Sentiment Analysis of Film Reviews Based on Deep Learning Model Collaborated with Content Credibility Filtering
- Conference paper
- First Online: 22 January 2021
- Cite this conference paper
- Xindong You 21 ,
- Xueqiang Lv 21 ,
- Shangqian Zhang 21 ,
- Dawei Sun 22 &
- Shang Gao 23
Part of the book series: Lecture Notes of the Institute for Computer Sciences, Social Informatics and Telecommunications Engineering ((LNICST,volume 349))
Included in the following conference series:
- International Conference on Collaborative Computing: Networking, Applications and Worksharing
1288 Accesses
Sentiment analysis of film reviews is the basis of obtaining the opinions of movie viewers. It has an important influence on movie public opinion control and stimulating potential viewers. Due to the natural openness and randomness of social media, there may exist a considerable amount of useless or false information in film review comments, making it challenging to analyze the credibility of the comments. This paper proposes a fine-grained sentiment analysis method based on the key-viewpoint sentences of Chinese film reviews, where a deep learning model is used to classify the fine-grained emotions in film reviews. Based on the analysis results, a method for calculating the credibility of review comments is proposed. Under the credibility criteria, corpus screened through credibility filtering algorithm, the overall sentiment classification can obtain 9% improvement on accuracy than the original corpus, which verifies the validity of the credibility algorithm. The higher quality corpus achieved by the credibility algorithm is benefit for improving the accuracy of the sentiment classification.
This is a preview of subscription content, log in via an institution to check access.
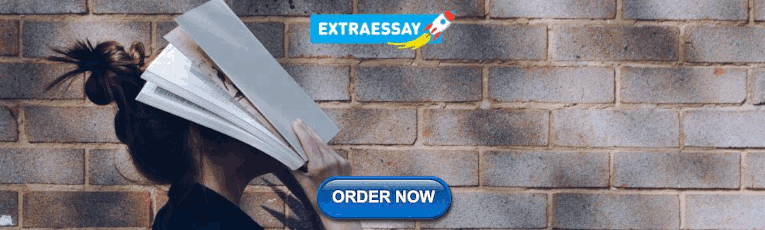
Access this chapter
- Available as PDF
- Read on any device
- Instant download
- Own it forever
- Available as EPUB and PDF
- Compact, lightweight edition
- Dispatched in 3 to 5 business days
- Free shipping worldwide - see info
Tax calculation will be finalised at checkout
Purchases are for personal use only
Institutional subscriptions
Zhao, Y.Y., Qin, B., Liu, T.: Sentiment analysis. J. Softw. 21 (8), 1834–1848 (2010)
Article Google Scholar
Chuang, J.L., Xu, S.Z., Hu, Q.F.: Market research report on movie box office, an empirical study of internet word-of-mouth and movie box office. Chin. Bus. Theor. 17 , 6–7 (2016)
Google Scholar
Huang, S.: Research on fine-grained opinion mining technologies of web review text. Beijing Institute of Technology (2014)
Li, Z., Wei, Y., Zhang, Y., et al.: Exploiting coarse-to-fine task transfer for aspect-level sentiment classification. In: Proceedings of the AAAI Conference on Artificial Intelligence, vol. 33, pp. 4253–4260 (2019)
Yang, M., Yin, W., Qu, Q., et al.: Neural attentive network for cross-domain aspect-level sentiment classification. IEEE Transactions on Affective Computing, 1–11 (2019)
Pontiki, M., Galanis, D., Pavlopoulos, J., et al.: Semeval-2014 task4: aspect based sentiment analysis. In: Proceedings of the 8th International Workshop on Semantic Evaluation, pp. 27–35 (2014)
Rosenthal, S., Farra, N., Nakov, P.: SemEval-2017 task4: sentiment analysis in twitter. In: Proceedings of SemEval (2017)
Guo, C., Wang, Z.Y.: Auto-construct of sentiment ontology tree for fine-grained opinion mining. J. Chin. Inform. Process. 27 (5), 75–83 (2014)
Liu, L., Wang, Y.H., Hang, W.: Fine-grained sentiment analysis oriented to product comment. J. Comput. Appl. 35 (12), 3481–3486 (2015)
Tang, X.B., Lan, Y.T.: Sentiment analysis of microblog product reviews based on feature ontology. Libr. Inform. Serv. 60 (16), 121–127 + 136 (2016)
Tang, D.Y., Qin, B., et al.: Effective LSTMs for target-dependent sentiment classification. In: Proceedings of the 26th International Conference on Computational Linguistics, pp. 3298–3307 (2016)
Ruder, S., Ghaffari, P., Breslin, J.G.: A hierarchical model of reviews for aspect-based sentiment analysis. In: EMNLP, pp. 999–1005 (2016)
Ma, D.H., Li, S.J., Zhang, X.D., Wang, H.F.: Interactive attention networks for aspect-level sentiment classification. In: IJCAI, pp. 4068–4074 (2017)
Xue, W., Li, T.: Aspect Based Sentiment Analysis with Gated Convolutional Networks (2018). arXiv preprint, arXiv:1805.07043
Guan, P.F., Li, B.A., Lv, X.Q.: Attention enhanced bi-directional LSTM for sentiment analysis. J. Chin. Inform. Process. 33 (02), 105–111 (2019)
Zhang, S.Q., Lv, X.Q.: Movie short-text reviews sentiment analysis based on multi-feature fusion. In: International Conference Proceeding Series. ACM (2018)
Paul, A.: Who gives a tweet evaluating microblog content value. In: Proceedings of the ACM 2012 Conference on Computer Supported Cooperative, pp. 471–474 (2012)
Sheng, Y.: Research on micro-blog information quality evaluation based on content—with sina micro-blog as an example. Inform. Sci. 31 (05), 51–54 + 66 (2013)
Hochreiter, S., Schmidhuber, J.: Long short-term memory. Neural Comput. 9 (8), 1735–1780 (1997)
Download references
Acknowledgment
This work is supported by National Natural Science Foundation of China under Grants No. 61671070, 61972364. National Science Key Lab Fund project 6142006190301, National Language Committee of China under Grants ZDI135-53, and Project of Developing University Intension for Improving the Level of Scientific Research–No.2019KYNH226, Qin Xin Talents Cultivation Program, Beijing Information Science & Technology University No. QXTCP B20190.
Author information
Authors and affiliations.
Beijing Key Laboratory of Internet Culture and Digital Dissemination Research, Beijing Information Science and Technology University, Beijing, China
Xindong You, Xueqiang Lv & Shangqian Zhang
School of Information Engineering, China University of Geosciences, Beijing, China
School of Information Technology, Deakin University, Waurn Ponds Victoria, Geelong, Australia
You can also search for this author in PubMed Google Scholar
Corresponding author
Correspondence to Dawei Sun .
Editor information
Editors and affiliations.
Shanghai University, Shanghai, China
Honghao Gao
Xi’an Jiaotong-Liverpool University, Suzhou, China
Xinheng Wang
London South Bank University, London, UK
Muddesar Iqbal
Hangzhou Dianzi University, Hangzhou, China
Zhejiang University, Hangzhou, China
Jianwei Yin
Fudan University, Shanghai, China
Rights and permissions
Reprints and permissions
Copyright information
© 2021 ICST Institute for Computer Sciences, Social Informatics and Telecommunications Engineering
About this paper
Cite this paper.
You, X., Lv, X., Zhang, S., Sun, D., Gao, S. (2021). Sentiment Analysis of Film Reviews Based on Deep Learning Model Collaborated with Content Credibility Filtering. In: Gao, H., Wang, X., Iqbal, M., Yin, Y., Yin, J., Gu, N. (eds) Collaborative Computing: Networking, Applications and Worksharing. CollaborateCom 2020. Lecture Notes of the Institute for Computer Sciences, Social Informatics and Telecommunications Engineering, vol 349. Springer, Cham. https://doi.org/10.1007/978-3-030-67537-0_19
Download citation
DOI : https://doi.org/10.1007/978-3-030-67537-0_19
Published : 22 January 2021
Publisher Name : Springer, Cham
Print ISBN : 978-3-030-67536-3
Online ISBN : 978-3-030-67537-0
eBook Packages : Computer Science Computer Science (R0)
Share this paper
Anyone you share the following link with will be able to read this content:
Sorry, a shareable link is not currently available for this article.
Provided by the Springer Nature SharedIt content-sharing initiative
- Publish with us
Policies and ethics
- Find a journal
- Track your research
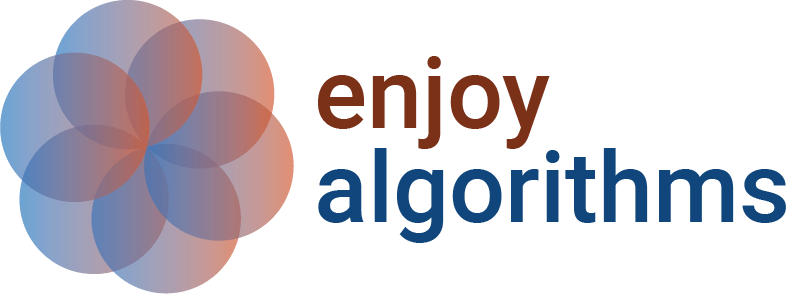
EnjoyMathematics
Movie Review Sentiment Analysis Using Machine Learning
Sentiment Analysis is a technique that uses Natural Language Processing (NLP), Text Mining, and Computational Linguistics to identify and extract the emotions present in the text. It has become increasingly valuable in today's digital age, as the proliferation of reviews, blogs, ratings, and feedback on the internet has created a wealth of information for businesses looking to understand their customers, identify new opportunities, and manage their reputation.
This technique has a wide range of applications and is used by many different industries, such as Market Research, Customer Feedback, Brand Monitoring, Employee Engagement, and Social Media Monitoring. By analyzing the emotions expressed in customer feedback, for example, businesses can gain insight into how their products or services are perceived and make improvements accordingly.
Key takeaways from this blog
In this blog, we will explore the following topics:
- How are different industries using sentiment analysis?
- Data analysis of the IMDB movie review dataset.
- The various steps involved in text processing or data processing, such as tokenization, lemmatization, word embedding, and tf-idf.
- The building of a Light GBM model for predicting positive and negative reviews.
- Real-world examples of sentiment analysis in use by companies such as Twitter and IBM.
- Potential interview questions related to this project.
Traditionally, sentiment classification involves a multi-step process that includes organizing text data and understanding customer emotions. However, with the arrival of deep learning, sentiment analysis has been revolutionized. The introduction of advanced techniques such as Transformers and Transfer Learning has made it possible to quickly build models for sentiment classification.
While the new deep-learning approaches have greatly simplified the process, it is still beneficial to have a basic understanding of sentiment classification. This understanding can help to fine-tune and improve the model, as well as provide a deeper understanding of customer sentiment.
Let’s build a model for classifying the sentiments using the conventional approach!
Data Analysis
In this tutorial, we will be using Kaggle’s IMDB movie review dataset for demonstration. This dataset contains more than 40,000 Reviews & sentiments, and most of the reviews are described in 200-plus words in this dataset.
Let’s load the dataset!
Text Preprocessing
It is important to clean text data before applying machine learning models to it because machines cannot understand the unstructured text. To prepare the text data, we will create a text preprocessing pipeline that includes the following operations on our movie review corpus:
- Converting the text to lowercase
- Removing any URLs from the text
- Removing punctuation marks from the text
- Removing common words (stopwords) from the text
- Correcting any misspelt words
Tokenization and Lemmatization
Tokenization.
Tokenization is the process of breaking down a sentence into individual words, known as tokens. These tokens are used to understand the context of the sentence and to create a vocabulary. Tokenization is achieved by separating the words in a sentence using spaces or punctuation marks. This process helps to make the text more structured, which makes it easier for machine learning models to understand and analyze the data.
Lemmatization
Lemmatization is a process that helps to reduce a word to its most basic root form. It uses linguistic analysis to determine the root form of a word, and it is necessary to have a comprehensive dictionary for the algorithm to reference in order to link the word form to its root. This process can help to improve the accuracy and performance of machine learning models by reducing the number of variations of a word and making the text more structured.
Applying tokenization and Lemmatization to our Clean Movie Reviews:
Now, we have a clean dataset ready for Exploratory data analysis.
Text Exploratory Analysis
We are also interested in the most frequent words other than the stopwords but highly frequent in reviews. Let’s find those words!
Since our dataset contains movie reviews, the resultant word frequency plot is pretty intuitive.
A bigram is a sequence of two adjacent elements from a string of tokens, typically letters, syllables, or words. Let’s also check the highly frequent bigrams in our data.
Almost all the above bigrams make sense in our data. We could go further with trigrams, but that would not be as informative as these bigrams and unigrams.
Visualization of Sentimental Words
Let’s visualize the most practical words representing positive or negative sentiment in reviews.
Let’s quickly summarise our findings:
- The red cluster represents the words used in most of the positive sentiments. Words farthest from the yellow shade have an even higher positive sentimental context.
- On the contrary, the blue cluster represents the words that have appeared majorly in the negative sentiments. The farther they are from the yellow shade, the higher will be negative sentimental context.
- The thin yellow-shaded cluster represents the neutral words.
- The words on the extreme right side more frequently appear in the reviews than those on the extreme left.
Word Embeddings
Word embedding is a technique used to represent words as numerical vectors. This method encodes words in real-valued vectors, such that words with similar meaning and context are located close to each other in the vector space. In other words, word embeddings connect the way humans understand language to the way machines understand it. They are critical for solving natural language processing (NLP) tasks, as they provide a way for machines to understand the meaning and context of words in a text.
There are several methods available for producing word embeddings, but their main idea is the same: to capture as much contextual and semantic information as possible. Choosing the best word embedding method often requires experimentation and can be a difficult task.
Some popular and straightforward methods for creating vector representations of words include:
- Bag-of-words
- ELMO (Embeddings for Language Models)
In this blog, we will keep ourselves confined to the TF-IDF Vectorizer.
TF-IDF Vectorizer
TF-IDF is a short notation for "Term Frequency and Inverse Document Frequency". It is commonly used to transform text into a meaningful representation of numeric vectors. Initially, it is an information retrieval method that relies on Term Frequency (TF) and Inverse Document Frequency (IDF) to measure the importance of a word in a document.
Term Frequency (TF) tracks the occurrence of words in a document; Inverse Document Frequency (IDF) assigns a weightage to each word in the corpus. The IDF weightage is high for infrequently appearing words and low for frequent words. This allows us to detect how important a word is to a document.
Let’s implement TF-IDF on our movie reviews:
Model Building
We are ready to build our Sentiment Classification model, but first, we must select a supervised classification model that satisfies our requirements.
We have several algorithms for classification tasks, each with their own pros and cons. One algorithm may produce superior results compared to others but may require more explainability. Even if explainability is not compromised, deploying such complex algorithms can be tedious. In other words, there is a trade-off between performance, model complexity, and model explainability. The ideal algorithm should be explainable, reliable, and easy to deploy, but again, there is no such thing as a perfect algorithm.
For example, XGBoost is a high-performance and explainable algorithm, but on the other hand, it is quite complex and requires high computational power. On the other hand, Logistic Regression is relatively fast, simple to implement, and explainable, but the performance of logistic regression on non-linear datasets is considerably disappointing. As the number of features in the dataset increases, Logistic Regression tends to become slower and its performance deteriorates.
For this blog, we will be using the Light GBM Classifier!
Light Gradient Boosting Machine (Light GBM)
Light GBM is a gradient-boosting framework that is similar to XGBoost and utilizes tree-based learning algorithms. It is designed to be distributed and efficient, with the following benefits:
- Faster training speed and increased efficiency
- Lower memory usage
- Improved accuracy
- Support for parallel and GPU learning
- Capable of handling large-scale data
Light GBM is an excellent alternative to XGBoost as it is roughly six times faster than XGBoost without compromising performance. It can handle large datasets and requires low memory to operate.
Let’s implement Light-GBM for Sentiment Classification:
Accuracy on the Testing dataset
Classification Report
Industrial Use Cases of Sentiment Analysis
Twitter allows businesses to engage personally with consumers by using real-time sentiment classification models to support and manage the marketing strategies of several brands. With so much data available, Twitter's Sentiment analysis enables companies to understand their customers, keep track of what's being said about their brand and competitors, and discover trends in the market.
IBM is one of the few companies that uses sentiment analysis to understand employee concerns. They are also developing programs to improve employees' likelihood of staying on the job. This helps human-resource managers figure out how workers feel about their company and where management can make changes to improve the experience of their employees.
Nielsen Holdings Inc.
Nielsen relies on Sentiment Analysis to discover market trends and find the popularity of their customer's products. Based on sentimental trends, they also provide consultation for building marketing strategies and campaigns.
Possible Interview Questions
Sentiment analysis projects are a common category of project that is often found in beginners' resumes. However, it's important to be prepared for potential questions on this topic, such as:
- What steps did you take to preprocess the data?
- Why did you choose to perform lemmatization instead of stemming?
- How did you convert the text into a machine-readable or trainable format?
- Can you explain how Light GBM works and what are the hyperparameters involved with the Light GBM model?
- How do you know that your model is better and what can be done to improve accuracy further?
We started with a brief introduction to Sentiment Analysis and why it is required in industries. Moving on, we applied a text preprocessing pipeline to our movie review dataset to remove the redundant expressions from the text. We implemented tokenization and Lemmatization to understand the context of those words used in the reviews and limit the recurring words appearing in diverse forms. Further, we performed a text exploratory analysis to understand the frequent unigrams and bigrams used in the reviews and visualize the clusters of positive, negative, and neutral words available in reviews.
Finally, we applied the TF-IDF vectorizer to the processed reviews, built a Light GBM model to classify the reviews, and evaluated the performance on the testing dataset. We also looked at some industrial use cases of Sentiment analysis.
Enjoy Learning, Enjoy Algorithms!
Share your insights.
Don’t fill this out if you’re human:
More from EnjoyAlgorithms
Self-paced courses and blogs, coding interview, machine learning, system design, oop concepts, our newsletter.
Subscribe to get well designed content on data structure and algorithms, machine learning, system design, object orientd programming and math.
©2023 Code Algorithms Pvt. Ltd.
All rights reserved.

Movie Reviews Sentiment Analysis -Binary Classification with Machine Learning
- May 25, 2020
- Machine Learning

In this Machine Learning Project, we’ll build binary classification that puts movie reviews texts into one of two categories — negative or positive sentiment. We’re going to have a brief look at the Bayes theorem and relax its requirements using the Naive assumption.
Let’s start by importing the Libraries
You can download the data set you need for this task from here:

No null values, Label encode sentiment to 1(positive) and 0(negative)

STEPS TO CLEAN THE REVIEWS :
- Remove HTML tags
- Remove special characters
- Convert everything to lowercase
- Remove stopwords
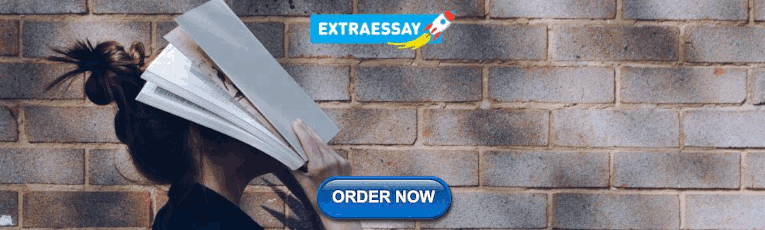
1. Remove HTML tags
Regex rule : ‘<.*?>’
2. Remove special characters
3. convert everything to lowercase, 4. remove stopwords, 5. stem the words.

CREATING THE MODEL
1. creating bag of words (bow), 2. train test split, 3. defining the models and training them, 4. prediction and accuracy metrics to choose best model.
0 mean negative .
I hope it will help you .
Follow us on Instagram for all your Queries
Aman Kharwal
Data Strategist at Statso. My aim is to decode data science for the real world in the most simple words.
Recommended For You

Image Data Preprocessing Techniques You Should Know
- June 5, 2024

Python Libraries for LLMs You Should Know
- June 4, 2024

Companies Offering Data Science Jobs
- May 31, 2024

LLMs Project Ideas
- May 30, 2024
One comment
[…] Movie Reviews Sentiment Analysis -Binary Classification with Machine Learning […]
Leave a Reply Cancel reply
Discover more from thecleverprogrammer.
Subscribe now to keep reading and get access to the full archive.
Type your email…
Continue reading
Navigation Menu
Search code, repositories, users, issues, pull requests..., provide feedback.
We read every piece of feedback, and take your input very seriously.
Saved searches
Use saved searches to filter your results more quickly.
To see all available qualifiers, see our documentation .
- Notifications You must be signed in to change notification settings
omaarelsherif/Movie-Reviews-Sentiment-Analysis-Using-Machine-Learning
Folders and files, repository files navigation, movie reviews sentiment analysis.

➲ Project description
Movie reviews sentiment analysis is a project which is based on natural language processing, where we use NLP techniques to extract useful words of each review and based on these words we can use binary classification to predict the movie sentiment if it's positive or negative
➲ Prerequisites
This is list of required packages and modules for the project to be installed :
- Scikit-learn
Install all required packages :
➲ The Dataset

➲ Coding Sections
In this part we will see the project code divided to sections as follows:
Section 1 | Data Preprocessing : In this section we aim to do some operations on the dataset before training the model on it, processes like :
- Loading the dataset
- Encoding ouput to binary (Positive : 1 , Negative : 0)
- Data cleaning : Remove HTML tags
- Data cleaning : Remove special characters
- Data cleaning : Convert everything to lowercase
- Data cleaning : Remove stopwords
- Data cleaning : Stemming
Section 2 | Model Creation : The dataset is ready for training, so we create a Naive Bayes model using scikit-learn and then fit it to the data.
Section 3 | Model Evaluation : Finally we evaluate the model by getting accuracy, classification report and confusion matrix.
➲ Installation
- Clone the repo git clone https://github.com/omaarelsherif/Movie-Reviews-Sentiment-Analysis-Using-Machine-Learning.git
- Run the code from cmd python movie_reviews_sentiment_analysis.py
Now let's see the project output after running the code :

➲ References
These links may help you to better understanding of the project idea and techniques used :
- Natural Language Processing (NLP) : https://ibm.co/38bN03T
- Sentiment analysis : https://bit.ly/3yi9BGq
- Naive Bayes classifier : https://bit.ly/3zhoWIO
- Model evaluation : https://bit.ly/3B12VOO
- E-mail : [email protected]
- LinkedIn : https://www.linkedin.com/in/omaarelsherif/
- Facebook : https://www.facebook.com/omaarelshereif
- Python 100.0%
Sentiment Analysis on IMDB Movie Reviews using Machine Learning and Deep Learning Algorithms
Ieee account.
- Change Username/Password
- Update Address
Purchase Details
- Payment Options
- Order History
- View Purchased Documents
Profile Information
- Communications Preferences
- Profession and Education
- Technical Interests
- US & Canada: +1 800 678 4333
- Worldwide: +1 732 981 0060
- Contact & Support
- About IEEE Xplore
- Accessibility
- Terms of Use
- Nondiscrimination Policy
- Privacy & Opting Out of Cookies
A not-for-profit organization, IEEE is the world's largest technical professional organization dedicated to advancing technology for the benefit of humanity. © Copyright 2024 IEEE - All rights reserved. Use of this web site signifies your agreement to the terms and conditions.
Help | Advanced Search
Computer Science > Computation and Language
Title: performance evaluation of reddit comments using machine learning and natural language processing methods in sentiment analysis.
Abstract: Sentiment analysis, an increasingly vital field in both academia and industry, plays a pivotal role in machine learning applications, particularly on social media platforms like Reddit. However, the efficacy of sentiment analysis models is hindered by the lack of expansive and fine-grained emotion datasets. To address this gap, our study leverages the GoEmotions dataset, comprising a diverse range of emotions, to evaluate sentiment analysis methods across a substantial corpus of 58,000 comments. Distinguished from prior studies by the Google team, which limited their analysis to only two models, our research expands the scope by evaluating a diverse array of models. We investigate the performance of traditional classifiers such as Naive Bayes and Support Vector Machines (SVM), as well as state-of-the-art transformer-based models including BERT, RoBERTa, and GPT. Furthermore, our evaluation criteria extend beyond accuracy to encompass nuanced assessments, including hierarchical classification based on varying levels of granularity in emotion categorization. Additionally, considerations such as computational efficiency are incorporated to provide a comprehensive evaluation framework. Our findings reveal that the RoBERTa model consistently outperforms the baseline models, demonstrating superior accuracy in fine-grained sentiment classification tasks. This underscores the substantial potential and significance of the RoBERTa model in advancing sentiment analysis capabilities.
Submission history
Access paper:.
- Other Formats
References & Citations
- Google Scholar
- Semantic Scholar
BibTeX formatted citation

Bibliographic and Citation Tools
Code, data and media associated with this article, recommenders and search tools.
- Institution
arXivLabs: experimental projects with community collaborators
arXivLabs is a framework that allows collaborators to develop and share new arXiv features directly on our website.
Both individuals and organizations that work with arXivLabs have embraced and accepted our values of openness, community, excellence, and user data privacy. arXiv is committed to these values and only works with partners that adhere to them.
Have an idea for a project that will add value for arXiv's community? Learn more about arXivLabs .
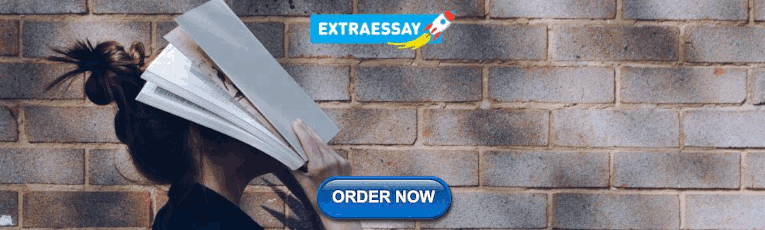
IMAGES
VIDEO
COMMENTS
This tutorial is ideal for beginning machine learning practitioners who want a project-focused guide to building sentiment analysis pipelines with spaCy. You should be familiar with basic machine learning techniques like binary classification as well as the concepts behind them, such as training loops, data batches, and weights and biases.
The reviews were originally released in 2002, but an updated and cleaned up version was released in 2004, referred to as "v2.0". The dataset is comprised of 1,000 positive and 1,000 negative movie reviews drawn from an archive of the rec.arts.movies.reviews newsgroup hosted at IMDB.
Want to know how to perform sentiment analysis and machine learning on movie reviews dataset? This machine learning project tutorial uses the IMDB movie revi...
This paper aims to analyze the reviews of various movies and determine the factors that influenced the ratings of the movies. It also aims to formulate a strategy to improve the customer experience. The reviews play a significant role in the success or failure of a movie; a good sentiment analysis model is needed to classify movie reviews.
This research paper presents a comprehensive comparison of traditional machine learning techniques and advanced transformer-based models for IMDb movie reviews sentiment analysis.
Fig. 1. Flowchart of proposed method. Three datasets have been used for study. 2 of them are for Movie Recommendation and 1 is for Sentiment Analysis. The ones used for recommendation are 'tmdb_5000_movies.csv', 'tbmd_5000_credits.csv' and the one used for sentiment analysis is 'reviews.txt'.
Sentiment analysis is a natural language processing problem where text is understood, and the underlying intent is predicted. In this post, you will discover how you can predict the sentiment of movie reviews as either positive or negative in Python using the Keras deep learning library. After reading this post, you will know: About the IMDB sentiment analysis problem for natural language
In this paper, we do sentiment analysis in the two different movie review datasets using various machine learning techniques including decision tree, naïve Bayes, support vector machine, blending, voting, and recurrent neural networks (RNN). We propose a few frameworks of senti-ment classification using these techniques on the given datasets.
We can learn about the movie's strengths and weaknesses from a textual review, and a morein-depth analysis of a movie review can tell us if the movie overall meets the reviewer's expectations. One of the most important areas of machine learning is sentiment analysis, which seeks to extract subjective information from written reviews.
Sentiment analysis of a movie review can rate how positive or negative a movie review is and hence the overall rating for a movie. Therefore, the process of understanding if a review is positive or negative can be automated as the machine learns through training and testing the data. This project aims to rate reviews using two classifiers and ...
The report utilizes a methodology to conduct the analysis of the sentiment analysis of IMDb reviews, as shown in Fig. 1. First, the report illustrates and feeds the data into the data cleaning and preprocess. Next, the report removes the stop words and some irrelevant words from the original data; then, the vectorization techniques are applied ...
Sentiment analysis is most widely used in NLP to extract and observe opinions of an individual or a group of people based on their own words or on their perspective or views on certain incidents, which could be based on a social media post, a review on a movie or even a feedback for a product purchased online. This technique can directly be used to predict the emotions conveyed by the text ...
Sentiment analysis of film reviews is the basis of obtaining the opinions of movie viewers. It has an important influence on movie public opinion control and stimulating potential viewers. ... Early scholars often used traditional machine learning and rule-based methods to extract emotion evaluation units in texts to perform fine-grained ...
Well, this is the process of looking at data and making inferences; in this case, using machine learning to learn and predict whether a movie review is positive or negative. Maybe you're interested in knowing whether movie reviews are positive or negative, companies use sentiment analysis in a variety of settings, particularly for marketing ...
Explore and run machine learning code with Kaggle Notebooks | Using data from IMDB Dataset of 50K Movie Reviews ... Explore and run machine learning code with Kaggle Notebooks | Using data from IMDB Dataset of 50K Movie Reviews. code. New Notebook. table_chart. New Dataset. tenancy. New Model. emoji_events. New Competition. corporate_fare.
The labeled data set consists of 50,000 IMDB movie reviews, specially selected for sentiment analysis. The sentiment of reviews is binary, meaning the IMDB rating <5 results in a sentiment score of 0, and rating 7 have a sentiment score of 1. No individual movie has more than 30 reviews. The 25,000 review labeled training set does not include ...
Sentiment Analysis is a technique that uses Natural Language Processing (NLP), Text Mining, and Computational Linguistics to identify and extract the emotions present in the text. It has become increasingly valuable in today's digital age, as the proliferation of reviews, blogs, ratings, and feedback on the internet has created a wealth of ...
Machine Learning. 1. In this Machine Learning Project, we'll build binary classification that puts movie reviews texts into one of two categories — negative or positive sentiment. We're going to have a brief look at the Bayes theorem and relax its requirements using the Naive assumption. Let's start by importing the Libraries.
Sentiment analysis is a machine learning approach in which machine learns and analyze the sentiments, emotions etc about some text data like reviews about movies or products. These reviews are ...
Abstract: Movie Reviews Using Sentiment Analysis is a research project that explores the application of natural language processing techniques for analyzing and classifying sentiments in movie reviews. The project involves collecting a large dataset of movie reviews from various sources, processing and cleaning the data, and then applying machine learning algorithms to train a model that can ...
Movie reviews sentiment analysis is a project which is based on natural language processing, where we use NLP techniques to extract useful words of each review and based on these words we can use binary classification to predict the movie sentiment if it's positive or negative.
2 DEPARTMENT OF COMPUTER SCIENCE AND ENGINEERING RCC INSTITUTE OF INFORMATION TECHNOLOGY TO WHOM IT MAY CONCERN I hereby recommend that the project Sentimental Analysis of Product-Based Reviews using Machine Learning Approaches prepared under my supervision by Anusuya Dhara (University Roll No.:11700114008 | Class Roll No.: CSE/2014/041), Arkadeb Saha
Sentiment analysis is the study, to classify the text based on customer reviews which can provide valuable information to improve business. Previously the analysis was carried out based on the information provided by the customers using natural language processing and machine learning. In this paper, sentiment analysis on IMDB movie reviews dataset is implemented using Machine Learning (ML ...
View PDF Abstract: Sentiment analysis, an increasingly vital field in both academia and industry, plays a pivotal role in machine learning applications, particularly on social media platforms like Reddit. However, the efficacy of sentiment analysis models is hindered by the lack of expansive and fine-grained emotion datasets. To address this gap, our study leverages the GoEmotions dataset ...