REVIEW article
The impact of cognitive biases on professionals’ decision-making: a review of four occupational areas.
- 1 Université de Lorraine, 2LPN, Nancy, France
- 2 Psychology and Neuroscience Lab, Centre d’Économie de la Sorbonne, Université de Lorraine, CNRS UMR 8174, Paris, France
The author reviewed the research on the impact of cognitive biases on professionals’ decision-making in four occupational areas (management, finance, medicine, and law). Two main findings emerged. First, the literature reviewed shows that a dozen of cognitive biases has an impact on professionals’ decisions in these four areas, overconfidence being the most recurrent bias. Second, the level of evidence supporting the claim that cognitive biases impact professional decision-making differs across the areas covered. Research in finance relied primarily upon secondary data while research in medicine and law relied mainly upon primary data from vignette studies (both levels of evidence are found in management). Two research gaps are highlighted. The first one is a potential lack of ecological validity of the findings from vignette studies, which are numerous. The second is the neglect of individual differences in cognitive biases, which might lead to the false idea that all professionals are susceptible to biases, to the same extent. To address that issue, we suggest that reliable, specific measures of cognitive biases need to be improved or developed.
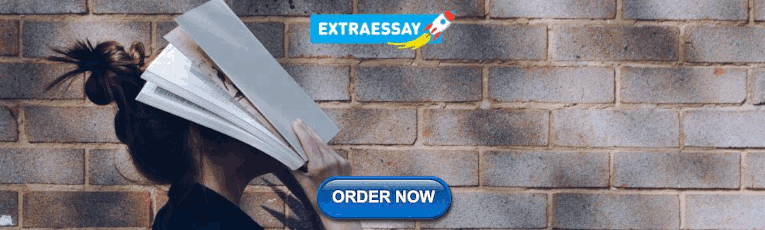
Introduction
When making judgments or decisions, people often rely on simplified information processing strategies called heuristics, which may result in systematic, predictable errors called cognitive biases (hereafter CB). For instance, people tend to overestimate the accuracy of their judgments (overconfidence bias), to perceive events as being more predictable once they have occurred (hindsight bias), or to seek and interpret evidence in ways that are partial to existing beliefs and expectations (confirmation bias). In fact, the seminal work of Kahneman and Tversky on judgment and decision-making in the 1970s opened up a vast research program on how decision-making deviates from normative standards (e.g., Tversky and Kahneman, 1974 ; Kahneman et al., 1982 ; Gilovich et al., 2002 ).
The “heuristics and biases” program has been remarkably fruitful, leading to unveiling dozens of CB and heuristics in decision-making (e.g., Baron, 2008 , listed 53 such biases). While this research turned out to have a large impact in the academic field and beyond ( Kahneman, 2011 ), it is worth noting that it led to some debate ( Vranas, 2000 ; Pohl, 2017 ). In particular, Gigerenzer (1991 , 1996) ( Gigerenzer et al., 2008 ) outlined that Kahneman and Tversky relied upon a narrow view of normative rules (probability theory), leading them to ask participants to make artificial judgments (e.g., estimating the probability of single events) likely to result in so-called “errors.” Gigerenzer also pointed out the overemphasis on decision errors and the lack of theory behind the heuristics-and-biases approach, which eventually results in a list of cognitive errors with no theoretical framework. However, there have been several attempts to overcome this shortcoming, such as the reframing of the heuristics-and-biases literature in terms of the concept of attribute substitution ( Kahneman and Frederick, 2002 ) and the various taxonomies of CB advanced based on dual-process models (e.g., Stanovich et al., 2008 ).
While early research on CB was conducted on lay participants to investigate decision-making in general, there has been a large interest in how such biases may impede professional decision-making in areas, such as management (e.g., Maule and Hodgkinson, 2002 ), finance (e.g., Baker and Nofsinger, 2002 ), medicine (e.g., Blumenthal-Barby and Krieger, 2015 ), and law (e.g., Rachlinski, 2018 ). Consider, for example, the framing effect, when making risky decisions, people prefer sure gains over more risky ones, whereas they prefer risky losses over sure ones ( Kahneman and Tversky, 1979 ). Therefore, framing a problem in terms of gains versus losses can significantly impact decision-making. In most lawsuits for instance, plaintiffs choose between a sure gain (the settlement payment) and a potential larger gain (in the case of further litigation) while defendants choose between a sure loss (the settlement payment) and a potential larger loss (in the case of further litigation). In fact, when considering whether the parties should settle the case, judges evaluating the case from the plaintiff’s perspective are more likely to recommend settlement than those evaluating the case from the defendant’s perspective ( Guthrie et al., 2001 ). Likewise, when asking to rate the effectiveness of a drug, presenting the results of a hypothetical clinical trial in terms of absolute survival (gain), absolute mortality (loss), or relative mortality reduction (gain) influences the ratings of doctors ( Perneger and Agoritsas, 2011 ).
For the sake of convenience, we list below the common definition of the main CB considered in this review.
Anchoring Bias is the tendency to adjust our judgments (especially numerical judgments) toward the first piece of information ( Tversky and Kahneman, 1974 ).
Availability bias is the tendency by which a person evaluates the probability of events by the ease with which relevant instances come to mind ( Tversky and Kahneman, 1973 ).
Confirmation bias is the tendency to search for, to interpret, to favor, and to recall information that confirms or supports one’s prior personal beliefs ( Nickerson, 1998 ).
Disposition effect is the tendency among investors to sell stock market winners too soon and hold on to losers too long ( Shefrin and Statman, 1985 ). This tendency is typically related to loss aversion ( Kahneman and Tversky, 1979 ).
Hindsight bias is a propensity to perceive events as being more predictable, once they have occurred ( Fischhoff, 1975 ).
Omission bias is the preference for harm caused by omissions over equal or lesser harm caused by acts ( Baron and Ritov, 2004 ).
Outcome bias is the tendency to judge the quality of a decision based on the information about the outcome of that decision. These judgments are erroneous in respect to normative assumption that “information that is available only after decision is made is irrelevant to the quality of the decision” ( Baron and Hershey, 1988 , p. 569).
Overconfidence bias is a common inclination of people to overestimate their own abilities to successfully perform a particular task ( Brenner et al., 1996 ).
Relative risk bias is a stronger inclination to choose a particular treatment when presented with the relative risk than when presented with the same information described in terms of the absolute risk ( Forrow et al., 1992 ).
Susceptibility to framing is the tendency for people to react differently to a single choice depending on whether it is presented as a loss or a gain ( Tversky and Kahneman, 1981 ).
In the present paper, we review the research on the impact of CB on professional decision-making in four areas: management, finance, medicine, and law. Those applied areas were selected as they have led to the highest number of publications on this topic so far (see “Materials and Methods”). This study aims to address the following research questions:
1. Assess the claim that CB impact professionals’ decision-making
2. Assess the level of evidence reported in the empirical studies
3. Identify the research gaps
We take a narrative approach to synthesizing the key publications and representative empirical studies to answer these research questions. To the best of our knowledge, this study is the first literature review on this research topic covering multiple areas together. This review is narrative, as opposed to a systematic review, which is one of its limitations. However, it aims to be useful both to researchers and professionals working in the areas covered.
The present paper is structured as follows. The Methods section provides details about the methodology used to conduct the literature review. In the following sections, we review the key findings in each of the four occupational areas covered. Finally, in the Discussion section, we answer the three research questions addressed in light of the findings reviewed.
Materials and Methods
We conducted a systematic literature search using the Web of Science (WoS) database with the search terms “cognitive biases AND decision making.” The search criteria included research articles, review articles, or book chapters with no restriction regarding the time period. We focused on the WoS database as the “Web of Science Categories” filter would offer a practical mean to select the applied areas covered. Admittedly, the results of our review might have been different had we covered more databases; however, as our strategy was to review the key publications and representative empirical studies in each of the areas selected, we reasoned that virtually every database would have led to these records.
The PRISMA flowchart in Figure 1 illustrates the process of article search and selection in this study. The WoS search led to a total of 3,169 records. Before screening, we used the “Web of Science Categories” filter to identify and select the four applied areas with the highest number of publications. Those areas were management ( n = 436), which merged the categories “Management” ( n = 260) and “Business” ( n = 176); medicine ( n = 517), which merged the categories “Psychiatry” ( n = 261), “Health Care Sciences Services” ( n = 112), “Medicine General Internal” ( n = 94), “Radiology Nuclear Medicine Medical Imaging” ( n = 22), “Critical Care Medicine” ( n = 14), and “Emergency Medicine” ( n = 14); and law ( n = 110) and finance ( n = 70). Noteworthy, while the category “Psychology Applied” was associated with a significant number of publications ( n = 146), a closer examination revealed that the majority of them was related to other applied areas (e.g., management, medicine, law, and ergonomics). Accordingly, this category was not included in the review. The abstracts selected were reviewed according to two inclusion criteria: (1) the article had a clear focus on cognitive biases and decision-making (e.g., not on implicit biases); (2) the article reported a review (narrative or systematic) on the topic or a representative empirical study. This screening led to a selection of 79 eligible articles, which were all included in the review.
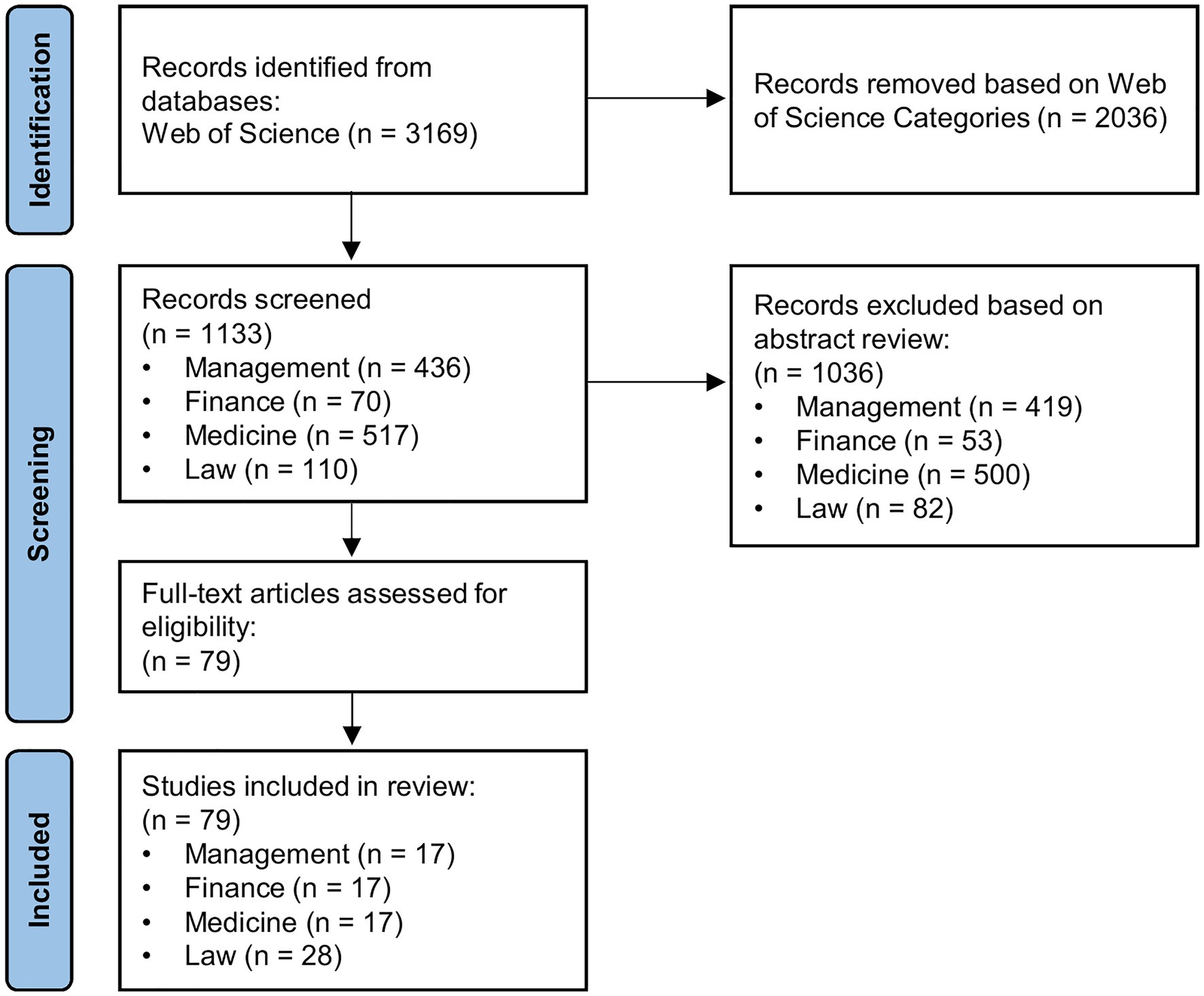
Figure 1 . PRISMA flowchart of article search and collection.
The life of any organization is made of crucial decisions. According to Eisenhardt and Zbaracki (1992 , p. 17), strategic decisions are “those infrequent decisions made by the top leaders of an organization that critically affect organizational health and survival.” For instance, when Disney decided to locate Euro Disney in Paris or when Quaker decided to acquire Snapple, these companies took strategic decisions.
A defining feature of strategic decisions is their lack of structure. While other areas of management deal with recurring, routinized, and operationally specific decisions, strategic issues and problems tend to be relatively ambiguous, complex, and surrounded by risk and uncertainty ( Hodgkinson, 2001 ). How do managers actually deal with such decisions? Much of early research on strategic decision-making was based on a neoclassical framework with the idea that strategists in organizations are rational actors. However, the seminal work of Kahneman and Tversky in the 1970s questioned this assumption ( Hardman and Harries, 2002 ). In fact, the very notion of “bounded rationality” emerged in the study of organizations ( March and Simon, 1958 ). One might argue that the issue of individual biases in strategic decision-making is of limited relevance as strategic decisions are the product of organizations rather than individuals within the context of a wider sociopolitical arena ( Mintzberg, 1983 ; Johnson, 1987 ). However, individual (micro) factors might help explain organizational (macro) phenomena, an idea promoted by behavioral strategy ( Powell et al., 2011 ).
The “heuristics and biases” program revived the interest for bounded rationality in management with the idea that decision-makers may use heuristics to cope with complex and uncertain environments, which in turn may result in inappropriate or suboptimal decisions (e.g., Barnes, 1984 ; Bazerman, 1998 ). Indeed, it is relatively easy to see how biases, such as availability, hindsight, or overconfidence, might play out in the strategic decision-making process. For instance, it may seem difficult in hindsight to understand why IBM and Kodak failed to see the potential that Haloid saw (which led to the Xerox company). The hindsight bias can actually lead managers to distort their evaluations of initial decisions and their predictions ( Bukszar and Connolly, 1988 ). Likewise, practicing auditors of major accounting firms are sensitive to anchoring effects ( Joyce and Biddle, 1981 ) and prospective entrepreneurs tend to neglect base rates for business failures ( Moore et al., 2007 ).
To our knowledge, no systematic review of empirical research on the impact of heuristics and CB on strategic decision-making has been published to date. Whereas the idea that CB could affect strategic decisions is widely recognized, the corresponding empirical evidence is quite weak. Most research on this topic consists in narrative papers relying upon documentary sources and anecdotal evidence (e.g., Duhaime and Schwenk, 1985 ; Lyles and Thomas, 1988 ; Huff and Schwenk, 1990 ; Zajac and Bazerman, 1991 ; Bazerman and Moore, 2008 ). In fact, the typical paper describes a few CB and provides for each one examples of how a particular bias can lead to poor strategic decisions (see Barnes, 1984 , for a representative example). While the examples provided are often compelling, such research faces severe methodological limitations.
The work of Schwenk (1984 , 1985) is representative of that type of research. This author identified three different stages of the strategic decision process (goal formulation and problem identification, strategic alternatives generation, evaluation of alternatives, and selection of the best one) and a set of heuristics and biases that might affect decisions at each stage. Schwenk also provided for each bias an illustrative example of how the bias may impede the overall quality of strategic decisions. For example, the representativeness heuristics may affect the stage of evaluation and selection of the alternatives. To illustrate this, Schwenk mentioned the head of an American retail organization (Montgomery Ward) who held a strong belief that there would be a depression at the end of the Second World War as was the case after World War I. Based on this belief, this executive decided not to allow his company to expand to meet competition from his rival (Sears), which led to a permanent loss of market share to Sears. Schwenk (1988) listed ten heuristics and biases of potential key significance in the context of strategic decision-making (availability, selective perception, illusory correlation, conservatism, law of small numbers, regression bias, wishful thinking, illusion of control, logical reconstruction, and hindsight bias).
In a similar vein, Das and Teng (1999) proposed a framework to explore the presence of four basic types of CB (prior hypotheses and focusing on limited targets, exposure to limited alternatives, insensitivity to outcome probabilities, and illusion of manageability) under five different modes of decision-making (rational, avoidance, logical incrementalist, political, and garbage can). They proposed that not all basic types of biases are robust across all kinds of decision processes; rather, their selective presence is contingent upon the specific processes that decision makers engage in. For instance, the garbage can mode ( Cohen et al., 1972 ) depicts decision-making processes as organized anarchies, in which a decision is largely dependent on chance and timing. In this kind of process, decision makers do not know their objectives ex ante , but merely look around for decisions to make. Das and Teng (1999) hypothesized that managers under the garbage can mode will be exposed to limited alternatives and insensitive to outcome probabilities. On the contrary, managers under the rational mode would be exposed to prior hypotheses and illusion of manageability. This framework, however, is not supported by rigorous empirical evidence.
It is not difficult to list examples of poor strategic decisions that can be readily interpreted – in hindsight – as the result of heuristics and biases. However, the claim that CB influence strategic decisions requires to be tested more directly through laboratory research and experimental studies ( Maule and Hodgkinson, 2002 ). It is worth noting that such research is scarce, probably because of its lack of ecological validity, an issue of primary importance in the field of management research ( Schwenk, 1982 ). Still, two CB in particular have been studied quantitatively, the framing effect and CEO overconfidence.
Hodgkinson et al. (1999) used an experimental setting to investigate the effect of framing on strategic decisions. Following the “Asian Disease” problem ( Tversky and Kahneman, 1981 ), they presented subjects (undergraduate management students) with a 500-word case vignette giving a brief history of a company that manufactured and distributed fast paint-drying systems. A positive and a negative frame were used and participants were asked to adopt the role of a board member facing a major strategic decision and to indicate which of two alternative options they would choose. The positive frame emphasized gains from a reference point of no profit, whereas the negative frame highlighted losses from a reference point where the target profit is achieved (£3 million). In addition, participants were either asked to choose between the presented options directly or to represent the ways in which they thought about the problem in the form of a causal map prior to making their choice. It turned out that when participants made their decisions directly, a massive framing effect was found (45.5% of participants chose the risk-averse option in the positive frame versus 9% in the negative frame). However, no framing effect was observed when participants were asked to draw a causal map before making their choice (36.4% of the participants opted for the risk-averse option in both versions). Interestingly, Hodgkinson et al. reported the same findings on experienced participants (senior managers in a banking organization).
Another CB that led to a large amount of empirical research in strategic management is CEO overconfidence. Overconfidence has various aspects: overprecision, overestimation, and overplacement ( Moore and Schatz, 2017 ). Regarding overprecision, Ben-David et al. (2013) investigated the accuracy of stock market predictions made by senior finance executives (the majority of them being CFOs). The data were collected in 40 quarterly surveys conducted between June 2001 and March 2011. Ben-David et al. asked participants to predict one- and 10-year market-wide stock returns and to provide an 80% confidence interval for their predictions (“Over the next year, I expect the annual SandP 500 return will be: There is a 1-in-10 chance the actual return will be less than ___%; I expect the return to be: ___%; There is a 1-in-10 chance the actual return will be greater than ___%.”). It turned out that the CFOs were severely miscalibrated as: the realized one-year SandP 500 returns fall within their 80% confidence intervals only 36.3% of the time. Even during the least volatile quarters in the sample, only 59% of realized returns fall within the 80% confidence intervals provided. The comparison of the size of the CFOs’ confidence intervals to the distribution of historical one-year returns revealed that their confidence intervals were too narrow. Indeed, CFOs provide an average confidence interval of 14.5%, whereas the difference between the 10th and 90th return percentiles from the realized distribution of the one-year SandP 500 returns is 42.2% (only 3.4% of CFOs provided confidence intervals wider than 42.2%).
Managers also overestimate their abilities, particularly with regard to the illusion of control. In their review on risk perception among managers, March and Shapira (1987) reported that most managers (1) consider that they take risks wisely and that they are less risk-averse than their colleagues, (2) perceive risk as largely controllable, and (3) attribute this controllability to skills and information.
Finally, executives also appear to be overconfident with regard to overplacement. Malmendier and Tate (2005) assessed CEO overconfidence through revealed preferences, examining how they exercised their options. A CEO persistently exercising options later than suggested by the benchmark reveals his belief in his ability to keep the company’s stock price rising and that he or she wants to profit from expected price increases by holding the options. Using panel data on personal portfolio and corporate investment decisions of Forbes 500 CEOs, Malmendier and Tate reported that most of CEO excessively hold company stock options, thereby failing to reduce their personal exposure to company-specific risk. CEO overconfidence is also believed to be involved in merger decisions. As overconfident CEOs overestimate their ability to generate returns, they are supposed to overpay for target companies and undertake value-destroying mergers. Using two measures of CEO overconfidence (CEOs’ personal over-investment in their company and their press portrayal), Malmendier and Tate (2008) provided support for that hypothesis: the odds of making an acquisition are 65% higher if the CEO is classified as overconfident.
The case of CB in finance is special. In the 1980s, CB were invoked to account for observations on markets in disagreement with the predictions of standard finance. This paradigm relies upon expected utility theory, assuming that investors make rational decisions under uncertainty (i.e., maximizing utility). Standard finance produced core theoretical concepts, such as arbitrage, portfolio theory, capital asset pricing theory, and efficient market hypothesis, all assuming rational investors. In the 1970s, some observations on financial markets relative to trading behavior, volatility, market returns, and portfolio selection turned out to be inconsistent with the framework of standard finance (“anomalies”). Psychological biases (micro level) were invoked as theoretical explanations of these market anomalies (macro level), launching the field of behavioral finance ( Shiller, 2003 ). In particular, behavioral finance capitalized on prospect theory ( Kahneman and Tversky, 1979 ), a more realistic view of decision-making under uncertainty that expected utility theory. A prime example is how (myopic) loss aversion – a key concept of prospect theory – can account for the equity premium puzzle (i.e., the excessively high difference between equity returns and the return of Treasury bills; Benartzi and Thaler, 1995 ).
Here, we focus on investment decision-making in individual investors ( Shefrin, 2000 ; Baker and Nofsinger, 2010 ; Baker and Ricciardi, 2014 ) and how CB may impede such decisions (see Baker and Nofsinger, 2002 , and Kumar and Goyal, 2015 , for reviews). 1 In fact, financial economists have distinguished between two types of investors in the market, arbitrageurs and noise traders. While the latter is assumed to be fully rational, noise traders are investors prone to CB ( De Long et al.,1990 ), which results in under-diversified portfolios. Various CB have been invoked to account for poor individual investment decisions, resulting in suboptimal portfolio management. For example, investors tend to favor stocks that performed well during the past 3–5 years (“winners”) over stocks that performed poorly (“losers”), neglecting that because of regression to the mean, the losers will tend to outperform the winners over the next years (actually by 30%; De Bondt and Thaler, 1985 ). Investors may exhibit a home bias (an instance of familiarity bias), a tendency to invest the majority of their portfolio in domestic equities rather than diversifying it into foreign equities ( Coval and Moskowitz, 1999 ). Investors may also fall prey to herding, a tendency to follow blindly what other investors do ( Grinblatt et al., 1995 ).
Two CB have been particularly studied in investment decision-making: overconfidence and disposition effect (see the systematic review of Kumar and Goyal, 2015 ). On the one hand, investors are usually overconfident with regard to the precision of their forecasts. When asked to predict the future return or price of a stock, investors report confidence intervals that are too narrow compared to the actual variability of prices (e.g., De Bondt, 1998 ). Investors also overestimate their ability to beat the market. Baker and Nofsinger (2002) reported a finding of a Gallup survey in 2001 revealing that on average, investors estimated that the stock market return during the next 12 months would be 10.3% while estimating that their portfolio return would be 11.7%. Barber and Odean (2001) reported evidence that overconfidence in investors is related to gender. Based on a sample of 35,000 individual accounts over a six-year period, their findings showed that males exhibit more overconfidence regarding their investing abilities and also trade more often than females. Overconfidence in investors makes them more prone to take high risks ( Chuang and Lee, 2006 ) and trade too much ( Odean, 1999 ; Statman et al., 2006 ; Glaser and Weber, 2007 ), which results in poor financial performance (consequent transaction costs and losses). For instance, trading turnover and portfolio returns are negatively correlated: of 66,465 households with accounts at a large discount broker during 1991–1996, households that trade most had an annual return of 11.4% while the average annual return was 16.4% ( Barber and Odean, 2000 ).
On the other hand, the disposition effect is the tendency by which investors tend to sell winning stocks too early while holding on to losing positions for too long ( Shefrin and Statman, 1985 ). Based on trading records for 10,000 accounts at a large discount brokerage house, Odean (1998) reported that on average, winning investments are 50% more likely to be sold than losing investment (similar results were obtained in other countries, such as France; Boolell-Gunesh et al., 2009 ). The disposition effect originates in loss aversion described by prospect theory ( Kahneman and Tversky, 1979 ).
The idea that cognitive failures are a primary source of medical errors has become prevalent in the medical literature (e.g., Detmer et al., 1978 ; Dawson and Arkes, 1987 ; Schmitt and Elstein, 1988 ; Elstein, 1999 ; Croskerry, 2003 ; Klein, 2005 ). In fact, emergency medicine has been described as a “natural laboratory of error” ( Bogner, 1994 ). Among medical errors, diagnostic errors have received particular attention ( Graber, 2013 ). Indeed, there is increasing evidence that mental shortcuts during information processing contribute to diagnostic errors (e.g., Schnapp et al., 2018 ).
It is not difficult to see how CB may impact medical decisions. Blumenthal-Barby and Krieger (2015) provided the following examples. A parent might refuse to vaccinate her child after she sees a media report of a child who developed autism after being vaccinated (availability bias). A patient with atrial fibrillation might refuse to take warfarin because she is concerned about causing a hemorrhagic stroke despite greater risk of having an ischemic stroke if she does not take warfarin (omission bias). Indeed, early papers on this topic were primarily narrative reviews suggesting a possible impact of CB on medical decision-making. These papers follow the same logic: they first provide a general description of a couple of CB and then describe how these shortcuts can lead physicians to make poor decisions, such as wrong diagnoses (e.g., Dawson and Arkes, 1987 ; Elstein, 1999 ; Redelmeier, 2005 ). But narrative reviews provide limited evidence. As Zwaan et al. (2017 , p.105) outlined, “While these papers make a formidable argument that the biases described in the literature might cause a diagnostic error, empirical evidence that any of these biases actually causes diagnostic errors is sparse.”
On the other hand, studies that investigated the actual impact of CB on medical decisions are mainly experimental studies using written cases (hypothetical vignettes) designed to elicit a particular bias. A typical example of vignette study is that of Mamede et al. (2010) on the effect of availability bias on diagnostic accuracy. In a first phase, participants (first-year and second-year internal medicine residents) were provided with 6 different cases and they were asked to rate the likelihood that the indicated diagnosis was correct (all cases were based on real patients with a confirmed diagnosis). Then, participants were asked to diagnose 8 new cases as quickly as possible, that is, relying on non-analytical reasoning. Half of those new cases were similar to the cases encountered in phase 1, so that the availability bias was expected to reduce diagnostic accuracy for those four cases. Second-year residents had actually lower diagnostic accuracy on cases similar to those encountered in phase 1 as compared to other cases, as they provided the phase 1 diagnosis more frequently for phase 2 cases they had previously encountered than for those they had not.
While vignette-based studies are the most frequent, researchers in this area have used diverse strategies ( Blumenthal-Barby and Krieger, 2015 ). For instance, Crowley et al. (2013) developed a computer-based method to detect heuristics and biases in diagnostic reasoning as pathologists examine virtual slide cases. Each heuristic or bias is defined as a particular sequence of hypothesis, findings, and diagnosis formulation in the diagnostic reasoning interface (e.g., availability bias is considered to occur if in a sequence of three cases where the third case has a different diagnosis than the two previous ones, the participant makes an incorrect diagnosis in the third case such that the diagnosis is identical to the correct diagnosis in the two immediately preceding cases). Such a procedure allows for examining the relationships between heuristics and biases, and diagnostic errors.
Another methodology consists in reviewing instances where errors occurred, to which CB presumably contributed (e.g., Graber et al., 2005 ). However, studies following this methodology are vulnerable to hindsight bias: since reviewers are aware that an error was committed, they are prone to identify biases ex post ( Wears and Nemeth, 2007 ). The fact that bias can be in the eye of the beholder has been supported by Zwaan et al. (2017) who asked 37 physicians to read eight cases and list which CB were present from a list provided. In half the cases, the outcome implied a correct diagnosis; in the other half, it implied an incorrect diagnosis. Physicians identified more biases when the case outcome implied an incorrect diagnosis (3.45 on average) than when it implied a correct one (1.75 on average).
To date, two systematic reviews have been published on the impact of CB on medical decision-making. Reviewing a total of 213 studies, Blumenthal-Barby and Krieger (2015) reported the following findings: (1) 77% of the studies ( N = 164) were based on hypothetical vignettes; (2) 34% of studies ( N = 73) investigated medical personnel; (3) 82% of the studies ( N = 175) were conducted with representative populations; (4) 68% of the studies ( N = 145 studies) confirmed a bias or heuristic in the study population; (5) the most studied CB are loss/gain framing bias (72 studies, 24.08%), omission bias (18 studies, 6.02%), relative risk bias (29 studies, 9.70%), and availability bias (22 studies, 7.36%); (6) the results regarding loss/gain framing bias are mixed with 39% of studies ( N = 28) confirming an effect, 39% ( N = 28) confirming an effect only in a subpopulation, and 22% ( N = 16) disconfirming any effect; (7) 25 of 29 studies (86%) supported the impact of relative risk bias on medical decisions; and (8) 14 of 18 studies (78%) supported the impact of omission bias on medical decisions.
Saposnik et al. (2016) conducted a similar review but including only 20 studies. These authors reported that as: (1) 60% of the studies ( N = 12) targeted CB in diagnostic tasks; (2) framing effect ( N = 5) and overconfidence ( N = 5) were the most common CB while tolerance to risk or ambiguity was the most commonly studied personality trait ( N = 5); and (3) given that the large majority of the studies (85%) targeted only one or two biases, the true prevalence of CB influencing medical decisions remains unknown. Moreover, there was a wide variability in the reported prevalence of CB. For example, when analyzing the three most comprehensive studies that accounted for several CB ( Ogdie et al., 2012 ; Stiegler and Ruskin, 2012 ; Crowley et al., 2013 ), it turned out that the availability bias ranged from 7.8 to 75.6% and anchoring bias from 5.9 to 87.8%; (4) the presence of CB was associated with diagnostic inaccuracies in 36.5 to 77% of case-scenarios. Physicians’ overconfidence, anchoring effect, and information or availability bias may be associated with diagnostic inaccuracies; (5) only seven studies (35%) provided information to evaluate the association between physicians’ CB and therapeutic or management errors. Five of these studies (71.4%) showed an association between CB (anchoring, information bias, overconfidence, premature closure, representativeness, and confirmation bias) and therapeutic or management errors.
Based on the legal realism’ premise that “judges are human,” the recent years have seen a growing interest for judicial decision-making (e.g., Klein and Mitchell, 2010 ; Dhami and Belton, 2017 ; Rachlinski, 2018 ). This topic covers issues, such as cognitive models of judicial decision-making (e.g., the story model), the impact of extralegal factors on decisions, prejudice (e.g., gender bias and racial bias), moral judgments, group decision-making, or the comparison of lay and professional judges. It is worth noting that most research on judicial decision-making has focused on how jurors decide cases, relying on jury simulations ( MacCoun, 1989 ). Here, we focus on how professional judges might be prone to CB. One might easily consider how CB could hamper judicial decisions. In a narrative fashion, Peer and Gamliel (2013) reviewed how such biases could intervene during the hearing process (confirmation bias and hindsight bias), ruling (inability to ignore inadmissible evidence), and sentencing (anchoring effects). In fact, research suggests that judges, prosecutors, and other professionals in the legal field might rely on heuristics to produce their decisions, which leaves room for CB (e.g., Guthrie et al., 2007 ; Helm et al., 2016 ; Rachlinski and Wistrich, 2017 ). 2
Researchers investigating judges’ decision-making have mainly relied upon archival studies (document analyses of court records) and experimental studies in which judges are asked to decide on hypothetical cases. In archival studies, researchers examine if judges’ decisions in actual cases exhibit features of irrationality. For instance, Ebbesen and Konecni (1975) investigated which information felony court judges considered when deciding the amount of bail to set. When presented with fictitious cases, the judges’ decisions were influenced by relevant information, such as prior criminal record, but their actual bail decisions relied almost exclusively on prosecutorial recommendations. That is, judges seem to be (too) heavily affected by prosecutors’ recommendations. Another example of archival study is the infamous research of Danziger et al. (2011) who highlighted a cycle in repeated judicial rulings: judges are initially lenient, then progressively rule more in favor of the status quo over time, and become lenient again after a food break. This would suggest that psychological factors, such as mental fatigue, could influence legal decisions (but see Weinshall-Margel and Shapard, 2011 ). Archival studies, however, are limited by the difficulty to control for unobserved variables.
On the other hand, vignette studies consist in presenting judges with hypothetical scenarios simulating real legal cases. As in the medical field, researchers have primarily relied on such studies. A representative study is that of Guthrie et al. (2001) who administered a survey to 167 federal magistrate judges in order to assess the impact of five CB (anchoring, framing, hindsight bias, inverse fallacy, and egocentric bias) on their decisions regarding litigation problems (see Guthrie et al., 2002 , for a summary of the research). Using materials adapting classic cognitive problems into legal ones, Guthrie et al. (2001) reported that judges fell prey to these biases but to various extent. For instance, in order to assess whether judges were susceptible to hindsight bias, Guthrie et al. (2001) presented them with a hypothetical case in which the plaintiff appealed the district court’s decision and asked them to indicate which of three possible outcomes of the appeal was most likely to have occurred. Crucially, they also provided them with the actual outcome of the court of appeals. The outcome significantly influenced judges’ assessments: those informed of a particular outcome were more likely to have identified that outcome as the most likely to have occurred.
In particular, numerous studies have investigated the impact of anchoring effects on judicial decisions (see Bystranowski et al., 2021 , for a recent meta-analysis). Judges and jurors are often required to translate qualitative judgments into quantitative decisions ( Hans and Reyna, 2011 ; Rachlinski et al., 2015 ). While their qualitative judgments on matters, such as the severity of the plaintiff’s injury or the appropriate severity of punishment, show a high degree of consistence and predictability ( Wissler et al., 1999 ), a great amount of variability appears (especially for non-economic and punitive damages) when these qualitative judgments are translated into numbers (e.g., civil damage awards and criminal sentences; Hart et al., 1997 ; Diamond et al., 1998 ). This might be explained by the fact that numerical assessments can be prone to anchoring. Facing uncertainty about the amount to determine, judges and especially juries (due to their lack of experience and information about standard practice) tend to rely on any numerical point of reference and make their judgment through adjustments from that number. As these adjustments are often insufficient, the judgments are biased toward the anchor (see Kahneman et al., 1998 , for a model describing how individual jurors set punitive damages and the role of anchoring in that process).
Accordingly, numerical values, such as a damage cap (e.g., Hinsz and Indahl, 1995 ; Robbennolt and Studebaker, 1999 ), the amount of damages claimed by the plaintiff ( Chapman and Bornstein, 1996 ), the amount of economic damage ( Eisenberg et al., 1997 , 2006 ), the sentence imposed in the preceding case, a sentence urged by the prosecutor, or a sentence recommended by a probation officer, might act as anchors in the courtroom, moving the judges’ decisions toward them. Guthrie et al. (2001) reported that in a personal injury suit, an irrelevant factor, such as a number in a pre-trial motion (used to determine whether the damages met the minimum limit for federal court), could act as an anchor. They presented judges with a description of a serious personal injury suit in which only damages were at issue and asked them to estimate how much they would award the plaintiff in compensatory damages. Prior to this estimation, half of the judges were asked to rule on a pre-trial motion filed by the defendant to have the case dismissed for failing to meet the jurisdictional minimum in a diversity suit ($75,000). It turned out that the judges who were asked only to determine the damage award provided an average estimate of $1,249,000 while the judges who first ruled on the motion provided an average estimate of $882,000.
Enough and Mussweiler (2001) conducted a series of research on how recommendations anchor judicial decisions, even when they are misleading. In their 2001 paper, they showed that sentencing decisions tend to follow the sentence demanded by the prosecutor. When told that the prosecutor recommended a sentence of 34 months, criminal trial judges recommended on average 8 months longer in prison ( M = 24.41 months) than when told that the sentence should be 12 months ( M = 17.64) for the same crime. This anchoring effect was independent of the perceived relevance of the sentencing demand, and judges’ experience. Englich et al. (2006) reported that anchoring even occurs when the sentencing demand is determined randomly (the result of a dice throw). Interestingly, Englich et al. (2005) found that the defense’s sentencing recommendation is actually anchored on the prosecutor’s demand, so that the former mediates the impact of the latter on the judge’s decision. Therefore, while it is supposed to be at their advantage, the fact that defense attorneys present their sentencing recommendation after the prosecution might be a hidden disadvantage for the defense.
Along with anchoring, the impact of hindsight bias in the courtroom has been also well documented, mainly in liability cases ( Harley, 2007 ; Oeberst and Goeckenjan, 2016 ). When determining liability or negligence, judges and juries must assess whether the defendant is liable for a negative outcome (damage or injury). The difficulty is that jurors accomplish this task in retrospect: having knowledge of the outcome, jurors tend to perceive it as foreseeable and accordingly rate highly the negligence or liability of the defendant ( Rachlinski et al., 2011 ). To avoid this bias, the law requires jurors to ignore the outcome information while evaluating the extent to which it should have been foreseen by the defendant. However, research suggests that jurors tend to fall prey to hindsight bias as much as lay persons. When evaluating the precautions took by a municipality to protect a riparian property owner from flood damage, participants assessing the situation in foresight concluded that a flood was too unlikely to justify further precautions. However, participants assessing the situation in hindsight considered that such a decision was negligent and also gave higher estimates for the probability of the disaster occurring ( Kamin and Rachlinski, 1995 ).
Outcome information has been shown to affect jurors’ decisions about punitive damage awards ( Hastie et al., 1999 ) and their decisions about the legality of a search ( Casper et al., 1989 ). In addition, more severe outcomes tend to produce a larger hindsight bias, a result particularly stressed in medical malpractice litigation ( LaBine and LaBine, 1996 ). While the assessment of negligence of the accused physician should be based on his course of action regardless of the outcome, jurors are highly influenced by the severity of a negative medical outcome when determining negligence in medical malpractice cases ( Berlin and Hendrix, 1998 ). Cheney et al. (1989) reviewed 1,004 cases of anesthesia-related negligence and reported that the court had imposed liability on the defendant in over 40 percent of the cases, even though the physician acted appropriately.
There is also significant evidence that confirmation bias ( Nickerson, 1998 ) may impact professional judges’ decisions. In the legal field, confirmation bias has been primarily studied with regard to criminal investigations ( Findley and Scott, 2006 ). Once they become convinced that the suspect is guilty, professionals involved in criminal proceedings (e.g., police officers and judges) may engage in guilt-confirming investigation endeavors (or tunnel vision) by which they undermine alternative scenarios in which the suspect is actually innocent. Several studies reported evidence of confirmation bias in criminal cases. For instance, O’Brien (2009) found that participants (College students) who articulated a hypothesis regarding the suspect early in their review of a mock police file showed bias in seeking and interpreting evidence to favor that hypothesis, thereby demonstrating a case-building mentality against a chosen suspect. Similarly, Lidén et al. (2019) showed that judges’ detentions of suspects trigger a confirmation bias that influences their assessment of guilt and that this bias is affected by who decided about detention. In fact, judges perceived the detained defendants’ statements as less trustworthy and were also more likely to convict when they themselves had previously detained the suspect as compared to when a colleague had decided to detain. 3
Table 1 provides a summary of the main CB in the four occupational areas reviewed and the corresponding evidence.
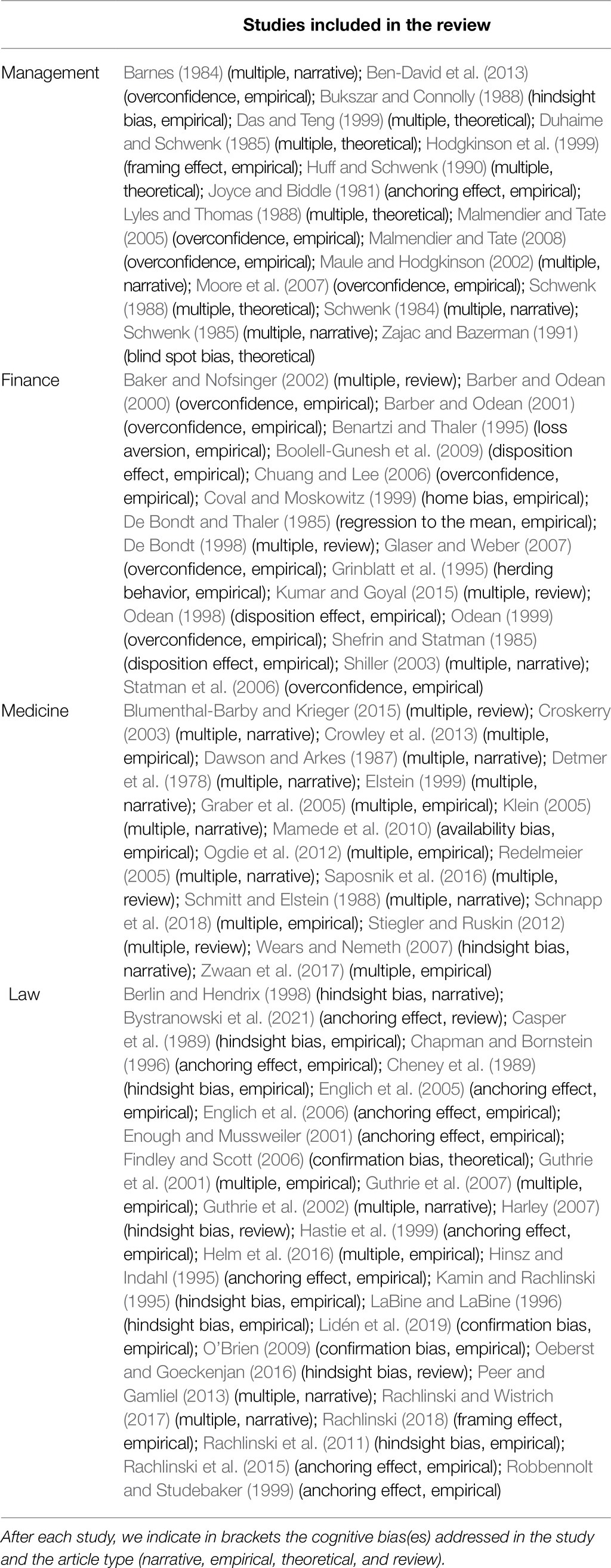
Table 1 . Summary of the main cognitive biases studied in the fields of management, finance, medicine, and law, and corresponding evidence.
The goal of the present paper was to provide an overview of the impact of CB on professional decision-making in various occupational areas (management, finance, medicine, and law). In all of them, there has been tremendous interest in that issue as revealed by a vast amount of research. Our review provided significant answers to the three research questions addressed.
First, the literature reviewed shows that, overall, professionals in the four areas covered are prone to CB. In management, there is evidence that risky-choice (loss/gain) framing effects and overconfidence (among CEOs) impact decision-making. In finance, there is strong evidence that overconfidence and the disposition effect (a consequence of loss aversion) impact individual investors’ decision-making. Regarding medical decision-making, the systematic review of Blumenthal-Barby and Krieger (2015) revealed that (1) 90% of the 213 studies reviewed confirmed a bias or heuristic in the study population or in a subpopulation of the study; (2) there is strong evidence that omission bias, relative risk bias, and availability bias have an impact on medical decisions, and mixed evidence for the risky-choice framing effect. On the other hand, the systematic review of Saposnik et al. (2016) – based on 20 studies only – reported that physicians’ overconfidence, anchoring, and availability bias were associated with diagnostic errors. Finally, the effects of anchoring, hindsight bias, and confirmation bias on judicial decision-making are well documented. Overall, overconfidence appears as the most recurrent CB over the four areas covered.
Second, the level of evidence supporting the claim that CB impact professionals’ decision-making differs across the four areas covered. In medicine and law, this issue has been primarily evidenced in vignettes studies. Such primary data provide a relevant assessment of CB in decision-making but they face the issue of ecological validity (see below). Accordingly, a mid-level of evidence can be assigned to these findings. On the other hand, following the method of revealed preference by which the preferences of individuals are uncovered through the analysis of their choices in real-life settings, the impact of CB on financial decision-making has been evidenced through secondary data (e.g., trading records), indicating a higher level of evidence. In management, both levels of evidence are found (framing effects were demonstrated in vignette studies while CEO overconfidence was evidenced through secondary data).
A practical implication of these findings is the need for professionals to consider concrete, practical ways of mitigating the impact of CB on decision-making. In finance, this issue has been tackled with programs that aimed to improve financial literacy ( Lusardi and Mitchell, 2014 ). In medicine, debiasing has been considered as a way to reduce the effects of CB ( Graber et al., 2002 , 2012 ; Croskerry, 2003 ; Croskerry et al., 2013 ). In fact, recent research has reported evidence that the debiasing of decisions can be effective ( Morewedge et al., 2015 ; Sellier et al., 2019 ). However, a preliminary step to considering practical means of mitigating the impact of CB is to acknowledge this diagnosis. In fact, professionals are reluctant to accept the idea that their decisions may be biased (e.g., Kukucka et al., 2017 ). Judges, for instance, tend to dismiss the evidence showing the impact of CB on judicial decisions, arguing that most studies did not investigate decisions on real cases ( Dhami and Belton, 2017 ).
Thirdly, our review highlights two major research gaps. The first one is a potential lack of ecological validity of the findings from vignette studies, which are numerous ( Blumenthal-Barby and Krieger, 2015 ). Consider for instance a study designed to test whether sentencing decisions could be anchored by certain information, such as the sentence demanded by the prosecutor ( Enough and Mussweiler, 2001 ). A typical study consists in presenting judges with a vignette describing a hypothetical criminal case and asking them to sentence the defendant (e.g., Rachlinski et al., 2015 ). If a statistically significant difference is observed between the different anchor conditions, it is concluded that anchoring impacts judges’ sentencing decisions. Does such a finding mean that judges’ sentencing decisions in real cases are affected by anchoring too? Likewise, it has been reported that 90% of judges solve the Wason task incorrectly ( Rachlinski et al., 2013 ) but this does not imply per se that confirmation bias impedes judges’ decisions in their regular work. Addressing that issue requires to use more ecological settings, such as mock trials in the case of judicial decision-making ( Diamond, 1997 ).
The second research gap is the neglect of individual differences in CB. This limit was found in the four areas covered. Individual differences have been neglected in decision-making research in general ( Stanovich et al., 2011 ; Mohammed and Schwall, 2012 ). Indeed, most of the current knowledge about the impact of CB on decision-making relies upon experimental research and group comparisons ( Gilovich et al., 2002 ). For instance, based on the experimental result described above, one might wrongly infer that all judges are susceptible to anchoring, to the same extent. That is why Guthrie et al. (2007 , p. 28) clarified that “the fact that we generally observed statistically significant differences between the control group judges and experimental group judges does not mean that every judge made intuitive decisions. […] Our results only show that, as a group, the judges were heavily influenced by their intuition – they do not tell us which judges were influenced and by how much.” In fact, there is clear evidence for individual differences in susceptibility to CB (e.g., Bruine de Bruin et al., 2007 ).
The issue of individual differences is of primary importance when considering CB in decision-making, especially among professionals. In finance for example, the measurement of the disposition effect at the individual level revealed significant individual differences, 20% of investors showing no disposition effect or a reverse effect ( Talpsepp, 2011 ). Taking full account of individual differences is crucial when considering public interventions aiming to mitigate individual biases: any single intervention might work on individuals highly susceptible to the bias addressed while having no or even harmful effects on individuals moderately susceptible to it ( Rachlinski, 2006 ).
Addressing the issue of individual differences in bias susceptibility requires having standardized, reliable measures ( Berthet, 2021 ). While reliable measures of a dozen CB are currently available, measures of key biases are still lacking (e.g., confirmation bias and availability bias). Most importantly, these measures are generic, using non-contextualized items. Such measures are relevant for research with the purpose of describing general aspects of decision-making ( Parker and Fischhoff, 2005 ; Bruine de Bruin et al., 2007 ). However, research on individual differences in professional decision-making requires specific measures which items are adapted to the context in which a particular decision is made (e.g., diagnostic decision and sentencing decision). An example is the inventory of cognitive biases in medicine ( Hershberger et al., 1994 ) which aims to measure 10 CB in doctors (e.g., insensitivity to prior probability and insensitivity to sample size) through 22 medical scenarios. The development of such instruments in the context of management, finance, and law is an important avenue for future research on professional decision-making.
Author Contributions
The author confirms being the sole contributor of this work and has approved it for publication.
Conflict of Interest
The author declares that the research was conducted in the absence of any commercial or financial relationships that could be construed as a potential conflict of interest.
Publisher’s Note
All claims expressed in this article are solely those of the authors and do not necessarily represent those of their affiliated organizations, or those of the publisher, the editors and the reviewers. Any product that may be evaluated in this article, or claim that may be made by its manufacturer, is not guaranteed or endorsed by the publisher.
1. ^ It should be noted that most research in behavioral finance has focused on individual rather than professional investors (e.g., mutual funds, hedge funds, pension funds, and investment advisors). Findings suggest that institutional investors are prone to various CB but to a lesser extent than individual investors (e.g., Kaustia et al., 2008 ).
2. ^ Interestingly, the notion of cognitive bias might also shed light on certain rules of law. For example, Guthrie et al. (2001) presented judges with a problem based on the classic English case Byrne v. Boadle (1863) asked them to assess the likelihood that a warehouse was negligent for an accident involving a barrel that injured a bystander. The materials indicated that when the warehouse is careful, accidents occur one time in 1,000, but that when the warehouse is negligent, accidents occur 90% of the time. The materials also indicated that the defendant is negligent only 1% of the time. Judges overestimated the probability that the defendant was negligent, failing to consider the base rate of negligence. Interestingly, this logical fallacy is implemented in the doctrine (res ipsa loquitur), which instructs judges to take no account of the base rates ( Kaye, 1979 ).
3. ^ Note that other CB, such as framing and omission bias, might also shed light on judicial decision-making ( Rachlinski, 2018 ). In fact, judges decide cases differently depending on whether the underlying facts are presented as gains or losses ( Rachlinski and Wistrich, 2018 ). Moreover, viewing the acceptance of a claim as the path of action and its dismissal as the path of inaction, omission bias might explain why the acceptance threshold of judges of a plaintiff’s claim is particularly high ( Zamir and Ritov, 2012 ). However, those biases have been much less studied than anchoring and hindsight bias.
Baker, K. H., and Nofsinger, J. R. (2002). Psychological biases of investors. Financ. Ser. Rev. 11, 97–116.
Google Scholar
Baker, H. K., and Nofsinger, J. R. (Eds.). (2010). Behavioral Finance: Investors, Corporations, and Markets. Vol. 6. New York: John Wiley & Sons.
Baker, H. K., and Ricciardi, V. (Eds.). (2014). Investor Behavior: The Psychology of Financial Planning and Investing. New York: John Wiley and Sons.
Barber, B. M., and Odean, T. (2000). Trading is hazardous to your wealth: The common stock investment performance of individual investors. J. Financ. 55, 773–806. doi: 10.1111/0022-1082.00226
CrossRef Full Text | Google Scholar
Barber, B., and Odean, T. (2001). Boys will be boys: gender, overconfidence, and common stock investment. Q. J. Econ. 116, 261–292. doi: 10.1162/003355301556400
Barnes, J. H. (1984). Cognitive biases and their impact on strategic planning. Strateg. Manag. J. 5, 129–137. doi: 10.1002/smj.4250050204
Baron, J. (2008). Thinking and Deciding. 4th Edn. Cambridge: Cambridge University Press.
Baron, J., and Hershey, J. C. (1988). Outcome bias in decision evaluation. J. Pers. Soc. Psychol. 54, 569–579. doi: 10.1037/0022-3514.54.4.569
Baron, J., and Ritov, I. (2004). Omission bias, individual differences, and normality. Organ. Behav. Hum. Decis. Process. 94, 74–85. doi: 10.1016/j.obhdp.2004.03.003
Bazerman, M. H. (1998). Judgment in Managerial Decision Making. New York: Wiley.
Bazerman, M. H., and Moore, D. (2008). Judgment in Managerial Decision Making. 7th Edn. Hoboken, NJ: Wiley.
Benartzi, S., and Thaler, R. H. (1995). Myopic loss aversion and the equity premium puzzle. Q. J. Econ. 110, 73–92. doi: 10.2307/2118511
Ben-David, I., Graham, J., and Harvey, C. (2013). Managerial miscalibration. Q. J. Econ. 128, 1547–1584. doi: 10.1093/qje/qjt023
Berlin, L., and Hendrix, R. W. (1998). Perceptual errors and negligence. AJR Am. J. Roentgenol. 170, 863–867. doi: 10.2214/ajr.170.4.9530024
PubMed Abstract | CrossRef Full Text | Google Scholar
Berthet, V. (2021). The measurement of individual differences in cognitive biases: A review and improvement. Front. Psychol. 12:630177. doi: 10.3389/fpsyg.2021.630177
Blumenthal-Barby, J. S., and Krieger, H. (2015). Cognitive biases and heuristics in medical decision making: A critical review using a systematic search strategy. Med. Decis. Mak. 35, 539–557. doi: 10.1177/0272989X14547740
Bogner, M. S. (Ed.) (1994). Human Error in Medicine. Hillsdale, NJ: Lawrence Erlbaum Associates, Inc.
Boolell-Gunesh, S., Broihanne, M., and Merli, M. (2009). Disposition effect, investor sophistication and taxes: Some French specificities. Finance 30, 51–78. doi: 10.3917/fina.301.0051
Brenner, L. A., Koehler, D. J., Liberman, V., and Tversky, A. (1996). Overconfidence in probability and frequency judgments: A critical examination. Organ. Behav. Hum. Decis. Process. 65, 212–219. doi: 10.1006/obhd.1996.0021
Bruine de Bruin, W., Parker, A. M., and Fischhoff, B. (2007). Individual differences in adult decision-making competence. J. Pers. Soc. Psychol. 92, 938–956. doi: 10.1037/0022-3514.92.5.938
Bukszar, E., and Connolly, T. (1988). Hindsight bias and strategic choice: Some problems in learning from experience. Acad. Manag. J. 31, 628–641.
Bystranowski, P., Janik, B., Próchnicki, M., and Skórska, P. (2021). Anchoring effect in legal decision-making: A meta-analysis. Law Hum. Behav. 45, 1–23. doi: 10.1037/lhb0000438
Casper, J. D., Benedict, K., and Perry, J. L. (1989). Juror decision making, attitudes, and the hindsight bias. Law Hum. Behav. 13, 291–310. doi: 10.1007/BF01067031
Chapman, G. B., and Bornstein, B. H. (1996). The more you ask for, the more you get: anchoring in personal injury verdicts. Appl. Cogn. Psychol. 10, 519–540. doi: 10.1002/(SICI)1099-0720(199612)10:6<519::AID-ACP417>3.0.CO;2-5
Cheney, F. W., Posner, K., Caplan, R. A., and Ward, R. J. (1989). Standard of care and anesthesia liability. JAMA 261, 1599–1603. doi: 10.1001/jama.1989.03420110075027
Chuang, W.-I., and Lee, B.-S. (2006). An empirical evaluation of the overconfidence hypothesis. J. Bank. Financ. 30, 2489–2515. doi: 10.1016/j.jbankfin.2005.08.007
Cohen, M. D., March, J. G., and Olsen, J. P. (1972). A garbage can model of organizational choice. Adm. Sci. Q. 17, 1–25. doi: 10.2307/2392088
Coval, J. D., and Moskowitz, T. J. (1999). Home bias at home: local equity preference in domestic portfolios. J. Financ. 54, 2045–2073. doi: 10.1111/0022-1082.00181
Croskerry, P. (2003). The importance of cognitive errors in diagnosis and strategies to minimize them. Acad. Med. 78, 775–780. doi: 10.1097/00001888-200308000-00003
Croskerry, P., Singhal, G., and Mamede, S. (2013). Cognitive debiasing 1: origins of bias and theory of debiasing. BMJ Qual. Saf. , 22(Suppl 2), 58–64. doi: 10.1136/bmjqs-2012-001712
Crowley, R. S., Legowski, E., Medvedeva, O., Reitmeyer, K., Tseytlin, E., Castine, M., et al. (2013). Automated detection of heuristics and biases among pathologists in a computer-based system. Adv. Health Sci. Educ. Theory Pract. 18, 343–363. doi: 10.1007/s10459-012-9374-z
Danziger, S., Levav, J., and Avnaim-Pesso, L. (2011). Extraneous factors in judicial decisions. Proc. Natl. Acad. Sci. 108, 6889–6892. doi: 10.1073/pnas.1018033108
Das, T. K., and Teng, B. (1999). Cognitive biases and strategic decision processes: An integrative perspective. J. Manag. Stud. 36, 757–778. doi: 10.1111/1467-6486.00157
Dawson, N. V., and Arkes, H. R. (1987). Systematic errors in medical decision making. J. Gen. Intern. Med. 2, 183–187. doi: 10.1007/BF02596149
De Bondt, W. F. (1998). A portrait of the individual investor. Eur. Econ. Rev. 42, 831–844. doi: 10.1016/S0014-2921(98)00009-9
De Bondt, W. F. M., and Thaler, R. (1985). Does the stock market overreact? J. Financ. 40, 793–805. doi: 10.1111/j.1540-6261.1985.tb05004.x
De Long, J. B., Shleifer, A., Summers, L. H., and Waldmann, R. J. (1990). Noise Trader Risk in Financial Markets. J. Polit. Econ. 98, 703–738.
PubMed Abstract | Google Scholar
Detmer, D. E., Fryback, D. G., and Gassner, K. (1978). Heuristics and biases in medical decision-making. J. Med. Educ. 53, 682–683.
Dhami, M. K., and Belton, I. K. (2017). On getting inside the judge’s mind. Trans. Issues Psychol. Sci. 3, 214–226. doi: 10.1037/tps0000115
Diamond, S. S. (1997). Illuminations and shadows from jury simulations. Law Hum. Behav. 21, 561–571. doi: 10.1023/A:1024831908377
Diamond, S. S., Saks, M. J., and Landsman, S. (1998). Jurors judgments about liability and damages: sources of variability and ways to increase consistency. DePaul Law Rev. 48, 301–325.
Duhaime, I. M., and Schwenk, C. R. (1985). Conjectures on cognitive simplification in acquisition and divestment decision making. Acad. Manag. Rev. 10, 287–295. doi: 10.5465/amr.1985.4278207
Ebbesen, E. B., and Konecni, V. J. (1975). Decision making and information integration in the courts: The setting of bail. J. Pers. Soc. Psychol. 32, 805–821. doi: 10.1037/0022-3514.32.5.805
Eisenberg, T., Goerdt, J., Ostrom, B., Rottman, D., and Wells, M. T. (1997). The predictability of punitive damages. J. Leg. Stud. 26, 623–661. doi: 10.1086/468010
Eisenberg, T., Hannaford-Agor, P. L., Heise, M., LaFountain, N., Munsterman, G. T., Ostrom, B., et al. (2006). Juries, judges, and punitive damages: empirical analyses using the civil justice survey of state courts 1992, 1996, and 2001 data. J. Empir. Leg. Stud. 3, 263–295. doi: 10.1111/j.1740-1461.2006.00070.x
Eisenhardt, K. M., and Zbaracki, M. J. (1992). Strategic decision making. Strateg. Manag. J. 13, 17–37. doi: 10.1002/smj.4250130904
Elstein, A. S. (1999). Heuristics and biases: selected errors in clinical reasoning. Acad. Med. 74, 791–794. doi: 10.1097/00001888-199907000-00012
Englich, B., Mussweiler, T., and Strack, F. (2005). The last word in court--A hidden disadvantage for the defense. Law Hum. Behav. 29, 705–722. doi: 10.1007/s10979-005-8380-7
Englich, B., Mussweiler, T., and Strack, F. (2006). Playing dice with criminal sentences: the influence of irrelevant anchors on experts’ judicial decision making. Personal. Soc. Psychol. Bull. 32, 188–200. doi: 10.1177/0146167205282152
Enough, B., and Mussweiler, T. (2001). Sentencing Under uncertainty: anchoring effects in the courtroom. J. Appl. Soc. Psychol. 31, 1535–1551. doi: 10.1111/j.1559-1816.2001.tb02687.x
Findley, K. A., and Scott, M. S. (2006). The multiple dimensions of tunnel vision in criminal cases. Wis. Law Rev. 2, 291–398.
Fischhoff, B. (1975). Hindsight is not equal to foresight: The effect of outcome knowledge on judgment under uncertainty. J. Exp. Psychol. Hum. Percept. Perform. 1, 288–299.
Forrow, L., Taylor, W. C., and Arnold, R. M. (1992). Absolutely relative: how research results are summarized can affect treatment decisions. Am. J. Med. 92, 121–124. doi: 10.1016/0002-9343(92)90100-P
Gigerenzer, G. (1991). “How to make cognitive illusions disappear: Beyond “heuristics and biases,”” in European Review of Social Psychology. Vol. 2 W. Stroebe and M. Hewstone (Eds.) (Chichester: Wiley), 83–115.
Gigerenzer, G. (1996). On narrow norms and vague heuristics: A reply to Kahneman and Tversky. Psychol. Rev. 103, 592–596. doi: 10.1037/0033-295X.103.3.592
Gigerenzer, G., Hertwig, R., Hoffrage, U., and Sedlmeier, P. (2008). “Cognitive illusions reconsidered,” in Handbook of Experimental Economics Results. eds. C. R. Plott and V. L. Smith (Amsterdam: Elsevier), 1018–1034. doi: 10.1016/S1574-0722(07)00109-6
Gilovich, T., Griffin, D., and Kahneman, D. (Eds.) (2002). Heuristics and Biases: The Psychology of Intuitive Judgment. Cambridge: Cambridge University Press.
Glaser, M., and Weber, M. (2007). Overconfidence and trading volume. Geneva Risk Insur. Rev. 32, 1–36. doi: 10.1007/s10713-007-0003-3
Graber, M. L. (2013). The incidence of diagnostic error in medicine. BMJ Qual. Saf. , 22(Suppl 2), 21–27. doi: 10.1136/bmjqs-2012-001615
Graber, M. L., Franklin, N., and Gordon, R. (2005). Diagnostic error in internal medicine. Arch. Intern. Med. 165, 1493–1499. doi: 10.1001/archinte.165.13.1493
Graber, M., Gordon, R., and Franklin, N. (2002). Reducing diagnostic errors in medicine: what’s the goal? Acad. Med. 77, 981–992. doi: 10.1097/00001888-200210000-00009
Graber, M. L., Kissam, S., Payne, V. L., Meyer, A. N., Sorensen, A., Lenfestey, N., et al. (2012). Cognitive interventions to reduce diagnostic error: a narrative review. BMJ Qual. Saf. 21, 535–557. doi: 10.1136/bmjqs-2011-000149
Grinblatt, M., Titman, S., and Wermers, R. (1995). Momentum investment strategies, portfolio performance, and herding: A study of mutual fund behavior. Am. Econ. Rev. 85, 1088–1105.
Guthrie, C., Rachlinski, J. J., and Wistrich, A. J. (2001). Inside the judicial mind. Cornell Law Rev. 86, 777–830. doi: 10.2139/ssrn.257634
Guthrie, C., Rachlinski, J. J., and Wistrich, A. J. (2002). Judging by heuristic: cognitive illusions in judicial decision making. Judicature 86, 44–50.
Guthrie, C., Rachlinski, J., and Wistrich, A. J. (2007). Blinking on the bench: how judges decide cases. Cornell Law Rev. 93, 1–43.
Hans, V. P., and Reyna, V. F. (2011). To dollars from sense: qualitative to quantitative translation in jury damage awards. J. Empir. Leg. Stud. 8, 120–147. doi: 10.1111/j.1740-1461.2011.01233.x
Hardman, D., and Harries, C. (2002). How rational are we? Psychologist 15, 76–79.
Harley, E. M. (2007). Hindsight bias in legal decision making. Soc. Cogn. 25, 48–63. doi: 10.1521/soco.2007.25.1.48
Hart, A. J., Evans, D. L., Wissler, R. L., Feehan, J. W., and Saks, M. J. (1997). Injuries, prior beliefs, and damage awards. Behav. Sci. Law 15, 63–82. doi: 10.1002/(SICI)1099-0798(199724)15:1<63::AID-BSL254>3.0.CO;2-9
Hastie, R., Schkade, D. A., and Payne, J. W. (1999). Juror judgments in civil cases: effects of plaintiff’s requests and plaintiff’s identity on punitive damage awards. Law Hum. Behav. 23, 445–470. doi: 10.1023/A:1022312115561
Helm, R. K., Wistrich, A. J., and Rachlinski, J. J. (2016). Are arbitrators human? J. Empir. Leg. Stud. 13, 666–692. doi: 10.1111/jels.12129
Hershberger, P. J., Part, H. M., Markert, R. J., Cohen, S. M., and Finger, W. W. (1994). Development of a test of cognitive bias in medical decision making. Acad. Med. 69, 839–842. doi: 10.1097/00001888-199410000-00014
Hinsz, V. B., and Indahl, K. E. (1995). Assimilation to anchors for damage awards in a mock civil trial. J. Appl. Soc. Psychol. 25, 991–1026. doi: 10.1111/j.1559-1816.1995.tb02386.x
Hodgkinson, G. (2001). “Cognitive processes in strategic management: some emerging trends and future directions,” in Handbook of Industrial, Work and Organizational Psychology Organizational Psychology. Vol. 2. eds. N. Anderson, D. S. Ones, and H. K. Sinangil (London: SAGE Publications Ltd.), 416–440.
Hodgkinson, G. P., Bown, N. J., Maule, A. J., Glaister, K. W., and Pearman, A. D. (1999). Breaking the frame: an analysis of strategic cognition and decision making under uncertainty. Strateg. Manag. J. 20, 977–985. doi: 10.1002/(SICI)1097-0266(199910)20:10<977::AID-SMJ58>3.0.CO;2-X
Huff, A. S., and Schwenk, C. (1990). “Bias and sensemaking in good times and bad,” in Mapping Strategic Thought. ed. A. S. Huff (Ed.) (Chichester, England: Wiley), 89–108.
Johnson, G. (1987). Strategic Change and the Management Process. Oxford: Basil Blackwell.
Joyce, E., and Biddle, G. (1981). Anchoring and adjustment in probabilistic inference in auditing. J. Account. Res. 19, 120–145. doi: 10.2307/2490965
Kahneman, D. (2011). Thinking, Fast and Slow. New York: Farrar, Straus and Giroux.
Kahneman, D., and Frederick, S. (2002). “Representativeness revisited: attribute substitution in intuitive judgment,” in Heuristics and Biases: The Psychology of Intuitive Judgment. T. Gilovich, D. Griffin, and D. Kahneman (Eds.) (Cambridge: Cambridge University Press), 103–119.
Kahneman, D., Schkade, D., and Sunstein, C. (1998). Shared outrage and erratic awards: The psychology of punitive damages. J. Risk Uncertain. 16, 49–86. doi: 10.1023/A:1007710408413
Kahneman, D., Slovic, P., and Tversky, A. (Eds.) (1982). Judgment Under Uncertainty: Heuristics and Biases. New York: Cambridge University Press.
Kahneman, D., and Tversky, A. (1979). Prospect theory: An analysis of decision under risk. Econometrica 47, 263–291. doi: 10.2307/1914185
Kamin, K. A., and Rachlinski, J. J. (1995). Ex post ≠ ex ante: determining liability in hindsight. Law Hum. Behav. 19, 89–104. doi: 10.1007/BF01499075
Kaustia, M., Alho, E., and Puttonen, V. (2008). How much does expertise reduce behavioral biases? The case of anchoring effects in stock return estimates. Financ. Manag. 37, 391–412. doi: 10.1111/j.1755-053X.2008.00018.x
Kaye, D. (1979). Probability theory meets res Ipsa loquitur. Mich. Law Rev. 77, 1456–1484. doi: 10.2307/1288109
Klein, J. G. (2005). Five pitfalls in decisions about diagnosis and prescribing. BMJ 330, 781–783. doi: 10.1136/bmj.330.7494.781
Klein, D. E., and Mitchell, G. (Eds.) (2010). The Psychology of Judicial Decision Making. New York, NY: Oxford University Press.
Kukucka, J., Kassin, S. M., Zapf, P. A., and Dror, I. E. (2017). Cognitive bias and blindness: A global survey of forensic science examiners. J. Appl. Res. Mem. Cogn. 6, 452–459. doi: 10.1016/j.jarmac.2017.09.001
Kumar, S., and Goyal, N. (2015). Behavioural biases in investment decision making – A systematic literature review. Qual. Res. Financ. Markets 7, 88–108. doi: 10.1108/QRFM-07-2014-0022
LaBine, S. J., and LaBine, G. (1996). Determinations of negligence and the hindsight bias. Law Hum. Behav. 20, 501–516. doi: 10.1007/BF01499038
Lidén, M., Gräns, M., and Juslin, P. (2019). ‘Guilty, no doubt’: detention provoking confirmation bias in judges’ guilt assessments and debiasing techniques. Psychol. Crime Law 25, 219–247. doi: 10.1080/1068316X.2018.1511790
Lusardi, A., and Mitchell, O. S. (2014). The economic importance of financial literacy: theory and evidence. J. Econ. Lit. 52, 5–44.
Lyles, M. A., and Thomas, H. (1988). Strategic problem formulation: biases and assumptions embedded in alternative decision-making models. J. Manag. Stud. 25, 131–145. doi: 10.1111/j.1467-6486.1988.tb00028.x
MacCoun, R. J. (1989). Experimental research on jury decision-making. Science 244, 1046–1050. doi: 10.1126/science.244.4908.1046
Malmendier, U., and Tate, G. (2005). CEO overconfidence and corporate investment. J. Financ. 60, 2661–2700. doi: 10.1111/j.1540-6261.2005.00813.x
Malmendier, U., and Tate, G. (2008). Who makes acquisitions? CEO overconfidence and the market’s reaction. J. Financ. Econ. 89, 20–43. doi: 10.1016/j.jfineco.2007.07.002
Mamede, S., van Gog, T., van den Berge, K., Rikers, R. M., van Saase, J. L., van Guldener, C., et al. (2010). Effect of availability bias and reflective reasoning on diagnostic accuracy among internal medicine residents. JAMA 304, 1198–1203. doi: 10.1001/jama.2010.1276
March, J. G., and Shapira, Z. (1987). Managerial perspectives on risk and risk taking. Manag. Sci. 33, 1404–1418. doi: 10.1287/mnsc.33.11.1404
March, J. G., and Simon, H. A. (1958). Organizations. New York: Wiley.
Maule, A. J., and Hodgkinson, G. P. (2002). Heuristics, biases and strategic decision making. Psychologist 15, 68–71.
Mintzberg, H. (1983). Power In and Around Organizations. Englewood Cliffs, N.J: Prentice-Hall.
Mohammed, S., and Schwall, A. (2012). Individual differences and decision making: what we know and where we go from here. Int. Rev. Ind. Organ. Psychol. 24, 249–312. doi: 10.1002/9780470745267.ch8
Moore, D. A., Oesch, J. M., and Zietsma, C. (2007). What competition? Myopic self-focus in market-entry decisions. Organ. Sci. 18, 440–454. doi: 10.1287/orsc.1060.0243
Moore, D. A., and Schatz, D. (2017). The three faces of overconfidence. Soc. Personal. Psychol. Compass 11:e122331. doi: 10.1111/spc3.12331
Morewedge, C. K., Yoon, H., Scopelliti, I., Symborski, C., Korris, J., and Kassam, K. S. (2015). Debiasing decisions: improved decision making with a single training intervention. Policy Insights Behav. Brain Sci. 2, 129–140. doi: 10.1177/2372732215600886
Nickerson, R. S. (1998). Confirmation bias: A ubiquitous phenomenon in many guises. Rev. Gen. Psychol. 2, 175–220. doi: 10.1037/1089-2680.2.2.175
O’Brien, B. (2009). Prime suspect: An examination of factors that aggravate and counteract confirmation bias in criminal investigations. Psychol. Public Policy Law 15, 315–334. doi: 10.1037/a0017881
Odean, T. (1998). Are investors reluctant to realize their losses? J. Financ. 53, 1775–1798. doi: 10.1111/0022-1082.00072
Odean, T. (1999). Do Investors trade too much? Am. Econ. Rev. 89, 1279–1298. doi: 10.1257/aer.89.5.1279
Oeberst, A., and Goeckenjan, I. (2016). When being wise after the event results in injustice: evidence for hindsight bias in judges’ negligence assessments. Psychol. Public Policy Law 22, 271–279. doi: 10.1037/law0000091
Ogdie, A. R., Reilly, J. B., Pang, W. G., Keddem, S., Barg, F. K., Von Feldt, J. M., et al. (2012). Seen through their eyes: residents’ reflections on the cognitive and contextual components of diagnostic errors in medicine. Acad. Med. 87, 1361–1367. doi: 10.1097/ACM.0b013e31826742c9
Parker, A. M., and Fischhoff, B. (2005). Decision-making competence: external validation through an individual-differences approach. J. Behav. Decis. Mak. 18, 1–27. doi: 10.1002/bdm.481
Peer, E., and Gamliel, E. (2013). Heuristics and biases in judicial decisions. Court Rev. 49, 114–118.
Perneger, T. V., and Agoritsas, T. (2011). Doctors and patients’ susceptibility to framing bias: A randomized trial. J. Gen. Intern. Med. 26, 1411–1417. doi: 10.1007/s11606-011-1810-x
Pohl, R. F. (2017). “Cognitive illusions,” in Cognitive Illusions: Intriguing Phenomena in Thinking, Judgment and Memory (London; New York, NY: Routledge/Taylor&Francis Group), 3–21.
Powell, T. C., Lovallo, D., and Fox, C. (2011). Behavioral strategy. Strateg. Manag. J. 32, 1369–1386. doi: 10.1002/smj.968
Rachlinski, J. J. (2006). Cognitive errors, individual differences, and paternalism. Univ. Chicago Law Rev. 73, 207–229. doi: 10.1093/acprof:oso/9780199211395.003.0008
Rachlinski, J. J. (2018). “Judicial decision-making,” in Behavioral Law and Economics. E. Zamir and D. Teichman (Eds.) (New York, NY: Oxford University Press), 525–565.
Rachlinski, J. J., Guthrie, C., and Wistrich, A. J. (2011). Probable cause, probability, and hindsight. J. Empir. Leg. Stud. 8, 72–98. doi: 10.1111/j.1740-1461.2011.01230.x
Rachlinski, J. J., Guthrie, C., and Wistrich, A. J. (2013). How lawyers’ intuitions prolong litigation. South. Calif. Law Rev. 86, 571–636.
Rachlinski, J. J., and Wistrich, A. J. (2017). Judging the judiciary by the numbers: empirical research on judges. Ann. Rev. Law Soc. Sci. 13, 203–229. doi: 10.1146/annurev-lawsocsci-110615-085032
Rachlinski, J. J., and Wistrich, A. J. (2018). Gains, losses, and judges: framing and the judiciary. Notre Dame Law Rev. 94, 521–582.
Rachlinski, J., Wistrich, A., and Guthrie, C. (2015). Can judges make reliable numeric judgments? Distorted damages and skewed sentences. Indiana Law J. 90, 695–739.
Redelmeier, D. A. (2005). The cognitive psychology of missed diagnoses. Ann. Intern. Med. 142, 115–120. doi: 10.7326/0003-4819-142-2-200501180-00010
Robbennolt, J. K., and Studebaker, C. A. (1999). Anchoring in the courtroom: The effects of caps on punitive damages. Law Hum. Behav. 23, 353–373. doi: 10.1023/A:1022312716354
Saposnik, G., Redelmeier, D., Ruff, C. C., and Tobler, P. N. (2016). Cognitive biases associated with medical decisions: a systematic review. BMC Med. Inform. Decis. Mak. 6:138. doi: 10.1186/s12911-016-0377-1
Schmitt, B. P., and Elstein, A. S. (1988). Patient management problems: heuristics and biases. Med. Decs. Making 8, 224–225.
Schnapp, B. H., Sun, J. E., Kim, J. L., Strayer, R. J., and Shah, K. H. (2018). Cognitive error in an academic emergency department. Diagnosis 5, 135–142. doi: 10.1515/dx-2018-0011
Schwenk, C. R. (1982). Dialectical inquiry in strategic decision-making: A comment on the continuing debate. Strateg. Manag. J. 3, 371–373. doi: 10.1002/smj.4250030408
Schwenk, C. R. (1984). Cognitive simplification processes in strategic decision-making. Strateg. Manag. J. 5, 111–128. doi: 10.1002/smj.4250050203
Schwenk, C. R. (1985). Management illusions and biases: their impact on strategic decisions. Long Range Plan. 18, 74–80. doi: 10.1016/0024-6301(85)90204-3
Schwenk, C. R. (1988). The cognitive perspective on strategic decision making. J. Manag. Stud. 25, 41–55. doi: 10.1111/j.1467-6486.1988.tb00021.x
Sellier, A. L., Scopelliti, I., and Morewedge, C. K. (2019). Debiasing training improves decision making in the field. Psychol. Sci. 30, 1371–1379. doi: 10.1177/0956797619861429
Shefrin, H. (2000). Beyond Greed and Fear: Understanding Behavioral Finance and the Psychology of Investing. Boston: Harvard Business School Press.
Shefrin, H., and Statman, M. (1985). The disposition to sell winners too early and ride losers too long: theory and evidence. J. Financ. 40, 777–790. doi: 10.1111/j.1540-6261.1985.tb05002.x
Shiller, R. J. (2003). From efficient markets theory to behavioral finance. J. Econ. Perspect. 17, 83–104. doi: 10.1257/089533003321164967
Stanovich, K. E., Toplak, M. E., and West, R. F. (2008). The development of rational thought: a taxonomy of heuristics and biases. Adv. Child Dev. Behav. 36, 251–285. doi: 10.1016/S0065-2407(08)00006-2
Stanovich, K. E., West, R. F., and Toplak, M. E. (2011). “Individual differences as essential components of heuristics and biases research,” in The Science of Reason: A Festschrift for Jonathan St B. T. Evans. K. Manktelow, D. Over, and S. Elqayam (Eds.) (New York: Psychology Press), 355–396.
Statman, M., Thorley, S., and Vorkink, K. (2006). Investor overconfidence and trading volume. Rev. Financ. Stud. 19, 1531–1565. doi: 10.1093/rfs/hhj032
Stiegler, M. P., and Ruskin, K. J. (2012). Decision-making and safety in anesthesiology. Curr. Opin. Anaesthesiol. 25, 724–729. doi: 10.1097/ACO.0b013e328359307a
Talpsepp, T. (2011). Reverse disposition effect of foreign investors. J. Behav. Financ. 12, 183–200. doi: 10.1080/15427560.2011.606387
Tversky, A., and Kahneman, D. (1973). Availability: A heuristic for judging frequency and probability. Cogn. Psychol. 5, 207–232. doi: 10.1016/0010-0285(73)90033-9
Tversky, A., and Kahneman, D. (1974). Judgment under uncertainty: heuristics and biases. Science 185, 1124–1131. doi: 10.1126/science.185.4157.1124
Tversky, A., and Kahneman, D. (1981). The framing of decisions and the psychology of choice. Science 211, 453–458. doi: 10.1126/science.7455683
Vranas, P. B. M. (2000). Gigerenzer’s normative critique of Kahneman and Tversky. Cognition 76, 179–193. doi: 10.1016/S0010-0277(99)00084-0
Wears, R. L., and Nemeth, C. P. (2007). Replacing hindsight with insight: toward better understanding of diagnostic failures. Ann. Emerg. Med. 49, 206–209. doi: 10.1016/j.annemergmed.2006.08.027
Weinshall-Margel, K., and Shapard, J. (2011). Overlooked factors in the analysis of parole decisions. Proc. Natl. Acad. Sci. 108:E833. doi: 10.1073/pnas.1110910108
Wissler, R. L., Hart, A. J., and Saks, M. J. (1999). Decision-making about general damages: A comparison of jurors, judges, and lawyers. Mich. Law Rev. 98, 751–826. doi: 10.2307/1290315
Zajac, E. J., and Bazerman, M. H. (1991). Blind spots in industry and competitor analysis: implications of interfirm (mis)perceptions for strategic decisions. Acad. Manag. Rev. 16, 37–56. doi: 10.5465/amr.1991.4278990
Zamir, E., and Ritov, I. (2012). Loss aversion, omission bias, and the burden of proof in civil litigation. J. Leg. Stud. 41, 165–207. doi: 10.1086/664911
Zwaan, L., Monteiro, S., Sherbino, J., Ilgen, J., Howey, B., and Norman, G. (2017). Is bias in the eye of the beholder? A vignette study to assess recognition of cognitive biases in clinical case workups. BMJ Qual. Saf. 26, 104–110. doi: 10.1136/bmjqs-2015-005014
Keywords: decision-making, cognitive biases, heuristics, management, finance, medicine, law
Citation: Berthet V (2022) The Impact of Cognitive Biases on Professionals’ Decision-Making: A Review of Four Occupational Areas. Front. Psychol . 12:802439. doi: 10.3389/fpsyg.2021.802439
Received: 26 October 2021; Accepted: 03 December 2021; Published: 04 January 2022.
Reviewed by:
Copyright © 2022 Berthet. This is an open-access article distributed under the terms of the Creative Commons Attribution License (CC BY) . The use, distribution or reproduction in other forums is permitted, provided the original author(s) and the copyright owner(s) are credited and that the original publication in this journal is cited, in accordance with accepted academic practice. No use, distribution or reproduction is permitted which does not comply with these terms.
*Correspondence: Vincent Berthet, [email protected]
Disclaimer: All claims expressed in this article are solely those of the authors and do not necessarily represent those of their affiliated organizations, or those of the publisher, the editors and the reviewers. Any product that may be evaluated in this article or claim that may be made by its manufacturer is not guaranteed or endorsed by the publisher.
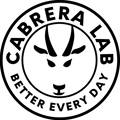
- Get Started Resources
- Main Glossaries
- Individual glossary pages
- DSRP 'Shards'
- Model Classroom
- Case Studies
- Systems Models
- White Papers
- Publications
- Common Core Standards
- Systems Mapping
- Systems Mapping (SM) Software Review Library
- Systemic Program Models
- Vision-Mission Library
- Help Center
Case Study: Reality Bias: The Mother of All Cognitive Biases
Part of the Cognitive Case Study Series from Cabrera Research Lab, this case explores reality bias, the role of metacognition in reducing it, and the foundational role of reality bias in creating all other cognitive biases.
A “cognitive case study”—inspired by the cases used in business and policy schools that involve students in real-world problem solving—is designed to engage students in metacognition (thinking about thinking). Cognitive cases introduce the cognitive patterns underlying our mental models, and then encourage us to explore how our and others’ mental models affect our emotions, behavior, action, and even our reality. These cases explore a broad range of topics, from politics to social issues to the physical sciences to everyday phenomena, with the purpose of enabling readers to see the cognitive structures at play across a variety of realms


- The Interactions of Heuristics and Biases in the Making of Decisions
Alice Newkirk
Given the sheer number of decisions the average person makes on any given day, the brain's use of shortcuts to help assess different choices makes perfect sense. It would be a waste of time and energy if someone had to do an exhaustive cost-benefit analysis to decide which brand of laundry detergent to buy, or which kind of pizza to order. As a result, people use a number of mental shortcuts, or heuristics, to help make decisions, which provide general rules of thumb for decision making (Tversky & Kahneman, 1982). However, the same glossing over of factors that makes heuristics a convenient and quick solution for many smaller issues means that they actually hinder the making of decisions about more complicated issues (Tversky & Kahneman, 1982). Heuristics are simplifications, and while simplifications use fewer cognitive resources, they also, well, simplify. Furthermore, since people mostly use these shortcuts automatically, they can also preempt analytical thinking in situations where a more logical process might yield better results. Although heuristics are useful shortcuts for everyday judgment calls, they can lead people to make hasty, sometimes incorrect decisions about issues that are more complicated.
An excellent case study for the flaws and complications of heuristics is the hypothetical case of Audrey, a hypochondriac whose vitamin-taking regimen is challenged by a new study linking vitamins with increased risk of death. Audrey attributes her good health to her vitamins, and her decision making process is further complicated by the advice of her friend, who tells her that the study is worthless and she should ignore it completely. Whether or not Audrey later goes through a more thorough reasoning process, her initial judgment will be highly influenced by common decision making heuristics. Audrey’s case is an excellent lens through which to look at common heuristics and the problems they create because her hypochondria makes her perceive her decision as having potentially dire consequence; she has a strong emotional investment in the decision, which has the potential to override her reasoning self. Although her situation is unique, the way she uses heuristics will follow common patterns of thinking. In Audrey's case, heuristics will lead her to believe that vitamins can only either be completely toxic or utterly harmless; her emotional attachment to her vitamins will give her a strong bias in favor of the second conclusion, and as a result she will reject the study entirely. This extreme reaction will highlight common heuristics and biases in an extreme way.
From the start, Audrey will be looking at her vitamin dilemma through the lens of her emotions. The affect heuristic suggests that strong emotional reactions often take the place of more careful reasoning (Sunstein, 2002), and Audrey has plenty of reason to have strong emotional reactions. Hypochondria is a mental illness centered around an irrational fear of serious disease, and hypochondriacs are obsessed with staying healthy as a result of this fear (Medline, 2012). As a result, by challenging Audrey's beliefs, the study presents her with massive emotional turmoil. Her vitamin regime, which provides her with a way to control her irrational fear of illness, is being called into question, and as a result her fear and anxiety levels are likely to be even greater than usual. Both giving up and continuing to take her vitamins are choices with massive emotional weight: giving up her vitamins means giving up a source of security, and continuing to take them means possibly continuing to expose herself to future harm.
Audrey's emotional complications will be further exacerbated by a whole category of mental shortcuts known as intuitive toxicology. Intuitive toxicology governs the ways people think about chemicals, compounds and toxins, and includes the false notion that chemical compounds are either entirely dangerous or entirely safe: in other words, that there is no such thing as moderately dangerous or dangerous only in excess (Sunstein, 2002). While not technically heuristics, these simplifications often erase the complexity associated with carcinogens and chemical health risks (Sunstein, 2002). By falling prey to the all-or-nothing model of risk, Audrey will not be able to think of the risk presented by the vitamins as a slight increase in the statistical probability of death. In her mind, her vitamins will either be completely harmless or dangerously toxic.
Furthermore, other effects of the affect heuristic will increase the stakes, and her emotional investment, even more. The affect heuristic links the perception of risks and the perception of benefits: when people perceive something to be high risk they perceive it to be low benefit, and vice versa (Sunstein, 2002). People have trouble believing that something is simultaneously risky and beneficial, especially where the risks are perceived to be very high (Sunstein, 2002). So as a result of the affect heuristic, if Audrey thinks that her vitamins are high risk, she will also think that they are low benefit. For Audrey, choosing to give up her vitamins as a result of the study would not only be admitting that she has been doing something actively harmful, but also that the regime on which she based her good health and safety had no benefits at all.
These high emotional stakes will give Audrey a bias in terms of what she wants to be true, even if her emotions play no further part in her reasoning process: accepting the study as true would mean that her main source of safety and support was extremely dangerous and not beneficial through the lenses of the all-or-nothing and affect heuristic biases. As a result, she will be motivated to show that the study is completely wrong. Her emotional investment in this hypothesis will lead to a number of other biases which will further affect her reasoning process, especially since she already strongly believes vitamins are healthy. Most notably, she will be subject to the belief-bias effect and confirmation bias.
The belief-bias effect, the first of these biases, has two parts: when a conclusion is unbelievable, it is much harder for people to accept, even when the logic is sound; and when a conclusion is believable people are much less likely to question its logic (Evans & Feeney, 2004). There are two potential explanations for these effects, both with implications for Audrey's decision making process. The first, the Selective Scrutiny Model, suggests that people are more likely to think critically about evidence when presented with a conclusion they disagree with (Evans & Feeney, 2004). In Audrey's case, she is more likely to be skeptical about the evidence provided by the study because she disagrees with its findings. The second, the Misinterpreted Necessity Model, suggests that people rely on prior beliefs to guide their judgments when the evidence is unclear (Evans & Feeney, 2004). This model has clear applications to Audrey's situation: when presented with the conflicting evidence provided by her friend and by the study, she is likely to rely on her previous belief to make her choice, i.e. that vitamins are healthy and harmless. Both of these models will lead Audrey to be far more skeptical of the studies findings, and far more accepting of evidence supporting her original beliefs.
Not only will Audrey be far more accepting of evidence supporting her preferred hypothesis, she will actively seek out evidence, as suggested by confirmation bias, that validates her beliefs. Confirmation bias leads to people seeking out information that confirms their hypotheses instead of refuting it (Evans & Feeney, 2004). Once Audrey has decided on a hypothesis—in this case, the one suggested by her previous beliefs and emotional reaction—she will look for pieces of evidence that support it, instead of searching for conflicting evidence and revising her theory based on that. As a result of the belief bias effect and confirmation bias, Audrey will actively search for information that supports her belief in vitamins, accept it more easily than she would other information and scrutinize conflicting evidence more aggressively.
Audrey will be able to find plenty of support for her hypothesis through other heuristics and biases. A variety of heuristics and biases can take the place of empirical evidence in decision making (Tversky & Kahneman, 1982); These heuristics, and their resulting biases, will provide Audrey with 'evidence' in favor of her all-natural vitamin regime. This evidence might not stand up to critical, unbiased analysis, but since she is looking for evidence that confirms her hypothesis and not scrutinizing confirming evidence too carefully as a result of belief bias and confirmation bias, her shortcuts will have a strong effect on her decision making. The first of these biases is another facet of intuitive toxicology. A number of specific biases come into play when people think about chemical risks, and one of these is the bias concerning the benevolence of nature (Sunstein, 2002). The chemicals produced in nature are not inherently safer than manufactured ones- for example, arsenic is a natural chemical, and is definitely not harmless. But as a rule of thumb, people tend to instinctively assume that natural compounds are somehow healthier and more benevolent than compounds which are man-made (Sunstein, 2002). This has clear implications for Audrey's all-natural vitamin regimen: since nature is fundamentally benevolent according to intuitive toxicology, Audrey's natural vitamins cannot be dangerous.
Audrey will find further evidence for her hypothesis through her previous positive experience with her vitamins. The representative heuristic, describes the different ways people often misattribute causes to various effects (Tversky & Kahneman, 1982). (Tversky & Kahneman, 1982). One example of this is the misconception that past experience is a good indicator of future forecasting. Even when present experience has little to no bearing on what someone is trying to predict, they are likely to try to use their present evidence to support their hypotheses for the future (Tversky & Kahneman, 1982). In Audrey's case, she will base her expectations of her vitamins off of her past experience with them, whether or not the two things are at all connected or if the effects of vitamins are supposed to be instantaneous. Since she attributes her good health to them, she presumably thinks of them very positively. Furthermore, the affect heuristic applies here as well; in this case, instead of high risks being associated with low benefits, high benefits are associated with low risk. Because she has previously seen vitamins as being extremely beneficial, she will also see them as having previously been low risk. She will use this as confirming evidence that the study is wrong: because she has in the past experienced only the positive effects of vitamins, she will assume that vitamins only have positive effects.
Audrey's confidence in her vitamins will be further strengthened by her conversation with her friend, who provides direct evidence to confirm her hypothesis. Audrey will be subject to the effects of group polarization: when multiple people of similar beliefs talk about something they share an opinion on, the opinion of the entire group is likely to shift further to the extreme, since people both have their beliefs confirmed and may be exposed to the beliefs of more radical people (Sunstein, 2002). Audrey is already motivated to prove the study wrong, already believes in the healthiness of vitamins and already has 'evidence' supporting these claims as a result of intuitive toxicology and the representative heuristic; her friend's rejection of the study will support her beliefs and polarize them even further. As a result, Audrey is likely to have her beliefs about vitamins confirmed and strengthened, and feel confident rejecting the results of the study completely.
Her previous positive associations with vitamins will help mitigate some of the potential negative effects of heuristics as well. Specifically, she will be less susceptible to alarmist bias, increased fear and urgency surrounding alarmingly vivid threats (Sunstein, 2002). Although the 'risk of death' mentioned by the study sounds very dangerous, it is also extremely vague. Death by vitamin does not have the urgency or vivid imagery of a plane crash or a terrorist attack. The threat of death will also be lessened by the availability heuristic, a mental shortcut for estimating the size or probability of something with how many examples come to mind—for example, estimating the number of five letter words ending in -ing by thinking of a few examples (Tversky & Kahneman, 1982). Audrey will not be able to think of examples of people who have died by vitamin overdose because that sort of thing doesn't make the news and is not particularly graphic, so her estimation of the threat will be severely diminished. Conversely, she will be able to think of a great many positive instances associated with vitamins, since she has used them for a long time and attributes her good health to them. As a result, she is likely to underestimate the severity of the negative consequences of her vitamin regime and overestimate their positive effects. The fear and anxiety brought up by these heuristics will be mitigated, and these heuristics will therefore have a much smaller effect on her reasoning process.
One of the other biases of intuitive toxicology also seems to work against Audrey's hypothesis. Laypeople often assume that it is possible and desirable for a chemical to have absolutely no associated risk, which trained toxicologists know to be untrue (Sunstein, 2002). At first, this seems to be a strike against Audrey's vitamins. They cannot be healthy or worthwhile if they have any associated risk at all, and the study suggests that they do. However, this fallacy's interactions with a number of other biases negates its effect. First, since Audrey is more critical of things she finds unbelievable as a result of the belief-bias effect, she is more likely to subject the zero-risk fallacy to critical examination. As a result, she is more likely to think logically about it and dismiss it as illogical than she is any of her other assumptions. Second, if she does not examine it critically, its interaction with the all-or-nothing fallacy will actually strengthen her notions about the safety of her vitamins. If her vitamins have associated risk, then by the all-or-nothing fallacy they must be dangerously toxic, a hypothesis which she is eager to reject. On the other hand, if they are completely healthy, the other option presented by the all-or-nothing fallacy, then they must have no risk associated, because the zero risk fallacy suggests that no risk is optimal and attainable for compounds. The zero-risk fallacy initially seems to counter Audrey's theories about risk, but as a result of her emotional investment combined with the biases driving her reasoning process, it will actually strengthen her argument.
Audrey's emotional reaction to the information presented by the study will dominate her initial thought process, and will guide her reasoning along with a number of general heuristics. Her mental polarization of the dilemma and her emotional investment in proving her original beliefs correct will lead her to instinctively reject the study in its entirety. However, her reasoning process does not have to end there, should she so choose. Heuristics are fundamentally shortcuts for reasoning, and people are perfectly capable of taking the long route to reach a better result. But whether or not Audrey decides to analyze the potential effects of her vitamins more critically, her beliefs and biases will play a role in the ways she initially thinks about her situation. Audrey's particular biases may be exacerbated by her intense situation, but they are the analogues of biases common to everyone. While our instincts can provide easy guidance in simple decisions where they accurately represent what's actually going on, in multifaceted issues like Audrey's vitamin dilemma, they can often lead us astray. By knowing when these heuristics may be working against us rather than for us, we can choose when to engage in deeper critical thinking and learn to overcome our own biases.
Bibliography
Evans, J. & Feeney, A. (2004). The role of prior belief in reasoning. In J.P. Leighton & R.J. Sternberg (eds.) The nature of reasoning . (pp.78-102). Cambridge, UK: Cambridge University Press.
Sunstein, C. R. (2002). Risk and reason: Safety, law, and the environment . Cambridge, UK: Cambridge University Press. Ch 2: Thinking About Risks, (pp. 28-58)
Tversky, A. & Kahneman, D. (1982). Judgment under uncertainty: Heuristics and biases. In D. Kahenman, P. Slovic, & A. Tversky (Eds.) Judgment under uncertainty: Heuristics and biases. (pp 3-20). Cambridge, UK: Cambridge University Press.
Issue Contents
- An Answer to Langer and Lopate: Two-Layered Representation in Art Spiegelman’s Maus
- Beyond the Biographical: Modern Meaning in Gilje's Susanna and the Elders, Restored
- Colombia: A Case Study of Archaeology and Nationalism
- I Am Become President: The Rhetorical Choreography of Johnson’s Nuclear Propaganda
- Interpreting the Failure of the Poor People’s Campaign
- On Uncertainty and Possibility: Consequences of an Unproven Science
- The BBC’s Pride and Prejudice: Falling in Love through Nature
- Then and Now: Healing in the Aftermath of Cambodian Genocide

- SUGGESTED TOPICS
- The Magazine
- Newsletters
- Managing Yourself
- Managing Teams
- Work-life Balance
- The Big Idea
- Data & Visuals
- Reading Lists
- Case Selections
- HBR Learning
- Topic Feeds
- Account Settings
- Email Preferences
Outsmart Your Own Biases
- Jack B. Soll,
- Katherine L. Milkman,
- John W. Payne

When making decisions, we all rely too heavily on intuition and use flawed reasoning sometimes. But it’s possible to fight these pernicious sources of bias by learning to spot them and using the techniques presented in this article, gleaned from the latest research. They’ll open up your thinking about possible outcomes, objectives, and options and lead to better choices.
To broaden your perspective on the future, the authors suggest, you can use proven tactics for improving the accuracy of estimates and preparing for contingencies. You’ll think more expansively about your objectives if you come up with many possibilities before deciding what’s most important, get input from others, and then carefully examine one goal at a time. And you’ll generate better options if you identify several and evaluate them side by side. Don’t settle for the first one that’s acceptable; imagine that you can’t pursue it, and you might find an even stronger alternative.
Strong emotional attachments or investments make cognitive biases even harder to overcome. When that’s the case, use checklists and algorithms to stay focused on the right things, and set “trip wires” to trigger planned responses at key points in the decision-making process.
HBR Reprint R1505D
How to broaden your thinking and make better decisions
Idea in Brief
The problem.
Cognitive biases muddy our decision making. We rely too heavily on intuitive, automatic judgments, and even when we try to use reason, our logic is often lazy or flawed.
Instead of exploring risks and uncertainties, we seek closure—it’s much easier. This narrows our thinking about what could happen in the future, what our goals are, and how we might achieve them.
The Solution
By knowing which biases tend to trip us up and using certain tricks and tools to outsmart them, we can broaden our thinking and make better choices.
Suppose you’re evaluating a job candidate to lead a new office in a different country. On paper this is by far the most qualified person you’ve seen. Her responses to your interview questions are flawless. She has impeccable social skills. Still, something doesn’t feel right. You can’t put your finger on what—you just have a sense. How do you decide whether to hire her?
- JS Jack B. Soll is an associate professor of management at Duke University’s Fuqua School of Business. He is a coauthor of “A User’s Guide to Debiasing,” a chapter in The Wiley Blackwell Handbook of Judgment and Decision Making, forthcoming in 2015.
- Katherine L. Milkman is the James G. Campbell Jr. Assistant Professor of Operations and Information Management at the University of Pennsylvania’s Wharton School. She is a coauthor of “A User’s Guide to Debiasing,” a chapter in The Wiley Blackwell Handbook of Judgment and Decision Making, forthcoming in 2015.
- JP John W. Payne is the Joseph J. Ruvane Jr. Professor of Business Administration at Fuqua. He is a coauthor of “A User’s Guide to Debiasing,” a chapter in The Wiley Blackwell Handbook of Judgment and Decision Making, forthcoming in 2015.
Partner Center
Thank you for visiting nature.com. You are using a browser version with limited support for CSS. To obtain the best experience, we recommend you use a more up to date browser (or turn off compatibility mode in Internet Explorer). In the meantime, to ensure continued support, we are displaying the site without styles and JavaScript.
- View all journals
- My Account Login
- Explore content
- About the journal
- Publish with us
- Sign up for alerts
- Open access
- Published: 26 June 2023
The persistence of cognitive biases in financial decisions across economic groups
- Kai Ruggeri 1 , 2 na1 ,
- Sarah Ashcroft-Jones 3 na1 ,
- Giampaolo Abate Romero Landini 4 ,
- Narjes Al-Zahli 5 , 6 ,
- Natalia Alexander 7 ,
- Mathias Houe Andersen 8 ,
- Katherine Bibilouri 6 ,
- Katharina Busch 9 ,
- Valentina Cafarelli 1 ,
- Jennifer Chen 10 ,
- Barbora Doubravová 11 ,
- Tatianna Dugué 6 ,
- Aleena Asfa Durrani 1 ,
- Nicholas Dutra 6 ,
- Eduardo Garcia-Garzon 12 ,
- Christian Gomes 6 ,
- Aleksandra Gracheva 13 ,
- Neža Grilc 14 ,
- Deniz Mısra Gürol 15 ,
- Zoe Heidenry 6 ,
- Clara Hu 6 ,
- Rachel Krasner 16 ,
- Romy Levin 16 ,
- Justine Li 17 ,
- Ashleigh Marie Elizabeth Messenger 18 ,
- Melika Miralem 19 ,
- Fredrik Nilsson 20 ,
- Julia Marie Oberschulte 21 ,
- Takashi Obi 22 ,
- Anastasia Pan 16 ,
- Sun Young Park 6 ,
- Daria Stefania Pascu 23 ,
- Sofia Pelica 24 ,
- Maksymilian Pyrkowski 25 ,
- Katherinne Rabanal 26 ,
- Pika Ranc 27 ,
- Žiga Mekiš Recek 27 ,
- Alexandra Symeonidou 28 ,
- Olivia Symone Tutuska 29 ,
- Milica Vdovic 30 ,
- Qihang Yuan 6 &
- Friederike Stock 31
Scientific Reports volume 13 , Article number: 10329 ( 2023 ) Cite this article
25k Accesses
2 Citations
533 Altmetric
Metrics details
- Human behaviour
While economic inequality continues to rise within countries, efforts to address it have been largely ineffective, particularly those involving behavioral approaches. It is often implied but not tested that choice patterns among low-income individuals may be a factor impeding behavioral interventions aimed at improving upward economic mobility. To test this, we assessed rates of ten cognitive biases across nearly 5000 participants from 27 countries. Our analyses were primarily focused on 1458 individuals that were either low-income adults or individuals who grew up in disadvantaged households but had above-average financial well-being as adults, known as positive deviants. Using discrete and complex models, we find evidence of no differences within or between groups or countries. We therefore conclude that choices impeded by cognitive biases alone cannot explain why some individuals do not experience upward economic mobility. Policies must combine both behavioral and structural interventions to improve financial well-being across populations.
Similar content being viewed by others
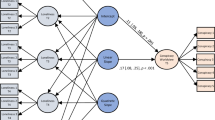
Loneliness trajectories over three decades are associated with conspiracist worldviews in midlife
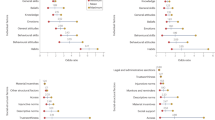
Determinants of behaviour and their efficacy as targets of behavioural change interventions
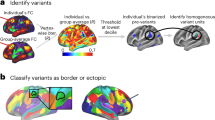
Two common and distinct forms of variation in human functional brain networks
Introduction.
Economic inequality is a direct and global barrier to upward mobility and positive socioeconomic outcomes, perpetuating negative effects for individual and population health, well-being, and sustainability 1 . In broad terms, economic mobility is a measurement of substantive change in financial well-being status, such as going from middle-class to wealthy or poverty to low-income. In this paper, economic mobility is understood beyond simply income, but through comparative financial security, such as wealth, debt, employment opportunity, and ability to withstand economic shocks.
Within countries, economic inequality continues to rise, made worse by the COVID-19 pandemic, disrupting decades of improvement in which inequality between countries had declined 2 . There are myriad established links between economic inequality and decision-making, such as how individuals from disadvantaged communities are more prone to higher rates of impulsivity 3 and temporal discounting 4 . Individual factors such as existing wealth and education are known to influence financial decision-making 5 . However, while it is widely studied that such financial behaviors may be influenced by cognitive biases (e.g., familiarity heuristics 6 , 7 , optimism 8 , proximity 8 ), there is an absence of definitive evidence whether individual decision-making ability is directly associated with upward economic mobility on a population level. There is a general view that poverty leads to attentional focus on scarcity demands, which amplifies biases such as risk aversion 9 .
However, others 10 , 11 , 12 argue that low-income individuals are not substantively different in decision-making 13 , but instead face narrower margins 14 and greater impact from their immediate environment. There is also evidence to suggest that even making generally good financial decisions can have differentiated outcomes for low-income individuals due to large transactional costs against relatively small investment gains 15 . Inequality is also associated with lower self-belief in achieving socio-economic success, diminishing the motivation to engage in behaviors associated with long-term socioeconomic growth 1 .
Behavioral interventions have attempted to reduce inequalities by informing individuals of the decisions associated with better socioeconomic outcomes. For instance, the Swedish government’s opt-out pension plan for workers facilitated better pension investment strategies 16 . Similarly, across several studies from different countries, Reñosa et al. 17 found that vaccination hesitancy was lower following simple behavioral nudges that made information more salient or were linked to incentives. In Kenya, unconditional cash transfers (UCTs) promoted better socioeconomic outcomes by enabling the coverage of immediate costs and the investment of any excess funds (e.g. in durable assets or business activities) 18 .
Unfortunately, despite some positive effects, behavioral approaches to reducing economic inequality have been largely ineffective at making substantive impacts. This may be due to measures focused on the modal person without considering marginalized groups. Consider three examples: first, the U.S. Earned Income Tax Credit aims to help low- to moderate-income workers reduce their tax burden, yet is under-subscribed by those that stand to benefit the most. When state agencies and non-profit organizations attempted established behavioral nudges to promote the utilization of and access to credits among the lowest-income families, effects were null and even linked to distrust among targeted groups 19 . Similarly, a large UCT experimental trial in the U.S. was followed by worsened subjective financial and psychological outcomes, rather than indicating positive benefits amongst recipients 20 . A conditional cash transfer program in Indonesia failed to support the needs of the lowest-income beneficiaries due to inadequate distribution of funds 21 . The incongruent effects between economic classes of such programs are a strong indication of need for new approaches.
“Positive deviance” is a framework which studies individuals from disadvantaged circumstances that experience notably better outcomes or routinely make more optimal choices than similarly disadvantaged peers 22 . Positive deviance approaches focus on understanding observed behaviors of individuals, thus lending practical policy suggestions 23 . As general interventions for improving financial well-being may inadvertently backfire among underprivileged groups 24 , considering the patterns of positive deviants may aid in developing programs with more successful impact.
While positive deviance has been identified around the world 25 , 26 , 27 , 28 no substantive work across countries and economic contexts exists to determine its viability as a frame for research or policy design. To ensure reliability, replicability, and generalizability prior to proposing a new construct for explaining behavior, there is considerable value in taking a multi-country, large-sample approach 29 . This both limits methodological biases based on sample or language 30 and presents more globalized contours of psychological and behavioral constructs 12 .
Secondary analysis of data from 60 countries 12 shows that rates of positive deviance are highly varied (Fig. 1 ), indicating a number of potential environmental and/or individual factors may contribute to population-level mobility. While most work on such economic matters will understandably focus on incomes, employment, education, and other systemic factors, how individuals make decisions under scarcity will also help develop more effective policies (in response to those failed attempts described).
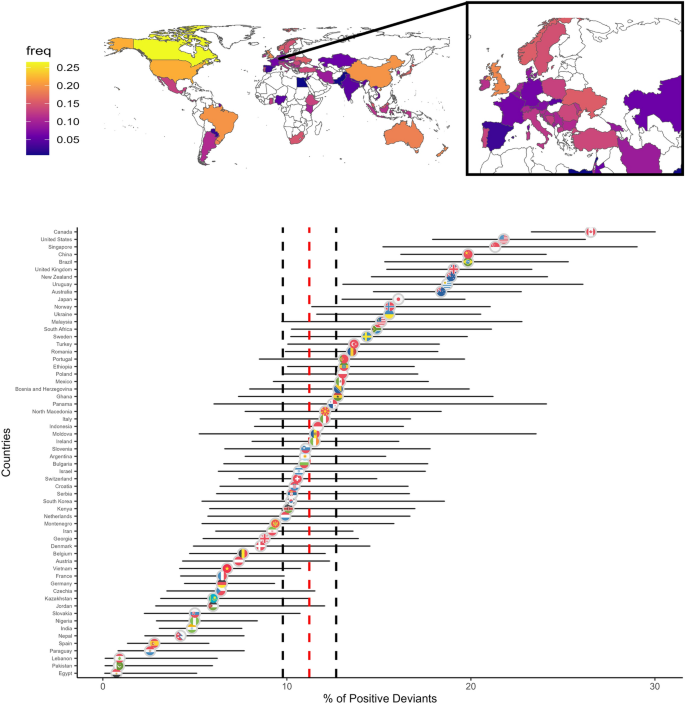
Frequency of positive deviance across country samples with a cross section on Europe, as taken from Ruggeri et al. 12 . Map generated with rnaturalearth.
One low-cost way to test potential differences in decision-making on a large scale is through cognitive biases known to influence (and harm) decision-making, particularly financial choices 31 . Assessing cognitive biases across multiple countries helps assess if patterns of preferences reflect specific environments and if choice patterns are highly similar but barriers impede consistent outcomes. If biases emerge consistently and vary between positive deviants and those who remain low-income, upward economic movement may be heavily explained by choices. If no such patterns emerge, it strongly suggests that barriers and absence of resources or opportunities are the most critical indicators of outcomes rather than unique choice patterns. Focusing our assessment on a global sample provides a robust insight compared to presenting findings from a single location and making assumptions about large applicability. With this approach, meaningful results may address economic inequalities in different settings.
The aim of this study was to test whether cognitive biases were observed at different rates between positive deviants and those who remain in disadvantaged circumstances as adults. Because of varying economic systems, we attempted to engage participants from around the world to produce a more robust first attempt at this research approach, rather than only those in similar environments. This was important in terms of added value of this research because most biases used here have primarily been tested and validated in contexts considered to meet the WEIRD (Western, Educated, Industrialized, Rich, Democratic) classification.
We expected to identify small to moderate differences in cognitive biases between positive deviants and low-income adults, looking at both the full sample as well as analyzing within each country. We also anticipated heterogeneity in differences in proportions of cognitive biases between countries. As this was the first such approach on the topic, some aspects were highly exploratory and we planned to report general patterns even if in the opposite direction than anticipated (i.e., if there were certain biases more common among positive deviants).
Ultimately, the primary research question was to understand if some individuals may overcome extremely disadvantaged financial circumstances in part due to resistance against cognitive biases that may impede optimal decision-making. If so, it may explain why some behavioral interventions aimed at reducing inequality have been unsuccessful. However, if no substantive differences exist, it would give strong evidence against the idea that individuals remain poor through choices alone. It would also indicate a more robust understanding of human behavior is necessary to develop effective policies for meaningful impact across populations.
To test our pre-registered hypotheses (osf.io/wj9yn), we ran binomial logistic regressions to predict differences in the presence of individual cognitive biases between positive deviants and low-income individuals (we mostly ignore comparisons with high-income individuals for this research, though data are available for such use). Bayesian meta-analyses were used to assess overall presentation of cognitive biases to account for potential heterogeneity within countries. Pooled Bayesian meta-analysis checked for differences among positive deviants across countries.
Across ten cognitive biases, rates observed ranged from 28.2% (temporal discounting) to 70% (ambiguity bias). On average, participants exhibited 3.23 ( SD = 1) cognitive biases. As indicated in Fig. 2 , individual biases were not highly correlated within individuals, which is why we treated them in discrete analysis rather than creating an index.
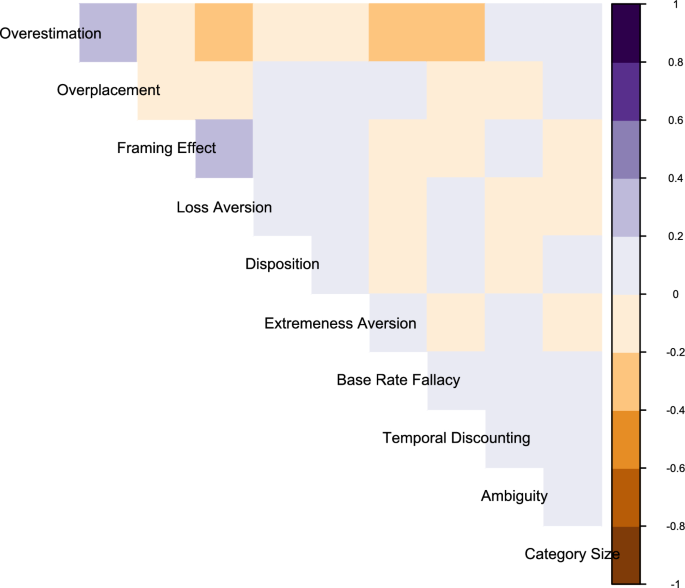
Correlation between ten biases within 3346 participants showed each bias was largely unique and not collinear with other biases assessed, with the exception of overplacement and overestimation (which rely on the presence of some biases).
Rates of cognitive biases between income groups
Chi-squared tests showed no significant differences between the rates of any of the eight cognitive biases demonstrated by low-income individuals or positive deviants, as indicated in Fig. 3 A,B (see also Supplementary Material, Table S8 ). Next, we conducted binomial logistic regressions to predict the presence of cognitive bias based on income group and country of residence. Prediction coefficients were not significant in any of ten logistic regressions; positive deviants were equally likely to exhibit cognitive bias compared to low-income individuals (see Table 1 ). As a robustness check, we also ran complementary Bayesian logistic regressions, whose results are consistent with these. We conclude that this additional analysis provides further evidence that rates of cognitive biases do not seem to differ between positive deviants and low-income adults. A table reporting credible intervals from all Bayesian logistic regressions can be found in the Supplementary Materials (Table S6 ).
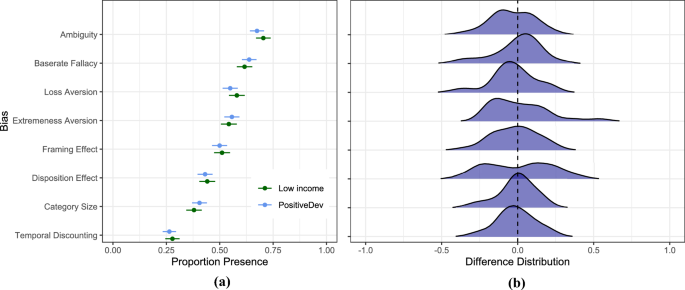
( A ) Presence of cognitive biases for low income and positive deviant participants for the pooled sample and ( B ) distribution of country mean differences in observed biases between low income participants and positive deviants. Note that overplacement and overestimation are not included as they are measured in counts rather than proportion.
To examine the optimal choice patterns between low-income and positive deviants, we calculated the mean difference between the overplacement score and number of presented biases. One-way ANOVA showed no significant difference; ( F (2) = 0.281, P = 0.755). We conducted additional ANOVAs for each country and found no significant differences between the three groups.
Because there were no substantive differences between groups, there are no additional insights to report on our second hypothesis anticipating positive deviants would show more optimal choice patterns (see Supplementary Material ).
Rates of cognitive biases between countries
Our third hypothesis expected differences in biases between countries in a way that might highlight how specific systems interacted with choice patterns. For example, recent work 12 indicated that temporal discounting is much higher in countries where inflation is extreme. In our case, we wanted to present limits and heterogeneity in differences that would be explained by local contexts, but we did not anticipate systematically different results given that not all biases should be context-dependent (e.g., category size bias). Context-dependent patterns, such as how temporal discounting rates in our data were substantially higher in Turkey, will be reported in a separate paper.
First, we found that within countries, positive deviants did not significantly differ in the probability of showing cognitive bias from either low-income, or high-income groups (see Supplementary Materials, Table S5 ). Next, we built four models (two with all countries for eight biases; two with only the countries where all 10 biases were assessed—see Selection of Countries for why seven countries were not included for overplacement and overestimation) using Bayesian meta-analysis to assess differences in probability of showing cognitive biases within countries, twice for the entire population (N = 3194) and twice with positive deviants only (N = 528). In all models, we found no significant differences in the probability of showing biases between countries (0.22 < τ < 0.43; 3.98 < SMD < 4.95; see Supplementary Materials, Table S4 ).
This study aimed to determine if rates of cognitive biases were different between positive deviants and low-income adults in a way that might explain some elements of what impedes or facilitates upward economic mobility. We anticipated finding small-to-moderate effects between groups indicating positive deviants were less prone to biases involving risk and uncertainty in financial choices. However, across a sample of nearly 5000 participants from 27 countries, of which 1458 were low-income or positive deviants, we find no evidence of any difference in the rates of cognitive biases—minor or otherwise—and no systematic variability to indicate patterns vary globally.
In sum, we find clear evidence that resistance to cognitive biases is not a factor contributing to or impeding upward economic mobility in our sample. Taken along with related work showing that temporal choice anomalies are tied more to economic environment rather than individual financial circumstances 12 , our findings are (unintentionally) a major validation of arguments (especially that of Bertrand, Mullainathan, and Shafir 11 ) stating that poorer individuals are not uniquely prone to cognitive biases that alone explain protracted poverty. It also supports arguments that scarcity 14 is a greater driver of decisions, as individuals of different income groups are equally influenced by biases and context-driven cues 13 , 32 .
What makes these findings particularly reliable is that multiple possible approaches to analyses had to be considered while working with the data, some of which were considered into extreme detail before selecting the optimal approach. As our measures were effective at eliciting biases on a scale to be expected based on existing research, and as there were relatively low correlations between individual biases (e.g., observing loss aversion in one participant is not necessarily a strong predictor of also observing any other specific bias), we conclude that there is no evidence from our sample to support that biases are directly associated with potentially harming optimal choices uniquely amongst low-income individuals.
Of course, though our sample was reasonably well powered, it is possible that our focus on two subsets of the overall population may have been too small to detect small effects. First, some perspective on this may be useful: ensuring that 17% of our sample met the criteria for being positive deviants indicated that our recruitment strategy was effective at finding a sufficient number of participants for a small (by rule) group within a population. When using existing datasets, this can yield as low as 1% 22 . However, since we did not want to over-represent a group, but instead have a reasonable reflection of groups while also sufficient samples for analyses, we were satisfied that overall we had over 750 participants meeting the criteria out of a total sample of nearly 5000. With that said, future work may wish to focus on expanding the sample of low income or positive deviant groups in case large samples yield small but significant effects. Given the consistency of our null findings, however, we do not speculate a likelihood for this.
We do not argue that behavior has no link to individuals overcoming or remaining in negative financial circumstances. On the contrary, it is very evident that biases do exist despite income levels, and that targeting those may be beneficial. However, we argue that further work is particularly necessary to understand why similar choice patterns do not lead to similar outcomes. If those patterns were validated and still produced differential outcomes, it would likely be a result of substantial system barriers and scarcity of opportunities 11 , 33 . If validated, it would provide even stronger arguments toward investment in substantive structural changes to reducing inequality, rather than assuming that individual changes can alone overcome broader barriers 34 . This again does not mean there is no place for individually targeted behavioral interventions, but that they should be developed in combination with those that involve addressing systems and barriers 35 .
Limitations
This is one of the first large-scale studies on positive deviance tested between countries and using cognitive biases as a frame. Our approach is therefore limited by not having been previously validated and used items that only superficially elicit biases but not necessarily reflect behaviors in real-world settings. Also, frames used may not have been truly reflecting biases but simply a random preference set based on the options given. This was evident in the intended items on mental accounting, which were removed after the study began based on a later determination that the items did not measure the intended choice pattern as written. However, it may also be true of the category size bias measure, which showed essentially a 50-50 behavior and may not be especially useful.
We are also limited in how we identified income groups, both due to the self-report nature and that participants were typically higher income as adults. This may also be collinear with the number of positive deviants that identified as immigrants: if they were born in low-income communities but migrated to a high-income country, whether they should qualify as positive deviants may be up for further debate. Similarly, we only measure a narrow set of biases, which are each tested discretely, rather than in combination (or controlling for) other factors such as personality, resilience, numeracy, personal beliefs (e.g., political or religious), or financial literacy. Future work may find that factoring in these aspects may elucidate different patterns.
Given our findings, one advantage of this approach is that there does not appear to be a need for longitudinal study on if or when positive deviants shift decision-making styles. That approach is typically recommended in static studies, where it is unclear if choices would have been the same prior to achieving financial wealth. Our findings indicate this may not be mandatory. However, we have attempted to avoid speaking to absolutely generalizability from our findings. Though we have a large and diverse sample, as the first study of this type and a sample that was intentionally not representative in order to engage many low-income participants, we strongly encourage further, multi-site studies to validate (or refute) our findings.
We sought to determine if individuals that had overcome low-income childhoods showed significantly different rates of cognitive biases from individuals that remained low-income as adults. We comprehensively reject our initial hypotheses and conclude that outcomes are not tied—at least not exclusively or potentially even meaningfully—to resistance to cognitive biases. Our research does not reject the notion that individual behavior and decision-making may directly relate to upward economic mobility. Instead, we narrowly conclude that biased decision-making does not alone explain a significant proportion of population-level economic inequality. Thus, any attempts to reduce economic inequality must involve both behavioral and structural aspects. Otherwise, similar decisions between disadvantaged individuals may not lead to similar outcomes. Where combined effectively, it will be possible to assess if genuine impact has been made on the financial well-being of individuals and populations.
Ethical approval for this research was given by the Institutional Review Board at Columbia University. All methods were carried out following relevant guidelines and regulations. All country surveys were provided in at least one primary local language, as well as screened for cultural appropriateness, flow, and overall quality. Each participant provided informed consent to participate in the study. All materials and methods followed our pre-registered plan (osf.io/wj9yn), except for certain deviations, which are described later. Further details are provided in the Supplementary Materials .
Selection of countries
There was no systematic approach to country inclusion, but we explicitly emphasized including some countries that are not typically represented in behavioral research. Countries were essentially chosen based on locations and languages where study volunteers were capable of recruiting substantive samples ethically (i.e., with reasonable oversight and appropriate methods). This means selection was not entirely at random, but there was no specific guiding criterion in which countries were included apart from representation in the study team. No country was added based on any unique factors, such as wealth, economic systems, or idiosyncratic contexts.
Following data collection, 27 countries were fully included, using 22 languages. Two countries were attempted but were unable to fulfill certain tasks or were removed for ethical concerns. Several countries (Bosnia and Herzegovina, Chile, Germany, Italy Sweden, Turkey, and the United States) were part of preliminary work in developing the full study. Participants in those countries answered slightly more questions, some of which were removed for the full study. For this reason, those countries are not included in the overestimation and overplacement analyses, as participants in those countries saw slightly different versions of the items.
Translations
All survey instruments utilized forward-and-back translations for all countries in their primary language. At least one native speaker was involved throughout each process, requiring translation into local currencies (and cost standards) as well as applicable aspects such as race, education, and employment reporting standards. In some countries, varying demographic measures were modified for cultural and ethical appropriateness. Guidelines for race and ethnicity were observed in countries with specific rules, such as where racial identity questions are regulated or prohibited. Additional details and full surveys for each country can be found under the pre-registration link (osf.io/wj9yn).
To measure cognitive biases with implications for decision-making in financial situations, we used 15 decision items that assessed 10 cognitive biases. These items were selected following preliminary data from a parallel study that was pre-registered using the Open Science Framework (osf.io/hmk9s) prior to data collection. Following an exhaustive process in which a large number of biases were reviewed from multiple scientific repositories, biases used in this study were ultimately selected on several criteria. Biases had to be directly relevant to financial decision-making, sufficient at eliciting cognitive biases in a large sample using simple discrete choice methods, and not require long or complex statements. The final list of biases used was the ambiguity effect 36 , base rate fallacy 37 , category size bias 38 , extremeness aversion 39 , disposition effect 40 , temporal discounting 12 , overplacement bias 41 , overestimation bias 42 , framing effect 43 , and loss aversion 44 . The pre-registered study mentioned earlier details additional biases that were piloted separately from this study, but removed for lack of sufficiently meeting these criteria.
Biases and their associated items were also selected specifically to meet certain practical criteria related to ease of understanding and avoiding complications related to translations. For example, we did not use vignettes or lengthy statements on scenarios to present choices. Instead, we used the most direct and singular approaches that were possible. While this was not always perfectly doable, some potential measures were excluded if they were deemed to be overly complicated or if the specific aspects might have been unfamiliar to most participants. This was particularly true for items that would have presented complex financial options only known to financially active individuals. Finally, we did not select items that would implicitly or explicitly appear to relate to poverty on inequality. Instead, we chose items that would be relevant to any economic class, in a way that may elicit any differences in choice patterns between groups if such differences would explain differential economic outcomes.
Financial values were adapted to local currencies and income standards (see: osf.io/wj9yn for the information on financial values and supplementary information on them) The survey also includes employment, bill management, income, debit and credit circumstances, and socioeconomic status as a child. We also collect age, gender, education level, parent education level, race, and ethnicity (where permitted and appropriate).
Participant recruitment utilized Qualtrics surveying software to collect data. Most participants were recruited using the Demić-Većkalov method 12 , which included posting links on discussion threads and online news articles (social media, popular forums, and news websites). We also implemented the Jarke method of identifying popular communication media associated with specific groups that were not represented (e.g., rugby forums on social media to recruit males from New Zealand). The survey was also circulated to local non-governmental and non-profit organizations, and for-profit corporations to generate informal “snowballing.” Some participants were recruited by convenience sampling. Only residents of Japan were compensated (less than US$1 total). This approach helped to minimize sample bias across countries and generate diverse backgrounds among participants, with the main exception of mostly including populations with direct internet access and social media accounts.
Because this study requires internet access and largely relies on visibility on popular (but not universally used) platforms, the team made concerted efforts to make direct contact with organizations, institutions, and government agencies to recruit participants through different media. Some of these methods included contacting Human Resource officers at large employers in different countries and specifically requesting circulation among individuals from lower-income backgrounds. We also communicated with a number of NGOs and non-profits to see if they would recruit community members as participants if they visited their sites in order to use computers or access the internet.
After confirming eligibility and giving consent, participants were presented 15 binary choice scenarios. For example, to measure category size biases, participants were asked to choose if they would prefer a scenario with one winning ticket out of 10 or 10 winning tickets out of 100 (see Supplement Table S9 ). Decision-making items were shown in a randomized order, except for choices that required a specific sequence (such as overestimation being required to appear last). Financial and demographic questions came at the end of the survey. The median duration to complete the longer version of the instrument was 14.41 min (from 13.67 min in the US to 18.07 min in Chile). The median duration to complete the shorter version of the instrument was 9.15 min (from 7.45 min in Canada to 16.55 min in Pakistan).
Participants
The final dataset consisted of 4958 (46.2% women) responses from 27 countries, ranging from 62 responses in Peru to 380 responses in the U.S. Gender participation was hugely varied, with women making up as few as 21.3% of participants in France, to 82.2% in Bosnia and Herzegovina. The median age of the entire sample was 38 (median of 34 in two countries to 46 in two countries). Of all participants, 78.8% had completed higher education. Most participants (71.4%) were employed full-time. Across countries, 30.1% of participants came from below-average or poor households, ranging from 17.5% in Pakistan to 51.6% in Peru. We then excluded participants with entries that did not align with our pre-registration requirements and tracked these changes in our “Exclusion Table” (Supplementary Materials, Table S3 ) which displays total participants removed and percent rate of change in each country. Comprehensive details on data inclusion are provided in the Supplementary Materials (Table S7 a,b).
Classification
The classification employed in the paper—positive deviants, low-income participants, and above average participants—is based on survey questions eliciting (1) participants’ financial situation in the household they grew up in, (2) their current income, (3) national income data from participant country of residence, and (4) the sample spread of income data from participant country of residence. Positive deviants are defined as adults who reported growing up in low-income households but who demonstrate a reasonable level of financial wellness in adulthood.
Specifically, to define the cut-off point, we calculated the midpoint between the average national income in each country and the median income within our country samples. This was done so that the cut off did not rely solely on nationally reported averages from each country, as these come from different sources and may not account for recent economic changes such as high rates of inflation.
As a result, our midpoint line sits above the national average and below our sample median. Positive deviants are thus defined as adults who reported growing up in low-income households and whose income falls above this line. Low-income individuals are also adults who reported growing up in low-income households but whose income falls below this line, which means that they started off in a low-income environment but were not able to achieve significantly higher incomes as adults. Everyone else was classified as above average and excluded from the analyses unless otherwise specified.
Deviations from pre-registered plan
Due to the complexity of the study, primarily based on including countries with entirely different economic systems and standards, recent extreme inflation and related taxation policies, as well as differing availability of reliable income estimates, several critical adjustments had to be made, though did not appear to impact outcomes. We removed the income buffer zone (40th to 50th percentile) for individuals that were born low-income, as this was determined to only be appropriate in a small number of countries and would have resulted in excluding many entirely legitimate participants from analyses. We also excluded mental accounting as, after starting data collection, it was agreed that our measures simply did not test for nor elicit this bias. For posterity and any future attempts to utilize these data, we provide a comprehensive and annotated source and decision table for all countries, which will be posted with all pre-registration material, code, and data.
Data availability
All data will be posted open access via https://psyarxiv.com/mrxy6/ and in interactive form via https://public.tableau.com/app/profile/kai.ruggeri . We will post these only once an accepted version of all analyses is possible to avoid confusion based on version control.
Browman, A. S., Destin, M., Kearney, M. S. & Levine, P. B. How economic inequality shapes mobility expectations and behaviour in disadvantaged youth. Nat. Hum. Behav. 3 , 214–220 (2019).
Article PubMed Google Scholar
Jetten, J. et al. Consequences of economic inequality for the social and political vitality of society: A social identity analysis. Polit. Psychol. 42 , 241–266 (2021).
Article Google Scholar
Williams, K. E. G., Sng, O. & Neuberg, S. L. Ecology-driven stereotypes override race stereotypes. Proc. Natl. Acad. Sci. 113 , 310–315 (2016).
Article ADS CAS PubMed Google Scholar
Falk, A. et al. Global evidence on economic preferences. Q. J. Econ. 133 , 1645–1692 (2018).
Calvet, L. E., Campbell, J. Y. & Sodini, P. Down or out: Assessing the welfare costs of household investment mistakes. J. Polit. Econ. 115 , 707–747 (2007).
Huberman, G. Familiarity breeds investment. Rev. Financ. Stud. 14 , 659–680 (2001).
Massa, M. & Simonov, A. Hedging, familiarity and portfolio choice. Rev. Financ. Stud. 19 , 633–685 (2006).
Branikas, I., Hong, H. & Xu, J. Location choice, portfolio choice. J. Financ. Econ. 138 , 74–94 (2020).
de Bruijn, E.-J. & Antonides, G. Poverty and economic decision making: A review of scarcity theory. Theory Decis. 92 , 5–37 (2022).
Article MathSciNet MATH Google Scholar
Blank, R. M. & Barr, M. S. Insufficient Funds: Savings, Assets, Credit, and Banking Among Low-Income Households (Russell Sage Foundation, 2009).
Google Scholar
Bertrand, M., Mullainathan, S. & Shafir, E. A behavioral-economics view of poverty. Am. Econ. Rev. 94 , 419–423 (2004).
Ruggeri, K. et al. The globalizability of temporal discounting. Nat. Hum. Behav. https://doi.org/10.1038/s41562-022-01392-w (2022).
Article PubMed PubMed Central Google Scholar
Plantinga, A., Krijnen, J. M. T., Zeelenberg, M. & Breugelmans, S. M. Evidence for opportunity cost neglect in the poor. J. Behav. Decis. Mak. 31 , 65–73 (2018).
Shah, A. K., Shafir, E. & Mullainathan, S. Scarcity frames value. Psychol. Sci. 26 , 402–412 (2015).
Vissing-Jorgensen, A. Towards an explanation of household portfolio choice heterogeneity: Nonfinancial income and participation cost structures. Working Paper at https://doi.org/10.3386/w8884 (2002).
Cronqvist, H., Thaler, R. H. & Yu, F. When nudges are forever: Inertia in the Swedish Premium Pension Plan. AEA Pap. Proc. 108 , 153–158 (2018).
Reñosa, M. D. C. et al. Nudging toward vaccination: A systematic review. BMJ Glob. Health 6 , e006237 (2021).
Haushofer, J. & Shapiro, J. The short-term impact of unconditional cash transfers to the poor: Experimental evidence from Kenya. Q. J. Econ. 131 , 1973 (2016).
Linos, E., Prohofsky, A., Ramesh, A., Rothstein, J. & Unrath, M. Can nudges increase take-up of the EITC?: Evidence from multiple field experiments. Am. Econ. J. Econ. Policy https://doi.org/10.1257/pol.20200603 (2022).
Jaroszewicz, A., Jachimowicz, J., Hauser, O. & Jamison, J. How effective is (more) money? Randomizing unconditional cash transfer amounts in the US. SSRN Scholarly Paper https://doi.org/10.2139/ssrn.4154000
Hadna, A. H. & Askar, M. W. The impact of conditional cash transfers on low-income individuals in Indonesia. Adv. Southeast Asian Stud. 15 , 23–41 (2022).
Ruggeri, K. & Folke, T. Unstandard deviation: The untapped value of positive peviance for reducing inequalities. Perspect. Psychol. Sci. 17 , 711–731 (2022).
Marsh, D. R., Schroeder, D. G., Dearden, K. A., Sternin, J. & Sternin, M. The power of positive deviance. BMJ 329 , 1177–1179 (2004).
Sussman, A. B. & O’Brien, R. L. Knowing when to spend: Unintended financial consequences of earmarking to encourage savings. J. Mark. Res. 53 , 790–803 (2016).
Mackintosh, U. A. T., Marsh, D. R. & Schroeder, D. G. Sustained positive deviant child care practices and their effects on child growth in Viet Nam. Food Nutr. Bull. 23 , 18–27 (2002).
Sripaipan, T. et al. Effect of an integrated nutrition program on child morbidity due to respiratory infection and diarrhea in Northern Viet Nam. Food Nutr. Bull. 23 , 67–74 (2002).
Chavez, A. & Martinez, C. Growing up in a Developing Community: A Bio-ecologic Study of the Development of Children from Poor Peasant Families in Mexico (Instituto Nacional de la Nutrición, 1982).
Roche, M. L. et al. A community-based positive deviance/hearth infant and young child nutrition intervention in Ecuador improved diet and reduced underweight. J. Nutr. Educ. Behav. 49 , 196-203.e1 (2017).
Yarkoni, T. The generalizability crisis. Behav. Brain Sci. https://doi.org/10.1017/S0140525X20001685 (2020).
Ghai, S. It’s time to reimagine sample diversity and retire the WEIRD dichotomy. Nat. Hum. Behav. 5 , 971–972 (2021).
Costa, D. F., de Melo Carvalho, F., de Melo Moreira, B. C. & do Prado, J. W. Bibliometric analysis on the association between behavioral finance and decision making with cognitive biases such as overconfidence, anchoring effect and confirmation bias. Scientometrics 111 , 1775–1799 (2017).
Hilbert, L. P., Noordewier, M. K. & van Dijk, W. W. Financial scarcity increases discounting of gains and losses: Experimental evidence from a household task. J. Econ. Psychol. 92 , 102546 (2022).
Ruggeri, K. et al. Nudging New York: Adaptive models and the limits of behavioral interventions to reduce no-shows and health inequalities. BMC Health Serv. Res. 20 , 363 (2020).
Hagger, M. S. & Weed, M. DEBATE: Do interventions based on behavioral theory work in the real world?. Int. J. Behav. Nutr. Phys. Act. 16 , 36 (2019).
Chater, N. & Loewenstein, G. The i-frame and the s-frame: How focusing on individual-level solutions has led behavioral public policy astray. Behav. Brain Sci. https://doi.org/10.1017/S0140525X22002023 (2022).
Ellsberg, D. Risk, ambiguity, and the savage axioms. Q. J. Econ. 75 , 643–669 (1961).
Bar-Hillel, M. The base-rate fallacy in probability judgments. Acta Psychol. (Amst.) 44 , 211–233 (1980).
Isaac, M. S. & Brough, A. R. The Category size bias and consumers’ perceptions of risk. ACR North Am. Adv. NA-40 , (2012).
Simonson, I. & Tversky, A. Choice in context: Tradeoff contrast and extremeness aversion. J. Market. Res. 29 , 281–295 (1992).
Weber, M. & Camerer, C. F. The disposition effect in securities trading: An experimental analysis. J. Econ. Behav. Organ. 33 , 167–184 (1998).
Moore, D. A. & Healy, P. J. The trouble with overconfidence. Psychol. Rev. 115 , 502–517 (2008).
Prims, J. P. & Moore, D. A. Overconfidence over the lifespan. Judgm. Decis. Mak. 12 , 29–41 (2017).
Kühberger, A., Schulte-Mecklenbeck, M. & Perner, J. Framing decisions: Hypothetical and real. Organ. Behav. Hum. Decis. Process. 89 , 1162–1175 (2002).
Tversky, A. & Kahneman, D. The framing of decisions and the psychology of choice. Science 211 , 453–458 (1981).
Article ADS MathSciNet CAS PubMed MATH Google Scholar
Download references
Acknowledgements
We thank the Junior Researcher Programme, Global Behavioral Science (GLOBES), Department of Psychology, Columbia University; the Centre for Business Research, Judge Business School, University of Cambridge; Corpus Christi College, Cambridge; and Dr Ceren Sönmez, Michal Goldstein, Abby Yucht, and Anastasia Gracheva. This research was supported in part by the National Science Foundation (#2218595) and by Undergraduate Global Engagement at Columbia University. Additional support was provided to individual researchers from the Columbia University Office of the Provost, Masaryk University Centre for International Cooperation, and the Benjamin A. Gilman International Fund from the United States Department of State. This research was funded in part, by the UKRI [MR/N013468/1]. For the purpose of Open Access, the author has applied a CC BY public copyright licence to any Author Accepted Manuscript version arising from this submission.
Author information
These authors contributed equally: Kai Ruggeri and Sarah Ashcroft-Jones.
Authors and Affiliations
Department of Health Policy and Management, Columbia University, 722 W 168th Street, New York, NY, 10032, USA
Kai Ruggeri, Valentina Cafarelli & Aleena Asfa Durrani
Centre for Business Research, Judge Business School, University of Cambridge, Cambridge, CB2 1AG, UK
Kai Ruggeri
Department of Experimental Psychology, University of Oxford, Oxford, UK
Sarah Ashcroft-Jones
Department of Psychology, University of Padua, Via VIII Febbraio, 35122, Padua, PD, Italy
Giampaolo Abate Romero Landini
Department of Computer Science, Columbia University, 500 W 120th Street, New York, NY, 10027, USA
Narjes Al-Zahli
Department of Psychology, Columbia University, 1180 Amsterdam Avenue, New York, NY, 10027, USA
Narjes Al-Zahli, Katherine Bibilouri, Tatianna Dugué, Nicholas Dutra, Christian Gomes, Zoe Heidenry, Clara Hu, Sun Young Park & Qihang Yuan
Columbia University, 116th and Broadway, New York, NY, 10027, USA
Natalia Alexander
Department of Psychology and Behavioural Sciences, Aarhus University, Bartholins Allé 11, 8000, Aarhus, Denmark
Mathias Houe Andersen
German Center for Addiction Research in Childhood and Adolescence, University Medical Center Hamburg-Eppendorf, Martinistr 52, 20246, Hamburg, Germany
Katharina Busch
Department of Economics, Columbia University, 420 W 118th Street, New York, NY, 10027, USA
Jennifer Chen
Department of Psychology, Faculty of Social Studies, Masaryk University, Joštova 218/10, 60200, Brno, Czech Republic
Barbora Doubravová
Department of Health, Universidad Camilo José Cela, Madrid, Spain
Eduardo Garcia-Garzon
Department of Political Science, Columbia University, 420 W 118th Street, New York, NY, 10027, USA
Aleksandra Gracheva
Department of Life Sciences, University of Roehampton, Whitelands College, London, SW15 4JD, UK
Department of Psychology, Koc University, 34349, Istanbul, Turkey
Deniz Mısra Gürol
Department of Psychology, Barnard College, Columbia University, 3009 Broadway, New York, NY, 10027, USA
Rachel Krasner, Romy Levin & Anastasia Pan
Department of Biological Sciences, Columbia University, 1212 Amsterdam Avenue, New York, NY, 10027, USA
Department of Psychology, University of Stirling, Stirling, Scotland, FK9 4L, UK
Ashleigh Marie Elizabeth Messenger
Lund University, Lund, Sweden
Melika Miralem
Department of Clinical Neuroscience, Division of Psychology, Karolinska Institutet, Solna, 171 77, Stockholm, Sweden
Fredrik Nilsson
Department of Psychology, Ludwig-Maximilians-Universität München, Munich, Germany
Julia Marie Oberschulte
Department of Public Administration, Columbia University, 420 West 118th Street, New York, NY, 10027, USA
Takashi Obi
Department of Developmental Psychology and Socialisation, University of Padua, Via Venezia 12, 35131, Padua, PD, Italy
Daria Stefania Pascu
Department of Social and Organizational Psychology, Iscte-University Institute of Lisbon, Avenida das Forças Armadas, 1649-026, Lisbon, Portugal
Sofia Pelica
SWPS University, Chodakowska 19/31, Warsaw, Poland
Maksymilian Pyrkowski
Department of Cognitive Science, Columbia University, 116th & Broadway, New York, NY, 10027, USA
Katherinne Rabanal
Department of Psychology, University of Ljubljana, Aškerčeva Cesta 2, 1000, Ljubljana, Slovenia
Pika Ranc & Žiga Mekiš Recek
Department of Clinical Psychology, Leiden University, Wassenaarseweg 52, 2333 AK, Leiden, South Holland, The Netherlands
Alexandra Symeonidou
Department of Sociology, Columbia University, 606 W 122nd Street, New York, NY, 10027, USA
Olivia Symone Tutuska
Department of Psychology, Faculty of Media and Communications, Singidunum University, Karadjordjeva 65, Belgrade, 11000, Serbia
Milica Vdovic
University of Cologne, Albertus-Magnus-Platz, 50923, Cologne, Germany
Friederike Stock
You can also search for this author in PubMed Google Scholar
Contributions
K.R. and S.A.J. were were the lead authors for all aspects. The rest of the team shared equally in instrument building, translation, data collection, analysis, and writing. K.R. coordinated revisions and was responsible for administrative aspects. V.C. was heavily involved in revisions as well.
Corresponding author
Correspondence to Sarah Ashcroft-Jones .
Ethics declarations
Competing interests.
The authors declare no competing interests.
Additional information
Publisher's note.
Springer Nature remains neutral with regard to jurisdictional claims in published maps and institutional affiliations.
Supplementary Information
Supplementary information., rights and permissions.
Open Access This article is licensed under a Creative Commons Attribution 4.0 International License, which permits use, sharing, adaptation, distribution and reproduction in any medium or format, as long as you give appropriate credit to the original author(s) and the source, provide a link to the Creative Commons licence, and indicate if changes were made. The images or other third party material in this article are included in the article's Creative Commons licence, unless indicated otherwise in a credit line to the material. If material is not included in the article's Creative Commons licence and your intended use is not permitted by statutory regulation or exceeds the permitted use, you will need to obtain permission directly from the copyright holder. To view a copy of this licence, visit http://creativecommons.org/licenses/by/4.0/ .
Reprints and permissions
About this article
Cite this article.
Ruggeri, K., Ashcroft-Jones, S., Abate Romero Landini, G. et al. The persistence of cognitive biases in financial decisions across economic groups. Sci Rep 13 , 10329 (2023). https://doi.org/10.1038/s41598-023-36339-2
Download citation
Received : 11 October 2022
Accepted : 01 June 2023
Published : 26 June 2023
DOI : https://doi.org/10.1038/s41598-023-36339-2
Share this article
Anyone you share the following link with will be able to read this content:
Sorry, a shareable link is not currently available for this article.
Provided by the Springer Nature SharedIt content-sharing initiative
By submitting a comment you agree to abide by our Terms and Community Guidelines . If you find something abusive or that does not comply with our terms or guidelines please flag it as inappropriate.
Quick links
- Explore articles by subject
- Guide to authors
- Editorial policies
Sign up for the Nature Briefing newsletter — what matters in science, free to your inbox daily.

"Lessons From Everest: The Interaction of Cognitive Bias, Psychological Safety, and System Complexity"
What went wrong on Mount Everest on May 10, 1996? That day, twenty-three climbers reached the summit. Five climbers, however, did not survive the descent. Two of these, Rob Hall and Scott Fischer, were extremely skilled team leaders with much experience on Everest. As the world's mightiest mountain, Everest has never been a cakewalk: 148 people have lost their lives attempting to reach the summit since 1922.
Newspaper and magazine articles and booksmost famously, Jon Krakauer's Into Thin Air: A Personal Account of the Mount Everest Disaster have attempted to explain how events got so out of control that particular day. Several explanations compete: human error, weather, all the dangers inherent in human beings pitting themselves against the world's most forbidding peak.
A single cause of the 1996 tragedy may never be known, says HBS professor Michael A. Roberto . But perhaps the events that day hold lessons, some of them for business managers. Roberto's new working paper describes how. Here follows an excerpt from "Lessons From Everest: The Interaction of Cognitive Bias, Psychological Safety, and System Complexity."
Implications for leaders This multi-lens analysis of the Everest case provides a framework for understanding, diagnosing, and preventing serious failures in many types of organizations. However, it also has important implications for how leaders can shape and direct the processes through which their organizations make and implement high-stakes decisions. The Everest analysis suggests that leaders must pay close attention to how they balance competing pressures in their organizations, and how their words and actions shape the perceptions and beliefs of organization members. In addition, the case provides insight regarding how firms approach learning from past failures.
Balancing competing forces The Everest case suggests that leaders need to engage in a delicate balancing act with regard to nurturing confidence, dissent, and commitment within their organizations. First, executives must strike a balance between overconfidence on the one hand and insufficient confidence on the other. Leaders must act decisively when faced with challenges, and they must inspire others to do so as well. A lack of confidence can enhance anticipatory regret, or the apprehension that individuals often experience prior to making a decision. High levels of anticipatory regret can lead to indecision and costly delays. 71 This anxiety can be particularly problematic for executives in fast-moving industries. Successful management teams in turbulent industries develop certain practices to cope with this anxiety. For instance, some leaders develop the confidence to act decisively in the face of considerable ambiguity by seeking the advice of one or more "expert counselors," i.e. highly experienced executives who can serve as a confidante and a sounding board for various ideas. 72 Naturally, too much confidence can become dangerous as well, as the Everest case clearly demonstrates. To combat overconfidence, leaders must seek out information that disconfirms their existing views, and they should discourage subordinates from hiding bad news. Leaders also must take great care to separate facts from assumptions, and they must encourage everyone to test critical assumptions vigorously to root out overly optimistic projections.
Fostering constructive dissent poses another challenge for managers. As we see in the Everest case, insufficient debate among team members can diminish the extent to which plans and proposals undergo critical evaluation. Flawed ideas remain unchallenged, and creative alternatives are not generated. On the other hand, when leaders arrive at a final decision, they need everyone to accept the outcome and support its implementation. They cannot allow continued dissension to disrupt the effort to turn that decision into action. As Cyrus the Great once said, leaders must balance the need for "diversity in counsel, unity in command." To accomplish this, leaders must insure that each participant has a fair and equal opportunity to voice their opinions during the decision process, and they must demonstrate that they have considered those views carefully and genuinely. Moreover, they must clearly explain the rationale for their final decision, including why they chose to accept some input and advice while rejecting other suggestions. 73 By doing so, leaders can encourage divergent thinking while building decision acceptance.
Finally, leaders must balance the need for strong buy-in against the danger of escalating commitment to a failing course of action over time. To implement effectively, managers must foster commitment by providing others with ample opportunities to participate in decision making, insuring that the process is fair and legitimate, and minimizing the level of interpersonal conflict that emerges during the deliberations. Without strong buy-in, they risk numerous delays including efforts to re-open the decision process after implementation is underway. However, leaders must be aware of the dangers of over-commitment to a flawed course of action, particularly after employees have expended a great deal of time, money, and effort. The ability to "cut your losses" remains a difficult challenge as well as a hallmark of courageous leadership. Simple awareness of the sunk cost trap will not prevent flawed decisions. Instead, leaders must be vigilant about asking tough questions such as: What would another executive do if he assumed my position today with no prior history in this organization? 74 Leaders also need to question themselves and others repeatedly about why they wish to make additional investments in a particular initiative. Managers should be extremely wary if they hear responses such as: "Well, we have put so much money into this already. We don't want to waste all of those resources." Finally, leaders can compare the benefits and costs of additional investments with several alternative uses of those resources. By encouraging the consideration of multiple options, leaders may help themselves and others recognize how over-commitment to an existing project may be preventing the organization from pursuing other promising opportunities.
Shaping perceptions and beliefs The Everest case also demonstrates how leaders can shape the perceptions and beliefs of organization members, and thereby affect how these individuals will interact with one another and with their leaders in critical situations. Hall and Fischer made a number of seemingly minor choices about how the teams were structured that had an enormous impact on people's perceptions of their roles, status, and relationships with other climbers. Ultimately, these perceptions and beliefs constrained the way that people behaved when the groups encountered serious obstacles and dangers.
Leaders can shape the perceptions and beliefs of others in many ways. In some cases, the leaders' words or actions send a clear signal as to how they expect people to behave. For instance, Hall made it very clear that he did not wish to hear dissenting views while the expedition made the final push to the summit. Most leaders understand the power of these very direct commands or directives. However, this case also demonstrates that leaders shape the perceptions and beliefs of others through subtle signals, actions, and symbols. For example, the compensation differential among the guides shaped people's beliefs about their relative status in the expedition. It is hard to believe that the expedition leaders recognized that their compensation decisions would impact perceptions of status, and ultimately, the likelihood of constructive dissent within the expedition teams. Nevertheless, this relatively minor decision did send a strong signal to others in the organization. The lesson for managers is that they must recognize the symbolic power of their actions and the strength of the signals they send when they make decisions about the formation and structure of work teams in their organizations.
Learning from failure Often, when an organization suffers a terrible failure, others attempt to learn from the experience. Trying to avoid repeating the mistakes of the past seems like an admirable goal. Naturally, some observers attribute the poor performance of others to human error of one kind or another. They blame the firm's leaders for making critical mistakes, at times even going so far as to accuse them of ignorance, negligence, or indifference. Attributing failures to the flawed decisions of others has certain benefits for outside observers. In particular, it can become a convenient argument for those who have a desire to embark on a similar endeavor. By concluding that human error caused others to fail, ambitious and self-confident managers can convince themselves that they will learn from those mistakes and succeed where others did not. 75
This research demonstrates a more holistic approach to learning from large-scale organizational failures. It suggests that we cannot think about individual, group, and organizational levels of analysis in isolation. Instead, we need to examine how cognitive, interpersonal, and systemic forces interact to affect organizational processes and performance. System complexity, team structure and beliefs, and cognitive limitations are not alternative explanations for failures, but rather complementary and mutually reinforcing concepts.
Business executives and other leaders typically recognize that equifinality characterizes many situations. In other words, most leaders understand that there are many ways to arrive at the same outcome. Nevertheless, we have a natural tendency to blame other people for failures, rather than attributing the poor performance to external and contextual factors. 76 We also tend to pit competing theories against one another in many cases, and try to argue that one explanation outperforms the others. The Everest case suggests that both of these approaches may lead to erroneous conclusions and reduce our capability to learn from experience. We need to recognize multiple factors that contribute to large-scale organizational failures, and to explore the linkages among the psychological and sociological forces involved at the individual, group, and organizational system level. In sum, all leaders would be well-served to recall Anatoli Boukreev's closing thoughts about the Everest tragedy: "To cite a specific cause would be to promote an omniscience that only gods, drunks, politicians, and dramatic writers can claim." 77
Excerpted with permission from the working paper "Lessons From Everest: The Interaction of Cognitive Bias, Psychological Safety, and System Complexity," Michael A. Roberto , 2002.
All images © Eyewire unless otherwise indicated.
Five Questions for Michael A. Roberto
Why study Mount Everest? Professor Roberto described what managers can learn from mountain climbing in an e-mail interview with HBS Working Knowledge senior editor Martha Lagace.
Lagace: In your new research, you tried to learn from a tragic episode on Mount Everest. You've applied a variety of theories from management to study why events on May 10, 1996 went horribly wrong. What interested you in the Everest case, and why did you decide to delve further using the tools of management?
Roberto: When I read Jon Krakauer's best-selling account of this tragedy, entitled Into Thin Air , I became fascinated with the possibility of using this material as a tool for teaching students about high-stakes decision-making. After all, here you had two of the most capable and experienced high altitude climbers in the world, and they both perished during one of the deadliest days in the mountain's history. It struck me that the disastrous consequences had more to do with individual cognition and group dynamics than with the tactics of mountain climbing.
In addition, I am always searching for material from outside of the business environment that can be used in our classrooms at HBS. I believe that there are important lessons that we can learn by examining case studies from other fields. Students find the material refreshing, and they enjoy trying to learn about management by studying experts in other domains.
Q: In hindsight, it is very easy to point a finger and assign blame to individuals involved in the climb. You resist that temptation. Why?
A: If we simply attribute the tragedy to the inadequate capabilities of a few climbers, then we have missed an opportunity to identify broader lessons from this episode. Many of us often fall into the trap of saying to ourselves, "That could never happen to me," when we observe others fail. The fact is that there may be powerful reasons why many people would fail under similar circumstances. It seemed that this might be the case here, and that's what motivated me to consider several different conceptual explanations for the tragedy.
Q: Overconfidence, an unwillingness to "cut one's losses," and a reliance on the most recent information are all psychological factors that can play into high-stakes decisions. You suggest that people dealing with riskbe they expedition leaders or executivesare very susceptible to these emotions. How might they have applied on Mount Everest that day?
A: First and foremost, I would advocate strict adherence to a turn-around time. In this case, the climbers ignored the conventional wisdom, which suggests that they should turn back if they cannot reach the summit by one o'clock in the afternoon. A strictly enforced rule would help protect them against the sunk cost effect, i.e., the tendency to continue climbing because of the substantial prior commitment of time, money, and other resources.
As for the overconfidence bias, I would suggest that expeditions assign someone with a great deal of credibility and experience to be the contrarian during the climb. That person would be responsible for identifying risks, questioning the judgment of other guides and climbers, and reminding everyone of the reasons why many people have died on the slopes of Everest.
Finally, I think the climbers should maintain radio communication with some expert hikers who are not involved in their expedition. Their emotional distance from the effort may enable these experts to offer unbiased guidance and to provide a more balanced assessment of the risks involved in particular situations.
Q: You also looked at the Everest tragedy through the lens of group dynamics. How, in a nutshell, do you think group dynamics could have influenced climbers' actions that day?
A: I would argue that the groups developed a climate that was hostile to open discussion and constructive dissent. One expedition leader went so far as to say, "I will tolerate no dissension...my word will be absolute law." Not surprisingly, people suppressed their concerns and doubts about some of the poor judgment and choices that were made during the climb.
For instance, one survivor lamented that he did not "always speak up when maybe I should have." One factor that contributed to the lack of candid discussion was the perceived differences in status among expedition members. For example, one climber said that he did not speak up when things began to go wrong because he "was quite conscious of his place in the expedition pecking order."
The unwillingness to question team procedures and exchange ideas openly prevented the group from revising and improving their plans as conditions changed.
Q: Many pieces of a puzzle need to interlock successfully for a team to climb a mountain or execute a high-pressure business decision. What is often the role of complexity in these kinds of situations?
A: The idea here is that climbing Everest entails a complex system of activities and behaviors. Two characteristics of this systemcomplex interactions and tight couplingenhanced the likelihood of a serious accident.
First, complex interactions means that different elements of the system interacted in ways that were unexpected and difficult to perceive or comprehend in advance. This led to a series of small, but interconnected, breakdowns and failures that became part of a dangerous "domino effect."
Second, tight coupling means that there was a fairly rigid sequence of time-dependent activities, one dominant path to achieving the goal, and very little slack in the system. These characteristics made it easier for a problem in one area to quickly trigger failures in other aspects of the climb.
71. For a more extensive discussion of anticipatory regret, see I. Janis & L. Mann, Decision Making: A Psychological Analysis of Conflict, Choice, and Commitment, (New York: Free Press, 1977).
72. For more on the issue of developing confidence to make decisions quickly in turbulent environments, see: K. Eisenhardt, "Making Fast Strategic Decisions in High-Velocity Environments," Academy of Management Journal , 32 (1989): 543-576.
73. See A. Korsgaard, D. Schweiger, & H. Sapienza, "Building Commitment, Attachment, and Trust in Strategic Decision-Making Teams: The Role of Procedural Justice," Academy of Management Journal , 38 (1995): 60-84.
74. In the famous story of Intel's exit from the DRAM business, this is exactly what Gordon Moore and Andrew Grove asked themselves as they were contemplating whether to continue investing in the loss-making DRAM business.
75. Jon Krakauer has cautioned that this could occur quite easily with respect to the Everest tragedy. In his book, he wrote, "If you can convince yourself that Rob Hall died because he made a string of stupid errors and that you are too clever to repeat those same errors, it makes it easier for you to attempt Everest in the face of some rather compelling evidence that doing so is injudicious." (p. 356-357).
76. E. Jones and R. Nisbett, "The Actor and the Observer: Divergent Perceptions of the Causes of Behavior," in E. Jones, D. Kanouse, H. Kelley, R. Nisbett, S. Valins, and B. Weiner, eds., Attribution: Perceiving the Causes of Behavior (General Learning Press, 1971).
77. Boukreev and DeWalt [p. 226-227], op cit.
Advertisement
Cognitive Biases in Criminal Case Evaluation: A Review of the Research
- Review Article
- Open access
- Published: 23 June 2021
- Volume 37 , pages 101–122, ( 2022 )
Cite this article
You have full access to this open access article
- Vanessa Meterko ORCID: orcid.org/0000-0002-1207-8812 1 &
- Glinda Cooper 1
21k Accesses
9 Citations
2 Altmetric
Explore all metrics
Psychological heuristics are an adaptive part of human cognition, helping us operate efficiently in a world full of complex stimuli. However, these mental shortcuts also have the potential to undermine the search for truth in a criminal investigation. We reviewed 30 social science research papers on cognitive biases in criminal case evaluations (i.e., integrating and drawing conclusions based on the totality of the evidence in a criminal case), 18 of which were based on police participants or an examination of police documents. Only two of these police participant studies were done in the USA, with the remainder conducted in various European countries. The studies provide supporting evidence that lay people and law enforcement professionals alike are vulnerable to confirmation bias, and there are other environmental, individual, and case-specific factors that may exacerbate this risk. Six studies described or evaluated the efficacy of intervention strategies, with varying evidence of success. Further research, particularly in the USA, is needed to evaluate different approaches to protect criminal investigations from cognitive biases.
Similar content being viewed by others
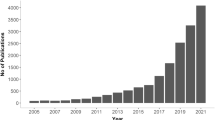
The disaster of misinformation: a review of research in social media
Ethical decision-making theory: an integrated approach.
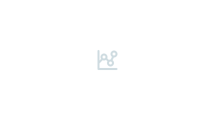
Implicit bias in healthcare professionals: a systematic review
Avoid common mistakes on your manuscript.
Introduction
Decades of research in cognitive and social psychology have taught us that there are limitations to human attention and decision-making abilities (see, for example, Gilovich et al. 2002 ). We cannot process all the stimuli that surround us on a daily basis, so instead we have adapted for efficiency by attuning to patterns and developing mental shortcuts or rules of thumb to help us effectively navigate our complex world. While this tendency to rely on heuristics and biases can serve us well by allowing us to make quick decisions with little cognitive effort, it also has the potential to inadvertently undermine accuracy and thus the fair administration of justice.
Cognitive bias is an umbrella term that refers to a variety of inadvertent but predictable mental tendencies which can impact perception, memory, reasoning, and behavior. Cognitive biases include phenomena like confirmation bias (e.g., Nickerson 1998 ), anchoring (e.g., Tversky & Kahneman 1974 ), hindsight bias (e.g., Fischhoff 1975 ), the availability heuristic (e.g., Tversky & Kahneman 1973 ), unconscious or implicit racial (or other identifying characteristics) bias (e.g., Greenwald et al. 1998 ; Staats et al. 2017 ), and others. In this context, the word “bias” does not imply an ethical issue (e.g., Dror 2020 ) but simply suggests a probable response pattern. Indeed, social scientists have demonstrated and discussed how even those who actively endorse egalitarian values harbor unconscious biases (e.g., Pearson et al. 2009 ; Richardson 2017 ) and how expertise, rather than insulating us from biases, can actually create them through learned selective attention or reliance on expectations based on past experiences (e.g., Dror 2020 ). Consequently, we recognize the potential for these human factors to negatively influence our criminal justice process.
In an effort to explore the role of cognitive biases in criminal investigations and prosecutions, we conducted a literature review to determine the scope of available research and strength of the findings. The questions guiding this exercise were as follows: (1) what topics have been researched so far and where are the gaps?; (2) what are the methodological strengths and limitations of this research?; and (3) what are the results, what do we know so far, and where should we go from here?
We searched PsycINFO for scholarly writing focused on cognitive biases in criminal investigations and prosecutions in December 2016 and again in January 2020. Footnote 1 We reviewed all results by title and then reviewed the subset of possibly-relevant titles by abstract, erring on the side of over-inclusivity. We repeated this process using the Social Sciences Full Text, PubMed, and Criminal Justice Abstracts with Full Text databases to identify additional papers. Finally, we manually reviewed the reference lists in the identified papers for any unique sources we may have missed in prior searches.
We sorted the articles into categories by the actor or action in the criminal investigation and prosecution process that they addressed, including physical evidence collection, witness evaluation, suspect evaluation, forensic analysis and testimony, police case evaluation (i.e., integrating and drawing conclusions based on the totality of the evidence), prosecutors, defense attorneys, judges, juries, and sentencing. Within each of these categories, we further sorted the articles into one of three types of sources: “primary data studies” describing experimental or observational studies that involved data collection or analysis, “intervention studies” that were solution-oriented and involved implementing some type of intervention or training to prevent or mitigate a phenomenon, and “secondary sources” (e.g., commentaries, letters, reviews, theoretical pieces, general book chapters) that discussed cognitive biases but did not present primary data.
To narrow the scope of this review, we did not include articles that focus solely on implicit racial bias or structural racial bias in the criminal legal system. The foundational and persistent problem of racial (particularly anti-Black) bias throughout our legal system—from policing to sentencing (e.g., Voigt et al. 2017 ; NYCLU 2011 ; Blair et al. 2004 ; Eberhardt et al. 2006 )—has been clearly demonstrated in laboratory experiments and analyses of real-world data and is well-documented in an ever-growing body of academic publications and policy reports (e.g., Correll et al. 2002 ; Chanin et al. 2018 ; Owens et al. 2017 ; Staats et al. 2017 ).
Scope of Available Research and Methodology
Cognitive biases in forensic science have received the most attention from researchers to date (for a review of these forensic science studies, see Cooper & Meterko 2019 ). The second most substantial amount of scholarship focused on case evaluation (i.e., integrating and drawing conclusions based on the totality of the evidence in a case). Ultimately, we found 43 scholarly sources that addressed various issues related to the evaluation of the totality of evidence in criminal cases: 25 primary data (non-intervention) studies, five intervention studies, and one additional paper that presented both primary data and interventions, and 12 secondary sources. For the remainder of this article, we focus solely on the primary data and intervention studies. One of the primary data studies (Fahsing & Ask 2013 ) described the development of materials that were used in two subsequent studies included in this review (Fahsing & Ask 2016 ; 2017 ), and thus, this materials-development paper is not reviewed further here. Table 1 presents an overview of the research participants and focus of the other 30 primary data and intervention studies included in our review.
One challenge in synthesizing this collection of research is the fact that these studies address different but adjacent concepts using a variety of measures and—in some instances—report mixed results. The heterogeneity of this research reveals the complex nature of human factors in criminal case evaluations.
Eighteen of the 30 papers (13 primary data and three intervention) included participants who were criminal justice professionals (e.g., police, judges) or analyzed actual police documents. An appendix provides a detailed summary of the methods and results of the 18 criminal justice participant (or document) studies. Fifteen papers were based on or presented additional separate analyses with student or lay participants. Recruiting professionals to participate in research is commendable as it is notoriously challenging but allows us to identify any differences between those with training and experience versus the general public, and to be more confident that conclusions will generalize to real-world behavior. Of course, representativeness (or not) must still be considered when making generalizations about police investigations.
Reported sample sizes ranged from a dozen to several hundred participants and must be taken into account when interpreting individual study results. Comparison or control groups and manipulation checks are also essential to accurately interpreting results; some studies incorporated these components in their designs while others did not.
Most studies used vignettes or case materials—both real and fictionalized—as stimuli. Some studies did not include enough information about stimulus or intervention materials to allow readers to critically interpret the results or replicate an intervention test. Future researchers would benefit from publishers making more detailed information available. Further, while the use of case vignettes is a practical way to study these complex scenarios, this approach may not completely mimic the pressures of a real criminal case, fully appreciate how the probative value of evidence can depend on context, or accurately reflect naturalistic decision-making.
Notably, only two of the criminal case evaluation studies using professional participants were conducted in the USA; all others were based in Europe (Austria, Netherlands, Norway, Sweden, and the UK). The differences between police training, operations, and the criminal justice systems writ large should be considered when applying lessons from these studies to the USA or elsewhere.
Finally, all of these papers were published relatively recently, within the past 15 years. This emerging body of research is clearly current, relevant, and has room to grow.
Research Findings
The primary data studies address a constellation of concepts that demonstrate how human factors can inadvertently undermine the seemingly objective and methodical process of a criminal investigation. To organize these concepts, we used a taxonomy originally developed to describe potential sources of bias in forensic science observations and conclusions as a guide (Dror 2017 ; Dror et al. 2017 ) and adapted it to this collection of case evaluation literature. Footnote 2 As in Dror’s taxonomy, the broad base of this organizing pyramid is “human nature,” and as the pyramid narrows to its peak, potential sources of bias become increasingly dependent on environmental, individual, and case-specific circumstances and characteristics (Fig. 1 ). Some authors in this collection address more than one of these research areas within the same paper through multiple manipulations or a series of studies (Table 1 ).
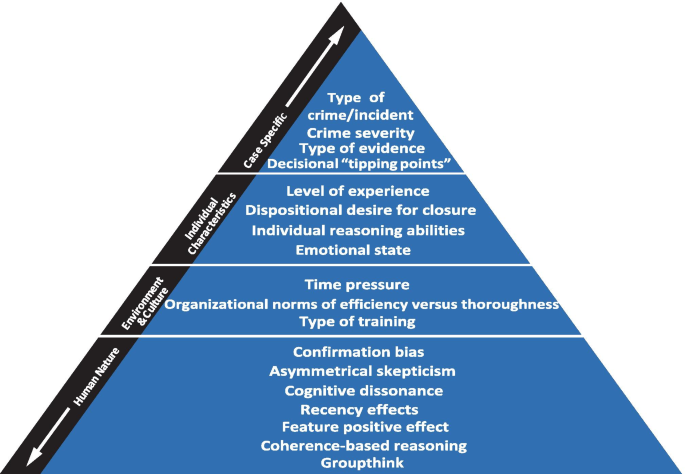
Organizational framework for case evaluation studies, adapted from Dror’s ( 2017 ) taxonomy of different sources of potential bias that may cognitively contaminate forensic observations and conclusions. The specific factors listed in this pyramid are those that were examined in the collection of studies in the present literature review
Human Nature
The “human nature” studies include those that demonstrate universal psychological phenomena and their underlying mechanisms in the context of a criminal case evaluation. Several studies focused on confirmation bias. Confirmation bias, sometimes colloquially referred to as “tunnel vision,” denotes selective seeking, recalling, weighting, and/or interpreting information in ways that support existing beliefs, expectations, or hypotheses, while simultaneously avoiding or minimizing inconsistent or contradictory information (Nickerson 1998 ; Findley 2012 ). Some authors in this collection of studies used other terms to describe this concept or elements of it, including “context effects,” the term used by Charman et al. ( 2015 ) to describe when “a preexisting belief affects the subsequent interpretation of evidence” (p. 214), and asymmetrical skepticism (Ask & Granhag 2007b ; Marksteiner et al. 2010 ).
Eight studies with law enforcement personnel (Ask & Granhag 2007b ; Ask et al. 2008 ; Charman et al. 2017 ; Ditrich 2015 ; Groenendaal & Helsloot 2015 ; Marksteiner et al. 2010 ; Rassin 2010 ; Wallace 2015 ) examined aspects of confirmation bias; one addressed the distinct but related phenomenon of groupthink (Kerstholt & Eikelboom 2007 ). The importance of this issue was demonstrated by a survey of an unspecified number of professional crime scene officers conducted by Ditrich ( 2015 ), asking for their opinions about the relative frequency and severity of various cognitive errors that could potentially negatively affect a criminal investigation; based on their experiences, respondents highlighted confirmation bias (as well as overestimating the validity of partial information and shifting the burden of proof to the suspect). The other studies within this group used experimental designs to assess police officers’ evaluation of evidence. Charman et al. ( 2017 ) reported that police officers’ initial beliefs about the innocence or guilt of a suspect in a fictional criminal case predicted their evaluation of subsequent ambiguous evidence, which in turn predicted their final beliefs about the suspect’s innocence or guilt. This is not the only study to demonstrate that, like the rest of us, police officers are susceptible to confirmation bias. Ask and colleagues ( 2008 ) found that police recruits discredited or supported the same exact evidence (“the viewing distance of 10 m makes the witness identification unreliable” versus “from 10 m one ought to see what a person looks like”) depending on whether it was consistent or inconsistent with their hypothesis of a suspect’s guilt. Ask and Granhag ( 2007b ) found that when experienced criminal investigators read a vignette that implied a suspect’s guilt (but left room for an alternative explanation), they rated subsequent guilt-consistent evidence as more credible and reliable than evidence that was inconsistent with their theory of guilt; similar results were seen in a study of police officers, district attorneys, and judges by Rassin ( 2010 ).
Marksteiner et al. ( 2010 ) investigated the motivational underpinnings of this type of asymmetrical skepticism among police trainees, asking whether it is driven by a desire to reconcile inconsistent information with prior beliefs or by the goal of case closure, and encountered mixed results. The group who initially hypothesized guilt reacted as expected, rating subsequent incriminating evidence as more reliable, but in the group whose initial hypothesis was innocence, there was no difference in the way that they rated additional consistent or inconsistent information. Wallace ( 2015 ) found that the order in which evidence was presented influenced guilt beliefs. When police officers encountered exculpatory evidence prior to inculpatory evidence, guilt belief scores decreased, suggesting their final decisions were influenced by their initial impressions. Kerstholt and Eikelboom ( 2007 ) describe how teams tend to converge on one interpretation, and once such an interpretation is adopted, individual members are less able to examine underlying assumptions critically. They asked independent crime analysts to evaluate a realistic criminal investigation with fresh eyes and found that they were demonstrably influenced when they were aware of the investigative team’s existing working hypothesis.
Studies in student and general populations examining confirmation bias and other aspects of human cognition (Ask et al. 2011b ; Charman et al. 2015 ; Eerland et al. 2012 ; Eerland & Rassin 2012 ; Greenspan & Surich 2016 ; O’Brien 2007 ; 2009 ; Price & Dahl 2014 ; Rassin et al. 2010 ; Simon et al. 2004 ; Wastell et al. 2012 ) reported similar patterns to those described above with police participants. O’Brien ( 2007 ; 2009 ) found that students who named a suspect early in a mock criminal investigation were biased towards confirming that person’s guilt as the investigation continued. O’Brien measured memory for hypothesis-consistent versus hypothesis-inconsistent information, interpretation of ambiguous evidence, participants’ decisions to select lines of inquiry into the suspect or an alternative, and ultimate opinions about guilt or innocence. In a novel virtual crime scene investigation, Wastell et al. ( 2012 ) found that all students (those who ultimately chose the predetermined “correct” suspect from the multiple available people of interest and those who chose incorrectly) sought more chosen-suspect-consistent information during the exercise. However, those who were ultimately unsuccessful (i.e., chose the wrong person) spent more time in a virtual workspace (a measure of the importance placed on potential evidence) after accessing confirmatory information. They also found that students who settled on a suspect early in the exercise—measured by prompts throughout the virtual investigation—were comparatively unsuccessful.
Other psychological phenomena such as recency effects (i.e., our ease of recalling information presented at the end of a list relative to information presented at the beginning or middle) and the feature positive effect (i.e., our tendency to generally attune to presence more than absence) were also examined in studies with student or general population participants. Price and Dahl ( 2014 ) explored evidence presentation order and found that under certain circumstances, evidence presented later in an investigation had a greater impact on student participant decision-making in a mock criminal investigation. Charman and colleagues also found order of evidence presentation influenced ratings of strength of evidence and likelihood of guilt in their 2015 study of evidence integration with student participants. These results appear to provide evidence against the presence of confirmation bias, but recency effects still demonstrate the influence of human factors as, arguably, the order in which one learns about various pieces of evidence -whether first or last- should not impact interpretation. Several research teams found that a positive eyewitness identification is seen as more credible than a failure to identify someone (Price & Dhal 2014 , p.147) and the presence of fingerprints—as opposed to a lack of fingerprints—is more readily remembered and used to make decisions about a criminal case (Eerland et al. 2012 ; Eerland & Rassin 2012 ), even though the absence of evidence can also be diagnostic. Other researchers highlighted our psychic discomfort with cognitive dissonance (Ask et al. 2011b ) and our tendency to reconcile ambiguity and artificially impose consistency in a criminal case by engaging in “ bidirectional coherence-based reasoning” (Simon et al. 2004 ; Greenspan & Surich 2016 ).
Environment and Culture
The three “environment and culture” studies with police personnel (Ask & Granhag 2007b ; Ask et al. 2011a ; Fahsing & Ask 2016 ) revealed the ways in which external factors can influence an investigation. For instance, type of training appears to impact the ability to generate a variety of relevant hypotheses and actions in an investigation. English and Norwegian investigators are trained and performed differently when faced with semi-fictitious crime vignettes (Fahsing & Ask 2016 ). Organizational culture can impact the integrity of an investigation as well. Ask and colleagues ( 2011a ) concluded that a focus on efficiency—as opposed to thoroughness—produces more cursory processing among police participants, which could be detrimental to the accurate assessment of evidence found later in an investigation. Ask and Granhag ( 2007b ) observed that induced time pressure influenced officers’ decision-making, creating a higher tendency to stick with initial beliefs and a lower tendency to be influenced by the evidence presented.
Individual Characteristics
Seven “individual characteristics” studies with police personnel (Ask & Granhag 2005 ; 2007a ; Dando & Ormerod 2017 ; Fahsing & Ask 2016 ; 2017 ; Kerstholt & Eikelboom 2007 ; Wallace 2015 ) plus two studies with student populations (Rassin 2010 , 2018a ) examined ways in which personal attributes can influence an investigation. Varying amounts of professional experience may matter when it comes to assessments of potential criminal cases and assumptions about guilt. For instance, police recruits appear to have a strong tendency toward criminal—as opposed to non-criminal—explanations for an ambiguous situation like a person’s disappearance (Fahsing & Ask 2017 ) and less experienced recruits show more suspicion than seasoned investigators (Wallace 2015 ). In a departure from the typical mock crime vignette method, Dando and Ormerod ( 2017 ) reviewed police decision logs (used for recording and justifying decisions made during serious crime investigations) and found that senior officers generated more hypotheses early in an investigation, and switched between considering different hypotheses both early and late in an investigation (suggesting a willingness to entertain alternative theories) compared with inexperienced investigators. An experimental study, however, found that professional crime analyst experience level (mean 7 months versus 7 years) was not related to case evaluation decisions and did not protect against knowledge of prior interpretations of the evidence influencing conclusions (Kerstholt & Eikelboom 2007 ).
Two studies examined differences in reasoning skills in relation to the evaluation of evidence. Fahsing and Ask ( 2017 ) found that police recruits’ deductive and inductive reasoning skills were not associated with performance on an investigative reasoning task. In contrast, in a study with undergraduate students, accuracy of decision-making regarding guilt or innocence in two case scenarios was associated with differences in logical reasoning abilities as measured by a test adapted from the Wason Card Selection Test (Rassin 2018a ).
Ask and Granhag ( 2005 ) found inconsistent results in a study of police officers’ dispositional need for cognitive closure and the effect on criminal investigations. Those with a high need for cognitive closure (measured with an established scale) were less likely to acknowledge inconsistencies in case materials when those materials contained a potential motive for the suspect, but were more likely to acknowledge inconsistencies when made aware of the possibility of an alternative perpetrator. In a replication study with undergraduate students, Ask & Granhag ( 2005 ) found that initial hypotheses significantly affected subsequent evidence interpretation, but found no interaction with individual need for cognitive closure. Students who were aware of an alternative suspect (compared with those aware of a potential motive for the prime suspect) were simply less likely to evaluate subsequent information as evidence supporting guilt.
In another study, when Ask and Granhag ( 2007a ) induced negative emotions in police officers and then asked them to make judgments about a criminal case, sad participants were better able to substantively process the consistency of evidence or lack thereof, whereas angry participants used heuristic processing.
Case-Specific
Four studies of police personnel (Ask et al. 2008 ; Fahsing & Ask 2016 ; 2017 ; Wallace 2015 ), one using police records (Dando & Omerod 2017 ), and three studies of student populations (Ask et al. 2011b ; O’Brien 2007 ; 2009 ; Rassin et al. 2010 ) examined “case-specific” and evidence-specific factors. In a study of police officers, Ask and colleagues ( 2008 ) showed that the perceived reliability of some types of evidence (DNA versus photographs versus witnesses) is more malleable than others; similar results pertaining to DNA versus witness evidence were found in a study of law students (Ask et al. 2011b ).
Fahsing and Ask ( 2016 ) found that police recruits who were presented with a scenario including a clear “tipping point” (an arrest) did not actually produce significantly fewer hypotheses than those who were not presented with a tipping point (though they acknowledge that the manipulation—one sentence embedded in a case file—may not have been an ecologically valid one). In a subsequent study with police recruits, the presence of a tipping point resulted in fewer generated hypotheses, but the difference was not statistically significant (Fahsing & Ask 2017 ).
Other studies using law students (Rassin et al. 2010 ) or undergraduate students (O’Brien 2007 ) examined the influence of crime severity on decision-making. Rassin et al. ( 2010 ) observed that the affinity for incriminating evidence increases with crime severity, but in one of O’Brien’s ( 2007 ) studies, crime severity did not have a demonstrable impact on confirmation bias.
Interventions
Taken together, this body of work demonstrates vulnerabilities in criminal investigations. Some researchers have suggested theoretically supported solutions to protect against these vulnerabilities, such as gathering facts rather than building a case (Wallace 2015 ) or institutionalizing the role of a “contrarian” in a criminal investigation (MacFarlane 2008 ). Few studies have tested and evaluated these potential remedies, however. Testing is an essential prerequisite to any advocacy for policy changes because theoretically sound interventions may not, in fact, have the intended effect when applied (e.g., see below for a description of O’Brien’s work testing multiple interventions with differing results).
Four studies have examined various intervention approaches with police departments or investigators (Groenendaal & Helsloot 2015 ; Jones et al. 2008 ; Rassin 2018b ; Salet & Terpstra 2014 ). Jones et al. ( 2008 ) created a tool that helped an experimental group of investigators produce higher quality reviews of a closed murder case than those working without the aid of the review tool. Their article provides an appendix with “categories used in the review tool” (e.g., crime scene management, house-to-house enquiries, community involvement) but lacks a detailed description of the tool itself and the outcome measures. Importantly, the authors raise the possibility that a review tool like this may improve how officers think through a case because of the structure or content of the tool or it may succeed by simply slowing them down so they can think more critically and thoroughly. Another approach that shows promise in reducing tunnel vision is using a pen and paper tool to prompt investigators to consider how well the same evidence supports different hypotheses (Rassin 2018b ). In a study of actual case files, supplemented with interviews, Salet and Terpstra ( 2014 ) explored “contrarians” and found that there are real-world challenges to the position’s efficacy (e.g., personal desire to be a criminal investigator, desire for solidarity with colleagues) and considerable variability in the way contrarians approach their work, with some opting for closeness to an investigation and others opting for distance; individuals also embraced different roles (e.g., supervisor, devil’s advocate, focus on procedure). The researchers concluded that, in practice, these contrarians appear to have exerted subtle influence on investigations but there is no evidence of a radical change in case trajectory. Similarly, members of criminal investigation teams in the Netherlands reported that, in practice, designated devil’s advocates tend to provide sound advice but do not fundamentally change the course of investigations (Groenendaal & Helsloot 2015 ). Groenendaal and Helsloot describe the development and implementation of the Criminal Investigation Reinforcement Programme in the Netherlands, which was prompted by a national reckoning stemming from a widely publicized wrongful conviction. The program included new policies aimed at, among other things, reducing tunnel vision (including the use of devil’s advocates, structured decision-making around “hypotheses and scenarios,” and professionalized, permanent “Command Core Teams” dedicated to major crimes). This deliberate intervention provided an opportunity for researchers to interview investigators who were directly impacted by the new policies. Groenendaal and Helsloot conclude that the main effect of this intervention was an increased awareness about the potential problem of tunnel vision, and they focus on an unresolved a tension between “efficacy” (more convictions) and “precaution” (minimizing wrongful convictions). Their work underscores the importance of collecting criminal legal system data, as interviewees reported their experiences and impressions but could not report whether more correct convictions had been obtained or more wrongful convictions avoided.
Other studies have examined various intervention ideas with student populations (Haas et al. 2015 ; O’Brien 2007 ; 2009 ). Haas et al. ( 2015 ) found that using a checklist tool to evaluate evidence appears to improve students’ abductive reasoning and reduce confirmation bias. O’Brien ( 2007 ; 2009 ) found that orienting participants to being accountable for good process versus outcome had no impact, and that when participants expected to have to persuade someone of their hypothesis, this anticipation actually worsened bias. More promisingly, she discovered that participants who were asked to name a suspect early in an investigation, but were then told to consider how their selected suspect could be innocent and then generate counter-arguments, displayed less confirmation bias across a variety of measures (they looked the same as those who did not name a suspect early). But another approach—asking participants to generate two additional alternative suspects—was not effective (these participants showed the same amount of bias as those who identified just one suspect).
Zalman and Larson ( 2016 ) have observed “the failure of innocence movement advocates, activists, and scholars to view the entirety of police investigation as a potential source of wrongful convictions, as opposed to exploring arguably more discrete police processes (e.g., eyewitness identification, interrogation, handling informants)” (p.3). While the thorough examination of these discrete processes has led to a better understanding of risk factors and, ultimately, reforms in police practices (e.g., see the Department of Justice 2017 guidelines for best practices with eyewitnesses), a recent shift towards viewing wrongful convictions from a “sentinel events” Footnote 3 perspective advances the conversation around these criminal justice system failures (Doyle 2012 ; 2014 ; Rossmo & Pollock 2019 ).
This literature review has identified a body of research that lends support to this holistic perspective. The studies reviewed here address a constellation of concepts that demonstrate how the human element—including universal psychological tendencies, predictable responses to situational and organizational factors, personal factors, and characteristics of the crime itself—can unintentionally undermine truth-seeking in the complex evidence integration process. Some concepts are addressed by one study, some are addressed by several, and some studies explored multiple variables (e.g., demonstrating the existence of confirmation bias and measuring how level of professional experience plays a role).
Several contemporary studies have demonstrated the existence of confirmation bias in police officers within the context of criminal investigations. Other psychological phenomena have not been examined in police populations but have been examined in student or general populations using study materials designed to assess the interpretation of criminal case evidence and decision-making. This collection of studies also investigates the role of environmental factors that may be specific to a department or organization, characteristics of individual investigators, or of the specific case under review. At the environmental level, type of training and organizational customs were influential and are promising areas for further research as these factors are within the control of police departments and can be modified. With respect to individual characteristics, a better understanding of advantageous dispositional tendencies and what is gained by professional experience, as well as the unique risks of expertise, could lead to better recruitment and training methods. Case-specific factors are outside the control of investigators, but awareness of factors that pose a greater risk for bias could serve as an alert and future research could identify ways to use this information in practice (see also Rossmo & Pollock 2019 for an in-depth discussion of “risk recipes”).
Charman and colleagues ( 2017 ) present a particularly interesting illustration of the way in which a criminal case is not merely the sum of its parts. In this study, the researchers presented law enforcement officers with exonerating, incriminating, or neutral DNA or eyewitness evidence, collected initial beliefs about guilt, asked participants to evaluate a variety of other ambiguous evidence (alibi, composite sketch, handwriting comparison, and informant information that could be reasonably interpreted in different ways), and then provide a final rating of guilt. As hypothesized, the researchers found those who were primed with incriminating evidence at the beginning were more likely to believe the suspect guilty at the end. However, even those who initially received exonerating information and initially rated the likelihood of suspect guilt as relatively low ended up increasing their guilt rating after reviewing the other ambiguous evidence. It appears that the cumulative effect of ambiguous evidence tilted the scales towards guilt. This unexpected outcome underscores the value of understanding how the totality of evidence in a criminal case is evaluated, and has implications for the legal doctrine of “harmless error” rooted in assumptions of evidentiary independence (e.g., Hasel & Kassin 2009 ).
Consistently incorporating control groups into future study designs and including complete stimulus materials in future publications could build on this foundation. This would help future researchers fully interpret and replicate study results and would assist in determining what elements of intervention strategies work. Since the majority of these studies were conducted in Europe, it would be worthwhile to explore whether or not these results can be replicated in the USA, given the similarities and differences in our criminal justice systems and the variety of approaches used to select and train detectives across police departments. Finally, valuable future research will move beyond the demonstration of these human vulnerabilities and will design and test strategies to mitigate them in the complex real world. Footnote 4 Vignettes and mock-investigations are clever ways of studying criminal investigations, but it is worth remembering that these approaches cannot fully capture the dynamics of a real criminal investigation. Collaboration between academic researchers and criminal investigators could generate robust expansions of this work.
Evidence evaluation and synthesis in criminal investigations is, of course, just one part of a larger legal process. In addition to police, defense attorneys, prosecutors, and judges have powerful roles in determining case outcomes, especially in a system that is heavily reliant on plea bargaining. Critically addressing the potential influence of cognitive biases throughout this system, and promoting and implementing proven, practical protections against these tendencies will advance accuracy and justice.
We used the following search terms and Boolean Operators: (criminal OR justice OR police OR investigat* OR forensic* OR jury OR juries OR judge* OR conviction* OR prosecut* OR defense OR defender* OR attorn*) in any field (e.g., text, title) AND (“cognitive bias” OR “cognitive dissonance” OR “tunnel vision” OR “confirmation bias” OR “interpretive bias” OR “belief perseverance” OR “asymmetrical skepticism”) in any field (e.g., text, title).
As Dror ( 2017 ) notes, the development of this taxonomy began in a paper in 2009 (Dror 2009 ) and was further developed in a 2014 paper (Stoel et al. 2014 ), with additional sources of bias added subsequently (in Dror 2015 , and Zapf & Dror 2017 ).
According to the National Institute of Justice ( 2017 ), a sentinel event is a significant negative outcome that (1) signals underlying weaknesses in the system or process, (2) is likely the result of compound errors, and (3) may provide, if properly analyzed and addressed, important keys to strengthen the system and prevent future adverse outcomes.
As Snook and Cullen ( 2008 ) assert, “it is unrealistic to expect police officers to investigate all possible suspects, collect evidence on all of those suspects, explore all possible avenues concerning the circumstances surrounding a crime, search for disconfirming and confirming evidence of guilt for every suspect, and integrate all of this information” (p. 72). Dando and Ormerod ( 2017 ) illustrate this real-world complexity when they describe an investigation that was delayed because a call for tips led to a flood of false leads, suggesting that more information is not always better. Further, though it addresses procedural justice in street policing rather than evidence integration in a criminal investigation (and thus was not included in this review), Owens et al. ( 2018 ) provide an example of a field study, complete with published scripts. Recognizing the automated thinking and behavior that comes with job experience, these researchers tested an intervention to reduce the number of incidents resolved with arrests and use of force by implementing a training program aimed at encouraging beat officers to think more slowly and deliberately during routine encounters; they also assessed the cost of this intervention in the police department.
Ask K, Granhag PA (2005) Motivational sources of confirmation bias in criminal investigations: the need for cognitive closure. J Investig Psychol Offender Profiling 2(1):43–63. https://doi.org/10.1002/jip.19
Article Google Scholar
Ask K, Granhag PA (2007a) Hot cognition in investigative judgments: the differential influence of anger and sadness. Law Hum Behav 31(6):537–551. https://doi.org/10.1007/s10979-006-9075-3
Article PubMed Google Scholar
Ask K, Granhag PA (2007b) Motivational bias in criminal investigators’ judgments of witness reliability. J Appl Soc Psychol 37(3):561–591. https://doi.org/10.1111/j.1559-1816.2007.00175.x
Ask K, Granhag PA, Rebelius A (2011a) Investigators under influence: how social norms activate goal-directed processing of criminal evidence. Appl Cogn Psychol 25(4):548–553. https://doi.org/10.1002/acp.1724
Ask K, Rebelius A, Granhag PA (2008) The ‘elasticity’ of criminal evidence: a moderator of investigator bias. Appl Cogn Psychol 22(9):1245–1259. https://doi.org/10.1002/acp.1432
Ask K, Reinhard M-A, Marksteiner T, Granhag PA (2011b) Elasticity in evaluations of criminal evidence: exploring the role of cognitive dissonance. Leg Criminol Psychol 16:289–306
Blair IV, Judd CM, Chapleau KM (2004) The influence of Afrocentric facial features in criminal sentencing. Psychol Sci 15(10):674–679. https://doi.org/10.1111/j.0956-7976.2004.00739.x
Chanin J, Welsh M, Nurge D (2018) Traffic enforcement through the lens of race: a sequential analysis of post-stop outcomes in San Diego. California Criminal Justice Policy Review 29(6–7):561–583. https://doi.org/10.1177/0887403417740188
Charman SD, Carbone J, Kekessie S, Villalba DK (2015) Evidence evaluation and evidence integration in legal decision-making: order of evidence presentation as a moderator of context effects. Appl Cogn Psychol 30(2):214–225. https://doi.org/10.1002/acp.3181
Charman SD, Kavetski M, Mueller DH (2017) Cognitive bias in the legal system: police officers evaluate ambiguous evidence in a belief-consistent manner. J Appl Res Mem Cogn 6(2):193–202. https://doi.org/10.1016/j.jarmac.2017.02.001
Cooper GS, Meterko V (2019) Cognitive bias research in forensic science: a systematic review. Forensic Sci Int 297:35–46. https://doi.org/10.1016/j.forsciint.2019.01.016
Correll J, Park B, Judd CM, Wittenbrink B (2002) The police officer’s dilemma: using ethnicity to disambiguate potentially threatening individuals. J Pers Soc Psychol 83(6):1314–1329. https://doi.org/10.1037/0022-3514.83.6.1314
Dando CJ, Ormerod TC (2017) Analyzing decision logs to understand decision making in serious crime investigations. Human Factors: The Journal of the Human Factors and Ergonomics Society 59(8):1188–1203. https://doi.org/10.1177/0018720817727899
Department of Justice (2017) Retrieved from: https://www.justice.gov/file/923201/download
Ditrich H (2015) Cognitive fallacies and criminal investigations. Sci Justice 55:155–159
Dror IE (2009) How can Francis Bacon help forensic science? The four idols of human biases. Jurimetrics: The Journal of Law, Science, and Technology 50(1):93–110
Dror IE (2015) Cognitive neuroscience in forensic science: understanding and utilizing the human element. Philos Trans R Soc B 370(1674). https://doi.org/10.1098/rstb.2014.0255
Dror IE (2017) Human expert performance in forensic decision making: seven different sources of bias. Aust J Forensic Sci 49(5):541–547. https://doi.org/10.1080/00450618.2017.1281348
Dror IE (2020) Cognitive and human factors in expert decision making: six fallacies and the eight sources of bias. Anal Chem 92(12):7998–8004. https://pubs.acs.org/doi/10.1021/acs.analchem.0c00704
Dror IE, Morgan RM, Rando C, Nakhaeizadeh S (2017) Letter to the Editor: The bias snowball and the bias cascade effects: two distinct biases that may impact forensic decision making. J Forensic Sci 62(3):832–833. https://doi.org/10.1111/1556-4029.13496
Doyle JM (2012) Learning about learning from error. Police Foundation 14:1–16
Google Scholar
Doyle JM (2014) NIJ’s sentinel events initiative: looking back to look forward. National Institute of Justice Journal 273:10–14. https://www.ncjrs.gov/pdffiles1/nij/244144.pdf
Eberhardt JL, Davies PG, Purdie-Vaughns VJ, Johnson SL (2006) Looking deathworthy: perceived stereotypicality of Black defendants predicts capital-sentencing outcomes. Psychol Sci 17(5):383–386. https://doi.org/10.1111/j.1467-9280.2006.01716.x
Eerland A, Post LS, Rassin E, Bouwmeester S, Zwaan RA (2012) Out of sight, out of mind: the presence of forensic evidence counts more than its absence. Acta Physiol (Oxf) 140(1):96–100. https://doi.org/10.1016/j.actpsy.2012.02.006
Eerland A, Rassin E (2012) Biased evaluation of incriminating and exonerating (non)evidence. Psychol Crime Law 18(4):351–358. https://doi.org/10.1080/1068316X.2010.493889
Fahsing I, Ask K (2013) Decision making and decisional tipping points in homicide investigations: an interview study of British and Norwegian detectives. J Investig Psychol Offender Profiling 10(2):155–165. https://doi.org/10.1002/jip.1384
Fahsing I, Ask K (2016) The making of an expert detective: the role of experience in English and Norwegian police officers’ investigative decision-making. Psychol Crime Law 22(3):203–223. https://doi.org/10.1080/1068316X.2015.1077249
Fahsing IA, Ask K (2017) In search of indicators of detective aptitude: police recruits’ logical reasoning and ability to generate investigative hypotheses. J Police Crim Psychol 33(1):21–34. https://doi.org/10.1007/s11896-017-9231-3
Findley KA (2012) Tunnel vision. In B. L. Cutler (Ed.), Conviction of the innocent: Lessons from psychological research (pp. 303–323). American Psychological Association. https://doi.org/10.1037/13085-014
Fischhoff B (1975) Hindsight does not equal foresight: the effect of outcome knowledge on judgment under uncertainty. J Exp Psychol Hum Percept Perform 1(3):288–299
Gilovich T, Griffin DW, Kahneman D (Eds.) (2002) Heuristics and biases: The psychology of intuitive judgment. Cambridge, U.K.; New York: Cambridge University Press
Greenspan R, Scurich N (2016) The interdependence of perceived confession voluntariness and case evidence. Law Hum Behav 40(6):650–659. https://doi.org/10.1037/lhb0000200
Greenwald AG, McGhee DE, Schwartz JLK (1998) Measuring individual differences in implicit cognition: the implicit association test. J Pers Soc Psychol 74(6):1464–1480
Groenendaal J, Helsloot I (2015) Tunnel vision on tunnel vision? A preliminary examination of the tension between precaution and efficacy in major criminal investigations in the Netherlands. Police Pract Res 16(3):224–238
Haas HS, Pisarzewska Fuerst M, Tönz P, Gubser-Ernst J (2015) Analyzing the psychological and social contents of evidence-experimental comparison between guessing, naturalistic observation, and systematic analysis. J Forensic Sci 60(3):659–668. https://doi.org/10.1111/1556-4029.12703
Hasel LE, Kassin SM (2009) On the presumption of evidentiary independence. Psychol Sci 20(1):122–126
Jones D, Grieve J, Milne B (2008) Reviewing the reviewers: a tool to aid homicide reviews. The Journal of Homicide and Major Incident Investigation 4(2):59–70
Kerstholt JH, Eikelboom AR (2007) Effects of prior interpretation on situation assessment in crime analysis. J Behav Decis Mak 20(5):455–465. https://doi.org/10.1002/bdm.570
MacFarlane BA (2008) Wrongful convictions: the effect of tunnel vision and predisposing circumstances in the criminal justice system. Goudge Inquiry Research Paper. https://www.attorneygeneral.jus.gov.on.ca/inquiries/goudge/policy_research/pdf/Macfarlane_Wrongful-Convictions.pdf
Marksteiner T, Ask K, Reinhard M-A, Granhag PA (2010) Asymmetrical scepticism towards criminal evidence: the role of goal- and belief-consistency. Appl Cogn Psychol 25(4):541–547. https://doi.org/10.1002/acp.1719
National Institute of Justice (2017) Retrieved from: https://nij.ojp.gov/topics/articles/sentinel-events-initiative
Nickerson RS (1998) Confirmation bias: a ubiquitous phenomenon in many guises. Rev Gen Psychol 2(2):175–220
NYCLU (2011) NYCLU Stop-And-Frisk Report 2011. New York Civil Liberties Union
O’Brien BM (2007) Confirmation bias in criminal investigations: an examination of the factors that aggravate and counteract bias. ProQuest Information & Learning, US. (2007–99016–280)
O’Brien B (2009) Prime suspect: an examination of factors that aggravate and counteract confirmation bias in criminal investigations. Psychol Public Policy Law 15(4):315–334. https://doi.org/10.1037/a0017881
Owens E, Kerrison EM, Da Silveira BS (2017) Examining racial disparities in criminal case outcomes among indigent defendants in San Francisco. Quattrone Center for the Fair Administration of Justice
Owens E, Weisburd D, Amendola KL, Alpert GP (2018) Can you build a better cop?: Experimental evidence on supervision, training, and policing in the community. Criminol Public Policy 17(1):41–87. http://doi.wiley.com/10.1111/1745-9133.12337
Pearson AR, Dovidio JF, Gaertner SL (2009) The nature of contemporary prejudice: insights from aversive racism. Soc Pers Psychol Compass 3(3):314–338. https://doi.org/10.1111/j.1751-9004.2009.00183.x
Price HL, Dahl LC (2014) Order and strength matter for evaluation of alibi and eyewitness evidence: Recency effects. Appl Cogn Psychol 28(2):143–150. https://doi.org/10.1002/acp.2983
Rassin E (2010) Blindness to alternative scenarios in evidence evaluation. J Investig Psychol Offender Profiling 7:153–163. https://doi.org/10.1002/jip.116
Rassin E (2018a) Fundamental failure to think logically about scientific questions: an illustration of tunnel vision with the application of Wason’s Card Selection Test to criminal evidence. Appl Cogn Psychol 32(4):506–511. https://doi.org/10.1002/acp.3417
Rassin E (2018b) Reducing tunnel vision with a pen-and-paper tool for the weighting of criminal evidence. J Investig Psychol Offender Profiling 15(2):227–233. https://doi.org/10.1002/jip.1504
Rassin E, Eerland A, Kuijpers I (2010) Let’s find the evidence: an analogue study of confirmation bias in criminal investigations. J Investig Psychol Offender Profiling 7:231–246
Richardson LS (2017) Systemic triage: Implicit racial bias in the criminal courtroom. Yale Law J 126(3):862–893
Rossmo DK, Pollock JM (2019) Confirmation bias and other systemic causes of wrongful convictions: a sentinel events perspective. Northeastern University Law Review 11(2):790–835
Salet R, Terpstra J (2014) Critical review in criminal investigation: evaluation of a measure to prevent tunnel vision. Policing 8(1):43–50. https://doi.org/10.1093/police/pat039
Simon D, Snow CJ, Read SJ (2004) The redux of cognitive consistency theories: evidence judgments by constraint satisfaction. J Pers Soc Psychol 86(6):814–837. https://doi.org/10.1037/0022-3514.86.6.814
Snook B, Cullen RM (2008) Bounded rationality and criminal investigations: has tunnel vision been wrongfully convicted? In D. K. Rossmo (Ed.), Criminal Investigative Failures (pp. 71–98). Taylor & Francis
Staats C, Capatosto K, Tenney L, Mamo S (2017) State of the science: implicit bias review. The Kirwan Institute
Stoel R, Berger C, Kerkhoff W, Mattijssen E, Dror I (2014) Minimizing contextual bias in forensic casework. In M. Hickman & K. Strom (Eds.), Forensic science and the administration of justice: Critical issues and directions (pp. 67–86). SAGE
Tversky A, Kahneman D (1973) Availability: a heuristic for judging frequency and probability. Cogn Psychol 5:207–232
Tversky A, Kahneman D (1974) Judgment under uncertainty: heuristics and biases. Science 185(4157):1124–1131
Voigt R, Camp NP, Prabhakaran V, Hamilton WL, Hetey RC, Griffiths CM, Eberhardt JL (2017) Language from police body camera footage shows racial disparities in officer respect. Proc Natl Acad Sci 114(25):6521–6526. https://doi.org/10.1073/pnas.1702413114
Article PubMed PubMed Central Google Scholar
Wallace WA (2015) The effect of confirmation bias in criminal investigative decision making. ProQuest Information & Learning, US. (2016–17339–014)
Wastell C, Weeks N, Wearing A, Duncan P (2012) Identifying hypothesis confirmation behaviors in a simulated murder investigation: implications for practice. J Investig Psychol Offender Profiling 9:184–198
Zalman M, Larson M (2016) Elephants in the station house: Serial crimes, wrongful convictions, and expanding wrongful conviction analysis to include police investigation. Retrieved from: https://papers.ssrn.com/sol3/papers.cfm?abstract_id=2716155
Zapf PA, Dror IE (2017) Understanding and mitigating bias in forensic evaluation: lessons from forensic science. Int J Forensic Ment Health 16(3):227–238. https://doi.org/10.1080/14999013.2017.1317302
Download references
Acknowledgments
Thank you to Dr. Karen Amendola (Police Foundation), Ms. Prahelika Gadtaula (Innocence Project), and Dr. Kim Rossmo (Texas State University) for their thoughtful reviews of earlier drafts.
Author information
Authors and affiliations.
Science & Research Department, Innocence Project, New York, NY, USA
Vanessa Meterko & Glinda Cooper
You can also search for this author in PubMed Google Scholar
Corresponding author
Correspondence to Vanessa Meterko .
Ethics declarations
Conflict of interest.
The authors declare that they have no conflict of interest.
Ethical Statement
This article does not contain any studies with human participants or animals performed by either of the authors.
Additional information
Publisher’s note.
Springer Nature remains neutral with regard to jurisdictional claims in published maps and institutional affiliations.
Appendix. Detailed Summary of 18 Studies with Police Participants or Source Materials
- a Homicide case vignette was the same as the others with this designation
- b Assault case vignette was the same as the others with this designation
- c Homicide case vignette was the same as the others with this designation
- d Missing person case vignettes were the same as others with this designation
- e The second study reported in this article used undergraduate student participants
Rights and permissions
Open Access This article is licensed under a Creative Commons Attribution 4.0 International License, which permits use, sharing, adaptation, distribution and reproduction in any medium or format, as long as you give appropriate credit to the original author(s) and the source, provide a link to the Creative Commons licence, and indicate if changes were made. The images or other third party material in this article are included in the article's Creative Commons licence, unless indicated otherwise in a credit line to the material. If material is not included in the article's Creative Commons licence and your intended use is not permitted by statutory regulation or exceeds the permitted use, you will need to obtain permission directly from the copyright holder. To view a copy of this licence, visit http://creativecommons.org/licenses/by/4.0/ .
Reprints and permissions
About this article
Meterko, V., Cooper, G. Cognitive Biases in Criminal Case Evaluation: A Review of the Research. J Police Crim Psych 37 , 101–122 (2022). https://doi.org/10.1007/s11896-020-09425-8
Download citation
Accepted : 08 December 2020
Published : 23 June 2021
Issue Date : March 2022
DOI : https://doi.org/10.1007/s11896-020-09425-8
Share this article
Anyone you share the following link with will be able to read this content:
Sorry, a shareable link is not currently available for this article.
Provided by the Springer Nature SharedIt content-sharing initiative
- Cognitive bias
- Confirmation bias
- Investigation
- Find a journal
- Publish with us
- Track your research

An official website of the United States government
The .gov means it’s official. Federal government websites often end in .gov or .mil. Before sharing sensitive information, make sure you’re on a federal government site.
The site is secure. The https:// ensures that you are connecting to the official website and that any information you provide is encrypted and transmitted securely.
- Publications
- Account settings
Preview improvements coming to the PMC website in October 2024. Learn More or Try it out now .
- Advanced Search
- Journal List
- Front Psychol
- PMC10071311
Cognitive bias and how to improve sustainable decision making
Associated data.
The original contributions presented in the study are included in the article/supplementary material, further inquiries can be directed to the corresponding author/s.
The rapid advances of science and technology have provided a large part of the world with all conceivable needs and comfort. However, this welfare comes with serious threats to the planet and many of its inhabitants. An enormous amount of scientific evidence points at global warming, mass destruction of bio-diversity, scarce resources, health risks, and pollution all over the world. These facts are generally acknowledged nowadays, not only by scientists, but also by the majority of politicians and citizens. Nevertheless, this understanding has caused insufficient changes in our decision making and behavior to preserve our natural resources and to prevent upcoming (natural) disasters. In the present study, we try to explain how systematic tendencies or distortions in human judgment and decision-making, known as “cognitive biases,” contribute to this situation. A large body of literature shows how cognitive biases affect the outcome of our deliberations. In natural and primordial situations, they may lead to quick, practical, and satisfying decisions, but these decisions may be poor and risky in a broad range of modern, complex, and long-term challenges, like climate change or pandemic prevention. We first briefly present the social-psychological characteristics that are inherent to (or typical for) most sustainability issues. These are: experiential vagueness, long-term effects, complexity and uncertainty, threat of the status quo, threat of social status, personal vs. community interest, and group pressure. For each of these characteristics, we describe how this relates to cognitive biases, from a neuro-evolutionary point of view, and how these evolved biases may affect sustainable choices or behaviors of people. Finally, based on this knowledge, we describe influence techniques (interventions, nudges, incentives) to mitigate or capitalize on these biases in order to foster more sustainable choices and behaviors.
1. Introduction: The challenges of human welfare
Supported by science and technology, the world has undergone an explosively rapid change in only a few centuries which offers humanity enormous practical advantages in a large number of areas. Misery and misfortune as a result of food shortages, diseases, and conflicts that were previously considered unsolvable have been adequately tackled ( Pinker, 2018 ). A large part of the world has achieved unprecedented economic growth, and on the waves of globalization, it is assumed that the less developed countries can in principle also benefit from this development ( Harari, 2017 ). However, the technologies we use to increase our welfare today have effects, not only across the whole planet, but also stretching far into the future. In the wake of our pursuit of prosperity, humanity has created a number of new, and possibly even greater, problems. The economic growth, that has provided us with an abundance of food, energy, medicines, and living comfort, simultaneously destabilizes the ecological balance. To date, scientists have gathered broad and convincing evidence that under the influence of fossil energy consumption, there is a rapid global warming that may have devastating consequences for the health, wellbeing, and flourish of future generations. This includes sea level rise, droughts, floods, water shortage, and refugee flows (e.g., Meadows et al., 1972 ; Meadows, 1997 ; Kates and Parris, 2003 ; Millenium Ecosystem Assessment, 2005 ; Biermann et al., 2012 ; IPCC, 2013 , 2014 , 2021 , 2022 ; Steffen et al., 2015 ). Other examples of ecological destabilization are: environmental pollution, pandemics, and massive extinction of plant and animal species. All these ecological imbalances pose a serious threat to the continued existence of the world and the survival of our civilization. In the Stone Age, the average person had around 4,000 cal. of energy per day at their disposal. Today, the average American uses around 230,000 cal., sixty times as much ( Harari, 2017 ). To offer everyone in this world the same standard of living as persons living in the USA, we would need at least four planets, but we only have one ( OECD, 2012 ). At the same time, the world seems hesitating to take decisive preventative action. 1 So, despite that most scientists and an increasing number of politicians and citizens acknowledge these facts, this common understanding has not caused much change in our collective behavior. Humanity thus seems to lack the kind of rationality or wisdom that is needed to make substantial financial, social, or material changes in order to stop possible disasters that threaten long-term wellbeing, i.e., to create a world in which people can flourish and be happy.
1.1. Cognitive bias in sustainability issues
How can this be? Human decision making can be quite questionable at times. For example, it often seems to underestimate the long-term dangers of things like global warming and species extinction. This can make even major future threats seem insufficient motivation for determined action ( Berger, 2009 ). In general, we see these types of typical, and often flawed, decision making patterns in many different contexts of our society ( Eigenauer, 2018 ). For instance, Flyvbjerg (2009) showed that 9 out of 10 transportation infrastructure projects end up in large cost overrun, which did not improve over time, even over a period of 70 years. Other examples of persisting problems that for a major part follow from poor decision making are: improper and incorrect diagnoses as well as harmful patient decisions in medicine and health care ( Croskerry, 2003 ; Groopman, 2007 ); overly optimistic growth assessments and ill-advised lending policies in global finance ( Shiller, 2015 ); optimistic decision making in personal finance, like susceptibility to scams ( Modic and Lea, 2013 ); against all knowledge continue a chosen course or investment with negative outcomes rather than alter it ( Arkes and Blumer, 1985 ; Garland and Newport, 1991 ); perpetuating injustice through personal prejudice and unjust sentencing ( Benforado, 2015 ); and accepting superstitions or conspiracy theories while rejecting scientific findings that contradict these beliefs ( Yasynska, 2019 ).
In this article, we will focus on how the human brain and its evolved psychological characteristics affect people’s decision making. Effects of the workings of our brain and of our evolutionary heritage on decision making manifest most prominently in cognitive biases ( Kahneman et al., 1982 ; Hastie and Dawes, 2001 ; Shafir and LeBoeuf, 2002 ; Haselton et al., 2005 ; van Vugt et al., 2014 ; Korteling et al., 2018 ). Cognitive biases can be generally described as systematic, universally occurring, tendencies, inclinations, or dispositions in human decision making that may make it vulnerable for inaccurate, suboptimal, or wrong outcomes (e.g., Tversky and Kahneman, 1974 ; Kahneman, 2011 ; Korteling and Toet, 2022 ). Well-known examples of biases are hindsight bias (once we know the outcome, we tend to think we knew that all along), tunnel vision (when we are under pressure, we tend to overfocus on our goal and ignore all other things that are happening), and confirmation bias (we tend to only see information that confirms our existing ideas and expectations). People typically tend to pursue self-interest at the expense of the community (Tragedy of the commons). We tend to over-value items we possess (Endowment effect) and we have a strong urge to persist in courses of action, with negative outcomes (Sunk-cost fallacy). What is more, biased decision making feels quite natural and self-evident, such that we are quite blind to our own biases ( Pronin et al., 2002 ). This means we often do not recognize it, and therefore do not realize how our biases influence our decision making.
Cognitive biases are robust and universal psychological phenomena, extensively demonstrated, described, and analyzed in the scientific literature. In a wide range of different conditions, people show the same, typical tendencies in the way they pick up and process information to judge and decide. In line with their systematic and universal character, cognitive biases are also prominent in societal issues and policymaking (e.g., Levy, 2003 ; McDermott, 2004 ; Mercer, 2005 ; Baron, 2009 ; Flyvbjerg, 2009 ; Vis, 2011 ; Arceneaux, 2012 ; Shiller, 2015 ; Bellé et al., 2018 ). For example, Arceneaux (2012) has shown that in discussing political arguments, individuals are more likely to be persuaded by arguments that evoke loss aversion, even in the face of a strong counterargument. And it has been demonstrated in many instances that policy makers tend to make risk-aversive decisions when they expect gains, whereas when facing losses they accept taking more risk (e.g., McDermott, 2004 ; Vis, 2011 ).
There are already many publications on cognitive biases showing how human psychological tendencies underly the choices and behaviors of people (e.g., Kahneman et al., 1982 ; Shafir and LeBoeuf, 2002 ; Kahneman, 2011 ). There is also some literature on which biases and human mechanisms play a role in our difficulties with preventing climate change (e.g., Gifford, 2011 ; van Vugt et al., 2014 ; Marshall, 2015 ; Stoknes, 2015 ). However, there is still lack of insight into how biases play a role in the process of environmental policymaking and how this knowledge may be used to deal with the major systemic challenges that the modern world is confronted with. Despite their possible substantial effects on society and human wellbeing, cognitive biases have never been a serious matter of concern in the social and political domain ( Eigenauer, 2018 ). In this paper, we will therefore analyze the constellation of psychological biases that may hinder behavioral and policy practices addressing sustainability challenges. We will also look for ways to mitigate the potential negative effects of biases through influence techniques, like nudging (e.g., Thaler and Sunstein, 2008 ).
1.2. The rationale and drawback of biases
Given the inherent constraints of our information processing system (i.e., the limited cognitive capacities of the human brain) our intuitive inclinations, or heuristics, may be considered effective, efficient, and pragmatic. And indeed, intuitive or heuristic decision making may typically be effective in; natural (primal) conditions with time-constraints, lack (or overload) of relevant information, when no optimal solution is evident, or when we have built up sufficient expertise and experience with the problem ( Simon, 1955 ; Kahneman and Klein, 2009 ; Gigerenzer and Gaissmaier, 2011 ). In these cases, the outcomes of heuristic decision making may be quite acceptable given the invested time, effort, and resources (e.g., Gigerenzer et al., 1999 ).
The fact that heuristic thinking deals with information processing limitations and/or data limitations ( Simon, 1955 ) does not alter the fact that many of our judgments and decisions may systematically deviate from what may be considered optimal, advisable, or utile given the available information and potential gain or risk ( Shafir and LeBoeuf, 2002 ). This has been demonstrated by a large body of literature, showing how cognitive heuristics or biases may lead to poor decisions in a broad range of situations, even including those without complexity, uncertainty, or time constraints ( Korteling et al., 2018 ). Imagine, for instance, a board of directors that has to decide about the continuation of a big project. Typically, the more they have invested so far, the less likely they are to pull the plug. This is not rational (and is therefore called the sunk cost fallacy), because what should matter is what the costs and benefits will be from this point forward, not what has already been spent. The Sunk-cost fallacy, like various other psychological biases affecting decision making, may continuously pop up in the world we live in. Examples are the Anchoring bias ( Tversky and Kahneman, 1974 ; Furnham and Boo, 2011 ), Authority bias ( Milgram, 1963 ), Availability bias ( Tversky and Kahneman, 1973 , 1974 ), and Conformity bias ( Cialdini and Goldstein, 2004 ).
A large number of different biases have been identified so far and specific biases are also likely to occur in the domain of public decision making. By public decision making, we mean not only collective and democratic decision making, but also individual decision making. For different kinds and domains of decision making, different biases may occur. It may be expected that in decision making within the sustainability domain, certain (categories of) biases may more often occur than others. In this paper, we try to present the most relevant biases and the associated nudges, focusing on public decision making with regard to sustainability challenges.
Decision making in our modern society may be done on an individual basis, but may also involve many participants or stakeholders with their own perspectives and background, i.e., citizens, policy makers, company representatives, and interest groups (e.g., Steg and Vlek, 2009 ). To come to a comprehensive understanding of which psychological biases are likely to pop up in this context, we selected those biases that would likely be most prominent, given the typical (psychological) characteristics of sustainability issues. Next, we described interventions or influence techniques (incentives, nudges) to overcome, mitigate, or capitalize on these biases. This was done in three steps.
Step 1: Defining psychological characteristics of sustainability problems
Sustainability issues have characteristics that may evoke certain biases. Here, we define “sustainability” as: a balanced development in which the exploitation of resources, the direction of investments, the orientation of technological development, and institutional change are all in harmony and enhance both current and future potential to meet long-term wellbeing. First, on the basis of the literature (e.g., Schultz, 2002 ; Steg and Vlek, 2009 ; van Vugt, 2009 ; van Vugt et al., 2014 ; Engler et al., 2018 ; Toomey, 2023 ) and a workshop with experts we defined a set of general social-psychologically relevant characteristics or factors, like “experiential vagueness” or “long-term effects” or “threat of the status quo” that are associated with most sustainability issues.
Step 2: Biases per sustainability characteristic
Each characteristic of sustainability issues may relate to a few specific biases that may hamper sustainable choices and behaviors of people. For example, the long-term character of sustainability implies may be in conflict with our tendency to short-term thinking (Hyperbolic time discounting) or the tendency to underestimate both the likelihood of a disaster and its possible consequences, and to believe that things will always function the way they normally function (Normalcy bias). The subsequent identification of thinking tendencies and biases related to these characteristics was based on the literature entailing overviews of multiple biases (e.g., Korteling et al., 2020a ), a Neuro-Evolutionary Bias Framework ( Korteling et al., 2020a , b ; Korteling and Toet, 2022 ), and on the literature on cognitive biases and sustainability challenges (e.g., Gardner and Stern, 2002 ; Penn, 2003 ; Fiske, 2004 ; Wilson, 2006 ; Steg and Vlek, 2009 ; van Vugt, 2009 ; van Vugt et al., 2014 ; Marshall, 2015 ; Engler et al., 2018 ).
Step 3: Influence techniques per sustainability characteristic
Also, for each group of biases, some relevant intervention techniques that can be used, by for example government or policy makers, were briefly described. These interventions, incentives, or nudges, may be applied to mitigate the relevant biases or to capitalize on them for the purpose of stimulating decision making that is more in line with sustainability goals in the context of the current world. On the basis of a previous literature review ( Korteling et al., 2021 ), we have chosen not to advocate specific educational approaches, aiming at bias mitigation training in order to foster sustainable decision making. Instead, our approach aims at interventions with regard to the context or environment in which people live order to promote more sustainable choices.
Example of the approach
Finally, we will illustrate our approach with the help of an example: A conflict between personal versus community interest is a typical characteristic that is associated with sustainability issues. Natural selection has favored individuals who prioritize personal benefits over those of unrelated others ( Hardin, 1968 ; van Vugt et al., 2014 ). This means that making choices in the public interest is often hindered by our personal interests (Step 1). Sustainability also often involves a trade-off between personal interests, such as driving a car or flying, against collective interests, such as fresh air and a peaceful environment. This conflict relates to the bias called the Tragedy of the commons , i.e., the tendency to prioritize one’s own interests over the common good of the community (Step 2). Because we share our genes with our relatives, this tendency may be countered by invoking kinship as a nudge. Pro-environmental actions or appeals may thus be more effective if they emphasize the interests of our ingroup, children, siblings, and grand-children (Step 3).
3. Most relevant psychological characteristics of sustainability challenges
Below, we list a set of prominent psychological characteristics that we consider relevant for sustainability issues. Although biases are inherent to the thinking and decision making in all people, it may be supposed that biases may differ depending on peoples’ places, functions, and roles in decision situations. On the other hand, there are many mutual influences and dependencies in the policymaking arena. Therefore, we have decided not to make clear distinctions between the specific roles people play in this arena. So, we do not discern biases for citizens, politicians or policy makers.
- Experiential vagueness: Sustainability problems are slowly and gradually evolving. Therefore, the impact of the issue is difficult, if not impossible, to perceive or experience directly with our body and senses. Our knowledge of the issue is largely built on indirect and abstract cognitive information, i.e., on conceptual reasoning, abstract figures, written papers, and quantitative models.
- Long-term effects and future risk : The negative consequences of green practices follow directly, whereas the positive aspects of green practices may emerge only after many years in the (far) future. The same counts for the positive consequences of not taking green action. In addition, sustainability concerns an unknown future with an abundance of possibilities that easily go beyond our imagination.
- Complexity and uncertainty: The sustainability issue is very complicated (socially, technically, logistically, economically) and even “wicked.” Being able to judge and reason over most topics within the field requires multi- and transdisciplinary knowledge. Sustainability challenges are (therefore) accompanied by a high degree of uncertainty about their future progression and how it should be tackled and addressed.
- Threat to the status quo: Many sustainability measures more or less have impact on (sometimes even threaten) our established way of living and basic societal infrastructure. When new measures have an impact on our “normal,” established way of living and basic societal infrastructure, this may be experienced as a threat that will result in losing our freedom and/or comfort (“fear of falling”).
- Threat of social status : Many environmental problems result from a desire to possess or consume as much as possible, instead of consuming “enough” for a good life. Consumptive behavior and high energy consumption are intrinsically related to high social status, which is something most people do not want to lose.
- Social dilemma’s: The sacrifices that have to be made in order to foster sustainability are mainly beneficial for the collective, whereas direct individual gains are often limited. In this “social dilemma,” humans tend to prioritize direct personal interests relative to more sustainable ones that benefit the planet.
- Group pressure : Norms, values, and standards for what is considered as ‘normal’ or what is considered “desirable” are determined and reinforced by group pressure. Also with regard to green choices, we are often more strongly influenced by the behaviors and opinions of our peers than by our personal views and attitudes toward conservation.
4. Biases and interventions per psychological sustainability characteristic
For each of the above-mentioned general psychological characteristics of sustainability issues, the next subsections will provide an analysis and inventory of the (kinds of) cognitive biases that are probably most relevant and critically involved in the associated public and political decision making processes. Finally, for each general characteristic, influence techniques (interventions) to mitigate or capitalize on the relevant/critical biases will be briefly described. These interventions are based on the literature concerning “psychological influence” (e.g., Jowett and O’Donnell, 1992 ; Cialdini, 2006 ; Adams et al., 2007 ; Cialdini, 2009 ; Hansen, 2013 ; Heuer, 2013 ; Korteling and Duistermaat, 2018 ; Toomey, 2023 ). The influence techniques have an informational nature. They can be utilized in public communication, education, and policy making, especially in communication to the public, in different forms of media. Because the biases mentioned show a great deal of overlap and similarity—it was more about groups or types of similar biases—we chose not to make explicit links between specific biases and the associated nudge.
4.1. Experiential vagueness
Social scientists have long been puzzled as to why people are so poor at recognizing environmental risks and ignore global environmental hazards ( Slovic, 1987 ; Hardin, 1995 ). Such apathy is probably a product of our evolutionary heritage that produced a brain that is optimized to perform biological and perceptual-motor functions ( Haselton and Nettle, 2006 ; Korteling et al., 2018 ; Korteling and Toet, 2022 ). For example, the vertebrate eye evolved some 500 billion years ago, compared to 50,000 years ago for human speech; while the first cave drawings are dated at 30,000 years, compared to the earliest writing system approximately 5,000 years ago ( Parker, 2003 ; see also Grabe and Bucy, 2009 ). This comparatively more ancient visual perceptual and communicative apparatus enables us to quickly extract meaning from eye-catching images ( Powel, 2017 ). In addition, there was always a tangible link between behavior and the environment. That is: if you do not eat, you will become hungry and search for food. If it starts raining, you may look for shelter in order to prevent becoming wet. A critical difference between the modern world and our ancestral environment is that we rarely see, feel, touch, hear, or smell how our behaviors gradually impact the environment ( Uzzell, 2000 ; Gifford, 2011 ). Because our ancestors were not confronted with the relatively remote, slowly evolving, or abstract problems ( Toomey, 2023 ), we probably are not well-evolved to be alarmed when confronted with potential or novel dangers that we cannot directly see, hear, or feel with our perceptual systems ( van Vugt et al., 2014 ).
The human senses and nervous system show a gradual decrease in responsiveness to constant situations. In general, we are more sensitive to, and more easily triggered by, sudden changes and differences in the stimulus (contrasts). Because of this neural adaptation, we often may have difficulty with perceiving and appreciating slow and gradual processes of change. Therefore, the gradual changes that are implied in our environment, like global warming, are not very easily noticed. So, most people are generally not really alarmed by the gradual evolving and remote environmental challenges that the world is facing. This may contribute to the relatively low public interest in the issue of environmental threats such as global climate change, pollution of the oceans, extinction of species, the negative health effects of particulate matter, and decreasing biodiversity ( Swim et al., 2011 ).
4.1.1. Most relevant biases with regard to experiential vagueness
- Experience effect : the tendency to believe and remember things easier when they are experienced directly with our physical body and senses instead of abstract representations, like graphs and statistics, or text about scientific data ( van Vugt et al., 2014 ).
- Contrast effect : having difficulty with perceiving and appreciating gradual changes or differences (instead of contrasting ones), such as gradually decreasing biodiversity and climate change ( Plous, 1993 ).
- Story bias : the tendency to accept and remember more easily than simple or basic facts ( Alexander and Brown, 2010 ).
4.1.2. Interventions to mitigate these biases
Key: make the consequences of possible ecological breakdown tangible.
- To increase awareness of environmental threats people should experience by their senses (e.g., vision, sound, proprioception, and smell) how future situations will look and feel, e.g., by gaming, simulation or “experience tanks.” In raising and education, positive “nature experiences” can be used in order to promote a pro-environmental perspective of the world.
- People have difficulty with correctly perceiving and judging abstract figures. Quantitative data, tables, and numbers do not really make an impression and are thus easily ignored or forgotten. 2 Make people therefore aware of environmental challenges using concrete examples and narratives that are related to real individuals with whom they can empathize and reinforce messages with vivid and appealing images, frames, and metaphors.
- Use pictures, animations, artist impressions, podcasts, and video’s instead of (or to support) written information.
- Focus on the concrete consequences of severe threats.
- Humans are evolved to love nature. So, increase the availability and number of opportunities (especially for city dwellers) to appreciate, experience and protect the healing value of the real nature, i.e., the fields, the woods, the waters, and the mountains ( Schultz, 2002 ).
- Sustainability interventions that imply the loss of assets or privileges should proceed slowly, gradual, and in small steps. The more positive and rewarding aspects of transitions can be presented as more contrasting, sudden and discrete events.
- Narratives and stories consisting of coherent events and elements—real or imaginary—are more easily accepted and remembered than plain facts, which may be useful to create or enhance feelings of connectedness and commitment to pro-environmental initiatives.
- From a psycho-social perspective face-to-face communication is probably the richest (and most natural) form of communication and interaction. Use therefore face-to-face communication to promote pro-environmental behavior.
4.2. Long-term effects and future risk
Sustainable choices are often only rewarded in the long-term future, while the costs and sacrifices have to take place in the present. Given two similar rewards, humans show a preference for one that arrives sooner rather than later. So, humans (and other animals) are said to discount the value of the later reward and/or delayed feedback ( Alexander and Brown, 2010 ). In addition, this effect increases with the length of the delay. According to van Vugt et al. (2014) , our tendency to discount future outcomes may have had substantial benefits in primitive ancestral environments, suggesting it is an evolved psychological trait ( Wilson and Daly, 2005 ). If our ancestors had put too much effort into meeting future needs rather than their immediate needs, they would have been less likely to survive and pass on their genes in the harsh and unpredictable natural environment in which they lived ( Boehm, 2012 ). Human psychology is thus naturally formed to maximize outcomes in the here and now, rather than in the uncertain future ( van Vugt et al., 2014 ). Thus people in modern societies still may weigh immediate outcomes much more heavily than distant ones ( Green and Myerson, 2004 ). This preference for today’s desires over tomorrow’s needs—and the conflict between people’s desire for immediate rather than delayed rewards—may be the cause of the persistence of many environmental problems.
Our brain tends to build general conclusions and predictions on the basis of a (small) number of consistent, previous observations (inductive thinking). A typical and flawed inductive statement is: “Of course humanity will survive. Up to now, we have always survived our major threats and disasters.” 3 Even in highly educated and experienced people, inductive reasoning may lead to poor intuitive predictions concerning the risks in the (long-term) future ( Taleb, 2007 ). We tend to focus on risks that we clearly see, but whose consequences are often relatively small, while ignoring the less obvious, but perhaps more serious ones. Next to such poor statistical intuitions, we have a preference for optimistic perspectives. This leads us to ignore unwelcome information and to underestimate the severity and probability of future (environmental) challenges and hazards ( Ornstein and Ehrlich, 1989 ). This may be especially devasting when considering rare and unpredictable outlier events with high impact (“black swans”). Examples of black swans from the past were the discovery of America (for the native population), World War I, the demise of the Titanic, the rise of the Internet, the personal computer, the dissolution of the Soviet Union, and the 9/11 attacks. Many people ignore possible rare events at the edges of a statistical distribution that may carry the greatest consequences. According to Taleb (2007) , black swans (or “unknown-unknowns”) rarely factor into our planning, our economics, our politics, our business models, and in our lives. Although these black swans have never happened before and cannot be precisely predicted, they nevertheless need much more attention than we give them. Also global warming may trigger currently unknown climate tipping points when change in a part of the climate system becomes self-perpetuating beyond a warming threshold, which will lead to unstoppable earth system impact ( IPCC, 2021 , 2022 ).
4.2.1. Most relevant biases related to long-term effects
- Hyperbolic time discounting: the tendency to prefer a smaller reward that arrives sooner over a larger reward that arrives later. We therefore have a preference for immediate remuneration or payment compared to later, which makes it hard to withhold the temptation of direct reward ( Alexander and Brown, 2010 ).
- Normalcy bias : the tendency to underestimate both the likelihood of a disaster and its possible consequences, and to believe that things will always function the way they normally function ( Drabek, 2012 ). By inductive reasoning, we fail to imagine or recognize possible rare events at the edges of a statistical distribution that often carry the greatest consequences, i.e., black swans ( Taleb, 2007 ).
- Optimism bias: (Positive outcome bias, Wishful thinking): the tendency to overestimate the probability of positive (favorable, pleasing) outcomes and to underestimate the probability of negative events ( O’Sullivan, 2015 ).
4.2.2. Interventions to deal with these biases
Key: bring the rewards of more sustainable choices to the present.
- In general, immediate reinforcements are usually better recognized or appreciated and have more effect. Provide thus immediate rewards for green choices, e.g., through subsidy and tax policy, so that it pays more directly to make them.
- Bring long-term benefits in line with short-term ones. For example: investing in solar panels with a quick payback period, subsidizing the purchase of pro-environmental goods, or taxing the use of fossil fuels.
- Make people aware that we live in a world that inherently involves unpredictable and (system-) risks with high impact, e.g., like the corona pandemic. These risks may have severe negative consequences, maybe not yet for themselves in the short term, but much more for their beloved children and grandchildren.
- Present required changes as much as possible in terms of positive challenges, that is in terms of potential benefits rather than negative terms: a more “relaxed and natural way of life” instead of “costs of energy transition.” Green policy will deliver a stable and predictable future within the foreseeable future that makes prosperity and well-being possible.
4.3. Complexity and uncertainty
The modern global world we live in is very complex with many intricate causal relationships. Everything is connected to everything, making it very difficult to see what exactly is going on in this dense network and how the interplay of societal, technological, economic, environmental, and (geo)political forces develops. Our wealth and comfort are made possible by many “hidden” enablers, such as child labor in third world sweatshops and animal suffering out of sight in the bio industry. The complexity of interrelated and hidden causes, consequences, or remedies is also very prominent in sustainability issues. Sustainability issues are about by a fine-grained logistic infrastructure and sophisticated technological inventions and their massive application. For example, the energy transition involves complex socio-technical systems that usually involve a high degree of uncertainty about how this will ultimately work out. Our cognitive capacities to pick up and understand all this technical, statistical, and scientific information are inherently limited (e.g., Engler et al., 2018 ; Korteling et al., 2018 ). How can we intuitively calculate how much CO 2 emission reduction is required and how much (or little) certain technical or economical interventions contribute to the reduction of greenhouse gases? Many people have also poor capacities for calculation and logic reasoning and a poor intuitive sense for coincidence, randomness, statistics, and probability reasoning (e.g., Monat et al., 1972 ; Sunstein, 2002 ; Engler et al., 2018 ). For instance, concepts like “exponential growth”—i.e., when the instantaneous rate of change of a quantity in time is proportional to the quantity itself—are generally poorly understood.
The inherent constraints of our cognitive system to collect and weight of all this information in a proper and balanced way may result in various biases preventing good judgment and decision making on the basis of the most relevant evidence. Our brain tends to selectively focus on specific pieces of information that ‘resonate’ with what we already know or expect and/or what associatively most easily pops up in the forming of judgments, ideas, and decisions ( Tversky and Kahneman, 1974 ; Korteling et al., 2018 ; Toomey, 2023 ). The fact that other (possible relevant or disconfirming) information may exist beyond what comes up in our mind may be insufficiently recognized or ignored ( Kahneman, 2011 ). This often may lead to a rather simplistic view of the world (e.g., populism). We trust and focus on what is clearly visible or (emotionally) charged, what we (accidentally) know, what we happened to see or hear, what we understand, what intuitively feels true, or what associatively comes to mind (the known-knowns). In contrast, we are rather insensitive to the fact that much information does not easily come to us, is not easily comprehensible, or simply is unknown to us. So we easily may ignore the fact that there usually is a lot that we do not know (The unknowns). This characteristic of neural information processing has been termed: the Focus principle ( Korteling et al., 2018 ) or “What You See Is All There Is” (WYSIATI, Kahneman, 2011 ). An important consequence of this principle is that we tend to overestimate our knowledge with regard to complex issues about which we lack experience or expertise ( Kruger and Dunning, 1999 ). A situation may also be deemed as too uncertain or complicated and a decision is never made due to the fear that a new approach may be wrong or even worse. An abundance of possible options may aggravate this situation rendering one unable to come to a conclusion. In sustainability challenges, people may thus be very motivated to improve the situation, but still can be hampered by uncertainty and lack of understanding to take action.
4.3.1. Most relevant biases related to complexity and uncertainty
- Confirmation bias : the tendency to select, interpret, focus on and remember information in a way that confirms one’s preconceptions, views, and expectations ( Nickerson, 1998 ).
- Neglect of probability : the tendency to completely disregard probability when making a decision under uncertainty ( Sunstein, 2002 ).
- Zero-risk bias : The tendency to overvalue choice options that promise zero risk compared to options with non-zero risk ( Viscusi et al., 1987 ; Baron et al., 1993 ).
- Anchoring bias : Biasing decisions toward previously acquired information. In this way, the early arrival of irrelevant information can seriously affect the outcome ( Tversky and Kahneman, 1974 ; Furnham and Boo, 2011 ).
- Availability bias : the tendency to judge the frequency, importance, or likelihood of an event (or information) by the ease with which relevant instances just happen to pop up in our minds ( Tversky and Kahneman, 1973 ; Tversky and Kahneman, 1974 ).
- Focusing illusion : the tendency to place too much emphasis on one or a limited number of aspects of an event or situation when estimating the utility of a future outcome ( Kahneman et al., 2006 ).
- Affect heuristic : basing decisions on what intuitively or emotionally feels right ( Kahneman, 2011 ).
- Framing bias : the tendency to base decisions on the way the information is presented (with positive or negative connotations), as opposed to just on the facts themselves ( Tversky and Kahneman, 1981 ; Plous, 1993 ).
- Knowledge illusion (Dunning-Kruger Effect): the tendency in laymen to over-estimate their own competence ( Kruger and Dunning, 1999 ).
- Surrogation (means-goal): the tendency to concentrate on an intervening process instead of on the final objective or result, e.g., concentrating on means vs goals or on measures vs intended objectives ( Choi et al., 2012 ).
- Ambiguity effect: the tendency to avoid options or actions for which the probability of a favorable outcome is unknown ( Baron, 1994 ).
4.3.2. Interventions to deal with these biases
Key: provide more information and education especially to better understand the environmental consequences of human decisions and actions.
- Consistency is more convincing than quantity. We believe that our judgments are accurate, especially when available information is consistent and representative for a known situation. Therefore, conclusions based on a very small body of consistent information are more convincing for most people than much larger bodies of (less consistent) data (i.e., “The law of small numbers”).
- Repetition of a pro-environmental message has more impact than just one attempt. This exposure effect can be enhanced by using all possible communication channels and media.
- Start with providing information the positive way you want it to taken by the target audience. Later the message may be extended by the less favorable nuances and details.
- Provide better statistical education and training and improve the communication on uncertainty and risk. When it comes to numbers, quantities, and changes therein, focus on total amounts rather than on proportions.
- Make pro-environmental information (e.g., about actions, initiatives, techniques etc….) salient and conspicuous. Focus (in a simple visual way) on the severe consequences of global warming and biodiversity loss (desertification, crop failure, and famine, millions of homeless and displaced people, risk of wars) instead of on the complex underlying mechanisms and processes.
- Influence is unlikely to fail due to information that is not provided. Therefore, in setting up an information campaign, it is generally not needed to invest all efforts in providing maximum possible “evidence” that is intended to confirm the deception. Consistency is dominant. In general, clear, recognizable, and simple information will be most easily picked up and accepted.
- Influence and persuasion is not only determined by what is, or is not, communicated (i.e., the content) but also by how it is communicated or presented (i.e., the frame or form). These latter superficial aspects are more easily, intuitively, and quickly processed than the deeper content of the message. This “framing” can thus be very well exploited for influencing peoples’ choices. Each message can be framed in numerous ways. So it may be very effective to analyze how to wrap up a message in the way you want it to be taken.
- Different people value, and pick-up, different information at different levels. Therefore, communicate messages at different levels of understanding, from the direct immediate consequences for the individual (micro) to the overarching long-term consequences for the world of the future and for future generations (macro).
- Present and facilitate as much as possible “total solutions.” Which are tailor-made to the target audiences.
4.4. Threat of the status quo
A basic premise of evolution is that all organisms strive for the continuation of their existence. This not only concerns the existence per se , but also the maintenance of stable living conditions (that are instrumental to this ultimate goal). For this reason (under normal circumstances and to prevent unexpected risk), we tend to strive at maintaining the present situation and to remain consistent with previous patterns (default effect). So, we easily accept, or prefer, to continue on the path taken and to maintain the status quo (default options) and we are afraid of choosing alternative, options that may turn out suboptimal ( Kahneman and Tversky, 1979 ; Johnson and Goldstein, 2003 ; Chorus, 2010 ). Energy transition, as a possible solution of a future problem, is by many people experienced as threatening, not only to our established comfortable way of living, but to our individual and social basic needs as well. A transition to more sustainable practices may thus cause bad feelings of losing security and possessions, sometimes termed “fear of falling.”
In line with this, people have an overall tendency to experience the disutility of giving up an object as greater than the utility associated with acquiring it (i.e., Loss aversion). Thaler (1980) recognized this pattern, and articulated it as such: people often demand much more to give up an object than they would be willing to pay to acquire it. This is called the Endowment effect. In contrast to what most authors on cognitive biases suppose, we here speculate that the emotions that we feel when we anticipate possible loss of our assets are not the cause of our bias to avoid loss. Instead, they are the result of our pervasive bias for self-preservation and for maintenance our (neurobiological) integrity ( Korteling et al., 2018 ). So in brief: we often prefer to hold on to the current situation and to continue on previous (al) choices. As such, we default to the current situation or status quo.
4.4.1. Most relevant biases related to threat of the status quo
- Status Quo bias : the tendency to maintain the current state of affairs ( Samuelson and Zeckhauser, 1988 ).
- Default effect : the tendency to favor the option that would be obtained if the actor does nothing when given a choice between several options ( Johnson and Goldstein, 2003 ).
- Sunk cost fallacy (also known as Irrational escalation or Concorde effect): the tendency to consistently continue a chosen course with negative outcomes rather than alter it. The effort previously invested is the main motive to continue ( Arkes and Ayton, 1999 ).
- System justification : the tendency to believe that the current or prevailing systems are fair and just, justifying the existing inaccuracies or inequalities within them (social, political, legal, organizational, and economical) ( Jost and Banaji, 1994 ; Jost et al., 2004 ).
- Cognitive dissonance : the tendency to search for and select consistent information in order to try to reduce discomfort when confronted with facts that contradict own choices, beliefs, and values ( Festinger, 1957 ).
- Fear of regret : feeling extra regret for a wrong decision if it deviates from the default ( Dobelli, 2011 ; Kahneman, 2011 ).
- Loss aversion : the tendency to prefer avoiding losses to acquiring equivalent gains. Loss takes an (emotionally) heavier toll than a profit of the same size does ( Kahneman and Tversky, 1984 ).
- Endowment effect : the tendency to value or prefer objects that you already own over those that you do not ( Thaler, 1980 ).
4.4.2. Interventions to deal with these biases
Key: make sustainable options the default or easiest choice and present them as a gains rather than losses.
- Make desired pro-environmental choices and behavior the default (the normal standard) or easiest choice. For example, providing only reusable unless specifically request a single-use plastic shopping bag, or designing buildings and cities to make walking and biking more convenient.
- Encourage active participation can be a major tool for triggering cognitive consistency pressures to build more sustainable habits. In general: active participation signals commitment to subjects, increasing their likely identification with the message or goal of the persuasion. Subsequently, they will tend to make choices that are consistent with their previous—in this case pro-environmental—actions.
- Based on cognitive dissonance theory ( Festinger, 1957 ), the expression of self-criticism in peer (discussion) groups is a major influence technique. Making people vocalize promises (or sins) in public drives subjects to remain consistent with their and words.
- We believe that our judgements are accurate, especially when available information is consistent and representative for a known situation. It is therefore always important to provide consistent information.
- People tend to focus on, interpret, and remember information in ways that confirm their existing ideas, expectations or preconceptions. Therefore, in order to create an open mind, it is better to start with undeniable, true evidence and take care to not to start with highly disputable information evidence. The more complicated and contradictory aspects can be tackled later.
- The first goal in any effort to change another person’s mind must be to ensure that the subject is at least seriously considering the desired alternative. This requires to start with strong and obvious evidence which fits into the target’s existing conceptions of the world. In contrast, starting with less dramatic evidence tends to be unsuccessful since the information will be ignored, unnoticed, forgotten, or misperceived.
- Present changes in terms of gains instead of losses and circumvent the loss felt by people when they are asked to invest funds and provide support to acquire the necessary funds for the transition.
- Create a story different from loss: what are we gaining? For example: more rest, less rat race. Do not address people as consumers, but as citizens, changemakers, parents, etc.
4.5. Threat of social status
People are more focused on relative status than absolute status. This is, for example, demonstrated by the fact that people find an increase in wealth relative to their peers more important than their absolute wealth ( Diener and Suh, 2000 ). In an experimental setting, researchers found that when presented with financial options, most people chose to earn less in absolute terms, as long as they relatively earned more than their peers ( Frank, 1985 ). Not unrelated to our status-seeking tendency, humans tend to consume more than they need. In many historical civilizations, we find a penchant toward (excessive) consumption and showing of materials and riches ( Bird and Smith, 2005 ; Godoy et al., 2007 ). From an evolutionary point of view, such displays of status may be rooted in a social advantage ( Penn, 2003 ; Saad, 2007 ; Miller, 2009 ). Ancestors who strived for improvement of their situation and who tried to do better than their peers, probably have passed their genes better than those who had a more comfortable attitude. The wry side effects, however, are that the tendency to seek status through material goods—nowadays more than ever—may contribute substantially to the production of waste and the depletion of nonrenewable resources. Because we seek relative wealth, as opposed to seeking an absolute point of satisfaction, we are not easily satisfied and we tend to persistently strive for ever more status and wealth. Whether it be our smartphone, our sense of fashion, or our household appliances, they all rapidly become outdated as soon as newer or more fashionable versions enter the horizon. As economists say: we compare ourselves continuously with our neighbors; we want to “keep up with the Joneses.” Finally, items that are scarce or hard to obtain have typically more perceived quality and status than those that are easy to acquire. So many environmental problems can therefore be the result of a conflict between status-enhancing overconsumption versus having enough for a good life. This ‘Hedonic treadmill’ is encouraged by commercials offering us a never ending stream of new products that should make us, in one way or the other, happy and thus hungry to buy more.
4.5.1. Most relevant biases related to threat of social status
- Affective forecasting (Hedonic forecasting, Impact bias): the tendency to overestimate the duration and intensity of our future emotions and feelings regarding events, encouraging putting effort into favorable results (greed) and into avoiding threats ( Wilson and Gilbert 2005 ).
- Hedonic adaptation (Hedonic treadmill): the tendency to quickly return to a relatively stable level of happiness despite major positive or negative life events ( Brickman and Campbell, 1971 ).
- Social comparison bias: The tendency, when making decisions, to favor individuals who do not compete with one’s own particular strengths ( Garcia et al., 2010 ).
- Scarcity bias: the tendency to attribute greater subjective value to items that are more difficult to acquire or in greater demand ( Mittone and Savadori, 2009 ).
4.5.2. Interventions to deal with these biases 4
Key: connect sustainable options and choices with concepts, persons or goods that emanate a high social status.
- Frame pro-environmental choices or options (like solar panels, bikes, or electric cars) as status symbols that show good beliefs and an exemplary way of life.
- In contrast, frame counter-environmental options (mopeds, flying, and meat consumption) as unattractive or associate them with low-status.
- Use high-status and admired or popular influencers and celebrities to promote pro-environmental options, e.g., in social media campaigns.
- Educate people to assess their quality of life in absolute terms of health, freedom, and comfort instead of in relative terms towards ‘the Jonesses’.
- Present the benefits of environmental as scarce. This can be done, for example, by pointing out others (competitors) who want the same goods or by drawing attention to possible future supply problems.
4.6. Personal versus community interest
Individual self-interest is often in conflict with the interest of the whole group. This is generally conceptualized as a social dilemma. This dilemma is usually referred to as the Tragedy of the Commons story ( Hardin, 1968 ). This hypothetical example demonstrates the effects of unregulated grazing (of cattle) on a common piece of land, also known as “the commons.” In modern economic terms, ‘commons’ are any shared or unregulated resources to which all individuals have equal and open access, like the atmosphere, roads, or even the fridge of the office. Searching for direct individual profit, most individuals increase their use or exploitation of these common resources, thereby unintentionally causing it to collapse ( Hawkes, 1992 ; Dietz et al., 2003 ). According to Hardin (1968) and van Vugt et al. (2014) the human mind is shaped to prioritize their personal interests over collective interests because natural selection favors individuals who can gain a personal benefit at the expense of unrelated others. Of course, there are situations under which the collective benefit will be prioritized over that of the induvial. But the conditions under which the human mind is triggered to prioritize the collective good over its own are generally less prevalent ( Hardin, 1968 ).
According to Dawkins (1976) , natural selection is the replication of one’s genes, which often comes at the expense of the survival of others’ genes. Power is thereby often instrumentally used for self-interest at the cost of others. So, survival of the species is not what primarily matters. However, this prioritizing of self-interest is dependent on the relationship of the individual to the group. In tight-knit communities where the individual knows himself to be dependent on the community, his behavior will be in line with this dependency and more likely be in favor of the in-group’s interests. When the individual does not feel this connection to an in-group (community), he is probably more likely to prioritize self-interest. Evidence for this strategy is seen in social dilemma research showing that most individuals tend to make selfish choices when they interact with other people in one-shot encounters ( Komorita and Parks, 1994 ; Fehr and Gächter, 2002 ; van Lange et al., 2013 ). The evolutionary tendency to let self-interest prevail at the expense of others has direct implications for environmental practice, which often concerns the overexploitation of limited resources, such as the oceans, natural areas, fish stocks, clean air, etc. Consequently, many sustainability problems result from this conflict between personal and collective interests.
4.6.1. Most relevant biases related to personal versus community interest
- Tragedy of the commons (Selfishness and self-interest): the tendency to prioritize one’s own interests over the common good of the community ( Hardin, 1968 ).
- Perverse incentive effect (Cobra effect): the tendency to respond to incentives in a way that best serves our own interests and that does not align with the beneficial goal or idea behind the incentives, which may lead to “perverse behaviors” ( Siebert, 2001 ).
- Anthropocentrism: the tendency to take the own, human perspective as the starting point for interpreting and reasoning about all sorts of things, such as nature and other living animals ( Coley and Tanner, 2012 ).
4.6.2. Interventions to deal with these biases
Key: introduce and present sustainable options as the most favorable and profitable.
- Because we share our genes with our relatives, kinship may be a good motivator of pro-environmental behavior. Pro-environmental appeals may be more effective if they emphasize the interests of our ingroup, children, siblings, and grand-children.
- Create programs where pro-environmental choices result in direct personal (or business) gain, e.g., by proper incentives or rewards, like tax exemptions.
- Create close-knit, stable, and small communities to foster pro-collective behavior and cooperation.
- In all species, behaviors reinforced by rewards or positive feedback tend to be repeated ( Thorndike, 1927 , 1933 ), and the more reinforcement, the greater the effect. Therefore, multiple reinforcements on desired social choices increase the chance that this will remain the case or repeat itself in the future.
4.7. Group pressure
Social psychologists have long known that people tend to adapt to the choices and behavior of others ( Asch, 1956 ). Our tendency of following the majority is adaptive since for most species, the costs of individual learning, through trial and error, are substantial ( Simon, 1990 ; Richerson and Boyd, 2006 ; Sundie et al., 2006 ; Sloman and Fernbach, 2018 ). Also for our ancestors, living in uncertain environments it would probably be better to follow and copy others’ behavior than figuring things out for yourself ( Kameda et al., 2003 ; Gorman and Gorman, 2016 ). This is therefore probably an ancient and natural adaptive tendency which may also help maintaining or strengthening a position within the social group ( Korteling et al., 2020a ). We thus easily follow leaders or people with high status and authority in groups. We adapt to people around us with which we feel connected, but have an aversion against strangers. We have difficulty being indebted to others and we like and support kind, attractive and agreeable people. This can lead, for example, to after-talk and blind copying of the behavior of others and the faithful following of persuasive and charismatic persons. In line with this, it has been found that green practices are more strongly influenced by the behaviors of our peers than by our personal attitudes toward conservation. For example, when people see that their neighbors are not conserving, they tend to increase their own energy consumption as well, even when they had been conserving energy in the past ( Schultz et al., 2007 ). This herd behavior is unconscious, and is mediated by mirror neurons in the brain ( Chartrand and Van Baaren, 2009 ). However, the unconscious nature of this herd behavior is often not acknowledged or even denied by the conformers themselves ( Nolan et al., 2008 ) and is thus hard to battle. Our modern world is built on the basis of an enormous amount of unsustainable methods, tools, practices, and applications, so there is still a long way to go to achieve a sustainable world. Hence, the human tendency to copy the behavior of others and to regard other people’s behaviors as the norm and justification of undesirable behavioral choices can be very detrimental to the achievement of sustainable goals.
4.7.1. Most relevant biases related to group pressure
- Bandwagon effect: the tendency to adopt beliefs and behaviors more easily when they have already been adopted by others ( Colman, 2003 ).
- Conformity bias: the tendency to adjust one’s thinking and behavior to that of a group standard.
- Ingroup (−outgroup) bias: the tendency to favor one’s own group above that of others ( Cialdini and Goldstein, 2004 ).
- Authority bias: the tendency to attribute greater accuracy to the opinion of authority figures (unrelated to its content) and to be more influenced by their opinions ( Milgram, 1963 ).
- Liking bias: the tendency to help or support another person the more sympathetically they feel, which is largely determined by: kindness, attractiveness, and affinity ( Cialdini, 2006 ).
- Reciprocity: the tendency to respond to a positive action with another positive action (“You help me then I help you”) and having difficulty being indebted to the other person ( Fehr and Gächter, 2002 ).
- Social proof: the tendency to mirror or copy the actions and opinions of others, causing (groups of) people to converge too quickly upon a single distinct choice ( Cialdini, 2006 ).
4.7.2. Interventions to deal with these biases
Key: use social norms and peer pressure to encourage sustainable choices and behaviors.
- When a behavioral change is requested, it will probably be better to focus peoples’ attention on others who already show the desired pro-environmental behavior instead of educating people about the bad behavior of others.
- People can be seduced to choose for a certain option if they see this in many other people. So, present desirable pro-environmental behaviors as behaviors of the majority of the people (or at least large groups) people. Foster, for example, the desired behavioral choices by advertisements suggesting this behavior is already adopted by groups of people.
- Use people with authority, powerful people, and/or attractive people to promote pro-environmental behavior.
- Create feelings of commitment and indebtment for people who make sacrifices for the community in order to foster sustainability.
5. Discussion and conclusion
5.1. biases and nudges.
In the present paper we have described how ingrained cognitive biases in human thinking may counter the development of green policy practices aimed at fostering a more sustainable and livable world. We have focused our study on how the form, content and communication of information affects our decisions and behavior with regard to sustainability. The influence techniques advocated in this paper are informational and psychological interventions, incentives, and/or nudges that could be effective with regard to biased thinking in the context of the current modern world. In general, biased information processing has served us for almost our entire existence (e.g., Haselton et al., 2005 ; Korteling et al., 2018 ). However, these natural and intuitive thinking patterns may be very counterproductive for coping with the global and complex problems the world is facing today. The many possible incentives and nudges presented show that there are many ways to deliberately capitalize on biased thinking in people in order to promote more sustainable behavioral choices.
In previous publications we have explained how biases originate from ingrained neuro-evolutionary characteristics of our evolved brain (e.g., Korteling et al., 2018 ; Korteling and Toet, 2022 ). This neuro-evolutionary framework provides more fundamental explanations for human decision making than ‘explanations’ provided by most social- or psychological studies. These latter (social-) psychological explanations are more ‘proximate’ in terms of “limitations of information processing capacity” ( Simon, 1955 ; Broadbent, 1958 ; Kahneman, 1973 ; Norman and Bobrow, 1975 ; Morewedge and Kahneman, 2010 ), two metaphorical “Systems of information processing” ( Stanovich and West, 2000 ; Kahneman, 2003 ; Evans, 2008 ; Kahneman, 2011 ), “emotions” ( Kahneman and Tversky, 1984 ; Damasio, 1994 ), “prospects” prospects (e.g., Kahneman and Tversky, 1979 ; Mercer, 2005 ). “lack of training and experience” ( Simon, 1992 ; Klein, 1997 , 1998 ). Our neuro-evolutionary bias framework explains in terms of structural (neural network) and functional (evolutionary) mechanisms the origin of cognitive biases, why they are so systematic, persistent, and pervasive, and why biased thinking feels so normal, natural, and self-evident. Given the inherent/structural (“neural”) and ingrained/functional (“evolutionary”) character of biases, it seems unlikely that simple education or training interventions would be effective to improve human decision making beyond the specific educational context (transfer) and/or for a prolonged period of time (retention). On the basis of a systematic review of the literature, this indeed appears the case ( Korteling et al., 2021 ). When it comes to solving the problems of the modern world, it will probably be impossible to defeat or eliminate biases in human thinking. Thus, we should always be aware of the pervasive effects of cognitive biases and be modest about our cognitive abilities to solve complex long-term problems in an easy way.
So, the effects on decision making of bias-mitigation training interventions are likely to be rather ineffective, in the same way that it is difficult to get people to change their eating habits by persuading them that chocolate or meat does not taste good. What is more: denying the ultimate and deep-seated neuro-evolutionary causes of the particularities and limitations of human thinking, may hamper adequate development and usage of effective interventions. For example: if governments strive to decrease the demand for energy-inefficient jacuzzi baths, but they ignore the influence of human evolutionary biases, this might lead to an intervention strategy that fails. Perhaps the government would try to persuade people that buying energy-consuming baths is unwise for the future. But in the context of our tendency to discount the value of future consequences, such a strategy on its own is likely to be rather ineffective. It would probably be more effective to use our knowledge of cognitive biases to our advantage. For example, the fact that we compare ourselves to our peers (Social comparison) might lead to a campaign in which the purchase of sustainable solar panels or a sustainable heat pump or fancy e-bike is related to status and prestige. Likewise, it is better to convey pro-environmental messages in a simple, consistent, repetitive, and tangible way and to focus on the consequences (bad or good) of ones choices, rather than on complex intervening processes. Finally, it is better to communicate information about the many aspects of sustainability at different levels of understanding at the same time, i.e., from the instant aspects for the individual to the global consequences for the world of the future.
5.2. The ethics of nudging
Above we have listed tips and tricks to provoke “sustainable decision making.” But as we write this, we realize all the more that this knowledge of how biases work, can be used for all kinds of purposes. In the ‘wrong’ hands, this knowledge about biases can be used to manipulate or incite the population to destructive. That is not even speculative, history has already shown this over and over again. Fossil industries that succeeded in holding back measures against global warming, doctors recommending brands of cigarettes, smear campaigns that led to witch-hunts, and anti-Semitic propaganda during World War II are just a few examples.
There is a serious ethical issue with using our knowledge of biases to our advantage (e.g., Bovens, 2009 ; Raihani, 2013 ). Who decides whether it is ethical to nudge citizens and use our knowledge of evolutionary biases to steer the choices and behavior of people? It sometimes may seem obvious that it is a good thing if you want to prevent incitement to hatred and violence, genocide or destructive such as smoking. But there is also a gray area. In the current pandemic, for example, we see that governments are doing their best to silence dissenting voices “for a good cause.” But counter voices also represent the basis of a democratic constitutional state, where counter voices must always be welcomed. Can we afford to go beyond our democratic boundaries, by nudging our citizens, for the sake of the climate? Our thought on this is as follows: Democracy means that everyone is allowed to make their voice heard about the goals that you want to achieve as a society. This report is about how to make your voice heard more effectively. It provides tools that everyone (not just politicians and policy makers) can use, for better or for worse. This applies to any instrument, AI, weapons, robots, ICT, etc.… The evil is not in the instrument, but in the purpose for which it is used. If we democratically choose to achieve certain goals, then it can be deemed defendable that governments use those instruments as effectively as possible to achieve those goals. It leaves people still free to choose their own path and goals.
5.3. A vision-based agenda
Politics can ensure that we as humanity behave more sustainably. In that case, our societal and physical environment will have to be organized differently, for example with far-reaching legislation (eg CO2 tax), a different market-oriented economy and a different transport system. However, these changes are held back by our ingrained preferences for short-term thinking, maintaining the status quo, personal interest, or herd behavior, which may result in fears like losing jobs or losing freedom. These thinking tendencies and fears are exploited by the lobbies of many powerful (e.g. fossil) parties with vested interests. That is why we have to search for ways to get moving as a society. An important part of this is managing well-being, and thereby discovering that there are ways to live sustainably, and also to be happy. This means that, more than ever, there is a need for knowledge and a substantiated vision about the core values that represent us, as humans, and our world, about who we are, how we want to live and where we want to go. This is not just a vision with long-term goals for human well-being, but also one that builds on our natural needs and that takes into account the hidden and inherent systemic risks of the modern, globalized world. This is essential in determining the course and the agenda for the future of humanity.
Data availability statement
Author contributions.
The literature search, analysis, conceptual work, and the writing of the manuscript was done by JEK. GP provided knowledge and information concerning sustainability. JM critically reviewed the manuscript several times. All authors contributed to the article and approved the submitted version.
Conflict of interest
The authors declare that the research was conducted in the absence of any commercial or financial relationships that could be construed as a potential conflict of interest.
Publisher’s note
All claims expressed in this article are solely those of the authors and do not necessarily represent those of their affiliated organizations, or those of the publisher, the editors and the reviewers. Any product that may be evaluated in this article, or claim that may be made by its manufacturer, is not guaranteed or endorsed by the publisher.
Acknowledgments
The present paper was a deliverable of the Wise Policy Making project and the Responsible Hypernudging project. These Early Research Projects (ERPs) were funded by the Netherlands Organization for Applied Scientific Research (TNO).
1 The problem of climate change was put on the agenda by the Club of Rome, with their report Limits to Growth ( Meadows et al., 1972 ). Since then numerous countries have agreed that action is needed. Climate goals were set numerous times, of which the last two were the Paris climate goals (Paris Climate Conference, COP21, 2015, and COP26 in Glasgow, 2021).
2 Although exact “numbers” may sometimes provide information with an aura of objectivity and certainty.
3 However, most human-like races, such as the Neanderthals, are now extinct and real major threats of humanity are those of a globalized world (which only exists for less than a couple of centuries) such as nuclear or biochemical weapons, global warming, or pandemics.
4 Governments will want to consider the ethical preconditions and repercussions of these forms of nudging before engaging in it. Though it is a widely applied strategy in our neoliberal system where commercial advertisements are deemed acceptable to nudge the potential customer into buying their product. However, governments should uphold important ethical guidelines that concur with our values of freedom of choice and democracy. For a more in depth study of this, please read, e.g., van Vugt (2009) and Raihani (2013) .
- Adams B. D., Sartory J., Waldherr S. (2007). Military Influence Operations: Review of Relevant Scientific Literature. Report No. CR 2007-146 . Toronto: Defence Research and Development Canada. [ Google Scholar ]
- Alexander W. H., Brown J. W. (2010). Hyperbolically discounted temporal difference learning . Neural Comput. 22 , 1511–1527. doi: 10.1162/neco.2010.08-09-1080, PMID: [ PMC free article ] [ PubMed ] [ CrossRef ] [ Google Scholar ]
- Arceneaux K. (2012). Cognitive biases and the strength of political arguments . Am. J. Polit. Sci. 56 , 271–285. doi: 10.1111/j.1540-5907.2011.00573.x [ CrossRef ] [ Google Scholar ]
- Arkes H. R., Ayton P. (1999). The sunk cost and Concorde effects: are humans less rational than lower animals? Psychol. Bull. 125 , 591–600. doi: 10.1037/0033-2909.125.5.591 [ CrossRef ] [ Google Scholar ]
- Arkes H. R., Blumer C. (1985). The psychology of sunk cost . Organ. Behav. Hum. Decis. Process. 35 , 124–140. doi: 10.1016/0749-5978(85)90049-4 [ CrossRef ] [ Google Scholar ]
- Asch S. (1956). Studies of independence and conformity . Psychol. Monogr. 70 , 1–70. doi: 10.1037/h0093718 [ CrossRef ] [ Google Scholar ]
- Baron J. (1994). Thinking and Deciding . 2nd Edn. Cambridge, UK: Cambridge University Press. [ Google Scholar ]
- Baron J. (2009). Cognitive biases in moral judgments that affect political behaviour . Synthese 172 , 7–35. doi: 10.1007/s11229-009-9478-z [ CrossRef ] [ Google Scholar ]
- Baron J., Gowda R., Kunreuther H. (1993). Attitudes toward managing hazardous waste: what should be cleaned up and who should pay for it? Risk Anal. 13 , 183–192. doi: 10.1111/j.1539-6924.1993.tb01068.x [ CrossRef ] [ Google Scholar ]
- Bellé N., Cantarelli P., Belardinelli P. (2018). Prospect theory goes public: experimental evidence on cognitive biases in public policy and management decisions . Public Admin Rev. 78 , 828–840. doi: 10.1111/puar.12960 [ CrossRef ] [ Google Scholar ]
- Benforado A. (2015). Unfair: The New Science of Criminal Injustice . New York: Broadway Books. [ Google Scholar ]
- Berger L. S. (2009). Averting Global Extinction: Our Irrational Society as Therapy Patient . Plymouth, UK: Jason Aronson. [ Google Scholar ]
- Biermann F., Abbott K., Andresen S., Baeckstrand K., Bernstein S., Betsill M. M., et al.. (2012). Navigating the 23 Anthropocene: improving earth system governance . Science 335 , 1306–1307. doi: 10.1126/science.1217255, PMID: [ PubMed ] [ CrossRef ] [ Google Scholar ]
- Bird R., Smith E. A. (2005). Signaling theory, strategic interaction, and symbolic capital . Curr. Anthropol. 46 , 221–248. doi: 10.1086/427115 [ CrossRef ] [ Google Scholar ]
- Boehm C. (2012). Moral Origins . London: Basic Books. [ Google Scholar ]
- Bovens L. (2009). “ The ethics of nudge ” in Preference Change: Approaches from Philosophy, Economics and Psychology . eds. Grüne-Yanoff T., Hansson S. O. (Dordrecht, Netherlands: Springer Sciences; ), 207–220. [ Google Scholar ]
- Brickman P., Campbell D. T. (1971). “ Hedonic Relativism and Planning the Good Society ,” in Adaptation Level Theory . ed. Appley M. H.. (New York, NY: Academic Press; ), 287–301. [ Google Scholar ]
- Broadbent B. E. (1958). Perception and communication . New York: Pergamon Press. [ Google Scholar ]
- Chartrand T., Van Baaren R. (2009). Human mimicry . Adv. Exp. Soc. Psychol. 41 , 219–274. doi: 10.1016/S0065-2601(08)00405-X [ CrossRef ] [ Google Scholar ]
- Choi W., Hecht G., Tayler W. B. (2012). Lost in translation: the effects of incentive compensation on strategy Surrogation . Account. Rev. 87 , 1135–1163. doi: 10.2308/accr-10273 [ CrossRef ] [ Google Scholar ]
- Chorus C. G. (2010). A new model of random regret minimization . Eur. J. Transp. Infrastruct. Res. 10 . doi: 10.18757/ejtir.2010.10.2.2881 [ CrossRef ] [ Google Scholar ]
- Cialdini R. D. (2006). Influence: The Psychology of Persuasion . Revised Edition. New York: William Morrow. [ Google Scholar ]
- Cialdini R. B. (2009). Influence: Science and Practice (Vol. 4 ). Boston: Pearson Education. [ Google Scholar ]
- Cialdini R. B., Goldstein N. J. (2004). Social influence: compliance and conformity . Annu. Rev. Psychol. 55 , 591–621. doi: 10.1146/annurev.psych.55.090902.142015 [ PubMed ] [ CrossRef ] [ Google Scholar ]
- Coley J. D., Tanner K. D. (2012). Common origins of diverse misconceptions: cognitive principles and the development of biology thinking . CBE Life Sci. Educ. 11 , 209–215. doi: 10.1187/cbe.12-06-0074, PMID: [ PMC free article ] [ PubMed ] [ CrossRef ] [ Google Scholar ]
- Colman A. M. (2003). Oxford Dictionary of Psychology . New York, NY, USA: Oxford University Press. [ Google Scholar ]
- Croskerry P. (2003). The importance of cognitive errors in diagnosis and strategies to minimize them . Acad. Med. 78 , 775–780. doi: 10.1097/00001888-200308000-00003, PMID: [ PubMed ] [ CrossRef ] [ Google Scholar ]
- Damasio A. R. (1994). Descartes’ Error: Emotion, Reason and the Human Brain , New York, NY, USA. G. P. Putnam’s Sons. [ Google Scholar ]
- Dawkins R. (1976). The Selfish Gene . Oxford: Oxford University Press. [ Google Scholar ]
- Diener E., Suh E. M. (2000). Culture and Subjective Wellbeing . Boston: MIT Press. [ Google Scholar ]
- Dietz T., Ostrom E., Stern P. C. (2003). The struggle to govern the commons . Science 302 , 1907–1912. doi: 10.1126/science.1091015, PMID: [ PubMed ] [ CrossRef ] [ Google Scholar ]
- Dobelli R. (2011). Die Kunst des Klaren Denkens: 52 Denkfehler die sie Besser Anderen Uberlassen . Munchen: Karl Hanser Verlag. [ Google Scholar ]
- Drabek T. E. (2012). Human System Responses to Disaster: An Inventory of Sociological Findings , New York, NY, USA, Springer Verlag. [ Google Scholar ]
- Eigenauer J. D. (2018). The problem with the problrm of human irrationality . Int. J. Educ. Reform 27 , 341–358. doi: 10.1177/105678791802700402 [ CrossRef ] [ Google Scholar ]
- Engler J. O., Abson D. J., von Wehrden H. (2018). Navigating cognition biases in the search of sustainability . Ambio 48 , 605–618. doi: 10.1007/s13280-018-1100-5, PMID: [ PMC free article ] [ PubMed ] [ CrossRef ] [ Google Scholar ]
- Evans J. S. (2008). Dual-processing accounts of reasoning, judgment, and social cognition . Annu. Rev. Psychol. 59 , 255–278. doi: 10.1146/annurev.psych.59.103006.093629, PMID: [ PubMed ] [ CrossRef ] [ Google Scholar ]
- Fehr E., Gächter S. (2002). Altruistic punishment in humans . Nature 415 , 137–140. doi: 10.1038/415137a [ PubMed ] [ CrossRef ] [ Google Scholar ]
- Festinger L. (1957). A Theory of Cognitive Dissonance , Stanford, CA, USA, Stanford University Press. [ Google Scholar ]
- Fiske S. (2004). Social Beings: Core Motives in Social Psychology . New York: Wiley and Sons. [ Google Scholar ]
- Flyvbjerg B. (2009). Survival of the unfittest: why the worst infrastructure gest built – and what can we do about is . Oxf. Rev. Econ. Policy 25 , 344–367. doi: 10.1093/oxrep/grp024 [ CrossRef ] [ Google Scholar ]
- Frank R. (1985). Choosing the Right Pond: Human and the Quest for Status . New York: Oxford University Press. [ Google Scholar ]
- Furnham A., Boo H. C. (2011). A literature review of the anchoring effect . J. Socio-Econ. 40 , 35–42. doi: 10.1016/j.socec.2010.10.008 [ CrossRef ] [ Google Scholar ]
- Garcia S. M., Song H., Tesser A. (2010). Tainted recommendations: the social comparison bias . Organ. Behav. Hum. Decis. Process. 113 , 97–101. doi: 10.1016/j.obhdp.2010.06.002 [ CrossRef ] [ Google Scholar ]
- Gardner G., Stern P. C. (2002). Environmental Problems and Human Behaviour . London: Pearson. [ Google Scholar ]
- Garland H., Newport S. (1991). Effects of absolute and relative sunk costs on the decision to persist with a course of action . Organ. Behav. Hum. Decis. Process. 48 , 55–69. doi: 10.1016/0749-5978(91)90005-E [ CrossRef ] [ Google Scholar ]
- Gigerenzer G., Todd P. M. and ABC Research Group. (1999). Simple heuristics that make us smart . Oxford, GB: Oxford University Press. [ Google Scholar ]
- Gifford R. (2011). The dragons of inaction: psychological barriers that limit climate change mitigation and adaptation . Am. Psychol. 66 , 290–302. doi: 10.1037/a0023566, PMID: [ PubMed ] [ CrossRef ] [ Google Scholar ]
- Gigerenzer G., Gaissmaier W. (2011). Heuristic decision making . Annu. Rev. Psychol. 62 , 451–482. doi: 10.1146/annurev-psych-120709-145346 [ PubMed ] [ CrossRef ] [ Google Scholar ]
- Godoy R., Reyes-García V., Leonard W. R., Huanca T., McDade T., Vadez V., et al.. (2007). Signaling by consumption in a native Amazonian society . Evol. Hum. Behav. 28 , 124–134. doi: 10.1016/j.evolhumbehav.2006.08.005 [ CrossRef ] [ Google Scholar ]
- Gorman S. E., Gorman J. M. (2016). Denying to the Grave: Why We Ignore the Facts that Will Save Us . London, UK: Oxford University Press. [ Google Scholar ]
- Grabe M. E., Bucy E. P. (2009). Image Bite Politics: News and the Visual Framing of Elections: News and the Visual Framing of Elections . Oxford University Press, USA. [ Google Scholar ]
- Green L., Myerson J. (2004). A discounting framework for choice with delayed and probabilistic rewards . Psychol. Bull. 130 , 769–792. doi: 10.1037/0033-2909.130.5.769, PMID: [ PMC free article ] [ PubMed ] [ CrossRef ] [ Google Scholar ]
- Groopman J. (2007). How Doctors Think . New York: Houghton Mifflin. [ Google Scholar ]
- Hansen W. G. (2013). Influence: Theory and Practice . Montery, California: Naval Postgraduate School. [ Google Scholar ]
- Harari Y. N. (2017). Homo Deus: A Brief History of Tomorrow . London: Jonathan Cape. [ Google Scholar ]
- Hardin G. (1968). Tragedy of the commons . Science 162 , 1243–1248. doi: 10.1126/science.162.3859.1243 [ PubMed ] [ CrossRef ] [ Google Scholar ]
- Hardin G. (1995). Living with limits: Ecology, economics, and population taboos . Oxford, UK: Oxford University Press. [ Google Scholar ]
- Haselton M. G., Nettle D. (2006). The paranoid optimist: an integrative evolutionary model of cognitive biases . Personal. Soc. Psychol. Rev. 10 , 47–66. doi: 10.1207/s15327957pspr1001_3, PMID: [ PubMed ] [ CrossRef ] [ Google Scholar ]
- Haselton M. G., Nettle D., Andrews P. W. (2005). “ The evolution of cognitive bias ” in The Handbook of Evolutionary Psychology . ed. Buss D. M. (Hoboken, NJ, USA: John Wiley and Sons Inc; ). [ Google Scholar ]
- Hastie R., Dawes R. M. (2001). Rational Choice in an Uncertain World: The Psychology of Judgement and Decision Making . Thousand Oaks: Sage. [ Google Scholar ]
- Hawkes K. (1992). “ Sharing and collective action ” in Evolutionary Ecology and Human Behaviour . eds. Smith E., Winterhalder B. (New York: Aldine de Gruyter; ), 269–300. [ Google Scholar ]
- Heuer R. J. (2013). “ Cognitive factors in deception and counter deception ” in The Art and Science of Military Deception . eds. Rothstein H., Whaley B. (Boston/London: Artech House; ), 105–133. [ Google Scholar ]
- IPCC (2013). Summary for Policymakers. In: Climate Change 2013: The Physical Science Basis. Contribution of Working Group I to the Fifth Assessment Report of the Intergovernmental Panel on Climate Change.
- IPCC (2014). “ Summary for policymakers ,” in Climate Change 2014: Mitigation of Climate Change. Contribution of Working Group III to the Fifth Assessment Report of the Intergovernmental Panel on Climate Change . eds. Edenhofer O., Pichs-Madruga R., Sokona Y., Farahani E., Kadner S., Seyboth K., et al.. (Cambridge, United Kingdom and New York, NY, USA: Cambridge University Press; ). [ Google Scholar ]
- IPCC (2021). Summary for Policymakers. In: The Physical Science Basis. Available at: https://IPCC_AR6_WGI_SPM_final.pdf (Accessed February 02, 2022).
- IPCC (2022). Critical Findings of the Sixth Assessment Report (AR6) of Working Group I of the Intergovernmental Panel on Climate Change (IPCC) for Global Climate Change Policymaking: A Summary for Policymakers (SPM) Analysis.
- Johnson E. J., Goldstein D. (2003). Do defaults save lives? Science 302 , 1338–1339. doi: 10.1126/science.1091721, PMID: [ PubMed ] [ CrossRef ] [ Google Scholar ]
- Jost J. T., Banaji M. R. (1994). The role of stereotyping in system-justification and the production of false consciousness . Br. J. Soc. Psychol. 33 , 1–27. doi: 10.1111/j.2044-8309.1994.tb01008.x [ CrossRef ] [ Google Scholar ]
- Jost J. T., Banaji M. R., Nosek B. A. (2004). A decade of system justification theory: accumulated evidence of conscious and unconscious bolstering of the status quo . Polit. Psychol. 25 , 881–919. doi: 10.1111/j.1467-9221.2004.00402.x [ CrossRef ] [ Google Scholar ]
- Jowett G, O’Donnell V. (1992). Propaganda and Persuasion , 2nd, Newbury Park, CA: Sage Publications, 122–154. [ Google Scholar ]
- Kahneman D. (1973). Attention and Effort . Englewood Cliffs, New Jersey, Prentice-Hall Inc. [ Google Scholar ]
- Kahneman D. (2003). A perspective on judgment and choice: mapping bounded rationality . Am. Psychol. 58 , 697–720. doi: 10.1037/0003-066X.58.9.697, PMID: [ PubMed ] [ CrossRef ] [ Google Scholar ]
- Kahneman D. (2011). Thinking Fast and Slow . New York, USA: Farrar, Straus and Giroux. [ Google Scholar ]
- Kahneman D., Klein G. (2009). Conditions for intuitive expertise: a failure to disagree . Am. Psychol. 64 , 515–526. doi: 10.1037/a0016755, PMID: [ PubMed ] [ CrossRef ] [ Google Scholar ]
- Kahneman D., Krueger A. B., Schkade D., Schwarz N., Stone A. A. (2006). You be happier if you were richer: a focusing illusion . Science 312 , 1908–1910. doi: 10.1126/science.1129688, PMID: [ PubMed ] [ CrossRef ] [ Google Scholar ]
- Kahneman D., Slovic P., Tversky A. (1982). Judgment Under Uncertainty: Heuristics and Biases , Cambridge, UK, Cambridge University Press. [ PubMed ] [ Google Scholar ]
- Kahneman D., Tversky A. (1979). Prospect theory: an analysis of decision under risk . Econometrica 47 :263. doi: 10.2307/1914185 [ CrossRef ] [ Google Scholar ]
- Kahneman D., Tversky A. (1984). Choices, values, and frames . Am. Psychol. 39 , 341–350. doi: 10.1037/0003-066X.39.4.341 [ CrossRef ] [ Google Scholar ]
- Kameda T., Takezawa M., Hastie R. (2003). The logic of social sharing: an evolutionary game analysis of adaptive norm development . Personal. Soc. Psychol. Rev. 7 , 2–19. doi: 10.1207/S15327957PSPR0701_1, PMID: [ PubMed ] [ CrossRef ] [ Google Scholar ]
- Kates R. W., Parris T. M. (2003). Long-term trends and a sustainability transition . Proc. Natl. Acad. Sci. 100 , 8062–8067. doi: 10.1073/pnas.1231331100, PMID: [ PMC free article ] [ PubMed ] [ CrossRef ] [ Google Scholar ]
- Klein G. (1997). “ The recognition-primed decision (RPD) model: looking back, looking forward ” in Naturalistic Decision Making . eds. Zsambok C. E., Klein G. (New York, USA: Psychology Press; ). [ Google Scholar ]
- Klein G. (1998). Sources of Power: How People Make Decisions , Cambridge, MA, USA, MIT Press. [ Google Scholar ]
- Komorita S., Parks C. D. (1994). Social Dilemmas . Madison, WI: Brown and Benchmark. [ Google Scholar ]
- Korteling J. E., Brouwer A. M., Toet A. (2018). A neural network framework for cognitive bias . Front. Psychol. 9 :1561. doi: 10.3389/fpsyg.2018.01561, PMID: [ PMC free article ] [ PubMed ] [ CrossRef ] [ Google Scholar ]
- Korteling J. E., Duistermaat M. (2018). Psychological Deception. Report TNO R11532 . Soesterberg: TNO Defence, Safety and Security. [ Google Scholar ]
- Korteling J. E., Gerritsma J., Toet A. (2021). Retention and transfer of cognitive bias mitigation interventions: a systematic literature study . Front. Psychol. 12 :629354. doi: 10.3389/fpsyg.2021.629354, PMID: [ PMC free article ] [ PubMed ] [ CrossRef ] [ Google Scholar ]
- Korteling J. E., Sassen-van Meer J., Toet A. (2020a). Neuro-Evolutionary Framework for Cognitive Biases. Rapport TNO 2020 R10611 . Soesterberg: TNO Defence, Safety and Security [ Google Scholar ]
- Korteling J. E., Sassen-van Meer J., Toet A. (2020b). Neuro-Evolutionary Bias Framework. Report TNO 2020 R11451 . Soesterberg: TNO Defence, Safety and Security. [ Google Scholar ]
- Korteling J. E., Toet A. (2022). “ Cognitive biases ” in Encyclopedia of Behavioural Neuroscience . ed. Della Sala S.. 2nd Edn. (Elsevier; ), 610–619. [ Google Scholar ]
- Kruger J., Dunning D. (1999). Unskilled and unaware of it: how difficulties in recognizing one’s own incompetence lead to inflated self-assessments . J. Pers. Soc. Psychol. 77 , 1121–1134. doi: 10.1037/0022-3514.77.6.1121, PMID: [ PubMed ] [ CrossRef ] [ Google Scholar ]
- Levy J. S. (2003). Applications of Prospect theory to political science . Synthese 135 , 215–241. doi: 10.1023/A:1023413007698 [ CrossRef ] [ Google Scholar ]
- Marshall G. (2015). Don’t Even Think About It: Why Our Brains are Wired to Ignore Climate Change . Bloomsbury Publishing, USA. [ Google Scholar ]
- McDermott R. (2004). Prospect theory in political science: gains and losses from the first decade . Polit. Psychol. 25 , 289–312. doi: 10.1111/j.1467-9221.2004.00372.x [ CrossRef ] [ Google Scholar ]
- Meadows D. (1997). Places to intervene in a system . Whole Earth 91 , 78–84. [ Google Scholar ]
- Meadows D., Randers J., Behrens W. W. (1972). The Limits to Growth . New York: Universe Books. [ Google Scholar ]
- Mercer J. (2005). Prospect theory and political science . Annu. Rev. Polit. Sci. 8 , 1–21. doi: 10.1146/annurev.polisci.8.082103.104911 [ CrossRef ] [ Google Scholar ]
- Milgram S. (1963). Behavioral study of obedience . J. Abnorm. Soc. Psychol. 67 , 371–378. doi: 10.1037/h0040525 [ PubMed ] [ CrossRef ] [ Google Scholar ]
- Millennium Ecosystem Assessment . (2005). Ecosystems and Human Well-Being: Synthesis . Washington, DC: Island Press. [ Google Scholar ]
- Miller G. F. (2009). Spent: Sex, Evolution, and Consumer Behaviour . New York: Viking. [ Google Scholar ]
- Mittone L., Savadori L. (2009). The scarcity bias . Appl. Psychol. 58 , 453–468. doi: 10.1111/j.1464-0597.2009.00401.x [ CrossRef ] [ Google Scholar ]
- Modic D., Lea S. E. G. (2013). Scam compliance and the psychology of persuasion . Soc. Sci. Res. Network 34. doi: 10.2139/ssrn.2364464 [ CrossRef ] [ Google Scholar ]
- Monat A., Averill J., Lazarus R. S. (1972). Anticipatory stress and coping reactions under various conditions of uncertainty . J. Pers. Soc. Psychol. 24 , 237–253. doi: 10.1037/h0033297, PMID: [ PubMed ] [ CrossRef ] [ Google Scholar ]
- Morewedge C. K., Kahneman D. (2010). Associative processes in intuitive judgment . Trends Cogn. Sci. 14 , 435–440. doi: 10.1016/j.tics.2010.07.004, PMID: [ PMC free article ] [ PubMed ] [ CrossRef ] [ Google Scholar ]
- Nickerson R. S. (1998). Confirmation bias: a ubiquitous phenomenon in many guises . Rev. Gen. Psychol. 2 , 175–220. doi: 10.1037/1089-2680.2.2.175 [ CrossRef ] [ Google Scholar ]
- Nolan J. P., Schultz P. W., Cialdini R. B., Goldstein N. J., Griskevicius V. (2008). Normative social influence is underdetected . Pers. Soc. Psychol. Bull. 34 , 913–923. [ PubMed ] [ Google Scholar ]
- Norman D. A., Bobrow D. G. (1975). On data-limited and resource-limited processes . Cogn. Psychol. 7 , 44–64. doi: 10.1016/0010-0285(75)90004-3 [ CrossRef ] [ Google Scholar ]
- O’Sullivan O. P. (2015). The neural basis of always looking on the bright side . Dialogues Philos. Ment. Neuro Sci. 8 , 11–15. [ Google Scholar ]
- OECD (2012). OECD Environmental Outlook to 2050 . Paris, France. [ Google Scholar ]
- Ornstein R., Ehrlich P. (1989). New world, New Mind: Moving Toward Conscious Evolution . New York: Touchstone Books. [ Google Scholar ]
- Parker A. (2003). In the Blink of an Eye: How Vision Sparked the Big Bang of Evolution . New York: Basic Books. [ Google Scholar ]
- Penn D. J. (2003). The evolutionary roots of our environmental problems: toward a Darwinian ecology . Q. Rev. Biol. 78 , 275–301. doi: 10.1086/377051, PMID: [ PubMed ] [ CrossRef ] [ Google Scholar ]
- Pinker S. (2018). Enlightment Now: The Case for Reason, Science, Humanism, and Progress . London, GB: Viking. [ Google Scholar ]
- Plous S. (1993). The Psychology of Judgment and Decision Making . New York: McGraw-Hill. [ Google Scholar ]
- Powel T. E. (2017). Multimodal News Framing Effects. Dissertation . Amsterdam, NL: University of Amsterdam. [ Google Scholar ]
- Pronin E., Lin D. Y., Ross L. (2002). The bias blind spot: perceptions of bias in self versus others . Personal. Soc. Psychol. Bull. 28 , 369–381. doi: 10.1177/0146167202286008 [ CrossRef ] [ Google Scholar ]
- Raihani N. J. (2013). Nudge politics: efficacy and ethics . Front. Psychol. 4. doi: 10.3389/fpsyg.2013.00972 [ PMC free article ] [ PubMed ] [ CrossRef ] [ Google Scholar ]
- Richerson P. J., Boyd R. (2006). Not by Genes Alone: How Culture Transformed Human Evolution . Chicago: Chicago University Press. [ Google Scholar ]
- Saad G. (2007). The Evolutionary Bases of Consumption . New York: Lawrence Erlbaum Associates. [ Google Scholar ]
- Samuelson W., Zeckhauser R. (1988). Status quo bias in decision making . J. Risk Uncertain. 1 , 7–59. doi: 10.1007/BF00055564 [ CrossRef ] [ Google Scholar ]
- Schultz P. W. (2002). “ Inclusion with nature: understanding the psychology of human-nature interactions ” in The psychology of sustainable development . eds. Schmuck P., Schultz P. W. (New York: Kluwer; ), 61–78. doi: 10.1007/978-1-4615-0995-0_4 [ CrossRef ] [ Google Scholar ]
- Schultz P. W., Nolan J. P., Cialdini R. B., Goldstein N. J., Griskevicius V. (2007). The constructive, destructive, and reconstructive power of social norms . Psychol. Sci. 18 , 429–434. doi: 10.1111/j.1467-9280.2007.01917.x, PMID: [ PubMed ] [ CrossRef ] [ Google Scholar ]
- Shafir E., LeBoeuf R. A. (2002). Rationality . Annu. Rev. Psychol. 53 , 491–517. doi: 10.1146/annurev.psych.53.100901.135213 [ PubMed ] [ CrossRef ] [ Google Scholar ]
- Shiller R. (2015). Irrational Exuberance . Princeton: Princeton University Press. [ Google Scholar ]
- Siebert H. (2001). Der Kobra-Effekt. Wie Man Irrwege der Wirtschaftspolitik Vermeidet . Munich: Deutsche Verlags-Anstalt [ Google Scholar ]
- Simon H. A. (1955). A behavioural model of rational choice . Q. J. Econ. 69 , 99–118. doi: 10.2307/1884852 [ CrossRef ] [ Google Scholar ]
- Simon H. A. (1990). A mechanism for social selection and successful altruism . Science 250 , 1665–1668. doi: 10.1126/science.2270480 [ PubMed ] [ CrossRef ] [ Google Scholar ]
- Simon H. A. (1992). What is an “explanation” of behaviour? Psychol. Sci. 3 , 150–161. doi: 10.1111/j.1467-9280.1992.tb00017.x [ CrossRef ] [ Google Scholar ]
- Sloman S. A., Fernbach P. (2018). The Knowledge Illusion: Why We Never Think Alone . Illinois: Penguin. [ Google Scholar ]
- Slovic P. (1987). Perception of risk. Science 236 , 280–285. [ PubMed ] [ Google Scholar ]
- Stanovich K. E., West R. F. (2000). Individual differences in reasoning: implications for the rationality debate? Behav. Brain Sci. 23 , 645–665. [ PubMed ] [ Google Scholar ]
- Steffen W., Richardson K., Rockstrom J., Cornell S. E., Fetzer I., Bennett E. M., et al.. (2015). Planetary boundaries: guiding human development on a changing planet . Science 347 :1259855. doi: 10.1126/science.1259855, PMID: [ PubMed ] [ CrossRef ] [ Google Scholar ]
- Steg L., Vlek C. (2009). Encouraging prosocial behaviour: an integrative review and research agenda . J. Environ. Psychol. 29 , 309–317. doi: 10.1016/j.jenvp.2008.10.004 [ CrossRef ] [ Google Scholar ]
- Stoknes P. E. (2015). What We Think About When We Try Not to Think About Global Warming: Toward a New Psychology of Climate Action . Vermont: Chelsea Green Publishing. [ Google Scholar ]
- Sundie J. M., Cialdini R. B., Griskevicius V., Kenrick D. T. (2006). “ Evolutionary social influence ” in Evolution and Social Psychology . ed. Schaller M. (New York: Psychology Press; ), 287–316. [ Google Scholar ]
- Sunstein C. R. (2002). Probability neglect: emotions, worst cases, and law . Yale Law J. 112 , 61–107. doi: 10.2307/1562234 [ CrossRef ] [ Google Scholar ]
- Swim J. K., Stern P. C., Doherty T., Clayton S., Reser J., Weber E., et al.. (2011). Psychology’s contributions to understanding and addressing global climate change . Am. Psychol. 66 , 241–250. doi: 10.1037/a0023220, PMID: [ PubMed ] [ CrossRef ] [ Google Scholar ]
- Taleb N. N. (2007). The Black Swan: The Impact of the Highly Improbable . New York: The random House. [ Google Scholar ]
- Thaler R. (1980). Toward a positive theory of consumer choice . J. Econ. Behav. Organ. 1 , 39–60. doi: 10.1016/0167-2681(80)90051-7 [ CrossRef ] [ Google Scholar ]
- Thaler R. H., Sunstein C. R. (2008): Nudge–Improving Decisions about Health, Wealth, and Happiness . Yale University Press, New Haven, CT. [ Google Scholar ]
- Thorndike E. L. (1927). The law of effect . Am. J. Psychol. 39 , 212–222. doi: 10.2307/1415413 [ CrossRef ] [ Google Scholar ]
- Thorndike E. L. (1933). A proof of the law of effect . Science 77 , 173–175. doi: 10.1126/science.77.1989.173.b [ PubMed ] [ CrossRef ] [ Google Scholar ]
- Toomey A. H. (2023). Why facts don’t change minds: insights from cognitive science for the improved communication of conservation research . Biol. Conserv. 278 :109886. doi: 10.1016/j.biocon.2022.109886 [ CrossRef ] [ Google Scholar ]
- Tversky A., Kahneman D. (1973). Availability: a heuristic for judging frequency and probability . Cogn. Psychol. 5 , 207–232. doi: 10.1016/0010-0285(73)90033-9 [ CrossRef ] [ Google Scholar ]
- Tversky A., Kahneman D. (1974). Judgment under uncertainty: heuristics and biases . Science 185 , 1124–1131. doi: 10.1126/science.185.4157.1124 [ PubMed ] [ CrossRef ] [ Google Scholar ]
- Tversky A., Kahneman D. (1981). The framing of decisions and the psychology of choice . Science 211 , 453–458. doi: 10.1126/science.7455683 [ PubMed ] [ CrossRef ] [ Google Scholar ]
- Uzzell D. L. (2000). The psycho-spatial dimension of global environmental problems . J. Environ. Psychol. 20 , 307–318. doi: 10.1006/jevp.2000.0175 [ CrossRef ] [ Google Scholar ]
- van Lange P. A. M., Balliet D. P., Parks C. D., Vugt M. (2013). Social Dilemmas: The Psychology of Human Cooperation . Oxford: Oxford University Press. [ Google Scholar ]
- van Vugt M. (2009). Averting the tragedy of the commons: using social psychological science to protect the environment . Curr. Dir. Psychol. Sci. 18 , 169–173. doi: 10.1111/j.1467-8721.2009.01630.x [ CrossRef ] [ Google Scholar ]
- van Vugt M., Griskevicius V., Schultz P. W. (2014). Natrurally green: harnessing stone age psychological biases to foster environmental behaviour . Soc. Issues Policy Rev. 8 , 1–32. doi: 10.1111/sipr.12000 [ CrossRef ] [ Google Scholar ]
- Vis B. (2011). Prospect theory and political decision making . Polit. Stud. Rev. 9 , 334–343. doi: 10.1111/j.1478-9302.2011.00238.x [ CrossRef ] [ Google Scholar ]
- Viscusi W. K., Magat W. A., Huber J. (1987). An investigation of the rationality of consumer valuation of multiple health risks . RAND J. Econ. 18 , 465–479. doi: 10.2307/2555636 [ CrossRef ] [ Google Scholar ]
- Wilson E. O. (2006). The Creation: An Appeal to Save Life on Earth . New York: Norton. [ Google Scholar ]
- Wilson M., Daly M. (2005). Carpe diem: adaptation and devaluing the future . Q. Rev. Biol. 80 , 55–60. doi: 10.1086/431025 [ PubMed ] [ CrossRef ] [ Google Scholar ]
- Wilson T. D., Gilbert D. T. (2005). Affective forecasting: knowing what to want . Curr. Dir. Psychol. Sci. 14 , 131–134. doi: 10.1111/j.0963-7214.2005.00355.x [ CrossRef ] [ Google Scholar ]
- Yasynska K. (2019). Can I Trust My Brain? Brainy Sundays. Available at: https://scanberlin.com/2019/09/29/can-i-trust-my-brain (Accessed November 08, 2021).
What Is Cognitive Bias? 7 Examples & Resources (Incl. Codex)
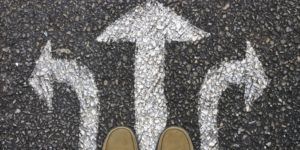
For example, we might:
- Trust someone more if they’re an authority figure than if they’re not
- Assume someone’s gender based on their profession
- Make poor decisions based on the information that we’re given
The reasons for our poor decision making can be a consequence of heuristics and biases. In general, heuristics and biases describe a set of decision-making strategies and the way that we weigh certain types of information. The existing literature on cognitive biases and heuristics is extensive, but this post is a user-friendly summary.
Central to this post’s topic is how cognitive heuristics and biases influence our decision making. We will also learn more about how to overcome them.
Before you continue, we thought you might like to download these Positive CBT Exercises for free . These science-based exercises will provide you with detailed insight into Positive CBT and give you the tools to apply it in your therapy or coaching.
This Article Contains:
What are cognitive biases.
- List and Types of Biases: The Codex
4 Examples of Cognitive Biases
Examples in business and everyday life, role of biases in decision making, 2 popular experiments, 4 ways to overcome your biases, bias modification exercises and activities, a look at cognitive bias modification apps, 5 relevant books, our favorite ted talks on the topic, resources from positivepsychology.com, a take-home message.
When considering the term ‘ cognitive biases ,’ it’s important to note that there is overlap between cognitive biases and heuristics . Sometimes these two terms are used interchangeably, as though they are synonyms; however, their relationship is nuanced.
In his book, Thinking, Fast and Slow , Professor Daniel Kahneman (2011, p. 98) defines heuristics as
“ a simple procedure that helps find adequate, though often imperfect, answers to difficult questions. ”
Tversky and Kahneman (1974, p. 1130) define the relationship between biases and heuristics as follows:
“ … cognitive biases that stem from the reliance on judgmental heuristics. ”
Gonzalez (2017, p. 251) also described the difference between the two terms:
“ Heuristics are the ‘ shortcuts ’ that humans use to reduce task complexity in judgment and choice, and biases are the resulting gaps between normative behavior and the heuristically determined behavior. ”
Lists and Types of Biases: The Codex
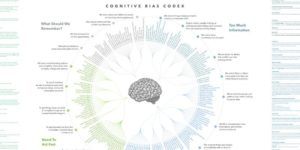
Created by John Manoogian III and Buster Benson, this codex is a useful tool for visually representing all of the known biases that exist to date.
The biases are arranged in a circle and can be divided into four quadrants. Each quadrant is dedicated to a specific group of cognitive biases:
- What should we remember? Biases that affect our memory for people, events, and information
- Too much information Biases that affect how we perceive certain events and people
- Not enough meaning Biases that we use when we have too little information and need to fill in the gaps
- Need to act fast Biases that affect how we make decisions
The Cognitive Bias Codex is a handy visual tool that organizes biases in a meaningful way; however, it is worth pointing out that the codex lists heuristics and biases both as ‘biases.’
If you decide to rely on the Cognitive Bias Codex, then keep in mind the distinction between heuristics and biases mentioned above.
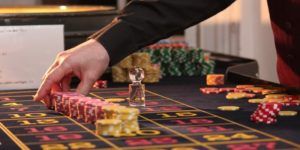
1. Confirmation bias
This bias is based on looking for or overvaluing information that confirms our beliefs or expectations (Edgar & Edgar, 2016; Nickerson, 1998). For example, a police officer who is looking for physical signs of lying might mistakenly classify other behaviors as evidence of lying.
2. Gambler’s fallacy
This false belief describes our tendency to believe that something will happen because it hasn’t happened yet (Ayton & Fischer, 2004; Clotfelter & Cook, 1993).
For example, when betting on a roulette table, if previous outcomes have landed on red, then we might mistakenly assume that the next outcome will be black; however, these events are independent of each other (i.e., the probability of their results do not affect each other).
3. Gender bias
Gender bias describes our tendency to assign specific behavior and characteristics to a particular gender without supporting evidence (Garb, 1997).
For example, complaints of pain are taken more seriously when made by male, rather than female, patients (Gawande, 2014); women are perceived as better caregivers than men (Anthony, 2004); specific clinical syndromes are more readily diagnosed in women than in men (Garb, 1997); and students often rate female lecturers lower than male lecturers (MacNell, Driscoll, & Hunt; 2014; Mitchell & Martin, 2018).
4. Group attribution error
This error describes our tendency to overgeneralize how a group of people will behave based on an interaction with only one person from that group (Pettigrew, 1979).
For example, a negative experience with someone from a different group (e.g., a different culture, gender, religion, political party, etc.) might make us say that all members of that group share the same negative characteristics. Group attribution error forms part of the explanation for prejudice in social psychology.
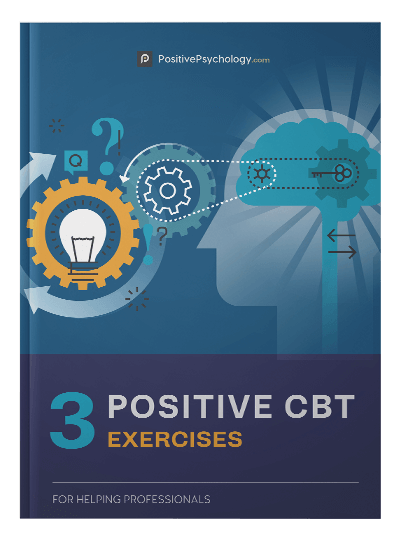
Download 3 Free Positive CBT Exercises (PDF)
These detailed, science-based exercises will equip you or your clients with tools to find new pathways to reduce suffering and more effectively cope with life stressors.
Download 3 Free Positive CBT Tools Pack (PDF)
By filling out your name and email address below.
Gender bias in the workplace is a well-documented and researched area of cognitive bias. Women often do not occupy top senior positions. For example, in 2010, only 15.2% of top positions in US Fortune-500 companies were held by women (Soares, 2010). Women tend to earn less than their male counterparts, and women’s salaries differ according to their marital status.
For example, consider these statistics reported by Güngör and Biernat (2009, p. 232):
“ [In 2005] … 68.1% of married and 79.8% of single mothers in the U.S. participate in the workforce, but while non-mothers earn 90 cents to a man’s dollar, mothers earn 73 cents, and single mothers earn about 60 cents.”
The social desirability bias is a concern for anyone who uses self-report data. Companies that run internal surveys investigating topics that may cast an employee in a poor light must be aware of how the social desirability bias will affect the validity of their data.
Knowing that people adjust their answers to appear more socially desirable, investigators (such as researchers and clinicians) can try to reframe their questions to be less direct, use formal tests, or anonymize responses.
Another sphere of our lives where biases can have devastating effects is in personal finance. According to Hershey, Jacobs-Lawson, and Austin (2012), there are at least 40 cognitive biases that negatively affect our ability to make sound financial decisions, thus hindering our ability to plan for retirement properly. Some of these biases include:
- Halo effect (just because that real estate agent was nice doesn’t mean it’s a good deal)
- Optimistic overconfidence (“I’ll be fine in the future, so I don’t need to save that much now.”)
- Confirmation bias (looking for information to confirm or validate unwise financial decisions)
Below you might find revealing insight into how biases affect our decision making.
The Monty Hall problem
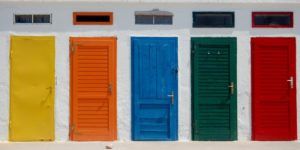
Assume that there are three doors.
- Behind one door is a fantastic prize: a car.
- Behind the other two doors are mediocre prizes: $1,000.
You initially choose Door 1. Before revealing what’s behind your chosen door, the presenter opens a different door, Door 2, to reveal the mediocre prize. The presenter then gives you the option to either keep what’s behind your initial chosen door or change your choice, knowing what’s behind Door 2. What should you do now? Should you stay with your initial choice, Door 1, or should you switch to Door 3?
The correct answer is that you have the best chances of winning the car if you change your choice. This is called the Monty Hall problem. Here’s why you should switch:
- When you made your initial decision, you didn’t know what the outcome would be (mediocre prize versus awesome prize).
- After the host reveals more information, you have a better idea about which prizes are behind which doors.
- Based on this information, you’re more likely to find the car if you change your chosen door, an improvement from odds of 1 in 3 for your initial choice, to 2 in 3 if you switch.
Despite the statistics being in favor of switching, most people are hesitant to abandon their first choice and don’t accept the offer to change it.
Other cognitive biases
The Monty Hall problem is an excellent example of how our intuitions and heuristics lead us to make poor decisions. However, there are lots of other cognitive biases and heuristics that also affect our decision making.
Kahneman, Slovic, Slovic, & Tversky (1982) list 13 biases that arise from the following three heuristics:
- A cognitive bias that may result from this heuristic is that we ignore the base rate of events occurring when making decisions. For example, I am afraid of flying; however, it’s more likely that I might be in a car crash than in a plane crash. Despite this, I still hate flying but am indifferent to hopping into my car.
- For example, when a violent crime occurs in a neighborhood, neighbors in that neighborhood will give a bigger estimate of the frequency of these crimes, compared to the reported statistics. The reason for their overestimate is that the memory of the violent crime is easy to retrieve, which makes it seems like violent crime happens more frequently than it actually does.
- For example, assume that I offer to sell you a car and I ask for $250. You counter with $200. You might think that this is a good deal because you bought the car for less than the asking price; however, your counteroffer was heavily influenced by my asking price, and you’re not likely to deviate too much from it.
To further illustrate the effect of cognitive bias, below are two popular experiments.
1. Anchoring and adjustment
Tversky and Kahneman (1974) found that our estimates are heavily influenced by the first number given to us. For example, participants were asked to estimate the percentage of African countries in the United Nations.
Before giving their answer, each participant had to spin a ‘Wheel of Fortune,’ which would determine their initial starting percentage. The result of the ‘Wheel of Fortune’ was random and meaningless. Despite this, participants’ estimate of African UN member-countries didn’t differ much from whatever random ‘Wheel of Fortune’ amount they landed on, regardless of what that amount was.
2. The attractiveness halo effect
Male students were asked to rate essays written by female authors (Landy & Sigall, 1974). The quality of the essays varied: some were poorly written, and others were well written.
Additionally, some of the essays were accompanied by a photograph of the author (who was either attractive or unattractive), and others were not. Male college students rated the quality of the essay and the talent of the authors higher when:
- the essay was written by an attractive author, and
- this effect was evident only when the essay was of poor quality.
In this study, the male students demonstrated the halo effect, applying the perceived attractiveness of the female author to the quality of the paper.
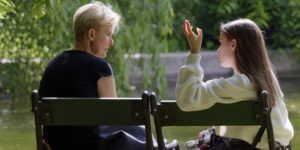
1. Reflect on past decisions
If you’ve been in a similar situation before, you can reflect on the outcomes of those previous decisions to learn how to overcome your biases.
An example of this is budgeting. We tend to underestimate how much money we need to budget for certain areas of our life. However, you can learn how much money to budget by tracking your expenditure for the last few months. Using this information from the past, you can better predict how much money you’ll need for different financial categories in the future.
2. Include external viewpoints
There is some evidence that we make better decisions and negotiations when we consult with other people who are objective, such as mediators and facilitators (Caputo, 2016).
Therefore, before making a decision, talk to other people to consider different viewpoints and have your own views challenged. Importantly, other people might spot your own cognitive biases.
3. Challenge your viewpoints
When making a decision, try to see the weaknesses in your thinking regardless of how small, unlikely, or inconsequential these weaknesses might seem. You can be more confident in your decision if it withstands serious, critical scrutiny.
4. Do not make decisions under pressure
A final way to protect yourself from relying on your cognitive biases is to avoid making any decisions under time pressure. Although it might not feel like it, there are very few instances when you need to make a decision immediately. Here are some tips for making a decision that can have substantial consequences:
- Take the necessary time to ruminate.
- List the pros and cons.
- Talk to friends or family members for advice (but remember that they may have their own biases).
- Try to poke holes in your reasoning.
In the last decade, research has looked at cognitive bias modification (CBM) since cognitive biases are associated with the severity of anxiety and depression. The relationship between cognitive biases and anxiety and depression is assumed to be causal; that is, cognitive biases cause an increase in the severity of symptoms.
CBM exercises are designed with this causal relationship in mind. If the cognitive bias is removed or reduced, then the severity of the symptoms should also lessen.
There are two categories of CBM exercises:
- Changing attentional bias: In this type of exercise, participants are trained to pay more attention to positive stimuli instead of negative stimuli.
- Changing interpretation bias: Participants are primed with positive information before completing an emotionally ambiguous task.
At least six meta-analyses report conflicting findings (Beard, Sawyer, & Hofmann, 2012; Cristea, Kok, & Cuijpers, 2015; Hakamata et al., 2010; Hallion & Ruscio, 2011; Heeren, Mogoașe, Philippot, & McNally, 2015; Mogoaşe, David, & Koster, 2014).
There are many reasons for these differences; for example, the types of studies included, the moderators included, the definition of the interventions, the outcome variable used, the clinical condition studied, and so forth. Therefore, the jury is still out on whether CBM affects symptom severity reliably.
There are many cognitive bias modification apps available for download. Before purchasing an app, research whether the creator of the app has followed sound research principles or done any research when developing the app (Zhang, Ying, Song, Fung, & Smith, 2018).
Most of the bias modification apps aim to change the attentional bias. For example, the following apps aim to train users to respond quicker to happy faces than to sad or angry faces. All hypothesize that repeated use will result in more positive moods.
- Bias Modification
- Upbeat Mind: Positivity Trainer
The Cognitive Bias Cheatsheet is a useful way to remind oneself of the different cognitive biases that exist.
Here is a list of books relevant for anyone interested in cognitive biases.
Firstly, any list about biases would be remiss without Thinking, Fast and Slow by Daniel Kahneman (2011). In this book, Kahneman unpacks some of the most common biases that we experience when making decisions. (Available on Amazon )
In the same vein is The Drunkard’s Walk: How Randomness Rules Our Lives by Leonard Mlodinow (2009). This book addresses how humans misjudge the effect that randomness has on our decision making. (Available on Amazon )
Predictably Irrational by Dan Ariely (2008) is an excellent and very accessible book about how our behavior is often governed by seemingly random and illogical thought processes. The opening chapter is jaw dropping. (Available on Amazon )
Nassim Nicholas Taleb published a series of books – five, in fact – and I include two of them on this list: Fooled by Randomness (2005) and The Black Swan (2007). The entire series discusses various aspects of uncertainty. (Available on Amazon )
We’ve put together a list of our favorite impressive TED talks on cognitive biases.
If you want to learn more about cognitive biases, then these talks are a great jumping-off point:
Are We in Control of Our Own Decisions? – Dan Ariely
Confirmation bias – nassor al hilal.
Confirmation Bias in 5 Minutes – Julia Galef
If you want to learn how to overcome your biases, then we can recommend the following:
How to Outsmart Your Own Unconscious Bias – Valerie Alexander
How to design gender bias out of your workplace – Sara Sanford
Unpacking the biases that shape our beliefs – Mike Hartmann
We have useful resources that you can use when tackling cognitive biases.
First, increasing awareness of Unhelpful Thinking Styles can change the way you think about yourself and your environment. Ultimately, users will increase their awareness of their cognitive biases, and through this awareness, be able to change their behavior.
Our Neutralizing Judgmental Thoughts worksheet is also useful for combating negative thoughts and biases. This exercise helps users apply the CLEAR acronym to adopt a less critical outlook when dealing with others.
The Core Beliefs Worksheet is a useful tool for reflecting on the origin and validity of our core beliefs. This technique might help us ‘step away’ from our biases.
An approach that is always beneficial, is to understand and find ways to apply positive psychology to your every day, and this selection of positive psychology TED Talks is a good starting point.
If you’re looking for more science-based ways to help others through CBT, this collection contains 17 validated positive CBT tools for practitioners. Use them to help others overcome unhelpful thoughts and feelings and develop more positive behaviors.
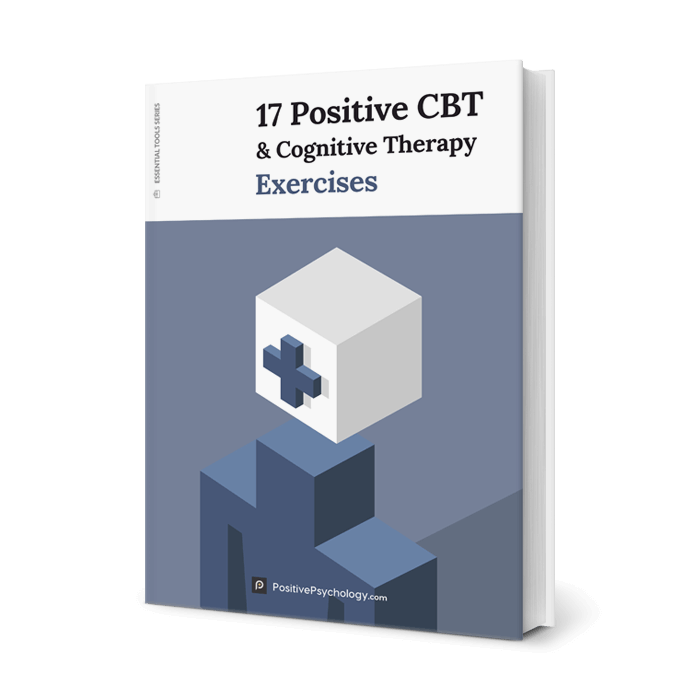
17 Science-Based Ways To Apply Positive CBT
These 17 Positive CBT & Cognitive Therapy Exercises [PDF] include our top-rated, ready-made templates for helping others develop more helpful thoughts and behaviors in response to challenges, while broadening the scope of traditional CBT.
Created by Experts. 100% Science-based.
We often rely on cognitive heuristics and biases when making decisions.
Heuristics can be useful in certain circumstances; however, heuristics and biases can result in poor decision making and reinforce unhealthy behavior.
There are many different types of cognitive biases, and all of us are victim to one or more.
However, being aware of our biases and how they affect our behavior is the first step toward resisting them.
We hope you enjoyed reading this article. For more information, don’t forget to download our three Positive CBT Exercises for free .
- Anthony, A. S. (2004). Gender bias and discrimination in nursing education: Can we change it? Nurse Educator, 29 (3), 121–125.
- Ariely, D. (2008). Predictably irrational. Harper Perennial.
- Ayton, P., & Fischer, I. (2004). The hot hand fallacy and the gambler’s fallacy: Two faces of subjective randomness? Memory & Cognition, 32 (8), 1369–1378.
- Beard, C., Sawyer, A. T., & Hofmann, S. G. (2012). Efficacy of attention bias modification using threat and appetitive stimuli: A meta-analytic review. Behavior Therapy, 43 (4), 724–740.
- Caputo, A. (2016). Overcoming judgmental biases in negotiations: A scenario-based survey analysis on third party direct intervention. Journal of Business Research, 69 (10), 4304–4312.
- Clotfelter, C. T., & Cook, P. J. (1993). The “gambler’s fallacy” in lottery play. Management Science, 39( 12), 1521–1525.
- Cristea, I. A., Kok, R. N., & Cuijpers, P. (2015). Efficacy of cognitive bias modification interventions in anxiety and depression: Meta-analysis. The British Journal of Psychiatry, 206 (1), 7–16.
- Edgar, G., & Edgar, H. (2016). Perception and attention: Errors and accidents. In D. Groome and M.W. Eysenck (Eds.), An introduction to applied cognitive psychology (2nd ed) (pp. 9–38). Routledge.
- Garb, H. N. (1997). Race bias, social class bias, and gender bias in clinical judgment. Clinical Psychology: Science and Practice, 4 (2), 99–120.
- Gawande, A. (2014). Being mortal: Medicine and what matters in the end. Metropolitan Books.
- Gonzalez, C. (2017). Decision-making: A cognitive science perspective. In S. Chipman (Ed.), The Oxford handbook of cognitive science (pp. 249–264). Oxford University Press. Accessed on July 9, 2020 from https://www.cmu.edu/dietrich/sds/ddmlab/papers/oxfordhb-9780199842193-e-6.pdf
- Güngör, G., & Biernat, M. (2009). Gender bias or motherhood disadvantage? Judgments of blue-collar mothers and fathers in the workplace. Sex Roles, 60 (3–4), 232–246.
- Hakamata, Y., Lissek, S., Bar-Haim, Y., Britton, J. C., Fox, N. A., Leibenluft, E., … & Pine, D. S. (2010). Attention bias modification treatment: A meta-analysis toward the establishment of novel treatment for anxiety. Biological Psychiatry, 68 (11), 982–990.
- Hallion, L. S., & Ruscio, A. M. (2011). A meta-analysis of the effect of cognitive bias modification on anxiety and depression. Psychological Bulletin, 137 (6), 940.
- Heeren, A., Mogoașe, C., Philippot, P., & McNally, R. J. (2015). Attention bias modification for social anxiety: A systematic review and meta-analysis. Clinical Psychology Review, 40 , 76–90.
- Hershey, D. A., Jacobs-Lawson, J. M., & Austin, J. T. (2012). Effective financial planning for retirement. In M. Wang (Ed.), Oxford handbook of retirement (pp. 402–430). Oxford University Press.
- Kahneman, D. (2011). Thinking, fast and slow. Farrar, Straus, and Giroux.
- Kahneman, D., Slovic, S. P., Slovic, P., & Tversky, A. (Eds.). (1982). Judgment under uncertainty: Heuristics and biases. Cambridge University Press.
- Landy, D., & Sigall, H. (1974). Beauty is talent: Task evaluation as a function of the performer’s physical attractiveness. Journal of Personality and Social Psychology, 29 (3), 299.
- MacNell, L., Driscoll, A., & Hunt, A. N. (2014). What’s in a name: Exposing gender bias in student ratings of teaching. Innovative Higher Education, 40 (4), 291–303.
- Mitchell, K. M., & Martin, J. (2018). Gender bias in student evaluations. PS: Political Science & Politics, 51 (3), 648–652.
- Mlodinow, L. (2009). The drunkard’s walk: How randomness rules our lives. Vintage.
- Mogoaşe, C., David, D., & Koster, E. H. (2014). Clinical efficacy of attentional bias modification procedures: An updated meta‐analysis. Journal of Clinical Psychology, 70 (12), 1133–1157.
- Nickerson, R. S. (1998). Confirmation bias: A ubiquitous phenomenon in many guises. Review of General Psychology, 2 (2), 175–220.
- Pettigrew, T. F. (1979). The ultimate attribution error: Extending Allport’s cognitive analysis of prejudice. Personality and Social Psychology Bulletin, 5 (4), 461–476.
- Soares, R. (2010). 2010 Catalyst census: Fortune 500 women board directors . Catalyst.
- Taleb, N. N. (2005). Fooled by randomness: The hidden role of chance in life and in the markets (vol. 1). Random House.
- Taleb, N. N. (2007). The black swan: The impact of the highly improbable (vol. 2). Random House.
- Tversky, A., & Kahneman, D. (1974). Judgment under uncertainty: Heuristics and biases. Science, 185 , 1124–1131.
- Zhang, M., Ying, J., Song, G., Fung, D. S., & Smith, H. (2018). Attention and cognitive bias modification apps: Review of the literature and of commercially available apps. JMIR mHealth and uHealth , 6 (5).

Share this article:
Article feedback
What our readers think.

Dumb. I wish the author would have used the “4 Ways to Overcome Bias” prior to writing this irrelevant article. Then maybe the contradictions could have been avoided such as the codex containing both heuristics and biases or the gender bias and marital status. Leading off with gender bias, by the way, is a dead giveaway that this is just propaganda disguised as pseudo-intellectual tripe. The author painted the issue as “women make less, so = bias.” Allow me to use the group attribution bias and call this as another article from majority female psychology “profession.”

I agree that awareness of our biases is the first step to overcoming them. But I think it’s important to also understand that we all have biases, and that they’re not necessarily a bad thing. We need biases to make decisions, and without them we would be paralyzed. The key is to be aware of our biases and to try to overcome them when they lead us astray.

You may be conflating heuristics with bias. Heuristics are shortcuts to see pattern and simplify things based on our experience and intuition. Heuristics are needed to make decisions and solve problems. But heuristics are also prone to thinking errors. Biases are thinking errors.

Thank you for this feast of information that I will be savoring over and over for weeks.

Thank you very much for the detailed information and resources. I plan to utilize this as a link with certain highlighted components for my social psychology unit with my students.

VERY GOOD ARTICLE. MAY USE IT TO TRAIN MY STUDENTS RESILIENCE AND GRIT AND PERCEPTIVE CAPACITY IN COUNSELLING SESSIONS
PATRICK MITI

Thanks for the detailed blog. I’m going to provide this as a link on my critical thinking course that I teach at a university.
Let us know your thoughts Cancel reply
Your email address will not be published.
Save my name, email, and website in this browser for the next time I comment.
Related articles
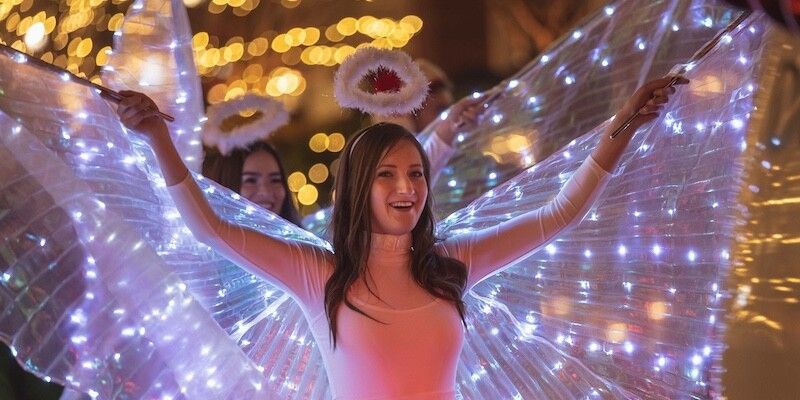
Halo Effect: Why We Judge a Book by Its Cover
Even though we may consider ourselves logical and rational, it appears we are easily biased by a single incident or individual characteristic (Nicolau, Mellinas, & [...]
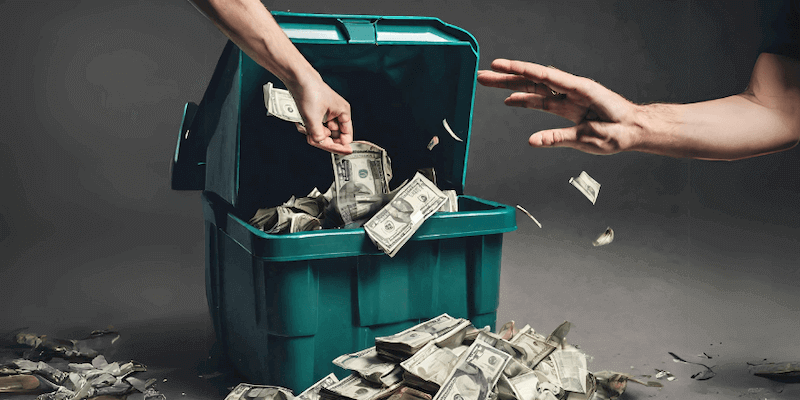
Sunk Cost Fallacy: Why We Can’t Let Go
If you’ve continued with a decision or an investment of time, money, or resources long after you should have stopped, you’ve succumbed to the ‘sunk [...]
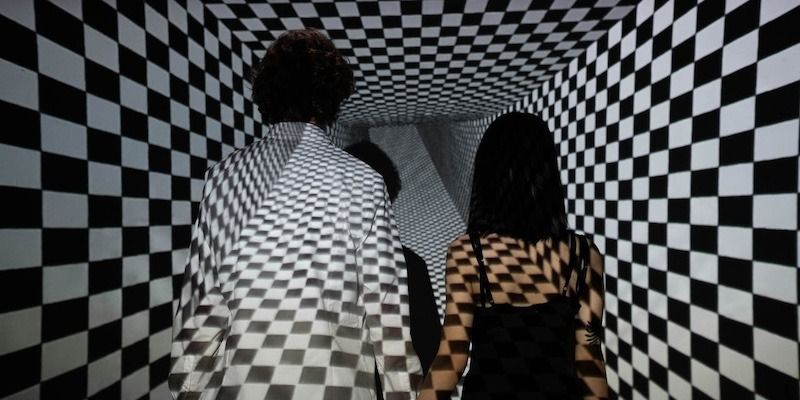
Confirmation Bias: Seeing What We Want to Believe
Believe it or not, we can’t always trust what we see or hear. It seems our memory is influenced by our expectations (Eysenk & Keane, [...]
Read other articles by their category
- Body & Brain (49)
- Coaching & Application (58)
- Compassion (25)
- Counseling (51)
- Emotional Intelligence (23)
- Gratitude (18)
- Grief & Bereavement (21)
- Happiness & SWB (40)
- Meaning & Values (26)
- Meditation (20)
- Mindfulness (44)
- Motivation & Goals (45)
- Optimism & Mindset (34)
- Positive CBT (29)
- Positive Communication (20)
- Positive Education (47)
- Positive Emotions (32)
- Positive Leadership (18)
- Positive Parenting (15)
- Positive Psychology (34)
- Positive Workplace (37)
- Productivity (17)
- Relationships (43)
- Resilience & Coping (37)
- Self Awareness (21)
- Self Esteem (38)
- Strengths & Virtues (32)
- Stress & Burnout Prevention (34)
- Theory & Books (46)
- Therapy Exercises (37)
- Types of Therapy (63)
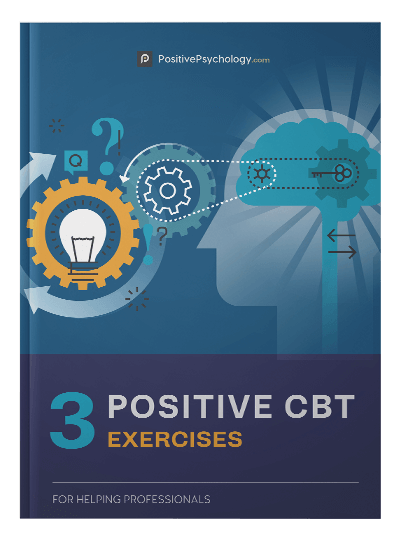
3 Positive CBT Exercises (PDF)
Cognitive Bias: How We Are Wired to Misjudge
Charlotte Ruhl
Research Assistant & Psychology Graduate
BA (Hons) Psychology, Harvard University
Charlotte Ruhl, a psychology graduate from Harvard College, boasts over six years of research experience in clinical and social psychology. During her tenure at Harvard, she contributed to the Decision Science Lab, administering numerous studies in behavioral economics and social psychology.
Learn about our Editorial Process
Saul Mcleod, PhD
Editor-in-Chief for Simply Psychology
BSc (Hons) Psychology, MRes, PhD, University of Manchester
Saul Mcleod, PhD., is a qualified psychology teacher with over 18 years of experience in further and higher education. He has been published in peer-reviewed journals, including the Journal of Clinical Psychology.
Olivia Guy-Evans, MSc
Associate Editor for Simply Psychology
BSc (Hons) Psychology, MSc Psychology of Education
Olivia Guy-Evans is a writer and associate editor for Simply Psychology. She has previously worked in healthcare and educational sectors.
On This Page:
Have you ever been so busy talking on the phone that you don’t notice the light has turned green and it is your turn to cross the street?
Have you ever shouted, “I knew that was going to happen!” after your favorite baseball team gave up a huge lead in the ninth inning and lost?
Or have you ever found yourself only reading news stories that further support your opinion?
These are just a few of the many instances of cognitive bias that we experience every day of our lives. But before we dive into these different biases, let’s backtrack first and define what bias is.
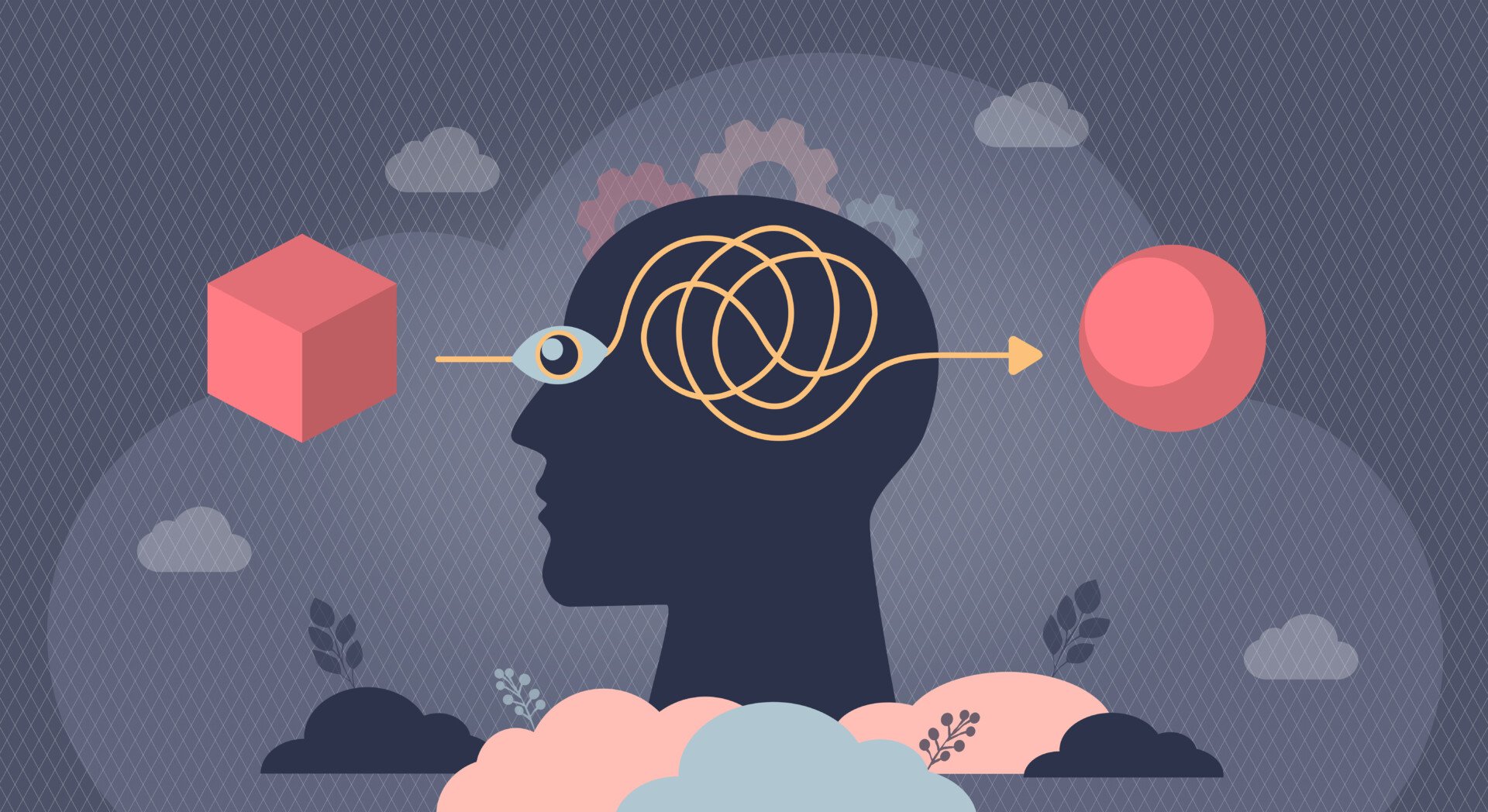
What is Cognitive Bias?
Cognitive bias is a systematic error in thinking, affecting how we process information, perceive others, and make decisions. It can lead to irrational thoughts or judgments and is often based on our perceptions, memories, or individual and societal beliefs.
Biases are unconscious and automatic processes designed to make decision-making quicker and more efficient. Cognitive biases can be caused by many things, such as heuristics (mental shortcuts) , social pressures, and emotions.
Broadly speaking, bias is a tendency to lean in favor of or against a person, group, idea, or thing, usually in an unfair way. Biases are natural — they are a product of human nature — and they don’t simply exist in a vacuum or in our minds — they affect the way we make decisions and act.
In psychology, there are two main branches of biases: conscious and unconscious. Conscious or explicit bias is intentional — you are aware of your attitudes and the behaviors resulting from them (Lang, 2019).
Explicit bias can be good because it helps provide you with a sense of identity and can lead you to make good decisions (for example, being biased towards healthy foods).
However, these biases can often be dangerous when they take the form of conscious stereotyping.
On the other hand, unconscious bias , or cognitive bias, represents a set of unintentional biases — you are unaware of your attitudes and behaviors resulting from them (Lang, 2019).
Cognitive bias is often a result of your brain’s attempt to simplify information processing — we receive roughly 11 million bits of information per second. Still, we can only process about 40 bits of information per second (Orzan et al., 2012).
Therefore, we often rely on mental shortcuts (called heuristics) to help make sense of the world with relative speed. As such, these errors tend to arise from problems related to thinking: memory, attention, and other mental mistakes.
Cognitive biases can be beneficial because they do not require much mental effort and can allow you to make decisions relatively quickly, but like conscious biases, unconscious biases can also take the form of harmful prejudice that serves to hurt an individual or a group.
Although it may feel like there has been a recent rise of unconscious bias, especially in the context of police brutality and the Black Lives Matter movement, this is not a new phenomenon.
Thanks to Tversky and Kahneman (and several other psychologists who have paved the way), we now have an existing dictionary of our cognitive biases.
Again, these biases occur as an attempt to simplify the complex world and make information processing faster and easier. This section will dive into some of the most common forms of cognitive bias.
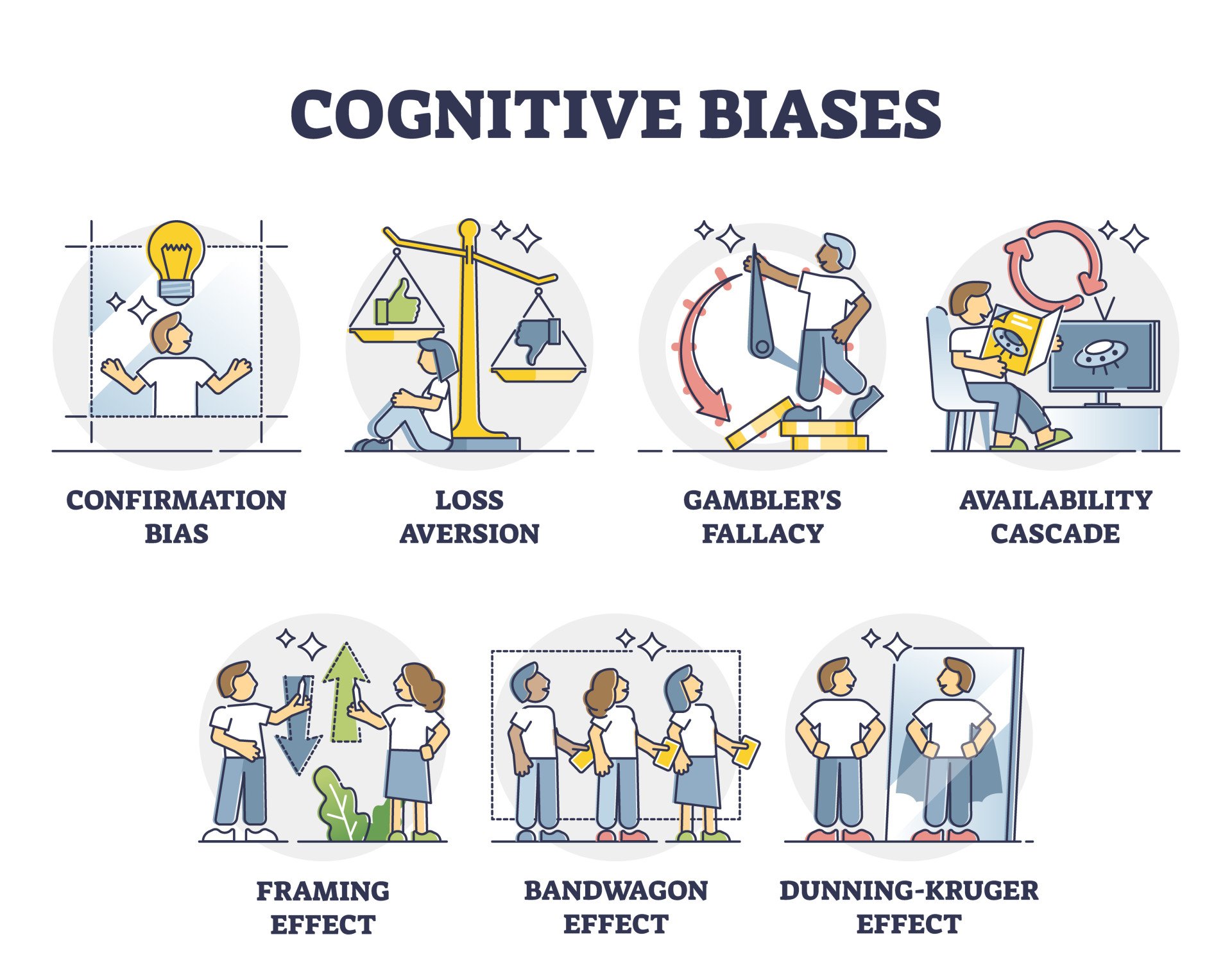
Confirmation Bias
Confirmation bias is the tendency to interpret new information as confirmation of your preexisting beliefs and opinions while giving disproportionately less consideration to alternative possibilities.
Real-World Examples
Since Watson’s 1960 experiment, real-world examples of confirmation bias have gained attention.
This bias often seeps into the research world when psychologists selectively interpret data or ignore unfavorable data to produce results that support their initial hypothesis.
Confirmation bias is also incredibly pervasive on the internet, particularly with social media. We tend to read online news articles that support our beliefs and fail to seek out sources that challenge them.
Various social media platforms, such as Facebook, help reinforce our confirmation bias by feeding us stories that we are likely to agree with – further pushing us down these echo chambers of political polarization.
Some examples of confirmation bias are especially harmful, specifically in the context of the law. For example, a detective may identify a suspect early in an investigation, seek out confirming evidence, and downplay falsifying evidence.
Experiments
The confirmation bias dates back to 1960 when Peter Wason challenged participants to identify a rule applying to triples of numbers.
People were first told that the sequences 2, 4, 6 fit the rule, and they then had to generate triples of their own and were told whether that sequence fits the rule. The rule was simple: any ascending sequence.
But not only did participants have an unusually difficult time realizing this and instead devised overly-complicated hypotheses, they also only generated triples that confirmed their preexisting hypothesis (Wason, 1960).
Explanations
But why does confirmation bias occur? It’s partially due to the effect of desire on our beliefs. In other words, certain desired conclusions (ones that support our beliefs) are more likely to be processed by the brain and labeled as true (Nickerson, 1998).
This motivational explanation is often coupled with a more cognitive theory.
The cognitive explanation argues that because our minds can only focus on one thing at a time, it is hard to parallel process (see information processing for more information) alternate hypotheses, so, as a result, we only process the information that aligns with our beliefs (Nickerson, 1998).
Another theory explains confirmation bias as a way of enhancing and protecting our self-esteem.
As with the self-serving bias (see more below), our minds choose to reinforce our preexisting ideas because being right helps preserve our sense of self-esteem, which is important for feeling secure in the world and maintaining positive relationships (Casad, 2019).
Although confirmation bias has obvious consequences, you can still work towards overcoming it by being open-minded and willing to look at situations from a different perspective than you might be used to (Luippold et al., 2015).
Even though this bias is unconscious, training your mind to become more flexible in its thought patterns will help mitigate the effects of this bias.
Hindsight Bias
Hindsight bias refers to the tendency to perceive past events as more predictable than they actually were (Roese & Vohs, 2012). There are cognitive and motivational explanations for why we ascribe so much certainty to knowing the outcome of an event only once the event is completed.
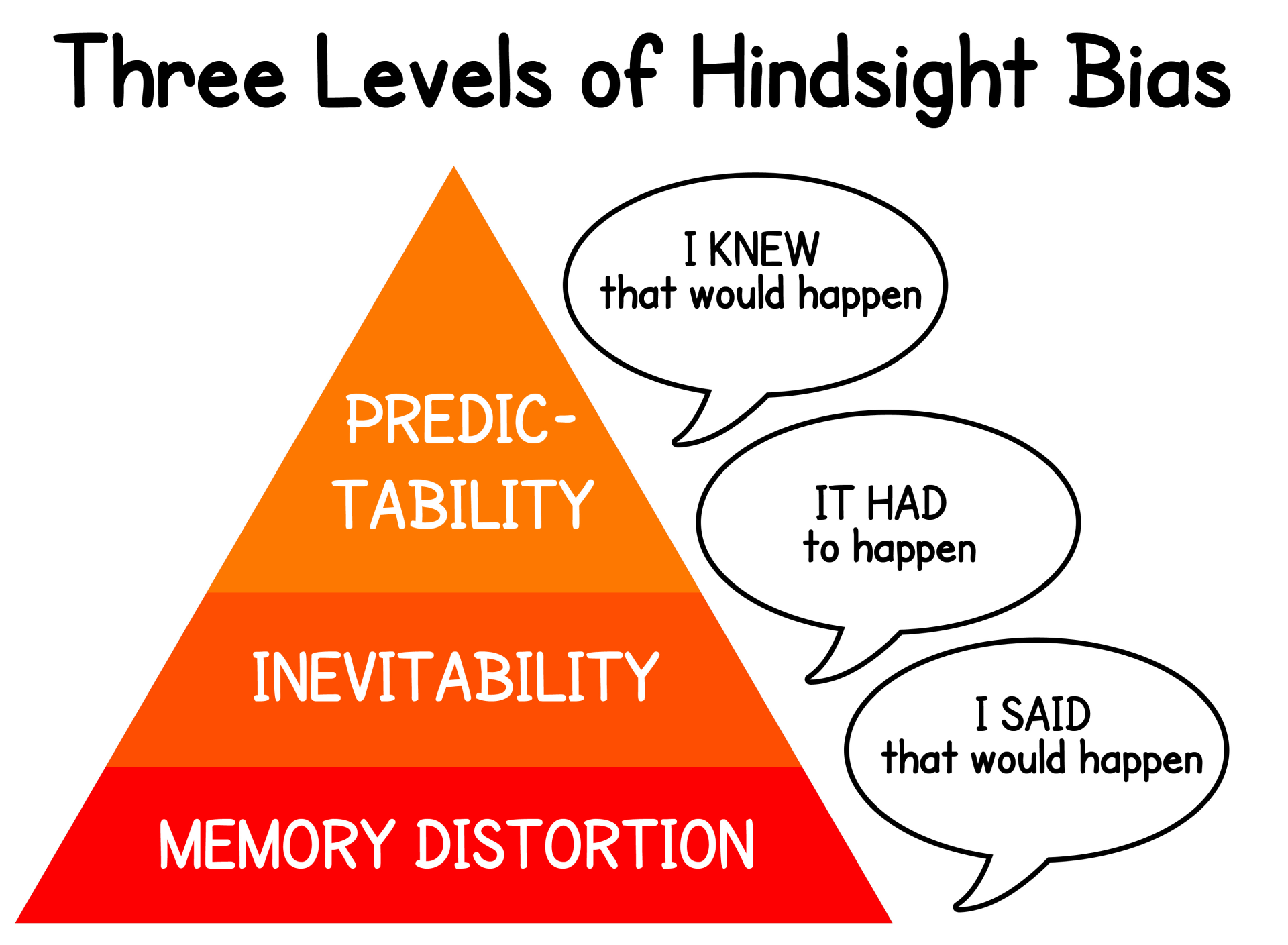
When sports fans know the outcome of a game, they often question certain decisions coaches make that they otherwise would not have questioned or second-guessed.
And fans are also quick to remark that they knew their team was going to win or lose, but, of course, they only make this statement after their team actually did win or lose.
Although research studies have demonstrated that the hindsight bias isn’t necessarily mitigated by pure recognition of the bias (Pohl & Hell, 1996).
You can still make a conscious effort to remind yourself that you can’t predict the future and motivate yourself to consider alternate explanations.
It’s important to do all we can to reduce this bias because when we are overly confident about our ability to predict outcomes, we might make future risky decisions that could have potentially dangerous outcomes.
Building on Tversky and Kahneman’s growing list of heuristics, researchers Baruch Fischhoff and Ruth Beyth-Marom (1975) were the first to directly investigate the hindsight bias in the empirical setting.
The team asked participants to judge the likelihood of several different outcomes of former U.S. president Richard Nixon’s visit to Beijing and Moscow.
After Nixon returned back to the States, participants were asked to recall the likelihood of each outcome they had initially assigned.
Fischhoff and Beyth found that for events that actually occurred, participants greatly overestimated the initial likelihood they assigned to those events.
That same year, Fischhoff (1975) introduced a new method for testing the hindsight bias – one that researchers still use today.
Participants are given a short story with four possible outcomes, and they are told that one is true. When they are then asked to assign the likelihood of each specific outcome, they regularly assign a higher likelihood to whichever outcome they have been told is true, regardless of how likely it actually is.
But hindsight bias does not only exist in artificial settings. In 1993, Dorothee Dietrich and Matthew Olsen asked college students to predict how the U.S. Senate would vote on the confirmation of Supreme Court nominee Clarence Thomas.
Before the vote, 58% of participants predicted that he would be confirmed, but after his actual confirmation, 78% of students said that they thought he would be approved – a prime example of the hindsight bias. And this form of bias extends beyond the research world.
From the cognitive perspective, hindsight bias may result from distortions of memories of what we knew or believed to know before an event occurred (Inman, 2016).
It is easier to recall information that is consistent with our current knowledge, so our memories become warped in a way that agrees with what actually did happen.
Motivational explanations of the hindsight bias point to the fact that we are motivated to live in a predictable world (Inman, 2016).
When surprising outcomes arise, our expectations are violated, and we may experience negative reactions as a result. Thus, we rely on the hindsight bias to avoid these adverse responses to certain unanticipated events and reassure ourselves that we actually did know what was going to happen.
Self-Serving Bias
Self-serving bias is the tendency to take personal responsibility for positive outcomes and blame external factors for negative outcomes.
You would be right to ask how this is similar to the fundamental attribution error (Ross, 1977), which identifies our tendency to overemphasize internal factors for other people’s behavior while attributing external factors to our own.
The distinction is that the self-serving bias is concerned with valence. That is, how good or bad an event or situation is. And it is also only concerned with events for which you are the actor.
In other words, if a driver cuts in front of you as the light turns green, the fundamental attribution error might cause you to think that they are a bad person and not consider the possibility that they were late for work.
On the other hand, the self-serving bias is exercised when you are the actor. In this example, you would be the driver cutting in front of the other car, which you would tell yourself is because you are late (an external attribution to a negative event) as opposed to it being because you are a bad person.
From sports to the workplace, self-serving bias is incredibly common. For example, athletes are quick to take responsibility for personal wins, attributing their successes to their hard work and mental toughness, but point to external factors, such as unfair calls or bad weather, when they lose (Allen et al., 2020).
In the workplace, people attribute internal factors when they have hired for a job but external factors when they are fired (Furnham, 1982). And in the office itself, workplace conflicts are given external attributions, and successes, whether a persuasive presentation or a promotion, are awarded internal explanations (Walther & Bazarova, 2007).
Additionally, self-serving bias is more prevalent in individualistic cultures , which place emphasis on self-esteem levels and individual goals, and it is less prevalent among individuals with depression (Mezulis et al., 2004), who are more likely to take responsibility for negative outcomes.
Overcoming this bias can be difficult because it is at the expense of our self-esteem. Nevertheless, practicing self-compassion – treating yourself with kindness even when you fall short or fail – can help reduce the self-serving bias (Neff, 2003).
The leading explanation for the self-serving bias is that it is a way of protecting our self-esteem (similar to one of the explanations for the confirmation bias).
We are quick to take credit for positive outcomes and divert the blame for negative ones to boost and preserve our individual ego, which is necessary for confidence and healthy relationships with others (Heider, 1982).
Another theory argues that self-serving bias occurs when surprising events arise. When certain outcomes run counter to our expectations, we ascribe external factors, but when outcomes are in line with our expectations, we attribute internal factors (Miller & Ross, 1975).
An extension of this theory asserts that we are naturally optimistic, so negative outcomes come as a surprise and receive external attributions as a result.
Anchoring Bias
individualistic cultures is closely related to the decision-making process. It occurs when we rely too heavily on either pre-existing information or the first piece of information (the anchor) when making a decision.
For example, if you first see a T-shirt that costs $1,000 and then see a second one that costs $100, you’re more likely to see the second shirt as cheap as you would if the first shirt you saw was $120. Here, the price of the first shirt influences how you view the second.
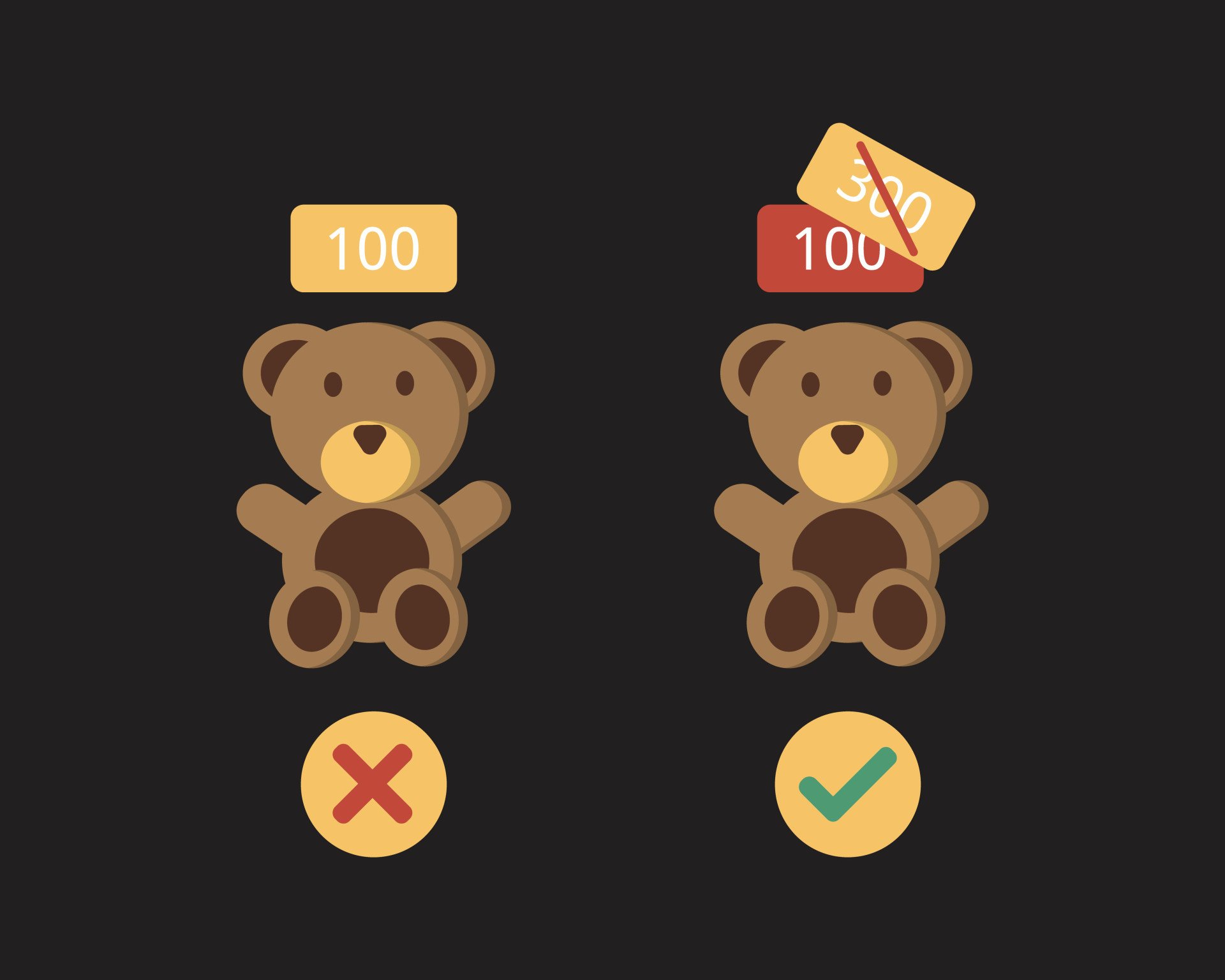
Sarah is looking to buy a used car. The first dealership she visits has a used sedan listed for $19,000. Sarah takes this initial listing price as an anchor and uses it to evaluate prices at other dealerships.
When she sees another similar used sedan priced at $18,000, that price seems like a good bargain compared to the $19,000 anchor price she saw first, even though the actual market value is closer to $16,000.
When Sarah finds a comparable used sedan priced at $15,500, she continues perceiving that price as cheap compared to her anchored reference price.
Ultimately, Sarah purchases the $18,000 sedan, overlooking that all of the prices seemed like bargains only in relation to the initial high anchor price.
The key elements that demonstrate anchoring bias here are:
- Sarah establishes an initial reference price based on the first listing she sees ($19k)
- She uses that initial price as her comparison/anchor for evaluating subsequent prices
- This biases her perception of the market value of the cars she looks at after the initial anchor is set
- She makes a purchase decision aligned with her anchored expectations rather than a more objective market value
Multiple theories seek to explain the existence of this bias.
One theory, known as anchoring and adjustment, argues that once an anchor is established, people insufficiently adjust away from it to arrive at their final answer, and so their final guess or decision is closer to the anchor than it otherwise would have been (Tversky & Kahneman, 1992).
And when people experience a greater cognitive load (the amount of information the working memory can hold at any given time; for example, a difficult decision as opposed to an easy one), they are more susceptible to the effects of anchoring.
Another theory, selective accessibility, holds that although we assume that the anchor is not a suitable answer (or a suitable price going back to the initial example) when we evaluate the second stimulus (or second shirt), we look for ways in which it is similar or different to the anchor (the price being way different), resulting in the anchoring effect (Mussweiler & Strack, 1999).
A final theory posits that providing an anchor changes someone’s attitudes to be more favorable to the anchor, which then biases future answers to have similar characteristics as the initial anchor.
Although there are many different theories for why we experience anchoring bias, they all agree that it affects our decisions in real ways (Wegner et al., 2001).
The first study that brought this bias to light was during one of Tversky and Kahneman’s (1974) initial experiments. They asked participants to compute the product of numbers 1-8 in five seconds, either as 1x2x3… or 8x7x6…
Participants did not have enough time to calculate the answer, so they had to estimate based on their first few calculations.
They found that those who computed the small multiplications first (i.e., 1x2x3…) gave a median estimate of 512, but those who computed the larger multiplications first gave a median estimate of 2,250 (although the actual answer is 40,320).
This demonstrates how the initial few calculations influenced the participant’s final answer.
Availability Bias
Availability bias (also commonly referred to as the availability heuristic ) refers to the tendency to think that examples of things that readily come to mind are more common than what is actually the case.
In other words, information that comes to mind faster influences the decisions we make about the future. And just like with the hindsight bias, this bias is related to an error of memory.
But instead of being a memory fabrication, it is an overemphasis on a certain memory.
In the workplace, if someone is being considered for a promotion but their boss recalls one bad thing that happened years ago but left a lasting impression, that one event might have an outsized influence on the final decision.
Another common example is buying lottery tickets because the lifestyle and benefits of winning are more readily available in mind (and the potential emotions associated with winning or seeing other people win) than the complex probability calculation of actually winning the lottery (Cherry, 2019).
A final common example that is used to demonstrate the availability heuristic describes how seeing several television shows or news reports about shark attacks (or anything that is sensationalized by the news, such as serial killers or plane crashes) might make you think that this incident is relatively common even though it is not at all.
Regardless, this thinking might make you less inclined to go in the water the next time you go to the beach (Cherry, 2019).
As with most cognitive biases, the best way to overcome them is by recognizing the bias and being more cognizant of your thoughts and decisions.
And because we fall victim to this bias when our brain relies on quick mental shortcuts in order to save time, slowing down our thinking and decision-making process is a crucial step to mitigating the effects of the availability heuristic.
Researchers think this bias occurs because the brain is constantly trying to minimize the effort necessary to make decisions, and so we rely on certain memories – ones that we can recall more easily – instead of having to endure the complicated task of calculating statistical probabilities.
Two main types of memories are easier to recall: 1) those that more closely align with the way we see the world and 2) those that evoke more emotion and leave a more lasting impression.
This first type of memory was identified in 1973, when Tversky and Kahneman, our cognitive bias pioneers, conducted a study in which they asked participants if more words begin with the letter K or if more words have K as their third letter.
Although many more words have K as their third letter, 70% of participants said that more words begin with K because the ability to recall this is not only easier, but it more closely aligns with the way they see the world (knowing the first letter of any word is infinitely more common than the third letter of any word).
In terms of the second type of memory, the same duo ran an experiment in 1983, 10 years later, where half the participants were asked to guess the likelihood of a massive flood would occur somewhere in North America, and the other half had to guess the likelihood of a flood occurring due to an earthquake in California.
Although the latter is much less likely, participants still said that this would be much more common because they could recall specific, emotionally charged events of earthquakes hitting California, largely due to the news coverage they receive.
Together, these studies highlight how memories that are easier to recall greatly influence our judgments and perceptions about future events.
Inattentional Blindness
A final popular form of cognitive bias is inattentional blindness . This occurs when a person fails to notice a stimulus that is in plain sight because their attention is directed elsewhere.
For example, while driving a car, you might be so focused on the road ahead of you that you completely fail to notice a car swerve into your lane of traffic.
Because your attention is directed elsewhere, you aren’t able to react in time, potentially leading to a car accident. Experiencing inattentional blindness has its obvious consequences (as illustrated by this example), but, like all biases, it is not impossible to overcome.
Many theories seek to explain why we experience this form of cognitive bias. In reality, it is probably some combination of these explanations.
Conspicuity holds that certain sensory stimuli (such as bright colors) and cognitive stimuli (such as something familiar) are more likely to be processed, and so stimuli that don’t fit into one of these two categories might be missed.
The mental workload theory describes how when we focus a lot of our brain’s mental energy on one stimulus, we are using up our cognitive resources and won’t be able to process another stimulus simultaneously.
Similarly, some psychologists explain how we attend to different stimuli with varying levels of attentional capacity, which might affect our ability to process multiple stimuli simultaneously.
In other words, an experienced driver might be able to see that car swerve into the lane because they are using fewer mental resources to drive, whereas a beginner driver might be using more resources to focus on the road ahead and unable to process that car swerving in.
A final explanation argues that because our attentional and processing resources are limited, our brain dedicates them to what fits into our schemas or our cognitive representations of the world (Cherry, 2020).
Thus, when an unexpected stimulus comes into our line of sight, we might not be able to process it on the conscious level. The following example illustrates how this might happen.
The most famous study to demonstrate the inattentional blindness phenomenon is the invisible gorilla study (Most et al., 2001). This experiment asked participants to watch a video of two groups passing a basketball and count how many times the white team passed the ball.
Participants are able to accurately report the number of passes, but what they fail to notice is a gorilla walking directly through the middle of the circle.
Because this would not be expected, and because our brain is using up its resources to count the number of passes, we completely fail to process something right before our eyes.
A real-world example of inattentional blindness occurred in 1995 when Boston police officer Kenny Conley was chasing a suspect and ran by a group of officers who were mistakenly holding down an undercover cop.
Conley was convicted of perjury and obstruction of justice because he supposedly saw the fight between the undercover cop and the other officers and lied about it to protect the officers, but he stood by his word that he really hadn’t seen it (due to inattentional blindness) and was ultimately exonerated (Pickel, 2015).
The key to overcoming inattentional blindness is to maximize your attention by avoiding distractions such as checking your phone. And it is also important to pay attention to what other people might not notice (if you are that driver, don’t always assume that others can see you).
By working on expanding your attention and minimizing unnecessary distractions that will use up your mental resources, you can work towards overcoming this bias.
Preventing Cognitive Bias
As we know, recognizing these biases is the first step to overcoming them. But there are other small strategies we can follow in order to train our unconscious mind to think in different ways.
From strengthening our memory and minimizing distractions to slowing down our decision-making and improving our reasoning skills, we can work towards overcoming these cognitive biases.
An individual can evaluate his or her own thought process, also known as metacognition (“thinking about thinking”), which provides an opportunity to combat bias (Flavell, 1979).
This multifactorial process involves (Croskerry, 2003):
(a) acknowledging the limitations of memory, (b) seeking perspective while making decisions, (c) being able to self-critique, (d) choosing strategies to prevent cognitive error.
Many strategies used to avoid bias that we describe are also known as cognitive forcing strategies, which are mental tools used to force unbiased decision-making.
The History of Cognitive Bias
The term cognitive bias was first coined in the 1970s by Israeli psychologists Amos Tversky and Daniel Kahneman, who used this phrase to describe people’s flawed thinking patterns in response to judgment and decision problems (Tversky & Kahneman, 1974).
Tversky and Kahneman’s research program, the heuristics and biases program, investigated how people make decisions given limited resources (for example, limited time to decide which food to eat or limited information to decide which house to buy).
As a result of these limited resources, people are forced to rely on heuristics or quick mental shortcuts to help make their decisions.
Tversky and Kahneman wanted to understand the biases associated with this judgment and decision-making process.
To do so, the two researchers relied on a research paradigm that presented participants with some type of reasoning problem with a computed normative answer (they used probability theory and statistics to compute the expected answer).
Participants’ responses were then compared with the predetermined solution to reveal the systematic deviations in the mind.
After running several experiments with countless reasoning problems, the researchers were able to identify numerous norm violations that result when our minds rely on these cognitive biases to make decisions and judgments (Wilke & Mata, 2012).
Key Takeaways
- Cognitive biases are unconscious errors in thinking that arise from problems related to memory, attention, and other mental mistakes.
- These biases result from our brain’s efforts to simplify the incredibly complex world in which we live.
- Confirmation bias , hindsight bias, mere exposure effect , self-serving bias , base rate fallacy , anchoring bias , availability bias , the framing effect , inattentional blindness, and the ecological fallacy are some of the most common examples of cognitive bias. Another example is the false consensus effect .
- Cognitive biases directly affect our safety, interactions with others, and how we make judgments and decisions in our daily lives.
- Although these biases are unconscious, there are small steps we can take to train our minds to adopt a new pattern of thinking and mitigate the effects of these biases.
Allen, M. S., Robson, D. A., Martin, L. J., & Laborde, S. (2020). Systematic review and meta-analysis of self-serving attribution biases in the competitive context of organized sport. Personality and Social Psychology Bulletin, 46 (7), 1027-1043.
Casad, B. (2019). Confirmation bias . Retrieved from https://www.britannica.com/science/confirmation-bias
Cherry, K. (2019). How the availability heuristic affects your decision-making . Retrieved from https://www.verywellmind.com/availability-heuristic-2794824
Cherry, K. (2020). Inattentional blindness can cause you to miss things in front of you . Retrieved from https://www.verywellmind.com/what-is-inattentional-blindness-2795020
Dietrich, D., & Olson, M. (1993). A demonstration of hindsight bias using the Thomas confirmation vote. Psychological Reports, 72 (2), 377-378.
Fischhoff, B. (1975). Hindsight is not equal to foresight: The effect of outcome knowledge on judgment under uncertainty. Journal of Experimental Psychology: Human Perception and Performance, 1 (3), 288.
Fischhoff, B., & Beyth, R. (1975). I knew it would happen: Remembered probabilities of once—future things. Organizational Behavior and Human Performance, 13 (1), 1-16.
Furnham, A. (1982). Explanations for unemployment in Britain. European Journal of social psychology, 12(4), 335-352.
Heider, F. (1982). The psychology of interpersonal relations . Psychology Press.
Inman, M. (2016). Hindsight bias . Retrieved from https://www.britannica.com/topic/hindsight-bias
Lang, R. (2019). What is the difference between conscious and unconscious bias? : Faqs. Retrieved from https://engageinlearning.com/faq/compliance/unconscious-bias/what-is-the-difference-between-conscious-and-unconscious-bias/
Luippold, B., Perreault, S., & Wainberg, J. (2015). Auditor’s pitfall: Five ways to overcome confirmation bias . Retrieved from https://www.babson.edu/academics/executive-education/babson-insight/finance-and-accounting/auditors-pitfall-five-ways-to-overcome-confirmation-bias/
Mezulis, A. H., Abramson, L. Y., Hyde, J. S., & Hankin, B. L. (2004). Is there a universal positivity bias in attributions? A meta-analytic review of individual, developmental, and cultural differences in the self-serving attributional bias. Psychological Bulletin, 130 (5), 711.
Miller, D. T., & Ross, M. (1975). Self-serving biases in the attribution of causality: Fact or fiction?. Psychological Bulletin, 82 (2), 213.
Most, S. B., Simons, D. J., Scholl, B. J., Jimenez, R., Clifford, E., & Chabris, C. F. (2001). How not to be seen: The contribution of similarity and selective ignoring to sustained inattentional blindness. Psychological Science, 12 (1), 9-17.
Mussweiler, T., & Strack, F. (1999). Hypothesis-consistent testing and semantic priming in the anchoring paradigm: A selective accessibility model. Journal of Experimental Social Psychology, 35 (2), 136-164.
Neff, K. (2003). Self-compassion: An alternative conceptualization of a healthy attitude toward oneself. Self and Identity, 2 (2), 85-101.
Nickerson, R. S. (1998). Confirmation bias: A ubiquitous phenomenon in many guises. Review of General Psychology, 2 (2), 175-220.
Orzan, G., Zara, I. A., & Purcarea, V. L. (2012). Neuromarketing techniques in pharmaceutical drugs advertising. A discussion and agenda for future research. Journal of Medicine and Life, 5 (4), 428.
Pickel, K. L. (2015). Eyewitness memory. The handbook of attention , 485-502.
Pohl, R. F., & Hell, W. (1996). No reduction in hindsight bias after complete information and repeated testing. Organizational Behavior and Human Decision Processes, 67 (1), 49-58.
Roese, N. J., & Vohs, K. D. (2012). Hindsight bias. Perspectives on Psychological Science, 7 (5), 411-426.
Ross, L. (1977). The intuitive psychologist and his shortcomings: Distortions in the attribution process. In Advances in experimental social psychology (Vol. 10, pp. 173-220). Academic Press.
Tversky, A., & Kahneman, D. (1973). Availability: A heuristic for judging frequency and probability. Cognitive Psychology, 5 (2), 207-232.
Tversky, A., & Kahneman, D. (1974). Judgment under uncertainty: Heuristics and biases. Science, 185 (4157), 1124-1131.
Tversky, A., & Kahneman, D. (1983). Extensional versus intuitive reasoning: The conjunction fallacy in probability judgment. Psychological Review , 90(4), 293.
Tversky, A., & Kahneman, D. (1992). Advances in prospect theory: Cumulative representation of uncertainty. Journal of Risk and Uncertainty, 5 (4), 297-323.
Walther, J. B., & Bazarova, N. N. (2007). Misattribution in virtual groups: The effects of member distribution on self-serving bias and partner blame. Human Communication Research, 33 (1), 1-26.
Wason, Peter C. (1960), “On the failure to eliminate hypotheses in a conceptual task”. Quarterly Journal of Experimental Psychology, 12 (3): 129–40.
Wegener, D. T., Petty, R. E., Detweiler-Bedell, B. T., & Jarvis, W. B. G. (2001). Implications of attitude change theories for numerical anchoring: Anchor plausibility and the limits of anchor effectiveness. Journal of Experimental Social Psychology, 37 (1), 62-69.
Wilke, A., & Mata, R. (2012). Cognitive bias. In Encyclopedia of human behavior (pp. 531-535). Academic Press.
Further Information
Test yourself for bias.
- Project Implicit (IAT Test) From Harvard University
- Implicit Association Test From the Social Psychology Network
- Test Yourself for Hidden Bias From Teaching Tolerance
- How The Concept Of Implicit Bias Came Into Being With Dr. Mahzarin Banaji, Harvard University. Author of Blindspot: hidden biases of good people5:28 minutes; includes transcript
- Understanding Your Racial Biases With John Dovidio, PhD, Yale University From the American Psychological Association11:09 minutes; includes transcript
- Talking Implicit Bias in Policing With Jack Glaser, Goldman School of Public Policy, University of California Berkeley21:59 minutes
- Implicit Bias: A Factor in Health Communication With Dr. Winston Wong, Kaiser Permanente19:58 minutes
- Bias, Black Lives and Academic Medicine Dr. David Ansell on Your Health Radio (August 1, 2015)21:42 minutes
- Uncovering Hidden Biases Google talk with Dr. Mahzarin Banaji, Harvard University
- Impact of Implicit Bias on the Justice System 9:14 minutes
- Students Speak Up: What Bias Means to Them 2:17 minutes
- Weight Bias in Health Care From Yale University16:56 minutes
- Gender and Racial Bias In Facial Recognition Technology 4:43 minutes
Journal Articles
- An implicit bias primer Mitchell, G. (2018). An implicit bias primer. Virginia Journal of Social Policy & the Law , 25, 27–59.
- Implicit Association Test at age 7: A methodological and conceptual review Nosek, B. A., Greenwald, A. G., & Banaji, M. R. (2007). The Implicit Association Test at age 7: A methodological and conceptual review. Automatic processes in social thinking and behavior, 4 , 265-292.
- Implicit Racial/Ethnic Bias Among Health Care Professionals and Its Influence on Health Care Outcomes: A Systematic Review Hall, W. J., Chapman, M. V., Lee, K. M., Merino, Y. M., Thomas, T. W., Payne, B. K., … & Coyne-Beasley, T. (2015). Implicit racial/ethnic bias among health care professionals and its influence on health care outcomes: a systematic review. American journal of public health, 105 (12), e60-e76.
- Reducing Racial Bias Among Health Care Providers: Lessons from Social-Cognitive Psychology Burgess, D., Van Ryn, M., Dovidio, J., & Saha, S. (2007). Reducing racial bias among health care providers: lessons from social-cognitive psychology. Journal of general internal medicine, 22 (6), 882-887.
- Integrating implicit bias into counselor education Boysen, G. A. (2010). Integrating Implicit Bias Into Counselor Education. Counselor Education & Supervision, 49 (4), 210–227.
- Cognitive Biases and Errors as Cause—and Journalistic Best Practices as Effect Christian, S. (2013). Cognitive Biases and Errors as Cause—and Journalistic Best Practices as Effect. Journal of Mass Media Ethics, 28 (3), 160–174.
- Empathy intervention to reduce implicit bias in pre-service teachers Whitford, D. K., & Emerson, A. M. (2019). Empathy Intervention to Reduce Implicit Bias in Pre-Service Teachers. Psychological Reports, 122 (2), 670–688.

Related Articles
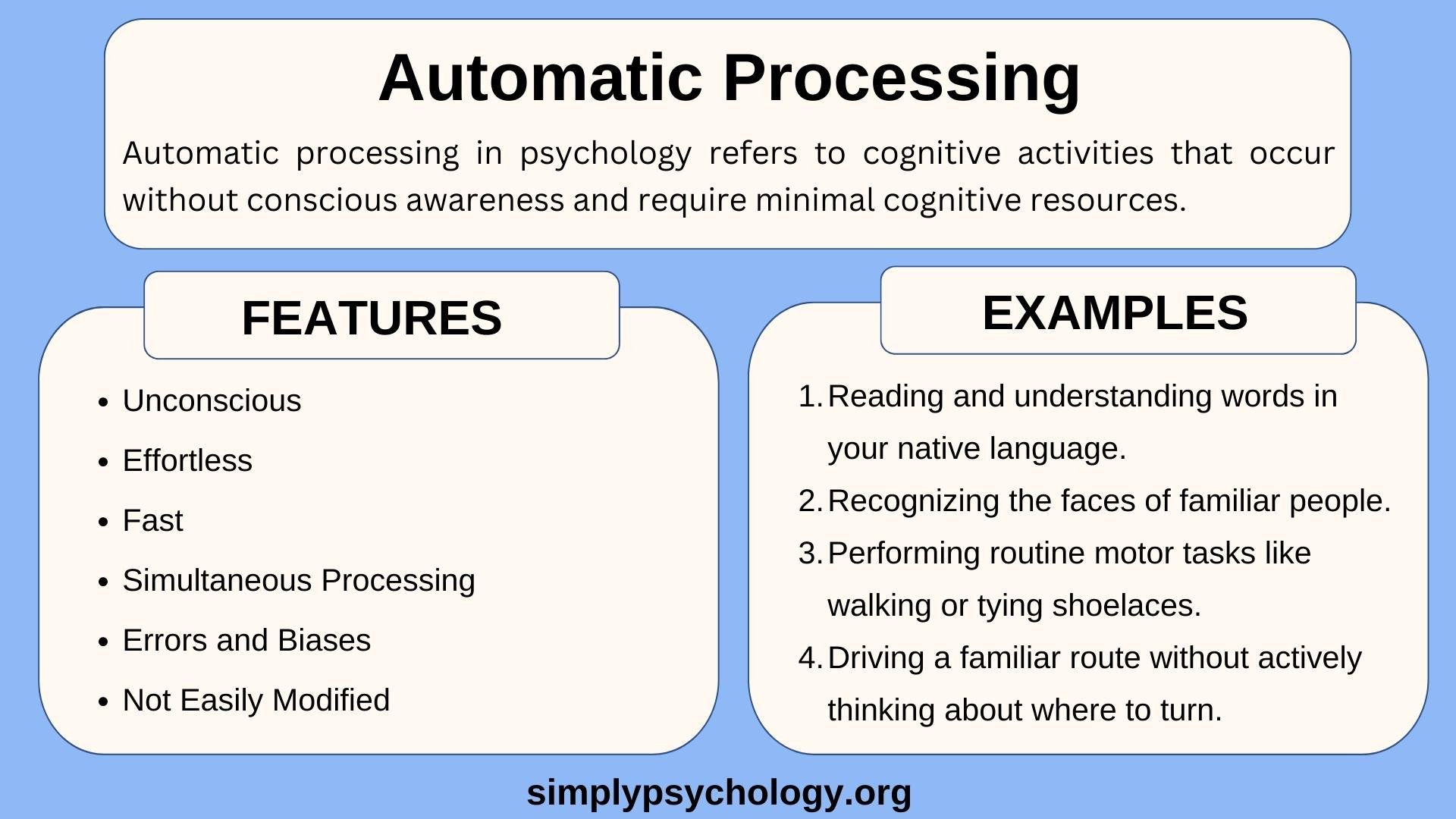
Cognitive Psychology
Automatic Processing in Psychology: Definition & Examples
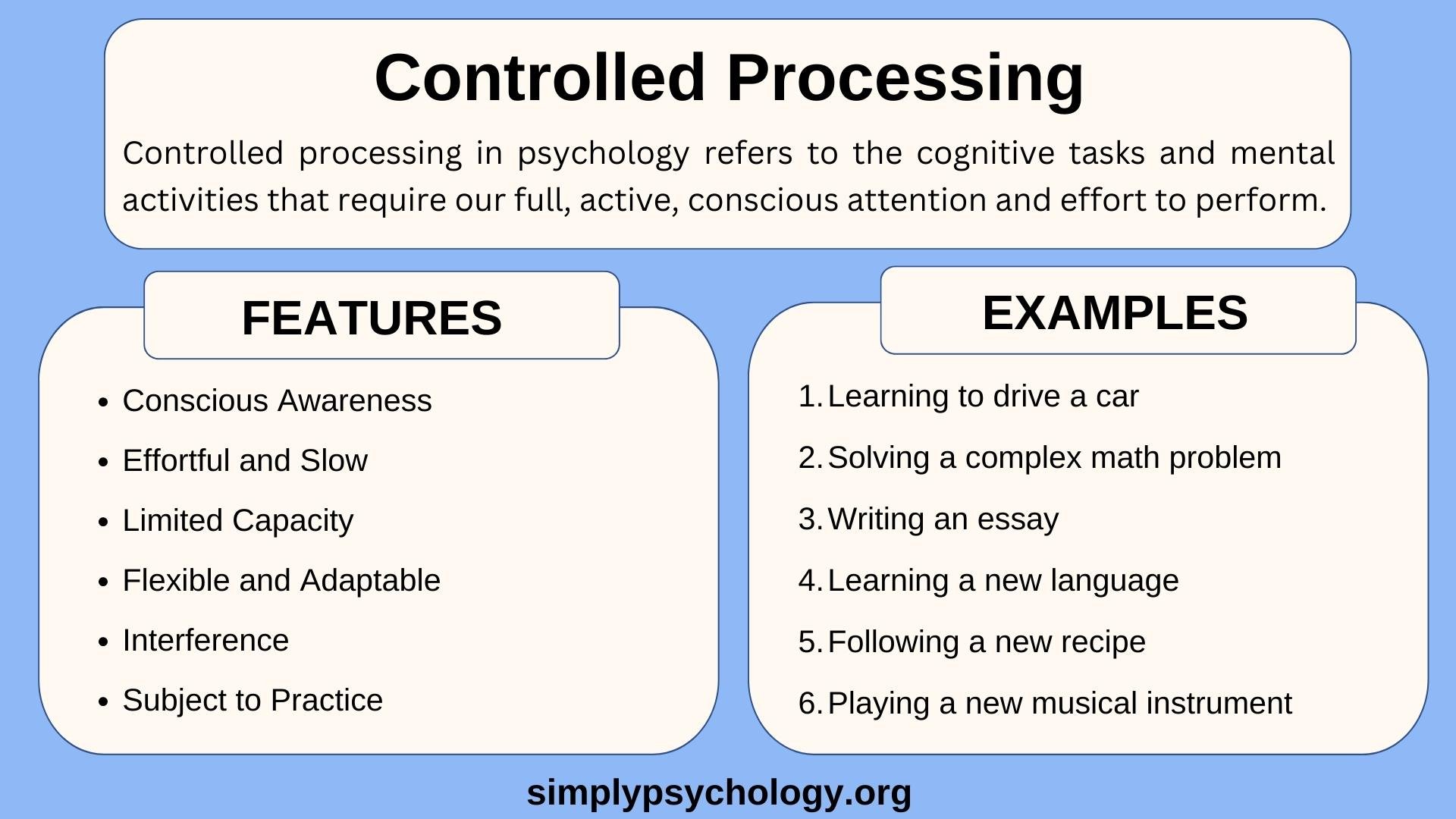
Controlled Processing in Psychology: Definition & Examples
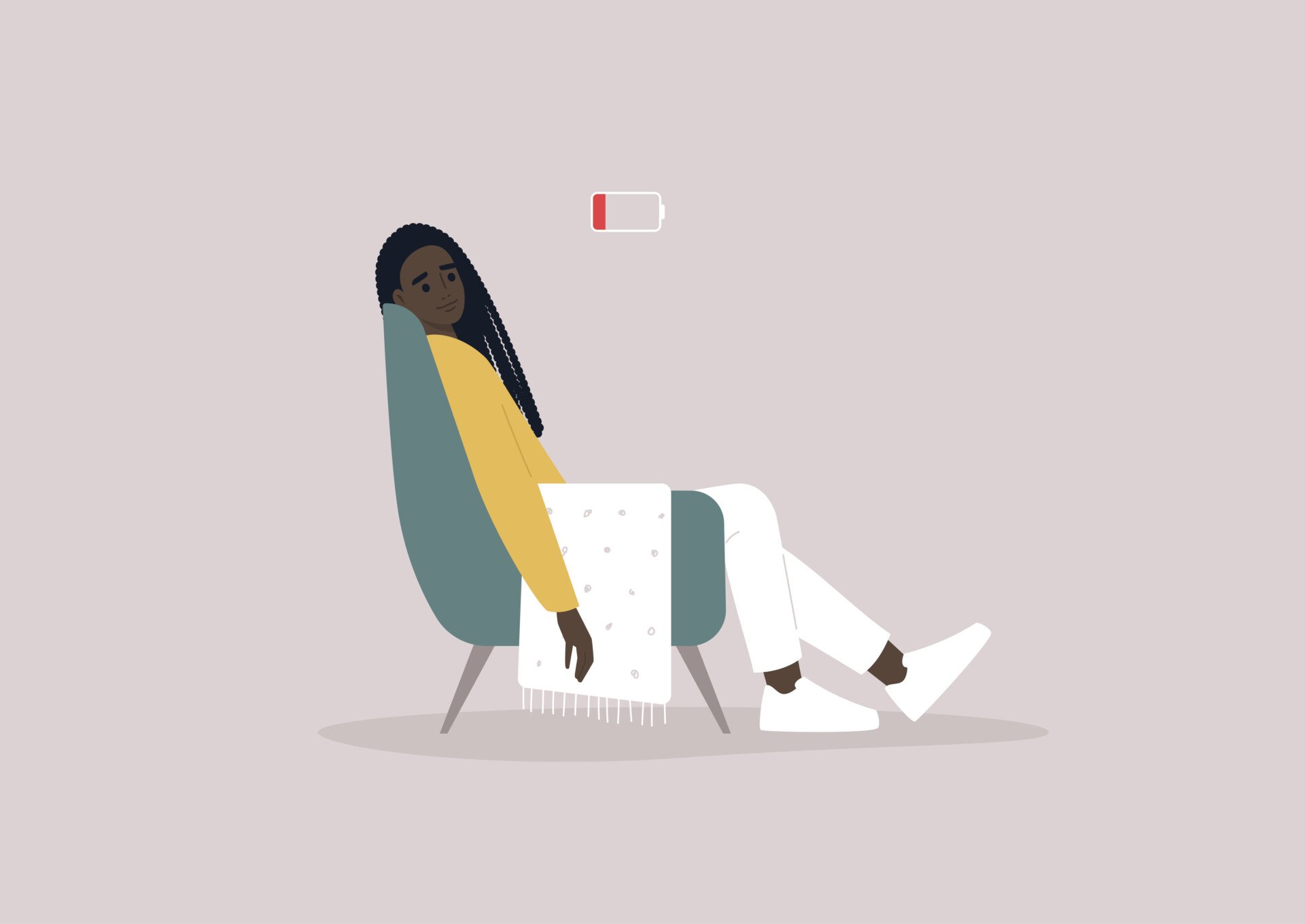
How Ego Depletion Can Drain Your Willpower
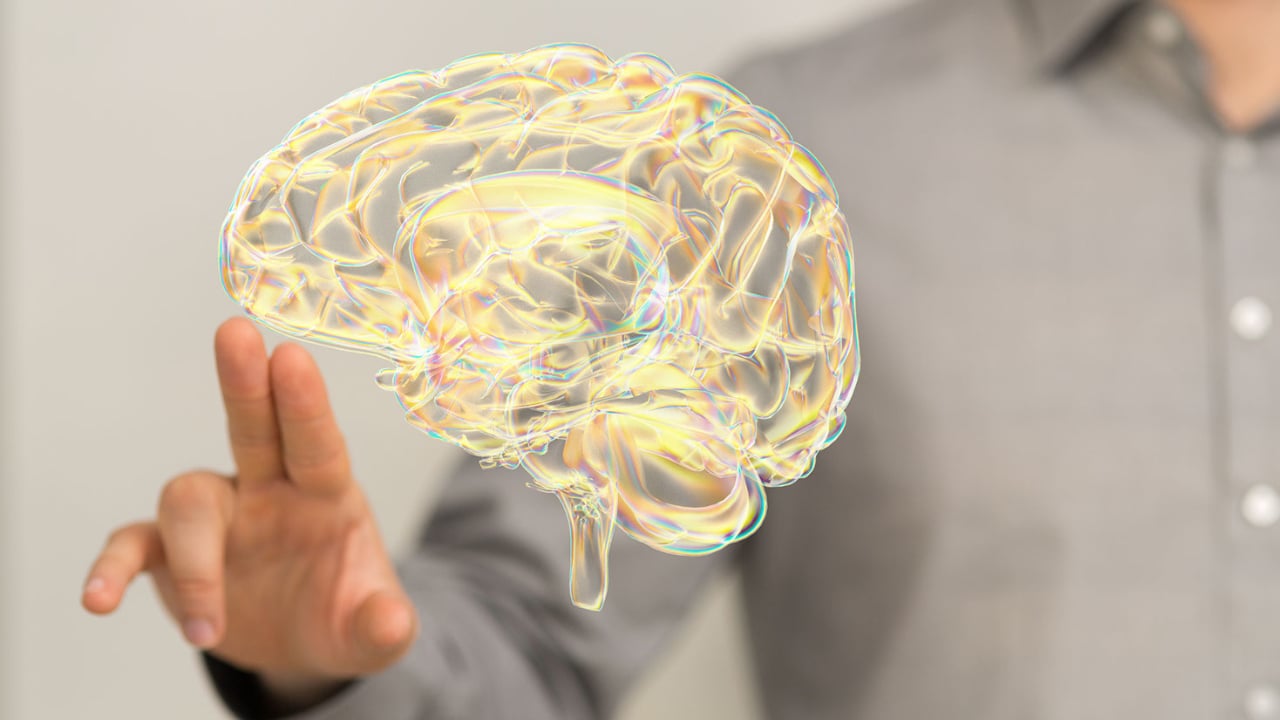
What is the Default Mode Network?
Theories of Selective Attention in Psychology
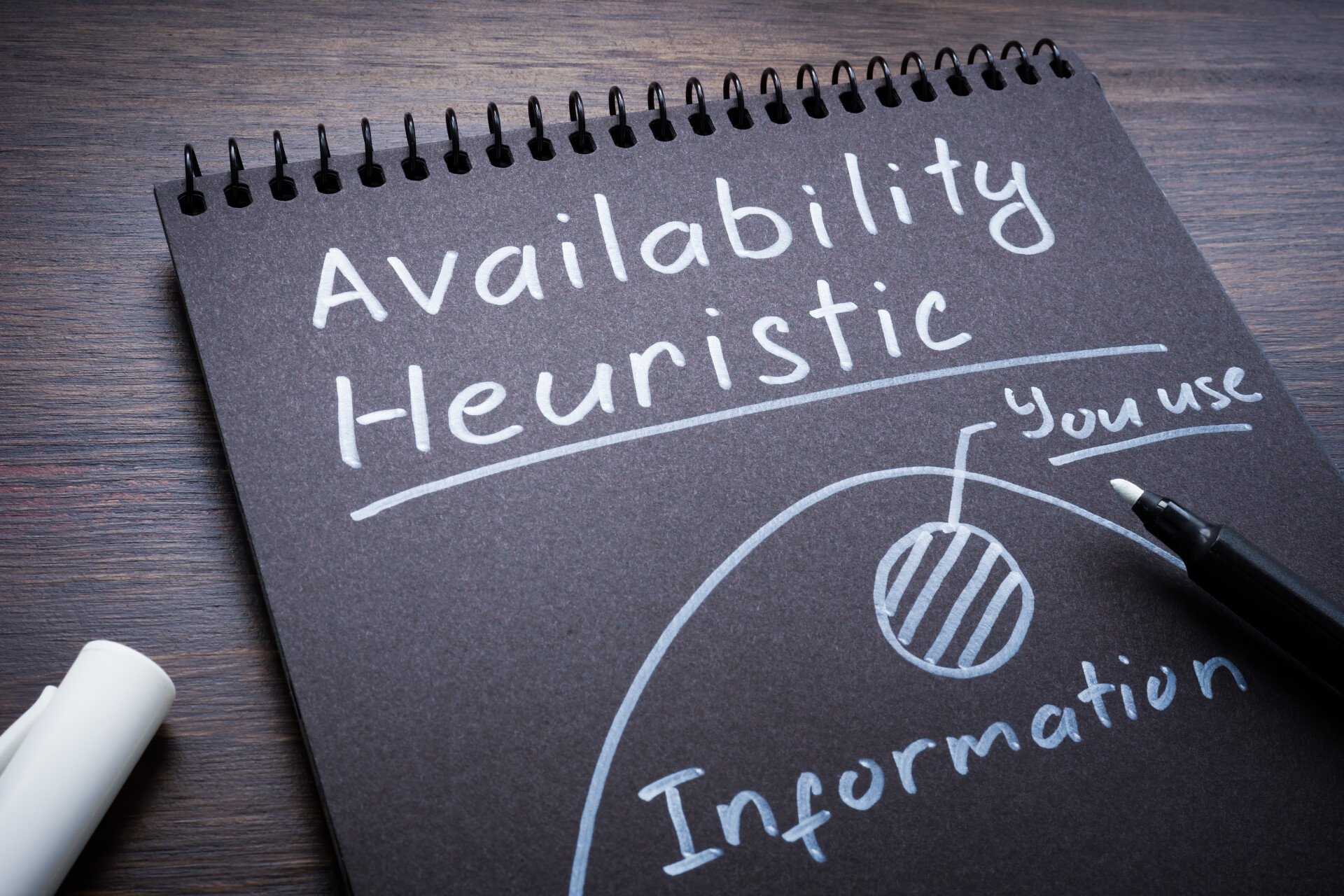
Availability Heuristic and Decision Making
A case study in combating bias
The Quarterly : Tell us a bit about the circumstances that motivated RWE’s management to undertake a broad debiasing operation.
Stay current on your favorite topics
Bernhard Günther: In the second half of the last decade, we spent more than €10 billion on big capital-expenditure programs and acquisitions in conventional power plants. In the business cases underlying these decisions, we were betting on the assumptions of ever-rising commodity prices, ever-rising power prices. We were not alone in our industry in hitting a kind of investment peak at that time. What we and most other peers totally underestimated was the turnaround in public sentiment toward conventional power generation—for example, the green transformation of the German energy system, and the technological progress in renewable generation and related production costs. These factors went in a completely opposite direction compared to our scenarios.
Conventional power generation in continental Europe went through the deepest crisis the industry has ever seen. This ultimately led to the split of the two biggest German players in the industry, E.ON and RWE. Both companies separated their ailing conventional power-generation businesses from the rest of the company.
The Quarterly : Was it difficult to convince members of the executive and supervisory boards to scrutinize your decision-making practices?
Bernhard Günther: Actually, it was the supervisory board asking, “Where has the shareholders’ money gone?” and we in the executive board wanted to learn our lessons from this experience as well. So we embarked on a postmortem analysis to understand what went wrong and why, by looking at a sample of these €10 billion investments. We asked ourselves, “Is there anything we could have done differently, and if so, how can we learn from this in the future?” The spirit of it was not about shaming and blaming, but about learning from our own mistakes.
The Quarterly : What were the main contributing factors that you identified in your investigation?
Bernhard Günther: There were a few outright areas of managerial under-performance such as some time and cost overruns on the €10 billion investments, totally unrelated to external factors. There were also exogenous factors that were not in our base-case assumption but that should have been within our solution space—the most obvious being the political intent to push renewables into the market, which was publicly known at the time our investment decisions were made. There was also at least one unforeseeable factor—the Fukushima disaster. The German government reacted by rushing into a sudden exit from nuclear-power generation. Roughly half of the nuclear plants were switched off immediately, significantly shortening the economic lifetime of the remaining plants. But even if you discount for Fukushima, I think the ultimate end game wouldn’t have looked much different from today’s perspective; it just speeded the whole thing up.
The Quarterly : As you analyzed the decision-making dynamics at work, what biases did you start to see?
Bernhard Günther: What became obvious is that we had fallen victim to a number of cognitive biases in combination. We could see that status quo and confirmation biases had led us to assume the world would always be what it used to be. Beyond that, we neglected to heed the wisdom of portfolio theory that you shouldn’t lay all your eggs in one basket. We not only laid them in the same basket, but also within a very short period of time—the last billion was committed before the construction period of the first billion had been finalized. If we had stretched this whole €10 billion program out over a longer period, say 10 or 15 years, we might still have lost maybe €1 billion or €2 billion but not the amount we incurred later.
We also saw champion and sunflower biases, which are about hierarchical patterns and vertical power distance. Depending on the way you organize decision processes, when the boss speaks up first, the likelihood that anybody who’s not the boss will speak up with a dissenting opinion is much lower than if you, for example, have a conscious rule that the bigwigs in the hierarchy are the ones to speak up last, and you listen to all the other evidence before their opinion is offered.
And we certainly overestimated our own abilities to deliver, due to a good dose of action-oriented biases like overconfidence and excessive optimism. Our industry, like many other capital-intensive ones, has had boom and bust cycles in investments. We embarked on a huge investment program with a whole generation of managers who hadn’t built a single power plant in their professional lives; there were just a few people left who could really remember how big investments were done. So we did something that the industry, by and large, hadn’t been doing on a large scale for 20 years.
The Quarterly : On the sunflower bias, how far down in the organization do you think that went? Were people having a hard time getting past their superiors’ views just on the executive level, or all the way down?
Bernhard Günther: Our investigation revealed that it went much farther down, to almost all levels of our organizational hierarchy. For example, there was a feeling within the rank and file who produced the investment valuations for major decisions that certain scenarios were not desired—that you exposed yourself to the risk of being branded an eternal naysayer, or worse, when you pushed for more pessimistic scenarios. People knew that there were no debiasing mechanisms upstairs, so they would have no champion too if they were to suggest, for example, that if we looked at a “brilliant” new investment opportunity from a different angle, it might not look that brilliant anymore.
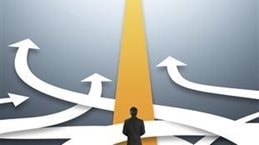
Are you ready to decide?
The Quarterly : So, what kind of countermeasures did you put in place to tackle these cultural issues?
Bernhard Günther: We started a cultural-change program early on, with the arrival of our new CEO, to address our need for a different management mind-set in light of an increasingly uncertain future. A big component of that was mindfulness—becoming aware of not only your own cognitive patterns, but also the likely ones of the people you work with. We also sought to embed this awareness in practical aspects of our process. For example, we’ve now made it mandatory to list the debiasing techniques that were applied as part of any major proposal that is put before us as a board.
It was equally important for us to start to create an atmosphere in which people are comfortable with a certain degree of conflict, where there is an obligation to dissent. This is not something I would say is part of the natural DNA of many institutions, including ours. We’ve found that we have to push it forward and safeguard it, because as soon as hierarchy prevails, it can be easily discouraged.
So, for example, when making big decisions, we now appoint a devil’s advocate—someone who has no personal stake in the decision and is senior enough in the hierarchy to be as independent as possible, usually a level below the executive board. And nobody blames the devil’s advocate for making the negative case because it’s not necessary for them to be personally convinced; it’s about making the strongest case possible. People see that constructive tension brings us further than universal consent.
Rapid reflections from Bernhard Günther
1. in your experience, what piece of common leadership advice is wrong or misleading.
People development based on weaknesses—or gaps versus “ideal candidate” profile—instead of building on strengths
2. Which historical figures do you admire the most?
Nelson Mandela and Martin Luther King Jr.
3. What’s the best book you’ve read in the past year?
Freedom , by Jonathan Franzen (fiction)
You! The Positive Force in Change: Leveraging Insights from Neuroscience and Positive Psychology , by Eileen Rogers and Nick van Dam (nonfiction)
4. What skill do you think is most undervalued in leaders today?
The Quarterly : How did you roll all this out?
Bernhard Günther: There were two areas of focus. First, over a period of two years, we sent the top 300 of our company’s management to a two-week course, which we had self-assembled with external experts. The main thrust of this program was self-awareness: being more open to dissent, more open to a certain amount of controlled risk taking, more agile, as with rapid prototyping, and so forth.
Then we also launched a training program for managers and experts, especially those involved in project work—for example, the financial controllers that have to run the models for big investment decisions. This was a combination of a training course, some desktop training you could do on your own, and some distributed materials.
This program explicitly focused on debiasing. It started with these typical examples where you can show everybody how easily we fall into those cognitive traps, framing it not as a personal defect but as something that’s just there. Secondly, it emphasized that debiasing can be done much more easily within a group, because it’s a collective, conscious effort. And not some kind of empty ritual either. We taught very specific things that people could apply in their daily practices. For example, you can do a kind of premortem analysis and ask your team, “Imagine we are five years into the future, and this whole project we’re deciding on today has turned out to be a complete disaster. What could have happened in the meantime? What could have gone wrong?” This is something that we are now doing regularly on big projects, especially when there are uncertain environmental factors—whether macroeconomic, technological, ecological, or political.
The Quarterly : Could you tell us about an example or two where you made a different decision as the result of debiasing practice, where it went the other way from what you initially thought was the right answer?
Bernhard Günther: Two examples immediately come to my mind. The first one came up in the middle of 2015, when it became obvious that our company was in a strategic deadlock with the power-generation business—the cash cow of the company for years but now with a broken business model. There was a growing awareness among senior management that trying to cure the crisis with yet another round of cost cutting might not be good enough, that we needed to consider more radical strategic options. We established a red team and a blue team to come up with different proposals, one staffed internally and one with externals. We wanted an unbiased view from the outside, from people who were not part of our company or industry; in this case, we brought in external people with backgrounds in investment banking.
The internal team came up with the kind of solution that I think everybody was initially leaning toward, which was more incremental. And the external team came up with a more disruptive solution. But because it was consciously pitched as an independent view, everybody on the board took their time to seriously consider it with an open mind. It planted the seedling of the strategy that we adopted to split the company into two parts, which now, a good year later, has successfully concluded with the IPO of Innogy. If we hadn’t taken this approach, maybe months later or years later, somebody would have come up with a similar idea, but it wouldn’t have happened that fast, with that kind of momentum.
The second example is a recent potential investment project in renewable energy that carried high reputational value for us, so there were emotional issues attached to winning the project. We were bidding for a wind park that was to be built, and the lowest bidder wins by offering the lowest electricity price. We knew it would be a very competitive auction for that project, and we had already decided in the run up to the decision making that we wanted to have a devil’s advocate involved.
We had the project team make the case first in the board meeting. Then we had the devil’s advocate put forward analysis of the risk–return trade-offs. All of this was in written form, so everybody had to read it before the meeting. This certainly helped our discussion a lot and made it much easier to have a nonemotional debate around the critical issues. And we came out of it with a different and I think better decision than we would have if we had just taken the proposal of our internal project team at face value.
The Quarterly : Now that these decision-making changes have taken hold, how do you see things running differently in the organization?
Bernhard Günther: Looking back at where we were three or four years ago, I’d say that this practice of awareness and debiasing has now become almost a part of our corporate decision-making DNA. But it’s something you have to constantly force yourself to practice again and again, because everyone at some point asks, “Do we really need to do it? Can’t we just decide?” It’s a very time-intensive process, which should be utilized only for the most important decisions of strategic relevance. About 30 percent of our board’s decisions fall into this category—for example, major resource-allocation decisions—and it’s similar elsewhere in the company.
Also, people’s general awareness of the complex set of issues around cognitive biases has grown dramatically. Before this, things easily degenerated into blaming exercises going both ways. The naysayers were critiquing the others for wanting to push their pet projects. And the people promoting these projects were saying that the naysayers were just narrow-minded financial controllers who were destroying the company by eternally killing good business ideas. But now there’s more mutual respect for these different roles that are needed to ultimately come up with as good a decision outcome as possible. It’s not just about debiasing; it’s given us a common language. It’s now routine for somebody to say in a meeting, “I think we need some debiasing here.” And then everybody can agree to this without any need to get emotional. When in doubt, we just go through the process.
The Quarterly : Do you have any recommendations for other senior leaders who might be reading this interview?
Bernhard Günther: I think when you read about these issues, it can seem a bit esoteric. You might say, “Well, maybe it’s just their problem, but not mine.” I think everyone should just do it; just start with it even on a pilot basis. You don’t have to start rolling it out across 1,000 people. You can start with your own board, with a few test examples, and see if you think it helps you. But if you do it, you have to do it right; you have to be serious about it. Looking back, there were a few key success factors for us. For one, top management has to set an example. That’s true of any kind of change, not just debiasing. If it’s not modeled at the very top, it’s unlikely to happen further down the hierarchy. Second, everyone has to be open to these ideas or it can be difficult to really make progress. At first glance, many of the tools might seem trivial to some, but we found them to have a very profound effect.
Bernhard Günther joined RWE in 1999 and served as the company’s chief financial officer from 2013 until the 2016 spin-off and IPO of Innogy, where he is now CFO. This interview was conducted by Sven Heiligtag , a partner in McKinsey’s Hamburg office, and Allen Webb, McKinsey Quarterly ’s editor in chief, who is based in the Seattle office.
Explore a career with us
Related articles.

Taking the bias out of meetings

Leadership and behavior: Mastering the mechanics of reason and emotion
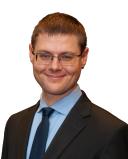
How to Evaluate Unconscious Cognitive Bias
Tackling dangerous judgment errors to improve decision-making..
Posted March 21, 2023 | Reviewed by Abigail Fagan
- Cognitive biases like the planning fallacy, the halo/horns effects, and the overconfidence effect negatively impact workplace performance.
- Emotional motivation and awareness are crucial for addressing cognitive biases effectively.
- Regular assessment and tailored interventions help mitigate cognitive biases and improve decision-making in the workplace.
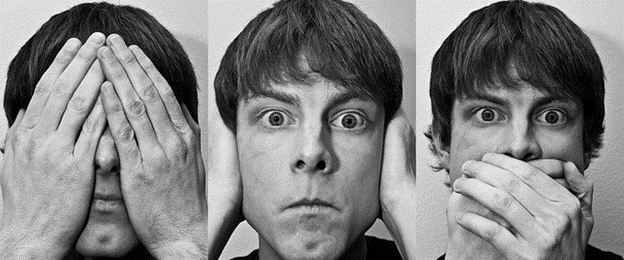
What percentage of projects in your workplace miss the deadline or go over budget?
How often do you see hiring decisions and employee performance and promotion assessments influenced by factors not relevant to job competency?
How frequently are your team members overconfident about their decisions?
If you didn’t answer “rare to none” for any of these, you've got a problem. In fact, these questions get at only three out of over 100 unconscious dangerous judgment errors that scholars in behavioral economics and cognitive neuroscience call cognitive biases.
Do you regularly see projects in your workplace go over deadline or budget, say over 10% of the time? It’s a sign that the cognitive bias known as the planning fallacy is undercutting performance. The planning fallacy refers to our intuitive belief that everything will go according to plan, resulting in us failing to plan for the many potential problems that cause projects to go over budget or deadline. Cost overruns and delays result in serious damage to the bottom lines of our businesses.
Are assessments for hiring, performance, and promotion impacted by non-relevant factors? Well, two dangerous judgment errors play a major role in causing such problematic evaluations, the halo effect and the horns effect . The halo effect refers to the fact that if we feel a significant positive emotion toward one characteristic of someone, then we will have an overly positive evaluation of that person as a whole. That’s why taller men get promoted at higher rates into positions of authority, and both men and women perceived as physically attractive are more likely to be hired. The horns effect is the opposite: If we don’t like a characteristic that is significant to us, we will tend to have a worse evaluation of that person as a whole. For instance, overweight people are less likely to be hired.
Finally, excessive confidence in making decisions — and other work areas — is a symptom of the mental blindspot known as the overconfidence effect. Overconfidence has been associated with many problems in the workplace. For example, overconfidence leads people into financial shenanigans such as overstating earnings. Overconfident leaders tend to resist constructive criticism and dismiss wise advice, letting their intuition drive their decision-making as opposed to making thoughtful plans.
So now that you know about the planning fallacy, the halo and horns effects, and overconfidence effect, you’re safe from these four cognitive biases, right? Unfortunately, just learning about these mental blindspots will not work to assess where they occur in your workplace or to defeat them, as research shows. In fact, some techniques that would seem intuitively to help address unconscious cognitive bias make them worse.
Fortunately, recent research shows how you can use pragmatic strategies to assess and address these dangerous judgment errors so as to make the best decisions, both day-to-day and major ones, and implement them efficiently and effectively. The first step to solving cognitive biases does involve learning about them. However, simply having knowledge doesn’t help. For instance, students who learned about mental blindspots showed the same vulnerability to these errors as students who didn’t.
What is much more helpful is making sure that people are strongly emotionally motivated to address cognitive biases. Our emotions determine 80-90 percent of our decisions, thoughts, and behaviors, and tapping our feelings is clearly effective in helping notice and address dangerous judgment errors. On a related note, it really helps for people to feel that the effort to address mental blindspots is important to them, getting them truly involved and bought into the outcome of debiasing unconscious cognitive bias.
To do so, you need to evaluate thoroughly the impact of each cognitive bias on your own professional activities, as well as more broadly in your team and organization. Then, you have to make and implement a plan to address the problem, again both for yourself, your team, and your business.
Fortunately, you don’t have to do all the cognitive biases. Just going through the 30 most dangerous judgment errors in the workplace will get you the large majority of the benefit from such an analysis. All of these mental blindspots, along with clear next steps on what to do after the evaluation, is in the “Assessment on Dangerous Judgment Errors in the Workplace.”
The assessment starts with an evaluation of how frequently each of the 30 cognitive biases occurred in your workplace in the last year in the form of percentages. Don’t feel obliged to be absolutely precise: Approximate numbers are fine.
If you don’t remember something occurring, give it a low percentage score, including 0 if you think it doesn’t occur. For instance, if all of your projects came under budget and within the deadline, then planning fallacy is not a problem for you.

Each of the 30 questions should take 10-15 seconds. Just put down the first number that seems to make the most sense for you. You can go back later and tweak it if needed. However, for the first run-through, do it fast. Remember if you tend to be an optimistic person in general, temper your optimism, and give a somewhat higher percentage than you intuitively feel is appropriate. The same goes for pessimism : Give a lower percentage if you tend to be pessimistic.
Following this evaluation, you will score the assessment to see the current state of dangerous judgment errors in your workplace. Next, you’ll evaluate the impact of these problems on the bottom line of your personal work, your organizational unit, or the company as a whole, to the extent that you can estimate this question. After all, knowing the bottom line impact will enable you to decide how much to invest into addressing the problem. You’ll then evaluate the performance of your workplace on the four broad competencies of addressing cognitive biases: How the people in your organization do on evaluating themselves, evaluating others, strategic evaluations of risks and rewards, and tactical evaluations in project implementation.
Finally, you’ll get to the next steps. There, each dangerous judgment error is explained, focusing on its business impact. You’ll also get to decide which of the mental blindspots you’ll focus on addressing in the short-term future.
The assessment will prove invaluable as you take the next steps to solve the problems you identified. You should have yourself and others in your organization do the assessment after you introduce the concept of cognitive biases but before you launch any interventions. Then, you can use your assessment results as a baseline to assess the impact of any interventions. To develop your interventions, see my book that’s based on this assessment and provides both techniques and business case studies for how to address cognitive biases, Never Go With Your Gut: How Pioneering Leaders Make the Best Decisions and Avoid Business Disasters.
Here are some techniques on addressing dangerous judgment errors in day-to-day decision-making and in major decisions, as well as on how to implement your choices most efficiently and effectively without falling into mental blindspots:
- While enacting the intervention, have yourself and the others in your workplace take the assessment regularly — once a week if the intervention is intense, once a month if it’s less intense — to evaluate the effectiveness of the intervention. Revise the intervention as needed to account for your results.
- After the intervention is complete and you are satisfied, keep taking the assessment every quarter. Doing so will help keep up vigilance and ensure that you keep protecting yourself from the disastrous consequences of falling into dangerous judgment errors.
Croskerry, P., Singhal, G., & Mamede, S. (2013). Cognitive debiasing 2: impediments to and strategies for change. BMJ quality & safety , 22 (Suppl 2), ii65-ii72.
Tsipursky, G. (2020). Never Go With Your Gut: How Pioneering Leaders Make the Best Decisions and Avoid Business Disasters . Wayne, NJ: Career Press.
What Is the Function of Confirmation Bias? Uwe Peters
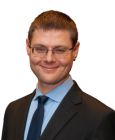
Gleb Tsipursky, Ph.D. , is on the editorial board of the journal Behavior and Social Issues. He is in private practice.
- Find a Therapist
- Find a Treatment Center
- Find a Psychiatrist
- Find a Support Group
- Find Online Therapy
- United States
- Brooklyn, NY
- Chicago, IL
- Houston, TX
- Los Angeles, CA
- New York, NY
- Portland, OR
- San Diego, CA
- San Francisco, CA
- Seattle, WA
- Washington, DC
- Asperger's
- Bipolar Disorder
- Chronic Pain
- Eating Disorders
- Passive Aggression
- Personality
- Goal Setting
- Positive Psychology
- Stopping Smoking
- Low Sexual Desire
- Relationships
- Child Development
- Therapy Center NEW
- Diagnosis Dictionary
- Types of Therapy
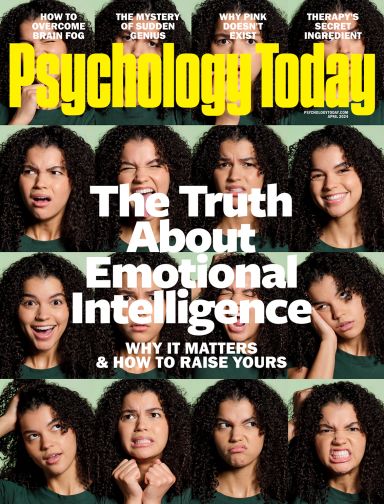
Understanding what emotional intelligence looks like and the steps needed to improve it could light a path to a more emotionally adept world.
- Emotional Intelligence
- Gaslighting
- Affective Forecasting
- Neuroscience
- Bipolar Disorder
- Therapy Center
- When To See a Therapist
- Types of Therapy
- Best Online Therapy
- Best Couples Therapy
- Best Family Therapy
- Managing Stress
- Sleep and Dreaming
- Understanding Emotions
- Self-Improvement
- Healthy Relationships
- Student Resources
- Personality Types
- Guided Meditations
- Verywell Mind Insights
- 2024 Verywell Mind 25
- Mental Health in the Classroom
- Editorial Process
- Meet Our Review Board
- Crisis Support
13 Types of Common Cognitive Biases That Might Be Impairing Your Judgment
Which of these sway your thinking the most?
Kendra Cherry, MS, is a psychosocial rehabilitation specialist, psychology educator, and author of the "Everything Psychology Book."
:max_bytes(150000):strip_icc():format(webp)/IMG_9791-89504ab694d54b66bbd72cb84ffb860e.jpg)
Amy Morin, LCSW, is a psychotherapist and international bestselling author. Her books, including "13 Things Mentally Strong People Don't Do," have been translated into more than 40 languages. Her TEDx talk, "The Secret of Becoming Mentally Strong," is one of the most viewed talks of all time.
:max_bytes(150000):strip_icc():format(webp)/VW-MIND-Amy-2b338105f1ee493f94d7e333e410fa76.jpg)
The Confirmation Bias
The hindsight bias, the anchoring bias, the misinformation effect, the actor-observer bias, the false consensus effect, the halo effect, the self-serving bias, the availability heuristic, the optimism bias.
- Other Kinds
Although we like to believe that we're rational and logical, the fact is that we are continually under the influence of cognitive biases . These biases distort thinking , influence beliefs, and sway the decisions and judgments that people make each and every day.
Sometimes, cognitive biases are fairly obvious. You might even find that you recognize these tendencies in yourself or others. In other cases, these biases are so subtle that they are almost impossible to notice.
At a Glance
Attention is a limited resource. This means we can't possibly evaluate every possible detail and event when forming thoughts and opinions. Because of this, we often rely on mental shortcuts that speed up our ability to make judgments, but this can sometimes lead to bias. There are many types of biases—including the confirmation bias, the hindsight bias, and the anchoring bias, just to name a few—that can influence our beliefs and actions daily.
The following are just a few types of cognitive biases that have a powerful influence on how you think, how you feel, and how you behave.
Tara Moore / Getty Images
The confirmation bias is the tendency to listen more often to information that confirms our existing beliefs. Through this bias, people tend to favor information that reinforces the things they already think or believe.
Examples include:
- Only paying attention to information that confirms your beliefs about issues such as gun control and global warming
- Only following people on social media who share your viewpoints
- Choosing news sources that present stories that support your views
- Refusing to listen to the opposing side
- Not considering all of the facts in a logical and rational manner
There are a few reasons why this happens. One is that only seeking to confirm existing opinions helps limit mental resources we need to use to make decisions. It also helps protect self-esteem by making people feel that their beliefs are accurate.
People on two sides of an issue can listen to the same story and walk away with different interpretations that they feel validates their existing point of view. This is often indicative that the confirmation bias is working to "bias" their opinions.
The problem with this is that it can lead to poor choices, an inability to listen to opposing views, or even contribute to othering people who hold different opinions.
Things that we can do to help reduce the impact of confirmation bias include being open to hearing others' opinions and specifically looking for/researching opposing views, reading full articles (and not just headlines), questioning the source, and [doing] the research yourself to see if it is a reliable source.
The hindsight bias is a common cognitive bias that involves the tendency to see events, even random ones, as more predictable than they are. It's also commonly referred to as the "I knew it all along" phenomenon.
Some examples of the hindsight bias include:
- Insisting that you knew who was going to win a football game once the event is over
- Believing that you knew all along that one political candidate was going to win an election
- Saying that you knew you weren't going to win after losing a coin flip with a friend
- Looking back on an exam and thinking that you knew the answers to the questions you missed
- Believing you could have predicted which stocks would become profitable
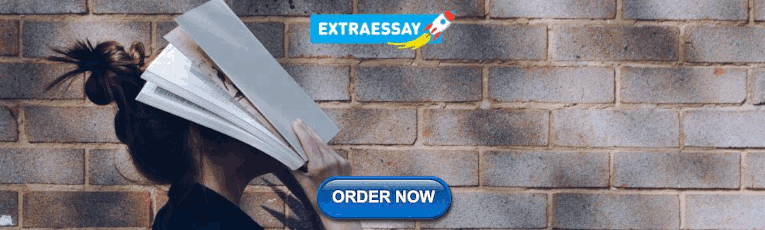
Classic Research
In one classic psychology experiment, college students were asked to predict whether they thought then-nominee Clarence Thomas would be confirmed to the U.S. Supreme Court.
Prior to the Senate vote, 58% of the students thought Thomas would be confirmed. The students were polled again following Thomas's confirmation, and a whopping 78% of students said they had believed Thomas would be confirmed.
The hindsight bias occurs for a combination of reasons, including our ability to "misremember" previous predictions, our tendency to view events as inevitable, and our tendency to believe we could have foreseen certain events.
The effect of this bias is that it causes us to overestimate our ability to predict events. This can sometimes lead people to take unwise risks.
The anchoring bias is the tendency to be overly influenced by the first piece of information that we hear. Some examples of how this works:
- The first number voiced during a price negotiation typically becomes the anchoring point from which all further negotiations are based.
- Hearing a random number can influence estimates on completely unrelated topics.
- Doctors can become susceptible to the anchoring bias when diagnosing patients. The physician’s first impressions of the patient often create an anchoring point that can sometimes incorrectly influence all subsequent diagnostic assessments.
While the existence of the anchoring bias is well documented, its causes are still not fully understood. Some research suggests that the source of the anchor information may play a role. Other factors such as priming and mood also appear to have an influence.
Like other cognitive biases, anchoring can have an effect on the decisions you make each day. For instance, it can influence how much you are willing to pay for your home. However, it can sometimes lead to poor choices and make it more difficult for people to consider other factors that might also be important.
The misinformation effect is the tendency for memories to be heavily influenced by things that happened after the actual event itself. A person who witnesses a car accident or crime might believe that their recollection is crystal clear, but researchers have found that memory is surprisingly susceptible to even very subtle influences.
For example:
- Research has shown that simply asking questions about an event can change someone's memories of what happened.
- Watching television coverage may change how people remember the event.
- Hearing other people talk about a memory from their perspective may change your memory of what transpired.
Classic Memory Research
In one classic experiment by memory expert Elizabeth Loftus , people who watched a video of a car crash were then asked one of two slightly different questions: “How fast were the cars going when they hit each other?” or “How fast were the cars going when they smashed into each other?”
When the witnesses were then questioned a week later whether they had seen any broken glass, those who had been asked the “smashed into” version of the question were more likely to report incorrectly that they had seen broken glass.
There are a few factors that may play a role in this phenomenon. New information may get blended with older memories. In other cases, new information may be used to fill in "gaps" in memory.
The effects of misinformation can range from the trivial to much more serious. It might cause you to misremember something you thought happened at work, or it might lead to someone incorrectly identifying the wrong suspect in a criminal case.
The actor-observer bias is the tendency to attribute our actions to external influences and other people's actions to internal ones. The way we perceive others and how we attribute their actions hinges on a variety of variables, but it can be heavily influenced by whether we are the actor or the observer in a situation.
When it comes to our own actions, we are often far too likely to attribute things to external influences. For example:
- You might complain that you botched an important meeting because you had jet lag.
- You might say you failed an exam because the teacher posed too many trick questions.
When it comes to explaining other people’s actions, however, we are far more likely to attribute their behaviors to internal causes. For example:
- A colleague screwed up an important presentation because he’s lazy and incompetent (not because he also had jet lag).
- A fellow student bombed a test because they lack diligence and intelligence (and not because they took the same test as you with all those trick questions).
While there are many factors that may play a role, perspective plays a key role. When we are the actors in a situation, we are able to observe our own thoughts and behaviors. When it comes to other people, however, we cannot see what they are thinking. This means we focus on situational forces for ourselves, but guess at the internal characteristics that cause other people's actions.
The problem with this is that it often leads to misunderstandings. Each side of a situation is essentially blaming the other side rather than thinking about all of the variables that might be playing a role.
The false consensus effect is the tendency people have to overestimate how much other people agree with their own beliefs, behaviors, attitudes, and values. For example:
- Thinking that other people share your opinion on controversial topics
- Overestimating the number of people who are similar to you
- Believing that the majority of people share your preferences
Researchers believe that the false consensus effect happens for a variety of reasons. First, the people we spend the most time with, our family and friends, do often tend to share very similar opinions and beliefs. Because of this, we start to think that this way of thinking is the majority opinion even when we are with people who are not among our group of family and friends.
Another key reason this cognitive bias trips us up so easily is that believing that other people are just like us is good for our self-esteem . It allows us to feel "normal" and maintain a positive view of ourselves in relation to other people.
This can lead people not only to incorrectly think that everyone else agrees with them—it can sometimes lead them to overvalue their own opinions. It also means that we sometimes don't consider how other people might feel when making choices.
The halo effect is the tendency for an initial impression of a person to influence what we think of them overall. Also known as the "physical attractiveness stereotype" or the "what is beautiful is 'good' principle" we are either influenced by or use the halo to influence others almost every day. For example:
- Thinking people who are good-looking are also smarter, kinder, and funnier than less attractive people
- Believing that products marketed by attractive people are also more valuable
- Thinking that a political candidate who is confident must also be intelligent and competent
One factor that may influence the halo effect is our tendency to want to be correct. If our initial impression of someone was positive, we want to look for proof that our assessment was accurate. It also helps people avoid experiencing cognitive dissonance , which involves holding contradictory beliefs.
This cognitive bias can have a powerful impact in the real world. For example, job applicants perceived as attractive and likable are also more likely to be viewed as competent, smart, and qualified for the job.
The self-serving bias is a tendency for people tend to give themselves credit for successes but lay the blame for failures on outside causes. When you do well on a project, you probably assume that it’s because you worked hard. But when things turn out badly, you are more likely to blame it on circumstances or bad luck.
Some examples of this:
- Attributing good grades to being smart or studying hard
- Believing your athletic performance is due to practice and hard work
- Thinking you got the job because of your merits
The self-serving bias can be influenced by a variety of factors. Age and sex have been shown to play a part. Older people are more likely to take credit for their successes, while men are more likely to pin their failures on outside forces.
This bias does serve an important role in protecting self-esteem. However, it can often also lead to faulty attributions such as blaming others for our own shortcomings.
The availability heuristic is the tendency to estimate the probability of something happening based on how many examples readily come to mind. Some examples of this:
- After seeing several news reports of car thefts in your neighborhood, you might start to believe that such crimes are more common than they are.
- You might believe that plane crashes are more common than they really are because you can easily think of several examples.
It is essentially a mental shortcut designed to save us time when we are trying to determine risk. The problem with relying on this way of thinking is that it often leads to poor estimates and bad decisions.
Smokers who have never known someone to die of a smoking-related illness, for example, might underestimate the health risks of smoking. In contrast, if you have two sisters and five neighbors who have had breast cancer, you might believe it is even more common than statistics suggest.
The optimism bias is a tendency to overestimate the likelihood that good things will happen to us while underestimating the probability that negative events will impact our lives. Essentially, we tend to be too optimistic for our own good.
For example, we may assume that negative events won't affect us such as:
The optimism bias has roots in the availability heuristic. Because you can probably think of examples of bad things happening to other people it seems more likely that others will be affected by negative events.
This bias can lead people to take health risks like smoking, eating poorly, or not wearing a seat belt. The bad news is that research has found that this optimism bias is incredibly difficult to reduce.
There is good news, however. This tendency toward optimism helps create a sense of anticipation for the future, giving people the hope and motivation they need to pursue their goals.
Other Kinds of Cognitive Bias
Many other cognitive biases can distort how we perceive the world. Just a partial list:
- Status quo bias reflects a desire to keep things as they are.
- Apophenia is the tendency to perceive patterns in random occurrences.
- Framing is presenting a situation in a way that gives a certain impression.
Keep in Mind
The cognitive biases above are common, but this is only a sampling of the many biases that can affect your thinking. These biases collectively influence much of our thoughts and ultimately, decision making.
Many of these biases are inevitable. We simply don't have the time to evaluate every thought in every decision for the presence of any bias. Understanding these biases is very helpful in learning how they can lead us to poor decisions in life.
Dietrich D, Olson M. A demonstration of hindsight bias using the Thomas confirmation vote . Psychol Rep . 1993;72(2):377-378. doi:/10.2466/pr0.1993.72.2.377
Lee KK. An indirect debiasing method: Priming a target attribute reduces judgmental biases in likelihood estimations . PLoS ONE . 2019;14(3):e0212609. doi:10.1371/journal.pone.0212609
Saposnik G, Redelmeier D, Ruff CC, Tobler PN. Cognitive biases associated with medical decisions: A systematic review . BMC Med Inform Decis Mak . 2016;16(1):138. doi:10.1186/s12911-016-0377-1
Furnham A., Boo HC. A literature review of anchoring bias . The Journal of Socio-Economics. 2011;40(1):35-42. doi:10.1016/j.socec.2010.10.008
Loftus EF. Leading questions and the eyewitness report . Cognitive Psychology . 1975;7(4):560-572. doi:10.1016/0010-0285(75)90023-7
Challies DM, Hunt M, Garry M, Harper DN. Whatever gave you that idea? False memories following equivalence training: a behavioral account of the misinformation effect . J Exp Anal Behav . 2011;96(3):343-362. doi:10.1901/jeab.2011.96-343
Miyamoto R, Kikuchi Y. Gender differences of brain activity in the conflicts based on implicit self-esteem . PLoS ONE . 2012;7(5):e37901. doi:10.1371/journal.pone.0037901
Weinstein ND, Klein WM. Resistance of personal risk perceptions to debiasing interventions . Health Psychol . 1995;14(2):132–140. doi:10.1037//0278-6133.14.2.132
Gratton G, Cooper P, Fabiani M, Carter CS, Karayanidis F. Dynamics of cognitive control: theoretical bases, paradigms, and a view for the future . Psychophysiology . 2018;55(3). doi:10.1111/psyp.13016
By Kendra Cherry, MSEd Kendra Cherry, MS, is a psychosocial rehabilitation specialist, psychology educator, and author of the "Everything Psychology Book."
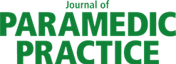
Search menu
Cognitive biases and their effects on practice: a case study reflection.
Philip Patenotte
Paramedic, South Western Ambulance Service, Poole
View articles · Email Philip
Programme Lead, Paramedic Science, Bournemouth University; Dorset
View articles · Email Iain
Clinical decision-making is a key aspect of the student paramedic journey. In particular, it is vital that students can understand the theories that underpin this vital facet of their practice. This article considers a case encountered in practice and the evidence that informs the decision process which was undertaken. The influence of biases, systems of thinking and an appreciation of probability are all considered in this call to a 55-year-old patient with chest pain. In addition, an appreciation of robust history taking is considered as part of this process. As ambulance clinicians can be limited in the tests and scoring systems appropriate to them, consideration is given to the value of traditional ‘in-hospital’ scoring systems and the advantages and limitations they present to the paramedic. These limitations can ultimately result in hospital attendance in many acute presentations such as chest pain incidents, and it may be that our practice is overdue a review of what can be done to address such limitations.
This clinical case study involved a 55-year-old woman who called 999 for severe central chest pain that radiated to her left arm and neck. The patient had an extensive medical history including a previous myocardial infarction (MI), bipolar disorder, anxiety and depression, as well as a complex social history.
Initially, I was unaware that the patient was a frequent caller. However, while en route to the patient, I was informed that there had been 15 ambulance attendances this month, most commonly with a presenting complaint of severe central chest pain.
Obtaining this information initiated an anchoring bias that influenced my clinical decision-making ( Collen, 2017 ). Anchoring bias commonly occurs because a clinician relies heavily on initial information provided, then fails to consider additional information ( Rutherford, 2020 ). This could occur if alternative factors, including vital signs and electrocardiogram (ECG) interpretation, as well as providing holistic patient centred care are dismissed. This can potentially lead to a misdiagnosis from interpreting the presentation as the patient's usual one. This was evident within this case study as the initial focus was on the fact that the patient frequently presented in this way, rather than on considering alternative differentials to determine a diagnosis.
A systematic review by Saposniik et al (2016) found that clinical decision-making is influenced by at least one cognitive bias. Anchoring effect and overconfidence were associated with 36.5%–77% of diagnostic inaccuracies in clinical cases. Similarly, Featherston et al (2020) identified that anchoring bias and stereotyping occurred in 77% of studies. The similarities among the research highlight the prevalence of bias within healthcare.
The Patient Assessment Triangle (PAT) ( Figure 1 ) is a rapid assessment tool based on visual and auditory clues ( Halliwell et al, 2013 ). This enables paramedics to articulate a global impression of the patient while assessing the urgency of intervention required and rule out any time-critical conditions ( Horeczko et al, 2011 ).

My initial visual assessment using the PAT of the patient permitted me to determine that the patient was ‘little sick’ and did not have a time-critical condition, which allowed me to conduct an in-depth assessment and obtain a thorough history (Jenkins, 2013).
The PAT is an example of system 1 thinking, which is informed by experience and generates rapid decisions through subconscious thoughts and pattern recognition ( Jenson et al, 2016 ). This triggers automated thinking more quickly than system 2 thinking, which is hypothetico-deductive ( Cabrera et al, 2015 ).
System 1 and system 2 thinking
Tay et al (2016) suggest that system 1 thinking is an essential element of clinical decision-making. However, decisions are generated without a great depth of conscious effort, and the model is relatively experimental and is often known as a gut feeling ( Tay et al, 2016 ).
Within the prehospital environment, type 1 thinking has a reported sensitivity of 74%–87% ( O'Sullivan and Schofield, 2018 ) for identifying whether a patient is sick. However, it is inadequate for predicting a final diagnosis as it can be associated with an increase in errors because decisions are made quickly, ultimately leaving the reasoning process highly susceptible to cognitive bias ( Croskerry et al, 2013 ).
However, bias does not only occur during type 1 thinking, and can be overcome by using a type 2 thinking process ( O'Sullivan and Schofield, 2018 ).
Realising I was unable to formulate a diagnosis through logical reasoning using system 1 prompted the use of system 2 thinking. System 2 thinking is analytical and is the second element of the dual system that formulates the decision-making process ( Julmi, 2019 ). A slower and more methodical approach that is less prone to error, it is used to seek new information to support decisions ( Tay et al, 2016 ). This thought process enabled me to conduct a further assessment and obtain an accurate history to formulate my diagnosis and management plan.
Student paramedics are more likely to use system 2 thinking because of inexperience ( Ryan and Halliwell, 2012 ), while established paramedics use system 1 thinking, most likely because of their greater time in practice ( Rutherford, 2020 ). However, this hypothesis is questionable as system 1 thinking is suggested to make up 98% of our overall thought process ( Kahneman et al, 2011 ). More research is needed to substantiate or disprove Ryan and Halliwell's (2012) claims within the prehospital environment as the literature is currently insubstantial ( Perona et al, 2019 ).
History-taking
The patient's history influences 80% of the diagnostic process, and taking a thorough history is essential for reaching an accurate diagnosis ( Keifeneim et al, 2015 ). Clinical history-taking provides information that may be useful during a secondary assessment ( Jayaprakash et al, 2016 ).
Although considered a challenging skill for paramedics to master, it is vital to enable adequate delivery of holistic care and treatment for patients ( Oyedokun et al, 2016 ).
Building rapport with patients is essential, and they are more likely to adhere to treatment suggestions and are more satisfied with care if rapport is built early ( Ross, 2013 ).
However, in this case, both building rapport and history-taking were difficult, as the patient disregarded clinical history questioning and, instead, talked irrationally about her historical social issues, which negatively affected my history-taking and the care I was able to deliver.
Instinct prompted me to conduct a full set of vital signs to assist my decision-making. The patient presented with tachycardia and hypertension ( Table 1 ), both of which are associated with a physiological stress response ( Dayoub and Jena, 2015 ). Initially, I was deliberately looking for signs that this was the patient's normal presentation of anxiety. However, I began to consider a differential diagnosis after obtaining a history and vital signs; this led me to believe that the patient could have acute coronary syndrome (ACS).
Confirmation bias
Because of my preconceived notion that the patient was anxious, I began looking for signs and symptoms that might have supported this rather than concentrating on the new onset of chest pain. Focusing on the fact the patient frequently presented with chest pain caused me to adopt a confirmation bias.
A confirmation bias is where a clinician favours information to support an earlier decision and dismisses confounding factors that do not support it ( Rutherford, 2020 ). Clinicians tend to overvalue the importance of clinically irrelevant information if it confirms their perception of the diagnosis ( Ross, 2013 ). This can lead to misdiagnosis and cause serious harm to patients as it begins a cascade of mistreatment that conflicts with the holistic and ethical care paramedics should provide ( Bhatti, 2018 ).
A systematic review by Sapsonik (2016) identified that 71% of 114 studies found an association between cognitive bias and mismanagement. However, 60% of the studies included were deemed low quality under the Newcastle-Ottawa scale. Nonetheless, the criteria in this scale have a satisfactory inter-rater reliability indicating a moderate level of homogeneity among authors ( Sapsonik, 2016 ). In addition, considering the number of studies included, 85% of them looked at only two biases. This indicates that the accurate number of cognitive biases influence healthcare decisions is unaccounted for ( Sapsonik, 2016 ). Further studies are required to understand the most common cognitive biases and their prevalence among the paramedic profession.
I can apply the principles of the study to my own practice by increasing my awareness of the influence of cognitive biases. Evidence shows that cognitive bias influences a paramedic's reasoning process when they need to make decisions, and this can lead to treatment errors and misdiagnosis ( Allen, 2019 ).
Self-awareness and adjustment to the potential impacts that confirmation bias can have on clinical decision-making can ultimately improve patient outcomes (Bhatti, 2019). Teaching on the influence of confirmation bias appears sparse in the College of Paramedics’ ( CoP, 2019 ) curriculum guidance (Allen, 2010). Additional teaching would improve the understanding of human factors to mitigate the risk of confirmation bias negatively impacting practice.
Likelihood and probability
Clinical tests aid decision-making by identifying the presence or absence of attributes that inform patient care and treatment ( Buehler et al, 2019 ). My automatic response was to conduct an ECG; this was because of my system 1 thinking combined with experience, knowledge and pattern recognition of patients presenting with chest pain. The patient's ECG showed normal sinus rhythm with no ischaemic changes. The European Society of Cardiology ( ESC, 2020 ) supports my decision to promptly conduct an ECG; this is recommended within 10 minutes of first contact with a patient with chest pain.
Likelihood ratios (LRs) are often applied to the results of diagnostic tests; specificity and sensitivity are used to produce the probability that informs how likely the patient is to have a disease ( Trevethan, 2017 ). LRs are more informative than sensitivity and specificity, which are often based on disease status only ( Whiting et al, 2015 ). LRs require the use of Bayes' theorem, which assists in determining pre-test odds of a disease based on test results providing post-test odds of a disease ( Karahan Şen et al, 2016 ).
Pre-test probability is the likelihood of having a disease before diagnostic testing is conducted; post-test probability is the probability of having a disease after the test has been carried out.
Mokhtari et al (2015) identified that non-ischaemic ECG changes have a sensitivity of 59% and a specificity of 5%, with a positive LR of 0.6 and a negative LR of 7.6. Using the figures presented by Mokhtari et al (2015) , I was able to conduct post-test probability calculations and create a nomograph ( Figure 2 ). The LR based on a non-ischaemic ECG gave a small probability of ACS, while using a clinical history increased the LR to 4.3. A benefit of the study is that the journal has been peer reviewed, which increases accuracy and validity ( Smithson et al, 2018 ). A limitation of the study is that it gives no definition for the typical presentation of ACS, leading to personal interpretation.

LRs cannot solely be used to provide a provisional diagnosis because of the possibility of false positives or negatives ( Llewelyn, 2012 ). The accuracy of the LR is dependent upon the quality of the research that informs the sensitivity and specificity used to produce LRs.
Tools and scoring systems
While the patient had an ECG indicting normal sinus rhythm, the absence of ECG abnormalities does not conclusively rule out ACS, so patient history plays an important role in diagnosis ( Ijkema et al, 2014 ).
As well as conducting an ECG, additional tools can be used to aid decision-making such as the HEART score, which is used to identify major adverse cardiac events (MACEs), assists in risk reduction and identifies undifferentiated chest pain ( Brady and de Souza, 2018 ). The tool has five elements: history; ECG; age; risk factors; and troponin measurement ( Table 2 ).
In addition, the HEART score has an increased sensitivity and negative predictive value than GRACE at 99.6 compared to 95.8 ( Brady and de Souza, 2018 ; Reaney, 2018 ). This shows the HEART score is more accurate regarding negative test results.
The HEART score was originally developed for use within EDs and has not been evaluated for prehospital use (Otsuka and Takeda, 2020). However, a modified HEART score could prove beneficial within paramedic practice. One suggestion could be a HEART score excluding the criterion of troponin, as prehospital troponin testing is outside a paramedic's scope of practice ( Health and Care Professions Council (HCPC), 2016 ; Stopyra et al, 2018 ), although research is required regarding reliability. There are no standard criteria for the history associated with the HEART score, which can lead to a bias resulting from individual understanding of the definition of history-taking ( Byrne et al, 2018 ). This shows additional assessment within a hospital environment is needed to provide a definitive diagnosis for a patient's cause of chest pain.
Diagnosis and next steps
After the HEART score was calculated, the patient was classified as being at moderate risk with a score of 5 and a MACE rate of up to 17% ( Brady and de Souza, 2018 ). Because of the combination of a poor history, associated risk factors and my initial anchoring bias, I was initially unaware of the patient's risk. In hindsight, awareness of the HEART score during my assessment and carrying out a structured clinical history taking may have rectified my anchoring bias and ensured more timely decision-making.
The patient had multiple risk factors identified by the HEART score, including a long-term history of smoking, hypertension and an increased BMI. These factors are considered to influence a person's health significantly ( Nash, 2014 ). Although these are referred to as lifestyle choices, this view has been criticised with suggestions that they are not necessarily choices but a consequence of social, physical and psychological effects that contribute to mental ill health ( Robson, 2013 ).
The paramedic's role in health promotion has changed over time, and is now being recognised as an important element that positively influences and promotes health (CoP, 2015). Furthermore, the CoP (2015) has recognised the importance of introducing it into their scope of practice. It is essential that student paramedics learn how to encourage patients to maintain their health and wellbeing, enabling them to make an informed decisions on these ( HCPC, 2016 ).
There are a multitude of health promotion models, which include the health belief model and the making every contact count programme; the latter aims to promote positive behaviour changes to improve physical and mental health by using the interaction between clinicians and patients ( NHS, 2023 ).
Brief interactions have been shown to be cost effective and prove useful ( McCambridge (2014) . However, paramedics' interactions with patients are often short and evidence suggests that there are concerns regarding long-term impact ( Upton and Thirlaway, 2014 ). This could result from time constraints when attending patients amid high operational demand. Paramedics also have limited resources to provide long-term health promotion as this is outside their remit ( HCPC, 2016 ). Nonetheless, offering the patient onward referral to additional services may prove beneficial. Robson (2013) highlight that positive change can occur through collaboration between emergency and primary care.
Hospital troponin testing
Taking into consideration the assessment and history, the author was considering a diagnosis of ACS. In spite of this, the author was unable to definitively identify the underlying cause of the patient's chest pain. Therefore, the patient would benefit from conveyance to the ED for troponin T measurement, assessment and monitoring ( Brown et al, 2019 ; National Institute of Health and Care Excellence, 2020a ; 2020b ). Troponin assays are an essential diagnostic tool used in conjunction with an ECG in the diagnosis and treatment of patients with cardiac events ( Fox and Diercks, 2016 ; ESC, 2020 ).
Wei-Ji et al (2015) conducted a meta-analysis involving 2863 patients to compare the sensitivity and specificity of high-sensitive troponin assays (Hs-TnT) and conventional cardiac troponin assays (cTn). The study found that Hs-TnT had a sensitivity of 79% compared to 59% for cTn. However, specificity was similar in the two with a difference of only 3%. The research identified that if conducted within 3 hours of symptom onset, Hs-TnT assays can rule MI in or out more quickly than cTn. The variation in specificity could result from different cardiac conditions raising troponin levels, thereby influencing results. Overall, the study identified the usefulness of troponin assays to aid the diagnostic process. This highlights the need for the patient to be transferred to the ED for a definitive diagnosis. The study was peer reviewed, which increases validity and reliability, and involved a large sample, which aids reproducibility.
I advised the patient that these additional investigations would be required to provide a definitive diagnosis. I provided her with the information needed to make an informed decision, which promotes autonomy and supports holistic, patient-centred care ( Pulvirenti et al, 2014 ).
Decision support and authority bias
Mentorship is a way in which an experienced clinician coaches a mentee to improve their knowledge, skills and professional development by progressing through the four stages of competence ( Burgess et al, 2018 ). Student paramedics prototype their behaviour based on their mentor's effectiveness to coach and act as a role model through concurrent observation and mimicking of behaviour ( Matheson, 2019 ).
As I am aware of the gaps in my knowledge, I identify myself as consciously competent on the four stages of competence model ( Taylor, 2016 ). However, I would class my mentor as unconsciously competent because of their vast experience and effortless performance ( Ryan and Halliwell, 2012 ).
Because of our different competence stages, I still look up to my mentor as a senior figure in my decision-making processes. This means there is a risk of an authority bias occurring as my mentor ultimately makes the final decision on patient care ( Burgess et al, 2018 ).
However, during this case, authority bias did not occur as my mentor supported my rationale and the decision to convey the patient to hospital.
Research on mentoring within the prehospital environment appears limited, making it difficult to fully evaluate the effectiveness of the mentor role.
Having an understanding of individual biases is the first step in identifying the unconscious influences that affect decision-making ( Rutherford, 2020 ). This essay acknowledges that cognitive bias occurs within paramedic practice and can influence practice both positively and negatively.
My improved awareness will allow me to mitigate such bias within my practice before it occurs, ultimately improving the care I am able to deliver to patients.
Acknowledging the limitations of diagnostic tools and considering the likelihood of disease will continue to improve my ability to differentiate diagnoses and reduce the likelihood of misdiagnosis.
In addition, being aware of my own limitations when using system 1 and 2 thinking and identifying myself as consciously incompetent mean I have an increased awareness of my own self-limitations that I can carry forward within my own clinical practice JPP
LEARNING OUTCOMES
After completing this module, the paramedic will be able to:
- Have an understanding of cognitive bias and how this can affect the decision-making process
- Recognise the differences in type 1 and 2 thinking and the importance of their application when encountering different clinical presentations
- Understand the differences between sensitivity, specificity and its application to common tests
- Understand how probability is affected by the above terms
If you would like to send feedback, please email [email protected]
🧠 The Psychology of Design 106 Cognitive Biases & Principles That Affect Your UX
Every time users interact with your product, they:
- 🙈 Filter the information
- 🔮 Seek the meaning of it
- ⏰ Act within a given time
- 💾 Store bits of the interaction in their memories
So to improve your user experience , you need to understand the biases & heuristics affecting those four decision-cycle steps.
Below is a list of cognitive biases and design principles (with examples and tips) for each category. Let’s dive right in.
PS: Don’t have time to read the whole list? Get the cheat sheet
🙈 Information
Users filter out a lot of the information that they receive, even when it could be important.
👀 Hick's Law
More options leads to harder decisions
💼 Confirmation Bias
People look for evidence that confirms what they think
Previous stimuli influence users' decision
🚛 Cognitive Load
Total amount of mental effort that is required to complete a task
⚓️ Anchoring Bias
Users rely heavily on the first piece of information they see
Subtle hints can affect users' decisions
🍰 Progressive Disclosure
Users are less overwhelmed if they're exposed to complex features later
🎯 Fitts's Law
Large and close elements are easier to interact with
🕶 Banner Blindness
Users tune out the stuff they get repeatedly exposed to
🕺 Decoy Effect
Create a new option that's easy to discard
The way information is presented affects how users make decisions
🐠 Attentional Bias
Users' thoughts filter what they pay attention to
💔 Empathy Gap
People underestimate how much emotions influence user behaviors
⛵️ Visual Anchors
Elements used to guide users' eyes
🌶 Von Restorff Effect
People notice items that stand out more
🎖 Visual Hierarchy
The order in which people perceive what they see
🔭 Selective Attention
People filter out things from their environment when in focus
✈️ Survivorship Bias
People neglect things that don't make it past a selection process
🍒 Juxtaposition
Elements that are close and similar are perceived as a single unit
🚦 Signifiers
Elements that communicate what they will do
Users' attention is drawn to higher visual weights
🚨 External Trigger
When the information on what to do next is within the prompt itself
🎪 Centre-Stage Effect
People tend to choose the middle option in a set of items
🍣 Law of Proximity
Elements close to each other are usually considered related
🍬 Tesler's Law
If you simplify too much, you'll transfer some complexity to the users
🧨 Spark Effect
Users are more likely to take action when the effort is small
🥏 Feedback Loop
When users take action, feedback communicates what happened
😻 Expectations Bias
People tend to be influenced by their own expectations
🚆 Aesthetic-Usability Effect
People perceive designs with great aesthetics as easier to use
When users try to give sense to information, they make stories and assumptions to fill the gaps.
👥 Social Proof
Users adapt their behaviors based on what others do
People value things more when they're in limited supply
💭 Curiosity Gap
Users have a desire to seek out missing information
🖲 Mental Model
Users have a preconceived opinion of how things work
👨👩👧👦 Familiarity Bias
People prefer familiar experiences
🕹 Skeuomorphism
Users adapt more easily to things that look like real-world objects
🎁 Reciprocity
People feel the need to reciprocate when they receive something
🤝 Singularity Effect
Users care disproportionately about an individual as compared to a group
🎰 Variable Reward
People especially enjoy unexpected rewards
🎉 Aha! moment
When new users first realize the value of your product
🥅 Goal Gradient Effect
Motivation increases as users get closer to their goal
💈 Occam’s Razor
Simple solutions are often better than the more complex ones
🎗 Noble Edge Effect
Users tend to prefer socially responsible companies
🧿 Hawthorne Effect
Users change their behavior when they know they are being observed
👼 Halo Effect
People judge things (or people) based on their feelings towards one trait
☎️ Miller’s Law
Users can only keep 7±2 items in their working memory
🍱 Unit Bias
One unit of something feels like the optimal amount
🌊 Flow State
Being fully immersed and focused on a task
👑 Authority Bias
Users attribute more importance to the opinion of an authority figure
🏺 Pseudo-Set Framing
Tasks that are part of a group are more tempting to complete
🎊 Group Attractiveness Effect
Individual items seem more attractive when presented in a group
🚰 Curse of Knowledge
Not realizing that people don't have the same level of knowledge
📮 Self-Initiated Triggers
Users are more likely to interact with prompts they setup for themselves
✏️ Survey Bias
Users tend to skew survey answers towards what's socially acceptable
🎭 Cognitive Dissonance
It's painful to hold two opposing ideas in our mind
💫 Feedforward
When users know what to expect before they take action
🏒 Hindsight Bias
People overestimate their ability to predict outcomes after the fact
🎏 Law of Similarity
Users perceive a relationship between elements that look similar
🌓 Law of Prägnanz
Users interpret ambiguous images in a simpler and more complete form
🐘 Streisand Effect
When trying to censor information ends up increasing awareness of that information
🔦 Spotlight Effect
People tend to believe they are being noticed more than they really are
🗓 Fresh Start Effect
Users are more likely to take action if there's a feeling of new beginnings
Users are busy so they look for shortcuts and jump to conclusions quickly.
🧗♂️ Labor Illusion
People value things more when they see the work behind them
🚶♂️ Default Bias
Users tend not to change an established behavior
🏦 Investment Loops
When users invest themselves, they're more likely to come back
🕯 Loss Aversion
People prefer to avoid losses more than earning equivalent gains
👞 Commitment & Consistency
Users tend to be consistent with their previous actions
🏝 Sunk Cost Effect
Users are reluctant to pull out of something they're invested in.
🌋 Reactance
Users are less likely to adopt a behavior when they feel forced
🔨 Law of the Instrument
If all you have is a hammer, everything looks like a nail
🍭 Temptation Bundling
Hard tasks are less scary when coupled with something users desire
🎩 Dunning-Kruger Effect
People tend to overestimate their skills when they don't know much
🔍 Discoverability
The ease with which users can discover your features
🐍 Second-Order Effect
The consequences of the consequences of actions
🌛 Decision Fatigue
Making a lot of decisions lowers users' ability to make rational ones
🥽 Observer-Expectancy Effect
When researchers' biases influence the participants of an experiment
🌱 Weber's Law
Users adapt better to small incremental changes
🎈 Parkinson’s Law
The time required to complete a task will take as much time as allowed
🌤 Affect Heuristic
People's current emotions cloud and influence their judgment
📉 Hyperbolic Discounting
People tend to prioritize immediate benefits over bigger future gains
⌚️ Chronoception
People's perception of time is subjective
💳 Cashless Effect
People spend more when they can't actually see the money
🌚 Self-serving bias
People take credits for positive events and blame others if negative
🥬 Pareto Principle
Roughly 80% of the effects come from 20% of the causes
🔫 Backfire Effect
When people's convictions are challenged, their beliefs get stronger
🌈 False Consensus Effect
People overestimate how much other people agree with them
🚋 Bandwagon Effect
Users tend to adopt beliefs in proportion of others who have already done so
🧙♂️ Barnum-Forer Effect
When you believe generic personality descriptions apply specifically to you.
🛋 IKEA Effect
When user partially create something, they value it way more
🧚♂️ Planning Fallacy
People tend to underestimate how much time a task will take
Users try to remember what's most important, but their brain prefers some elements over others.
🏕 Provide Exit Points
Invite users to leave your app at the right moment
🎢 Peak-End Rule
People judge an experience by its peak and how it ends.
👅 Sensory Appeal
Users engage more with things appealing to multiple senses
🧩 Zeigarnik Effect
People remember incomplete tasks better than completed ones
🧤 Endowment Effect
Users value something more if they feel it's theirs
People remember grouped information better
💚 Delighters
People remember more unexpected and playful pleasures
💛 Internal Trigger
When users are prompted to take action based on a memory
📸 Picture Superiority Effect
People remember pictures better than words
📌 Method of Loci
People remember things more when they're associated with a location
Incrementally reinforcing actions to get closer to a target behavior
💾 Recognition Over Recall
It's easier to recognize things than recall them from memory
🏰 Storytelling Effect
People remember stories better than facts alone
👹 Negativity Bias
Users recall negative events more than positive ones
⏰ Availability Heuristic
Users favor recent and available information over past information
🌌 Spacing Effect
People learn more effectively when study sessions are spaced out
🏁 Serial Position Effect
It's easier for users to recall the first and last items of a list
Product Psychology Resources
If you want to learn more about behavioral psychology and mental models , we recommend these resources:
📓 Cognitive Biases Codex
The four categories of our list come from Buster Benson's work
📘 Super Thinking
The big book of mental models and cognitive biases (Gabriel Weinberg)
How to build habit-forming products (Nir Eyal)
📕 Influence
The psychology of persuasion (Robert Cialdini)
📔 Predictably Irrational
The hidden forces that shape our decisions (Dan Ariely)
Cognitive Biases Cheat sheet
We took the time to summarize each principle in one line.
They are all in a free cheat sheet of cognitive biases principles .
You can download this cheatsheet as a PDF here .
Use it as a user empathy reminder while you build a feature.
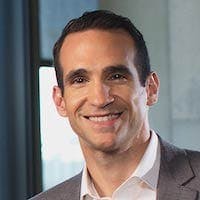
“ We all have a responsibility to build ethically-designed products and services to improve people’s lives. Growth.Design’s list of cognitive biases and psychological principles is a great reference for any team committed to improving their customers’ user experience. Dan & Louis-Xavier’s comic book case studies show you how. ” — Nir Eyal, bestselling author of Hooked and Indistractable
Now It’s Your Turn
So which principle are you going to try next?
Are there missing elements we should add to the list?
You can reach us at [email protected] , we reply to everyone!
- Open access
- Published: 11 May 2024
Does a perceptual gap lead to actions against digital misinformation? A third-person effect study among medical students
- Zongya Li ORCID: orcid.org/0000-0002-4479-5971 1 &
- Jun Yan ORCID: orcid.org/0000-0002-9539-8466 1
BMC Public Health volume 24 , Article number: 1291 ( 2024 ) Cite this article
217 Accesses
12 Altmetric
Metrics details
We are making progress in the fight against health-related misinformation, but mass participation and active engagement are far from adequate. Focusing on pre-professional medical students with above-average medical knowledge, our study examined whether and how third-person perceptions (TPP), which hypothesize that people tend to perceive media messages as having a greater effect on others than on themselves, would motivate their actions against misinformation.
We collected the cross-sectional data through a self-administered paper-and-pencil survey of 1,500 medical students in China during April 2022.
Structural equation modeling (SEM) analysis, showed that TPP was negatively associated with medical students’ actions against digital misinformation, including rebuttal of misinformation and promotion of corrective information. However, self-efficacy and collectivism served as positive predictors of both actions. Additionally, we found professional identification failed to play a significant role in influencing TPP, while digital misinformation self-efficacy was found to broaden the third-person perceptual gap and collectivism tended to reduce the perceptual bias significantly.
Conclusions
Our study contributes both to theory and practice. It extends the third-person effect theory by moving beyond the examination of restrictive actions and toward the exploration of corrective and promotional actions in the context of misinformation., It also lends a new perspective to the current efforts to counter digital misinformation; involving pre-professionals (in this case, medical students) in the fight.
Peer Review reports
Introduction
The widespread persistence of misinformation in the social media environment calls for effective strategies to mitigate the threat to our society [ 1 ]. Misinformation has received substantial scholarly attention in recent years [ 2 ], and solution-oriented explorations have long been a focus but the subject remains underexplored [ 3 ].
Health professionals, particularly physicians and nurses, are highly expected to play a role in the fight against misinformation as they serve as the most trusted information sources regarding medical topics [ 4 ]. However, some barriers, such as limitations regarding time and digital skills, greatly hinder their efforts to tackle misinformation on social media [ 5 ].
Medical students (i.e., college students majoring in health/medical science), in contrast to medical faculty, have a greater potential to become the major force in dealing with digital misinformation as they are not only equipped with basic medical knowledge but generally possess greater social media skills than the former generation [ 6 ]. Few studies, to our knowledge, have tried to explore the potential of these pre-professionals in tackling misinformation. Our research thus fills the gap by specifically exploring how these pre-professionals can be motivated to fight against digital health-related misinformation.
The third-person perception (TPP), which states that people tend to perceive media messages as having a greater effect on others than on themselves [ 7 ], has been found to play an important role in influencing individuals’ coping strategies related to misinformation. But empirical exploration from this line of studies has yielded contradictory results. Some studies revealed that individuals who perceived a greater negative influence of misinformation on others than on themselves were more likely to take corrective actions to debunk misinformation [ 8 ]. In contrast, some research found that stronger TPP reduced individuals’ willingness to engage in misinformation correction [ 9 , 10 ]. Such conflicting findings impel us to examine the association between the third-person perception and medical students’ corrective actions in response to misinformation, thus attempting to unveil the underlying mechanisms that promote or inhibit these pre-professionals’ engagement with misinformation.
Researchers have also identified several perceptual factors that motivate individuals’ actions against misinformation, especially efficacy-related concepts (e.g., self-efficacy and health literacy) and normative variables (e.g., subjective norms and perceived responsibility) [ 3 , 8 , 9 ]. However, most studies devote attention to the general population; little is known about whether and how these factors affect medical students’ intentions to deal with misinformation. We recruited Chinese medical students in order to study a social group that is mutually influenced by cultural norms (collectivism in Chinese society) and professional norms. Meanwhile, systematic education and training equip medical students with abundant clinical knowledge and good levels of eHealth literacy [ 5 ], which enable them to have potential efficacy in tackling misinformation. Our study thus aims to examine how medical students’ self-efficacy, cultural norms (i.e., collectivism) and professional norms (i.e., professional identification) impact their actions against misinformation.
Previous research has found self-efficacy to be a reliable moderator of optimistic bias, the tendency for individuals to consider themselves as less likely to experience negative events but more likely to experience positive events as compared to others [ 11 , 12 , 13 ]. As TPP is thought to be a product of optimistic bias, accordingly, self-efficacy should have the potential to influence the magnitude of third-person perception [ 14 , 15 ]. Meanwhile, scholars also suggest that the magnitude of TPP is influenced by social distance corollary [ 16 , 17 ]. Simply put, individuals tend to perceive those who are more socially distant from them to be more susceptible to the influence of undesirable media than those who are socially proximal [ 18 , 19 , 20 ]. From a social identity perspective, collectivism and professional identification might moderate the relative distance between oneself and others while the directions of such effects differ [ 21 , 22 ]. For example, collectivists tend to perceive a smaller social distance between self and others as “they are less likely to view themselves as distinct or unique from others” [ 23 ]. In contrast, individuals who are highly identified with their professional community (i.e., medical community) are more likely to perceive a larger social distance between in-group members (including themselves) and out-group members [ 24 ]. In this way, collectivism and professional identification might exert different effects on TPP. On this basis, this study aims to examine whether and how medical students’ perceptions of professional identity, self-efficacy and collectivism influence the magnitude of TPP and in turn influence their actions against misinformation.
Our study builds a model that reflects the theoretical linkages among self-efficacy, collectivism, professional identity, TPP, and actions against misinformation. The model, which clarifies the key antecedents of TPP and examines the mediating role of TPP, contribute to the third-person effect literature and offer practical contributions to countering digital misinformation.
Context of the study
As pre-professionals equipped with specialized knowledge and skills, medical students have been involved in efforts in health communication and promotion during the pandemic. For instance, thousands of medical students have participated in various volunteering activities in the fight against COVID-19, such as case data visualization [ 25 ], psychological counseling [ 26 ], and providing online consultations [ 27 ]. Due to the shortage of medical personnel and the burden of work, some medical schools also encouraged their students to participate in health care assistance in hospitals during the pandemic [ 28 , 29 ].
The flood of COVID-19 related misinformation has posed an additional threat to and burden on public health. We have an opportunity to address this issue and respond to the general public’s call for guidance from the medical community about COVID-19 by engaging medical students as a main force in the fight against coronavirus related misinformation.
Literature review
The third-person effect in the misinformation context.
Originally proposed by Davison [ 7 ], the third-person effect hypothesizes that people tend to perceive a greater effect of mass media on others than on themselves. Specifically, the TPE consists of two key components: the perceptual and the behavioral [ 16 ]. The perceptual component centers on the perceptual gap where individuals tend to perceive that others are more influenced by media messages than themselves. The behavioral component refers to the behavioral outcomes of the self-other perceptual gap in which people act in accordance with such perceptual asymmetry.
According to Perloff [ 30 ], the TPE is contingent upon situations. For instance, one general finding suggests that when media messages are considered socially undesirable, nonbeneficial, or involving risks, the TPE will get amplified [ 16 ]. Misinformation characterized as inaccurate, misleading, and even false, is regarded as undesirable in nature [ 31 ]. Based on this line of reasoning, we anticipate that people will tend to perceive that others would be more influenced by misinformation than themselves.
Recent studies also provide empirical evidence of the TPE in the context of misinformation [ 32 ]. For instance, an online survey of 511 Chinese respondents conducted by Liu and Huang [ 33 ] revealed that individuals would perceive others to be more vulnerable to the negative influence of COVID-19 digital disinformation. An examination of the TPE within a pre-professional group – the medical students–will allow our study to examine the TPE scholarship in a particular population in the context of tackling misinformation.
Why TPE occurs among medical students: a social identity perspective
Of the works that have provided explanations for the TPE, the well-known ones include self-enhancement [ 34 ], attributional bias [ 35 ], self-categorization theory [ 36 ], and the exposure hypothesis [ 19 ]. In this study, we argue for a social identity perspective as being an important explanation for third-person effects of misinformation among medical students [ 36 , 37 ].
The social identity explanation suggests that people define themselves in terms of their group memberships and seek to maintain a positive self-image through favoring the members of their own groups over members of an outgroup, which is also known as downward comparison [ 38 , 39 ]. In intergroup settings, the tendency to evaluate their ingroups more positively than the outgroups will lead to an ingroup bias [ 40 ]. Such an ingroup bias is typically described as a trigger for the third-person effect as individuals consider themselves and their group members superior and less vulnerable to undesirable media messages than are others and outgroup members [ 20 ].
In the context of our study, medical students highly identified with the medical community tend to maintain a positive social identity through an intergroup comparison that favors the ingroup and derogates the outgroup (i.e., the general public). It is likely that medical students consider themselves belonging to the medical community and thus are more knowledgeable and smarter than the general public in health-related topics, leading them to perceive the general public as more vulnerable to health-related misinformation than themselves. Accordingly, we propose the following hypothesis:
H1: As medical students’ identification with the medical community increases, the TPP concerning digital misinformation will become larger.
What influences the magnitude of TPP
Previous studies have demonstrated that the magnitude of the third-person perception is influenced by a host of factors including efficacy beliefs [ 3 ] and cultural differences in self-construal [ 22 , 23 ]. Self-construal is defined as “a constellation of thoughts, feelings, and actions concerning the relationship of the self to others, and the self as distinct from others” [ 41 ]. Markus and Kitayama (1991) identified two dimensions of self-construal: Independent and interdependent. Generally, collectivists hold an interdependent view of the self that emphasizes harmony, relatedness, and places importance on belonging, whereas individualists tend to have an independent view of the self and thus view themselves as distinct and unique from others [ 42 ]. Accordingly, cultural values such as collectivism-individualism should also play a role in shaping third-person perception due to the adjustment that people make of the self-other social identity distance [ 22 ].
Set in a Chinese context aiming to explore the potential of individual-level approaches to deal with misinformation, this study examines whether collectivism (the prevailing cultural value in China) and self-efficacy (an important determinant of ones’ behavioral intentions) would affect the magnitude of TPP concerning misinformation and how such impact in turn would influence their actions against misinformation.
The impact of self-efficacy on TPP
Bandura [ 43 ] refers to self-efficacy as one’s perceived capability to perform a desired action required to overcome barriers or manage challenging situations. He also suggests understanding self-efficacy as “a differentiated set of self-beliefs linked to distinct realms of functioning” [ 44 ]. That is to say, self-efficacy should be specifically conceptualized and operationalized in accordance with specific contexts, activities, and tasks [ 45 ]. In the context of digital misinformation, this study defines self-efficacy as one’s belief in his/her abilities to identify and verify misinformation within an affordance-bounded social media environment [ 3 ].
Previous studies have found self-efficacy to be a reliable moderator of biased optimism, which indicates that the more efficacious individuals consider themselves, the greater biased optimism will be invoked [ 12 , 23 , 46 ]. Even if self-efficacy deals only with one’s assessment of self in performing a task, it can still create the other-self perceptual gap; individuals who perceive a higher self-efficacy tend to believe that they are more capable of controlling a stressful or challenging situation [ 12 , 14 ]. As such, they are likely to consider themselves less vulnerable to negative events than are others [ 23 ]. That is, individuals with higher levels of self-efficacy tend to underestimate the impact of harmful messages on themselves, thereby widening the other-self perceptual gap.
In the context of fake news, which is closely related to misinformation, scholars have confirmed that fake news efficacy (i.e., a belief in one’s capability to evaluate fake news [ 3 ]) may lead to a larger third-person perception. Based upon previous research evidence, we thus propose the following hypothesis:
H2: As medical students’ digital misinformation self-efficacy increases, the TPP concerning digital misinformation will become larger.
The influence of collectivism on TPP
Originally conceptualized as a societal-level construct [ 47 ], collectivism reflects a culture that highlights the importance of collective goals over individual goals, defines the self in relation to the group, and places great emphasis on conformity, harmony and interdependence [ 48 ]. Some scholars propose to also examine cultural values at the individual level as culture is embedded within every individual and could vary significantly among individuals, further exerting effects on their perceptions, attitudes, and behaviors [ 49 ]. Corresponding to the construct at the macro-cultural level, micro-psychometric collectivism which reflects personality tendencies is characterized by an interdependent view of the self, a strong sense of other-orientation, and a great concern for the public good [ 50 ].
A few prior studies have indicated that collectivism might influence the magnitude of TPP. For instance, Lee and Tamborini [ 23 ] found that collectivism had a significant negative effect on the magnitude of TPP concerning Internet pornography. Such an impact can be understood in terms of biased optimism and social distance. Collectivists tend to view themselves as an integral part of a greater social whole and consider themselves less differentiated from others [ 51 ]. Collectivism thus would mitigate the third-person perception due to a smaller perceived social distance between individuals and other social members and a lower level of comparative optimism [ 22 , 23 ]. Based on this line of reasoning, we thus propose the following hypothesis:
H3: As medical students’ collectivism increases, the TPP concerning digital misinformation will become smaller.
Behavioral consequences of TPE in the misinformation context
The behavioral consequences trigged by TPE have been classified into three categories: restrictive actions refer to support for censorship or regulation of socially undesirable content such as pornography or violence on television [ 52 ]; corrective action is a specific type of behavior where people seek to voice their own opinions and correct the perceived harmful or ambiguous messages [ 53 ]; promotional actions target at media content with desirable influence, such as advocating for public service announcements [ 24 ]. In a word, restriction, correction and promotion are potential behavioral outcomes of TPE concerning messages with varying valence of social desirability [ 16 ].
Restrictive action as an outcome of third-person perceptual bias (i.e., the perceptual component of TPE positing that people tend to perceive media messages to have a greater impact on others than on themselves) has received substantial scholarly attention in past decades; scholars thus suggest that TPE scholarship to go beyond this tradition and move toward the exploration of corrective and promotional behaviors [ 16 , 24 ]. Moreover, individual-level corrective and promotional actions deserve more investigation specifically in the context of countering misinformation, as efforts from networked citizens have been documented as an important supplement beyond institutional regulations (e.g., drafting policy initiatives to counter misinformation) and platform-based measures (e.g., improving platform algorithms for detecting misinformation) [ 8 ].
In this study, corrective action specifically refers to individuals’ reactive behaviors that seek to rectify misinformation; these include such actions as debunking online misinformation by commenting, flagging, or reporting it [ 3 , 54 ]. Promotional action involves advancing correct information online, including in response to misinformation that has already been disseminated to the public [ 55 ].
The impact of TPP on corrective and promotional actions
Either paternalism theory [ 56 ] or the protective motivation theory [ 57 ] can act as an explanatory framework for behavioral outcomes triggered by third-person perception. According to these theories, people act upon TPP as they think themselves to know better and feel obligated to protect those who are more vulnerable to negative media influence [ 58 ]. That is, corrective and promotional actions as behavioral consequences of TPP might be driven by a protective concern for others and a positive sense of themselves.
To date, several empirical studies across contexts have examined the link between TPP and corrective actions. Koo et al. [ 8 ], for instance, found TPP was not only positively related to respondents’ willingness to correct misinformation propagated by others, but also was positively associated with their self-correction. Other studies suggest that TPP motivates individuals to engage in both online and offline corrective political participation [ 59 ], give a thumbs down to a biased story [ 60 ], and implement corrective behaviors concerning “problematic” TV reality shows [ 16 ]. Based on previous research evidence, we thus propose the following hypothesis:
H4: Medical students with higher degrees of TPP will report greater intentions to correct digital misinformation.
Compared to correction, promotional behavior has received less attention in the TPE research. Promotion commonly occurs in a situation where harmful messages have already been disseminated to the public and others appear to have been influenced by these messages, and it serves as a remedial action to amplify messages with positive influence which may in turn mitigate the detrimental effects of harmful messages [ 16 ].
Within this line of studies, however, empirical studies provide mixed findings. Wei and Golan [ 24 ] found a positive association between TPP of desirable political ads and promotional social media activism such as posting or linking the ad on their social media accounts. Sun et al. [ 16 ] found a negative association between TPP regarding clarity and community-connection public service announcements (PSAs) and promotion behaviors such as advocating for airing more PSAs in TV shows.
As promotional action is still underexplored in the TPE research, and existing evidence for the link between TPP and promotion is indeed mixed, we thus propose an exploratory research question:
RQ1: What is the relationship between TPP and medical students’ intentions to promote corrective information?
The impact of self-efficacy and collectivism on actions against misinformation
According to social cognitive theory, people with higher levels of self-efficacy tend to believe they are competent and capable and are more likely to execute specific actions [ 43 ]. Within the context of digital misinformation, individuals might become more willing to engage in misinformation correction if they have enough knowledge and confidence to evaluate information, and possess sufficient skills to verify information through digital tools and services [ 61 ].
Accordingly, we assumed medical students with higher levels of digital misinformation self-efficacy would be likely to become more active in the fight against misinformation.
H5: Medical students with higher levels of digital misinformation self-efficacy will report greater intentions to (a) correct misinformation and (b) promote corrective information on social media.
Social actions of collectivists are strongly guided by prevailing social norms, collective responsibilities, and common interest, goals, and obligations [ 48 ]. Hence, highly collectivistic individuals are more likely to self-sacrifice for group interests and are more oriented toward pro-social behaviors, such as adopting pro-environmental behaviors [ 62 ], sharing knowledge [ 23 ], and providing help for people in need [ 63 ].
Fighting against misinformation is also considered to comprise altruism, especially self-engaged corrective and promotional actions, as such actions are costly to the actor (i.e., taking up time and energy) but could benefit the general public [ 61 ]. Accordingly, we assume collectivism might play a role in prompting people to engage in reactive behaviors against misinformation.
It is also noted that collectivist values are deeply rooted in Chinese society and were especially strongly advocated during the outbreak of COVID-19 with an attempt to motivate prosocial behaviors [ 63 ]. Accordingly, we expected that the more the medical students were oriented toward collectivist values, the more likely they would feel personally obliged and normatively motivated to engage in misinformation correction. However, as empirical evidence was quite limited, we proposed exploratory research questions:
RQ2: Will medical students with higher levels of collectivism report greater intentions to (a) correct misinformation and (b) promote corrective information on social media?
The theoretical model
To integrate both the antecedents and consequences of TPP, we proposed a theoretical model (as shown in Fig. 1 ) to examine how professional identification, self-efficacy and collectivism would influence the magnitude of TPP, and how such impact would in turn influence medical students’ intentions to correct digital misinformation and promote corrective information. Thus, RQ3 was proposed:
RQ3: Will the TPP mediate the impact of self-efficacy and collectivism on medical students’ intentions to (a) correct misinformation, and (b) promote corrective information on social media? Fig. 1 The proposed theoretical model. DMSE = Digital Misinformation Self-efficacy; PIMC = Professional Identification with Medical Community; ICDM = Intention to Correct Digital Misinformation; IPCI = Intention to Promote Corrective Information Full size image
To examine the proposed hypotheses, this study utilized cross-sectional survey data from medical students in Tongji Medical College (TJMC) of China. TJMC is one of the birthplaces of Chinese modern medical education and among the first universities and colleges that offer eight-year curricula on clinical medicine. Further, TJMC is located in Wuhan, the epicenter of the initial COVID-19 outbreaks, thus its students might find the pandemic especially relevant – and threatening – to them.
The survey instrument was pilot tested using a convenience sample of 58 respondents, leading to minor refinements to a few items. Upon approval from the university’s Institutional Research Board (IRB), the formal investigation was launched in TJMC during April 2022. Given the challenges of reaching the whole target population and acquiring an appropriate sampling frame, this study employed purposive and convenience sampling.
We first contacted four school counselors as survey administrators through email with a letter explaining the objective of the study and requesting cooperation. All survey administrators were trained by the principal investigator to help with the data collection in four majors (i.e., basic medicine, clinical medicine, nursing, and public health). Paper-and-pencil questionnaires were distributed to students on regular weekly departmental meetings of each major as students in all grades (including undergraduates, master students, and doctoral students) were required to attend the meeting. The projected time of completion of the survey was approximately 10–15 min. The survey administrators indicated to students that participation was voluntary, their responses would remain confidential and secure, and the data would be used only for academic purposes. Though a total of 1,500 participants took the survey, 17 responses were excluded from the analysis as they failed the attention filters. Ultimately, a total of 1,483 surveys were deemed valid for analysis.
Of the 1,483 respondents, 624 (42.10%) were men and 855 (57.70%) were women, and four did not identify gender. The average age of the sample was 22.00 ( SD = 2.54, ranging from 17 to 40). Regarding the distribution of respondents’ majors, 387 (26.10%) were in basic medicine, 390 (26.30%) in clinical medicine, 307 (20.70%) in nursing, and 399 (26.90%) in public health. In terms of university class, 1,041 (70.40%) were undergraduates, 291 (19.70%) were working on their master degrees, 146 (9.90%) were doctoral students, and five did not identify their class data.
Measurement of key variables
Perceived effects of digital misinformation on oneself and on others.
Three modified items adapted from previous research [ 33 , 64 ] were employed to measure perceived effects of digital misinformation on oneself. Respondents were asked to indicate to what extent they agreed with the following: (1) I am frequently concerned that the information about COVID-19 I read on social media might be false; (2) Misinformation on social media might misguide my understanding of the coronavirus; (3) Misinformation on social media might influence my decisions regarding COVID-19. The response categories used a 7-point scale, where 1 meant “strongly disagree” and 7 meant “strongly agree.” The measure of perceived effects of digital misinformation on others consisted of four parallel items with the same statement except replacing “I” and “my” with “the general others” and “their”. The three “self” items were averaged to create a measure of “perceived effects on oneself” ( M = 3.98, SD = 1.49, α = 0.87). The three “others” items were also added and averaged to form an index of “perceived effects on others” ( M = 4.62, SD = 1.32, α = 0.87).
The perceived self-other disparity (TPP)
TPP was derived by subtracting perceived effects on oneself from perceived effects on others.
Professional identification with medical community
Professional identification was measured using a three item, 7-point Likert-type scale (1 = strongly disagree , 7 = strongly agree ) adapted from previous studies [ 65 , 66 ] by asking respondents to indicate to what extent they agreed with the following statements: (1) I would be proud to be a medical staff member in the future; (2) I am committed to my major; and (3) I will be in an occupation that matches my current major. The three items were thus averaged to create a composite measure of professional identification ( M = 5.34, SD = 1.37, α = 0.88).
Digital misinformation self-efficacy
Modified from previous studies [ 3 ], self-efficacy was measured with three items. Respondents were asked to indicate on a 7-point Linkert scale from 1 (strongly disagree) to 7 (strongly agree) their agreement with the following: (1) I think I can identify misinformation relating to COVID-19 on social media by myself; (2) I know how to verify misinformation regarding COVID-19 by using digital tools such as Tencent Jiaozhen Footnote 1 and Piyao.org.cn Footnote 2 ; (3) I am confident in my ability to identify digital misinformation relating to COVID-19. A composite measure of self-efficacy was constructed by averaging the three items ( M = 4.38, SD = 1.14, α = 0.77).
- Collectivism
Collectivism was measured using four items adapted from previous research [ 67 ], in which respondents were asked to indicate their agreement with the following statements on a 7-point scale, from 1 (strongly disagree) to 7 (strongly agree): (1) Individuals should sacrifice self-interest for the group; (2) Group welfare is more important than individual rewards; (3) Group success is more important than individual success; and (4) Group loyalty should be encouraged even if individual goals suffer. Therefore, the average of the four items was used to create a composite index of collectivism ( M = 4.47, SD = 1.30, α = 0.89).
Intention to correct digital misinformation
We used three items adapted from past research [ 68 ] to measure respondents’ intention to correct misinformation on social media. All items were scored on a 7-point scale from 1 (very unlikely) to 7 (very likely): (1) I will post a comment saying that the information is wrong; (2) I will message the person who posts the misinformation to tell him/her the post is wrong; (3) I will track the progress of social media platforms in dealing with the wrong post (i.e., whether it’s deleted or corrected). A composite measure of “intention to correct digital misinformation” was constructed by adding the three items and dividing by three ( M = 3.39, SD = 1.43, α = 0.81).
Intention to promote corrective information
On a 7-point scale ranging from 1 (very unlikely) to 7 (very likely), respondents were asked to indicate their intentions to (1) Retweet the corrective information about coronavirus on my social media account; (2) Share the corrective information about coronavirus with others through Social Networking Services. The two items were averaged to create a composite measure of “intention to promote corrective information” ( M = 4.60, SD = 1.68, r = 0.77).
Control variables
We included gender, age, class (1 = undergraduate degree; 2 = master degree; 3 = doctoral degree), and clinical internship (0 = none; 1 = less than 0.5 year; 2 = 0.5 to 1.5 years; 3 = 1.5 to 3 years; 4 = more than 3 years) as control variables in the analyses. Additionally, coronavirus-related information exposure (i.e., how frequently they were exposed to information about COVID-19 on Weibo, WeChat, and QQ) and misinformation exposure on social media (i.e., how frequently they were exposed to misinformation about COVID-19 on Weibo, WeChat, and QQ) were also assessed as control variables because previous studies [ 69 , 70 ] had found them relevant to misinformation-related behaviors. Descriptive statistics and bivariate correlations between main variables were shown in Table 1 .
Statistical analysis
We ran confirmatory factor analysis (CFA) in Mplus (version 7.4, Muthén & Muthén, 1998) to ensure the construct validity of the scales. To examine the associations between variables and tested our hypotheses, we performed structural equation modeling (SEM). Mplus was chosen over other SEM statistical package mainly because the current data set included some missing data, and the Mplus has its strength in handling missing data using full-information maximum likelihood imputation, which enabled us to include all available data [ 71 , 72 ]. Meanwhile, Mplus also shows great flexibility in modelling when simultaneously handling continuous, categorical, observed, and latent variables in a variety of models. Further, Mplus provides a variety of useful information in a concise manner [ 73 ].
Table 2 shows the model fit information for the measurement and structural models. Five latent variables were specified in the measurement model. To test the measurement model, we examined the values of Cronbach’s alpha, composite reliability (CR), and average variance extracted (AVE) (Table 1 ). Cronbach’s alpha values ranged from 0.77 to 0.89. The CRs, which ranged from 0.78 to 0.91, exceeded the level of 0.70 recommended by Fornell (1982) and thus confirmed the internal consistency. The AVE estimates, which ranged from 0.54 to 0.78, exceeded the 0.50 lower limit recommended by Fornell and Larcker (1981), and thus supported convergent validity. All the square roots of AVE were greater than the off-diagonal correlations in the corresponding rows and columns [ 74 ]. Therefore, discriminant validity was assured. In a word, our measurement model showed sufficient convergence and discriminant validity.
Five model fit indices–the relative chi-square ratio (χ 2 / df ), the comparative fit index (CFI), the Tucker–Lewis index (TLI), the root mean square error of approximation (RMSEA), and the standardized root-mean-square residual (SRMR) were used to assess the model. Specifically, the normed chi-square between 1 and 5 is acceptable [ 75 ]. TLI and CFI over 0.95 are considered acceptable, SRMR value less than 0.08 and RMSEA value less than 0.06 indicate good fit [ 76 ]. Based on these criteria, the model was found to have an acceptable fit to the data.
Figure 2 presents the results of our hypothesized model. H1 was rejected as professional identification failed to predict TPP ( β = 0.06, p > 0.05). Self-efficacy was positively associated with TPP ( β = 0.14, p < 0.001) while collectivism was negatively related to TPP ( β = -0.10, p < 0.01), lending support to H2 and H3.
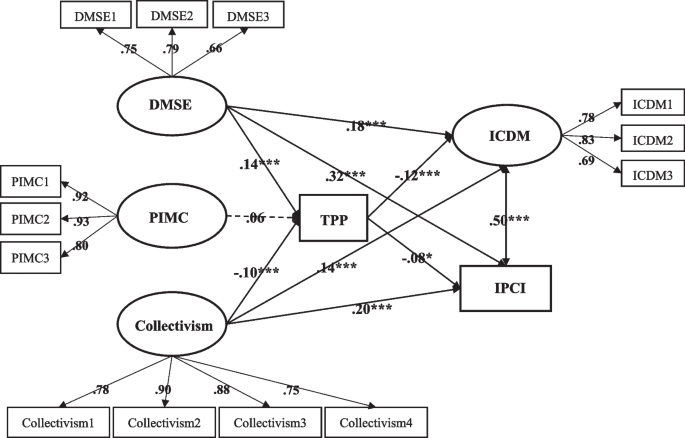
Note. N = 1,483. The coefficients of relationships between latent variables are standardized beta coefficients. Significant paths are indicated by solid line; non-significant paths are indicated by dotted lines. * p < .05, ** p < .01; *** p < .001. DMSE = Digital Misinformation Self-efficacy; PIMC = Professional Identification with Medical Community; ICDM = Intention to Correct Digital Misinformation; IPCI = Intention to Promote Corrective Information
H4 posited that medical students with higher degrees of TPP would report greater intentions to correct digital misinformation. However, we found a negative association between TPP and intentions to correct misinformation ( β = -0.12, p < 0.001). H4 was thus rejected. Regarding RQ1, results revealed that TPP was negatively associated with intentions to promote corrective information ( β = -0.08, p < 0.05).
Further, our results supported H5 as we found that self-efficacy had a significant positive relationship with corrective intentions ( β = 0.18, p < 0.001) and promotional intentions ( β = 0.32, p < 0.001). Collectivism was also positively associated with intentions to correct misinformation ( β = 0.14, p < 0.001) and promote corrective information ( β = 0.20, p < 0.001), which answered RQ2.
Regarding RQ3 (see Table 3 ), TPP significantly mediated the relationship between self-efficacy and intentions to correct misinformation ( β = -0.016), as well as the relationship between self-efficacy and intentions to promote corrective information ( β = -0.011). However, TPP failed to mediate either the association between collectivism and corrective intentions ( β = 0.011, ns ) or the association between collectivism and promotional intentions ( β = 0.007, ns ).
Recent research has highlighted the role of health professionals and scientists in the fight against misinformation as they are considered knowledgeable, ethical, and reliable [ 5 , 77 ]. This study moved a step further by exploring the great potential of pre-professional medical students to tackle digital misinformation. Drawing on TPE theory, we investigated how medical students perceived the impact of digital misinformation, the influence of professional identification, self-efficacy and collectivism on these perceptions, and how these perceptions would in turn affect their actions against digital misinformation.
In line with prior studies [ 3 , 63 ], this research revealed that self-efficacy and collectivism played a significant role in influencing the magnitude of third-person perception, while professional identification had no significant impact on TPP. As shown in Table 1 , professional identification was positively associated with perceived effects of misinformation on oneself ( r = 0.14, p < 0.001) and on others ( r = 0.20, p < 0.001) simultaneously, which might result in a diminished TPP. What explains a shared or joint influence of professional identification on self and others? A potential explanation is that even medical staff had poor knowledge about the novel coronavirus during the initial outbreak [ 78 ]. Accordingly, identification with the medical community was insufficient to create an optimistic bias concerning identifying misinformation about COVID-19.
Our findings indicated that TPP was negatively associated with medical students’ intentions to correct misinformation and promote corrective information, which contradicted our hypotheses but was consistent with some previous TPP research conducted in the context of perceived risk [ 10 , 79 , 80 , 81 ]. For instance, Stavrositu and Kim (2014) found that increased TPP regarding cancer risk was negatively associated with behavioral intentions to engage in further cancer information search/exchange, as well as to adopt preventive lifestyle changes. Similarly, Wei et al. (2008) found concerning avian flu news that TPP negatively predicted the likelihood of engaging in actions such as seeking relevant information and getting vaccinated. In contrast, the perceived effects of avian flu news on oneself emerged as a positive predictor of intentions to take protective behavior.
Our study shows a similar pattern as perceived effects of misinformation on oneself were positively associated with intentions to correct misinformation ( r = 0.06, p < 0.05) and promote corrective information ( r = 0.10, p < 0.001, See Table 1 ). While the reasons for the behavioral patterns are rather elusive, such findings are indicative of human nature. When people perceive misinformation-related risk to be highly personally relevant, they do not take chances. However, when they perceive others to be more vulnerable than themselves, a set of sociopsychological dynamics such as self-defense mechanism, positive illusion, optimistic bias, and social comparison provide a restraint on people’s intention to engage in corrective and promotional actions against misinformation [ 81 ].
In addition to the indirect effects via TPP, our study also revealed that self-efficacy and collectivism serve as direct and powerful drivers of corrective and promotive actions. Consistent with previous literature [ 61 , 68 ], individuals will be more willing to engage in social corrections of misinformation if they possess enough knowledge, skills, abilities, and resources to identify misinformation, as correcting misinformation is difficult and their effort would not necessarily yield positive outcomes. Collectivists are also more likely to engage in misinformation correction as they are concerned for the public good and social benefits, aiming to protect vulnerable people from being misguided by misinformation [ 82 ].
This study offers some theoretical advancements. First, our study extends the TPE theory by moving beyond the examination of restrictive actions and toward the exploration of corrective and promotional actions in the context of misinformation. This exploratory investigation suggests that self-other asymmetry biased perception concerning misinformation did influence individuals’ actions against misinformation, but in an unexpected direction. The results also suggest that using TPP alone to predict behavioral outcomes was deficient as it only “focuses on differences between ‘self’ and ‘other’ while ignoring situations in which the ‘self’ and ‘other’ are jointly influenced” [ 83 ]. Future research, therefore, could provide a more sophisticated understanding of third-person effects on behavior by comparing the difference of perceived effects on oneself, perceived effects on others, and the third-person perception in the pattern and strength of the effects on behavioral outcomes.
Moreover, institutionalized corrective solutions such as government and platform regulation are non-exhaustive [ 84 , 85 ]; it thus becomes critical to tap the great potential of the crowd to engage in the fight against misinformation [ 8 ] while so far, research on the motivations underlying users’ active countering of misinformation has been scarce. The current paper helps bridge this gap by exploring the role of self-efficacy and collectivism in predicting medical students’ intentions to correct misinformation and promote corrective information. We found a parallel impact of the self-ability-related factor and the collective-responsibility-related factor on intentions to correct misinformation and promote corrective information. That is, in a collectivist society like China, cultivating a sense of collective responsibility and obligation in tackling misinformation (i.e., a persuasive story told with an emphasis on collective interests of social corrections of misinformation), in parallel with systematic medical education and digital literacy training (particularly, handling various fact-checking tools, acquiring Internet skills for information seeking and verification) would be effective methods to encourage medical students to engage in active countering behaviors against misinformation. Moreover, such an effective means of encouraging social corrections of misinformation might also be applied to the general public.
In practical terms, this study lends new perspectives to the current efforts in dealing with digital misinformation by involving pre-professionals (in this case, medical students) into the fight against misinformation. As digital natives, medical students usually spend more time online, have developed sophisticated digital competencies and are equipped with basic medical knowledge, thus possessing great potential in tackling digital misinformation. This study further sheds light on how to motivate medical students to become active in thwarting digital misinformation, which can help guide strategies to enlist pre-professionals to reduce the spread and threat of misinformation. For example, collectivism education in parallel with digital literacy training would help increase medical students’ sense of responsibility for and confidence in tackling misinformation, thus encouraging them to engage in active countering behaviors.
This study also has its limitations. First, the cross-sectional survey study did not allow us to justify causal claims. Granted, the proposed direction of causality in this study is in line with extant theorizing, but there is still a possibility of reverse causal relationships. To establish causality, experimental research or longitudinal studies would be more appropriate. Our second limitation lies in the generalizability of our findings. With the focus set on medical students in Chinese society, one should be cautious in generalizing the findings to other populations and cultures. For example, the effects of collectivism on actions against misinformation might differ in Eastern and Western cultures. Further studies would benefit from replication in diverse contexts and with diverse populations to increase the overall generalizability of our findings.
Drawing on TPE theory, our study revealed that TPP failed to motivate medical students to correct misinformation and promote corrective information. However, self-efficacy and collectivism were found to serve as direct and powerful drivers of corrective and promotive actions. Accordingly, in a collectivist society such as China’s, cultivating a sense of collective responsibility in tackling misinformation, in parallel with efficient personal efficacy interventions, would be effective methods to encourage medical students, even the general public, to actively engage in countering behaviors against misinformation.
Availability of data and materials
The datasets used and/or analyzed during the current study available from the corresponding author on reasonable request.
Tencent Jiaozhen Fact-Checking Platform which comprises the Tencent information verification tool allow users to check information authenticity through keyword searching. The tool is updated on a daily basis and adopts a human-machine collaboration approach to discovering, verifying, and refuting rumors and false information. For refuting rumors, Tencent Jiaozhen publishes verified content on the homepage of Tencent's rumor-refuting platform, and uses algorithms to accurately push this content to users exposed to the relevant rumors through the WeChat dispelling assistant.
Piyao.org.cn is hosted by the Internet Illegal Information Reporting Center under the Office of the Central Cyberspace Affairs Commission and operated by Xinhuanet.com. The platform is a website that collects statements from Twitter-like services, news portals and China's biggest search engine, Baidu, to refute online rumors and expose the scams of phishing websites. It has integrated over 40 local rumor-refuting platforms and uses artificial intelligence to identify rumors.
Dhawan D, Bekalu M, Pinnamaneni R, McCloud R, Viswanath K. COVID-19 news and misinformation: do they matter for public health prevention? J Health Commun. 2021;26:799–808.
Article PubMed Google Scholar
Janmohamed K, Walter N, Nyhan K, Khoshnood K, Tucker JD, Sangngam N, et al. Interventions to mitigate COVID-19 misinformation: a systematic review and meta-analysis. J Health Commun. 2021;26:846–57.
Cheng Y, Chen ZF. The influence of presumed fake news influence: examining public support for corporate corrective response, media literacy interventions, and governmental regulation. Mass Commun Soc. 2020;23:705–29.
Article Google Scholar
Earnshaw VA, Katz IT. Educate, amplify, and focus to address COVID-19 misinformation. JAMA Health Forum. 2020;1:e200460.
Bautista JR, Zhang Y, Gwizdka J. Healthcare professionals’ acts of correcting health misinformation on social media. Int J Med Inf. 2021;148:104375.
O’Doherty D, Lougheed J, Hannigan A, Last J, Dromey M, O’Tuathaigh C, et al. Internet skills of medical faculty and students: is there a difference? BMC Med Educ. 2019;19:39.
Article PubMed PubMed Central Google Scholar
Davison WP. The third-person effect in communication.
Koo AZ-X, Su M-H, Lee S, Ahn S-Y, Rojas H. What motivates people to correct misinformation? Examining the effects of third-person perceptions and perceived norms. J Broadcast Electron Media. 2021;65:111–34.
Oktavianus J, Bautista JR. Motivating healthcare professionals to correct online health misinformation: the roles of subjective norm, third-person perception, and channel differences. Comput Hum Behav. 2023;147:107839.
Tang S, Willnat L, Zhang H. Fake news, information overload, and the third-person effect in China. Glob Media China. 2021;6:492–507.
Chapin J. Third-person perception and facebook. Int J Cyber Behav Psychol Learn. 2014;4:34–44.
Wei R, Lo V-H, Lu H-Y. Reconsidering the relationship between the third-person perception and optimistic bias. Commun Res. 2007;34:665–84.
Weinstein ND. Unrealistic optimism about future life events. J Pers Soc Psychol. 1980;39:802–20.
Liu X. Media exposure and third-person perception: the mediating role of social realism and proxy efficacy. 2021.
Yang J, Tian Y. “Others are more vulnerable to fake news than I Am”: Third-person effect of COVID-19 fake news on social media users. Comput Hum Behav. 2021;125:106950.
Sun Ye, Shen L, Pan Z. On the behavioral component of the third-person effect. Commun Res. 2008;35:257–78.
Article CAS Google Scholar
Wei R, Lo V-H. The third-person effects of political attack ads in the 2004 U.S. Presidential election. Media Psychol. 2007;9:367–88.
Duck JM, Hogg MA, Terry DJ. Social identity and perceptions of media persuasion: are we always less influenced than others? 1. J Appl Soc Psychol. 1999;29(9):1879–99.
Eveland WP, Nathanson AI, Detenber BH, McLEOD DM. Rethinking the social distance corollary: perceived likelihood of expsoure and the third-person perception. Commun Res. 1999;26:275–302.
Scharrer E. Third-person perception and television violence: the role of out-group stereotyping in perceptions of susceptibility to effects. Commun Res. 2002;29:681–704.
Brownlee K, Halverson G, Chassie A. Multiple relationships: maintaining professional identity in rural social work practice. J Compar Soc Work. 2012;7(1):81–91.
Hogg MA, Reid SA. Social identity, self-categorization, and the communication of group norms. Commun Theory. 2006;16:7–30.
Lee B, Tamborini R. Third-person effect and internet pornography: the influence of collectivism and internet self-efficacy. J Commun. 2005;55:292–310.
Wei R, Golan G. Political advertising on social media in the 2012 presidential election: exploring the perceptual and behavioral components of the third-person effect. Electron News. 2013;7:223–42.
Dong E, Du H, Gardner L. An interactive web-based dashboard to track COVID-19 in real time. Lancet Infect Dis. 2020;20:533–4.
Jun Z, Weili W, Xin Z, Wei Z. Recommended psychological crisis intervention response to the 2019 novel coronavirus pneumonia outbreak in China: a model of West China Hospital. Precis Clin Med. 2020;3(1):3–8.
Shi Y, Zhang S, Fan L, Sun T. What motivates medical students to engage in volunteer behavior during the COVID-19 Outbreak? A large cross-sectional survey. Front Psychol. 2021;11:569765.
Passemard S, Faye A, Dubertret C, Peyre H, Vorms C, Boimare V, ... & Ricard JD. Covid-19 crisis impact on the next generation of physicians: a survey of 800 medical students. BMC Med Educ. 2021;21(1):1–13.
Tempski P, Arantes-Costa FM, Kobayasi R, Siqueira MA, Torsani MB, Amaro BQ, Martins MA. Medical students’ perceptions and motivations during the COVID-19 pandemic. PLoS ONE. 2021;16(3):e0248627.
Article CAS PubMed PubMed Central Google Scholar
Perloff RM. Third-person effect research 1983–1992: a review and synthesis. Int J Public Opin Res. 1993;5:167–84.
Chen L, Fu L. Let’s fight the infodemic: the third-person effect process of misinformation during public health emergencies. Internet Res. 2022;32:1357–77.
Lee T. How people perceive influence of fake news and why it matters. Commun Q. 2021;69:431–53.
Liu PL, Huang LV. Digital disinformation about COVID-19 and the third-person effect: examining the channel differences and negative emotional outcomes. Cyberpsychol Behav Soc Netw. 2020;23:789–93.
Gunther AC, Thorson E. Perceived persuasive effects of product commercials and public service announcements: third-person effects in new domains. Commun Res. 1992;19:574–96.
Gunther A. What we think others think: cause and consequence in the third-person effect. Commun Res. 1991;18:355–72.
Reid SA, Hogg MA. A self-categorization explanation for the third-person effect. Hum Commun Res. 2005;31:129–61.
Cao B, Chen Z, Huang Y, Lo WH. Conflict between Mainland Chinese and Hong Kongers: a social identity perspective in explaining the hostile media phenomenon and the third-person effect. J Appl J Media Stud. 2014;3:225–40.
Google Scholar
Tajfel H. Experimental studies of intergroup behaviour. In: Cognitive analysis of social behavior: Proceedings of the NATO advanced study Institute on “The cognitive analysis of socio-psychological processes”, Aix-enProvence, France, July 12–31, 1981 Dordrecht: Springer Netherlands; 1982. p. 227–46.
Chapter Google Scholar
Tajfel H, Turner JC, Austin WG, Worchel S. An integrative theory of intergroup conflict. Organizational Identity. 1979;56(65):9780203505984–9780203505916.
Crocker J, Luhtanen R. Collective self-esteem and ingroup bias. J Pers Soc Psychol. 1990;58(1).
Singelis TM. The measurement of independent and interdependent self-construals. Pers Soc Psychol Bull. 1994;20(5):580–91.
Cho H, Lee JS. The influence of self-efficacy, subjective norms, and risk perception on behavioral intentions related to the H1N1 flu pandemic: a comparison between K orea and the US. Asian J Soc Psychol. 2015;18(4):311–24.
Bandura A, Freeman WH, Lightsey R. Self-efficacy: the exercise of control. J Cogn Psychother. 1999;13:158–66.
Bandura A, Guide for constructing self-efficacy scales. Self-efficacy beliefs of adolescents. 2006;5(1):307–37.
Pajares F. Self-efficacy beliefs in academic settings. Rev Educ Res. 1996;66:543–78.
Park JS, Ahn HY, Haley EJ. Optimistic bias, advertising skepticism, and consumer intentions for seeking information about the health risks of prescription medicine. Health Mark Q. 2017;34(2):81–96.
Hofstede GH. Culture’s consequences: comparing values, behaviors, institutions, and organizations across nations. 2nd ed. Thousand Oaks: Sage Publications; 2001.
Triandis HC. Individualism and Collectivism. 1st ed. New York: Routledge; 2018.
Wated G, Sanchez JI. Managerial tolerance of nepotism: the effects of individualism-collectivism in a Latin American Context. J Bus Ethics. 2015;130:45–57.
Markus HR, Kitayama S. Culture and the self."Implications for cognition, emotion, and motivation.
Sullivan D, Landau MJ, Kay AC, Rothschild ZK. Collectivism and the meaning of suffering. J Pers Soc Psychol. 2012;103:1023–39.
Lo V, Wei R. Third-person effect, gender, and pornography on the lnternet. J Broadcast Electron Media. 2002;46:13–33.
Barnidge M, Rojas H. Hostile media perceptions, presumed media influence, and political talk: expanding the corrective action hypothesis. Int J Public Opin Res. 2014;26:135–56.
Wintterlin F, Frischlich L, Boberg S, Schatto-Eckrodt T, Reer F, Quandt T. Corrective Actions in the information disorder. the role of presumed media influence and hostile media perceptions for the countering of distorted user-generated content. Polit Commun. 2021;38:773–91.
Wei R, Lo V-H, Lu H-Y, Hou H-Y. Examining multiple behavioral effects of third-person perception: evidence from the news about Fukushima nuclear crisis in Taiwan. Chin J Commun. 2015;8:95–111.
McLEOD DM, Eveland WP, Nathanson AI. Support for censorship of violent and misogynic rap lyrics: an analysis of the third-person effect. Commun Res. 1997;24:153–74.
Nathanson AI, Eveland WP Jr, Park H-S, Paul B. Perceived media influence and efficacy as predictors of caregivers’ protective behaviors. J Broadcast Electron Media. 2002;46:385–410.
McLeod DM, Detenber BH, Eveland WP. Behind the third-person effect: differentiating perceptual processes for self and other. J Commun. 2001;51:678–95.
Rojas H. “Corrective” Actions in the public sphere: how perceptions of media and media effects shape political behaviors. Int J Public Opin Res. 2010;22:343–63.
Chung M, Munno GJ, Moritz B. Triggering participation: exploring the effects of third-person and hostile media perceptions on online participation. Comput Hum Behav. 2015;53:452–61.
Zhao L, Yin J, Song Y. An exploration of rumor combating behavior on social media in the context of social crises. Comput Hum Behav. 2016;58:25–36.
Sherman DK, Updegraff JA, Handy MS, Eom K, Kim HS. Beliefs and social norms as precursors of environmental support: the joint influence of collectivism and socioeconomic status. Pers Soc Psychol Bull. 2022;48:463–77.
Zhu Y, Wei R, Lo V-H, Zhang M, Li Z. Collectivism and altruistic behavior: a third-person effect study of COVID-19 news among Wuhan residents. Glob Media China. 2021;6:476–91.
Yang F, Horning M. Reluctant to share: how third person perceptions of fake news discourage news readers from sharing “real news” on social media. Soc Media Soc. 2020;6:205630512095517.
Adams K, Hean S, Sturgis P, Clark JM. Investigating the factors influencing professional identity of first-year health and social care students. Learn Health Soc Care. 2006;5(2):55–68.
丁汉青, 王军. 冲突与协调: 传媒从业者后备军职业认同状况研究——以北京某高校新闻学院在校生为例. 国际新闻界, 2019;2 :113–131.
Yoo B, Donthu N, Lenartowicz T. Measuring Hofstede’s five dimensions of cultural values at the individual level: development and validation of. J Int Consum Mark. 2011;23(3-4):193-210.
Tandoc EC, Lim D, Ling R. Diffusion of disinformation: how social media users respond to fake news and why. Journalism. 2020;21:381–98.
Tan ASL, Lee C, Chae J. Exposure to health (Mis)Information: lagged effects on young adults’ health behaviors and potential pathways. J Commun. 2015.
Tully M, Bode L, Vraga EK. Mobilizing users: does exposure to misinformation and its correction affect users’ responses to a health misinformation post? Soc Media Soc. 2020;6:205630512097837.
Arbuckle JL. Full information estimation in the presence of in complete data. In: Marcoulides GA, Schumaker RE, editors. Advanced structural equation modeling: issues and techniques. Mahwah: Erlbaum; 1996. p. 243–77.
Narayanan A. A review of eight software packages for structural equation modeling. Am Stat. 2012;66(2):129–38.
Sakaria D, Maat SM, Mohd Matore MEE. Examining the optimal choice of SEM statistical software packages for sustainable mathematics education: a systematic review. Sustainability. 2023;15(4):3209.
Fornell C, Larcker DF. Evaluating structural equation models with unobservable variables and measurement error. J Mark Res. 1981;18(1):39–50.
Wheaton B, Muthen B, Alwin DF, Summers GF. Assessing reliability and stability in panel models. Sociol Methodol. 1977;8:84–136.
Hu LT, Bentler PM. Cutoff criteria for fit indexes in covariance structure analysis: conventional criteria versus new alternatives. Struct Equ Modeling. 1999;6(1):1–55.
Ho SS, Goh TJ, Leung YW. Let’s nab fake science news: predicting scientists’ support for interventions using the influence of presumed media influence model. Journalism. 2022;23:910–28.
Bhagavathula AS, Aldhaleei WA, Rahmani J, Mahabadi MA, Bandari DK. Knowledge and perceptions of COVID-19 among health care workers: cross-sectional study. JMIR Public Health Surveill. 2020;6(2):e19160.
Jung EH, Zhang L, Nekmat E. SNS usage and third-person effects in the risk perception of Zika virus among Singaporean Women. J Health Commun. 2020;25:736–44.
Stavrositu CD, Kim J. Social media metrics: third-person perceptions of health information. Comput Hum Behav. 2014;35:61–7.
Wei R, Lo VH, Lu HY. Third-person effects of health news: exploring the relationships among media exposure, presumed media influence, and behavioral intentions. Am Behav Sci. 2008;52:261–77.
Hong SC. Presumed effects of “fake news” on the global warming discussion in a cross-cultural context. Sustainability. 2020;12(5).
Neuwirth K, Frederick E. Extending the framework of third-, first-, and second-person effects. Mass Commun Soc. 2002;5:113–40.
Bastick Z. Would you notice if fake news changed your behavior? An experiment on the unconscious effects of disinformation. Comput Hum Behav. 2021;116.
Harff D, Bollen C, Schmuck D. Responses to social media influencers’ misinformation about COVID-19: a pre-registered multiple-exposure experiment. Media Psychol. 2022;25:831–50.
Download references
Acknowledgements
We thank all participants and staff working for the project.
This work was supported by Humanities and Social Sciences Youth Foundation of the Ministry of Education of China (Grant No. 21YJC860012).
Author information
Authors and affiliations.
Journalism and Information Communication School, Huazhong University of Science and Technology, Wuhan, Hubei, China
Zongya Li & Jun Yan
You can also search for this author in PubMed Google Scholar
Contributions
Zongya Li wrote the main manuscript and Jun yan collected the data. All authors reviewed the manuscript.
Corresponding author
Correspondence to Jun Yan .
Ethics declarations
Ethics approval and consent to participate.
This study was approved by the Medical Ethics Committee of Union Hospital Affiliated to Tongji Medical College, Huazhong University of Science and Technology (approval number: 2022S009). All the participants provided informed consent to engage in this research.
Consent for publication
The authors give their consent for the publication of identifiable details, which can include photograph(s) and/or videos and/or case history and/or details within the manuscript to be published in the BMC Public Health.
Competing interests
The authors declare no competing interests.
Additional information
Publisher’s note.
Springer Nature remains neutral with regard to jurisdictional claims in published maps and institutional affiliations.
Rights and permissions
Open Access This article is licensed under a Creative Commons Attribution 4.0 International License, which permits use, sharing, adaptation, distribution and reproduction in any medium or format, as long as you give appropriate credit to the original author(s) and the source, provide a link to the Creative Commons licence, and indicate if changes were made. The images or other third party material in this article are included in the article's Creative Commons licence, unless indicated otherwise in a credit line to the material. If material is not included in the article's Creative Commons licence and your intended use is not permitted by statutory regulation or exceeds the permitted use, you will need to obtain permission directly from the copyright holder. To view a copy of this licence, visit http://creativecommons.org/licenses/by/4.0/ . The Creative Commons Public Domain Dedication waiver ( http://creativecommons.org/publicdomain/zero/1.0/ ) applies to the data made available in this article, unless otherwise stated in a credit line to the data.
Reprints and permissions
About this article
Cite this article.
Li, Z., Yan, J. Does a perceptual gap lead to actions against digital misinformation? A third-person effect study among medical students. BMC Public Health 24 , 1291 (2024). https://doi.org/10.1186/s12889-024-18763-9
Download citation
Received : 08 December 2023
Accepted : 02 May 2024
Published : 11 May 2024
DOI : https://doi.org/10.1186/s12889-024-18763-9
Share this article
Anyone you share the following link with will be able to read this content:
Sorry, a shareable link is not currently available for this article.
Provided by the Springer Nature SharedIt content-sharing initiative
- Digital misinformation
- Third-person perception
- Pre-professionals
- Professional identification
BMC Public Health
ISSN: 1471-2458
- Submission enquiries: [email protected]
- General enquiries: [email protected]
Help | Advanced Search
Computer Science > Computation and Language
Title: quite good, but not enough: nationality bias in large language models -- a case study of chatgpt.
Abstract: While nationality is a pivotal demographic element that enhances the performance of language models, it has received far less scrutiny regarding inherent biases. This study investigates nationality bias in ChatGPT (GPT-3.5), a large language model (LLM) designed for text generation. The research covers 195 countries, 4 temperature settings, and 3 distinct prompt types, generating 4,680 discourses about nationality descriptions in Chinese and English. Automated metrics were used to analyze the nationality bias, and expert annotators alongside ChatGPT itself evaluated the perceived bias. The results show that ChatGPT's generated discourses are predominantly positive, especially compared to its predecessor, GPT-2. However, when prompted with negative inclinations, it occasionally produces negative content. Despite ChatGPT considering its generated text as neutral, it shows consistent self-awareness about nationality bias when subjected to the same pair-wise comparison annotation framework used by human annotators. In conclusion, while ChatGPT's generated texts seem friendly and positive, they reflect the inherent nationality biases in the real world. This bias may vary across different language versions of ChatGPT, indicating diverse cultural perspectives. The study highlights the subtle and pervasive nature of biases within LLMs, emphasizing the need for further scrutiny.
Submission history
Access paper:.
- HTML (experimental)
- Other Formats
References & Citations
- Google Scholar
- Semantic Scholar
BibTeX formatted citation

Bibliographic and Citation Tools
Code, data and media associated with this article, recommenders and search tools.
- Institution
arXivLabs: experimental projects with community collaborators
arXivLabs is a framework that allows collaborators to develop and share new arXiv features directly on our website.
Both individuals and organizations that work with arXivLabs have embraced and accepted our values of openness, community, excellence, and user data privacy. arXiv is committed to these values and only works with partners that adhere to them.
Have an idea for a project that will add value for arXiv's community? Learn more about arXivLabs .
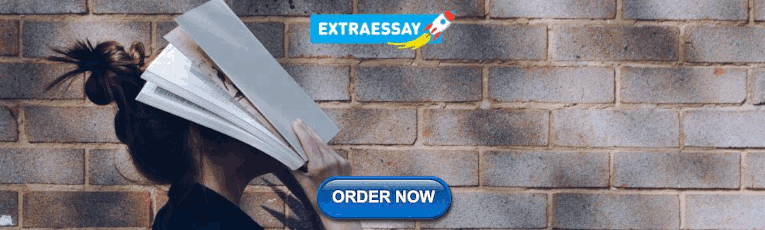
IMAGES
VIDEO
COMMENTS
Results. All studies found at least one cognitive bias or personality trait to affect physicians. Overconfidence, lower tolerance to risk, the anchoring effect, and information and availability biases were associated with diagnostic inaccuracies in 36.5 to 77 % of case-scenarios.
The abstracts selected were reviewed according to two inclusion criteria: (1) the article had a clear focus on cognitive biases and decision-making (e.g., not on implicit biases); (2) the article reported a review (narrative or systematic) on the topic or a representative empirical study.
Part of the Cognitive Case Study Series from Cabrera Research Lab, this case explores reality bias, the role of metacognition in reducing it, and the foundational role of reality bias in creating all other cognitive biases. A "cognitive case study"—inspired by the cases used in business and policy schools that involve students in real ...
However, the same glossing over of factors that makes heuristics a convenient and quick solution for many smaller issues means that they actually hinder the making of decisions about more complicated issues (Tversky & Kahneman, 1982). Heuristics are simplifications, and while simplifications use fewer cognitive resources, they also, well, simplify.
Katherine L. Milkman, and. John W. Payne. From the Magazine (May 2015) Artwork: Millo, 2014, B.ART-Arte in Barriera, Turin, Italy. Summary. When making decisions, we all rely too heavily on ...
The final list of biases used was the ambiguity effect 36, base rate fallacy 37, category size bias 38, extremeness aversion 39, disposition effect 40, temporal discounting 12, overplacement bias ...
For example, a diagnostic case study may have two versions specifically designed to test for a cognitive bias. That is, the way information is presented within the two versions, to two separate comparison groups of participants, may differ in a specific way (such as the ordering, framing or anchoring of the information).
Here follows an excerpt from "Lessons From Everest: The Interaction of Cognitive Bias, Psychological Safety, and System Complexity." Implications for leaders This multi-lens analysis of the Everest case provides a framework for understanding, diagnosing, and preventing serious failures in many types of organizations.
Cognitive biases can affect experts' judgments and decisions. In a recent article published in Law and Human Behavior, Tess M.S. Neal, Pascal Lienert, Emily Denne, and Jay P. Singh provided a broad descriptive model of how bias affects human judgment along two continuous dimensions: depth of cognitive processing and susceptibility to bias.More intuitive, low-effort cognitive processes can ...
Scope of Available Research and Methodology. Cognitive biases in forensic science have received the most attention from researchers to date (for a review of these forensic science studies, see Cooper & Meterko 2019).The second most substantial amount of scholarship focused on case evaluation (i.e., integrating and drawing conclusions based on the totality of the evidence in a case).
The practice of reflection reinforces behaviors that reduce bias in complex situations. Simply increasing physicians' familiarity with the many types of cognitive biases—and how to avoid them—may be one of the best strategies to decrease bias-related errors. 1 Thus, education for medical students, residents, and fellows could fruitfully ...
Objective: Cognitive and affective biases are essentially connected to heuristic shortcuts in thinking. These biases ordinarily function outside of conscious awareness and potentially affect clinical assessment, reasoning, and decision making in general medicine. However, little consideration has been given to how they may affect clinicians in the conduct of psychotherapy. This article aims to ...
Cognitive biases can be generally described as systematic, universally occurring, tendencies, inclinations, or dispositions in human decision making that may make it vulnerable for inaccurate, suboptimal, or wrong outcomes (e.g., Tversky and Kahneman, 1974; Kahneman, 2011; Korteling and Toet, 2022 ).
The Cognitive Bias Codex is a handy visual tool that organizes biases in a meaningful way; however, it is worth pointing out that the codex lists heuristics and biases both as 'biases.' If you decide to rely on the Cognitive Bias Codex, then keep in mind the distinction between heuristics and biases mentioned above. 4 Examples of Cognitive ...
As an example of why cognitive bias matters in the case of data-driven decision making, consider 'recency bias' and big data. ... (BI) systems can mitigate the effect of one cognitive bias, the anchoring effect. The study is based on the behavioral economics of decision making and involves a laboratory experiment. The results show that BI ...
Confirmation bias, hindsight bias, mere exposure effect, self-serving bias, base rate fallacy, anchoring bias, availability bias, the framing effect , inattentional blindness, and the ecological fallacy are some of the most common examples of cognitive bias. Another example is the false consensus effect. Cognitive biases directly affect our ...
A case study in combating bias. May 11, 2017 | Interview. ... What became obvious is that we had fallen victim to a number of cognitive biases in combination. We could see that status quo and confirmation biases had led us to assume the world would always be what it used to be. Beyond that, we neglected to heed the wisdom of portfolio theory ...
What is much more helpful is making sure that people are strongly emotionally motivated to address cognitive biases. Our emotions determine 80-90 percent of our decisions, thoughts, and behaviors ...
The Misinformation Effect. The Actor-Observer Bias. The False Consensus Effect. The Halo Effect. The Self-Serving Bias. The Availability Heuristic. The Optimism Bias. Other Kinds. Although we like to believe that we're rational and logical, the fact is that we are continually under the influence of cognitive biases.
An Introduction to Cognitive Bias. The word bias means a prejudice in favor or against a specific person, place, or thing. When we speak of cognitive bias, we are talking more specifically about a ...
A cognitive bias is a systematic pattern of deviation from norm or ... The study of cognitive biases has practical implications for areas including clinical judgment, entrepreneurship, finance, and management. ... tendency to judge the frequency or likelihood" of an occurrence by the extent of which the event "resembles the typical case." The ...
Clinical Case Reports aims to improve global health outcomes by sharing clinical knowledge through the ... Data suggests that cognitive biases affect all healthcare workers. 14 ... Another study showed that an implicit bias towards Hispanic and Black patient populations was a notably decreased perception of patient pain. 15 It is possible ...
This was evident within this case study as the initial focus was on the fact that the patient frequently presented in this way, rather than on considering alternative differentials to determine a diagnosis. A systematic review by Saposniik et al (2016) found that clinical decision-making is influenced by at least one cognitive bias. Anchoring ...
Growth.Design's list of cognitive biases and psychological principles is a great reference for any team committed to improving their customers' user experience. Dan & Louis-Xavier's comic book case studies show you how."— Nir Eyal, bestselling author of Hooked and Indistractable
To further combat cognitive biases, diversify your thinking by exposing yourself to different perspectives and methodologies. This could mean collaborating with professionals from various ...
Here we describe a 20-year arc of research to demonstrate the problem-solving utility of an evolutionary perspective by focusing, as a case study, on a particular cognitive bias - the endowment effect - that has implications for law.
1.2.Stress and depression. Most cognitive theories of depression propose diathesis-stress hypotheses that posit a link between a psychological vulnerability (e.g., certain cognitions or particular ways of processing information) and precipitating stressors (e.g., a negative life event or some other environmental factor) that together trigger the onset of depression (Gotlib and Joormann 2010, 286).
A researcher can bias a study by ... covariate reduced heterogeneity in outcomes. However, Tolin (Citation 2010), using a similar methodology to investigate studies of cognitive behavioural ... Gaffan, E. A., Tsaousis, J., & Kemp-Wheeler, S. M. (1995). Researcher allegiance and meta-analysis: The case of cognitive therapy for depression. ...
What influences the magnitude of TPP. Previous studies have demonstrated that the magnitude of the third-person perception is influenced by a host of factors including efficacy beliefs [] and cultural differences in self-construal [22, 23].Self-construal is defined as "a constellation of thoughts, feelings, and actions concerning the relationship of the self to others, and the self as ...
While nationality is a pivotal demographic element that enhances the performance of language models, it has received far less scrutiny regarding inherent biases. This study investigates nationality bias in ChatGPT (GPT-3.5), a large language model (LLM) designed for text generation. The research covers 195 countries, 4 temperature settings, and 3 distinct prompt types, generating 4,680 ...