Module 13: Theory of Evolution
Phylogenetic trees, read and analyze a phylogenetic tree that documents evolutionary relationships.
In scientific terms, the evolutionary history and relationship of an organism or group of organisms is called phylogeny. Phylogeny describes the relationships of an organism, such as from which organisms it is thought to have evolved, to which species it is most closely related, and so forth. Phylogenetic relationships provide information on shared ancestry but not necessarily on how organisms are similar or different.
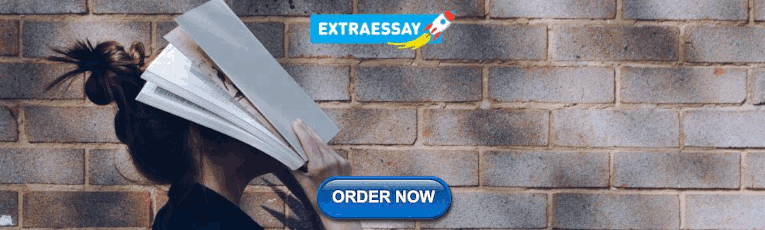
Learning Objectives
- Identify how and why scientists classify the organisms on earth
- Differentiate between types of phylogenetic trees and what their structure tells us
- Identify some limitations of phylogenetic trees
- Relate the taxonomic classification system and binomial nomenclature
Scientific Classification
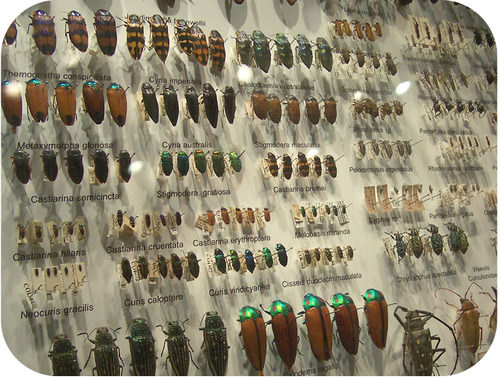
Figure 1. Only a few of the more than one million known species of insects are represented in this beetle collection. Beetles are a major subgroup of insects. They make up about 40 percent of all insect species and about 25 percent of all known species of organisms.
Why do biologists classify organisms? The major reason is to make sense of the incredible diversity of life on Earth. Scientists have identified millions of different species of organisms. Among animals, the most diverse group of organisms is the insects. More than one million different species of insects have already been described. An estimated nine million insect species have yet to be identified. A tiny fraction of insect species is shown in the beetle collection in Figure 1.
As diverse as insects are, there may be even more species of bacteria, another major group of organisms. Clearly, there is a need to organize the tremendous diversity of life. Classification allows scientists to organize and better understand the basic similarities and differences among organisms. This knowledge is necessary to understand the present diversity and the past evolutionary history of life on Earth.
Scientists use a tool called a phylogenetic tree to show the evolutionary pathways and connections among organisms. A phylogenetic tree is a diagram used to reflect evolutionary relationships among organisms or groups of organisms. Scientists consider phylogenetic trees to be a hypothesis of the evolutionary past since one cannot go back to confirm the proposed relationships. In other words, a “tree of life” can be constructed to illustrate when different organisms evolved and to show the relationships among different organisms (Figure 2).
Each group of organisms went through its own evolutionary journey, called its phylogeny. Each organism shares relatedness with others, and based on morphologic and genetic evidence, scientists attempt to map the evolutionary pathways of all life on Earth. Many scientists build phylogenetic trees to illustrate evolutionary relationships.
Structure of Phylogenetic Trees
A phylogenetic tree can be read like a map of evolutionary history. Many phylogenetic trees have a single lineage at the base representing a common ancestor. Scientists call such trees rooted, which means there is a single ancestral lineage (typically drawn from the bottom or left) to which all organisms represented in the diagram relate. Notice in the rooted phylogenetic tree that the three domains—Bacteria, Archaea, and Eukarya—diverge from a single point and branch off. The small branch that plants and animals (including humans) occupy in this diagram shows how recent and miniscule these groups are compared with other organisms. Unrooted trees don’t show a common ancestor but do show relationships among species.
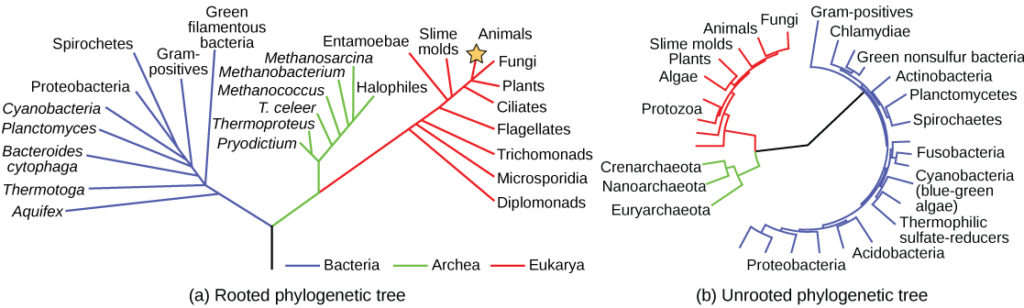
Figure 2. Both of these phylogenetic trees shows the relationship of the three domains of life—Bacteria, Archaea, and Eukarya—but the (a) rooted tree attempts to identify when various species diverged from a common ancestor while the (b) unrooted tree does not. (credit a: modification of work by Eric Gaba)
In a rooted tree, the branching indicates evolutionary relationships (Figure 3). The point where a split occurs, called a branch point , represents where a single lineage evolved into a distinct new one. A lineage that evolved early from the root and remains unbranched is called basal taxon . When two lineages stem from the same branch point, they are called sister taxa . A branch with more than two lineages is called a polytomy and serves to illustrate where scientists have not definitively determined all of the relationships. It is important to note that although sister taxa and polytomy do share an ancestor, it does not mean that the groups of organisms split or evolved from each other. Organisms in two taxa may have split apart at a specific branch point, but neither taxa gave rise to the other.
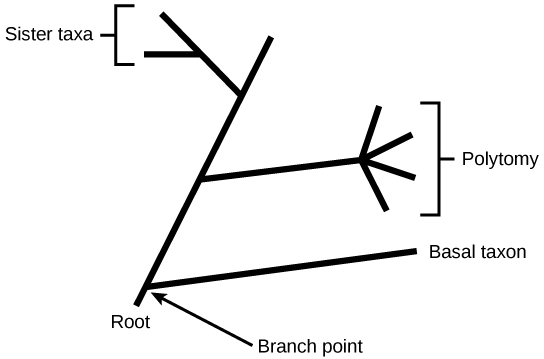
Figure 3. The root of a phylogenetic tree indicates that an ancestral lineage gave rise to all organisms on the tree. A branch point indicates where two lineages diverged. A lineage that evolved early and remains unbranched is a basal taxon. When two lineages stem from the same branch point, they are sister taxa. A branch with more than two lineages is a polytomy.
The diagrams above can serve as a pathway to understanding evolutionary history. The pathway can be traced from the origin of life to any individual species by navigating through the evolutionary branches between the two points. Also, by starting with a single species and tracing back towards the “trunk” of the tree, one can discover that species’ ancestors, as well as where lineages share a common ancestry. In addition, the tree can be used to study entire groups of organisms.
Another point to mention on phylogenetic tree structure is that rotation at branch points does not change the information. For example, if a branch point was rotated and the taxon order changed, this would not alter the information because the evolution of each taxon from the branch point was independent of the other.
Many disciplines within the study of biology contribute to understanding how past and present life evolved over time; these disciplines together contribute to building, updating, and maintaining the “tree of life.” Information is used to organize and classify organisms based on evolutionary relationships in a scientific field called systematics. Data may be collected from fossils, from studying the structure of body parts or molecules used by an organism, and by DNA analysis. By combining data from many sources, scientists can put together the phylogeny of an organism; since phylogenetic trees are hypotheses, they will continue to change as new types of life are discovered and new information is learned.
Video Review
Limitations of Phylogenetic Trees
It may be easy to assume that more closely related organisms look more alike, and while this is often the case, it is not always true. If two closely related lineages evolved under significantly varied surroundings or after the evolution of a major new adaptation, it is possible for the two groups to appear more different than other groups that are not as closely related. For example, the phylogenetic tree in Figure 4 shows that lizards and rabbits both have amniotic eggs, whereas frogs do not; yet lizards and frogs appear more similar than lizards and rabbits.
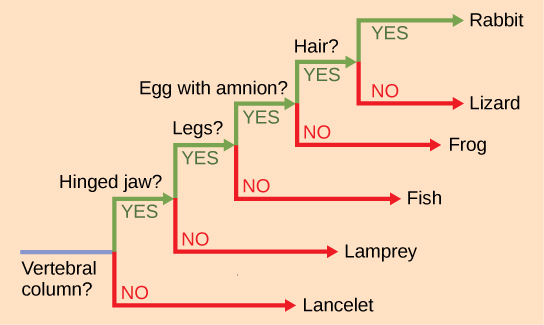
Figure 4. This ladder-like phylogenetic tree of vertebrates is rooted by an organism that lacked a vertebral column. At each branch point, organisms with different characters are placed in different groups based on the characteristics they share.
Another aspect of phylogenetic trees is that, unless otherwise indicated, the branches do not account for length of time, only the evolutionary order. In other words, the length of a branch does not typically mean more time passed, nor does a short branch mean less time passed— unless specified on the diagram. For example, in Figure 4, the tree does not indicate how much time passed between the evolution of amniotic eggs and hair. What the tree does show is the order in which things took place. Again using Figure 4, the tree shows that the oldest trait is the vertebral column, followed by hinged jaws, and so forth. Remember that any phylogenetic tree is a part of the greater whole, and like a real tree, it does not grow in only one direction after a new branch develops.
So, for the organisms in Figure 4, just because a vertebral column evolved does not mean that invertebrate evolution ceased, it only means that a new branch formed. Also, groups that are not closely related, but evolve under similar conditions, may appear more phenotypically similar to each other than to a close relative.
The Taxonomic Classification System
Taxonomy (which literally means “arrangement law”) is the science of classifying organisms to construct internationally shared classification systems with each organism placed into more and more inclusive groupings. Think about how a grocery store is organized. One large space is divided into departments, such as produce, dairy, and meats. Then each department further divides into aisles, then each aisle into categories and brands, and then finally a single product. This organization from larger to smaller, more specific categories is called a hierarchical system.
The taxonomic classification system (also called the Linnaean system after its inventor, Carl Linnaeus, a Swedish botanist, zoologist, and physician) uses a hierarchical model. Moving from the point of origin, the groups become more specific, until one branch ends as a single species. For example, after the common beginning of all life, scientists divide organisms into three large categories called a domain: Bacteria, Archaea, and Eukarya. Within each domain is a second category called a kingdom . After kingdoms, the subsequent categories of increasing specificity are: phylum , class , order , family , genus , and species (Figure 5).
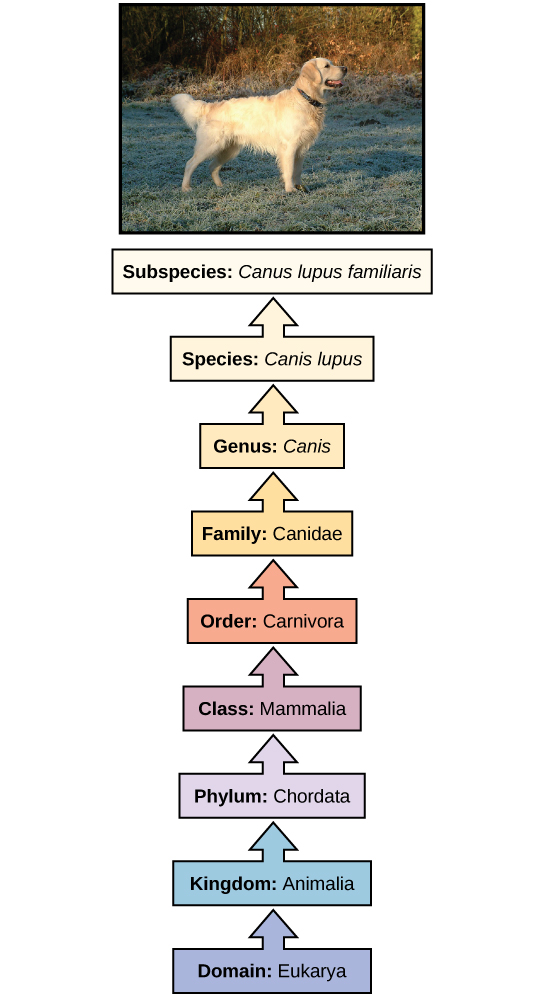
Figure 5. The taxonomic classification system uses a hierarchical model to organize living organisms into increasingly specific categories. The common dog, Canis lupus familiaris , is a subspecies of Canis lupus , which also includes the wolf and dingo. (credit “dog”: modification of work by Janneke Vreugdenhil)
The kingdom Animalia stems from the Eukarya domain. For the common dog, the classification levels would be as shown in Figure 5. Therefore, the full name of an organism technically has eight terms. For the dog, it is: Eukarya, Animalia, Chordata, Mammalia, Carnivora, Canidae, Canis, and lupus . Notice that each name is capitalized except for species, and the genus and species names are italicized. Scientists generally refer to an organism only by its genus and species, which is its two-word scientific name, in what is called binomial nomenclature . Therefore, the scientific name of the dog is Canis lupus . The name at each level is also called a taxon . In other words, dogs are in order Carnivora. Carnivora is the name of the taxon at the order level; Canidae is the taxon at the family level, and so forth. Organisms also have a common name that people typically use, in this case, dog. Note that the dog is additionally a subspecies: the “ familiaris ” in Canis lupus familiaris. Subspecies are members of the same species that are capable of mating and reproducing viable offspring, but they are considered separate subspecies due to geographic or behavioral isolation or other factors.
Figure 6 shows how the levels move toward specificity with other organisms. Notice how the dog shares a domain with the widest diversity of organisms, including plants and butterflies. At each sublevel, the organisms become more similar because they are more closely related. Historically, scientists classified organisms using characteristics, but as DNA technology developed, more precise phylogenies have been determined.
Practice Question
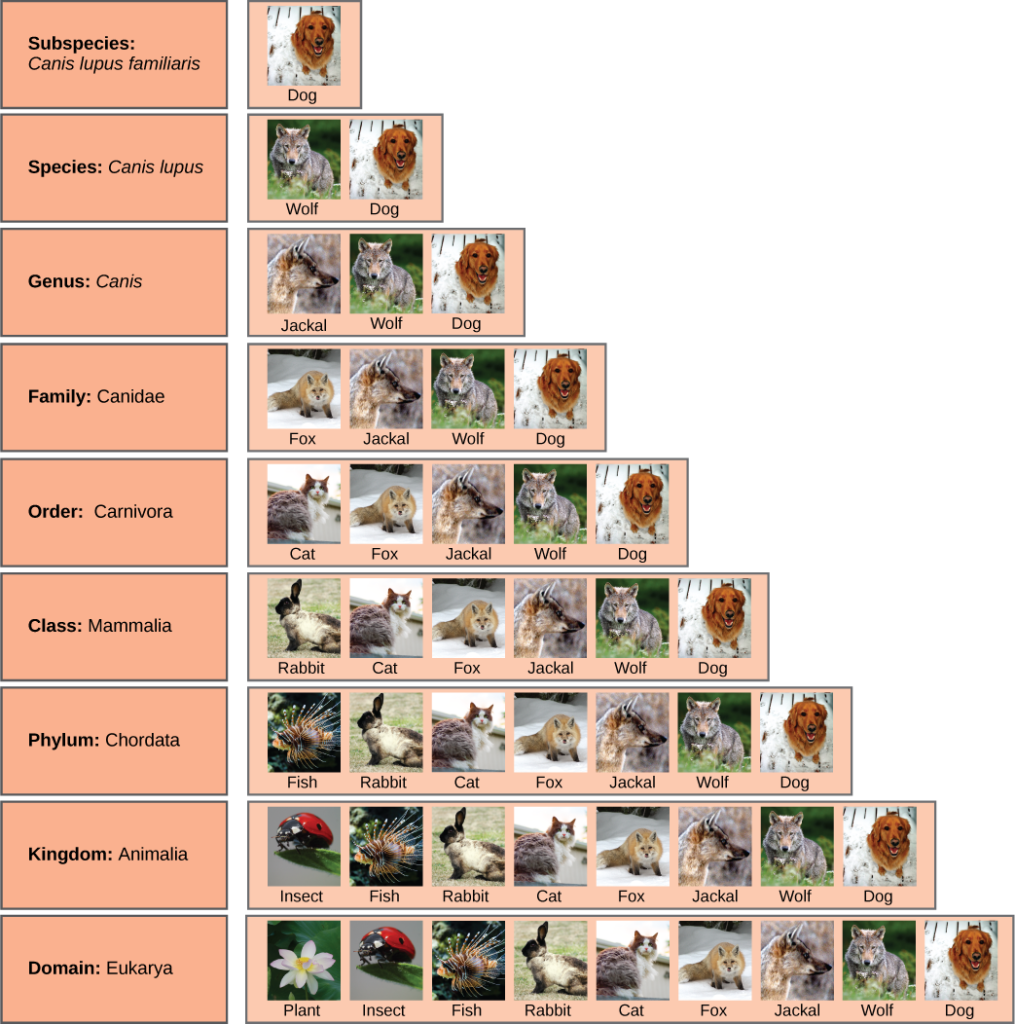
Figure 6. At each sublevel in the taxonomic classification system, organisms become more similar. Dogs and wolves are the same species because they can breed and produce viable offspring, but they are different enough to be classified as different subspecies. (credit “plant”: modification of work by “berduchwal”/Flickr; credit “insect”: modification of work by Jon Sullivan; credit “fish”: modification of work by Christian Mehlführer; credit “rabbit”: modification of work by Aidan Wojtas; credit “cat”: modification of work by Jonathan Lidbeck; credit “fox”: modification of work by Kevin Bacher, NPS; credit “jackal”: modification of work by Thomas A. Hermann, NBII, USGS; credit “wolf”: modification of work by Robert Dewar; credit “dog”: modification of work by “digital_image_fan”/Flickr)
At what levels are cats and dogs considered to be part of the same group?
Recent genetic analysis and other advancements have found that some earlier phylogenetic classifications do not align with the evolutionary past; therefore, changes and updates must be made as new discoveries occur. Recall that phylogenetic trees are hypotheses and are modified as data becomes available. In addition, classification historically has focused on grouping organisms mainly by shared characteristics and does not necessarily illustrate how the various groups relate to each other from an evolutionary perspective. For example, despite the fact that a hippopotamus resembles a pig more than a whale, the hippopotamus may be the closest living relative of the whale.
Check Your Understanding
Answer the question(s) below to see how well you understand the topics covered in the previous section. This short quiz does not count toward your grade in the class, and you can retake it an unlimited number of times.
Use this quiz to check your understanding and decide whether to (1) study the previous section further or (2) move on to the next section.
- Introduction to Phylogenetic Trees. Authored by : Shelli Carter and Lumen Learning. Provided by : Lumen Learning. License : CC BY: Attribution
- Biology. Provided by : OpenStax CNX. Located at : http://cnx.org/contents/[email protected] . License : CC BY: Attribution . License Terms : Download for free at http://cnx.org/contents/[email protected]
- 14.1: Form and Function. Provided by : CK-12. Located at : http://www.ck12.org/book/CK-12-Biology-I-Honors-CA-DTI3/section/14.1/ . License : CC BY-NC: Attribution-NonCommercial
- Phylogeny and Phylogentic Trees. Authored by : Complex Life. Located at : https://youtu.be/iyAOkzdO3vw . License : All Rights Reserved . License Terms : Standard YouTube License
If you're seeing this message, it means we're having trouble loading external resources on our website.
If you're behind a web filter, please make sure that the domains *.kastatic.org and *.kasandbox.org are unblocked.
To log in and use all the features of Khan Academy, please enable JavaScript in your browser.
AP®︎/College Biology
Course: ap®︎/college biology > unit 7.
- Taxonomy and the tree of life
- Discovering the tree of life
- Understanding and building phylogenetic trees
- Phylogenetic trees
Building a phylogenetic tree

Key points:
- Phylogenetic trees represent hypotheses about the evolutionary relationships among a group of organisms.
- A phylogenetic tree may be built using morphological (body shape), biochemical, behavioral, or molecular features of species or other groups.
- In building a tree, we organize species into nested groups based on shared derived traits (traits different from those of the group's ancestor).
- The sequences of genes or proteins can be compared among species and used to build phylogenetic trees. Closely related species typically have few sequence differences, while less related species tend to have more.
Introduction
Overview of phylogenetic trees, the idea behind tree construction, example: building a phylogenetic tree.
Feature | Lamprey | Antelope | Bald eagle | Alligator | Sea bass |
---|---|---|---|---|---|
Lungs | 0 | + | + | + | 0 |
Jaws | 0 | + | + | + | + |
Feathers | 0 | 0 | + | 0 | 0 |
Gizzard | 0 | 0 | + | + | 0 |
Fur | 0 | + | 0 | 0 | 0 |
- In the context of homework or a test, the question you are solving may tell you which traits are derived vs. ancestral.
- If you are doing your own research, you may have knowledge that allows you identify ancestral and derived traits (e.g., based on fossils).
- You may be given information about an outgroup , a species that's more distantly related to the species of interest than they are to one another.
Feature | Lamprey | Antelope | Bald eagle | Alligator | Sea bass |
---|---|---|---|---|---|
Lungs | + | + | + | 0 | |
Jaws | + | + | + | + | |
Feathers | 0 | + | 0 | 0 | |
Gizzard | 0 | + | + | 0 | |
Fur | + | 0 | 0 | 0 |
Parsimony and pitfalls in tree construction
- We may not always be able to distinguish features that reflect shared ancestry ( homologous features) from features that are similar but arose independently ( analogous features arising by convergent evolution ). See an example Imagine that the tree below shows the actual evolutionary history of a group of rodents. In this tree, whiskers arise two independent times. If we didn't know the true history of the group and were trying to reconstruct it, we might interpret the whiskers as arising from a single event. The whisker data would then conflict with data for the other traits.
- Traits can be gained and lost multiple times over the evolutionary history of a species. A species may have a derived trait, but then lose that trait (revert back to the ancestral form) over the course of evolution. See an example Imagine that the tree below shows the actual evolutionary history of a group of rodents. In this tree, species E undergoes a genetic change that causes it to lose its bushy tail and gain the skinny tail present in the group's ancestor. If we didn't know the true history of the group and were trying to reconstruct it, we might assume that the species E was descended from an ancestor without a bushy tail. Under this assumption, the tail data would conflict with data for other traits.
Using molecular data to build trees
- A larger number of differences corresponds to less related species
- A smaller number of differences corresponds to more related species
Attribution
Works cited.
- David Baum, "Reading a Phylogenetic Tree: The Meaning of Monophyletic Groups," Nature Education 1, no. 1 (2008): 190, http://www.nature.com/scitable/topicpage/reading-a-phylogenetic-tree-the-meaning-of-41956 .
Want to join the conversation?
- Upvote Button navigates to signup page
- Downvote Button navigates to signup page
- Flag Button navigates to signup page

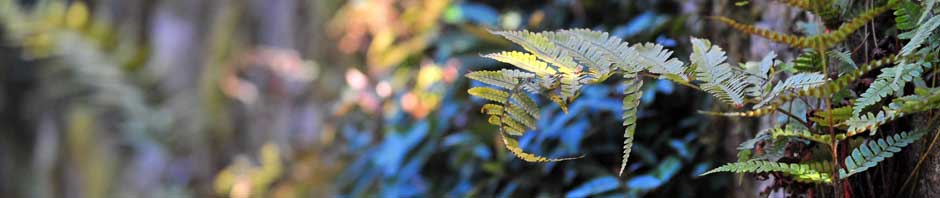
- Introduction to Ecology; Major patterns in Earth’s climate
- Behavioral Ecology
- Population Ecology 1
- Population Ecology 2
- Community Ecology 1
- Community Ecology 2
- Ecosystems 1
- Ecosystems 2
- Strong Inference
- What is life?
- What is evolution?
- Evolution by Natural Selection
- Other Mechanisms of Evolution
- Population Genetics: the Hardy-Weinberg Principle
Phylogenetic Trees
- Earth History and History of Life on Earth
- Origin of Life on Earth
- Gene expression: DNA to protein
- Gene regulation
- Cell division: mitosis and meiosis
- Mendelian Genetics
- Chromosome theory of inheritance
- Patterns of inheritance
- Chemical context for biology: origin of life and chemical evolution
- Biological molecules
- Membranes and Transport
- Energy and enzymes
- Respiration, chemiosmosis and oxidative phosphorylation
- Oxidative pathways: electrons from food to electron carriers
- Fermentation, mitochondria and regulation
- Why are plants green, and how did chlorophyll take over the world? (Converting light energy into chemical energy)
- Carbon fixation
- Recombinant DNA
- Cloning and Stem Cells
- Adaptive Immunity
- Human evolution and adaptation
Learning Objectives
- Know and use the terminology required to describe and interpret a phylogenetic tree.
- Know the different types of data incorporated into phylogenetic trees and recognize how this data is used to construct phylogenetic trees
- Interpret the relatedness of extant species based on phylogenetic trees
What is a phylogenetic tree?
A phylogenetic tree is a visual representation of the relationship between different organisms, showing the path through evolutionary time from a common ancestor to different descendants. Trees can represent relationships ranging from the entire history of life on earth, down to individuals in a population.
The diagram below shows a tree of 3 taxa (a singular taxon is a taxonomic unit; could be a species or a gene).

Terminology of phylogenetic trees
This is a bifurcating tree. The vertical lines, called branches , represent a lineage , and nodes are where they diverge, representing a speciation event from a common ancestor. The trunk at the base of the tree, is actually called the root . The root node represents the most recent common ancestor of all of the taxa represented on the tree. Time is also represented, proceeding from the oldest at the bottom to the most recent at the top. What this particular tree tells us is that taxon A and taxon B are more closely related to each other than either taxon is to taxon C. The reason is that taxon A and taxon B share a more recent common ancestor than they do with taxon C. A group of taxa that includes a common ancestor and all of its descendants is called a clade . A clade is also said to be monophyletic . A group that excludes one or more descendants is paraphyletic ; a group that excludes the common ancesto r is said to be polyphyletic.
The image below shows several monophyletic (top row) vs a polyphyletic (bottom left) or paraphyletic (bottom right) trees. Notice how the clades include the common ancestor and all of its descendants (the green and blue examples), while those labeled “not a clade” leave out some common ancestors (polyphyletic in red) or some descendants (paraphyletic in orange).
From http://evolution.berkeley.edu/evolibrary/article/side_0_0/evo_06
The video below focuses on terminology and explores some misconceptions about reading trees:
Misconceptions and how to correctly read a phylogenetic tree
Trees can be confusing to read. A common mistake is to read the tips of the trees and think their order has meaning. In the tree above, the closest relative to taxon C is not taxon B. Both A and B are equally distant from, or related to, taxon C. In fact, switching the labels of taxa A and B would result in a topologically equivalent tree. It is the order of branching along the time axis that matters. The illustration below shows that one can rotate branches and not affect the structure of the tree, much like a hanging mobile:
http://evolution.berkeley.edu/evolibrary/article/%3C?%20echo%20$baseURL;%20?%3E_0_0/evotrees_primer_08
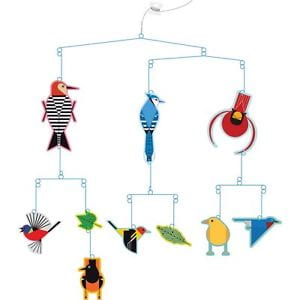
Hanging bird mobile by Charlie Harper
It can also be difficult to recognize how the trees model evolutionary relationships. One thing to remember is that any tree represents a minuscule subset of the tree of life.

Given just the 5-taxon tree (no dotted branches), it is tempting to think that taxon S is the most “primitive” or most like the common ancestor represented by the root node, because there are no additional nodes between S and the root. However, there were undoubtedly many branches off that lineage during the course of evolution, most leading to extinct taxa (99% of all species are thought to have gone extinct), and many to living taxa (like the purple dotted line) that are just not shown in the tree. What matters, then, is the total distance along the time axis (vertical axis, in this tree) – taxon S evolved for 5 million years, the same length of time as any of the other 4 taxa. As the tree is drawn, with the time axis vertical, the horizontal axis has no meaning, and serves only to separate the taxa and their lineages. So none of the currently living taxa are any more “primitive” nor any more “advanced” than any of the others; they have all evolved for the same length of time from their most recent common ancestor.
The time axis also allows us to measure evolutionary distances quantitatively. The distance between A and Q is 4 million years (A evolved for 2 million years since they split, and Q also evolved independently of A for 2 million years after the split). The distance between A and D is 6 million years, since they split from their common ancestor 3 million years ago.
Phylogenetic trees can have different forms – they may be oriented sideways, inverted (most recent at bottom), or the branches may be curved, or the tree may be radial (oldest at the center). Regardless of how the tree is drawn, the branching patterns all convey the same information: evolutionary ancestry and patterns of divergence.
This video does a great job of explaining how to interpret species relatedness using trees, including describing some of the common incorrect ways to read trees:
Constructing phylogenetic trees
Many different types of data can be used to construct phylogenetic trees, including morphological data, such as structural features, types of organs, and specific skeletal arrangements; and genetic data, such as mitochondrial DNA sequences, ribosomal RNA genes, and any genes of interest.
These types of data are used to identify homology, which means similarity due to common ancestry. This is simply the idea that you inherit traits from your parents, only applied on a species level: all humans have large brains and opposable thumbs because our ancestors did; all mammals produce milk from mammary glands because their ancestors did.
Trees are constructed on the principle of parsimony, which is the idea that the most likely pattern to is the one requiring the fewest changes. For example, it is much more likely that all mammals produce milk because they all inherited mammary glands from a common ancestor that produced milk from mammary glands, versus multiple groups of organisms each independently evolving mammary glands.
Here is an excellent resource on phylogenetic trees: https://evolution.berkeley.edu/evolibrary/article/0_0_0/evotrees_intro
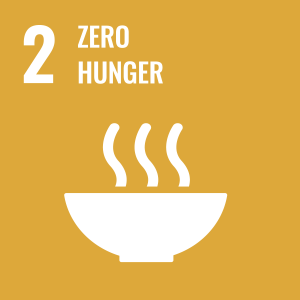
UN Sustainable Development Goal (SDG) 2: Zero Hunger – Understanding relatedness is important to the aim of ending hunger, achieving food security, and improving nutrition and sustainable agriculture. Determining the evolutionary relationships and relatedness within and between different plant species can aid in the development of sustainable agricultural practices and the preservation of crop diversity, which is essential for food security.
- Entries RSS
- Comments RSS
- Sites@GeorgiaTech
- Search for:
Creative Commons License

- eradication of Asian tiger mosquitos
- gut bacteria and stunted growth
- gut pathogens and autoimmune disease
- human milk oligosaccharides
- Legionella and mitochondria
- mannosides and uropathogenic E. coli
- origin of sickle cell mutation
- trehalose and C. difficile
- Uncategorized
- Skip to primary navigation
- Skip to main content
- Skip to primary sidebar
- Skip to footer
- Image & Use Policy
- Translations
UC MUSEUM OF PALEONTOLOGY
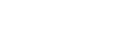
Understanding Evolution
Your one-stop source for information on evolution
Phylogenetic systematics (evolutionary trees)
Using trees for classification.
Clearly, evolutionary trees convey a lot of information about a group’s evolutionary history. Biologists are taking advantage of this by using a system of phylogenetic classification , which conveys the same sort of information that is conveyed by trees. In contrast to the traditional Linnaean system of classification , phylogenetic classification names only clades. For example, a strictly Linnaean system of classification might place the birds and the non-Avian dinosaurs into two separate groups. However, the phylogeny of these organisms reveals that the bird lineage actually branches off of the dinosaur lineage, and so, in phylogenetic classification, the birds should be considered a part of the group Dinosauria.
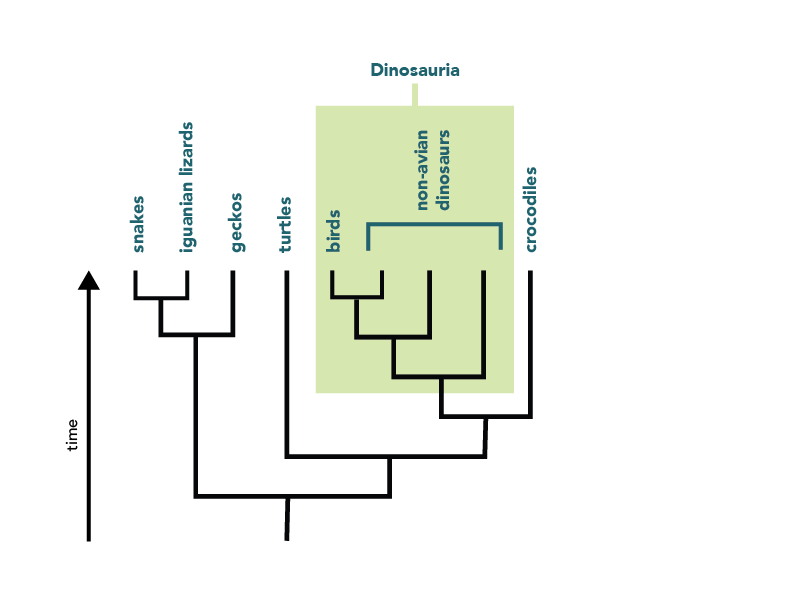
Advantages of phylogenetic classification
Phylogenetic classification has two main advantages over the Linnaean system. First, phylogenetic classification tells you something important about the organism: its evolutionary history. Second, phylogenetic classification does not attempt to “rank” organisms. Linnaean classification “ranks” groups of organisms artificially into kingdoms, phyla, orders, etc. This can be misleading as it seems to suggest that different groupings with the same rank are equivalent. For example, the cats (Felidae) and the orchids (Orchidaceae) are both family level groups in Linnaean classification. However, the two groups are not comparable:
- One has a longer history than the other. The first representatives of the cat family Felidae probably lived about 30 million years ago, while the first orchids may have lived more than 100 million years ago.
- The have different levels of diversity. There are about 35 cat species and 20,000 orchid species.
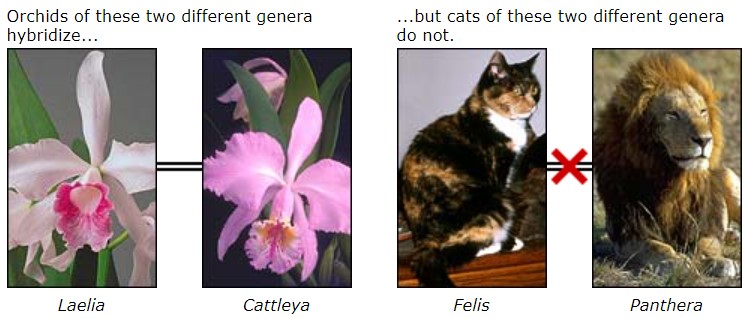
There is just no reason to think that any two identically ranked groups are comparable and by suggesting that they are, the Linnaean system is misleading. So it seems that there are many good reasons to switch to phylogenetic classification. However, organisms have been named using the Linnaean system for many hundreds of years. How are biologists making the transition to phylogenetic classification?
Switching to phylogenetic classification
Biologists deal with phylogenetic classification by de-emphasizing ranks and by reassigning names so that they are only applied to clades. This means that your use of biological names doesn’t have to change very much. In many cases, the Linnaean names are perfectly good in the phylogenetic system. For example, Aves, which is the class of birds in the Linnaean system, is also used as a phylogenetic name, since birds form a clade.
Most of the specific names that you are accustomed to using (e.g., Homo sapiens , Drosophila melanogaster ) have not changed at all with the rise of phylogenetic classification. However, there are some names from Linnaean classification that do NOT work in a phylogenetic classification. For example, the reptiles do not form a clade (and cannot be a named group in the phylogenetic system) — unless you count birds as members of Reptilia too.
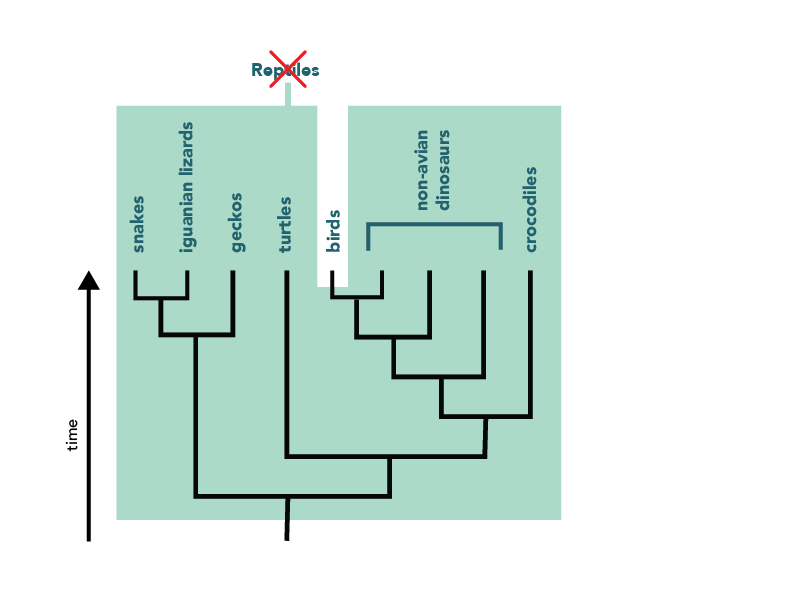
- More Details
Need a review of phylogenetic classification? See a summary of the topic in Evolution 101 .
Phylogenetic pitchforks
Reconstructing trees: Cladistics
Subscribe to our newsletter
- Teaching resource database
- Correcting misconceptions
- Conceptual framework and NGSS alignment
- Image and use policy
- Evo in the News
- The Tree Room
- Browse learning resources
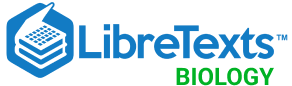
- school Campus Bookshelves
- menu_book Bookshelves
- perm_media Learning Objects
- login Login
- how_to_reg Request Instructor Account
- hub Instructor Commons
Margin Size
- Download Page (PDF)
- Download Full Book (PDF)
- Periodic Table
- Physics Constants
- Scientific Calculator
- Reference & Cite
- Tools expand_more
- Readability
selected template will load here
This action is not available.
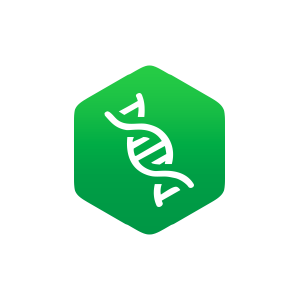
5.23: Perspectives on the Phylogenetic Tree
- Last updated
- Save as PDF
- Page ID 46114
\( \newcommand{\vecs}[1]{\overset { \scriptstyle \rightharpoonup} {\mathbf{#1}} } \)
\( \newcommand{\vecd}[1]{\overset{-\!-\!\rightharpoonup}{\vphantom{a}\smash {#1}}} \)
\( \newcommand{\id}{\mathrm{id}}\) \( \newcommand{\Span}{\mathrm{span}}\)
( \newcommand{\kernel}{\mathrm{null}\,}\) \( \newcommand{\range}{\mathrm{range}\,}\)
\( \newcommand{\RealPart}{\mathrm{Re}}\) \( \newcommand{\ImaginaryPart}{\mathrm{Im}}\)
\( \newcommand{\Argument}{\mathrm{Arg}}\) \( \newcommand{\norm}[1]{\| #1 \|}\)
\( \newcommand{\inner}[2]{\langle #1, #2 \rangle}\)
\( \newcommand{\Span}{\mathrm{span}}\)
\( \newcommand{\id}{\mathrm{id}}\)
\( \newcommand{\kernel}{\mathrm{null}\,}\)
\( \newcommand{\range}{\mathrm{range}\,}\)
\( \newcommand{\RealPart}{\mathrm{Re}}\)
\( \newcommand{\ImaginaryPart}{\mathrm{Im}}\)
\( \newcommand{\Argument}{\mathrm{Arg}}\)
\( \newcommand{\norm}[1]{\| #1 \|}\)
\( \newcommand{\Span}{\mathrm{span}}\) \( \newcommand{\AA}{\unicode[.8,0]{x212B}}\)
\( \newcommand{\vectorA}[1]{\vec{#1}} % arrow\)
\( \newcommand{\vectorAt}[1]{\vec{\text{#1}}} % arrow\)
\( \newcommand{\vectorB}[1]{\overset { \scriptstyle \rightharpoonup} {\mathbf{#1}} } \)
\( \newcommand{\vectorC}[1]{\textbf{#1}} \)
\( \newcommand{\vectorD}[1]{\overrightarrow{#1}} \)
\( \newcommand{\vectorDt}[1]{\overrightarrow{\text{#1}}} \)
\( \newcommand{\vectE}[1]{\overset{-\!-\!\rightharpoonup}{\vphantom{a}\smash{\mathbf {#1}}}} \)
Learning Objectives
- Identify different perspectives and criticisms of the phylogenetic tree
The concepts of phylogenetic modeling are constantly changing. It is one of the most dynamic fields of study in all of biology. Over the last several decades, new research has challenged scientists’ ideas about how organisms are related. New models of these relationships have been proposed for consideration by the scientific community.
Many phylogenetic trees have been shown as models of the evolutionary relationship among species. Phylogenetic trees originated with Charles Darwin, who sketched the first phylogenetic tree in 1837 (Figure 1a), which served as a pattern for subsequent studies for more than a century. The concept of a phylogenetic tree with a single trunk representing a common ancestor, with the branches representing the divergence of species from this ancestor, fits well with the structure of many common trees, such as the oak (Figure 1b). However, evidence from modern DNA sequence analysis and newly developed computer algorithms has caused skepticism about the validity of the standard tree model in the scientific community.

Limitations to the Classic Model
Classical thinking about prokaryotic evolution, included in the classic tree model, is that species evolve clonally. That is, they produce offspring themselves with only random mutations causing the descent into the variety of modern-day and extinct species known to science. This view is somewhat complicated in eukaryotes that reproduce sexually, but the laws of Mendelian genetics explain the variation in offspring, again, to be a result of a mutation within the species. The concept of genes being transferred between unrelated species was not considered as a possibility until relatively recently. Horizontal gene transfer (HGT), also known as lateral gene transfer, is the transfer of genes between unrelated species. HGT has been shown to be an ever-present phenomenon, with many evolutionists postulating a major role for this process in evolution, thus complicating the simple tree model. Genes have been shown to be passed between species which are only distantly related using standard phylogeny, thus adding a layer of complexity to the understanding of phylogenetic relationships.
The various ways that HGT occurs in prokaryotes is important to understanding phylogenies. Although at present HGT is not viewed as important to eukaryotic evolution, HGT does occur in this domain as well. Finally, as an example of the ultimate gene transfer, theories of genome fusion between symbiotic or endosymbiotic organisms have been proposed to explain an event of great importance—the evolution of the first eukaryotic cell, without which humans could not have come into existence.
Horizontal Gene Transfer
Horizontal gene transfer (HGT) is the introduction of genetic material from one species to another species by mechanisms other than the vertical transmission from parent(s) to offspring. These transfers allow even distantly related species to share genes, influencing their phenotypes. It is thought that HGT is more prevalent in prokaryotes, but that only about 2% of the prokaryotic genome may be transferred by this process. Some researchers believe such estimates are premature: the actual importance of HGT to evolutionary processes must be viewed as a work in progress. As the phenomenon is investigated more thoroughly, it may be revealed to be more common. Many scientists believe that HGT and mutation appear to be (especially in prokaryotes) a significant source of genetic variation, which is the raw material for the process of natural selection. These transfers may occur between any two species that share an intimate relationship (Table 1).
Table 1. Summary of Mechanisms of Prokaryotic and Eukaryotic HGT | |||
---|---|---|---|
Mechanism | Mode of Transmission | Example | |
transformation | DNA uptake | many prokaryotes | |
transduction | bacteriophage (virus) | bacteria | |
conjugation | pilus | many prokaryotes | |
gene transfer agents | phage-like particles | purple non-sulfur bacteria | |
from food organisms | unknown | aphid | |
jumping genes | transposons | rice and millet plants | |
epiphytes/parasites | unknown | yew tree fungi | |
from viral infections |
Genome Fusion and the Evolution of Eukaryotes
Scientists believe the ultimate in HGT occurs through genome fusion between different species of prokaryotes when two symbiotic organisms become endosymbiotic. This occurs when one species is taken inside the cytoplasm of another species, which ultimately results in a genome consisting of genes from both the endosymbiont and the host. This mechanism is an aspect of the Endosymbiont Theory, which is accepted by a majority of biologists as the mechanism whereby eukaryotic cells obtained their mitochondria and chloroplasts. However, the role of endosymbiosis in the development of the nucleus is more controversial. Nuclear and mitochondrial DNA are thought to be of different (separate) evolutionary origin, with the mitochondrial DNA being derived from the circular genomes of bacteria that were engulfed by ancient prokaryotic cells.
The nucleus-first hypothesis proposes that the nucleus evolved in prokaryotes first (Figure 2 a ), followed by a later fusion of the new eukaryote with bacteria that became mitochondria. The mitochondria-first hypothesis proposes that mitochondria were first established in a prokaryotic host (Figure 2 b ), which subsequently acquired a nucleus, by fusion or other mechanisms, to become the first eukaryotic cell. Most interestingly, the eukaryote-first hypothesis proposes that prokaryotes actually evolved from eukaryotes by losing genes and complexity (Figure 2 c ). All of these hypotheses are testable. Only time and more experimentation will determine which hypothesis is best supported by data.

Web and Network Models
The recognition of the importance of HGT, especially in the evolution of prokaryotes, has caused some to propose abandoning the classic “tree of life” model. In 1999, W. Ford Doolittle proposed a phylogenetic model that resembles a web or a network more than a tree. The hypothesis is that eukaryotes evolved not from a single prokaryotic ancestor, but from a pool of many species that were sharing genes by HGT mechanisms. As shown in Figure 3 a , some individual prokaryotes were responsible for transferring the bacteria that caused mitochondrial development to the new eukaryotes, whereas other species transferred the bacteria that gave rise to chloroplasts. This model is often called the “ web of life .” In an effort to save the tree analogy, some have proposed using the Ficus tree (Figure 3 b ) with its multiple trunks as a phylogenetic to represent a diminished evolutionary role for HGT.

Ring of Life Models

Others have proposed abandoning any tree-like model of phylogeny in favor of a ring structure, the so-called “ ring of life ” (Figure 4); a phylogenetic model where all three domains of life evolved from a pool of primitive prokaryotes. Lake, again using the conditioned reconstruction algorithm, proposes a ring-like model in which species of all three domains—Archaea, Bacteria, and Eukarya—evolved from a single pool of gene-swapping prokaryotes. His laboratory proposes that this structure is the best fit for data from extensive DNA analyses performed in his laboratory, and that the ring model is the only one that adequately takes HGT and genomic fusion into account. However, other phylogeneticists remain highly skeptical of this model.
In summary, the “tree of life” model proposed by Darwin must be modified to include HGT. Does this mean abandoning the tree model completely? Even Lake argues that all attempts should be made to discover some modification of the tree model to allow it to accurately fit his data, and only the inability to do so will sway people toward his ring proposal.
This doesn’t mean a tree, web, or a ring will correlate completely to an accurate description of phylogenetic relationships of life. A consequence of the new thinking about phylogenetic models is the idea that Darwin’s original conception of the phylogenetic tree is too simple, but made sense based on what was known at the time. However, the search for a more useful model moves on: each model serving as hypotheses to be tested with the possibility of developing new models. This is how science advances. These models are used as visualizations to help construct hypothetical evolutionary relationships and understand the massive amount of data being analyzed.
The phylogenetic tree, first used by Darwin, is the classic “tree of life” model describing phylogenetic relationships among species, and the most common model used today. New ideas about HGT and genome fusion have caused some to suggest revising the model to resemble webs or rings.
Contributors and Attributions
- Biology. Provided by : OpenStax CNX. Located at : http://cnx.org/contents/[email protected] . License : CC BY: Attribution . License Terms : Download for free at http://cnx.org/contents/[email protected]
- Original Scientific Article
- Open access
- Published: 25 September 2010
Why Trees Are Important
- Edward O. Wiley 1
Evolution: Education and Outreach volume 3 , pages 499–505 ( 2010 ) Cite this article
9721 Accesses
6 Citations
3 Altmetric
Metrics details
The Tree of Life is the result of the interplay of changes in information and speciation. Almost 100 years after publication of Darwin’s Origin , the inception of Phylogenetic Systematics has resulted in a revolution in data inference. I briefly trace the development of this revolution and show examples of how data are interpreted relative to phylogenetic trees. I then provide brief discussions of how to read tree diagrams and the need to access the quality of phylogenetic inference.
As a first principle, we adopt the Darwinian idea that all life is related. Life is diverse, being composed of many species, not one. So while there may have been only one line of descent initially, there are now many lines of descent, many “families” reproducing through time. This means that evolution is not simply “change through time,” although it certainly is that; it means, minimally, that speciation is also occurring such that lines of descent are divided by various processes into two or more lines of descent which can then follow their own, independent, evolutionary pathways. I say minimally because speciation mechanisms are diverse, sometimes two lineages found a third through other mechanisms, or one lineage spins off a new lineage through other processes. So we can conceive of the Tree of Life in nature as a diverging hierarchy of lineages composed of one or more populations with a few too many individual organisms, with most of the divergence being caused by the establishment of new lineages through speciation. Thus there are two general processes at work in evolutionary descent. One is change in information; ultimately change in the genetic code and how genes interact during development. When played out over time, this general process is termed “anagenesis” and the mechanisms include natural selection, sexual selection, and genetic drift operating on single evolving lineages. The other general process is speciation, the origin of new species. Although speciation can take many forms (various modes of speciation), these forms involve the establishment of two or more lineages where only a single lineage existed before: an ancestral species gives rise to daughter species through lineage splitting. This lineage splitting has been called “cladogenesis” and this is the origin of the term “cladist.”
Over the past 40 or so years, a revolution has occurred in the way that many biologists look at data. The revolution is fairly simple but profound. Data are interpreted relative to trees of descent which are the inferred genealogical relationships of entities linked by history. From this perspective, the data are dynamic; information changes through time, and these changes can be studied by following lines of genealogical descent. Trees can depict our hypotheses of the histories of individual organisms, populations, genes, proteins, morphological characters, developmental patterns, species, groups of species, and even areas of the Earth. In short, trees can convey our ideas of the historical relationships that exist among entities that share a common history and serve to organize and summarize where and how information has changed during historical descent.
In evolutionary biology, the more common kind of tree portrays the inferred evolutionary histories of species. They represent attempts to estimate the macroscopic properties of the Tree of Life, the genealogical nexus that ties together all of the living organisms on Earth. Such trees have been around for some time; Darwin ( 1859 ) included one as the only illustration in the Origin . However, it took more than 100 years for biologists to put together a coherent and logical methodology that allows them both to consistently estimate the Tree of Life and to estimate it in a manner that can be tested by new data in a rigorous manner. This is the “long march” from Darwin to Hennig, who proposed an integrated framework for the research program.
The Phylogenetics Revolution
From 1859, when Darwin first published a hypothetical genealogy of species, until just after World War II, there was no unified method for reconstructing the genealogical relationship among species. This is not to say there were no attempts to do so or that there were no trees; rather, that there was a lack of empirical rigor in the way those trees were formulated. For the most part, trees represented scientists’ opinions, based on their experience. Experience is frequently a good guide, but it lacks a mechanism for independent confirmation using new data from other sources or a consistent way to resolve conflicting ideas. Building on the work of such biologists as Karl Zimmerman ( 1943 ), the German entomologist Willi Hennig began synthesizing a method of reconstructing phylogenetic relationships before World War II and published his first synthesis, in German, in 1950 . This received a bit of attention (e.g., Simpson 1961 mentions the work) but was overshadowed by the “phenetics revolution” (e.g., Sokal and Michener 1958 ) in the U.S. until the publication of his second synthetic work, Phylogenetic Systematics , in English, in 1966. In the U.S., this book caught the attention of a core of future phylogeneticists lead by Gareth Nelson of the American Museum of Natural History. Hennig seems to have thought himself a Darwinian, and his method as firming up basic Darwinian principles, forging a method of reconstructing phylogenies, and bringing Darwinian principles to the classification of organisms. Hennig’s basic ideas are fairly simple.
“Relationship” in the Darwinian sense means genealogical relationship. It does not mean anything like the pre-Darwinian ideas of “similarity” or conformation to an ideal type.
Darwinian classifications are purely genealogical. Historians and biologists who think they can interpret history argue over whether Darwin advocated purely genealogical classifications. It appears he did (Ghiselin 2004 ), but how to translate that thought into a functioning system has taken over 150 years, and we are still working on it. Here are the problems. First, one had to develop a methodology to consistently reconstruct phylogeny in a way that we could argue about different hypotheses in a rigorous manner, without appeal to “authority.” This did not happen in a consistent manner until the rise of phylogenetic thinking brought on by Hennig and his advocates some 100+ years after the publication of the Darwin/Wallace thesis. We should not forget that Hennig built on the work of others, in particular Othenio Abel, Adolf Naef, and Walter Zimmermann (Willmann 2003 ). But it was left to Hennig to forge the now accepted principles of classification used by phylogeneticists today. Second, there was the pervasive idea, a holdover of pre-Darwininan thinking, that classifications could be based on similarity even at the expense of what we think we know about phylogenetic relationships. Third, a particular term, “monophyly,” was as confused as the term “homology.” Interestingly, the second problem is wrapped up in the third problem, discussed below.
“Similarity” is a complex concept requiring parsing. There is nothing wrong with similarity per se, but we must parse out similarity that denotes unique, immediate, common ancestry from similarity that denotes ancient common ancestry from similarity unrelated to common ancestry (i.e., similarity due to convergence). Homology is basically similarity due to descent of information from a common ancestor to its descendants, and sharing homologous similarities may signal unique ancestry or it may signal more ancient ancestry. For example, hair is homologous in horses and humans, and toes are homologous in horses, humans, and lizards. When we ask if horses are more closely related to humans than to lizards, we would answer “yes” because hair originated in the common ancestor of horses and humans but not in the common ancestor of all three species. When we ask if humans are more closely related to lizards than to horses because humans and lizards have multiple toes while (living) horses have only one toe, we would answer “no,” because having multiple toes is found in the common ancestor of humans, horses, and lizards, not simply in the common ancestor of humans and lizards. There is nothing wrong with the homology of human and lizard toes; it is just that this particular homology originated in an earlier ancestor, an ancestor that was common to lizards, horses, and humans. It signals a more ancient ancestor, one common to salamanders as well as lizards, horses, and humans. Since we think that the homologous similarity of having multiple digits arose once in evolutionary descent, we use it only once, at the level signaling the common ancestry of all tetrapods.
Hennig ( 1966 ) used a particular set of terms to describe homologous characters. Characters that demonstrated a unique common ancestry relative to other organisms in analysis (humans+horses versus lizards+humans) were termed apomorphic characters or apomorphic homologies. Organisms that had such characters were said to share a synapomorphy. Homologous characters that denoted a deeper relationship (humans+horses+lizards) were termed plesiomorphic characters at that level of inquiry. Lizards and humans share a symplesiomorphy, multiple digits, when we also consider horses in the mix. It is important to understand that these are relative terms. The common ancestor of all tetrapods, which includes horses, humans, lizards, salamanders, turtles, dinosaurs, etc. as well as some advanced lobe-finned fishes, is hypothesized to have multiple digits attached to legs. A more ancient common ancestor, the ancestor of tetrapods and bony fishes, had only fin rays and fins. An even more ancient ancestor, the ancestor of sharks, bony fishes, and tetrapods, also had fins with rays. Unless we are quite wrong about the relationships, we can conclude that sharks and bony fishes share the homology of having fins. Relative to having legs, the presence of fins is a symplesiomorphy of sharks and bony fishes, a “shared primitive character.” Relative to having fins, having legs is a synapomorphy of lizards, horses, and humans, a “shared advanced character.” Deeper in the phylogeny, having fins is a synapomorphy of jawed vertebrates. The ancestor of sharks, bony fishes, tetrapods, etc. is thought to have had fins. Having a relatively unmodified body wall is a plesiomorphy of lampreys and a symplesiomorphy of lampreys and hagfishes. So, apomorophy and plesiomorphy are relative terms; they describe the dynamics of character change of homologous features over the phylogeny. The unmodified body wall of lampreys was transformed by changes in information (probably using the same genes in different ways during development) to fins in some (unknown at this point) ancestor that gave rise to jawed vertebrates. Fins were transformed to limbs with multiple digits in the ancestor of tetrapods and their closest lobefin relatives, the multiple digits of mammals and early horses were transformed into the single digit we see today in the ancestor of our living species of horses.
It is also important to note that Hennig was not the first to understand this distinction. The importance of parsing homologous characters into those that denoted unique common ancestry and those that denoted more ancient common ancestry was recognized by several workers in the early half of the twentieth century. Willmann ( 2003 ) provides a detailed account of the early development of phylogenetics and points out many of the contributions of Hennig’s predecessors such as Sinai Tschulok, who provided criteria for parsing primitive and derived characters and the idea that it was the characters that are primitive and derived and not the whole organism (see Willmann 2003 and Rieppel 2010 , for discussions of Tschulok’s contributions). But it was Hennig who melded these concepts and brought them to a wider audience.
Monophyly is strict. Before Hennig, “monophyly” was applied inexactly. We had two commonly used terms, “monophyly” and “polyphyly” just as we had two terms “homology” and “convergence.” Everyone agreed that polyphyly was bad because the characters that support a polyphyletic group are known to be convergent. Mammals and birds are both warm-blooded, but they gained this character independently. Homeothermia is a class based on convergence. However, few took note of the fact that “monophyletic groups” could be based on either plesiomorphies (Pisces, with fins) or apomorphies (Tetrapods, with legs). The distinction between these two kinds of homologous characters was largely unrecognized. This created a tension: how do you justify calling a group “monophyletic?” There were no less than three reactions.
Pheneticists advocated abandoning the pursuit of phylogeny reconstruction and monophyly entirely (Sneath and Sokal 1973 ). Simply group by some measure of overall similarity and be done with it. This didn’t work for two reasons. First, pheneticists could not agree among themselves as to exactly what constituted a measure of overall similarity; there were simply too many measures from which to choose. Second, there is no standard by which one could judge the resulting classifications. Is a 70% difference the mark of one genus from another, or is it 85% dissimilarity? And, of course, there was a third reason. Who would be interested in phenograms (trees of overall similarity) when one could work with phylogenetic trees (trees of genealogy)? If we can reconstruct phylogeny, such trees are much more useful as prediction machines (see examples below) because they parse homology and convergence, which phenograms cannot accomplish.
The “old guard,” evolutionary biologists such George Simpson ( 1961 ) and Ernst Mayr advocated a hybrid system (Mayr and Bock 2002 ). Some groups are groups of unique common ancestry, but other groups can exclude some descendants of a common ancestor if they are really different. The usual criterion for “really different” was the occupation of a unique adaptive zone. For example, birds have descended from the common ancestor of reptiles and birds. But birds are really distinctive; they fill an adaptive zone much different than the adaptive zone of, say, crocodiles. So they will be placed in their own class Aves, while reptiles will be placed in the class Reptilia. Humans have their own family, Hominidae, while their relatives, the great apes, are classified in a different family, Pongidae. But, there are problems with such “half-measures.” Without even being aware of Hennig’s work, David Hull ( 1964 ) pointed out that this approach resulted in classifications that were logically inconsistent (read illogical) with the phylogenies they were supposed to summarize. Inexplicably, Hull’s conclusions were largely ignored (but see Wiley 1981 ); yet, they form the necessary and sufficient conditions for rejecting the entire “school” of evolutionary taxonomy.
Hennig’s choice, made independently of Hull’s observations, was genealogy. The problem was that the commonly used term “monophyly” was a complex term. In some cases, a monophyletic group included an (inferred) ancestral species and all of its descendants; in other cases, a monophyletic group included an (inferred) ancestral species and only some of its descendants. Groups that include an ancestral species and only some of its descendants were, to Hennig, incomplete groups. The analogy is including your cousins but not your sister in your family. Hennig called such groups as Reptilia (excludes birds) and Pongidae (excludes humans) “paraphyletic,” while he called complete groups “monophyletic.” Using Hull’s choice ( 1964 ; also see Wiley, 1981), only classifications containing monophyletic groups and only monophyletic groups were logical classifications relative to the phylogenies they represent. Only these kinds of classifications were truly “Darwinian.” This attitude was expressed as early as 1919 by Naef who advocated dissolving “stem groups” into their component branches if one wished a strictly evolutionary classification (a step Naef did not take, fearing disruption of existing classifications; see Willmann 2003 ). Classifications, it would seem, can express some ideas, but not every idea you choose: do you wish to express similarity or genealogy? Take your choice. One route leads to phenetics, the other to phylogenetics. To put it bluntly, no one would argue with a pheneticist who claimed that his similarity tree was consistent with his phenetic classification, given the pheneticist’s own criteria of grouping by a particular measure of similarity. No one would argue with a phylogeneticist whose classification contained only monophyletic groups found in his phylogenetic tree. But when you mix the two, the result is an illogical system that does not fully cover either phenomenon (see Wiley 1981 for additional discussion).
Of What Use Are Phylogenetic Trees?
As dynamic hypotheses of genealogy and character change, phylogenetic trees can be used both to describe and understand character evolution and, as devices, to predict what we do not yet know. If Theodosius Dobzhansky ( 1973 ) was correct in stating that “nothing in biology makes sense except in the light of evolution,” and if all similarities and differences among organisms are the result of the evolutionary processes of cladogenesis (lineage splitting) and anagenesis (character change), then trees should be very useful to a wider audience. Indeed, “tree thinking” is beginning to be felt in many disciplines (see Baum and Offner 2008 , for a perspective on tree thinking and the classroom). I illustrate some examples of the use of trees below. Two of these come directly from a review paper by Bull and Wichman ( 2001 ), a paper I highly recommend to educators and one that is required reading in my systematics course.
The origin of HIV in humans (from Bull and Wichman 2001 ). Retroviruses evolve and HIV is a notoriously fast evolving virus. There are actually two different forms, HIV-1 and HIV-2. By performing phylogenetic analysis on human HIV strains as well as HIV strains from a number of primate species, Gao et al. ( 1999 ) were able to demonstrate that HIV-1 was more closely related to the HIV strains in chimpanzees while Hahn et al. ( 2000 ) traced HIV-2 to the sooty mangabey monkey. Interestingly, HIV-2 is both less prevalent and less often fatal than HIV-1 in humans.
Diagnosing cancer. Abu-Asab et al. ( 2006 ) have proposed a novel way of diagnosing cancer through a combination of proteomics and phylogenetic analysis (“phyloproteomics”). The resulting phylogenetic analyses of three types of cancer (ovarian, prostate, and pancreatic) that included samples from non-cancerous individuals grouped all cancerous samples into one group, at the bottom was a healthy group or groups and in between are what Abu-Asb and colleagues call a transitional zone. This raises the exciting possibility of relatively simple diagnoses of cancers in very early stages of development since the cancers have a predictable phylogenetic position relative to healthy and cancerous samples. Note the power of using phylogenetics. Such analyses do not depend on a “magic bullet” approach to diagnosing a complex disease but rather using the history and evolution of the development of the serum proteins in cancer cells to provide a broad spectrum diagnostic tool.
Phylogenetics and the law (from Bull and Wichman 2001 ). In December 1994, the former mistress of a Louisiana physician was diagnosed with HIV and hepatitis C. She had tested negative only a few months before the diagnosis. She suspected that the physician was the source. Since he was HIV negative, the HIV had to come from another source, which turned out to be one of the physician’s patients, while another patient had hepatitis C. A phylogenetic analysis of the woman’s HIV DNA sequence clustered with another patients HIV sequence: the physician had used the tainted blood in a vitamin injection given to the mistress in August 1994. The physician is now serving a 50-year sentence for attempted murder. Just like the cancer example, the ultimate origin of the unfortunate women’s HIV viruses is not dependent on some sort of exact match with the original sample. HIV evolves rapidly enough that an exact match may or may not obtain. Rather, the outcome hinged on placing the woman’s HIV strain within the historical context of the evolution of HIV and showing the historical origin of her strain, which lay with the sample of another patient of the felonious physician.
Global climate change and the fate of species. Every species is associated with a complex set of environmental parameters that characterize its Grinnellian niche, which are essentially the general environmental parameters that allow the species to live and prosper. This niche is not some single set of parameters, such as a specific range of temperature and moisture, but a complex set of parameters than can vary geographically due to local adaptation. It can vary over time and space. Many of the broader parameters, such as maximum and minimum yearly temperature, total and seasonal rainfall, vegetation cover, and the like are those parameters subject to global climate change. Sets of these global environmental parameters can be successfully used to predict the potential niches of species and geographic information system technology can be used to project these predictions onto the surface of the Earth (for a good review, see Peterson 2003 ). This forms a prediction of where a species might potentially be found, its potential range. This is useful for all sorts of things, like prediction of the spread of invasive species. There are other uses when we consider the evolution of niches. Peterson et al. ( 1999 ) pointed out that the broader parameters of the Grinnellian niche are shared among closest taxonomic relatives. That is, these niches are conserved over speciation events and thus can be thousands, if not millions of years old and retained by the descendants of ancient ancestral species. McNyset ( 2009 ) modeled the dynamics of niche change over explicit phylogenies, demonstrating that this was not a taxonomic anomaly. The implication is clear: the broader aspects of species’ niches evolve slowly; the rate of change is slower than the speciation rate. This implies that the ability of species to adapt to phenomena such as global climate change may be very limited. We can feed the niche model of a species into a global climate change model and see where, in geographic space, the niche shifts in response to global climate change (Peterson et al. 2002 ).
Phylogenetic trees are so useful because they provide the historical narrative for explaining the similarities and differences among those entities placed on the tree. It is not so important that the DNA sequence of the HIV virus recovered from the victim exactly matches that of the former patient, what is important is that the two strains appear on the phylogenetic tree as more closely related that other HIV strains, indicating that they had a common origin. But, we must know exactly what information they convey.
The Tree of Life Versus Our Tree Hypotheses
When we draw a tree, we are attempting to capture a limited but accurate picture of the Tree of Life as it exists in nature. As such, trees are rather like highway maps that help us navigate along the path of evolutionary descent. All such trees have two things in common. First, they explicitly show ancestor and descendant relationships. Second, they all have a relative time axis. This makes them different from other kinds of graphs such as phenograms; there, the vertical axis is an axis of relative similarity, not time.
Figure 1 shows two basic kinds of tree diagrams. The one on the left (Fig. 1a ) is what I term a “stem-based tree.” The ancestral species are symbolized by the lines (technically edges or internodes) and the branching points (technically nodes or vertices) are speciation events. This diagram shows that to account for the evolution of humans, chimps, and gorillas, we need a minimum of two speciation events and a minimum of two common ancestral species. Now, it is important to understand that two speciation events and two ancestors is the minimum number of speciation events and ancestors needed to account for these three species. It does not mean that these are all the ancestors in this part of the Tree of Life. In fact, as we add fossil chimps and fossil humans to our tree, we will add additional ancestors. It is also important to note that while the ancestors may be unsampled or unrecognized as ancestral species, they are not “hypothetical” in the sense that this term is commonly applied. To assert that ancestors are hypothetical is to assert that evolutionary descent itself is hypothetical. And, the monophyletic groups to which chimp and human are parts extend back to the split between the common ancestor of all chimps and humans which occurred after the split of the common ancestor of chimps, humans and gorillas. Finally, the common ancestor of chimps and humans is neither a chimp nor a human.
A hypothesis of relationships among gorillas, chimpanzees, and humans shown as two different, but complementary, tree graphs. a A phylogenetic tree. b A Hennig tree showing the identical genealogical relationships as ( a ) in alternative form. In ( a ), each lineage is traced back to a speciation event shown at each node. In ( a ), the ancestors (X and Y) are unsampled, encompass the entire lineage between speciation events, and represent only the minimum number of ancestors needed to account for descendant lineages. In ( b ), each ancestral lineage and descendant group is folded into a single node and the arrow lines represent statements of parent–child relationships, not lineages. In ( b ), speciation events are not shown but implied by the parent–child relationships. Two hypothetical synapomorphies uniting chimps and humans are placed on each tree graph. A similar mapping is shown in Hennig ( 1966 )
The tree on the right shows exactly the same kind of relationship ancestors and descendants have, but it is organized differently. I term it a “node-based tree.” You could also term it a “Hennig tree” based on Hennig’s detailed description of the two kinds of tree he presented in Hennig ( 1966 : see p. 59, Fig. 15 and p. 60, Fig. 15). It is much more like a human genealogy, turned upside down, with ancestors at nodes connected to descendants (children) at the tips. The edges are, symbolically, explicit statements of genealogical relationship, the equivalent of parent–child statements, just like a family tree of a human family except that there is usually only one parent. So, in Fig. 1b , we would read “X is the parent of gorillas and Y.” And we would read “gorillas are the children of X.”
I have garnished both trees with two hypothetical characters that are synapomorphies shared by humans and chimps, but not gorillas. In Fig. 1a , these are attached to the ancestral lineage, but do not be misled. Just because one is lower than two does not mean that we know that one arose before two. We do not even know if both characters arose in one ancestor or in two ancestors. The only sense of the plotting of these characters on Fig. 1a is that both were characters evolved or fixed sometime before the speciation event that established the human and chimp lineages. In Fig. 1b , we see that one and two are simply listed beside the ancestor that appears in Fig. 1a as an edge rather than as a node.
I use the terms node-based and stem-based trees as usefully neutral terms. But do not be misled; they are both phylogenetic trees, and one can be converted into the other. However, one can get in trouble if they are mixed. Nodes must either be taxa or speciation events and internodes must be either taxa or statement of relationships over the entire tree. There is another way of thinking of these trees. Stem-based trees (such as Fig. 1a ) treat ancestral and descendant taxa as lineages. Node-based trees (Fig. 1b ) treat taxa as objects. Figure 1a is probably the natural way that people think about phylogenetic trees but Fig. 1b is the way computers think about objects that are analyzed.
If there were only two kinds of trees in the world, then interpretation of trees would be easy and straightforward. Alas, graph theory is much richer. Stem-based and node-based trees of the sorts discussed by Hennig ( 1966 ) are simply two kinds of acyclic graphs and acyclic graphs are simply graphs with no loops. Gene trees are acyclic graphs and gene trees do not always portray the descent of the species of which the genes are a part. Phenetic trees (phenograms) are acyclic graphs. Cladograms are acyclic graphs usually thought of as common ancestry trees. Figures drawn by Louis Agassiz in the 1840s look very much like those drawn later by Romer. Yet, they are not meant to represent evolutionary descent (Agassiz rejected evolution). Imposing an evolutionary interpretation on an acyclic graph that is not meant to portray evolutionary descent is a category mistake; yet, the graphs may take exactly the same form. Thus, we must exercise caution: we must know the intention of the graph, what it is meant to portray; we cannot divine it purely from the form. There are other problems, relatively minor but vexing in our quest for full understanding of the diagrams we draw and the evolutionary biology they are meant to document. For example, when Baum et al. ( 2005 ) mark ancestral species at the nodes of their tree, do we assume that their tree is a node-based tree, as in Fig. 1b without the mark of the “circle” convention? Surely this must be so, for in a stem-based tree, a node (branch point) is an event (speciation) and not a thing (ancestral species). Fortunately, this should not cause major problems in interpretation of the relationships of descendants, but they are relevant to meaning. Ancestral species do not exist on a stem-based tree at nodes; they exist between nodes (Hennig 1966 ). Descent species may or may not exist only at tips on a phylogenetic tree, but the lineage to which they belong has existed since the speciation event that founded the edge that connects them to their closest analyzed relative. And, we have no idea how many other species join that edge until we have a full account of the diversity represented by that edge. In the chimp–human case, there are a number of other lineages that join along both edges. But, if we accept the hypothesis of chimp+human as opposed to chimp+gorilla, the tree is still accurate in giving an account of relative relationships among the organisms analyzed. Such graphs may be accurate in a relative sense without having to be accurate in an absolute sense. The analogy to a highway map is apt. Highway maps may not show all the intersections, but the intersection they do show must be accurately drawn.
Assessing Tree Quality
Robustness in phylogenetic inference refers to how well methods work in the face of violations of the assumption of the method or model used in an analysis. A robust tree would be one that is relatively immune to violations of the assumptions used to generate the tree hypothesis and might be expected to stand the test of new data, perhaps analyzed using different methods. Hopefully, a robust tree is an accurate tree. Phylogeneticists have put a great deal of effort in exploring how violations of assumption affect the results of an analysis (for example, Holder et al. 2008 ), and I will not review that extensive literature here. Suffice to say, how robust a phylogenetic tree needs to be depends on the use to which it is put. If the goal is to convict a physician of second-degree murder, then we require a very robust tree that is likely to closely estimate the actual descent of HIV strains. If the goal is to estimate rates of speciation, then not only must the tree be a robust estimate of the Tree of Life but it must also be populated by a significant number of species of the group. Every missing species represents an underestimation of speciation events. If the goal is to use the tree to forecast the potential distribution of an invasive species based on the ecological niche of it and its nearest relatives, then the result could influence policy decision on a national or international level. The major point is that before using a tree, one should access the relative strength of the hypothesis, and the greater the consequences, the more closely we should question the strength of the tree hypothesis. We must remind ourselves that tree hypotheses, like all scientific hypotheses, are conjectures, not facts.
Abu-Asab M, Chaouchi M, Amri H. Phyloproteomics: What phylogenetic analysis reveals about serum proteomics. J Proteome Res. 2006;5:2236–40.
Article CAS Google Scholar
Baum DA, Offner S. Phylogenies and tree thinking. Am Biol Teach. 2008;70:222–9.
Google Scholar
Baum DA, DeWitt Smith S, Donovan SS. The tree thinking challenge. Science. 2005;310:979–80.
Bull JJ, Wichman HA. Applied evolution. Annu Rev Evol Syst. 2001;32:183–217.
Article Google Scholar
Darwin C. On the origin of species by means of natural selection; or the preservation of favored races in the struggle for life (Reprinted 1st edition). Cambridge: Harvard University Press; 1859.
Dobzhansky T. Nothing in biology makes sense except in the light of evolution. Am Biol Teach. 1973;35:125–9.
Gao F, Bailes E, Robertson DL, Chen Y, Rodenburg CM, et al. Origin of HIV-1 in the chimpanzee Pan troglodytes troglodytes . Nature. 1999;397:436–41.
Ghiselin MT. Mayr and Bock versus Darwin on genealogical classification. J Zool Syst Evol Res. 2004;42:165–9.
Hahn BH, Shaw GM, De Cock KM, Sharp PM. AIDS as a zoonosis: scientific and public health implications. Science. 2000;287:607–14.
Hennig W. Grundzuge einer Theorie der phylogenetischen Systematik. Berlin: Deutscher Zentralverlag; 1950.
Hennig W. Phylogenetic systematics. Urbana: University of Illinois Press; 1966.
Holder MT, Zwickl DJ, Dessimoz C. Evaluating the robustness of phylogenetic methods to among-site variability in substitution processes. Philos Trans R Soc B Biol Sci. 2008;363:4013–2.
Hull DL. Consistency and Monophyly. Syst Zool. 1964;13:1–11.
Mayr E, Bock WJ. Classification and other ordering systems. J Zool Syst Evol Res. 2002;40:169–94.
McNyset KM. Ecological niche conservatism in North American freshwater fishes. Biol J Linn Soc. 2009;96:282–95.
Peterson AT. Predicting the geography of species’ invasions via ecological niche modeling. Quart Rev Biol. 2003;78:419–33.
Peterson A, Soberón TJ, Sanchez-Cordero V. Conservation of ecological niches in evolutionary time. Science. 1999;285:1265–7.
Peterson AT, Ortega-Huerta MA, Bartley J, Sanchez-Cordero V, Soberon J, Buddemeier RH, et al. Future projections for Mexican faunas under global climate change scenarios. Nature. 2002;416:626–9.
Rieppel O. Sinai Tschulok (1875-1945)—a pioneer of cladistics. Cladistics. 2010;26:103–11.
Simpson GG. The principles of animal taxonomy. New York: Columbia University Press; 1961.
Sneath PHA, Sokal RR. Numerical taxonomy. San Francisco: Freeman; 1973.
Sokal RR, Michener CD. A statistical method for evaluating systematic relationships. Univ Kans Sci Bull. 1958;38:1409–38.
Wiley EO. Convex groups and consistent classifications. Syst Bot. 1981;6:346–58.
Willmann R. From Haeckel to Hennig: the early development of phylogenetics in German-speaking Europe. Cladistics. 2003;19:449–79.
Zimmermann W. Die Methoden der Phylogenetik. In: Henberer G, editor. Dei Evolution der Organismsn 1, Aulf G. Jena: Justav Fisher; 1943. p. 20–56.
Download references
Acknowledgements
My thanks to Dr. Daniel R. Brooks (University of Toronto) for inviting me to participate in this special issue and to Teresa E. McDonald (University of Kansas) for her discussions of understanding of public awareness of the meaning of phylogenetic trees in educational and museum settings. This paper is supported by NSF DEB 0732819, the Euteleost Tree of Life project, which includes an educational component designed to increase understanding of phylogenetic trees.
Author information
Authors and affiliations.
Ecology and Evolutionary Biology and Biodiversity Institute, University of Kansas, Lawrence, KS, 66045, USA
Edward O. Wiley
You can also search for this author in PubMed Google Scholar
Corresponding author
Correspondence to Edward O. Wiley .
Rights and permissions
Open Access This is an open access article distributed under the terms of the Creative Commons Attribution Noncommercial License ( https://creativecommons.org/licenses/by-nc/2.0 ), which permits any noncommercial use, distribution, and reproduction in any medium, provided the original author(s) and source are credited.
Reprints and permissions
About this article
Cite this article.
Wiley, E.O. Why Trees Are Important. Evo Edu Outreach 3 , 499–505 (2010). https://doi.org/10.1007/s12052-010-0279-0
Download citation
Published : 25 September 2010
Issue Date : December 2010
DOI : https://doi.org/10.1007/s12052-010-0279-0
Share this article
Anyone you share the following link with will be able to read this content:
Sorry, a shareable link is not currently available for this article.
Provided by the Springer Nature SharedIt content-sharing initiative
- Phylogenetic systematics
- Phylogenetic trees
Evolution: Education and Outreach
ISSN: 1936-6434
- Submission enquiries: [email protected]
Information
- Author Services
Initiatives
You are accessing a machine-readable page. In order to be human-readable, please install an RSS reader.
All articles published by MDPI are made immediately available worldwide under an open access license. No special permission is required to reuse all or part of the article published by MDPI, including figures and tables. For articles published under an open access Creative Common CC BY license, any part of the article may be reused without permission provided that the original article is clearly cited. For more information, please refer to https://www.mdpi.com/openaccess .
Feature papers represent the most advanced research with significant potential for high impact in the field. A Feature Paper should be a substantial original Article that involves several techniques or approaches, provides an outlook for future research directions and describes possible research applications.
Feature papers are submitted upon individual invitation or recommendation by the scientific editors and must receive positive feedback from the reviewers.
Editor’s Choice articles are based on recommendations by the scientific editors of MDPI journals from around the world. Editors select a small number of articles recently published in the journal that they believe will be particularly interesting to readers, or important in the respective research area. The aim is to provide a snapshot of some of the most exciting work published in the various research areas of the journal.
Original Submission Date Received: .
- Active Journals
- Find a Journal
- Proceedings Series
- For Authors
- For Reviewers
- For Editors
- For Librarians
- For Publishers
- For Societies
- For Conference Organizers
- Open Access Policy
- Institutional Open Access Program
- Special Issues Guidelines
- Editorial Process
- Research and Publication Ethics
- Article Processing Charges
- Testimonials
- Preprints.org
- SciProfiles
- Encyclopedia
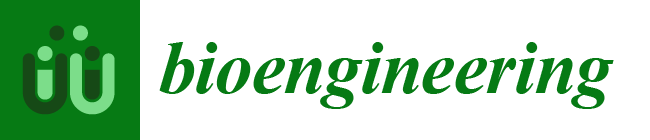
Article Menu
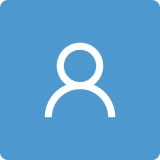
- Subscribe SciFeed
- Recommended Articles
- Author Biographies
- PubMed/Medline
- Google Scholar
- on Google Scholar
- Table of Contents
Find support for a specific problem in the support section of our website.
Please let us know what you think of our products and services.
Visit our dedicated information section to learn more about MDPI.
JSmol Viewer
Common methods for phylogenetic tree construction and their implementation in r.

Graphical Abstract
1. Introduction
2. the popular methods for inferring phylogenetic trees, 2.1. distance-based method, 2.2. maximum parsimony (mp) method, 2.3. maximum likelihood (ml) method, 2.4. bayesian inference (bi) method, 3. advanced computational integrative methods for inferring phylogenetic tree, 3.1. concatenation phylogeny method, 3.2. coalescence phylogeny method, 4. construction and evaluation of phylogenetic trees in r language environment, 4.1. implementation of distance-based methods in r, 4.2. implementation of mp method in r, 4.3. implementation of ml method in r, 4.4. implementation of bi method in r, 4.5. building the consensus phylogenetic tree using multiple genes in r, 5. summary and perspectives.
R Package | Description | Source | Reference |
---|---|---|---|
ape | Providing both utility functions for reading and writing data and manipulating phylogenetic trees, as well as several advanced methods for phylogenetic and evolutionary analysis. | CRAN * | [ ] |
phangorn | Estimating phylogenetic trees and networks using maximum likelihood, maximum parsimony, distance methods, and Hadamard conjugation; offering methods for tree comparison, model selection, and visualization of phylogenetic networks. | CRAN * | [ ] |
babette | Providing an alternative workflow to the BEAST2; conducting complex Bayesian phylogenetics easily and reproducibly from R. | Github | [ ] |
BAMMtools | Reconstructing and visualizing changes in evolutionary rates through time and across clades in a Bayesian statistical framework. | CRAN * | [ ] |
apex | Implementing new object classes for storing and handling multiple genes data. | CRAN * | [ ] |
phytools | Concentrating on phylogenetic comparative biology; including numerous techniques for visualizing, analyzing, manipulating, reading or writing, and inferring phylogenetic trees. | CRAN * | [ ] |
ggtree | Annotating phylogenetic trees with their associated data of different types and from various sources. | Bioconductor | [ ] |
RPANDA | Characterizing and comparing phylogenies using spectral densities; fitting models of diversification to phylogenies. | CRAN * | [ ] |
TreeSearch | Dataset construction and validation; phylogenetic search (including with inapplicable data); the interrogation of optimal tree sets. | CRAN * | [ ] |
paleotree | Analyzing the combined paleontological and phylogenetic data sets, particularly the time-scaling of phylogenetic trees, which include extinct fossil lineages. | CRAN * | [ ] |
treeman | Containing a new class called TreeMan for representing phylogenetic trees that has a list structure that allows for more efficient manipulation of phylogenetic trees; demonstrating intuitive tree manipulation, both conceptually and as computationally efficient as possible, within the R environment. | Github | [ ] |
Author Contributions
Data availability statement, acknowledgments, conflicts of interest.
- Sanderson, M.J.; Driskell, A.C. The challenge of constructing large phylogenetic trees. Trends Plant Sci. 2003 , 8 , 374–379. [ Google Scholar ] [ CrossRef ] [ PubMed ]
- Hug, L.A.; Baker, B.J.; Anantharaman, K.; Brown, C.T.; Probst, A.J.; Castelle, C.J.; Butterfield, C.N.; Hernsdorf, A.W.; Amano, Y.; Ise, K.; et al. A new view of the tree of life. Nat. Microbiol. 2016 , 1 , 16048. [ Google Scholar ] [ CrossRef ] [ PubMed ]
- Abaza, S. What is and why do we have to know the phylogenetic tree? Parasitol. United J. 2020 , 13 , 68–71. [ Google Scholar ] [ CrossRef ]
- de Queiroz, K. Nodes, branches, and phylogenetic definitions. Syst. Biol. 2013 , 62 , 625–632. [ Google Scholar ] [ CrossRef ] [ PubMed ]
- Dissanayake, A.; Bhunjun, C.; Maharachchikumbura, S.; Liu, J. Applied aspects of methods to infer phylogenetic relationships amongst fungi. Mycosphere 2020 , 11 , 2652–2676. [ Google Scholar ] [ CrossRef ]
- Gupta, M.K.; Gouda, G.; Sabarinathan, S.; Donde, R.; Rajesh, N.; Pati, P.; Rathore, S.K.; Behera, L.; Vadde, R. Phylogenetic analysis. In Bioinformatics in Rice Research: Theories and Techniques ; Springer: Singapore, 2021; pp. 179–207. [ Google Scholar ]
- Feng, H.; Liu, M.; Wang, B.; Feng, J.; Han, J.; Liu, J. HCPC: A New Parsimonious Clustering Method based on Hierarchical Characters for Morphological Phylogenetic Reconstruction. Res. Sq. 2021 . [ Google Scholar ] [ CrossRef ]
- Mc, C.E.; Verdeflor, L.; Weinsztok, A.; Wiles, J.R.; Dorus, S. Exploratory Activities for Understanding Evolutionary Relationships Depicted by Phylogenetic Trees: United but Diverse. Am. Biol. Teach. 2020 , 82 , 333–337. [ Google Scholar ] [ CrossRef ]
- Jetz, W.; Thomas, G.H.; Joy, J.B.; Hartmann, K.; Mooers, A.O. The global diversity of birds in space and time. Nature 2012 , 491 , 444–448. [ Google Scholar ] [ CrossRef ]
- Hinchliff, C.E.; Smith, S.A.; Allman, J.F.; Burleigh, J.G.; Chaudhary, R.; Coghill, L.M.; Crandall, K.A.; Deng, J.; Drew, B.T.; Gazis, R.; et al. Synthesis of phylogeny and taxonomy into a comprehensive tree of life. Proc. Natl. Acad. Sci. USA 2015 , 112 , 12764–12769. [ Google Scholar ] [ CrossRef ]
- Denamur, E.; Clermont, O.; Bonacorsi, S.; Gordon, D. The population genetics of pathogenic Escherichia coli. Nat. Rev. Microbiol. 2021 , 19 , 37–54. [ Google Scholar ] [ CrossRef ]
- Smith, S.D.; Pennell, M.W.; Dunn, C.W.; Edwards, S.V. Phylogenetics is the New Genetics (for Most of Biodiversity). Trends Ecol. Evol. 2020 , 35 , 415–425. [ Google Scholar ] [ CrossRef ]
- Lee, M.S.; Palci, A. Morphological Phylogenetics in the Genomic Age. Curr. Biol. CB 2015 , 25 , R922–R929. [ Google Scholar ] [ CrossRef ] [ PubMed ]
- Lemmon, E.M.; Lemmon, A.R. High-throughput genomic data in systematics and phylogenetics. Annu. Rev. Ecol. Evol. Syst. 2013 , 44 , 99–121. [ Google Scholar ] [ CrossRef ]
- Morel, B.; Williams, T.A.; Stamatakis, A. Asteroid: A new algorithm to infer species trees from gene trees under high proportions of missing data. Bioinformatics 2023 , 39 , btac832. [ Google Scholar ] [ CrossRef ]
- James, T.Y.; Stajich, J.E.; Hittinger, C.T.; Rokas, A. Toward a Fully Resolved Fungal Tree of Life. Annu. Rev. Microbiol. 2020 , 74 , 291–313. [ Google Scholar ] [ CrossRef ] [ PubMed ]
- Ashkenazy, H.; Sela, I.; Levy Karin, E.; Landan, G.; Pupko, T. Multiple Sequence Alignment Averaging Improves Phylogeny Reconstruction. Syst. Biol. 2019 , 68 , 117–130. [ Google Scholar ] [ CrossRef ]
- Francis, W.R.; Canfield, D.E. Very few sites can reshape the inferred phylogenetic tree. PeerJ 2020 , 8 , e8865. [ Google Scholar ] [ CrossRef ] [ PubMed ]
- Talavera, G.; Castresana, J. Improvement of phylogenies after removing divergent and ambiguously aligned blocks from protein sequence alignments. Syst. Biol. 2007 , 56 , 564–577. [ Google Scholar ] [ CrossRef ] [ PubMed ]
- Williams, T.A.; Heaps, S.E. An introduction to phylogenetics and the tree of life. In Methods in Microbiology ; Elsevier: Amsterdam, The Netherlands, 2014; Volume 41, pp. 13–44. [ Google Scholar ]
- Desper, R.; Gascuel, O. Theoretical foundation of the balanced minimum evolution method of phylogenetic inference and its relationship to weighted least-squares tree fitting. Mol. Biol. Evol. 2004 , 21 , 587–598. [ Google Scholar ] [ CrossRef ]
- Wang, Z.; Sun, J.; Gao, Y.; Xue, Y.; Zhang, Y.; Li, K.; Zhang, W.; Zhang, C.; Zu, J.; Zhang, L. Fusang: A framework for phylogenetic tree inference via deep learning. Nucleic Acids Res. 2023 , 51 , 10909–10923. [ Google Scholar ] [ CrossRef ]
- Balaban, M.; Jiang, Y.; Roush, D.; Zhu, Q.; Mirarab, S. Fast and accurate distance-based phylogenetic placement using divide and conquer. Mol. Ecol. Resour. 2022 , 22 , 1213–1227. [ Google Scholar ] [ CrossRef ] [ PubMed ]
- Vaz, C.; Nascimento, M.; Carriço, J.A.; Rocher, T.; Francisco, A.P. Distance-based phylogenetic inference from typing data: A unifying view. Brief. Bioinform. 2021 , 22 , bbaa147. [ Google Scholar ] [ CrossRef ] [ PubMed ]
- Coorens, T.H.; Spencer Chapman, M.; Williams, N.; Martincorena, I.; Stratton, M.R.; Nangalia, J.; Campbell, P.J. Reconstructing phylogenetic trees from genome-wide somatic mutations in clonal samples. Nat. Protoc. 2024 , 1–21. [ Google Scholar ] [ CrossRef ] [ PubMed ]
- Scossa, F.; Fernie, A.R. Ancestral sequence reconstruction—An underused approach to understand the evolution of gene function in plants? Comput. Struct. Biotechnol. J. 2021 , 19 , 1579–1594. [ Google Scholar ] [ CrossRef ] [ PubMed ]
- Ojha, K.K.; Mishra, S.; Singh, V.K. Computational molecular phylogeny: Concepts and applications. In Bioinformatics ; Academic Press: New York, NY, USA, 2022; pp. 67–89. [ Google Scholar ]
- Kapli, P.; Yang, Z.; Telford, M.J. Phylogenetic tree building in the genomic age. Nat. Rev. Genet. 2020 , 21 , 428–444. [ Google Scholar ] [ CrossRef ] [ PubMed ]
- Mount, D.W. Distance methods for phylogenetic prediction. CSH Protoc. 2008 , 2008 , pdb.top33. [ Google Scholar ] [ CrossRef ] [ PubMed ]
- Davidson, R.; Martín Del Campo, A. Combinatorial and Computational Investigations of Neighbor-Joining Bias. Front. Genet. 2020 , 11 , 584785. [ Google Scholar ] [ CrossRef ] [ PubMed ]
- Saitou, N.; Nei, M. The neighbor-joining method: A new method for reconstructing phylogenetic trees. Mol. Biol. Evol. 1987 , 4 , 406–425. [ Google Scholar ] [ CrossRef ] [ PubMed ]
- Kuhner, M.K.; Felsenstein, J. A simulation comparison of phylogeny algorithms under equal and unequal evolutionary rates. Mol. Biol. Evol. 1994 , 11 , 459–468. [ Google Scholar ] [ CrossRef ]
- Godini, R.; Fallahi, H. A brief overview of the concepts, methods and computational tools used in phylogenetic tree construction and gene prediction. Meta Gene 2019 , 21 , 100586. [ Google Scholar ] [ CrossRef ]
- Tamura, K.; Nei, M.; Kumar, S. Prospects for inferring very large phylogenies by using the neighbor-joining method. Proc. Natl. Acad. Sci. USA 2004 , 101 , 11030–11035. [ Google Scholar ] [ CrossRef ]
- Zhang, L.-N.; Rong, C.-H.; He, Y.; Guan, Q.; He, B.; Zhu, X.-W.; Liu, J.-N.; Chen, H.-J. A bird’s eye view of the algorithms and software packages for reconstructing phylogenetic trees. Zool. Res. 2013 , 34 , 640–650. [ Google Scholar ] [ CrossRef ]
- Santiago-Alarcon, D.; Tapia-McClung, H.; Lerma-Hernández, S.; Venegas-Andraca, S.E. Quantum aspects of evolution: A contribution towards evolutionary explorations of genotype networks via quantum walks. J. R. Soc. Interface 2020 , 17 , 20200567. [ Google Scholar ] [ CrossRef ]
- Farris, J.S. Methods for computing Wagner trees. Syst. Biol. 1970 , 19 , 83–92. [ Google Scholar ] [ CrossRef ]
- Fitch, W.M. Toward defining the course of evolution: Minimum change for a specific tree topology. Syst. Biol. 1971 , 20 , 406–416. [ Google Scholar ] [ CrossRef ]
- Liu, D.K.; Tu, X.D.; Zhao, Z.; Zeng, M.Y.; Zhang, S.; Ma, L.; Zhang, G.Q.; Wang, M.M.; Liu, Z.J.; Lan, S.R.; et al. Plastid phylogenomic data yield new and robust insights into the phylogeny of Cleisostoma-Gastrochilus clades (Orchidaceae, Aeridinae). Mol. Phylogenetics Evol. 2020 , 145 , 106729. [ Google Scholar ] [ CrossRef ] [ PubMed ]
- Azouri, D.; Abadi, S.; Mansour, Y.; Mayrose, I.; Pupko, T. Harnessing machine learning to guide phylogenetic-tree search algorithms. Nat. Commun. 2021 , 12 , 1983. [ Google Scholar ] [ CrossRef ] [ PubMed ]
- Felsenstein, J. Evolutionary trees from DNA sequences: A maximum likelihood approach. J. Mol. Evol. 1981 , 17 , 368–376. [ Google Scholar ] [ CrossRef ]
- Jukes, T.H.; Cantor, C.R. Evolution of protein molecules. Mamm. Protein Metab. 1969 , 3 , 21–132. [ Google Scholar ]
- Wascher, M.; Kubatko, L. Consistency of SVDQuartets and Maximum Likelihood for Coalescent-Based Species Tree Estimation. Syst. Biol. 2021 , 70 , 33–48. [ Google Scholar ] [ CrossRef ]
- Kimura, M. A simple method for estimating evolutionary rates of base substitutions through comparative studies of nucleotide sequences. J. Mol. Evol. 1980 , 16 , 111–120. [ Google Scholar ] [ CrossRef ] [ PubMed ]
- Tamura, K.; Nei, M. Estimation of the number of nucleotide substitutions in the control region of mitochondrial DNA in humans and chimpanzees. Mol. Biol. Evol. 1993 , 10 , 512–526. [ Google Scholar ] [ CrossRef ] [ PubMed ]
- Hasegawa, M.; Kishino, H.; Yano, T. Dating of the human-ape splitting by a molecular clock of mitochondrial DNA. J. Mol. Evol. 1985 , 22 , 160–174. [ Google Scholar ] [ CrossRef ] [ PubMed ]
- Tavaré, S. Some probabilistic and statistical problems on the analysis of DNA sequence. Lect. Math. Life Sci. 1986 , 17 , 57. [ Google Scholar ]
- Jacob, S.S.; Sengupta, P.P.; Chandu, A.G.S.; Shamshad, S.; Yogisharadhya, R.; Sudhagar, S.; Ramesh, P. Existence of genetic lineages within Asian genotype of Taenia solium-Genetic characterization based on mitochondrial and ribosomal DNA markers. Transbound. Emerg. Dis. 2022 , 69 , 2256–2265. [ Google Scholar ] [ CrossRef ]
- Heaps, S.E.; Nye, T.M.; Boys, R.J.; Williams, T.A.; Embley, T.M. Bayesian modelling of compositional heterogeneity in molecular phylogenetics. Stat. Appl. Genet. Mol. Biol. 2014 , 13 , 589–609. [ Google Scholar ] [ CrossRef ] [ PubMed ]
- Amiroch, S.; Pradana, M.S.; Irawan, M.I.; Mukhlash, I. Maximum Likelihood Method on The Construction of Phylogenetic Tree for Identification the Spreading of SARS Epidemic. In Proceedings of the 2018 International Symposium on Advanced Intelligent Informatics (SAIN), Yogyakarta, Indonesia, 29–30 August 2018; pp. 137–141. [ Google Scholar ]
- Rannala, B.; Yang, Z. Probability distribution of molecular evolutionary trees: A new method of phylogenetic inference. J. Mol. Evol. 1996 , 43 , 304–311. [ Google Scholar ] [ CrossRef ] [ PubMed ]
- Flouri, T.; Huang, J.; Jiao, X.; Kapli, P.; Rannala, B.; Yang, Z. Bayesian Phylogenetic Inference using Relaxed-clocks and the Multispecies Coalescent. Mol. Biol. Evol. 2022 , 39 , msac161. [ Google Scholar ] [ CrossRef ] [ PubMed ]
- Nascimento, F.F.; Reis, M.D.; Yang, Z. A biologist’s guide to Bayesian phylogenetic analysis. Nat. Ecol. Evol. 2017 , 1 , 1446–1454. [ Google Scholar ] [ CrossRef ]
- Cornuault, J.; Sanmartín, I. A road map for phylogenetic models of species trees. Mol. Phylogenetics Evol. 2022 , 173 , 107483. [ Google Scholar ] [ CrossRef ]
- Spade, D.A. Geometric ergodicity of a Metropolis-Hastings algorithm for Bayesian inference of phylogenetic branch lengths. Comput. Stat. 2020 , 35 , 2043–2076. [ Google Scholar ] [ CrossRef ]
- Csősz, S.; Loss, A.C.; Fisher, B.L. Exploring the diversity of the Malagasy Ponera (Hymenoptera: Formicidae) fauna via integrative taxonomy. Org. Divers. Evol. 2023 , 23 , 917–927. [ Google Scholar ] [ CrossRef ]
- Larget, B.; Simon, D.L. Markov Chasin Monte Carlo Algorithms for the Bayesian Analysis of Phylogenetic Trees. Mol. Biol. Evol. 1999 , 16 , 750. [ Google Scholar ] [ CrossRef ]
- Whidden, C.; Matsen, F.A.t. Quantifying MCMC exploration of phylogenetic tree space. Syst. Biol. 2015 , 64 , 472–491. [ Google Scholar ] [ CrossRef ]
- Inagaki, Y.; Nakajima, Y.; Sato, M.; Sakaguchi, M.; Hashimoto, T. Gene sampling can bias multi-gene phylogenetic inferences: The relationship between red algae and green plants as a case study. Mol. Biol. Evol. 2009 , 26 , 1171–1178. [ Google Scholar ] [ CrossRef ]
- Lax, G.; Kolisko, M.; Eglit, Y.; Lee, W.J.; Yubuki, N.; Karnkowska, A.; Leander, B.S.; Burger, G.; Keeling, P.J.; Simpson, A.G.B. Multigene phylogenetics of euglenids based on single-cell transcriptomics of diverse phagotrophs. Mol. Phylogenetics Evol. 2021 , 159 , 107088. [ Google Scholar ] [ CrossRef ]
- Kanzi, A.M.; Trollip, C.; Wingfield, M.J.; Barnes, I.; Van der Nest, M.A.; Wingfield, B.D. Phylogenomic incongruence in Ceratocystis: A clue to speciation? BMC Genom. 2020 , 21 , 362. [ Google Scholar ] [ CrossRef ] [ PubMed ]
- Williams, T.A.; Cox, C.J.; Foster, P.G.; Szöllősi, G.J.; Embley, T.M. Phylogenomics provides robust support for a two-domains tree of life. Nat. Ecol. Evol. 2020 , 4 , 138–147. [ Google Scholar ] [ CrossRef ]
- Pardo-De la Hoz, C.J.; Magain, N.; Piatkowski, B.; Cornet, L.; Dal Forno, M.; Carbone, I.; Miadlikowska, J.; Lutzoni, F. Ancient Rapid Radiation Explains Most Conflicts Among Gene Trees and Well-Supported Phylogenomic Trees of Nostocalean Cyanobacteria. Syst. Biol. 2023 , 72 , 694–712. [ Google Scholar ] [ CrossRef ]
- Shen, X.X.; Li, Y.; Hittinger, C.T.; Chen, X.X.; Rokas, A. An investigation of irreproducibility in maximum likelihood phylogenetic inference. Nat. Commun. 2020 , 11 , 6096. [ Google Scholar ] [ CrossRef ]
- Zhao, P.; Kakishima, M.; Uzuhashi, S.; Ishii, H. Multigene phylogenetic analysis of inter- and intraspecific relationships in Venturia nashicola and V. pirina. Eur. J. Plant Pathol. 2012 , 132 , 245–258. [ Google Scholar ] [ CrossRef ]
- Abeysundera, M.; Field, C.; Gu, H. Phylogenetic Analysis Based on Spectral Methods. Mol. Biol. Evol. 2012 , 29 , 579–597. [ Google Scholar ] [ CrossRef ]
- Bi, G.; Mao, Y.; Xing, Q.; Cao, M. HomBlocks: A multiple-alignment construction pipeline for organelle phylogenomics based on locally collinear block searching. Genomics 2018 , 110 , 18–22. [ Google Scholar ] [ CrossRef ]
- Steenwyk, J.L.; Li, Y.; Zhou, X.; Shen, X.X.; Rokas, A. Incongruence in the phylogenomics era. Nat. Rev. Genet. 2023 , 24 , 834–850. [ Google Scholar ] [ CrossRef ] [ PubMed ]
- Wolsan, M.; Sato, J.J. Effects of data incompleteness on the relative performance of parsimony and Bayesian approaches in a supermatrix phylogenetic reconstruction of Mustelidae and Procyonidae (Carnivora). Cladistics Int. J. Willi Hennig Soc. 2010 , 26 , 168–194. [ Google Scholar ] [ CrossRef ]
- Rannala, B.; Yang, Z. Phylogenetic inference using whole genomes. Annu. Rev. Genom. Hum. Genet. 2008 , 9 , 217–231. [ Google Scholar ] [ CrossRef ] [ PubMed ]
- Zou, X.-H.; Song, G. Conflicting gene trees and phylogenomics. J. Syst. Evol. 2008 , 46 , 795. [ Google Scholar ]
- Delsuc, F.; Brinkmann, H.; Philippe, H. Phylogenomics and the reconstruction of the tree of life. Nat. Rev. Genet. 2005 , 6 , 361–375. [ Google Scholar ] [ CrossRef ]
- Bininda-Emonds, O.R.; Sanderson, M.J. Assessment of the accuracy of matrix representation with parsimony analysis supertree construction. Syst. Biol. 2001 , 50 , 565–579. [ Google Scholar ] [ CrossRef ]
- Zhao, T.; Zwaenepoel, A.; Xue, J.-Y.; Kao, S.-M.; Li, Z.; Schranz, M.E.; Van de Peer, Y. Whole-genome microsynteny-based phylogeny of angiosperms. Nat. Commun. 2021 , 12 , 3498. [ Google Scholar ] [ CrossRef ]
- Cotton, J.A.; Wilkinson, M. Majority-rule supertrees. Syst. Biol. 2007 , 56 , 445–452. [ Google Scholar ] [ CrossRef ]
- Delucchi, E.; Hoessly, L.; Paolini, G. Impossibility Results on Stability of Phylogenetic Consensus Methods. Syst. Biol. 2020 , 69 , 557–565. [ Google Scholar ] [ CrossRef ] [ PubMed ]
- Goloboff, P.A.; Pol, D. Semi-strict supertrees. Cladistics Int. J. Willi Hennig Soc. 2002 , 18 , 514–525. [ Google Scholar ] [ CrossRef ]
- Fischer, M.; Hendriksen, M. Refinement-stable Consensus Methods. arXiv 2021 , arXiv:2102.04502. [ Google Scholar ] [ CrossRef ]
- Lapointe, F.-J.; Cucumel, G. The Average Consensus Procedure: Combination of Weighted Trees Containing Identical or Overlapping Sets of Taxa. Syst. Biol. 1997 , 46 , 306–312. [ Google Scholar ] [ CrossRef ]
- Mavrodiev, E.V.; Williams, D.M.; Ebach, M.C. On the Typology of Relations. Evol. Biol. 2019 , 46 , 71–89. [ Google Scholar ] [ CrossRef ]
- Lu, L.; Sun, M.; Zhang, J.; Li, H.; Lin, L.; Yang, T.; Chen, M.; Chen, Z. Tree of life and its applications. Biodivers. Sci. 2014 , 22 , 3–20. [ Google Scholar ] [ CrossRef ]
- Jiang, X.; Edwards, S.V.; Liu, L. The Multispecies Coalescent Model Outperforms Concatenation Across Diverse Phylogenomic Data Sets. Syst. Biol. 2020 , 69 , 795–812. [ Google Scholar ] [ CrossRef ]
- Retief, J.D. Phylogenetic analysis using PHYLIP. Methods Mol. Biol. 2000 , 132 , 243–258. [ Google Scholar ] [ CrossRef ]
- Wilgenbusch, J.C.; Swofford, D. Inferring evolutionary trees with PAUP*. In Current Protocols in Bioinformatics ; Wiley: Hoboken, NJ, USA, 2003; Chapter 6, Unit 6.4. [ Google Scholar ] [ CrossRef ]
- Guindon, S.; Dufayard, J.F.; Lefort, V.; Anisimova, M.; Hordijk, W.; Gascuel, O. New algorithms and methods to estimate maximum-likelihood phylogenies: Assessing the performance of PhyML 3.0. Syst. Biol. 2010 , 59 , 307–321. [ Google Scholar ] [ CrossRef ]
- Ronquist, F.; Teslenko, M.; van der Mark, P.; Ayres, D.L.; Darling, A.; Höhna, S.; Larget, B.; Liu, L.; Suchard, M.A.; Huelsenbeck, J.P. MrBayes 3.2: Efficient Bayesian phylogenetic inference and model choice across a large model space. Syst. Biol. 2012 , 61 , 539–542. [ Google Scholar ] [ CrossRef ]
- Tamura, K.; Stecher, G.; Kumar, S. MEGA11: Molecular Evolutionary Genetics Analysis Version 11. Mol. Biol. Evol. 2021 , 38 , 3022–3027. [ Google Scholar ] [ CrossRef ] [ PubMed ]
- Xiang, C.Y.; Gao, F.; Jakovlić, I.; Lei, H.P.; Hu, Y.; Zhang, H.; Zou, H.; Wang, G.T.; Zhang, D. Using PhyloSuite for molecular phylogeny and tree-based analyses. iMeta 2023 , 2 , e87. [ Google Scholar ] [ CrossRef ]
- Huber, W.; Carey, V.J.; Gentleman, R.; Anders, S.; Carlson, M.; Carvalho, B.S.; Bravo, H.C.; Davis, S.; Gatto, L.; Girke, T.; et al. Orchestrating high-throughput genomic analysis with Bioconductor. Nat. Methods 2015 , 12 , 115–121. [ Google Scholar ] [ CrossRef ] [ PubMed ]
- Giorgi, F.M.; Ceraolo, C.; Mercatelli, D. The R Language: An Engine for Bioinformatics and Data Science. Life 2022 , 12 , 648. [ Google Scholar ] [ CrossRef ] [ PubMed ]
- Yu, G.; Smith, D.K.; Zhu, H.; Guan, Y.; Lam, T.T.Y. ggtree: An R package for visualization and annotation of phylogenetic trees with their covariates and other associated data. Methods Ecol. Evol. 2017 , 8 , 28–36. [ Google Scholar ] [ CrossRef ]
- Paradis, E.; Schliep, K. ape 5.0: An environment for modern phylogenetics and evolutionary analyses in R. Bioinformatics 2019 , 35 , 526–528. [ Google Scholar ] [ CrossRef ] [ PubMed ]
- Schliep, K.P. phangorn: Phylogenetic analysis in R. Bioinformatics 2011 , 27 , 592–593. [ Google Scholar ] [ CrossRef ] [ PubMed ]
- Galili, T. dendextend: An R package for visualizing, adjusting and comparing trees of hierarchical clustering. Bioinformatics 2015 , 31 , 3718–3720. [ Google Scholar ] [ CrossRef ]
- Wang, L.G.; Lam, T.T.; Xu, S.; Dai, Z.; Zhou, L.; Feng, T.; Guo, P.; Dunn, C.W.; Jones, B.R.; Bradley, T.; et al. Treeio: An R Package for Phylogenetic Tree Input and Output with Richly Annotated and Associated Data. Mol. Biol. Evol. 2020 , 37 , 599–603. [ Google Scholar ] [ CrossRef ]
- Yu, G. Data Integration, Manipulation and Visualization of Phylogenetic Trees ; Chapman and Hall/CRC: Boca Raton, FL, USA, 2022. [ Google Scholar ]
- Xu, S.; Li, L.; Luo, X.; Chen, M.; Tang, W.; Zhan, L.; Dai, Z.; Lam, T.T.; Guan, Y.; Yu, G. Ggtree: A serialized data object for visualization of a phylogenetic tree and annotation data. iMeta 2022 , 1 , e56. [ Google Scholar ] [ CrossRef ]
- Wilkinson, L. ggplot2: Elegant Graphics for Data Analysis by WICKHAM, H. Biometrics 2011 , 67 , 678–679. [ Google Scholar ] [ CrossRef ]
- Cock, P.J.; Antao, T.; Chang, J.T.; Chapman, B.A.; Cox, C.J.; Dalke, A.; Friedberg, I.; Hamelryck, T.; Kauff, F.; Wilczynski, B.; et al. Biopython: Freely available Python tools for computational molecular biology and bioinformatics. Bioinformatics 2009 , 25 , 1422–1423. [ Google Scholar ] [ CrossRef ]
- Sukumaran, J.; Holder, M.T. DendroPy: A Python library for phylogenetic computing. Bioinformatics 2010 , 26 , 1569–1571. [ Google Scholar ] [ CrossRef ] [ PubMed ]
- Hao, J.; Ho, T.K. Machine learning made easy: A review of scikit-learn package in python programming language. J. Educ. Behav. Stat. 2019 , 44 , 348–361. [ Google Scholar ] [ CrossRef ]
- Ketkar, N.; Moolayil, J.; Ketkar, N.; Moolayil, J. Introduction to pytorch. In Deep Learning with Python: Learn Best Practices of Deep Learning Models with PyTorch ; Apress: Berkeley, CA, USA, 2021; pp. 27–91. [ Google Scholar ]
- Jombart, T. adegenet: A R package for the multivariate analysis of genetic markers. Bioinformatics 2008 , 24 , 1403–1405. [ Google Scholar ] [ CrossRef ]
- Bilderbeek, R.J.; Laudanno, G.; Etienne, R.S. Quantifying the impact of an inference model in Bayesian phylogenetics. Methods Ecol. Evol. 2021 , 12 , 351–358. [ Google Scholar ] [ CrossRef ]
- Zou, Z.; Zhang, H.; Guan, Y.; Zhang, J. Deep Residual Neural Networks Resolve Quartet Molecular Phylogenies. Mol. Biol. Evol. 2020 , 37 , 1495–1507. [ Google Scholar ] [ CrossRef ] [ PubMed ]
- Bilderbeek, R.J.C.; Etienne, R.S. babette: BEAUti 2, BEAST2 and Tracer for R. Methods Ecol. Evol. 2018 , 9 , 2034–2040. [ Google Scholar ] [ CrossRef ]
- Rabosky, D.L.; Grundler, M.; Anderson, C.; Title, P.; Shi, J.J.; Brown, J.W.; Huang, H.; Larson, J.G. BAMMtools: An R package for the analysis of evolutionary dynamics on phylogenetic trees. Methods Ecol. Evol. 2014 , 5 , 701–707. [ Google Scholar ] [ CrossRef ]
- Jombart, T.; Archer, F.; Schliep, K.; Kamvar, Z.; Harris, R.; Paradis, E.; Goudet, J.; Lapp, H. apex: Phylogenetics with multiple genes. Mol. Ecol. Resour. 2017 , 17 , 19–26. [ Google Scholar ] [ CrossRef ]
- Revell, L.J. phytools 2.0: An updated R ecosystem for phylogenetic comparative methods (and other things). PeerJ 2024 , 12 , e16505. [ Google Scholar ] [ CrossRef ]
- Morlon, H.; Lewitus, E.; Condamine, F.L.; Manceau, M.; Clavel, J.; Drury, J. RPANDA: An R package for macroevolutionary analyses on phylogenetic trees. Methods Ecol. Evol. 2016 , 7 , 589–597. [ Google Scholar ] [ CrossRef ]
- Smith, M.R. TreeSearch: Morphological phylogenetic analysis in R. bioRxiv 2021 . [ Google Scholar ] [ CrossRef ]
- Bapst, D.W. paleotree: An R package for paleontological and phylogenetic analyses of evolution. Methods Ecol. Evol. 2012 , 3 , 803–807. [ Google Scholar ] [ CrossRef ]
- Bennett, D.J.; Sutton, M.D.; Turvey, S.T. treeman: An R package for efficient and intuitive manipulation of phylogenetic trees. BMC Res. Notes 2017 , 10 , 30. [ Google Scholar ] [ CrossRef ] [ PubMed ]
- Burgstaller-Muehlbacher, S.; Crotty, S.M.; Schmidt, H.A.; Reden, F.; Drucks, T.; von Haeseler, A. ModelRevelator: Fast phylogenetic model estimation via deep learning. Mol. Phylogenetics Evol. 2023 , 188 , 107905. [ Google Scholar ] [ CrossRef ]
- Sarkar, R. Low distortion delaunay embedding of trees in hyperbolic plane. In Proceedings of the International Symposium on Graph Drawing, Eindhoven, The Netherlands, 21–23 September 2011; pp. 355–366. [ Google Scholar ]
- Matsumoto, H.; Mimori, T.; Fukunaga, T. Novel metric for hyperbolic phylogenetic tree embeddings. Biol. Methods Protoc. 2021 , 6 , bpab006. [ Google Scholar ] [ CrossRef ]
- Jiang, Y.; Tabaghi, P.; Mirarab, S. Learning Hyperbolic Embedding for Phylogenetic Tree Placement and Updates. Biology 2022 , 11 , 1256. [ Google Scholar ] [ CrossRef ]
- Macaulay, M.; Darling, A.; Fourment, M. Fidelity of hyperbolic space for Bayesian phylogenetic inference. PLoS Comput. Biol. 2023 , 19 , e1011084. [ Google Scholar ] [ CrossRef ]
- Thirunavukarasu, A.J.; Ting, D.S.J.; Elangovan, K.; Gutierrez, L.; Tan, T.F.; Ting, D.S.W. Large language models in medicine. Nat. Med. 2023 , 29 , 1930–1940. [ Google Scholar ] [ CrossRef ] [ PubMed ]
- Lubiana, T.; Lopes, R.; Medeiros, P.; Silva, J.C.; Goncalves, A.N.A.; Maracaja-Coutinho, V.; Nakaya, H.I. Ten quick tips for harnessing the power of ChatGPT in computational biology. PLoS Comput. Biol. 2023 , 19 , e1011319. [ Google Scholar ] [ CrossRef ] [ PubMed ]
Click here to enlarge figure
Algorithm | Principle | Hypothesis | Criteria for Selecting the Final Tree | Scope of Application |
---|---|---|---|---|
NJ * | Minimal evolution: Minimizing the total branch length of the phylogenetic tree. | BME branch length estimation model: Ensuring general statistical consistency of minimum length phylogeny and non-negativity of its branch lengths [ ]. | In the end, only one tree was constructed. | Short sequences with small evolutionary distance and few informative sites. |
MP | Maximum-parsimony criterion: Minimize the number of evolutionary steps required to explain the data set. | No model required. | The phylogenetic tree with the smallest number of base (or amino acid) substitutions during evolution. | Sequences with high sequence similarity, sequences for which it is difficult to design appropriate characteristic evolution models. |
ML | Maximize likelihood value. | The sites in the alignment are independent; each branch is allowed to evolve at different rates. | Phylogenetic tree with maximum likelihood value. | Distantly related and small number of sequences. |
BI | Bayes theorem. | Continuous-time Markov substitution model: Substitution probability is only related to the current nucleotide and has nothing to do with past nucleotides. | The most sampled phylogenetic tree in MCMC. | A small number of sequences. |
The statements, opinions and data contained in all publications are solely those of the individual author(s) and contributor(s) and not of MDPI and/or the editor(s). MDPI and/or the editor(s) disclaim responsibility for any injury to people or property resulting from any ideas, methods, instructions or products referred to in the content. |
Share and Cite
Zou, Y.; Zhang, Z.; Zeng, Y.; Hu, H.; Hao, Y.; Huang, S.; Li, B. Common Methods for Phylogenetic Tree Construction and Their Implementation in R. Bioengineering 2024 , 11 , 480. https://doi.org/10.3390/bioengineering11050480
Zou Y, Zhang Z, Zeng Y, Hu H, Hao Y, Huang S, Li B. Common Methods for Phylogenetic Tree Construction and Their Implementation in R. Bioengineering . 2024; 11(5):480. https://doi.org/10.3390/bioengineering11050480
Zou, Yue, Zixuan Zhang, Yujie Zeng, Hanyue Hu, Youjin Hao, Sheng Huang, and Bo Li. 2024. "Common Methods for Phylogenetic Tree Construction and Their Implementation in R" Bioengineering 11, no. 5: 480. https://doi.org/10.3390/bioengineering11050480
Article Metrics
Article access statistics, further information, mdpi initiatives, follow mdpi.
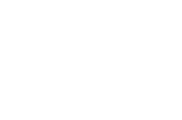
Subscribe to receive issue release notifications and newsletters from MDPI journals
Phylogenetics
An introduction
- Course overview
- Search within this course
- Why use molecular data?
Why is phylogenetics important?
- The example of a family tree
- Relating distance, rate and time
- Alternative representation of phylogenies
- Interpreting patterns of relatedness
- Major stages in phylogenetic analyses
- Phylogenetics resources at EMBL-EBI
- Discovering the phylogeny of all flowering plants
- Quiz: test your knowledge
- Your feedback

All materials are free cultural works licensed under a Creative Commons Attribution 4.0 International (CC BY 4.0) license , except where further licensing details are provided.
Share this page with:
Phylogenetics is important because it enriches our understanding of how genes, genomes, species (and molecular sequences more generally) evolve. Through phylogenetics, we learn not only how the sequences came to be the way they are today, but also general principles that enable us to predict how they will change in the future. This is not only of fundamental importance but also extremely useful for numerous applications (Figure 2).
Applications of phylogenetics

Classification : Phylogenetics based on sequence data provides us with more accurate descriptions of patterns of relatedness than was available before the advent of molecular sequencing. Phylogenetics now informs the Linnaean classification of new species.
Forensics : Phylogenetics is used to assess DNA evidence presented in court cases to inform situations, e.g. where someone has committed a crime, when food is contaminated, or where the father of a child is unknown.
Identifying the origin of pathogens : Molecular sequencing technologies and phylogenetic approaches can be used to learn more about a new pathogen outbreak. This includes finding out about which species the pathogen is related to and subsequently the likely source of transmission. This can lead to new recommendations for public health policy.
Conservation : Phylogenetics can help to inform conservation policy when conservation biologists have to make tough decisions about which species they try to prevent from becoming extinct.
Bioinformatics and computing : Many of the algorithms developed for phylogenetics have been used to develop software in other fields.
With the advent of newer, faster sequencing technologies, it is now possible to take a sequencing machine out to the field and sequence species of interest in situ . Phylogenetics is needed to add biological meaning to the data.
Congratulations!
You have completed this tutorial.
Why not share your success on social media?
Continue on to the final pages of this online tutorial for recommendations on what to learn next and to tell us what you thought of this tutorial.
Phylogenetic Trees: Applications, Construction, and Assessment
- First Online: 02 November 2019
Cite this chapter
- Surekha Challa 5 &
- Nageswara Rao Reddy Neelapu 5
1983 Accesses
7 Citations
Molecular phylogeny is used to study the relationships among the set of objects by generating phylogenetic or evolutionary tree. The objects in the study can be organisms or biomolecules such as gene or protein. The evolutionary history hidden in the biomolecules establishes the evolutionary patterns in the form of a tree when a suitable data, data substitution models, and tree construction methods are used. These evolutionary patterns are used to study the relationships among the objects. These patterns sometimes make it difficult to infer the relationship among the objects. In addition, different tree construction methods like unweighted pair group method with arithmetic mean (UPGMA), neighbor joining, minimum evolution, Fitch-Margoliash, maximum parsimony, maximum likelihood, Monte Carlo’s simulation, Bayes, and so on and types of data used in the analysis make it much more complicated to infer the relationships. The above tree construction methods follow different principles to construct a phylogenetic tree. Most often, the tree topologies generated by different methods for the same data will be the same, whereas in some cases the tree topologies may be different in their internal branching. These differences in the tree topologies may make it difficult to assess the confidence of the phylogenetic tree. Further, combination of the tree construction methods and data used by phylogeny program packages such as MEGA, Molphy, Phylip, PAML, and PAUP also make it difficult to assess the confidence of the phylogenetic tree. Molecular phylogeny has a wide range of applications such as affiliating taxonomy of an organism, studying reproductive biology in lower organisms, assessing the process of cryptic speciation in a species, understanding the history of life, resolving controversial history of life, reconstructing the paths of infection in an epidemiology, classifying proteins or genes into families, and many more. If the interpretation of the evolutionary patterns is not appropriate, then the inference of the study may be misleading. Thus, interpretation of the tree and relationships among the organisms is always dependent on assessing the confidence of the phylogenetic tree. Literature review shows that sampling methods such as bootstrapping, jackknifing, and Bayesian simulation and statistical methods such as Kishino-Hasegawa test and Shimodaira-Hasegawa test are used to assess the confidence of the phylogenetic tree. Thus, this chapter reviews the applications, construction, and assessment of phylogenetic tree.
This is a preview of subscription content, log in via an institution to check access.
Access this chapter
- Available as PDF
- Read on any device
- Instant download
- Own it forever
- Available as EPUB and PDF
- Compact, lightweight edition
- Dispatched in 3 to 5 business days
- Free shipping worldwide - see info
- Durable hardcover edition
Tax calculation will be finalised at checkout
Purchases are for personal use only
Institutional subscriptions
Similar content being viewed by others
Molecular Phylogenetics: Concepts for a Newcomer
Short Introduction to Phylogenetic Analysis of Molecular Sequence Data
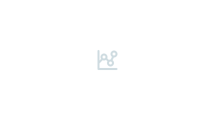
Tutorial on phylogenetic inference — 2
Adachi J, Hasegawa M (1996) Molphy, version 2.3. Programs for molecular phylogenetics based on maximum likelihood. In: Ishiguro M, Kitagawa G, Ogata Y, Takagi H, Tamura Y, Tsuchiya T (eds) Computer science monographs. Institute of Statistical Mathematics, Tokyo
Google Scholar
Ané C, Larget B, Baum DA, Smith SD, Rokas A (2007) Bayesian estimation of concordance among gene trees. Mol Biol Evol 24(2):412–426
Article CAS PubMed Google Scholar
Baldauf SL, Roger AJ, Wenk-Siefert I, Doolittle WF (2000) A kingdom-level phylogeny of eukaryotes based on combined protein data. Science 290(5493):972–977
Castro-Nallar E, Perez-Losada M, Burton GF, Crandall KA (2012) The evolution of HIV: inferences using phylogenetics. Mol Phylogenet Evol 62:777–792
Cavalli-Sforza LL, Edwards AWF (1967) Phylogenetic analysis: models and estimation procedures. Evolution 21:550–570
Clement M, Posada D, Crandall K (2000) TCS: a computer program to estimate gene genealogies. Mol Ecol 9:1657–1660
Devi KU, Reineke A, Reddy NNR, Rao CUM, Padmavathi J (2006) Genetic diversity, reproductive biology, and speciation in the entomopathogenic fungus Beauveria bassiana (Balsamo) Vuillemin. Genome 49(5):495–504
Devi UK, Reineke A, Rao UCM, Reddy NRN, Khan APA (2007) AFLP and single-strand conformation polymorphism studies of recombination in the entomopathogenic fungus Nomuraea rileyi . Mycol Res 111(6):716–725
Drummond A, Strimmer K (2001) PAL: an object-oriented programming library for molecular evolution and phylogenetics. Bioinformatics 17:662–663
Drummond AJ, Suchard MA, Xie D, Rambaut A (2012) Bayesian phylogenetics with BEAUti and the BEAST 1.7. Mol Biol Evol 29:1969–1973
Article CAS PubMed PubMed Central Google Scholar
Felsenstein J (1973) Maximum likelihood and minimum-steps methods for estimating evolutionary trees from data on discrete characters. Syst Zool 22:240–249
Article Google Scholar
Felsenstein J (1981) Evolutionary trees from gene-frequencies and quantitative characters – finding maximum-likelihood estimates. Evolution 35:1229–1242
Article PubMed Google Scholar
Felsenstein J (1989) PHYLIP – phylogeny inference package (version 3.2). Cladistics 5:164–166
Fitch WM (1971) Towards defining the course of evolution: minimum change for a specific tree topology. Syst Zool 20:406–416
Frech C, Chen N (2010) Genome-wide comparative gene family classification. PLoS One 5(10):e13409. https://doi.org/10.1371/journal.pone.0013409
Gao F, Yue L, White AT, Pappas PG, Barchue J, Hanson AP, Greene BM, Sharp PM, Shaw GM, Hahn BH (1992) Human infection by genetically diverse SIVSM-related HIV-2 in West Africa. Nature 358:495–499
Gao F, Bailes E, Robertson DL, Chen Y, Rodenburg CM, Michael SF, Cummins LB, Arthur LO, Peeters M, Shaw GM, Sharp PM, Hahn BH (1999) Origin of HIV-1 in the chimpanzee Pan troglodytes troglodytes . Nature 397:436–441
Gilbert MTP, Rambaut A, Wlasiuk G, Spira TJ, Pitchenik AE, Worobey M (2007) The emergence of HIV/AIDS in the Americas and beyond. Proc Natl Acad Sci U S A 104:18566–18570
Goloboff PA (1999) Analyzing large data sets in reasonable times: solutions for composite optima. Cladistics 15:415–428
Grenfell B, Pybus O, Gog J, Wood J, Daly J (2004) Unifying the epidemiological and evolutionary dynamics of pathogens. Science 303:327–332
Hahn BH, Shaw GM, De Cock KM, Sharp PM (2000) AIDS as a zoonosis: scientific and public health implications. Science 287:607–614
Hardison RC (2012) Evolution of hemoglobin and its genes. Cold Spring Harb Perspect Med 2(12):a011627. https://doi.org/10.1101/cshperspect.a011627
Hasegawa M, Kishino H, Yano T (1985) Dating of the human-ape splitting by a molecular clock of mitochondrial DNA. J Mol Evol 22(2):160–174
Holmes EC (2009) The evolution and emergence of RNA viruses. Oxford University Press, New York
Huelsenbeck JP, Ronquist F (2001) MrBayes: Bayesian inference of phylogeny. Bioinformatics 17:754–755
Huet T, Cheynier R, Meyerhans A, Roelants G, Wain-Hobson S (1990) Genetic organization of a chimpanzee lentivirus related to HIV-1. Nature 345:356–359
Kishino H, Hasegawa M (1989) Evaluation of the maximum likelihood estimate of the evolutionary tree topologies from DNA sequence data, and the branching order in Hominoidea. J Mol Evol 29:170–179
Kumar S, Tamura K, Nei M (2004) MEGA3: an integrated software for molecular evolutionary genetics analysis and sequence alignment. Brief Bioinform 5:150–163
Lemey P, Pybus OG, Wang B, Saksena NK, Salemi M, Vandamme A-M (2003) Tracing the origin and history of the HIV-2 epidemic. Proc Natl Acad Sci U S A 100:6588–6592
Lord E, Leclercq M, Boc A, Diallo AB, Makarenkov V (2012) Armadillo 1.1: an original workflow platform for designing and conducting phylogenetic analysis and simulations. PLoS One 7(1):e29903. https://doi.org/10.1371/journal.pone.002990
Maddison WP, Maddison DR (1992) MacClade. Sinauer Associates, Sunderland
Maddison WP, Maddison DR (2011) Mesquite: a modular system for evolutionary analysis. Version 2.75. http://mesquiteproject.org
Maeshima M (2000) Vacuolar H + -pyrophosphatase. Biochim Biophys Acta 1465:37–51
Margos G, Vollmer SA, Ogden NH, Fish D (2011) Population genetics, taxonomy, phylogeny and evolution of Borrelia burgdorferi sensu lato. Infect Genet Evol 11(7):1545–1563
Article PubMed PubMed Central Google Scholar
McGuire G, Wright F (2000) TOPAL 2.0: improved detection of mosaic sequences within multiple alignments. Bioinformatics 16(2):130–134
Neelapu NRR (2007) Investigation on existence and mechanism of recombination and molecular phylogeny of mitosporic entomopathogenic fungi Beauveria bassiana (Balsamo) Vuillemin and Nomuraea rileyi (Farlow) Samson. Doctoral dissertation, Andhra University, Visakhapatnam, India
Neelapu NRR, Reineke A, Chanchala UMR, Koduru UD (2009) Molecular phylogeny of asexual entomopathogenic fungi with special reference to Beauveria bassiana and Nomuraea rileyi . Rev Iberoam Micol 26(2):129–145
Nei M (1975) Molecular population genetics and evolution. North-Holland, Amsterdam
Nguyen L-T, Schmidt HA, von Haeseler A, Minh BQ (2015) IQ-TREE: a fast and effective stochastic algorithm for estimating maximum-likelihood phylogenies. Mol Biol Evol 32(1):268–274
Olsen GJ, Matsuda H, Hagstrom R, Overbeek R (1994) FastDNAml: a tool for construction of phylogenetic trees of DNA sequences using maximum likelihood. Bioinformatics 10(1):41–48
Article CAS Google Scholar
Opazo JC, Homan FG, Storz JF (2008) Genomic evidence for independent origins of like globin genes in monotremes and therian mammals. Proc Natl Acad Sci U S A 105:1590–1595
Pace NR (1997) A molecular view of microbial diversity and the biosphere. Science 276:734–740
Padmavathi J, Uma Devi K, Rao CUM, Reddy NNR (2003) Telomere fingerprinting for assessing chromosome number, isolating typing and recombination in the entomopathogen Beauveria bassiana . Mycol Res 107(5):572–580
Page RDM (1998) GeneTree: comparing gene and species phylogenies using reconciled trees. Bioinformatics 14:819–820
Pagel M, Meade A (2004) A phylogenetic mixture model for detecting pattern-heterogeneity in gene sequence or character-state data. Syst Biol 53:571–581
Pérez-Losada M, Jobes DV, Sinangil F, Crandall KA, Posada D, Berman PW (2010) Phylodynamics of HIV-1 from a phase-III AIDS vaccine trial in North America. Mol Biol Evol 27:417–425
Plantier J-C, Leoz M, Dickerson JE, De Oliveira F, Cordonnier F, Lemee V, Damond F, Robertson DL, Simon F (2009) A new human immunodeficiency virus derived from gorillas. Nat Med 15:871–872
Posada D, Crandall KA, Templeton AR (2000) GeoDis: a program for the cladistic nested analysis of the geographical distribution of genetic haplotypes. Mol Ecol 9:487–488
Pozio E, Hoberg E, La Rosa G, Zarlenga DS (2009) Molecular taxonomy, phylogeny and biogeography of nematodes belonging to the Trichinella genus . Infect Genet Evol 9(4):606–616
Price MN, Dehal PS, Arkin AP (2009) FastTree: computing large minimum-evolution trees with profiles instead of a distance matrix. Mol Biol Evol 26:1641–1650
Ramírez-Flandes S, Ulloa O (2008) Bosque: integrated phylogenetic analysis software. Bioinformatics 24(21):2539–2541
Raphaël H, Milinkovitch MC (2010) MetaPIGA v2.0: maximum likelihood large phylogeny estimation using the metapopulation genetic algorithm and other stochastic heuristics. BMC Bioinforma 11:379
Rea PA, Kim Y, Sarafian V, Poole RJ, Davies JM, Sanders D (1992) Vacuolar H + -translocating pyrophosphatase: a new category of ion translocase. Trends Biochem Sci 17(9):348–352
Saitou N, Nei M (1987) The neighbor-joining method: a new method for reconstructing phylogenetic trees. Mol Biol Evol 4(4):406–425
CAS PubMed Google Scholar
Salemi M, Lamers SL, Yu S, de Oliveira T, Fitch WM, McGrath MS (2005) Phylodynamic analysis of human immunodeficiency virus type 1 in distinct brain compartments provides a model for the neuropathogenesis of AIDS. J Virol 79:11343–11352
Schmidt HA, Strimmer K, Vingron M, von Haeseler A (2002) TREE-PUZZLE: maximum likelihood phylogenetic analysis using quartets and parallel computing. Bioinformatics 18:502–504
Shimodaira H, Hasegawa M (1999) Multiple comparisons of log-likelihoods with applications to phylogenetic inference. Mol Biol Evol 16:1114–1116
Sokal RR, Michener CD (1958) A statistical method for evaluating systematic relationships. J Univ Kans Sci Bull 28:1409–1438
Suchard MA, Redelings BD (2006) BAli-Phy: simultaneous Bayesian inference of alignment and phylogeny. Bioinformatics 22:2047–2048
Suneetha G, Neelapu NRR, Surekha C (2016) Plant vacuolar proton pyrophosphatases (VPPases): structure, function and mode of action. Int J Recent Sci Res 7(6):12148–12152
Swofford DL (1991) PAUP: Phylogenetic Analysis Using Parsimony, version 3.1 Computer program distributed by the Illinois Natural History Survey, Champaign, Illinois
Swofford DL, Olsen GJ, Waddell PJ, Hillis DM (1996) Phylogenetic inference. In: Hillis DM, Moritz C, Mable BK (eds) Molecular systematics. Sinauer, Sunderland
Takahashi K, Nei M (2000) Efficiencies of fast algorithms of phylogenetic inference under the criteria of maximum parsimony, minimum evolution, and maximum likelihood when a large number of sequences are used. Mol Biol Evol 17:1251–1258
Teugels G (1996) Taxonomy, phylogeny and biogeography of catfishes ( Ostariophysi , Siluroidei ): an overview. Aquat Living Resour 9(S1):9–34. https://doi.org/10.1051/alr:1996039
Thompson RCA (2008) The taxonomy, phylogeny and transmission of Echinococcus . Exp Parasitol 119(4):439–446
Van Heuverswyn F, Peeters M (2007) The origins of HIV and implications for the global epidemic. Curr Infect Dis Rep 9:338–346
Vinh LS, von Haeseler A (2004) IQPNNI: moving fast through tree space and stopping in time. Mol Biol Evol 21(8):1565–1571
Woese CR (1987) Bacterial evolution. Microbiol Rev 51:221–271
CAS PubMed PubMed Central Google Scholar
Woese CR, Fox GE (1997) Phylogenetic structure of the prokaryotic domain: the primary kingdoms. Proc Natl Acad Sci U S A 74:5088–5090
Woese CR, Kandler O, Wheelis ML (1990) Towards a natural system of organisms: proposal for the domains Archaea, Bacteria, and Eucarya. Proc Natl Acad Sci U S A 87:4576–4579
Worobey M, Gemmel M, Teuwen DE, Haselkorn T, Kunstman K, Bunce M, Muyembe J-J, Kabongo J-MM, Kalengayi RM, Van Marck E, Gilbert MTP, Wolinsky SM (2008) Direct evidence of extensive diversity of HIV-1 in Kinshasa by 1960. Nature 455:661–664
Xia X, Xie Z (2001) DAMBE: data analysis in molecular biology and evolution. J Hered 92:371–373
Yang Z (2000) Phylogenetic analysis by maximum likelihood (PAML). University College, London
Download references
Acknowledgment
The authors are grateful to Gandhi Institute of Technology and Management (GITAM) Deemed-to-be-University, for providing necessary facilities to carry out the research work and for extending constant support in writing this review.
Author information
Authors and affiliations.
Department of Biochemistry and Bioinformatics, Institute of Science, Gandhi Institute of Technology and Management (GITAM) (Deemed to be University), Visakhapatnam, Andhra Pradesh, India
Surekha Challa & Nageswara Rao Reddy Neelapu
You can also search for this author in PubMed Google Scholar
Editor information
Editors and affiliations.
Princess Dr. Najla Bint Saud Al-Saud Center for Excellence Research in Biotechnology, Department of Biological Sciences, King Abdulaziz University, Jeddah, Saudi Arabia
Khalid Rehman Hakeem
Princess Al-Jawhara Center of Excellence in Research of Hereditary Disorders, Department of Genetic Medicine, Faculty of Medicine, King Abdulaziz University, Jeddah, Saudi Arabia
Noor Ahmad Shaik
Babajan Banaganapalli
Ramu Elango
Rights and permissions
Reprints and permissions
Copyright information
© 2019 Springer Nature Switzerland AG
About this chapter
Challa, S., Neelapu, N.R.R. (2019). Phylogenetic Trees: Applications, Construction, and Assessment. In: Hakeem, K., Shaik, N., Banaganapalli, B., Elango, R. (eds) Essentials of Bioinformatics, Volume III. Springer, Cham. https://doi.org/10.1007/978-3-030-19318-8_10
Download citation
DOI : https://doi.org/10.1007/978-3-030-19318-8_10
Published : 02 November 2019
Publisher Name : Springer, Cham
Print ISBN : 978-3-030-19317-1
Online ISBN : 978-3-030-19318-8
eBook Packages : Biomedical and Life Sciences Biomedical and Life Sciences (R0)
Share this chapter
Anyone you share the following link with will be able to read this content:
Sorry, a shareable link is not currently available for this article.
Provided by the Springer Nature SharedIt content-sharing initiative
- Publish with us
Policies and ethics
- Find a journal
- Track your research
We use cookies to enhance our website for you. Proceed if you agree to this policy or learn more about it.
- Essay Database >
- Essay Examples >
- Essays Topics >
- Essay on Science
Phylogenetic Tree Essay Example
Type of paper: Essay
Topic: Science , Development , Life , Information , DNA , Evolution , Genetics , Species
Words: 1250
Published: 12/07/2019
ORDER PAPER LIKE THIS
Introduction:
Phylogenetic tree also known as evolutionary tree depicts the connection between various species of animals and plants based on their physical and genetic traits. Top points of the trees symbolize descendent common species whereas nodes symbolize common ancestor of descendents. Phylogenetic trees very effectively show the evolution that took place by adaptive and sudden ancestry splits. The Phylogenetic trees can be classified mainly in four types rooted tree, un-rooted tree, bifurcating tree and special tree types.
The basal universal phylogenetic tree inferred from comparative analyses of rRNA sequences.
1. Horizontally acquired variations – Horizontal acquire variations occurs due to horizontal gene transfer. Horizontal gene transfer also known as lateral gene transfer is a procedure where a species integrate genetic material of any other species even when other species is not parent of that species. Horizontal gene transfer is a process which defines cellular evolution due to its nature of bringing variation in species. Horizontal gene transfer was known for a long time but earlier scientists were aware about the limited impact on evolution. For the last two decades we have realized the power of Horizontal gene transfer. It can change the whole genome. For example we can see various protein trees and RNA tree are different in topology because of Horizontal gene transfer. Earlier we use to think that the trees are having flaws or plotted wrongly but those interpretations were not right. Horizontal gene transfer can be done by various methods. Transformation is a method in which cell get altered due to introduction of genetic material from strange species. In transduction method genetic material moved from one species to another by virus. Another method is bacterial conjugation in which genetic material get transferred by cell contact. Horizontal gene transfer also conducted by gene transfer agents. Vertically generated variations – Vertically generated variation occurs due to vertical gene transfer. Vertical gene transfer is a procedure where a species integrate genetic material from its parent or any ancestors. Earlier when knowledge about horizontal acquired variations and horizontal gene transfer was not completely developed, genetics was majorly derived from vertical gene transfer. Vertically generated variations are very limited in character. The variations between parents and its offspring’s are very limited in nature. Horizontal derived variations are major sources of evolution. Vertically generated variations on lineages are the main way for biological difficulties and cellular integration. 2. Cambrian explosion can be recalled as a most puzzling occasion in the history of human life. Before 550 to 600 million year’s changes on the earth were very rapid. Majority of the phylum encountered various diversifications by other species including plants, animals and calcimicrobes. During that time all the species were very simple and formed by one cell. Year after years the rate of change was growing and after approx 80 years the ancestors of all species existing today were emerged. As per scientist the possible reasons for explosion could be increased level of oxygen, snowfall on the earth and increased level of calcium in Cambrian seawater. Scientist faced lot of difficulties in describing Cambrian explosion as the event happened long time ago but there are few evidence and techniques are existing that provide proof of Cambrian explosion. Some evidences are: body fossils- a very informative way of evidence. Presently we do not have complete records of fossils because of the time and metamorphism. The fossils available from Cambrian fauna are lagerstatten and have supple tissues and it is not difficult to study complete anatomy of those species. Trace fossils – these types of fossils mainly have holes, warren, tracks, feces and feeding marks. These fossils help in studying behavior of that era. Geochemical technique – various chemical marks indicate the rapid changes around Cambrian. These marks are consistent with extinction or with enormous warming due to methane ice. Phylogenetic techniques – this technique helps in working out family tree for specific species. These techniques work by establishing a relationship between species and its ancestors after evaluating the structure of DNA and RNA. Cladistics is one of the techniques which establish link between species by linking similar characteristics. Special tree type branch of phylogenetic tree is involved in Cambrian explosion. Cladogram type of phylogenetic tree is used in developing tree for species during Cambrian explosion time. 3. Genotype information provide better detailed than phenotype information. Genotype information is more useful while developing phylogenetic tree. Some information that required for phylogenetic tree can only be obtained by genotype like; distinct difference in molecular progression, information contained in genes encoding, phenotype or external features of species change very slowly compare to genetic features. Genotype information provides full information on species hereditary even if information is not articulated. Phenotype is a type of information that developed by observing the properties of species like behavior, various development and morphology. Phenotype information provides external information about species. Genotype information is internally coded information that used in forming and maintaining a live species. Genotype information is used during the whole life of a species or cell and transferred from one generation to other generation. Genotype information describes the complete process of protein formation, regulation and synthesis. Phenotype information is based on observation and sometimes can be misleading. It is not necessary that genotype always provide complete information. For example DNA molecule consist four nucleotides and while comparing two different species DNA the sequence may be same because of random mutation. 4. The debate on the existence on tree of life is still going on. Scientists around the world are skeptical about its existence. The trees of life have different definitions in different subjects. People are having tree of life in biology, religion, mythology and various areas. In the field of science there are various theories about tree of life which conflict with each other. The theory of life is based on cell evolutions. All the theories developed by scientist are based on their own understanding, assumptions, available evidence and other supporting facts. The existence of life on earth happened long time ago and till now scientist are not having a complete information about the various species and their phylogenetic trees. During the evolutions lot of variations horizontal and vertical took places, lot of information due to random mutation gone missing. There is still a big question mark on the existence of tree of life.
Conclusion:
The universal phylogenetic tree represents the true picture of the genealogy and it opens the doors of the past for the people and allows them to see the past. Further it also portrays a picture of the future and helps the biologist in their several researches and studies.
Willi Hennig, D. Davis and Rainer Zangerl. (1999). Phylogenetic systematics. Champaign: University of Illinois Press. Cambrian Explosion. (n.d.). Retrieved December 25, 2011, from www.fossilmuseum.net: http://www.fossilmuseum.net/Paleobiology/CambrianExplosion.htm E. O. Wiley and Bruce S. Lieberman. (2011). Phylogenetics: Theory and Practice of Phylogenetic Systematics. New Jersey: John Wiley & Sons. Modern Phylogenetics. (n.d.). Retrieved December 25, 2011, from www.bio.cmu.edu: https://www.bio.cmu.edu/courses/03441/TermPapers/99TermPapers/GenEvo/phylogeny.htm Woese, C. R. (2000, May 22). Interpreting the universal phylogenetic tree. Retrieved December 25, 2011, from www.pnas.org: http://www.pnas.org/content/97/15/8392.full.pdf Woese, C. R. (2002). On the evolution of cells. PNAS , 8742–8747.
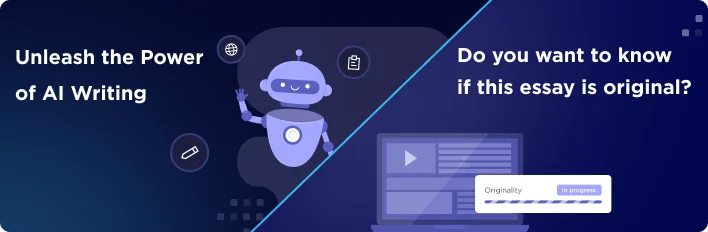
Cite this page
Share with friends using:
Removal Request

Finished papers: 2578
This paper is created by writer with
If you want your paper to be:
Well-researched, fact-checked, and accurate
Original, fresh, based on current data
Eloquently written and immaculately formatted
275 words = 1 page double-spaced
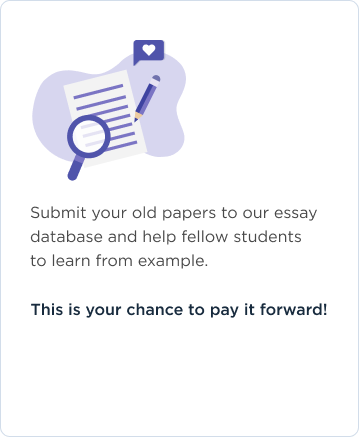
Get your papers done by pros!
Other Pages
Increase essays, hatsue essays, synthroid essays, strassburger essays, pneumocystis essays, fiedlers essays, debra essays, final exam essays, study time essays, calculates essays, asha essays, dysarthria essays, franklin stove essays, del rio essays, steiner essays, deems essays, regulatory authority essays, distillation essays, being human essays, cultural difference essays, good example of report on finance, good essay about active and passive euthanasia, emergency management essay 3, sample essay on failure, research paper on diabetes, equity premium article reviews example, good example of research paper on whistleblowing, how does a model of system behavior help in understanding long term consequences case study example, good case study on training programs at bongo, free basic historical chronology essay sample, nursing meaningful use of electronic health records research paper, free business plan about panera bread competitive analysis management team, chinese telecommunication companies essay examples, good example of essay on facebook innovation, free essay about difference between jails and prisons, free corporate and social responsibility essay example, good gone baby gone essay example, sample essay on biometric ethics, transplantation literature reviews, tenure literature reviews, col literature reviews, cont literature reviews, cells literature reviews.
Password recovery email has been sent to [email protected]
Use your new password to log in
You are not register!
By clicking Register, you agree to our Terms of Service and that you have read our Privacy Policy .
Now you can download documents directly to your device!
Check your email! An email with your password has already been sent to you! Now you can download documents directly to your device.
or Use the QR code to Save this Paper to Your Phone
The sample is NOT original!
Short on a deadline?
Don't waste time. Get help with 11% off using code - GETWOWED
No, thanks! I'm fine with missing my deadline
Thank you for visiting nature.com. You are using a browser version with limited support for CSS. To obtain the best experience, we recommend you use a more up to date browser (or turn off compatibility mode in Internet Explorer). In the meantime, to ensure continued support, we are displaying the site without styles and JavaScript.
- View all journals
- Explore content
- About the journal
- Publish with us
- Sign up for alerts
- Open access
- Published: 03 February 2020
Standardized phylogenetic and molecular evolutionary analysis applied to species across the microbial tree of life
- Migun Shakya ORCID: orcid.org/0000-0003-3876-691X 1 ,
- Sanaa A. Ahmed 1 ,
- Karen W. Davenport ORCID: orcid.org/0000-0002-2740-1344 1 ,
- Mark C. Flynn 1 ,
- Chien-Chi Lo 1 &
- Patrick S. G. Chain 1
Scientific Reports volume 10 , Article number: 1723 ( 2020 ) Cite this article
27k Accesses
56 Citations
10 Altmetric
Metrics details
- Data processing
- Genome informatics
There is growing interest in reconstructing phylogenies from the copious amounts of genome sequencing projects that target related viral, bacterial or eukaryotic organisms. To facilitate the construction of standardized and robust phylogenies for disparate types of projects, we have developed a complete bioinformatic workflow, with a web-based component to perform phylogenetic and molecular evolutionary (PhaME) analysis from sequencing reads, draft assemblies or completed genomes of closely related organisms. Furthermore, the ability to incorporate raw data, including some metagenomic samples containing a target organism (e.g. from clinical samples with suspected infectious agents), shows promise for the rapid phylogenetic characterization of organisms within complex samples without the need for prior assembly.
Similar content being viewed by others
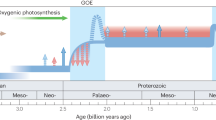
Co‐evolution of early Earth environments and microbial life
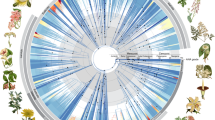
Phylogenomics and the rise of the angiosperms
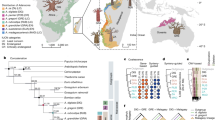
The rise of baobab trees in Madagascar
Introduction.
The reconstruction of organismal evolutionary history using phylogenetics is a fundamental method applied to many areas of biology. Single nucleotide polymorphisms (SNPs), one of the dominant forms of evolutionary change, have become an indispensable tool for phylogenetic analyses 1 , 2 , 3 , 4 . Phylogenies in the pre-genomic era relied on SNPs and conserved sites within a single locus, and was later extended to multiple loci, such as in multiple locus sequence typing (MLST). Although still valuable, these methods only consider evolutionary signals originating within a small fraction of the genome, are unable to capture the complete variation within species, and generally provide a weak phylogenetic signal, particularly within a species, and do not always reflect the true evolutionary history of species 5 . While phylogenetic analyses that use many conserved genes (orthologs) are a great improvement, these methods require annotated coding regions, whose predictions are not always accurate or available 6 . Furthermore, they are impacted by horizontal gene transfer (HGT) 7 , recombination 8 , rate heterogeneity 9 , and incomplete lineage sorting.
Genome-wide SNPs are one of the best measures of phylogenetic diversity as they can discriminate among closely related organisms and help resolve both short and long branches in a tree 10 , 11 . Since selectively neutral SNPs accumulate at a uniform rate, they can be used to measure divergence between species as well as strains 12 , 13 . Furthermore, due to the large number of SNPs found along the length of entire genomes, the use of whole-genome SNPs minimizes the impact of random sequencing and assembly errors that can impact individual loci, as well as biases due to individual genes under strong selective pressure. Some inherent biases remain with whole genome SNP approaches that are similar to loci-based phylogenies such as HGT, recombination, and rate heterogeneity. Although genome-wide sequencing now allows examination of the full complement of genomic variation, the number of completed and finished genomes are increasingly falling behind the generation of new draft genomes, due to the lack of computational or other resources. For example, of 94,126 total genomes in the NCBI RefSeq genome database, only 13.25% are complete (December 5, 2018 from ftp://ftp.ncbi.nlm.nih.gov/genomes/refseq/assembly_summary_refseq.txt) and a large fraction of available sequencing data still remains unassembled as evident by the much larger number (e.g. 360,929 for bacteria only) of whole genome projects in the sequence read archive (SRA) database (June 21, 2018 from https://www.ncbi.nlm.nih.gov/sra/). Several methods for whole-genome SNP discovery or phylogenetics have been previously described: SNPsFinder 14 , PhyloSNP 2 , kSNP 15 , WG-FAST 16 , NASP 17 , CFSAN 18 , CSI phylogeny 19 , REALPHY 20 , SNVPhyl 21 , SPANDx 22 , Snippy 23 , Lyve-set 24 , and Parsnp 25 . Some of these (e.g. SNPsFinder and PhyloSNP) are no longer under active development. Although the others are able to analyze raw reads to identify a core genome (the conserved portion among all genomes) and the SNPs within it, several of them cannot process assembled contigs or multiple complete genomes (e.g., CFSAN, SPANDx, Lyve-set), or will perform only a portion of the required functions to obtain a tree (e.g., Snippy), or identify SNPs from metagenomes (e.g. WG-FAST), and only few (CSI Phylogeny, REALPHY, SNVPhyl) can be accessed with a graphical user interface, limiting the user base to well-trained bioinformatics scientists. Moreover, almost all these tools have been restricted in their testing to bacterial organisms, and have only been used with genomes from within a single species. None have shown broad utility incorporating multiple species (i.e. genus-level phylogeny) or genera within a single tree, nor have any been tested on microbial eukaryotic genomes. In addition, most of these tools require users to select a reference genome, which can have dramatic impact on the alignments and resulting SNP calls 26 , and are unable to distinguish or map SNPs to their functional annotation, and hence cannot perform molecular evolution analysis.
Here, we present an open source workflow using a collection of existing bioinformatic tools for Phylogenetic and Molecular Evolutionary (PhaME) analysis that incorporates these additional features to allow more flexibility when studying the evolutionary relationships between closely related genomes (genera, species, and strains). PhaME is a whole-genome SNP-based phylogeny tool that identifies the core genome from input datasets (finished genomes, draft assembly contigs, and/or raw FASTQ reads), extracts core SNPs, parses them to coding or non-coding regions and as synonymous or non-synonymous SNPs, reconstructs a phylogeny, and performs molecular evolutionary analysis to identify genes under selection (Fig. 1 ). With any of the inputs there must be sufficient data covering a target genome of interest for acceptable SNP calling. PhaME thus accepts FASTA or FASTQ inputs corresponding either to genome sequencing data from isolates, or metagenomic data where the target organism has sufficient reads to allow SNP calling along much of the length of the genome. PhaME can be run either via the command line, or accessed through an accompanying webserver that can be installed locally. Here, we demonstrate PhaME’s ability to construct robust genus and species phylogenies using examples that span the tree of life, with up to thousands of genomes as input in the form of raw sequencing reads, draft assembled contigs, fully completed genomes, and even unassembled metagenomic reads.
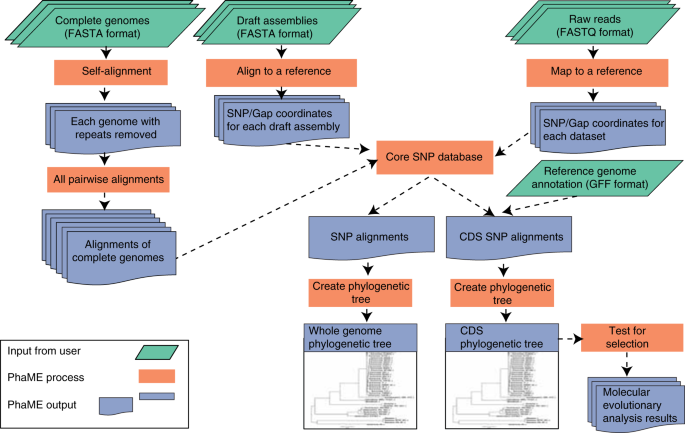
PhaME analysis workflow. The PhaME analysis workflow first identifies SNPs at orthologous positions in complete genomes, assembled contigs, and read datasets. First, nucmer is used to identify and mask repeats, and to perform pairwise alignments among all complete genomes. A reference genome is selected based on user criteria (See Methods). Contigs are then compared with the reference genome using nucmer, and reads are then mapped to the reference using Bowtie 2 or BWA. The SNP and gap coordinates are used to generate whole-genome core alignment. If an annotation file is provided, a separate alignment consisting of conserved positions only found in the CDS regions are also reported. RAxML, FastTree or IQ-TREE phylogenies are constructed using these alignments. If specified, PAML or HyPhy packages are used to test for selective pressure on genes with SNPs.
Results and Discussions
Implementation of phame with examples from across the tree of life.
To demonstrate different capabilities of PhaME and to validate the underlying algorithms, we tested PhaME on available bacterial genomes of Escherichia (together with related genera Shigella , and Salmonella ) and Burkholderia (together with recently reclassified genera of Caballeronia and Paraburkholderia , and Ralstonia as an outgroup), as well as on eukaryotic genomes from Saccharomyces , and on viral genomes of Zaire ebolavirus . We further examined the robustness of how PhaME handles raw reads, by comparing the placement of these datasets with the genome assemblies that resulted from these data, and have also investigated how well PhaME performs when including metagenomic samples in the form of raw reads (Table 1 ).
High resolution Escherichia phylotyping using PhaME
The model bacterium Escherichia coli has been extensively studied, including its diversity and phylogenetic history 27 . In previous studies, phylogenetic analysis using a single gene 28 , a set of genes 27 , 29 , 30 , 31 , SNPs 32 , 33 , and k -mer profiles 34 have consistently shown that E. coli strains are clustered into phylogenetic groups (A, B1, B2, D1, D2, and E) and different ‘species’ of Shigella also form distinct groups within the E. coli lineage and are not a separate genus 35 . To test whether PhaME can recapitulate the established E. coli tree topology, we first analyzed 35 complete genomes of E. coli , Shigella , using E. fergusonii as an outgroup (Table S1 ). PhaME detected 266,969 SNPs within the conserved core genome which consists of 2,159,296 aligned nucleotides (Table 1 ) . Similar to previously published phylogenies 27 , 31 , the maximum likelihood phylogeny constructed from these core SNPs grouped all E. coli and Shigella strains into their expected phylotypes (Fig. 2 ).
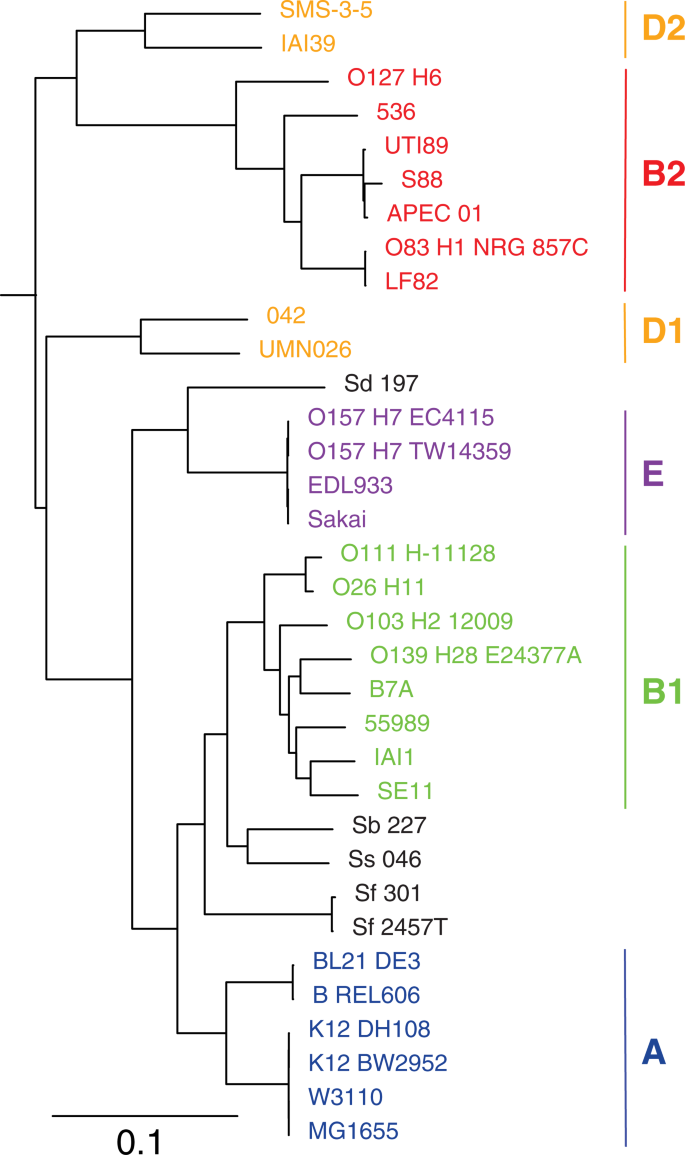
SNP based phylogeny of 35 Escherichia and Shigella genomes. All nodes have bipartition bootstrap support of 60% or greater. Clades are labeled with their corresponding E. coli phylogroups on the right. The tree was rooted with E. fergusonii ATCC 35469 as an outgroup that was removed in the figure. The scale bar indicates the number of substitutions per site.
To further test PhaME’s ability to successfully group the E. coli phylotypes when incorporating a larger number of genomes as well as representatives of related genera, we expanded our dataset to 676 genomes. We included genomes of Salmonella , the incorrectly named E. blattae (now reclassified as Shimwellia blattae 36 ) and E. hermannii (now reclassified as Atlantibacter 37 ), several ‘cryptic clades’ of Escherichia that have shown inconsistent phylogenetic placement in past studies 38 , 39 , and additional Escherichia and Shigella datasets (Table S2 ). Due to the significant increase in number and diversity of genomes in this expanded dataset, PhaME detected a much smaller conserved core genome of 134,062 positions, with 40,675 SNPs (Table 1 ). The resulting phylogeny showed genomes from E. coli phylotypes and Shigella accurately grouped into their respective clades (Fig. 3 ); Salmonella spp. S. bongori and S. enterica were clearly distinguished and were an outgroup to all Escherichia (Fig. S1 ). This tree also resolved contested evolutionary relationships among the environmental cryptic Escherichia lineages. For example, consistent with the 2009 MLST study, but in contrast with the 2011 single copy core gene study, the E. albertii lineage diverged before E. fergusonii 38 , 39 and E. fergusonii grouped with cryptic clade CI and not as an outgroup to all four cryptic clades (Fig. 3 ). In addition, the tree also supports reclassification and renaming of E. blattae to Shimwellia blattae 36 and E. hermanii to Atlantibacter hermanii 37 as these genomes clearly fell outside of Escherichia and Salmonella . In a separate naming issue, E. fergusonii FDAARGOS 170 (GCA_001471755.1) was placed within an E. coli clade. Since the construction of this tree, in its most recent assembly version in NCBI (GCA_001471755.2; May 1, 2018), it has now been reclassified as E. coli . PhaME was therefore able to recapitulate the established phylogeny of these related organisms, including distinguishing among E. coli phylotypes using hundreds of genomes from multiple genera while maintaining the internal Escherichia coli/Shigella topology (Fig. 3 ). Additionally, PhaME provides supporting evidence for reclassification of organisms that have only recently been renamed, and has helped resolve the evolutionary history among the cryptic clades of Escherichia .
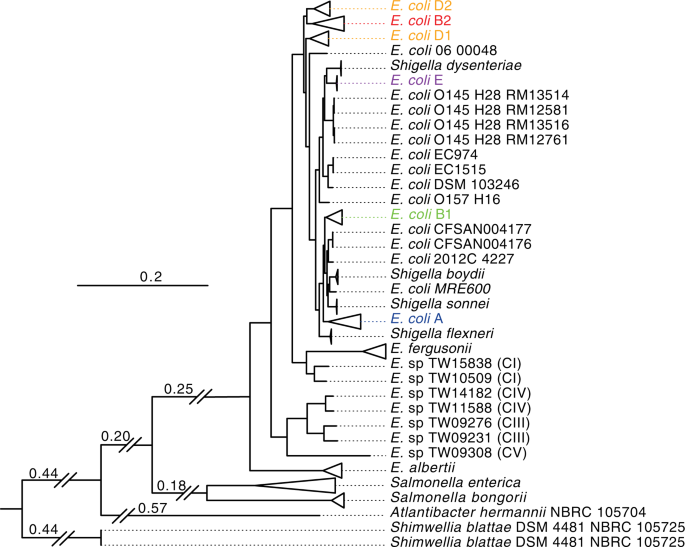
Inter-genus phylogeny using 676 Escherichia, Shigella, Salmonella, Shimwellia, and Atlantibacter datasets. Branches containing genomes from clades representing E. coli phylotypes and species with multiple strains are collapsed and labeled on the right with their corresponding phylotypes or species name. Genomes that did not form clades with any phylotypes are labeled with their full name. Genomes of cryptic Escherichia clades have their groups labeled in parenthesis from CI-CV. Two forward slashes in branches represents branches that were trimmed and the corresponding numbers represent the actual branch lengths. The tree was rooted with outgroup Shimwellia spp. The scale bar indicates the number of substitutions per site. A detailed tree that displays the names of all genomes and support values is shown in Fig. S1 .
At a granular level, we observed several additional cases of phylogenetic placement of genomes that were not in agreement with their designated species name. Four genomes annotated as E. coli are found with Shigella , namely E. coli MRE600 40 , E. coli 2012C 4227 41 , E. coli CFSAN004176 42 , 43 , and E. coli CFSAN004177 42 . Among these, E. coli MRE600 was previously shown to reside in a clade with S. flexneri , using a phylogeny inferred from seven housekeeping genes 40 . Our analysis instead places MRE600 as an outgroup of the S. boydii clade, based on core SNPs that are spread across 157 genes (Fig. S1 ). The other three outliers have been previously described as closely related to one another, and are known to express Shiga toxin 44 , which is consistent with the PhaME placement of this clade as an outgroup to S. sonnei , S. boydii and MRE600. Likewise, Shigella sp. PAMC 28760 was placed within phylotype A of E. coli , warranting a review of its name/description. With the rapid increase in available E. coli and related genomes and a shifting view of their phylogeny, we find that classic nomenclature with named phylotypes may be insufficient to categorize all new or future strains (Fig. 3 ). For example, four strains of E. coli O145 H28 form a sister clade to phylotype E and S. dysenteriae and do not group with any previously named phylotypes, consistent with prior observations 45 .
With the above examples, we have shown that PhaME is able to reconstruct known phylogenetic relationships using genome-wide scans for polymorphisms. The use of core genome SNPs allows for highly detailed trees capable or resolving strain to strain relationships. We have also illustrated how PhaME can help resolve long standing questions regarding species and genus-level relationships, and to better understand the granular relationship among strains, including the discovery of misnamed strains or species and potential issues with our current taxonomic nomenclature.
Burkholderia phylogeny from genomes, contigs, and raw reads
We used the large and diverse group of Burkholderia genomes (which have been recently divided into additional genera ( Paraburkholderia and Caballeronia ), to show the ability of PhaME to recreate correct phylogenies of a highly divergent set of related genomes, regardless of input data type. We used 158 complete and draft genomes and 55 raw (FASTQ) read datasets (Tables 1 , S3 ) to infer a genus-level phylogenetic tree (Figs. 4 , S2 ). PhaME calculated a core genome of 43,124 positions with a total of 15,180 core positions with SNPs (Table 1 ). The genomic plasticity of this disparate group, including genome sizes ranging from 3.6 Mbp for P. rhizoxinica (1 chromosome and 1 megaplasmid) (42) to 9.8 Mbp for P. xenovorans (2 chromosomes and a megaplasmid) (43), has contributed to the observed small core genome size. This also supports the hypothesis that Burkholderia are highly diverse lineage with a large ‘accessory genome’ 46 not shared among all of its members.
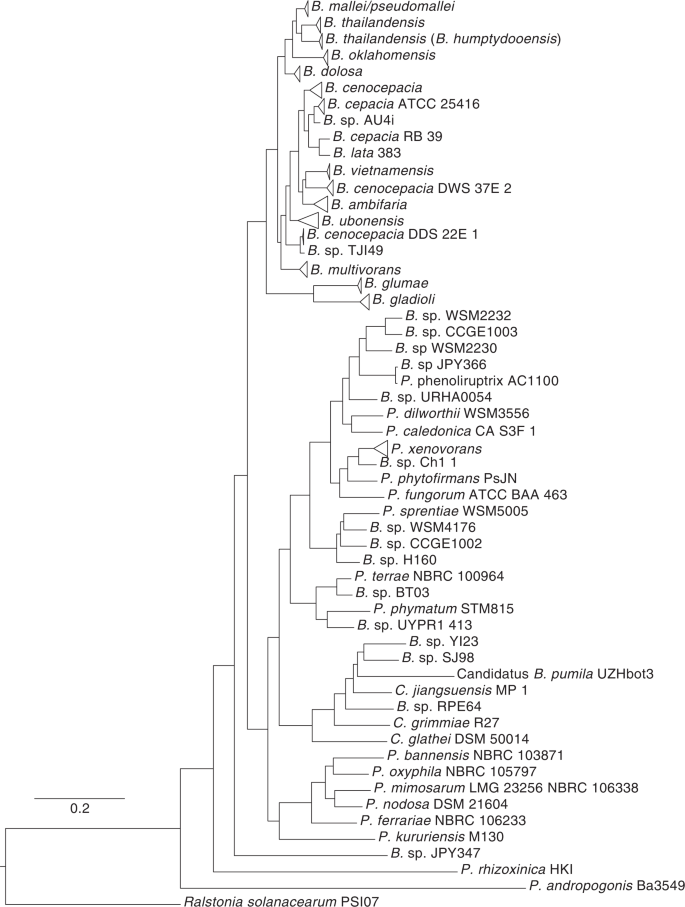
Phylogeny of Burkholderia, Paraburkholderia, Caballeronia, and Ralstonia using reads, contigs, and finished genomes. Maximum likelihood phylogeny from 213 samples (genomes, assemblies, and reads). Clades of the same species were collapsed and only the name of that species is shown. Ralstonia solanacearum PSI07 was used as an outgroup. The scale bar indicates the number of substitutions per site. A fully expanded and detailed tree can be found in Fig. S2 . Detailed trees showing relationships among genomes of only the Bc c or within the B. pseudomallei/mallei group can be found in Figs. S3 and S4 respectively.
PhaME recapitulated all major known clades 47 such as the B. cepacia complex ( Bc c) and the B. pseudomallei group from the input reads, assemblies and genomes (Figs. 4 , S2 ). While the overall topology of the tree grossly agrees with previously published phylogenies derived from concatenated housekeeping genes 47 , 48 , several novel observations can be made. Similar to the ribosomal protein tree 49 but disagreeing with a 21 conserved protein tree 48 , PhaME supports the placement of the P. kururiensis clade as ancestral to the remaining named Paraburkholderia as well as the Caballeronia clade, bringing into question the recent renaming of Burkholderia into three separate genera. The PhaME tree also shows two well-supported (bootstrap value ≥60) and separate clades of B. thailandensis agreeing with a proposal to rename one of the clades as B. humptydooensis (Figs. 4 , S2 ) 50 .
Similar to issues observed with the Escherichia-Salmonella phylogeny above, we also detected two B. cenocepacia genomes that are likely misnamed in NCBI taxonomy database (last accessed on September 26, 2019) 51 . Strain DDS 22E (GCA_000755725.1) is a close relative to the mango tree isolate B . TJI49 52 in the PhaME tree, and strain DWS 37E (GCA_000764955.1) lies within the B. ambifaria - B. vietnamiensis lineage. These examples further illustrate how PhaME, using a high-resolution whole genome SNP approach, can be used to resolve disputed phylogenetic placement and nomenclature of taxonomic groups.
Since PhaME also allows the inclusion of raw read datasets into whole genome SNP phylogenies, we evaluated the accuracy of their placement compared with the assemblies and finished genomes obtained from those datasets. We found that PhaME accurately places all 55 raw FASTQ read datasets as immediate sister lineages to their respective draft assemblies or complete genomes. These results illustrate the ability of PhaME to conduct highly robust strain-level phylogenetic analysis without the need for assembly of raw sequencing data.
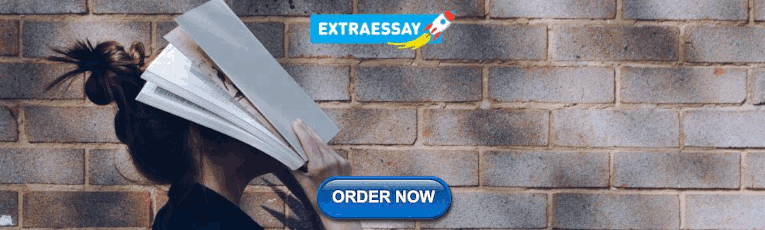
Rapid reexamination of sublineages using PhaME
The Burkholderia genera and clades therein have been uncharacteristically difficult to discriminate using conventional polyphasic, 16 S, recA, or MLST approaches 47 . For cases like these, the ability to select a subset of genomes for analysis from within a larger phylogeny, without the need to recalculate alignments, can provide more refined insight into not only the consistency and topology within the larger tree, but can help display differences in the core genome size and the SNPs within the core. The topology of the Bc c subtree (Fig. S3 ) remained the same as in the larger tree with all Burkholderia (and renamed genera). The core genome size with only Bc c increased more than ten-fold to 699,313 bp and the core SNPs increased six-fold to 97,524 SNPs (Table 1 ). These changes did not result in topology differences but instead improved branch length resolution. Likewise, when PhaME recalculated the core genome of the highly similar B. pseudomallei group, the core genome and the corresponding SNPs increased by 64 and 50-fold respectively (Table 1 ) with no changes in the overall topology (Fig. S4 ). This zoomed-in phylogenetic tree also highlights the recent clonal derivation of B. mallei from B. pseudomallei , with B. pseudomallei 576 as the most closely related sequenced ancestor and recapitulates the paraphyletic nature of the B. pseudomallei strains when B. mallei is considered its own species 53 .These results highlight the unique functionality of PhaME to zoom into clades within a larger tree, recalculate the core genome, and rapidly generate a finer-grained phylogeny without the need to realign all the data.
PhaME can be implemented on small eukaryotic genomes
Because PhaME can be readily applied to any taxonomic group of closely related genomes, we tested its implementation beyond bacterial lineages to larger eukaryotic genomes. Fungi are known to be a difficult group to resolve in terms of phylogenetic analysis 49 ; the phylogenetic placement of fungal species displays disparities between trees based on gene sequence analyses and those based on morphological characteristics (such as modes of reproduction). This is especially true of the ‘ Saccharomyces complex’, where the ITS regions and 26 S rDNA-based phylogenies do not show many well-supported clades 54 .
Due to the complexity and cost of assembling and finishing eukaryotic genomes, there are fewer complete genomes for many eukaryotic species. This is even the case for well-studied Saccharomyces , which only has 2 complete genomes. Therefore, the ability to make use of raw reads or draft assemblies/contigs can be of great value in characterizing eukaryotic genomes. We analyzed 194 Saccharomyces genome projects, including 7 sets of raw reads, 2 complete genomes, and 185 draft assemblies/contigs (Table S4 ) as input using PhaME. These datasets represent every major species from the Saccharomyces species complex , aside from hybrid species. PhaME calculated a core genome of 96,665 bp which consists of 24,244 SNP positions. The resulting tree topology agrees with previously published Saccharomyces species trees (Fig. S5 ) 55 , 56 , displaying PhaME’s ability to align and correctly recapitulate the phylogeny for small eukaryotic genomes.
A refined analysis focusing solely on the large S. cerevisiae clade consisting of 172 genomes increased the core genome size to 2,224,283 bp, highlighting the degree to which the core may change if a more closely related set of genomes is used, and highlights the great sequence divergence among eukaryotic species which resulted in a very small core genome size for genus-wide analysis. With such a dramatic increase in the core genome used for tree inference, one can observe much improved discrimination among strains of S. cerevisiae , with strong (>60) bootstrap support for most ancestral nodes (Fig. S6 ). This whole genome SNP analysis is a novel approach for reconstructing eukaryotic phylogenies, as the standard practice in the field is still reliant on one or several annotated genes 57 , 58 . PhaME can therefore provide rapid and robust discrimination among eukaryotic strains, and help better describe the relationships among closely related eukaryotic species, even when using raw read datasets.
Using PhaME with viral samples
The Zaire ebolavirus outbreak that began in 2014 was rapidly characterized by large-scale sequencing and assembly of genomes from several hundred patients 59 , 60 , 61 , 62 , 63 and provides a rich dataset for phylogenetic exploration. Many of the genomes and draft assemblies sequenced during the 2014/2015 Zaire ebolavirus outbreak, which encompassed a wide number of studies 59 , 60 , 61 , 62 , 63 , were recently combined into a phylogenetic study by Dudas et al . 64 . We used PhaME to re-analyze this dataset, and calculated 17,639 bp as the core genome size with 1,787 core SNP positions, using 1,359 Zaire ebolavirus genomes. The resulting PhaME tree topology is consistent with the combined maximum likelihood tree 64 , where distinct lineages are observed based largely on their geographical region of origin (Fig. S7 ) 59 , 60 , 61 , 62 , 63 .
Outbreaks such as this 2014–2015 Zaire ebolavirus scenario provide real-world situations where assembly of genomes is often the first step for epidemiological analysis. However, obtaining pure isolates for genome assembly is often difficult or time consuming, and assembly from metagenomic data can result in poor assembly, particularly if the target organism is not dominant or well represented in the sample. Since PhaME can accurately place raw reads in a phylogeny (as shown above for pure cultures/isolates) and because it directly aligns reads to a reference genome, it can potentially provide targeted phylogenetic analyses of an organism present within complex samples. We therefore tested PhaME’s ability to accurately place a known infectious agent within a phylogeny using reads derived directly from clinical samples.
For detailed analysis of the placement of read datasets in a phylogenetic context, we focused our analysis on viral genomes isolated from Sierra Leone. In addition to 1,031 genome assemblies, we included 93 raw read datasets that covered 99% of the Zaire ebolavirus genome, resulting in a 18,050 bp core genome, with 1,269 core SNP positions (Tables 1 , S5 ). These 93 raw read datasets were quite different from one another with respect to dataset size (from 30MB to 1.2GB), average depth of Zaire ebolavirus genome coverage (16× to 24,204×), and percentage of Zaire ebolavirus reads (0.21% to 99.88%) within the sample. Regardless of these differences and the abundance of Zaire ebolavirus reads, the PhaME tree placed 89/93 (96%) of the raw read datasets within the same branch as the sample-matched assembled genomes (Fig. S8 ). Compared with their respective assemblies, the variant analysis of the four remaining datasets differed by only one or two SNPs, which resulted in their slightly different placement within the tree. The SNP differences reflect existing allelic variation within the population of viruses in the samples, which can only be captured looking at the raw sequencing data, while assemblies generally reflect the consensus sequence. PhaME provides functionality to include or exclude variants based on fold coverage and proportion of reads that support the variant. These results highlight the power of PhaME to accurately phylogenetically characterize a target organism from a wide range of clinical viral samples without the need for assembly, even when it comprises only a minute fraction of a complex sample.
Analyzing raw metagenomic reads with PhaME
As demonstrated with the Zaire ebolavirus examples, we hypothesize that a target pathogen infecting a host (assuming a mostly clonal lineage of the target organism) will be accurately placed within a phylogeny due to the read mapping and SNP calling strategy in PhaME. We further investigated fecal samples from US patients having returned from Germany during the 2011 stx2 -positive Enteroaggregative E. coli (StxEAggEC) outbreak. In the context of metagenomic data, the ability to accurately phylogenetically place a target genome has two requirements: a) that a sufficient number of reads be sequenced from a target organism whose phylogeny is to be established; and b) that the target organism be a dominant clonal member of the population (including potential commensal members of that same species) in order to accurately identify SNPs belonging to the target strain. With E. coli as a commensal resident within the human gut, we tested the ability of PhaME to analyze fecal samples derived from two patients suspected to be infected with the 2011 StxEAggEC strain. Two fecal sample datasets (SRR2000383 and SRR2164314), each with >270 M reads, were included in a PhaME phylogeny using Escherichia and Shigella phylotype representatives ( Table S6 , Fig. 5 ). The target E. coli within the SRR2164314 fecal sample was clearly placed within the StxEAggEC phylogroup B1 outbreak strains, while the other sample was placed within a different E. coli clade not related to the outbreak strains (Fig. 5 ). These results suggest that one of the patients was indeed infected with the outbreak strain, while the other patient carried a strain from a different E. coli lineage.
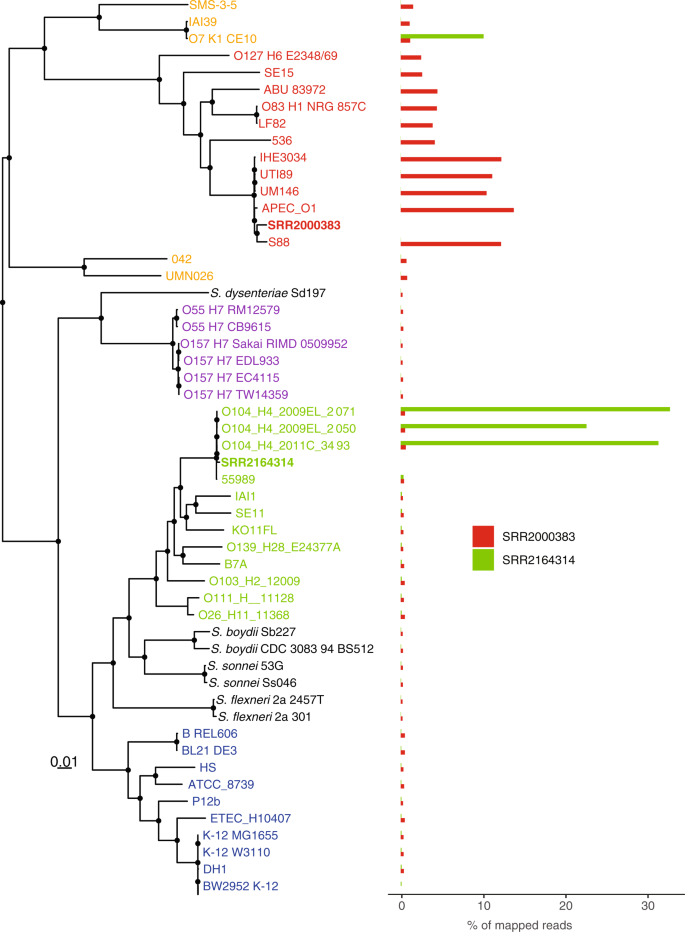
Read-based PhaME phylogenetic analysis of two human fecal metagenomics samples. Maximum likelihood tree showing 53 E. coli and Shigella genomes and the placement within the tree of the dominant E. coli present in the two metagenomes. The tree was rooted using outgroup E. fergusonii ATCC 35469. Nodes with bipartition bootstrap ≥60% are labeled with circles. The scale bar indicates the number of substitutions per site. The bar graph on the right shows the percentage of reads that mapped to each genome from the two metagenomic samples. Names of genomes are colored based on their phylotype association similar to Fig. 2 .
To validate the placement of these samples within the E. coli phylogeny, we further characterized the metagenomes by performing taxonomy classification on the reads and also by mapping them to the human reference genome. While only the SRR2000383 sample had a strong human signal (95.73%), the majority of the bacterial hits within both samples was E. coli , followed by a list of other enterics common in gut microbiomes (e.g. Eubacterium rectale , Enterococcus faecium , Lactococcus spp ., Bacteroides spp ., etc.; Table S7 ). We also independently mapped the metagenome reads from both samples to their best match among all the reference genomes used in the PhaME tree, in order to evaluate the distribution of reads among the genomes. In total, 68.23% of the reads from SRR2164314 and only 0.77% of SRR2000383 mapped to the E. coli genomes used in the PhaME tree. While all E. coli genomes recruited some reads from the metagenome datasets, the dominant signal from each sample corroborated their phylogenetic placement in the PhaME generated tree (Fig. 5 ). This further supports the use of PhaME to establish the phylogenetic placement of target organisms, including the ability to characterize complex human fecal microbiome samples, even when in the presence of host signal, other microbial community members, and also the conflicting presence of less abundant commensal strains of the same species.
Detecting signs of positive selections
Identifying SNPs found in coding regions enables further molecular evolutionary analyses as a post-phylogeny option that is provided in PhaME. By default, PhaME will use the HyPhy program with the Adaptive Branch-Site Random Effects Likelihood (aBSREL) 65 , 66 model for detecting episodic diversifying selection on genes containing at least one SNP. Using the reference E. coli - Shigella tree (Fig. 1 ), we tested the application of molecular evolutionary analysis within PhaME. A total of 1387/4388 genes were found to contain at least one SNP, of which 52 genes showed statistically significant evidence of positive selection (Table S8 ). Among these, 37 genes showed a single lineage under positive selection, while one gene (OmpA) showed signs of positive selection in 12 lineages. OmpA is an outer member protein that is usually abundantly found on the outer surface of the cell and plays an important role in pathogenesis through its contribution to adhesion, invasion, intracellular survival, and evasion of host defenses 67 . As this protein is consistently interacting with the host, E. coli OmpA has been previously shown to be under strong positive selection 68 . A more detailed analyses will be required to further characterize such signals of positive selection. Among phylogenetic tools that analyze genomes, this analytical feature is unique to PhaME and allows users to explore the evolution of organisms of interest beyond simple phylogenetic trees.
PhaME accessibility and performance
PhaME can be used on a wide variety of computing platforms from laptops with Mac OSX to Linux servers with multiple processors. Its source code is freely available in GitHub (https://github.com/LANL-Bioinformatics/PhaME) and can be installed for command line access with Bioconda (https://anaconda.org/bioconda/phame). PhaME can also be rapidly installed as a Docker container which supports use via command line, and also provides a web interface (Fig. S9 ) through which users can select data files, run jobs and view PhaME results (instructions at https://phame.readthedocs.io/). An example of the PhaME web interface is also hosted at https://www.edgebioinformatics.org/ 69 for use by the community.
In terms of PhaME performance, the overall computing load increases with the number and size of genomes, amount of alignments (to find the core genome), the number of SNPs, and the number of genes included in the molecular evolutionary analysis. We evaluated the wall clock time performance of PhaME to complete the full or partial analytical workflow ( Table S9 ) using genomes of Escherichia and Shigella (Table S6 ) and a metagenome dataset (SRR2000383). Because of PhaME’s flexibility in terms of processing raw data or using previously aligned data, we examined the performance of various components separately. We tested the performance of generating the core genome and SNP matrix after conducting all possible pairwise alignments of 53 complete genomes. PhaME took 27.8 hours using a single processor, or 2.7 hours when increasing the number of threads and processors to 16 (see Methods for details; Table S9 ). Performing all pairwise comparisons is a computationally demanding task, which is why PhaME can, as an alternative, pick a single reference based on smallest average MinHash distance which approximately represents k-mers that are shared between two genomes 70 . The performance with the same aforementioned dataset was assessed using this MinHash-based approach for the pairwise comparison step, and using FastTree to create a phylogeny. This option reduced the runtime to 1.5 hours using a single processor and 36 minutes using 16 processors. Because PhaME also allows the addition of new datasets to be added to an existing tree (SNP matrix), we evaluated the addition of a single raw read dataset (62GB, 317 M reads) to the 53 genome SNP matrix using PhaME, which performs read mapping, variant calling, and extraction of SNPs. The process took 4 hours using a single processor. A full-fledged PhaME analysis with the 53 genomes, including MinHash-based reference selection, pairwise alignments, SNP extraction, RAxML phylogeny inference, along with molecular evolution analysis with HyPhy, took 15.16 hours to complete with 32 processors. Additional performance tests can be found in Table S9 .
Conclusions
With the rapidly growing number of available genomes and NGS read datasets, it is becoming increasingly important to have holistic yet modular analysis tools that can deal with common sequencing outputs, such as complete genomes, assembled contigs, and raw sequencing data in a standardized fashion. It is also pertinent that tools are capable of accommodating a wide variety of research goals and applications, while catering to the needs of biologists without substantial bioinformatics background or training. Here, we described a new Phylogenetic and Molecular Evolutionary analysis package, PhaME, that can rapidly process hundreds of genomes and/or raw reads from organisms across the tree of life, that produces highly robust whole genome SNP phylogenetic trees, and that can additionally estimate selective pressure in core genes along lineages of the tree. PhaME is a unique phylogenetic tool that can correctly and quickly place raw sequencing data into phylogenetic context without the need for assembly, can zoom into select lineages for rapid reanalysis of a subset of genomes, and can incrementally add samples to previously analyzed datasets. While the full functionality of PhaME can be accessed through the command line, we have implemented an easy-to-use web-based interface that can accommodate biologists with a range of bioinformatics expertise. While phylogenetic analysis has traditionally required annotated genes, PhaME represents an automated workflow for today’s genomics era that enables computing the core whole genome alignment, phylogenetic trees, and molecular evolutionary analyses within a single tool.
Materials and Methods
Phame overview.
We present a tool for Phylogenetic and Molecular Evolution Analyses (PhaME) that can take raw NGS reads or assembled contig(s) that represent draft or complete genomes, will align the sequences to find conserved ‘core’ sections among the input genomes, identify all SNPs (in coding and non-coding regions of the genome), infer a phylogeny, and perform evolutionary analyses to identify signals of selective pressure in genes with SNPs. PhaME is primarily written in Perl incorporating several open source software packages including the BBMap v37.66 71 for MinHash distance calculations, MUMmer package with nucmer v3.1 72 for genome alignment, Bowtie 2 v2.1.0 73 or BWA v0.7.17 for read mapping, SAMtools v1.6 74 and BCFtools v1.6 for parsing mapped reads and calling SNPs, RAxML v8.2.10 75 , FastTree v2.1.10 76 , or IQ-TREE v1.5.5 77 for reconstruction of phylogenetic trees, and HyPhy v2.3.11 65 or PAML 78 for molecular evolution analyses. The overarching architecture of the PhaME analysis workflow is outlined in Fig. 1 and all steps are explained in detail in both the Supplementary Methods and online documentation at https://phame.readthedocs.io. All of the analyses were performed using PhaME v1.0.4 (DOI: 10.5281/zenodo.3458556).
PhaME can be used both via a command line interface and a web-based interface (Fig. S9 ). For command line use, PhaME can be installed using the source code from GitHub, or as a Bioconda package 79 . Detailed instructions on installation and for the GUI can be found on the GitHub page as well as in the online documentation at http://phame.readthedocs.io. Alternatively, we provide Docker containers that allow both command line use as well as an interactive web-interface that provides the ability to both submit jobs and view results. The PhaME web interface is deployed using a microservices framework in Docker containers that combines Flask (a python framework for user interfaces; http://flask.pocoo.org/), PostGREs (for user account database handling), Celery (for maintaining and executing PhaME; http://www.celeryproject.org/), and Redis (to keep track of task status; https://redis-py.readthedocs.io). After logging in, users are prompted to upload and select their input data through a web interface, select parameters using drop-down menus, and submit their jobs. Upon completion of a run, the users are emailed a link to a results page that contains an interactive tree viewer (https://github.com/cmzmasek/archaeopteryx-js) and pre-formatted tables. We have integrated PhaME as part of the EDGE bioinformatics platform 69 and have made available a PhaME webserver at https://edgebioinformatics.org/. This online web service requires registration via an email which will enable running the PhaME workflow and keep track of projects.
After installation, PhaME requires a “control file” that provides parameter information and the location of input and output folders. An example control file is shown in Fig. S10 . PhaME requires at least one reference genome, preferably a complete genome in FASTA format, consisting of one or more sequences that can be chromosomes, other replicons, contigs, etc. If molecular evolutionary analysis is desired, or if the user wishes to explore coding vs noncoding or synonymous vs nonsynonymous differences, the reference genome must have an associated annotation file (GFF or GFF3 file). Additional genomes in the form of raw next generation sequencing reads in FASTQ format (single or paired ends), or assembled contigs in FASTA format can also be included.
PhaME produces a number of output result files. The main outputs include pairwise alignment files, the final multiple sequence alignments of all positions with one or more SNPs, core genome alignment, maximum likelihood tree(s), text files summarizing the number of SNPs in pairwise comparisons between all aligned genomes, the position of SNPs in all input genomes, and information on whether these SNPs alter a codon and its associated amino acid. The molecular evolutionary analysis, when selected, are performed on each gene that contains a SNP and are presented in a series of files per gene.
Whole genome alignment and core genome and SNP discovery from genomes, contigs, and reads
All complete genomes input into PhaME are initially subjected to self-comparisons using nucmer in order to remove duplicated regions or other highly similar ‘repetitive’ elements to avoid possible misleading alignments. The complete genomes then undergo pairwise whole genome alignment using nucmer in all combinations when the user wants to create a database for faster future analysis or wants all vs. all comparisons. Otherwise (default) only pairwise alignments against a designated reference genome is carried out. The reference genome can be specified by the user in the control file (from among the input genomes), picked randomly from the input genomes, or (default) identified using the MinHash distance calculated using BBMap v. 37.66 71 to identify a complete genome with the shortest total distance among all input genomes. Moreover, based on the proportion of query genomes that aligned with a reference genome, users can automatically control the inclusion or exclusion of similar or divergent genomes by specifying it in “cutoff” parameter in the control file. This option also allows users to remove incomplete genomes that are not of desirable completion compared to the reference. Gap regions from the alignments (unaligned segments ≥1 nucleotide) are removed from downstream analyses. Input raw read datasets (either single or paired-end) are then aligned to the reference genome using Bowtie 2 (default parameters) or BWA MEM (default parameters). The mapping results are then parsed using SAMtools, BCFtools and Perl scripts to identify SNPs found in shared genomic locations. An orthologous SNP alignment is created for each genome, contig, and/or read set, and contains the nucleotides that are found in all genomes, and where at least one genome differs at that position. Given an annotation file in GFF or GFF3 format, the workflow can distinguish SNPs present within coding sequences (CDS) from those present in intergenic regions. The SNPs identified in the pairwise genome alignments as well as those identified using mapped reads are available as text files or vcf files (*.snps/*.vcfs). These SNP matrices allow for rapid recalculation of the core SNPs for any subset of genomes and for reconstruction of subtrees. In addition, pairwise SNP profiles for the core genome (*coreMatrix.txt) as well as for the core coding genome (*CDSMatrix.txt) and the core intergenic genome (*intergenicMatrix.txt) are also available.
Phylogenetic reconstruction
The core genome or SNP alignment is used to construct a phylogenetic tree. If a GFF annotation file was provided, an additional tree can be generated from the subset of SNPs found only within coding sequences or only within intergenic regions. The phylogenetic trees are inferred using FastTree (default) and/or the RAxML maximum likelihood method and/or the IQ-TREE method. In the first two cases, PhaME builds the tree using General Time Reversible (GTR) model, accounting for gamma rate variation and proportion of invariable sites (-m GTRGAMMAI in RAxML). If IQ-TREE is chosen, the program picks a model that fits the data using their ModelFinder 80 . If RAxML or IQ-TREE are chosen, one can also perform a number of bootstraps (specified in the control file).
Molecular evolutionary analyses
PhaME can automatically perform some of the basic molecular evolutionary analyses. Using the reference GFF file, all homologous genes containing SNPs are used to test for positive or purifying selection through the implementation of methods within the HyPhy (hyphy.org) 65 or PAML 78 packages. Both packages can test for the presence of positively selected sites and lineages by allowing the dN/dS ratio (ω) to vary among sites and lineages. The adaptive branch-site REL test for episodic diversification (aBSREL) 66 model in the HyPhy package is used to detect instances of episodic diversifying and positive selection. If PAML is selected, the M1a-M2a and M7-M8 nested models are implemented. In the latter case, the likelihood ratio test between the null models (M1a and M8) and the alternative model (M2a and M7) at a significance cutoff of 5% provides information on how the genes are evolving. The results for each gene are then summarized in a table containing information on whether the gene is evolving under positive, neutral, or purifying selection, along with p-values. HyPhy is run with a model, which specifically looks for sign of positive selection in given sets of genes. The analysis produces a list of JSON files corresponding to each gene which can be uploaded to vision.hyphy.org/absrel for further analysis. We opted to provide PAML as an option, however we recommend using HyPhy for large projects due to its speed and concise output.
Analysis of complete E. coli , Shigella spp. genomes
Complete genomes of E. coli from different phylotypes and Shigella spp. and Escherichia fergusonii were analyzed using PhaME (Table S1 ). Briefly, PhaME picked E. coli IAI1 as the reference genome based on MinHash distance and all other genomes/assemblies were aligned against the reference using nucmer. Orthologous positions were kept, the core genome was calculated, and the subset consisting of only the polymorphic sites were used to reconstruct a maximum likelihood phylogenetic tree using RAxML (GTRGAMMAI) with 100 bootstraps. E. fergusonii was used to root the tree.
Analysis of Escherichia spp., Shigella spp., and Salmonella spp
Complete genomes of E. coli, Salmonella , and Shigella that were available during the time of analyses (assembly_summary_genbank.txt accessed June 20, 2017) including available genomes (complete or/and draft) for other species of Escherichia were used in the analyses. S. enterica CFSAN033543 was picked as the reference by PhaME based on MinHash distances and the resultant polymorphic sites were used to reconstruct a phylogenetic tree using FastTree, and was rooted with the Salmonella clade.
Analysis of Burkholderia spp., Paraburkholderia spp., and Caballeronia spp. using PhaME
Complete, draft genomes, and raw reads of Burkholderia spp. including former Burkholderia genomes from the newly renamed genera Paraburkholderia and Caballeronia ( Table S3 ) were analyzed using PhaME. Genomes from genera that have multiple available genomes were randomly selected to have a mixture of complete and draft genomes. Ralstonia solanacearum PSI07 was also included and used as an outgroup and PhaME picked B. mallei NCTC 10247 as the reference genome based on MinHash distances. Raw reads were first quality controlled using FaQCs v2.09 81 and then added to PhaME analysis. Orthologous polymorphic positions were kept and used to build a maximum likelihood tree using RAxML (GTRGAMMAI) with 100 bootstrap supports.
Subsets of the genomes that belong to the Bc c or the B. pseudomallei groups were further analyzed using PhaME (Table S3 ). Genomes that belong to the corresponding clades were selected from the whole Burkholderia tree and the original alignments were used to recalculate the core genome and core SNPs, which were then used to reconstruct maximum likelihood tree using RAxML (GTRGAMMAI) with 100 bootstraps.
Analysis of Saccharomyces spp
210 available complete, draft, and raw reads of Saccharomyces genomes were analyzed using PhaME (Table S4 ). Since the majority of available genomes were from S. cerevisiae , we randomly sub sampled those genomes so that the results were not too heavily biased with S. cerevisiae genomes. Although the majority of available genomes were from S. cerevisiae , there were genomes from most of the recognized species of Saccharomyces , including S. kudriavzevii , S. bayanus, S. eubayanus, S. paradoxus, S. mikatae, S. pastorianus , and S. arboricola . The complete genome of S. cerevisiae S288C was selected as a reference based on MinHash distances and all genomes were aligned to the reference using nucmer. To increase the size of the core genome while including all divergent species of Saccharomyces , we removed datasets that aligned to less than 15% of the reference genome. The conserved polymorphic sites were then used to reconstruct a phylogenetic tree using RAxML (GTRGAMMAI) with 100 bootstraps. Polymorphic sites were further divided into coding and non-coding regions. We also analyzed the subset of genomes that were found in the monophyletic lineage of S. cerevisiae using PhaME to obtain higher resolution phylogeny with the same reference that was used for Saccharomyces spp. analysis.
Analysis of Zaire ebolavirus
1,610 Zaire ebolavirus genomes that were summarized in a recent overview publication was obtained from https://github.com/ebov/space-time 64 . Only genomes that had a linear coverage of 99% or greater to the PhaME picked reference genome (Accession ID: KT725295) were kept (i.e. 18,693 bp or greater) and processed through PhaME. For the purpose of this analysis, all genomes were treated as “complete” to perform self-alignments using nucmer.
For raw reads analysis, we focused on a subset of genomes (1,031) that were isolated from Sierra Leone and added 138 randomly selected raw reads from the Sequence Read Archive (SRA) that correspond to analyzed genomes. We also included some Guinea samples (5) for rooting the tree. These raw reads and assembled genomes were analyzed together using PhaME (Table S5 ). Briefly, raw reads were quality controlled with FaQCs v2.09 81 before they were mapped to reference genome (KR105277) using BWA and only samples that had a linear coverage of 99% or greater were kept to only analyze high quality genomes. To be reported as a SNP for the purpose of tree inference, the default requirement is set to 60% of the reads mapped to the SNP position must agree with the alternate allelic variant. For both analyses, the conserved orthologous positions that included monomorphic positions were then used to reconstruct a phylogenetic tree using IQ-TREE 77 .
Analysis of metagenomes using PhaME
Two metagenomes (SRR2000383 and SRR2164314) from the 2011 German outbreak, along with a suite of E. coli and Shigella genomes (Table S6 ) representing all phylotypes were used as input into PhaME. Raw reads from metagenomes were first quality controlled with FaQCs v2.09 81 . A reference genome was picked based on MinHash distances, and all other genomes and the two metagenomes were aligned against it ( E. coli str. K-12 substr. W3110). The resulting orthologous polymorphic positions were then used to reconstruct a maximum likelihood tree using RAxML with 100 bootstraps. As an orthogonal method to evaluate the placement of metagenomic data within the tree, we mapped the two metagenome datasets to all genomes used in the phylogeny. All genomes were thus concatenated into a single FASTA file, which was used to create a Bowtie 2 index and then reads from the metagenomes were mapped and the percentage of the reads (best hit) that were mapped to each genome was reported.
An additional independent analysis of the reads was undertaken to observe the broader taxonomic composition of the metagenomic samples. Briefly, the EDGE Bioinformatics platform 69 was used to map the reads to the human reference genome to look at the contribution of host-derived data. The remaining reads were processed using GOTTCHA (version 2) 82 to find the proportion of reads that map to taxonomically unique segments of RefSeq genomes.
Molecular evolution analysis of E. coli genomes
53 genomes (Table S6 ) consisting of E. coli, E. fergusonii , and Shigella spp. were processed using PhaME to detect the list of genes that are evolving under positive selection, using HyPhy. Genes with at least one SNP and 0 gapped regions within them were identified, converted to amino acid sequences, aligned, and then checked for positive selection using aBSREL 66 model of HyPhy. Because the size of the core genome decreases with the inclusion of additional genomes, the core genome becomes increasingly enriched in highly conserved genes and depleted in accessory genes making the choice of genomes to be included in PhaME analysis, a critical step for molecular evolutionary studies.
Performance analysis of PhaME
We tested the performance of PhaME using a set of E. coli , Shigella , and E. fergusonii genomes (Table S6 ) on a dedicated server of a Dell PowerEdge R815 model with 512GB of RAM and a quad-processor AMD Opteron(tm) Processor 6376 @ 2.3 GHz with Bright Computing’s version of CentOS 7 of kernel version 3.10.0–229.el7.x86_64. Since PhaME is highly customizable and can process a wide range of genomic file types, we tested the performance of PhaME under different scenarios (Table S8 ) and reported some of the performance values using total wall clock time.
Data availability
Genomes, complete and incomplete, were downloaded based on ftp addresses from the assembly_summary_genbank.txt file downloaded from NCBI (ftp://ftp.ncbi.nlm.nih.gov/genomes/genbank/assembly_summary_genbank.txt) (accessed June 20, 2017). Reads were downloaded from SRA database ( https://www.ncbi.nlm.nih.gov/sra ). GenBank accession numbers for the sequencing data and genomes used in this study can be found in Tables S1 – S9 . The PhaME workflow together with documentation can be found at https://github.com/LANL-Bioinformatics/PhaME. PhaME Control files that were used for the analyses can be found at https://github.com/mshakya/PhaME-manuscript-data ( https://doi.org/10.5281/zenodo.3610728 ).
Lee, T. H., Guo, H., Wang, X., Kim, C. & Paterson, A. H. SNPhylo: a pipeline to construct a phylogenetic tree from huge SNP data. BMC Genomics 15 , 162, https://doi.org/10.1186/1471-2164-15-162 (2014).
Article PubMed PubMed Central Google Scholar
Faison, W. J. et al . Whole genome single-nucleotide variation profile-based phylogenetic tree building methods for analysis of viral, bacterial and human genomes. Genomics 104 , 1–7, https://doi.org/10.1016/j.ygeno.2014.06.001 (2014).
Article CAS PubMed Google Scholar
McNally, K. L. et al . Genomewide SNP variation reveals relationships among landraces and modern varieties of rice. Proc. Natl Acad. Sci. USA 106 , 12273–12278, https://doi.org/10.1073/pnas.0900992106 (2009).
Article ADS PubMed Google Scholar
Sankarasubramanian, J., Vishnu, U. S., Gunasekaran, P. & Rajendhran, J. A genome-wide SNP-based phylogenetic analysis distinguishes different biovars of Brucella suis. Infect. Genet. Evol. 41 , 213–217, https://doi.org/10.1016/j.meegid.2016.04.012 (2016).
Pamilo, P. & Nei, M. Relationships between gene trees and species trees. Mol. Biol. Evol. 5 , 568–583 (1988).
CAS PubMed Google Scholar
Lomsadze, A., Gemayel, K., Tang, S. & Borodovsky, M. Modeling leaderless transcription and atypical genes results in more accurate gene prediction in prokaryotes. Genome Res. 28 , 1079–1089, https://doi.org/10.1101/gr.230615.117 (2018).
Article CAS PubMed PubMed Central Google Scholar
Doolittle, W. F. Phylogenetic classification and the universal tree. Sci. 284 , 2124–2129, https://doi.org/10.1126/science.284.5423.2124 (1999).
Article CAS Google Scholar
Posada, D. & Crandall, K. A. The effect of recombination on the accuracy of phylogeny estimation. J. Mol. Evol. 54 , 396–402, https://doi.org/10.1007/s00239-001-0034-9 (2002).
Article ADS CAS PubMed Google Scholar
Bevan, R. B., Bryant, D. & Lang, B. F. Accounting for gene rate heterogeneity in phylogenetic inference. Syst. Biol. 56 , 194–205, https://doi.org/10.1080/10635150701291804 (2007).
Girault, G., Blouin, Y., Vergnaud, G. & Derzelle, S. High-throughput sequencing of Bacillus anthracis in France: investigating genome diversity and population structure using whole-genome SNP discovery. BMC Genomics 15 , 288, https://doi.org/10.1186/1471-2164-15-288 (2014).
Griffing, S. M. et al . Canonical Single Nucleotide Polymorphisms (SNPs) for High-Resolution Subtyping of Shiga-Toxin Producing Escherichia coli (STEC) O157:H7. PLoS One 10 , e0131967, https://doi.org/10.1371/journal.pone.0131967 (2015).
Schork, N. J., Fallin, D. & Lanchbury, J. S. Single nucleotide polymorphisms and the future of genetic epidemiology. Clin. Genet. 58 , 250–264 (2000).
Filliol, I. et al . Global phylogeny of Mycobacterium tuberculosis based on single nucleotide polymorphism (SNP) analysis: insights into tuberculosis evolution, phylogenetic accuracy of other DNA fingerprinting systems, and recommendations for a minimal standard SNP set. J. Bacteriol. 188 , 759–772, https://doi.org/10.1128/JB.188.2.759-772.2006 (2006).
Song, J., Xu, Y., White, S., Miller, K. W. & Wolinsky, M. SNPsFinder–a web-based application for genome-wide discovery of single nucleotide polymorphisms in microbial genomes. Bioinforma. 21 , 2083–2084, https://doi.org/10.1093/bioinformatics/bti176 (2005).
Gardner, S. N., Slezak, T. & Hall, B. G. kSNP3.0: SNP detection and phylogenetic analysis of genomes without genome alignment or reference genome. Bioinforma. 31 , 2877–2878, https://doi.org/10.1093/bioinformatics/btv271 (2015).
Sahl, J. W. et al . Phylogenetically typing bacterial strains from partial SNP genotypes observed from direct sequencing of clinical specimen metagenomic data. Genome Med. 7 , 52, https://doi.org/10.1186/s13073-015-0176-9 (2015).
Sahl, J. W. et al . NASP: an accurate, rapid method for the identification of SNPs in WGS datasets that supports flexible input and output formats. Microb. Genom. 2 , e000074, https://doi.org/10.1099/mgen.0.000074 (2016).
Davis, S. et al . CFSAN SNP Pipeline: an automated method for constructing SNP matrices from next-generation sequence data. PeerJ Computer Sci. 1 , e20 (2015).
Article Google Scholar
Kaas, R. S., Leekitcharoenphon, P., Aarestrup, F. M. & Lund, O. Solving the problem of comparing whole bacterial genomes across different sequencing platforms. PLoS One 9 , e104984, https://doi.org/10.1371/journal.pone.0104984 (2014).
Article ADS CAS PubMed PubMed Central Google Scholar
Bertels, F., Silander, O. K., Pachkov, M., Rainey, P. B. & van Nimwegen, E. Automated reconstruction of whole-genome phylogenies from short-sequence reads. Mol. Biol. Evol. 31 , 1077–1088, https://doi.org/10.1093/molbev/msu088 (2014).
Petkau, A. et al . SNVPhyl: a single nucleotide variant phylogenomics pipeline for microbial genomic epidemiology. Microb. Genom. 3 , e000116, https://doi.org/10.1099/mgen.0.000116 (2017).
Sarovich, D. S. & Price, E. P. SPANDx: a genomics pipeline for comparative analysis of large haploid whole genome re-sequencing datasets. BMC Res. Notes 7 , 618, https://doi.org/10.1186/1756-0500-7-618 (2014).
snippy: fast bacterial variant calling from NGS reads (2015).
Katz, L. S. et al . A Comparative Analysis of the Lyve-SET Phylogenomics Pipeline for Genomic Epidemiology of Foodborne Pathogens. Front. Microbiol. 8 , 375, https://doi.org/10.3389/fmicb.2017.00375 (2017).
Treangen, T. J., Ondov, B. D., Koren, S. & Phillippy, A. M. The Harvest suite for rapid core-genome alignment and visualization of thousands of intraspecific microbial genomes. Genome Biol. 15 , 524, https://doi.org/10.1186/PREACCEPT-2573980311437212 (2014).
Bush, S. J. et al . Genomic diversity affects the accuracy of bacterial SNP calling pipelines. bioRxiv , 653774 (2019).
Touchon, M. et al . Organised genome dynamics in the Escherichia coli species results in highly diverse adaptive paths. PLoS Genet. 5 , e1000344, https://doi.org/10.1371/journal.pgen.1000344 (2009).
Fukushima, M., Kakinuma, K. & Kawaguchi, R. Phylogenetic analysis of Salmonella, Shigella, and Escherichia coli strains on the basis of the gyrB gene sequence. J. Clin. Microbiol. 40 , 2779–2785 (2002).
Sahl, J. W. & Rasko, D. A. Analysis of global transcriptional profiles of enterotoxigenic Escherichia coli isolate E24377A. Infect. Immun. 80 , 1232–1242, https://doi.org/10.1128/IAI.06138-11 (2012).
Ahmed, S. A. et al . Genomic comparison of Escherichia coli O104:H4 isolates from 2009 and 2011 reveals plasmid, and prophage heterogeneity, including shiga toxin encoding phage stx2. PLoS One 7 , e48228, https://doi.org/10.1371/journal.pone.0048228 (2012).
Ogura, Y. et al . Comparative genomics reveal the mechanism of the parallel evolution of O157 and non-O157 enterohemorrhagic Escherichia coli. Proc. Natl Acad. Sci. USA 106 , 17939–17944, https://doi.org/10.1073/pnas.0903585106 (2009).
Hommais, F., Pereira, S., Acquaviva, C., Escobar-Paramo, P. & Denamur, E. Single-nucleotide polymorphism phylotyping of Escherichia coli. Appl. Env. Microbiol. 71 , 4784–4792, https://doi.org/10.1128/AEM.71.8.4784-4792.2005 (2005).
Pettengill, E. A., Pettengill, J. B. & Binet, R. Phylogenetic Analyses of Shigella and Enteroinvasive Escherichia coli for the Identification of Molecular Epidemiological Markers: Whole-Genome Comparative Analysis Does Not Support Distinct Genera Designation. Front. Microbiol. 6 , 1573, https://doi.org/10.3389/fmicb.2015.01573 (2015).
Article PubMed Google Scholar
Sims, G. E. & Kim, S. H. Whole-genome phylogeny of Escherichia coli/Shigella group by feature frequency profiles (FFPs). Proc. Natl Acad. Sci. USA 108 , 8329–8334, https://doi.org/10.1073/pnas.1105168108 (2011).
Chaudhuri, R. R. & Henderson, I. R. The evolution of the Escherichia coli phylogeny. Infect. Genet. Evol. 12 , 214–226, https://doi.org/10.1016/j.meegid.2012.01.005 (2012).
Priest, F. G. & Barker, M. Gram-negative bacteria associated with brewery yeasts: reclassification of Obesumbacterium proteus biogroup 2 as Shimwellia pseudoproteus gen. nov., sp. nov., and transfer of Escherichia blattae to Shimwellia blattae comb. nov. Int. J. Syst. Evol. Microbiol. 60 , 828–833, https://doi.org/10.1099/ijs.0.013458-0 (2010).
Hata, H. et al . Phylogenetics of family Enterobacteriaceae and proposal to reclassify Escherichia hermannii and Salmonella subterranea as Atlantibacter hermannii and Atlantibacter subterranea gen. nov., comb. nov. Microbiol. Immunol. 60 , 303–311, https://doi.org/10.1111/1348-0421.12374 (2016).
Walk, S. T. et al . Cryptic lineages of the genus Escherichia. Appl. Env. Microbiol. 75 , 6534–6544, https://doi.org/10.1128/AEM.01262-09 (2009).
Luo, C. et al . Genome sequencing of environmental Escherichia coli expands understanding of the ecology and speciation of the model bacterial species. Proc. Natl Acad. Sci. USA 108 , 7200–7205, https://doi.org/10.1073/pnas.1015622108 (2011).
Kurylo, C. M. et al . Genome Sequence and Analysis of Escherichia coli MRE600, a Colicinogenic, Nonmotile Strain that Lacks RNase I and the Type I Methyltransferase, EcoKI. Genome Biol. Evol. 8 , 742–752, https://doi.org/10.1093/gbe/evw008 (2016).
Lindsey, R. L. et al . Complete Genome Sequences of Two Shiga Toxin-Producing Escherichia coli Strains from Serotypes O119:H4 and O165:H25. Genome Announc 3 , https://doi.org/10.1128/genomeA.01496-15 (2015).
Lorenz, S. C., Monday, S. R., Hoffmann, M., Fischer, M. & Kase, J. A. Plasmids from Shiga Toxin-Producing Escherichia coli Strains with Rare Enterohemolysin Gene (ehxA) Subtypes Reveal Pathogenicity Potential and Display a Novel Evolutionary Path. Appl. Env. Microbiol. 82 , 6367–6377, https://doi.org/10.1128/AEM.01839-16 (2016).
Lorenz, S. C. et al . Complete Genome Sequences of Four Enterohemolysin-Positive (ehxA) Enterocyte Effacement-Negative Shiga Toxin-Producing Escherichia coli Strains. Genome Announc 4 , https://doi.org/10.1128/genomeA.00846-16 (2016).
Lorenz, S. C., Gonzalez-Escalona, N., Kotewicz, M. L., Fischer, M. & Kase, J. A. Genome sequencing and comparative genomics of enterohemorrhagic Escherichia coli O145:H25 and O145:H28 reveal distinct evolutionary paths and marked variations in traits associated with virulence & colonization. BMC Microbiol. 17 , 183, https://doi.org/10.1186/s12866-017-1094-3 (2017).
Cooper, K. K. et al . Comparative genomics of enterohemorrhagic Escherichia coli O145:H28 demonstrates a common evolutionary lineage with Escherichia coli O157:H7. BMC Genomics 15 , 17, https://doi.org/10.1186/1471-2164-15-17 (2014).
Chain, P. S. et al . Burkholderia xenovorans LB400 harbors a multi-replicon, 9.73-Mbp genome shaped for versatility. Proc. Natl Acad. Sci. USA 103 , 15280–15287, https://doi.org/10.1073/pnas.0606924103 (2006).
Depoorter, E. et al . Burkholderia: an update on taxonomy and biotechnological potential as antibiotic producers. Appl. Microbiol. Biotechnol. 100 , 5215–5229, https://doi.org/10.1007/s00253-016-7520-x (2016).
Sawana, A., Adeolu, M. & Gupta, R. S. Molecular signatures and phylogenomic analysis of the genus Burkholderia: proposal for division of this genus into the emended genus Burkholderia containing pathogenic organisms and a new genus Paraburkholderia gen. nov. harboring environmental species. Front. Genet. 5 , 429, https://doi.org/10.3389/fgene.2014.00429 (2014).
Hittinger, C. T. Saccharomyces diversity and evolution: a budding model genus. Trends Genet. 29 , 309–317, https://doi.org/10.1016/j.tig.2013.01.002 (2013).
Tuanyok, A. et al . Burkholderia humptydooensis sp. nov., a New Species Related to Burkholderia thailandensis and the Fifth Member of the Burkholderia pseudomallei Complex. Appl Environ Microbiol 83 , https://doi.org/10.1128/AEM.02802-16 (2017).
Daligault, H. E. et al . Whole-genome assemblies of 56 burkholderia species. Genome Announc 2 , : https://doi.org/10.1128/genomeA.01106-14 (2014).
Khan, A., Asif, H., Studholme, D. J., Khan, I. A. & Azim, M. K. Genome characterization of a novel Burkholderia cepacia complex genomovar isolated from dieback affected mango orchards. World J. Microbiol. Biotechnol. 29 , 2033–2044, https://doi.org/10.1007/s11274-013-1366-5 (2013).
Godoy, D. et al . Multilocus sequence typing and evolutionary relationships among the causative agents of melioidosis and glanders, Burkholderia pseudomallei and Burkholdefia mallei (vol 41, pg 2068, 2003). J. Clin. Microbiology 41 , 4913–4913, https://doi.org/10.1128/Jcm.41.10.4913.2003 (2003).
Kurtzman, C. P. & Robnett, C. J. Phylogenetic relationships among yeasts of the ‘Saccharomyces complex’ determined from multigene sequence analyses. FEMS Yeast Res. 3 , 417–432 (2003).
Peter, J. et al . Genome evolution across 1,011 Saccharomyces cerevisiae isolates. Nat. 556 , 339–344, https://doi.org/10.1038/s41586-018-0030-5 (2018).
Article ADS CAS Google Scholar
Dujon, B. A. & Louis, E. J. Genome Diversity and Evolution in the Budding Yeasts (Saccharomycotina). Genet. 206 , 717–750, https://doi.org/10.1534/genetics.116.199216 (2017).
Gallone, B. et al . Domestication and Divergence of Saccharomyces cerevisiae Beer Yeasts. Cell 166 , 1397–1410 e1316, https://doi.org/10.1016/j.cell.2016.08.020 (2016).
Sulo, P. et al . The evolutionary history of Saccharomyces species inferred from completed mitochondrial genomes and revision in the ‘yeast mitochondrial genetic code’. DNA Res. 24 , 571–583, https://doi.org/10.1093/dnares/dsx026 (2017).
Gire, S. K. et al . Genomic surveillance elucidates Ebola virus origin and transmission during the 2014 outbreak. Sci. 345 , 1369–1372, https://doi.org/10.1126/science.1259657 (2014).
Carroll, M. W. et al . Temporal and spatial analysis of the 2014-2015 Ebola virus outbreak in West Africa. Nat. 524 , 97–101, https://doi.org/10.1038/nature14594 (2015).
Simon-Loriere, E. et al . Distinct lineages of Ebola virus in Guinea during the 2014 West African epidemic. Nat. 524 , 102–104, https://doi.org/10.1038/nature14612 (2015).
Park, D. J. et al . Ebola Virus Epidemiology, Transmission, and Evolution during Seven Months in Sierra Leone. Cell 161 , 1516–1526, https://doi.org/10.1016/j.cell.2015.06.007 (2015).
Baize, S. et al . Emergence of Zaire Ebola virus disease in Guinea. N. Engl. J. Med. 371 , 1418–1425, https://doi.org/10.1056/NEJMoa1404505 (2014).
Dudas, G. et al . Virus genomes reveal factors that spread and sustained the Ebola epidemic. Nat. 544 , 309–315, https://doi.org/10.1038/nature22040 (2017).
Pond, S. L., Frost, S. D. & Muse, S. V. HyPhy: hypothesis testing using phylogenies. Bioinforma. 21 , 676–679, https://doi.org/10.1093/bioinformatics/bti079 (2005).
Smith, M. D. et al . Less is more: an adaptive branch-site random effects model for efficient detection of episodic diversifying selection. Mol. Biol. Evol. 32 , 1342–1353, https://doi.org/10.1093/molbev/msv022 (2015).
Confer, A. W. & Ayalew, S. The OmpA family of proteins: roles in bacterial pathogenesis and immunity. Vet. Microbiol. 163 , 207–222, https://doi.org/10.1016/j.vetmic.2012.08.019 (2013).
Petersen, L., Bollback, J. P., Dimmic, M., Hubisz, M. & Nielsen, R. Genes under positive selection in Escherichia coli. Genome Res. 17 , 1336–1343, https://doi.org/10.1101/gr.6254707 (2007).
Li, P. E. et al . Enabling the democratization of the genomics revolution with a fully integrated web-based bioinformatics platform. Nucleic Acids Res. 45 , 67–80, https://doi.org/10.1093/nar/gkw1027 (2017).
Ondov, B. D. et al . Mash: fast genome and metagenome distance estimation using MinHash. Genome Biol. 17 , 132, https://doi.org/10.1186/s13059-016-0997-x (2016).
BBMap v. 37.66 (sourceforge.net/projects/bbmap/).
Kurtz, S. et al . Versatile and open software for comparing large genomes. Genome Biol. 5 , R12, https://doi.org/10.1186/gb-2004-5-2-r12 (2004).
Langmead, B. & Salzberg, S. L. Fast gapped-read alignment with Bowtie 2. Nat. Methods 9 , 357–359, https://doi.org/10.1038/nmeth.1923 (2012).
Li, H. et al . The Sequence Alignment/Map format and SAMtools. Bioinforma. 25 , 2078–2079, https://doi.org/10.1093/bioinformatics/btp352 (2009).
Stamatakis, A. RAxML version 8: a tool for phylogenetic analysis and post-analysis of large phylogenies. Bioinforma. 30 , 1312–1313, https://doi.org/10.1093/bioinformatics/btu033 (2014).
Price, M. N., Dehal, P. S. & Arkin, A. P. FastTree 2–approximately maximum-likelihood trees for large alignments. PLoS One 5 , e9490, https://doi.org/10.1371/journal.pone.0009490 (2010).
Nguyen, L. T., Schmidt, H. A., von Haeseler, A. & Minh, B. Q. IQ-TREE: a fast and effective stochastic algorithm for estimating maximum-likelihood phylogenies. Mol. Biol. Evol. 32 , 268–274, https://doi.org/10.1093/molbev/msu300 (2015).
Yang, Z. PAML 4: phylogenetic analysis by maximum likelihood. Mol. Biol. Evol. 24 , 1586–1591, https://doi.org/10.1093/molbev/msm088 (2007).
Gruning, B. et al . Bioconda: sustainable and comprehensive software distribution for the life sciences. Nat. Methods 15 , 475–476, https://doi.org/10.1038/s41592-018-0046-7 (2018).
Kalyaanamoorthy, S., Minh, B. Q., Wong, T. K. F., von Haeseler, A. & Jermiin, L. S. ModelFinder: fast model selection for accurate phylogenetic estimates. Nat. Methods 14 , 587–589, https://doi.org/10.1038/nmeth.4285 (2017).
Lo, C. C. & Chain, P. S. Rapid evaluation and quality control of next generation sequencing data with FaQCs. BMC Bioinforma. 15 , 366, https://doi.org/10.1186/s12859-014-0366-2 (2014).
Freitas, T. A., Li, P. E., Scholz, M. B. & Chain, P. S. Accurate read-based metagenome characterization using a hierarchical suite of unique signatures. Nucleic Acids Res. 43 , e69, https://doi.org/10.1093/nar/gkv180 (2015).
Download references
Acknowledgements
We thank the LANL Genome Programs Group and Jason Gans for reading and providing feedback with the manuscript. The authors report no conflict of interests. This work was supported by the U.S. Defense Threat Reduction Agency’s Joint Science and Technology Office (DTRA J9-CB/JSTO) under contract number CB10152, and by the U.S. Department of Energy, Office of Science, Biological and Environmental Research Division, under award number LANL-F59T, to P.S.G.C.
Author information
Authors and affiliations.
Bioscience Division, Los Alamos National Laboratory, MS-M888, Los Alamos, NM, 87545, USA
Migun Shakya, Sanaa A. Ahmed, Karen W. Davenport, Mark C. Flynn, Chien-Chi Lo & Patrick S. G. Chain
You can also search for this author in PubMed Google Scholar
Contributions
P.S.G.C. conceived the study. M.S., C.L., and S.A.A. designed the algorithm. M.S. performed bioinformatics analyses. M.F designed and implemented web server. K.W.D. generated the flowchart for workflow design. P.S.G.C., S.A.A. and M.S. interpreted the data and wrote the manuscript with input from the other authors.
Corresponding authors
Correspondence to Migun Shakya or Patrick S. G. Chain .
Ethics declarations
Competing interests.
The authors declare no competing interests.
Additional information
Publisher’s note Springer Nature remains neutral with regard to jurisdictional claims in published maps and institutional affiliations.
Supplementary information
Supplementary information ., supplementary tables s1-s9, ., rights and permissions.
Open Access This article is licensed under a Creative Commons Attribution 4.0 International License, which permits use, sharing, adaptation, distribution and reproduction in any medium or format, as long as you give appropriate credit to the original author(s) and the source, provide a link to the Creative Commons license, and indicate if changes were made. The images or other third party material in this article are included in the article’s Creative Commons license, unless indicated otherwise in a credit line to the material. If material is not included in the article’s Creative Commons license and your intended use is not permitted by statutory regulation or exceeds the permitted use, you will need to obtain permission directly from the copyright holder. To view a copy of this license, visit http://creativecommons.org/licenses/by/4.0/ .
Reprints and permissions
About this article
Cite this article.
Shakya, M., Ahmed, S.A., Davenport, K.W. et al. Standardized phylogenetic and molecular evolutionary analysis applied to species across the microbial tree of life. Sci Rep 10 , 1723 (2020). https://doi.org/10.1038/s41598-020-58356-1
Download citation
Received : 18 July 2019
Accepted : 06 January 2020
Published : 03 February 2020
DOI : https://doi.org/10.1038/s41598-020-58356-1
Share this article
Anyone you share the following link with will be able to read this content:
Sorry, a shareable link is not currently available for this article.
Provided by the Springer Nature SharedIt content-sharing initiative
This article is cited by
Isolation of burkholderia pseudomallei from a goat in new caledonia: implications for animal and human health monitoring and serological tool comparison.
- Anais Desoutter
- Thomas Deshayes
- Karine Laroucau
BMC Veterinary Research (2024)
Population structure and ongoing microevolution of the emerging multidrug-resistant Salmonella Typhimurium ST213
- Isela Serrano-Fujarte
- Edmundo Calva
- José L. Puente
npj Antimicrobials and Resistance (2024)
VBCG: 20 validated bacterial core genes for phylogenomic analysis with high fidelity and resolution
- Renmao Tian
- Behzad Imanian
Microbiome (2023)
Beyond the spore, the exosporium sugar anthrose impacts vegetative Bacillus anthracis gene regulation in cis and trans
- Michael H. Norris
- Andrew P. Bluhm
- Jason K. Blackburn
Scientific Reports (2023)
Arbuscular mycorrhizal fungi heterokaryons have two nuclear populations with distinct roles in host–plant interactions
- Jana Sperschneider
- Gokalp Yildirir
- Nicolas Corradi
Nature Microbiology (2023)
By submitting a comment you agree to abide by our Terms and Community Guidelines . If you find something abusive or that does not comply with our terms or guidelines please flag it as inappropriate.
Quick links
- Explore articles by subject
- Guide to authors
- Editorial policies
Sign up for the Nature Briefing: AI and Robotics newsletter — what matters in AI and robotics research, free to your inbox weekly.

Loading metrics
Open Access
Peer-reviewed
Research Article
A Molecular Phylogeny of Living Primates
Current address: Laboratory of Cytogenetics of Animals, Institute of Chemical Biology and Fundamental Medicine, Novosibirsk, Russia
Affiliation Laboratory of Genomic Diversity, National Cancer Institute–Frederick, Frederick, Maryland, United States of America
Affiliation Gene Bank of Primates and Primate Genetics Laboratory, German Primate Center, Göttingen, Germany
Affiliation Division of Genetics, Instituto Nacional de Câncer and Department of Genetics, Universidade Federal do Rio de Janeiro, Rio de Janeiro, Brazil
Affiliation Department of Evolutionary Anthropology and Institute for Genome Sciences and Policy, Duke University, Durham, North Carolina, United States of America
Affiliation SAIC–Frederick, Laboratory of Genomic Diversity, National Cancer Institute–Frederick, Frederick, Maryland, United States of America
Affiliation Physiopathologie et Médecine Translationnelle, Faculté de Médecine, Université Louis Pasteur, Strasbourg, France
Affiliation Universidade Federal do Pará, Belém, Brazil
* E-mail: [email protected]
- Polina Perelman,
- Warren E. Johnson,
- Christian Roos,
- Hector N. Seuánez,
- Julie E. Horvath,
- Miguel A. M. Moreira,
- Bailey Kessing,
- Joan Pontius,
- Melody Roelke,
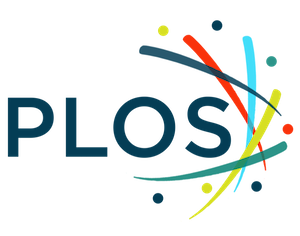
- Published: March 17, 2011
- https://doi.org/10.1371/journal.pgen.1001342
- Reader Comments
Comparative genomic analyses of primates offer considerable potential to define and understand the processes that mold, shape, and transform the human genome. However, primate taxonomy is both complex and controversial, with marginal unifying consensus of the evolutionary hierarchy of extant primate species. Here we provide new genomic sequence (∼8 Mb) from 186 primates representing 61 (∼90%) of the described genera, and we include outgroup species from Dermoptera, Scandentia, and Lagomorpha. The resultant phylogeny is exceptionally robust and illuminates events in primate evolution from ancient to recent, clarifying numerous taxonomic controversies and providing new data on human evolution. Ongoing speciation, reticulate evolution, ancient relic lineages, unequal rates of evolution, and disparate distributions of insertions/deletions among the reconstructed primate lineages are uncovered. Our resolution of the primate phylogeny provides an essential evolutionary framework with far-reaching applications including: human selection and adaptation, global emergence of zoonotic diseases, mammalian comparative genomics, primate taxonomy, and conservation of endangered species.
Author Summary
Advances in human biomedicine, including those focused on changes in genes triggered or disrupted in development, resistance/susceptibility to infectious disease, cancers, mechanisms of recombination, and genome plasticity, cannot be adequately interpreted in the absence of a precise evolutionary context or hierarchy. However, little is known about the genomes of other primate species, a situation exacerbated by a paucity of nuclear molecular sequence data necessary to resolve the complexities of primate divergence over time. We overcome this deficiency by sequencing 54 nuclear gene regions from DNA samples representing ∼90% of the diversity present in living primates. We conduct a phylogenetic analysis to determine the origin, evolution, patterns of speciation, and unique features in genome divergence among primate lineages. The resultant phylogenetic tree is remarkably robust and unambiguously resolves many long-standing issues in primate taxonomy. Our data provide a strong foundation for illuminating those genomic differences that are uniquely human and provide new insights on the breadth and richness of gene evolution across all primate lineages.
Citation: Perelman P, Johnson WE, Roos C, Seuánez HN, Horvath JE, Moreira MAM, et al. (2011) A Molecular Phylogeny of Living Primates. PLoS Genet 7(3): e1001342. https://doi.org/10.1371/journal.pgen.1001342
Editor: Jürgen Brosius, University of Münster, Germany
Received: September 15, 2010; Accepted: February 16, 2011; Published: March 17, 2011
This is an open-access article, free of all copyright, and may be freely reproduced, distributed, transmitted, modified, built upon, or otherwise used by anyone for any lawful purpose. The work is made available under the Creative Commons CC0 public domain dedication.
Funding: This project has supported with federal funds from the National Cancer Institute, National Institutes of Health, under contract N01-CO-12400. This research has been supported (in part) by the Intramural Research Program of the NIH, NCI, Center for Cancer Research, the Duke Primate Genomics Initiative, and Institute for Genome Sciences and Policy at Duke University. In Brazil, support included CNPq grant 303583/2007-0 (HNS) and CNPq grant 304403/2008-3 (MAMM). The content of this publication does not necessarily reflect the views or policies of the Department of Health and Human Services, nor does its mention of trade names, commercial products, or organizations imply endorsement by the U.S. Government. The funders had no role in study design, data collection and analysis, decision to publish, or preparation of manuscript.
Competing interests: The authors have declared that no competing interests exist.
Introduction
The human genome project has revolutionized such fields as genomics, proteomics and medicine. Markedly absent from these many advances however, is a formal evolutionary context to interpret these findings, as the phylogenetic hierarchy of primate species has only modest local (family and genus level) molecular resolution with little consensus on overall primate radiations. The exact number of primate genera is controversial and species counts range from 261–377 [1] - [3] . Although whole genome sequencing of 12 primate species are now completed, or nearly so, broader genome representation of man's closest relatives is necessary to interpret human evolution, adaptation and genome structure to assist in biomedical advances.
Primate taxonomy has undergone considerable revision but current views [1] - [3] concur that 67–69 primate genera originated from a common ancestor during the Cretaceous/Paleocene boundary roughly 80–90 MYA. An Eocene expansion formed the major extant lineages of 1) Strepsirrhini, which is composed of Lorisiformes (galagos, pottos, lorises), Chiromyiformes (Malagasy aye-aye) and Lemuriformes (Malagasy lemurs); 2) Tarsiiformes (tarsiers) and 3) Simiiformes composed of Platyrrhini (New World monkeys) and Catarrhini, which includes Cercopithecoidea (Old World monkeys) and Hominoidea (human, great apes, gibbons) (see Figure 1 ). Primate taxonomy, initially imputed from morphological, adaptive, bio-geographical, reproductive and behavioral traits, with inferences from the fossil record [1] - [3] is complex. Recent application of molecular genetic data to resolve primate systematics has been informative, but limited in scope and constrained to just specific subsets of taxa. Efforts to overcome this deficiency using a supermatrix approach [4] , [5] with published sequences culled from these prior studies are inherently flawed by a prohibitively large proportion of missing data for each taxon (e.g. 59–85% see [5] ).
- PPT PowerPoint slide
- PNG larger image
- TIFF original image
Shown is the maximum likelihood tree based on 34,927 bp sequenced from 54 genes amplified from selected single species representing each genus. All unmarked nodes have bootstrap support of 100%. Nodes with green circles have bootstrap proportions<70%, grey circles 71–80%, black circles 81–90% and red circles 91–99%. Boxes indicate genus of species with completed, nominated or draft whole genome sequence accomplished. Numbers in parenthesis next to each genus indicate number of species present in study followed by the total number described [3] . Numbers in parentheses next to family names indicate number of genera included in study followed by total described [3] . Numbers in bold refer to nodes on Figure 2 , Figure S1 , Table 1 , Table 2 , Table 3 . Reference fossil dates used for calibration of tree in dating algorithms are represented by letters A-H on nodes (see Materials and Methods ). Fossil dates are as follows and sources are listed in Materials and Methods : A) Galagidae-Lorisidae split 38–42 MYA, B) Simiiformes emerge 36–50 MYA, C) Catarrhini emerge 20–38 MYA, D) Platyrrhini emerge 20–27 MYA, E) Tribe Papionini emerge 6–8 MYA, F) Theropithecus emerge 3.5–4.5 MYA, G) Family Hominidae emerge 13–18 MYA, H) Homo-Pan split 6–7 MYA.
https://doi.org/10.1371/journal.pgen.1001342.g001
https://doi.org/10.1371/journal.pgen.1001342.t001
https://doi.org/10.1371/journal.pgen.1001342.t002
https://doi.org/10.1371/journal.pgen.1001342.t003
Here we employ large-scale sequencing and extensive taxon sampling to provide a highly resolved phylogeny that affirms, reforms and extends previous depictions of primate speciation. In turn, the clarity of the primate phylogeny forms a solid framework for a novel depiction of diverse patterns of genome evolution among primate lineages. Such insights are essential in ongoing and future comparative genomic investigation of adaptation and selection in humans and across primates.
Results/Discussion
A comprehensive molecular phylogeny based on 34,927 bp (after correction for ambiguous sites from the original dataset of 43,493 bp per operational taxonomic unit, OTU) amplified from 54 nuclear genes in 191 taxa including 186 primates representing 61 genera is presented ( Figure 1 , Figure 2 , Figure S1 , Table S1 , and Table S2 ). The phylogeny is highly resolved, with bootstrap values of 90–100% and Bayesian posterior probabilities of 0.9–1.0 at 166 of the 189 nodes (88%)( Table 1 , Table 2 , Table 3 ). Further, only 3 of 189 nodes (nodes 28, 38, 158) are polytomies in the bootstrap analyses ( Table 1 and Table 3 ; Figure 2 , Figure S1 ). ( Note: nodes listed hereafter refer to Figure 2 , Figure S1 , Table 1 , Table 2 , Table 3 ) . Roughly equal amounts of coding (14742 bp) and non-coding (17185 bp) genomic regions were sampled from X chromosome (4870 bp), Y chromosome (2630 bp) and autosomes (27427 bp) ( Table 4 ) using newly developed PCR primers derived from a bioinformatics approach specific to primates in addition to primers from previous large-scale phylogenetic analyses ( Materials and Methods , Tables S2 , S3 , S4 ).
(See also Figure S1 ). Shown is the maximum likelihood tree derived from 34,927 bp of sequence from 54 genes. Node support is >90% for 166 nodes. Each node within the tree is numbered and listed in Table 1 , Table 2 , Table 3 to provide all node support values for ML, MP and Bayesian methods of analysis as well as estimated dates of divergence. Numbers in boxes represent estimate divergence times for major nodes as listed in Table 1 , Table 2 , Table 3 . * denotes nodes whose divergence time is estimated to be less than 1 MYA.
https://doi.org/10.1371/journal.pgen.1001342.g002
https://doi.org/10.1371/journal.pgen.1001342.t004
Separate phylogenetic analyses of these data partitions are generally concordant. The greatest proportion of phylogenetically informative sites occurred in Y-linked genes (56%) compared with regions sequenced from the X-chromosome (40%) and autosomes (42%) ( Table 4 , Table S4 ), a finding also observed in carnivores [6] , [7] . However, greater frequency of phylogenetic inconsistencies or unresolved nodes occur in these subset trees ( Figures S2 , S3 , S4 , S5 , S6 , S7 , S8 , S9 , S10 , S11 , S12 , S13 ), compared with the entire concatenated data set ( Figure 2 , Figure S1 ). Thus, these findings illustrate the need for both genome-wide datasets and maximum representation of species to resolve differences among previous studies that used only single genes, the uniparentally inherited mtDNA molecular marker and smaller numbers of primate taxa.
Resolution of Early Primate Divergence
The relative placement of suborder Strepsirrhini and infraorder Tarsiiformes at an early stage of primate evolution has been difficult to resolve [8] - [11] . Presently distributed in the islands of Borneo, Sumatra, Sulawesi and the Philippines, Tarsiiformes had a broad Holarctic distribution during the Eocene [10] . Phylogenetic placement of tarsiers has alternatively been defined as 1) sister taxa to Strepsirrhini to form Prosimii [2] , [8] , [12] , 2) allied with Simiiformes (Anthropoidea) to form Haplorrhini [1] , [13] , [14] and 3) a separate relict lineage with an independent origin [15] . Here we provide strong evidence that strepsirrhines split with suborder Haplorrhini approximately 87 MYA (node 185). The ancient lineage is monophyletic and defined by a long branch and eight shared insertions/deletions (indels) (node 144). Rooted by Lagomorpha, the phylogeny affirms Dermoptera as the closest mammalian order relative to Primates, followed by Scandentia [16] , [17] .
A long continuous Tarsiiformes branch (node 142), marked by 25 synapomorphic indels, is consistent with a relict lineage of ancient origin. The sequence phylogeny unambiguously supports tarsiers as a sister lineage (albeit distant) to Simiiformes (BS = 85 MP; 98 ML; 0.99 PP) to form Haplorrhini (node 143). A few indels ( Table S5 ) define alternate evolutionary topologies, such as tarsiers aligned with Strepsirrhini (1 indel, ZFX ) or Scandentia (1 indel, DCTN2 ), compared with those that support an ancestral grouping of Tarsiiformes +Strepsirrhini +Dermoptera +Scandentia (2 indels, PLCB4, POLA1 ). These incongruent alternatives suggest further investigation of more complex rare genomic changes as cladistic markers of ancient speciation is needed [17] , [18] .
Strepsirrhini
Aided by samples of rare taxa, the phylogeny expands upon recent findings [19] - [21] to better resolve long-standing questions on the evolution of Lorisiformes and the two endemic Madagascar infraorders of Chiromyiformes and Lemuriformes. Our data affirm the ancient split of Strepsirrhini, approximately 68.7 MYA (node 144), into the progenitors of Lemuriformes/Chiromyiformes (origin 58.6 MYA, node 174) and Lorisiformes (origin 40.3 MYA, node 184).
Lorisiformes evolution includes the radiation of Lorisidae (pottos and lorises, 37 MYA, node 179) and Galagidae (19.9 MYA, node 183) species. Within Lorisidae, the four extant genera split into the African subfamily Perodicticinae ( Arctocebus, Perodictus ) and the Asian subfamily Lorisinae ( Nycticebus, Loris ) and are the most divergent within all of primates. For example, mean nucleotide divergence between Lorisidae species is 4–5 times that observed in family Hominidae ( Figure 3 ) and significantly (p<0.05) exceed the average genetic divergence across all of Strepsirrhini (nodes 176–178, Table S7 , Figure 3 ). Galagidae are found only in Africa and currently are divided into four genera. However, the Otolemur lineage (node 180) is placed as part of a paraphyletic grouping (node 182) along with two other extant Galago lineages (nodes 181, 183), suggesting that further taxonomic investigation of Galago is warranted.
1) infraorders Simiiformes, Lemuriformes, and Lorisiformes (Chiromyiformes and Tarsiiformes excluded due to small numbers of species); 2) parvorders Catarrhini and Platyrrhini; 3) superfamilies Cercopithecoidea and Hominoidea; 4) catarrhine families Cercopithecidae, Hominidae and Hylobatidae, 5) platyrrhine families Pitheciidae Atelidae, and Cebidae; 6) Malagasy strepsirrhine families of Lemuridae, Indriidae, Lepilemuridae, and Cheirogaleidae; 7) strepsirrhine families of Lorisidae and Galagidae; 8) catarrhine subfamilies of Cercopithecinae, Colobinae, Homininae, and Ponginae; 9) platyrrhine subfamilies of Callitrichinae, Aotinae, Cebinae, Saimirinae, Alouattinae, Atelidae, Calicebinae and Pitheciinae; 10) strepsirrhine subfamilies of Lorisinae and Perodicticinae. (A) Mean nucleotide divergence and standard error computed from branch lengths per taxonomic level from Figure 2 , Figure S1 , Table 1 , Table 2 , Table 3 , and Tables S6 , S7 , S8 . (B) Mean rate of nucleotide substitution and standard error computed from BEAST analysis for each branch within taxonomic level from Table 1 , Table 2 , Table 3 , and Tables S6 , S7 , S8 . (C) Mean number of synapomorphic and autapomorphic indels per branch and standard error computed from Table 1 , Table 2 , Table 3 , and Tables S6 , S7 , S8 . Horizontal lines reflect global mean for primate phylogeny for each parameter.
https://doi.org/10.1371/journal.pgen.1001342.g003
Common ancestors of Chiromyiformes and Lemuriformes likely colonized the island of Madagascar prior to 58.6 MYA (node 174). Noted for extensive adaptive evolution, the relative hierarchical branching patterns of the four Lemuriformes families (Indriidae, Lepilemuridae, Lemuridae, Cheirogaleidae) recognized by taxonomists, has proven difficult to resolve conclusively. Inferences on species versus subspecies classification are controversial with as many as 97 Malagasy lemurs [22] under taxonomic review. Chiromyiformes diverged from a common ancestor with Lemuriformes shortly after colonisation of Madagascar [14] , [19] and today consists of a single relict genus Daubentonia defined by a long branch with high indel frequency (N = 14) ( Figure 2 , Figure S1 , Table S7 ). The evolution of the four Lemuriformes families began 38.6 MYA (node 173) with the emergence of Lemuridae, followed by Indriidae and a monophyletic lineage that split 32.9 MYA (node 152) to form the sister lineages of Lepilemuridae and Cheirogaleidae. This branching pattern among families agrees with earlier nuclear gene segment findings [20] that differ from studies using mtDNA sequence and Alu insertion variation which were unable to resolve these hierarchical associations [19] . Further, relatively weak nodal support here collapses Lemuriformes into an unresolved trichotomy of Lemuridae, Indriidae, and the Lepilemuridae + Cheirogaleidae lineage (node 158). Optimal resolution of this node is observed with exon sequences ( Figures S8 and S9 ), indicating that intron sites may be saturated, while more conserved coding regions remain informative and reflect the ancient rapid radiation of Lemuriformes families.
New World Primates (Platyrrhini)
The phylogeny clarifies formerly unresolved questions concerning New World primate evolution including branching order among families, relative divergence of genera within families, and phylogenetic placement of Aotus , and provides genetic support for examples of adaptive evolution that led to nocturnalism, “phyletic dwarfism” and species diversification within the Amazonian rainforest. Here, Platyrrhini clearly diverged from a common ancestor with Catarrhini (node 141) roughly 43.5 MYA during the Eocene. Although questions remain about the route and nature of primate colonization of the New World [23] , [24] and the impact of historic global climate change in neotropical regions [25] , [26] , the phylogeny unambiguously resolves the relative divergence pattern among families from a common ancestor 24.8 MYA (node 78).
The common ancestor to Pitheciidae (uakaris, titis and sakis) originated 20.2 MYA (node 140) and the majority of these species currently are distributed in the neotropical Amazonian basin extending from the Andean slopes to the Atlantic. Next to radiate are the Atelidae (node 126), with the most basal lineage leading to Alouatta (howler monkeys), currently widely distributed from Mexico to northern Argentina, followed by the divergence of Ateles (spider monkeys) from South American lineage comprised of sister genera (node 121) of Lagothrix (woolly monkeys) and Brachyteles (muriquis).
The Cebidae radiation initiated with the emergence of sister taxa Cebus (Cebinae) and Saimiri (Saimirinae) approximately 20 MYA (node 113), in agreement with other molecular studies [27] - [30] . Subsequently, during a relatively brief interval (∼700,000 years) a lineage arose (node 112) that split to form the Callitrichinae (marmosets and tamarins) and Aotus (night monkeys). The Aotus lineage (node 98) radiated with unusually high numbers of synapomorphic indels (N = 15), the most observed in Simiiformes ( Table 2 and Table 3 ), to form a complex species group of controversial taxonomic designation as subfamily or family and uncertainty over its exact placement relative to other Cebidae lineages. Here, Aotus is the sister lineage to Callitrichinae (marmosets, tamarins) as originally hypothesized by Goodman (1998) [1] , [28] . Aotus species divide into sister lineages, with the “grey-necked” species ( A. trivirgatus + A. lemurinus griseimembra ) distributed north of the Amazon River, and “red-necked” species A. nancymaae , A. azarae species and associated subspecies located most to the south (nodes 98, 101, 102). The unusual depth of divergence (i.e. sizeable nucleotide substitutions/site; high indel frequency) may exemplify adaptive speciation as Aotus are the only nocturnal Simiiformes [31] , and thereby may have reduced competition with diurnal small-bodied platyrrhines inhabiting the same neotropical environments.
Another case of adaptation termed “phyletic dwarfism,” defined as a gradient in morphological size partially correlated with evolutionary time [32] , is supported in Cebidae. Aotus , Cebus and Saimiri species are larger than the more derived and smaller squirrel-sized Callitrichinae of Saguinus, Leontopithecus , Callimico, Mico, Cebuella and Callithrix . In Callitrichinae, Saguinus is the first to diverge with S. fuscicollis currently distributed south of the Amazon River. Subsequently, the genus diversified into northern ( S. bicolor, S. midas, S. martinsi, S. geoffroyi, S. oedipus ) and south Amazonian species ( S. imperator, S. mystax, S. labiatus ); a trend generally similar to findings based on mtDNA [33] and single nuclear genes [34] . The hierarchical branching order among the remaining Callitrichinae of Leontopithecus , Callimico , Callithrix and Mico mirrors decreasing body size and culminates with the smallest platyrrhine species, Cebuella pygmaea, as most derived. This phylogenetic depiction of Callitrichinae is concordant with several other morphological and reproductive traits [32] , [35] related to dwarfism and perhaps reflects adaptive evolution selected by fluctuating resource availability within the Amazon and Atlantic coast rainforests [36] .
Old World Primates (Cercopithecoidea)
Cercopithecoidea (family Cercopithecidae) speciation patterns are confounded by symplesiomorphic traits in morphology, behavior and reproduction, and are further confused by hybridization between sympatric species, subspecies and populations (summarized in [2] ). Cercopithecidae includes two subfamilies, Colobinae and Cercopithecinae, which diverged 18 MYA (node 62), but classification schemes [2] are marked by inconsistencies between morphological [37] , [38] and genetic data, as well as differences among genetic data studies [4] , [27] , [39] - [44] .
Colobinae radiation started approximately 12 MYA (node 42) with species adapted to an arboreal, leaf-eating existence. Asian (tribe Presbytini) and African (tribe Colobini) genera are monophyletic (nodes 53 and 61, respectively), supporting earlier genetic findings [4] , [40] over morphology-based taxonomy [2] , [45] . Whilst African genera Piliocolobus and Colobus are commonly recognized, the taxonomic schemes for the critically endangered Asian langur and leaf monkeys, all sharing digestive adaptations for an arboreal folivorous diet, have ranged from a single genus Presbytis to three distinct genera ( Trachypithecus , Semnopithecus, Presbytis ). Here, the Presbytis lineage, distinguished by 3 indel events (node 56), diverged first within Asian Colobinae, followed by the odd-nosed group (Rhinopithecus, Nasalis, Pygathrix) , Trachypithecus and Semnopithecus . As odd-nosed species are not exclusively arboreal and folivorous, the results indicate either 1) morphological convergence between Presbytis with Trachypithecus and Semnopithecus , 2) adaptation for an expanded diet in the odd-nosed group, or 3) that a folivorous diet is a symplesiomorphic trait within Asian colobines.
Trachypithecus and Semnopithecus genera consist of closely related, often sympatric species (node 51), distributed in the Indian subcontinent and SE Asia, with inconsistent phylogenetic resolution among species [4] , [27] , [40] , [44] , [46] . Nonetheless, all genetic studies, including the present, place Trachypithecus vetulus (monticola) nested within the Semnopithecus clade (node 50), suggesting the need for taxonomic revision. Further, previously ambiguous associations between Trachypithecus and Semnopithecus (nodes 43–51) are clarified. Inter-specific genetic differences are roughly half those observed among other colobine genera ( Figure 2 , Figure S1 , Table 3 , Table S9 ) and may indicate that recent speciation, taxonomic over-splitting, reticulate evolution, or a combination thereof, (e. g. see [40] , [44] , [46] ) are common within the Asian Colobinae radiation.
The remainder of Old World monkeys (tribes Papionini and Cercopithecini) [2] arose from a common ancestor approximately 11.5 MYA (node 41). Considerable interest in Cercopithecinae speciation is motivated not only by primate conservation, but increased biomedical surveillance for novel zoonotic agents and comparative research of host-pathogen adaptation relevant to the study of deadly human viral pandemics such as HIV/SIV.
Cercopithecini (guenons, patas monkey, talapoin, green monkeys) include lineages rooted by divergent monotypic genera followed by more recent speciation, characterized by transition from an arboreal to a terrestrial lifestyle. Generally arboreal, Miopithecus and Allenopithecus are early offshoots with respect to the two Cercopithecini subclades formed approximately 7 MYA. The Cercopithecus lineage (node 34) radiated after Miopithecus and retained an arboreal lifestyle. The second, rooted by Allenopithecus , forms a terrestrial clade of Erythrocebus patas and Chlorocebus species, with Cercopithecus l'hoesti separated the other Cercopithecus . This paraphyly, also reported in earlier genetic studies [39] , [47] , [48] and counter to initial morphological classifications [2] , suggests taxonomic revision of Cercopithecus . Further, resolution of Allenopithecus (node 40) and Miopithecus (node 35) speciation herein suggests a single evoluiontary transition from an arboreal to a terrestrial lifestyle in E. patas, C. l'hoesti, and Chlorocebus species.
Papionini (macaques, mandrills, drills, baboons, geladas, mangabeys) is a taxonomically complex tribe [2] . One of the more familiar genera within Cercopithecoidea, Macaca (macaques) diverged 5.1 MYA and today is represented by an African lineage comprised of a single species M. sylvanus , and an Asian lineage consisting of well-defined species groups ( fascicularis, sinica, mulatta, nemestrina, Sulawesi ) inhabiting India and Asia, SE Asia and Sundaland [49] . With the exception of the fascicularis group, which is split in this study whereby M. arctoides [ fascicularis ] is more closely aligned with M. thibetana [ sinica ] rather than M. fascicularis as expected, our data otherwise strongly support these macaque species groups (nodes 6, 11). Moreover, the phylogeny affirms Groves [2] proposal that Lophocebus and Theropithecus are distinct clades apart from Papio (nodes 18, 19), although the average nucleotide divergence among these three genera are generally less than between other recognized Papionin genera ( Macaca , Mandrillus , Cercocebus) ( Figure 2 , Figure S1 , Table 3 , Table S9 ). Lastly, sequence divergence between tribes is unequal with Cercopithecini nearly twice that of Papionini (mean branch length = 13.1, 7.43, respectively, p<0.005) and there are numerous instances of discordance between the present phylogeny with previous mtDNA studies [4] , [5] suggesting that continued resolution of Cercopithecinae speciation and of Papionini in particular, will likely include evidence of reticulate evolution represented by ongoing and historic episodes of hybridization (e.g. see [39] , [48] ).
Once contentiously debated, the closest human relative of chimpanzee ( Pan ) within subfamily Homininae ( Gorilla, Pan, Homo ) is now generally undisputed. The branch forming the Homo and Pan lineage apart from Gorilla is relatively short (node 73, 27 steps MP, 0 indels) compared with that of the Pan genus (node 72, 91 steps MP, 2 indels) and suggests rapid speciation into the 3 genera occurred early in Homininae evolution. Based on 54 gene regions, Homo-Pan genetic distance range from 6.92 to 7.90×10 −3 substitutions/site ( P. paniscus and P. troglodytes , respectively), which is less than previous estimates based on large scale sequencing of specific regions such as chromosome 7 [50] . The highly endangered orangutan forms the single genus Pongo in subfamily Ponginae (nodes 75–76), the sister lineage to Homininae. Currently restricted to the islands of Borneo and Sumatra, orangutans once inhabited all of Southeast Asia during the Pleistocene [51] . Differences in behavior, morphology, karyology, and genetic data between the two island populations [2] support the taxonomic designation as two separate species of Bornean ( P. pygmaeus ) and Sumatran orangutans ( P. abelii ), and these designations are upheld by the data presented here.
Hylobatidae (siamang, gibbons, hoolock) are noted for exceptional rates of chromosome re-arrangement [52] , [53] , 10–20 times faster than in most mammals [54] . Classification schemes of the 12 species range from two genera ( Hylobates and Symphalangus ) to four subgenera and/or genera ( Hylobates , Nomascus, Symphalangus, Hoolock ), defined by unique numbers of chromosomes [54] , [55] . The eight species included in this study form three clades that coincide with genus designation (absent is Hoolock ; nodes 64–69) that diverged rapidly 8.9 MYA. Moreover, Nomascus species appear more recent than Symphalangus and Hylobates , with node divergence dates estimated at less than 1 MY ( Table 3 , Table S9 , Figure 2 ). Thus, Hylobatidae exhibits episodes of rapid divergence perhaps related to excessive genome re-organization and warrants additional investigation.
Genome Divergence, Rate Heterogeneity, and Indels
The clarity of the primate phylogeny here can be used to assess nucleotide divergence patterns, rates of substitution and accumulation of synapomorphic and autapomorphic indels. Genome divergence varies across primate lineages, with the least inter-specific differences observed in Cercopithecidae lineages and the most in Lorisidae, reflecting recent speciation in the former and the more ancient origins of the latter ( Figure 3 , Table 1 , Table 3 , Tables S7 and S9 ).
The global rate of nucleotide substitution across the entire primate phylogeny is 6.163×10 −4 substitutions/ site/ MY, but exhibits significant heterogeneity across lineages ( Figure 3 ) and among branches ( Table 1 , Table 2 , Table 3 ; Tables S6 , S7 , S8 ). For example, the “hominoid slow-down” hypothesized to have occurred in human evolution, is confounded by the reduced rates observed in all Catarrhini (not just Homininae) compared with Platyrrhini and Strepsirrhini ( Figure 3 , Table S10 ). By contrast, the “phyletic dwarfism” of the Callitrichinae (nodes 97, 85) and the evolution of nocturnalism in Aotinae are correlated with increased rates along specific branches (see nodes 99, 100) rather than an being a function of an average rate among all branches within the lineage ( Figure 3 ), suggesting that an adaptive “speed-up” occurred in the common ancestors of these extant species.
The genome accumulates indels over evolutionary time, altering the degree of sequence homology between taxa. Further, large-scale genome sequence analysis demonstrate that indel formation is an indicator of genome plasticity, positively correlated with adjacent nucleotide substitution rate [56] , [57] , gene segmental duplication, chromosomal position, hybridization between species and speciation, and is enhanced by molecular mechanisms of recombination among repetitive elements [58] - [60] . Here, the distribution of indels is ubiquitous in both coding and noncoding segments ( Tables S4 , S5 , S6 ), but is markedly disjunct among primate lineages ( Figure 3 ). Excluding the infraorders Tarsiiformes (25 indels) and Chiromyiformes (14 indels) due to statistically inadequate sampling, the indel frequency per branch varies by a factor of 20 ( Table 1 , Table 2 , Table 3 ; Tables S7 , S8 , S9 ) with the greatest accumulation within Lorisidae (particularly Arctocebus calabarensis ) and the least in Cercopithecidae ( Figure 3 ). The major correlate of indel frequency is not substitution rate, but overall genome divergence represented by branch length (R-square = 0.659 Lorisiformes; 0.610 Lemuriformes; 0.3286 Simiiformes; P<0.05).
Conclusions
The molecular genetic resolution of the primate phylogeny provides a robust comparative genomic resource to affirm, alter, and extend previous taxonomic inferences. Approximately half of the 261–377 species and 90% of the genera are included facilitating resolution of long-standing phylogenetic ambiguities. Early events within primate evolution are resolved such as: Dermoptera is the closest mammalian order to Primates; Tarsiiformes are sister taxa with Simiiformes to form Haplorrhini; Chiromyiformes (Daubentoniidae) and Lemuriformes are monophyletic indicating a common ancestral lineage colonized the island of Madagascar once; and the hierarchical divergence pattern among New World families Pitheciidae, Atelidae, and Cebidae is clarified.
Additional insights are possible because the relative branching patterns among infraorders, parvorders, superfamilies, families, subfamilies, genera and species are resolved with high measures of support for all but three nodes. For example, Old World monkeys (Cercopithecoidea) display remarkably low levels of divergence, particularly within Papionini, consistent with reticulate evolution, recent speciation and possibly augmented by taxonomic over-splitting. By contrast, the Lorisidae are marked by extraordinary divergence relative to other primate lineages. In the New World, the phylogenetic placement of the unique, nocturnal Aotinae is unambiguously resolved, diverging rapidly after the sister lineage of Cebinae+Saimirinae and prior to the Callitrichinae within the family Cebidae. Further, the pattern of divergence of Callitrichinae is correlated with a gradation in species size, supporting “phyletic dwarfism” [32] , [35] . In the context of human evolution, the large amount of sequence available here for each well-recognized species in Hominidae provides a baseline estimate of average genetic divergence per taxonomic level in primates. However, deviations from these values observed across diverse lineages illustrate the remarkable biodiversity and species richness within the Primate order.
One of the more intriguing unresolved questions is the origin of primates. Generally concordant, most molecular data suggest extant primates arose approximately 85 MYA from a common ancestor. However, the debate continues over the geographic locale most consistent with the existing fossil record [9] , [10] , [12] , [16] , [23] , [26] , [61] - [63] . A parsimonious interpretation of the present data would suggest an Asian origin as the ancient Asian Tarsiiformes and the strepsirrhine Lorisinae are most basal and the closest relatives of primates, Dermoptera and Scandentia, are also exclusive to Asia.
Primate genomes harbor remarkable differences in patterns of speciation, genome diversity, rates of evolution and frequency of insertion/deletion events that are fascinating in their own right, but also provide needed insight into human evolution. Advances in human biomedicine including those focused on changes in genes triggered or disrupted in development, resistance/susceptibility to infectious disease, cancers, mechanisms of recombination and genome plasticity, cannot be adequately interpreted in the absence of a precise evolutionary context or hierarchy. Resolution of the primate species phylogeny here provides a validated framework essential in the development, interpretation and discovery of the genetic underpinnings of human adaptation and disease.
Materials and Methods
Ethics statement.
Primate DNA samples were obtained following the guidelines of Institutional Animal Care and Use Committee policies of respective research institutions (see Table S1 ). All tissue samples for the Laboratory of Genomic Diversity were collected in full compliance with specific Federal Fish and Wildlife permits from the Conservation of International Trade in Endangered Species of Wild flora and Fauna: Endangered and Threatened Species, Captive Bred issued to the National Cancer Institute (NCI)-National Institutes of Health (NIH) (S.J.O. principal officer) by the U.S. Fish and Wildlife Services of the Department of the Interior. Duke University Lemur samples (J.E.H.) were collected under research project BS-4-06-1 and Institutional Animal Care and Use Committee (IACUC) project A094-06-03, and this paper is Duke Lemur Center publication #1192.
DNA Specimens
A complete list of individual and source DNA are presented in Table S1 . DNA was extracted from whole blood, buffy coat, hair or buccal swab samples using DNeasy Blood & Tissue Kit (Qiagen) following manufacture's protocol. DNA from different tissues (muscle, kidney etc) or cell culture pellets was extracted using standard phenol∶chloroform extraction methods. Proteinase K digestion in lysis buffer (100 mM NaCl, 10 mM Tris-HCl pH 8.0, 25 mM EDTA pH 8.0, 0.6% SDS, 100 µg/ml RNAse A) at 56 °C for 3–12 hours rotating was followed by 30 minute phenol, phenol∶chloroform 70∶30, and chloroform extractions using phase-lock gel tubes (Eppendorf) followed by ethanol precipitation and 70% ethanol wash. Dried DNA was reconstituted in TE pH 7.4 buffer and stored at 4 °C. DNA was quantified using Nanodrop (Thermo Scientific) and its quality was assessed using 0.7% agarose gel electrophoresis.
DNA of limited quantity was used for whole-genome amplification using REPLI-g Midi Kit (Qiagen). 50–100 ng of genomic DNA (depending on its quality) was used per one 50 µl reaction according to the manufacturer's protocol. A negative control (no template) was included in every WGA and was verified by downstream PCR and sequencing. Some strepsirrhine DNA was extracted and/or whole genome amplified as previously described [21] .
Amplification of Gene Segments
A complete list of 54 primer sets used in this study is presented in Table S2 . This list includes primers from earlier studies [12] , [16] , [21] , [64] - [68] , as well as those designed specifically for this study using a unique bioinformatics approach (Pontius, unpublished data). A panel of species representing the breadth of primate diversity was used in the testing and optimization of PCR primers and included: Gorilla gorilla, Pan paniscus, Nomascus leucogenys, Symphalanges syndactylus, Erythrocebus patas, Macaca fuscata, Macaca tonkeana, Chiropotes satanas, Saimiri boliviensis, Saimiri sciureus, Callithrix jacchus, Ateles fusciceps, Saguinus fuscicollis, Cheirogaleus medius, Lemur catta and Tupaia minor.
All nuclear gene regions in all the samples were amplified with the following conditions. Either 30 ng of genomic DNA or 1 µl of WGA product was diluted 1∶10 with 0.1XTE per PCR reaction. DNA quantity was increased for poor quality DNA. Genomic and WGA DNA was aliquoted into plates, dried at room temperature and stored at 4 °C. Each 15 µl PCR reaction contained 2 mM MgCl 2 , 250 µM of each dNTP, 150 µM of each forward and reverse primer, 0.8 units of AmpliTaq Gold polymerase (ABI) with 1X GeneAmp 10X PCR Gold Buffer. PCR was performed in PE ABI GeneAmp 9700 and Biometra T1 thermal cyclers. PCRs were carried out using a touchdown program with the following parameters: initial denaturation for 10 min at 95 °C; followed by 10 cycles of 95 °C for 15 s, 60–52 °C (2 cycles for each of the five down gradient annealing temperature steps: 60 °C, 58 °C, 56 °C, 54 °C and 52 °C) for 30 s, and 72 °C for 1 min; and followed by 25 cycles of 95 °C for 15 s, 50 °C for 30 s, and 72 °C for 1 min; and a final extension at 72 °C for 30 min. PCR products were analyzed on 2% agarose gels. Only PCR products that produced single bands were sequenced. PCR products were purified using AMPure kit (Agencourt) or Mag-Bind EZ Pure (OMEGA). PCR products were sequenced directly in two reactions with forward and reverse primers. The sequencing reactions were carried out with the BigDye Terminator v1.1 cycle sequencing kit (Applied Biosystems, Inc.). For 10 µl sequencing reactions we used 0.25 µl of BigDye, 2 µl of 5X Sequencing buffer, 0.32 µM primer and 2.5 µl of PCR product (we diluted PCR product if bands on the gel were too bright). Sequencing reactions were performed as following: 25 cycles of 96 °C for 10 s, 50 °C for 5 s, 60 °C for 4 minutes. Sequencing products were purified using paramagnetic sequencing clean-up CleanSEQ (Agencourt) or Mag-bind SE DTR (OMEGA). PCR and sequencing cleanups were performed on Beckman Coulter Biomek FX laboratory automation workstation. The sequencing products were analyzed with an ABI PRISM 3730 XL 96-well capillary sequencer. Some of the prosimian PCR products and sequences were obtained following earlier published methods [21] . Consensus sequences for each individual were generated from sequences in forward and reverse directions using Sequencher 4.9 program (Gene Codes Corporation). All sequences were deposited in GenBank under accession numbers presented in Table S11 .
DNA Sequence Analyses
Multiple sequence files for each gene segment amplified were aligned by MAFFT version 6 [69] , [70] , imported into Se-Al ver 2.0a11 [71] and verified by eye. Regions of sequence ambiguity within the alignment were identified by GBLOCK version 0.91b [72] , and removed from subsequent phylogenetic analyses. A FilemakerPro database was created to manage all sequence records for each individual DNA specimen and the concatenated dataset was exported. The final, post-GBLOCK, edited, annotated PAUP* nexus alignment of the 54 concatenated genes used for this study is publically available at the following website:
http://lgdfm3.ncifcrf.gov/190Taxa_Rabbit_PAUP.zip
The file is a compressed zip file that can be viewed in either a generic text editor, PAUP*, or alignment programs that read large nexus format files.
Phylogenetic Reconstruction of Primates
Gene partitions were analyzed separately, as well as combined, for genome comparison and phylogenetic reconstruction. Six gene partitions were created, corresponding to X-chromosome, Y-chromosome, autosome, intron, exon and UTR segments. A separate phylogenetic analysis was conducted for each of the six data partitions to compare the concordance among tree topologies derived from each partition. It should be noted that the Y-chromosome tree is not directly comparable to the topologies of the other data partitions because the number of males (N = 127) was a subset of the total (N = 191). In the concatenated data set of all 54 genes, females were coded as “missing” for the Y-chromosome gene sequence. Aligned multiple sequence files of either combined data or gene partitions were imported into ModelTest ver 3.7 [73] and the optimal model of nucleotide substitution was selected using the AIC criterion. Models are listed in Table S12 .
Phylogenetic trees based on nucleotide data were obtained using a heuristic search with different optimality criteria of maximum likelihood (ML) and maximum parsimony (MP) as implemented in PAUP* ver 4.0a109 [74] for Macintosh (X86) and additional runs of ML as implemented in GARLI ver 0.96 [75] . In PAUP*, conditions for the ML analysis included starting trees obtained by stepwise addition, and branch swapping using the tree-bisection-reconnection (TBR) algorithm. The MP analyses used step-wise addition of taxa, TBR branch swapping and excluded indels. Support for nodes within the phylogeny used bootstrap analysis with identical settings established for each method of phylogenetic reconstruction and values greater than 50% were retained. The number of bootstrap iterations consisted of 1000 for MP methods and 100 for ML. Detailed control files used for GARLI ML analyses are available from corresponding author.
Bayesian Analyses of Primate Sequences: Posterior Probability, Node Support, and Divergence Dating
We estimated the phylogeny and divergence time splits simultaneously using a Bayesian approach as implemented in the program BEAST ver 1.5.3 [76] , [77] . Due to computational constraints, analyses were performed with 5 different sets of species: 1) genus-level data set including 61 Primate genera, two Dermoptera genera and one Scandentia genus rooted by Lagomorpha, 2) Catarrhini species with outgroups, 3) Platyrrhini species with outgroups, 4) Strepsirrhini species with outgroups and 5) genus-level analysis with a partitioned data set allowing for rate heterogeneity and different substitution models for autosome, X-chromosome, and Y-chromosome sequences.
By using the uncorrelated lognormal relaxed-clock model, rates were allowed to vary among branches without the a priori assumption of autocorrelation between adjacent branches. This model allows sampling of the coefficient of variation of rates, which reflects the degree of departure from a global clock. Based on the results of ModelTest, we assumed a GTR+I+G model of DNA substitution with four rate categories. Uniform priors were employed for GTR substitution parameters (0, 100), gamma shape parameter (0, 100) and proportion of invariant sites parameter (0, 1). The uncorrelated lognormal relaxed molecular clock model was used to estimate substitution rates for all nodes in the tree, with uniform priors on the mean (0, 100) and standard deviation (0, 10) of this clock model. We employed the Yule process of speciation as the tree prior and a Unweighted Pair Group Method with Arithmetic Mean (UPGMA) tree to construct a starting tree, with the ingroup assumed to be monophyletic with respect to the outgroup. To obtain the posterior distribution of the estimated divergence times, nine calibration points were applied as normal priors to constrain the age of the following nodes (labeled A-H in Figure 1 of main text): A) mean = 40.0 MYA, standard deviation (stdev) = 3.0 for time to most recent common ancestor (TMRCA) of galagids and lorisids [78] , B) mean = 43.0 MYA, stdev = 4.5 for TMRCA of Simiiformes [79] , [80] , C) mean = 29.0 MYA, stdev = 6.0 for TMRCA of Catarrhini [80] , D) mean = 23.5 MYA, stdev = 3.0 for TMRCA of Platyrrhini [26] , [81] , E) mean = 7 MYA, stdev = 1.0 for TMRCA of Papionini [82] , F) mean = 4.0 MYA, stdev = 0.4 for TMRCA of Theropithecus clade [40] , [83] , G) mean = 15.5 MYA, stdev = 2.5 for TMRCA of Hominidae [14] and H) mean = 6.5 MYA, stdev = 0.8 for TMRCA of Homo-Pan [84] . A normal prior for the mean root height of 90.0 MYA with stdev = 6.0 was used based on molecular estimates of MRCA of all Primates [14] , [82] , [85] . The calibration points selected are based on fossil dates that have undergone extensive review in previous publications and are supported by a consensus of paleoanthropologists. Rather than re-iterate the considerable amount of information forming the basis for each calibration point, we list the respective citations with the most detailed overview and attendant references.
Four to seven independent Markov chain Monte Carlo (MCMC) runs for each analysis were run for 20–100 million generations to ensure sampling of estimated sample size (ESS) values. The Auto Optimize Operators function was enabled to maximize efficiency of MCMC runs. Trees were saved every 1000 generations. Log files from each run were imported into Tracer ver 1.4.1, and trees sampled from the first 1 million generations were discarded. Mixing of trees was assessed in Tracer by examination of ESS values. Analysis of these parameters in Tracer suggested that the number of MCMC steps was more than adequate, with ESS of all parameters often exceeding 200, and Tracer plots showing strong equilibrium after discarding burn-in. Tree files from the individual runs were combined using LogCombiner ver 1.5.3 after removing 1000 trees from each sample. The maximum-clade-credibility tree topology and mean node heights were calculated from the posterior distribution of the trees. Final summary trees were produced in TreeAnnotator ver 1.5.3 and viewed in FigTree ver 1.3.1.
Computation of Nucleotide Substitution Rates
Heterogeneity in nucleotide substitution rates among primate taxa was assessed by a Bayesian approach, allowing for unequal rates of nucleotide substitution among lineages as implemented in BEAST. Rate estimates provided for each branch within the primate phylogeny were analyzed by ANOVA as implemented in SAS (SAS Institute Inc., SAS 9.1.3). Significant differences among means used the Duncan multiple means test.
Statistical Analyses of Insertion/Deletion Events among Primate Lineages
Indels were assessed as possible indicators of genome plasticity among primate lineages. An a priori approach was developed that used the derived primate phylogenetic tree ( Figure 2 ) as a guide for identification of synapomorphic and autapomorphic indels. First, all indels were identified using FASTGAP on GBLOCKED alignments and verified by eye. Second, only indels that correctly conformed to the species associations of the primate phylogeny ( Figure 2 ) were used and identified as a subset of synapomorphic events ( Table 1 , Table 2 , Table 3 ; Tables S5 , S6 ). Third, another subset of autapomorphic indels were identified and assessed as potential signatures of genome plasticity for a given species ( Tables S7 , S8 , S9 ). Infrequently, some indels included in the analysis were positioned in regions that did not amplify across all species. In these cases, indels were identified as synapomorphic for a lineage providing ∼70% of the relevant species were successfully PCR amplified, and that species with missing sequence for the indel did not all occur on the same node within the lineage. The hypothesis that patterns of nucleotide substitution are influenced by indel frequency was tested by regression of ln-transformed branch length against ln-transformed indels per branch. Tests of the association between genome rates of evolution and indel frequency were conducted by regression of the rate of nucleotide substitution (substitution/site/MY) versus ln-transformed indel frequency per branch. Statistical software used was SAS (SAS Institute Inc., SAS 9.1.3).
Supporting Information
Large tabloid format of Figure 2 from main text.
https://doi.org/10.1371/journal.pgen.1001342.s001
(1.23 MB PDF)
Maximum likelihood phylogeny of autosome data partition (27,427 bp).
https://doi.org/10.1371/journal.pgen.1001342.s002
(0.22 MB PDF)
Maximum likelihood bootstrap consensus phylogeny of autosome data partition (27,427 bp).
https://doi.org/10.1371/journal.pgen.1001342.s003
(0.28 MB PDF)
Maximum likelihood phylogeny of X-linked data partition (4,870 bp).
https://doi.org/10.1371/journal.pgen.1001342.s004
Maximum likelihood bootstrap consensus phylogeny of X-linked data partition (4,870 bp).
https://doi.org/10.1371/journal.pgen.1001342.s005
(0.29 MB PDF)
Maximum likelihood phylogeny of intron data partition (16,371 bp).
https://doi.org/10.1371/journal.pgen.1001342.s006
Maximum likelihood bootstrap consensus phylogeny of intron data partition (16,371 bp).
https://doi.org/10.1371/journal.pgen.1001342.s007
Maximum likelihood phylogeny of exon data partition (14,742 bp).
https://doi.org/10.1371/journal.pgen.1001342.s008
Maximum likelihood bootstrap consensus phylogeny of exon data partition (14,742 bp).
https://doi.org/10.1371/journal.pgen.1001342.s009
Figure S10.
Maximum likelihood phylogeny of UTR data partition (3,814 bp).
https://doi.org/10.1371/journal.pgen.1001342.s010
Figure S11.
Maximum likelihood bootstrap consensus phylogeny of UTR data partition (3,814 bp).
https://doi.org/10.1371/journal.pgen.1001342.s011
Figure S12.
Maximum likelihood phylogeny of Y-linked data partition of N = 127 males only (2,630 bp).
https://doi.org/10.1371/journal.pgen.1001342.s012
(0.03 MB PDF)
Figure S13.
Maximum likelihood bootstrap consensus phylogeny of Y-linked data partition of N = 127 males only (2,630 bp).
https://doi.org/10.1371/journal.pgen.1001342.s013
List of DNA specimens used in study.
https://doi.org/10.1371/journal.pgen.1001342.s014
(0.08 MB PDF)
List of 54 gene regions, primers and source used in study.
https://doi.org/10.1371/journal.pgen.1001342.s015
(0.05 MB PDF)
Putative gene ontology of 54 genes used in this study.
https://doi.org/10.1371/journal.pgen.1001342.s016
(0.07 MB PDF)
Chromosome location, phylogenetic informativity and nucleotide variation of 54 genes.
https://doi.org/10.1371/journal.pgen.1001342.s017
(0.04 MB PDF)
Synapomorphic and autapomorphic indels sorted by branch node and species listed in Figure 2 .
https://doi.org/10.1371/journal.pgen.1001342.s018
Synapomorphic and autapomorphic indels sorted by gene.
https://doi.org/10.1371/journal.pgen.1001342.s019
Genome rates, branch lengths, and indels for Strepsirrhini species and Tarsiiformes.
https://doi.org/10.1371/journal.pgen.1001342.s020
Genome rate, branch length, and indels within Platyrrhini species.
https://doi.org/10.1371/journal.pgen.1001342.s021
Genome rates, branch lengths, and indels for catarrhine species.
https://doi.org/10.1371/journal.pgen.1001342.s022
Genome rates across primate genera.
https://doi.org/10.1371/journal.pgen.1001342.s023
NCBI Accession Numbers.
https://doi.org/10.1371/journal.pgen.1001342.s024
Models of nucleotide substitution for maximum likelihood phylogenetic analyses of combined and partitioned data in Figure 2 , Figures S1 , S2 , S3 , S4 , S5 . NOTE: Y genes only 127 individuals (males).
https://doi.org/10.1371/journal.pgen.1001342.s025
Acknowledgments
We acknowledge generous support from D. Swofford for use of PAUP_dev_ICC. We thank explicitly sample providers listed in Table S1 . This research was not possible without the outstanding technical assistance from Amy Chen, Alex Peters, Christina Walker, Nathan Follin, Joseph Bullard, Amy Zheng, Rasshmi Shankar, Gary Chen, Matthew Healy, Lisa Maslan, and Carrie McCracken at the Laboratory of Genomic Diversity; Stephanie Merrett, Wendy Parris, and Lisa Bukovnik at Duke University; Christiane Schwarz at the German Primate Center; Kelly Rose Lobo de Souza and Shayany Pinto Felix at the Genetics Division-INCA; and Soraya Silva Andrade at the UFPA.
Author Contributions
Conceived and designed the experiments: JPS. Performed the experiments: PP HNS JEH MAMM MPCS AS JPS. Analyzed the data: PP WEJ JPS. Contributed reagents/materials/analysis tools: PP WEJ CR HNS JEH MAMM BK JP MR YR MPCS AS SJO JPS. Wrote the paper: PP WEJ CR HNS JEH MAMM JP MR MPCS AS SJO JPS. Implemented, supervised, and directed research project: JPS. Conducted phylogenetic and statistical analyses: JPS. Curated DNA samples and DNA sequences: PP. Performed BEAST analysis: WEJ.
- View Article
- Google Scholar
- 2. Groves CP (2001) Primate taxonomy. Washington, DC: Smithsonian Institution Press. 350 p.
- 3. Wilson DE, Reeder DM (2005) Mammal species of the world : a taxonomic and geographic reference. Baltimore: Johns Hopkins University Press. 2142 p.
- 8. Eizirik E MW, Springer MS, O'Brien SJ (2004) Molecular Phylogeny and Dating of Early Primate Divergences. In: Ross CF KR, editor. Anthropoid Origins: New Visions. New York: Kluwer Academic/Plenum. pp. 45–65.
- 11. Nowak RM (1999) Walker's mammals of the world. Baltimore: Johns Hopkins University Press. 1936 p.
- 36. Moynihan M (1976) The New World primates : adaptive radiation and the evolution of social behavior, languages, and intelligence. Princeton, N.J.: Princeton University Press. 262 p.
- 37. Fleagle JG (1988) Primate adaptation and evolution. San Diego: Academic Press. 486 p.
- 38. Delson E (1992) Evolution of Old World Monkeys. In: Jones S MR, Pilbeam D, editors. The Cambridge Encyclopedia of Human Evolution. Cambridge UK: Cambridge University Press. pp. 217–222.
- 71. Rambaut A (2007) Se-Al. Sequence Alignment Editor. Oxford: University of Oxford.
- 74. Swofford DL (2002) PAUP*. Phylogenetic Analysis Using Parsimony (*and Other Methods). Version 4. Sinauer Associates, Sunderland, Massachusetts.
- 75. Zwickl DJ (2006) Genetic algorithm approaches for the phylogenetic analysis of large biological sequence datasets under the maximum likelihood criterion. Austin: The University of Texas.
phylogenetic tree Recently Published Documents
Total documents.
- Latest Documents
- Most Cited Documents
- Contributed Authors
- Related Sources
- Related Keywords
Genetic similarities and phylogenetic analysis of Muntjac (Muntiacus spp.) by comparing the nucleotide sequence of 16S rRNA and cytochrome B genome
Abstract This study aimed to identify the phylogenetic similarities among the muntjac (Muntiacus spp.). The phylogenetic similarities among seven major muntjac species were studied by comparing the nucleotide sequence of 16s rRNA and cytochrome b genome. Nucleotide sequences, retrieved from NCBI databases were aligned by using DNASTAR software. A phylogenetic tree was created for the selected species of muntjac by using the maximum likelihood method on MEGA7 software. The results of nucleotide sequences (16s rRNA) showed phylogenetic similarities between, the M. truongsonensis and M. rooseveltorum had the highest (99.2%) while the lowest similarities (96.8%) found between M. crinifrons and M. putaoensi. While the results of nucleotide sequences (Cty b) showed the highest similarity (100%) between M. muntjak and M. truongsonensis and the lowest s (91.5%) among M. putaoensis and M. crinifrons. The phylogenetic tree of muntjac species (16s rRNA gene) shows the main two clusters, the one including M. putaoensis, M. truongsonensis, M. rooseveltorum, and M. muntjak, and the second one including M. crinifrons and M. vuquangensis. The M. reevesi exists separately in the phylogenetic tree. The phylogenetic tree of muntjac species using cytochrome b genes shows that the M. muntjak and M. truongsonensis are clustered in the same group.
matOptimize: A parallel tree optimization method enables online phylogenetics for SARS-CoV-2
Phylogenetic tree optimization is necessary for precise analysis of evolutionary and transmission dynamics, but existing tools are inadequate for handling the scale and pace of data produced during the COVID-19 pandemic. One transformative approach, online phylogenetics, aims to incrementally add samples to an ever-growing phylogeny, but there are no previously-existing approaches that can efficiently optimize this vast phylogeny under the time constraints of the pandemic. Here, we present matOptimize, a fast and memory-efficient phylogenetic tree optimization tool based on parsimony that can be parallelized across multiple CPU threads and nodes, and provides orders of magnitude improvement in runtime and peak memory usage compared to existing state-of-the-art methods. We have developed this method particularly to address the pressing need during the COVID-19 pandemic for daily maintenance and optimization of a comprehensive SARS-CoV-2 phylogeny. Thus, our approach addresses an important need for daily maintenance and refinement of a comprehensive SARS-CoV-2 phylogeny.
Distribution of Therapeutic Efficacy of Ranunculales Plants Used by Ethnic Minorities on the Phylogenetic Tree of Chinese Species
The medicinal properties of plants can be evolutionarily predicted by phylogeny-based methods, which, however, have not been used to explore the regularity of therapeutic effects of Chinese plants utilized by ethnic minorities. This study aims at exploring the distribution law of therapeutic efficacy of Ranunculales plants on the phylogenetic tree of Chinese species. We collected therapeutic efficacy data of 551 ethnomedicinal species belonging to five species-rich families of Ranunculales; these therapeutic data were divided into 15 categories according to the impacted tissues and organs. The phylogenetic tree of angiosperm species was used to analyze the phylogenetic signals of ethnomedicinal plants by calculating the net relatedness index (NRI) and nearest taxon index (NTI) in R language. The NRI results revealed a clustered structure for eight medicinal categories (poisoning/intoxication, circulatory, gastrointestinal, eyesight, oral, pediatric, skin, and urinary disorders) and overdispersion for the remaining seven (neurological, general, hepatobiliary, musculoskeletal, otolaryngologic, reproductive, and respiratory disorders), while the NTI metric identified the clustered structure for all. Statistically, NRI and NTI values were significant in 5 and 11 categories, respectively. It was found that Mahonia eurybracteata has therapeutic effects on all categories. iTOL was used to visualize the distribution of treatment efficacy on species phylogenetic trees. By figuring out the distribution of therapeutic effects of Ranunculales medicinal plants, the importance of phylogenetic methods in finding potential medicinal resources is highlighted; NRI, NTI, and similar indices can be calculated to help find taxonomic groups with medicinal efficacy based on the phylogenetic tree of flora in different geographic regions.
Isolation of purple non-sulfur bacteria from the digestive tract of ayu (Plecoglossus altivelis)
Abstract Purple non-sulfur bacteria (PNSB) reportedly have probiotic effects in fish, but whether they are indigenous in the digestive tract of fish is a question that requires answering. We attempted to isolate PNSB from the digestive tract of ayu (Plecoglossus altivelis) from the Kuma River (Kumamoto, Japan), and successfully isolated 12 PNSB strains. All the isolated PNSB belonged to the genus Rhodopseudomonas. Five Rhodopseudomonas strains were also isolated from the soil samples collected along the Kuma River. The phylogenetic tree based on the partial sequence of pufLM gene indicated that the PNSB from ayu and soil were similar. The effects of NaCl concentration in growth medium on growth were also compared between the PNSB from ayu and soil. The PNSB from ayu showed a better growth performance at a higher NaCl concentration, suggesting that the intestinal tract of ayu, a euryhaline fish, might provide suitable environment for halophilic microorganisms.
Phylogeny of different Helicobacter pylori strains could not be explained by 16S rRNA gene due to high similarity in gene sequence across strains
Abstract Understanding the evolutionary relatedness of different strains of a species helped identify strain-specific differences that may be useful for disease diagnosis and treatment. Typically, such strain level typing would be augmented by molecular assays such as DNA sequencing, and phylogenetic tree analysis. This work utilizes public data on the 16S rRNA gene sequence of different strains of Helicobacter pylori to help plot the phylogenetic tree that describes the evolutionary trajectories of the different strains. Results from multiple sequence alignment reveals high level of conservation in 16S rRNA gene sequence across strains. This then translates into a phylogenetic tree structure that suggests very close evolutionary relationships of the different strains except for one outlier strain. Even in the case of the outlier strain, its evolutionary distance from other brethren was also not large. Overall, the results obtained in this study indicates that 16S rRNA gene may not capture strain-level phylogeny between different strains of the same species, and point to efforts in elucidating this phylogenetic effect in other genes of the species. Such genes may be involved in virulence during pathogenesis in humans, and may thus be subjected to higher evolutionary pressure and natural selection.
Phylogenetic tree analysis for Bali Cattle based on partial sequence 16S rRNA Mitochondrial DNA
Mitochondria DNA (mtDNA) as a source of genetic information based on the maternal genome, can provide important information for phylogenetic analysis and evolutionary biology. The objective of this study was to analyze the phylogenetic tree of Bali cattle with seven gene bank references (Bos indicus, Bos taurus, Bos frontalis, and Bos grunniens) based on partial sequence 16S rRNA mitochondria DNA. The Bayesian phylogenetic tree was constructed using BEAST 2.4. and visualization in Figtree 1.4.4 (tree.bio.ed.ac.uk/software/figtree/). The best model of evolution was carried out using jModelTest 2.1.7. The most optimal was the evolutionary models GTR + I + G with p-inv (I) 0,1990 and gamma shape 0.1960. The main result indicated that the Bali cattle were grouped into Bos javanicus. Phylogenetic analysis also successfully classifying Bos javanicus, Bos indicus, Bos taurus, Bos frontalis and Bos grunniens. These results will complete information about Bali cattle and useful for the preservation and conservation strategies of Indonesian animal genetic resources.
Identification of Single Nucleotide Polymorphism in Y-chromosome Specific DDX3Y Gene in Murrah Buffalo Bulls
Background: Molecular markers based approaches are essential to select fertile bulls for frozen semen production at an early age. The present investigation was undertaken to perform the molecular characterization and identify single nucleotide polymorphisms (SNP) in Y-chromosome specific DDX3Y gene in Murrah buffalo bulls. Methods: The genomic DNA isolated from the blood samples of 70 Murrah buffalo bulls, covering bulls with normal seminal traits and poor production performance (poor semen quality, freezability, libido), were subjected to PCR amplification. The sequences of DDX3Y gene were analyzed for single nucleotide polymorphism using the seqman module of DNASTAR LASERGENE software. The single nucleotide variations in the sequences with reference to the Bos taurus sequence were determined using Clustal W. The phylogenetic tree and genetic distance were constructed using the MegAlign module. Result: The analysis of sequences revealed that the exons and their adjacent intronic regions of the DDX3Y gene are monomorphic in nature without any variations indicating that the sequences are highly conserved in the studied population of Murrah buffalo bulls. However, a considerable number of single nucleotide variations were observed in the sequences of Murrah buffalo compared with Bos taurus sequences. Furthermore, the phylogenetic tree analysis revealed less divergence and close genetic association between the sequences of Murrah buffalo and other species in the bovinae family than the caprinea species. Further studies on DDX3Y gene in a more extensive and diverse population of Murrah buffalo bulls distributed in different regions could aid to discover substantial SNPs.
A multi-modal algorithm based on an NSGA-II scheme for phylogenetic tree inference
Morphological and molecular characterization of paragonimus species isolated from freshwater crabs in southern yunnan, china.
Paragonimus species are highly prevalent in various regions of China. The study’s objective is to isolate and identify Paragonimus from natural habitats and compare the phylogenetic diversity of Paragonimus in southern Yunnan province, China. Metacercariae of Paragonimus was isolated from crabs, and morphologic identification was performed by microscopy. Metacercariae were injected into experimental Paragonimus free Sprague Dawley rats. After 114 days, adult worms and eggs were isolated from multiple organs. Morphologic identification confirmed the initial identification. DNA was extracted from 5 adult worms, and molecular characterization was performed by amplification and sequencing of CO1 and ITS2 regions, followed by phylogenetic analysis. Out of 447 crabs captured, 186 crabs were found to be infected. A total of 4 species of Paragonimus was observed from naturally infected crabs. Paragonimus microrchis (2), Paragonimus heterotremus (1), Paragonimus proliferus (1), and Paragonimus skrjabini (1) were isolated and identified. A total of 32 sequences were downloaded from the National Center for Biotechnology Information, and 5 sequences generated in the study were used for phylogenetic analysis. In the phylogenetic tree of the CO1 gene, Paragonimus proliferus, Paragonimus heterotremus, and Paragonimus skrjabini were clustered with the same species, and the confidence values of their branches were >95%. A congruent phylogenetic relationship was observed with the ITS2 phylogenetic tree. In the phylogenetic tree constructed with the combined dataset of CO1 and ITS2 datasets, Paragonimus proliferus, Paragonimus heterotremus, and Paragonimus skrjabini clustered with the same species, and their branch confidence values were >94%. Paragonimus microrchis clustered with Paragonimus bangkokensis in both datasets. Phylogenetic analysis revealed robustness of the double loci method as against the single-locus method with either CO1 or ITS2 alone. Paragonimus species isolated from the southern Yunnan province, China, was phylogenetically diverse, and the analysis revealed the clustering of multiple species of Paragonimus isolated from different geographic locations.
A new subspecies of Stellaria alsine (Caryophyllaceae) from Yakushima, Japan
An unknown taxon of Stellaria was discovered in Yakushima, a Japanese island known to harbor several endemic species. To determine the identity of this taxon, this study employed MIG-seq for the reconstruction of a finely resolved phylogenetic tree of the newly discovered taxon, along with some related species of Stellaria. The results showed that the newly discovered taxon is a relative of S. alsine. Based on this result, Stellaria alsine subsp. nanasubsp. nov. was published.
Export Citation Format
Share document.


An official website of the United States government
The .gov means it’s official. Federal government websites often end in .gov or .mil. Before sharing sensitive information, make sure you’re on a federal government site.
The site is secure. The https:// ensures that you are connecting to the official website and that any information you provide is encrypted and transmitted securely.
- Publications
- Account settings
Preview improvements coming to the PMC website in October 2024. Learn More or Try it out now .
- Advanced Search
- Journal List
- Elsevier - PMC COVID-19 Collection

Phylogeny of Viruses ☆
Biological species, including viruses, change through generations and over time in the process known as evolution. Viruses may evolve at high, uneven, and fluctuating rates among genome sites. The accumulated changes, through either mutation or recombination with other species, are first fixed in the genome of successful individuals that give rise to genetic lineages. The relationship between biological lineages related by common descent is called ‘phylogeny’. For inferring phylogeny, the differences between aligned sequences of genomes and proteins are quantified and depicted in the form of a tree, in which contemporary species and their intermediate and common ancestors occupy, respectively, the terminal nodes, internal nodes, and the root. The tree is characterized by a topology, length of branches, shape, and the root position. A complex mathematical apparatus has been developed for phylogeny inference that can evaluate inter-species differences, facilitate tree building and comparison of trees, and assess the fit between data and tree through, typically, computationally intensive calculations. A reconstructed tree is an approximation of the true phylogeny that practically remains unknown. The phylogenetic analysis is used in applied and fundamental virus research, including epidemiology, diagnostics, forensic studies, phylogeography, evolutionary studies, and virus taxonomy. It can provide an evolutionary perspective on variation of any trait that can be measured for a group of viruses.
Introduction: Evolution, Phylogeny, and Viruses
Biological species, including viruses, change through generations and over time in the process known as evolution. These changes are first fixed in the genome of successful individuals that give rise to genetic lineages. Due to either limited fidelity of the replication apparatus copying the genome or physico-chemical activity of the environment, nucleotides may be changed, inserted, or deleted. Genomes of other origin may also be a source of innovation for a genome through the use of specially evolved mechanisms of genetic exchange (recombination). Accepted changes, known as mutations, may be neutral, advantageous, or deleterious, and depending on the population size and environment, the mutant lineage may proliferate or go extinct. Overall, advantageous mutations and large population size increase the chances for a lineage to succeed. The fitness of a lineage is constantly re-assessed in the ever-changing environment and lineages that, due to mutation, became a success in the past could be unfit in the new environment. Due to the growing number of mutations accumulating in the genomes, lineages diverge over time, although occasionally, due to stochastic reasons or under similar selection pressure, they may converge.
The relationship between biological lineages related by common descent is called phylogeny; the same term also embodies the methodology of reconstructing these relationships. Phylogeny deals with past events and, therefore, it is reconstructed by quantification of differences accumulated between lineages. Due to the lack of fossils and (relatively) high mutation rates, viruses were not considered to provide a recoverable part of phylogeny until the advent of molecular data proved otherwise. Comparison of nucleotide and amino acid sequences, and, occasionally, other quantitative characteristics such as distances between three-dimensional structures of biopolymers, have been used to reconstruct virus phylogenies. Results of phylogenetic analysis are typically depicted in the form of a tree that may be used as a synonym for phylogeny. For instance, the all-inclusive phylogeny of cellular species is known as the Tree of Life (ToL) ( Fig. 1A ). More recently, two techniques, networks and forests of trees, are used to depict the complexity of phylogenetic relations and the uncertainty of phylogenetic inference, respectively.

Phylogeny of nidoviruses in comparison to the Tree of life (ToL). Bayesian phylogenies under the WAG amino acid substitution model with rate heterogeneity across sites and relaxed molecular clock with log-normal distribution of nidoviruses (A) and ToL (B) are drawn to a common scale of 0.2 amino acid substitutions per position. Major lineages are indicated by vertical bars and names; arteri: Arteriviridae , mesoni: Mesoniviridae , roni: Roniviridae , toro: Torovirinae , corona: Coronavirinae . Support values at basal internal nodes are posterior probability support values. (C) Distributions of pair-wise patristic distances extracted from (A) and (B). The combined set of distances was normalized relative to the largest distance that was set to one.
With few exceptions, virus phylogeny follows the theory and practice developed for phylogeny of cellular life forms. For inferring phylogeny, differences between the sequences of species members, assumed to be of a discernable common origin, are analyzed. If species in all lineages evolve at a uniform constant rate, like clocks tick, their evolution conforms to a molecular clock model. The utility of this model in relation to viruses may be very limited. Rather, related virus lineages may evolve at different and fluctuating rates and some sites may mutate repeatedly, including reverse substitutions. As a result, reconstruction of a full record of change at all sites is associated with ever increasing uncertainty with each new mutation. Furthermore, the accumulation of inter-species residue differences may progress nonlinearly with the time elapsed. At present, our understanding of these parameters of virus evolution is poor and this limits our ability to assess the fit between a reconstructed phylogeny and the true phylogeny, with the latter practically remaining unknown for most virus isolates. This gap in our knowledge does not undermine the conceptual strength and utility of phylogenetic analysis for reconstructing the relationships between biological species including viruses.
The ultimate goal of virus phylogeny is reconstructing the relationships between ‘all’ virus isolates and species. For instance, cellular species form three (or two) compact domains (kingdoms) and their origin can be traced back to a common ancestor in the ToL, using either ribosomal RNA or a common set of single-copy genes. Such inference is not feasible for viruses due to their diversity and the lack of a universal molecular denominator (trait). Thus, reconstructing the comprehensive virus phylogeny may require comparisons that involve genomes of viral and cellular origins. This formidable task remains largely ‘work in progress’. In fact, most efforts in virus phylogeny are invested in reconstructing the relationships at the micro, rather than grand, scale and they focus on well-sampled lineages that have practical (e.g., medical) relevance. Most recently, due to the advent of high-throughput next generation sequencing (NGS) and metagenomics, phylogeny of distant relations to characterize diverse viromes and the entire Virosphere has become an active area of research. Phylogeny itself or in combination with other data may provide a deep insight into virus evolution and diverse aspects of virus life cycles, including virus interactions with their hosts.
Our knowledge about contemporary virus diversity has been steadily advancing with new viruses being constantly described by systematic efforts as well as occasional discoveries. These developments indicate that only a small part of virus diversity has so far been unraveled and has become available for phylogenetic studies. It is also likely that many more lineages existed in the past; some of these lineages are likely to have ancestral relationships with contemporary lineages.
Tree Definitions
Species share similarity that varies depending on the rate of evolution and time of divergence. The entire process of generating contemporary species diversity from a common ancestor is believed to proceed through a chain of intermediate ancestors specific for different subsets of the analyzed species ( Fig. 2 ). Typically, these ancestral sequences are estimated internally during the tree building process or are not required at all, depending on the method used. The relationship between the common ancestor, intermediate ancestors, and contemporary species may be likened to the relationship between, respectively, root, internal nodes, and terminal nodes (leaves) of a tree, an abstraction that is widely used for the visualization of this relationship ( Fig. 2 ). Alignment of the contemporary sequences with the reconstructed tree side by side, like shown for the toy example in Fig. 2 , may reveal the full chain of sequence changes that have happened during evolution which, however, is rarely the case for real data sets due to repeated substitutions and incomplete species sampling. Trees are also part of graph theory, a branch of mathematics, whose apparatus is used in phylogeny. Formally and due to a strong link between phylogeny and taxonomy, leaves may be called operational taxonomy units (OTUs) and internal nodes and roots, since they have not been directly observed, are known as hypothetical taxonomy units (HTUs). Nodes are connected by branches or edges.

Phylogenetic tree and molecular evolution. Shown is a toy example of evolution of an ancestral sequence (to the right of the black-filled circle) of length four into five extant sequences (to the right of the open circles) and the corresponding phylogenetic tree. The respective substitutions and sequence positions are indicated at the tree branches. Sequences at internal nodes (gray-filled circles) are reconstructions of the tree building process. Note the multiple substitutions at sequence position two in the second extant sequence resulting in reversion to the ancestral state (denoted with *).
The tree may be characterized by topology, length of branches, shape, and the position of the root ( Fig. 3 ). The topology is determined by relative positions of internal and terminal nodes; it defines branching events leading to contemporary species diversity. If two or more trees obtained for different data sets feature a common topology, these trees are called congruent. The branch length of a tree may define either the amount of change fixed or the time passed between two nodes connected in a tree, and is known as ‘additive’ or ‘ultrametric’, respectively ( Fig. 3B and C ). The tree shape may be linked to particulars of the evolutionary process and reflect changes in population size and diversity due to genetic drift and natural selection. The position of the root at the tree defines the direction of evolution. Species that descend from an internal node in a rooted tree form a lineage (cluster) and the node is called most recent common ancestor (MRCA) of the lineage that thus has a monophyletic origin ( Fig. 2 ). The branch lengths and the root position may be left undefined for a tree that is then called ‘cladogram’ and ‘unrooted tree’, respectively ( Fig. 3A ).

Tree types and pitfalls of phylogeny reconstruction. (A) Unrooted tree for five hypothetical viruses that was reconstructed based on their gene or protein sequences. Branch lengths represent the amount of genetic change between two viruses typically measured in units of substitutions per site. The direction of evolution is undetermined. (B) The tree for the five ingroup viruses in A was rooted using an outgroup (o). The direction of evolution is from left to right. (C) The same tree as in B but with branches calibrated to represent time. Note that time does not necessarily correlate with the amount of genetic change (for instance, compare length of the branch leading to the cluster joining i4 and i5 with that of the same branch in B. (D) The relative positions of three highly divergent lineages is unresolved by the phylogeny (polytomy). (E) The true relationship of four hypothetical sequences (top) is not recovered by the phylogenetic reconstruction (bottom) due to long branch attraction involving i2 and i3. (F) Phylogenetic trees (bottom) reconstructed for three adjacent genomic regions (top) are different with respect to the position of i2 which was subject to a recombination event in middle genome region.
Phylogenetic Analysis
Multiple alignments of polynucleotide or amino acid sequences representing analyzed species and maximized for similarity are traditionally used as input for phylogenetic analysis. The quality of alignment is among the most significant factors affecting the quality of phylogenetic inference. Due to the redundancy of the genetic code, changes in polynucleotide sequences are accumulated at a higher rate than those in amino acid sequences. In viruses, including RNA viruses, this difference is not counterbalanced by other local or global constraints on variation of genomes that are linked to e.g. di-nucleotide frequency or RNA secondary (tertiary) structure. Because of these differences, polynucleotide sequences are commonly used for phylogeny reconstruction of only those species that are closely related, while protein sequences, preserving better phylogenetic signal, may be used to infer phylogeny of distantly related species.
Differences between species, as calculated from alignment, may be quantified as either pairwise distances forming a distance matrix or position-specific substitution columns (discrete characters of states of alignment), the latter preserving the knowledge about location of differences. The respective methods dealing with these quantitative characteristics are known as distance and discrete (character state). The distance methods are praised for their speed and are considered a technique of choice for analysis of very large data sets, although character state methods caught up in this respect due to recent algorithmic advancements (see also below). Distance methods are often designed to converge on a unique phylogeny by clustering, with none others being even considered. The unweighted pair group method with arithmetic means (UPGMA) in which a constantly recalculated distance matrix is used to define the hierarchy of similarities through systematic and stepwise merging of most similar pairs at a time was the first technique introduced for clustering. The neighbor-joining (NJ) method uses a more sophisticated algorithm of clustering that minimizes branch lengths, and is the most popular among distance methods.
Although different trees may be compared in how they fit a distance matrix, it is character-based methods that are routinely used to assess numerous alternative phylogenies in search for the best one in a computationally intensive process. Due to the calculation time involved, assessing all possible phylogenies is found to be impractical for data sets including more than 10 sequences; for larger data sets different heuristic approximations are used that may not guarantee a recovered phylogeny to be the best overall. There are two major criteria for selecting the best phylogeny using character-state based information through either maximum parsimony (MP) or maximum likelihood (ML). In MP analysis, a phylogeny with a minimal number of substitutions separating the analyzed species is sought. The ML analysis offers a statistical framework for comparing the likelihood of fitting different trees to the data under competing models of evolution with parameters including population size change and rate of mutation in search for one with the best fit. The latter approach is mathematically robust and its statistical power may also be used in combination with other techniques of tree generation. Recently, a Bayesian variant of the ML approach has gained popularity. It can utilize prior knowledge about the evolutionary process, like known substitution rates or clustering of species subsets or dates of species isolation, in combination with repeated sampling from subsequently derived hypotheses. The result of a Bayesian analysis is thus a forest of trees that reflects the uncertainty associated with the reconstructed phylogeny and which forms the basis to derive a consensus tree and statistic support for its branches. In phylogenetic analysis of viruses the dates of species isolation are often used to date the MRCA of the analyzed viruses under a Bayesian framework, while fossil information is routinely used to time-calibrate trees of cellular organisms. Bayesian methods have the highest computational cost due to their sampling approach and thus show the lowest speed, while realization of the similarly advanced ML algorithm may be largely comparable in speed to distance methods, allowing for the phylogenetic analysis of very large data sets like genome-wide tree reconstructions of cellular organisms or thousands of viruses.
One should keep in mind that different methods for phylogeny reconstruction can produce different trees, concerning both topology and branch lengths, for the same data set, although better agreement between ML and Bayesian trees is common, especially in respect to branch lengths ( Fig. 4 ). None of the methods is considered superior to the other methods with respect to all aspects of phylogeny reconstruction, and which method to use under what circumstances is often a point of debate. A valid approach to gain further confidence in phylogenetic results is to apply several methods on the data and to only trust HTUs that are inferred by more than one method.

Comparison of phylogenetic results between methods. Shown are unrooted NJ, ML and Bayesian trees reconstructed for the same dataset of 287 aligned VP1 protein positions of 93 polyomaviruses. The LG amino acid substitution model with site heterogeneity modeled by a gamma distribution with four categories, as selected by ProtTest, was used. In the Bayesian analysis, a relaxed molecular clock approach with log-normally distributed rate was applied. The trees are drawn to the same scale of average amino acid substitutions per site, as indicated by the bar in the middle. Note the considerably shorter branch lengths of the NJ tree compared to the other two trees. Robinson-Foulds distances measuring the topological differences between tree pairs are shown in gray.
After a tree is chosen, it is common to assign support values to internal nodes through assessing the nodes’ persistence in trees related to the chosen tree. One particular technique, called bootstrap analysis, in which trees are generated for numerous randomly modified derivatives of the original data set, is most frequently used in distance-based as well as MP and ML analysis. Each internal node in the original tree is characterized by a so-called bootstrap value that is equal to the number of times a node appears in all tested trees. Although the relationship between bootstrap and statistical values is not linear, nodes with very high bootstrap values are considered to be reliable. In a Bayesian analysis, the support of internal nodes is quantified through posterior probability values.
If species evolve according to a molecular clock model, the root position in a tree could directly be calculated from the observed inter-species differences as a midpoint of cumulative inter-species differences. Alternatively, the root position may be assigned to a tree from knowledge about the analyzed species that was gained independently from phylogenetic analysis. Commonly, this knowledge comes in the form of a single or more species which are assumed (or known) to have emerged before the ‘birth’ of the analyzed cluster. These early diverged species are collectively defined as ‘outgroup’, while the analyzed species may be called ‘in group’ ( Fig. 3B, C ). Also, a tree may be generated unrooted, a common practice in phylogenetic analysis of viruses for which the applicability of the molecular clock model remains largely untested and reliable outgroups may not be routinely available ( Fig. 4 ). In an unrooted tree, grouping of species in separate clusters may be apparent, although these clusters may not be treated as monophyletic as long as the direction of evolution has not been defined. These challenges are addressed by the development of new approaches that infer rooted trees without artificially restricting species evolution to a constant rate (known as relaxed molecular clock models).
Virus phylogeny can be inferred using either genomes or distinct genes and each of these approaches, standard in phylogenomics, may be considered as complementary. Under the first approach, genome-wide alignments are used for analysis. Due to complexities of the evolutionary process that may be region specific, reliable genome-wide alignments can routinely be built only for relatively closely related viruses whose analysis, however, may be further complicated by recombination events (see below). Using the second approach, genes with no evidence for recombination may be merged (concatenated) in a single data set that may be used to produce a superior phylogenetic signal compared to those generated for distinct genes or entire genomes. For viruses with small genomes or for a diverse set of viruses, it is common practice to use a single gene to infer virus phylogeny. Although the results produced may be the best models describing evolutionary history of a group of viruses, the validity of this gene-based approach for the genome-wide extrapolation remains a point of debate. Recently, network methods were used to infer and depict evolutionary relationships of multigene virus genomes taking into account gene-specific sequence affinities.
When the gene tree is used as representing the phylogeny of the entire genome, an underlying most common assumption is that its topology but not branch lengths holds for different genomic regions in reflection of their coevolution with potentially different rates of substitution. This assumption may be violated due to several evolutionary processes, including orthologous gene exchange between (closely) related viruses, gene duplication and horizontal gene transfer (HGT), all involving one or another form of recombination, or incomplete lineage sorting. In phylogenetic terms, this violation may be revealed through incongruency of trees built for different genome regions ( Fig. 3F ). Trees may also become incongruent due to various technical reasons related to the size and diversity of a virus data set. These characteristics complicate interpretation of the congruency test, which is widely used in different programs to identify recombination in viruses. Other pitfalls of phylogenetic reconstruction include the inability to resolve basal branching patterns of highly divergent lineages ( Fig. 3D ) and the relatively close clustering of lineages that are only distantly related and do not form a monophyletic group in the true (unknown) phylogeny ( Fig. 3E ). The latter phenomenon is known as long branch attraction (LBA) and the phylogenetic artifacts produced by LBA are most frequently observed for isolated, that is, long branches in the tree which represent distant lineages with no close relatives known.
Applications of Phylogeny in Virology
Phylogenetic analysis is used in a wide range of studies to address both applied and fundamental issues of virus research, including epidemiology, diagnostics, forensic studies, phylogeography, origin, evolution, and taxonomy of viruses. The first questions to be answered during an outbreak of a virus epidemic concern the virus identity and origin. Answers to these questions form the basis for implementing immediate practical measures and prospective planning, enabling specific and rapid virus detection and epidemic containment, which may include the use and development of antiviral drugs and vaccines. Among different analyses performed for virus identification at the early stage of a virus epidemic, the phylogenetic characterization is used for determining the relationship of a newly identified virus with all other previously characterized and sequenced viruses.
Results of this analysis may be sufficient to provide answers to the questions posed, as regularly happens with closely monitored viruses that include most human viruses of high social impact, for example, influenza virus, human immunodeficiency virus (HIV), hepatitis C virus (HCV), poliovirus, and others. For these viruses, there exist large databases of previously characterized isolates and strains that comprehensively cover the so far characterized natural diversity. Should a newly identified virus belong to one of these species, chances are that it has evolved from a previously sampled isolate or a close variant and this immediately becomes evident in the clustering of these viruses in the phylogenetic tree. Combining the results of gene-specific and genome-wide phylogenetic analysis allows one to determine whether recombination contributed to the isolate origin. For instance, recombination was found to be extremely uncommon in the evolution of HCV, but not for poliovirus lineages that recombine promiscuously, also with closely related human coxsackie A viruses, both of which belong to the same virus species of human enteroviruses known as Enterovirus C .
When an emerging infection is caused by a new never-before-detected virus, the phylogenetic analysis is instrumental for classification of this virus and in the case of a zoonotic infection, for determining the dynamic of virus introduction into the (human) population and initiating the search for the natural virus reservoir. This was the case with many emerging infections including those caused by Nipah virus, a paramyxovirus, SARS coronavirus (SARS-CoV), MERS coronavirus (MERS-CoV), ebolavirus, and Zika virus. In the case of SARS-CoV, poor sampling of the coronavirus diversity in the lineage at the time, some uncertainty over the relationship between phylogeny and taxonomy of coronaviruses, and the complexity of phylogenetic analysis of a virus data set including isolated distant lineages led to considerable controversy over the exact evolutionary position of SARS-CoV among coronaviruses. Since then, the matter has fully been resolved but this experience illustrates some challenges in inferring virus phylogeny.
The search for a zoonotic reservoir of an emerging virus may involve a significant and time-consuming effort that requires numerous phylogenetic analyses of ever-expanding sampling of the virus diversity generated in pursuit of the goal. In this quest, phylogenetic analysis canalizes the effort and provides crucial information for reconstructing parameters of major evolutionary events that promoted the virus origin and spread. For instance, intertwining HIV and simian immunodeficiency virus (SIV) lineages in the primate lentivirus tree led to the postulation that the existing diversity of HIV in the human population originated from several ancestral viruses independently introduced from primates over a number of years. Similar phylogenetic reasoning was used to trace the origin of a local HIV outbreak to a common source of HIV introduction through dental practice (known as ‘HIV dentist’ case). These are typical examples illustrating the utility of phylogenetic analysis for epidemiological and forensic studies.
Geographic distribution of places of virus isolation is another important characteristic relative to which virus phylogeny may be evaluated. This field of study belongs to phylogeography. The evolution of human JC polyomavirus provides an example of confinement of circulation of virus clusters to geographically isolated areas, represented by three continents. Identification of West Nile virus in the USA illustrates a geographical expansion of an Old World virus into the New World. Analysis of phylogenies of field isolates of rabies virus of the family Rhabdoviridae sampled from different animals across Europe led to the recognition that interspecies virus expansion occurs faster when compared to geographical expansion.
Phylogenies can also reveal information about the relative strength of the virus–host association over time. In some virus families (e.g., the Coronaviridae ) host-jumping events may be relatively frequent in establishing new species, including the emergence of at least three human viruses, dead-end SARS-CoV and MERS-CoV and successfully circulating human coronavirus OC43 (HCoV-OC43). At the other end of the spectrum one finds the family Herpesviridae . Extensive phylogenetic analysis of herpesviruses and their hosts showed a remarkable congruency of topologies of trees indicating that this virus family may have emerged some 400 million years ago and that herpesviruses largely cospeciate with their hosts. Moreover, through phylogenetic analysis one can show that most viruses, and in particular RNA viruses, evolve at rates that are orders of magnitude faster than those of cellular organisms. For instance, even the most conserved enzymes encoded by nidoviruses, that comprise just four RNA virus families, accumulated more than twice as many substitutions during evolution than their counterparts across the ToL, as estimated through branch lengths of the respective phylogenetic trees ( Fig. 1 ). Taking into account that the MRCA of all cellular organisms predates that of nidoviruses, this reveals that most residues of viral proteins changed repeatedly and more frequently than cellular protein residues during long-term evolution. In fact, this high evolutionary rate seems to be a prerequisite for RNA viruses to stay fit in the ever-changing environment considering their tiny genomes that would otherwise not be able to produce enough genetic variation.
Phylogenetic analysis becomes increasingly important in virus classification (taxonomy) whose development relies on complex multicharacter rules applied to separate virus families by respective ‘study groups’. For viruses united in high-rank taxa above the genus level, phylogenetic clustering for most conserved replicative genes is commonly observed and used in the decision making process. For instance, human hepatitis E virus, originally classified as a calicivirus using largely virion properties, was eventually expelled from the family due to poor fit of genome characteristics, including results of phylogenetic analysis. Phylogenetic considerations also played an important role in establishing new families, for example, the Marnaviridae and Dicistroviridae . In contrast, phylogenetic analysis has been of relatively little use in the taxonomy of large DNA phages which has been developed in such a way that existing families may unite phages with different gene layouts and phylogenies. The relationship between phylogeny and taxonomy is evolving and efforts were made in extracting taxa structure from monophyletic clusters in trees using analysis of pairwise evolutionary distances. In future one might hope for important advancements of virus taxonomy that improve cross-family consistency in relation to phylogeny.
Acknowledgements
AEG research was partially supported by Leiden University Fund and EU Horizon2020 project EVAg 653316.
☆ Change History: December 2016. AE Gorbalenya and C Lauber updated sections Introduction, Tree Definitions, Phylogenetic Analysis and Applications of Phylogeny in Virology, the reference section. Introduced Figures 1-4.
Further Reading
- Dolja VV, Koonin EV, editors. Comparative genomics and evolution of complex viruses Virus Research. 2006; 117 :1–184. [ PubMed ] [ Google Scholar ]
- Domingo E. Virus evolution. In: Knipe D.M., Howley P.M., Griffin D.E., editors. Fields virology. 5th edn. Wolters Kluwer, Lippincott Williams and Wilkins; Philadelphia, PA: 2007. pp. 389–421. [ Google Scholar ]
- Domingo E, Webster RG, Holland JJ, editors. Origin and evolution of viruses. Academic Press; San Diego: 1999. [ Google Scholar ]
- Drummond A.J., Suchard M.A., Xie D., Rambaut A. Bayesian phylogenetics with BEAUti and the BEAST 1.7. Molecular Biology and Evolution. 2012; 29 :1969–1973. [ PMC free article ] [ PubMed ] [ Google Scholar ]
- Felsenstein J. Sinauer Associates, Inc; Sunderland, MA: 2004. Inferring phylogenies. [ Google Scholar ]
- Gibbs A.J., Calisher C.H., Garcia-Arenal F. Cambridge University Press; Cambridge: 1995. Molecular basis of virus evolution. [ Google Scholar ]
- King A.M.Q., Adams M.J., Carstens E.B., Lefkowitz E.J., editors. Virus taxonomy: the 9th report of the international committee on taxonomy of viruses. Elsevier, Academic Press; San Diego, CA: 2012. [ Google Scholar ]
- Lauber C., Goeman J., Parquet M.D.C., Nga P.T., Snijder E.J., Morita K., Gorbalenya A.E. The footprint of genome architecture in the largest genome expansion in RNA viruses. PLoS Pathogens. 2013; 9 (7):e1003500. [ PMC free article ] [ PubMed ] [ Google Scholar ]
- Moya A., Holmes E.C., Gonzalez-Candelas F. The population genetics and evolutionary epidemiology of RNA viruses. Nature Reviews Microbiology. 2004; 2 :279–288. [ PMC free article ] [ PubMed ] [ Google Scholar ]
- Page R.D., Holmes E.C. Blackwell Publishing; Boston: 1998. Molecular evolution. A phylogenetic approach. [ Google Scholar ]
- Salemi M, Vandamme AM, editors. The phylogenetic handbook. A practical approach to DNA and protein phylogeny. Cambridge University Press; Cambridge: 2003. [ Google Scholar ]
- Stamatakis A. RAxML version 8: a tool for phylogenetic analysis and post-analysis of large phylogenies. Bioinformatics. 2014; 30 :1312–1313. [ PMC free article ] [ PubMed ] [ Google Scholar ]
- Villarreal L.P. ASM Press; Washington, DC: 2005. Viruses and evolution of life. [ Google Scholar ]
- Weaver S.C., Denison M., Roosinck M., Vignuzzi M., editors. Virus Evolution, Current Research and Future Directions. Academic Press; Caister: 2016. [ Google Scholar ]
20.3 Perspectives on the Phylogenetic Tree
Learning objectives.
- Describe horizontal gene transfer
- Illustrate how prokaryotes and eukaryotes transfer genes horizontally
- Identify the web and ring models of phylogenetic relationships and describe how they differ from the original phylogenetic tree concept
The concepts of phylogenetic modeling are constantly changing. It is one of the most dynamic fields of study in all of biology. Over the last several decades, new research has challenged scientists’ ideas about how organisms are related. New models of these relationships have been proposed for consideration by the scientific community.
Many phylogenetic trees have been shown as models of the evolutionary relationship among species. Phylogenetic trees originated with Charles Darwin, who sketched the first phylogenetic tree in 1837 ( Figure 20.12 a ), which served as a pattern for subsequent studies for more than a century. The concept of a phylogenetic tree with a single trunk representing a common ancestor, with the branches representing the divergence of species from this ancestor, fits well with the structure of many common trees, such as the oak ( Figure 20.12 b ). However, evidence from modern DNA sequence analysis and newly developed computer algorithms has caused skepticism about the validity of the standard tree model in the scientific community.
Limitations to the Classic Model
Classical thinking about prokaryotic evolution, included in the classic tree model, is that species evolve clonally. That is, they produce offspring themselves with only random mutations causing the descent into the variety of modern-day and extinct species known to science. This view is somewhat complicated in eukaryotes that reproduce sexually, but the laws of Mendelian genetics explain the variation in offspring, again, to be a result of a mutation within the species. The concept of genes being transferred between unrelated species was not considered as a possibility until relatively recently. Horizontal gene transfer (HGT), also known as lateral gene transfer, is the transfer of genes between unrelated species. HGT has been shown to be an ever-present phenomenon, with many evolutionists postulating a major role for this process in evolution, thus complicating the simple tree model. Genes have been shown to be passed between species which are only distantly related using standard phylogeny, thus adding a layer of complexity to the understanding of phylogenetic relationships.
The various ways that HGT occurs in prokaryotes is important to understanding phylogenies. Although at present HGT is not viewed as important to eukaryotic evolution, HGT does occur in this domain as well. Finally, as an example of the ultimate gene transfer, theories of genome fusion between symbiotic or endosymbiotic organisms have been proposed to explain an event of great importance—the evolution of the first eukaryotic cell, without which humans could not have come into existence.
Horizontal Gene Transfer
Horizontal gene transfer (HGT) is the introduction of genetic material from one species to another species by mechanisms other than the vertical transmission from parent(s) to offspring. These transfers allow even distantly related species to share genes, influencing their phenotypes. It is thought that HGT is more prevalent in prokaryotes, but that only about 2% of the prokaryotic genome may be transferred by this process. Some researchers believe such estimates are premature: the actual importance of HGT to evolutionary processes must be viewed as a work in progress. As the phenomenon is investigated more thoroughly, it may be revealed to be more common. Many scientists believe that HGT and mutation appear to be (especially in prokaryotes) a significant source of genetic variation, which is the raw material for the process of natural selection. These transfers may occur between any two species that share an intimate relationship ( Table 20.1 ).
Summary of Mechanisms of Prokaryotic and Eukaryotic HGT | |||
---|---|---|---|
Mechanism | Mode of Transmission | Example | |
transformation | DNA uptake | many prokaryotes | |
transduction | bacteriophage (virus) | bacteria | |
conjugation | pilus | many prokaryotes | |
gene transfer agents | phage-like particles | purple non-sulfur bacteria | |
from food organisms | unknown | aphid | |
jumping genes | transposons | rice and millet plants | |
epiphytes/parasites | unknown | yew tree fungi | |
from viral infections |
HGT in Prokaryotes
The mechanism of HGT has been shown to be quite common in the prokaryotic domains of Bacteria and Archaea, significantly changing the way their evolution is viewed. The majority of evolutionary models, such as in the Endosymbiont Theory, propose that eukaryotes descended from multiple prokaryotes, which makes HGT all the more important to understanding the phylogenetic relationships of all extant and extinct species.
The fact that genes are transferred among common bacteria is well known to microbiology students. These gene transfers between species are the major mechanism whereby bacteria acquire resistance to antibiotics. Classically, this type of transfer has been thought to occur by three different mechanisms:
- Transformation: naked DNA is taken up by a bacteria
- Transduction: genes are transferred using a virus
- Conjugation: the use a hollow tube called a pilus to transfer genes between organisms
More recently, a fourth mechanism of gene transfer between prokaryotes has been discovered. Small, virus-like particles called gene transfer agents (GTAs) transfer random genomic segments from one species of prokaryote to another. GTAs have been shown to be responsible for genetic changes, sometimes at a very high frequency compared to other evolutionary processes. The first GTA was characterized in 1974 using purple, non-sulfur bacteria. These GTAs, which are thought to be bacteriophages that lost the ability to reproduce on their own, carry random pieces of DNA from one organism to another. The ability of GTAs to act with high frequency has been demonstrated in controlled studies using marine bacteria. Gene transfer events in marine prokaryotes, either by GTAs or by viruses, have been estimated to be as high as 10 13 per year in the Mediterranean Sea alone. GTAs and viruses are thought to be efficient HGT vehicles with a major impact on prokaryotic evolution.
As a consequence of this modern DNA analysis, the idea that eukaryotes evolved directly from Archaea has fallen out of favor. While eukaryotes share many features that are absent in bacteria, such as the TATA box (found in the promoter region of many genes), the discovery that some eukaryotic genes were more homologous with bacterial DNA than Archaea DNA made this idea less tenable. Furthermore, the fusion of genomes from Archaea and Bacteria by endosymbiosis has been proposed as the ultimate event in eukaryotic evolution.
HGT in Eukaryotes
Although it is easy to see how prokaryotes exchange genetic material by HGT, it was initially thought that this process was absent in eukaryotes. After all, prokaryotes are but single cells exposed directly to their environment, whereas the sex cells of multicellular organisms are usually sequestered in protected parts of the body. It follows from this idea that the gene transfers between multicellular eukaryotes should be more difficult. Indeed, it is thought that this process is rarer in eukaryotes and has a much smaller evolutionary impact than in prokaryotes. In spite of this fact, HGT between distantly related organisms has been demonstrated in several eukaryotic species, and it is possible that more examples will be discovered in the future.
In plants, gene transfer has been observed in species that cannot cross-pollinate by normal means. Transposons or “jumping genes” have been shown to transfer between rice and millet plant species. Furthermore, fungal species feeding on yew trees, from which the anti-cancer drug TAXOL® is derived from the bark, have acquired the ability to make taxol themselves, a clear example of gene transfer.
In animals, a particularly interesting example of HGT occurs within the aphid species ( Figure 20.13 ). Aphids are insects that vary in color based on carotenoid content. Carotenoids are pigments made by a variety of plants, fungi, and microbes, and they serve a variety of functions in animals, who obtain these chemicals from their food. Humans require carotenoids to synthesize vitamin A, and we obtain them by eating orange fruits and vegetables: carrots, apricots, mangoes, and sweet potatoes. On the other hand, aphids have acquired the ability to make the carotenoids on their own. According to DNA analysis, this ability is due to the transfer of fungal genes into the insect by HGT, presumably as the insect consumed fungi for food. A carotenoid enzyme called a desaturase is responsible for the red coloration seen in certain aphids, and it has been further shown that when this gene is inactivated by mutation, the aphids revert back to their more common green color ( Figure 20.13 ).
Genome Fusion and the Evolution of Eukaryotes
Scientists believe the ultimate in HGT occurs through genome fusion between different species of prokaryotes when two symbiotic organisms become endosymbiotic. This occurs when one species is taken inside the cytoplasm of another species, which ultimately results in a genome consisting of genes from both the endosymbiont and the host. This mechanism is an aspect of the Endosymbiont Theory, which is accepted by a majority of biologists as the mechanism whereby eukaryotic cells obtained their mitochondria and chloroplasts. However, the role of endosymbiosis in the development of the nucleus is more controversial. Nuclear and mitochondrial DNA are thought to be of different (separate) evolutionary origin, with the mitochondrial DNA being derived from the circular genomes of bacteria that were engulfed by ancient prokaryotic cells. Mitochondrial DNA can be regarded as the smallest chromosome. Interestingly enough, mitochondrial DNA is inherited only from the mother. The mitochondrial DNA degrades in sperm when the sperm degrades in the fertilized egg or in other instances when the mitochondria located in the flagellum of the sperm fails to enter the egg.
Within the past decade, the process of genome fusion by endosymbiosis has been proposed by James Lake of the UCLA/NASA Astrobiology Institute to be responsible for the evolution of the first eukaryotic cells ( Figure 20.14 a ). Using DNA analysis and a new mathematical algorithm called conditioned reconstruction (CR), his laboratory proposed that eukaryotic cells developed from an endosymbiotic gene fusion between two species, one an Archaea and the other a Bacteria. As mentioned, some eukaryotic genes resemble those of Archaea, whereas others resemble those from Bacteria. An endosymbiotic fusion event, such as Lake has proposed, would clearly explain this observation. On the other hand, this work is new and the CR algorithm is relatively unsubstantiated, which causes many scientists to resist this hypothesis.
More recent work by Lake ( Figure 20.14 b ) proposes that gram-negative bacteria, which are unique within their domain in that they contain two lipid bilayer membranes, indeed resulted from an endosymbiotic fusion of archaeal and bacterial species. The double membrane would be a direct result of the endosymbiosis, with the endosymbiont picking up the second membrane from the host as it was internalized. This mechanism has also been used to explain the double membranes found in mitochondria and chloroplasts. Lake’s work is not without skepticism, and the ideas are still debated within the biological science community. In addition to Lake’s hypothesis, there are several other competing theories as to the origin of eukaryotes. How did the eukaryotic nucleus evolve? One theory is that the prokaryotic cells produced an additional membrane that surrounded the bacterial chromosome. Some bacteria have the DNA enclosed by two membranes; however, there is no evidence of a nucleolus or nuclear pores. Other proteobacteria also have membrane-bound chromosomes. If the eukaryotic nucleus evolved this way, we would expect one of the two types of prokaryotes to be more closely related to eukaryotes.
The nucleus-first hypothesis proposes that the nucleus evolved in prokaryotes first ( Figure 20.15 a ), followed by a later fusion of the new eukaryote with bacteria that became mitochondria. The mitochondria-first hypothesis proposes that mitochondria were first established in a prokaryotic host ( Figure 20.15 b ), which subsequently acquired a nucleus, by fusion or other mechanisms, to become the first eukaryotic cell. Most interestingly, the eukaryote-first hypothesis proposes that prokaryotes actually evolved from eukaryotes by losing genes and complexity ( Figure 20.15 c ). All of these hypotheses are testable. Only time and more experimentation will determine which hypothesis is best supported by data.
Web and Network Models
The recognition of the importance of HGT, especially in the evolution of prokaryotes, has caused some to propose abandoning the classic “tree of life” model. In 1999, W. Ford Doolittle proposed a phylogenetic model that resembles a web or a network more than a tree. The hypothesis is that eukaryotes evolved not from a single prokaryotic ancestor, but from a pool of many species that were sharing genes by HGT mechanisms. As shown in Figure 20.16 a , some individual prokaryotes were responsible for transferring the bacteria that caused mitochondrial development to the new eukaryotes, whereas other species transferred the bacteria that gave rise to chloroplasts. This model is often called the “ web of life .” In an effort to save the tree analogy, some have proposed using the Ficus tree ( Figure 20.16 b ) with its multiple trunks as a phylogenetic to represent a diminished evolutionary role for HGT.
Ring of Life Models
Others have proposed abandoning any tree-like model of phylogeny in favor of a ring structure, the so-called “ ring of life ” ( Figure 20.17 ); a phylogenetic model where all three domains of life evolved from a pool of primitive prokaryotes. Lake, again using the conditioned reconstruction algorithm, proposes a ring-like model in which species of all three domains—Archaea, Bacteria, and Eukarya—evolved from a single pool of gene-swapping prokaryotes. His laboratory proposes that this structure is the best fit for data from extensive DNA analyses performed in his laboratory, and that the ring model is the only one that adequately takes HGT and genomic fusion into account. However, other phylogeneticists remain highly skeptical of this model.
In summary, the “tree of life” model proposed by Darwin must be modified to include HGT. Does this mean abandoning the tree model completely? Even Lake argues that all attempts should be made to discover some modification of the tree model to allow it to accurately fit his data, and only the inability to do so will sway people toward his ring proposal.
This doesn’t mean a tree, web, or a ring will correlate completely to an accurate description of phylogenetic relationships of life. A consequence of the new thinking about phylogenetic models is the idea that Darwin’s original conception of the phylogenetic tree is too simple, but made sense based on what was known at the time. However, the search for a more useful model moves on: each model serving as hypotheses to be tested with the possibility of developing new models. This is how science advances. These models are used as visualizations to help construct hypothetical evolutionary relationships and understand the massive amount of data being analyzed.
As an Amazon Associate we earn from qualifying purchases.
This book may not be used in the training of large language models or otherwise be ingested into large language models or generative AI offerings without OpenStax's permission.
Want to cite, share, or modify this book? This book uses the Creative Commons Attribution License and you must attribute OpenStax.
Access for free at https://openstax.org/books/biology/pages/1-introduction
- Authors: Connie Rye, Robert Wise, Vladimir Jurukovski, Jean DeSaix, Jung Choi, Yael Avissar
- Publisher/website: OpenStax
- Book title: Biology
- Publication date: Oct 21, 2016
- Location: Houston, Texas
- Book URL: https://openstax.org/books/biology/pages/1-introduction
- Section URL: https://openstax.org/books/biology/pages/20-3-perspectives-on-the-phylogenetic-tree
© Feb 14, 2022 OpenStax. Textbook content produced by OpenStax is licensed under a Creative Commons Attribution License . The OpenStax name, OpenStax logo, OpenStax book covers, OpenStax CNX name, and OpenStax CNX logo are not subject to the Creative Commons license and may not be reproduced without the prior and express written consent of Rice University.
- Search Menu
- Sign in through your institution
- Advance Articles
- Virtual Issues
- High-Impact Research Collection
- Celebrate 40 years of MBE
- Perspectives
- Discoveries
- Cover Archive
- Brief Communications
- Submission site
- Author guidelines
- Open access
- Self-archiving policy
- Reasons to submit
- About Molecular Biology and Evolution
- About the Society for Molecular Biology and Evolution
- Editorial Board
- Advertising and Corporate Services
- Journals Career Network
- Journals on Oxford Academic
- Books on Oxford Academic
Article Contents
Phylobarcoder: a web tool for phylogenetic classification of eukaryote metabarcodes using custom reference databases.

- Article contents
- Figures & tables
- Supplementary Data
Jun Inoue, Chuya Shinzato, Junya Hirai, Sachihiko Itoh, Yuki Minegishi, Shin-ichi Ito, Susumu Hyodo, phyloBARCODER: A web tool for phylogenetic classification of eukaryote metabarcodes using custom reference databases, Molecular Biology and Evolution , 2024;, msae111, https://doi.org/10.1093/molbev/msae111
- Permissions Icon Permissions
We developed phyloBARCODER ( https://github.com/jun-inoue/phyloBARCODER ), a new web tool that can identify short DNA sequences to the species level using metabarcoding. phyloBARCODER estimates phylogenetic trees based on uploaded anonymous DNA sequences and reference sequences from databases. Without such phylogenetic contexts, alternative, similarity-based methods independently identify species names and anonymous sequences of the same group by pairwise comparisons between queries and database sequences, with the caveat that they must match exactly or very closely. By putting metabarcoding sequences into a phylogenetic context, phyloBARCODER accurately identifies (1) species or classification of query sequences and (2) anonymous sequences associated with the same species or even with populations of query sequences, with clear and accurate explanations. Version 1 of phyloBARCODER stores a database comprising all eukaryotic mitochondrial gene sequences. Moreover, by uploading their own databases, phyloBARCODER users can conduct species identification specialized for sequences obtained from a local geographic region or those of non-mitochondrial genes, e.g., ITS or rbcL.
Supplementary data
Email alerts, citing articles via.
- Author Guidelines
Affiliations
- Online ISSN 1537-1719
- Copyright © 2024 Society for Molecular Biology and Evolution
- About Oxford Academic
- Publish journals with us
- University press partners
- What we publish
- New features
- Institutional account management
- Rights and permissions
- Get help with access
- Accessibility
- Advertising
- Media enquiries
- Oxford University Press
- Oxford Languages
- University of Oxford
Oxford University Press is a department of the University of Oxford. It furthers the University's objective of excellence in research, scholarship, and education by publishing worldwide
- Copyright © 2024 Oxford University Press
- Cookie settings
- Cookie policy
- Privacy policy
- Legal notice
This Feature Is Available To Subscribers Only
Sign In or Create an Account
This PDF is available to Subscribers Only
For full access to this pdf, sign in to an existing account, or purchase an annual subscription.
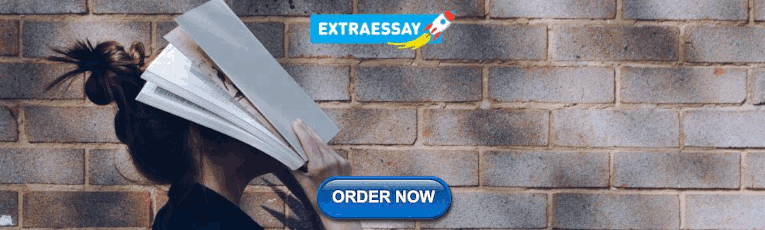
IMAGES
VIDEO
COMMENTS
A phylogenetic tree is a diagram that represents evolutionary relationships among organisms. Phylogenetic trees are hypotheses, not definitive facts. The pattern of branching in a phylogenetic tree reflects how species or other groups evolved from a series of common ancestors. In trees, two species are more related if they have a more recent ...
A phylogenetic tree is a diagram used to reflect evolutionary relationships among organisms or groups of organisms. Scientists consider phylogenetic trees to be a hypothesis of the evolutionary past since one cannot go back to confirm the proposed relationships. In other words, a "tree of life" can be constructed to illustrate when ...
A phylogenetic tree may be built using morphological (body shape), biochemical, behavioral, or molecular features of species or other groups. In building a tree, we organize species into nested groups based on shared derived traits (traits different from those of the group's ancestor). The sequences of genes or proteins can be compared among ...
In biology, phylogenetics (/ ˌ f aɪ l oʊ dʒ ə ˈ n ɛ t ɪ k s,-l ə-/) is the study of the evolutionary history and relationships among or within groups of organisms.These relationships are determined by phylogenetic inference, methods that focus on observed heritable traits, such as DNA sequences, protein amino acid sequences, or morphology.The result of such an analysis is a ...
A phylogenetic tree, phylogeny or evolutionary tree is a graphical representation which shows the evolutionary history between a set of species or taxa during a specific time. In other words, it is a branching diagram or a tree showing the evolutionary relationships among various biological species or other entities based upon similarities and differences in their physical or genetic ...
A phylogenetic tree is a visual representation of the relationship between different organisms, showing the path through evolutionary time from a common ancestor to different descendants. Trees can represent relationships ranging from the entire history of life on earth, down to individuals in a population. The diagram below shows a tree of 3 ...
Phylogenetic trees originated with Charles Darwin, who sketched the first phylogenetic tree in 1837 (Figure 20.12a). This served as a prototype for subsequent studies for more than a century. The phylogenetic tree concept with a single trunk representing a shared ancestry, with the branches representing the divergence of species from this ...
Knowing phylogenetic relationships among species is fundamental for many studies in biology. An accurate phylogenetic tree underpins our understanding of the major transitions in evolution, such ...
1.B.1 Organisms share many conserved core processes and features that evolved and are widely distributed among organisms today. Science Practice. 7.2 The student can connect concepts in and across domain (s) to generalize or extrapolate in and/or across enduring understandings and/or big ideas. Learning Objective.
Family trees tend to be drawn as if they were hanging upside down, like a cluster of grapes. Phylogenetic trees are depicted somewhat differently. Imagine that you are holding the family tree for the big cats shown in Fig. 2a. Now, flip it sideways (rotate 90° counterclockwise) and you have the image shown in 2b.
Clearly, evolutionary trees convey a lot of information about a group's evolutionary history. Biologists are taking advantage of this by using a system of phylogenetic classification, which conveys the same sort of information that is conveyed by trees.In contrast to the traditional Linnaean system of classification, phylogenetic classification names only clades.
Learning Objectives. The phylogenetic tree, first used by Darwin, is the classic "tree of life" model describing phylogenetic relationships among species, and the most common model used today. New ideas about HGT and genome fusion have caused some to suggest revising the model to resemble webs or rings.
The Tree of Life is the result of the interplay of changes in information and speciation. Almost 100 years after publication of Darwin's Origin, the inception of Phylogenetic Systematics has resulted in a revolution in data inference. I briefly trace the development of this revolution and show examples of how data are interpreted relative to phylogenetic trees.
Modern phylogenetic trees reflect a commitment to hierarchical classification that precedes evolutionary thought. Centuries before On the Origin of Species, taxonomic trees grouped organisms into hierarchical, non-overlapping sets (species nested within genera, genera within families, families within orders, and so on).Darwin's insight was that these categories corresponded to, and were thus ...
A phylogenetic tree, also known as a cladogram, tree of life, or evolutionary tree, is a graphical representation resembling a tree that illustrates the evolutionary and phylogenetic relationships between biological taxa based on their physical or genetic characteristics [1,2,3].Comprising nodes and branches, a phylogenetic tree uses nodes to stand for taxonomic units and branches to depict ...
Our phylogenetic tree includes 58% of the approximately 13,600 currently accepted genera of angiosperms (Fig. 1 and Supplementary Table 1; ref. 2). Together, the 7,923 genera encompass 85.7% of ...
Phylogenetics is important because it enriches our understanding of how genes, genomes, species (and molecular sequences more generally) evolve. Through phylogenetics, we learn not only how the sequences came to be the way they are today, but also general principles that enable us to predict how they will change in the future.
Binary data ("0"/"1") from RAPD, RFLP, AFLP, SSCP, and sequence data (DNA or protein) from the set of objects are used to construct phylogenetic tree. The different tree construction methods are UPGMA, NJ, ME, FM, MP, and ML. Molecular phylogeny has a wide range of applications and if the interpretation of the evolutionary patterns is ...
give the best result and the most informative tree. Rooting trees: Root is the common ancestor of the species under study. Most phylogenetic methods do not locate the root of a tree and the unrooted trees only reflect the relationship among species but not the evolutionary path. Fig5 (a) shows an unrooted tree of species A, B, C and D.
Introduction: Phylogenetic tree also known as evolutionary tree depicts the connection between various species of animals and plants based on their physical and genetic traits. Top points of the trees symbolize descendent common species whereas nodes symbolize common ancestor of descendents. Phylogenetic trees very effectively show the ...
This zoomed-in phylogenetic tree also highlights the recent clonal derivation of B. mallei from B. pseudomallei, with B. pseudomallei 576 as the most closely related sequenced ancestor and ...
A separate phylogenetic analysis was conducted for each of the six data partitions to compare the concordance among tree topologies derived from each partition. It should be noted that the Y-chromosome tree is not directly comparable to the topologies of the other data partitions because the number of males (N = 127) was a subset of the total ...
Phylogenetic tree optimization is necessary for precise analysis of evolutionary and transmission dynamics, but existing tools are inadequate for handling the scale and pace of data produced during the COVID-19 pandemic. One transformative approach, online phylogenetics, aims to incrementally add samples to an ever-growing phylogeny, but there ...
Phylogenetic tree and molecular evolution. Shown is a toy example of evolution of an ancestral sequence (to the right of the black-filled circle) of length four into five extant sequences (to the right of the open circles) and the corresponding phylogenetic tree. The respective substitutions and sequence positions are indicated at the tree ...
The concept of a phylogenetic tree with a single trunk representing a common ancestor, with the branches representing the divergence of species from this ancestor, fits well with the structure of many common trees, such as the oak (Figure 20.12b). However, evidence from modern DNA sequence analysis and newly developed computer algorithms has ...
By putting metabarcoding sequences into a phylogenetic context, phyloBARCODER accurately identifies (1) species or classification of query sequences and (2) anonymous sequences associated with the same species or even with populations of query sequences, with clear and accurate explanations.
The ML phylogenetic tree based on protein-coding genes in the chloroplast genomes suggested distinguishing between P. kingianum var. grandifolium and P. kingianum. The chloroplast genomes of the three herbs will provide information for identification and classification of the species that can be used to explore the evolution of the genus ...