Spatio-temporal analysis of urban expansion using remote sensing data and GIS for the sustainable management of urban land: the case of Burayu, Ethiopia
- Talema, Abebe Hambe
- Nigusie, Wubshet Berhanu
PurposeThe purpose of this study is to analyze the horizontal expansion of Burayu Town between 1990 and 2020. The study typically acts as a baseline for integrated spatial planning in small- and medium-sized towns, which will help to plan sustainable utilization of land.Design/methodology/approachLandsat5-TM, Landsat7 ETM+, Landsat5 TM and Landsat8 OLI were used in the study, along with other auxiliary data. The LULC map classifications were generated using the Random Forest Package from the Comprehensive R Archive Network. Post-classification, spatial metrics, and per capita land consumption rate were used to understand the manner and rate of expansion of Burayu Town. Focus group discussions and key informant interviews were also used to validate land use classes through triangulation.FindingsThe study found that the built-up area was the most dynamic LULC category (85.1%) as it increased by over 4,000 ha between 1990 and 2020. Furthermore, population increase did not result in density increase as per capita land consumption increased from 0.024 to 0.040 during the same period.Research limitations/implicationsAs a result of financial limitations, there were no high-resolution satellite images available, making it challenging to pinpoint the truth as it is on the ground. Including senior citizens in the study region allowed this study to overcome these restrictions and detect every type of land use and cover.Practical implicationsData on urban growth are useful for planning land uses, estimating growth rates and advising the government on how best to use land. This can be achieved by monitoring and reviewing development plans using satellite imaging data and GIS tools.Originality/valueThe use of Random Forest for image classification and the employment of local knowledge to validate the accuracy of land cover classification is a novel approach to properly customize remote sensing applications.
Skip to content
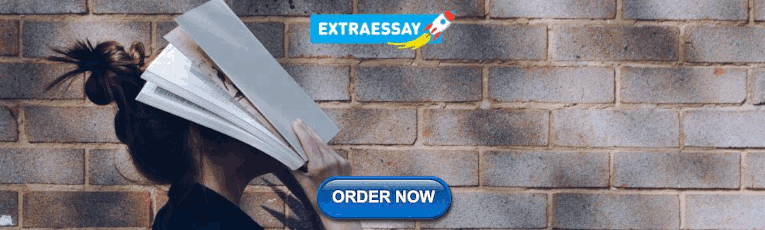
Get Revising
Join get revising, already a member.
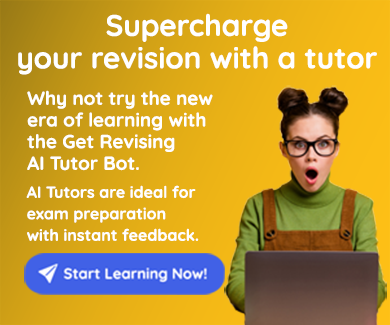
Dynamic Development: Ethiopia
- Development
- Created by: 15patersond
- Created on: 09-02-20 11:21
- Economy growing 2.6%/year
- HDI: 165/188 countries worldwide
- Vegetables and Legumes
- 80% of all jobs
- 33% birth rate
- 28% of population with malnutrition
- Was once called Abyssinia - avoided colonial rule.
- Italy took control, but regained independence via rebels + Britain support
- Years of unrest after WW2 led to a successful military coup by USSR in 1974.
- 1977-78: the Ethiopian "Red Terror" - 50,000 people killed and 1.5 million relocated
- Ethiopia is still food deficient.
- Became Federal Democratic Republic: free trade, farmers given benefits
- Since 2012, new training has increased crop yield for farmers.
- Unreliable Rainfall
- Climate change altering monsoon and dry seasons
- Eastern Areas = Drought, desertification Western Areas = Mild and Wet
- Central and Western = Mountainous
- Livestock limited to lowlands = desertification
- Livestock at risk of disease or malnutrition
- Economy based on primary sector
- Negative balance of trade
- Government spending improving healthcare
- Arrival of TNCs investing in their economy
- Newer methods of agriculture is improving QOL
- Eradicate extreme poverty + hunger
- Achieve universal primary education
- Gender Equaltiy
- Global partnerships for development
- Reduce Child Mortality
- Improve maternal heath
- Combat disease
No comments have yet been made
Similar Geography resources:
Dynamic Development: Ethiopia 2 3.0 / 5 based on 1 rating
Ethiopia Links with other states 0.0 / 5
Geography People and Planet (notes on whole topic) 5.0 / 5 based on 1 rating Teacher recommended
Hydro Electric Power - HEP 2.0 / 5 based on 1 rating
Aid/Sustainable Development Case Study - WaterAid 4.0 / 5 based on 3 ratings
Geography Topics 1.5 / 5 based on 2 ratings
Geography 0.0 / 5
Worldwide effect of CLIMATE CHNAGE: 0.0 / 5
Geography:People And Society: Case studies 3.0 / 5 based on 2 ratings
Case Study - Drought, Ethiopia, 2006 0.0 / 5
- Utility Menu

Development in a Complex World: The Case of Ethiopia
14.52 MB |
This research compendium provides an explanation of Ethiopia’s fundamental economic challenge of slowing economic growth after an exceptional growth acceleration — a challenge that has been compounded by COVID-19, conflict, and climate change impacts. Ethiopia has experienced exceptional growth since the early 2000s but began to see a slowdown in the capacity of the economy to grow, export, and produce jobs since roughly 2015. This intensified a set of macroeconomic challenges, including high, volatile, and escalating inflation. This compendium identifies a path forward for more sustainable and inclusive growth that builds on the government’s Homegrown Economic Reform strategy. It includes growth diagnostics and economic complexity research as well as applications to unpack interacting macroeconomic distortions and inform diversification strategies. Drawing on lessons from past success in Ethiopia and new constraints, this compendium offers insights into what the Government of Ethiopia and the international community must do to unlock resilient, post-conflict economic recovery across Ethiopia.
The research across the chapters of this compendium was developed during the Growth Lab’s research project in Ethiopia from 2019 to 2022, supported through a grant by the United States Agency of International Development (USAID). This research effort, which was at times conducted in close collaboration with government and non-government researchers in Ethiopia, pushed the boundaries of Growth Lab research. The project team worked to understand to intensive shocks faced by the country and enable local capability building in the context of limited government resources in a very low-income country. Given the value of this learning, this compendium not only discusses challenges and opportunities in Ethiopia in significant detail but also describes how various tools of diagnostic work and economic strategy-building were used in practice. As such, it aims to serve as a teaching resource for how economic tools can be applied to unique development contexts. The compendium reveals lessons for Ethiopian policymakers regarding the country’s development path as well as numerous lessons that the development community and development practitioners can learn from Ethiopia.
SORT BY POLICY AREA
- Cities/Regions (53)
- COVID-19 (14)
- Diasporas (9)
- Economic Complexity (58)
- Gender (11)
- Green Growth (16)
- Growth Diagnostics (45)
- Inclusive Growth (20)
- Industrial Policy (24)
- Migration & Mobility (39)
- Poverty (5)
- Product Space (18)
- Remoteness (9)
- Skills & Human Capital (41)
- Structural Transformation/Diversification (34)
- Telework (3)
- Tourism (3)
- Trade/Foreign Direct Investment (26)
SORT BY FORMAT
- Book Chapter (14)
- Faculty Working Papers (138)
- Fellow Working Papers (99)
- Journal Articles (88)
- Reports (35)
- Web Articles (10)
SORT BY COUNTRY/REGION
- Africa (39)
- Europe (55)
- Latin America (84)
- Middle East (15)
- North America (24)
For additional research view the CID Publications page and the HKS Faculty Research Connection .
Thank you for visiting nature.com. You are using a browser version with limited support for CSS. To obtain the best experience, we recommend you use a more up to date browser (or turn off compatibility mode in Internet Explorer). In the meantime, to ensure continued support, we are displaying the site without styles and JavaScript.
- View all journals
- Explore content
- About the journal
- Publish with us
- Sign up for alerts
- Open access
- Published: 22 June 2024
Modeling and forecasting the volatility of some industry development indicators in Ethiopia using multivariate GARCH models
- Getachew Abate Dagnew 1 ,
- Birhan Walie Alamneh 2 &
- Wosenie Gebireamanuel Hailu 3
Scientific Reports volume 14 , Article number: 14395 ( 2024 ) Cite this article
99 Accesses
Metrics details
- Environmental social sciences
- Mathematics and computing
Industry development indicators refer to a set of measures used to assess the performance and growth of industries. The main aim of this study was to assess the relationship between industry development indicators in Ethiopia using a multivariate GARCH model based on World Bank data from 1982 to 2021. A time series technique using annual data for the period 1982–2021 is utilized, and multivariate generalized autoregressive conditional heteroscedasticity was performed for volatility modeling. The results of the diagonal BEKK (1, 1)-GARCH model showed that there is strong evidence for a GARCH effect and the presence of a weaker ARCH effect, Equations show a statistically significant co-variation in shocks, which depends more on their lags than on past errors. Consequently, development indicator shocks are influenced by past information. The cross-volatility effects are higher than the own-volatility effects in Industry GDP, manufacturing GDP, and manufacturing exports. However, past volatility shocks in industry growth have less effect on cross-volatility than its own volatility shock. The implication of the study is that both domestic policymakers and development partners should support and motivate the growth of manufacturing sectors and manufacturing exports, since this is a necessary condition for promoting industry growth.
Similar content being viewed by others
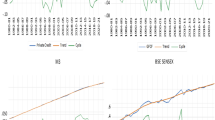
Cyclical dynamics and co-movement of business, credit, and investment cycles: empirical evidence from India
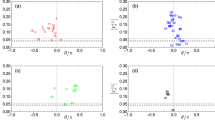
Relationship between Macroeconomic Indicators and Economic Cycles in U.S.
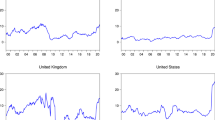
Quantifying nonlinear effects of BRIC and G4 liquidity on oil prices
Introduction.
Industrialization, as history has shown, is essential for economic growth. A structural shift from conventional to modern industrial sectors boosts output, encourages creativity, speeds up the spread of new technologies, and has other advantageous knock-on effects 1 . Any economic activity that includes the production of goods, the acquisition of service, and the provision of services is referred to as an industry 2 .
Industrialization has had a substantial impact on economic growth in countries like China, the Republic of Korea (Korea), the Taiwan Province of China (Taiwan), and Indonesia. Along with rapid growth, poverty rates have declined in many countries. Inequality has worsened in certain countries while remaining an issue in others 3 .
Ethiopia is one of the few African countries that has formulated and implemented a full-fledged industrial development strategy since the early 2000s, when industrial policy was taboo in international policy forums. The industry's manufacturing sector must expand in order to build national technological capacity, manufacturing capability, technological progress, productivity, and capital accumulation 4 .
Manufacturing is described as the physical or chemical transformation of material components into new products. The definition also includes the assembly of various elements of manufacture Products as a manufacturing activity, whether the production is done at a factory or at home, sold at retail or wholesale, and whether a power-driven machine is used or not 5 . Currently, Sub-Saharan Africa has the lowest per-capita manufacturing output of any inhabited region on the planet. Most African economies, in contrast to the newly industrialized (NIC) Asian countries, have so far failed to supplement agricultural and extractive output by raising average productivity through the creation of a substantial number of jobs in higher-value-added manufacturing sectors 6 .
Ethiopian manufacturing sectors contribute a great deal to exports, employment, and national output. Currently, Ethiopia is ranked among the five fastest-growing economies in the world. However, the sectors within manufacturing in Ethiopia are still in their infancy due to the shortage of skilled workers, lower competitiveness, lack of advanced technology, and sustainability awareness 7 .
Manufacturing firms are viewed as an essential element of a healthy and vibrant economy. They are seen as vital to the promotion of an enterprise culture and to the creation of jobs within the financial system 8 . Industry manufacturers are believed to provide an impetus to the economic progress of developing nations, and their importance is gaining widespread recognition 9 . There are several industrial development indicators, like industrial output, value added, employment, wages, industrial labor force, and manufactured and semi- manufactured exports at the aggregated sector level; GNP, population, geographical area, and total merchandise trade; and human resources: skills, education, and nutrition 10 , 11 , 12 .
The industry promotes various aspects of social integration through its general thrust towards modernization and makes a specific contribution to national economic integration 13 . From this, four features can be deduced. First, industrialization is not a one-time occurrence but rather a sustained process. Second, industrialization requires the application of modern science and technology to the production process. Third, the manufacturing sector plays the most important role in the industrialization process; that is, the key and dynamic roles in this process are played by the manufacturing sector. Last but not least, industrialization brings about a structural transformation of the national economy. As a result, this makes the manufacturing sector a dynamic component of the industrial sector 14 .
Growth in industrial manufacturing can be possible only through external demand with a high growth rate, that is, through export. The higher the growth rate in the manufacturing industry that exports determine, the faster the transfer of labor will be from sectors in which economic productivity is low to the industrial sector, which leads to a faster productivity increase 15 . Therefore, studying the linkage between manufacturing exports and various development indicators helps us understand the status of industry growth and the causes of manufacturing growth in Ethiopia.
Thus, the main aim of this study was to investigate the volatility and relationship between industry development indicators in Ethiopia from 1982 to 2021. Specifically, this research attempts to address the trends in industry development indicators, fit volatility models, and determines the appropriate one for the data and to forecast the volatility of industry development indicators for the next five years.
Ethiopia’s manufacturing sector was growing in terms of the number of firms, employment, output, value addition, exports, and investment. Growth figures appear to be high, mainly because of the country’s low industrial base. In practice, the sector has not grown as per the country’s growth potential and goals as stipulated in Growth and Transformation Plan-I (GTP-I) and GTP-II. For instance, its share in GDP remained low (only 7.4% in 2019, well below the African average and far below the 17% envisioned in GTP II). Exports grow slowly and erratically, with still around 10% of the export proceeds for the country 16 . Ethiopia is virtually at the beginning of the manufacturing industry's expansion 17 . Therefore, the manufacturing sector has an impact on both industry and overall economic growth. Thus, it is better to place too much emphasis on the impact of manufacturing exports on the annual growth of the industry, and all the above facts were the motive for investigate this issue.
As far as our knowledge is concerned, some studies related to manufacturing industry growth and the relationship between development indicators have been done in Ethiopia using fixed effect regression model, tobit regression model, multiple regression model and co-integration analyses 7 , 14 , 18 , 19 , 20 , 21 , 22 . In addition, there are many studies done in Indonesia, Sub-Saharan Africa, East Java Province, Europe, Nigeria, and Pakistan using panel data regression with the OLS model, generalized autoregressive conditional heteroscedasticity model, fixed effect regression model, co-integration tests, and a bounds testing approach to co-integration 6 , 10 , 13 , 23 , 24 , 25 , 26 , 27 . But most of them emphasize manufacturing growth and its determinants rather than examining its instability, and they do not see the relationships and trends of many indicators simultaneously. In addition, the aforementioned studies showed that manufacturing growth itself and the effects of manufacturing growth on industrial development are subject to regime shifts 23 .
Thus, this study addressed the volatilities and the relationships of industry development indicators using the Multivariate Generalized Autoregressive Conditional Heteroscedasticity (MGARCH) models.
Hypothesis tests
Hypothesis 1:.
The industry development indicators are stationary.
Hypothesis 2:
The multivariate GARCH model will be able to capture the volatility of the industry development indicators.
Hypothesis 3:
The tests for significance will be statistically significant.
Literature review
Theoretical literature review.
Industry is an economic activity that deals with the processing of raw materials, the manufacture of goods in factories, and the services that go along with their use. In its most restricted sense, the term "industry" may only refer to manufacturing- the making of goods, but in its broadest sense, it can refer to all phases and forms of economic activity, such as resource extraction, construction, and providing services 28 .
In order to come to a consensus on the link between manufacturing and industry growth, the industrial revolution brought about four significant transformations. Economic systems based on large-scale industry, automated manufacturing, and the factory system took the place of economies based on agriculture and handicrafts throughout the Industrial Revolution. Existing industries become more productive and efficient as a result of new equipment, power sources, and organizational techniques. The four industrial eras are coal, gas, electronics and nuclear, and the internet and renewable energy 29 . These revolutions were summarized in the following ways:
The first industrial revolution started in 1760 with the invention of the steam engine. The invention of the steam engine made it possible to go from farming and a feudal society to a modern manufacturing system. This transition included the use of coal as the main energy while trains were the main means of transportation. The two industries that dominated in terms of employment, output value, and capital invested were textile and steel 30 .
Since there is no clear distinction between the two revolutions from a technological and sociological standpoint, the second industrial revolution is sometimes known as the second phase of the industrial revolution. It was, in many ways, the continuation of the first. In many industries there was direct continuity. Yet it differed from it in a number of crucial aspects. First, it had a direct effect on real wages and standards of living which clearly differed significantly in 1914 from 1870. Second, it maintained the monopoly of the industrialised Western world on technological leadership while moving the geographic focus away from Britain to a more dispersed locus. Finally, it permanently altered how technological change occurs by altering the relationship between knowledge of nature and how it affected technological practices 31 .
The Third Industrial Revolution started in the 1950s, peaked in the dot.com period in the late 1990s, and is still continues at present. IR3 is anticipated to come to an end in the 2030s. The IR3 is seen as the transition from mechanical and analog electronics to digital electronics, which includes dispersed manufacturing, green buildings, and electric vehicles. It is based on the internet, digital technology, and the energy shift. The IR3 emerges from the corporate industry’s opportunity brought by nanotechnology, intelligent systems, 3D printing and robotics for industrial production and domestic services 32 . The third industrial revolution, often referred to as the digital revolution, which started in the late 1900s, is distinguished by the development of automation and digitalization through the use of electronics and computers, the invention of the internet and the discovery of nuclear energy. This era witnessed the rise of electronics like never before, from computers new technologies that allow the automation of industrial operations. Telecommunications development paved the groundwork for extensive globalization, which in turn enabled industries to offshore production to low-cost economies and radicalize business models worldwide 32 .
The design of computer-generated products and three-dimensional (3D) printing, which can produce solid objects by stacking successive layers of materials, are now two aspects of the fourth industrial revolution 33 . It is characterized by a fusion of technologies that is blurring the lines between the physical, digital, and biological spheres. There are three reasons why today’s transformations don't just reflect a continuation of the Third Industrial Revolution but rather the arrival of a Fourth and distinct one: velocity, scope, and systems impact. There is no historical parallel for the rapidity of modern advances. Comparing the Fourth Industrial Revolution to prior ones, it is growing exponentially rather than linearly. Almost every industry worldwide is also being disrupted by it. Additionally, the magnitude and scope of these changes denote a complete transformation of the production, management, and governance systems 34 .
Prisecaru 35 shows a short presentation of the industrial revolutions from 1760 to the present. The main characteristics of industrial revolution-I from the period 1760–1900 and the transition period 1860–1900, the energy resource was coal, main technical achievement was steam engine internal, main developed industries were textile, steel and metallurgy and the accessed transport was train, industrial revolution-II from the period 1900–1960 and transition period 1940–1960 were characterized by oil electricity energy source, combustion engine main technical achievement, auto, machine building main developed industries and train and car transport means were utilized.
From the period 1960–2000, industrial revolutions were introduced, and the transaction period between 1980–2000, which is characterized by nuclear energy and natural gas used as energy sources, computers, robots, internets, and 3D were the main technical achievements, and auto and chemistry were the main development industries with car and plane transport means. The fourth industrial revolution from the period 2000–present, characterized by green energy resources, printers, and genetic engineering as technical achievements, developed high-tech industries with electric cars, and ultra-fast trains were used as transport mechanisms 35 .
There are also theories that justified by drawing upon several key theoretical frameworks and concepts. Here are some theoretical justifications that can support the research objective outlined in the title:
Financial economics theory
Financial Economics Theory is a branch of economics that focuses on understanding how financial markets, institutions, and instruments operate and how they impact economic outcomes? It applies economic principles and theories to analyze financial decisions, asset pricing, risk management, and the allocation of resources in financial markets. One of the foundational theories in financial economics is the Efficient Market Hypothesis (EMH), which posits that financial markets are efficient in reflecting all available information, making it impossible to consistently outperform the market through active trading or investment strategies. This theory has important implications for understanding asset pricing and the behavior of financial markets 36 .
Risk management theory
Risk management theory is a field of study that focuses on understanding and managing risks in various contexts, including finance, business, industry, and economics. It involves identifying, assessing, and mitigating risks to achieve organizational objectives and protect against potential losses. One of the foundational theories in risk management is Value at Risk (VaR), which quantifies the maximum potential loss that a portfolio or investment may face over a specified time horizon at a given confidence level. VaR is widely used in financial risk management to measure and monitor market risk 37 .
Forecasting theory
Forecasting theory is a set of principles and methods used to make predictions about future events or trends based on historical data and other relevant information. It involves analyzing patterns and relationships in data to make informed projections about what may happen in the future 38 .
Empirical literature review
According to 10 studies using time series analysis and dynamics indicators, the world leader in terms of industrial production is China, accounting for 28.4% of world industrial production. The second and third places are occupied by the USA and India. The fourth place is occupied by Russia, so in 2021 industrial production increased by 2.3%. The highest values of the studied indicator can be traced in the following countries: Belarus, Germany, and Japan. This suggests that the manufacturing sector makes a significant contribution to the development of the country's gross domestic product. If the studied indicators have a positive development tendency and the industrial output itself appears to be promising, then the current development trend contributes to economic growth and the independence of the state.
Zhong 12 studies on linear analysis of the correlation between industrial development and foreign trade development in Guangdong Province show that the degree of synergy between the developments level of the secondary industry in Guangdong Province and the indicators of foreign trade is relatively high, indicating that foreign trade can significantly promote the development level of the secondary industry, which is consistent with the status of Guangdong Province as a manufacturing province. At the same time, relative to the secondary industry, the development of tertiary industry in Guangdong Province is sensitive to changes in foreign trade, which may be related to the rapid transition of Guangdong Province from the industrial economy stage to the service economy stage, making the coefficient of elasticity of tertiary industry and foreign trade higher. The number of workers employed in this industrial sector has grown yearly along with the expansion of industry in East Java Province. This, combined with the indirect growth of industry, can also influence the provision of employment and maximize employment, helping to combat the high unemployment rate and lessen poverty in the area. The development and performance of the industrial sector are very rapid; this is seen from the output produced from the industrial activity process, which is the result of the value of production. Components of production value are goods produced from the production process 23 .
Elahinia et al. 24 Conclude that there is a strong causal relationship between manufacturing growth and GDP growth in any nation and analyze the correlation between the manufacturing value added (MVA) and GDP for 92 countries in the period of 1950–1970, 1970–1990 and 1990–2005 using random effects, fixed effects and Hausman tests. According to these empirical results, the relationship between manufacturing output and economic growth is significantly positive.
According to 22 Study on the Determinants of Manufactured Products Export Performance in Ethiopia using fixed effect, tobit and probit models, the manufacturing industry in Ethiopia is at its lowest stage of development. However, it has registered a 9.7% annual growth rate between the periods 2000 and 2015 on average. This has indicated a significant improvement from the period between1990 and 2000 where the growth was nearly zero. The sector contributes about 5% of the GDP and remains stagnant over time and the export share of the manufacturing sector has remained stagnant at around 15% of total merchandise exports and even exhibited a declining trend despite the growth of the sector. This is one of the indicators of the poor performance of the manufacturing sector in the export market.
Different study has shown the MVA share's relationship to GDP. Various studies using panel data found that credit availability had a significant direct impact on Manufacturing value Added in Kenya, Africa, South Asia and Nigeria 39 , 40 and 11 .
Samouel 39 studied the determinants of industrialization in 35 African countries. Using time series data ranging from 1970 to 2012 and a dynamic panel model, their study revealed that human capital, labor market conditions, the real effective exchange rate, and GDP per capita were found to be the determinants of industrialization in Africa. Moreover, the study depicted that the determinants of industrialization vary between regions on the continent and evolve over time.
The study by 41 examines the determinants of manufacturing growth in Nigeria using dynamic ordinary least squares methods of econometric analysis. The result of the study indicates that the main determinants of manufacturing growth are foreign direct investment (FDI), the interest rate, the labor force, inflation, and the exchange rate. According to the study, factors affecting the determinant of manufacturing exports were analyzed using the vector error correction model (VECM), and the result indicates that inflation at lag1 and GDP at lag1 and lag2 negatively and positively affect manufacturing exports, respectively, in the short run.
Levinson 5 estimated the response of manufacturing capacity utilization in Nigeria to changes in key macroeconomic indicators using annual data over the period 1975–2012. Accordingly, a co-integration between the endogenous and exogenous variables was found and variations in manufacturing capacity utilization in Nigeria are largely driven by their own shocks. The study further shows that the exchange rate, interest rate and terms of trade contribute significantly but negatively to variations in manufacturing capacity utilization. The study also presents evidence of the causal impact of manufacturing capacity utilization on the exchange rate and manufacturing capacity utilization on the interest rate, not vice versa, by using the Granger causality test.
The study by 42 analyzed the drivers of successful industrialization in developing countries. By considering two different periods (1970–1990 and 1991–2014). The empirical analysis reveals that successful industrialization is driven by a combination of factors, including the country’s initial economic conditions, its factor endowments, and other characteristics such as demographic structure and geography. The study also shows that other variables such as the promotion of investments in capital and education; the management of trade and capital openness; financial sector development; and the promotion of both macroeconomic and institutional stability, which policymakers can control, play a crucial role.
Beyene and Singh 31 studies on the effectiveness of monetary policy on industrial growth in Ethiopia using an autoregressive distributed lag model, revealed that Ethiopia's industrial problems could be tamed by appropriate management of monetary policies. In doing so, the structural shift observed in Ethiopia and its impacts on economic transformation need a close and systematic look. Moreover, the government’s policies towards the exchange rate, interest rate and domestic inflation need a closer check-up, as the unintended results become a pitfall for Ethiopian industry. They conclude that the export share of firms has a positive effect on their growth rate. Again, it is plausible that this effect is of a permanent nature. Firms that are highly export-oriented should show continuously higher growth rates.
In the case of this study, annual (yearly) data for the period 1982–2021 were used in the analysis. Multivariate GARCH models are applied in modeling and investigating the volatility and relationship between industry development indicators in Ethiopia. MGARCH is a powerful method to discuss volatility issues in clustered and equally integrated returns series. A kind of general to specific estimation technique is utilized, see the theoretical frame work of time series analysis in Fig. 1 .
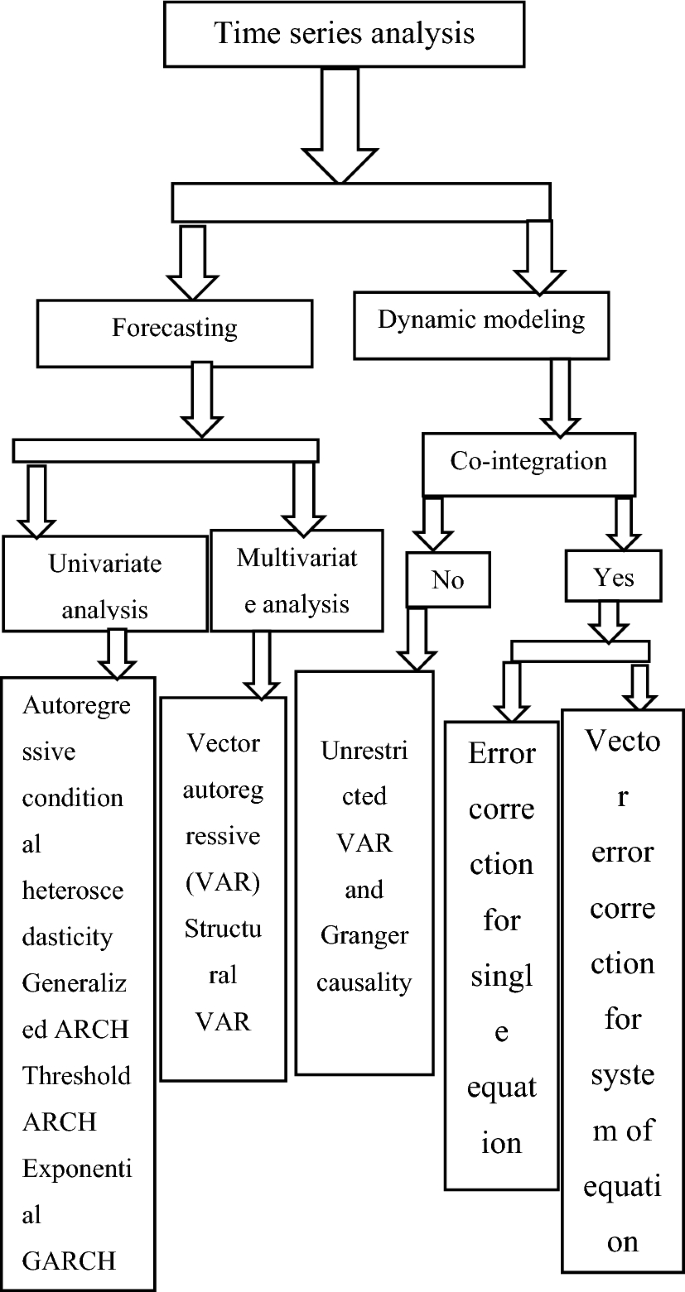
Conceptual frame work for time series analysis.
Methodology
Description of study area.
The study was conducted in Ethiopia. Ethiopia is a diverse cultural nation and the only country in the horn of Africa that has never been colonized. It is bordered by Sudan to the west, Eritrea to the north, Djibouti and Somalia to the east, and Kenya to the south. The population is concentrated in the northern and southern highlands, with the lowlands in the south-east, south, and west, for the most part, being far more sparsely inhabited.
Data source and description
The source of data for this study was secondary, and obtained from the World Bank via https://data.worldbank.org/indicator/ . World development indicators (WDI) offer metrics for social advancement, lifestyle improvement, economic growth, societal infrastructure development, environmental protection, and governmental performance. WDI is the principal collection of development indicators maintained by the World Bank, compiled from officially sanctioned worldwide sources.
The data existed yearly in the database of the Word Bank. To attain normality, time series analysis needs a large enough number of observations, since 40 observations are enough for multivariate time series modeling 43 . The data was analyzed by Eviews 12 and Ox Metrics 7 statistical software.
Variables in the study
Industry development (growth) is partially explained by its key indicators, which are manufacturing output (growth), manufacturing imports, manufacturing exports, employment, industry GDP, manufacturing GDP, industrial labor force, wages, and other development indicators. Based on the availability of data and the main target of the study, the variables that would be considered in this study are: industry including construction value added as a proxy of industry growth (INDG), manufacturing value added as a proxy of manufacturing growth (MANG), industry including construction value added (% GDP) as a proxy of industry GDP (INDGDP), manufacturing value added (% of GDP) as a proxy of manufacturing GDP (MANGDP) and medium and high technology (tech) exports as a proxy of manufacturing exports (MANEXP) would be taken as dependent variables; time and the lagged values of dependent variables on the side of independent variables, and also all variables are measured by percent.
Methods of data analysis
Time series analysis.
A time series is broadly defined as any series of measurements taken at different times. It can be divided into two major parts: univariate and multivariate time series. Univariate time series analysis uses only the past history of the time series being forecast plus current and past random error terms. Autoregressive integrated moving average (ARIMA) modeling is a specific subset of univariate modeling in which a time series is expressed in terms of past values of itself (the autoregressive component) plus current and lagged values of a "white noise” error term (the moving average component), and the seasonal autoregressive integrated moving average (SARIMA) model is used in non-stationary data 41 . On the other hand, multivariate time series analysis is used when one wants to model and explain the interactions and co-movements among a group of time series variables. This study was concerned with modeling multivariate time series.
Before the models are presented, some basic concepts are mentioned, such as time series plots, a test of stationarity, and transformation if needed.
Time series plot
The first step in the analysis of time series is usually to plot the data and obtain simple descriptive measures of the main property of the series via a visual inspection of the time series plot. This may reveal one or more of the following characteristics: seasonality, trends either in the mean level or the variance of the series, long-term cycles, and so on. If any such patterns are present, then these are signs of non-stationarity.
Test of stationarity
The foundation of time series analysis is stationarity. A time series \({Y}_{t}\) is said to be strictly stationary if and only if the joint distribution of \({y}_{t1},\cdots ,{y}_{tk}\) is identical to that of \({y}_{t1+t},\dots ,{y}_{tk+t}\) for all \(\text{t}\) , where \(\text{k}\) is an arbitrary positive integer and \({t}_{1},{t}_{2}\dots ,{t}_{k}\) is a collection of \(\text{k}\) positive integers. A weaker version of stationarity is often assumed. A time series \({Y}_{t}\) is weakly stationary if both the mean of \({Y}_{t}\) and the covariance between \({Y}_{t}\) and \({Y}_{t-k}\) are time-invariant. More specifically, \({Y}_{t}\) is weakly stationary if \(E\left({Y}_{t}\right)=\mu \) , which is constant \({\forall }_{t}\) , and \(\text{Cov}\left({Y}_{t},{Y}_{t-j}\right)={\Gamma }_{j},{\forall }_{t}\) and \(\text{j}=\text{0,1},2,.\) . In applications, weak stationarity enables one to make inferences concerning future observations 44 .
There are different stationarity tests; among them the following two were used for this study.
Augmented Dickey-Fuller (ADF) test
In econometrics, an ADF tests the null hypothesis that a unit root is present in a series 45 . The testing procedure for the ADF test is applied to the model.
where \(\mu \) is a constant, \(\beta \) is the coefficient on a time trend, \(\gamma =\left({\sum }_{i=1}^{p} {\phi }_{i}\right)-1,{\delta }_{i}=-{\sum }_{k=i+1}^{p} {\delta }_{k}\) \(,\Delta \) is the first difference operator, and \(\text{p}\) the lag order of the AR process. Assuming \(\mu =\beta =0\) , the ADF test is then carried out under \({H}_{0}:\gamma =0\) against \({H}_{1}:\gamma >0\) .
The test statistic is: \(DFT=\frac{\widehat{\gamma }}{\text{se}(\widehat{\gamma })}\)
Where DFT is the ADF test statistic at \(\text{t}\) degree of freedom, is compared with the relevant critical value. The ADF statistic is always negative for stationary series. The more negative it is, the stronger the rejections of null hypothesis that there is a unit root at some level of confidence.
Phillips Perron (PP) test
The PP test named after 46 is a unit root test. That is used in time series analysis to test the null hypothesis that a time series is integrated of order 1. It builds on the DFT of the null hypothesis \(\rho =1\) in \(\Delta {y}_{t}=(\rho -1){y}_{t-1}+{\epsilon }_{t}\) . Where \(\Delta \) is the first difference operator. Like the ADF test, the PP test addresses the issue that the process generating data for \({y}_{t}\) might have a higher order of auto-correlation than is admitted in the test equation making \({y}_{t}\) endogenous and thus invalidating the Dickey Fuller t-test. Then, PP test makes a non-parametric correction to the t-test statistic.
Modeling volatility
Volatility provides a measure of the possible variation or movement in particular industry development indicators from period t-1 to t. It indicates how much and how quickly a value changes over time. Volatility connects two principal concepts: variability and uncertainty, with the former describing overall movement and the latter referring to movement that is unpredictable. Wide movements over a short period of time typify the term “high volatility”. The lack of predictability and uncertainty associated with increased volatility may influence both producers and consumers to secure supplies and control input costs. Volatility is often measured as the sample standard deviation.
where \({R}_{t}\) is the return (i.e. natural logarithm transformed and differenced series) at time t and \(\mu \) is the estimated average return over period \(T\) . Since variance is the square of standard deviation, it makes no difference which ever measure S or \({S}^{2}\) to compare volatilities 47 . Most financial studies involved returns instead of original series. 48 Gives two main reasons for using returns. First, for average investors, the return of a series is a complete and scale-free summary of the investment opportunity. Second, return series are easier to handle than original series because the former have more attractive statistical properties. Let \({Y}_{t}\) be the series at time index \(\text{t}\) , which often displays unit-root behavior and thus cannot be modeled as stationary, we often analyze log-returns on \({Y}_{t}\) given as:
where \({R}_{t}\) is the \(\text{log}\) return series of \({y}_{t}\) , and \({y}_{t-1}\) is the series at time \(t-1\) . The returns for multivariate time series is \({R}_{t}=\left({R}_{1t},\cdots ,{R}_{kt}\right)\) be the log returns of \(\text{k}\) series at time \(t\) . The squared returns are used as a proxy for volatility.
If there is a large variation from period \(t-1\) to \(\text{t},{R}_{t}\) be large and we speak of large returns or large volatility. Hence, extreme values for returns reflect extreme variation (volatility). If there is no variation over time, \({R}_{t}=\text{log}\left({y}_{t}\right)-\text{log}\left({y}_{t-1}\right)=0\)
MGARCH models
GARCH models have featured prominently in the analysis of financial time series and are used to study the heteroscedasticity problem. Financial time-series such as foreign exchange rates, inflation rates, and stock prices may exhibit some volatility that varies over time, and this variation is an indicator of ARCH effects or heteroscedasticity problems. Among the numerous specifications of MGARCH models, the most popular seem to be the Vector Error Correction Heteroscedasticity (VECH ), the Baba, Engle, Kraft and Kroner (BEKK) of 49 , Constant Conditional Correlations (CCC) introduced by 50 , and Dynamic Conditional Correlations (DCC) proposed by 51 and 52 . VECH- and BEKK-GARCH models are the models of conditional covariance matrix, while CCC- and DCC-GARCH are the models of conditional variances and correlations 53 .
Before fitting any GARCH model, it is better to test for the ARCH effect. According to 48 , there are two available methods to test for ARCH effects. The first test is to apply the usual Ljung-Box statistics \({Q}_{(m)}\) to the \({\epsilon }_{t}^{2}\) series. The second test for conditional heteroscedasticity is the Lagrange multiplier test of Engle 54 . In the presence of an ARCH effect or time-varying variance in the residuals of a linear time series model, an ARCH or GARCH model is fitted to the squared residuals of the model in order to remove the heteroscedasticity from the residuals.
The dynamic time dependence (i.e., conditional heteroscedasticity) of \({\varepsilon }_{t}\) is the subject of multivariate volatility modeling. For a k-dimensional time series, \({Y}_{t}\) the volatility matrix \({\Sigma }_{t}\) consists of \(k\) conditional variances and \(\frac{k(k-1)}{2}\) conditional covariance. In other words, \({\Sigma }_{t}\) consists of \(\frac{k(k+1)}{2}\) different time-varying elements and the volatility matrix \({\Sigma }_{t}\) must be positive definite for all t.
The complexity of MGARCH models has been a major obstacle to their use in applied work. However, as the dimension of the cross section increases, the number of parameters can become very large in MGARCH models, making estimation increasingly cumbersome. This "dimensionality curse" is general in multivariate time series but is particularly problematic in GARCH models 52 . When the number of parameters becomes too large \((m>9)\) the computation time of the Maximum Likelihood Estimation (MLE) becomes prohibitive, and more importantly, the optimization fails to give a reasonable value.
VECH- and BEKK-GARCH models
The difficulty of multivariate volatility model is evident because of the number of parameters involved. For a k-dimensional process, the number of parameters in VECH \((\text{1,1})\) is \(\frac{k(k+1)}{2}+\) \(2{\left[\frac{k(k+1)}{2}\right]}^{2}\) and the number of parameters in \(\text{BEKK}(\text{1,1})\) model is \(\frac{k(k+1)}{2}+2[k{]}^{2}\) , which is much smaller than the number of parameters for VECH model. Other than the large number of parameters in the model, the other problem with the VECH model is that the conditional covariance matrix may not be positive definite. The BEKK ( \(p, q, k\) ) model is given as:
where \(C\) is a \(mxm\) triangular matrix; which is to ensure \({\Sigma }_{t}\) (i.e.Conditional variance covariance matrix) to be definitely positive and is a necessary condition for the estimated variances to be zero or positive, \({\Phi }_{k}\) is kxk matrix which shows the influence of past volatility on the current volatility (i.e. GARCH effect), \({\Theta }_{k}\) is a kxk matrix which shows the effect of past shocks irrespective of their sign (i.e. ARCH effect). \(\Phi \) and \(\Theta \) are symmetric. The sum, \(\sum {\Phi }_{k}+\sum {\Theta }_{k}\) measures the persistence of volatility. Any shock to volatility is permanent if \(\sum {\Phi }_{k}+\sum {\Theta }_{k}=1\) , that is; past volatility is significant in predicting future volatility. Volatility is explosive if \(\sum {\Phi }_{k}+\sum {\Theta }_{k}>1\) , meaning, a shock to volatility in one period will lead to even greater volatility in the next period. If \(\sum {\Phi }_{k}+\sum {\Theta }_{k}<1\) , volatility is neither permanent nor explosive and past volatility prediction is not as such important 55 . The \(\text{BEKK}(\text{1,1},\text{k})\) model is:
The model will be stationary if and only if the Eigen values of \({\Phi }_{k}\otimes {\Phi }_{k}+{\Theta }_{k}\otimes {\Theta }_{k}\) are in the unit circle, where \(\otimes \) is the kronecker product of two matrices. Partly because of numerical difficulties are so common in the estimation of BEKK models, it is typically assumed that \(p=q=1\) . In this study the BEKK \((\text{1,1})\) model, its explicit form is given by:
The interpretation of parameters in the VECH and BEKK models is not easy for the general case with a large p and q, even after imposing several restrictions (i.e., the associated matrices in the model such as \({\phi }_{k}\) and \({\theta }_{k}\) are taken to be diagonal or scalar). The VECH and BEKK models are only applied in low dimensions ( \(d\le 4\) ). In Diagonal BEKK-GARCH model; the conditional variances are functions of their own lagged values and lagged squared returns, the conditional covariance’s are functions of the lagged covariance and lagged cross-products of the corresponding returns. It reduces the number of parameters estimated by restricting the parameter matrices to be diagonal and addresses the difficulty with VECH by ensuring that the conditional covariance matrix is always positive definite. The BEKK model doesn’t impose the restriction of constant correlation among returns over time. The CCC and DCC-GARCH models are implemented in dimensions larger than 4 in the financial industry 50 .
Basic properties of MGARCH family model
Any MGARCH should be unique and stationary; a necessary and sufficient condition to have a unique and stationary solution is \({\sum }_{k=1}^{p} {\phi }_{k}+{\sum }_{k=1}^{q} {\theta }_{k}<1\) , Zero in mean: any model in which \({\sigma }_{t}\) is measurable, the mean of \({\varepsilon }_{t}\) is zero. i.e. \(E[{\varepsilon }_{t}]=0\) , Correlation-free: in GARCH, even though it is conditional, heteroscedasticity is inevitable. Hence, the auto-covariance of any pair of elements from the series is expected to be zero resulting in lack of serial autocorrelation. For \(\text{k}>0,{\epsilon }_{t}\) is not correlated with \({\epsilon }_{\text{t}+k}:E\left[{\varepsilon }_{t}{\varepsilon }_{\text{t}+\text{k}}]=0\right.\) , and Unconditional variance.
Order determination of the MGARCH family model
A model selection criterion considers the "best approximating model" from a set of competing models. An important practical problem is the determination of the GARCH order \(p\) and the ARCH order \(q\) for a particular series. The Akaike information criterion (AIC) proposed by Akaike (1994), Schwartz Bayesian information criterion (SBIC) proposed by Schwart (1978) and Hannan-Quinn information criterion (HOIC) were employed. The model satisfying minimum AIC or SBIC or HQIC is most representative of the true model. The formal expressions for the above criterion in terms of the log-likelihood are discussed.
where \(\hat{L}\) = the maximized value of the likelihood function of the model M, i.e. \(\hat{L} = {\text{P}}({\text{R}}/\hat{\theta },{\text{M}})\) , where \(\hat{\theta }\) are the parameter vlues that maximine the likelibood function; \(\text{R}=\) the oberved returns; \(T\) = the number of data points in \(R\) , the number of observations, or equivalently, the sample size; \(\text{P}\) = the number of parameters estimated by the model.
Parameter estimation of MGARCH models
To be able to predict the volatility of a series, one first has to fit the GARCH model to the time series in question. We can estimate the parameters of MGARCH models by maximum likelihood (ML), assuming that the errors come from a multivariate normal distribution or Student's t-distribution. Both the ML estimator and the quasi-maximum likelihood (QML) estimator, which drops the normality assumption, are assumed to be consistent and normally distributed in large samples. The QML parameter estimates are the same as the ML estimates. As suggested by 48 , 56 , the estimation of GARCH mode is usually carried out using the MLE method for the VECH and BEKK-GARCH models, while two step estimation method for the CCC and DCC-GARCH models. In MLE, the distributional assumption on residuals is the core point. Financial time series data possess volatility clustering and leptokurtosis characteristics, which lead to the use of different distributional assumptions for residuals such as Gauss and Student-t.
Suppose that \({R}_{\text{t}}(\text{for} t=1,\cdots ,T)\) is the vector of returns with conditional mean, conditional variance matrix and conditional distribution are respectively \({\mu }_{\text{t}}\left({\theta }_{0}\right),{H}_{t}\left({\theta }_{0}\right)\) , and \(p\left({R}_{t}\mid {\upzeta }_{0} ,{\Omega }_{t-1}\right)\) . Where \({\zeta }_{0}=\left({\theta }_{0}{\eta }_{0}\right)\) is r-dimensional parameter vector and \({\eta }_{0}\) is the vector that contains the parameters of the distribution of the innovations \({z}_{t}\) (there may be no such parameter). Importantly, to justify the choice of the estimation procedure, the model to be estimated encompasses the true formulations of \({\mu }_{t}\left({\theta }_{0}\right)\) and \({H}_{\text{t}}\left({\theta }_{0}\right)\) .
The most commonly employed distribution in the literature is the multivariate normal, uniquely determined by its first two moments (so that \(\zeta =\theta \) since \(\eta \) is empty). In this case, the sample log-likelihood is:
where, \(\text{T}\) is the number of observations, \(\text{k}\) is the number of returns, \(\theta \) is the vector of parameters to be estimated.
Forecasting volatility
Formally, forecasting volatility could be seen as finding such \({\widehat{\Sigma }}_{t}\) that will minimize the error.
\(\varepsilon =f({\Sigma }_{t}-{\widehat{\Sigma }}_{t})\) , \(\text{where}\) \({\Sigma }_{t}\) is an actual (or observed) volatility over period \(t\) and \(f(.)\) is an error function. To forecast volatility on a certain time frame one could use data of a smaller time frame and compute the standard deviation. For example, if we are interested in monthly volatility, we can compute the standard deviation of yearly returns. In order to test the forecasting performance of some methods or to compare several methods we should define error functions. The following are the commonly used error functions:
Root Mean Square Error (RMSE) = \(\sqrt{\frac{1}{N} \sum_{i=1}^{N}({\widehat{\Sigma }}_{t}-{\Sigma }_{t}{)}^{2}}\)
Mean Absolute Error (MAE) = \(\frac{1}{N} \sum_{i=1}^{N}({|\widehat{\Sigma }}_{t}-{\Sigma }_{t}|)\)
Mean Absolute percent Error (MAPE) = \(\frac{1}{N} \sum_{i=1}^{N}\frac{({|\widehat{\Sigma }}_{t}-{\Sigma }_{t}|)}{{\Sigma }_{t}}\)
Mean Error (ME) = \(\frac{1}{N} \sum_{i=1}^{N}({\widehat{\Sigma }}_{t}-{\Sigma }_{t})\)
Mean Square Error (MSE) = \(\frac{1}{N} \sum_{i=1}^{N}({\widehat{\Sigma }}_{t}-{\Sigma }_{t}{)}^{2}\)
Ethics approval and consent to participate
Permission to access the data was obtained from the measure World Bank https://data.worldbank.org/indicator/ via online request. The website and the data used were publicly available with no personal identifier. The proposal was submitted to the Mekdela Amba University ethics committee and they gave permission to conduct the research. All methods were carried out in accordance with relevant guidelines and regulations.
Results and discussion
Descriptive statistics.
The study is based on secondary data obtained from the World Bank during a 40-year period, collected annually from 1982 to 2021. The data were expressed in natural logarithms to achieve stationarity and make the data have a better goodness of fit for multivariate GARCH models in this study. The data was analyzed by Oxmetrics 7 and Eviews 12 software. In the empirical analysis, INDG, INDGDP, MANG, MANGDP, and MANEXP were used aggregately. Some descriptive statistics, including mean, maximum, minimum, standard deviation, skewness, kurtosis, coefficient of variation (CV), Jarque–Bera statistics, and the corresponding probability values (P-values) of the natural log-transformed and return series under study, are presented in Tables 1 and 2 . The return series is the log-transformed and differenced series, which is used as a proxy of volatility.
Table 1 show that the trend of the series indicated some volatile characteristics during the investigation period. This is highly evident from the fact that the series has a high standard deviation, which is 0.82, 0.35, 0.28, 0.19, and 0.17, respectively. The result showed that the natural log-transformed industry growth at an average rate of 3.30% from 1982 to 2021 in Ethiopia. Moreover, the natural log-transformed average annual manufacturing growth rate was 3.71%, with maximum and minimum values of 4.02 and 3.22, respectively. The mean of the natural log-transformed values for lnINDGDP, lnMANGDP, and lnMANEXP are 2.46, 1.55 and 2.74, respectively. Skewness is negative for lnINDG, lnMANG, lnMANGDP, and lnMANEXP (i.e., the distribution has a longer tail to the left) and positive for lnINDGDP (i.e., the distribution has a longer tail to the right). On the other hand, the kurtosis of series assures that lnINDG, lnINDGDP, and lnMANG are leptokurtic and lnMANGDP and lnMANEXP are platykurtic. The result of Jarque–Bera with corresponding probability shows that all natural log-transformed series except lnINDGDP are normally distributed. Since volatility modeling is applicable for the leptokurtic distribution, the series are returned once.
Table 2 reveals that the return series INDG, INDGDP, MANGDP, MANG, and MANEXP were approximately growing at an average of − 0.002, 0.023, 0.002, − 0.001, and 0.049, respectively, while the standard deviations are almost equal except for MANEXP. Summary statistics show a longer tail to left INDG, MANGDP, and MANG returns and a longer tail to right INDGDP and MANEXP returns, as well as excess kurtosis in all return series except INDGDP, meaning they are highly leptokurtic and INDGDP is platykurtic. The Jarque–Bera test also assures a significant departure from normality due to the excess skewness and kurtosis found in the two returns (INDG and MANG). This implies that the two return series are heteroscedastic.
In most practices, time plots of certain data are very helpful to detect and extract useful insight and pre-information about the data. Thus, inspection should be made on the time plots of the natural log-transformed series. Figure 2 provides the individual plots against the time period covered by the study.
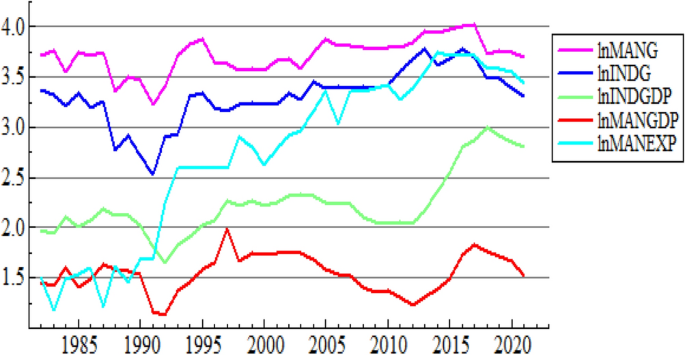
Time plot of the natural Log transformed series.
Figure 2 depicts that all natural log-transformed series have some downward trends after 2018, and they showed upward linear trends from 1991 to 2018, but lnMANEXP was stable from 1992 to 1998 and lnINDG had some downward trends from 1982 to 1991. To know which one is increasing more, it is better to see Table 1 , the CV row. From the table, the variability of natural log-transformed INDG, INDGDP, MANG, MANGDP, and MANEXP are 8.5%, 14.2%, 4.6%, 12.3% and 29.9%, respectively. Natural log-transformed INDGDP and MANGDP vary almost equally, and MANEXP has the greatest variability above all. Therefore, the variability of INDG (8.5%) is significantly greater than the variability of MANG (4.6%). So industry growth volatility is more than manufacturing growth uncertainty as shown by CV. This might be a motive to see the sort of relationship between major industry development indicators.
From Fig. 2 , it can also be seen that there is no clear seasonality. This implies that all the data are non-seasonal and non-stationary. But it should be strongly noticed that only graph inspections are not enough to conclude that the series are non-stationary. Therefore, standard tests for stationarity, which have been discussed previously in methodology, are applied in the analysis as follows:
Unit root properties of individual series
In practice, using non-stationary time series is problematic with regard to statistical inference and before making any statistical inference, the stationarity of the series was tested by using ADF and PP tests. The hypothesis to be tested as: H 0 the series is non-stationary against H 1 the series is stationary. The results of tests with an intercept at the level and after the first difference are presented in Table 3 . The critical values used for the tests are the MacKinnon (1996) critical values.
The test results presented in Table 3 indicate that the null hypothesis (H O : the series is unit root) could not be rejected for all series at this level. That is, the respective test statistics are less than critical values at the 5% significance level. In order to determine the order of integration of the non-stationary time series, the same tests were applied to their first differences. Thus, the null hypothesis that the series at first difference contains a unit root could be rejected for all series at the 5% significance level. That is, the absolute value of the respective test statistic is greater than the critical value at the 5% significance level. Therefore, the order of integration is the number of unit roots that are contained in the series so as to be stationary. This implies that all series are integrated in the same order one (I(1)).
To build a volatility model for the return series, the first step is to specify the mean equation based on the information criterion. Once we specify the mean equation, we have to test for ARCH effects using the residuals of the mean equation. If ARCH effects are statistically significant, specifying a volatility model and carrying out a joint estimation of the mean and volatility equations is necessary. The conditional mean specification is, in general, arbitrary for GARCH models of conditional volatility. Various modifications to the conditional means in GARCH models are possible (see, for example, 57 ).
Checking and testing for the presence of the ARCH effect
The squared returns are used as a proxy for volatility and are plotted in Fig. 3 . The y-axis limits give an opportunity to compare the volatility of the different series. It seems as though the returns on MANEXP, INDG, MANGDP, MANG and INDGDP are the first, second, third, fourth, and fifth most volatile, respectively. Moreover, the INDGDP seems to be the least volatile. However, the graphical analysis does not give an indication of how volatility reacts to positive and negative news. The first crucial step in the volatility modeling procedure is to assess the presence of ARCH/GARCH effects, which is usually carried out by the Lagrange multiplier (LM) test.

Volatility of industry development indicators.
ARCH effect test
Based on the residuals from the mean equation, it is possible to test for the existence of the ARCH effect, which will allow continuing the analysis using the GARCH model. In order to estimate the optimal parameters of different GARCH models, it is necessary to set up the presence of ARCH effects. This is done by testing the hypothesis H O : there is no ARCH effect (i.e., homoscedasticity) in the residuals Vs H a : there is ARCH effect in the residuals using ARCH LM test, and the result is shown in Table 4 . The LM-statistic showed the presence of ARCH effects at the 1% and 5% significance levels. Hence, there is volatility clustering, and it is possible to estimate the optimal parameters of MGARCH family models, excluding MANG (i.e., there is no ARCH effect in the return series MANG).
In the presence of the ARCH effect or time-varying variance in the residuals of a model, an ARCH or GARCH model is fitted to the squared residuals of the model in order to remove the heteroskedasticity from the residuals. Before going to estimate any of the MGARCH models, it is better to determine the best model by using conditional correlation tests and information criteria. But the conditional correlation models (i.e., CCC and DCC-GARCH) did not reach convergence, so these models are not appropriate for this data, and in this case, the researchers fitted only the conditional variance–covariance GARCH model for this study.
Lag length selection
If an ARCH effect is found to be significant, the ACFs and PACFs are helpful to determine the order of an ARCH model. This is due to the expectation that is linearly related to it in a manner similar to that of the AR model. In practice, for ARCH modeling a long lag is often needed and this requires estimating a large number of parameters. To reduce the computational burden, a GARCH model with low lags can be helpful. This results in a more parsimonious representation of the conditional variance process. To estimate and evaluate the forecasts of the competing GARCH models, different p and q values for the standard symmetric GARCH models are tested using different statistics such as Akaike Information Criterion (AIC), Bayesian Information Criterion (BIC), and Hannan-Quinn Information Criterion (HQIC) in order to choose the best model based on the in-sample data. Before fitting any MGARCH model, the optimal lag should be determined because too many lags result in loss of degree of freedom, insignificant coefficients, and multi-collinearity, and too few lags result in specification errors (i.e., a poorly specified model). The result is presented in Table 5 . The appropriate specification is chosen, and then for these specific p and q, the alternative GARCH models are estimated and tested, and finally one GARCH model is chosen based on forecasting performance.
The results of the information criteria in Table 5 showed that both diagonal VECH (1, 1) and BEKK (1, 1) GARCH models were adopted. But since the values of the information criterion are comparable and small for the diagonal BEKK (DBEKK)-GARCH model, so DBEKK-GARCH (1, 1) is the best multivariate generalized autoregressive conditional heteroscedasticity model adopted for this data. This outcome is a desirable since a smaller number of parameters to estimate remarkably simplify the computational procedure.
Estimation of diagonal BEKK-GARCH model
Once the optimal lag length and the appropriate model are selected for the data, the next step is estimating the parameters and obtaining the estimates of each unknown coefficient to interpret the result. This section assesses the ARCH and GARCH effects of each variable’s previous value. ARCH effects measure the impact of previous information on the volatility of the development indicator returns and GARCH effects show the persistence of the return volatility. As shown in Table 6 , the sum of ϕ and θ is close to one, which means that the returns are under the influence of shocks. The larger the ARCH coefficient θ is, the greater the impact of the previous shocks. For the GARCH coefficient ϕ, it shows the characteristics of each series itself. Overall, ARCH coefficients were much smaller than GARCH coefficients in this study.
The multivariate diagonal BEKK-GARCH (1, 1) parameter estimations were summarized in Table 6 . The estimated model shows that Φ 11, Φ 22, Φ33 , Θ 11, Θ 22, and Θ 44 coefficients are significant. The own volatility effect of return series annual industry growth (Θ 11 = 0.783) is the largest compared with other industry development indicators and the own volatility effect of manufacturing exports (Θ 44 = 0.414) is the second largest compared with other industry development indicators. And also, the lagged own-volatility effect for industry growth (0.622) and industry GDP (0.598) are the first and second largest compared with others, respectively.
Conditional variance–covariance equations effectively capture the volatility and cross-volatility among the four returns (INDG, INDGDP, MANGDP, and MANEXP) of industry development indicators because most coefficients are statistically significant (Table 6 ). Specifically, conditional variances and covariance implied by the diagonal BEKK-GARCH (1, 1) specifications are presented in the equations below.
The equations above are used as a representation of the diagonal BEKK-GARCH model constructed for the period 1982–2021 using four industry development indicators. The model takes into account both its own specific volatility and cross-volatility impacts. The log likelihood for this diagonal BEKK-GARCH model is 113.64; the higher log likelihood for multivariate GARCH models makes them suitable for presenting the interaction among the return volatility. First, we compare the equations of own volatilities. The constant of conditional variance \({\Sigma }_{44,\text{t}}\) (0.03) is larger than any other conditional variance, which suggests greater volatility in manufacturing exports.
From these empirical results, we conclude that there is strong evidence for a higher GARCH effect and the presence of a weaker ARCH effect. Equations show a statistically significant co-variation in shocks, which depends more on their lags than on past errors. Consequently, development indicator shocks are influenced by past information.
Own-volatility effects (ARCH effects) are positive and significant for three return development indicators (INDG, INDGDP, and MANEXP). The effect is higher for INDG (0.613) and MANEXP (0.172) than for INDGDP (0.077) and MANGDP (0.049). These coefficients show the volatility persistence for each indicator in terms of its own past errors.
As for cross-volatility effects, the strongest ARCH effect (0.324) is detected between INDG and MANEXP, which shows that previous information from manufacturing exports affects the growth of industry. The ARCH effect (0.218) is the second strongest, which is between industry growth and industry GDP, and the weakest ARCH effect (0.062) is detected between industry GDP and manufacturing GDP. The cross-volatility effects are higher than the own-volatility effects in INDGDP, MANGDP, and MANEXP. However, past volatility shocks in INDG have less effect on cross-volatility than its own volatility shock. These results suggest that MANGDP is the least vulnerable indicator to outside shocks.
The lagged own-volatility persistence effects (GARCH effects) are INDG (0.387), INDGDP (0.358), MANGDP (0.340), and MANEXP (0.013). These results suggest that INDG derives more of their volatility and persistence from within themselves. Moreover, the own volatility effects for four indicators do not remain within a tight range. This further implies that each indicator of growth faces a different risk-return profile and different levels of vulnerability to outside conditions.
For the return industry growth (INDG), the lagged cross-volatility persistence goes from 0.372 (INDGDP) to -0.070 (MANEXP), and in INDGDP, it goes from 0.372 (INDG) to -0.067 (MANEXP). Conversely, in MANGDP, the cross-volatility persistence varies between 0.363 (INDG) and -0.065 (MANEXP), while in MANEXP it goes from -0.070 (INDG) to 0.065 (MANGDP). Hence, in terms of cross-volatility persistence, the least influential indicator in the study is MANEXP, while the most influential would appear to be INDGDP. On the other hand, past volatility shocks in INDGDP have the greatest effect on the future volatility of INDG.
It is an important finding here that although cross-volatility persistence is heterogeneous for four indicators. The influence of lagged covariance on future covariance is positive for all pairs but negative for MANEXP pairs. The analysis implied that the magnitude of the cross-volatility persistence of the development indicators.
The plots for the conditional variance covariance estimated by the diagonal BEKK model are illustrated below. They suggest that the co-movements of the industry development indicators in Ethiopia display an extremely volatile trend for the study period.
The conditional variance, covariance, and correlation of four industry development indicators are shown in Figs. 4 and 5 . The plot indicates that there is a positive association between INDGDP and MANGDP, but all the other relationships between the return series vary between positive and negative values. Taking manufacturing GDP and manufacturing exports into account, as the share of manufacturing GDP increases, the general level of industry GDP also increases. The positive relationship between industry GDP and manufacturing exports means that an increase in the share of manufacturing exports leads to an increase in industry gross domestic product in a modernized system, which affects the health of one’s country's economy in a positive way, especially in Ethiopia, which depends heavily on import goods.

Conditional Variance and Covariance’s plot for Industry development indicators.
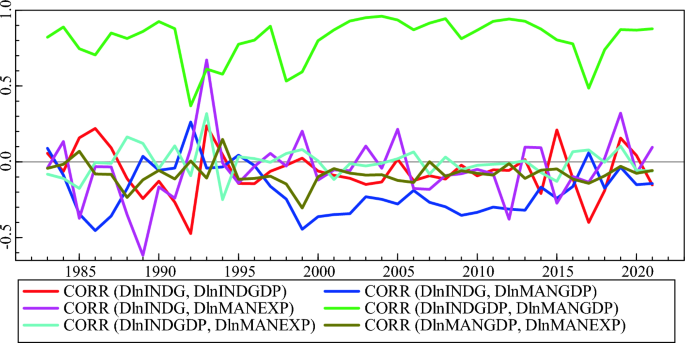
Conditional correlation plot for Industry development indicators.
Diagonal BEKK-GARCH model adequacy checking
Checking the adequacy of MGARCH models is essential in identifying whether a well-specified MGARCH model can obtain reliable estimates and inferences. Starting by looking at the residuals, they should be following a white noise process. The ACF plot for the squared standardized residuals, given in Fig. A.1 (Appendix), shows that the residuals are not auto-correlated for all return series. By looking at the ACF graphs, it can be determined whether the processed data has any trace of correlation in the residuals. If more than 5% of the lags in the autocorrelation plot fall outside the confidence interval, the residuals cannot be treated as white noise. In this model, almost all lags out of 20 fall within the confidence level. It can therefore be concluded that the residuals do not suffer from heteroscedasticity. The portmanteau test of white noise in Table 7 confirms the graphical view. After a diagonal BEKK-GARCH model had been fitted, the Ljung Box statistics (Q-test) and Li Mcloed (Q*-test) were employed for residuals to check for the model adequacy in Table 7 to test the null hypothesis of no serial correlation. The overall result showed that the model performed well statistically since p-values were insignificant. It means that the null hypothesis, which says there is no serial correlation in the squared residual, was accepted.
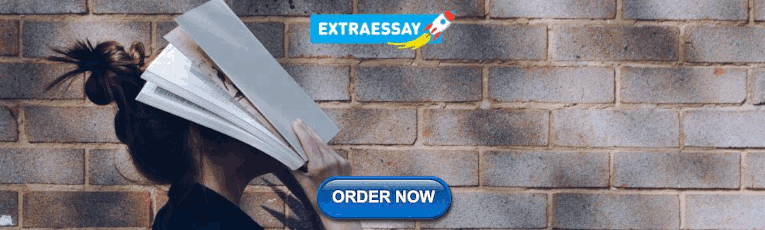
The ARCH LM test of residuals
The results from the ARCH LM test of the return series in Table 4 indicate the presence of volatility clustering in the data. The next model diagnostic is to determine whether there are still ARCH effects left in the residuals or not. If there are still unexplained effects left, this means that the chosen model suits the sample poorly since the return series suffers from heteroscedasticity according to the first ARCH LM test. Table A.1 in the appendix, Lagrange multiplier test approach, shows insignificant p-values for all standardized residuals. The conclusion of the LM tests is that a model captures volatility clustering successfully.
Normality of standardized residuals
GARCH estimates could be sensitive to the assumption of normally distributed errors. So it is important to check the extent to which this assumption is correct. Since the standardized errors are not directly observable and have to be estimated, chi-squared based tests such as the skewness and kurtosis tests for normality are not strictly applicable. Instead, it is advisable to plot the quantiles of the standardized residuals against the quantiles of the standardized normal distribution (Q-Q plot) and check if they are similar 58 .
Figure 6 shows that in all return series, the points form a roughly straight line, with approximately half the data below 0 and the other above 0. Therefore, standardized errors are approximately normally distributed, except for deviations at the beginning and end of the tails. The two outlier points, as shown in the residual Q-Q plots of the return series of manufacturing GDP and manufacturing exports do not have an impact on the model because the predicted response, estimated coefficient, and hypothesis test result are not affected by the inclusion of the point. Therefore, the data point is neither influential nor an outlier, but it does have leverage. This is confirmed in Fig. A.2 (Appendix), a histogram of the standardized residuals from the diagonal BEKK-GARCH (1, 1) model.

Normality (Q-Q) plot of standardized residuals, diagonal BEKK-GARCH model.
Forecasting from the DBEKK-GARCH model
Out of samples, volatility forecasting.
Forecasting is a deep-seated ambition of time series analysis or developing a time series model. The previous discussions validated that the diagonal BEKK-GARCH (1, 1) model is the paramount model to suitably describe the volatility of the return series of this study. This section conducts an examination of the forecasting accuracy of the fitted model and then makes a forecast for 2022–2026. A five year forecast is made for conditional variances, and the results are plotted in Fig. 6 .
Figure 7 exhibits the time series forecast of volatility in INDG, INDGDP, MANGDP, and MANEXP for the next five years. The forecast indicates that there is likely to be stability in all variables for the next five years. This implies that the returned series are expected to increase at a low rate, but the return series INDG is increasing very fast. Therefore, analyzing and forecasting volatility is helpful as it informs investors of the measures of risk involved in holding an asset because investors are not only interested in the average returns.
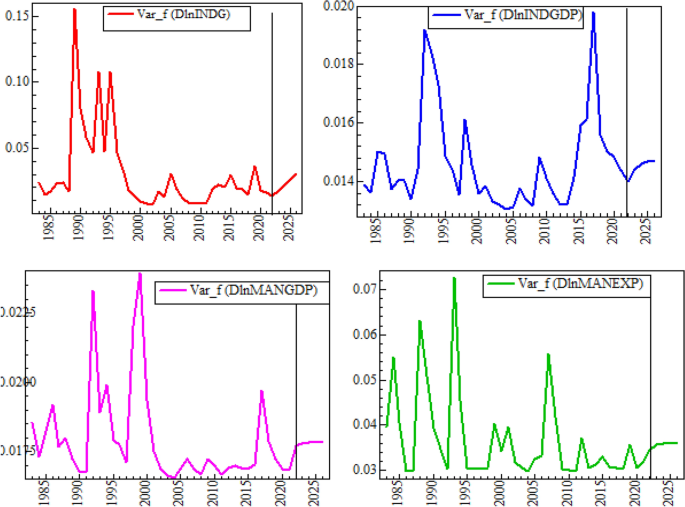
In (1982 to 2021) and Out (2022 to 2026) of Samples Forecasted Volatility.
Conclusions
The general objective of the study was to assess the volatility and relationship between industry development indicators in Ethiopia using a multivariate GARCH model based on World Bank data from 1982 to 2021, and the data were transformed to natural logarithms (by adding a constant for the variables that have a negative value) for easy analysis. However, without understanding the direction of relationships between industry development indicators, it is not possible to draw important lessons for policy-making purposes in an effort to pursue more effective policies that promote industry growth. Initially, all components were identified as non-stationary using Augmented Dickey-Fuller (ADF) and Phillips-Perron (PP) unit root tests. Thus, the result showed that all the series were, firstly non-seasonal, some are upward trended, volatile and non-stationary. The problems of non-stationarity were solved by differencing all the series once.
The ARCH Lagrange Multiplier test showed evidence of volatility clustering, and therefore the DBEKK-GARCH model was estimated excluding annual percent of manufacturing growth because there is no ARCH effect in manufacturing growth.
Among the four multivariate GARCH models, DBEKK-GARCH (1, 1) is found to be the appropriate volatility model, which is adopted by using different tests and information criteria.
The model takes into account both its own specific volatility and cross-volatility impacts. And manufacturing GDP is positively correlated with industry GDP and manufactured exports, and industry growth is positively correlated with manufactured exports.
Since ARCH coefficients are lower than GARCH term coefficients, there is evidence of a high rate of change in conditional volatility and significant time dependence.
Industry growth is the largest volatility effect in both cases (i.e., ARCH effect and the lagged own volatility (GARCH effect)) compared with others, as indicated by the diagonal BEKK-GARCH (1, 1) model.
Finally, the forecast showed an increment in industry growth, industry GDP, and manufacturing exports, while stability was showed in the volatility of manufacturing GDP.
Recommendations
As a result of these findings, policy-makers should prioritize the industry sector's development by enacting the following incentive conditions: The government and investors must work together to change and stabilize the volatile manufacturing exports and industry growth by stabilizing recurring policy changes and other unstable conditions. Policy makers should focus as much on industry and manufacturing growth as possible and there must be a national consensus to reduce imports of manufacturing goods into the country by improving production and increasing manufacturing exports.
We recommend and encourage future researchers studying the forecasting performance of MGARCH models to pay particular attention to the measurement of realized volatility and employ high-frequency data whenever feasible.
Limitation of the study
This study is limited to some extent due to inexplicitly recorded data and small number of observations on some variables like manufacturing imports, employment, wages, industrial labor force, skills, and education.
Significance of the study
Fitting and investigating the relationship and volatilities between industry development indicators are necessary for several reasons. First, to overcome the debates existing in their relationship and the uncertainty about future growth, which causes unexpected gains and losses in trade and industry, thus discouraging long-term contracts and investment, and to serve as a mirror in showing the trends and the major forces causing manufacturing industry growth, which helps policy-makers with proper ways of intervention for working the growth of the manufacturing industry and the whole industry growth.
Data availability
The datasets analyzed during the current study are available in the World Bank repository, https://data.worldbank.org/indicator/ .
Zheng, Y. et al . Development and industrialization in Ethiopia: Reflections from China’s experience. Fudan SIRPA Think Tank Report Series 1–31 (2017).
David Gorton, J. M. & Y. P. Industry Definition in Business and Investing 1–8 (2022).
Kniivilä, M. Industrial development and economic growth : Implications for poverty reduction and income inequality. Ind. Dev 1956 , 295–332 (2004).
Google Scholar
EDRI. Handbook of Industrial Development in Ethiopia policies, performance and Literature (Issue March). (2016).
Levinson, M. What is manufacturing ? Why does the definition matter ?. Congr. Res. Serv. 7 , 1–14 (2017).
Austin, G., Frankema, E. & Jerven, M. Patterns of manufacturing growth in Sub-Saharan Africa: From colonization to the present. Spread Mod. Ind. Periphery 1871 , 345–375 (2017).
Wakjira, M. W., Altenbach, H. & Ramulu, P. J. Optimization of manufacturing sustainability in the Ethiopian industries. Procedia Manuf. 21 (2017), 890–897. https://doi.org/10.1016/j.promfg.2018.02.197 (2018).
Article Google Scholar
Herman, E. The importance of the manufacturing sector in the romanian economy. Procedia Technol. 22 (February), 976–983. https://doi.org/10.1016/j.protcy.2016.01.121 (2016).
Agegnew, A. & Gujral, T. Determinants of profitability: Evidence from selected manufacturing company in Hawassa, Ethiopia. J. Posit. School Psychol. 6 (6), 8309–8322 (2022).
Klevtsova, M., Vertakova, Y. & Polozhentseva, Y. Analysis of the development of the industrial sector in the context of global transformation of economic processes. SHS Web Conf. 92 , 07031. https://doi.org/10.1051/shsconf/20219207031 (2021).
Maroof, Z., Hussain, S., Jawad, M. & Naz, M. Determinants of industrial development: A panel analysis of South Asian economies Determinants of industrial development: A panel analysis of South Asian economies. Qual. Quant. https://doi.org/10.1007/s11135-018-0820-8 (2019).
Zhong, J. Linear analysis of the correlation between industrial development and foreign trade development in Guangdong province-based on time series data from 2005 to 2018. IOP Conf. Ser. 474 (5), 052064. https://doi.org/10.1088/1755-1315/474/5/052064 (2020).
Hamzah, L. M. Efficiency and productivity of textile industry sub-sector with total factor productivity approaches. Int. J. Econ. Stat. 9 , 41–46. https://doi.org/10.46300/9103.2021.9.7 (2021).
Girma, A. Determinants of Export Performance and Employmnet of Labor in Large and Medium Scale Manufacturing Industry of Ethiopia an Ardl Cointegration Approach (2015).
Altaee, H. Trade openness and economic growth in the GCC countries: A panel data analysis approach. Int. J. Bus. Econ. Sci. Appl. Res. 11 (3), 57–64. https://doi.org/10.25103/ijbesar.113.05 (2018).
Gebeyehu, W. What is New in Ethiopia ’ s New Industrial Policy : Policy Direction and Sectoral Priorities 37 (2022)
Jagemma, M. & Worku, H. The effectiveness of industrial development strategy in Ethiopia. J. Contemp. Issues Bus. Govt. 27 (2), 5280–5298. https://doi.org/10.47750/cibg.2021.27.02.537 (2021).
Alemnew MW. (2022). Manufacturing Value-Added (MVA) Share Development in Ethiopia: Causes and Determinants. China-USA Business Review , 21 (2), 70–88. https://doi.org/10.17265/1537-1514/2022.02.005
Mishra, M. K. Determinants of manufacturing sector growth in ethiopia. Amity J. Manag. VI (2), 1–12 (2018).
Rao, K. R. M. & Tesfahunegn, S. Z. Performance measurement of manufacturing industries in Ethiopia: An analytical study. Economics 7 , 42–54 (2015).
Worku, D. School of Graduate Studies Trade Libralization and Industrial Growth in Ethiopia: A Cointegration Analysis (2008).
Zeleke, Y. T. A Study on the Determinants of Manufactured Products Export Performance in Ethiopia: Evidence from Firm Level Data 1–48 (2020).
Anggriawan, R., Maskie, G. & Syafitri, W. Analysis of the performance of the manufacturing industry sector in east Java Province and implications for strategy preparation. Int. J. Sci. Technol. Res. 8 (2), 72–77 (2019).
Elahinia, N., Karami, S. & Karami, M. The effect of manufacturing value added on economic growth: empirical evidence from Europe. Pressacademia 8 (2), 133–147. https://doi.org/10.17261/pressacademia.2019.1044 (2019).
Muhammad, I., Hafiz, K. A., Muhammad, A. & Tahir, M. Determinants of manufacturing value added in Pakistan: An application value added in Pakistan: An application of bounds testing approach to cointegration. Pak. Econ. Soc. Rev. 48 (2), 209–223 (2010).
MathSciNet Google Scholar
Okoye, L. & Clement, N. The influence of finance and macroeconomic variables on manufacturing capacity utilization in Nigeria ?. Niger. Account. Assoc. 6 (1), 176–188 (2015).
Saptioratri Budiono, H. D., Nurcahyo, R. & Habiburrahman, M. Relationship between manufacturing complexity, strategy, and performance of manufacturing industries in Indonesia. Heliyon 7 (6), e07225. https://doi.org/10.1016/j.heliyon.2021.e07225 (2021).
Article PubMed PubMed Central Google Scholar
Aliyu, U. Location of industry. Intellect. Arch. 7 (6), 23–27. https://doi.org/10.32370/2018_11_3 (2018).
Teker, S. & Koc, T. C. Industrial revolutions and its effects on quality of life. Pressacademia 9 (9), 304–311. https://doi.org/10.17261/pressacademia.2019.1109 (2019).
Clark, G. & Jacks, D. Coal and the Industrial Revolution, 1700–1869. Eur. Rev. Econ. Hist. 11 (1), 39–72 (2007).
Beyene, T. & Singh, I. Effectiveness of monetary policy on industrial growth of Ethiopia: ARDL modeling on monetary framework. IOSR J. Econ. Financ. 10 (3), 1–13. https://doi.org/10.9790/5933-1003010113 (2019).
Magal, P. Timeline of Revolutions. Manufacturing Data Summit Events Newsroom 1–8. https://manufacturingdata.io/newsroom/timeline-of-revolutions/ (2019).
Xu, M., David, J. M. & Kim, S. H. The Fourth Industrial Revolution : Opportunities and challenges. Int. J. Financ. Res. 9 (2), 90–95 (2018).
Schwab, K. The Fourth Industrial Revolution: what it means and how to respond. World Economic Forum 1–7 (2016).
Prisecaru, P. Challenges of the fourth industrial revolution. Knowl. Horizons 8 (1), 57–62 (2016).
Armstrong, S. Principles of Forecasting: A Handbook for Researchers and Practitioners (Springer, 2001).
Book Google Scholar
Fama, E. F. Efficient capital markets: A review of theory and empirical work. J. Financ. 25 (2), 383–417 (1970).
Jorion, P. Value at Risk: The New Benchmark for Managing Financial Risk (McGraw-Hill Education, 2006).
Samouel, B. The determinants of industrialization : Empirical Evidence For Africa. Eur. Sci. J 7881 (April), 219–239 (2016).
Were, M., Nzomoi, J. & Rutto, N. Assessing the impact of private sector credit on economic performance: Evidence from sectoral panel data for Kenya. Kenya Inst. Public Policy Res. Anal. 4 (3), 182–190 (2012).
Pierce, B. On a measure of lack of fit in time series models. Biometrika 65 (2), 297–303 (1970).
Haraguchi, N., Martorano, B. & Sanfilippo, M. What factors drive successful industrialization ? Evidence and implications for developing countries. Struct. Change Econ. Dyn. 49 , 266–276. https://doi.org/10.1016/j.strueco.2018.11.002 (2019).
Liu, X., Swift, S. & Tucker, A. Modelling multivariate time series. Time Ser. Econom. https://doi.org/10.1057/9781137525338.0010 (2015).
Thomas, S. Multivariate time series analysis of Ethiopian International Air Travel Demand. Unpublished Master’s Thesis, Addis Ababa University 23–34 (2014).
Dickey, D. & Fuller, W. Distribution of the estimation for autoregressive time series with a unit root. J. Am. Stat. Assoc. 74 , 427–431 (1979).
Phillips, P. & Perron, P. Testing for a unit root in times series regression. Biometrika 75 , 335–346. https://doi.org/10.11648/j.ijema.20200804.12 (1988).
Article MathSciNet Google Scholar
Godana, A. A., Ashebir, Y. A. & Yirtaw, T. G. Statistical analysis of domestic price volatility of sugar in Ethiopia. Am. J. Theor. Appl. Stat. 3 (6), 177–183. https://doi.org/10.11648/j.ajtas.20140306.12 (2014).
Ruey, S. T. Analysis of Financial Time Series Second Edition . (2005).
Engle, R. F. Multivariate Simultaneous Generalized Arch Published by : Cambridge University Press Stable. https://www.jstor.org/stable/3532933 . Cambridge University Press is collaborating with JSTOR to digitize, preserve and extend access Multivariate Simultaneou 11 (1), 122–150 (1995).
Bollerslev, T. & Wooldridge, J. M. Quasi-Maximum Likelihood Estimation and Inference in Dynamic Models with Time-Varying Covariances May 2013 37–41 (1992).
Brownlees, C. & Llorens, J. Projected Dynamic Conditional Correlations 1–32 (2020).
Engle, R. Dynamic conditional correlation: A simple class of multivariate generalized autoregressive conditional heteroskedasticity models. J. Bus. Econ. Stat. 20 (3), 339–350. https://doi.org/10.1198/073500102288618487 (2002).
Joseph, N. Modeling time-varying variance-covariance for exchange rate using multivariate GARCH model. Int. J. Thesis Projects Dissert. 4 (2), 49–65 (2016).
Engle, R. F. Autoregressive conditional heteroscedasticity with estimates of the variance of United Kingdom inflation. Econometrica 50 (4), 987–1007 (1982).
Sinha, D. Effects of Volatility of Exports in the Philippines and Thailand 2563 (2007).
Bollerslev, T. Generalized autoregressive conditional heteroskedasticity. J. Econom. 31 , 307–327 (1986).
Asai, M. & Mcaleer, M. Asymptotic Theory for Extended Asymmetric Multivariate GARCH Processes 2341–2356 (2016).
Wang, Y., Steele, T. & Zhang, E. QQ PLOT 1–13 (2016).
Download references
Acknowledgements
The authors are thank full to World Bank for data availability.
Author information
Authors and affiliations.
Department of Statistics, Mekdela Amba University, Tulu Awulia, Ethiopia
Getachew Abate Dagnew
Department of Statistics, Bahir Dar University, Bahir Dar, Ethiopia
Birhan Walie Alamneh
Department of Statistics, Wollo University, Dessie, Ethiopia
Wosenie Gebireamanuel Hailu
You can also search for this author in PubMed Google Scholar
Contributions
All authors reviewed the manuscript.
Corresponding author
Correspondence to Getachew Abate Dagnew .
Ethics declarations
Competing interests.
The authors declare no competing interests.
Additional information
Publisher's note.
Springer Nature remains neutral with regard to jurisdictional claims in published maps and institutional affiliations.
Supplementary Information
Supplementary information., rights and permissions.
Open Access This article is licensed under a Creative Commons Attribution 4.0 International License, which permits use, sharing, adaptation, distribution and reproduction in any medium or format, as long as you give appropriate credit to the original author(s) and the source, provide a link to the Creative Commons licence, and indicate if changes were made. The images or other third party material in this article are included in the article's Creative Commons licence, unless indicated otherwise in a credit line to the material. If material is not included in the article's Creative Commons licence and your intended use is not permitted by statutory regulation or exceeds the permitted use, you will need to obtain permission directly from the copyright holder. To view a copy of this licence, visit http://creativecommons.org/licenses/by/4.0/ .
Reprints and permissions
About this article
Cite this article.
Dagnew, G.A., Alamneh, B.W. & Hailu, W.G. Modeling and forecasting the volatility of some industry development indicators in Ethiopia using multivariate GARCH models. Sci Rep 14 , 14395 (2024). https://doi.org/10.1038/s41598-024-64749-3
Download citation
Received : 15 November 2023
Accepted : 12 June 2024
Published : 22 June 2024
DOI : https://doi.org/10.1038/s41598-024-64749-3
Share this article
Anyone you share the following link with will be able to read this content:
Sorry, a shareable link is not currently available for this article.
Provided by the Springer Nature SharedIt content-sharing initiative
By submitting a comment you agree to abide by our Terms and Community Guidelines . If you find something abusive or that does not comply with our terms or guidelines please flag it as inappropriate.
Quick links
- Explore articles by subject
- Guide to authors
- Editorial policies
Sign up for the Nature Briefing: Anthropocene newsletter — what matters in anthropocene research, free to your inbox weekly.


- Sign Up for Free Sign Up
dynamic development and Ethiopia case study
Description.
over 3 years ago | ||
Resource summary
Question | Answer |
name 3 physical factors that affect global uneven development | natural resources-clean water might not be available climate- dry climate with little rain makes it hard to grow crops hazards- frequent hazards make it difficult to re develop areas |
name 3 human factors that affect global uneven development | education- educated people tend to get paid more, they also pay more taxes, these can be used to further develop the country health- lack of clean water and formal housing makes people vulnerable to diseases trade- having good trade relationships improves economy |
what is 1.GNI per capita 2.Literacy rate 3.Life expectancy 4.Infant mortality rate | 1. average income a year per person 2. the percentage of the population that can read and write 3.average lifespan of a person born in a certain country 4. number of live births per 1000 *These are development indicators |
define Landlock | almost or entirely surrounded by land |
where is Ethiopia and what's its population | Horn of Africa, bordered by Djibouti, Eritrea, Kenya, Somalia, and Sudan. *it is a landlocked country it has a population of 94 million |
what is a TNC | transnational corporation: a business that operates in many different countries |
advantages of TNCs | well-known names may attract people to an area taxes can be used to improve the country |
Disadvantages of TNCs | tend to pay poorly to the local population (especially in factories) tend to take profits to source country and host country (Ethiopia) does not benefit much |
define 1. bottom up 2. top down strategies | 1.community led, tend to have an immediate impact and are less expensive 2. government led, tend to have a lasting impact of reducing poverty and increasing sustainability, tend to be expensive |
2 bottom up strategies in Ethiopia | Farm Africa has worked with local communities to breed goats and chickens. They have also created beehives in rural areas Local community is educated in the use of fertilizers and planting legumes between crops to keep soil fertile and rich in nitrogen, this improves crops yields |
2 top-down strategies | around $3.6 billion has been spent on converting rural mud roads into asphalt roads, reducing travel time HEP systems have been built on the Omo River, it has produced energy for industry but also flooded large areas and increased nearby water pollution |
Want to create your own Flashcards for free with GoConqr? Learn more .
- Leaderboard
- Where is Ethiopia located? On the Horn of Africa
- What are some of the barriers to Ethiopia's development? The country is landlocked , natural disasters (e.g. droughts, famine) happen frequently , their history , their government , and previous war with Eritrea
- How has being a landlocked country restricted Ethiopia's development? Because they are landlocked , it is harder to receive goods from trade / export goods because they either have to drive through other countries or fly the goods in, which is more expensive.
- How has their past restricted Ethiopia's development? Ethiopia was colonised by Italy from 1935 - 1941. Upon independence , their government was left very unstable and communism gained popularity due to people having to go through famine, unrest and droughts. This led to a successful military coup in 1974. Ethiopia was under the communist Derg government from 1974 - 1991. They grabbed tracts of land and evicted the owners, which caused migration, refugees and economic decline.
- How have natural disasters restricted Ethiopia's development? Ethiopia frequently suffers from droughts and famines. During these times, farmers cannot grow any crops to sell , so they cannot trade with other countries.
- How has the government restricted Ethiopia's government? The government is still not perfect (although fairly stable ) and due to their history of corrupt and unstable governments many years of development have been lost.
- What are the 5 stages of Rostow's Model? The traditional society , the preconditions for takeoff , the takeoff , the drive to maturity , and the age of mass consumption
- What happens at the Traditional Society stage of Rostow's Model + give an example of a country in this stage Most people are working in the primary sector ( mostly in agriculture ), local trade happens ( no international trade), an example of this is Burkina Faso
- What happens Precondition for Takeoff in Rostow's Model + give an example of a country in this stage The industrial and manufacturing society begins, agriculture is commercialised and trade expands overseas , an example of this is Ghana
- What happens at Takeoff in Rostow's Model + give an example of a country in this stage Substantial manufacturing sectors become developed, there is a technological breakthrough , an example of this is Thailand
- What happens at the Drive to Maturity in Rostow's Model + give an example of a country in this stage The economy is steadily growing, more modern technology, workers in agriculture drastically decrease, workers gain greater skills and are paid more, an example of this is China
- What happens at the High Mass Consumption stage of Rostow's Model + give an example of a country in this stage High value goods are normalised for purchase , consumers are beyond their basic needs , many people are living in cities ( urban society ), most people are working in the tertiary sector , an example of this is the US
- What are some of the TNCs in Ethiopia? Hilton Hotels , Siemens , General Electric (GE), Atriflora , Dow Chemicals , H&M , Huajian Shoe Factory ,
- What are some of the advantages to TNCs in Ethiopia? - Products are tailored to African customers - New and improved infrastructure - Helped build new dams are trade links to other countries - TNCs invest in development , causing economic growth
- What are some of the disadvantages to TNCs in Ethiopia? - Locals are only employed in low-paying jobs - Highly qualified Ethiopians who are capable of taking up higher positions within TNCs are not given these jobs - The government takes out massive loans , and cannot pay this debt - Concerns about sustainability , some of the roads are deteriating
- What are the Millennium Development Goals? 1. Eradicate extreme poverty and hunger 2. Achieve universal primary education 3. Promote gender equality and empower women 4. Reduce child mortality 5. Improve maternal health 6. Combat HIV / AIDS , malaria and other diseases 7. Ensure environmental sustainability 8. Develop a global partnership for development
- How much does Ethiopia export every year? 3 billion USD
- How much does Ethiopia import every year? 11 billion USD
- How much debt is Ethiopia in? 8 billion USD
- What are Ethiopia's main exports? Coffee , vegetables and legumes , pulses and oilseeds , flowers , livestock
- What are Ethiopia's main imports? Petroleum , trucks , fertilisers , construction , wheat
- How much did the tourist sector in Ethiopia grow by in 2018, and how much did it contribute to the economy? 48.6 %, contributed 7.4 billion USD ( 9.4 % of total economy)
- How many people are employed in the tourist sector in Ethiopia? Over 2.5 million people
- What percentage of visitors to Ethiopia are tourists? 79%
- What percentage of visitors to Ethiopia are international? 77%
- How many visitors does the Addis Ababa Bole International Airport attract every year? 22 million people
- How many people need food aid in Ethiopia? 8 million people
- What is Direct Foreign Investment ? Active ownership of a foreign company or of overseas manufacturing or marketing facilities
- Which country funded the first 6 lane highway in Ethiopia? China
- Because China has been demanding repayments, what has the Ethiopian government resorted to, and how does this cause further problems for the country? Borrowing from the World Bank and IMF , which causes more debt
- What are some of the benefits of Goat Aid in Ethiopia? - The manure can be used for crops - Goats are cheap - Can breed more goats - Use goat fur for clothes - Can get milk and meat
- What are some of the problems of Goat Aid in Ethiopia? - Can be hard to transport - Diseases can spread - People have to be educated on looking after the goats properly - Veterinary bills can be expensive , sometimes vets are inaccessible - Goats need to be fed
- What is a bottom up development strategy + give an example These strategies focus on the needs of individual communities , and are funded by charities or NGOs. An example is when the charity Farm Africa trained locals to be better farmers so that they can sell their goods and provided them with animals that they can breed and make money from.
- What is a top down development strategy + give an example These strategies are large scale projects, funded by the government and have an impact on many people, not just certain communities. An example is the Gibe III dam on the Omo River , which provides many people with electricity , and also makes the water safer so that it can be stored in case of droughts.
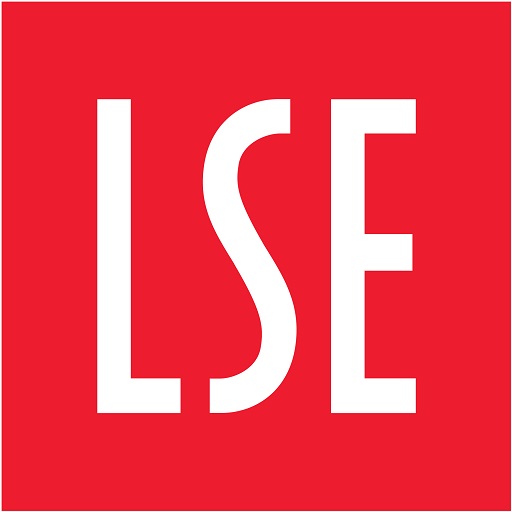
- Publications
- Student Ambassadors
Basil Oberholzer
Dawit ayele haylemariam, june 27th, 2024, finance, economic stability, and green growth in ethiopia.
Estimated reading time: 6 minutes
Guest bloggers Basil Oberholzer and Dawit Ayele Haylemariam argue for maintaining Ethiopia’s developmental financial system and implementing economic reforms to address inflation, currency shortages, and slowing growth while promoting green development.
Ethiopia, Africa’s second-most populous country, has experienced twenty years of high economic growth and great success in poverty reduction. This growth has been fuelled by substantial public investment in critical infrastructure and social development programs. To continue on this path, the challenges emerged in past years, such as slowing growth, high inflation, foreign exchange shortage, and harmful climate change impacts must be addressed quickly. How can Ethiopia get out of these constraints by fostering green growth and development while avoiding austerity and more instability, which jeopardize development success? Answers can be found in the analysis of Ethiopia’s banking system and its key role in sustaining macroeconomic and monetary stability.
Ethiopia’s banks feature a high share of public ownership, which has effectively served the government’s successful developmentalist strategy driven by public investment. At the same time, the country conducts monetary policy in a rather monetarist fashion where banks are subject to minimum reserve requirements while the growth of reserves is the main policy target. Restricting the supply of reserves thus sets an upper limit to money growth. Additionally, interest rates are also controlled by setting a minimum deposit rate. Commercial banks are obliged to purchase treasury bonds to cover the government’s budget deficit at least partially. Moreover, banks face requirements to surrender large shares of foreign exchange earnings to the National Bank of Ethiopia (NBE). The currency is pegged to the US Dollar, capital controls limit currency convertibility, and the banking system is closed to foreign banks.
Misleading analysis of macroeconomic challenges
Several analyses, notably by international financial institutions , identify the roots of Ethiopia’s macroeconomic problems as follows: money supply growth due to public budget deficits financed by advances from the NBE drives inflation; government interventions in the financial sector lead to inefficiencies in resource allocation; government dominance creates finance shortage and crowds out the private sector; negative real interest rates discourage saving; the fixed exchange rate leads to a loss in international competitiveness due to inflation while capital controls keep away foreign capital, thus intensifying the lack of finance in the economy. The recommendations of those analysts are clear: reduce the government’s budget deficit, adopt market interest rates, liberalize capital flows by removing controls and exchange rate pegging, and open the market to foreign banks. As a result, inflation will come down, resource allocation will be more efficient, the current account will improve, and the financing constraint lifted.
The above perspective would possibly be useful if money was as a scarce commodity where a limited amount of savings is available to finance investment. Yet, in the world we are living in, money is created via the issuance of loans, meaning that every loan creates a deposit of an equal amount. Savings are not a precondition of investment but, instead, the result of investment. Hence, money is driven by demand for credit and associated to economic production, which means that money is elastic. The quantity of money is neither limited by an external force nor does it say anything about the causes of inflation.
Once capital expenditures are excluded from Ethiopia’s government budget, i.e. when comparing total government revenue and government recurrent spending, the public finance exhibited a surplus in the last 20 years. Distinguishing between current and capital expenditures is relevant because the former create new output and income and hence should be financed via new loans much like private investment. Moreover, government expenses involving payments abroad for external debt servicing and specific military spending amounted to more than 20 percent of the total budget deficit. Such payments involve a drainage rather than an increase in the amount of money. Explaining inflation via the growth of the domestic quantity of money, which is itself supposedly driven by the government budget deficit, does not work. There are enough other factors that can explain inflation in Ethiopia: currency devaluation, global commodity price spikes, urbanization, domestic supply-side shocks such as in agriculture due to conflict and climate change. As a result, more money is required to deal with higher prices of everything but that does not mean that money causes inflation.
The consequences of restricting money supply
Crowding-out of the private sector via government deficit financing is a possibility in Ethiopia because of the monetary authority’s endeavor to control the growth of reserves and, by extension, money supply. This is more evident in the National Bank’s recent policy to cap credit expansion at 14% in inflation fight . In this situation, restrictions to commercial banks’ balance sheets combined with their obligation to purchase treasury bonds may leave an insufficient space for credit to the private sector. Yet, this is a policy choice rather than a necessity. It is the monetarist idea of restricting money supply that leads to this crowding-out. This is economically harmful while not even addressing the causes of inflation. If the central bank accommodates demand for loans, crowding-out can be avoided without the government cutting down its spending, which would have negative economic and social consequences (which does not mean that expenses should not be reviewed and checked for their social benefits before making them).
In this context, there is an additional driver of inflation, which has not yet been mentioned: due to overall inflation, wealth holders store their wealth in real estate, which drives up their prices, thus contributing to more cost-push inflation in the rest of the economy. Data show that real estate prices in Ethiopia’s capital, Addis Ababa, have risen at a much faster rate than consumer prices and also house rent prices. This points to the possibility of a speculative bubble in the making. Moreover, due to imposed restrictions on money growth, real estate investment leaves less space for real productive and green investment. It is likely that speculative investment is the much more relevant source of crowding-out real private investment than the government deficit. Yet, productive investment is more than ever required to address the economy’s supply-side constraints, particularly in agriculture, industries, and post-conflict reconstruction. While money supply control is not able to tackle inflation, it is an obstacle to an investment-driven, growth-enhancing, and climate-friendly anti-inflation strategy.
Simple reforms for stability and green growth
Financing constraints can also be addressed without devaluing the exchange rate or removing capital controls. Obviously, foreign exchange is essential to pay for imports. Yet, financial account liberalization will most likely intensify forex shortage rather than easing it. Capital flight will set in with devastating and self-enforcing consequences including severe inflation spikes. If the trade deficit improves, it will mostly not do so due to adjusted prices thanks to currency devaluation, but due to shrinking import demand arising from the shock effect on the Ethiopian economy.
Instead of dismantling the government’s developmentalist strategy that could trigger additional instability, the Ethiopian banking system should take a few key steps towards a growth-led and green framework. First, the NBE should accommodate commercial banks’ demand for reserves to remove financing constraints to productive green investment. Second, speculative investment should be restricted by instruments such as, for instance, limits to mortgage volumes or higher reserve requirements than for productive investment. What matters is not the fixation on the quantity of money but ensuring that finance flows to investment with green and social benefits rather than inflation-driving speculative investment. In this way, structural change can be enforced to reduce import dependence, promote exports, and grow out of foreign exchange shortage.
The views expressed in this post are those of the author and do not reflect those of the International Development LSE blog or the London School of Economics and Political Science.
Featured image credit: Addis Ababa, snapped by Gideon Abate via www.ethiopia-insight.com .
Share this:
- Click to share on Facebook (Opens in new window)
- Click to share on Twitter (Opens in new window)
- Click to email this to a friend (Opens in new window)
- Click to print (Opens in new window)
About the author

Basil Oberholzer is a Senior Economist at the Global Green Growth Institute advising governments in the Global South on matters of green economic development. He holds a PhD in macroeconomics from the University of Fribourg and has published books and peer-reviewed research articles on development economics, macroeconomics, and ecological economics.

Dawit Ayele Haylemariam, an economist, is the Founder of Growth Capital Analytics in Addis Ababa. He holds a Master’s in Good Governance and Public Policy from the University of Passau, a Master’s in Development Policy from Korea Development Institute School, and a Bachelor’s in Economics from Jimma University. He has served in advisory and consultancy roles with the Ethiopian Ministry of Finance, the World Bank, UNIDO, UNDP, GGGI, and the Tony Blair Institute for Global Change.
Leave a Reply Cancel reply
Your email address will not be published. Required fields are marked *
Notify me of follow-up comments by email.
Notify me of new posts by email.
Related Posts
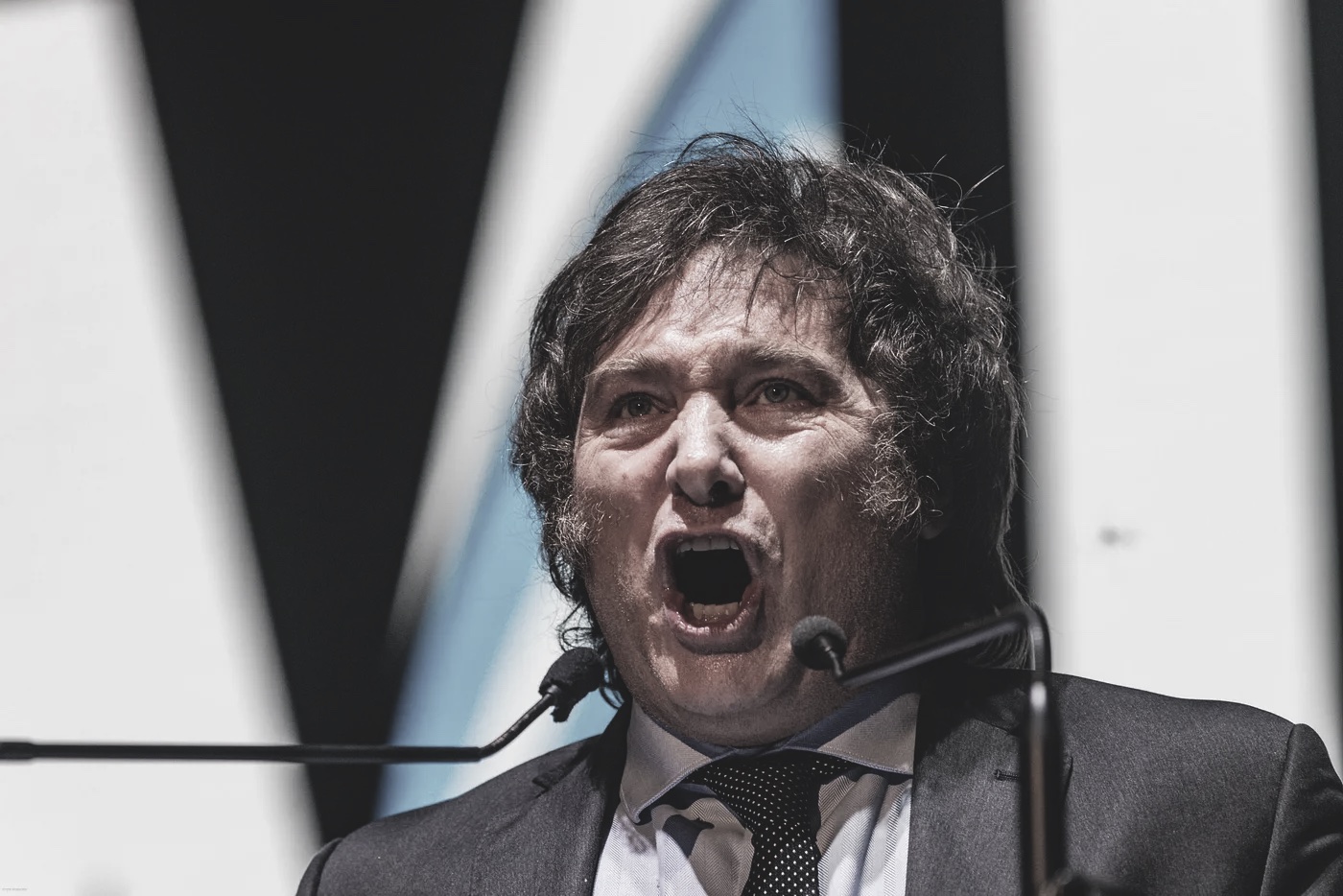
Argentina and Türkiye: Two very different countries, the same problem
February 6th, 2024.
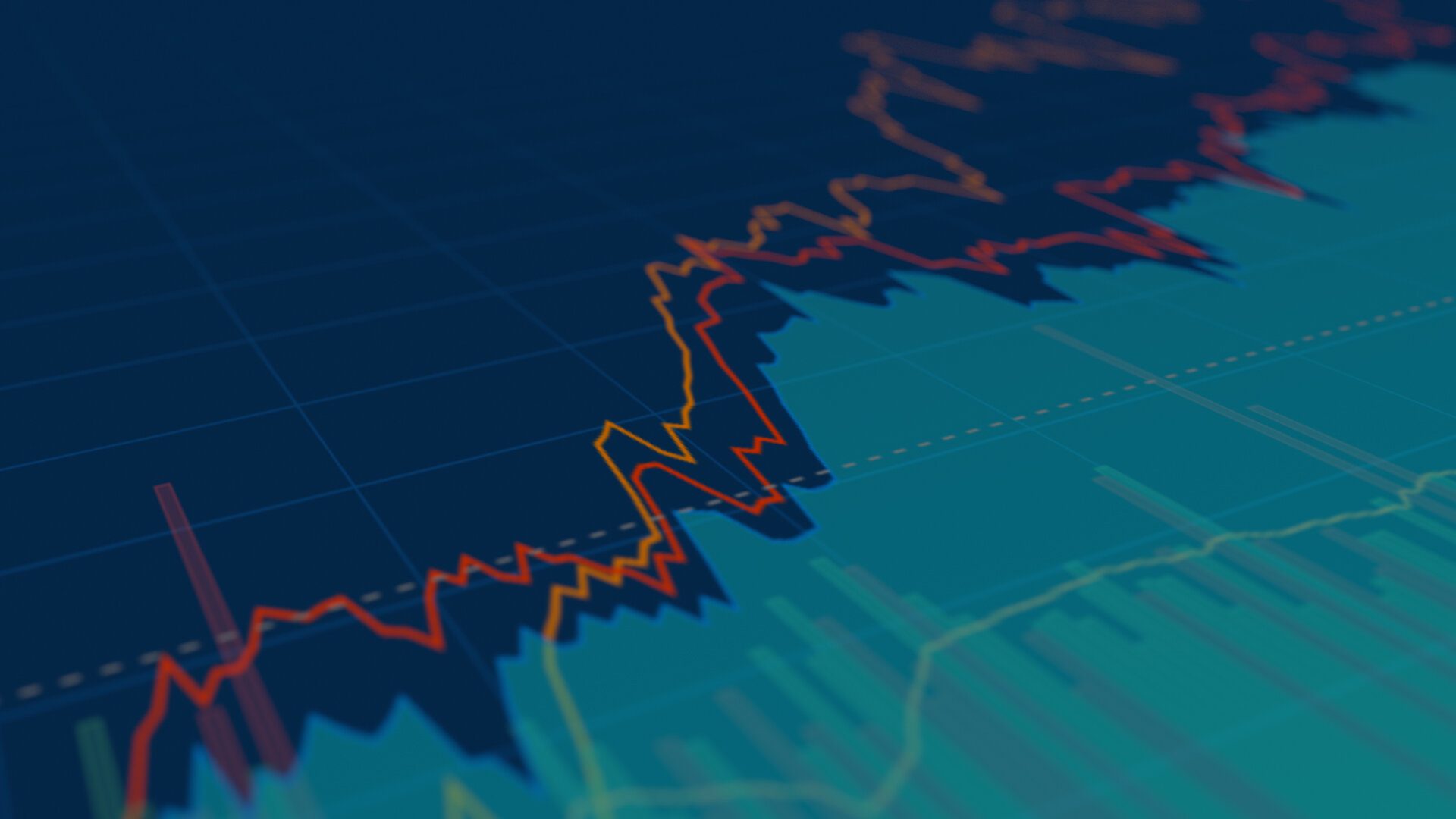
Financial crisis predictions in emerging markets: Then and now
October 27th, 2022.
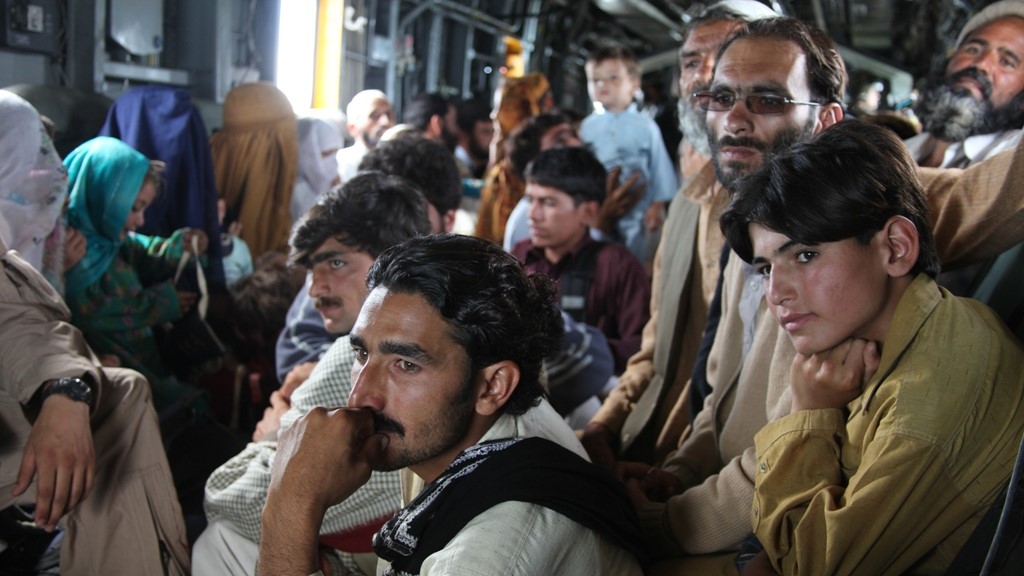
Can Pakistan survive the next 75 years without strong human capital?
December 5th, 2022.

Justice and Security Research Programme
Lse’s engagement with south asia.
- The Coup d’état in Myanmar and Collective Punishment of Minorities As the military junta loses direct control over most of the country to ethnic armed organisations (EAOs) and other local resistance groups, their repression has often targeted specific minority communities. Political analyst Tluang Kip Thang discusses the targeting of Christian minorities in Myanmar, alongside ‘partisan’ Buddhists who are also vulnerable to the junta‘s actions. […]
- Polling Agents: An Unlikely Agent of Development in South Asia For the topic ‘Politics is a Barrier to Societal Development in South Asia’ — Zaid Ahmed Abro, winner of the LSE South Asia Centre’s Vera Anstey South Asia Essay Competition 2024 — argues that polling agents in South Asia should be seen as agents of development, providing crucial transparency and voting education for the electorate at polling booths […]
- International
- Education Jobs
- Schools directory
- Resources Education Jobs Schools directory News Search
GCSE 9-1: Global development - Ethiopia case study

Last updated
8 December 2021
- Share through email
- Share through twitter
- Share through linkedin
- Share through facebook
- Share through pinterest
Resources included (7)
Gcse 9-1; global development - ethiopia case study, political development.
GCSE 9-1; Global development- Ethiopia case study , population and employment structure
GCSE 9-1; Global development - Ethiopia case study, location, climate and ecosystems
GCSE 9-1; Global development - Ethiopia case study, trade
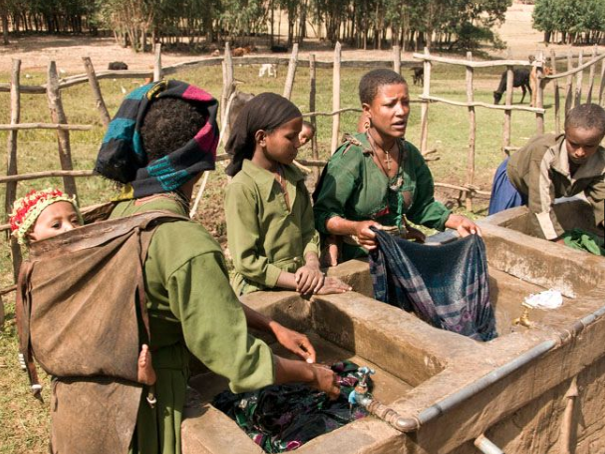
GCSE 9-1; Global development - Ethiopia aid project- Charity water
GCSE 9-1; Global development - Ethiopia case study, DME solving trade problems
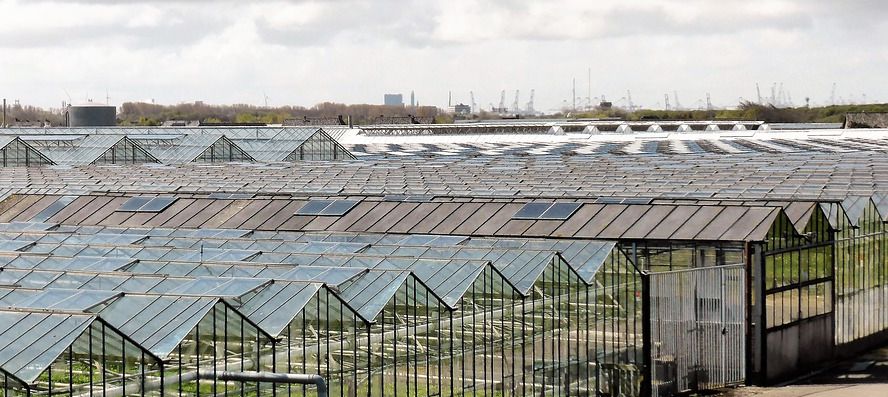
GCSE 9-1; Global development - Ethiopia case study, international investment
Tes paid licence How can I reuse this?
Your rating is required to reflect your happiness.
It's good to leave some feedback.
Something went wrong, please try again later.
This resource hasn't been reviewed yet
To ensure quality for our reviews, only customers who have purchased this resource can review it
Report this resource to let us know if it violates our terms and conditions. Our customer service team will review your report and will be in touch.
Not quite what you were looking for? Search by keyword to find the right resource:
Academia.edu no longer supports Internet Explorer.
To browse Academia.edu and the wider internet faster and more securely, please take a few seconds to upgrade your browser .
Enter the email address you signed up with and we'll email you a reset link.
- We're Hiring!
- Help Center
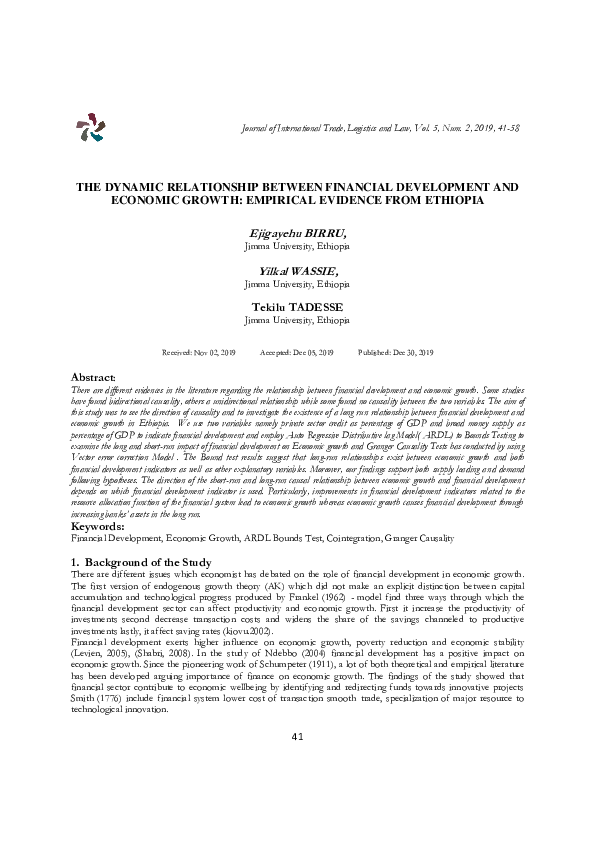
The Dynamic Relationship between Financial Development and Economic Growth: Empirical Evidence from Ethiopia

2019, Journal of International Trade, Logistics and Law
There are different evidences in the literature regarding the relationship between financial development and economic growth. Some studies have found bidirectional causality, others a unidirectional relationship while some found no causality between the two variables. The aim of this study was to see the direction of causality and to investigate the existence of a long run relationship between financial development and economic growth in Ethiopia. We use two variables namely private sector credit as percentage of GDP and broad money supply as percentage of GDP to indicate financial development and employ Auto Regressive Distributive lag Model( ARDL) to Bounds Testing to examine the long and short-run impact of financial development on Economic growth and Granger Causality Tests has conducted by using Vector error correction Model . The Bound test results suggest that long-run relationships exist between economic growth and both financial development indicators as well as other expla...
Related Papers
Yvonne Gwenhure
This paper investigates the dynamic causal linkage between bank-based financial development and economic growth in Ethiopia during the period from 1980 to 2014. The study includes savings and investment as intermittent variables in an attempt to address the omission of variable bias – thereby creating a multivariate Granger-causality model. Using the newly developed autoregressive distributed lag bounds testing approach to cointegration and the error-correction model-based causality model, the study finds that in the short run, both financial development and economic growth Granger-cause each other in Ethiopia. However, in the long run, there is unidirectional Granger-causality from bank-based financial development to economic growth. The study, therefore, recommends that policies aimed at enhancing both economic growth and financial development should be pursued in the short run. However, in the long run, policies that target the development of the banking sector should be prioriti...
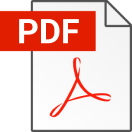
RePEc: Research Papers in Economics
Sheilla Nyasha
Orginal article
Tekilu Tadesse
This paper investigates linkage between financial development and economic growth in Ethiopia during the period from 1975 to 2016 using Autoregressive Distributed Lag (ARDL) approach. The paper also schedules Vector Error Correction Model (VECM) in order to observe how fast the cointegrated variables convergence in long-run. Accordingly, the results of bound test confirmed that the long run relationship between explanatory variables and economic growth. The empirical results implied evidence of a long-run and short run positive impact of financial development on economic growth in Ethiopia. This implies financial sector and financial institution the input to support and accelerate economic growth short and long run. With regard to other control variables, except inflation and government expenditure as percentage of GDP, all variables significantly influence economic growth in the long-run. Other than inflation, government expenditure and trade openness, human capital and gross investment were the pioneer determinant of economic growth in the short run. Furthermore, VECM granger causality tests show that the direction of causality is running from financial development to economic growth both in short run and long run. This study found the 'supply-leading' hypothesis held in the case of Ethiopia.
International Journal of All Research Education and Scientific Methods (IJARESM)
Bealu Tukela
Finance-growth nexus is one of the main issues in the major economies of the world.This study aimed at examining effects of financial development on economic growth in Ethiopia using annual time series data from 1980 to 2019. Stationary tests were conducted using the Augmented Dicker Fuller (ADF) test statistic. The Autoregressive Distributed Lag (ARDL) and Bounds test for co-integration was conducted. The Granger Causality technique was employed. The findings reveal that financial sector development causes economic growth. Inflation had a negative impact on economic growth in line with a priori expectation.Credit to private sector and savings had a positive impact on economic growth. It is recommended that monetary policy makers ensure all stakeholders adhere strictly to laid down goals to guarantee financial development. Government should implement policies that promote financial sector development which will result in investment and economic growth. Key Words: ADF, ARDL,Financial Development, Granger Causality, Time Series.
Journal of Development Studies
Grace Ofori-Abebrese
International Journal of Research in Business and Social Science (2147- 4478)
Oscar Chiwira
This study investigated the nexus between financial development and economic growth, exploring the short-run and long-run impact of financial development on economic growth in the Sothern African Development Community (SADC). The SADC was fragmented into middle-income countries and low-income countries so as to ascertain the income effect on the nature of the relationship, an exploration not considered by previous studies. The study used panel data covering the period 1980 to 2020. It employed the Autoregressive Distributed Lag (ARDL) Bounds and the Toda and Yamamoto and Dolado and Lütkepohl (TYDL) models to examine the relationship and the direction of causality respectively. In the short run, financial development, through domestic credit to the private sector, spurs economic growth and in the long run financial development focus should be on promoting bank deposits. Causality tests between economic growth and financial development tests showed mixed results depending on the varia...
Grace Ofori-Abebrese , Robert Becker Pickson
Although the finance-growth relationship has been looked at in many African countries such as Ghana, the outcomes have been mixed while the causal direction between the two variables has particularly not been examined. This study, therefore, applied ARDL approach and Granger causality test to investigating the relationship and the causal direction between financial development and economic growth in Ghana during the period 1970-2013. The study revealed that the amount of credit from domestic sources to the private sector maintained a positively significant nexus with the growth of the economy whereas the domestic deposit was not the case. Also, the results established that there is a dependence of the Ghanaian economy to the changes in domestic credit to private sector whilst there exists a uni-directional causality running from the variations in economic growth to the domestic deposit in Gha-na. Consistent with the findings, it is recommended that the authorities concentrate on improving the efficiency of the financial system to allow deposits to be channelled to growth-inducing investments to bring about the long-term growth of the economy.
International Journal of Economics & Management Sciences
Tekilu Tadesse , Wondaferahu Mulugeta
This paper aimed to investigate the linkage between financial development and sectoral output growth with special emphasis on Agriculture, industry and service sectors in Ethiopia during the period from 1975 to 2016. The study has used Autoregressive Distributive lag (ARDL) bound testing approach via an augmented growth model to examine the linkage between the financial development, proxy by bank credit to sectors, and sectoral output growth. Furthermore, Vector Error Correction Model (VECM) was employed to investigate the direction of causality between financial development and sectoral output growth. The results of bound test confirmed that the long run relationship between explanatory variables and sector output growth with less co integration of agricultural output growth and respective independent variables. The empirical results of this study showed, that in the long run financial development had a less significant positive impact on agricultural and service sector output growth but, short run relationship was found to be insignificant. However, financial development has a positive and significant impact on industrial and aggregate output growth both in the short run and long run. Furthermore, VECM granger causality tests show that there is no causality between financial development and agricultural output growth both in long run and short run. However, uni-directional causality running from (1) financial development to industrial output growth both in the long run and short run which confirmed supply leading growth hypothesis (2) financial development to service sector output growth in the long run (supply leading) and in the short run running from service sector to financial development which supports demand leading hypothesis only in short run.
International journal trade, economics and finance
Ariuna Taivan
felician mutasa
This paper examines causality relationship between financial development and economic growth in Tanzania over the period 1980 to 2012. In time series context, recently econometric techniques were used; namely Augmented Dickey and Fuller test (ADF) for unit roots test, Johansen test for Co-intergration test, Vector error correction model (VECM) tested for short run and long run causality, a pairwise Granger causality test used to establish the direction of causality and Variance decomposition (VD) under VAR framework applied for validating strengths of findings outside the estimated sampling period. In overall empirical findings can be summarized as follows. Firstly, there is long-run relationship between financial development and economic growth. Secondly, granger causality test suggests economic growth causes financial development in a short-run when broad money to nominal GDP and liquidity liability to nominal GDP used, however when credit to private sector to nominal GDP was used...
Loading Preview
Sorry, preview is currently unavailable. You can download the paper by clicking the button above.
RELATED PAPERS
Journal of Economic and Financial Sciences
thobeka ncanywa
Review of Development Economics
Suleiman Abu-Bader
GYLYCH JELILOV
Bankole Falana
Zenodo (CERN European Organization for Nuclear Research)
Emeka Okereke
Aisha M Abubakar
alper aslan
Chiamaka Eneh
Abdul wadud
The Review of Finance and Banking
Anthony Akinlo
Charles Ricky-Okine
Cedric Komnang
Michael Adusei , Efthymios Tsionas
Iranian Economic Review
Oluyemi A Okunlola , Emilomo Omons Masade
Chief Editor
Mohammad Kashem
South African Journal of Economic and Management Sciences
Ilhan Ozturk
Economic Development and Cultural Change
Eric Pentecost
Chekwube Madichie
Zubair Uddin
Business And Management Studies: An International Journal
Sevilay Atlama Küçüksakarya
International Journal of Management Sciences and Business Research
Samuel K O F I Otchere
erasmus hyera
RELATED TOPICS
- We're Hiring!
- Help Center
- Find new research papers in:
- Health Sciences
- Earth Sciences
- Cognitive Science
- Mathematics
- Computer Science
- Academia ©2024
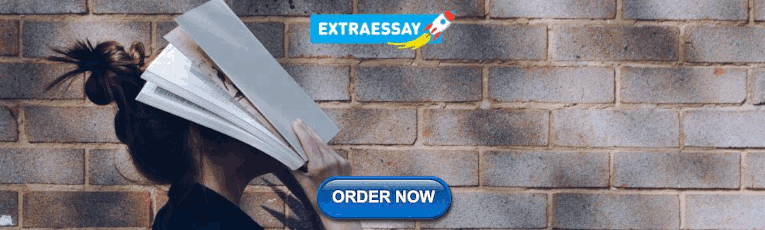
IMAGES
VIDEO
COMMENTS
How has their past restricted Ethiopia's development? Ethiopia was colonised by Italy from 1935 - 1941. Upon independence, their government was left very unstable and communism gained popularity due to people having to go through famine, unrest and droughts. This led to a successful military coup in 1974.
During the build up to WWII, Italy colonized Ethiopia and had control from 1935-41 when rebels and British troops claimed back independence. - at least 1.4m people died in the civil war and the derg government remained in power until 1987. - the 1984-85 famine killed a million people in just one year due to drought and high food prices.
Ethiopia has seen a steady increase in demand for dairy products recently. Between 2007 and 2017 the number of dairy processors in Ethiopia increased from 8 to 25 and annual overall dairy production has increased from 927 million liters in 1996 to 3.3 billion liters in 2018 (Tschopp, Gizachew, & Wood, 2021).
6.2- DYNAMIC DEVELOPMENT- Ethiopia case study (complete) 2. Measures of Development: Click the card to flip 👆. HDI- takes into account a range of social and economic factors. GNI/per capita- just an economic measure, it is calculated by finding the gross national income on average of those living in Ethiopia. Click the card to flip 👆.
Ethiopia has made considerable effort to improve girls' access to education. The girls/boys ratio in primary education has improved from 84% in 2005 to 94% in 2013. At secondary level, the ratio of girls to boys reached 82% in 2013. This progress is key to promoting gender equality across Ethiopia (see Box 1.7).
Focus group discussions and key informant interviews were also used to validate land use classes through triangulation.FindingsThe study found that the built-up area was the most dynamic LULC category (85.1%) as it increased by over 4,000 ha between 1990 and 2020.
Dynamic Development: Ethiopia. Level of Development in Ethiopia. GNI/Capita= $505. Economy growing 2.6%/year; HDI: 165/188 countries worldwide; ... Aid/Sustainable Development Case Study - WaterAid. 4.0 / 5 based on 3 ratings. Geography Topics. 1.5 / 5 based on 2 ratings. Geography. 0.0 / 5. Worldwide effect of CLIMATE CHNAGE:
Abstract: This research compendium provides an explanation of Ethiopia's fundamental economic challenge of slowing economic growth after an exceptional growth acceleration — a challenge that has been compounded by COVID-19, conflict, and climate change impacts. Ethiopia has experienced exceptional growth since the early 2000s but began to ...
This is a dynamic contribution to the developmental state literature that conceptually centres the interactions between states and the international sphere which has been largely unaddressed until now. ... Industrial Park Development in Ethiopia: Case Study Report. Inclusive and Sustainable Industrial Development Working Article Series WP 21 ...
reproductive health in Ethiopia. He offers background on Ethiopia and population policy; an assessment of progress made toward reaching large development goals; a review of the role of UNFPA and other population organizations in Ethiopia; and challenges and opportunities for the population and development communities in Ethiopia.
The main aim of this study was to assess the relationship between industry development indicators in Ethiopia using a multivariate GARCH model based on World Bank data from 1982 to 2021.
define 1. bottom up 2. top down strategies. 1.community led, tend to have an immediate impact and are less expensive 2. government led, tend to have a lasting impact of reducing poverty and increasing sustainability, tend to be expensive. 2 bottom up strategies in Ethiopia. Farm Africa has worked with local communities to breed goats and chickens.
PDF | On Dec 1, 2020, Belayneh Bogale Zewdie published Status, Challenges, and Prospects of the Ethiopian Developmental State (Emphasis on Democratic Governance) | Find, read and cite all the ...
Ethiopia was colonised by Italy from 1935 - 1941. Upon independence, their government was left very unstable and communism gained popularity due to people having to go through famine, unrest and droughts. This led to a successful military coup in 1974. Ethiopia was under the communist Derg government from 1974 - 1991. They grabbed tracts of land and evicted the owners, which caused migration ...
Guest bloggers Basil Oberholzer and Dawit Ayele Haylemariam argue that to address Ethiopia's current challenges, such as high inflation, foreign exchange shortage, and slowing growth, the financial system's developmental structure should not be dismantled in the hope that private capital will fill the gap. Instead, they make a case for a few economic reforms to maintain it as a robust engine ...
Study Dynamic Development Case Study - Ethiopia with flashcards, multiple choice questions, and games. Master concepts like Ethiopia GNI per capita, Ethiopia population and more.
1.1 This study of Ethiopia is one of six country case studies undertaken as part of an independent evaluation by the World Bank's Operations Evaluation Department (OED) of the relevance and effectiveness of World Bank support for public sector capacity building in Sub-Saharan Africa over the past ten years (1995-2004).
Study with Quizlet and memorise flashcards containing terms like where is ethiopia located, examples of development indicators for ethiopia, what are ethiopias exports and others. Scheduled maintenance: May 29, 2024 from 10:00 PM to 12:00 AM
Ethiopia was colonised by Italy from 1935 - 1941. Upon independence, their government was left very unstable and communism gained popularity due to people having to go through famine, unrest and droughts. This led to a successful military coup in 1974. Ethiopia was under the communist Derg government from 1974 - 1991.
GCSE 9-1; Global Development - Ethiopia case study, Political development. In this lesson students will learn about political development in Ethiopia from 1935 and its impacts on the economy. to the current Tigran civil War satrted in 2020, which threatens to further development of the country. Firstly, the students are given a time line of ...
Resources included (7) This bundle contains a series of lessons to teach students about economic development in Ethiopia. it includes the following content: employment structure, population, trade, international investment, aid, political development, climate, ecosystems and location. It also contains a range of teaching and learning strategies ...
GCSE Geography Dynamic development learning resources for adults, children, parents and teachers. ... Case study - emerging and developing country - India - OCR.
This result is contradict with early causality study done by Mckinnon (1973) and 54 The Dynamic Relationship between Financial Development and Economic Growth: Empirical Evidence from Ethiopia Shaw (1973), works of King and Levine (1993), and the later study by Helmi et al. (2013) and Agyei (2015) which supply leading hypothesis of financial ...
LIDC case study Factors that impact Ethiopia's economic development 'everyone' 80% work in traditional work and small scale agriculture w low wages, population is 94million growing annually by 2.6% not enough resources for everyone, civil war
Higher; Differences in levels of development between developing countries Comparing Ethiopia and South Korea. There are many reasons, physical and human, to explain differences in levels of ...
1. Western Highlands 2.Central area 3. Eastern Lowlands. What are 3 effects of unreliable rainfall in eastern lowlands of Ethiopia. drought, soil erosion and desertification. Livestock is kept in eastern area. What can this lead to. overgrazing --> soil erosion --> agricultural unreliability. Study with Quizlet and memorize flashcards ...