Non-specific nature of specific language impairment: a review of the literature with regard to concomitant motor impairments
Affiliation.
- 1 Department of Experimental Psychology, University of Cambridge, UK. [email protected]
- PMID: 11344592
- DOI: 10.1080/13682820010019874
In the light of emerging suggestions that language and motor deficits may co-occur, the literature on specific language impairment (SLI) was reviewed to investigate the prevalence of co-morbidity between SLI and poor limb motor skill in children diagnosed with language impairments. An extensive literature search was undertaken and the subsequent findings evaluated with particular reference to issues surrounding symptom co-occurrence, as well as to theoretical and aetiological accounts of SLI. Clearly substantial co-morbidity exists between SLI and poor motor skill, suggesting that SLI is not a specific disorder of language, but rather that children with SLI experience a broader range of difficulties, of which motor incoordination is one. Current theoretical explanations of SLI do not account fully for such wide-ranging difficulties and it may be useful in the future to focus on a more detailed explanation in terms of shared cognitive processes or neuromaturational delay to understand further the nature of the disorder, to explain it theoretically and to deal with it practically.
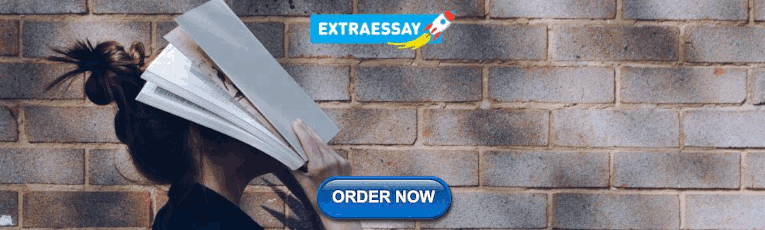
Publication types
- Case-Control Studies
- Child, Preschool
- Cognition / physiology
- Language Development Disorders / classification
- Language Development Disorders / complications*
- Language Development Disorders / physiopathology
- Motor Skills Disorders / classification
- Motor Skills Disorders / complications*
- Motor Skills Disorders / physiopathology
- Reaction Time / physiology
- Submission of manuscripts
- About the journal
- Editorial Board
- Instructions to authors
- Text (English)
- Texto (Spanish)
- Download PDF (English)
- Download PDF (Spanish)
- StambleUpon
Grammar intervention in children with specific language impairment: an integrative literature review
to review the literature reporting grammar-based interventions designed to improve morphosyntactic skills among children with specific language impairment (SLI).
several studies reporting grammar-based interventions were analyzed. The criteria for selecting the articles were determined as follows: a) publication date within the last ten years; b) studies that reported a grammar-based intervention; c) groups of study constituted by children with SLI, aged 3.0 to 12.0 years; d) design including Pre and Post measures; e) articles reporting quantitative/qualitative data analyses. The databases selected for this review were: Lilacs, PubMed, Embase, Scopus, ISI-Web of Science, and EBSCOhost.
most studies reported interventions focused on expressive grammar, providing no particular details about the specific grammar contents considered. Interventions usually consisted of implicit approaches implemented as individual therapy. Studies reported children with SLI as generally improving on intervened skills.
Conclusion:
all of the grammar-based intervention programs described in the selected studies, seemed to be equally adequate when working with children with SLI.
Keywords: Child Language; Language Disorders; Language Therapy; Speech, Language and Hearing Sciences
revisar la literatura que aborda la intervención gramatical destinada a incrementar las habilidades morfosintácticas en niños con TEL.
se analizaron diferentes artículos que exponían el tema de la intervención gramatical en niños con TEL. Los criterios de selección fueron: a) ser publicados en los últimos 10 años, b) exponer la aplicación de un programa gramatical, c) contar con un grupo de estudio constituido por niños con TEL, cuyas edades fluctuaran entre 3.0 y 12.0 años, d) considerar evaluaciones previas y posteriores a la aplicación y e) informar sobre los análisis cualitativo o cuantitativo utilizados para la obtención de los resultados. Las bases de datos utilizadas fueron: Lilacs, Pubmed, Embase, Scopus, ISI-Web of Science y EBSCOhost.
Resultados:
la mayoría de las investigaciones muestran una tendencia a trabajar la gramática expresiva, sin definir contenidos específicos. Las aplicaciones propenden a usar un enfoque implícito en un modo de terapia individual. Los estudios señalan que los niños con TEL tienden a beneficiarse de la intervención implementada.
Conclusión:
los trabajos analizados no permiten identificar un tipo de intervención gramatical que sea más apropiado para los niños con TEL.
Descriptor: Lenguaje Infantil; Trastornos del Lenguaje; Terapia del Lenguaje; Fonoaudiología
Introduction
Specific Language Impairment (SLI) is generally defined as a disorder of the linguistic development, which occurs in the absence of evident neurological damage, hearing deficit, severe environmental deprivation or mental retardation 1 1. Ullman M, Pierpont E. Specific language impairment is not specific to language: the procedural deficit hypothesis. Cortex. 2005;41(3):399-433. . It has been estimated that this disorder affects approximately 7% of children 2 2. Tomblin J, Records N, Buckwalter P, Zhang X, Smith E, O'Brien M. Prevalence of specific language impairment in kindergarten children. J Speech Lang Hear Res. 1997;40(6):1245-60. , 3 3. Leonard LB. Children with specific language impairment. 1°ed. Cambridge (MA): MIT Press;1998. and that it persists until adolescence 4 4. Stothard S, Snowling M, Bishop D, Chipchase B, Kaplan C. Language-impaired preschoolers. J Speech Lang Hear Res. 1998;41(2):407-18. , 5 5. Johnson C, Beitchman J, Young A, Escobar M, Atkinson L, Wilson B et al. Fourteen-year follow-up of children with and without speech/language impairments. J Speech Lang Hear Res. 1999;42(3):744-60. .
One of the most outstanding linguistic characteristics in children with SLI is their grammatical problems 6 6. Aguado G. Apuntes acerca de la investigación sobre el TEL. Rev Logop Foniatr Audiol. 2007;27(3):103-9. . These are generally considered one of the nuclear symptoms of this disorder 7 7. Leonard L. Specific language impairment across languages. In: Bishop B, Leonard L (orgs). In speech and language impairments in children. New York: Psychology Press;2014. p.115-30. . In addition, there are children with SLI who are characterized by only demonstrating difficulties in grammatical aspects 8 8. De Anda S, Blossom M, Abel A. A complexity approach to treatment of tense and agreement deficits: a case study. Commun Disord Q. 2019:1-11. .
Grammar difficulties occur both at an expressive and comprehensive level. In this regard, it has been observed that expressive morphosyntactic disturbances are more evident in young children (up to 8 years) and that receptive errors are expressed even in adolescence 9 9. Rice M, Hoffman L, Wexler K. Judgments of omitted BE and DO in questions as extended finiteness clinical markers of Specific Language Impairment (SLI) to 15 years: a study of growth and asymptote. J Speech Lang Hear Res. 2009;52(6):1417-33. . Although grammatical errors are a transversal symptom, difficulties may manifest differently in speakers of different languages 1 1. Ullman M, Pierpont E. Specific language impairment is not specific to language: the procedural deficit hypothesis. Cortex. 2005;41(3):399-433. , 10 10. Leonard L. Some reflections on the study of children with specific language impairment. Child Lang Teach Ther. 2009;25(2):169-71. . For example, English-speaking children show mainly a compromise in the morphology of verbs, in particular related to the use of third person singular and to the morphemes of the past tense of regular verbs 11 11. Norbury C, Bishop D, Briscoe J. Production of English finite verb morphology. J Speech Lang Hear Res. 2001;44(1):165-78. . On the other hand, Spanish speakers, in addition to their difficulties with verbs, exhibit difficulties with the use of articles, clitic pronouns and prepositions 12 12. Anderson R, Souto S. The use of articles by monolingual Puerto Rican Spanish-speaking children with specific language impairment. Appl Psycholinguist. 2005;26(04):621-47. 13. Bedore L, Leonard L. Verb inflections and noun phrase morphology in the spontaneous speech of Spanish-speaking children with specific language impairment. Appl Psycholinguist. 2005;26(02):195-225. - 14 14. Morgan G, Restrepo M, Auza A. Comparison of Spanish morphology in monolingual and Spanish-English bilingual children with and without language impairment. Biling Lang Cogn. 2013;16(03):578-96. .
The Procedural Deficit Hypothesis is one of the explanations proposed to understand the grammatical difficulties of children with SLI. This hypothesis states that grammar involves the learning of rules, which requires the use of procedural memory. Instead, lexical knowledge depends on declarative memory. In children with SLI, procedural memory would be compromised and therefore grammatical areas would be affected. In contrast, declarative memory would be unscathed 1 1. Ullman M, Pierpont E. Specific language impairment is not specific to language: the procedural deficit hypothesis. Cortex. 2005;41(3):399-433. .
The relevance of grammatical difficulties in children with SLI requires developing interventions that contribute to overcome or compensate this problem.
Kamhi 15 15. Kamhi A. Improving clinical practices for children with language and learning disorders. Lang Speech Hear Ser. 2014;45(2):92-103. states that in therapy, there can be a differentiation between the approaches (referring to the how) and the contents (referring to the what) that should be used. Regarding the approaches, Ebbels 16 16. Ebbels S. Effectiveness of intervention for grammar in school-aged children with primary language impairments: a review of the evidence. Child Lang Teach Ther. 2014;30(1):7-40. distinguishes two types: implicit and explicit. Implicit approaches address the teaching of grammatical aspects through procedures such as reformulation, imitation, expansion or modeling. This perspective seeks that children are able to infer morphosyntactic rules without being explicitly instructed. From this approach, it has been found that the use of reformulation is appropriate for children with SLI when programs with specific grammatical objectives are applied 17 17. Cleave P, Becker S, Curran M, Owen A, Fey M. The efficacy of recasts in language intervention: A systematic review and meta-analysis. Am J Speech Lang Pathol. 2015;24(2):237-55. .
In turn, explicit approaches directly teach the rules of grammar, through procedures that involve metalinguistic reflection, which is generally based on visual clues 16 16. Ebbels S. Effectiveness of intervention for grammar in school-aged children with primary language impairments: a review of the evidence. Child Lang Teach Ther. 2014;30(1):7-40. . It is argued that visual clues are useful to support the teaching of grammar, because children with SLI have strong visual skills 18 18. Ebbels S. Teaching grammar to school-aged children with specific language impairment using Shape Coding. Child Lang Teach Ther. 2007;23(1):67-93. .
According to Kamhi 15 15. Kamhi A. Improving clinical practices for children with language and learning disorders. Lang Speech Hear Ser. 2014;45(2):92-103. , the approaches just described correspond to the same intervention model that varies in intensity and specificity. Thus, at one end of the therapy specter would be the explicit approach in which specific grammatical structures are explicitly taught through metalinguistic reflection. At the other extreme would be the implicit approach where, through procedures such as modeling or reformulation, these structures are learned, without direct instruction.
Regarding which approach is best for children with SLI, it seems that the explicit ones would be more appropriate. This is because these children show difficulties with implicit grammatical learning, due to their issues in procedural memory 1 1. Ullman M, Pierpont E. Specific language impairment is not specific to language: the procedural deficit hypothesis. Cortex. 2005;41(3):399-433. , 16 16. Ebbels S. Effectiveness of intervention for grammar in school-aged children with primary language impairments: a review of the evidence. Child Lang Teach Ther. 2014;30(1):7-40. . On the other hand, declarative memory, based on explicit learning, would not be affected 1 1. Ullman M, Pierpont E. Specific language impairment is not specific to language: the procedural deficit hypothesis. Cortex. 2005;41(3):399-433. , 16 16. Ebbels S. Effectiveness of intervention for grammar in school-aged children with primary language impairments: a review of the evidence. Child Lang Teach Ther. 2014;30(1):7-40. .
Also, it has been proposed that at different stages and ages children respond differently to each of these approaches. Thus, implicit approaches would be more suitable for young children 16 16. Ebbels S. Effectiveness of intervention for grammar in school-aged children with primary language impairments: a review of the evidence. Child Lang Teach Ther. 2014;30(1):7-40. , while explicit ones are more appropriate for older children 18 18. Ebbels S. Teaching grammar to school-aged children with specific language impairment using Shape Coding. Child Lang Teach Ther. 2007;23(1):67-93. . The previous statement is consistent with a study in which speech therapists reported that the most used procedures vary according to the school period in which the child is. Thus, in pre-school stage, modeling is the most used procedure, followed by reformulation and imitation (implicit approach). In the school stage, modeling is practically not used and the most common procedure is explicit teaching (explicit approach) 19 19. Finestack L, Satterlund K. Current practice of child grammar intervention: a survey of speech-language pathologists. Am J Speech Lang Pathol. 2018;27(4):1329-51. .
Finally, it is important to point out that implicit approaches fundamentally help children with morphosyntactic expression difficulties 15 15. Kamhi A. Improving clinical practices for children with language and learning disorders. Lang Speech Hear Ser. 2014;45(2):92-103. . On the other hand, explicit approaches support mainly schoolchildren with receptive grammatical difficulties 16 16. Ebbels S. Effectiveness of intervention for grammar in school-aged children with primary language impairments: a review of the evidence. Child Lang Teach Ther. 2014;30(1):7-40. .
As previously stated, in addition to the approaches that are used in an intervention, there are grammatical contents that must be addressed 15 15. Kamhi A. Improving clinical practices for children with language and learning disorders. Lang Speech Hear Ser. 2014;45(2):92-103. . The establishment of these contents should consider the language spoken by the child, since grammatical difficulties manifest differently in each language 1 1. Ullman M, Pierpont E. Specific language impairment is not specific to language: the procedural deficit hypothesis. Cortex. 2005;41(3):399-433. , 10 10. Leonard L. Some reflections on the study of children with specific language impairment. Child Lang Teach Ther. 2009;25(2):169-71. . In English, the contents most commonly addressed in the pre-school stage, according to language therapists, are plural form and verbs in present progressive. In the school period the contents most usually applied are regular and irregular verbs in the past tense, pronouns and plurals 19 19. Finestack L, Satterlund K. Current practice of child grammar intervention: a survey of speech-language pathologists. Am J Speech Lang Pathol. 2018;27(4):1329-51. .
Another topic that has been discussed regarding grammatical intervention in children with SLI is the duration that the session should have for the treatment to be beneficial. Recent work shows that there are no differences between sessions that last fifteen minutes and the ones that last thirty minutes. Consequently, it is suggested to prefer a shorter session 20 20. Plante E, Mettler H, Tucci A, Vance R. Maximizing treatment efficiency in developmental language disorder: positive effects in half the time. Am J Speech Lang Pathol. 2019;28(3):1233-47. .
Despite the importance of treating grammatical difficulties in children with SLI, it is noteworthy that there are significant gaps in the literature on the effectiveness and efficiency of the intervention, both in children with receptive grammatical problems and those with expressive grammatical difficulties 16 16. Ebbels S. Effectiveness of intervention for grammar in school-aged children with primary language impairments: a review of the evidence. Child Lang Teach Ther. 2014;30(1):7-40. .
Thus, the question of how the intervention used to support grammatical development in children with SLI is, turns out to be relevant and valid. Therefore, the objective of this paper is to review the literature that addresses grammar intervention aimed at increasing morphosyntactic abilities in children with SLI.
Literature Search Procedures
An integrative review of the literature was carried out between August and October of 2018. For this review, two procedures were used.
The first one consisted in researching the following databases: Lilacs, PubMed, Embase, Scopus, ISI-Web of Science and EBSCOhost. These were chosen because they address issues related to therapeutic intervention, grammar and SLI. Thus, some focus primarily on biological and medical sciences and others address content both related to philosophy and humanities. The cross-reference between databases that address medical and biological contents and the ones that address philosophy and humanities allowed to find the articles analyzed in this review.
The terms used for the search were traced in English and Spanish. English was chosen because it is the language in which most scientific works are disseminated. As a matter of fact, abstracts of articles published in any language count with an English translation. In addition to the aforementioned, it is known that evidence on grammar in children with SLI has been obtained mainly from studies conducted with English speakers 21 21. Leonard L, Kueser J. Five overarching factors central to grammatical learning and treatment in children with developmental language disorder. Int J Lang Commun Disord. 2019;54(3):341-61. . On the other hand, Spanish was privileged as it is the most spoken Romance language in the world 22 22. Zambrana JR. La situación actual de la lengua española en el mundo. [septiembre 2009]. Disponible en http://www.eumed.net/rev/cccss/05/jrz.htm http://www.eumed.net/rev/cccss/05/jrz.ht... . Along with the above, most journals that address the issue of language difficulties in Latin America and Spain disseminate their articles in Spanish. finally, Brazilian magazines disseminate articles in Portuguese and English and some of them accept works in Spanish.
The terms used in English were: "classroom and grammar and intervention", "grammar and intervention", "intervention and grammar and SLI", "classroom and children and SLI and grammar", "grammatical and treatment and SLI". In turn, the words used in Spanish were: “sala de clase y gramática e intervención” “gramática e intervención”, “intervención y gramática y TEL”, “sala de clase y niños y TEL y gramática” and “gramática y tratamiento y TEL”. The terms “intervention / intervención” and “treatment / tratamiento” were used because they involve the idea of therapy, support plans, programs and training. On the other hand, the words "grammar / gramática" and "grammatical / gramatical" were used since they include the concepts of morphology and syntax. The descriptor “classroom / sala de clases” was included, because when using only the descriptors “grammar / gramática”, “intervention / intervención” and “SLI / TEL” very few articles were found. Thus, when considering it within the search, more studies emerged.
All descriptors were examined without limiting the search, with the exception of Scopus database, in which the search for all terms was limited to the option of “title, abstract and keywords”, because when considering all fields, many articles appeared which did not relate to the topic.
For the rest of the databases, there was only restriction of the terms “Grammar and intervention”. In the case of PubMed and Embase, the search was limited to the title and the abstract simultaneously. In ISI, Lilacs and Ebscohost, which did not present the simultaneity option, the exploration was restricted to the title. This is because when these terms were not limited, the number of articles without relation to the topic increased considerably.
The second procedure consisted in searching for articles from the ones already selected in the mentioned databases. Specifically, the references found in those articles were placed in the Google Scholar search engine. All those that appeared in the Related Articles section were reviewed and those that met the inclusion criteria were selected.
Inclusion Criteria for Articles
Articles must contain original data.
Articles should report on applications of grammar intervention programs exclusively for children with language difficulties.
Articles should have been published in the last 10 years, that is, between 2008 and 2018.
The research design should include: a) study group, b) evaluation pre and post program implementation and c) quantitative or qualitative analysis of the data.
The intervention must be carried out in preschoolers and / or in schoolchildren with SLI.
The age range of children with SLI should fluctuate between 3.0 years and 12.0 years.
Accepted Articles and Rejected Articles
The databases showed 1,171 articles and 4 articles were found through the Google Scholar search engine, and 1,057 articles were excluded due to their titles, 73 for being duplicated, 15 after the revision of the abstract and 7 after reading the complete text. Finally, there were 23 articles left which met the inclusion criteria, consequently they were analyzed in their full version.
Image 1 summarizes the search process performed for the selection of articles.
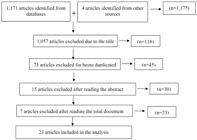
It should be noted that when the descriptors were tracked in Spanish, no results were obtained in any database. The only exception was EBSCOhost which when using the terms “sala de clases y niños y TEL y gramática” showed 10 articles. None were considered into the analysis, because they did not meet the inclusion criteria. Due to the aforementioned, the tables presented contain information on the articles that correspond to the search performed with the terms in English.
Table 1 shows the total number of articles found (number on the left) and the total number of articles selected (number on the right). Table 2 shows the distribution of the selected articles by their source. The numbers correspond to the ones under which they are found in the references section.
Review of Literature
Tables 3 and 4 can be found below, which summarize the most important information from the selected articles. The complete bibliographic information of the articles analyzed is in the references section and they are marked with an asterisk.
Table 3 shows the objectives / hypotheses, the participants to whom the grammatical intervention was applied and the language spoken by the children.
The data in Table 3 indicate that 83% of the researches are carried out in English-speaking children. This implies a limitation because the results cannot always be extrapolated to children who speak other languages, since the grammatical difficulties in children with SLI manifest differently in each language 10 10. Leonard L. Some reflections on the study of children with specific language impairment. Child Lang Teach Ther. 2009;25(2):169-71. . This is consistent with what has been previously stated by Leonard 21 21. Leonard L, Kueser J. Five overarching factors central to grammatical learning and treatment in children with developmental language disorder. Int J Lang Commun Disord. 2019;54(3):341-61. , regarding the fact that the greatest amount of information about grammar comprehension in children with SLI has been obtained from studies with English speakers.
Another noteworthy aspect is that 87% of the interventions focus on supporting expressive grammar. These findings agree with what Ebbels’ 16 16. Ebbels S. Effectiveness of intervention for grammar in school-aged children with primary language impairments: a review of the evidence. Child Lang Teach Ther. 2014;30(1):7-40. proposes, which indicates that there are persistent gaps in the intervention, in particular in treatments aimed at receptive aspects. It is important to note that 3 investigations (in which the therapies were not focused on grammatical comprehension) showed that children also benefited at a comprehensive level. Thus, the work of Calder et al. 42 42. Calder S, Claessen M, Leitão S. Combining implicit and explicit intervention approaches to target grammar in young children with developmental language disorder. Child Lang Teach Ther. 2017;34(2):171-89. , which uses combined approaches, Zwitserlood et al 40 40. Zwitserlood R, Wijnen F, van Weerdenburg M, Verhoeven L. 'MetaTaal': enhancing complex syntax in children with specific language impairment-a metalinguistic and multimodal approach. Int J Lang Commun Disord. 2015;50(3):273-97. , which uses the metalinguistic approach and Camarata et al. 26 26. Camarata S, Nelson K, Gillum H, Camarata M. Incidental receptive language growth associated with expressive grammar intervention in SLI. First Lang. 2009;29(1):51-63. that uses implicit approaches to work expressive grammar, indicated that children were favored both expressively and comprehensively. These results suggest that the approach to grammatical comprehension can be performed indirectly, in particular the explicit or metalinguistic approach seems to be useful for receptive grammatical difficulties. Ebbels 16 16. Ebbels S. Effectiveness of intervention for grammar in school-aged children with primary language impairments: a review of the evidence. Child Lang Teach Ther. 2014;30(1):7-40. also agrees that explicit approaches are appropriate to support students with receptive grammatical difficulties.
In addition, it can be observed that the age ranges are different in the studies. Thus, it is appreciated that 78% of the participants are preschoolers whose ages range between 2 and 6 years old. Moreover, in 26% of the studies the subjects are under 5 years old, which is controversial since before this age the diagnosis of SLI is debatable. This is because it is possible to confuse this diagnosis with a language delay. This may impact the results of the investigation, given that children with language delay generally progress faster than children with SLI when an intervention program is applied 45 45. Ramírez-Santana G, Acosta-Rodríguez V, Moreno-Santana A, del Valle-Hernández N, Axpe-Caballero Á. El uso combinado de narraciones orales y actividades morfosintácticas para mejorar habilidades gramaticales de alumnado con trastorno específico del lenguaje (TEL). Revista de Psicodidáctica. 2018;23(1):48-55. .
Regarding the type of studies, it is observed that 83% of them compare independent samples, that is, they contrast groups that receive different types of interventions or groups with different diagnosis (SLI and TD) to which the same intervention is applied.
Table 4 shows the characteristics of the treatment and the conclusions obtained after the intervention.
The characteristics of the treatment were analyzed using the following categories: modality (individual and group), dose (number of sessions, session length and duration of treatment), approach and intervention techniques or procedures. The elements referred to the dose are relevant to specify the nature of the treatment 16 16. Ebbels S. Effectiveness of intervention for grammar in school-aged children with primary language impairments: a review of the evidence. Child Lang Teach Ther. 2014;30(1):7-40. . Finally, it is important to note that the approach was inferred from information about the techniques or procedures of the treatment.
Table 4 shows that 60% of the studies are carried out individually. This suggests that there is some consensus that individual therapy is more appropriate for children with SLI than group therapy. This may be due to the fact that the persistence and severity of this disorder would require focused support for these children. This result differs from a study 46 46. Eidsvåg S, Plante E, Oglivie T, Privette CH, Mailend M. Individual versus small group treatment of morphological errors for children with developmental language disorder. Lang Speech Hear Ser. 2019;50:237-52. in which it was found that the individual modality shows a similar effect as group therapy. It should be noted that in the mentioned study each subject had their own therapeutic goal. Consequently, the results, rather than favoring a treatment modality, indicate the importance of the intervention being specific and directed to a particular grammatical aspect.
Regarding the frequency and duration of the sessions, it is noted that 65% of the studies perform between 1 and 2 sessions per week and their duration is variable, fluctuating between 5 minutes and 1 hour. It is noteworthy that the highest percentage was 39%, which corresponds to studies that carried out therapy sessions of 30 minutes.
In relation to the length of the treatment, the data shows that the duration ranges from 1 week to 8 months. However, there is a tendency to execute treatments that last between 10 and 12 weeks. It is important to note that the Ramírez-Santana 45 45. Ramírez-Santana G, Acosta-Rodríguez V, Moreno-Santana A, del Valle-Hernández N, Axpe-Caballero Á. El uso combinado de narraciones orales y actividades morfosintácticas para mejorar habilidades gramaticales de alumnado con trastorno específico del lenguaje (TEL). Revista de Psicodidáctica. 2018;23(1):48-55. study implements the treatment for more than 1 year.
Despite the importance of evaluating the dose (number and duration of sessions / length of treatment), it was found that only the study by Smith-Lock et al 35 35. Smith-Lock K, Leitão S, Lambert L, Prior P, Dunn A, Cronje J et al. Daily or weekly? The role of treatment frequency in the effectiveness of grammar treatment for children with specific language impairment. Int J Speech Lang Pathol. 2013;15 (3):255-67. analyzes some aspects of it. The purpose of this study was to determine which dose was most effective. Their results showed that weekly therapy, carried out for 8 weeks, was more effective than daily treatment. The findings of this investigation are consistent with what was raised by Kamhi 15 15. Kamhi A. Improving clinical practices for children with language and learning disorders. Lang Speech Hear Ser. 2014;45(2):92-103. . This author mentions that a higher dose in therapy will not always lead to better results. It is suggested that progress may depend on other aspects such as the area to be supported and the level of development that the child manifests in that area.
This is also consistent with what is proposed by Proctor-Williams and Fey 47 47. Proctor-Williams K, Fey M. Recast density and acquisition of novel irregular past tense verbs. J Speech Lang Hear Res. 2007;50(4):1029-47. who express that therapy is effective when it provides varied and repeated learning opportunities that are distributed over a considerable period of time.
In relation to the approaches used, there is a tendency to perform the intervention with one type of approach. Thus, 60% of the studies were carried out with the implicit approach, 26% used therapies with the explicit or metalinguistic approach and 14% used both approaches. The results of the investigations showed that children in treatment, independent of the approach, show improvements in the areas that are stimulated. These findings are not in accord with the proposal by Ullman & Pierpont 1 1. Ullman M, Pierpont E. Specific language impairment is not specific to language: the procedural deficit hypothesis. Cortex. 2005;41(3):399-433. and Ebbels 16 16. Ebbels S. Effectiveness of intervention for grammar in school-aged children with primary language impairments: a review of the evidence. Child Lang Teach Ther. 2014;30(1):7-40. in which the metalinguistic approach would be better for children with SLI, since this approach is based on declarative memory, which is not compromised 1 1. Ullman M, Pierpont E. Specific language impairment is not specific to language: the procedural deficit hypothesis. Cortex. 2005;41(3):399-433. .
The predominance of treatments that use implicit approaches may be due to the age of the subjects who are supported with such treatments. Thus, 13 of the 14 studies in which the implicit approach is used work with participants who are under 6 years old. In contrast, of the 6 works in which the explicit approach is used, 5 were applied to children who were 6 years old or older. This relationship between age and type of approach is consistent with what is proposed by Ebbels 16 16. Ebbels S. Effectiveness of intervention for grammar in school-aged children with primary language impairments: a review of the evidence. Child Lang Teach Ther. 2014;30(1):7-40. , 18 18. Ebbels S. Teaching grammar to school-aged children with specific language impairment using Shape Coding. Child Lang Teach Ther. 2007;23(1):67-93. . The author indicates that implicit approaches are more suitable for younger children 16 16. Ebbels S. Effectiveness of intervention for grammar in school-aged children with primary language impairments: a review of the evidence. Child Lang Teach Ther. 2014;30(1):7-40. and explicit ones are more suitable for older children 18 18. Ebbels S. Teaching grammar to school-aged children with specific language impairment using Shape Coding. Child Lang Teach Ther. 2007;23(1):67-93. .
Finally, it is important to note that the studies analyzed do not show explicit criteria to determine the contents that will be applied in therapy. In general, syntactic structures are mentioned, but their selection is not substantiated. This indicates that this aspect of therapy is not a focus of attention when assessing the treatments.
The limitations of this review are related to the age of the subjects and one of the inclusion criteria. The age range to which the search was limited restricted the analysis of interventions for older children with SLI. In this regard, it is important to review treatments in older subjects, because the linguistic aspect of grammar acquires relevance when learning the written language. The inclusion criterion which considered studies exclusively with grammatical interventions, limited the analysis of treatments where the grammatical component works in conjunction with other linguistic aspects in children with SLI.
The review of the articles shows that in 10 years of studies analyzed, research on grammatical treatments in children with SLI is scarce, since only 23 articles were found using the established criteria. In addition, most of them have been done with English-speaking children. Regarding the modality and the dose, the treatments tend to be individual, predominantly with therapies of one or two weekly sessions of 30 minutes. The intervention period tends not to exceed 6 months. Concerning the approach, most treatments use the implicit therapy and the results indicate that children with SLI benefit from any type of approach. In regards to the intervention of expressive or comprehensive aspects, there is a prevalence of studies in which expressive grammar is intervened. Finally, aspects related to grammatical contents are not specified. This suggests that there is a gap regarding the criteria applied to select the grammatical contents that can be used to support the morphosyntactic difficulties in children with SLI.
- 1 Ullman M, Pierpont E. Specific language impairment is not specific to language: the procedural deficit hypothesis. Cortex. 2005;41(3):399-433.
- 2 Tomblin J, Records N, Buckwalter P, Zhang X, Smith E, O'Brien M. Prevalence of specific language impairment in kindergarten children. J Speech Lang Hear Res. 1997;40(6):1245-60.
- 3 Leonard LB. Children with specific language impairment. 1°ed. Cambridge (MA): MIT Press;1998.
- 4 Stothard S, Snowling M, Bishop D, Chipchase B, Kaplan C. Language-impaired preschoolers. J Speech Lang Hear Res. 1998;41(2):407-18.
- 5 Johnson C, Beitchman J, Young A, Escobar M, Atkinson L, Wilson B et al. Fourteen-year follow-up of children with and without speech/language impairments. J Speech Lang Hear Res. 1999;42(3):744-60.
- 6 Aguado G. Apuntes acerca de la investigación sobre el TEL. Rev Logop Foniatr Audiol. 2007;27(3):103-9.
- 7 Leonard L. Specific language impairment across languages. In: Bishop B, Leonard L (orgs). In speech and language impairments in children. New York: Psychology Press;2014. p.115-30.
- 8 De Anda S, Blossom M, Abel A. A complexity approach to treatment of tense and agreement deficits: a case study. Commun Disord Q. 2019:1-11.
- 9 Rice M, Hoffman L, Wexler K. Judgments of omitted BE and DO in questions as extended finiteness clinical markers of Specific Language Impairment (SLI) to 15 years: a study of growth and asymptote. J Speech Lang Hear Res. 2009;52(6):1417-33.
- 10 Leonard L. Some reflections on the study of children with specific language impairment. Child Lang Teach Ther. 2009;25(2):169-71.
- 11 Norbury C, Bishop D, Briscoe J. Production of English finite verb morphology. J Speech Lang Hear Res. 2001;44(1):165-78.
- 12 Anderson R, Souto S. The use of articles by monolingual Puerto Rican Spanish-speaking children with specific language impairment. Appl Psycholinguist. 2005;26(04):621-47.
- 13 Bedore L, Leonard L. Verb inflections and noun phrase morphology in the spontaneous speech of Spanish-speaking children with specific language impairment. Appl Psycholinguist. 2005;26(02):195-225.
- 14 Morgan G, Restrepo M, Auza A. Comparison of Spanish morphology in monolingual and Spanish-English bilingual children with and without language impairment. Biling Lang Cogn. 2013;16(03):578-96.
- 15 Kamhi A. Improving clinical practices for children with language and learning disorders. Lang Speech Hear Ser. 2014;45(2):92-103.
- 16 Ebbels S. Effectiveness of intervention for grammar in school-aged children with primary language impairments: a review of the evidence. Child Lang Teach Ther. 2014;30(1):7-40.
- 17 Cleave P, Becker S, Curran M, Owen A, Fey M. The efficacy of recasts in language intervention: A systematic review and meta-analysis. Am J Speech Lang Pathol. 2015;24(2):237-55.
- 18 Ebbels S. Teaching grammar to school-aged children with specific language impairment using Shape Coding. Child Lang Teach Ther. 2007;23(1):67-93.
- 19 Finestack L, Satterlund K. Current practice of child grammar intervention: a survey of speech-language pathologists. Am J Speech Lang Pathol. 2018;27(4):1329-51.
- 20 Plante E, Mettler H, Tucci A, Vance R. Maximizing treatment efficiency in developmental language disorder: positive effects in half the time. Am J Speech Lang Pathol. 2019;28(3):1233-47.
- 21 Leonard L, Kueser J. Five overarching factors central to grammatical learning and treatment in children with developmental language disorder. Int J Lang Commun Disord. 2019;54(3):341-61.
- 22 Zambrana JR. La situación actual de la lengua española en el mundo. [septiembre 2009]. Disponible en http://www.eumed.net/rev/cccss/05/jrz.htm » http://www.eumed.net/rev/cccss/05/jrz.htm
- 23 Leonard L, Camarata S, Pawlowska M, Brown B, Camarata M. The acquisition of tense and agreement morphemes by children with specific language impairment during intervention: phase 3. J Speech Lang Hear Res. 2008;51(1):120-5.
- 24 Leonard L, Haebig H, Deevy P, Brown B. Tracking the growth of tense and agreement in children with specific language impairment: differences between measures of accuracy, diversity, and productivity. J Speech Lang Hear Res. 2017;60:3590-600.
- 25 Motsch H, Riehemann S. Effects of 'Context-Optimization' on the acquisition of grammatical case in children with specific language impairment: an experimental evaluation in the classroom. Int J Lang Commun Disord. 2008;43(6):683-98.
- 26 Camarata S, Nelson K, Gillum H, Camarata M. Incidental receptive language growth associated with expressive grammar intervention in SLI. First Lang. 2009;29(1):51-63.
- 27 Yoder P, Molfese D, Gardner E. Initial mean length of utterance predicts the relative efficacy of two grammatical treatments in preschoolers with specific language impairment. J Speech Lang Hear Res. 2011;54(4):1170-81.
- 28 Yoder P, Molfese D, Murray M, Key A. Normative topographic ERP analyses of speed of speech processing and grammar before and after grammatical treatment. Dev Neuropsychol. 2013;38(8):514-33.
- 29 Gallego JL. La enseñanza de habilidades lingüísticas en morfosintaxis: evaluación de un programa de intervención. Investigación en la escuela. 2012;76:77-91.
- 30 Kulkarni A, Pring T, Ebbels S. Evaluating the effectiveness of therapy based around Shape Coding to develop the use of regular past tense morphemes in two children with language impairments. Child Lang Teach Ther. 2013;30(3):245-54.
- 31 Hoover J, Storkel H. Grammatical treatment and specific language impairment: Neighbourhood density & third person singular -s. Clin Linguist Phon. 2013;27(9):661-80.
- 32 Washington K. The association between expressive grammar intervention and social and emergent literacy outcomes for preschoolers with SLI. Am J Speech Lang Pathol. 2013;22(1):113-25.
- 33 Washington K, Warr-Leeper G. Growth in expressive grammar following intervention for 3- to 4-year-old preschoolers with SLI. JCPSLP. 2013;15(1):7-12.
- 34 Washington K, Warr-Leeper G. Visual support in intervention for preschoolers with specific language impairment. Top Lang Disord. 2013;33(4):347-65.
- 35 Smith-Lock K, Leitão S, Lambert L, Prior P, Dunn A, Cronje J et al. Daily or weekly? The role of treatment frequency in the effectiveness of grammar treatment for children with specific language impairment. Int J Speech Lang Pathol. 2013;15 (3):255-67.
- 36 Smith-Lock KM, Leitao S, Lambert L, Nickels L. Effective intervention for expressive grammar in children with specific language impairment. Int J Lang Commun Disord. 2013;48(3):265-82.
- 37 Smith-Lock KM, Leitão S, Prior P, Nickelsa L. The effectiveness of two grammar treatment procedures for children with SLI: a randomized clinical trial. Lang Speech Hear Ser. 2015;46(4):312-24.
- 38 Plante E, Ogilvie T, Vance R, Aguilar J, Dailey N, Meyers C et al. Variability in the language input to children enhances learning in a treatment context. Am J Speech Lang Pathol. 2014;23(4):530-46.
- 39 Hsu HJ, Bishop D. Training understanding of reversible sentences: a study comparing language-impaired children with age-matched and grammar-matched controls. Peer J. 2014;2:e656.
- 40 Zwitserlood R, Wijnen F, van Weerdenburg M, Verhoeven L. 'MetaTaal': enhancing complex syntax in children with specific language impairment-a metalinguistic and multimodal approach. Int J Lang Commun Disord. 2015;50(3):273-97.
- 41 Meyers-Denman Ch, Plante E. Dose schedule and enhanced conversational recast treatment for children with specific language impairment. Lang Speech Hear Ser. 2016;47:334-46.
- 42 Calder S, Claessen M, Leitão S. Combining implicit and explicit intervention approaches to target grammar in young children with developmental language disorder. Child Lang Teach Ther. 2017;34(2):171-89.
- 43 Owen A, Fey M, Curran M. Do the hard things first: a randomized controlled trial testing the effects of exemplar selection on generalization following therapy for grammatical morphology. J Speech Lang Hear Res. 2017;60(9):2569-88.
- 44 Owen A, Curran M, Larson C, Fey M. Effects of a complexity-based approach on generalization of past tense -ed and related morphemes. Lang Speech Hear Ser. 2018;49:681-93.
- 45 Ramírez-Santana G, Acosta-Rodríguez V, Moreno-Santana A, del Valle-Hernández N, Axpe-Caballero Á. El uso combinado de narraciones orales y actividades morfosintácticas para mejorar habilidades gramaticales de alumnado con trastorno específico del lenguaje (TEL). Revista de Psicodidáctica. 2018;23(1):48-55.
- 46 Eidsvåg S, Plante E, Oglivie T, Privette CH, Mailend M. Individual versus small group treatment of morphological errors for children with developmental language disorder. Lang Speech Hear Ser. 2019;50:237-52.
- 47 Proctor-Williams K, Fey M. Recast density and acquisition of novel irregular past tense verbs. J Speech Lang Hear Res. 2007;50(4):1029-47.
- Research support Source: Regular FONDECYT nº1170705 from Comisión Nacional de Investigación y Tecnología (CONICYT); Basal Fund for Centers of Excellence, project FB0003 from PIA-CONICYT.
Publication Dates
- Publication in this collection 25 Nov 2019
- Date of issue 2019
- Received 30 May 2019
- Accepted 07 Oct 2019

- https://orcid.org/0000-0002-9116-0450
- https://orcid.org/0000-0002-3954-8327
- https://orcid.org/0000-0001-6449-7712
- Corresponding address: Carmen Julia Coloma Tirapegui, Avenida Independencia 1027, Independencia, Postal Code: 8380453 - Santiago, Chile, E-mail: [email protected]
- Conflict of interests: Nonexistent
SCIMAGO INSTITUTIONS RANKINGS
Figures | tables.
- Figures (1)
Figure 1: Flowchart for article selection
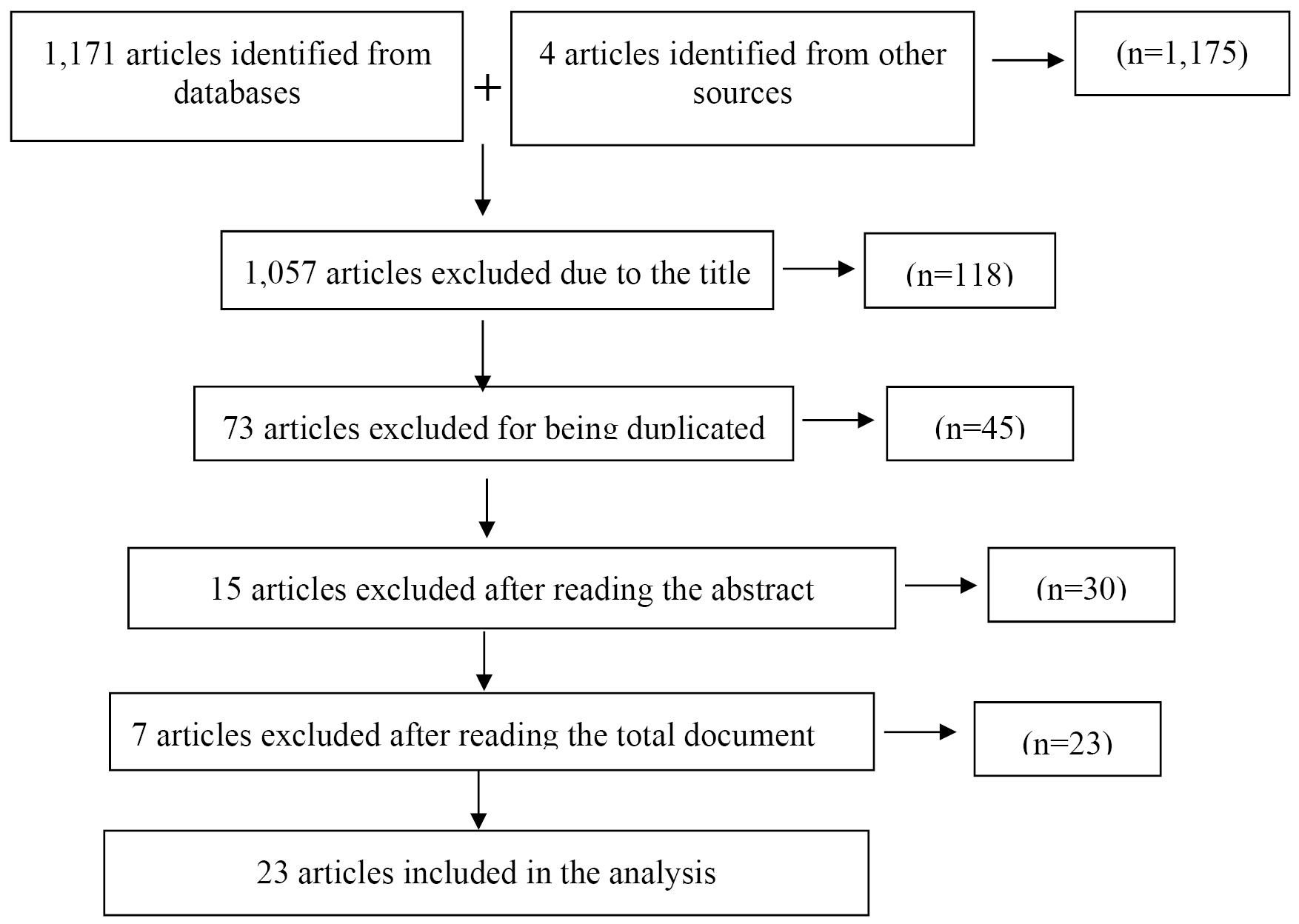
Table 1: Quantity and distribution of the articles found and selected, according to descriptor and database used
- Legend: SLI (specific language impairment)
Table 2: Distribution of the selected articles, according to descriptor and source
Table 3: objectives/hypotheses, participants and language.
- Legend: SLI (specific language impairment) / ASD (autistic spectrum disorder) /MLT ( milieu language teaching ) /BTR ( broad target recasts ) / CAI ( Computer-assisted intervention) / TTI (Table-top intervention)
Table 4: Characteristics of the treatment and conclusions of the intervention
- Legend: CO (Context-Optimization ) / MLT (milieu language teaching) /BTR (broad target recasts) /SLI (specific language impairment) /PO.DE.MOS ( Programa para el Desarrollo de la Morfosintaxis ) /CAI (Computer-assisted intervention ) /TTI ( Table-top intervention )
How to cite
Pdf version for download, related articles.
- Google Scholar
Versões e tradução automática
- Google Translator
- Microsoft Translator

An official website of the United States government
The .gov means it’s official. Federal government websites often end in .gov or .mil. Before sharing sensitive information, make sure you’re on a federal government site.
The site is secure. The https:// ensures that you are connecting to the official website and that any information you provide is encrypted and transmitted securely.
- Publications
- Account settings
Preview improvements coming to the PMC website in October 2024. Learn More or Try it out now .
- Advanced Search
- Journal List
- J Speech Lang Hear Res
Advances in Specific Language Impairment Research and Intervention: An Overview of Five Research Symposium Papers
Mabel l. rice.
a Child Language Doctoral Program, University of Kansas, Lawrence

Associated Data
This article provides an overview of five papers appearing together on the topic of “Advances in Specific Language Impairment Research and Intervention,” which was the 2019 program in an ongoing series of research symposia presented at the Annual Convention of the American Speech-Language-Hearing Association.
The article provides a historical context for the set of papers, then a short summation of each paper's content, followed by the identification of overarching themes and working conclusions.
Each paper provides summations of empirical results, and some papers provide new empirical evidence.
The papers collectively highlight six points: (a) Children with specific language impairment (SLI) are likely to be unidentified among their age peers. (b) There is great need for better identification of children with SLI across developmental levels. (c) Progress is evident toward a better understanding of causal pathways, as examined across different research designs involving comparison of children with typical language acquisition to children with SLI and other possibly co-occurring atypical conditions. (d) Measuring multiple dimensions of language brings enhanced informativeness, with differing outcomes for differing dimensions. (e) Replicated research findings require precision of methods in order to reduce unexplained error variance especially when defining groups. (f) Accurate identification of children with SLI is the first step toward a sound treatment plan for SLI and reading disorders as well.
Presentation Video
https://doi.org/10.23641/asha.13063721
Under the leadership of Margaret Rogers, Chief Staff Officer for Science and Research at the American Speech-Language-Hearing Association (ASHA), there is an annual research forum offered at the time of the Annual Convention, funded by competitive grant support provided by the National Institute on Deafness and Other Communicative Disorders (NIDCD) and documented by follow-up publications within a year of the Convention. In Spring 2015, planning began for the research forum reported in the following papers on the topic, “Advances in Specific Language Impairment Research and Intervention.” The first step was the recruitment of a panel of active researchers with a history of funding from the National Institutes of Health (NIH), encompassing a range of topics adding to our knowledge of specific language impairment (SLI). Each of the panelists/contributing authors carry out a program of investigation of children with SLI, consisting of multiple studies exploring multiple dimensions of SLI over different ages of children.
SLI is defined by the NIDCD (2019 , para. 1) as “a communication disorder that interferes with the development of language skills in children who have no hearing loss or intellectual disabilities. SLI can affect a child's speaking, listening, reading, and writing.” The causes of the condition of SLI are unknown. The reference group for identification of children with SLI is a same-age group of typically developing children whose language acquisition is seemingly effortless, around the world, across many languages. The causal sources of this ubiquitous human ability are also not known beyond a general conclusion that they are related to children's brain development and opportunities to communicate with people. There is a common assumption that language acquisition is easy for all healthy children, barring extreme limitations or mitigating circumstances. For example, a renown scholar of children's language acquisition once wrote in an important book on the topic, “In general, language acquisition is a stubbornly robust process; from what we can tell there is virtually no way to prevent it from happening short of raising a child in a barrel” ( Pinker, 1984 , p. 29). The same author later adjusted that perspective, recognizing the existence of SLI as a likely inherited condition leading to difficulties in language acquisition ( Pinker, 1994 , p. 48).
The point here is that studies of children with SLI invariably straddle what is known about language acquisition in two groups of children: typically developing children, as compared to apparently typically developing children who nevertheless lag behind their same-age peers in language acquisition. This reciprocity contributes to new knowledge about possible causal pathways for universal human language acquisition (shared robustness) as well as vulnerabilities in language acquisition (unexplained departures from typical expectations). Informativeness can be further enhanced with research designs involving comparisons with strategically selected groups of atypical children, such as young children at risk for future SLI, children with social (pragmatic) communication disorder or S(P)CD, children with attention-deficit/hyperactivity disorder (ADHD), children with reading disorders, and children with low nonverbal intelligence. Such comparisons bring valuable insights about the timing of early language acquisition, possible differences in developmental change for children with and without SLI, and the extent to which other atypical developmental conditions are independent of SLI (suggesting nonshared causal pathways and potentially discrete developmental mechanisms underlying language acquisition).
Across the papers included in the forum, group comparison studies clarify linguistic details in the identification of relative ease or difficulty of acquisition among the various linguistic elements to be mastered. For example, one paper in this collection focuses on what young English-speaking children need to know to generate diverse simple sentences and avoid grammatical errors. Another question across papers is whether the components of linguistic systems (e.g., speech, vocabulary, syntax, morphosyntax, narratives, and social pragmatics) in children with SLI or clinical comparison groups are synchronized across elements in the same way as children with typically developing language or whether some components are more likely to lag behind other components. Studies of instructional methods shine light on possible teaching strategies that could be used to enhance the rate of acquisition in children with SLI to close the gap with their age peers. Such studies also provide essential information about how to benchmark training methods for children with SLI relative to their age peers whose language acquisition is ahead of children with SLI, following the educational practice of grouping children by age for instruction. These issues are among the ones in the papers reported in this research symposium. Highlights from each of the papers within the SLI research forum are briefly summarized here, in order according to a developmental progression, from gestation to elementary school age reading levels.
The topic of the first paper ( Rice, 2020 ) literally begins with gestation, in investigations of twin pairs of children defined by their biological relationship—that is, monozygotic (MZ) versus dizygotic pairs (DZ). Comparisons of language acquisition between twins and singletons inform us of biological/developmental risks associated with twinning, and comparisons of children within twin pairs inform us of the relative contributions of shared environment versus shared genes as indexed by heritability estimates. Comparison of heritability estimates across multiple dimensions of language, speech, and nonverbal cognition provides indications of possible causal pathways across elements of language and cognition. A population base sample provides evidence of the extent to which low levels of nonverbal IQ and language variables overlap or diverge (e.g., revealing the existence of children with low nonverbal IQ who nevertheless have language levels equivalent to their age peers) and possible change over time in a longitudinal study. Key outcomes reveal the value of the differences in empirical measures that index levels of performance (i.e., how children differ in their score levels) versus how empirical measures are associated with each other (e.g., if co-twin MZ children are more like each other than co-twin DZ children, or if there is an association between a child's score on a language assessment with their score on a nonverbal IQ assessment). The third key outcome is that language is composed of dimensions that can be measured separately, such as speech versus vocabulary versus grammar, with different dimensions revealing different outcomes. The indices of multiple levels of performance reveal differences between twins and singleton children during the early period of language acquisition, such that twins are at risk for delay in reaching language milestones. The indices of within–twin pair associations reveal moderate to high levels of heritability for speech and language, although the size of the heritability estimates varies by linguistic dimension, age, and whether it is calculated as heritability of a clinical group (such as SLI) or across the full sample of twins (all children regardless of clinical status). Results from this study indicate that the relationship between nonverbal IQ and speech and language measures is not straightforward and that the classic clinical definition of SLI brings different outcomes than those from a nonspecific language impairment (NLI) group that includes children with clinical levels of nonverbal cognitive impairments. Heritability of language replicates from 2 to 16 years on measures of grammar and vocabulary, although the pattern of heritability does not replicate across SLI and NLI groups in a longitudinal study at ages 4 and 6 years, cautioning us about the need to keep these groups separate when examining potential underlying causal mechanisms. Overall, the outcomes of the reported twin studies add support to the generalization that language acquisition and language impairments are each heritable, with additional details needed regarding heritability of grammar, vocabulary, and nonverbal IQ. Greater precision in outcomes is evident when language impairment is differentiated from low levels of nonverbal IQ, suggesting some degree of independence in causal pathways for SLI versus NLI. Outcomes have implications for theoretical models of genetic effects on language, suggesting the need to incorporate recognition of timing effects in the start of language acquisition and in growth over time in childhood.
The second paper ( Hadley, 2020 ) focuses on early stages of language acquisition in toddlers as they begin to generate sentences, comparing toddlers developing language typically and toddlers at risk for SLI. The methods of investigation are precise, sentence-focused analyses of children's spontaneous utterances, informed by linguistic perspectives and high standards of empirical analyses. Analytic outcomes are literally at the level of individual words as well as carefully specified interpretations of intended syntactic structures. The sentence-focused framework predicts that children's early verb vocabulary will be more strongly associated with later grammatical outcomes than early noun vocabulary, and first-person subjects (i.e., “I”) lead the way for diverse simple sentences versus third-person subjects (e.g., “she” or “he”). Conversely, reliance on first-person subjects for necessary sentence diversity could be diagnostic of a young child at risk for SLI as a preschooler. A review of previous studies in the author's lab is provided, encompassing data from ages 21, 24, 27, and 30 months. In addition, new descriptive analyses of archival data from ages 30, 33, and 36 months are reported. Key outcomes are as follows: Documentation of precise methods of assessing sentence diversity to chart how this linguistic growth occurs in toddlers and a strong case for how individual differences in the developmental patterns of emerging sentences are candidates for methods of identifying SLI early in children's development, as well as providing important documentation of the ways in which children with typical language acquisition build their early sentences.
The third paper ( Leonard & Deevy, 2020 ) focuses on the possible role of verbal memory on word learning and retention in children with SLI, whose risk for problems with word learning are well documented. Although it is apparent to most parents that early word learning is a rapidly accelerating process, as well as to every teacher that a child's readiness for reading instruction requires the expected vocabulary size by school age, the exact ways in which children accomplish the rapid growth without explicit tutoring are not well documented. Further, by the time children enter school, any vocabulary instruction they receive is usually incidental to a focus on new content. There is a great need for precise information about exactly how to teach new words to children, with identification of methods that would be beneficial for young children with SLI and their same-age peers with typical development. The third paper reviews outcomes of a program of experimental studies investigating the role of retrieval practice in experimental settings for learning novel words under different retrieval conditions, comparing preschool-age children with SLI and their same-age peers with typical development. Four logically related experimental studies are reported, with outcomes summarized in detail. With the caveat that if future experimental refinements support the findings, the interpretive and clinical implications are that repeated spaced retrieval activities in naturalistic clinical settings and educational activities could help children with SLI acquire new words to add to their vocabulary. If this proves to be the case, it would be a significant addition to instructional methods. Such detailed experimental training studies inform our understanding of typically developing children's language acquisition as well as children with SLI.
The fourth paper ( Redmond, 2020 ), addresses the issue of possible co-occurrence of SLI ( Stark & Tallal, 1981 ) with ADHD, as compared to two other operationalizations of idiopathic language disorder, in an analysis of the study sample compiled by his lab's program of investigation of SLI and ADHD. The three definitions were classic SLI ( Stark & Tallal, 1981 ), the recent criteria for developmental language disorder (DLD; Bishop et al., 2017 ), and the criteria of the Diagnostic and Statistical Manual of Mental Disorders, 5th Edition (DSM5; American Psychiatric Association, 2013 ) definition of language disorder. Another category of communication disorder must also be considered, that of S(P)CD, as defined and included in the recent DLD criteria. The key outcome is that the amount of overlap between ADHD and language impairment diagnostic groups varied according to the criteria for language impairment: Co-occurrence of language impairment and ADHD was 2% under the DSM5 definition of “language disorders,” which separates language disorder from the S(P)CD designation, whereas co-occurrence increased to 22.3% under the broader DLD criteria, which includes S(P)CD as well as cases of NLI. The SLI designation yielded 16.9% co-occurrence. It is of interest that the presence of pragmatic symptoms exerted a stronger influence on observed co-occurrence rates than low nonverbal abilities. These outcomes are clearly relevant to research aimed at sorting out the co-occurrence of SLI with other clinical conditions as well as clinical practice decisions involving eligibility for services, risk for co-occurring conditions, and determination of treatment goals.
The fifth paper ( Adlof, 2020 ) addresses the possible overlap of SLI and dyslexia. A review of definitions and inclusionary and exclusionary criteria is provided, followed by a review of the intersection/overlap of the two disorders, focusing on language, working memory, and academic achievement. The detailed comparisons of groups of children with SLI-only, dyslexia-only, and SLI + dyslexia versus neither SLI or dyslexia across different outcomes supports the conclusion that SLI and dyslexia are separate disorders, yet frequently co-occur. Children in the SLI + dyslexia group are likely to have the most severe deficits. The results support the need for further studies in how to differentially identify children with SLI and children with dyslexia, such that it is not assumed that the presence of one condition entails the presence of the other, although both confer risk to academic achievement. The paper concludes with advocacy for screenings of oral language at school entry as well as screenings of dyslexia as precursors to response-to-intervention (RTI) methods for identifying children with dyslexia, a method heavily weighted toward word-reading skills.
Collectively, the papers address overarching themes and working conclusions. One is the need for identification of affected children across developmental levels. This objective requires precise knowledge of language acquisition in children developing typically for indications of unexpected variance such that some children, for no apparent reason, are lagging behind age expectations. The identification of accurate clinical markers of SLI in children across age levels is an ongoing and important research goal. Detailed linguistic analyses of early sentences are promising for the challenging toddler period of dynamic language development, a time of wide variation across children. Screenings at school entry are needed for identification of children with low levels of language acquisition and/or early reading skills to avoid delayed detection of children when intervention should already be underway.
A second theme is a focus on causal pathways and the extent to which language impairment is independent of other developmental disorders. The situation is complicated by cases of clinical co-occurrences that may appear to confirm an expected pattern that does not hold up in population-ascertained samples. Although some may assume that children with SLI are just not very smart (or were raised in a barrel), recent twin studies document the independence of low nonverbal IQ and language impairments. There is evidence of heritability for both, albeit heritability is stronger for speech and language variables. In studies of singleton children, SLI can overlap with some other disorders (e.g., dyslexia, ADHD), yet no consistent evidence for a single shared causal pathway has emerged, as reported in two papers in this collection. At the same time, it is good to keep in mind that studies of twin children indicate moderate to high heritability for language—higher for analyses investigating heritability of SLI as compared to estimates of heritability across all children in a sample. It is possible that the ways in which children with SLI differ from most children will be an age-dependent part of the causal pathway, perhaps related to underlying timing mechanisms for the onset of language acquisition in early childhood.
A third theme is the need to consider the multidimensionality of language. Across the papers, there is a focus on the particular dimensions of language of interest for the purpose of the investigation, ranging from first words, first sentences, word learning in experimental conditions, precise morphosyntactic and grammatical elements, composite language scores across elements, and language adjustments for social uses. Across the papers' outcomes, one conclusion is that it matters which linguistic element is operationalized in a study. Language is complex, multidimensional, and age-dependent in children, and precise measurement is required.
A fourth theme is the need to reduce unexplained error variance, especially in the definition of groups. This is most explicit in the paper comparing ADHD co-occurrence with three different definitions of language impairment ( Redmond, 2020 ). If the definition of language impairment was loosely defined in a way that any of the three possible definitions could be used to place a child in the “language impairment group” (i.e., identified according to the classic SLI criteria, the DSM5 criteria, or the DLD criteria), this combined criterion would generate a larger group size than a more conservative definition. Note that under such a broad definition, it would be possible to miss the distinctive contribution of S(P)CD and low nonverbal IQ. The conclusion could be quite misleading, due to unexplained error variance (i.e., unidentified sources of spurious overlap in causal pathways for ADHD and language impairments), which could work against the identification of real overlap or real independence—a first step for understanding possible shared or unique causal pathways. Note, also, that such a broad definition of language impairment could undermine replicability of outcomes across studies, because the samples could vary considerably in the proportion of children with or without S(P)CD or children with or without very low levels of nonverbal IQ. Another example is evident in the paper on dyslexia and SLI ( Adlof, 2020 ). The paper highlights that predictors differ for dyslexia and SLI: Poor phonemic awareness is associated with dyslexia more than SLI, whereas SLI is more likely to be associated with grammatical weakness. Regarding nonverbal IQ, studies of SLI and dyslexia aimed at understanding causal mechanisms could have benefited from nonverbal IQ cutoff levels to arrive at the generalizations. Common cut-point levels could avoid increased error variance attributable to the effects introduced by increased shared variance of low nonverbal IQ with SLI and dyslexia than is evident among the variables when all children have nonverbal IQ levels clearly within typical range. Much of the current literature focuses on SLI or dyslexia, not studies of comorbidity. It will be important to conduct studies in which measures of language, reading, and nonverbal IQ are collected from the same children in a large population–based sample. Methods of analyses in the twin studies ( Rice, 2020 ) generate outcomes most likely to replicate across studies within one lab or across different labs if the measurement methods are precise, including criteria for differentially grouping children as language impaired with or without low nonverbal IQ. Note, it is valuable to also include the children in the population who have low nonverbal IQ with co-occurring language skills within or even above typical range. In summary, the point here is that control of unexplained variance in the grouping variable can be enhanced by using cutoffs for low performance, preferably at the same level for language and nonverbal IQ, which, in the case of low nonverbal IQ, is also likely to screen/avoid children with undiagnosed neurological conditions ( Stark & Tallal, 1981 ).
A common theme across the papers is concern with clinical issues related to identification of children with SLI, across a wide age span. Evaluation of diagnostic definitions or clinical markers are addressed in four of the papers ( Adlof, 2020 ; Hadley, 2020 ; Redmond, 2020 ; Rice, 2020 ). Two of the papers focus on intervention methods ( Adlof, 2020 ; Leonard & Deevy, 2020 ), and a third paper addresses intervention in the literature review ( Hadley, 2020 ).
Collectively, the papers here are consistent with a robust scientific commitment to better understand how to account for unexpected individual differences in language acquisition in children with SLI who seem to have the essential prerequisites, how to identify them, and how to provide effective treatment to help enhance their language abilities in a time when language is vital to the well-being of children and adults. In turn, studies of children with SLI increase our knowledge of language acquisition in general, the underlying causal pathways, how language unfolds over a developmental trajectory, and our knowledge of how nonlinguistic cognitive or social abilities are associated (or not) with language acquisition. The papers in this collection provide valuable new information and replicate findings reported in the many valuable precedents in our scientific literature on the condition of SLI.
Supplementary Material
Acknowledgments.
This article summarizes papers from the Research Symposium at the 2019 Annual ASHA Convention, which was supported by the National Institute on Deafness and Other Communication Disorders of the National Institutes of Health under National Institute of Health Grant R13DC003383. Support for preparation of this paper was provided by the National Institute on Deafness and Other Communication Disorders of the National Institutes of Health under National Institute of Health Grants R01DC001803, R01DC005226, and T32DC000052 to the University of Kansas (Principal Investigator: Rice). The content is solely the responsibility of the author and does not necessarily represent the official views of the National Institutes of Health.
I appreciate editorial feedback from the authors of the other papers from the Symposium and from Kathleen Kelsey. During the preparation of this article, I was sorry to learn of the death of Donna Kelly, one of the early graduates of the Child Language Doctoral Program at the University of Kansas. She was an Associate Professor in Audiology and Speech Pathology at the University of Arkansas, Little Rock. I appreciate her many contributions to clinical training and research, and I know she would have read this set of articles. I also appreciate her choice of me as her academic advisor, a collaboration beneficial to each of us, which surely played a role in the research over the years that followed.
Funding Statement
- Adlof, S. M. (2020). Promoting reading achievement in children with developmental language disorders: What can we learn from research on specific language impairment and dyslexia? Journal of Speech, Language, and Hearing Research , 63 ( 10 ), 3277–3292. https://doi.org/10.1044/2020_JSLHR-20-00118 [ PMC free article ] [ PubMed ] [ Google Scholar ]
- American Psychiatric Association. (2013). Diagnostic and statistical manual of mental disorders (5th ed.). https://doi.org/10.1176/appi.books.9780890425596
- Bishop, D. V. M. , Snowling, M. J. , Thompson, P. A. , Greenhalgh, T. , &. the CATALISE-2 consortium. (2017). Phase 2 of CATALISE: A multinational and multidisiplinary Delphi consensus study of problems with language development: Terminology . Journal of Child Psychology & Psychiatry , 58 ( 10 ), 1068–1080. https://doi.org10.1111/jcpp.12721 [ PMC free article ] [ PubMed ] [ Google Scholar ]
- Hadley, P. A. (2020). Exploring sentence diversity at the boundary of typical and impaired language abilities . Journal of Speech, Language, and Hearing Research , 63 ( 10 ), 3236–3251. https://doi.org/10.1044/2020_JSLHR-20-00031 [ PMC free article ] [ PubMed ] [ Google Scholar ]
- Leonard, L. B. , & Deevy, P. (2020). Retrieval practice and word learning in children with specific language impairment and their typically developing peers . Journal of Speech, Language, and Hearing Research , 63 ( 10 ), 3252–3262. https://doi.org/10.1044/2020_JSLHR-20-00006 [ PMC free article ] [ PubMed ] [ Google Scholar ]
- National Institute on Deafness and Other Communication Disorders. (2019). Fact sheet: Specific language impairment . https://www.nidcd.nih.gov/health/specific-language-impairment
- Pinker, S. (1984). Language learnability and language development . Harvard University Press. [ Google Scholar ]
- Pinker, S. (1994). The language instinct: How the mind creates language . William Morrow Co. [ Google Scholar ]
- Redmond, S. M. (2020). Clinical intersections among idiopathic langauge disorder, social (pragmatic) communication disorder, and attention-deficit/hyperactivity disorder . Journal of Speech, Language, and Hearing Research , 63 ( 10 ), 3263–3276. https://doi.org/10.1044/2020_JSLHR-20-00050 [ PMC free article ] [ PubMed ] [ Google Scholar ]
- Rice, M. L. (2020). Causal pathways for specific language impairment: Lessons from studies of twins . Journal of Speech, Language, and Hearing Research , 63 ( 10 ), 3224–3235. https://doi.org/10.1044/2020_JSLHR-20-00169 [ PMC free article ] [ PubMed ] [ Google Scholar ]
- Stark, R. , & Tallal, P. (1981). Selection of children with specific language deficits . Journal of Speech and Hearing Disorders , 46 ( 2 ), 114–122. https://doi.org/10.1044/jshd.4602.114 [ PubMed ] [ Google Scholar ]
- Open access
- Published: 25 May 2024
Spatial transcriptomic brain imaging reveals the effects of immunomodulation therapy on specific regional brain cells in a mouse dementia model
- Eun Ji Lee 1 , 2 ,
- Minseok Suh 1 , 3 , 4 ,
- Hongyoon Choi 1 , 3 ,
- Yoori Choi 1 , 5 ,
- Do Won Hwang 6 ,
- Sungwoo Bae 1 , 3 , 4 &
- Dong Soo Lee 1 , 2 , 3 , 4 , 7
BMC Genomics volume 25 , Article number: 516 ( 2024 ) Cite this article
Metrics details
Increasing evidence of brain-immune crosstalk raises expectations for the efficacy of novel immunotherapies in Alzheimer’s disease (AD), but the lack of methods to examine brain tissues makes it difficult to evaluate therapeutics. Here, we investigated the changes in spatial transcriptomic signatures and brain cell types using the 10x Genomics Visium platform in immune-modulated AD models after various treatments. To proceed with an analysis suitable for barcode-based spatial transcriptomics, we first organized a workflow for segmentation of neuroanatomical regions, establishment of appropriate gene combinations, and comprehensive review of altered brain cell signatures. Ultimately, we investigated spatial transcriptomic changes following administration of immunomodulators, NK cell supplements and an anti-CD4 antibody, which ameliorated behavior impairment, and designated brain cells and regions showing probable associations with behavior changes. We provided the customized analytic pipeline into an application named STquantool. Thus, we anticipate that our approach can help researchers interpret the real action of drug candidates by simultaneously investigating the dynamics of all transcripts for the development of novel AD therapeutics.
Peer Review reports
Introduction
Central nervous system (CNS) and central immune system (bone marrow: BM) interactions, specifically brain-immune cross-talk, can occur by a pathway from the skull BM, meninges and their lymphatics, and cerebrospinal fluid (CSF) to the brain parenchyma [ 1 , 2 , 3 , 4 , 5 , 6 , 7 , 8 , 9 , 10 , 11 , 12 , 13 , 14 ] and/or by another pathway from the choroidal plexus (CP) capillary-stroma-epithelium and CSF to brain parenchyma [ 15 , 16 , 17 , 18 , 19 ] in addition to by the classic pathway of crossing the blood‒brain barrier (BBB) [ 20 , 21 , 22 , 23 ]. In explicit neuroinflammatory diseases such as multiple sclerosis in humans or experimental autoimmune encephalomyelitis (EAE) in animal model, immunoglobulins or immune cells have been considered to enter the brain parenchyma via the BBB [ 20 ] of the brain parenchyma or via the brain-CSF barrier of the CP [ 24 , 25 ], or recently via the arachnoid barrier cell (ABC) layer of skull BM-meningeal lymphatics and CSF/perivascular spaces reaching the brain parenchyma [ 3 , 4 , 5 , 17 , 26 , 27 , 28 ].
Novel immunomodulatory therapy in Alzheimer’s disease (AD) transgenic models, such as 5xFAD mice, should be accompanied by the improvement of cognitive decline associated with aging and/or the amelioration of the transgenes’ adverse effects, such as priming brain cells or immune responses during development and aging. When we inadvertently found the effect of the anti-CD4 antibody while investigating the effect of aducanumab [ 29 ] and encountered the probable effect of allogeneic natural killer (NK) cell supplements in AD models [ 30 ], we questioned which cells or transcriptomic markers in the brain areas would be the best to predict the outcome of these novel, currently unaccounted therapeutic candidates. In AD mouse models including 5xFAD mice, the surrogate effect markers of previous findings/trials of systemic or intraventricular administration of CD8 + T cells [ 31 ], anti-CD8 [ 32 ] or anti-CD3 [ 33 ] antibodies, Treg cells [ 34 , 35 , 36 ] (or for stroke model [ 37 ] or DEREG model for traumatic brain injury model [ 38 ]), and amyloid-sensitized Th1 cells [ 39 , 40 , 41 ] were amyloid plaques/Aβ on immunohistochemistry and transcriptional signatures of major brain cells and brain parenchyma [ 32 , 33 , 35 ] or CP infiltrating cells [ 25 , 42 ]. As systemically injected cells and immunoglobulins were not examined for their location or biodistribution, direct CNS effects or systemic actions on immune systems were always the alternative to explain the probable effect of novel immunomodulatory therapies, which inevitably led to the insufficient understanding of the target cells and areas. This resulted in inconsistent findings among the reporting investigators.
Single-cell or single-nucleus RNA sequencing (scRNAseq/snRNAseq) based on tissue dissociation and preparation of a single-cell suspension followed by next-generation sequencing allows comprehensive characterization of cell types in the tissue [ 43 , 44 , 45 , 46 ]. Recently available barcode-based spatial transcriptomics (ST) using the solid-phase capture of RNA on slides, such as Visium® [ 47 , 48 , 49 , 50 ], HDST [ 51 ], slideSeqV2 [ 52 ], Seq-Scope [ 53 ], or stereo-Seq [ 54 , 55 , 56 ], adds a spatial dimension to transcriptomics and enables spatial characterization of genes and cell types through robust regional segmentation of the tissue. Regional and cell-type specific characterization of one or more sections of the mouse brain based on this reliable anatomical segmentation mostly allowed for the comparison of the basal states between groups or even the task-related active states by calcium two-photon imaging and scRNAseq of the visual cortex [ 57 , 58 ]. Given that ST allows us to obtain genome-wide spatial expression profiles, ST can be considered multiplexed molecular imaging of the brain. We can now use spatial transcriptomic brain imaging to investigate whether a probable immunomodulatory therapy yields its effect on major brain cells (and infiltrating or rare cells of the brain) in each segmented brain area after systemic administration [ 30 ]. Biodistribution studies after systemic injection can inform whether immune cells or antibodies enter and directly interact with brain cells; however, if we do not see the immediate presence of cells (usually none) and antibodies (less than 1% of the injected dose), we assumed that they would influence brain cells and pathologic processes. Transcriptomic changes owing to proper novel immunomodulatory therapy will enable us to explore the probable target cells/genes that would have caused or at least be associated with the expected behavioral effects of these therapies [ 30 ]. This is especially helpful early in the pursuit of potential new drugs so that investigators can be confident that they are moving in the right direction to modify and optimize new therapeutic candidates. Transcriptomic changes of a region or regions and a cell type or cells in a group are expected to explain the behavioral results of the mouse model. Finally, the transcriptomic changes might predate the behavioral improvements. In both cases, we expect that transcriptomic profiles at a high spatial resolution would excel for drug screening, in sensitivity and target-cell specificity [ 47 , 54 ], over histological results of immunohistochemistry. Additionally, single-spot ST yields tissue-globally searchable data that can later be reanalyzed repeatedly when the marker gene combination [ 46 , 50 , 59 , 60 , 61 , 62 ] becomes available.
To do this, we needed to advance the single-spot RNA sequencing and its analyses using a customized method to derive cell type/state-specific distribution of the Visium sections. Paying attention to the proper dissociation of cell types/states using the optimum/minimum number of genes and cell‒cell interaction (CCI) and cell‒cell communication (CCC) [ 63 ], marker gene combinations should be established with the existing public database of scRNAseq/snRNAseq [ 46 , 49 , 59 , 64 , 65 , 66 , 67 , 68 ] and our own data [ 30 ]. For both tasks, ready-to-adopt methods are available by the Creative Commons regulations in previous investigations.
Stromal and parenchymal cells of various organs, including the brain, are now known to show common and specific characteristics of cell identity and their ontological characteristics, the best known of which are microglia and perivascular macrophages or resident macrophages [ 67 , 68 ]. Immune cells of monocyte-derived and resident macrophages have distinctive transcriptomic signatures, which predict their immune roles and tissue integrity-preserving roles per characteristic signatures [ 69 ]. This was also the case for T cells, where resident memory T (T RM ) cells for intestines, effector memory T (T EM/EMRA ) cells for blood, liver, and BM, and mixed T RM/EM cells for various organs and BM yield their own characteristic transcriptomic signatures that determine their differentiation of T cells in every tissue of interest, dictating their respective functional roles [ 70 , 71 ]. Unfortunately, neither of these recent cross-tissue, resident immune cell studies [ 69 , 71 ] included the brain, which mandates our own analysis.
In this investigation, we performed segmentation of brain regions on coronal/sagittal sections per dozens of animals using readily available methods and characterized the common pathologic transcriptomic signatures of 7-month-old 5xFAD mice. Immunomodulatory drugs were administered to these mice to confirm behavioral improvement. 99m Tc-hexamethylpropyleneamineoxime (HMPAO)-labeled cell-tracking imaging [ 72 ] ruled out the immediate infiltration of NK cells in the brain. Spatial transcriptomic changes in mice were examined after anti-CD4 immunoglobulin administration and expanded NK cell supplement treatment with a dose schedule, which improved Y-maze alternation behavior impairment at this age range in 5xFAD mice. Transcriptomic changes were dissected across areas and cell types/states using publicly available methods and databases, and the analytical pipelines were organized as an application named STquantool. We found that regional/areal gene set-defined type/state-specific cells showed characteristic differences after each trial treatment in a genetic model of AD, 5xFAD mice, upon spatial transcriptomic Visium analysis. Combined brain major cells including neurons, astrocytes, microglia, and oligodendrocytes with their associated types and states and brain resident/infiltrating rare immune cells per region were explored for their distinctive transcriptomic changes among mouse groups to yield their probable association with behavior improvements.
Spatial transcriptomic characterization of gene-set-defined type/state-specific major brain cells in 7-month-old wild-type and 5xFAD mice
In total, 35 coronal and 28 sagittal brain sections from 63 mice of either wild-type or 5xFAD background were included in the analysis. A spatial barcode was given for every spot, the unit tissue domain of spatial transcriptomics, and at least 50,000 reads were obtained from each of the 4,992 spots in a capture region. The brain tissues were covered by an average of 3,000 spots across all samples. Using the count matrices computed from Space Ranger as inputs to the reciprocal principal component analysis (RPCA) based integration and clustering pipelines supplied by Seurat (Seurat 4.1; https://satijalab.org/seurat/ ), the multiple brain tissues were segmented based on their transcriptome patterns [ 73 ]. By optimizing parameters such as the resolution of spatial clusters and others, we yielded segmented spatial cluster images in every case, including those with various treatments and manipulations. The treatments and manipulations are listed in Supplementary Fig. 1 A and Supplementary Table 1 . These included anti-CD4 antibody treatment and NK cell supplement treatment groups. Others were 3-month-old 5xFAD mice, cervical lymphatic ligation, a P301L model with or without amyloid/tau-rich lysate injection, and fingolimod hydrochloride (FTY720) injection with or without lipopolysaccharide (LPS) pretreatment.
The difference between 10 ST data points from wild-type and 11 from 5xFAD animals was compared for each of 14 spatial clusters using 7 to 8 coronal and 3 sagittal sections. For the integration of slides based on RPCA, the transcriptomes of wild-type animals were used as pivots, and the spots from diseased animals were mapped to the PCA space of the wild-type reference [ 74 ]. The corrected counts derived from the integration were used to cluster the spots. Spots clusters were visualized in all individual sections and verified for their accuracy in designating the areas according to already-known anatomical correlates (Supplementary Fig. 1 B-D). Only the askew sagittal section in a few mice missed the dorsal striatum and instead supplied the septal lobe in a more median position, but the spatial clustering correctly showed the pair of dorsal/ventral striatum in some sections and septal/ventral striatum in others. The spots from each cluster and group were represented by a UMAP plot, a dimensionality reduction method for visualization, and the clusters were well separated in terms of gene expression (Supplementary Fig. 1 E, F).
Using the developed platform, STquantool, the representative genes for each cell type were determined by literature or data-driven methods and validated based on spatial gene expression patterns (Materials and Methods). Cell signature scores for each cell type were calculated by the difference between the average expression of the marker genes and that of the randomly selected control genes. Since the cell scores are derived from the curated marker genes and indirectly measure the abundance of the cell type in each spot, it can be postulated that the spatial distribution of the cell scores reflects the spatial distribution of the corresponding cell type. Regional/areal transcriptomes representing major brain cell types/states were then compared between groups by averaging the major cell scores in wild-type and 5xFAD mice using the Wilcoxon rank-sum test (Supplementary Fig. 2 ). In addition to the major cell types, scores were also calculated in 32 subtypes of neurons [ 75 ], several types of astrocytes [ 76 ], microglia, and oligodendrocytes [ 66 ]. In addition, the reactive state-specific changes and marker genes of astrocytes and microglia were compared between wild-type and 5xFAD animals in 14 brain regions.
To investigate brain regional changes during pathological progression in AD, we obtained ST data from coronal brain sections of the 5xFAD mouse model and age-matched wild-type mice at three and seven months of age. The 5xFAD AD model is known to show amyloid deposition and reactive gliosis from two months of age and synaptic loss and cognitive impairment from four to six months of age (Supplementary Fig. 3 A). Amyloid deposition was observed in the adjacent sections of the samples used for ST analysis (Supplementary Fig. 3 B). In both coronal and sagittal sections, beta-amyloid levels began to increase at three months of age, and dramatic increases were observed remarkably in the deep cortex, thalamus, hippocampus, and amygdala of seven-month-old 5xFAD mice. The major brain cells were classified into neurons, astrocytes, microglia and oligodendrocytes and their associated cell types (Supplementary Fig. 4 A). Neurons were classified according to the reports of Hodge et al. [ 75 ] of the Allen Institute with Aeverman et al.’s [ 59 ] random forest hierarchical clustering method (NSForest) to define the optimal marker gene combination for neuron subtypes, which was verified by the reports of BICCN [ 77 ] and another group’s approach [ 78 , 79 , 80 , 81 , 82 ]. Astrocytes were classified according to the types of white matter-associated and gray matter-associated astrocytes [ 76 ] once and again into region-specific astrocytes for the cortex/hippocampus (telencephalon), thalamus, and other brain regions (diencephalon) [ 49 , 83 ]. Reactive astrocytes and their marker gene combinations were determined by the suggestions of Escartin et al. [ 84 ] and other investigators (Habib et al. [ 85 ], Ioannou et al. [ 86 ], Chamling et al. [ 87 ] etc.). Oligodendrocytes and their associated cell types were designated by following an initial report by Marques et al. [ 66 ] and were verified by other investigators’ suggestions [ 86 , 87 ]. Microglia were classified according to their states but not types considering their homeostatic and reactive (microglia with neurodegeneration: MgND [ 88 ], disease-associated microglia: DAM [ 89 ], lipid-droplet associated microglia: LDAM [ 90 ], etc.) states [ 68 , 91 , 92 ], and thus, no subtypes of microglia were assigned. Instead, the aging-related effect on its own or associated with amyloid pathology was examined to show amyloid pathology excluding the confounding effect of aging [ 93 ].
Using the data by Ximerakis et al. [ 64 ] for defining cell types, the hierarchical clustering method suggested by Hodge et al. [ 75 ] and NSForest (version 2.0) by Aeverman et al. [ 59 ], neurons were typed and subtyped into 20 GABAergic neurons and 12 glutamatergic neurons. Their pattern of expression is displayed for wild-type and 5xFAD mice in Fig. 1 A. The differences between wild-type and 5xFAD mice in coronal and sagittal sections of 9 areas of interest were quantitatively analyzed (Supplementary Fig. 4 B). Subtypes of GABAergic and glutamatergic neurons showed unique patterns in wild-type and 5xFAD mice. The expression patterns of the GABAergic somatostatin (Sst) subtypes in the amygdala differed between wild-type and 5xFAD mice (Fig. 1 B and Supplementary Fig. 4 C). Increased expression in the amygdala was prominent in the 5xFAD mice compared to the wild-type mice. Notably, the individual genes ( Sst, Nr2f2, Tac1 , and Moxd1 ) tended to be expressed at higher levels in the amygdala of 5xFAD mice among the gene combinations (Supplementary Fig. 5 ). Furthermore, increased expression of Sst was identified at the protein level in the amygdala and striatum regions but not in the deep cortex, thalamus, or hippocampal regions of seven-month-old 5xFAD mice (Supplementary Fig. 6 ). The most pronounced increase was found in the amygdala, with only a slight change in the striatum, and the results were consistent with those at the transcript level. Thus, after the accumulation of amyloid plaques in the 5xFAD mice, a marked increase in specific subclasses of inhibitory neuron-associated genes in the amygdala was remarkably identified.
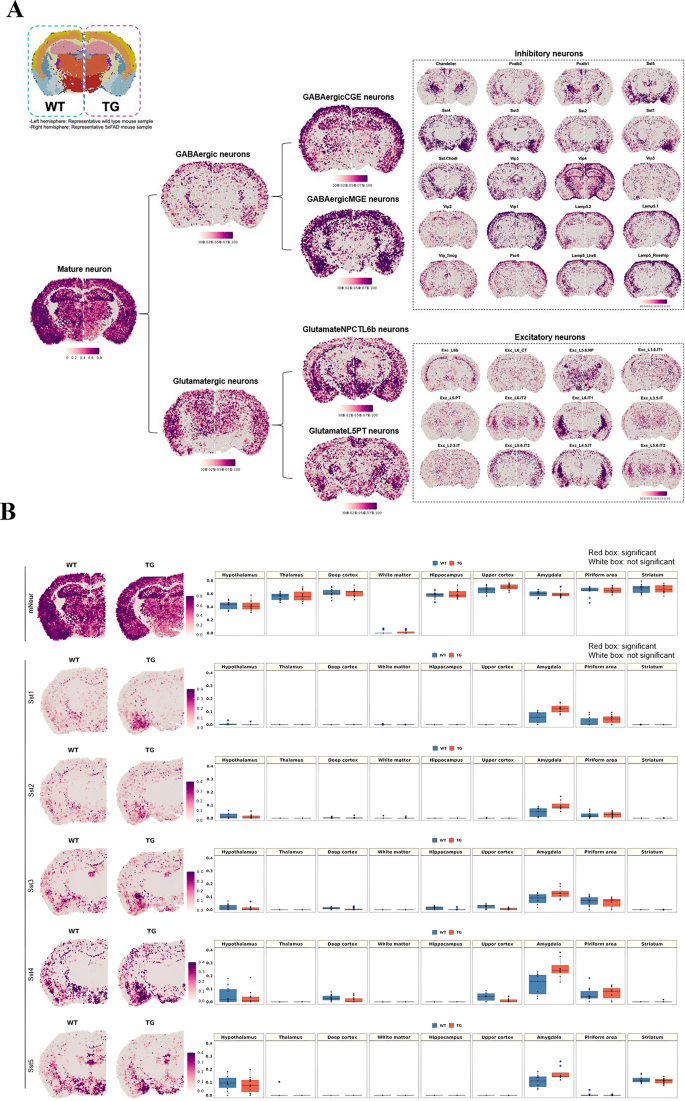
Brain region-specific expression patterns of the signatures of neuronal subclasses and spatial changes in neurons in 5xFAD mice compared to wild-type mice. ( A ) Spatial patterns of diverse neuronal subclass signatures. The left side is a representative slide of a wild-type mouse, and the right side is that of a 5xFAD mouse. Each cell type showed distinct region-specific expression. First, mature neurons were subdivided into GABAergic and glutamatergic neurons, and then the cells were further divided into subclasses to show the regional distribution of subclasses of inhibitory and excitatory neurons. ( B ) Spatial pattern of the neuronal signatures (mature neurons, Sst1, Sst2, Sst3, Sst4, and Sst5; left). Representative images of each group were selected among 10 spatial transcriptome datasets of wild-type mice and 11 of 5xFAD mice. Spatial patterns of the Sst-subclass of inhibitory neuronal signatures of the 5xFAD mice were the most remarkably different compared to those of wild-type mice. The spatial distribution of Sst subclass neurons was similar between wild-type and 5xFAD mice, and the expression of Sst subclasses was higher in 5xFAD exclusively in the amygdala. The boxplot revealed the average module score of Sst-subclass inhibitory neurons, and expression tended to be higher in the amygdala in 5xFAD mice, especially for the Sst4-subclass. Each dot represents a mouse in each group. (mNeur: mature neurons; Sst: somatostatin; WT: wild type; TG: 5xFAD mice; GABAergicCGE: caudal germinal eminence; GABAergicMGE: medial germinal eminence; GlutamateNPCTL6b: near projection, corticothalamic, and layer 6b; GlutamateL5PT: layer 5 and pyramidal tract)
The difference between wild-type and 5xFAD mice was differential according to the definition (by gene combination to define reactivity) of reactive astrocytes and reactive microglia in their density and distribution (Fig. 2 and Supplementary Fig. 7 ). Reactive astrocytes and reactive microglia shared gene signatures and were supposed to collaborate to do the job of waste disposal in situ and out of the brain while promoting the interstitial fluid space (ISF) to perivascular/CSF space to meningeal lymphatics. Astrocytes were classified into deep or upper cortical layer-specific and telencephalon- or diencephalon-origin according to Bayraktar et al. [ 83 ] and Kleshchevnikov et al. [ 49 ]. This classification did not reveal a difference between wild-type and 5xFAD mice (Supplementary Fig. 7 A). However, another two types of astrocytes, white matter-associated and gray matter-associated, according to Werkman et al. [ 76 ], yielded differences in density and distribution between wild-type and 5xFAD mice. The cell score of white matter-associated astrocytes was significantly increased in the white matter and other gray matter regions in the 5xFAD mice, but no differences were observed in the gray matter-associated astrocyte signatures (Fig. 2 A). In addition, reactive astrocytes defined by various ways [ 84 , 85 , 86 , 87 , 94 ] that showed an increase in density in white matter and neighboring gray matter areas (cortex and thalamus) in 5xFAD mice. Their distribution of reactive states was characterized to be diffuse but was prominent around the white matter in coronal and sagittal sections from 7-month-old 5xFAD mice compared with that of wild type mice. Aging astrocytes showed significant but small differences between wild-type and 5xFAD mice in the white matter, deep cortex, thalamus, and striatum (Fig. 2 A). Further analysis with individual transcriptomes used as markers for each state-specific astrocyte revealed the following findings. The expression of individual transcriptomes defining white matter-associated and reactive astrocytes showed similar patterns between wild-type and 5xFAD mice, but the dominant individual transcriptomes were different (Supplementary Fig. 8 A, B). In the gene combination of white matter-associated astrocytes, Lyz2, C1qa, Ctss, C1qb , and C1qc were the top five genes with significant differences. In reactive astrocytes, Gfap, Serpina3n, Vim , and C1qb showed dramatic increases in 5xFAD mice compared to wild-type mice.
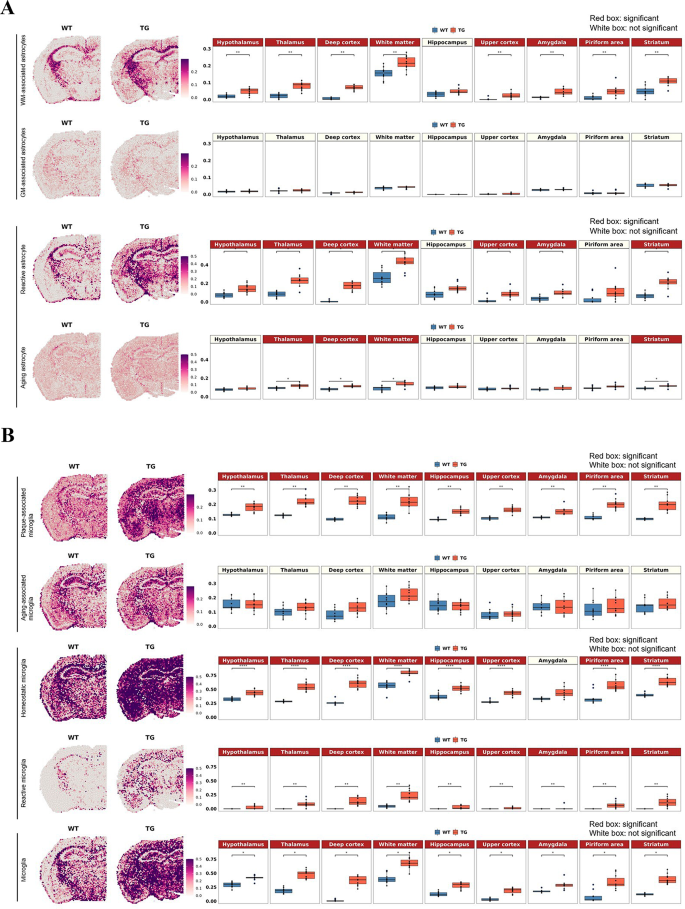
Spatial changes in the distribution of the region- or state-specific signatures of microglia and astrocytes in 5xFAD mice compared to wild-type mice. ( A ) Spatial pattern of the region-specific signatures (white matter-associated and gray matter-associated astrocytes) and the state-specific signatures (reactive astrocytes and aging astrocytes; left). Representative images of each group were selected among 10 spatial transcriptome datasets from wild-type mice and 11 from 5xFAD mice. Boxplot showing average module scores (right). Each dot represents a mouse in each group. The average module score of white matter-associated astrocytes was significantly increased in the white matter and other gray matter regions in 5xFAD mice compared to wild-type mice, but no differences were observed in gray matter-associated astrocyte signatures. Moreover, the average module score of reactive astrocytes showed a similar expression pattern to that of white matter-associated astrocytes, while significant but smaller differences were observed in white matter and several areas in the aging astrocyte signatures. ( B ) Spatial pattern of the state-specific signatures (plaque-associated, aging-associated, homeostatic, reactive, and panmicroglia). The average module score of plaque-associated microglia showed a significant increase in 5xFAD mice compared to wild-type mice, whereas aging-associated microglia showed no difference. Interestingly, both homeostatic and reactive microglia signatures showed a dramatic increase in the average module score in 5xFAD mice. Microglia in general (representing all the state-specific and nonspecific signatures) showed increased expression in all the regions without showing any regional distinctiveness. Bonferroni-adj. *p value < 0.05, **p value < 0.01, ****p value < 0.0001. (WM: white matter; GM: gray matter; WT: wild type; TG: 5xFAD mice)
Microglia, classified into homeostatic and reactive states [ 89 ] and aging-related and plaque-related states [ 93 ], showed increased density in wide areas for homeostatic state microglia and reactive microglia (disease-associated microglia, according to Keren-Shaul et al. [ 89 ]) and plaque-related (aging-nonrelated but plaque-related) reactive microglia. Interestingly, both homeostatic and reactive microglia showed a dramatic increase throughout brain regions in 5xFAD mice compared to wild-type mice (Fig. 2 B). Plaque-associated microglia also showed a significant increase in 5xFAD mice, but aging-associated microglia showed no difference (Fig. 2 B). Plaque-associated and reactive microglia shared a similar set of genes (Supplementary Fig. 8 C, D). In particular, Cst7, Spp1, Ccl6 , and Axl showed remarkable increases in 5xFAD mice compared to wild-type mice for both microglial signatures. For homeostatic microglial signatures, other genes, such as Hexb, Cst3, Cx3cr1, Tmem119 , and P2ry12 , showed dramatic increases in 5xFAD mice. Of note, microglial signatures did not show differences by brain region.
Oligodendrocytes and their lineage cells classified by Marques et al. [ 66 ], which comprise mature oligodendrocytes, myelin-forming oligodendrocytes, newly formed oligodendrocytes, committed oligodendrocyte precursors (COP) and oligodendrocyte precursor cells (OPC), showed distinct distribution along the areas, mainly identified in the white matter and faintly in the thalamus and lateral hypothalamus (Supplementary Fig. 7 B). A significant difference in newly formed oligodendrocytes in the deep cortex and thalamus was observed between wild-type and 5xFAD mice, but the expression was very low, and the difference was also small. The classification according to Chamling et al. [ 87 ], consisting of oligodendrocytes, OPCs and cycling progenitors, also showed similar characteristic distributions. The oligodendrocyte signatures showed relatively little difference between wild-type and 5xFAD mice.
Astrocytes and microglia, specifically white matter-associated astrocytes, reactive astrocytes, plaque-associated microglia, and homeostatic and reactive microglia, tended to increase exclusively in the white matter in 3-month-old 5xFAD mice compared to age-matched wild-type mice. This meant that the changes with the signatures started at an earlier age and occurred around white matter, reflecting a similar result to our previous report [ 95 ] (Supplementary Fig. 9 ). The most interesting finding was that homeostatic microglia also revealed increased expression in most gray matter regions at the later stage of amyloid pathology, similar to the expression pattern of reactive microglia. Increased expression of Tmem119 (a marker for homeostatic microglia) and Cst7 (a marker for reactive microglia) in the gray matter regions, especially in the deep cortex, thalamus, hippocampus, and amygdala, was validated at the protein level in 7-month-old 5xFAD mice compared to 3-month-old 5xFAD mice (Supplementary Fig. 10 A). In addition, increased expression of GFAP, S100beta, and Ctss (markers for reactive astrocytes) was confirmed at the protein level in the deep cortex, thalamus, amygdala, and white matter regions (Supplementary Fig. 10 B). Thus, the results of verifying the protein expression level were consistent with the ST analysis results.
Finally, DEGs were explored between the groups using the MAST model [ 96 ] to find regional differences at the gene level. Of note, we considered gene abundance in addition to the log fold change of mean expression in the spots corresponding to the two groups to classify DEGs in each brain region. Based on the properties of barcode-based spatial transcriptomics, adding abundance information for the corresponding gene within one barcode can increase confidence in identifying DEGs. The spatial expression of individual DEGs in 5xFAD mice compared to wild-type mice was visually assessed by STquantool (Supplementary Fig. 11 and Supplementary Table 2 ). Venn diagrams of the significantly different transcripts per region were drawn, and the GO terms associated with the genes were visualized as dot plots to examine the differences between wild-type and 5xFAD mice. The reliability of the applied DE analysis was validated by quantitative reverse-transcription PCR (qRT‒PCR analysis) in the thalamus and hippocampus (Supplementary Fig. 12 ). Among the DEGs from the thalamus, Hexb, Lyz2, Cst7, H2-K1, Ctss , and Gfap were increased in the thalamus of 5xFAD mice compared to wild-type mice. Additionally, the detected DEGs in the hippocampus, Scg5, C1qb, Ctss, Hexb, Cst3, S100a6, Cst7, Gfap , and Lyz2 , were also significantly increased. Thus, we demonstrated that our transcriptomic approach faithfully captured changes in DE analysis. In 5xFAD mice, both the white matter and gray matter regions showed significant increases in gliogenesis- and glial cell activation-related genes. For downregulated genes-associated pathways, none were detected in the white matter, but ATP biosynthetic process and purine nucleoside triphosphate biosynthetic process were significantly decreased in deep cortex of 5xFAD mice compared to wild type. The DEG-related upregulated and downregulated pathways in other regions are listed in Supplementary Table 3 .
Spatial transcriptomic characterization of rare brain-resident or infiltrating cells in 7-month-old wild-type and 5xFAD mice
Spatial transcriptomic characterization of rare brain cells poses problems of finding the proper unique set of gene combinations for determining these rare cells residing among the confounding major cells. Unlike major cells, the distribution of which is already known, rare cells are low in number and do not have any presumed distribution. Information on the propensity (rarity) of these cells is either derived from scRNAseq studies using dissociated samples from various areas of the brain, even collected from a number of animals, or from the zoomed-in small areas observed by histochemistry. Abundance studies of rare immune cells in the brain reported that the abundance of T cells was 4/mm 3 , that of neurons was 90,000/mm 3 and that of microglia was 6,500/mm 3 [ 97 , 98 , 99 ]. Other cells such as B cells, monocytes, infiltrating macrophages, dendritic cells (DCs) either conventional or plasmacytoid, or neutrophils were counted and reported for the brain tissue as a whole because all these studies were from scRNAseq analysis using suspended cells from dissociated brain tissue.
In contrast to the previous studies that disregarded the heterogeneous distribution of rare immune cells in the brain, solid-phase spot RNA sequencing enabled genome-wide quantification and localization of transcripts, as first documented by Ståhl et al. [ 47 ]. In this method and in the now available Visium, a spot has its own count (log1p of the count ratio), which was measured by in situ capture of transcripts in the tissue. However, a spot is composed of a mixture of multiple cells, and it can be difficult to distinguish the transcripts of the rare immune cells from those of the major cell types. In line with this, the problem is to find an appropriate gene (transcriptome) combination to sort out only the specific marker transcriptomes that can discern rare cells from others. Selecting the possible key gene sets defining rare cells with the highest specificity is influenced by the choice of the input data, which are composed of participating cells [ 50 ]. For example, T or B lymphoid cells, quite unique with their high propensity for ribosomal protein genes such as Rpl or Rps , are characterized by any cell-type annotation method to yield candidate marker gene combinations. However, other major brain cells are also equipped with these protein-producing genes expressed in sufficient amounts to appear to be rare brain cells, confounding the presence/density of rare lymphoid cells in any area of the brain. Additionally, since rare immune cells are commonly investigated by combining cell sorting strategies with scRNAseq, the rare cell markers acquired from the subpopulation single-cell dataset may overlap with the major cell type markers. This caused serious overestimation, which was disclosed immediately upon visual assessment. This was also the case despite the use of the recent data available by Schafflick et al. [ 68 ] and NSForest by Aeverman et al. [ 59 ]. We adopted visual curation to exclude the frankly absurd transcriptomes as marker gene candidates and finally sorted out the rare cells with optimal marker gene combinations to compare wild-type and 5xFAD mice (Fig. 3 and Supplementary Fig. 13 ).
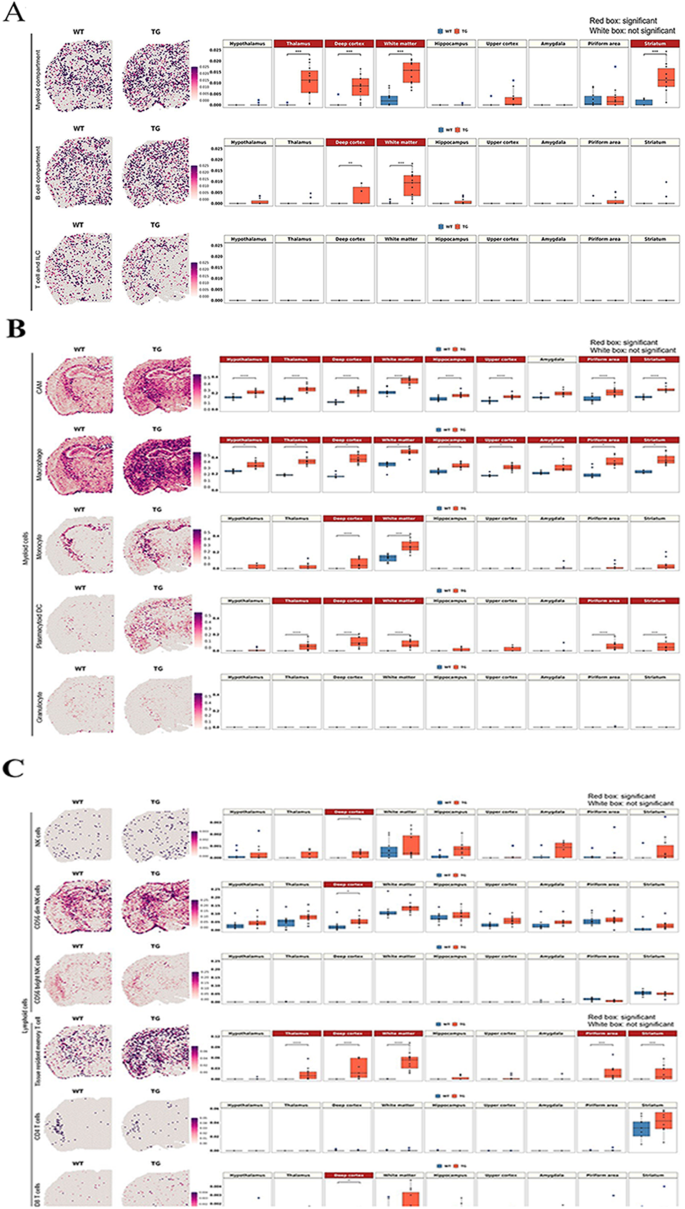
Spatial changes in the distribution of myeloid and lymphoid cell signatures in 5xFAD mice compared to wild-type mice. ( A ) Spatial pattern of the signatures of myeloid, B cell, and T-cell and ILC compartments according to the marker gene combination reported by Dominguez Conde et al. [ 71 ] (left) and the boxplot showing the average module scores (right). Each dot represents a mouse in each group. The average module score of the myeloid compartment showed a significant increase in the white matter and gray matter regions adjacent to the white matter, including the thalamus, deep cortex, and striatum. B-cell compartment signatures showed an increase in the deep cortex and white matter. In contrast, T-cell/ILC compartment signatures were low without differences between wild-type and 5xFAD mice. ( B ) Spatial pattern of the subtype signatures of myeloid cells, including CAM, macrophage, monocyte, plasmacytoid DC, and granulocyte according to marker gene combination from Schafflick et al. [ 68 ]. Notably, CAM and macrophage signatures showed the most pronounced increase in 5xFAD mice compared to wild-type mice in most of the regions. Monocytes and plasmacytoid DCs were increased in the deep cortex and white matter, and plasmacytoid DCs were further increased in the thalamus, pyriform area and striatum. ( C ) Spatial pattern of the subtype signatures of lymphoid cells, including NK and T cells, according to marker gene combination from Xiemrakis et al. [ 64 ]. In the case of the NK cell signature, a significant increase was observed in the deep cortex of 5xFAD mice, which is associated with an increase in CD56dim NK cells. Among T-cell signatures, tissue-resident memory T-cell signatures were higher in 5xFAD mice in the deep cortex, white matter, thalamus, pyriform area and striatum. The CD4 signature was explicit in the striatum in both wild-type and 5xFAD mice, but the expression was too low to show a quantitative difference between wild-type and 5xFAD mice. Bonferroni-adj. *p value < 0.05, ***p value < 0.001, ****p value < 0.0001. (WT: wild type; TG: 5xFAD mice; ILC: innate lymphoid cells; CAM: CNS-associated macrophage; DC: dendritic cells; NK: natural killer)
Immune resident cells were classified in three ways (1) using novel data by Eraslan et al. [ 69 ] (Supplementary Fig. 13 A) for tissue-specific monocyte-derived macrophages and data by Dominguez Conde et al. [ 71 ] (Fig. 3 A) for tissue-resident T cells, (2) using the data by Schafflick et al. [ 68 ] (Fig. 3 B) and markers refined using NSForest 2.0 and (3) using the data by Xiemrakis et al. [ 64 ] and refined using NSForest 2.0 by Aeverman et al. [ 59 ] (Fig. 3 C). The first two reports [ 69 , 71 ] were derived by using various tissues excluding the brain, while the other two reports [ 64 , 68 ] were derived by using brain tissues.
Defining marker gene combinations was more intricate for these rare immune resident/infiltrating cells, as they were defined by surface markers in the report of Eraslan et al. [ 69 ], in organs/tissues other than the brain, or by transcriptome signatures suited for each study. Although the data were from body tissues, not the brain, in the first approaches, as the tissue stromal cells are included in DEG analysis and assuming stromal cells might be more similar between tissues including brain, transcriptomes of major parenchymal/stromal cells coexpressing with rare immune cells were to be correctly excluded. We chose NSForest to help exclude confounding stromal tissues. Monocyte-derived macrophages include two types, according to Eraslan et al. [ 69 ]: one for immune function (MHCII+) and the other (LYVE1+) for vascular integrity and repulsion of infiltrating immune cells. For immune function, specifically for the brain, conventional DCs with MHCII + cells were found to be effective for antigen presentation by adaptive immune cells (T cells and B cells), although border-associated macrophages or microglia were not [ 100 ]. We asked whether this surface marker-defined characterization can be translated to mouse (5xFAD) amyloidosis using the signature of MHCII+-related immune-functioning macrophages and LYVE1+-related integrity-charged macrophages described by Eraslan et al. [ 69 ] (Supplementary Fig. 13 A). Integrity-charged macrophage signature scores were not different among the groups of 7-month-old wild-type and 5xFAD mice in all regions of brain. Spot signatures of immune macrophages were higher in 5xFAD mice than in wild-type mice in the white matter.
Signature gene combinations used in the cross-tissue immune cell analysis by Dominguez Conde et al. [ 71 ] revealed no difference between wild type and 5xFAD mice for T cells and innate lymphoid cell T/ILCs. However, significant differences were found among these mice for myeloid compartment cells in the white matter and the gray matter regions adjacent to the white matter, including the thalamus, deep cortex, and striatum and for B cells in the deep cortex and white matter (Fig. 3 A).
This result came from the following stepped analysis including the curation procedure. At the first step of curation, individual transcriptomes belonging to the three compartments described by Dominguez Conde et al. [ 71 ] were examined visually for their distribution/intensity, and several transcriptomes that were already reported in the literature as signatures for major brain cells and their reactive states were removed, which excluded the background effects of abundant brain cells, eventually yielding marker gene combinations for the three compartments and their cell types. Having removed (1) Cx3cr1 and Tyrobp (microglia) from T/ILCs, (2) Ighm (Scheurer et al. [ 101 ] for neurons) and C1qa (microglia) from the B-cell compartment, and (3) Trem2 (microglia), Clu (astrocytes), Selenop (microglia, astrocytes, oligodendrocytes), Igf1 (reactive microglia and reactive astrocytes), C1qa and Cx3cr1 from the myeloid compartment, the scores of T/ILCs were still not different between wild-type and 5xFAD mice, and the scores of B-cell or myeloid compartments revealed a slight but significant increase in 5xFAD mice compared with wild-type mice. Individual variations within 5xFAD mice could also be recognized upon visual assessment. For individual genes for T/ILCs, localization was prominent for Cd4 (striatum) and showed little difference regardless of abundance ( Slc4a4, Spry2, Ncam1 , and Pcdh 9 are abundant), and no difference was observed except for Pdcd1 (smaller cell fraction in various T cells including Trm/em_CD8 according to Dominguez Conde et al. [ 71 ]), which was slightly increased in 5xFAD mice. For the B-cell compartment, the difference between mouse groups, if any, was presumed to be due to Itgax and Fcrls , both of which were related to aging-associated B cells, and Fcrls was related to memory B cells and plasma cells/plasmablasts. For the myeloid compartment, the difference in scores among mouse groups was contributed by Tyrobp, Lyz2, Fcer1g, C1qc , and Apoe , all of which are related to various types of tissue-specific macrophages and classical/nonclassical monocytes (Supplementary Fig. 14 ).
The second one by Schafflick et al.’s data [ 68 ] was tested for either the marker gene combination recommended by Schafflick et al. according to their supplementary table (log fold change: LFC > 0.5) for 12 border cell leukocytes (including microglia) or the marker gene combination curated by NSForest upon inputting their data. Schafflick’s own data yielded obviously too high intensity for CD4 and CD8 T cells among 12 border-associated leukocytes, such as B1, B2, CD4 T, CD8 T and NK cells, microglia, CNS-associated macrophages (CAM), macrophages, monocytes, myeloid DC (mDC), plasmacytoid DC (pDC) and granulocytes. When we surveyed the constituents of the tentative marker transcriptomes for these inappropriate signatures, ribosomal genes (many isoforms of Rpl and Rps ) were presented as false positive markers of CD4 T and CD8 T cells. This misclassification of marker genes is assumed to be caused originally by the fact that the parenchymal and border leukocytes were included after their selection for CD45 positivity, meaning that they could not exclude the differential expression of these cells from the major brain cells, including stromal cells. Individual transcriptomes per spot were easily observed to disclose whether we chose the highest LFC with adjusted p values for determining marker genes, Ighm for b1 cells (also found in the cortex not related to B cells) [ 101 ], H3f3b (histone protein also nonspecific for the brain) for b1 cells, Stmn1 for b2 cells (rather brain-wide expression), Dut (enzyme for nucleotide and ubiquitous, including brain cells) for B cells and many similar examples (Supplementary Fig. 13 B). Although DEG analysis depends upon the input data composition, we tried NSForest on Schafflick’s data and obtained a better marker gene combination. This Schafflick/NSForest analysis yielded improved intensity matching considering the prevalence of cell populations in the brain parenchyma except for b2 cells (still too dense due to Tuba1b (tubulin related)) and CD4 T cells (depending heavily upon one transcriptome Trbc2 (T-cell receptor beta constant 2 but also expressed in the cortex)). The other 10 cell signatures appeared to represent the cell intensity/distribution correctly; however, they also included nonspecific and dense Apoe for CAM, dense Cst3 for macrophages, Mal (Myelin And Lymphocyte Protein, implying its localization both in lymphocytes and myelin of neurons) for monocytes, and Tyrobp (in association with Trem2 , a well-known marker for microglia) for both monocytes and pDCs. Upon the application of NSForest, 6 to 10 marker genes were obtained, and zero to three genes were adjusted (kept or removed, meaning curated by operators’ consensus). The application of curated gene combination to our ST samples revealed that CAM and macrophages showed the most pronounced increase in 5xFAD mice compared to wild-type mice globally throughout the brain regions (Fig. 3 B). Additionally, pDCs showed increases in the white matter and some gray matter regions. However, the transcriptome density of DCs was considered inappropriate, as it yielded much higher intensity along the entire brain, considering that DCs occupy only 0.14% of myeloid/lymphoid cells of the brain and border, including microglia (0.8% among myeloid/lymphoid cells excluding microglia) [ 68 ].
Among lymphoid cell signatures, an increase in tissue-resident memory T cells was inferred in 5xFAD mice compared to wild-type mice (Fig. 3 C and Supplementary Fig. 14 ). However, it is necessary to consider the technical limitations of spot-based transcriptomic analysis for evaluating rare brain cell signatures. It is still unclear which genes specifically define rare cells, while gene combinations may overlap with major brain cells on ST brain imaging.
Using a single gene as a marker would be better and more convenient for designating rare cell types. It was possible to designate infiltrating macrophages derived from circulating monocytes originating from BM (Supplementary Fig. 15 ). The CD11c surface marker and its gene Itgax were used as markers for these cells. Resident T cells were suggested to be CD73 positive, and its gene Nt5e was identified by Fang et al. [ 102 ]. CD56 bright and its gene Ncam1 are considered to be circulating and immature NK cell markers but are also highly expressed in neurons [ 103 , 104 ]. Perivascular macrophages cause a great problem in distinguishing them from microglia, and Lyve1 is the discriminator of pvMϕs and microglia ( Sall1 ) [ 105 ]. Similarly, for brain major cells, Trem2 and Tyrobp were suggested to be conjoint markers for microglia, and Cspg and Olig2 were expected to represent OPCs, not any other cell types. Homeostatic microglia could have been defined by Sall1 ; however, a transgenic mouse study [ 105 ] found that Hexb was the better marker for authentic microglia than Sall1 . The importance of Aif1 (IBA1) as an activated microglial marker and of Gfap as an activated astrocyte marker was disclosed to be nonspecific or at least subtype specific, respectively. Once a marker was well defined for designating rare cell types well discriminated from major brain cells, including microglia and perivascular space (pv) macrophages (and submeningeal macrophages), then that marker in a spot could disclose the fact that the gene signature of that spot might be from the rare cell of interest, but it does not mean that the signature was not from the rare cells if no signal was observed. Genes widely expressed over all cell types but with specific isoforms could be used to define the cell types, and Prdx (for peroxiredoxin) was one of the examples ( Prdx6 and Prdx2 for astrocytes, Prdx4 for microglia and Prdx1 for oligodendrocytes) (Supplementary Fig. 16 ).
To validate the results obtained from the cell signature score based method of measuring cell type abundance, we performed the cell type deconvolution analysis and compared the results between the two methods. The cell type deconvolution method captures the gene expression patterns of cell types from the single-cell reference dataset and predicts the cell type composition in the ST spot, which is a mixture of multiple cells. We performed the analysis mainly for microglia and infiltrating immune cells, which showed significant changes between 5xFAD mice (TG) and wild-type mice (WT) in the signature score-based method. For microglia, the proportions of both homeostatic and reactive microglia were globally increased in the gray and white matter regions of TG mice, which was consistent with the results obtained from the cell signature scores (Supplementary Fig. 17 ). Minor immune cells, including myeloid cells such as macrophages, monocytes, and dendritic cells, were also upregulated in multiple gray and white matter regions of TG, and the results were similar to those obtained using cell signature scores (Supplementary Fig. 18 A, B). However, for lymphoid cell types such as innate lymphoid cells, natural killer cells and T cells, which are rare, the patterns of change between the two methods were inconsistent for a few gray matter regions, while the biological effect may be small due to very low cell abundance (Supplementary Fig. 18 C, D). Overall, the results suggest that cell signature scores derived from curated markers are an accurate and reliable measure of cell abundance for the relatively common cell types, while rare cell types require special attention in interpretation.
Improvement of behaviors with much variation by immunomodulatory therapy of anti-CD4 antibody and NK supplements in the 5xFAD AD mouse model
During a preliminary behavioral study to prove the effect of aducanumab, pretreatment with anti-CD4 antibody caused a larger degree of changes in alternation scores in the control animals (meaning higher improvement in the group of animals treated with anti-CD4 antibody) [ 29 , 30 ]. Three batches of several animals with anti-CD4 antibody treatment reproduced the previous groupwise behavioral improvement with similar variation (67.7% ± 18.4%) at 7 months of age in 5xFAD model mice (Fig. 4 ). We assumed that anti-CD4 antibody treatment modulated the systemic adaptive immune system, as transgenic insertion of five types of mutated human APP/PS1 genes would have caused immune disturbance due to their presence in the mouse chromosome. The presence of human mutated genes would have resulted in brain-immune interaction dysfunction as well as plaque-prone amyloid burden in animals. Spatial transcriptomic analysis was considered to reveal the eventual response of brain cells, either major or rare resident and infiltrating immune cells, if any.
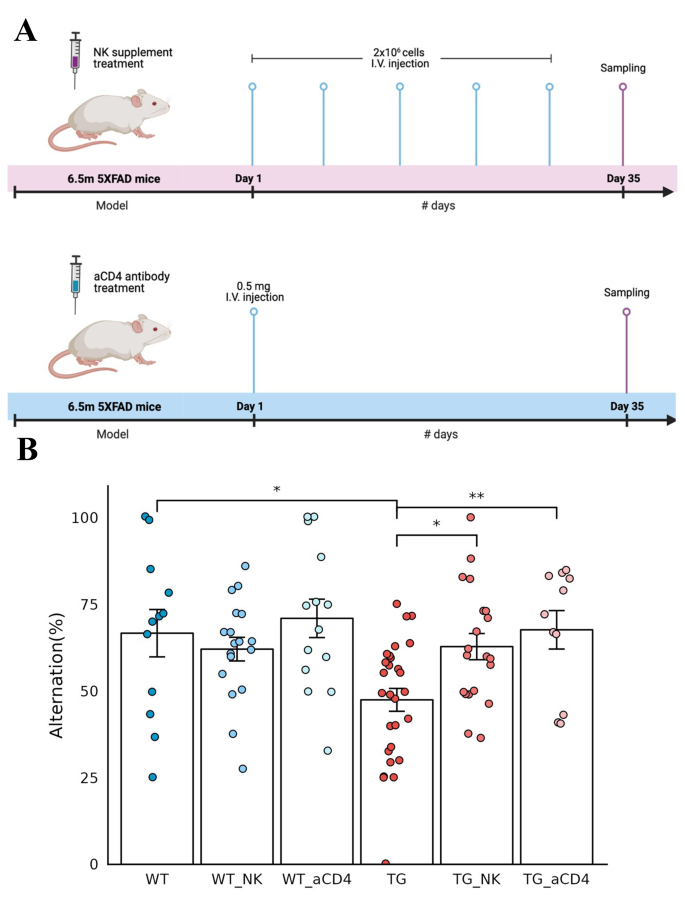
Improved behavior after intravenous administration of NK cell supplement and anti-CD4 antibody in 5xFAD mice. ( A ) Timeline of the experiments for intravenous NK cell supplements (upper) and anti-CD4 antibody (lower) administration in 6.5-month-old wild-type and 5xFAD mice. NK cells (2 × 10 6 cells/injection) were administered once a week for a total of five times, and anti-CD4 antibody (0.5 mg/injection) was administered once as a single injection. After a month, behavior analysis was performed, and brain tissue samples were obtained for spatial transcriptomic brain imaging analysis. ( B ) The behavioral function of exploring new environments was examined using the Y-maze test and expressed as alternating percentages. Each dot represents a mouse in each group. The alternation rate was decreased in 5xFAD mice compared to wild-type mice, with much variation at this age in wild-type and 5xFAD mice. The alternation percentage score of 5xFAD mice increased significantly after injection of NK cell supplements and anti-CD4 antibody treatments compared with that of 5xFAD mice without treatments. Wild-type mice also showed variation; however, their alternation scores were not different between the no treatment and either treatment group. Wilcoxon *p value < 0.05, **p value < 0.01. (aCD4: anti-CD4 antibody; WT: wild type; TG: 5xFAD mice; WT_NK: NK cell-treated wild type; WT_aCD4: anti-CD4 antibody-treated wild type; TG_NK: NK cell-treated 5xFAD; TG_aCD4: anti-CD4 antibody-treated 5xFAD)
In another preliminary study with APP/PS1 model mice using the water maze with expanded NK cell supplements derived from the spleen of wild-type BALB/c mice, anecdotal cases of behavioral improvement were observed (data not shown). Three batches of allogeneic NK cell supplements, as 5xFAD mice are on a B6 background, reproduced the behavioral improvement of alternation scores on Y-maze tests on average, however, with much variation (Fig. 4 ). Much variation in both the anti-CD4 antibody treatment study and NK supplementation study indicated that 5xFAD mice at 7 months of age were undergoing their own course of aggravation of the pathological changes of Aβ oligomer insults and amyloid plaque burden, resulting in later pathological and behavioral dysfunction at approximately 12 months of age or later. Spatial transcriptomic analysis was also considered to reveal the regional and cell-type specific changes of transcriptomes of major and rare brain cells corresponding to each individual mouse’s degree of behavioral dysfunction in the NK supplement-treated group.
Regional cell-type/state-specific transcriptome changes in 5xFAD mice compared with wild-type mice after intravenous administration of NK cell supplements
Three mice with higher alternation scores on the Y-maze test were selected for both the saline-treated and NK cell supplement-treated groups (Supplementary Fig. 19 ). Coronal/sagittal brain sections of these mice were subjected to Visium analysis. Each group was paired to the same plates so that the batch effect of the read per slide would be minimized. Using 30,000 to 50,000 counts per mouse, we retrieved the count data, which were normalized for their total count, and log1p of the ratio data were used for further analysis. Spatial clustering allowed anatomical segmentation to yield 14 regions with almost similar sizes (Supplementary Table 4 ). Cell type- and state-specific marker gene combinations were also used to analyze cell-specific and/or cell state-specific changes after NK cell supplement treatment. For the 5xFAD case with NK cell supplements, one mouse with a very high behavior score was chosen as the ‘behaviorally best’ representative of the group, and another mouse with a very low score was chosen as the ‘behaviorally worst already at 7 months of age’ representative. This essentially allowed us to examine the transcriptomic changes according to the behavioral impairment of the 5xFAD mice at the age of 7 months. NK cell supplements contributed at least to the widening of the distribution of scores of behavioral impairments at this middle age.
GABAergic Sst subtype neurons showed a significant decrease after NK supplementation in the amygdala, which showed an abnormally increased signature in 5xFAD ( n = 11) compared with wild-type mice ( n = 10) (Fig. 5 A). Among the Sst neuronal signatures, Sst, Tac1 , and Nr2f2 showed dramatic decreases in the amygdala after NK cell supplement administration in 5xFAD mice, but there were no distinct differences in the other regions (Supplementary Fig. 20 A, B). The Sst-expressing neurons in the cortex are known to contribute to modulating cortical circuits, synaptic plasticity and maintaining spatial working memory [ 106 ]. Patients with AD exhibited low Sst expression in the cortex and hippocampus. However, the function of Sst-inhibitory neurons in the amygdala remains poorly understood [ 107 ]. No significant difference was found either in the mature neuron score or in any other subtype of neurons other than Sst neurons between 5xFAD mice without NK supplements and those with NK supplement treatment. Thus, normalization of excitatory and inhibitory neuronal imbalances in the amygdala may improve behavior function. Further investigation of the neurons in the amygdala region could play an important role in understanding the pathology of AD and in providing therapeutic directions. Additionally, the NK cell signature tended to increase after administration of NK cell supplements exclusively in the white matter region of 5xFAD mouse brains (Supplementary Fig. 21 A). The change in the module score level was not observed with the anti-CD4 antibody treatment, which was in contrast with the change after NK cell supplement treatment (Supplementary Fig. 21 B). The signatures of astrocytes, microglia, and oligodendrocytes did not show any difference. Additionally, no difference in rare brain cells, either resident or infiltrating, was observed (Supplementary Fig. 22 ). The biodistribution of 99m Tc-HMPAO-labeled NK cells was examined using SPECT/CT to determine how systemically injected NK cells caused changes in the brain (Supplementary Fig. 23 ). Within 1 h after injection, the labeled NK cells were mainly taken up by the liver, and this radioactivity decreased gradually by 16 h. Of note, no definite brain uptake of the labeled NK cells was observed with the resolution of SPECT/CT images. Thus, NK cells may have caused changes in brain cells at the transcriptional level indirectly via cytokines or other secretory factors released by NK cells and/or inherent peripheral immune cells influenced by supplemented NK cells.
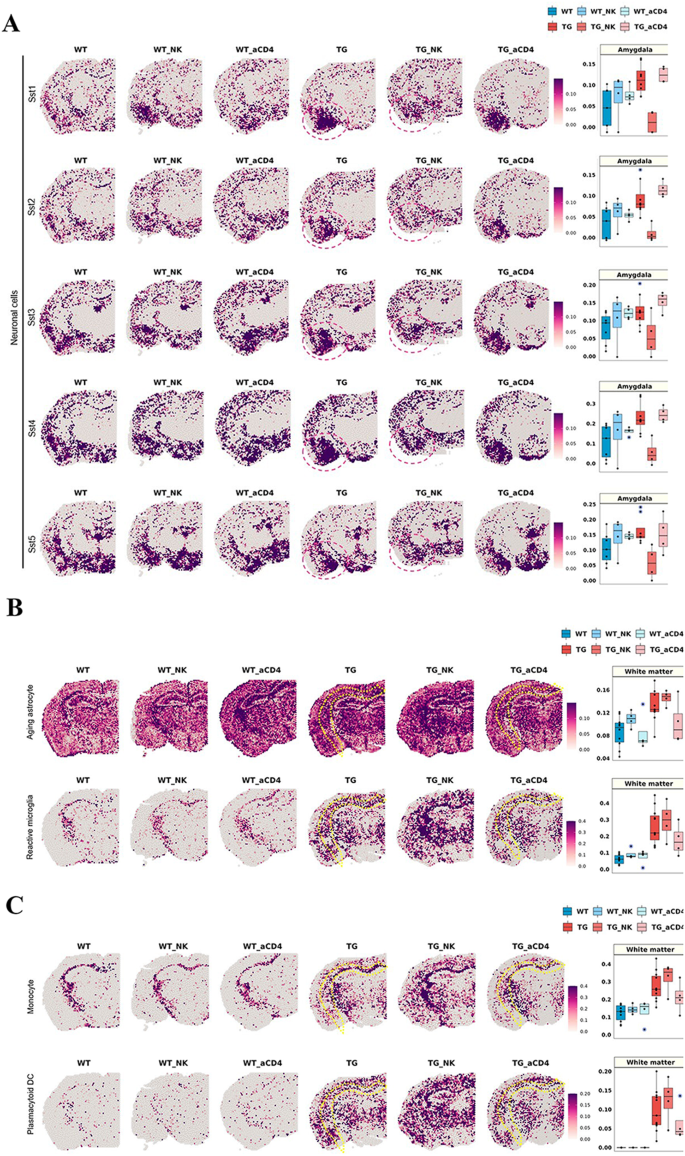
Brain region-specific transcriptome changes in cell signatures after NK cell supplementation and anti-CD4 antibody treatment in 5xFAD mice. ( A ) Spatial pattern of the signatures of somatostatin (Sst)-inhibitory neuronal signatures (Sst1, Sst2, Sst3, Sst4, and Sst5; left) and the boxplot showing the average module scores in the amygdala (right). Each dot represents a mouse in each group. The average module scores of Sst neuronal subclasses tended to decrease specifically in the amygdala after administration of NK cell supplement in 5xFAD mice. Interestingly, the Sst neuronal signatures, which were increased in expression in the amygdala of 5xFAD mice, were decreased to the expression level in wild-type mice by NK cell supplements. In contrast, the module score was not different between the no treatment and anti-CD4 antibody treatment groups, while there were differences between the no treatment and NK cell supplement groups. ( B ) Spatial pattern of the signatures of state-specific glial cells (aging astrocytes and reactive microglia; left) and ( C ) immune cells (monocytes and plasmacytoid DCs) and the boxplot showing the average module scores in the white matter (right). The expression of state-specific subtypes of glial cell and immune cell signatures, which showed a significant increase in 5xFAD mice compared to wild-type mice, tended to slightly decrease in the white matter after anti-CD4 antibody treatment. Considering that NK cell supplementation showed no appreciable differences in glial cell and immune cell signatures, anti-CD4 treatment effects on these cell subtypes of state-specifics looked real. Expression, however, did not decrease to the level observed in wild-type mice. In summary, NK cell supplementation and anti-CD4 antibody treatment affected different state/type-cell signatures and brain regions, respectively. (aCD4: anti-CD4 antibody; WT: wild type; TG: 5xFAD mice; WT_NK: NK cell-treated wild type; WT_aCD4: anti-CD4 antibody-treated wild type; TG_NK: NK cell-treated 5xFAD; TG_aCD4: anti-CD4 antibody-treated 5xFAD; Sst: somatostatin; DC: dendritic cells; NK: natural killer)
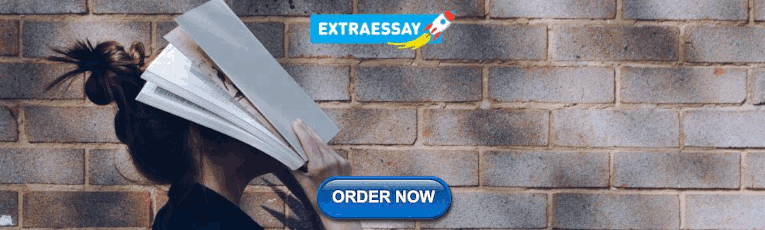
Regional cell-type/state-specific transcriptome changes in 5xFAD model mice compared with wild-type mice after intravenous anti-CD4 antibody treatment
Three mice in the anti-CD4 antibody treatment group were selected, and their coronal brain sections were plated on Visium slides. The frame of sample distribution on the quadrants of each Visium slide was the same as above for NK cell supplement treatment. Further analysis of transcriptomes per spot and spatial clustering and designation of transcriptomes to the approximately 3,000 spots were also the same.
Among major brain cells, state-specific glial cells, such as aging astrocytes and reactive microglia, which showed a significant increase in 5xFAD mice compared to wild-type mice, showed a slight decrease exclusively in the white matter after administration of anti-CD4 antibody in 5xFAD mice (Fig. 5 B). In the gene combination of aging astrocytes, the expression of Lgmn, Gsn, Mt1, Fcrls , and Hexb was noticeably decreased in the white matter after anti-CD4 antibody administration in 5xFAD mice, and expression decreased slightly in the other regions (Supplementary Fig. 20 C, D). In the reactive microglial signature, Cst7, Spp1 , and Cd9 showed a decreased pattern throughout the region, while other genes, such as Axl , Csf1 , and Ccl6 showed decreased patterns mainly in the white matter (Supplementary Fig. 20 C, D). Interestingly, the CD4 T-cell signature tended to decrease slightly in the deep and upper cortex (striatum) after anti-CD4 antibody treatment (Supplementary Fig. 21 B). However, mature neuronal signatures showed typical and very similar patterns of distribution between 9 major brain regions and within each region irrespective of whether the sample was from wild type, 5xFAD, wild type with anti-CD4 antibody treatment or 5xFAD with anti-CD4 antibody treatment mice (Supplementary Fig. 22 A). No significant differences in other types of glial cells, including white matter-associated astrocytes, reactive astrocytes, plaque-associated microglia, homeostatic microglia, and oligodendrocytes, were found, meaning that all 10 wild-type mice and all 11 5xFAD mice could not be differentiated between no treatment and anti-CD4 antibody treatment (Supplementary Fig. 22 B, C). The difference between wild-type and 5xFAD mice was sustained but did not reveal any dramatic effect of anti-CD4 antibody treatment.
Rare brain cells, resident or infiltrating, were distinguished between the no treatment group and the anti-CD4 antibody treatment group. The expression levels of monocytes and pDCs, which showed a significant increase in 5xFAD mice compared to wild-type mice, tended to slightly decrease only in the white matter after anti-CD4 antibody treatment (Fig. 5 C). In both monocyte and pDC signatures, the expression of Tyrobp was dramatically reduced throughout the brain, whereas S100a4, S100a10 , and Mal in the monocyte signature showed decreased expression patterns exclusively in the white matter after anti-CD4 antibody treatment. A reduction in the expression of individual genes by the anti-CD4 antibody was mainly identified in the white matter region (Supplementary Fig. 20 C, D).
While NK cell supplementation showed no appreciable differences in immune cell signatures, the fact that anti-CD4 antibody treatment showed effects on subtypes of immune cells is noteworthy. However, the expression level was not decreased to that observed in wild-type mice.
Methods to scrutinize spatial cell-type and cell-state specific changes upon the platform of setting norms and characterization of abnormality of a test mouse
As spatial distribution is critical for characterization of a new mouse specimen for their status of normalcy, pathology, and response to therapy, the specimen can be on every section, but on a limited number of coronal sections (per monkey samples in a report by Chen et al. [ 55 ]) or sagittal sections. To acquire representative information regarding mouse groups, we combined multi-individual mouse sections to yield the apparently correct spatial segmentation. Each region was then prepared to present their norms for various scores for cell types, cell states and response to the tentative immunomodulatory drugs. We tried to establish methods to reveal the regional cell-type/state-specific norms and their probable changes by drug intervention. We set up norms for normal mice using wild type mouse data genotype, and then the effect of the age or the influence of drug treatments were characterized. For example, the presence of anomaly were examined for individual mice according to their disease states (5xFAD mice of certain age with diverse behavior/Aβ abnormality, P301L mice with no behavioral/pathological abnormality) and the effect of therapy (anti-CD4 antibody or NK cell treatments).
Cell types should have been annotated to the then-best knowledge of the scientific community based on the resource reports in the literature up to the date of this investigation run by trial-and-evaluation and then the choice; for example, for neurons and neuronal subtypes, Hodge et al.’s report [ 75 ] was adopted as is or after NS Forest [ 59 ] to define 20 GABAergic neurons and 12 glutamatergic neurons. Available data were downloaded from specific sites or supplementary tables of each report. Thus, for example, Scng-VIP neuron subtypes described in a more recent report by Bugeon et al. [ 57 ] were ignored but later can be reanalyzed with the current Visium data by specifying their markers along the regions segmented after integration of slides using RPCA. For astrocytes and microglia, the reactive state signature was surveyed by scrutinizing the counts of transcriptomics at each spot according to the reports by Keren-Shaul et al. [ 89 ], Friedman et al. [ 91 ], Grubman et al. [ 93 ] and others. Coexpression of the same transcriptome by astrocytes and microglia, such as Apoe, Gfap, Tspo and others, was removed from the tentative marker gene combination. The same procedure was performed for astrocytes and oligodendrocytes or microglia and oligodendrocytes. Oligodendrocytes and their lineage cells did not have a ‘reactive’ transcriptome signature. Signature transcriptomes between reactive and homeostatic microglia (and astrocytes) were also surveyed for their conjoint expression between both states. Homeostatic transcriptomes were designated to exclude the signature of reactive transcriptomes and vice versa, referring to literature reports by Prinz et al. [ 108 , 109 , 110 , 111 , 112 , 113 , 114 ], and Kim et al. [ 105 ], so that Sall1 and Hexb were used to measure the abundance of microglia as each microglia express these genes constitutively while assuming that these transcriptomes did not increase in quantity per microglia when reactive [ 105 , 113 ]. As spatial transcriptomic imaging using Visium yielded a linear (semi)quantitative (due to log1p transformation for further processing using Seurat 4.1)) metric, no fractional presentation was adopted to disclose that homeostatic microglia were increased in quantity of signature per spot in 5xFAD mice compared with wild-type or P301L mice.
Quantification was performed for 9 regions (hypothalamus, thalamus, deep cortex, white matter, upper cortex, hippocampus, amygdala, piriform area, and striatum) for major cell types, including neuron subtypes, reactive and homeostatic glial cells, astrocyte subtypes, oligodendrocyte lineage cell subtypes and rare resident/infiltrating immune cells. For regions, for example, white matter-associated astrocytes or white matter-localized microglia were quantified and correlated with white matter-localized oligodendrocyte lineage subtypes. Dense and thus intense quantities of mature oligodendrocytes could be compared among mouse groups. In contrast, diffuse and sparsely distributed rare immune cells were found in three compartments by Dominguez Conde et al. [ 71 ], four types by Xiemerakis/NSForest [ 59 , 64 ], and 12 types by Schafflick et al. [ 68 ]. Tissue resident macrophages by Dogra et al. [ 115 ] and Eraslan et al. [ 69 ] were also quantified for intensity to yield the difference for each region among mouse groups. The Wilcoxon rank-sum test was used to determine the significance between pairs of groups (uncorrected p value or corrected by three for paired group comparisons). Beyond group comparison, an anomaly detection procedure (or confirmation of normalcy meaning no difference found on any regional, cell-type, cell-state specific signature by density per region) was performed for each mouse.
Once a region and cell type and its state were found, we performed DEG analysis to find the transcriptomes of interest in each brain region. Then, the association of discovered genes with biological pathways was examined using an overrepresentation test based on evidence-driven databases. This was to determine the significance of the found transcriptome designating their functional role in pathology (amyloid pathology or tauopathy, glial cell dysfunction, etc.), physiology (aging) and their participation in the response to tentative immunomodulatory therapy. Considering the diversity of behavior improvement after anti-CD4 antibody and NK cell supplement treatments and the unpaired nature of the Visium study, we could only detect the treatment effect (and non-effect) of the transcriptome signature of regional cell types/states upon treatment per individual. Transcriptomes of the marker gene combination that we used were all checked for their individual transcriptomes to elucidate key transcriptomes for specifying type/state characteristics or therapy effects. We also tried to assess their individual contribution to this specification to find one, two or more distinctive transcriptomes to predict their presence in each spot. This means that curation by operator in addition to the readymade Wilcoxon, logistic, or NSForest methods was used in at least two steps, first to choose a seemingly optimal combination ruling out cross-expressing, background, or confounding genes and then at last to find the succinct combination of transcriptomes for cell-type/state annotation or if any, the sole transcriptome (Supplementary Fig. 2 ). The above pipelines for dissecting cell-type- and cell-state-specific regional transcriptomic changes can be readily implemented with our in-house application STquantool, which facilitates the visualization of spatial gene expression and enables quantification across multiple transcriptomic datasets.
In this investigation, we used ST for its superiority over scRNAseq/snRNAseq to localize the specific transcriptomic signature of cell type or cell state in almost 5 thousand spots, among which 3,000 or more spots harbored either coronal or sagittal sections of brain tissue. Before going further to use this transcriptomic signature to disclose the effects of novel but unproven neuromodulatory treatments, we trimmed the method of the use of this Visium-based ST imaging to elucidate regional, cell-type/state-specific changes. The method for choosing one or two optimal transcriptomic marker combinations among so many possible combinations was adjusted to yield the best contrast between cell types/subtypes using the literature resources and our in-house method of curation. A simple and easy method to sort out the candidate transcriptomes was set up to ensure that we found the best cell type/state annotation methods for either abundant brain cells or rare immune cells. The challenge was to separate 4 or more major brain cell types and their subtypes with transcriptome combinations and to define rare immune cells for their exact propensity and distribution/location. Stahl et al.’s [ 47 ] suggestion of counting the transcriptomes per spot using the original ST Visium methods and Tirosh et al.’s [ 44 ] approach to generate cell signature scores based on the curated marker genes and comparing them between mouse groups with genuine or sham treatments worked well for this endeavor. We overcame the problem of high dependence of this endeavor on the choice of tentative marker gene combinations varying upon the diverse input data derived from the preliminary DEG studies using single-cell data of brain tissues [ 67 , 68 , 108 ] and even tissues other than brain tissues. Assessing the sophisticated use of the public database and scrutinizing the individual transcriptomes visually by the operators (neuroimaging experts) were essential. Curation by operators is heuristic at best and is surely subject to operator arbitrariness; however, this was eventually the key step to enhance the authenticity of the observation of large number of cells (2 to 10 per spot) admixed in spots and a dozen specimens from individually different but syngeneic mice. From the neuroimaging perspective, integrated single spot imaging (100 µ x 100 µ x 10 µ) containing an average of 5 cells (2 to 10) in each unit domain did not have a significant batch/individual variation effect to confound further analysis, as we observed dozens/hundreds of spots at the same time, and the batch effect was corrected during sample integration. With this visual investigation, we soon became confident that spatial transcriptomic brain spot imaging with visual assessment and its quantitative analysis using the framework of voxel (spot) imaging of mouse/human brains was suitable for the evaluation of the effect of certain drugs/treatments for disease-course modification in dementia mouse models.
The transcriptomic signature of brain cells could clearly segment every section from the mice, regardless of disease or treatment status, taking advantage of 22,000 or more transcriptomes per cell to identify the cell type/state with thousands of variable transcriptomes. Unlike functional neuroimaging such as functional magnetic resonance imaging (fMRI) or positron emission tomography (PET), which needs coregistration and segmentation considering individual variation for further analysis, the segmentation of neuroanatomical entities on Visium could be performed without any more assumptions, except that functional regional entities could be determined by transcriptomes belonging to spots and their conglomerated spots make explicit functional regions. An eccentric case of regionally remote but similar transcriptome composition was observed in that the cortical amygdala and subcortical septal lobe were categorized as the same cluster on sagittal section, but excluding this exception, all the other spatial clusters were within the expected anatomical border definition (https://connectivity.brain-map.org/3d-viewer?v=1&types=IMAGEPLANE&IMAGEPLANE=imageplanes). Thus, spatial or regional representation of characteristic changes related to pathology and treatment response could then be described and quantified. Finding marker gene combinations to define the spots as belonging to certain functional regions of interest then could be achieved by finding the optimal or best combination, which would be appropriate and succinct.
Determination of the best annotation of neurons and other major brain cells was initially dependent upon previous reports mainly derived from nonspatial scRNAseq/snRNAseq analysis [ 63 , 73 , 80 , 116 ]. In these previous studies, the spatially expected designation of cells was suggested as a success of cell clustering, raising concerns that there was no gold standard information regarding their true location; nevertheless, the cell clustering and annotation allows assignment of regional identity of brain cells based on anatomical region-specific marker genes. ST brain imaging obviated this concern. In ST imaging, however, there remain two major problematic ambiguities for spatial clustering and cell type/state identification per spot. The first one is spatially agnostic annotation by transcriptome signature, which previous researchers tried to solve by sampling regions of brain such as posterior isocortex, hippocampus (or hippocampal formation), striatum, thalamus and hypothalamus, etc., in the reports of Saunders et al. [ 65 ] or Chai et al. [ 117 ]. This problem was easily solved by ST imaging using Visium of 3 to 5 thousand spots, which allows capturing transcriptomic changes across the broad area of the brain. Now, imaging with a resolution of 100 µ x 100 µ on 2D is available, allowing easy segmentation; this differs from fMRI/PET in that the huge multiplexing capability of ST brain imaging allows almost infinite reanalyses using combinatorics. The second is cell/state identification per spot by using the transcriptomic signature of the marker gene combination determined by previous DEG studies using detached and sometimes surface-marker-sorted brain cells. When scRNAseq/snRNAseq was used for detached brain cells to determine the effect of drug/treatment on those brain cells, lack of spatial localization was the major hurdle blocking the understanding of the role of any treatment. In situ hybridization of immunohistochemistry complemented transcriptomic global/regional brain signatures to address this, but without reassuring results to explain the therapy effect. ST brain imaging solved this problem. As shown in this study, ST brain imaging is equipped with the expression profile per spot for the entirety of genes of the individual cells localized on each spot, and the data could be analyzed in an unsupervised fashion without any assumption or in appropriate cases by using a priori knowledge derived from the literature resources of scRNAseq/snRNAseq. Considering the challenges and difficulties in overcoming these problems, we streamlined the use of visual reading by expert operators called curation. The steps required for curation were kept minimal and practical, and it was performed initially to exclude nonspecific and cross-expressing transcriptomes between major cells, and finally to exclude cross-tissue, stromal cell-dependent and confounding background signatures. It would have been better to base curation on individual transcriptomic features of any types of cells for their association with disease states or drug/treatment responsiveness.
To tackle these problems, we asked how we could use individual mouse ST brain imaging data to determine the disease states, which are variable even in syngeneic animals, and the variable treatment responses affecting major and rare brain cells. Taking advantage of the automatic segmentation results for groups of individual mouse specimens, irrespective of section planes and stereotaxic coordinates, we tried to individualize the transcriptomic features of each individual specimen compared with the norms we constructed. Comparing regional, cell type/state-specific transcriptomic signatures using visual and quantitative decisions of an individual mouse with norms was performed. This analysis method allowed for the individuation-based evaluation of animals for their behavior correlates. We were able to obtain and reproduce a wide variety of behavior metrics, which is in this study included the alternation score on the Y-maze; the Y-maze alternation scores of 7-month-old wild-type mice ranged widely as well as those of 5xFAD mice, but those of 8.5-month-old wild-type mice converged with smaller variation to lower values, meaning commonly poorer performance at this age even in wild-type mice. After anti-CD4 antibody treatment, the variation was sustained with a slight improvement in their average scores (Fig. 4 B). After NK cell supplement treatment, variation was also sustained, with slight improvement in their average scores (Fig. 4 B). We assumed that these behavior variabilities are the keystone for proving the feasibility of tentative novel immunomodulatory treatments and that we would find that the mouse behavior scores concord with the transcriptomic signature [ 57 ]. NK cell-treated 5xFAD mice with higher Y-maze alternation scores definitely showed that their amygdala GABAergic Sst neuronal subtypes decreased in intensity (Fig. 5 A). This decrease (or increase, if any) did not prove the efficacy of NK cell supplement treatment on 5xFAD mice but definitely disclosed that transcriptomics of the neuronal subtype of that region were correlated with the degree of behavior impairment. More importantly, this meant that many other neuronal subtypes, other homeostatic or reactive glial cells and their subtypes, did not show any change in intensity over all the regions examined on these sections despite the improved behavior score. Anti-CD4 antibody treatment recapitulated only a slight decrease in specific immune cell signatures in the white matter, but beyond this finding, no other discovery of drug-responsive transcriptomic changes in any region or in any cell type or cell state was found. This was even observed on individual interpretations both visually and quantitatively for each mouse (Fig. 5 B and Supplementary Fig. 21 ). We could say that anti-CD4 immunoglobulins did not affect the transcriptomic signatures of major brain cells (on this single coronal section), and this was also the case with rare immune cells. Due to the lack of Y-maze score measures of the anti-CD4 antibody-treated wild-type and 5xFAD mice, behavior correlation could not be reported here.
The interpretation of rare immune cell signatures for the localization of immune cells presented different challenges from major brain cells. First, due to the intrinsic limitations of Visium, rare cell transcripts may not be well captured compared to the abundant cell type. Additionally, Visium captures a mixture of transcripts from multiple cells and lacks single-cell resolution. Therefore, it relies on cell type abundance estimation tools, which may be less reliable than image-based ST methods that capture transcript expression at the single-cell level. Nevertheless, we attempted to overcome the limitations with several strategies. The first was to remove the background effects of major brain cells. Homeostatic and reactive microglia and their coexpressed transcriptomes between microglia and infiltrating monocytes were the major challenge but were easy to remove, and astrocytes and oligodendrocytes followed by reactive glial cells expressed the same/similar transcriptomes. Double-checking the unique transcriptomes and their combinations was attempted with the data by Ximerakis et al. [ 64 ] and Schafflick et al. [ 68 ]. based on brain tissue studies. The study by Schafflick et al. [ 68 ]. used cells sorted by FACS for CD45 (gene Ptprc ) positivity and thus we were unable to remove the coexpressed transcriptomes of ribosomal protein transcriptomes for lymphoid cells, which if removed, would have enabled correct classification of myeloid and lymphoid cells among major brain cells in terms of intensity and distribution. Nevertheless, visual/manual curation by surveying individual transcriptomes helped to remove absurdly intense and unrealistically distributed transcriptomic signatures. When we used only the data of Schafflick et al. [ 68 ]. , we could not correct the inappropriate signature for B and T-cell compartments even after NSForest application to their data. The data came to look realistic after we adopted cross-tissue data and visual curation upon the two reports by Eraslan et al. [ 69 ] and Dominguez Conde et al. [ 71 ]. DEG data with an arbitrary threshold of 2.0 higher or -2.0 lower log fold change (LFC) for MHC + infiltrating immune macrophages (Mϕs) or LYVE + infiltrating integrity Mϕs produced 200 or more or 100 or more transcriptomes, respectively. We needed to remove, upon visual curation, 30% or 20% of transcriptomes to annotate the infiltrating monocyte-derived Mϕ. Infiltrating Mϕ and border-associated Mϕ [ 67 , 68 ] should have been differentiated but this was not possible due to the lack of clear distinction between the two cell types in the literature and the sparsity of the cells of both types. Tissue-resident and effector memory cells were traced with the transcriptomic signature by Dominguez Conde et al. [ 71 ]. As these authors included a variety of tissues (unfortunately, brain was not included) and stromal tissue specificity was considered a possible confounder in common for every tissue and thus, as expected, they yielded the signature for three compartments of T/ILC, B-cell and myeloid compartments. Of course, the types/subtypes of classically well-known immune cells belonging to these three compartments represented well the rare immune cells that would have originated from the bone marrow. We found differences in the intensity and distribution of the three compartments in the brain sections between 5xFAD mice and wild-type mice (Fig. 3 ). Drug/treatment effects should have been disclosed with this comparison, but we simply state that further investigation is warranted with a larger number of mice to avoid confounding factors which may hide or spuriously render probable false-negative/positive results regarding the effect of any tentative immunomodulatory treatments (Supplementary Tables 5 and 6 ).
The ultimate objective of using ST brain imaging with its visual and quantitative analysis is to convincingly designate the target cells, either major or rare, with regional localization; this can be for either brain parenchymal/stromal or rare immune cells, either resident or infiltrating immune cells and their homeostatic/reactive states, and target genes with significant contributions to pathologic changes in cells/regional tissues and their response to effective or ineffective treatments. More importantly, we could be sure that the unfound cells and transcriptomes were innocent, meaning that they were not affected by the test trial of a novel immunomodulatory therapy. For neuroimmune interactions during the disease process or in response to disease modifying drugs, we now know that the skull BM communicates with the dural sinus and peri-sinus regions, dural lymphatics as well as across ABC and CSF and thus perivascular spaces and ISF; communication can also take a totally different and unique route via the capillary endothelium and stroma of the choroid plexus, and choroid epithelium despite its tight junctions as well as the brain blood vessels’ microvascular endothelium despite its tight junctions. Once immune cells from the three compartments of T/ILCs, B cells and myeloid cells infiltrate the brain parenchyma, dynamically changing along the aging or disease process (in 5xFAD or P301L mice), they can respond to systemic immunomodulatory drug treatment directly or at least indirectly. The abundance of T cells (average 4/mm3) relative to neurons (90,000/mm3) or microglia (6,500/mm3) suggests that a few immune cells could change the response of major brain cells by significantly changing the transcriptomic signature of major brain cells. How the signals are transferred and/or translated from systemically administered anti-CD4 immunoglobulins or NK cell supplements should be investigated further. In this study, the study scheme and analysis methods were proposed to be applied to use ST brain imaging to investigate the impact of novel tentative disease-modifying treatments on neurodegenerative diseases and to elucidate whether regional brain cell-type/state-specific changes in the entire transcriptome per spot/region/cells of the brain or immune system would respond. The comprehensiveness and resolution of the results will be much improved with more novel technology [ 54 , 55 ] that will be available soon in many institutions, such as Visium methods [ 47 ]. Accordingly, methodology for analyzing spatial transcriptomics can be incorporated into high-resolution ST technologies to determine changes in cell types and abundance of rare immune cells with greater confidence.
Materials and methods
Ad models at different ages.
Three-month- and 7.5-month-old male 5xFAD mice (Tg6799; on a C57BL/6-SJL background) containing five FAD mutations in human APP (the Swedish mutation, K670N/M671L; the Florida mutation, I716V; and the London mutation, V717I; and the PS1 mutations M146L/L286V) and wild-type mice were used for spatial transcriptomic brain imaging data. Six- and seventeen-month-old male tau P301L mice (MAPT P301L mutations; on an FVB/N background) were used. Mice of all strains were raised in a laboratory cage with controlled temperature and humidity and on a 12 h light-dark cycle with free access to food and water. All experimental protocols and animal usage were approved (SNU-181018-6, SNU-190221-1-5) by the Institutional Animal Care and Use Committee (IACUC) at Seoul National University. All animals were handled in accordance with the Animal Research: Reporting of in vivo Experiments (ARRIVE) guidelines ( https://arriveguidelines.org ). Details are in Supplementary Notes.
Peripheral CD4 T-cell blockade in the 5xFAD AD model
Anti-CD4 antibody (0.5 mg/mouse; Bio X Cell) was intravenously injected into 6.5-month-old 5xFAD and wild-type mice according to group. Samples of different tissues were obtained after a month. Coronal sections of brain samples were used for spatial transcriptomic data acquisition.
Administration of NK cell supplement in the 5xFAD AD model
NK cells were expanded for 7 days after the isolation of NK cells from BALB/c mouse spleens. NK cells (2 × 10 6 cells/mouse in saline) were intravenously administered once a week for a total of five times to 6.5-month-old 5xFAD and wild-type mice. Brain samples were obtained after five weeks and used for spatial transcriptomic data.
Spatial gene expression library construction
Mice were anesthetized with isoflurane inhalation and perfused intracardially with cold DPBS (Dulbecco’s Phosphate-Buffered Saline; Gibco). Then, whole brains were removed. Brain hemispheres were prepared in frozen blocks using OCT compound (Sakura) and cryosectioned into 10 μm coronal and sagittal sections. According to the manufacturer’s protocols using Visium Spatial Tissue Optimization slides (10X Genomics), the permeabilization time was optimized to 12 min. The brain sections were methanol-fixed, hematoxylin and eosin (H&E)-stained and imaged on a TissueFAXS PLUS (TissueGenostics). The slides were merged into a picture of the whole brain using TissueFAXS imaging software. Then, the sections were permeabilized and processed to obtain cDNA Visium Spatial Gene Expression libraries according to the manufacturer’s protocol. To verify the size of PCR-enriched fragments, the template size distribution was checked using a high-sensitivity DNA assay (Agilent Technologies 2100 Bioanalyzer).
Generation of the count matrix
The libraries were sequenced using HiSeqXten (Illumina) with a read length of 28 bp for read 1 (Spatial Barcode and UMI), 10 bp index read (i7 index), 10 bp index read (i5 index), and 90 bp for read 2 (RNA read). Raw FASTQ data and H&E images were processed by the Space Ranger v1.1.0 (10X Genomics) pipeline for the gene expression analysis of Visium Spatial Gene Expression library data. Illumina base call files from the Illumina sequencing instrument were converted to FASTQ format for each sample using the ‘mkfastq’ command. Visium spatial expression libraries were analyzed with the ‘count’ command. Image alignment to predefined spots was performed using the fiducial alignment grid of the tissue image to determine the orientation and position of the input image. Sequencing reads were aligned to the mm10 reference genome (mm10-2020-A) using STAR (v2.5.1b) aligner. Gene expression profiling in each spot was performed with unique molecular identifier (UMI) and 10X barcode information.
Integration and spot clustering
A total of 63 spatial transcriptomic datasets, including brain tissue from wild-type and 5xFAD mice, with 32,885 genes in common were integrated and analyzed. The generated gene counts were normalized using ‘LogNormalize’ methods with a scale factor of 10,000. The top highly variable genes (HVGs; n = 2,000) in each tissue slide were identified using the variance stabilizing transformation (vst) method. The 2000 integration genes across all slides were then selected by ranking the genes by the number of slides in which they are variable in and their median rank of variability across the slides. For each slide, the log-normalized count matrix for the selected genes was scaled and the total RNA counts in each spot was regressed to remove the influence of the total count in the integration process. Principal component analysis (PCA) was performed for dimensionality reduction. Integration was performed for multiple spatial datasets prior to spot clustering to remove the batch effect. To flexibly integrate a large number of slides with both coronal and sagittal sections, reciprocal PCA (RPCA) was used to discover a set of anchors between the datasets, and normal (wild-type) mice were used as a reference during integration. The anchors were utilized to correct the count matrix in each spatial spot. The corrected counts were then scaled and PCA was performed. For spot clustering, a shared nearest neighbor (SNN) graph was constructed and graph-based clustering was performed using the Louvain algorithm. The resulting spot clusters were visualized using two different approaches: spatially mapped to the tissue based on spatial barcodes, or plotted in 2-dimensional space using Uniform Manifold Approximation and Projection (UMAP). The optimal resolution of the spot clusters was determined by decreasing the resolution value and visually examining the appropriate granularity of the spatial clusters that corresponded well to the anatomical structure. The anatomical location of each cluster was visually determined by comparison with the Allen Mouse Brain Reference Atlas ( https://mouse.brain-map.org/static/atlas ). As a result, the resolution was set to 0.15 for subsequent analysis. All analyses were performed using the R package, Seurat (version 4.1.1) [ 74 ].
Differential gene expression analysis
MAST (Model-based Analysis of Single-cell Transcriptomics) was used to perform differential gene expression analysis [ 96 ]. MAST accounts for the bimodal distribution of counts in the spatial transcriptomics and uses a generalized linear model with the proportion of genes expressed in each spot as a covariate to model the normalized counts. Differentially expressed genes (DEGs) were extracted from the comparison of wild-type and 5xFAD mice in each spot cluster defining the anatomical region in the brain. The cutoff for significantly different genes was false discovery rate (FDR)-adjusted p < 0.05 and log FC > 0.25.
Overrepresentation analysis
Overrepresentation analysis was performed and the Gene Ontology (GO) biological process terms associated with DEGs were identified. The count ratio was defined as the ratio of the proportion of the genes constituting GO terms among the DEGs to the proportion of genes constituting GO terms among total genes. Statistical significance was calculated based on the hypergeometric model, and correction for multiple comparisons was performed using the Benjamini-Hochberg procedure. The dot plots for the significant GO terms were drawn by showing the number of overlapping genes between the DEGs and each GO term, the count ratio, and the adjusted p-values. Overrepresentation tests were performed using clusterProfiler [ 118 ], which supports statistical analysis and visualization of functional profiles for gene sets. The packages ‘enrichplot’ and ‘igraph’ were additionally used to visualize the results.
Marker panel selection and curation
To analyze the spatial patterns of major cell types and immune cell types, the panel of marker genes was constructed and curated for each cell type. For the cell types identified in studies not using scRNAseq, individual genes were determined based on reference papers. For the cell types defined by scRNAseq, Necessary and Sufficient Forest (NSForest) version 2 [ 59 ] was applied and signature genes for the cell type were determined based on the cell type annotation information. The NSForest algorithm scores genes according to binary expression profiles in a specific cell type compared to other cell types. Then, based on the random forest algorithm, the minimum gene set that best describes the given cell type was searched. After selecting signature genes based on NSForest, the gene sets were refined to exclude the genes that are highly expressed in major cell types. This is particularly important when the scRNAseq data represent a subpopulation of the cells in the brain, such as immune cell sorted datasets. As a validation process, the spatial expression of the selected marker genes was examined and the genes were excluded if they showed a non-specific distribution pattern for the cell type. As a final step, genes that were not present in our spatial transcriptomic data were excluded. The curated gene sets are listed in Supplementary Table 7 .
Comparison of cellular signatures across groups
After curation of the marker panel, a gene set that best represents a particular cell type, the signature score of each cell type was computed on the spatial transcriptomic data by utilizing the AddModuleScore function in Seurat [ 44 ] with default parameters. For each gene in the gene set, a fixed number of control genes with the same average expression level as the gene were randomly selected. The difference between the average expression of the gene set and that of the control gene sets was calculated and named the cell signature score. The score in each spot was spatially mapped to the tissue using the SpatialFeaturePlot function in Seurat, and the spatial distribution pattern was identified. The average of the signature scores in a given region of interest was calculated and the values were compared between groups using the Wilcoxon rank-sum test. Correction for multiple comparison was performed using the Bonferroni method. The cutoff for the adjusted p-value was 0.05.
Cell type deconvolution analysis
The cell type distribution represented by the cell signature scores was compared to that derived by the cell type deconvolution method, CellDART [ 46 ]. CellDART first trains a model to extract cell type proportions from the synthetic mixture of cells generated from the reference scRNAseq dataset, and then adapts the model to predict the cell type composition of the spot, which is a mixture of multiple cells. For the major brain cell types, snRNAseq datasets from mouse brain coronal slices [ 49 ] were used as a reference for predicting spatial cell distribution. However, in the case of immune cells, the majority of scRNAseq datasets are obtained after cell sorting strategies such as fluorescence-activated cell sorting (FACS), and there is a mismatch in cell type and composition between spatial and single-cell datasets. Therefore, the cell type deconvolution tool spSeudoMap was used to compensate for this discrepancy [ 50 ]. For lymphoid and myeloid brain cell types, scRNAseq samples from CNS border immune cells were used [ 119 ], and for microglia, scRNAseq samples from brain immune cells were used [ 89 ]. The distribution of representative cell types that showed significant differences between wild-type and 5xFAD mice was evaluated: homeostatic microglia, reactive microglia, macrophages, monocytes, dendritic cells, innate lymphoid cells, natural killer cells, and T cells. The cell type annotation information from the reference single-cell dataset was used for the deconvolution analysis. Default parameter values suggested in the user manual were applied for the analysis.
Statistical analysis
For the spatial transcriptomic data, plots in R were created either with the ggplot2 R package or Seurat modified by custom codes for data visualization. All p-values reported in this study were adjusted by FDR (for DE analysis using MAST) using Benjamini-Hochberg procedure or Bonferroni method (all other analyses). The p-values below 0.05 were considered statistically significant.
Development of an application to visualize and quantify ST datasets
An R shiny-based application named STquantool was developed to comprehensively analyze ST datasets to explore cell type- and cell state-specific regional changes in wild-type, 5xFAD, and treatment mouse models. The application allows users to easily load and integrate the multiple ST datasets and visualize the spatial expression of genes and cell type scores based on Seurat [ 74 ] and shiny running on R (ver. 4.1.1). One of the key features of STquantool is that it facilitates the curation of cell type-specific marker combinations by sorting out key genes based on the NSForest [ 59 ] algorithm and finalizing the markers by visually assessing the spatial expression patterns. As an adjunct, the cell type decomposition method CellDART [ 46 ] can be implemented to find the spatial distribution patterns of major cell types constituting brain tissues. Moreover, the spatial patterns of the cell scores and cell fraction can be quantified and statistically analyzed with STquantool. Finally, the gene-level transcriptomic alterations between the mouse groups can be explored by performing the DEG analysis provided in the application. Then, the functional implications of the selected genes can be represented by gene ontology (GO) and Kyoto Encyclopedia of Genes and Genomes (KEGG) terms [ 120 , 121 , 122 ]. The suggested platform was packaged and can be readily installed from GitHub ( https://github.com/bsungwoo/STquantool.git ).
Data availability
All data are available in the main text or the supplementary materials. Additionally, the Visium spatial transcriptomics datasets utilized in this research are accessible on the data repository ( https://zenodo.org/records/10404412 ). The spatial transcriptomics analytical pipeline, STquantool, is available for installation from GitHub at https://github.com/bsungwoo/STquantool.git
Brioschi S, Wang WL, Peng V, Wang M, Shchukina I, Greenberg ZJ et al. Heterogeneity of meningeal B cells reveals a lymphopoietic niche at the CNS borders. Science. 2021;373.
Cugurra A, Mamuladze T, Rustenhoven J, Dykstra T, Beroshvili G, Greenberg ZJ et al. Skull and vertebral bone marrow are myeloid cell reservoirs for the meninges and CNS parenchyma. Science. 2021;373.
Cai R, Pan C, Ghasemigharagoz A, Todorov MI, Förstera B, Zhao S, et al. Panoptic imaging of transparent mice reveals whole-body neuronal projections and skull-meninges connections. Nat Neurosci. 2019;22:317–27.
Article CAS PubMed Google Scholar
Shibata-Germanos S, Goodman JR, Grieg A, Trivedi CA, Benson BC, Foti SC, et al. Structural and functional conservation of non-lumenized lymphatic endothelial cells in the mammalian leptomeninges. Acta Neuropathol. 2020;139:383–401.
Kutomi O, Takeda S. Identification of lymphatic endothelium in cranial arachnoid granulation-like dural gap. Microscopy (Oxf). 2020;69:391–400.
Louveau A, Smirnov I, Keyes TJ, Eccles JD, Rouhani SJ, Peske JD, et al. Structural and functional features of central nervous system lymphatic vessels. Nature. 2015;523:337–41.
Article CAS PubMed PubMed Central Google Scholar
Aspelund A, Antila S, Proulx ST, Karlsen TV, Karaman S, Detmar M, et al. A dural lymphatic vascular system that drains brain interstitial fluid and macromolecules. J Exp Med. 2015;212:991–9.
Bedussi B, van der Wel NN, de Vos J, van Veen H, Siebes M, VanBavel E, et al. Paravascular channels, cisterns, and the subarachnoid space in the rat brain: a single compartment with preferential pathways. J Cereb Blood Flow Metab. 2017;37:1374–85.
Article PubMed Google Scholar
Da Mesquita S, Louveau A, Vaccari A, Smirnov I, Cornelison RC, Kingsmore KM, et al. Functional aspects of meningeal lymphatics in ageing and Alzheimer’s disease. Nature. 2018;560:185–91.
Article PubMed PubMed Central Google Scholar
Ahn JH, Cho H, Kim JH, Kim SH, Ham JS, Park I, et al. Meningeal lymphatic vessels at the skull base drain cerebrospinal fluid. Nature. 2019;572:62–6.
Frederick N, Louveau A. Meningeal lymphatics, immunity and neuroinflammation. Curr Opin Neurobiol. 2020;62:41–7.
Papadopoulos Z, Herz J, Kipnis J. Meningeal lymphatics: from anatomy to Central Nervous System Immune Surveillance. J Immunol. 2020;204:286–93.
Alves de Lima K, Rustenhoven J, Kipnis J. Meningeal immunity and its function in maintenance of the Central Nervous System in Health and Disease. Annu Rev Immunol. 2020;38:597–620.
Hsu M, Sandor M, Fabry Z. Current concepts on communication between the central nervous system and peripheral immunity via lymphatics: what roles do lymphatics play in brain and spinal cord disease pathogenesis? Biol Futur. 2021;72:45–60.
Solár P, Zamani A, Kubíčková L, Dubový P, Joukal M. Choroid plexus and the blood-cerebrospinal fluid barrier in disease. Fluids Barriers CNS. 2020;17:35.
Lun MP, Monuki ES, Lehtinen MK. Development and functions of the choroid plexus-cerebrospinal fluid system. Nat Rev Neurosci. 2015;16:445–57.
Damkier H, Praetorius J. Structure of the Mammalian Choroid Plexus. In: Role of the Choroid Plexus in Health and Disease Edited by Praetorius J, Blazer-Yost B, Damkier H. New York, NY: Springer US; 2020: 1–33.
Fame RM, Lehtinen MK. Emergence and developmental roles of the Cerebrospinal Fluid System. Dev Cell. 2020;52:261–75.
Dani N, Herbst RH, McCabe C, Green GS, Kaiser K, Head JP, et al. A cellular and spatial map of the choroid plexus across brain ventricles and ages. Cell. 2021;184:3056–74.
Agarwal N, Carare RO. Cerebral vessels: an overview of anatomy, physiology, and role in the drainage of fluids and solutes. Front Neurol. 2020;11:611485.
Ross JM, Kim C, Allen D, Crouch EE, Narsinh K, Cooke DL, et al. Expanding Cell Divers Brain Vasculature Front Physiol. 2020;11:600767.
Google Scholar
Kalucka J, de Rooij L, Goveia J, Rohlenova K, Dumas SJ, Meta E, et al. Single-cell transcriptome atlas of murine endothelial cells. Cell. 2020;180:764–79.
Vanlandewijck M, He L, Mäe MA, Andrae J, Ando K, Del Gaudio F, et al. A molecular atlas of cell types and zonation in the brain vasculature. Nature. 2018;554:475–80.
Dixon GA, Pérez CA. Multiple sclerosis and the Choroid Plexus: emerging concepts of Disease Immunopathophysiology. Pediatr Neurol. 2020;103:65–75.
Stock AD, Der E, Gelb S, Huang M, Weidenheim K, Ben-Zvi A, et al. Tertiary lymphoid structures in the choroid plexus in neuropsychiatric lupus. JCI Insight. 2019;4:e124203.
Ma Q, Decker Y, Müller A, Ineichen BV, Proulx ST. Clearance of cerebrospinal fluid from the sacral spine through lymphatic vessels. J Exp Med. 2019;216:2492–502.
Jacob L, Boisserand L, Geraldo BS, de Brito Neto LMH. Anatomy and function of the vertebral column lymphatic network in mice. Nat Commun. 2019;10:4594.
Petrova TV, Koh GY. Biological functions of lymphatic vessels. Science. 2020;369:eaax4063.
Lee EJ, Choi Y, Park EJ, Lee DS. Lymphatic dysfunction sustains memory impairment despite Abeta reduction in an Alzheimer’s disease model. Immunity & Ageing; 2022. (in revision).
Lee EJ. Investigation of the spatial transcriptomic signatures and therapeutic mode of action in an Alzheimer’s disease model. Seoul National University (Thesis). 2022.
Huang Q, Belz GT. Parallel worlds of the adaptive and innate immune cell networks. Curr Opin Immunol. 2019;58:53–9.
Unger MS, Li E, Scharnagl L, Poupardin R, Altendorfer B, Mrowetz H, et al. CD8(+) T-cells infiltrate Alzheimer’s disease brains and regulate neuronal- and synapse-related gene expression in APP-PS1 transgenic mice. Brain Behav Immun. 2020;89:67–86.
Laurent C, Dorothée G, Hunot S, Martin E, Monnet Y, Duchamp M, et al. Hippocampal T cell infiltration promotes neuroinflammation and cognitive decline in a mouse model of tauopathy. Brain. 2017;140:184–200.
Faridar A, Thome AD, Zhao W, Thonhoff JR, Beers DR, Pascual B, et al. Restoring regulatory T-cell dysfunction in Alzheimer’s disease through ex vivo expansion. Brain Commun. 2020;2:fcaa112.
Dansokho C, Ait Ahmed D, Aid S, Toly-Ndour C, Chaigneau T, Calle V, et al. Regulatory T cells delay disease progression in Alzheimer-like pathology. Brain. 2016;139:1237–51.
Gingele S, Pul R, Sardari M, Borbor M, Henkel F, Moellenkamp TM, et al. FoxP3 deficiency causes no inflammation or neurodegeneration in the murine brain. J Neuroimmunol. 2020;342:577216.
Ito M, Komai K, Mise-Omata S, Iizuka-Koga M, Noguchi Y, Kondo T, et al. Brain regulatory T cells suppress astrogliosis and potentiate neurological recovery. Nature. 2019;565:246–50.
Krämer TJ, Hack N, Brühl TJ, Menzel L, Hummel R, Griemert EV, et al. Depletion of regulatory T cells increases T cell brain infiltration, reactive astrogliosis, and interferon-γ gene expression in acute experimental traumatic brain injury. J Neuroinflammation. 2019;16:163.
Fisher Y, Strominger I, Biton S, Nemirovsky A, Baron R, Monsonego A. Th1 polarization of T cells injected into the cerebrospinal fluid induces brain immunosurveillance. J Immunol. 2014;192:92–102.
Martinez B, Peplow PV. Amelioration of Alzheimer’s disease pathology and cognitive deficits by immunomodulatory agents in animal models of Alzheimer’s disease. Neural Regen Res. 2019;14:1158–76.
Mittal K, Eremenko E, Berner O, Elyahu Y, Strominger I, Apelblat D, et al. CD4 T cells induce a subset of MHCII-Expressing microglia that attenuates Alzheimer Pathology. iScience. 2019;16:298–311.
Raha-Chowdhury R, Henderson JW, Raha AA, Vuono R, Bickerton A, Jones E, et al. Choroid Plexus acts as Gatekeeper for TREM2, abnormal Accumulation of ApoE, and Fibrillary Tau in Alzheimer’s Disease and in Down Syndrome Dementia. J Alzheimers Dis. 2019;69:91–109.
Satija R, Farrell JA, Gennert D, Schier AF, Regev A. Spatial reconstruction of single-cell gene expression data. Nat Biotechnol. 2015;33:495–502.
Tirosh I, Izar B, Prakadan SM, Wadsworth MH 2nd, Treacy D, Trombetta JJ, et al. Dissecting the multicellular ecosystem of metastatic melanoma by single-cell RNA-seq. Science. 2016;352:189–96.
Butler A, Hoffman P, Smibert P, Papalexi E, Satija R. Integrating single-cell transcriptomic data across different conditions, technologies, and species. Nat Biotechnol. 2018;36:411–20.
Bae S, Na KJ, Koh J, Lee DS, Choi H, Kim YT. CellDART: cell type inference by domain adaptation of single-cell and spatial transcriptomic data. Nucleic Acids Res. 2022;50:e57.
Ståhl PL, Salmén F, Vickovic S, Lundmark A, Navarro JF, Magnusson J, et al. Visualization and analysis of gene expression in tissue sections by spatial transcriptomics. Science. 2016;353:78–82.
Cable DM, Murray E, Zou LS, Goeva A, Macosko EZ, Chen F, et al. Robust decomposition of cell type mixtures in spatial transcriptomics. Nat Biotechnol. 2022;40:517–26.
Kleshchevnikov V, Shmatko A, Dann E, Aivazidis A, King HW, Li T, et al. Cell2location maps fine-grained cell types in spatial transcriptomics. Nat Biotechnol. 2022;40:661–71.
Bae S, Choi H, Lee DS, spSeudoMap. Cell type mapping of spatial transcriptomics using unmatched single-cell RNA-seq data. Genome Med. 2023;15:19.
Vickovic S, Eraslan G, Salmén F, Klughammer J, Stenbeck L, Schapiro D, et al. High-definition spatial transcriptomics for in situ tissue profiling. Nat Methods. 2019;16:987–90.
Stickels RR, Murray E, Kumar P, Li J, Marshall JL, Di Bella DJ, et al. Highly sensitive spatial transcriptomics at near-cellular resolution with Slide-seqV2. Nat Biotechnol. 2021;39:313–9.
Cho CS, Xi J, Si Y, Park SR, Hsu JE, Kim M, et al. Microscopic examination of spatial transcriptome using seq-scope. Cell. 2021;184:3559–72.
Chen A, Liao S, Cheng M, Ma K, Wu L, Lai Y, et al. Spatiotemporal transcriptomic atlas of mouse organogenesis using DNA nanoball-patterned arrays. Cell. 2022;185:1777–92.
Chen A, Sun Y, Lei Y, Li C, Liao S, Liang Z, et al. Single-cell spatial transcriptome reveals cell-type organization in the macaque cortex. Cell. 2023;186:3726–43.
Wei X, Fu S, Li H, Liu Y, Wang S, Feng W, et al. Single-cell stereo-seq reveals induced progenitor cells involved in axolotl brain regeneration. Science. 2022;377:eabp9444.
Bugeon S, Duffield J, Dipoppa M, Ritoux A, Prankerd I, Nicoloutsopoulos D, et al. A transcriptomic axis predicts state modulation of cortical interneurons. Nature. 2022;607:330–8.
Zeng H, de Vries SEJ. A gene-expression axis defines neuron behaviour. Nature. 2022;607:243–4.
Aevermann B, Zhang Y, Novotny M, Keshk M, Bakken T, Miller J, et al. A machine learning method for the discovery of minimum marker gene combinations for cell type identification from single-cell RNA sequencing. Genome Res. 2021;31:1767–80.
Nelson ME, Riva SG, Cvejic A. SMaSH: a scalable, general marker gene identification framework for single-cell RNA-sequencing. BMC Bioinformatics. 2022;23:328.
Vargo AHS, Gilbert AC. A rank-based marker selection method for high throughput scRNA-seq data. BMC Bioinformatics. 2020;21:477.
Delaney C, Schnell A, Cammarata LV, Yao-Smith A, Regev A, Kuchroo VK, et al. Combinatorial prediction of marker panels from single-cell transcriptomic data. Mol Syst Biol. 2019;15:e9005.
Armingol E, Officer A, Harismendy O, Lewis NE. Deciphering cell-cell interactions and communication from gene expression. Nat Rev Genet. 2021;22:71–88.
Ximerakis M, Lipnick SL, Innes BT, Simmons SK, Adiconis X, Dionne D, et al. Single-cell transcriptomic profiling of the aging mouse brain. Nat Neurosci. 2019;22:1696–708.
Saunders A, Macosko EZ, Wysoker A, Goldman M, Krienen FM, de Rivera H, et al. Molecular diversity and specializations among the cells of the adult mouse brain. Cell. 2018;174:1015–30.
Marques S, Zeisel A, Codeluppi S, van Bruggen D, Mendanha Falcao A, Xiao L, et al. Oligodendrocyte heterogeneity in the mouse juvenile and adult central nervous system. Science. 2016;352:1326–9.
Van Hove H, Martens L, Scheyltjens I, De Vlaminck K, Pombo Antunes AR, De Prijck S, et al. A single-cell atlas of mouse brain macrophages reveals unique transcriptional identities shaped by ontogeny and tissue environment. Nat Neurosci. 2019;22:1021–35.
Schafflick D, Wolbert J, Heming M, Thomas C, Hartlehnert M, Börsch AL, et al. Single-cell profiling of CNS border compartment leukocytes reveals that B cells and their progenitors reside in non-diseased meninges. Nat Neurosci. 2021;24:1225–34.
Eraslan G, Drokhlyansky E, Anand S, Fiskin E, Subramanian A, Slyper M, et al. Single-nucleus cross-tissue molecular reference maps toward understanding disease gene function. Science. 2022;376:eabl4290.
Miragaia RJ, Gomes T, Chomka A, Jardine L, Riedel A, Hegazy AN, et al. Single-Cell Transcriptomics of Regulatory T Cells reveals trajectories of tissue adaptation. Immunity. 2019;50:493–504.
Domínguez Conde C, Xu C, Jarvis LB, Rainbow DB, Wells SB, Gomes T, et al. Cross-tissue immune cell analysis reveals tissue-specific features in humans. Science. 2022;376:eabl5197.
Kim DG, Lee JI, Lee DS, Lee MC, Choi KS, Han DH. 99mTc-HMPAO labeled leukocyte SPECT in intracranial lesions. Surg Neurol. 1995;44:338–45.
Tappan SJ, Eastwood BS, O’Connor N, Wang Q, Ng L, Feng D, et al. Automatic navigation system for the mouse brain. J Comp Neurol. 2019;527:2200–11.
Hao Y, Hao S, Andersen-Nissen E, Mauck WM 3rd, Zheng S, Butler A, et al. Integrated analysis of multimodal single-cell data. Cell. 2021;184:3573–e35873529.
Hodge RD, Bakken TE, Miller JA, Smith KA, Barkan ER, Graybuck LT, et al. Conserved cell types with divergent features in human versus mouse cortex. Nature. 2019;573:61–8.
Werkman IL, Dubbelaar ML, van der Vlies P, de Boer-Bergsma JJ, Eggen BJL, Baron W. Transcriptional heterogeneity between primary adult grey and white matter astrocytes underlie differences in modulation of in vitro myelination. J Neuroinflammation. 2020;17:373.
A multimodal cell. Census and atlas of the mammalian primary motor cortex. Nature. 2021;598:86–102.
Article Google Scholar
Tasic B, Yao Z, Graybuck LT, Smith KA, Nguyen TN, Bertagnolli D, et al. Shared and distinct transcriptomic cell types across neocortical areas. Nature. 2018;563:72–8.
Zeng H, Sanes JR. Neuronal cell-type classification: challenges, opportunities and the path forward. Nat Rev Neurosci. 2017;18:530–46.
Tasic B, Menon V, Nguyen TN, Kim TK, Jarsky T, Yao Z, et al. Adult mouse cortical cell taxonomy revealed by single cell transcriptomics. Nat Neurosci. 2016;19:335–46.
Yuste R, Hawrylycz M, Aalling N, Aguilar-Valles A, Arendt D, Armañanzas R, et al. A community-based transcriptomics classification and nomenclature of neocortical cell types. Nat Neurosci. 2020;23:1456–68.
Yao Z, van Velthoven CTJ, Nguyen TN, Goldy J, Sedeno-Cortes AE, Baftizadeh F, et al. A taxonomy of transcriptomic cell types across the isocortex and hippocampal formation. Cell. 2021;184:3222–e32413226.
Bayraktar OA, Bartels T, Holmqvist S, Kleshchevnikov V, Martirosyan A, Polioudakis D, et al. Astrocyte layers in the mammalian cerebral cortex revealed by a single-cell in situ transcriptomic map. Nat Neurosci. 2020;23:500–9.
Escartin C, Galea E, Lakatos A, O’Callaghan JP, Petzold GC, Serrano-Pozo A, et al. Reactive astrocyte nomenclature, definitions, and future directions. Nat Neurosci. 2021;24:312–25.
Habib N, McCabe C, Medina S, Varshavsky M, Kitsberg D, Dvir-Szternfeld R, et al. Disease-associated astrocytes in Alzheimer’s disease and aging. Nat Neurosci. 2020;23:701–6.
Ioannou MS, Jackson J, Sheu SH, Chang CL, Weigel AV, Liu H, et al. Neuron-astrocyte metabolic coupling protects against Activity-Induced fatty acid toxicity. Cell. 2019;177:1522–35.
Chamling X, Kallman A, Fang W, Berlinicke CA, Mertz JL, Devkota P, et al. Single-cell transcriptomic reveals molecular diversity and developmental heterogeneity of human stem cell-derived oligodendrocyte lineage cells. Nat Commun. 2021;12:652.
Krasemann S, Madore C, Cialic R, Baufeld C, Calcagno N, El Fatimy R, et al. The TREM2-APOE pathway drives the Transcriptional phenotype of dysfunctional microglia in neurodegenerative diseases. Immunity. 2017;47:566–81.
Keren-Shaul H, Spinrad A, Weiner A, Matcovitch-Natan O, Dvir-Szternfeld R, Ulland TK, et al. A Unique Microglia Type Associated with Restricting Development of Alzheimer’s Disease. Cell. 2017;169:1276–e12901217.
Marschallinger J, Iram T, Zardeneta M, Lee SE, Lehallier B, Haney MS, et al. Lipid-droplet-accumulating microglia represent a dysfunctional and proinflammatory state in the aging brain. Nat Neurosci. 2020;23:194–208.
Friedman BA, Srinivasan K, Ayalon G, Meilandt WJ, Lin H, Huntley MA, et al. Diverse brain myeloid expression profiles reveal distinct microglial activation States and aspects of Alzheimer’s Disease Not Evident in Mouse models. Cell Rep. 2018;22:832–47.
Uriarte Huarte O, Richart L, Mittelbronn M, Michelucci A. Microglia in Health and Disease: the strength to be diverse and reactive. Front Cell Neurosci. 2021;15:660523.
Grubman A, Choo XY, Chew G, Ouyang JF, Sun G, Croft NP, et al. Transcriptional signature in microglia associated with Aβ plaque phagocytosis. Nat Commun. 2021;12:3015.
Pan J, Ma N, Yu B, Zhang W, Wan J. Transcriptomic profiling of microglia and astrocytes throughout aging. J Neuroinflammation. 2020;17:97.
Choi H, Lee EJ, Shin JS, Kim H, Bae S, Choi Y et al. Spatiotemporal characterization of glial cell activation in an Alzheimer’s disease model by spatially resolved transcriptomics. Exp Mol Med. 2023.
Finak G, McDavid A, Yajima M, Deng J, Gersuk V, Shalek AK, et al. MAST: a flexible statistical framework for assessing transcriptional changes and characterizing heterogeneity in single-cell RNA sequencing data. Genome Biol. 2015;16:278.
Locatelli G, Engelhardt B. Microglia get a little help from Th-eir friends. Immunity. 2020;53:484–6.
Erö C, Gewaltig MO, Keller D, Markram H. A cell atlas for the mouse brain. Front Neuroinform. 2018;12:84.
Keller D, Erö C, Markram H. Cell densities in the mouse brain: a systematic review. Front Neuroanat. 2018;12:83.
Mundt S, Mrdjen D, Utz SG, Greter M, Schreiner B, Becher B. Conventional DCs sample and present myelin antigens in the healthy CNS and allow parenchymal T cell entry to initiate neuroinflammation. Sci Immunol. 2019;4:eaau8380.
Scheurer L, Das Gupta RR, Saebisch A, Grampp T, Benke D, Zeilhofer HU et al. Expression of immunoglobulin constant domain genes in neurons of the mouse central nervous system. Life Sci Alliance. 2021;4.
Fang F, Cao W, Zhu W, Lam N, Li L, Gaddam S, et al. The cell-surface 5′-nucleotidase CD73 defines a functional T memory cell subset that declines with age. Cell Rep. 2021;37:109981.
Yang C, Siebert JR, Burns R, Gerbec ZJ, Bonacci B, Rymaszewski A, et al. Heterogeneity of human bone marrow and blood natural killer cells defined by single-cell transcriptome. Nat Commun. 2019;10:3931.
Crinier A, Milpied P, Escalière B, Piperoglou C, Galluso J, Balsamo A, et al. High-dimensional single-cell analysis identifies organ-specific signatures and conserved NK Cell subsets in humans and mice. Immunity. 2018;49:971–86.
Kim JS, Kolesnikov M, Peled-Hajaj S, Scheyltjens I, Xia Y, Trzebanski S, et al. A binary cre Transgenic Approach dissects Microglia and CNS border-Associated macrophages. Immunity. 2021;54:176–90.
Song YH, Yoon J, Lee SH. The role of neuropeptide somatostatin in the brain and its application in treating neurological disorders. Exp Mol Med. 2021;53:328–38.
Davies P, Katzman R, Terry RD. Reduced somatostatin-like immunoreactivity in cerebral cortex from cases of Alzheimer disease and Alzheimer senile dementa. Nature. 1980;288:279–80.
Jordão MJC, Sankowski R, Brendecke SM, Sagar, Locatelli G, Tai YH, et al. Single-cell profiling identifies myeloid cell subsets with distinct fates during neuroinflammation. Science. 2019;363:eaat7554.
Masuda T, Sankowski R, Staszewski O, Bottcher C, Amann L, Sagar, et al. Spatial and temporal heterogeneity of mouse and human microglia at single-cell resolution. Nature. 2019;566:388–92.
Sankowski R, Böttcher C, Masuda T, Geirsdottir L, Sagar, Sindram E, et al. Mapping microglia states in the human brain through the integration of high-dimensional techniques. Nat Neurosci. 2019;22:2098–110.
Kierdorf K, Masuda T, Jordão MJC, Prinz M. Macrophages at CNS interfaces: ontogeny and function in health and disease. Nat Rev Neurosci. 2019;20:547–62.
Prinz M, Jung S, Priller J. Microglia Biology: one century of evolving concepts. Cell. 2019;179:292–311.
Masuda T, Amann L, Sankowski R, Staszewski O, Lenz M. Author correction: Novel Hexb-based tools for studying microglia in the CNS. Nat Immunol. 2020;21:1302.
Borst K, Prinz M. Deciphering the heterogeneity of myeloid cells during neuroinflammation in the single-cell era. Brain Pathol. 2020;30:1192–207.
Dogra P, Rancan C, Ma W, Toth M, Senda T, Carpenter DJ, et al. Tissue determinants of human NK Cell Development, function, and Residence. Cell. 2020;180:749–63.
Fulcher BD, Arnatkeviciute A, Fornito A. Overcoming false-positive gene-category enrichment in the analysis of spatially resolved transcriptomic brain atlas data. Nat Commun. 2021;12:2669.
Chai H, Diaz-Castro B, Shigetomi E, Monte E, Octeau JC, Yu X, et al. Neural circuit-specialized astrocytes: Transcriptomic, Proteomic, Morphological, and functional evidence. Neuron. 2017;95:531–49.
Yu G, Wang LG, Han Y, He QY. clusterProfiler: an R package for comparing biological themes among gene clusters. Omics. 2012;16:284–7.
Posner DA, Lee CY, Portet A, Clatworthy MR. Humoral immunity at the brain borders in homeostasis. Curr Opin Immunol. 2022;76:102188.
Ashburner M, Ball CA, Blake JA, Botstein D, Butler H, Cherry JM, et al. Gene Ontology: tool for the unification of biology. Nat Genet. 2000;25:25–9.
The Gene Ontology. Resource: enriching a GOld mine. Nucleic Acids Res. 2021;49:D325–34.
Kanehisa M, Goto S. KEGG: kyoto encyclopedia of genes and genomes. Nucleic Acids Res. 2000;28:27–30.
Download references
Acknowledgements
We thank Joo Young Park for his technical assistance. The work was supported by grants from the National Research Foundation of Korea (NRF-2017M3C7A1048079, NRF- 2020R1A2C2101069, NRF- 2022R1A5A6000840).
This work was supported by National Research Foundation of Korea (NRF) Grants funded by the Korean Government (MSIP) (No. 2017M3C7A1048079, No. 2020R1A2C2101069 and No. 2017R1A5A1015626).
Author information
Authors and affiliations.
Department of Nuclear Medicine, Seoul National University College of Medicine, 101 Daehak-ro, Jongno-gu, Seoul, 03080, Republic of Korea
Eun Ji Lee, Minseok Suh, Hongyoon Choi, Yoori Choi, Sungwoo Bae & Dong Soo Lee
Department of Molecular Medicine and Biopharmaceutical Sciences, Graduate School of Convergence Science and Technology, Seoul National University, Seoul, Republic of Korea
Eun Ji Lee & Dong Soo Lee
Department of Nuclear Medicine, Seoul National University Hospital, Seoul, Republic of Korea
Minseok Suh, Hongyoon Choi, Sungwoo Bae & Dong Soo Lee
Institute of Radiation Medicine, Medical Research Center, Seoul National University, 103 Daehak-ro, Jongno-gu, Seoul, 03080, Republic of Korea
Minseok Suh, Sungwoo Bae & Dong Soo Lee
Cliniclal Research Institute, Seoul National University Hospital, Seoul, Republic of Korea
Research and Development Center, THERABEST Inc., Seocho-daero 40-gil, Seoul, 06657, Republic of Korea
Do Won Hwang
Medical Science and Engineering, School of Convergence Science and Technology, POSTECH, Pohang, Republic of Korea
Dong Soo Lee
You can also search for this author in PubMed Google Scholar
Contributions
EJL: Methodology: Investigation: Visualization: Writing; SB: Methodology: Investigation: Visualization: Revision; MS: Methodology: Investigation: Visualization; HC: Methodology: Investigation: Funding acquisition; YC: Methodology: Investigation; DWH: Methodology: Investigation; DSL: Conceptualization: Methodology: Investigation: Visualization: Funding acquisition: Project administration: Supervision: Writing – original draft: Writing – review & editing.
Corresponding authors
Correspondence to Sungwoo Bae or Dong Soo Lee .
Ethics declarations
Ethics approval and consent to participate.
All experimental protocols and animal usage were approved (SNU-181018-6, SNU-190221-1-5) by the Institutional Animal Care and Use Committee (IACUC) at Seoul National University. All animals were handled in accordance with the Animal Research: Reporting of in vivo Experiments (ARRIVE) guidelines ( https://arriveguidelines.org ). Details are in Supplementary Notes.
Consent for publication
Not applicable.
Competing interests
The authors declare no competing interests.
Additional information
Publisher’s note.
Springer Nature remains neutral with regard to jurisdictional claims in published maps and institutional affiliations.
Electronic supplementary material
Below is the link to the electronic supplementary material.
Supplementary Material 1
Supplementary material 2, supplementary material 3, rights and permissions.
Open Access This article is licensed under a Creative Commons Attribution 4.0 International License, which permits use, sharing, adaptation, distribution and reproduction in any medium or format, as long as you give appropriate credit to the original author(s) and the source, provide a link to the Creative Commons licence, and indicate if changes were made. The images or other third party material in this article are included in the article’s Creative Commons licence, unless indicated otherwise in a credit line to the material. If material is not included in the article’s Creative Commons licence and your intended use is not permitted by statutory regulation or exceeds the permitted use, you will need to obtain permission directly from the copyright holder. To view a copy of this licence, visit http://creativecommons.org/licenses/by/4.0/ . The Creative Commons Public Domain Dedication waiver ( http://creativecommons.org/publicdomain/zero/1.0/ ) applies to the data made available in this article, unless otherwise stated in a credit line to the data.
Reprints and permissions
About this article
Cite this article.
Lee, E.J., Suh, M., Choi, H. et al. Spatial transcriptomic brain imaging reveals the effects of immunomodulation therapy on specific regional brain cells in a mouse dementia model. BMC Genomics 25 , 516 (2024). https://doi.org/10.1186/s12864-024-10434-8
Download citation
Received : 18 February 2024
Accepted : 20 May 2024
Published : 25 May 2024
DOI : https://doi.org/10.1186/s12864-024-10434-8
Share this article
Anyone you share the following link with will be able to read this content:
Sorry, a shareable link is not currently available for this article.
Provided by the Springer Nature SharedIt content-sharing initiative
- Spatial transcriptomics
- Brain imaging
- Cell type decomposition
- Cell state annotation
- Major brain cells
- Rare immune cells
- Immunomodulatory therapy
BMC Genomics
ISSN: 1471-2164
- Submission enquiries: [email protected]
- General enquiries: [email protected]
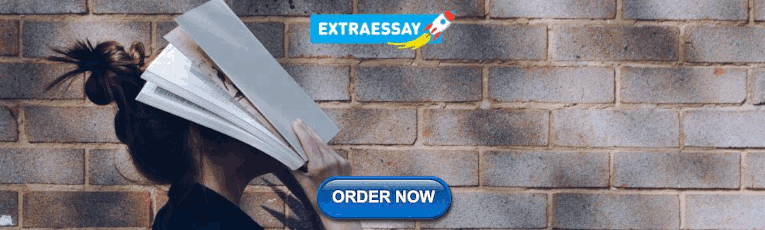
COMMENTS
If the definition of language impairment was loosely defined in a way that any of the three possible definitions could be used to place a child in the "language impairment group" (i.e., identified according to the classic SLI criteria, the DSM5 criteria, or the DLD criteria), this combined criterion would generate a larger group size than a ...
Specific language impairment (SLI) is a developmental language disorder in the absence of obvious accompanying conditions such as mental retardation, ... A brief review on literature indicates that the criteria for selecting SLI subjects vary among studies from scores of standardized tests to assessing child's language in naturalistic contexts ...
The purpose of this study is to provide a review of the literature regarding the treatment of specific language impairment (SLI) in the long-term perspective. We develop the paper along the three issues we consider to be of the most importance in relation to counseling practice with SLI children and young people: the importance of assessment procedures of SLI, the importance of reading skills ...
Introduction and background. Specific language impairment (SLI) is a developmental disorder that is multifactorial in nature and has a high prevalence rate; it is known to be a risk factor for psychosocial and academic challenges that have a significant impact on school-age children when compared to their typically developing peers [1-3].This study aims to elucidate the impact of SLI on ...
In comparison to other childhood disorders (i.e. autism), Developmental Language Disorder (DLD) (previously known as specific language impairment or SLI) receives little public attention despite affecting around 7% of the population (Bishop, Snowling, Thompson, Greenhalgh, & the CATALISE Consortium, 2017).
Analysing language characteristics and understanding their dynamics is the key for a successful intervention by speech and language therapists (SLT). Thus, this review aims to investigate a possible overlap in language development shared by autism spectrum disorders (ASD), specific language impairment (SLI) and social (pragmatic) communication disorder (SPCD). The sources of this work were the ...
Language impairment was defined as a score more than 1.25 SD below the mean. The children were grouped as follows: Language within normal limits at 4 and 7 years (75.6%). Language impairment at 4 but not 7 years (6.2%). Language impairment at 7 but not at 4 years (8.4%). Language impairment at 4 and 7 years (9.8%).
1 INTRODUCTION. The term "pragmatic language impairment" (hereafter PLI) has been widely used to refer to a disorder in which someone manifests problems in the (social) use of language (Perkins, 2008, 2010).This impairment affects the ability to communicate and engage in social interactions, with negative consequences for the quality of interpersonal relationships, academic performance ...
A review of specific language impairment: language characteristics and linguistic interpretations January 1997 Asia Pacific Journal of Speech Language and Hearing 2(3):149-164
Specific Language Impairment Literature Review Specific Language Impairment (SLI) is when a child has a communication disorder that interferes with the development of language skills in children who have no hearing loss or intellectual disabilities (NIH). To be a type of SLI students will be delayed in language and will not be eligible for the ...
language impairments at 4 and 6 years of age, comparing children with specific language impairment (SLI) and nonspecific language impairment (NLI); the likelihood that affectedness will be shared within monozygotic versus dizygotic twin pairs; and estimated levels of heritability for SLI and NLI. Twinning effects are predicted to result in
There is debate regarding the relationship between autism and specific language impairment (SLI), with some researchers proposing aetiological overlap between the conditions and others maintaining their aetiological distinction. ... A comprehensive review of the empirical literature further supported this position, finding little evidence for ...
This review explores these issues, focusing on three disor - ders impacting on language that are dened symptomatically and that are often correlated by researchers: autism spectrum disorders (ASD), specic language impairment (SLI) and social (pragmatic) communication disorder (SPCD). The ultimate aim is to investigate whether a distinctive symp-
Abstract. Background: Children with specific language impairment (SLI) are at considerable risk for later language development. However there has not been a good literature review to provide understanding and to get information relative to parental counseling and decision making for further management. Objective: To summarize the literature ...
In the light of emerging suggestions that language and motor deficits may co-occur, the literature on specific language impairment (SLI) was reviewed to investigate the prevalence of co-morbidity between SLI and poor limb motor skill in children diagnosed with language impairments. An extensive literature search was undertaken and the ...
In the light of emerging suggestions that language and motor deficits may co-occur, the literature on specific language impairment (SLI) was reviewed to investigate the prevalence of co-morbidity between SLI and poor limb motor skill in children diagnosed with language impairments. An extensive lite …
DOI: 10.21608/ejhm.2023.313893 Corpus ID: 261220347; An Overview about Specific Language Impairment: Review Article @article{Abdelbaset2023AnOA, title={An Overview about Specific Language Impairment: Review Article}, author={Amany Abdelbaset and Sabry Mohammed and Elham Amal Saeed Quriba and Nasser Magdy Hassan and Labeb Alnakeb}, journal={The Egyptian Journal of Hospital Medicine}, year={2023 ...
What is "specific language impairment (SLI)?" Specific language impairment is a communication disorder that interferes with the development of language skills in students who have no hearing loss or intellectual disabilities and is identified by National Institute on Deafness and other Communication Disorders. (Adlof, n.) SLI can affect a ...
Specific language impairment (SLI) is a communication disorder that interferes with the development of language skills in children who have no hearing loss. SLI can affect a child's speaking, listening, reading, and writing. SLI is also called developmental language disorder, language delay, or developmental dysphasia.
All of the grammar-based intervention programs described in the selected studies, seemed to be equally adequate when working with children with SLI. Corresponding address: Carmen Julia Coloma Tirapegui Avenida Independencia 1027, Independencia Casilla: 8380453 Santiago, Chile E-mail: [email protected] ABSTRACT Aim: to review the literature reporting grammar-based interventions designed to ...
Ullman M, Pierpont E. Specific language impairment is not specific to language: the procedural deficit hypothesis. Cortex. 2005;41(3):399-433. and Ebbels 16 16. Ebbels S. Effectiveness of intervention for grammar in school-aged children with primary language impairments: a review of the evidence.
Background/Objectives: The intranasal delivery of various neurotropic substances is considered a new attractive therapeutic approach for treating neuropathologies associated with neuroinflammation and altered regeneration. Specific language impairment (SLI) that arises as a result of damage to the cortical speech zones during the developmental period is one of the most common problems in ...
A review of previous studies in the author's lab is provided, encompassing data from ages 21, 24, 27, and 30 months. ... 2020), and a third paper addresses intervention in the literature review (Hadley, 2020). ... Causal pathways for specific language impairment: Lessons from studies of twins. Journal of Speech, Language, ...
Lisa Blanford Grand Canyon University SPD- Specific Language Impairment Literature Review October 16, 2021 Betty Swaney "Specific language impairment (SLI) is a communication disorder that interferes with the development of language skills in children who have no hearing loss or intellectual disabilities.
Increasing evidence of brain-immune crosstalk raises expectations for the efficacy of novel immunotherapies in Alzheimer's disease (AD), but the lack of methods to examine brain tissues makes it difficult to evaluate therapeutics. Here, we investigated the changes in spatial transcriptomic signatures and brain cell types using the 10x Genomics Visium platform in immune-modulated AD models ...