Have a language expert improve your writing
Run a free plagiarism check in 10 minutes, generate accurate citations for free.
- Knowledge Base
- Type I & Type II Errors | Differences, Examples, Visualizations
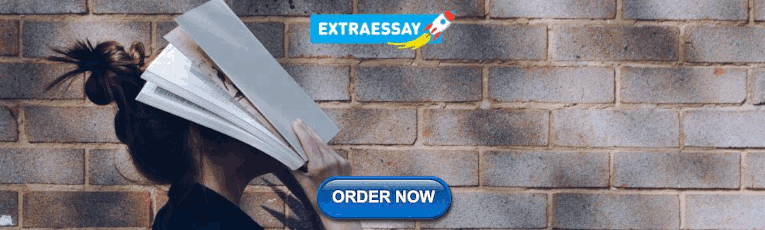
Type I & Type II Errors | Differences, Examples, Visualizations
Published on January 18, 2021 by Pritha Bhandari . Revised on June 22, 2023.
In statistics , a Type I error is a false positive conclusion, while a Type II error is a false negative conclusion.
Making a statistical decision always involves uncertainties, so the risks of making these errors are unavoidable in hypothesis testing .
The probability of making a Type I error is the significance level , or alpha (α), while the probability of making a Type II error is beta (β). These risks can be minimized through careful planning in your study design.
- Type I error (false positive) : the test result says you have coronavirus, but you actually don’t.
- Type II error (false negative) : the test result says you don’t have coronavirus, but you actually do.
Table of contents
Error in statistical decision-making, type i error, type ii error, trade-off between type i and type ii errors, is a type i or type ii error worse, other interesting articles, frequently asked questions about type i and ii errors.
Using hypothesis testing, you can make decisions about whether your data support or refute your research predictions with null and alternative hypotheses .
Hypothesis testing starts with the assumption of no difference between groups or no relationship between variables in the population—this is the null hypothesis . It’s always paired with an alternative hypothesis , which is your research prediction of an actual difference between groups or a true relationship between variables .
In this case:
- The null hypothesis (H 0 ) is that the new drug has no effect on symptoms of the disease.
- The alternative hypothesis (H 1 ) is that the drug is effective for alleviating symptoms of the disease.
Then , you decide whether the null hypothesis can be rejected based on your data and the results of a statistical test . Since these decisions are based on probabilities, there is always a risk of making the wrong conclusion.
- If your results show statistical significance , that means they are very unlikely to occur if the null hypothesis is true. In this case, you would reject your null hypothesis. But sometimes, this may actually be a Type I error.
- If your findings do not show statistical significance, they have a high chance of occurring if the null hypothesis is true. Therefore, you fail to reject your null hypothesis. But sometimes, this may be a Type II error.
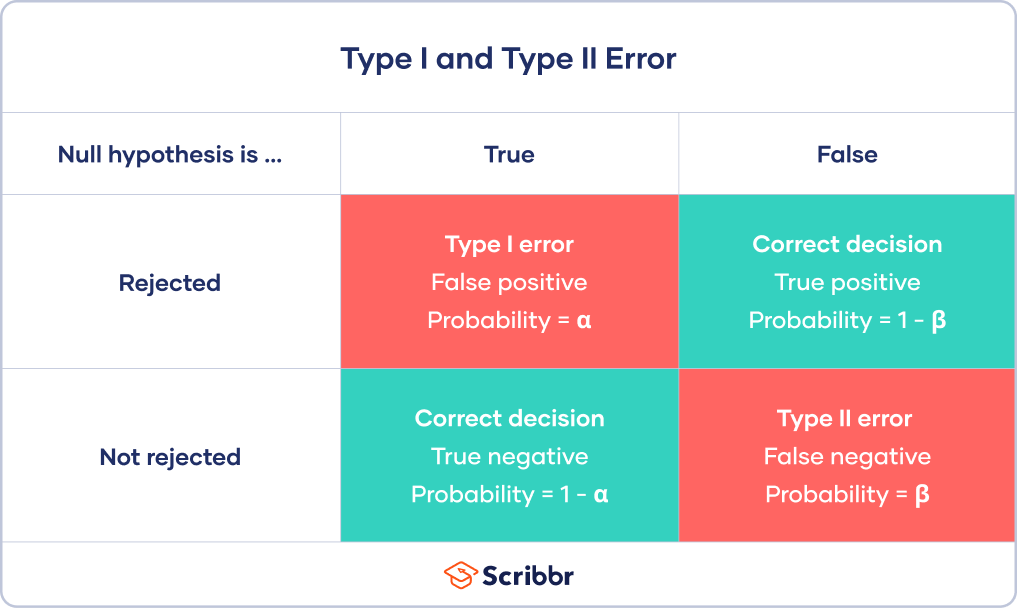
Prevent plagiarism. Run a free check.
A Type I error means rejecting the null hypothesis when it’s actually true. It means concluding that results are statistically significant when, in reality, they came about purely by chance or because of unrelated factors.
The risk of committing this error is the significance level (alpha or α) you choose. That’s a value that you set at the beginning of your study to assess the statistical probability of obtaining your results ( p value).
The significance level is usually set at 0.05 or 5%. This means that your results only have a 5% chance of occurring, or less, if the null hypothesis is actually true.
If the p value of your test is lower than the significance level, it means your results are statistically significant and consistent with the alternative hypothesis. If your p value is higher than the significance level, then your results are considered statistically non-significant.
To reduce the Type I error probability, you can simply set a lower significance level.
Type I error rate
The null hypothesis distribution curve below shows the probabilities of obtaining all possible results if the study were repeated with new samples and the null hypothesis were true in the population .
At the tail end, the shaded area represents alpha. It’s also called a critical region in statistics.
If your results fall in the critical region of this curve, they are considered statistically significant and the null hypothesis is rejected. However, this is a false positive conclusion, because the null hypothesis is actually true in this case!
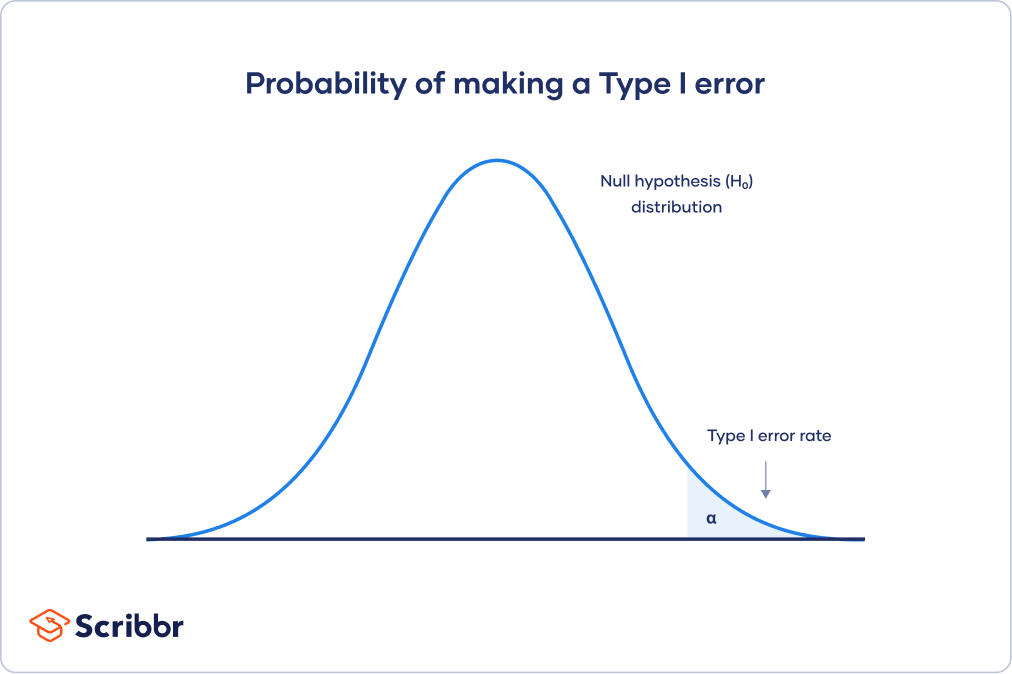
A Type II error means not rejecting the null hypothesis when it’s actually false. This is not quite the same as “accepting” the null hypothesis, because hypothesis testing can only tell you whether to reject the null hypothesis.
Instead, a Type II error means failing to conclude there was an effect when there actually was. In reality, your study may not have had enough statistical power to detect an effect of a certain size.
Power is the extent to which a test can correctly detect a real effect when there is one. A power level of 80% or higher is usually considered acceptable.
The risk of a Type II error is inversely related to the statistical power of a study. The higher the statistical power, the lower the probability of making a Type II error.
Statistical power is determined by:
- Size of the effect : Larger effects are more easily detected.
- Measurement error : Systematic and random errors in recorded data reduce power.
- Sample size : Larger samples reduce sampling error and increase power.
- Significance level : Increasing the significance level increases power.
To (indirectly) reduce the risk of a Type II error, you can increase the sample size or the significance level.
Type II error rate
The alternative hypothesis distribution curve below shows the probabilities of obtaining all possible results if the study were repeated with new samples and the alternative hypothesis were true in the population .
The Type II error rate is beta (β), represented by the shaded area on the left side. The remaining area under the curve represents statistical power, which is 1 – β.
Increasing the statistical power of your test directly decreases the risk of making a Type II error.
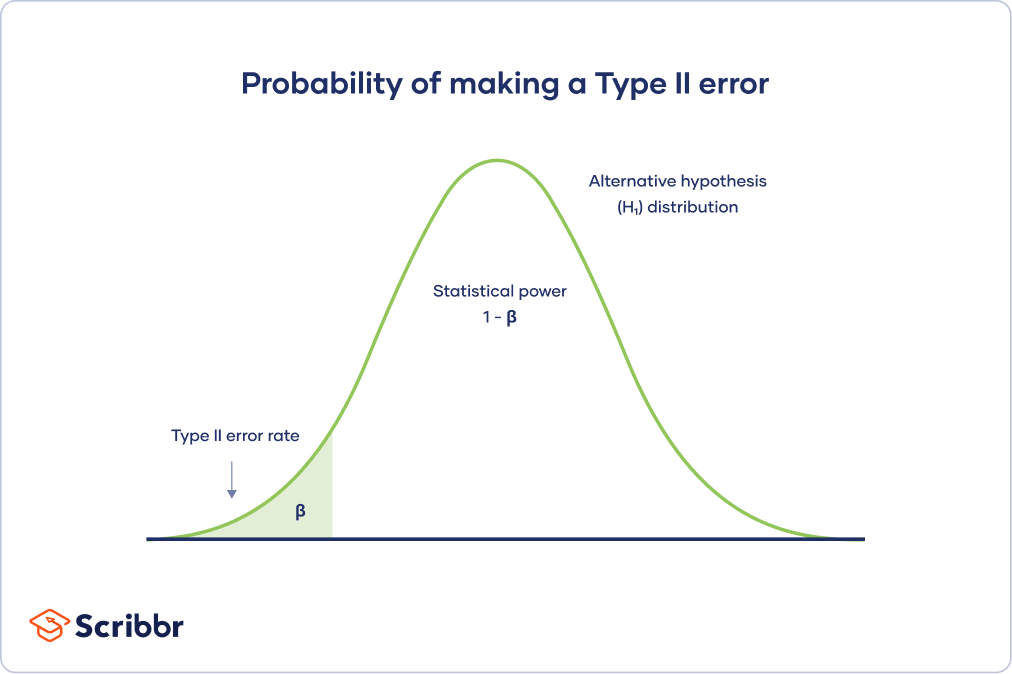
The Type I and Type II error rates influence each other. That’s because the significance level (the Type I error rate) affects statistical power, which is inversely related to the Type II error rate.
This means there’s an important tradeoff between Type I and Type II errors:
- Setting a lower significance level decreases a Type I error risk, but increases a Type II error risk.
- Increasing the power of a test decreases a Type II error risk, but increases a Type I error risk.
This trade-off is visualized in the graph below. It shows two curves:
- The null hypothesis distribution shows all possible results you’d obtain if the null hypothesis is true. The correct conclusion for any point on this distribution means not rejecting the null hypothesis.
- The alternative hypothesis distribution shows all possible results you’d obtain if the alternative hypothesis is true. The correct conclusion for any point on this distribution means rejecting the null hypothesis.
Type I and Type II errors occur where these two distributions overlap. The blue shaded area represents alpha, the Type I error rate, and the green shaded area represents beta, the Type II error rate.
By setting the Type I error rate, you indirectly influence the size of the Type II error rate as well.
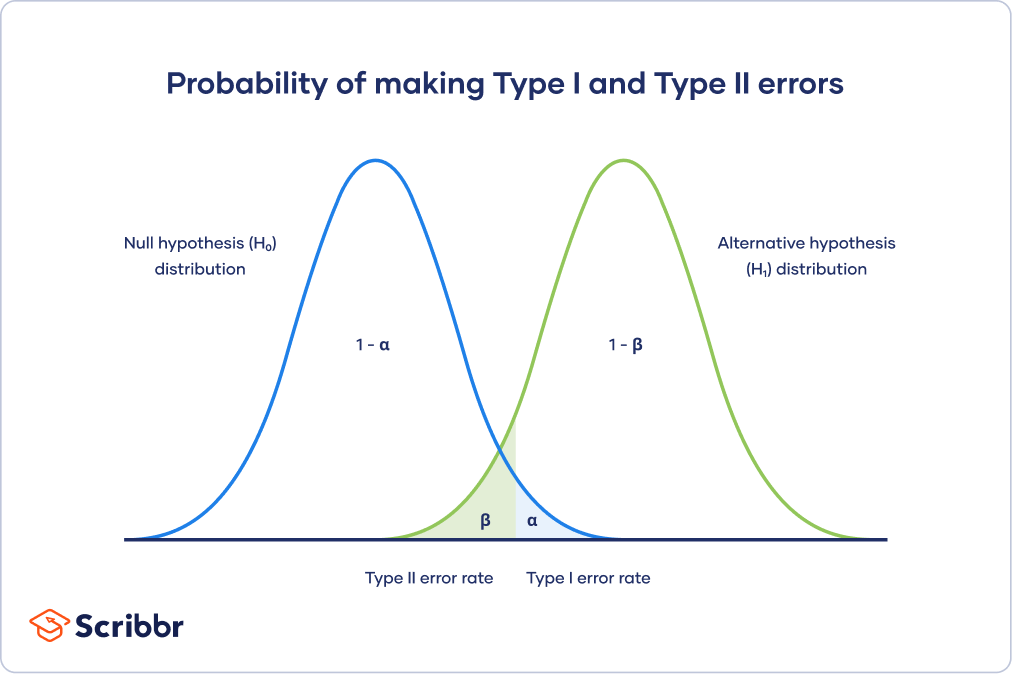
It’s important to strike a balance between the risks of making Type I and Type II errors. Reducing the alpha always comes at the cost of increasing beta, and vice versa .
Receive feedback on language, structure, and formatting
Professional editors proofread and edit your paper by focusing on:
- Academic style
- Vague sentences
- Style consistency
See an example
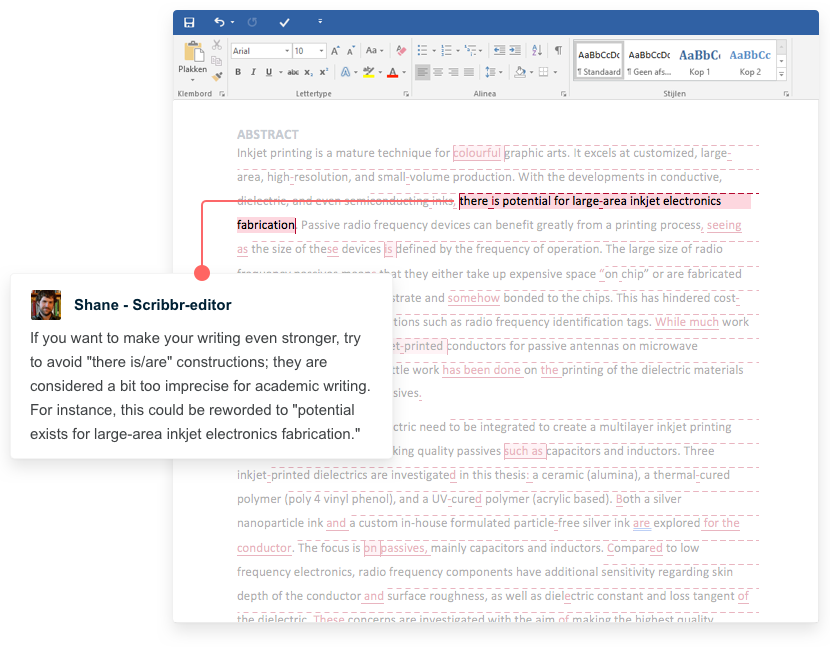
For statisticians, a Type I error is usually worse. In practical terms, however, either type of error could be worse depending on your research context.
A Type I error means mistakenly going against the main statistical assumption of a null hypothesis. This may lead to new policies, practices or treatments that are inadequate or a waste of resources.
In contrast, a Type II error means failing to reject a null hypothesis. It may only result in missed opportunities to innovate, but these can also have important practical consequences.
If you want to know more about statistics , methodology , or research bias , make sure to check out some of our other articles with explanations and examples.
- Normal distribution
- Descriptive statistics
- Measures of central tendency
- Correlation coefficient
- Null hypothesis
Methodology
- Cluster sampling
- Stratified sampling
- Types of interviews
- Cohort study
- Thematic analysis
Research bias
- Implicit bias
- Cognitive bias
- Survivorship bias
- Availability heuristic
- Nonresponse bias
- Regression to the mean
In statistics, a Type I error means rejecting the null hypothesis when it’s actually true, while a Type II error means failing to reject the null hypothesis when it’s actually false.
The risk of making a Type I error is the significance level (or alpha) that you choose. That’s a value that you set at the beginning of your study to assess the statistical probability of obtaining your results ( p value ).
To reduce the Type I error probability, you can set a lower significance level.
The risk of making a Type II error is inversely related to the statistical power of a test. Power is the extent to which a test can correctly detect a real effect when there is one.
To (indirectly) reduce the risk of a Type II error, you can increase the sample size or the significance level to increase statistical power.
Statistical significance is a term used by researchers to state that it is unlikely their observations could have occurred under the null hypothesis of a statistical test . Significance is usually denoted by a p -value , or probability value.
Statistical significance is arbitrary – it depends on the threshold, or alpha value, chosen by the researcher. The most common threshold is p < 0.05, which means that the data is likely to occur less than 5% of the time under the null hypothesis .
When the p -value falls below the chosen alpha value, then we say the result of the test is statistically significant.
In statistics, power refers to the likelihood of a hypothesis test detecting a true effect if there is one. A statistically powerful test is more likely to reject a false negative (a Type II error).
If you don’t ensure enough power in your study, you may not be able to detect a statistically significant result even when it has practical significance. Your study might not have the ability to answer your research question.
Cite this Scribbr article
If you want to cite this source, you can copy and paste the citation or click the “Cite this Scribbr article” button to automatically add the citation to our free Citation Generator.
Bhandari, P. (2023, June 22). Type I & Type II Errors | Differences, Examples, Visualizations. Scribbr. Retrieved June 15, 2024, from https://www.scribbr.com/statistics/type-i-and-type-ii-errors/
Is this article helpful?
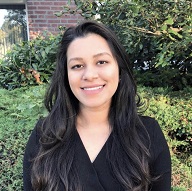
Pritha Bhandari
Other students also liked, an easy introduction to statistical significance (with examples), understanding p values | definition and examples, statistical power and why it matters | a simple introduction, what is your plagiarism score.
Type 1 and Type 2 Errors in Statistics
Saul Mcleod, PhD
Editor-in-Chief for Simply Psychology
BSc (Hons) Psychology, MRes, PhD, University of Manchester
Saul Mcleod, PhD., is a qualified psychology teacher with over 18 years of experience in further and higher education. He has been published in peer-reviewed journals, including the Journal of Clinical Psychology.
Learn about our Editorial Process
On This Page:
A statistically significant result cannot prove that a research hypothesis is correct (which implies 100% certainty). Because a p -value is based on probabilities, there is always a chance of making an incorrect conclusion regarding accepting or rejecting the null hypothesis ( H 0 ).
Anytime we make a decision using statistics, there are four possible outcomes, with two representing correct decisions and two representing errors.
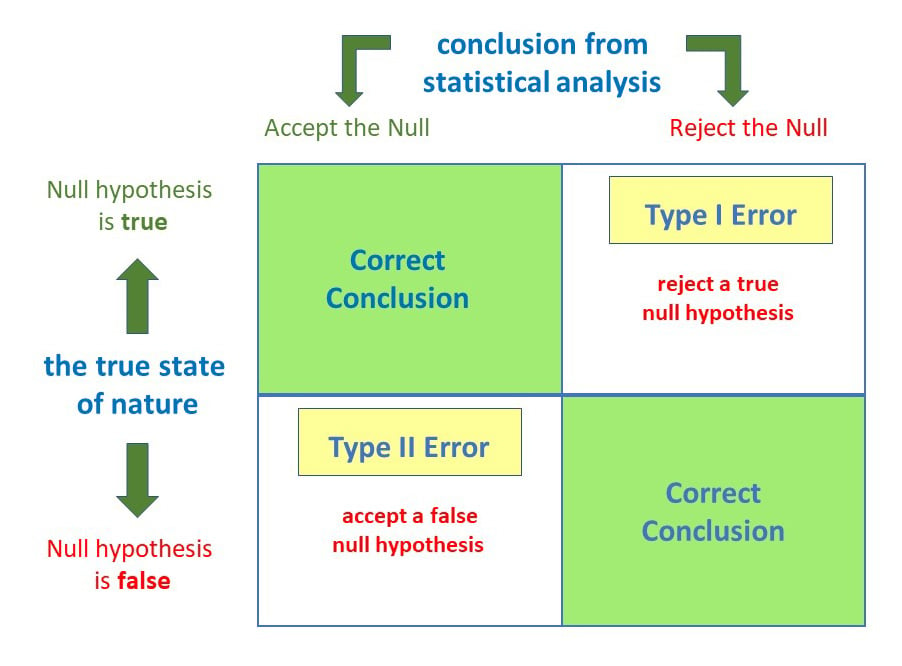
The chances of committing these two types of errors are inversely proportional: that is, decreasing type I error rate increases type II error rate and vice versa.
As the significance level (α) increases, it becomes easier to reject the null hypothesis, decreasing the chance of missing a real effect (Type II error, β). If the significance level (α) goes down, it becomes harder to reject the null hypothesis , increasing the chance of missing an effect while reducing the risk of falsely finding one (Type I error).
Type I error
A type 1 error is also known as a false positive and occurs when a researcher incorrectly rejects a true null hypothesis. Simply put, it’s a false alarm.
This means that you report that your findings are significant when they have occurred by chance.
The probability of making a type 1 error is represented by your alpha level (α), the p- value below which you reject the null hypothesis.
A p -value of 0.05 indicates that you are willing to accept a 5% chance of getting the observed data (or something more extreme) when the null hypothesis is true.
You can reduce your risk of committing a type 1 error by setting a lower alpha level (like α = 0.01). For example, a p-value of 0.01 would mean there is a 1% chance of committing a Type I error.
However, using a lower value for alpha means that you will be less likely to detect a true difference if one really exists (thus risking a type II error).
Scenario: Drug Efficacy Study
Imagine a pharmaceutical company is testing a new drug, named “MediCure”, to determine if it’s more effective than a placebo at reducing fever. They experimented with two groups: one receives MediCure, and the other received a placebo.
- Null Hypothesis (H0) : MediCure is no more effective at reducing fever than the placebo.
- Alternative Hypothesis (H1) : MediCure is more effective at reducing fever than the placebo.
After conducting the study and analyzing the results, the researchers found a p-value of 0.04.
If they use an alpha (α) level of 0.05, this p-value is considered statistically significant, leading them to reject the null hypothesis and conclude that MediCure is more effective than the placebo.
However, MediCure has no actual effect, and the observed difference was due to random variation or some other confounding factor. In this case, the researchers have incorrectly rejected a true null hypothesis.
Error : The researchers have made a Type 1 error by concluding that MediCure is more effective when it isn’t.
Implications
Resource Allocation : Making a Type I error can lead to wastage of resources. If a business believes a new strategy is effective when it’s not (based on a Type I error), they might allocate significant financial and human resources toward that ineffective strategy.
Unnecessary Interventions : In medical trials, a Type I error might lead to the belief that a new treatment is effective when it isn’t. As a result, patients might undergo unnecessary treatments, risking potential side effects without any benefit.
Reputation and Credibility : For researchers, making repeated Type I errors can harm their professional reputation. If they frequently claim groundbreaking results that are later refuted, their credibility in the scientific community might diminish.
Type II error
A type 2 error (or false negative) happens when you accept the null hypothesis when it should actually be rejected.
Here, a researcher concludes there is not a significant effect when actually there really is.
The probability of making a type II error is called Beta (β), which is related to the power of the statistical test (power = 1- β). You can decrease your risk of committing a type II error by ensuring your test has enough power.
You can do this by ensuring your sample size is large enough to detect a practical difference when one truly exists.
Scenario: Efficacy of a New Teaching Method
Educational psychologists are investigating the potential benefits of a new interactive teaching method, named “EduInteract”, which utilizes virtual reality (VR) technology to teach history to middle school students.
They hypothesize that this method will lead to better retention and understanding compared to the traditional textbook-based approach.
- Null Hypothesis (H0) : The EduInteract VR teaching method does not result in significantly better retention and understanding of history content than the traditional textbook method.
- Alternative Hypothesis (H1) : The EduInteract VR teaching method results in significantly better retention and understanding of history content than the traditional textbook method.
The researchers designed an experiment where one group of students learns a history module using the EduInteract VR method, while a control group learns the same module using a traditional textbook.
After a week, the student’s retention and understanding are tested using a standardized assessment.
Upon analyzing the results, the psychologists found a p-value of 0.06. Using an alpha (α) level of 0.05, this p-value isn’t statistically significant.
Therefore, they fail to reject the null hypothesis and conclude that the EduInteract VR method isn’t more effective than the traditional textbook approach.
However, let’s assume that in the real world, the EduInteract VR truly enhances retention and understanding, but the study failed to detect this benefit due to reasons like small sample size, variability in students’ prior knowledge, or perhaps the assessment wasn’t sensitive enough to detect the nuances of VR-based learning.
Error : By concluding that the EduInteract VR method isn’t more effective than the traditional method when it is, the researchers have made a Type 2 error.
This could prevent schools from adopting a potentially superior teaching method that might benefit students’ learning experiences.
Missed Opportunities : A Type II error can lead to missed opportunities for improvement or innovation. For example, in education, if a more effective teaching method is overlooked because of a Type II error, students might miss out on a better learning experience.
Potential Risks : In healthcare, a Type II error might mean overlooking a harmful side effect of a medication because the research didn’t detect its harmful impacts. As a result, patients might continue using a harmful treatment.
Stagnation : In the business world, making a Type II error can result in continued investment in outdated or less efficient methods. This can lead to stagnation and the inability to compete effectively in the marketplace.
How do Type I and Type II errors relate to psychological research and experiments?
Type I errors are like false alarms, while Type II errors are like missed opportunities. Both errors can impact the validity and reliability of psychological findings, so researchers strive to minimize them to draw accurate conclusions from their studies.
How does sample size influence the likelihood of Type I and Type II errors in psychological research?
Sample size in psychological research influences the likelihood of Type I and Type II errors. A larger sample size reduces the chances of Type I errors, which means researchers are less likely to mistakenly find a significant effect when there isn’t one.
A larger sample size also increases the chances of detecting true effects, reducing the likelihood of Type II errors.
Are there any ethical implications associated with Type I and Type II errors in psychological research?
Yes, there are ethical implications associated with Type I and Type II errors in psychological research.
Type I errors may lead to false positive findings, resulting in misleading conclusions and potentially wasting resources on ineffective interventions. This can harm individuals who are falsely diagnosed or receive unnecessary treatments.
Type II errors, on the other hand, may result in missed opportunities to identify important effects or relationships, leading to a lack of appropriate interventions or support. This can also have negative consequences for individuals who genuinely require assistance.
Therefore, minimizing these errors is crucial for ethical research and ensuring the well-being of participants.
Further Information
- Publication manual of the American Psychological Association
- Statistics for Psychology Book Download

Related Articles
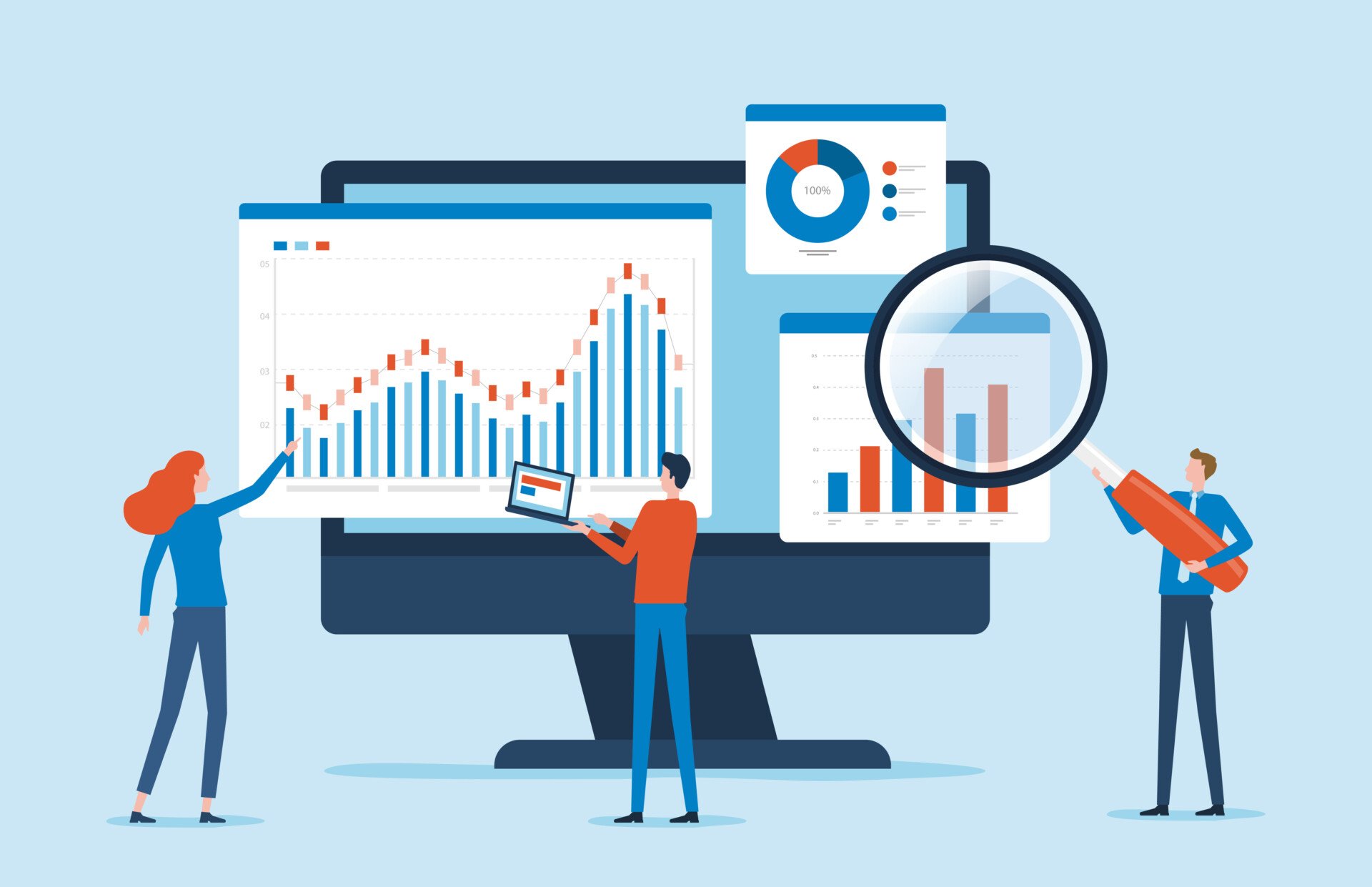
Exploratory Data Analysis
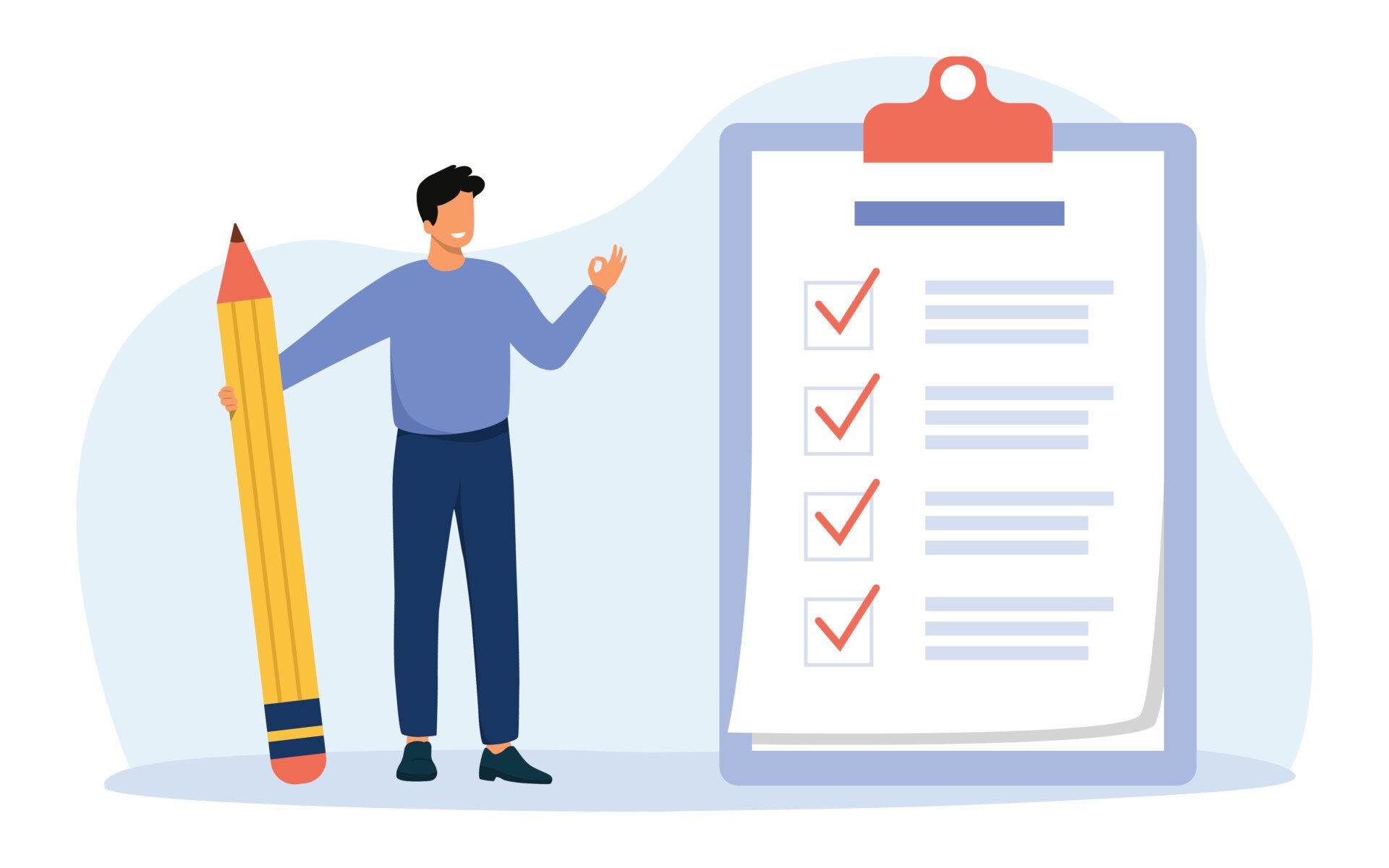
Research Methodology , Statistics
What Is Face Validity In Research? Importance & How To Measure
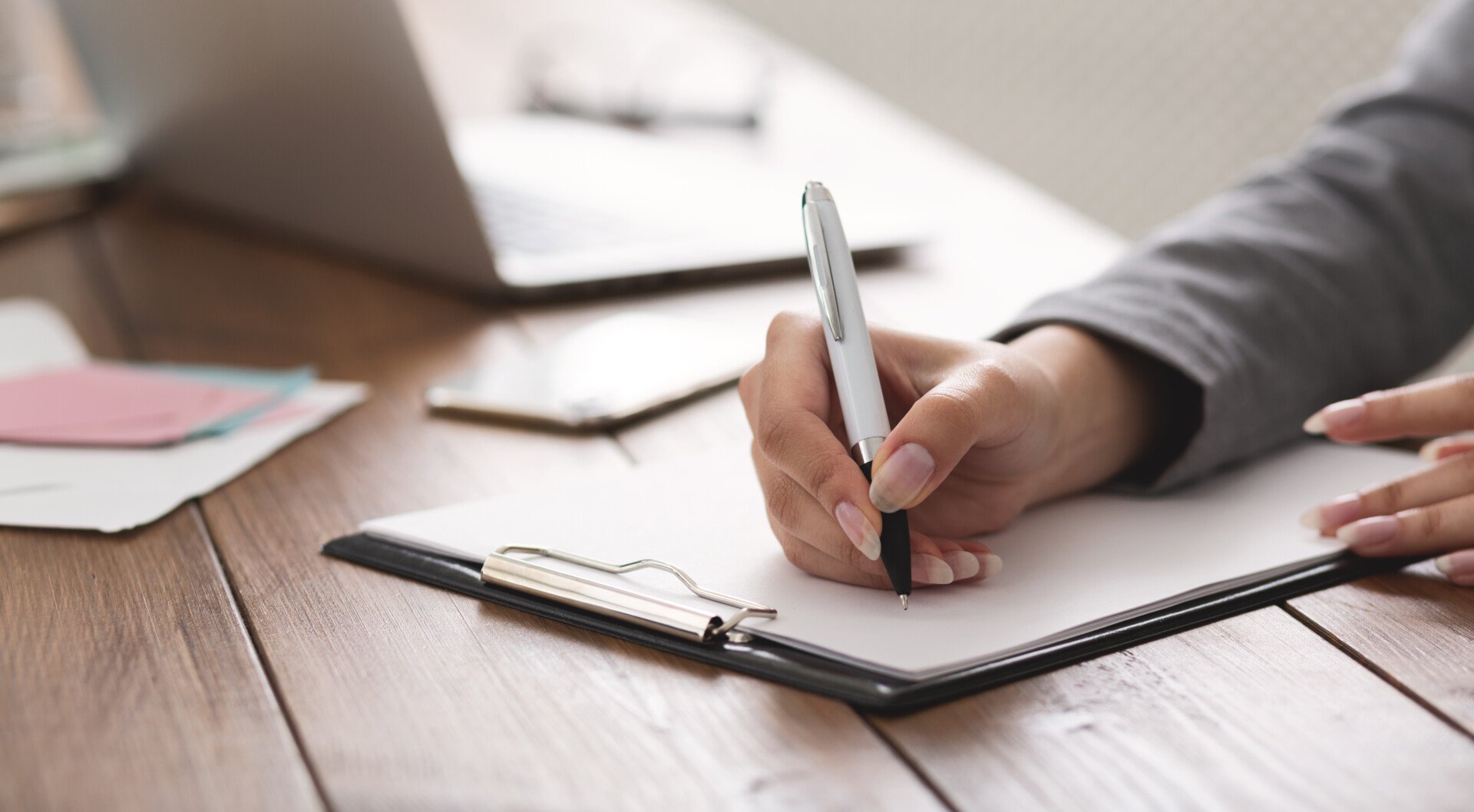
Criterion Validity: Definition & Examples
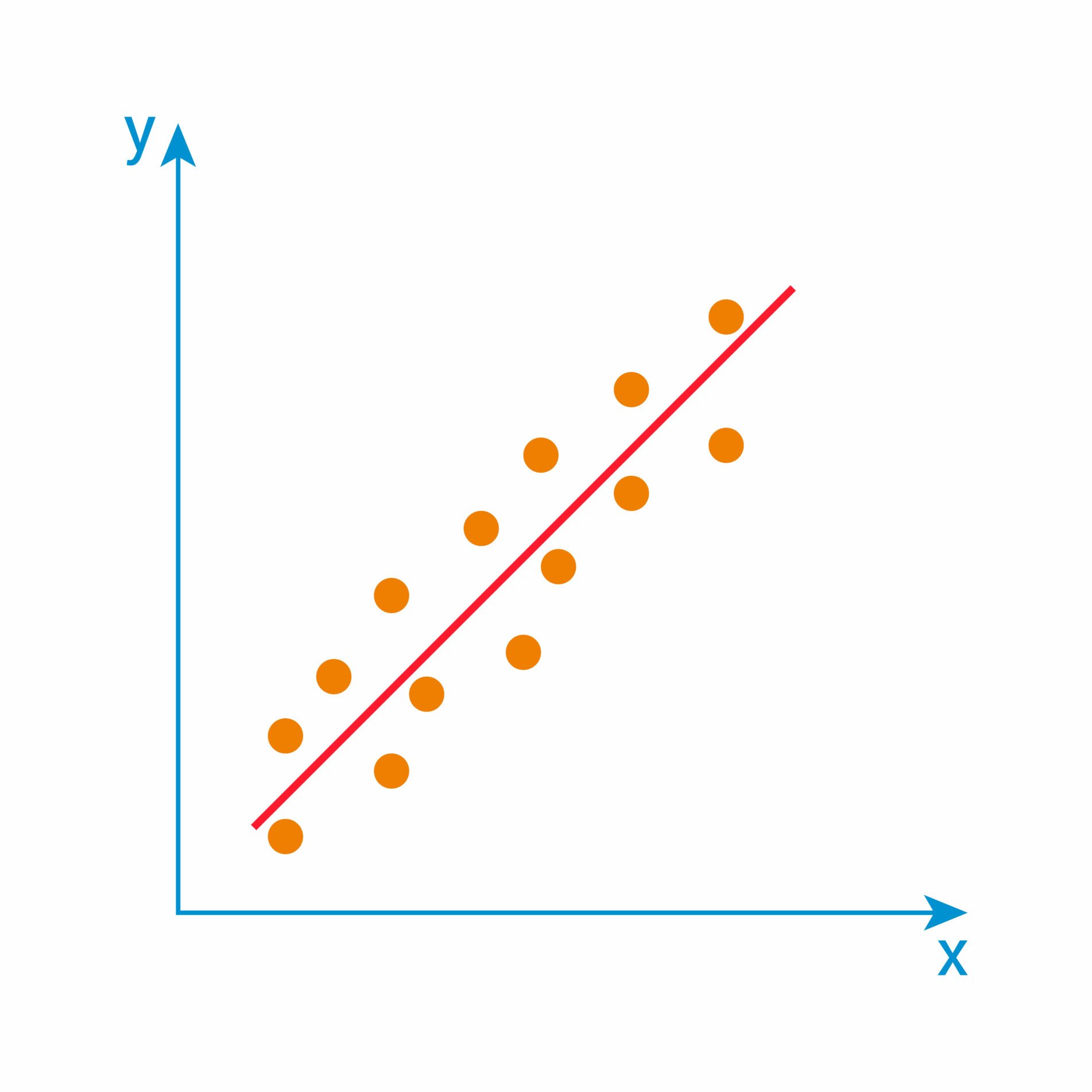
Convergent Validity: Definition and Examples
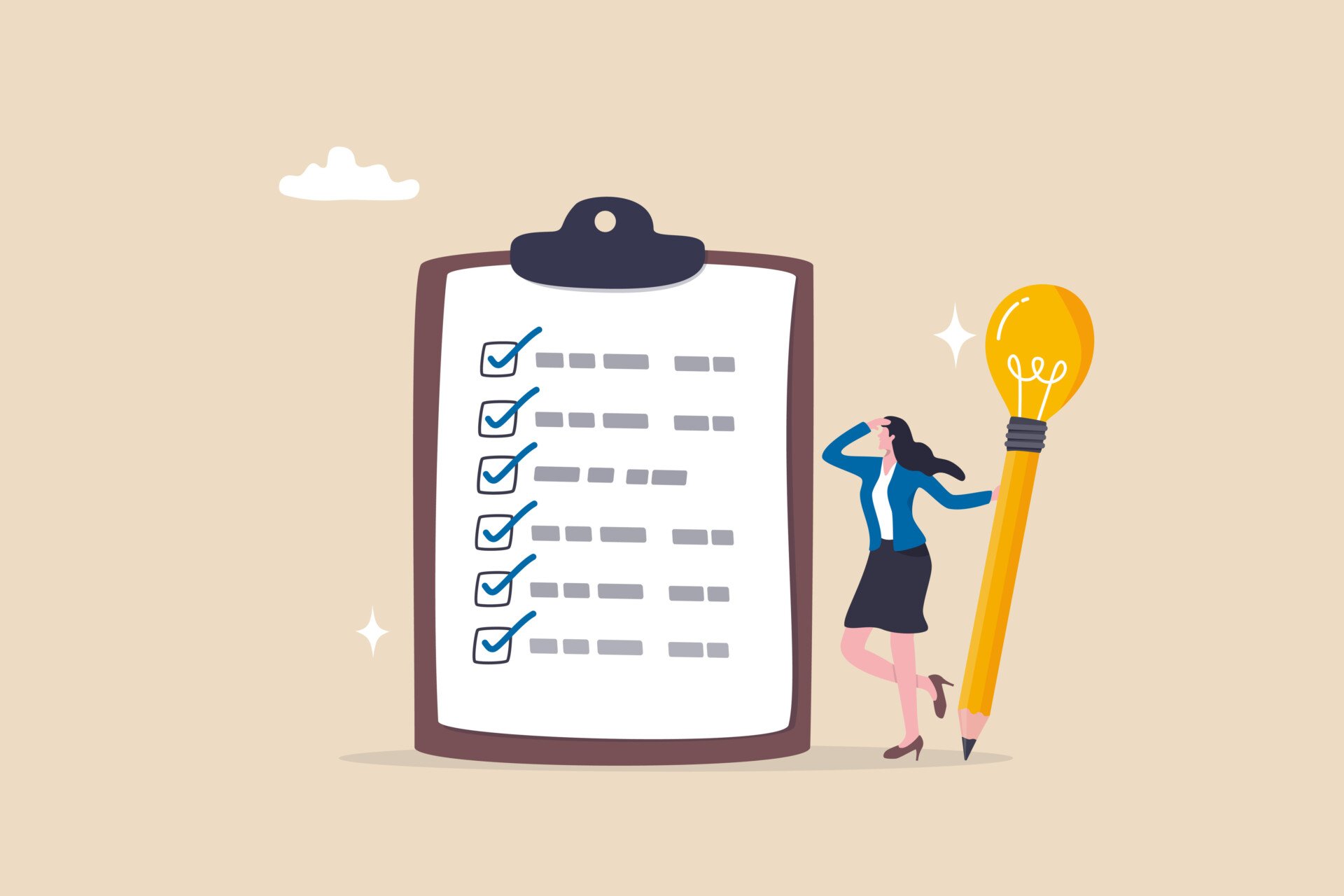
Content Validity in Research: Definition & Examples
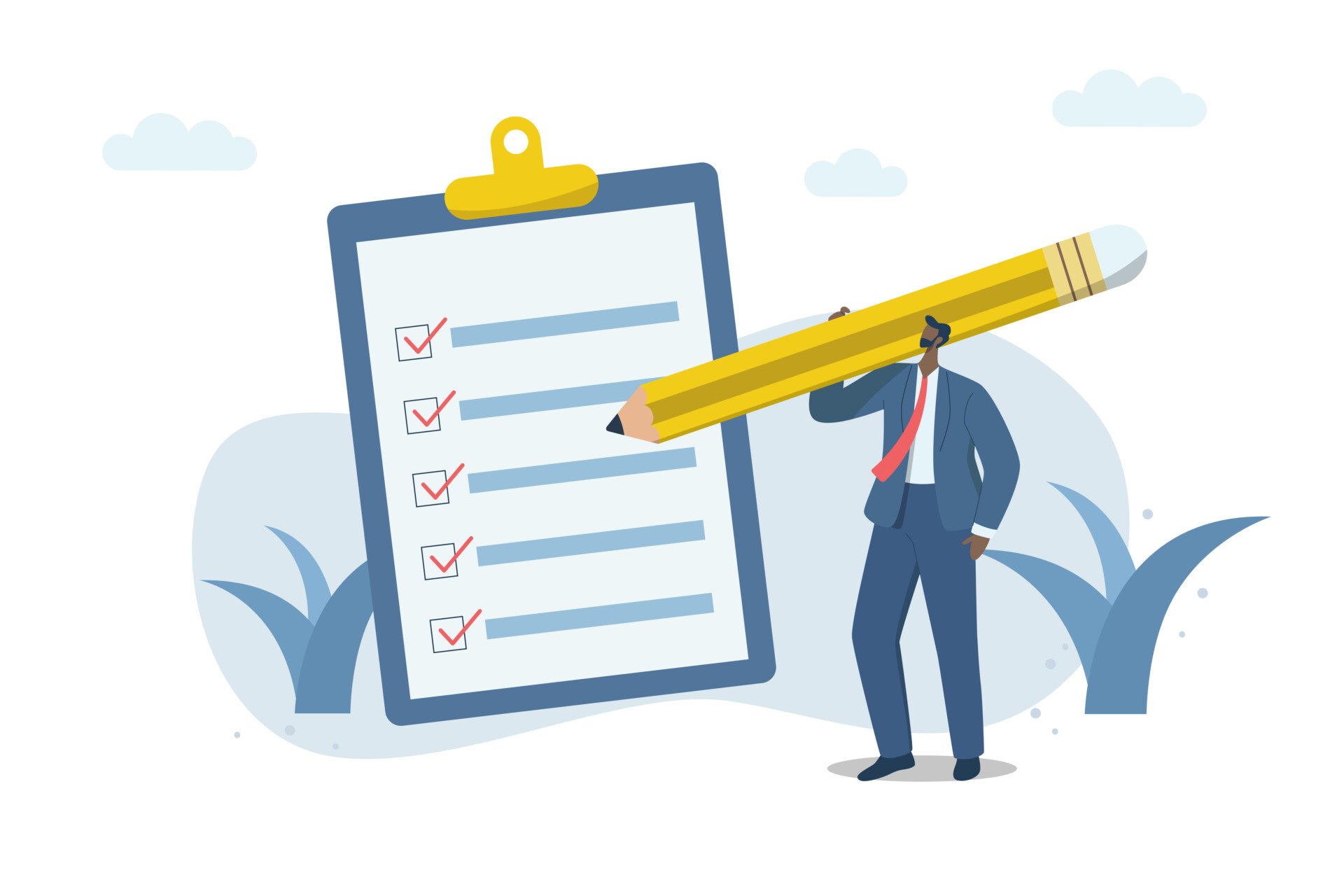
Construct Validity In Psychology Research
- Search Search Please fill out this field.
What Is a Type II Error?
- How It Works
- Type I vs. Type II Error
The Bottom Line
- Corporate Finance
- Financial Analysis
Type II Error: Definition, Example, vs. Type I Error
Adam Hayes, Ph.D., CFA, is a financial writer with 15+ years Wall Street experience as a derivatives trader. Besides his extensive derivative trading expertise, Adam is an expert in economics and behavioral finance. Adam received his master's in economics from The New School for Social Research and his Ph.D. from the University of Wisconsin-Madison in sociology. He is a CFA charterholder as well as holding FINRA Series 7, 55 & 63 licenses. He currently researches and teaches economic sociology and the social studies of finance at the Hebrew University in Jerusalem.
:max_bytes(150000):strip_icc():format(webp)/adam_hayes-5bfc262a46e0fb005118b414.jpg)
Michela Buttignol / Investopedia
A type II error is a statistical term used within the context of hypothesis testing that describes the error that occurs when one fails to reject a null hypothesis that is actually false. A type II error produces a false negative, also known as an error of omission.
For example, a test for a disease may report a negative result when the patient is infected. This is a type II error because we accept the conclusion of the test as negative, even though it is incorrect.
A type II error can be contrasted with a type I error , where researchers incorrectly reject a true null hypothesis. A type II error happens when one fails to reject a null hypothesis that is actually false. A type I error produces a false positive.
Key Takeaways
- A type II error is defined as the probability of incorrectly failing to reject the null hypothesis, when in fact it is not applicable to the entire population.
- A type II error is essentially a false negative.
- A type II error can be made less likely by making more stringent criteria for rejecting a null hypothesis, although this increases the chances of a false positive.
- The sample size, the true population size, and the preset alpha level influence the magnitude of risk of an error.
- Analysts need to weigh the likelihood and impact of type II errors with type I errors.
Understanding a Type II Error
A type II error, also known as an error of the second kind or a beta error, confirms an idea that should have been rejected—for instance, claiming that two observances are the same, despite them being different. A type II error does not reject the null hypothesis, even though the alternative hypothesis is actually correct. In other words, a false finding is accepted as true.
The likelihood of a type II error can be reduced by making more stringent criteria for rejecting a null hypothesis (H 0 ). For example, if an analyst is considering anything that falls within the +/- bounds of a 95% confidence interval as statistically insignificant (a negative result), then by decreasing that tolerance to +/- 90%, and subsequently narrowing the bounds, you will get fewer negative results, and thus reduce the chances of a false negative.
Taking these steps, however, tends to increase the chances of encountering a type I error—a false-positive result. When conducting a hypothesis test, the probability or risk of making a type I error or type II error should be considered.
The steps taken to reduce the chances of encountering a type II error tend to increase the probability of a type I error.
Type I Errors vs. Type II Errors
The difference between a type II error and a type I error is that a type I error rejects the null hypothesis when it is true (i.e., a false positive). The probability of committing a type I error is equal to the level of significance that was set for the hypothesis test. Therefore, if the level of significance is 0.05, there is a 5% chance that a type I error may occur.
The probability of committing a type II error is equal to one minus the power of the test, also known as beta. The power of the test could be increased by increasing the sample size, which decreases the risk of committing a type II error.
Some statistical literature will include overall significance level and type II error risk as part of the report’s analysis. For example, a 2021 meta-analysis of exosome in the treatment of spinal cord injury recorded an overall significance level of 0.05 and a type II error risk of 0.1.
Example of a Type II Error
Assume a biotechnology company wants to compare how effective two of its drugs are for treating diabetes. The null hypothesis states the two medications are equally effective. A null hypothesis, H 0 , is the claim that the company hopes to reject using the one-tailed test . The alternative hypothesis, H a , states that the two drugs are not equally effective. The alternative hypothesis, H a , is the state of nature that is supported by rejecting the null hypothesis.
The biotech company implements a large clinical trial of 3,000 patients with diabetes to compare the treatments. The company randomly divides the 3,000 patients into two equally sized groups, giving one group one of the treatments and the other group the other treatment. It selects a significance level of 0.05, which indicates it is willing to accept a 5% chance it may reject the null hypothesis when it is true or a 5% chance of committing a type I error.
Assume the beta is calculated to be 0.025, or 2.5%. Therefore, the probability of committing a type II error is 97.5%. If the two medications are not equal, the null hypothesis should be rejected. However, if the biotech company does not reject the null hypothesis when the drugs are not equally effective, then a type II error occurs.
What Is the Difference Between Type I and Type II Errors?
A type I error occurs if a null hypothesis is rejected that is actually true in the population. This type of error is representative of a false positive. Alternatively, a type II error occurs if a null hypothesis is not rejected that is actually false in the population. This type of error is representative of a false negative.
What Causes Type II Errors?
A type II error is commonly caused if the statistical power of a test is too low. The higher the statistical power, the greater the chance of avoiding an error. It’s often recommended that the statistical power should be set to at least 80% prior to conducting any testing.
What Factors Influence the Magnitude of Risk for Type II Errors?
As the sample size of a study increases, the risk of type II errors should decrease. As the true population effect size increases, the probability of a type II error should also decrease. Finally, the preset alpha level set by the research influences the magnitude of risk. As the alpha level set decreases, the risk of a type II error increases.
How Can a Type II Error Be Minimized?
It is not possible to fully prevent committing a type II error, but the risk can be minimized by increasing the sample size. However, doing so will also increase the risk of committing a type I error instead.
In statistics, a type II error results in a false negative—meaning that there is a finding, but it has been missed in the analysis (or that the null hypothesis is not rejected when it ought to have been). A type II error can occur if there is not enough power in statistical tests, often resulting from sample sizes that are too small. Increasing the sample size can help reduce the chances of committing a type II error.
Type II errors can be contrasted with type I errors, which are false positives.
Europe PMC. “ A Meta-Analysis of Exosome in the Treatment of Spinal Cord Injury .”
:max_bytes(150000):strip_icc():format(webp)/type_1_error.asp-final-157a4185cc544251ae1d79908393ca48.png)
- Terms of Service
- Editorial Policy
- Privacy Policy
Type II Error
"False negative" error
What is a Type II Error?
In statistical hypothesis testing, a type II error is a situation wherein a hypothesis test fails to reject the null hypothesis that is false. In other words, it causes the user to erroneously not reject the false null hypothesis because the test lacks the statistical power to detect sufficient evidence for the alternative hypothesis. The type II error is also known as a false negative.

The type II error has an inverse relationship with the power of a statistical test. This means that the higher power of a statistical test, the lower the probability of committing a type II error. The rate of a type II error (i.e., the probability of a type II error) is measured by beta (β) while the statistical power is measured by 1- β.
How to Avoid the Type II Error?
Similar to the type I error, it is not possible to completely eliminate the type II error from a hypothesis test . The only available option is to minimize the probability of committing this type of statistical error. Since a type II error is closely related to the power of a statistical test, the probability of the occurrence of the error can be minimized by increasing the power of the test.
1. Increase the sample size
One of the simplest methods to increase the power of the test is to increase the sample size used in a test. The sample size primarily determines the amount of sampling error, which translates into the ability to detect the differences in a hypothesis test. A larger sample size increases the chances to capture the differences in the statistical tests, as well as raises the power of a test.
2. Increase the significance level
Another method is to choose a higher level of significance . For instance, a researcher may choose a significance level of 0.10 instead of the commonly acceptable 0.05 level. The higher significance level implies a higher probability of rejecting the null hypothesis when it is true.
The larger probability of rejecting the null hypothesis decreases the probability of committing a type II error while the probability of committing a type I error increases. Thus, the user should always assess the impact of type I and type II errors on their decision and determine the appropriate level of statistical significance.
Practical Example
Sam is a financial analyst . He runs a hypothesis test to discover whether there is a difference in the average price changes for large-cap and small-cap stocks .
In the test, Sam assumes as the null hypothesis that there is no difference in the average price changes between large-cap and small-cap stocks. Thus, his alternative hypothesis states that a difference between the average price changes does exist.
For the significance level, Sam chooses 5%. This means that there is a 5% probability that his test will reject the null hypothesis when it is actually true.
If Sam’s test incurs a type II error, then the results of the test will indicate that there is no difference in the average price changes between large-cap and small-cap stocks. However, in reality, a difference in the average price changes does exist.
More Resources
CFI is the official provider of the global Business Intelligence & Data Analyst (BIDA)® certification program, designed to help anyone become a world-class financial analyst. To keep learning and advancing your career, the additional CFI resources below will be useful:
- Type I Error
- Conditional Probability
- Framing Bias
- Mutually Exclusive Events
- See all data science resources
- Share this article
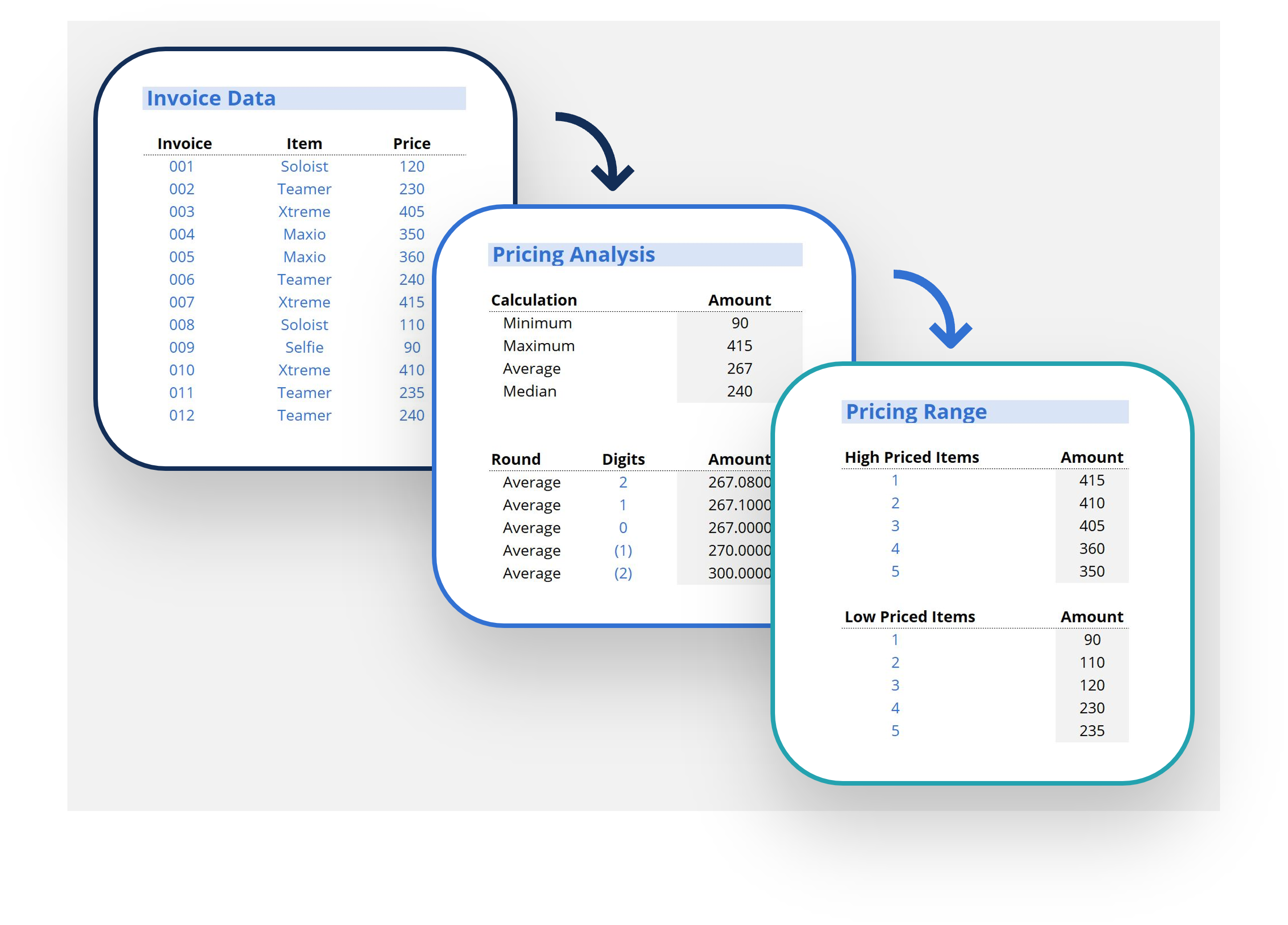
Create a free account to unlock this Template
Access and download collection of free Templates to help power your productivity and performance.
Already have an account? Log in
Supercharge your skills with Premium Templates
Take your learning and productivity to the next level with our Premium Templates.
Upgrading to a paid membership gives you access to our extensive collection of plug-and-play Templates designed to power your performance—as well as CFI's full course catalog and accredited Certification Programs.
Already have a Self-Study or Full-Immersion membership? Log in
Access Exclusive Templates
Gain unlimited access to more than 250 productivity Templates, CFI's full course catalog and accredited Certification Programs, hundreds of resources, expert reviews and support, the chance to work with real-world finance and research tools, and more.
Already have a Full-Immersion membership? Log in
Have a thesis expert improve your writing
Check your thesis for plagiarism in 10 minutes, generate your apa citations for free.
- Knowledge Base
- Type I & Type II Errors | Differences, Examples, Visualizations
Type I & Type II Errors | Differences, Examples, Visualizations
Published on 18 January 2021 by Pritha Bhandari . Revised on 2 February 2023.
In statistics , a Type I error is a false positive conclusion, while a Type II error is a false negative conclusion.
Making a statistical decision always involves uncertainties, so the risks of making these errors are unavoidable in hypothesis testing .
The probability of making a Type I error is the significance level , or alpha (α), while the probability of making a Type II error is beta (β). These risks can be minimized through careful planning in your study design.
- Type I error (false positive) : the test result says you have coronavirus, but you actually don’t.
- Type II error (false negative) : the test result says you don’t have coronavirus, but you actually do.
Table of contents
Error in statistical decision-making, type i error, type ii error, trade-off between type i and type ii errors, is a type i or type ii error worse, frequently asked questions about type i and ii errors.
Using hypothesis testing, you can make decisions about whether your data support or refute your research predictions with null and alternative hypotheses .
Hypothesis testing starts with the assumption of no difference between groups or no relationship between variables in the population—this is the null hypothesis . It’s always paired with an alternative hypothesis , which is your research prediction of an actual difference between groups or a true relationship between variables .
In this case:
- The null hypothesis (H 0 ) is that the new drug has no effect on symptoms of the disease.
- The alternative hypothesis (H 1 ) is that the drug is effective for alleviating symptoms of the disease.
Then , you decide whether the null hypothesis can be rejected based on your data and the results of a statistical test . Since these decisions are based on probabilities, there is always a risk of making the wrong conclusion.
- If your results show statistical significance , that means they are very unlikely to occur if the null hypothesis is true. In this case, you would reject your null hypothesis. But sometimes, this may actually be a Type I error.
- If your findings do not show statistical significance, they have a high chance of occurring if the null hypothesis is true. Therefore, you fail to reject your null hypothesis. But sometimes, this may be a Type II error.
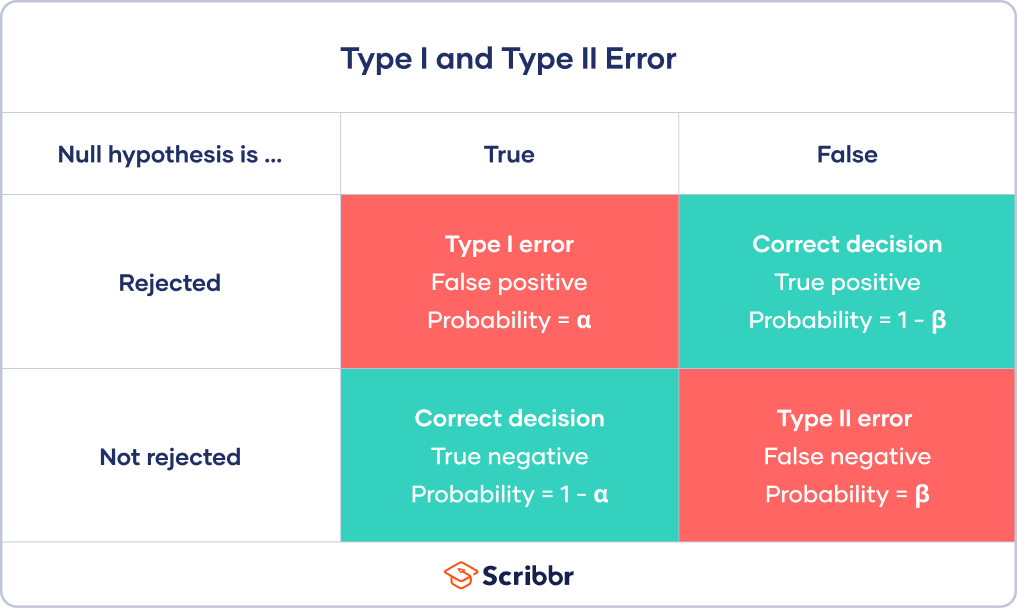
A Type I error means rejecting the null hypothesis when it’s actually true. It means concluding that results are statistically significant when, in reality, they came about purely by chance or because of unrelated factors.
The risk of committing this error is the significance level (alpha or α) you choose. That’s a value that you set at the beginning of your study to assess the statistical probability of obtaining your results ( p value).
The significance level is usually set at 0.05 or 5%. This means that your results only have a 5% chance of occurring, or less, if the null hypothesis is actually true.
If the p value of your test is lower than the significance level, it means your results are statistically significant and consistent with the alternative hypothesis. If your p value is higher than the significance level, then your results are considered statistically non-significant.
To reduce the Type I error probability, you can simply set a lower significance level.
Type I error rate
The null hypothesis distribution curve below shows the probabilities of obtaining all possible results if the study were repeated with new samples and the null hypothesis were true in the population .
At the tail end, the shaded area represents alpha. It’s also called a critical region in statistics.
If your results fall in the critical region of this curve, they are considered statistically significant and the null hypothesis is rejected. However, this is a false positive conclusion, because the null hypothesis is actually true in this case!
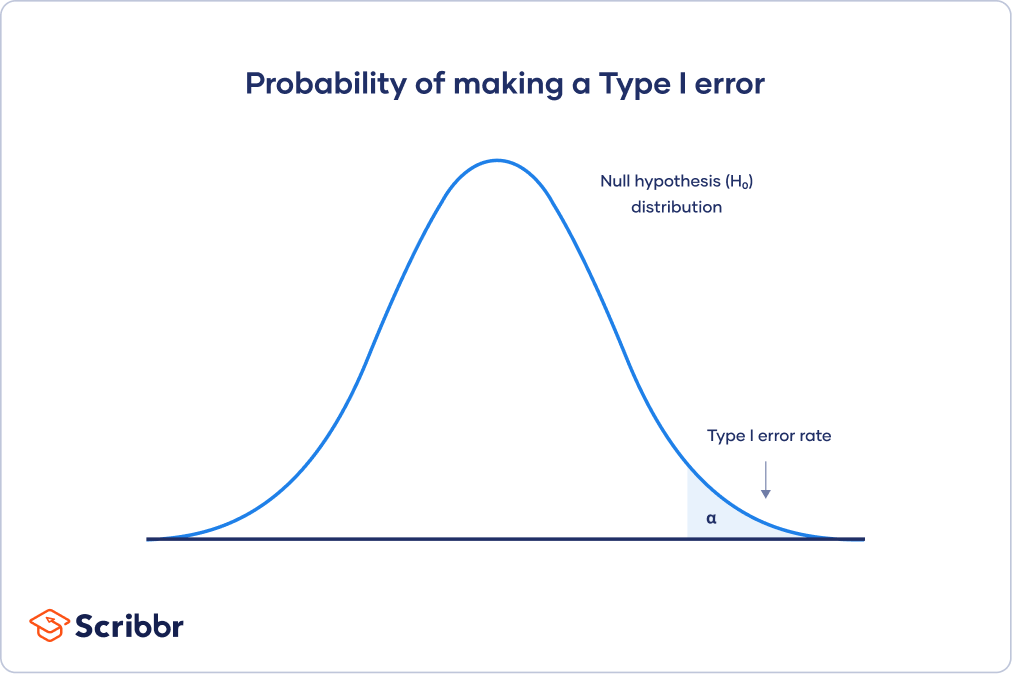
A Type II error means not rejecting the null hypothesis when it’s actually false. This is not quite the same as “accepting” the null hypothesis, because hypothesis testing can only tell you whether to reject the null hypothesis.
Instead, a Type II error means failing to conclude there was an effect when there actually was. In reality, your study may not have had enough statistical power to detect an effect of a certain size.
Power is the extent to which a test can correctly detect a real effect when there is one. A power level of 80% or higher is usually considered acceptable.
The risk of a Type II error is inversely related to the statistical power of a study. The higher the statistical power, the lower the probability of making a Type II error.
Statistical power is determined by:
- Size of the effect : Larger effects are more easily detected.
- Measurement error : Systematic and random errors in recorded data reduce power.
- Sample size : Larger samples reduce sampling error and increase power.
- Significance level : Increasing the significance level increases power.
To (indirectly) reduce the risk of a Type II error, you can increase the sample size or the significance level.
Type II error rate
The alternative hypothesis distribution curve below shows the probabilities of obtaining all possible results if the study were repeated with new samples and the alternative hypothesis were true in the population .
The Type II error rate is beta (β), represented by the shaded area on the left side. The remaining area under the curve represents statistical power, which is 1 – β.
Increasing the statistical power of your test directly decreases the risk of making a Type II error.
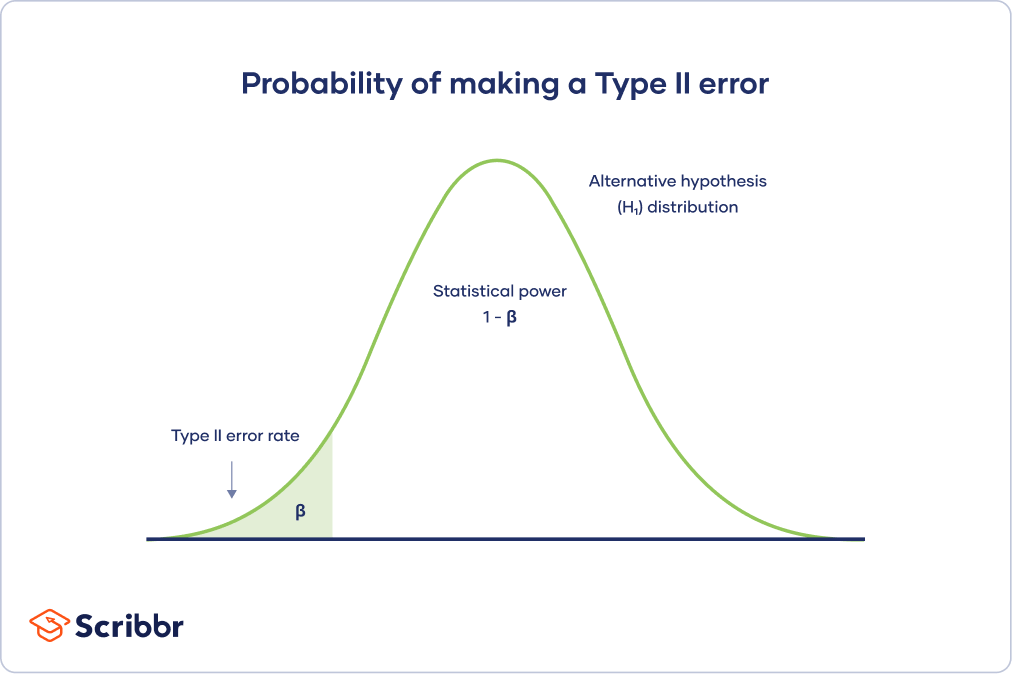
The Type I and Type II error rates influence each other. That’s because the significance level (the Type I error rate) affects statistical power, which is inversely related to the Type II error rate.
This means there’s an important tradeoff between Type I and Type II errors:
- Setting a lower significance level decreases a Type I error risk, but increases a Type II error risk.
- Increasing the power of a test decreases a Type II error risk, but increases a Type I error risk.
This trade-off is visualized in the graph below. It shows two curves:
- The null hypothesis distribution shows all possible results you’d obtain if the null hypothesis is true. The correct conclusion for any point on this distribution means not rejecting the null hypothesis.
- The alternative hypothesis distribution shows all possible results you’d obtain if the alternative hypothesis is true. The correct conclusion for any point on this distribution means rejecting the null hypothesis.
Type I and Type II errors occur where these two distributions overlap. The blue shaded area represents alpha, the Type I error rate, and the green shaded area represents beta, the Type II error rate.
By setting the Type I error rate, you indirectly influence the size of the Type II error rate as well.
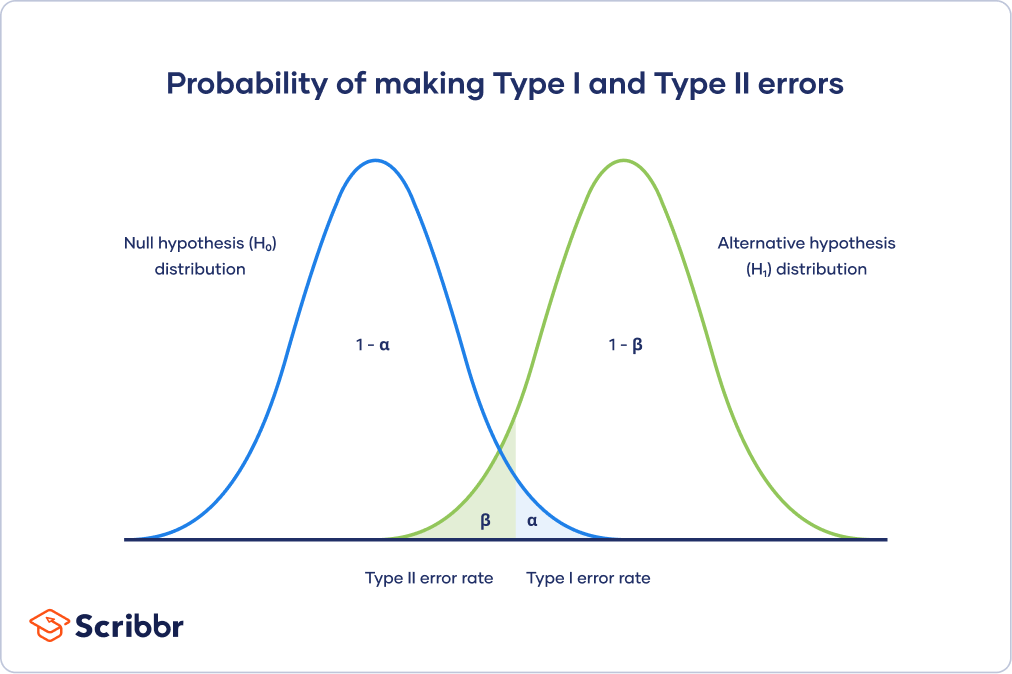
It’s important to strike a balance between the risks of making Type I and Type II errors. Reducing the alpha always comes at the cost of increasing beta, and vice versa .
For statisticians, a Type I error is usually worse. In practical terms, however, either type of error could be worse depending on your research context.
A Type I error means mistakenly going against the main statistical assumption of a null hypothesis. This may lead to new policies, practices or treatments that are inadequate or a waste of resources.
In contrast, a Type II error means failing to reject a null hypothesis. It may only result in missed opportunities to innovate, but these can also have important practical consequences.
In statistics, a Type I error means rejecting the null hypothesis when it’s actually true, while a Type II error means failing to reject the null hypothesis when it’s actually false.
The risk of making a Type I error is the significance level (or alpha) that you choose. That’s a value that you set at the beginning of your study to assess the statistical probability of obtaining your results ( p value ).
To reduce the Type I error probability, you can set a lower significance level.
The risk of making a Type II error is inversely related to the statistical power of a test. Power is the extent to which a test can correctly detect a real effect when there is one.
To (indirectly) reduce the risk of a Type II error, you can increase the sample size or the significance level to increase statistical power.
Statistical significance is a term used by researchers to state that it is unlikely their observations could have occurred under the null hypothesis of a statistical test . Significance is usually denoted by a p -value , or probability value.
Statistical significance is arbitrary – it depends on the threshold, or alpha value, chosen by the researcher. The most common threshold is p < 0.05, which means that the data is likely to occur less than 5% of the time under the null hypothesis .
When the p -value falls below the chosen alpha value, then we say the result of the test is statistically significant.
In statistics, power refers to the likelihood of a hypothesis test detecting a true effect if there is one. A statistically powerful test is more likely to reject a false negative (a Type II error).
If you don’t ensure enough power in your study, you may not be able to detect a statistically significant result even when it has practical significance. Your study might not have the ability to answer your research question.
Cite this Scribbr article
If you want to cite this source, you can copy and paste the citation or click the ‘Cite this Scribbr article’ button to automatically add the citation to our free Reference Generator.
Bhandari, P. (2023, February 02). Type I & Type II Errors | Differences, Examples, Visualizations. Scribbr. Retrieved 11 June 2024, from https://www.scribbr.co.uk/stats/type-i-and-type-ii-error/
Is this article helpful?
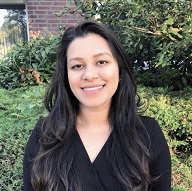
Pritha Bhandari
Type I and Type II Error (Decision Error): Definition, Examples
> Statistics Definitions > Type I and Type II Error
- What is Type I Error?
- What is Type II Error?
- Examples of Type I and Type II Error
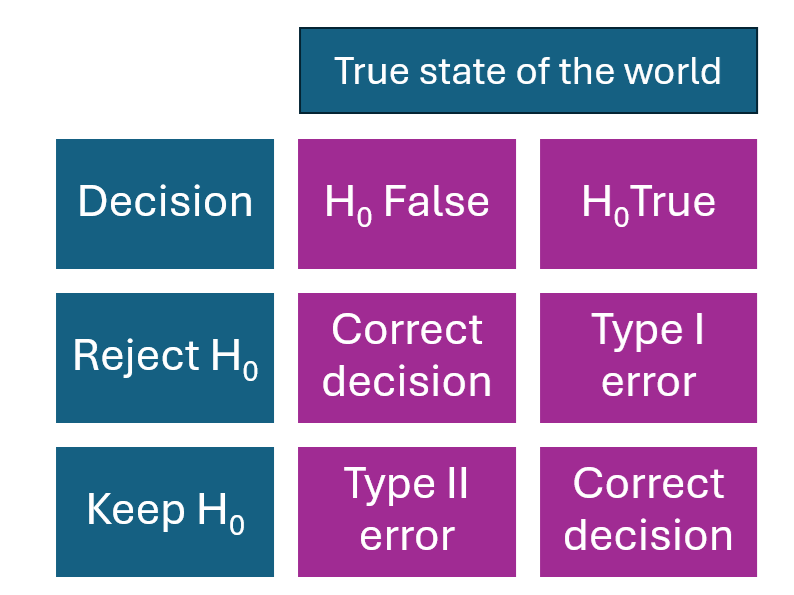
Type I & Type II Error: What is Type I Error?
A Type I error (or Type 1), is the incorrect rejection of a true null hypothesis . The alpha symbol, α, is usually used to denote a Type I error.
The null hypothesis , H 0 is a commonly accepted hypothesis ; it is the opposite of the alternate hypothesis . Researchers come up with an alternate hypothesis, one that they think explains a phenomenon, and then work to reject the null hypothesis. If that sounds a little convoluted, an example might help. Back in the day (way back!) scientists thought that the Earth was at the center of the Universe. That meant everything else—the sun, the planets, the Oort cloud—revolved around the Earth.
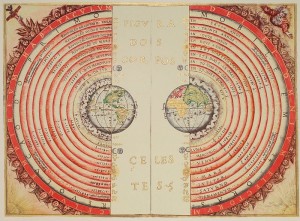
This Geocentric model , where the Earth is at the center of the universe, has since been proven false. So the current, accepted hypothesis (the null) is:
- H 0 : The Earth IS NOT at the center of the Universe.
And the alternate hypothesis (the challenge to the null hypothesis) would be:
- H 1 : The Earth IS at the center of the Universe.
Type I Error: Conducting a Test
In our sample test ( Is the Earth at the center of the Universe? ), the null hypothesis is: H 0 : The Earth is not at the center of the Universe Let’s say you’re an amateur astronomer and you’re convinced they’ve all got it wrong. You want to prove that the Earth IS at the center of the Universe. You set out to prove the alternate hypothesis and sit and watch the night sky for a few days, noticing that hey—it looks like all that stuff in the sky is revolving around the Earth! You therefore reject the null hypothesis and proudly announce that the alternate hypothesis is true; The Earth is, in fact, at the center of the Universe. That’s a very simplified explanation of a Type I Error. Of course, it’s a little more complicated than that in real life (or in this case, in statistics). But basically, when you’re conducting any kind of test, you want to minimize the chance that you could make a Type I error. In the case of the amateur astronomer, you could probably have avoided a Type I error by reading some scientific journals.
Type I & Type II Error: What is Type II Error?
A Type II error (sometimes called a Type 2 error) is the failure to reject a false null hypothesis. The probability of a type II error is denoted by the beta symbol β.
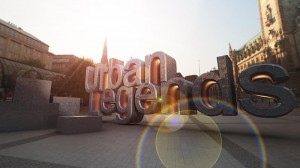
Let’s say you’re an urban legend researcher and you want to research if people believe in legends such as:
- Newton was hit by an apple (he wasn’t).
- Walt Disney drew Mickey mouse (he didn’t— Ub Werks did).
- Marie Antoinette said “Let them eat cake” (she didn’t).
The accepted fact is, most people probably believe in urban legends (or we wouldn’t need Snopes.com)*. So, your null hypothesis is:
- H 0 : Most people do believe in urban legends.
But let’s say that null hypothesis is completely wrong. It might have been true ten years ago, but with the advent of the Smartphone —we have Snopes.com and Google.com at our fingertips. Still, your job as a researcher is to try and disprove the null hypothesis. So you come up with an alternate hypothesis:
- H 1 : Most people DO NOT believe in urban legends.
You conduct your research by polling local residents at a retirement community and to your surprise you find out that most people do believe in urban legends. The problem is, you didn’t account for the fact that your sampling method introduced some bias: retired folks are less likely to have access to tools like Smartphones than the general population. So you incorrectly fail to reject the false null hypothesis that most people do believe in urban legends (in other words, most people do not, and you failed to prove that). You’ve committed an egregious Type II error, the penalty for which is banishment from the scientific community. *I used this simple statement as an example of Type I and Type II errors. I haven’t actually researched this statement, so as well as committing numerous errors myself, I’m probably also guilty of sloppy science.
Type I and Type II Error: Examples
We’ll start off using a sample size of 100 and .4 to .6 boundary lines to make a 95% confidence interval for testing coins. Any coin whose proportion of heads lies outside the interval we’ll declare unfair. Only 5% of the time will a fair coin mislead us and lie outside the interval, leading us to erroneously declare it unfair. This is Type I Error. What about unfair coins that mislead us and lie inside the interval? That will lead us to erroneously declare them fair. This is Type II Error. Let’s explore these two types of potential errors with some examples.
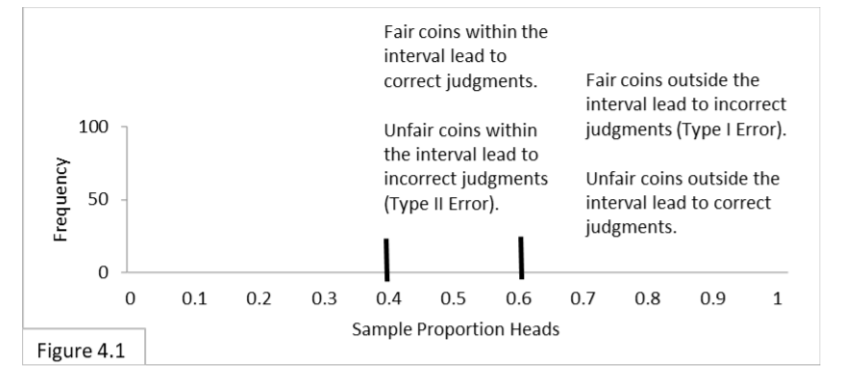
- That ½ of the coins in the basket are fair and ½ are unfair, and
- that the unfair coins are all identical and favor heads ¾ versus tails ¼,
then we could do some calculations to determine how likely it is that your judgments are correct. But we don’t know those things. We can make use of estimates of those things. In Frequentist statistics , we can, if we wish, estimate values for #2 in order to then estimate the likelihood of Type II Error. We’ll explore that next. But we do not make nor use estimates for #1. (Estimates for #1 are used to determine what’s called the false discovery rate .
Exploring Type II Error
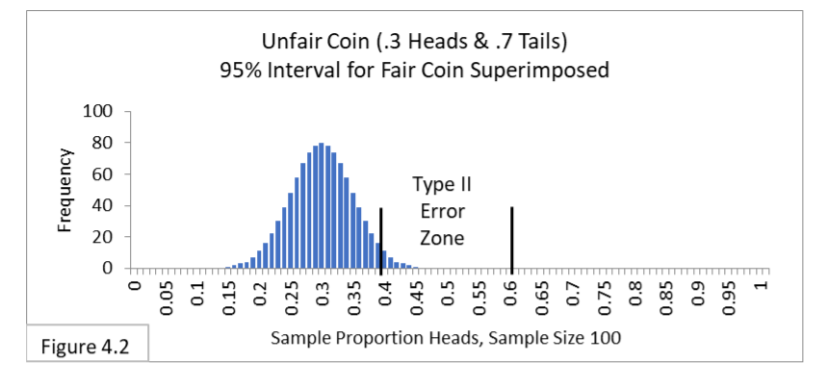
99% Intervals and Their Effects on Type I and Type II Error
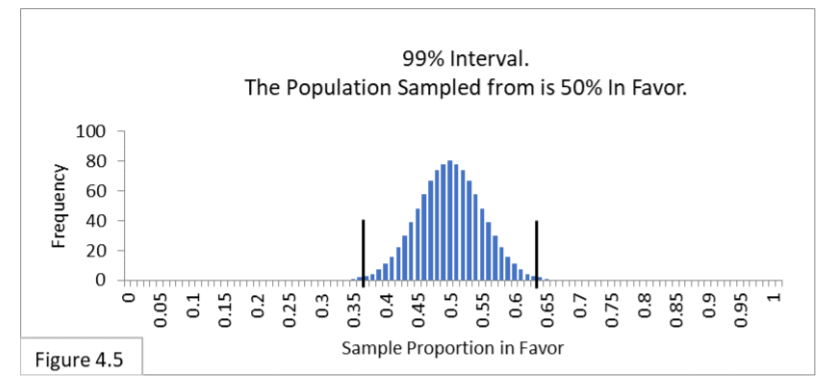
As we’ve just seen, the likelihood of Type II Error is determined by a number of factors, some within our control and some not. In summary, the likelihood of Type II Errors increases:
- the closer something is to what we’re testing for (.4 is closer to .5 than .3 is, and so .4 coins make Type II Errors more likely than .3 coins). We don’t control this.
- when we use smaller sample sizes (a sample size of 100 makes Type II Errors more likely than a sample size of 1000). We do control this, although larger samples cost more to gather.
- when we use stricter Type I Error criteria (a 99% interval is stricter for Type I Error and makes Type II Error more likely than a 95% interval—but keep in mind that Type I Error becomes less likely; it’s a trade-off). We do control this, but in practice the strictness of our Type I Error criteria is usually set by convention (social sciences use 95% and sometimes 99%; physical sciences use 95% through 99.9999% depending on the specific field).
Decreasing the likelihood of Type II Error increases the statistical power of our analysis. In practice, increasing the sample size is a common way to reduce the likelihood of Type II Error. Moreover, there are formulas and software tools (including online calculators) available that help researchers estimate what their sample size should be in order to achieve their desired levels of Type I and Type II Error. With these tools you enter your estimated value for the population proportion , your expected sample proportion value, and your desired Type I and II Error rates. Keep in mind that, since you don’t actually know what the population proportion is nor what your sample proportion will be, the sample size recommendations of these tools are “educated-guess” estimates.
In practice, Type I Error is feared more than Type II Error. In the social sciences the Type I Error limit is most often set at 5% via a 95% confidence interval. With surveys, for example, we want to limit how often we infer that a majority of the population is in favor of a new policy when in fact a majority is not in favor. What about Type II Error? That occurs if we infer that a majority of the population is not in favor of the new policy when in fact a majority is in favor. There is no well established convention, but the most common guidance is to try and limit Type II Error to 20% (by having large enough sample sizes). These guidelines of 5% and 20% imply that we prefer to error on the conservative side, maintaining the status quo. Using our surveying context, this means that statistical survey evidence is more likely to erroneously undermine new policies than to erroneously provide support for them. While 95% and 99% intervals are common, much stricter Type I Error criteria are used in particle physics: 99.9999% (approximately). Using this interval with coin flipping, we would insist that a coin come up heads at least 75 times in 100 flips before declaring it unfair. Biologists conducting what are called genome-wide association studies use a similarly strict interval. Since these researchers test hundreds of thousands or even millions of separate genome locations, Type I Errors would be expected to occur far too frequently if they used a 95% or 99% interval. After all, 5% of 1,000,000 is 50,000 and 1% of 1,000,000 is 10,000.
- Skip to secondary menu
- Skip to main content
- Skip to primary sidebar
Statistics By Jim
Making statistics intuitive
Types I & Type II Errors in Hypothesis Testing
By Jim Frost 8 Comments
In hypothesis testing, a Type I error is a false positive while a Type II error is a false negative. In this blog post, you will learn about these two types of errors, their causes, and how to manage them.
Hypothesis tests use sample data to make inferences about the properties of a population . You gain tremendous benefits by working with random samples because it is usually impossible to measure the entire population.
However, there are tradeoffs when you use samples. The samples we use are typically a minuscule percentage of the entire population. Consequently, they occasionally misrepresent the population severely enough to cause hypothesis tests to make Type I and Type II errors.
Potential Outcomes in Hypothesis Testing
Hypothesis testing is a procedure in inferential statistics that assesses two mutually exclusive theories about the properties of a population. For a generic hypothesis test, the two hypotheses are as follows:
- Null hypothesis : There is no effect
- Alternative hypothesis : There is an effect.
The sample data must provide sufficient evidence to reject the null hypothesis and conclude that the effect exists in the population. Ideally, a hypothesis test fails to reject the null hypothesis when the effect is not present in the population, and it rejects the null hypothesis when the effect exists.
Statisticians define two types of errors in hypothesis testing. Creatively, they call these errors Type I and Type II errors. Both types of error relate to incorrect conclusions about the null hypothesis.
The table summarizes the four possible outcomes for a hypothesis test.
|
| |
|
|
Related post : How Hypothesis Tests Work: P-values and the Significance Level
Fire alarm analogy for the types of errors

Using hypothesis tests correctly improves your chances of drawing trustworthy conclusions. However, errors are bound to occur.
Unlike the fire alarm analogy, there is no sure way to determine whether an error occurred after you perform a hypothesis test. Typically, a clearer picture develops over time as other researchers conduct similar studies and an overall pattern of results appears. Seeing how your results fit in with similar studies is a crucial step in assessing your study’s findings.
Now, let’s take a look at each type of error in more depth.
Type I Error: False Positives
When you see a p-value that is less than your significance level , you get excited because your results are statistically significant. However, it could be a type I error . The supposed effect might not exist in the population. Again, there is usually no warning when this occurs.
Why do these errors occur? It comes down to sample error. Your random sample has overestimated the effect by chance. It was the luck of the draw. This type of error doesn’t indicate that the researchers did anything wrong. The experimental design, data collection, data validity , and statistical analysis can all be correct, and yet this type of error still occurs.
Even though we don’t know for sure which studies have false positive results, we do know their rate of occurrence. The rate of occurrence for Type I errors equals the significance level of the hypothesis test, which is also known as alpha (α).
The significance level is an evidentiary standard that you set to determine whether your sample data are strong enough to reject the null hypothesis. Hypothesis tests define that standard using the probability of rejecting a null hypothesis that is actually true. You set this value based on your willingness to risk a false positive.
Related post : How to Interpret P-values Correctly
Using the significance level to set the Type I error rate
When the significance level is 0.05 and the null hypothesis is true, there is a 5% chance that the test will reject the null hypothesis incorrectly. If you set alpha to 0.01, there is a 1% of a false positive. If 5% is good, then 1% seems even better, right? As you’ll see, there is a tradeoff between Type I and Type II errors. If you hold everything else constant, as you reduce the chance for a false positive, you increase the opportunity for a false negative.
Type I errors are relatively straightforward. The math is beyond the scope of this article, but statisticians designed hypothesis tests to incorporate everything that affects this error rate so that you can specify it for your studies. As long as your experimental design is sound, you collect valid data, and the data satisfy the assumptions of the hypothesis test, the Type I error rate equals the significance level that you specify. However, if there is a problem in one of those areas, it can affect the false positive rate.
Warning about a potential misinterpretation of Type I errors and the Significance Level
When the null hypothesis is correct for the population, the probability that a test produces a false positive equals the significance level. However, when you look at a statistically significant test result, you cannot state that there is a 5% chance that it represents a false positive.
Why is that the case? Imagine that we perform 100 studies on a population where the null hypothesis is true. If we use a significance level of 0.05, we’d expect that five of the studies will produce statistically significant results—false positives. Afterward, when we go to look at those significant studies, what is the probability that each one is a false positive? Not 5 percent but 100%!
That scenario also illustrates a point that I made earlier. The true picture becomes more evident after repeated experimentation. Given the pattern of results that are predominantly not significant, it is unlikely that an effect exists in the population.
Type II Error: False Negatives
When you perform a hypothesis test and your p-value is greater than your significance level, your results are not statistically significant. That’s disappointing because your sample provides insufficient evidence for concluding that the effect you’re studying exists in the population. However, there is a chance that the effect is present in the population even though the test results don’t support it. If that’s the case, you’ve just experienced a Type II error . The probability of making a Type II error is known as beta (β).
What causes Type II errors? Whereas Type I errors are caused by one thing, sample error, there are a host of possible reasons for Type II errors—small effect sizes, small sample sizes, and high data variability. Furthermore, unlike Type I errors, you can’t set the Type II error rate for your analysis. Instead, the best that you can do is estimate it before you begin your study by approximating properties of the alternative hypothesis that you’re studying. When you do this type of estimation, it’s called power analysis.
To estimate the Type II error rate, you create a hypothetical probability distribution that represents the properties of a true alternative hypothesis. However, when you’re performing a hypothesis test, you typically don’t know which hypothesis is true, much less the specific properties of the distribution for the alternative hypothesis. Consequently, the true Type II error rate is usually unknown!
Type II errors and the power of the analysis
The Type II error rate (beta) is the probability of a false negative. Therefore, the inverse of Type II errors is the probability of correctly detecting an effect. Statisticians refer to this concept as the power of a hypothesis test. Consequently, 1 – β = the statistical power. Analysts typically estimate power rather than beta directly.
If you read my post about power and sample size analysis , you know that the three factors that affect power are sample size, variability in the population, and the effect size. As you design your experiment, you can enter estimates of these three factors into statistical software and it calculates the estimated power for your test.
Suppose you perform a power analysis for an upcoming study and calculate an estimated power of 90%. For this study, the estimated Type II error rate is 10% (1 – 0.9). Keep in mind that variability and effect size are based on estimates and guesses. Consequently, power and the Type II error rate are just estimates rather than something you set directly. These estimates are only as good as the inputs into your power analysis.
Low variability and larger effect sizes decrease the Type II error rate, which increases the statistical power. However, researchers usually have less control over those aspects of a hypothesis test. Typically, researchers have the most control over sample size, making it the critical way to manage your Type II error rate. Holding everything else constant, increasing the sample size reduces the Type II error rate and increases power.
Learn more about Power in Statistics .
Graphing Type I and Type II Errors
The graph below illustrates the two types of errors using two sampling distributions. The critical region line represents the point at which you reject or fail to reject the null hypothesis. Of course, when you perform the hypothesis test, you don’t know which hypothesis is correct. And, the properties of the distribution for the alternative hypothesis are usually unknown. However, use this graph to understand the general nature of these errors and how they are related.

The distribution on the left represents the null hypothesis. If the null hypothesis is true, you only need to worry about Type I errors, which is the shaded portion of the null hypothesis distribution. The rest of the null distribution represents the correct decision of failing to reject the null.
On the other hand, if the alternative hypothesis is true, you need to worry about Type II errors. The shaded region on the alternative hypothesis distribution represents the Type II error rate. The rest of the alternative distribution represents the probability of correctly detecting an effect—power.
Moving the critical value line is equivalent to changing the significance level. If you move the line to the left, you’re increasing the significance level (e.g., α 0.05 to 0.10). Holding everything else constant, this adjustment increases the Type I error rate while reducing the Type II error rate. Moving the line to the right reduces the significance level (e.g., α 0.05 to 0.01), which decreases the Type I error rate but increases the type II error rate.
Is One Error Worse Than the Other?
As you’ve seen, the nature of the two types of error, their causes, and the certainty of their rates of occurrence are all very different.
A common question is whether one type of error is worse than the other? Statisticians designed hypothesis tests to control Type I errors while Type II errors are much less defined. Consequently, many statisticians state that it is better to fail to detect an effect when it exists than it is to conclude an effect exists when it doesn’t. That is to say, there is a tendency to assume that Type I errors are worse.
However, reality is more complex than that. You should carefully consider the consequences of each type of error for your specific test.
Suppose you are assessing the strength of a new jet engine part that is under consideration. Peoples lives are riding on the part’s strength. A false negative in this scenario merely means that the part is strong enough but the test fails to detect it. This situation does not put anyone’s life at risk. On the other hand, Type I errors are worse in this situation because they indicate the part is strong enough when it is not.
Now suppose that the jet engine part is already in use but there are concerns about it failing. In this case, you want the test to be more sensitive to detecting problems even at the risk of false positives. Type II errors are worse in this scenario because the test fails to recognize the problem and leaves these problematic parts in use for longer.
Using hypothesis tests effectively requires that you understand their error rates. By setting the significance level and estimating your test’s power, you can manage both error rates so they meet your requirements.
The error rates in this post are all for individual tests. If you need to perform multiple comparisons, such as comparing group means in ANOVA, you’ll need to use post hoc tests to control the experiment-wise error rate or use the Bonferroni correction .
Share this:

Reader Interactions
June 4, 2024 at 2:04 pm
Very informative.
June 9, 2023 at 9:54 am
Hi Jim- I just signed up for your newsletter and this is my first question to you. I am not a statistician but work with them in my professional life as a QC consultant in biopharmaceutical development. I have a question about Type I and Type II errors in the realm of equivalence testing using two one sided difference testing (TOST). In a recent 2020 publication that I co-authored with a statistician, we stated that the probability of concluding non-equivalence when that is the truth, (which is the opposite of power, the probability of concluding equivalence when it is correct) is 1-2*alpha. This made sense to me because one uses a 90% confidence interval on a mean to evaluate whether the result is within established equivalence bounds with an alpha set to 0.05. However, it appears that specificity (1-alpha) is always the case as is power always being 1-beta. For equivalence testing the latter is 1-2*beta/2 but for specificity it stays as 1-alpha because only one of the null hypotheses in a two-sided test can fail at one time. I still see 1-2*alpha as making more sense as we show in Figure 3 of our paper which shows the white space under the distribution of the alternative hypothesis as 1-2 alpha. The paper can be downloaded as open access here if that would make my question more clear. https://bioprocessingjournal.com/index.php/article-downloads/890-vol-19-open-access-2020-defining-therapeutic-window-for-viral-vectors-a-statistical-framework-to-improve-consistency-in-assigning-product-dose-values I have consulted with other statistical colleagues and cannot get consensus so I would love your opinion and explanation! Thanks in advance!
June 10, 2023 at 1:00 am
Let me preface my response by saying that I’m not an expert in equivalence testing. But here’s my best guess about your question.
The alpha is for each of the hypothesis tests. Each one has a type I error rate of 0.05. Or, as you say, a specificity of 1-alpha. However, there are two tests so we need to consider the family-wise error rate. The formula is the following:
FWER = 1 – (1 – α)^N
Where N is the number of hypothesis tests.
For two tests, there’s a family-wise error rate of 0.0975. Or a family-wise specificity of 0.9025.
However, I believe they use 90% CI for a different reason (although it’s a very close match to the family-wise error rate). The 90% CI provides consistent results with the two one-side 95% tests. In other words, if the 90% CI is within the equivalency bounds, then the two tests will be significant. If the CI extends above the upper bound, the corresponding test won’t be significant. Etc.
However, using either rational, I’d say the overall type I error rate is about 0.1.
I hope that answers your question. And, again, I’m not an expert in this particular test.
July 18, 2022 at 5:15 am
Thank you for your valuable content. I have a question regarding correcting for multiple tests. My question is: for exactly how many tests should I correct in the scenario below?
Background: I’m testing for differences between groups A (patient group) and B (control group) in variable X. Variable X is a biological variable present in the body’s left and right side. Variable Y is a questionnaire for group A.
Step 1. Is there a significant difference within groups in the weight of left and right variable X? (I will conduct two paired sample t-tests)
If I find a significant difference in step 1, then I will conduct steps 2A and 2B. However, if I don’t find a significant difference in step 1, then I will only conduct step 2C.
Step 2A. Is there a significant difference between groups in left variable X? (I will conduct one independent sample t-test) Step 2B. Is there a significant difference between groups in right variable X? (I will conduct one independent sample t-test)
Step 2C. Is there a significant difference between groups in total variable X (left + right variable X)? (I will conduct one independent sample t-test)
If I find a significant difference in step 1, then I will conduct with steps 3A and 3B. However, if I don’t find a significant difference in step 1, then I will only conduct step 3C.
Step 3A. Is there a significant correlation between left variable X in group A and variable Y? (I will conduct Pearson correlation) Step 3B. Is there a significant correlation between right variable X in group A and variable Y? (I will conduct Pearson correlation)
Step 3C. Is there a significant correlation between total variable X in group A and variable Y? (I will conduct a Pearson correlation)
Regards, De
January 2, 2021 at 1:57 pm
I should say that being a budding statistician, this site seems to be pretty reliable. I have few doubts in here. It would be great if you can clarify it:
“A significance level of 0.05 indicates a 5% risk of concluding that a difference exists when there is no actual difference. ”
My understanding : When we say that the significance level is 0.05 then it means we are taking 5% risk to support alternate hypothesis even though there is no difference ?( I think i am not allowed to say Null is true, because null is assumed to be true/ Right)
January 2, 2021 at 6:48 pm
The sentence as I write it is correct. Here’s a simple way to understand it. Imagine you’re conducting a computer simulation where you control the population parameters and have the computer draw random samples from the populations that you define. Now, imagine you draw samples from two populations where the means and standard deviations are equal. You know this for a fact because you set the parameters yourself. Then you conduct a series of 2-sample t-tests.
In this example, you know the null hypothesis is correct. However, thanks to random sampling error, some proportion of the t-tests will have statistically significant results (i.e., false positives or Type I errors). The proportion of false positives will equal your significance level over the long run.
Of course, in real-world experiments, you never know for sure whether the null is true or not. However, given the properties of the hypothesis, you do know what proportion of tests will give you a false positive IF the null is true–and that’s the significance level.
I’m thinking through the wording of how you wrote it and I believe it is equivalent to what I wrote. If there is no difference (the null is true), then you have a 5% chance of incorrectly supporting the alternative. And, again, you’re correct that in the real world you don’t know for sure whether the null is true. But, you can still know the false positive (Type I) error rate. For more information about that property, read my post about how hypothesis tests work .
July 9, 2018 at 11:43 am
I like to use the analogy of a trial. The null hypothesis is that the defendant is innocent. A type I error would be convicting an innocent person and a type II error would be acquitting a guilty one. I like to think that our system makes a type I error very unlikely with the trade off being that a type II error is greater.
July 9, 2018 at 12:03 pm
Hi Doug, I think that is an excellent analogy on multiple levels. As you mention, a trial would set a high bar for the significance level by choosing a very low value for alpha. This helps prevent innocent people from being convicted (Type I error) but does increase the probability of allowing the guilty to go free (Type II error). I often refer to the significant level as a evidentiary standard with this legalistic analogy in mind.
Additionally, in the justice system in the U.S., there is a presumption of innocence and the prosecutor must present sufficient evidence to prove that the defendant is guilty. That’s just like in a hypothesis test where the assumption is that the null hypothesis is true and your sample must contain sufficient evidence to be able to reject the null hypothesis and suggest that the effect exists in the population.
This analogy even works for the similarities behind the phrases “Not guilty” and “Fail to reject the null hypothesis.” In both cases, you aren’t proving innocence or that the null hypothesis is true. When a defendant is “not guilty” it might be that the evidence was insufficient to convince the jury. In a hypothesis test, when you fail to reject the null hypothesis, it’s possible that an effect exists in the population but you have insufficient evidence to detect it. Perhaps the effect exists but the sample size or effect size is too small, or the variability might be too high.
Comments and Questions Cancel reply
User Preferences
Content preview.
Arcu felis bibendum ut tristique et egestas quis:
- Ut enim ad minim veniam, quis nostrud exercitation ullamco laboris
- Duis aute irure dolor in reprehenderit in voluptate
- Excepteur sint occaecat cupidatat non proident
Keyboard Shortcuts
6.1 - type i and type ii errors.
When conducting a hypothesis test there are two possible decisions: reject the null hypothesis or fail to reject the null hypothesis. You should remember though, hypothesis testing uses data from a sample to make an inference about a population. When conducting a hypothesis test we do not know the population parameters. In most cases, we don't know if our inference is correct or incorrect.
When we reject the null hypothesis there are two possibilities. There could really be a difference in the population, in which case we made a correct decision. Or, it is possible that there is not a difference in the population (i.e., \(H_0\) is true) but our sample was different from the hypothesized value due to random sampling variation. In that case we made an error. This is known as a Type I error.
When we fail to reject the null hypothesis there are also two possibilities. If the null hypothesis is really true, and there is not a difference in the population, then we made the correct decision. If there is a difference in the population, and we failed to reject it, then we made a Type II error.
Rejecting \(H_0\) when \(H_0\) is really true, denoted by \(\alpha\) ("alpha") and commonly set at .05
\(\alpha=P(Type\;I\;error)\)
Failing to reject \(H_0\) when \(H_0\) is really false, denoted by \(\beta\) ("beta")
\(\beta=P(Type\;II\;error)\)
Decision | Reality | |
---|---|---|
\(H_0\) is true | \(H_0\) is false | |
Reject \(H_0\), (conclude \(H_a\)) | Type I error | Correct decision |
Fail to reject \(H_0\) | Correct decision | Type II error |
Example: Trial Section
A man goes to trial where he is being tried for the murder of his wife.
We can put it in a hypothesis testing framework. The hypotheses being tested are:
- \(H_0\) : Not Guilty
- \(H_a\) : Guilty
Type I error is committed if we reject \(H_0\) when it is true. In other words, did not kill his wife but was found guilty and is punished for a crime he did not really commit.
Type II error is committed if we fail to reject \(H_0\) when it is false. In other words, if the man did kill his wife but was found not guilty and was not punished.
Example: Culinary Arts Study Section
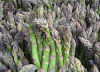
A group of culinary arts students is comparing two methods for preparing asparagus: traditional steaming and a new frying method. They want to know if patrons of their school restaurant prefer their new frying method over the traditional steaming method. A sample of patrons are given asparagus prepared using each method and asked to select their preference. A statistical analysis is performed to determine if more than 50% of participants prefer the new frying method:
- \(H_{0}: p = .50\)
- \(H_{a}: p>.50\)
Type I error occurs if they reject the null hypothesis and conclude that their new frying method is preferred when in reality is it not. This may occur if, by random sampling error, they happen to get a sample that prefers the new frying method more than the overall population does. If this does occur, the consequence is that the students will have an incorrect belief that their new method of frying asparagus is superior to the traditional method of steaming.
Type II error occurs if they fail to reject the null hypothesis and conclude that their new method is not superior when in reality it is. If this does occur, the consequence is that the students will have an incorrect belief that their new method is not superior to the traditional method when in reality it is.
9.2 Outcomes and the Type I and Type II Errors
When you perform a hypothesis test, there are four possible outcomes depending on the actual truth (or falseness) of the null hypothesis H 0 and the decision to reject or not. The outcomes are summarized in the following table:
ACTION | IS ACTUALLY | ... |
---|---|---|
True | False | |
Correct Outcome | Type II error | |
Type I Error | Correct Outcome |
The four possible outcomes in the table are:
- The decision is not to reject H 0 when H 0 is true (correct decision).
- The decision is to reject H 0 when H 0 is true (incorrect decision known as a Type I error ).
- The decision is not to reject H 0 when, in fact, H 0 is false (incorrect decision known as a Type II error ).
- The decision is to reject H 0 when H 0 is false ( correct decision whose probability is called the Power of the Test ).
Each of the errors occurs with a particular probability. The Greek letters α and β represent the probabilities.
α = probability of a Type I error = P (Type I error) = probability of rejecting the null hypothesis when the null hypothesis is true.
β = probability of a Type II error = P (Type II error) = probability of not rejecting the null hypothesis when the null hypothesis is false.
α and β should be as small as possible because they are probabilities of errors. They are rarely zero.
The Power of the Test is 1 – β . Ideally, we want a high power that is as close to one as possible. Increasing the sample size can increase the Power of the Test.
The following are examples of Type I and Type II errors.
Example 9.5
Suppose the null hypothesis, H 0 , is: Navah's rock climbing equipment is safe.
Type I error : Navah thinks that her rock climbing equipment may not be safe when, in fact, it really is safe. Type II error : Navah thinks that her rock climbing equipment may be safe when, in fact, it is not safe.
α = probability that Navah thinks her rock climbing equipment may not be safe when, in fact, it really is safe. β = probability that Navah thinks her rock climbing equipment may be safe when, in fact, it is not safe.
Notice that, in this case, the error with the greater consequence is the Type II error. (If Navah thinks her rock climbing equipment is safe, she will go ahead and use it.)
Suppose the null hypothesis, H 0 , is: the blood cultures contain no traces of pathogen X . State the Type I and Type II errors.
Example 9.6
Suppose the null hypothesis, H 0 , is: The victim of an automobile accident is alive when they arrive at the emergency room of a hospital.
Type I error : The emergency crew thinks that the victim is dead when, in fact, the victim is alive. Type II error : The emergency crew does not know if the victim is alive when, in fact, the victim is dead.
α = probability that the emergency crew thinks the victim is dead when, in fact, the victim is really alive = P (Type I error). β = probability that the emergency crew does not know if the victim is alive when, in fact, the victim is dead = P (Type II error).
The error with the greater consequence is the Type I error. (If the emergency crew thinks the victim is dead, they will not treat them.)
Suppose the null hypothesis, H 0 , is: a patient is not sick. Which type of error has the greater consequence, Type I or Type II?
Example 9.7
A company called Genetic Labs claims to be able to increase the likelihood that a pregnancy will result in a male being born. Statisticians want to test the claim. Suppose that the null hypothesis, H 0 , is: Genetic Labs has no effect on sex outcome.
Type I error : This results when a true null hypothesis is rejected. In the context of this scenario, we would state that we believe that Genetic Labs influences the sex outcome, when in fact it has no effect. The probability of this error occurring is denoted by the Greek letter alpha, α .
Type II error : This results when we fail to reject a false null hypothesis. In context, we would state that Genetic Labs does not influence the sex outcome of a pregnancy when, in fact, it does. The probability of this error occurring is denoted by the Greek letter beta, β .
The error of greater consequence would be the Type I error since people would use the Genetic Labs product in hopes of increasing the chances of having a male.
“Red tide” is a bloom of poison-producing algae–a few different species of a class of plankton called dinoflagellates. When the weather and water conditions cause these blooms, shellfish such as clams living in the area develop dangerous levels of a paralysis-inducing toxin. In Massachusetts, the Division of Marine Fisheries (DMF) monitors levels of the toxin in shellfish by regular sampling of shellfish along the coastline. If the mean level of toxin in clams exceeds 800 μg (micrograms) of toxin per kg of clam meat in any area, clam harvesting is banned there until the bloom is over and levels of toxin in clams subside. Describe both a Type I and a Type II error in this context, and state which error has the greater consequence.
Example 9.8
A certain experimental drug claims a cure rate of at least 75% for males with prostate cancer. Describe both the Type I and Type II errors in context. Which error is the more serious?
Type I : A cancer patient believes the cure rate for the drug is less than 75% when it actually is at least 75%.
Type II : A cancer patient believes the experimental drug has at least a 75% cure rate when it has a cure rate that is less than 75%.
In this scenario, the Type II error contains the more severe consequence. If a patient believes the drug works at least 75% of the time, this most likely will influence the patient’s (and doctor’s) choice about whether to use the drug as a treatment option.
Determine both Type I and Type II errors for the following scenario:
Assume a null hypothesis, H 0 , that states the percentage of adults with jobs is at least 88%.
Identify the Type I and Type II errors from these four statements.
- Not to reject the null hypothesis that the percentage of adults who have jobs is at least 88% when that percentage is actually less than 88%
- Not to reject the null hypothesis that the percentage of adults who have jobs is at least 88% when the percentage is actually at least 88%.
- Reject the null hypothesis that the percentage of adults who have jobs is at least 88% when the percentage is actually at least 88%.
- Reject the null hypothesis that the percentage of adults who have jobs is at least 88% when that percentage is actually less than 88%.
This book may not be used in the training of large language models or otherwise be ingested into large language models or generative AI offerings without OpenStax's permission.
Want to cite, share, or modify this book? This book uses the Creative Commons Attribution License and you must attribute OpenStax.
Access for free at https://openstax.org/books/introductory-statistics-2e/pages/1-introduction
- Authors: Barbara Illowsky, Susan Dean
- Publisher/website: OpenStax
- Book title: Introductory Statistics 2e
- Publication date: Dec 13, 2023
- Location: Houston, Texas
- Book URL: https://openstax.org/books/introductory-statistics-2e/pages/1-introduction
- Section URL: https://openstax.org/books/introductory-statistics-2e/pages/9-2-outcomes-and-the-type-i-and-type-ii-errors
© Dec 6, 2023 OpenStax. Textbook content produced by OpenStax is licensed under a Creative Commons Attribution License . The OpenStax name, OpenStax logo, OpenStax book covers, OpenStax CNX name, and OpenStax CNX logo are not subject to the Creative Commons license and may not be reproduced without the prior and express written consent of Rice University.
5. Differences between means: type I and type II errors and power
Large sample standard error of difference between means.
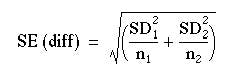
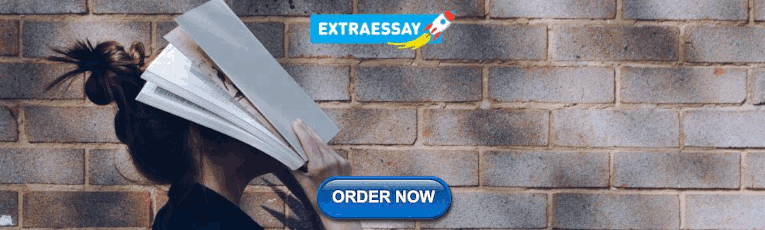
Hypothesis Testing
The process of hypothesis testing can seem to be quite varied with a multitude of test statistics. But the general process is the same. Hypothesis testing involves the statement of a null hypothesis and the selection of a level of significance . The null hypothesis is either true or false and represents the default claim for a treatment or procedure. For example, when examining the effectiveness of a drug, the null hypothesis would be that the drug has no effect on a disease.
After formulating the null hypothesis and choosing a level of significance, we acquire data through observation. Statistical calculations tell us whether or not we should reject the null hypothesis.
In an ideal world, we would always reject the null hypothesis when it is false, and we would not reject the null hypothesis when it is indeed true. But there are two other scenarios that are possible, each of which will result in an error.
Type I Error
The first kind of error that is possible involves the rejection of a null hypothesis that is actually true. This kind of error is called a type I error and is sometimes called an error of the first kind.
Type I errors are equivalent to false positives. Let’s go back to the example of a drug being used to treat a disease. If we reject the null hypothesis in this situation, then our claim is that the drug does, in fact, have some effect on a disease. But if the null hypothesis is true, then, in reality, the drug does not combat the disease at all. The drug is falsely claimed to have a positive effect on a disease.
Type I errors can be controlled. The value of alpha, which is related to the level of significance that we selected has a direct bearing on type I errors. Alpha is the maximum probability that we have a type I error. For a 95% confidence level, the value of alpha is 0.05. This means that there is a 5% probability that we will reject a true null hypothesis. In the long run, one out of every twenty hypothesis tests that we perform at this level will result in a type I error.
Type II Error
The other kind of error that is possible occurs when we do not reject a null hypothesis that is false. This sort of error is called a type II error and is also referred to as an error of the second kind.
Type II errors are equivalent to false negatives. If we think back again to the scenario in which we are testing a drug, what would a type II error look like? A type II error would occur if we accepted that the drug had no effect on a disease, but in reality, it did.
The probability of a type II error is given by the Greek letter beta. This number is related to the power or sensitivity of the hypothesis test, denoted by 1 – beta.
How to Avoid Errors
Type I and type II errors are part of the process of hypothesis testing. Although the errors cannot be completely eliminated, we can minimize one type of error.
Typically when we try to decrease the probability one type of error, the probability for the other type increases. We could decrease the value of alpha from 0.05 to 0.01, corresponding to a 99% level of confidence . However, if everything else remains the same, then the probability of a type II error will nearly always increase.
Many times the real world application of our hypothesis test will determine if we are more accepting of type I or type II errors. This will then be used when we design our statistical experiment.
- Null Hypothesis Examples
- Type I and Type II Errors in Statistics
- What Level of Alpha Determines Statistical Significance?
- Hypothesis Test Example
- What Is the Difference Between Alpha and P-Values?
- How to Conduct a Hypothesis Test
- What Is a P-Value?
- Scientific Method Vocabulary Terms
- An Example of a Hypothesis Test
- Hypothesis Test for the Difference of Two Population Proportions
- How to Find Critical Values with a Chi-Square Table
- What 'Fail to Reject' Means in a Hypothesis Test
- Null Hypothesis and Alternative Hypothesis
- The Runs Test for Random Sequences
- How to Do Hypothesis Tests With the Z.TEST Function in Excel
- Chi-Square Goodness of Fit Test
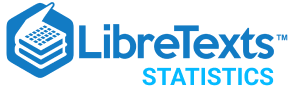
- school Campus Bookshelves
- menu_book Bookshelves
- perm_media Learning Objects
- login Login
- how_to_reg Request Instructor Account
- hub Instructor Commons
Margin Size
- Download Page (PDF)
- Download Full Book (PDF)
- Periodic Table
- Physics Constants
- Scientific Calculator
- Reference & Cite
- Tools expand_more
- Readability
selected template will load here
This action is not available.
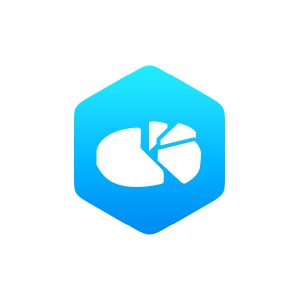
9.2: Outcomes, Type I and Type II Errors
- Last updated
- Save as PDF
- Page ID 16267
\( \newcommand{\vecs}[1]{\overset { \scriptstyle \rightharpoonup} {\mathbf{#1}} } \)
\( \newcommand{\vecd}[1]{\overset{-\!-\!\rightharpoonup}{\vphantom{a}\smash {#1}}} \)
\( \newcommand{\id}{\mathrm{id}}\) \( \newcommand{\Span}{\mathrm{span}}\)
( \newcommand{\kernel}{\mathrm{null}\,}\) \( \newcommand{\range}{\mathrm{range}\,}\)
\( \newcommand{\RealPart}{\mathrm{Re}}\) \( \newcommand{\ImaginaryPart}{\mathrm{Im}}\)
\( \newcommand{\Argument}{\mathrm{Arg}}\) \( \newcommand{\norm}[1]{\| #1 \|}\)
\( \newcommand{\inner}[2]{\langle #1, #2 \rangle}\)
\( \newcommand{\Span}{\mathrm{span}}\)
\( \newcommand{\id}{\mathrm{id}}\)
\( \newcommand{\kernel}{\mathrm{null}\,}\)
\( \newcommand{\range}{\mathrm{range}\,}\)
\( \newcommand{\RealPart}{\mathrm{Re}}\)
\( \newcommand{\ImaginaryPart}{\mathrm{Im}}\)
\( \newcommand{\Argument}{\mathrm{Arg}}\)
\( \newcommand{\norm}[1]{\| #1 \|}\)
\( \newcommand{\Span}{\mathrm{span}}\) \( \newcommand{\AA}{\unicode[.8,0]{x212B}}\)
\( \newcommand{\vectorA}[1]{\vec{#1}} % arrow\)
\( \newcommand{\vectorAt}[1]{\vec{\text{#1}}} % arrow\)
\( \newcommand{\vectorB}[1]{\overset { \scriptstyle \rightharpoonup} {\mathbf{#1}} } \)
\( \newcommand{\vectorC}[1]{\textbf{#1}} \)
\( \newcommand{\vectorD}[1]{\overrightarrow{#1}} \)
\( \newcommand{\vectorDt}[1]{\overrightarrow{\text{#1}}} \)
\( \newcommand{\vectE}[1]{\overset{-\!-\!\rightharpoonup}{\vphantom{a}\smash{\mathbf {#1}}}} \)
When you perform a hypothesis test, there are four possible outcomes depending on the actual truth (or falseness) of the null hypothesis H 0 and the decision to reject or not.
The outcomes are summarized in the following table:
is actually | ||
---|---|---|
True | False | |
Do not reject | Correct Outcome | Type II Error ( |
Reject | Type I Error ( | Correct Outcome |
The four possible outcomes in the table are:
- The decision is not to reject H 0 when H 0 is true (correct decision) .
- The decision is to reject H 0 when H 0 is true (incorrect decision known as a Type I error ).
- The decision is not to reject H 0 when H 0 is false (incorrect decision known as a Type II error ).
- The decision is to reject H 0 when H 0 is false ( correct decision whose probability is called the Power of the Test ).
Each of the errors occurs with a particular probability. The Greek letters α and β represent the probabilities.
α = probability of a Type I error = P (Type I error) = probability of rejecting the null hypothesis when the null hypothesis is true.
β = probability of a Type II error = P (Type II error) = probability of not rejecting the null hypothesis when the null hypothesis is false.
α and β should be as small as possible because they are probabilities of errors. They are rarely zero.
The Power of the Test is 1 – β . Since β is probability of making type II error, we want this probability to be small. In other words, we want the value 1 – β to be as closed to one as possible. Increasing the sample size can increase the Power of the Test.
Suppose the null hypothesis, H 0 , is: Frank’s rock climbing equipment is safe.
- Ty pe I error: Frank thinks that his rock climbing equipment may not be safe when, in fact, it really is safe.
- Type II error: Frank thinks that his rock climbing equipment may be safe when, in fact, it is not safe.
α = Probability that Frank thinks his rock climbing equipment may not be safe when it really is safe. β = Probability that Frank thinks his rock climbing equipment may be safe when it is not safe.
True (The equipment is safe) | False (The equipment is not safe.) | |
Not reject H | Correct decision | Type II Error |
Reject H | Type I Error | Correct decision |
Notice that, in this case, the error with the greater consequence is the Type II error. (If Frank thinks his rock climbing equipment is safe, he will go ahead and use it.)
Suppose the null hypothesis, H 0 , is: the blood cultures contain no traces of pathogen X . State the Type I and Type II errors.
[practice-area rows=”2″][/practice-area] [reveal-answer q=”313018″]Solution[/reveal-answer] [hidden-answer a=”313018″]
Type I error : The researcher thinks the blood cultures do contain traces of pathogen X, when in fact, they do not. Type II error : The researcher thinks the blood cultures do not contain traces of pathogen X, when in fact, they do.
True (The blood cultures contain no traces of pathogen X. ) | False (The blood cultures contain traces of pathogen X.) | |
Not reject | Correct decision | Type II Error |
Reject | Type I Error | Correct decision |
[/hidden-answer]
Suppose the null hypothesis, H 0 : The victim of an automobile accident is alive when he arrives at the emergency room of a hospital. State the 4 possible outcomes of performing a hypothesis test.
α = probability that the emergency crew thinks the victim is dead when, in fact, he is really alive = P (Type I error). β = probability that the emergency crew does not know if the victim is alive when, in fact, the victim is dead = P (Type II error).
True (The victim is alive.) | False (The victim is dead.) | |
Not reject | Correct decision | Type II Error |
Reject | Type I Error | Correct decision |
The error with the greater consequence is the Type I error. (If the emergency crew thinks the victim is dead, they will not treat him.)
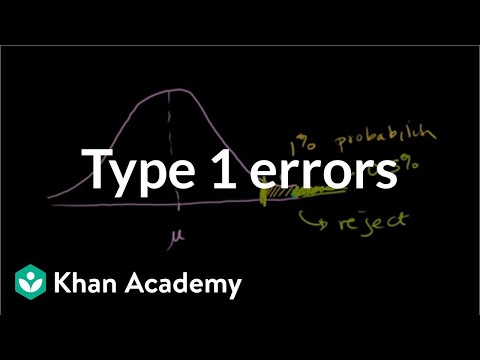
A YouTube element has been excluded from this version of the text. You can view it online here: http://pb.libretexts.org/esm/?p=120
Suppose the null hypothesis, H 0 , is: A patient is not sick. Which type of error has the greater consequence, Type I or Type II?
[practice-area rows=”1=2″][/practice-area] [reveal-answer q=”3933″]Solution[/reveal-answer] [hidden-answer a=”3933″]
Type I Error: The patient will not be thought well when, in fact, he is not sick.
Type II Error: The patient will be thought well when, in fact, he is sick.
True (The patient is not sick.) | False (The patient is sick.) | |
Not reject H | Correct decision | Type II Error |
Reject H | Type I Error | Correct decision |
The error with the greater consequence is the Type II error: the patient will be thought well when, in fact, he is sick. He will not be able to get treatment.[/hidden-answer]
Boy Genetic Labs claim to be able to increase the likelihood that a pregnancy will result in a boy being born. Statisticians want to test the claim. Suppose that the null hypothesis, H 0 , is: Boy Genetic Labs has no effect on gender outcome. Which type of error has the greater consequence, Type I or Type II?
H 0 : Boy Genetic Labs has no effect on gender outcome.
H a : Boy Genetic Labs has effect on gender outcome.
True (No Effect) | False (Effect) | |
Not reject | Correct decision | Type II Error |
reject | Type I Error | Correct decision |
- Type I error: This results when a true null hypothesis is rejected. In the context of this scenario, we would state that we believe that Boy Genetic Labs influences the gender outcome, when in fact it has no effect. The probability of this error occurring is denoted by the Greek letter alpha, α .
- Type II error: This results when we fail to reject a false null hypothesis. In context, we would state that Boy Genetic Labs does not influence the gender outcome of a pregnancy when, in fact, it does. The probability of this error occurring is denoted by the Greek letter beta, β .
The error of greater consequence would be the Type I error since couples would use the Boy Genetic Labs product in hopes of increasing the chances of having a boy.
“Red tide” is a bloom of poison-producing algae–a few different species of a class of plankton called dinoflagellates. When the weather and water conditions cause these blooms, shellfish such as clams living in the area develop dangerous levels of a paralysis-inducing toxin. In Massachusetts, the Division of Marine Fisheries (DMF) monitors levels of the toxin in shellfish by regular sampling of shellfish along the coastline. If the mean level of toxin in clams exceeds 800 μg (micrograms) of toxin per kg of clam meat in any area, clam harvesting is banned there until the bloom is over and levels of toxin in clams subside. Describe both a Type I and a Type II error in this context, and state which error has the greater consequence.
[reveal-answer q=”432609″]Solution[/reveal-answer] [hidden-answer a=”432609″]
In this scenario, an appropriate null hypothesis would be H 0 : the mean level of toxins is at most 800 μg. ( H 0 : μ 0 ≤ 800 μg ) H a : the mean level of toxins exceeds 800 μg. (H a : μ 0 > 800 μg )
Type I error: The DMF believes that toxin levels are still too high when, in fact, toxin levels are at most 800 μg. The DMF continues the harvesting ban. Type II error: The DMF believes that toxin levels are within acceptable levels (are at least 800 μg) when, in fact, toxin levels are still too high (more than 800 μg). The DMF lifts the harvesting ban.
True | False | |
Not reject H | Correct decision | Type II Error |
Reject H | Type I Error | Correct decision |
This error could be the most serious. If the ban is lifted and clams are still toxic, consumers could possibly eat tainted food. In summary, the more dangerous error would be to commit a Type II error, because this error involves the availability of tainted clams for consumption.[/hidden-answer]
A certain experimental drug claims a cure rate of higher than 75% for males with prostate cancer.
Describe both the Type I and Type II errors in context. Which error is the more serious?
H 0 : The cure rate is less than 75%.
H a : The cure rate is higher than 75%.
True (The cure rate is less than 75%.) | False (The cure rate is higher than 75%.) | |
Not reject H | Correct | Type II Error |
Reject H | Type I Error | Correct |
- Type I: A cancer patient believes the cure rate for the drug is more than 75% when the cure rate actually is less than 75%.
- Type II: A cancer patient believes the the cure rate is less than 75% cure rate when the cure rate is actually higher than 75%.
In this scenario, the Type II error contains the more severe consequence. If a patient believes the drug works at least 75% of the time, this most likely will influence the patient’s (and doctor’s) choice about whether to use the drug as a treatment option.
Determine both Type I and Type II errors for the following scenario:
Assume a null hypothesis, H 0 , that states the percentage of adults with jobs is at least 88%.
Identify the Type I and Type II errors from these four statements.
a)Not to reject the null hypothesis that the percentage of adults who have jobs is at least 88% when that percentage is actually less than 88%
b)Not to reject the null hypothesis that the percentage of adults who have jobs is at least 88% when the percentage is actually at least 88%.
c)Reject the null hypothesis that the percentage of adults who have jobs is at least 88% when the percentage is actually at least 88%.
d)Reject the null hypothesis that the percentage of adults who have jobs is at least 88% when that percentage is actually less than 88%.
[reveal-answer q=”76062″]Type I error:[/reveal-answer] [hidden-answer a=”76062″]c [/hidden-answer] [reveal-answer q=”975662″]Type II error:[/reveal-answer] [hidden-answer a=”975662″]b[/hidden-answer]
If H 0 : The percentage of adults with jobs is at least 88%, then H a : The percentage of adults with jobs is less than 88%.
[reveal-answer q=”864484″]Type I error: [/reveal-answer] [hidden-answer a=”864484″]c [/hidden-answer] [reveal-answer q=”126260″]Type II error:[/reveal-answer] [hidden-answer a=”126260″]b[/hidden-answer]
Concept Review
In every hypothesis test, the outcomes are dependent on a correct interpretation of the data. Incorrect calculations or misunderstood summary statistics can yield errors that affect the results. A Type I error occurs when a true null hypothesis is rejected. A Type II error occurs when a false null hypothesis is not rejected.
The probabilities of these errors are denoted by the Greek letters α and β , for a Type I and a Type II error respectively. The power of the test, 1 – β , quantifies the likelihood that a test will yield the correct result of a true alternative hypothesis being accepted. A high power is desirable.
Formula Review
- OpenStax, Statistics, Outcomes and the Type I and Type II Errors. Provided by : OpenStax. Located at : http://cnx.org/contents/[email protected] . License : CC BY: Attribution
- Introductory Statistics . Authored by : Barbara Illowski, Susan Dean. Provided by : Open Stax. Located at : http://cnx.org/contents/[email protected] . License : CC BY: Attribution . License Terms : Download for free at http://cnx.org/contents/[email protected]
- Type 1 errors | Inferential statistics | Probability and Statistics | Khan Academy. Authored by : Khan Academy. Located at : https://youtu.be/EowIec7Y8HM . License : All Rights Reserved . License Terms : Standard YouTube License

An official website of the United States government
The .gov means it’s official. Federal government websites often end in .gov or .mil. Before sharing sensitive information, make sure you’re on a federal government site.
The site is secure. The https:// ensures that you are connecting to the official website and that any information you provide is encrypted and transmitted securely.
- Publications
- Account settings
Preview improvements coming to the PMC website in October 2024. Learn More or Try it out now .
- Advanced Search
- Journal List
- Ind Psychiatry J
- v.18(2); Jul-Dec 2009
Hypothesis testing, type I and type II errors
Amitav banerjee.
Department of Community Medicine, D. Y. Patil Medical College, Pune, India
U. B. Chitnis
S. l. jadhav, j. s. bhawalkar, s. chaudhury.
1 Department of Psychiatry, RINPAS, Kanke, Ranchi, India
Hypothesis testing is an important activity of empirical research and evidence-based medicine. A well worked up hypothesis is half the answer to the research question. For this, both knowledge of the subject derived from extensive review of the literature and working knowledge of basic statistical concepts are desirable. The present paper discusses the methods of working up a good hypothesis and statistical concepts of hypothesis testing.
Karl Popper is probably the most influential philosopher of science in the 20 th century (Wulff et al ., 1986). Many scientists, even those who do not usually read books on philosophy, are acquainted with the basic principles of his views on science. The popularity of Popper’s philosophy is due partly to the fact that it has been well explained in simple terms by, among others, the Nobel Prize winner Peter Medawar (Medawar, 1969). Popper makes the very important point that empirical scientists (those who stress on observations only as the starting point of research) put the cart in front of the horse when they claim that science proceeds from observation to theory, since there is no such thing as a pure observation which does not depend on theory. Popper states, “… the belief that we can start with pure observation alone, without anything in the nature of a theory, is absurd: As may be illustrated by the story of the man who dedicated his life to natural science, wrote down everything he could observe, and bequeathed his ‘priceless’ collection of observations to the Royal Society to be used as inductive (empirical) evidence.
STARTING POINT OF RESEARCH: HYPOTHESIS OR OBSERVATION?
The first step in the scientific process is not observation but the generation of a hypothesis which may then be tested critically by observations and experiments. Popper also makes the important claim that the goal of the scientist’s efforts is not the verification but the falsification of the initial hypothesis. It is logically impossible to verify the truth of a general law by repeated observations, but, at least in principle, it is possible to falsify such a law by a single observation. Repeated observations of white swans did not prove that all swans are white, but the observation of a single black swan sufficed to falsify that general statement (Popper, 1976).
CHARACTERISTICS OF A GOOD HYPOTHESIS
A good hypothesis must be based on a good research question. It should be simple, specific and stated in advance (Hulley et al ., 2001).
Hypothesis should be simple
A simple hypothesis contains one predictor and one outcome variable, e.g. positive family history of schizophrenia increases the risk of developing the condition in first-degree relatives. Here the single predictor variable is positive family history of schizophrenia and the outcome variable is schizophrenia. A complex hypothesis contains more than one predictor variable or more than one outcome variable, e.g., a positive family history and stressful life events are associated with an increased incidence of Alzheimer’s disease. Here there are 2 predictor variables, i.e., positive family history and stressful life events, while one outcome variable, i.e., Alzheimer’s disease. Complex hypothesis like this cannot be easily tested with a single statistical test and should always be separated into 2 or more simple hypotheses.
Hypothesis should be specific
A specific hypothesis leaves no ambiguity about the subjects and variables, or about how the test of statistical significance will be applied. It uses concise operational definitions that summarize the nature and source of the subjects and the approach to measuring variables (History of medication with tranquilizers, as measured by review of medical store records and physicians’ prescriptions in the past year, is more common in patients who attempted suicides than in controls hospitalized for other conditions). This is a long-winded sentence, but it explicitly states the nature of predictor and outcome variables, how they will be measured and the research hypothesis. Often these details may be included in the study proposal and may not be stated in the research hypothesis. However, they should be clear in the mind of the investigator while conceptualizing the study.
Hypothesis should be stated in advance
The hypothesis must be stated in writing during the proposal state. This will help to keep the research effort focused on the primary objective and create a stronger basis for interpreting the study’s results as compared to a hypothesis that emerges as a result of inspecting the data. The habit of post hoc hypothesis testing (common among researchers) is nothing but using third-degree methods on the data (data dredging), to yield at least something significant. This leads to overrating the occasional chance associations in the study.
TYPES OF HYPOTHESES
For the purpose of testing statistical significance, hypotheses are classified by the way they describe the expected difference between the study groups.
Null and alternative hypotheses
The null hypothesis states that there is no association between the predictor and outcome variables in the population (There is no difference between tranquilizer habits of patients with attempted suicides and those of age- and sex- matched “control” patients hospitalized for other diagnoses). The null hypothesis is the formal basis for testing statistical significance. By starting with the proposition that there is no association, statistical tests can estimate the probability that an observed association could be due to chance.
The proposition that there is an association — that patients with attempted suicides will report different tranquilizer habits from those of the controls — is called the alternative hypothesis. The alternative hypothesis cannot be tested directly; it is accepted by exclusion if the test of statistical significance rejects the null hypothesis.
One- and two-tailed alternative hypotheses
A one-tailed (or one-sided) hypothesis specifies the direction of the association between the predictor and outcome variables. The prediction that patients of attempted suicides will have a higher rate of use of tranquilizers than control patients is a one-tailed hypothesis. A two-tailed hypothesis states only that an association exists; it does not specify the direction. The prediction that patients with attempted suicides will have a different rate of tranquilizer use — either higher or lower than control patients — is a two-tailed hypothesis. (The word tails refers to the tail ends of the statistical distribution such as the familiar bell-shaped normal curve that is used to test a hypothesis. One tail represents a positive effect or association; the other, a negative effect.) A one-tailed hypothesis has the statistical advantage of permitting a smaller sample size as compared to that permissible by a two-tailed hypothesis. Unfortunately, one-tailed hypotheses are not always appropriate; in fact, some investigators believe that they should never be used. However, they are appropriate when only one direction for the association is important or biologically meaningful. An example is the one-sided hypothesis that a drug has a greater frequency of side effects than a placebo; the possibility that the drug has fewer side effects than the placebo is not worth testing. Whatever strategy is used, it should be stated in advance; otherwise, it would lack statistical rigor. Data dredging after it has been collected and post hoc deciding to change over to one-tailed hypothesis testing to reduce the sample size and P value are indicative of lack of scientific integrity.
STATISTICAL PRINCIPLES OF HYPOTHESIS TESTING
A hypothesis (for example, Tamiflu [oseltamivir], drug of choice in H1N1 influenza, is associated with an increased incidence of acute psychotic manifestations) is either true or false in the real world. Because the investigator cannot study all people who are at risk, he must test the hypothesis in a sample of that target population. No matter how many data a researcher collects, he can never absolutely prove (or disprove) his hypothesis. There will always be a need to draw inferences about phenomena in the population from events observed in the sample (Hulley et al ., 2001). In some ways, the investigator’s problem is similar to that faced by a judge judging a defendant [ Table 1 ]. The absolute truth whether the defendant committed the crime cannot be determined. Instead, the judge begins by presuming innocence — the defendant did not commit the crime. The judge must decide whether there is sufficient evidence to reject the presumed innocence of the defendant; the standard is known as beyond a reasonable doubt. A judge can err, however, by convicting a defendant who is innocent, or by failing to convict one who is actually guilty. In similar fashion, the investigator starts by presuming the null hypothesis, or no association between the predictor and outcome variables in the population. Based on the data collected in his sample, the investigator uses statistical tests to determine whether there is sufficient evidence to reject the null hypothesis in favor of the alternative hypothesis that there is an association in the population. The standard for these tests is shown as the level of statistical significance.
The analogy between judge’s decisions and statistical tests
Judge’s decision | Statistical test |
---|---|
Innocence: The defendant did not commit crime | Null hypothesis: No association between Tamiflu and psychotic manifestations |
Guilt: The defendant did commit the crime | Alternative hypothesis: There is association between Tamiflu and psychosis |
Standard for rejecting innocence: Beyond a reasonable doubt | Standard for rejecting null hypothesis: Level of statistical significance (à) |
Correct judgment: Convict a criminal | Correct inference: Conclude that there is an association when one does exist in the population |
Correct judgment: Acquit an innocent person | Correct inference: Conclude that there is no association between Tamiflu and psychosis when one does not exist |
Incorrect judgment: Convict an innocent person. | Incorrect inference (Type I error): Conclude that there is an association when there actually is none |
Incorrect judgment: Acquit a criminal | Incorrect inference (Type II error): Conclude that there is no association when there actually is one |
TYPE I (ALSO KNOWN AS ‘α’) AND TYPE II (ALSO KNOWN AS ‘β’)ERRORS
Just like a judge’s conclusion, an investigator’s conclusion may be wrong. Sometimes, by chance alone, a sample is not representative of the population. Thus the results in the sample do not reflect reality in the population, and the random error leads to an erroneous inference. A type I error (false-positive) occurs if an investigator rejects a null hypothesis that is actually true in the population; a type II error (false-negative) occurs if the investigator fails to reject a null hypothesis that is actually false in the population. Although type I and type II errors can never be avoided entirely, the investigator can reduce their likelihood by increasing the sample size (the larger the sample, the lesser is the likelihood that it will differ substantially from the population).
False-positive and false-negative results can also occur because of bias (observer, instrument, recall, etc.). (Errors due to bias, however, are not referred to as type I and type II errors.) Such errors are troublesome, since they may be difficult to detect and cannot usually be quantified.
EFFECT SIZE
The likelihood that a study will be able to detect an association between a predictor variable and an outcome variable depends, of course, on the actual magnitude of that association in the target population. If it is large (such as 90% increase in the incidence of psychosis in people who are on Tamiflu), it will be easy to detect in the sample. Conversely, if the size of the association is small (such as 2% increase in psychosis), it will be difficult to detect in the sample. Unfortunately, the investigator often does not know the actual magnitude of the association — one of the purposes of the study is to estimate it. Instead, the investigator must choose the size of the association that he would like to be able to detect in the sample. This quantity is known as the effect size. Selecting an appropriate effect size is the most difficult aspect of sample size planning. Sometimes, the investigator can use data from other studies or pilot tests to make an informed guess about a reasonable effect size. When there are no data with which to estimate it, he can choose the smallest effect size that would be clinically meaningful, for example, a 10% increase in the incidence of psychosis. Of course, from the public health point of view, even a 1% increase in psychosis incidence would be important. Thus the choice of the effect size is always somewhat arbitrary, and considerations of feasibility are often paramount. When the number of available subjects is limited, the investigator may have to work backward to determine whether the effect size that his study will be able to detect with that number of subjects is reasonable.
α,β,AND POWER
After a study is completed, the investigator uses statistical tests to try to reject the null hypothesis in favor of its alternative (much in the same way that a prosecuting attorney tries to convince a judge to reject innocence in favor of guilt). Depending on whether the null hypothesis is true or false in the target population, and assuming that the study is free of bias, 4 situations are possible, as shown in Table 2 below. In 2 of these, the findings in the sample and reality in the population are concordant, and the investigator’s inference will be correct. In the other 2 situations, either a type I (α) or a type II (β) error has been made, and the inference will be incorrect.
Truth in the population versus the results in the study sample: The four possibilities
Truth in the population | Association + nt | No association |
---|---|---|
Reject null hypothesis | Correct | Type I error |
Fail to reject null hypothesis | Type II error | Correct |
The investigator establishes the maximum chance of making type I and type II errors in advance of the study. The probability of committing a type I error (rejecting the null hypothesis when it is actually true) is called α (alpha) the other name for this is the level of statistical significance.
If a study of Tamiflu and psychosis is designed with α = 0.05, for example, then the investigator has set 5% as the maximum chance of incorrectly rejecting the null hypothesis (and erroneously inferring that use of Tamiflu and psychosis incidence are associated in the population). This is the level of reasonable doubt that the investigator is willing to accept when he uses statistical tests to analyze the data after the study is completed.
The probability of making a type II error (failing to reject the null hypothesis when it is actually false) is called β (beta). The quantity (1 - β) is called power, the probability of observing an effect in the sample (if one), of a specified effect size or greater exists in the population.
If β is set at 0.10, then the investigator has decided that he is willing to accept a 10% chance of missing an association of a given effect size between Tamiflu and psychosis. This represents a power of 0.90, i.e., a 90% chance of finding an association of that size. For example, suppose that there really would be a 30% increase in psychosis incidence if the entire population took Tamiflu. Then 90 times out of 100, the investigator would observe an effect of that size or larger in his study. This does not mean, however, that the investigator will be absolutely unable to detect a smaller effect; just that he will have less than 90% likelihood of doing so.
Ideally alpha and beta errors would be set at zero, eliminating the possibility of false-positive and false-negative results. In practice they are made as small as possible. Reducing them, however, usually requires increasing the sample size. Sample size planning aims at choosing a sufficient number of subjects to keep alpha and beta at acceptably low levels without making the study unnecessarily expensive or difficult.
Many studies s et al pha at 0.05 and beta at 0.20 (a power of 0.80). These are somewhat arbitrary values, and others are sometimes used; the conventional range for alpha is between 0.01 and 0.10; and for beta, between 0.05 and 0.20. In general the investigator should choose a low value of alpha when the research question makes it particularly important to avoid a type I (false-positive) error, and he should choose a low value of beta when it is especially important to avoid a type II error.
The null hypothesis acts like a punching bag: It is assumed to be true in order to shadowbox it into false with a statistical test. When the data are analyzed, such tests determine the P value, the probability of obtaining the study results by chance if the null hypothesis is true. The null hypothesis is rejected in favor of the alternative hypothesis if the P value is less than alpha, the predetermined level of statistical significance (Daniel, 2000). “Nonsignificant” results — those with P value greater than alpha — do not imply that there is no association in the population; they only mean that the association observed in the sample is small compared with what could have occurred by chance alone. For example, an investigator might find that men with family history of mental illness were twice as likely to develop schizophrenia as those with no family history, but with a P value of 0.09. This means that even if family history and schizophrenia were not associated in the population, there was a 9% chance of finding such an association due to random error in the sample. If the investigator had set the significance level at 0.05, he would have to conclude that the association in the sample was “not statistically significant.” It might be tempting for the investigator to change his mind about the level of statistical significance ex post facto and report the results “showed statistical significance at P < 10”. A better choice would be to report that the “results, although suggestive of an association, did not achieve statistical significance ( P = .09)”. This solution acknowledges that statistical significance is not an “all or none” situation.
Hypothesis testing is the sheet anchor of empirical research and in the rapidly emerging practice of evidence-based medicine. However, empirical research and, ipso facto, hypothesis testing have their limits. The empirical approach to research cannot eliminate uncertainty completely. At the best, it can quantify uncertainty. This uncertainty can be of 2 types: Type I error (falsely rejecting a null hypothesis) and type II error (falsely accepting a null hypothesis). The acceptable magnitudes of type I and type II errors are set in advance and are important for sample size calculations. Another important point to remember is that we cannot ‘prove’ or ‘disprove’ anything by hypothesis testing and statistical tests. We can only knock down or reject the null hypothesis and by default accept the alternative hypothesis. If we fail to reject the null hypothesis, we accept it by default.
Source of Support: Nil
Conflict of Interest: None declared.
- Daniel W. W. In: Biostatistics. 7th ed. New York: John Wiley and Sons, Inc; 2002. Hypothesis testing; pp. 204–294. [ Google Scholar ]
- Hulley S. B, Cummings S. R, Browner W. S, Grady D, Hearst N, Newman T. B. 2nd ed. Philadelphia: Lippincott Williams and Wilkins; 2001. Getting ready to estimate sample size: Hypothesis and underlying principles In: Designing Clinical Research-An epidemiologic approach; pp. 51–63. [ Google Scholar ]
- Medawar P. B. Philadelphia: American Philosophical Society; 1969. Induction and intuition in scientific thought. [ Google Scholar ]
- Popper K. Unended Quest. An Intellectual Autobiography. Fontana Collins; p. 42. [ Google Scholar ]
- Wulff H. R, Pedersen S. A, Rosenberg R. Oxford: Blackwell Scientific Publicatons; Empirism and Realism: A philosophical problem. In: Philosophy of Medicine. [ Google Scholar ]
- Math Article
- Type I And Type Ii Errors
Type I and Type II Errors
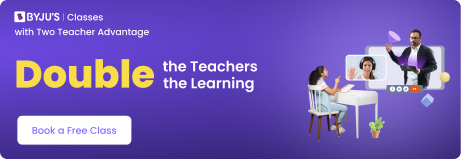
Type I and Type II errors are subjected to the result of the null hypothesis. In case of type I or type-1 error, the null hypothesis is rejected though it is true whereas type II or type-2 error, the null hypothesis is not rejected even when the alternative hypothesis is true. Both the error type-i and type-ii are also known as “ false negative ”. A lot of statistical theory rotates around the reduction of one or both of these errors, still, the total elimination of both is explained as a statistical impossibility.
Type I Error
A type I error appears when the null hypothesis (H 0 ) of an experiment is true, but still, it is rejected. It is stating something which is not present or a false hit. A type I error is often called a false positive (an event that shows that a given condition is present when it is absent). In words of community tales, a person may see the bear when there is none (raising a false alarm) where the null hypothesis (H 0 ) contains the statement: “There is no bear”.
The type I error significance level or rate level is the probability of refusing the null hypothesis given that it is true. It is represented by Greek letter α (alpha) and is also known as alpha level. Usually, the significance level or the probability of type i error is set to 0.05 (5%), assuming that it is satisfactory to have a 5% probability of inaccurately rejecting the null hypothesis.
Type II Error
A type II error appears when the null hypothesis is false but mistakenly fails to be refused. It is losing to state what is present and a miss. A type II error is also known as false negative (where a real hit was rejected by the test and is observed as a miss), in an experiment checking for a condition with a final outcome of true or false.
A type II error is assigned when a true alternative hypothesis is not acknowledged. In other words, an examiner may miss discovering the bear when in fact a bear is present (hence fails in raising the alarm). Again, H0, the null hypothesis, consists of the statement that, “There is no bear”, wherein, if a wolf is indeed present, is a type II error on the part of the investigator. Here, the bear either exists or does not exist within given circumstances, the question arises here is if it is correctly identified or not, either missing detecting it when it is present, or identifying it when it is not present.
The rate level of the type II error is represented by the Greek letter β (beta) and linked to the power of a test (which equals 1−β).
Also, read:
Table of Type I and Type II Error
The relationship between truth or false of the null hypothesis and outcomes or result of the test is given in the tabular form:
is True | is False | |
Correct Decision (True negative) Probability = 1 – α | Type II Error (False negative) Probability = β | |
Type II Error (False Positive) Probability = α | Correct Decision (True Positive) Probability = 1 – β |
Type I and Type II Errors Example
Check out some real-life examples to understand the type-i and type-ii error in the null hypothesis.
Example 1 : Let us consider a null hypothesis – A man is not guilty of a crime.
Then in this case:
Type I error (False Positive) | Type II error (False Negative) |
He is condemned to crime, though he is not guilty or committed the crime. | He is condemned not guilty when the court actually does commit the crime by letting the guilty one go free. |
Example 2: Null hypothesis- A patient’s signs after treatment A, are the same from a placebo.
Type I error (False Positive) | Type II error (False Negative) |
Treatment A is more efficient than the placebo | Treatment A is more powerful than placebo even though it truly is more efficient. |
MATHS Related Links | |
Leave a Comment Cancel reply
Your Mobile number and Email id will not be published. Required fields are marked *
Request OTP on Voice Call
Post My Comment

Register with BYJU'S & Download Free PDFs
Register with byju's & watch live videos.
- School Guide
- Mathematics
- Number System and Arithmetic
- Trigonometry
- Probability
- Mensuration
- Maths Formulas
- Class 8 Maths Notes
- Class 9 Maths Notes
- Class 10 Maths Notes
- Class 11 Maths Notes
- Class 12 Maths Notes
Type I and Type II Errors
- Explain Type assertions in TypeScript
- C# | Implicitly Typed Arrays
- TypeScript Assertions Type
- TypeScript Less Common Primitives Type
- Typescript Generic Type Array
- TypeScript Narrowing typeof type guards
- How to Debug paste Error in R
- How to use Type Guards in TypeScript ?
- C Program to Show Types of Errors
- C++ Program to Show Types of Errors
- TypeScript Interfaces Type
- PHP | Types of Errors
- Errors and Exceptions in Python
- C++ Error - Does not name a type
- What is Type Assertion in TypeScript ?
- Types of Issues and Errors in Programming/Coding
- Type Casting or Type Conversion in Golang
- What is Type Annotations in TypeScript ?
- Type-Safety and Type-Casting in Java Generics
Type I and Type II Errors are central for hypothesis testing in general, which subsequently impacts various aspects of science including but not limited to statistical analysis. False discovery refers to a Type I error where a true Null Hypothesis is incorrectly rejected. On the other end of the spectrum, Type II errors occur when a true null hypothesis fails to get rejected.
In this article, we will discuss Type I and Type II Errors in detail, including examples and differences.
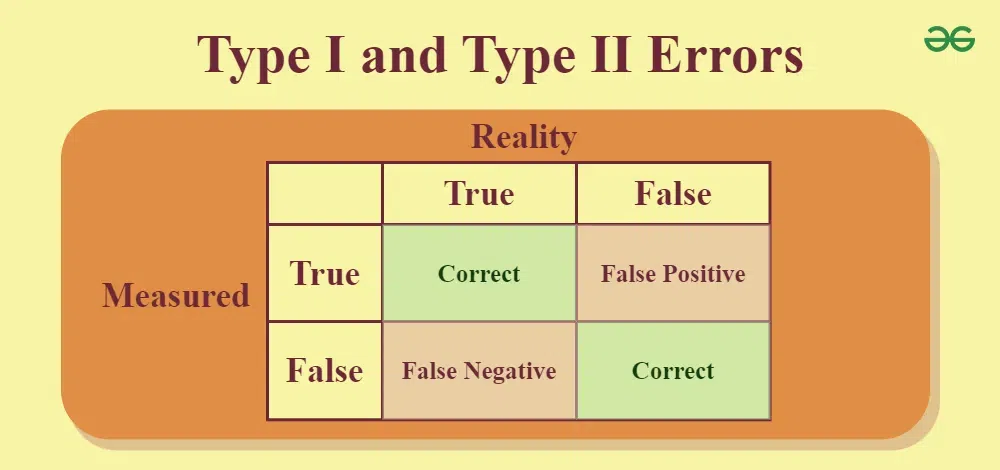
Table of Content
Type I and Type II Error in Statistics
What is error, what is type i error (false positive), what is type ii error (false negative), type i and type ii errors – table, type i and type ii errors examples, examples of type i error, examples of type ii error, factors affecting type i and type ii errors, how to minimize type i and type ii errors, difference between type i and type ii errors.
In statistics , Type I and Type II errors represent two kinds of errors that can occur when making a decision about a hypothesis based on sample data. Understanding these errors is crucial for interpreting the results of hypothesis tests.
In the statistics and hypothesis testing , an error refers to the emergence of discrepancies between the result value based on observation or calculation and the actual value or expected value.
The failures may happen in different factors, such as turbulent sampling, unclear implementation, or faulty assumptions. Errors can be of many types, such as
- Measurement Error
- Calculation Error
- Human Error
- Systematic Error
- Random Error
In hypothesis testing, it is often clear which kind of error is the problem, either a Type I error or a Type II one.
Type I error, also known as a false positive , occurs in statistical hypothesis testing when a null hypothesis that is actually true is rejected. In other words, it’s the error of incorrectly concluding that there is a significant effect or difference when there isn’t one in reality.
In hypothesis testing, there are two competing hypotheses:
- Null Hypothesis (H 0 ): This hypothesis represents a default assumption that there is no effect, no difference, or no relationship in the population being studied.
- Alternative Hypothesis (H 1 ): This hypothesis represents the opposite of the null hypothesis. It suggests that there is a significant effect, difference, or relationship in the population.
A Type I error occurs when the null hypothesis is rejected based on the sample data, even though it is actually true in the population.
Type II error, also known as a false negative , occurs in statistical hypothesis testing when a null hypothesis that is actually false is not rejected. In other words, it’s the error of failing to detect a significant effect or difference when one exists in reality.
A Type II error occurs when the null hypothesis is not rejected based on the sample data, even though it is actually false in the population. In other words, it’s a failure to recognize a real effect or difference.
Suppose a medical researcher is testing a new drug to see if it’s effective in treating a certain condition. The null hypothesis (H 0 ) states that the drug has no effect, while the alternative hypothesis (H 1 ) suggests that the drug is effective. If the researcher conducts a statistical test and fails to reject the null hypothesis (H 0 ), concluding that the drug is not effective, when in fact it does have an effect, this would be a Type II error.
The table given below shows the relationship between True and False:
Error Type | Description | Also Known as | When It Occurs |
---|---|---|---|
Type I | Rejecting a true null hypothesis | False Positive | You believe there is an effect or difference when there isn’t |
Type II | Failing to reject a false null hypothesis | False Negative | You believe there is no effect or difference when there is |
Some of examples of type I error include:
- Medical Testing : Suppose a medical test is designed to diagnose a particular disease. The null hypothesis ( H 0 ) is that the person does not have the disease, and the alternative hypothesis ( H 1 ) is that the person does have the disease. A Type I error occurs if the test incorrectly indicates that a person has the disease (rejects the null hypothesis) when they do not actually have it.
- Legal System : In a criminal trial, the null hypothesis ( H 0 ) is that the defendant is innocent, while the alternative hypothesis ( H 1 ) is that the defendant is guilty. A Type I error occurs if the jury convicts the defendant (rejects the null hypothesis) when they are actually innocent.
- Quality Control : In manufacturing, quality control inspectors may test products to ensure they meet certain specifications. The null hypothesis ( H 0 ) is that the product meets the required standard, while the alternative hypothesis ( H 1 ) is that the product does not meet the standard. A Type I error occurs if a product is rejected (null hypothesis is rejected) as defective when it actually meets the required standard.
Using the same H 0 and H 1 , some examples of type II error include:
- Medical Testing : In a medical test designed to diagnose a disease, a Type II error occurs if the test incorrectly indicates that a person does not have the disease (fails to reject the null hypothesis) when they actually do have it.
- Legal System : In a criminal trial, a Type II error occurs if the jury acquits the defendant (fails to reject the null hypothesis) when they are actually guilty.
- Quality Control : In manufacturing, a Type II error occurs if a defective product is accepted (fails to reject the null hypothesis) as meeting the required standard.
Some of the common factors affecting errors are:
- Sample Size: In statistical hypothesis testing, larger sample sizes generally reduce the probability of both Type I and Type II errors. With larger samples, the estimates tend to be more precise, resulting in more accurate conclusions.
- Significance Level: The significance level (α) in hypothesis testing determines the probability of committing a Type I error. Choosing a lower significance level reduces the risk of Type I error but increases the risk of Type II error, and vice versa.
- Effect Size: The magnitude of the effect or difference being tested influences the probability of Type II error. Smaller effect sizes are more challenging to detect, increasing the likelihood of failing to reject the null hypothesis when it’s false.
- Statistical Power: The power of Statistics (1 – β) dictates that the opportunity for rejecting a wrong null hypothesis is based on the inverse of the chance of committing a Type II error. The power level of the test rises, thus a chance of the Type II error dropping.
To minimize Type I and Type II errors in hypothesis testing, there are several strategies that can be employed based on the information from the sources provided:
- By setting a lower significance level, the chances of incorrectly rejecting the null hypothesis decrease, thus minimizing Type I errors.
- Increasing the sample size reduces the variability of the statistic, making it less likely to fall in the non-rejection region when it should be rejected, thus minimizing Type II errors.
Some of the key differences between Type I and Type II Errors are listed in the following table:
Aspect | Type I Error | Type II Error |
---|---|---|
Definition | Incorrectly rejecting a true null hypothesis | Failing to reject a false null hypothesis |
Also known as | False positive | False negative |
Probability symbol | α (alpha) | β (beta) |
Example | Concluding that a person has a disease when they do not (false alarm) | Concluding that a person does not have a disease when they do (missed diagnosis) |
Prevention strategy | Adjusting the significance level (α) | Increasing sample size or effect size (to increase power) |
Conclusion – Type I and Type II Errors
In conclusion, type I errors occur when we mistakenly reject a true null hypothesis, while Type II errors happen when we fail to reject a false null hypothesis. Being aware of these errors helps us make more informed decisions, minimizing the risks of false conclusions.
People Also Read:
Difference between Null and Alternate Hypothesis Z-Score Table
Type I and Type II Errors – FAQs
What is type i error.
Type I Error occurs when a null hypothesis is incorrectly rejected, indicating a false positive result, concluding that there is an effect or difference when there isn’t one.
What is an Example of a Type 1 Error?
An example of Type I Error is that convicting an innocent person (null hypothesis: innocence) based on insufficient evidence, incorrectly rejecting the null hypothesis of innocence.
What is Type II Error?
Type II Error happens when a null hypothesis is incorrectly accepted, failing to detect a true effect or difference when one actually exists.
What is an Example of a Type 2 Error?
An example of type 2 error is that failing to diagnose a disease in a patient (null hypothesis: absence of disease) despite them actually having the disease, incorrectly failing to reject the null hypothesis.
What is the difference between Type 1 and Type 2 Errors?
Type I error involves incorrectly rejecting a true null hypothesis, while Type II error involves failing to reject a false null hypothesis. In simpler terms, Type I error is a false positive, while Type II error is a false negative.
What is Type 3 Error?
Type 3 Error is not a standard statistical term. It’s sometimes informally used to describe situations where the researcher correctly rejects the null hypothesis but for the wrong reason, often due to a flaw in the experimental design or analysis.
How are Type I and Type II Errors related to hypothesis testing?
In hypothesis testing, Type I Error relates to the significance level (α), which represents the probability of rejecting a true null hypothesis. Type II Error relates to the power of the test (β), which represents the probability of failing to reject a false null hypothesis.
What are some examples of Type I and Type II Errors?
Type I Error: Rejecting a null hypothesis that a new drug has no side effects when it actually does (false positive). Type II Error: Failing to reject a null hypothesis that a new drug has no effect when it actually does (false negative).
How can one minimize Type I and Type II Errors?
Type I Error can be minimized by choosing a lower significance level (α) for hypothesis testing. Type II Error can be minimized by increasing the sample size or improving the sensitivity of the test.
What is the relationship between Type I and Type II Errors?
There is often a trade-off between Type I and Type II Errors. Decreasing the probability of one type of error typically increases the probability of the other.
How do Type I and Type II Errors impact decision-making?
Type I Errors can lead to false conclusions, such as mistakenly believing a treatment is effective when it’s not. Type II Errors can result in missed opportunities, such as failing to identify an effective treatment.
In which fields are Type I and Type II Errors commonly encountered?
Type I and Type II Errors are encountered in various fields, including medical research, quality control, criminal justice, and market research.
Please Login to comment...
Similar reads, improve your coding skills with practice.
What kind of Experience do you want to share?

In order to continue enjoying our site, we ask that you confirm your identity as a human. Thank you very much for your cooperation.

A Definitive Guide on Types of Error in Statistics
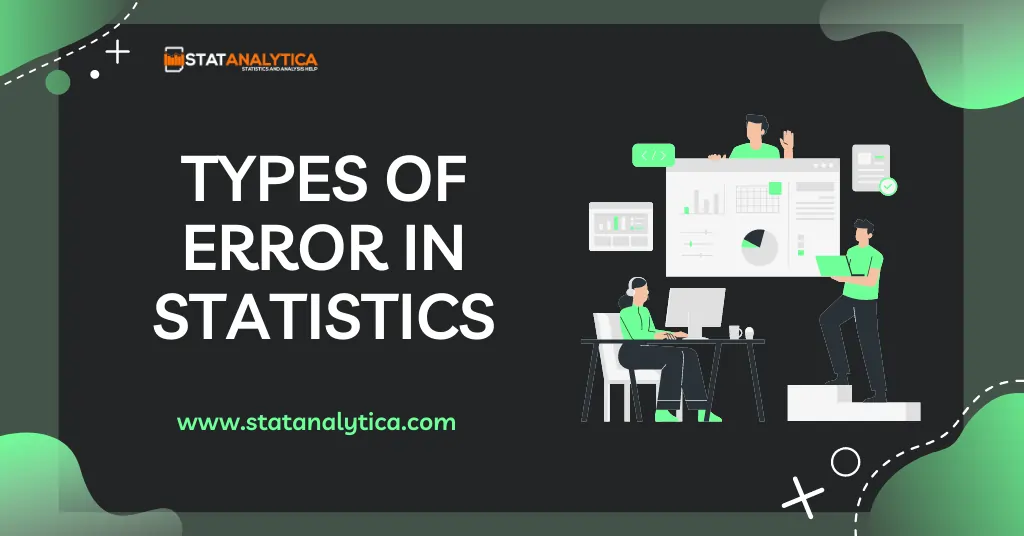
Most of the students are not aware of the types of error in statistics. This guide will help you to know everything about the types of error in statistics. Let’s explore the guide:-
As ‘statistics’ relates to the mathematical term, individuals start analyzing it as a problematic terminology, but it is the most exciting and straightforward form of mathematics. If you are looking for mathematics experts who can complete your math homework, then don’t worry. We are here to help you, our expert can provide you with the best maths homework help.
As the word ‘statistics’ refers that it consists of quantified statistic figures. That we use to represent and summarize the given data of an experiment or the real-time studies. In this article, we will discuss the following topics in detail:-
- What is the error in statistics?
- What is the standard error in statistics?
- How many types of errors in statistics?
- What are the sampling error and non-sampling error in statistics?
- What is the margin of error in statistics formula?
Before discussing these topics as mentioned above in this article, we want to say that if you are looking for a statistics homework helper, then don’t worry you can get the best statistics homework help from our experts. So, what are you waiting for get help now!
What Is The Error In Statistics?
Table of Contents
Statistics is a methodology of gathering, analyzing, reviewing, and drawing the particular information’s conclusion. The statistical error is the difference between the collected data’s obtained value and the actual value of the collected data. The higher the error value, the lesser will be the representative data of the community.
In simple words, a statistics error is a difference between a measured value and the actual value of the collected data. If the error value is more significant, then the data will be considered as the less reliable. Therefore, one has to keep in mind that the data must have minimal error value. So that the data can be considered to be more reliable.
Types Of Error In Statistics
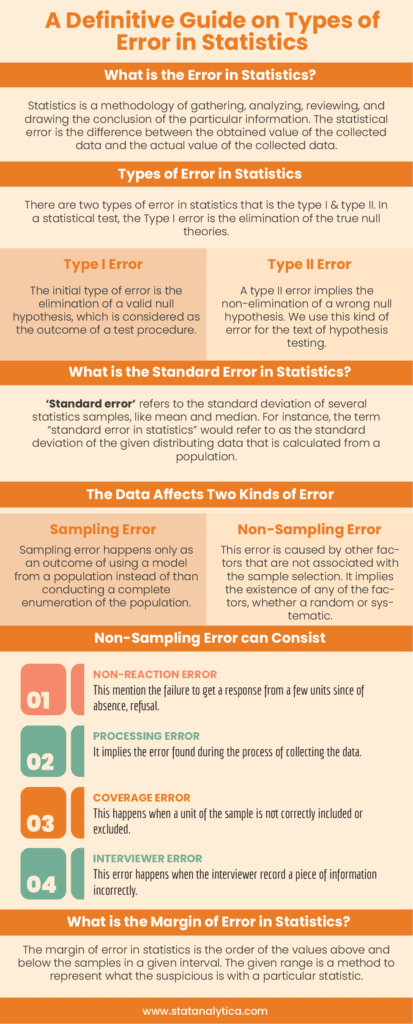
There are main two types of error in statistics that is the type I & type II. In a statistical test, the Type I error is the elimination of the true null theories. In contrast, the type II error is the non-elimination of the false null hypothesis.
Plenty of the statistical method rotates around the reduction of one or both kind of errors, although the complete rejection of either of them is impossible.
But by choosing the low threshold value and changing the alpha level, the features of the hypothesis test could be maximized. The information on type I error & type II error is used for bio-metrics, medical science, and computer science. On the other hand, we also mentioned type III error in this post.
Type I Error
The initial type of error is eliminating a valid null hypothesis, which is considered the outcome of a test procedure. This type of error is sometimes also called an error of the initial type/kind.
A null hypothesis is set before the beginning of an analysis. But for some situations, the null hypothesis is considered as not being present in the ‘cause and effect’ relationship of the items that are being tested.
This situation is donated as ‘n=0’ if you are conducting the test, and the outcome seems to signify that applied stimuli may cause a response, then the null hypothesis will be rejected.
Examples Of Type I Error
Let’s take an example of the trail of a condemned criminal. The null hypothesis can be considered as an innocent person, while others treat it as guilty. In this case, Type I error means that the individual is not found to be innocent. And individual needs to be sent to jail, despite being an innocent.
In another example, in medicinal tests, a Type I would bring its display as a cure of disease tends to minimize the seriousness of a disease, but actually, it is not doing the same.
Whenever a new dose of disease is tested, then the null hypothesis would consider as the dose will not disturb the progress of the particular ailment. Let’s take an example of it as a lab research a new cancer dose. The null hypothesis would be that the dose will not disturb the progress of the cancer cells.
After treating the cancer cells with medicine, the cancer cells will not progress. This may result to eliminate the null hypothesis of that drug that does not have any effect. The medicine is successful in stopping the growth of cancer cells, then the conclusion of rejecting the null value will be correct.
However, while testing the medicine, something else would help to stop the growth rather than the medicine, then this could be treated as an example of an incorrect elimination of the null hypothesis that is a type I error.
Type II Error
A type II error implies the non-elimination of a wrong null hypothesis. We use this kind of error for the text of hypothesis testing. In the statistical data analysis, type I errors are the elimination of the true null hypothesis.
On the other hand, type II error is a kind of error that happens when someone is not able to eliminate a null hypothesis, which is wrong. In simple words, type II error generates a false positive. The error eliminates the other hypothesis, even though that does not happen due to chance.
A type II error proves an idea that has been eliminated, demanding the two observances could be identical, even though both of them are dissimilar. Besides, a type II errors do not eliminate the null hypothesis. Even though the other hypothesis has the true nature, or we can say that a false value is treated as true. A type II error is well-known as a ‘beta error’.
Type II Error’s example
Suppose a biometric company likes to compare the effectivity of the two medicines that are used for treating diabetic patients. The null hypothesis refers to the two treatments that are of similar effectivity.
A null hypothesis (H 0 ) is the demand of the organization that concern to eliminate one-tailed test uses. The other hypothesis refers to the two medications are not identically effective. The other hypothesis (H a ) is the calculation, which is backed by eliminating the null hypothesis.
The biotechnical organization decided to conduct a test on 4,000 diabetic patients to evaluate the treating effectivity. The organization anticipates the two medicines should have a similar number of diabetic patients to ensure the effectivity of the drugs. It chooses a significant value of 0.05 that signifies that it is ready to adopt a 6% chance of eliminating the null hypothesis when it is considered to be real or a chance of 6% of carrying out a type I error.
Suppose beta would be measured as 0.035 or as 3.5%. So, the chances of carrying out a type II error is 3.5%. When the two cures are not identical, then the null hypothesis must be eliminated. Still, the biotechnical organization does not eliminate the null hypothesis if the medicine is not identically effective, then the type II error happens.
Type III Error
Type III errors, also known as conceptual errors, occur when researchers test the wrong hypothesis or design an experiment that does not accurately address the research question.
In simpler words, a type III error arises when a researcher investigates a relationship between two variables that are not related and concludes that they are related based on the results of the analysis. As a result, this error can result in misguided policies and inaccurate conclusions.
Example of Type III Error
For example, let’s say a researcher is interested in examining the relationship between ice cream consumption and crime rates. After collecting and analyzing data, the researcher finds a significant correlation between the two variables. However, this relationship is a type 3 error because ice cream consumption does not cause crime.
In this case, the researcher has failed to consider other important variables, such as temperature or time of day, that may influence ice cream consumption and crime rates. Therefore, the researcher’s conclusion that ice cream consumption is related to crime rates is incorrect, and any policies or interventions based on this conclusion would be misguided.
Test Your Knowledge About Types Of Error In Statistics
- A Type I error occurs if
- A null hypothesis needs to be not rejected but must be rejected.
- The given null hypothesis is rejected, but actually, it needs not be rejected.
- A test statistic is wrong.
- None of these.
The given null hypothesis is rejected, but actually, it needs not be rejected. |
2. A Type II error occurs if
A null hypothesis needs to be not rejected but must be rejected. |
3. Deciding on the significance level ‘α’ helps in determining
- the Type II error’s probability.
- the Type I error’s probability.
the Type I error’s probability. |
4. Suppose a water bottle has a label stating – the volume is 12 oz. A user group found that the bottle is under‐filled and decided to perform a test. In this case, a Type I error would mean
- The user group does not summarize that the bottle has less than 12 oz. The mean is also less than 12 oz.
- The user group has proof that the label is wrong.
- The user group summarizes that the bottle has less than 12 oz. The mean is also 12 oz.
The user group summarizes that the bottle has less than 12 oz. The mean is also 12 oz. |
5. A Type I error happens in the case the null hypothesis is
- either correct or incorrect.
correct. |
6. A Type II error happens in the case the null hypothesis is
incorrect. |
7. Suppose the significance level ‘α’ is raised; in this case, the uncertainty of a Type I error will also
- remain the same.
increase. |
8. Suppose the significance level ‘α’ is raised; in this case, the uncertainty of a Type II error will also
decrease. |
9. Suppose the significance level ‘α’ is raised; in this case, the power will also
10. The test’s power can increase by
- selecting a smaller value for α.
- using a normal approximation.
- using a larger sample size.
using a larger sample size. |
What Is The Standard Error In Statistics?
‘Standard error’ refers to the standard deviation of several statistics samples, like mean and median. For instance, the term “standard error in statistics” would refer to as the standard deviation of the given distributing data that is calculated from a population. The smaller the value of the standard error, the larger representative the overall data.
The relation between standard deviation and the standard error is that for a provided data, the standard error is equal to the standard deviation (SD) upon the square root of the offered data size.
Standard error = standard deviation
√ Given data
The standard error is inversely proportional to the given model size, which means the higher the model size, the lesser the value of standard error since the statistic will tend to the actual value.
Standard error ∝ 1/sample size
The standard error is taken as a portion of explanatory statistics. The standard error shows the standard deviation (SD) of an average value into a data set. It treats as a calculation of the random variables as well as to the extent. The smaller the extent, the higher the accuracy of the dataset.
The Data Affects Two Kinds Of Error
Here in this section, you get to know how the data affects two kinds of error:
1. Sampling error
Sampling error happens only as an outcome of using a model from a population instead of than conducting a complete enumeration of the population. It implies a difference between a prediction of the value of community and the ‘true or real’ value of that sample population that would result if a census would be taken. The sampling error does not happen in a census as it is based on the whole community.
Sampling error would cause when:
- The method of sampling is not accidental.
- The samples are smaller to show the population accurately.
- The proportions of several features within the sample would not be identical to the proportion of the features for the entire population.
Sampling error can be calculated and handled in random samples. Especially where every unit has a hope of selection, and the hope can be measured. In other words, the increment in the sample size would decrements in the sampling error.
2. Non-sampling error
This error is caused by other factors that are not associated with the sample selection. It implies the existence of any of the factors, whether a random or systematic, that output as the true value of the population. The non-sampling error can happen at any step of a census or study sample. And it is not easily quantified or identified.
Non-sampling error can consist
- Non-reaction error: This mention the failure to get a response from a few units since of absence, refusal, non-contact, or some other reason. The non-reaction error can be a partial reaction (that is, a chosen unit has not supported the solution to a few problems) or a complete reaction (that is no information has been taken at all from a chosen unit).
- Processing error: It implies the error found during the process of collecting the data, coding, data entry, editing, and outputting.
- Coverage error: This happens when a unit of the sample is not correctly included or excluded or is replicated in the sample (for example, an interviewer is not able to interview a chosen household).
- Interviewer error: This error happens when the interviewer record a piece of information incorrectly, is not objective or neutral or assumes reaction based on looks or other features.
What Is The Margin Of Error In Statistics?
The margin of error in statistics is the order of the values above and below the samples in a given interval. The given range is a method to represent what the suspicious is with a particular statistic.
For example, a survey may be referred that there is a 97% confidence interval of 3.88 and 4.89. This means that when a survey would be conducted again with the same technical method, 97% of the time, the real population statistic will lay within the estimated interval (i.e., 3.88 and 4.89) 97% of the time.
The Formula For Calculating The Margin Of Error Percentage
A marginal error implies to you how many different values would be resulted from the true population value. For instance, a 94% confidence interval by a 3% margin of error means that your calculated statistics would be within 3% points to the true population value 945 of the time.
The Margin Of Error Can Be Measured In Two Ways:
- The margin of error = Standard deviation of the statistics x Critical range value
- The margin of error = Standard error of the statistic x Critical value
Steps on Calculate Margin of Error
Step 1: Calculate the critical value. The critical value is either of a z-score or t-score. In general, for the smaller value (under 30) or when you do not have the standard deviation of the population. Then use a t-score, in another way, use a z-score.
Step 2: Calculate the standard error or standard deviation . These two are an identical thing, and merely you should have the population parameter value to measure standard deviation.
Step 3: Multiply the standard deviation and the critical value.
Sample problem: 100 students were polled and had a GPA of 2.5 with a standard deviation of 0.5. Calculate the margin of error in statistics for a 90% confidence range.
- The value of critical for a 90% confidence range is 1.645 (see the table of z-score).
- The SD is 0.5 (as it is a sample, we require the standard error for the mean.) the SE can be calculated as the standard deviation / √ Given data; therefore, 0.5/ √ (100) = 0.05.
- 1.645*0.05 = 0.08125.
The margin of error for a proportion formula:

p-hat = sample proportion; n= sample size; z= z-score
Steps to calculate margin error for a proportion
Step 1: Calculate p-hat. This can be calculated by the number of the population who have been responded positively. It means that they have given the answer related to the given statement of the question.
Step 2: Calculate the z-score with follows the confidence level value.
Step3: Put all the value in the given formula:

Step 4: Convert step 3 into the percentage.
Sample problem
1000 individuals were polled, and 380 think that climate alters not because of human pollution. Calculate the ME for a given 90% confidence value.
- The number of individuals who respond positively; 38%.
- A 90% confidence level has a critical value (z-score) of 1.645.
- Calculate the value by using the formula
=1.645*[ √ {(38*.62)/(1000)}]
4. Convert the value into a percentage.
0.0252= 2.52%
The margin of error in statistics is 2.52%.
This is all about types of error in statistics. Use the details as mentioned earlier, you can understand types of error in statistics. But, still, you find any issue related to the topic error in statistics. Then you can get in touch 24*7 with our professional experts. They have enough knowledge of this particular topic; therefore, they can resolve all the queries of yours.
Get the best statistics homework help from the professional experts at a reasonable price. We provide you the assignment before the deadline so that you can check your work. And we also provide a plagiarism-free report which defines the uniqueness of the content. We are providing world-class help on math assignment help to the students who are living across the globe. We are the most reliable math assignment helpers in the world.
Frequently Asked Question
Q1. what is the difference between type 1 and type 2 errors.
In statistical hypothesis testing, Type 1 error is caused by rejecting any of the null hypotheses (in case it has true value). Type II error occurs while a null hypothesis is taken (if it does not have a true value).
Q2. What is random error example?
A random error can happen because of the measuring instrument and can be affected by variations in the experimental environment. For example, a spring balance can produce some variation in calculations because of the unloading and loading conditions, fluctuations in temperature, etc.
Q3. What type of error is human error?
Random errors are considered to be natural errors. Systematic errors occur because of problems or imprecision with instruments. Now, human error is something that humans screwed up; they have made a mistake. These cause human errors.
Q4. What are Type 3 errors in statistics?
A type III error occurs in statistics when you correctly conclude that the two groups are statistically different but incorrect about the difference’s direction.
Related Posts
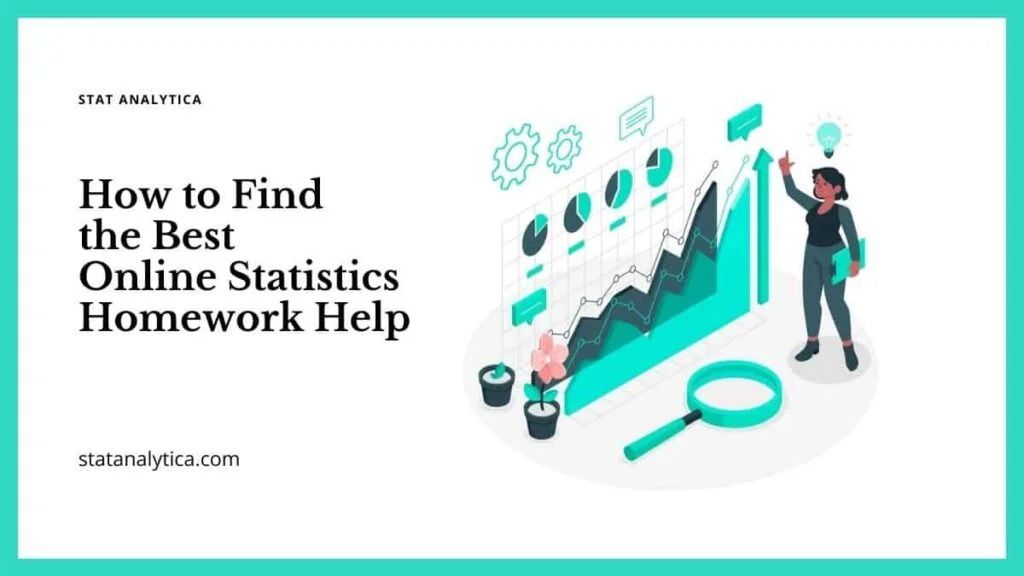
How to Find the Best Online Statistics Homework Help
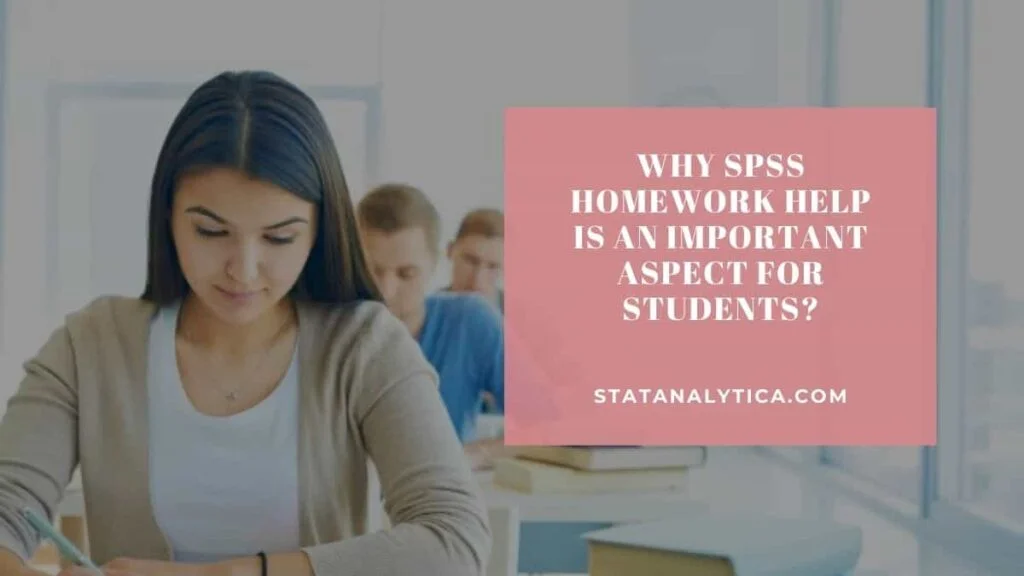
Why SPSS Homework Help Is An Important aspect for Students?
What is a scientific hypothesis?
It's the initial building block in the scientific method.
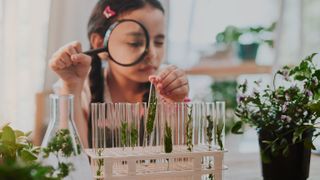
Hypothesis basics
What makes a hypothesis testable.
- Types of hypotheses
- Hypothesis versus theory
Additional resources
Bibliography.
A scientific hypothesis is a tentative, testable explanation for a phenomenon in the natural world. It's the initial building block in the scientific method . Many describe it as an "educated guess" based on prior knowledge and observation. While this is true, a hypothesis is more informed than a guess. While an "educated guess" suggests a random prediction based on a person's expertise, developing a hypothesis requires active observation and background research.
The basic idea of a hypothesis is that there is no predetermined outcome. For a solution to be termed a scientific hypothesis, it has to be an idea that can be supported or refuted through carefully crafted experimentation or observation. This concept, called falsifiability and testability, was advanced in the mid-20th century by Austrian-British philosopher Karl Popper in his famous book "The Logic of Scientific Discovery" (Routledge, 1959).
A key function of a hypothesis is to derive predictions about the results of future experiments and then perform those experiments to see whether they support the predictions.
A hypothesis is usually written in the form of an if-then statement, which gives a possibility (if) and explains what may happen because of the possibility (then). The statement could also include "may," according to California State University, Bakersfield .
Here are some examples of hypothesis statements:
- If garlic repels fleas, then a dog that is given garlic every day will not get fleas.
- If sugar causes cavities, then people who eat a lot of candy may be more prone to cavities.
- If ultraviolet light can damage the eyes, then maybe this light can cause blindness.
A useful hypothesis should be testable and falsifiable. That means that it should be possible to prove it wrong. A theory that can't be proved wrong is nonscientific, according to Karl Popper's 1963 book " Conjectures and Refutations ."
An example of an untestable statement is, "Dogs are better than cats." That's because the definition of "better" is vague and subjective. However, an untestable statement can be reworded to make it testable. For example, the previous statement could be changed to this: "Owning a dog is associated with higher levels of physical fitness than owning a cat." With this statement, the researcher can take measures of physical fitness from dog and cat owners and compare the two.
Types of scientific hypotheses
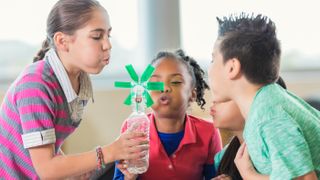
In an experiment, researchers generally state their hypotheses in two ways. The null hypothesis predicts that there will be no relationship between the variables tested, or no difference between the experimental groups. The alternative hypothesis predicts the opposite: that there will be a difference between the experimental groups. This is usually the hypothesis scientists are most interested in, according to the University of Miami .
For example, a null hypothesis might state, "There will be no difference in the rate of muscle growth between people who take a protein supplement and people who don't." The alternative hypothesis would state, "There will be a difference in the rate of muscle growth between people who take a protein supplement and people who don't."
If the results of the experiment show a relationship between the variables, then the null hypothesis has been rejected in favor of the alternative hypothesis, according to the book " Research Methods in Psychology " (BCcampus, 2015).
There are other ways to describe an alternative hypothesis. The alternative hypothesis above does not specify a direction of the effect, only that there will be a difference between the two groups. That type of prediction is called a two-tailed hypothesis. If a hypothesis specifies a certain direction — for example, that people who take a protein supplement will gain more muscle than people who don't — it is called a one-tailed hypothesis, according to William M. K. Trochim , a professor of Policy Analysis and Management at Cornell University.
Sometimes, errors take place during an experiment. These errors can happen in one of two ways. A type I error is when the null hypothesis is rejected when it is true. This is also known as a false positive. A type II error occurs when the null hypothesis is not rejected when it is false. This is also known as a false negative, according to the University of California, Berkeley .
A hypothesis can be rejected or modified, but it can never be proved correct 100% of the time. For example, a scientist can form a hypothesis stating that if a certain type of tomato has a gene for red pigment, that type of tomato will be red. During research, the scientist then finds that each tomato of this type is red. Though the findings confirm the hypothesis, there may be a tomato of that type somewhere in the world that isn't red. Thus, the hypothesis is true, but it may not be true 100% of the time.
Scientific theory vs. scientific hypothesis
The best hypotheses are simple. They deal with a relatively narrow set of phenomena. But theories are broader; they generally combine multiple hypotheses into a general explanation for a wide range of phenomena, according to the University of California, Berkeley . For example, a hypothesis might state, "If animals adapt to suit their environments, then birds that live on islands with lots of seeds to eat will have differently shaped beaks than birds that live on islands with lots of insects to eat." After testing many hypotheses like these, Charles Darwin formulated an overarching theory: the theory of evolution by natural selection.
"Theories are the ways that we make sense of what we observe in the natural world," Tanner said. "Theories are structures of ideas that explain and interpret facts."
- Read more about writing a hypothesis, from the American Medical Writers Association.
- Find out why a hypothesis isn't always necessary in science, from The American Biology Teacher.
- Learn about null and alternative hypotheses, from Prof. Essa on YouTube .
Encyclopedia Britannica. Scientific Hypothesis. Jan. 13, 2022. https://www.britannica.com/science/scientific-hypothesis
Karl Popper, "The Logic of Scientific Discovery," Routledge, 1959.
California State University, Bakersfield, "Formatting a testable hypothesis." https://www.csub.edu/~ddodenhoff/Bio100/Bio100sp04/formattingahypothesis.htm
Karl Popper, "Conjectures and Refutations," Routledge, 1963.
Price, P., Jhangiani, R., & Chiang, I., "Research Methods of Psychology — 2nd Canadian Edition," BCcampus, 2015.
University of Miami, "The Scientific Method" http://www.bio.miami.edu/dana/161/evolution/161app1_scimethod.pdf
William M.K. Trochim, "Research Methods Knowledge Base," https://conjointly.com/kb/hypotheses-explained/
University of California, Berkeley, "Multiple Hypothesis Testing and False Discovery Rate" https://www.stat.berkeley.edu/~hhuang/STAT141/Lecture-FDR.pdf
University of California, Berkeley, "Science at multiple levels" https://undsci.berkeley.edu/article/0_0_0/howscienceworks_19
Sign up for the Live Science daily newsletter now
Get the world’s most fascinating discoveries delivered straight to your inbox.
Hot Tub of Despair: The deadly ocean pool that traps and pickles creatures that fall in
Enormous deposit of rare earth elements discovered in heart of ancient Norwegian volcano
Melatonin may stave off age-related vision loss, study hints
Most Popular
- 2 Enormous deposit of rare earth elements discovered in heart of ancient Norwegian volcano
- 3 'At least 150,000 tons' of water frost discovered atop Mars' tallest volcanoes
- 4 DARPA's military-grade 'quantum laser' will use entangled photons to outshine conventional laser beams
- 5 James Webb telescope discovers most distant supernova ever seen
- 2 New RSV shots tied to rare nervous system disorder — should you worry?
- 3 Planet Nine: Is the search for this elusive world nearly over?
- 4 The 1st 'major lunar standstill' in more than 18 years is about to occur. Here's how to see it.
- 5 Leatherback turtle dives deeper than a Navy sub, smashing world record in the process
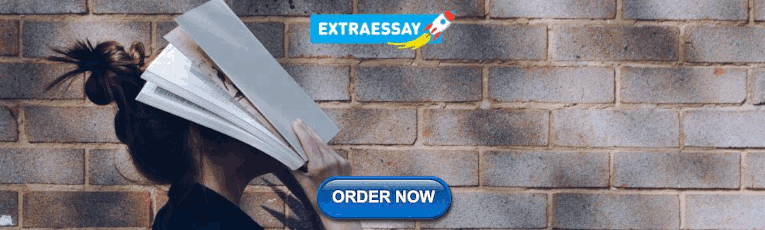
IMAGES
VIDEO
COMMENTS
In statistics, a Type I error is a false positive conclusion, while a Type II error is a false negative conclusion.
A statistically significant result cannot prove that a research hypothesis is correct (which implies 100% certainty). Because a p-value is based on probabilities, there is always a chance of making an incorrect conclusion regarding accepting or rejecting the null hypothesis (H 0).
Type II Error: A type II error is a statistical term used within the context of hypothesis testing that describes the error that occurs when one accepts a null ...
Type I and type II errors. In statistical hypothesis testing, a type I error, or a false positive, is the rejection of the null hypothesis when it is actually true. For example, an innocent person may be convicted. A type II error, or a false negative, is the failure to reject a null hypothesis that is actually false.
In hypothesis testing, understanding Type 2 errors is essential. They represent a false negative, where we fail to detect a significant effect that genuinely exists. By thoughtfully designing our studies, we can reduce the risk of these errors and make more informed statistical decisions.
In statistical hypothesis testing, a type II error is a situation wherein a hypothesis test fails to reject the null hypothesis that is false. In other
Statistical significance is a term used by researchers to state that it is unlikely their observations could have occurred under the null hypothesis of a statistical test.Significance is usually denoted by a p-value, or probability value.. Statistical significance is arbitrary - it depends on the threshold, or alpha value, chosen by the researcher.
In summary, the likelihood of Type II Errors increases: the closer something is to what we're testing for (.4 is closer to .5 than .3 is, and so .4 coins make Type II Errors more likely than .3 coins). We don't control this. when we use smaller sample sizes (a sample size of 100 makes Type II Errors more likely than a sample size of 1000).
We use the symbols \(\alpha\) = P(Type I Error) and β = P(Type II Error). The critical value is a cutoff point on the horizontal axis of the sampling distribution that you can compare your test statistic to see if you should reject the null hypothesis.
Type I error: The null hypothesis claims that the tomato plant is alive, and it is true, but the students make the mistake of thinking that the plant is already dead. Type II error: The tomato plant is already dead (the null hypothesis is false), but the students do not notice it, and believe that the tomato plant is alive.
Statisticians designed hypothesis tests to control Type I errors while Type II errors are much less defined. Consequently, many statisticians state that it is better to fail to detect an effect when it exists than it is to conclude an effect exists when it doesn't.
- [Instructor] What we're gonna do in this video is talk about Type I errors and Type II errors and this is in the context of significance testing. So just as a little bit of review, in order to do a significance test, we first come up with a null and an alternative hypothesis. And we'll do this on some population in question.
9.2: Type I and Type II Errors. When you perform a hypothesis test, there are four possible outcomes depending on the actual truth (or falseness) of the null hypothesis H0 H 0 and the decision to reject or not. The outcomes are summarized in the following table: The four possible outcomes in the table are: The decision is not to reject H0 H 0 ...
6.1 - Type I and Type II Errors. When conducting a hypothesis test there are two possible decisions: reject the null hypothesis or fail to reject the null hypothesis. You should remember though, hypothesis testing uses data from a sample to make an inference about a population. When conducting a hypothesis test we do not know the population ...
Introduction; 9.1 Null and Alternative Hypotheses; 9.2 Outcomes and the Type I and Type II Errors; 9.3 Probability Distribution Needed for Hypothesis Testing; 9.4 Rare Events, the Sample, Decision and Conclusion; 9.5 Additional Information and Full Hypothesis Test Examples; 9.6 Hypothesis Testing of a Single Mean and Single Proportion; Key Terms; Chapter Review; Formula Review
In this setting, Type I and Type II errors are fundamental concepts to help us interpret the results of the hypothesis test. 1 They are also vital components when calculating a study sample size. 2, 3 We have already briefly met these concepts in previous Research Design and Statistics articles 2, 4 and here we shall consider them in more detail.
Consider now the mean of the second sample. If the sample comes from the same population its mean will also have a 95% chance of lying within 196 standard errors of the population mean but if we do not know the population mean we have only the means of our samples to guide us.
What are type I and type II errors, and how we distinguish between them? Briefly: Type I errors happen when we reject a true null hypothesis. Type II errors happen when we fail to reject a false null hypothesis. We will explore more background behind these types of errors with the goal of understanding these statements.
9.2: Outcomes, Type I and Type II Errors. When you perform a hypothesis test, there are four possible outcomes depending on the actual truth (or falseness) of the null hypothesis H0 and the decision to reject or not. The outcomes are summarized in the following table: The four possible outcomes in the table are:
Hypothesis testing is an important activity of empirical research and evidence-based medicine. A well worked up hypothesis is half the answer to the research question. For this, both knowledge of the subject derived from extensive review of the literature ...
Type I and Type II errors are subjected to the result of the null hypothesis. In case of type I or type-1 error, the null hypothesis is rejected though it is true whereas type II or type-2 error, the null hypothesis is not rejected even when the alternative hypothesis is true.
In conclusion, type I errors occur when we mistakenly reject a true null hypothesis, while Type II errors happen when we fail to reject a false null hypothesis. Being aware of these errors helps us make more informed decisions, minimizing the risks of false conclusions.
The two types of errors that are possible in hypothesis testing are called type 1 and type 2 errors. These errors result in incorrect conclusions. If this happens, the whole study can be ...
Correct Answer: The given null hypothesis is rejected, but actually, it needs not be rejected.
A scientific hypothesis is a tentative, testable explanation for a phenomenon in the natural world. It's the initial building block in the scientific method. Many describe it as an "educated guess ...