoost have been employed to predict the AQI level with SMOTE and without SMOTE resampling technique. Table 4 shown below presents the results of used ML models in terms of accuracy, precision, recall, and F1-score during the training phase. Precision tells the fraction of relevant instances present in the retrieved instances, while recall is the fraction of relevant instances that have been retrieved. Accuracy is the ratio of the correctly labeled attributes to the whole pool of variables. F1-score is a weighted average of precision and recall. Note that the XGBoost model achieved the highest accuracy, while the SVM model exhibited the lowest accuracy.
The performances of the ML models for the training set are evaluated against the standard performance parameters, viz MAE , RMSE , Root Mean Squared Logarithmic Error (RMSLE) , and coefficient of determination, i.e. R 2 (Table 5 ). These performance measures have been exploited extensively in the literature. Table 5 given below provides error statistics of the ML models applied with and without SMOTE resampling technique on the training set. The XGBoost model outperformed other models in terms of error statistics when exercised without the SMOTE technique. On the other hand, the RF model performed relatively good among others in terms of error statistics when exercised with the SMOTE technique. The XGBoost model performed equally good in this area in terms of MAE and RMSLE. These observations are marked bold in Table 5 .
Table 6 shown below presents the results of employed ML models obtained during the testing phase. It is evident from Table 6 that the XGBoost model surpassed the other models again, whereas the SVM model attained the lowest accuracy in the testing phase too.
The performances of the ML models for the testing set are evaluated against the standard performance parameters as above (Table 7 ).
The above table summarizes the performances of various ML models applied with and without SMOTE resampling technique on the testing set. It is observed that all ML models exhibited improvement in almost all assessment metrics when applied with SMOTE resampling technique. The GNB model attained the best values of R 2 in both cases. The XGBoost model performed the best in terms of error statistics and attained the most optimum values in both experimental genres. These observations are marked bold in Table 7 .
Prediction of air quality is a challenging task because of the dynamic environment, unpredictability, and variability in space and time of pollutants. The grave consequences of air pollution on humans, animals, plants, monuments, climate, and environment call for consistent air quality monitoring and analysis, especially in developing countries. However, lesser attention for researchers has been observed for AQI prediction for India. In the present work, air pollution data of 23 Indian cities for a tenure of six years are investigated. The dataset is cleaned and preprocessed first by filling NAN values, addressing outliers, and normalising data values. Then correlation-based feature selection technique is exercised to filter AQI affecting pollutants for further study and logarithmic transformations are applied to the skewed features. The exploratory data analysis methods are exercised to find various hidden patterns present in the dataset. It was found that almost all pollutants exhibited a significant fall in 2020. The data imbalance problem is addressed by the SMOTE analysis. The dataset is split into train-test subsets by the ratio of 75–25% respectively. ML-based AQI prediction is carried out with and without SMOTE resampling technique and a comparative analysis is presented. The results of ML models for both the train-test subsets are presented in terms of standard metrics like accuracy, precision, recall, and F1-Score. For both the train-test sets, the XGBoost model attained the highest accuracy and the SVM model exhibited the lowest accuracy. The classical statistical error metrics, namely MAE, RMSE, RMSLE, and R 2 are then evaluated to assess and compare the performances of ML models. The XGBoost model comes out to be the overall best performer by attaining the optimum values in both training and testing phases. For the training phase, the RF model performed relatively good when exercised with SMOTE. On the other hand, almost all ML models exhibited improvements in the testing phase. In this phase, the GNB model attained the best results for R 2 in target predictions. The present research endeavors to contribute to the literature by addressing air quality analysis and prediction for India which might have not been properly studied. This work can be extended by employing deep learning techniques for AQI prediction.
The dataset can be downloaded from: https://app.cpcbccr.com/ccr/#/caaqm-dashboard-all/caaqm-landing/data .
BTX is the combined name given to Benzene , Toluene , and Xylene.
Alade IO, Rahman MAA, Saleh TA (2019a) Predicting the specific heat capacity of alumina/ethylene glycol nanofluids using support vector regression model optimized with Bayesian algorithm. Sol Energy 183:74–82. https://doi.org/10.1016/j.solener.2019.02.060
Article CAS Google Scholar
Alade IO, Rahman MAA, Saleh TA (2019b) Modeling and prediction of the specific heat capacity of Al 2 O 3 /water nanofluids using hybrid genetic algorithm/support vector regression model. Nano-Struct Nano-Objects 17:103–111. https://doi.org/10.1016/j.nanoso.2018.12.001
Al-Jamimi HA, Saleh TA (2019) Transparent predictive modelling of catalytic hydrodesulfurization using an interval type-2 fuzzy logic. J Clean Prod 231:1079–1088. https://doi.org/10.1016/j.jclepro.2019.05.224
Al-Jamimi HA, Al-Azani S, Saleh TA (2018) Supervised machine learning techniques in the desulfurization of oil products for environmental protection: a review. Process Saf Environ Prot 120:57–71. https://doi.org/10.1016/j.psep.2018.08.021
Al-Jamimi HA, Bagudu A, Saleh TA (2019) An intelligent approach for the modeling and experimental optimization of molecular hydrodesulfurization over AlMoCoBi catalyst. J Mol Liq 278:376–384. https://doi.org/10.1016/j.molliq.2018.12.144
Ayturan YA, Ayturan ZC, Altun HO, Kongoli C, Tuncez FD, Dursun S, Ozturk A (2020) Short-term prediction of PM2.5 pollution with deep learning methods. Global NEST J 22(1):126–131
CAS Google Scholar
Bellinger C, Jabbar MSM, Zaïane O, Osornio-Vargas A (2017) A systematic review of data mining and machine learning for air pollution epidemiology. BMC Public Health. https://doi.org/10.1186/s12889-017-4914-3
Article Google Scholar
Bhalgat P, Bhoite S, Pitare S (2019) Air Quality Prediction using Machine Learning Algorithms. Int J Comput Appl Technol Res 8(9):367–370. https://doi.org/10.7753/IJCATR0809.1006
Castelli M, Clemente FM, Popoviˇc A, Silva S, Vanneschi L (2020) A machine learning approach to predict air quality in California. Complexity 2020(8049504):1–23. https://doi.org/10.1155/2020/8049504
Dalberg (2019) Air pollution and its impact on business: the silent pandemic. https://www.cleanairfund.org/wp-content/uploads/2021/04/01042021_Business-Cost-of-Air-Pollution_Long-Form-Report.pdf
Deshpande T (2021) India Has 9 Of World's 10 most-polluted cities, but few air quality monitors. indiaspend. https://www.indiaspend.com/pollution/india-has-9-of-worlds-10-most-polluted-cities-but-few-air-quality-monitors-792521
Doreswamy HKS, Yogesh KM, Gad I (2020) Forecasting Air pollution particulate matter (PM2.5) using machine learning regression models. Procedia Comput Sci 171:2057–2066. https://doi.org/10.1016/j.procs.2020.04.221
Fahad S, Sönmez O, Saud S, Wang D, Wu C, Adnan M, Turan, V (2021a) Plant growth regulators for climate-smart agriculture (1st ed.). CRC Press. https://doi.org/10.1201/9781003109013
Fahad, S, Sönmez O, Saud S, Wang D, Wu C, Adnan M, Turan V (2021b) Sustainable soil and land management and climate change (1st ed.). CRC Press. https://doi.org/10.1201/9781003108894
Gopalakrishnan V (2021) Hyperlocal air quality prediction using machine learning. Towards data science. https://towardsdatascience.com/hyperlocal-air-quality-prediction-using-machine-learning-ed3a661b9a71
Gurjar BR (2021) Air pollution in india: major issues and challenges. energy future 9(2):12–27. https://www.magzter.com/stories/Education/Energy-Future/AIR-POLLUTION-IN-INDIA-MAJOR-ISSUES-AND-CHALLENGES
IHME (2019) State of global air 2019 report. http://www.healthdata.org/news-release/state-global-air-2019-report
Liang Y, Maimury Y, Chen AH, Josue RCJ (2020) Machine learning-based prediction of air quality. Appl Sci 10(9151):1–17. https://doi.org/10.3390/app10249151
Madan T, Sagar S, Virmani D (2020) Air quality prediction using machine learning algorithms–a review. In: 2nd international conference on advances in computing, communication control and networking (ICACCCN) pp 140–145. https://doi.org/10.1109/ICACCCN51052.2020.9362912
Madhuri VM, Samyama GGH, Kamalapurkar S (2020) Air pollution prediction using machine learning supervised learning approach. Int J Sci Technol Res 9(4):118–123
Google Scholar
Mahalingam U, Elangovan K, Dobhal H, Valliappa C, Shrestha S, Kedam G (2019) A machine learning model for air quality prediction for smart cities. In: 2019 international conference on wireless communications signal processing and networking (WiSPNET). IEEE 452–457. https://doi.org/10.1109/WiSPNET45539.2019.9032734
Monisri PR, Vikas RK, Rohit NK, Varma MC, Chaithanya BN (2020) Prediction and analysis of air quality using machine learning. Int J Adv Sci Technol 29(5):6934–6943
Nahar K, Ottom MA, Alshibli F, Shquier MA (2020) Air quality index using machine learning—a jordan case study. COMPUSOFT, Int J Adv Comput Technol 9(9):3831–3840
Patil RM, Dinde HT, Powar SK (2020) A literature review on prediction of air quality index and forecasting ambient air pollutants using machine learning algorithms 5(8):1148–1152
Rogers CD (2019) Pollution's impact on historical monuments pollution's impact on historical monuments. SCIENCING. https://sciencing.com/about-6372037-pollution-s-impact-historical-monuments.html
Rybarczyk Y, Zalakeviciute R (2017) Regression models to predict air pollution from affordable data collections. In: H. Farhadi (Ed.), Machine learning advanced techniques and emerging applications pp 15–48. IntechOpen. https://doi.org/10.5772/intechopen.71848
Rybarczyk Y, Zalakeviciute R (2021) Assessing the COVID-19 impact on air quality: a machine learning approach. Geophys Res Lett. https://doi.org/10.1029/2020GL091202
Sanjeev D (2021) Implementation of machine learning algorithms for analysis and prediction of air quality. Int. J. Eng. Res. Technol. 10(3):533–538
Sönmez O, Saud S, Wang D, Wu C, Adnan M, Turan V (2021) Climate change and plants: biodiversity, growth and interactions (S. Fahad, Ed.) (1st ed.). CRC Press. https://doi.org/10.1201/9781003108931
Soundari AG, Jeslin JG, Akshaya AC (2019) Indian air quality prediction and analysis using machine learning. Int J Appl Eng Res 14(11):181–186
Sweileh WM, Al-Jabi SW, Zyoud SH, Sawalha AF (2018) Outdoor air pollution and respiratory health: a bibliometric analysis of publications in peer-reviewed journals (1900–2017). Multidiscip Respiratory Med. https://doi.org/10.1186/s40248-018-0128-5
Zhu D, Cai C, Yang T, Zhou X (2018) A machine learning approach for air quality prediction: model regularization and optimization. Big Data and Cognitive Comput. https://doi.org/10.3390/bdcc2010005
Download references
Acknowledgements
No organization funded the present research.
Author information
Authors and affiliations.
Sikh National College, Qadian, Guru Nanak Dev University, Amritsar, Punjab, India
Department of Computer Applications, LSM, Government PG College, Pithoragarh, Uttarakhand, India
B. P. Pande
You can also search for this author in PubMed Google Scholar
Corresponding author
Correspondence to B. P. Pande .
Ethics declarations
Conflict of interest.
The authors declare that they have no conflict of interest.
Additional information
Editorial responsibility: M. Abbaspour.
Rights and permissions
Reprints and permissions
About this article
Kumar, K., Pande, B.P. Air pollution prediction with machine learning: a case study of Indian cities. Int. J. Environ. Sci. Technol. 20 , 5333–5348 (2023). https://doi.org/10.1007/s13762-022-04241-5
Download citation
Received : 18 December 2021
Revised : 17 February 2022
Accepted : 19 April 2022
Published : 15 May 2022
Issue Date : May 2023
DOI : https://doi.org/10.1007/s13762-022-04241-5
Share this article
Anyone you share the following link with will be able to read this content:
Sorry, a shareable link is not currently available for this article.
Provided by the Springer Nature SharedIt content-sharing initiative
- Air quality index
- Machine learning
- Indian air quality data
- Correlation-based feature selection
- Synthetic minority oversampling technique
- Find a journal
- Publish with us
- Track your research
- - Google Chrome
Intended for healthcare professionals
- Access provided by Google Indexer
- My email alerts
- BMA member login
- Username * Password * Forgot your log in details? Need to activate BMA Member Log In Log in via OpenAthens Log in via your institution

Search form
- Advanced search
- Search responses
- Search blogs
- Air pollution and...
Air pollution and family related determinants of asthma onset and persistent wheezing in children: nationwide case-control study
- Related content
- Peer review
- Gitte J Holst , PhD student 1 ,
- Carsten B Pedersen , professor 2 ,
- Malene Thygesen , doctoral student 2 ,
- Jørgen Brandt , professor 3 ,
- Camilla Geels , senior researcher 3 ,
- Jakob H Bønløkke , associate professor 4 ,
- Torben Sigsgaard , professor 1
- 1 Department of Public Health, Section of Environment, Occupation and Health, Aarhus University, Bartholin Allé 2, 8000 Aarhus C, Denmark
- 2 National Centre for Register-Based Research, School of Business and Social Sciences, Aarhus University, Denmark
- 3 Department of Environmental Science, Aarhus University, Roskilde, Denmark
- 4 Department of Occupational and Environmental Medicine, Danish Ramazzini Centre, Aalborg University Hospital, Aalborg, Denmark
- Correspondence to: T Sigsgaard ts{at}ph.au.dk (or @torbensigsgaard on Twitter)
- Accepted 24 June 2020
Objective To identify risk factors (air pollution and family related) for the onset of asthma and persistent wheezing in children.
Design Nationwide case-control study.
Setting Denmark.
Participants All Danish children born from 1997 to 2014 and followed for asthma onset and persistent wheezing from age 1 year to 15 years.
Main outcome measure Onset of asthma and persistent wheezing.
Results A higher incidence of asthma was found in children of parents with asthma (adjusted hazard ratio 2.29 (95% confidence interval 2.22 to 2.35) and mothers who smoked during pregnancy (1.20, 1.18 to 1.22), whereas a lower incidence was found in children of parents with high educational attainment (0.72, 0.69 to 0.75) and high incomes (0.85, 0.81 to 0.89). Exposure to particulate matter ≤2.5 µm (PM 2.5 ) and ≤10 µm (PM 10 ) and nitrate was associated with an increased risk of asthma and persistent wheezing, with hazard ratios per 5 µg/m 3 increase in pollutant concentrations 1.05 (1.03 to 1.07) for PM 2.5 , 1.04 (1.02 to 1.06) for PM 10 , and 1.04 (1.03 to 1.04) for nitrogen dioxide. Only the positive association of PM 2.5 with asthma and persistent wheezing remained robust across the different models and in sensitivity analyses.
Conclusions The findings of this study suggest that children exposed to higher levels of PM 2.5 are more likely to develop asthma and persistent wheezing than children who are not exposed. Other risk factors associated with these outcomes were parental asthma, parental education, and maternal smoking during pregnancy.
Introduction
Asthma is among the most prevalent diseases in children worldwide. 1 As children of parents with asthma are more prone to develop the disease, genetic susceptibility has been considered an important risk factor. 2 The rapid increase in prevalence of asthma and allergy globally suggests that besides genetic factors, environmental and social factors might also play a part.
Exposure to passive smoking is recognised as an important environmental risk factor for the development of asthma in children. 3 4 Urbanisation has been associated with asthma, 5 but it is unknown what factors, or combination of factors, such as environmental exposures, activities, or health behaviours might be part of the association. 5 6 Residential area might also reflect socioeconomic status, as measured by parental income, and educational level has been linked with asthma in children. 7 8
Peaks in exposure to air pollution have been associated with exacerbation of asthma, 9 10 whereas the role of long term exposure and timing of exposure for asthma onset is less clear. Many studies have been limited to non-representative populations, covering children in families from certain areas and socioeconomic groups. This might introduce selection bias and affect the analysis of the influence of socioeconomic status on asthma and the role of socioeconomic status and related factors in the effect of air pollution.
The development of asthma and wheezing is likely to result from a complex interplay between environmental and social factors, rather than from individual factors alone, underscoring the need to explore the relation between air pollution and asthma in large, representative populations. Using a registry based nationwide approach covering all Danish children born during 1997-2014, we explored a combination of air pollution and family related factors potentially contributing to asthma onset and persistent wheezing. We determined whether asthma onset and persistent wheezing was associated with family related factors, whether high levels of air pollution in the children’s residential area was associated with an increased risk of asthma and persistent wheezing, and whether associations of air pollution with asthma and persistent wheezing vary by family related factors.
Study design and study population
We used survival analysis methods to follow Danish children born from 1997 to 2014 for asthma onset and persistent wheezing using a national registry based, matched case-control design. Cases were drawn from the entire Danish population and comprised all children with a diagnosis of asthma or with a minimum of two prescriptions for asthma medicine (n=122 842) from their 1st to 15th birthday. For each case we selected 25 controls at random who could serve as controls several times (n=3 069 943), had no asthma diagnosis, and were matched by sex and birthday to within one week. All children and their parents were born in Denmark.
Asthma and wheezing outcome assessment
We identified children with asthma and persistent wheezing from the Danish National Patient Register, which contains data on all people admitted to public hospitals from 1977 onwards, and we obtained information on dispensed drugs from the Danish National Prescription Registry from 1994 and onwards. Cases were classified as having asthma or persistent wheezing, or both if they had been admitted to a public hospital or been treated as an outpatient and received a first diagnosis of asthma (international classification of diseases, 10th revision; ICD-10 code J45 or J46) as the final diagnosis at the visit (release diagnosis), or they had a minimum of two prescriptions for asthma medicine (based on the Anatomical Therapeutic Chemical Classification System; adrenergic inhalants (R03A), other drugs for obstructive airway diseases (R03B), adrenergics for systemic use (R03C), and other systemic drugs for obstructive airway disease (R03D)). A recent Danish validation study compared data on asthma discharge diagnosis from the national register with prescription data and found that true asthma in children could be identified with reasonable accuracy; the positive predictive value of having two different asthma drugs prescribed in one year was 80% for the presence of true asthma, with a sensitivity of 59%. 11 Moreover, validation studies concluded that by using this definition of asthma both true cases and virus related wheezing as well as persistent wheezing due to other environmental factors would likely be identified. 11 12 13 Therefore in this study we use both the terms asthma and persistent wheezing for the outcome. We defined the date of onset as either the day when asthma was first diagnosed or the date of a second prescription for asthma drugs, whichever came first.
Exposure to air pollution
Concentrations of pollutants were modelled on an hourly basis for all of Denmark from 1 January 1997 to 31 December 2014. Pollutants of interest comprised several different gases and aerosols or particles: ozone, nitrogen dioxide (NO 2 ), nitrate (NO 3 – ), combined nitrogen oxide (NO) and nitrogen dioxide, particulate matter ≤2.5 µm (PM 2.5 ) and ≤10 µm (PM 10 ), sulphur dioxide (SO 2 ), sulfate (SO 4 − ), ammonia (NH 3 ), ammonium (NH 4 + ), secondary inorganic aerosols (the sum of nitrate, sulfate, and ammonium), elemental carbon, organic carbon, and sea salt. Modelled air pollution data were obtained from the newly updated Danish air pollution modelling system DEHM/UBM(THOR) 14 15 16 (see supplementary file for further details). We used an average of hourly air pollution concentrations during the past 3, 6, and 12 months before the index date for which the case and matched controls were identified. Air pollution concentrations were linked to the children’s residential addresses with a detailed spatial resolution of 1 km×1 km grid cells, taking into account any changes in residence.
Assessment of family related factors
We obtained information about parental asthma from the Danish National Patient Register based on information on asthma diagnosis (ICD-8, 493; ICD-10, J45 or J46) and a minimum of two prescriptions of asthma medicine. From the Medical Birth Registry, for a subgroup of the study population (n=120 521), we had information on mothers’ reported smoking at the first meeting with a midwife during pregnancy. Smoking information was categorised into two variables. One variable categorised mothers as either non-smoker, quit smoking during pregnancy, and smoking during pregnancy. A second, more detailed variable also included daily number of cigarettes smoked. Information on parental education was derived from the education register and reflected the highest completed parental education for the mother or the father and was categorised into primary school and short, medium, and long education. Information on parental income was obtained from the income register and categorised into mother’s and father’s income based on the quintiles of income for the general population. To study both parental education and income, a combined variable was generated adding the level of income (level 1-5) or education (level 1-4) for each parent generating a seven level categorical variable for parental education and a nine level variable for parental income.
Statistical analysis
We used survival analyses techniques to follow all children from their 1st to their 15th birthday between 1998 and 2014 for the risk of asthma. Risk ratios equivalent to hazard ratios along with 95% confidence intervals were estimated using conditional logistic regression with each case-control set forming separate stratums to investigate the association of air pollution and family related factors of asthma onset and persistent wheezing.
Family related factors were analysed as categorical variables and air pollutant concentrations were analysed for each 1, 5, and 10 µg/m 3 increase in concentrations using exposure to air pollution during the past 12 months before asthma onset as the primary exposure of interest.
To study the association of air pollution with risk of asthma and persistent wheezing, we undertook three a priori specified confounder models. Model 1 included matching the variables of age, sex, date of birth, and calendar year. Model 2 additionally adjusted for parental asthma, and model 3 additionally adjusted for parental education and parental income.
We conducted several sensitivity analyses based on confounder model 3. We explored the past 3, 6, and 12 month exposure time windows, different exposure concentrations comparing each interquartile range with each µg increase in concentration, adjustment for other air pollutants one at a time, adjustment for maternal smoking during pregnancy, trends in risk over time, and first born children.
To study the consistency among effects of air pollution, we stratified model 3 by sex (boy versus girl), age groups (<6 years and ≥6 years), parental asthma (none, only father asthmatic, only mother asthmatic, both parents asthmatic), maternal smoking during pregnancy (non-smoker, quit smoking during pregnancy, smoking during pregnancy), parental educational level (level 1-7), and income level (level 1-9).
Statistical analyses were conducted using STATA (version 14. StataCorp, College Station, TX).
Patient and public involvement
As this is a register based study, patients and public were not involved in the design, conduct, or reporting of this research. The study did not include the funding or researchers and patient training necessary to include patients or members of the public in the design of the study.
In total, 3 192 785 children were included in the study. Of these, 122 842 children were identified as having asthma and persistent wheezing. Most cases (83%, n=101 348) occurred among children younger than 3 years (supplementary table E1), with a mean age of 1.9 years (SD 2.2) for developing asthma and persistent wheezing.
Asthma onset and persistent wheezing in relation to family related factors
Parental asthma was positively associated with a higher risk of asthma and persistent wheezing in offspring (supplementary table E1). If mothers had asthma this was associated with a higher risk than if fathers had asthma (hazard ratio 1.72 (95% confidence interval 1.72 to 1.77) versus 1.51 (1.48 to 1.53)). Having two parents with asthma was associated with a 2.40-fold (2.34-fold to 2.47-fold) increased risk of asthma and persistent wheezing. Maternal smoking during pregnancy was associated with an increased risk of asthma, and a positive dose-response relation was found between the number of cigarettes smoked daily and an increased risk of asthma and persistent wheezing. Moreover, both mother’s and father’s incomes as well as educational levels were independently associated with asthma and persistent wheezing, showing that higher household income and educational level were associated with a reduced risk of asthma and persistent wheezing. This finding was most pronounced for maternal education level, with a reduced risk of asthma and persistent wheezing (hazard ratio 0.58, 95% confidence interval 0.56 to 0.59) in children of mothers with the highest compared with the lowest education levels. The combined variables of parental income and education showed a similar trend in associations. When the determinants were mutually adjusted for one another, the association of parental income with asthma and persistent wheezing was only shown for the four highest income levels compared with the lowest income level ( fig 1 , see supplementary table E2). To study trends in asthma risk over time, five year intervals were compared: 1998-2002, 2003-08, and 2009-14. The results remained robust for parental asthma, parental education, and maternal smoking during pregnancy, but were not similarly consistent over time for parental income (supplementary table E3).

Effects of family related factors on risk of asthma and persistent wheezing after adjustment for age, calendar year, parental asthma, parental education, parental income, and mother’s smoking behaviour during pregnancy. Level 1 refers to the lowest level of parental education and parental income
- Download figure
- Open in new tab
- Download powerpoint
Risk of asthma and persistent wheezing from high levels of air pollution in children’s residential area
Mean air pollutant concentrations ranged considerably, from 0.4 µg/m 3 (SD 0.1) for elemental carbon to 58.8 µg/m 3 (SD 5.1) for ozone ( table 1 ). Air pollutant concentrations were generally moderately to highly correlated (supplementary table E4), with the highest correlations found between secondary inorganic aerosols, ammonium, sulfate, and nitrate (Pearson’s correlation coefficient r=0.71-0.95), elemental carbon and organic carbon (r=0.98), nitrogen dioxide and combined nitrogen oxide and nitrogen dioxide (r=0.98), PM 2.5 and PM 10 (r=0.95), and between ammonium and PM 2.5 (r=0.88) and ammonium and PM 10 (r=0.83).
Average air pollution concentrations during 12 months before the date of first diagnosis of asthma or persistent wheeze
- View inline
In the first analyses (model 1) ( fig 2 , supplementary table E5), PM 2.5 , PM 10 , nitrate, ammonium, and secondary inorganic aerosols were positively associated with asthma and persistent wheezing, whereas nitrogen dioxide, sulphur dioxide, elemental carbon, and organic carbon were inversely associated with asthma and persistent wheezing. When these effects were adjusted for parental asthma (model 2, fig 2 ), findings were similar, although the point estimates were slightly attenuated. After adjustment for parent’s education and income (model 3, fig 2 ), the positive association for secondary inorganic aerosols and ammonium disappeared, but the positive associations between PM 2.5 , PM 10 , nitrogen dioxide and asthma remained, with hazard ratios for asthma and persistent wheezing per 5 µg/m 3 increase in pollutant concentrations of 1.05 (1.03 to 1.07) for PM 2.5 , 1.04 (1.02 to 1.06) for PM 10 , and 1.04 (1.03 to 1.04) for nitrogen dioxide. Positive associations were also found for sulphur dioxide, nitrogen dioxide, and combined nitrogen oxide and nitrogen dioxide, whereas ozone and sea salt were inversely associated with asthma and persistent wheezing.

Effects of air pollutants on risk of asthma and persistent wheezing. Model 1 was adjusted for sex, age, and calendar year. Model 2 was additionally adjusted for parental asthma. Model 3 was additionally adjusted for parental income and parental education. A log10 scale is used
Robustness of the results in model 3 were investigated, considering: exposure time windows of 3, 6, and 12 months (supplementary table E6); differences in results for increases in interquartile ranges versus increases for each 1, 5, and 10 µg/m 3 increment in concentrations (supplementary table E7); adjusting for any other pollutant by including one pollutant simultaneously in each logistic regression analysis in a two pollutant model (supplementary table E8); adjusting for maternal smoking during pregnancy (supplementary table E9); investigating trends in risk for asthma and persistent wheezing in relation to air pollution over time (supplementary table E10); and studying firstborn children to explore whether associations were related to shared factors in certain families (supplementary table E11). These sensitivity analyses showed that associations of PM 2.5 with asthma and persistent wheezing remained robust for direction, magnitude, and statistical significance, whereas the findings for the other air pollutants were not consistently robust.
Effect of air pollution across subpopulations
Stratified analyses of air pollution with asthma and persistent wheezing by sex, age group, parental asthma, maternal smoking during pregnancy, and parental educational and income level showed consistency between boys and girls (supplementary table E12), parental asthma (supplementary table E13), parental educational level (supplementary table E14), income level (supplementary table E15), and maternal smoking status during pregnancy (supplementary table E16). When considering different age groups (<6 years and ≥6 years) ( fig 3 , supplementary table E17), associations between air pollutants and asthma and persistent wheezing showed a different pattern, and strength of associations for children younger than 6 years and 6 years or older. For children aged 6 years or older, no associations were found between PM 2.5 , PM 10 , and nitrate and asthma and persistent wheezing, whereas inverse associations were found for ammonium, sea salt, and ozone, and positive associations were found for elemental carbon and organic carbon.
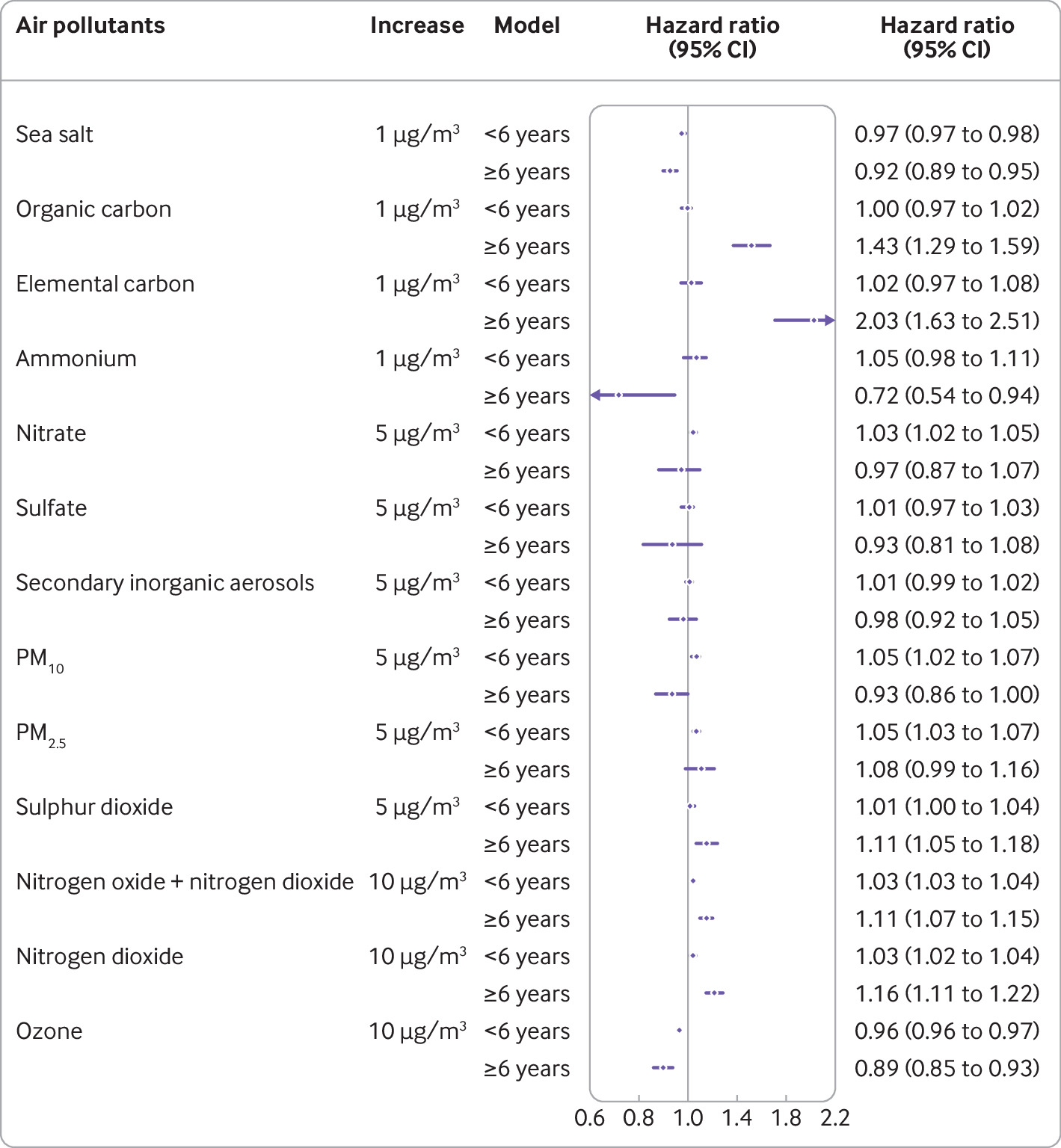
Effects of air pollutants on risk of asthma and persistent wheezing by age groups after adjustment for sex, age, calendar year, parental asthma, parental income, and parental education. A log10 scale is used
In this nationwide study, we found that Danish children who had parents with asthma, a mother who smoked during pregnancy, or parents with low education and low income were at a higher risk of developing asthma and persistent wheezing independently of exposure to air pollution. Sensitivity analyses showed robust associations between parental asthma, parental education, and maternal smoking during pregnancy and asthma and persistent wheezing in offspring. For associations of air pollution concentrations with asthma and persistent wheezing, the most consistent finding was the positive association of PM 2.5 with asthma indicating that exposure to PM 2.5 might be responsible for an increase in the numbers of children with asthma and persistent wheezing worldwide. Our analyses of effect modification indicated potential susceptibility by age as we found different associations between air pollutant concentrations and asthma in different age groups.
Comparison with previous studies
Family related determinants.
The strongest determinant for asthma incidence and persistent wheezing was parental asthma. This was expected, as genetic disposition is recognised as a strong risk factor for the development of asthma and allergy. 2 17 Using parental asthma as a proxy for children’s genetic disposition for asthma is, however, a proxy of the genetic liability of developing asthma.
We found that maternal smoking during pregnancy was associated with an increased risk of asthma and persistent wheezing in offspring. Exposure to tobacco is well established as an important risk factor for asthma. 18 Children whose mothers quit smoking during pregnancy had an increased risk of childhood asthma and persistent wheezing, indicating that even some exposure to tobacco smoke during gestation is harmful, and this is supported by other studies. 8 However, we had no information on exposures of the children to smoking after delivery, and therefore it is unclear whether it is exposure during pregnancy or after delivery that influences the results.
Among other risk factors for asthma and persistent wheezing, we found parental income and educational level to be significant. Generally, heterogeneous results have been found between socioeconomic status and asthma in previous studies, 7 19 20 possibly as a result of difficulties in assessment of socioeconomic status and the uncertainty about whether socioeconomic status is the driver for development of asthma. Health behaviour related to socioeconomic status—physical activity, diet, alcohol consumption, smoking, and healthcare—are suggested to be among the underlying drivers for the development of asthma. 21 Information on the children’s or their family’s lifestyle could have provided further insights. This information was not, however, available from the registries.
Air pollution and potential effect modifiers
Despite heterogeneity in study designs and methods used across studies, in our study the results were in line with a meta-analysis 22 and a population based birth cohort study, 23 together with other prospective studies that have identified PM 2.5 as a potential risk factor for asthma in children. 24 25 26 Notably, two of the mentioned studies even observed an increased risk of asthma at PM 2.5 levels lower than the ones reported in our study (overall mean 12.2 µg/m 3 (SD 1.5) in our study). The pollution levels in Denmark are lower than in, for example, highly populated regions in central Europe, but are higher than, for example, the rest of Scandinavia. The proposed annual limit in Europe for PM 2.5 is 20 µg/m 3 ; however, further reductions in PM 2.5 might reduce the number of children developing asthma and having persistent wheezing.
Traffic related air pollution has been suggested to influence the development of asthma, and besides exposure to PM 2.5 , the effects on asthma in children have been observed for exposure to nitrogen dioxide, 27 28 even at current low European levels. 29 This is in accordance with our findings, which showed a general pattern of positive associations for elemental carbon, organic carbon, PM 2.5 , PM 10 , sulphur dioxide, combined nitrogen oxide and nitrogen dioxide, and nitrogen dioxide with asthma and persistent wheezing. This indicates that for asthma onset, local sources of air pollution (eg, traffic) might be more of a factor than long distance sources. In line with this, the observed inverse associations of ozone and sea salt with asthma and persistent wheezing could reflect the more rural distribution pattern of these pollutants and that ozone is negatively correlated with concentrations of nitrogen dioxide and combined nitrogen oxide and nitrogen dioxide that are increased in urban areas. More long term studies are needed to explore and confirm the role of primary and secondary air pollution emissions and to explore both short term and long term exposure to identify the critical exposure window. Furthermore, the mechanisms underlying the association between air pollution and asthma have not been fully clarified. The present experimental studies have, however, proposed that oxidative stress and tissue damage, airway modelling, inflammatory pathways, and immunological responses might play a key role in combination with genetic susceptibility. 9
Different patterns of associations between air pollutants and asthma and persistent wheezing were found for children younger and older than 6 years. Again, associations were more pronounced for local sources of air pollution. Whether these associations reflect different effects of air pollution in themselves in different age groups or are related to different asthma phenotypes is unclear. Previous studies have suggested that younger children might be more susceptible to the harmful effects of air pollution than older children. 30 It has been proposed that early onset asthma is related to inflammation and later onset asthma (from around age 6 years) commonly has an allergic origin. 31 However, we had no information on atopic status, lung function, inflammatory markers, and disease progress for the children, which could have helped to explain potential differences of these factors. Therefore, in future studies, it would be interesting to distinguish between asthma phenotypes to rule out whether they might be associated with air pollutants in different ways.
Strengths and limitations of this study
A major strength of our study was the registry based design, which provides a large study population, highly comprehensive data, and no selection bias as all Danish children were included in the study with no losses to follow-up. Therefore, the study population is representative of Danish children across all social classes and varied lifestyles in a broad age group compared with previous studies, allowing us to examine the timing of exposure in relation to asthma onset. Additionally, the prospective data collection of both exposure and outcome data ensures temporality (the exposure precedes the outcome) and therefore enhances the possibility of causal interferences. The age and time matched design minimises confounding from factors related to age, sex, and calendar year. Another strength of the study is the high spatial and temporal resolution for various air pollutants, which allowed for a detailed exposure assessment over the full period.
In our study population, we defined asthma as either being diagnosed on hospital discharge or when a child had received a minimum of two prescriptions for asthma drugs. This ensured high accuracy as the asthma was diagnosed by a physician and treatment was prescribed by the child’s general practitioner. Asthma diagnosis based on hospital diagnosis might reflect more severe or more acute cases of asthma, whereas a diagnosis based on prescription of asthma drugs likely reflects less severe cases of asthma and comprises persistent wheezing related to viruses and other environmental factors. By including two prescriptions, we minimised the risk of including children with transient airway symptoms but no asthma diagnosis.
The moderate to high correlations between the air pollutants in this and similar studies hampers the investigation of pollutant specific effects. When we adjusted for other air pollutants in the two component analyses, the results were similar to those in the primary analyses (in model 3). We cannot, however, rule out confounding by other air related factors that were not adjusted for, such as meteorological conditions, pollen, and fungal spores. As this problem on high correlation is well known, it has been suggested to consider air pollutants as a dynamic mixture of pollutants that interact and change over time rather than as individual exposures in isolation from other pollutants. However, no such ideal statistical method exists.
Furthermore, to define the children’s personal exposure we used modelled exposure data to assess ambient air pollution at the children’s residential addresses. An underlying assumption was that the children spent most of their time in the area of their residential address. Although personal air monitoring can measure fewer air pollutants, personal monitoring could yield a more valid exposure assessment by monitoring the children’s exposure during daily activities and exact locations (ie, day care centres and schools). Owing to the design and size of the study, however, this was not possible. As most of the study population comprised preschool aged children, we believe that the children spent most of their time at home or at a local day care centre. Furthermore, we accounted for changes in residential address, increasing the validity of the exposure assessment.
Besides the studied determinants, other risk factors could have played a role as potential confounders in our study. A shortcoming of our study is therefore the lack of individual level information such as on nutrition and physical activity, factors related to the children’s indoor environment, and perinatal and childhood factors, such as delivery by caesarean section, 31 32 breast feeding, 33 and infections. 34
Conclusions
The findings from this study strengthen the evidence that parental asthma, parental education, and maternal smoking during pregnancy are risk factors for asthma onset and persistent wheezing in children. Moreover, we found that children exposed to high levels of PM 2.5 were more likely to develop asthma and to have persistent wheezing. These findings therefore support emerging evidence that exposure to air pollution might influence the development of asthma. This finding needs to be substantiated in future studies.
What is already known on this topic
The rapid increase in prevalence of asthma worldwide suggests that along with heredity (familial asthma history), environmental, behavioural, and social factors might also contribute to asthma incidence
Among risk factors, short term peak exposure to air pollution has been associated with exacerbation of asthma, whereas the risks of long term exposure and the timing of exposure for the onset of asthma is less clear
In addition, the role of air pollution and its occurrence with other risk factors, such as socioeconomic status, on asthma is unclear
What this study adds
This nationwide study found evidence that heredity, socioeconomic status, and maternal smoking during pregnancy are potential risk factors for new asthma onset and persistent wheezing in children
These findings contribute to the emerging evidence that ambient fine particles smaller than 2.5 µm (PM 2.5 ) contribute to the development of asthma and persistent wheezing
The results suggest that further reductions in PM 2.5 might help to reduce the number of children who develop asthma and persistent wheezing in highly exposed populations
Contributors: GJH, CBP, and TS conceived the study. GJH, CBP, MT, and TS contributed to the study design. MT, CG, and JB prepared and cleaned the data. JB and CG contributed to the exposure assessment. CBP provided scientific input on statistical methods. GJH performed the data analyses and took the lead in drafting the manuscript. All authors contributed to the interpretation of data, provided critical revisions to the manuscript, and approved the final draft. The corresponding author attests that all listed authors meet authorship criteria and that no others meeting the criteria have been omitted. GJH, TS, CBP, and JB act as guarantors.
Funding: This study was supported by NordForsk under the Nordic Programme on Health and Welfare (project No 75007): understanding the link between air pollution and distribution of related health impacts and welfare in the Nordic countries (NordicWelfAir), and by BERTHA—the Danish Big Data Centre for Environment and Health funded by the Novo Nordisk Foundation Challenge Programme (grant NNF17OC0027864).
Competing interests: All authors have completed the ICMJE uniform disclosure form at www.icmje.org/coi_disclosure.pdf and declare: support from NordForsk and the Danish Big Data Centre for Environment and Health, funded by the Novo Nordisk Foundation; no financial relationships with any organisations that might have an interest in the submitted work in the previous three years; no other relationships or activities that could appear to have influenced the submitted work.
Ethical approval: Not required.
Data sharing: Data are not available owing to a strict data sharing agreement. Data on individual characteristics were requested through Statistics Denmark from the Danish Civil Registration System and data on asthma from the Danish National Patient Register. Environmental data can be requested on payment from the Department of Environmental Sciences, Aarhus University. The overall link for information on residential coordinates with exposure assessment, personal characteristics, and diagnosis history was performed within Statistics Denmark, which requires all users to have permission to access data and carry out analyses.
The lead author (the manuscript’s guarantor) affirms that the manuscript is an honest, accurate, and transparent account of the study being reported; that no important aspects of the study have been omitted; and that any discrepancies from the study as planned (and, if relevant, registered) have been explained.
Dissemination to participants and related patient and public communities: We plan to disseminate the results to relevant patient communities, at conferences, and through popular science articles and newspapers.
This is an Open Access article distributed in accordance with the Creative Commons Attribution Non Commercial (CC BY-NC 4.0) license, which permits others to distribute, remix, adapt, build upon this work non-commercially, and license their derivative works on different terms, provided the original work is properly cited and the use is non-commercial. See: http://creativecommons.org/licenses/by-nc/4.0/ .
- ↵ Akinbami L, Simon A, Rossen L. Changing Trends in asthma Prevalence Among Children. Pediatrics. 2016; 137: e2 0152354.
- Haslam PL ,
- Gratziou CG ,
- Tobacco Control Committee of the European Respiratory Society
- Leonardi-Bee J ,
- Rodriguez A ,
- Brickley E ,
- Rodrigues L ,
- Normansell RA ,
- Barreto M ,
- Matsui EC ,
- McCormack MC ,
- Gilbreath S ,
- Grabenhenrich LB ,
- Guarnieri M ,
- Strickland MJ ,
- Darrow LA ,
- Christensen LB ,
- Langfrits MS ,
- Stensballe LG ,
- Haerskjold A ,
- Thomsen SF ,
- Maslova E ,
- Mortensen EL ,
- Granström C ,
- Christensen JH ,
- Palmgren F ,
- Berkowicz R ,
- Berkowicz R
- Silver JD ,
- Perzanowski MS ,
- Platts-Mills T ,
- Lundbäck B ,
- Hollams EM ,
- de Klerk NH ,
- Winquist A ,
- Tolbert P ,
- Flanders WD ,
- Shmool JL ,
- Kubzansky LD ,
- Newman OD ,
- Spengler J ,
- Shepard P ,
- Clougherty JE
- O’Lenick CR ,
- Mulholland JA ,
- Parslow R ,
- Nieuwenhuijsen M
- Gehring U ,
- Carlsten C ,
- Dybuncio A ,
- Chan-Yeung M ,
- Eeftens M ,
- Tétreault LF ,
- Gamache P ,
- Berhane KT ,
- Achakulwisut P ,
- Anenberg SC
- Koppelman GH ,
- Brunekreef B
- Brunst KJ ,
- Brokamp C ,
- Mahmoudi M ,
- Duong-Quy S ,
- Thavagnanam S ,
- Fleming J ,
- Bromley A ,
- Shields MD ,
- Cardwell CR
- Sonnenschein-van der Voort AM ,
- Jaddoe VW ,
- van der Valk RJ ,
- Papadopoulos NG ,
- Christodoulou I ,
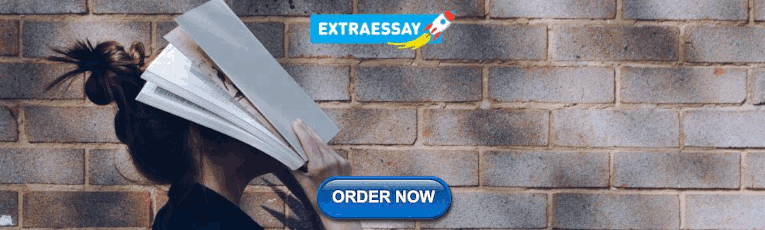
COMMENTS
The roles of air pollution and climate change in individual-level patient care are increasingly recognized. While air pollution has contributed to morbidity and mortality before these cases, the past 2 years brought the first cases in which individual deaths were officially linked with air pollution: in the death of a 9-year-old girl who suffered from asthma in the United Kingdom in whom ...
The study found that DALYs related to noise were more important than those related to air pollution, as the effects of environmental noise on cardiovascular disease were independent of air pollution . ... Case Study 2; Comparison of the EU and US Air Quality Standards and Planning Requirements. (2004). Available online at: ...
Most air pollution research has focused on assessing the urban landscape effects of pollutants in megacities, little is known about their associations in small- to mid-sized cities. Considering ...
Air pollution-related deaths account for around 17% of all ... So the propensity scores are treated as known in our study. In addition, since air pollution has rarely been considered an important ... (over 80,000 data points in our case), the air stagnation analysis leads to reliable and interpretable estimates of the associations between air ...
Air pollution contributes to the global burden of disease, with ambient exposure to fine particulate matter of diameters smaller than 2.5 μm (PM2.5) being identified as the fifth-ranking risk ...
This study assesses Delhi's air pollution scenario in the winter of 2021 and the actions to tackle it. Winter 2021 was unlike previous winters as the control measures mandated by the Commission of Air Quality Management (CAQM) in Delhi National Capital Region and adjoining areas were rolled out. These measures included the Graded Response ...
An analysis of different sources of air pollution in Kolkata has revealed that motor vehicles are the leading contributor to air pollution (51.4%) which is followed by industry (24.5%) and dust particles (21.1%), respectively ( Table 1) [ 48 ]. Table 1. Sources of air pollution emissions in Kolkata.
Air pollution control in the USA is focused on compliance with emission limits and meeting air quality standards. Over recent decades, US air quality regulations have greatly reduced emissions ...
Recognizing the value of SEI York's work integrating air pollution and climate change mitigation, the University of York's Environment and Geography Department included it as an impact case study in its REF2021 submission. Read about this work, which helped the department become ranked as one of the best of its kind for research impact.
A pictorial representation of the article, representing a case study reported in South Africa for 03 July 2021, a major reported case and to understand the influences of atmospheric weather (Temperature, Relative Humidity, Precipitation rate and vertical velocity) including the various major pollutants (particulate matters: PM 2.5 & PM 10, SO 2, NO 2 and surface ozone) changes.
A 2021 report estimated that air pollution costs the Philippines approximately US$87 billion annually due to health-care and welfare costs and labour and economic productivity loss. Even after three decades since the passage of the country's Clean Air Act, the Philippines is still nowhere near achieving comprehensive air pollution control.
Numerous epidemiologic studies have investigated the respiratory effects of air pollution on children, including lung function and respiratory symptoms/diseases. The systematic reviews and meta-analyses on ambient air pollution and children's respiratory health examined here are shown in Table 1 [ 8 - 21 ].
The current study presents a method for assessing individual-level exposure to traffic-related air pollution by integrating mobility patterns tracked by global positioning system (GPS) devices with dynamics of air pollutant concentrations. The study is based on a pool of 17 pregnant women residing in Hidalgo County, Texas.
The high burden of death and disease due to air pollution and its associated substantial adverse economic impact from loss of output could impede India's aspiration to be a $5 trillion economy by 2024. Successful reduction of air pollution in India through state-specific strategies would lead to substantial benefits for both the health of the population and the economy.
January 4, 2024 - The Harvard Six Cities Study, which had a profound impact on efforts to curb air pollution in the U.S., turned 30 in December. The study found that fine particulate pollution was linked with mortality at much lower levels than previously thought.. A December 29 article in The Guardian quoted Douglas Dockery, John L. Loeb and Frances Lehman Loeb Professor of Environmental ...
Air pollution affects many people in developed and developing countries worldwide. It is costing around 2% and 5% of GDP (gross domestic product) in developed and developing countries, respectively. The air qualities have been deteriorating day by day and now the situation has become worst. An increase in air pollution will worsen the environment and human health status. Hence, there is an ...
This article describes the impact of limiting human mobility related to the COVID-19 pandemic on the level of air pollution related to transport. The city of Krakow and the emission level of nitrogen oxides (NO x ) were used as a case study. This article describes the air quality monitoring system in Krakow and the measurement results from the measurement station at Krasinskiego Avenue. The ...
AIR POLLUTION TRAGEDY: A CASE STUDY. Lesson Concepts: Students will build awareness that many disciplines of study and areas of interest can contribute to solving a public problem. Students will build awareness of past air pollution disasters by reviewing historic accounts. Learning Objective — Students will be able to:
The analysis of chemical elements in the barks of trees is an alternative procedure to access spatial heterogeneity of traffic related air pollution. However, the role of tree species in the characterization of the variability of airborne pollution is poorly known. We present an observational study conducted in São Paulo, Brazil, based on the analysis of 498 trees from three common species ...
1. Introduction. Air pollution can be defined as the presence in the air of substances harmful to humans and is associated with a high risk for premature deaths due to cardio-vascular diseases (e.g., ischaemic heart disease and strokes), chronic obstructive pulmonary disease, asthma, lower respiratory infections and lung cancer [1,2].People living in developing and overpopulated countries ...
The survival of mankind cannot be imagined without air. Consistent developments in almost all realms of modern human society affected the health of the air adversely. Daily industrial, transport, and domestic activities are stirring hazardous pollutants in our environment. Monitoring and predicting air quality have become essentially important in this era, especially in developing countries ...
Objective To identify risk factors (air pollution and family related) for the onset of asthma and persistent wheezing in children. Design Nationwide case-control study. Setting Denmark. Participants All Danish children born from 1997 to 2014 and followed for asthma onset and persistent wheezing from age 1 year to 15 years. Main outcome measure Onset of asthma and persistent wheezing. Results A ...
The sulphur limits are 1% for heavy fuel oil and 0.2% for gas oil and marine gas oil (dropping to 0.1% in 2008). Volatile Organic Compounds (VOCs) have a direct effect on human health and the ...