- Browse All Articles
- Newsletter Sign-Up
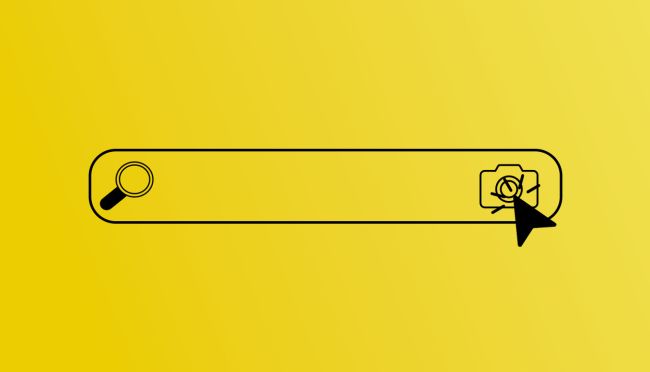
- 13 May 2024
- Research & Ideas
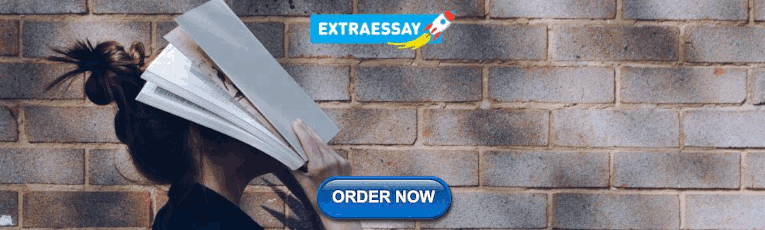
Picture This: Why Online Image Searches Drive Purchases
Smaller sellers' products often get lost on large online marketplaces. However, harnessing images in search can help consumers find these products faster, increasing sales and customer satisfaction, finds research by Chiara Farronato and colleagues.
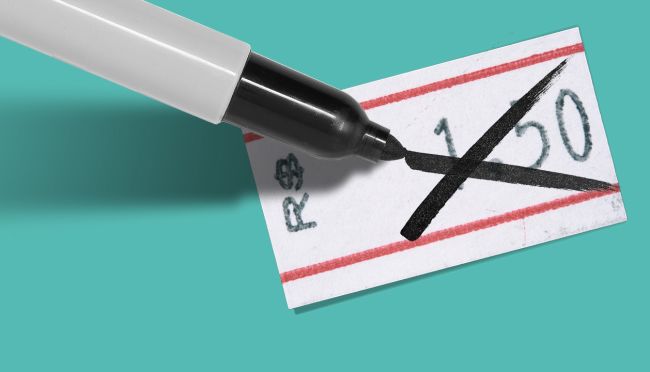
- 01 Apr 2024
- In Practice
Navigating the Mood of Customers Weary of Price Hikes
Price increases might be tempering after historic surges, but companies continue to wrestle with pinched consumers. Alexander MacKay, Chiara Farronato, and Emily Williams make sense of the economic whiplash of inflation and offer insights for business leaders trying to find equilibrium.
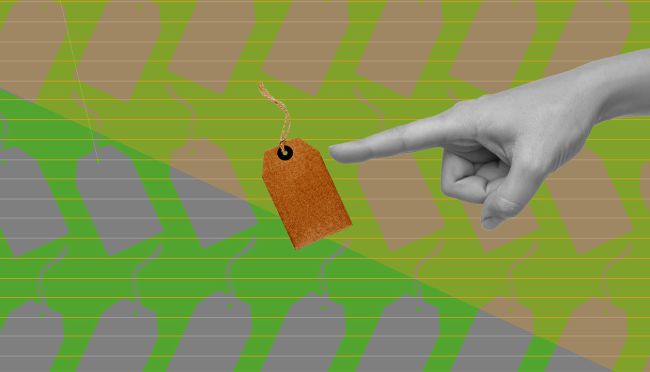
- 17 Jan 2024
Psychological Pricing Tactics to Fight the Inflation Blues
Inflation has slowed from the epic rates of 2021 and 2022, but many consumers still feel pinched. What will it take to encourage them to spend? Thoughtful pricing strategies that empower customers as they make purchasing decisions, says research by Elie Ofek.
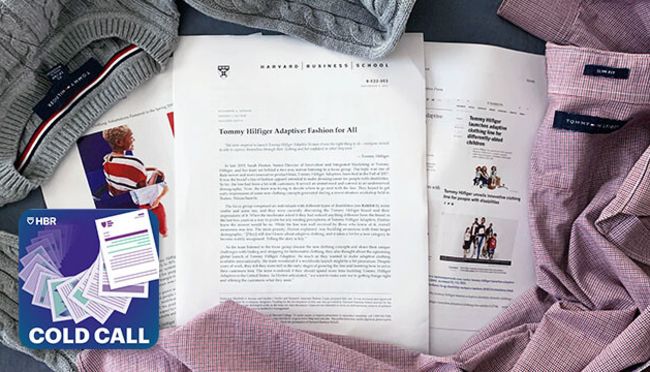
- 05 Dec 2023
- Cold Call Podcast
Tommy Hilfiger’s Adaptive Clothing Line: Making Fashion Inclusive
In 2017, Tommy Hilfiger launched its adaptive fashion line to provide fashion apparel that aims to make dressing easier. By 2020, it was still a relatively unknown line in the U.S. and the Tommy Hilfiger team was continuing to learn more about how to serve these new customers. Should the team make adaptive clothing available beyond the U.S., or is a global expansion premature? Assistant Professor Elizabeth Keenan discusses the opportunities and challenges that accompanied the introduction of a new product line that effectively serves an entirely new customer while simultaneously starting a movement to provide fashion for all in the case, “Tommy Hilfiger Adaptive: Fashion for All.”
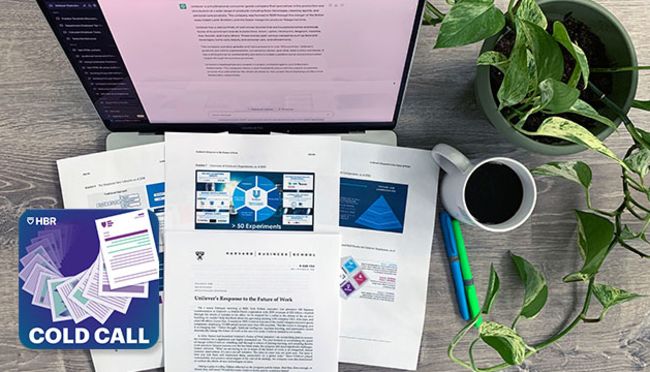
- 05 Jul 2023
How Unilever Is Preparing for the Future of Work
Launched in 2016, Unilever’s Future of Work initiative aimed to accelerate the speed of change throughout the organization and prepare its workforce for a digitalized and highly automated era. But despite its success over the last three years, the program still faces significant challenges in its implementation. How should Unilever, one of the world's largest consumer goods companies, best prepare and upscale its workforce for the future? How should Unilever adapt and accelerate the speed of change throughout the organization? Is it even possible to lead a systematic, agile workforce transformation across several geographies while accounting for local context? Harvard Business School professor and faculty co-chair of the Managing the Future of Work Project William Kerr and Patrick Hull, Unilever’s vice president of global learning and future of work, discuss how rapid advances in artificial intelligence, machine learning, and automation are changing the nature of work in the case, “Unilever's Response to the Future of Work.”
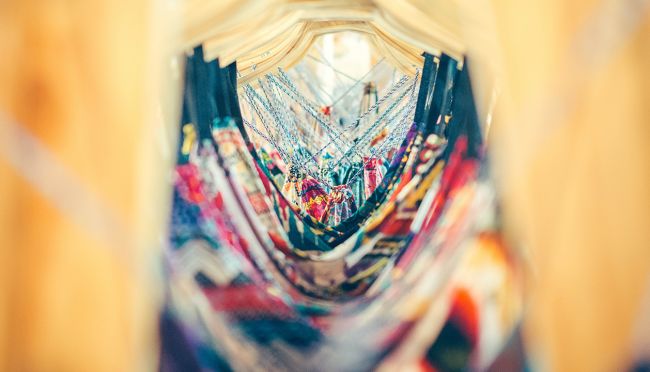
- 25 Apr 2023
How SHEIN and Temu Conquered Fast Fashion—and Forged a New Business Model
The platforms SHEIN and Temu match consumer demand and factory output, bringing Chinese production to the rest of the world. The companies have remade fast fashion, but their pioneering approach has the potential to go far beyond retail, says John Deighton.
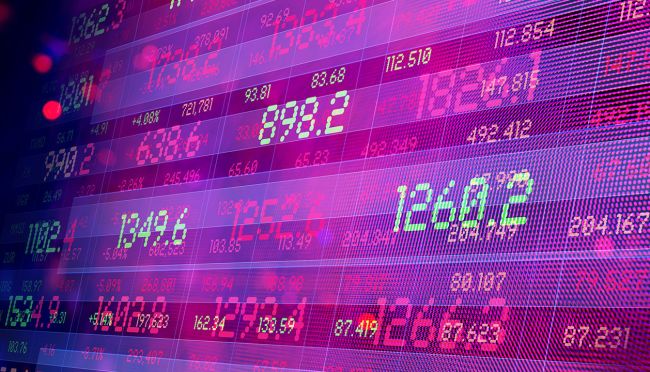
- 11 Apr 2023
Is Amazon a Retailer, a Tech Firm, or a Media Company? How AI Can Help Investors Decide
More companies are bringing seemingly unrelated businesses together in new ways, challenging traditional stock categories. MarcAntonio Awada and Suraj Srinivasan discuss how applying machine learning to regulatory data could reveal new opportunities for investors.
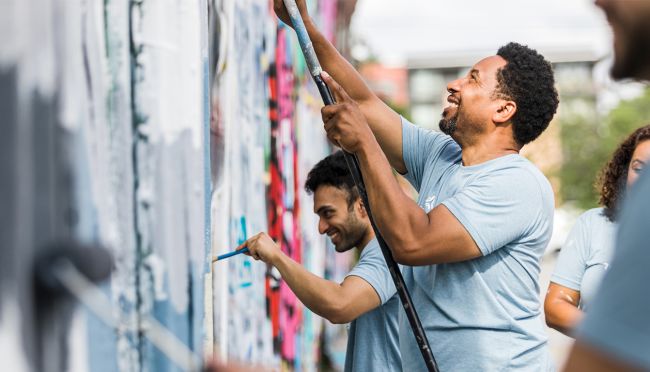
- 04 Apr 2023
Two Centuries of Business Leaders Who Took a Stand on Social Issues
Executives going back to George Cadbury and J. N. Tata have been trying to improve life for their workers and communities, according to the book Deeply Responsible Business: A Global History of Values-Driven Leadership by Geoffrey Jones. He highlights three practices that deeply responsible companies share.
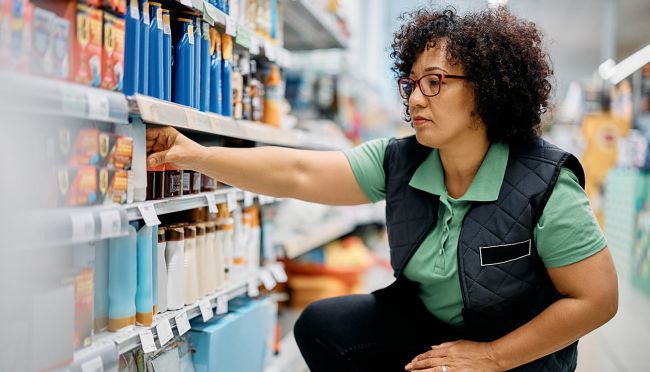
- 03 Mar 2023
When Showing Know-How Backfires for Women Managers
Women managers might think they need to roll up their sleeves and work alongside their teams to show their mettle. But research by Alexandra Feldberg shows how this strategy can work against them. How can employers provide more support?
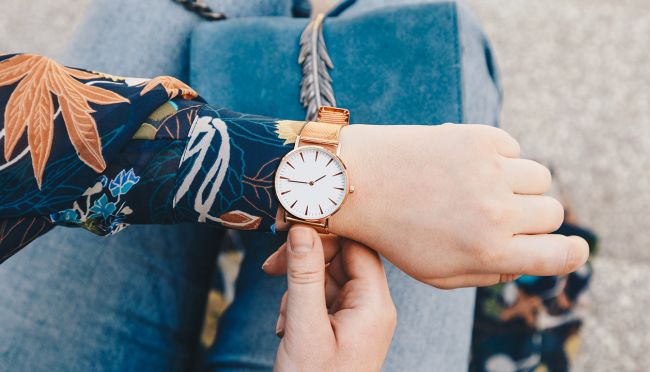
- 27 Feb 2023
How One Late Employee Can Hurt Your Business: Data from 25 Million Timecards
Employees who clock in a few minutes late—or not at all—often dampen sales and productivity, says a study of 100,000 workers by Ananth Raman and Caleb Kwon. What can managers do to address chronic tardiness and absenteeism?
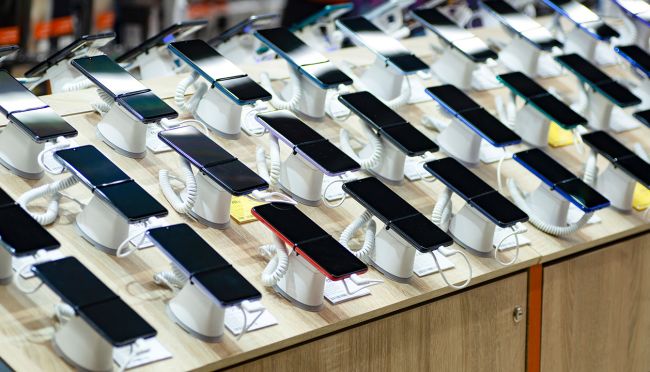
- 06 Dec 2022
Latest Isn’t Always Greatest: Why Product Updates Capture Consumers
Consumers can't pass up a product update—even if there's no improvement. Research by Leslie John, Michael Norton, and Ximena Garcia-Rada illustrates the powerful allure of change. Are we really that naïve?
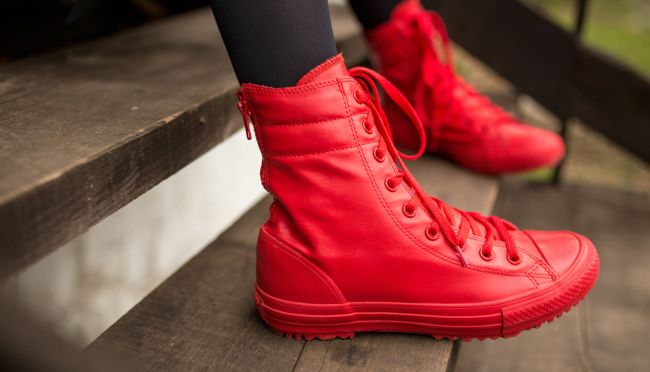
- 29 Nov 2022
How Much More Would Holiday Shoppers Pay to Wear Something Rare?
Economic worries will make pricing strategy even more critical this holiday season. Research by Chiara Farronato reveals the value that hip consumers see in hard-to-find products. Are companies simply making too many goods?
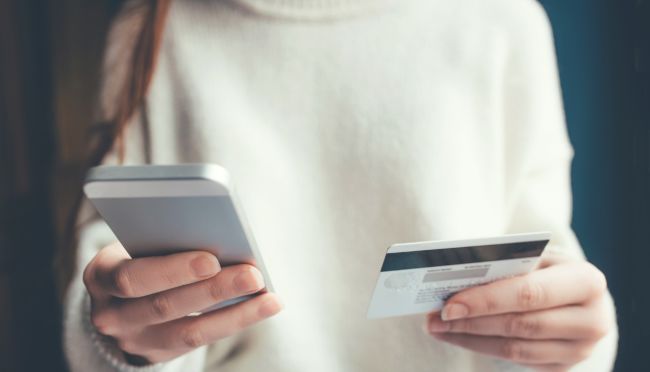
- 21 Nov 2022
Buy Now, Pay Later: How Retail's Hot Feature Hurts Low-Income Shoppers
More consumers may opt to "buy now, pay later" this holiday season, but what happens if they can't make that last payment? Research by Marco Di Maggio and Emily Williams highlights the risks of these financing services, especially for lower-income shoppers.
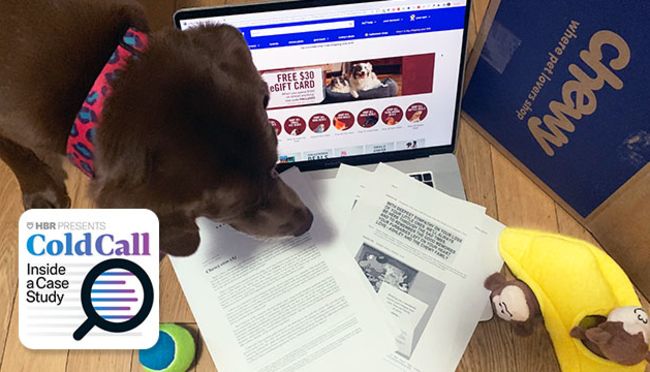
- 18 Oct 2022
Chewy.com’s Make-or-Break Logistics Dilemma
In late 2013, Ryan Cohen, cofounder and then-CEO of online pet products retailer Chewy.com, was facing a decision that could determine his company’s future. Should he stay with a third-party logistics provider (3PL) for all of Chewy.com’s e-commerce fulfillment or take that function in house? Cohen was convinced that achieving scale would be essential to making the business work and he worried that the company’s current 3PL may not be able to scale with Chewy.com’s projected growth or maintain the company’s performance standards for service quality and fulfillment. But neither he nor his cofounders had any experience managing logistics, and the company’s board members were pressuring him to leave order fulfillment to the 3PL. They worried that any changes could destabilize the existing 3PL relationship and endanger the viability of the fast-growing business. What should Cohen do? Senior Lecturer Jeffrey Rayport discusses the options in his case, “Chewy.com (A).”
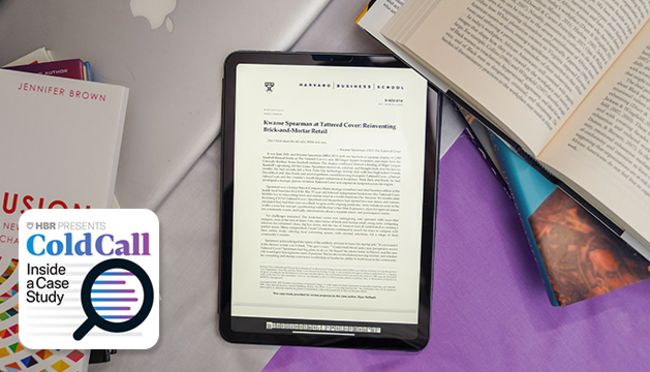
- 06 Sep 2022
Reinventing an Iconic Independent Bookstore
In 2020, Kwame Spearman (MBA 2011) made the career-shifting decision to leave a New York City-based consulting job to return to his hometown of Denver, Colorado, and take over an iconic independent bookstore, The Tattered Cover. Spearman saw an opportunity to reinvent the local business to build a sense of community after the pandemic. But he also had to find a way to meet the big challenges facing independent booksellers amid technological change and shifting business models. Professor Ryan Raffaelli and Spearman discuss Spearman’s vision for reinventing The Tattered Cover, as well as larger insights around how local businesses can successfully compete with online and big box retailers in the case, “Kwame Spearman at Tattered Cover: Reinventing Brick-and-Mortar Retail.”
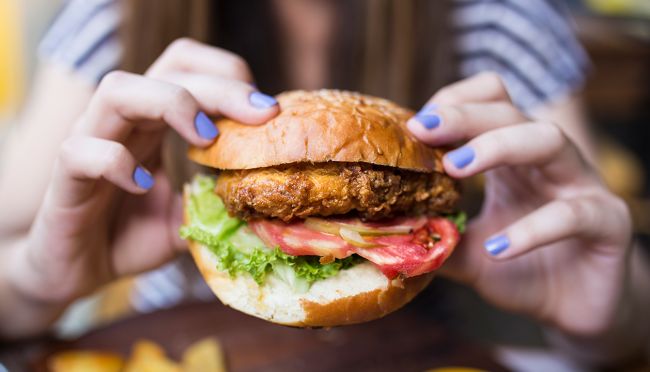
- 26 Jul 2022
Burgers with Bugs? What Happens When Restaurants Ignore Online Reviews
Negative Yelp reviews hold more sway with consumers than restaurateurs might think. A machine learning study by Chiara Farronato reveals how online platforms amplify the customer voice, and why business owners should listen.
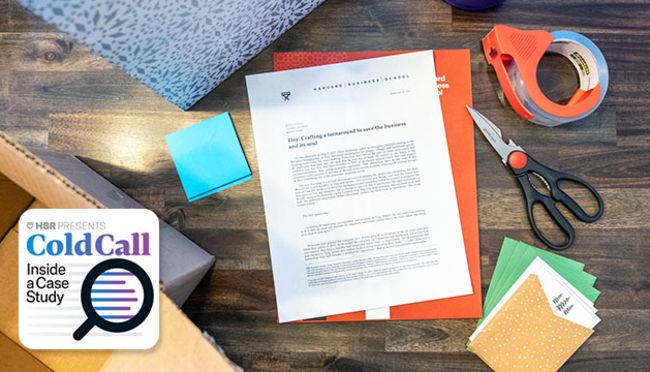
- 22 Mar 2022
How Etsy Found Its Purpose and Crafted a Turnaround
Etsy, the online seller of handmade goods, was founded in 2005 as an alternative to companies that sold mass-manufactured products. The company grew substantially, but remained unprofitable under the leadership of two early CEOs. Ten years later, Etsy went public and was forced into a new arena, where it was beholden to stakeholders who demanded financial success and accountability. Unable to contain costs, the company was almost bought out by private equity firms in 2017—until CEO Josh Silverman arrived with a mission to save the company financially and, in the process, save its soul. Harvard Business School professor Ranjay Gulati discusses the purpose-driven turnaround Silverman and his team led at Etsy—to make the company profitable and improve its social and environmental impact—in the case, “Etsy: Crafting a Turnaround to Save the Business and Its Soul.” Open for comment; 0 Comments.
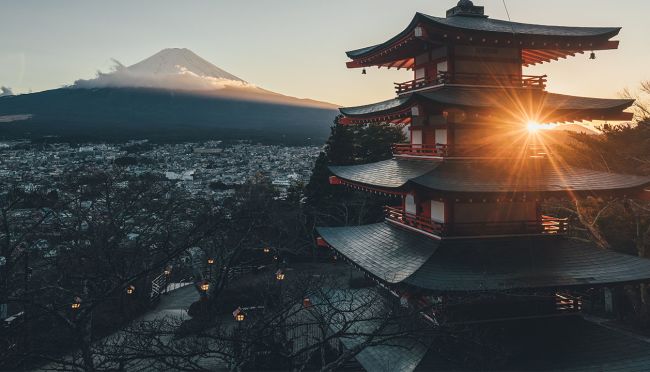
- 05 Nov 2021
Is the Business World Finally Ready for the Wisdom of Shibusawa?
Legendary financier Eiichi Shibusawa advocated for business prosperity that would also benefit society. One hundred years after his death, his message is resonating with a new generation of leaders, say Geoffrey Jones and Rei Morimoto. Open for comment; 0 Comments.
- 19 Oct 2021
Fed Up Workers and Supply Woes: What's Next for Dollar Stores?
Willy Shih discusses how higher costs, shipping delays, and worker shortages are putting the dollar store business model to the test ahead of the critical holiday shopping season. Open for comment; 0 Comments.
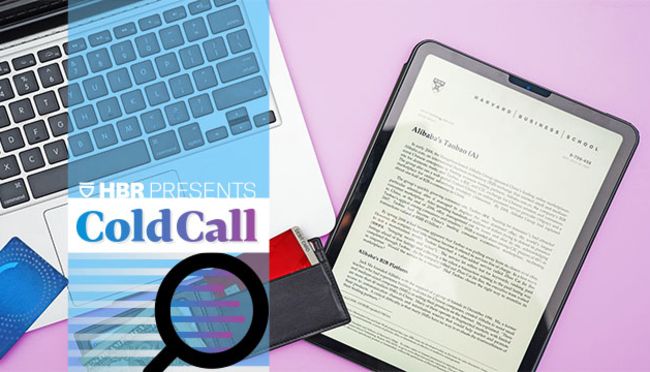
- 13 Jul 2021
Strategies for Underdogs: How Alibaba’s Taobao Beat eBay in China
In 2007, Alibaba’s Taobao became China’s leading consumer e-commerce marketplace, displacing the once dominant eBay. How did underdog Taobao do it? And will it be able to find a way to monetize its marketplace and ensure future success? Professor Felix Oberholzer-Gee discusses his case, “Alibaba’s Taobao,” and related strategy lessons from his new book, Better, Simpler Strategy: A Value-Based Guide to Exceptional Performance. Open for comment; 0 Comments.
Retail Insights
The state of grocery 2024, featured insights.

The State of Fashion 2024: Finding pockets of growth as uncertainty reigns
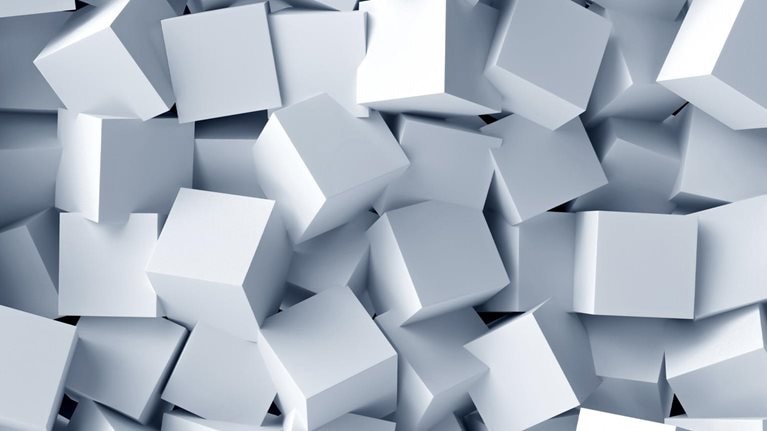
Why courting aspirational luxury consumers still matters
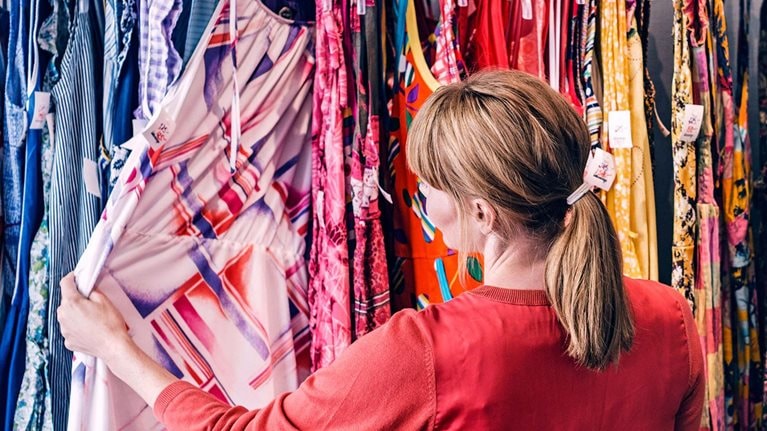
Sustainable style: How fashion can afford and accelerate decarbonization

Author Talks: Philip Kotler and Giuseppe Stigliano on retail’s next chapter

Luxury retail is about ‘emotions, not transactions’

McKinsey on Consumer and Retail Podcast: Insights from experts on business and management
Want to learn more about how we help clients in retail, more insights, related practice.
- Consumer Packaged Goods
Connect with our Retail Practice
Forecasting e-commerce consumer returns: a systematic literature review
- Open access
- Published: 21 May 2024
Cite this article
You have full access to this open access article
- David Karl ORCID: orcid.org/0000-0002-0326-5982 1
307 Accesses
Explore all metrics
The substantial growth of e-commerce during the last years has led to a surge in consumer returns. Recently, research interest in consumer returns has grown steadily. The availability of vast customer data and advancements in machine learning opened up new avenues for returns forecasting. However, existing reviews predominantly took a broader perspective, focussing on reverse logistics and closed-loop supply chain management aspects. This paper addresses this gap by reviewing the state of research on returns forecasting in the realms of e-commerce. Methodologically, a systematic literature review was conducted, analyzing 25 relevant publications regarding methodology, required or employed data, significant predictors, and forecasting techniques, classifying them into several publication streams according to the papers’ main scope. Besides extending a taxonomy for machine learning in e-commerce, this review outlines avenues for future research. This comprehensive literature review contributes to several disciplines, from information systems to operations management and marketing research, and is the first to explore returns forecasting issues specifically from the e-commerce perspective.
Similar content being viewed by others
An E-Commerce Prototype for Predicting the Product Return Phenomenon Using Optimization and Regression Techniques
Extreme Learning Machine for Business Sales Forecasts: A Systematic Review
Demand Forecasting Methods and the Potential of Machine Learning in the FMCG Retail Industry
Avoid common mistakes on your manuscript.
1 Introduction
E-commerce has witnessed substantial growth rates in recent years and continues growing by double-digit margins (National Retail Federation/Appriss Retail 2023 ). However, lenient consumer return policies have resulted in $212 Billion worth of merchandise being returned to online retailers in the U.S. in 2022, accounting for 16.5% of online sales (National Retail Federation/Appriss Retail 2023 ). While high rates of consumer returns mainly concern specific sectors and product categories, online fashion retailing is particularly affected (Diggins et al. 2016 ). Recent studies report average shipment-related return rates for fashion retailers in the 40–50% range (Difrancesco et al. 2018 ; Karl and Asdecker 2021 ). In addition to missed sales and reduced profits (Zhao et al. 2020 ), consumer returns pose operational challenges (Stock and Mulki 2009 ), including unavoidable processing costs (Asdecker 2015 ) and uncertainties regarding logistics capacities, inventory management, procurement decisions, and marketing activities. Hence, effectively managing consumer returns is an essential part of the e-commerce business model (Urbanke et al. 2015 ).
Similar to the research conducted by Abdulla et al. ( 2019 ), this work focuses on consumer returns in online retailing (e-commerce), excluding the larger body of closed-loop supply chain (CLSC) management, which encompasses product returns related to end-of-life and end-of-use scenarios involving raw material recycling or remanufacturing. In contrast to CLSC returns, retail consumer returns are typically sent or given back unused or undamaged shortly after purchase, without any quality-related defects. These returns should be reimbursed to the consumer and are intended to be resold “as new” (de Brito et al. 2005 ; Melacini et al. 2018 ; Shang et al. 2020 ).
Regarding forecasting aspects, demand forecasting is a crucial activity for successful retail management (Ge et al. 2019 ). In contrast to demand and sales, returns constitute the “supply” side of the return process (Frei et al. 2022 ). Consequently, forecasting becomes a complex task and a significant challenge in managing returns due to the inherently uncertain nature of customer decisions regarding product retention (Frei et al. 2022 ). Moreover, return forecasts are interconnected with sales forecasts and promotional activities (Govindan and Bouzon 2018 ; Tibben-Lembke and Rogers 2002 ). Hence, forecasting objectives may vary, encompassing return quantities, timing (Hachimi et al. 2018 ), and even individual return probabilities. Minimizing return forecast errors is critical to reduce and minimize reactive planning (Hess and Mayhew 1997 ). Accurate forecasts rely on (1) comprehensive data collection, e.g., regarding consumer behavior, and (2) information and communications technology (ICT) for data processing, such as big data analytics. Despite extensive research in supply chain management (SCM), Barbosa et al. ( 2018 ) noted a lack of relevant publications exploring the "returns management" process of SCM in conjunction with big data analytics. Specifically, “the topic of forecasting consumer returns has received little attention in the academic literature” (Shang et al. 2020 ). Nonetheless, precise return forecasts positively impact reverse logistics activities’ economic, environmental, and social performance, primarily concerning quantity, quality, and timing predictions (Agrawal and Singh 2020 ). Hence, forecasting returns holds significant relevance across various supply chain stages.
1.1 Previous meta-research
Hess and Mayhew ( 1997 ) emphasized the need for extensive data analysis concerning reverse flows, which forms the basis for returns forecasting. Subsequently, research on consumer returns and reverse logistics has proliferated. Thus, before collecting data and reviewing the topic of consumer returns forecasting, we first examined existing reviews and meta-studies relevant to the subject matter. To accomplish this, we referred to Web of Science, Business Source Ultimate via EBSCOhost, JSTOR and the AIS Electronic Library as primary sources of knowledge (search term: "literature review" AND "return*" AND "forecast*”). As a secondary source, we appended the results of Google Scholar, Footnote 1 for which a different search term was used (intitle:"literature review" ("product return" OR "consumer return" OR "retail return" OR "e-commerce return") forecast) due to unavailable truncations and to reduce the vast amount of literature with financial focus the search term “return” would lead to. Table 1 presents the most pertinent literature reviews related to the scope of this paper.
Agrawal et al. ( 2015 ) identified research gaps within the realm of reverse logistics, finding “forecasting product returns” as a crucial future research path. However, among 21 papers focusing on “forecasting models for product returns”, the emphasis was predominantly on CLSC, reuse, remanufacturing, and recycling, which do not align with the aim of this review. Agrawal et al. also noted a lack of comprehensive analysis of underlying factors in returns forecasting, such as demographics or consumer behavior.
Similarly, Hachimi et al. ( 2018 ) addressed forecasting challenges within the broader context of reverse logistics. They classified their literature using various forecasting approaches: time series and machine learning, operations research methods, and simulation programs. The research gaps they identified included a limited number of influencing factors taken into account, the absence of established performance indicators, and methodological issues related to dynamic lot-sizing with returns. Although this review focused on reverse logistics, the call for research into predictors of future returns is equally applicable to consumer returns in e-commerce.
The review of Abdulla et al. ( 2019 ) centers on consumer returns within the retail context, particularly in relation to return policies. While they discuss consumer behavior and planning and execution of returns, they do not present any sources explicitly focused on forecasting issues.
Micol Policarpo et al. ( 2021 ) reviewed the literature on the use of machine learning (ML) in e-commerce, encompassing common goals of e-commerce studies (e.g., purchase prediction, repurchase prediction, and product return prediction) and the ML techniques suitable for supporting these goals. Their primary contribution is a novel taxonomy of machine learning in e-commerce, covering most of the identified goals. However, within the taxonomy developed, the aspect of return predictions is disregarded.
The most exhaustive literature review to date regarding product returns, conducted by Ambilkar et al. ( 2021 ), analyzed 518 papers and adopted a holistic reverse logistics approach encompassing all supply chain stages. The authors categorized the papers into six categories, including “forecasting product returns”, for which they found and concisely described 13 papers. Due to the broader research scope, none of the analyzed papers focused on consumer returns within the retail context.
The review by Duong et al. ( 2022 ) employed a hybrid approach combining machine learning and bibliometric analysis. Regarding forecasts of product returns, they identified three relevant papers (Clottey and Benton 2014 ; Cui et al. 2020 ; Shang et al. 2020 ) within the “operations management” category. They explicitly call for further research on predicting customer returns behavior in the pre-purchase stage, highlighting the importance of a better understanding of online product reviews and customers’ online interactions.
1.2 Research gaps and research questions
Why is a systematic literature review necessary for investigating consumer returns and forecasting? On the one hand, there are empirical and conceptual papers that touch upon this topic, including brief literature reviews that align with the subject’s focus (e.g., Hofmann et al. 2020 ). However, narrative reviews lack transparency and replicability (Tranfield et al. 2003 ) and often induce selection bias (Srivastava and Srivastava 2006 ) as they tend to approach a field from a specific perspective. In contrast, systematic reviews strive to present a holistic, differentiated, and more detailed picture, incorporating the complete available literature (Uman 2011 ). On the other hand, existing systematic reviews provide structured yet relatively superficial overviews of literature on end-of-use and end-of-life forecasting (Shang et al. 2020 ), but they do not specifically address consumer returns. Furthermore, we contend that a review dedicated to general reverse logistics forecasting would not adequately capture the distinctive context and requirements inherent in the consumer-retailer relationship within the realm of e-commerce (Abdulla et al. 2019 ).
Consequently, based on existing reviews and papers, we have identified research gaps worth examining more in detail: (1) Returns forecasting techniques and relevant predictors for the respective underlying purposes, especially in the context of e-commerce (RQ1 and RQ2); (2) the integration of return forecasts into an existing but incomplete taxonomy of machine learning in e-commerce (Micol Policarpo et al. 2021 ; RQ3); and (3) future research directions pertaining to e-commerce returns forecasting (RQ4). Therefore, this review aims to shed more light on consumer returns forecasting in the retail context. The following research questions outline the primary objectives:
RQ1: What key research problems (e.g., forecasting purposes, technological approaches) have been addressed in the literature on forecasting consumer returns over time?
RQ2: What are the …
Publication outlets and research disciplines,
Research types and methodologies,
Product categories and industries,
Data sources and characteristics,
Relevant forecasting predictors,
Techniques and algorithms
… used to address these key problems?
RQ3: How can returns forecasting be integrated into a taxonomy of machine learning in e-commerce?
RQ4: What are promising or emerging future research directions regarding forecasting consumer returns?
The paper is organized as follows: Sect. 2 describes selected fundamental concepts and the delimitation of the research field on consumer returns forecasting. Section 3 contains the methodology for the review, drawing on the PRISMA guideline (Page et al. 2021 ) while integrating the approaches of Denyer and Tranfield ( 2009 ) and Webster and Watson ( 2002 ). Section 4 presents the review’s main results, answering RQs 1 (Sect. 4.1 ), RQ2 (Sects. 4.2 – 4.5 ), and RQ 3 (Sect. 4.6 ). A research framework developed in Sect. 5 structures the discussion regarding future research directions (RQ4). Section 6 subsumes the overall contribution of this review.
2 Consumer returns and forecasting
2.1 consumer returns and return reasons.
Reverse product flows, commonly referred to as product returns, can be classified into three categories: manufacturing returns, distribution returns, and consumer returns (Shaharudin et al. 2015 ; Tibben-Lembke and Rogers 2002 ). Among these, consumer returns are further differentiated between returns in brick-and-mortar retail or mail-order/e-commerce returns (Tibben-Lembke and Rogers 2002 ) and are also known as commercial returns (de Brito et al. 2005 ) or retail (product) returns (Bernon et al. 2016 ). With sky-rocketing e-commerce sales, online consumer returns have emerged as the dominant segment, making them a highly relevant field of research (Abdulla et al. 2019 ; Frei et al. 2020 ). Additionally, the digitization of retail provides numerous opportunities for data collection, as digital customer accounts facilitate more efficient analytical monitoring of customer behavior (Akter and Wamba 2016 ). Simultaneously, as competitive pressures intensify in e-commerce due to increased price transparency and substitution possibilites, retailers aiming to stimulate impulse purchases face hightened return rates (Cook and Yurchisin 2017 ; Karl et al. 2022 ).
The spatial decoupling of supply and demand introduces a higher level of uncertainty for e-commerce customers regarding various product attributes compared to bricks-and-mortar retailing (Hong and Pavlou 2014 ). As consumers are unable to physically assess the products they order, this translates into returns being essential part of the e-commerce business model. Besides fit uncertainty, other reasons for returns exist. Stöcker et al. ( 2021 ) classify the drivers triggering consumer returns into consumer behavior related reasons (e.g., impulsive purchases, showrooming), fulfillment/service related reasons (e.g., wrong/delayed delivery) and information gap related reasons (product fit, insufficient visualization). By mitigating customers’ return reasons, retailers try to reduce the return likelihood (“return avoidance”) (Rogers et al. 2002 ). Another, but less promising way of reducing returns, is preventing customers who intend to return from actually doing so (e.g., by incurring additional effort or by rejecting returns) (Rogers et al. 2002 ).
Adapted from Abdulla et al. ( 2019 ) and Vakulenko et al. ( 2019 ), a simplified parallel process of a return transaction from the consumer’s and retailer’s perspective is visualized in Fig. 1 . Retailers can use forecasting in all transaction phases (Hess and Mayhew 1997 ). Targeting customer interventions pre-purchase (real-time forecasting) could be implemented by using dynamically generated (Dalecke and Karlsen 2020 ) digital nudging elements (Kaiser 2018 ; Thaler and Sunstein 2009 ; Zahn et al. 2022 ) in case of a predicted high return propensity. In the post-purchase phase, forecasting could stimulate different interventions (e.g., customer support) or can be helpful for logistics and inventory planning activities (Hess and Mayhew 1997 ). In the phase after the return decision, data analysis, including segmentation on different levels, e.g., for customers, products, or brands (Shang et al. 2020 ), can support managerial decision-making regarding assortment or (individualized) return policies for future orders (Abdulla et al. 2019 ). In other words, forecasting (or modeling) of returns in later phases of the process can substantiate interventions in earlier phases of the process (e.g., a temporary return policy change, or the suspension of product promotions due to particular forecasts). However, such data-driven interventions itself also represent an influencing factor to be taken into account in future forecasts; thus, different forecasting purposes can be linked, at least when it comes to the data required. All these interdependencies hint at the circularity of the returns process, with an adequate management of returns representing an opportunity for generating customer satisfaction and retention (Ahsan and Rahman 2016 ; Röllecke et al. 2018 ).
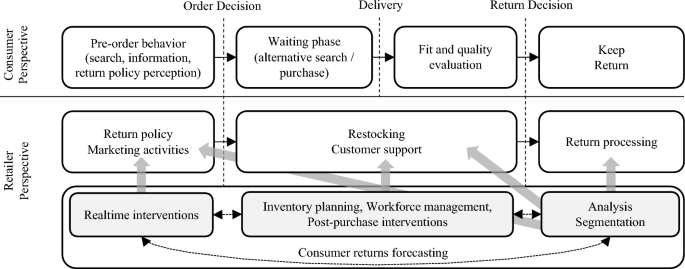
Purchase and return process concerning forecasting issues (adapted from Abdulla et al. 2019 ; Vakulenko et al. 2019 )
Although primarily focussing on the online retailers’ process, it is worth noting that the issue at hand is equally applicable to brick-and-mortar retail (Santoro et al. 2019 ), which can benefit from the application of advanced data analysis techniques for forecasting purposes (Hess and Mayhew 1997 ).
2.2 Forecasting purposes and corresponding techniques
Accurate forecasting holds significant importance in the realm of e-commerce. Precise demand forecasts (“predictions”) play a pivotal role in inventory planning, pricing, and promotions and ultimately impact the commercial success of retailers (Ren et al. 2020 ). Forecasting consumer returns affects similar business aspects and resorts to comparable existing technical procedures. The data science and statistics literature offers diverse methods and algorithms for forecasting consumer returns. The choice of approach depends on the specific objective, with the outcome variable being scaled accordingly. For instance, when forecasting whether a single product will be returned, the dependent variable is either binary or expressed as a propensity value ranging form 0 to 1. On the other hand, forecasting the quantitay or timing of returns entails continuous outcome variables. As a result, various techniques, from time-series forecasting to machine learning approaches can be applied, which will be briefly outlined in the subsequent sections.
2.2.1 Return classifications and propensities
A naïve method for determining the propensity or return decision forecast is using lagged (historical) return information (return rates), either for a given product, a given customer, or any other reference, to calculate a historical return probability (Hess and Mayhew 1997 ). Return rate forecasts are a reference-specific variant of forecasting return propensities.
Simple causal models based on statistical regression methods utilize one or more independent exogenous variables. The logistic regression (logit model) is employed when the dependent variable is binary or contains more nominal outcomes (multinomial logistic regression). For each observation, the binary logistic regression assesses the probability that the dependent variable takes the value “1” (Hastie et al. 2017 ). Consequently, this approach finds application for return decisions and return propensities. Comparatively, linear discriminant analysis (Fisher 1936 ) bears a resemblance to logistic regression by generating a linear combination of independent variables to best classify available data. This classification process involves determining a score for each observation, subsequently compared to a critical discriminant score threshold, and distinguishing between return and keep.
More sophisticated machine learning (ML) techniques such as neural networks, decision tree-based methods, ensemble learning, and boosting methods are highly suitable for this forecasting purpose. For a general exposition of ML techniques in the domain of e-commerce, we refer to Micol Policarpo et al. ( 2021 ). Additionally, for a comparative study of several state-of-the-art ML classification techniques, see Fernández-Delgado et al. ( 2014 ). Artificial Neural Networks (NN) consist of interconnected nodes (“neurons”) organized in layers, exchanging signals to ascertain a function that accurately assigns input data to corresponding outputs. Typically, supervised learning techniques such as backpropagation compare the network outputs with known actual values (Hastie et al. 2017 ). Notably, neural networks are the most popular machine learning algorithm in last years’ e-commerce research (Micol Policarpo et al. 2021 ), and deep learning extensions like Long Short-Term Memory (Bandara et al. 2019 ) are gaining attention. Decision Trees (DT) manifest as hierarchical structures of branches representing conjunctions of specific characteristics and leaf nodes denoting class labels. This approach endeavors to construct an optimal decision tree for classifying available observations. Many decision tree algorithms have been introduced to serve this purpose (e.g., Breiman et al. 1984 ; Pandya and Pandya 2015 ). Ensemble learning methods adopt a voting mechanism involving multiple algorithms to enhance predictive performance (Polikar 2006 ). Analogously, boosting and bagging techniques are incorporated in algorithms like AdaBoost or the tree-based Random Forest (RF) to augment the input data, aiming at more generalizable forecasting models less prone to overfitting issues (Hastie et al. 2017 ). Support Vector Machines (SVM) stand as another example of a supervised ML algorithm, having demonstrated efficacy in tackling classification problems within e-commerce (Micol Policarpo et al. 2021 ).
2.2.2 Return timing and volume forecasts
For product returns, timing is crucial in forecasting end-of-life, end-of-use, or remanufacturing returns that can occur years after the initial purchase (Petropoulos et al. 2022 ). In contrast, for consumer returns, the possible time window in which products are regularly returned in new condition with the aim of a refund is much shorter (usually less than 100 days and mostly less than 30 days), and priorities are more on forecasting return volumes. Forecasting return volumes can be multi-faceted, ranging from forecasting the total return volume a retailer has to process within its logistics department through forecasting product-specific return numbers up to forecasting costly return shares, e.g., return fraud volume. Because returns depend on fluctuating sales, time-series forecasting of return volumes performs only well with constant sales volumes or under risk-pooling (Petropoulos et al. 2022 ). Thus, for a naïve return volume forecast, sales forecasts for a given timeframe are multiplied by the lagged return rate (historical data of products/consumers or any other reference). Possible algorithms for estimating historical return rates include time series forecasting to causal predictions comprising ML approaches (Hachimi et al. 2018 ).
Time-series techniques, e.g., single exponential smoothing (SES) or Holt-Winters-approaches (HW), are based on the assumption that the future development of an outcome variable (e.g., return volume) is dependent on its past numbers, while time acts as the only predictor. Most of these models can be generalized as autoregressive moving averages (ARIMA) models, for which numerous extensions are available. These models can approximate more complex temporal relationships. Similarly, time-series regression models use univariate linear regression with time as a single exogenous variable.
The mentioned multivariate regression models are essential statistical tools and can predict metric variables such as return volume or time. The logic is to fit a linear function of a given set of input variables (“features”) to the outcome variable with the criteria of minimizing the residual sum of squares (Hastie et al. 2017 ). Many variants of regression models are derived from this logic (e.g., generalized linear models), and various extensions are built upon this base (e.g., LASSO for variable selection, Tibshirani 1996 ).
Emerging from more complex statistical methods and using the possibilities of continuously increasing computing power, IT-based machine learning (ML) approaches were developed. Some of these approaches have already been presented in Sect. 2.2.1, being suitable for predicting metric variables in addition to classification tasks, e.g., neural networks, decision tree algorithms, and especially ensemble techniques like random forests.
3 Methodology
Methodologically, the research process of this review follows the PRISMA guideline (Page et al. 2021 ) where applicable and is structured in five steps (Denyer and Tranfield 2009 ; Webster and Watson 2002 ): (1) question formulation; (2) locating studies; (3) study selection and evaluation; (4) (concept-centric) analysis and synthesis; and (5) reporting and using the results for defining an agenda for future research.
The first step refers to the research questions already formulated in the introduction. The second step involves selecting the databases and defining the search terms. In that respect, five scientific databases were selected, aiming at journal as well as conference publications: AIS Electronic Library (AISeL), Business Source Ultimate (BS) via EbscoHost, JSTOR (JS), Science Direct (SD), and Web of Science (WoS). To ensure inclusivity and to account for potential variations in spelling or phrasing, the final search strings incorporate truncations where applicable. The search query utilized in this review comprises two key components. Firstly, it pertains to consumer returns, encompassing products returned by consumers, primarily in the context of e-commerce, to the retailer. While it is recommended to use reasonably general search terms, the term “return” alone would yield results for various stages of reverse logistics and a vast amount of financial literature. Therefore, we conducted a more specific search using the phrase “consumer return*” and the related terms “e-commerce return*”, “product return*”, “return* product”, “customer return*”, and “retail return*”. Secondly, this paper specifically focuses on forecasting (“forecast*”), which can be alternately referred to as “predict*” or “prognos*”. The combination of these terms was searched for in the Title, Abstract and Keywords fields.
The search includes results up to the middle of 2022 and resulted in 725 initial search hits (see Fig. 2 ). As this review aims to identify papers dealing with consumer returns and forecasting, the inclusion criteria for eligibility were:
The title or keywords referred to consumer returns or forecasting (in a broader sense, including data preparation). A connection to the respective subject area and applicability to the retail domain should at least be plausible.
Manuscript in English: No important study would be written and published in a language different than English.
The paper has undergone a single- or double-blind peer-review process, either as a journal publication or as a publication in peer-reviewed conference proceedings.
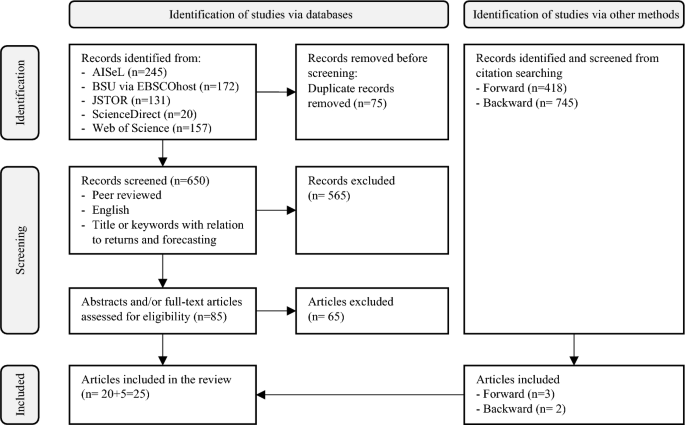
Research process flow diagram
In the third step, duplicates were removed, resulting in a set of 650 unique records. Subsequently, the papers underwent screening based on title, keywords, and language to determine whether they warranted further examination. This preliminary screening phase reduced the number of papers to 85. These papers’ abstracts and full texts were thoroughly reviewed to assess their relevance. This step encompasses all papers pertaining to returns forecasting for retailers or direct-selling manufacturers while excluding those focused on closed-loop supply chain management or remanufacturing, recycling, and end-of-life returns. Ultimately, a final sample of 20 publications was identified, serving as a foundation for identifying additional relevant papers (vom Brocke et al. 2009 ; Webster and Watson 2002 ) through a forward search using Google Scholar and snowballing via backward search. This process yielded an additional five papers, resulting in a total of 25 papers included for review (Table 2 ).
The fourth step comprises the analysis and synthesis of the relevant papers. Data, including bibliographic statistics, were collected in accordance with the research questions. A two-way concept-centric analysis, as described by Webster and Watson ( 2002 ), was conducted, encompassing confirmatory aspects based on the fundamentals outlined in Sect. 2 of this paper, as well as exploratory elements aimed at enriching existing categories and concepts. The objective was to comprehensively describe the relevant concepts, approaches, and dimensions discussed in the literature.
Moving on to the fifth and final step (Denyer and Tranfield 2009 ), the results are presented. Initially, the main scope of the papers included in the analysis is presented. Next, bibliographic data pertaining to the included papers are provided to offer a concise overview of the research area and its recent developments, followed by a content analysis and synthesis of the relevant literature to delve into the current state of research and highlight key findings. Finally, Sect. 5 outlines a research agenda for the domain (vom Brocke et al. 2009 ).
4 Results of the systematic review
After outlining the main scope of the relevant publications (4.1), a short bibliographic characterization (4.2) is given. Next, this section presents the results of the systematic review, focussing on the methodology and datasets used (4.3), predictors used for returns forecasting (4.4), and forecasting techniques employed (4.5). The integration of consumer returns forecasting into an existing taxonomy for e-commerce and machine learning (Micol Policarpo et al. 2021 ) summarizes and concludes the presentation of the results.
4.1 Overview and main scope of the relevant publications
Table 3 provides an overview of the forecasting purpose of the papers, the data source for the forecasting, the algorithms employed, and the predictors used in the forecasting models. The contributions of the respective papers regarding forecasting issues are summarized in the Appendix.
For identifying research streams, the publications are analyzed regarding the intention and main scope, as described in the abstract, the respective research questions, and the remainder of the papers. Most papers were assigned to an unequivocal research scope, while some contributed to two key topics (Fig. 3 ).
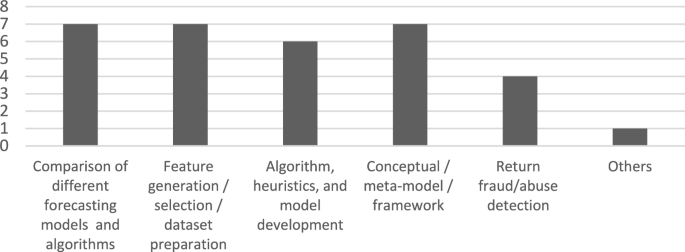
Classification of main scopes (n = 25; not mutually exclusive)
At first, we identified a stream of literature regarding the comparison of different forecasting models and algorithms (Asdecker and Karl 2018 ; Cui et al. 2020 ; Drechsler and Lasch 2015 ; Heilig et al. 2016 ; Hess and Mayhew 1997 ; Hofmann et al. 2020 ; Imran and Amin 2020 ). These papers use existing approaches, adapt them for individual forecasting purposes, apply models to one or more datasets, and compare and evaluate the resulting forecasting performance. One paper claims that the difference in forecasting accuracy of easily interpretable algorithms is relatively small compared to more sophisticated ML algorithms (Asdecker and Karl 2018 ). This statement is partially confirmed (Cui et al. 2020 ), as the ML algorithms show advantages over simpler models in the training data set but have lower prediction quality due to overfitting issues in the test data. Nevertheless, fine-tuned ML approaches (e.g., deep learning with TabNet) outperform simpler models and gain accuracy when correcting class imbalances during the data preparation phase (Imran and Amin 2020 ). When confronted with large class imbalances (e.g., low return rates), boosting algorithms like Gradient Boosting work well without oversampling (Hofmann et al. 2020 ). Fundamentally, ensemble models incorporating different techniques show the maximum possible accuracy (Asdecker and Karl 2018 ; Heilig et al. 2016 ). Forecasting of return timing is more erroneous than return decisions, and split-hazard-models outperform simple OLS approaches (Hess and Mayhew 1997 ). Time series prediction only works reliably when return rates do not fluctuate heavily (Drechsler and Lasch 2015 ).
The second stream we identified focuses on feature generation or selection and dataset preparation (Ahmed et al. 2016 ; Ding et al. 2016 ; Hofmann et al. 2020 ; Rezaei et al. 2021 ; Samorani et al. 2016 ; Urbanke et al. 2015 , 2017 ). Besides this central topic, some papers also compare different forecasting algorithms (Ahmed et al. 2016 ; Hofmann et al. 2020 ; Rezaei et al. 2021 ; Urbanke et al. 2015 , 2017 ). For example, random oversampling of data with large class imbalances can improve the performance of different forecasting algorithms, while models based only on sales/return history perform worse than models with more features (Hofmann et al. 2020 ). Two similar approaches are based on product, basket, and clickstream data, using different algorithms for feature extraction (Urbanke et al. 2015 , 2017 ). The first developed a Mahalanobis Feature Extraction algorithm, proving superior to other algorithms like principal component analysis or non-negative matrix factorization (Urbanke et al. 2015 ). The second develops a NeuralNet algorithm to extract interpretable features from a high-dimensional dataset, showing superior performance and giving reasonable interpretability of the most important factors (Urbanke et al. 2017 ). For the automated integration of different data sources into single flat tables and the generation of discriminating features, a rolling-path algorithm is developed, improving performance when data is imbalanced (Ahmed et al. 2016 ). Similarly, the software “Dataconda” can automatically generate and integrate relational attributes from different sources into a flat table, which is often the required prerequisite for forecasting algorithms (Samorani et al. 2016 ). A different selection approach clusters the features into groups and applies selection algorithms to the groups, aiming to select a smaller set of attributes (Rezaei et al. 2021 ). As quite an offshoot, one paper predicts a seller’s overall daily return volume dependent on his current “reputation” measured by tweets (Ding et al. 2016 ), which needs sentiment analysis to be integrated into the forecast.
A quite heterogenous research stream belongs to the development of algorithms, heuristics, and models that go beyond a straightforward adaption of existing approaches (Fu et al. 2016 ; Joshi et al. 2018 ; Li et al. 2018 ; Potdar and Rogers 2012 ; Rajasekaran and Priyadarshini 2021 ; Shang et al. 2020 ; Sweidan et al. 2020 ; Zhu et al. 2018 ). Potdar and Rogers ( 2012 ) developed a methodology for forecasting product returns based on reason codes and consumer behavior data. Fu et al. ( 2016 ) developed a conditional probability-based statistical model for predicting return propensities while revealing return reasons and outperforming some baseline benchmark models. Li et al. ( 2018 ) describe their “HyperGo” approach as a ‘framework’ and develop an algorithm for forecasting return intention after basket composition. Zhu et al. ( 2018 ) describe a “LoGraph” random walk algorithm for predicting returned customer/product combinations within their framework. Although Joshi et al. ( 2018 ) label their approach as a “framework”, they describe a specific two-stage algorithm for forecasting return decisions based on network science and ML. Rajasekaran and Priyadarshini ( 2021 ) developed a hybrid metaheuristic-based regression approach to predict return propensities.
Seven papers deal with concepts, meta-models, or substantial frameworks for returns forecasting (Fu et al. 2016 ; Fuchs and Lutz 2021 ; Heilig et al. 2016 ; Hofmann et al. 2020 ; Li et al. 2018 ; Shang et al. 2020 ; Zhu et al. 2018 ). A generic framework for a scalable cloud-based platform, which enables a vertical and horizontal adjustment of resources, could enable the practical real-time use of computationally intensive ML algorithms for forecasting returns in an e-commerce platform (Heilig et al. 2016 ). Two papers (Fuchs and Lutz 2021 ; Hofmann et al. 2020 ) are based on design science research (DSR, Hevner et al. 2004 ) for developing artifacts like meta models and frameworks. The first also refers to CRISP-DM, the “Cross Industry Standard Process for Data Mining” (Wirth and Hipp 2000 ), and develops a shopping-basket-based general forecasting approach suitable across different industries without domain knowledge and attributes needed (Hofmann et al. 2020 ). In a similar approach, based on the basket composition and user interactions, a generic model for real-time return prediction and intervention is developed (Fuchs and Lutz 2021 ) and prepared for integration into an ERP system. Fu et al. ( 2016 ) present a generalized return propensity latent model framework by decomposing returns into different inconsistencies (unmet product expectations, shipping issues, and both factors combined) and enriching the derived propensities with product features and customer profiles. Li et al. ( 2018 ) developed a “HyperGo” framework for forecasting the return intention in real-time after basket composition, including a hypergraph representation of historical purchase and return information. Similarly, Zhu et al. ( 2018 ) developed a “HyGraph” representation of historical customer behavior and customer/product similarity, combined with a “LoGraph” random-walk-based algorithm for predicting customer/product combinations that will be returned. Shang et al. ( 2020 ) discuss two opposing forecasting concepts, demonstrating that their predict-aggregate framework is superior to common and more naïve aggregate-predict approaches.
The last stream covers the detection and forecasting of return fraud and abuse (Drechsler and Lasch 2015 ; John et al. 2020 ; Ketzenberg et al. 2020 ; Li et al. 2019 ). On the employees’ side, one paper tries to automatically predict fraudulent return behavior of agents (employees), e.g., regarding unjustified refunds, by a penalized logit model, enabling a lift in detection (John et al. 2020 ). On the customers’ side, misused returns as a cost-incurring problem are the forecasting purpose of different time series prediction models (Drechsler and Lasch 2015 ). Instead of focussing on fraudulent transactions, a trust-aware random walk model identifies consumer anomalies, enabling retailers to apply targeted measures to specific customer groups (selfish, honest, fraud, and irrelevant customers) (Li et al. 2019 ). Similarly, returning customers can be categorized into abusive, legitimate, and nonreturners (Ketzenberg et al. 2020 ). Based on the characterization of abusive return behavior, a neural network classifier recaptures almost 50% of lost profits due to return abuse (Ketzenberg et al. 2020 ).
One paper (Sweidan et al. 2020 ) could not be assigned to the other scopes. It applies a single algorithm (RF) to a given dataset, and it contributes to the idea that only forecasted return decisions with high confidence should be used for targeted interventions due to their overproportional reliability.
4.2 Bibliographic literature analysis
Forecasting consumer returns has gained more research attention since 2016 (Fig. 4 ). The majority of the sample are conference publications, a couple of years ahead of the rise in journal publications. Compared to the publications on returns forecasting in the broader context of reverse logistics, which emerged in 2006 (Agrawal et al. 2015 ), the research on consumer returns moved into the spotlight about ten years later. This development is linked to a massive increase in e-commerce sales pre- and in-pandemic (Alfonso et al. 2021 ).
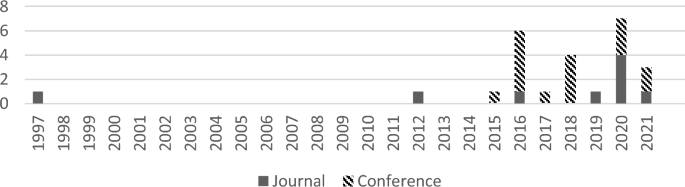
Publication trend by publication outlet
Out of 9 journal publications in the final sample, only two are published in the same journal (Journal of Operations Management). Out of 16 conference papers, 6 are published at conferences of the Association for Information Systems. In total, 16 of the 25 papers found are published in Information Systems (IS) and related outlets. Others can be assigned to the Management Science / Operations Research discipline (3), Strategy & Management in a broader sense (4), Marketing (1), and Research Methods (1) (Fig. 5 ).
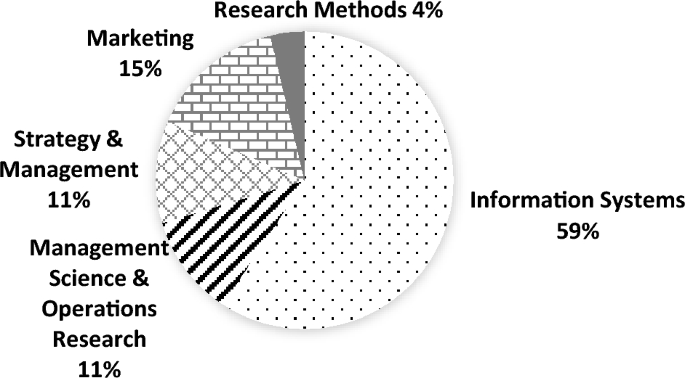
Distribution of publication disciplines
Regarding the researchers’ geographical perspective, one paper was jointly published by authors from the US and China, 10 of 25 papers were authored from North America, followed by authors from Germany (7), India (3), China (1), and one paper each from Bangladesh, Singapore, and Sweden.
The most cited paper (200 external citations Footnote 2 ) from Hess and Mayhew ( 1997 ) could be thought of as the root of this research field (Table 4 ). However, only 10 out of 24 papers reference this work. Although Urbanke et al. ( 2015 ) received only 15 citations in total, within the sample, it is the second most cited paper (8 citations) and could eventually be classified as a research strand and origin of returns forecasting in the IS domain. Concerning the remaining papers, no unique strands of literature are recognizable based on citation analysis.
4.3 Methodology and data characterization
Regarding methodology, most of the papers start with a short narrative literature review regarding their respective focus. Not a single paper was based on interviews, surveys, questionnaires, or field experiments. 3 out of 25 papers formulated and tested conventional hypotheses. All of the publications use quantitative data for analysis and forecasting in a “case study” style, including numerical experiments based on real or simulated data.
Table 5 lists further details about the data used in the publications. 4 out of 25 papers rely on simulated data, and 23 out of 25 integrate actual data gained from a retailer. Two papers use both data types. 5 papers use more than one dataset (Ahmed et al. 2016 ; Cui et al. 2020 ; Rezaei et al. 2021 ; Samorani et al. 2016 ; Shang et al. 2020 ). The most frequently studied industry is fashion/apparel (10 papers), followed by five consumer electronics datasets. Two publications are based on data from a Taobao cosmetics retailer, and two datasets originate from general and wide assortment retailers. Two datasets incorporate building material and hardware store articles, and the detailed products are not named for three publications. Based on the previous studies, it is evident that consumer returns forecasting is most relevant for e-commerce, as 19 of the 25 publications refer to e-tailers. Nevertheless, 7 publications refer to brick-and-mortar retailing. Direct selling/marketing is represented in 2 data sets.
4.4 Predictors for consumer returns
There is an individual stream of research into factors that influence or help avoid consumer returns (e.g., Asdecker et al. 2017 ; De et al. 2013 ; Walsh and Möhring 2017 ), which is not part of this review. Nevertheless, the forecasting literature gives insights into return drivers, as the input variables (features, predictors, exogenous variables) for forecasting models represent some of these factors. Table 6 presents the most used predictors and tries to map these to the return driver categorization from Sect. 2.2 (Stöcker et al. 2021 ).
Although only a part of the publications interprets the predictors, some insights can be extracted. For total return volume , sales volume is the most critical predictor (Cui et al. 2020 ; Shang et al. 2020 ). Historical return volume trends can include behavioral aspects (e.g., impulse purchases) in a given timeframe (Cui et al. 2020 ; Shang et al. 2020 ). The product type significantly impacts the volume of returns (Cui et al. 2020 ), confirmed by widely varying return rates between different industries/sectors. Adding transaction-, customer-, or product-level predictors led to a surprisingly small forecasting accuracy gain (4% reduction of RMSE, Shang et al. 2020 ). The latter input variables may be more critical in forecasting return decisions and propensities.
Regarding product attributes , product or order price is one of the most common predictors, while some papers also include price discounts. In most models, price is hypothesized to increase returns (e.g., Asdecker and Karl 2018 ; Hess and Mayhew 1997 ). Promotional (discounted) orders also seem to result in more returns (Imran and Amin 2020 ), which could be explained by the stimulation of impulse purchases. Footnote 3 Brand perception influences return decisions (positive brands, lower returns) (Samorani et al. 2016 ). The order and return history of products are also relevant for predicting future orders and returns (Hofmann et al. 2020 ). Fit importance as a product attribute does not significantly change return propensities (Hess and Mayhew 1997 ).
Concerning customer attributes , gender seems essential, as female customers return significantly more items than men (Asdecker and Karl 2018 ; Fu et al. 2016 ). Younger customers show a slightly lower propensity to return (Asdecker and Karl 2018 ), but age played a more prominent role in predicting return fraud among employees than in customers (John et al. 2020 observed more fraud among younger employees). Customers with low credit scores returned more (Fu et al. 2016 ). The return history of a customer is possibly the most important predictor of future return behavior (Samorani et al. 2016 ). Some papers argue that consumer attributes, including purchase and return history (e.g., number and value of orders), are more relevant predictors than product or transaction profiles, reflecting more or less stable consumer preferences (Li et al. 2019 ).
Basket interactions are significant (Urbanke et al. 2017 ) in returns prediction. E.g., the larger the basket, the higher the return propensity will be (Asdecker and Karl 2018 ). Selection orders (same product in different sizes or colors) increase the return propensity (Li et al. 2018 ). Logistics attributes like delivery times only show minor effects (Asdecker and Karl 2018 ). Regarding the payment method, prepaid products are sent back less frequently than those with post-delivery payment options (Imran and Amin 2020 ), confirming other research results (Asdecker et al. 2017 ).
One literature stream focuses on the automated generation of features , as different and large-scale data sources need to be integrated and prepared for forecasting algorithms. Thus, possible interrelationships are complex to find manually, and ML approaches might outperform human analysts (Rezaei et al. 2021 ). While some approaches generate a large number of features that are hard to make sense of (Ahmed et al. 2016 ), the approach of Urbanke et al. ( 2017 ) aims to maintain the interpretability of automatically generated input variables. Some unexpected but meaningful interrelations might be found by automatic feature generation, e.g., the price of the last returned orders (Samorani et al. 2016 ). Nevertheless, automatic feature generation might be computation-intensive; thus, a parallel integration of feature selection could be advantageous for large data sets (Rezaei et al. 2021 ).
A remarkable research path based on artificial intelligence is integrating qualitative information like product reviews as predictors, going beyond numerical feedback (Rajasekaran and Priyadarshini 2021 ) or tweets. These data can be processed and made accessible for forecasting with ML-based sentiment analysis techniques (Ding et al. 2016 ).
4.5 Forecasting techniques and algorithms
To describe the techniques and algorithms employed, we sorted the papers by forecasting purpose as described in Sect. 2 , then assigned them to different algorithms, either from time series forecasting, statistical techniques, or ML algorithms. Table 7 lists all papers for which an assignment was possible, and the respective techniques used. If a comparison was possible, the best-performing algorithm is marked in this table.
The approaches listed in Table 7 are overlap-free, but some papers use more than one version of an approach, i.e., more than one algorithm from a category. E.g., TabNet is a DeepLearning version of neural networks (NN), and different variants of GradientBoosting are compared in one paper (CatBoost/LightGBM, not differentiated in the table below) (Imran and Amin 2020 ).
The algorithm used most frequently (Fig. 6 ) is the Random Forest algorithm (RF, 10 papers), followed by Support Vector Machines (SVM, 8 papers), Neural Networks (NN, 6 papers), logistic regression (Logit, 6 papers), GradientBoosting (5 papers), Ordinary Least Squares regression (OLS, 4 papers), Adaptive Boosting (AdaBoost), Linear Discriminant Analysis (LDA), and CART (Classification and Regression Trees, 3 papers each).
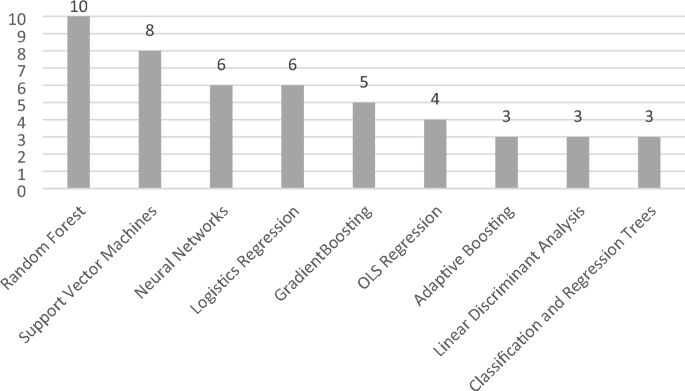
Most frequently used algorithms (used in at least three papers)
The papers focusing on return volume use time series forecasts like (AutoRegressive) Moving Averages (MA), Single Exponential Smoothing (SES), and Holt-Winters Smoothing (HWS) more frequently than ML algorithms. Nevertheless, when considering a predict-aggregate approach as proposed by Shang et al. ( 2020 ), these ML techniques could be helpful in forecasting return decisions first and cumulating the propensity results for the volume prediction in the second step.
In forecasting binary return decisions, Random Forests (RF) (Ahmed et al. 2016 ; Heilig et al. 2016 ; Ketzenberg et al. 2020 ), Neural Networks (NN) (Imran and Amin 2020 ; Ketzenberg et al. 2020 ), as well as Adaptive Boosting (AdaBoost) (Urbanke et al. 2015 , 2017 ) showed high prediction performance. The performance of different algorithms varies depending on the data set, the implementation, and the parameterization used. For this reason, it is hardly possible to make a generally valid statement regarding performance levels. Combining several algorithms in ensembles (Asdecker and Karl 2018 ; Heilig et al. 2016 ) seems advantageous, at least for retrospective analytical purposes, when the required computing resources are less relevant.
When evaluating different forecasting algorithms for return decisions, imbalanced classes (especially evident for low return shares in non-fashion datasets) seem to be handled differently depending on the algorithms. Class imbalances might distort comparison results in some publications. Random oversampling as a measure of data preparation can solve this problem (Hofmann et al. 2020 ).
High-performance algorithms are needed for real-time predictions, e.g., graph and random-walk-based (Li et al. 2018 ; Zhu et al. 2018 ). According to Li et al. ( 2018 ), the proposed algorithm “HyperGo” performs best for most performance metrics.
4.6 E-Commerce and machine learning taxonomy extension
In their literature review regarding the use of ML techniques in e-commerce, Micol Policarpo et al. ( 2021 ) propose a taxonomy to visualize specific ML algorithms in the context of e-commerce platforms. This novel kind of taxonomy is based on direct acyclic graphs, i.e., all input variables need to be fulfilled to reach the target. The first level of the taxonomy represents different target goals for the use of ML in e-commerce. While returns forecasting (“product return prediction”) is identified as an essential goal among others (purchase prediction, repurchase prediction, customer relationship management, discovering relationships between data, fraud detection, and recommendation systems), it was excluded from the taxonomy they developed, possibly because the review comprised only two relevant papers on this topic (Micol Policarpo et al. 2021 ). The review at hand proposes an extension of Micol Policarpo’s taxonomy, renaming the goal to “consumer returns forecasting”. This extension reflects and synthesizes the consumer returns forecasting studies reviewed.
The middle level of the taxonomy represents properties and features that support this superordinate goal. On this level, our extension does not include return fraud detection, which we propose to be integrated into the existing category of “fraud detection”, separated into transaction analysis and consumer analysis (Micol Policarpo et al. 2021 ). Circles represent the necessary data to execute the analysis, referring to categories introduced in (Micol Policarpo et al. 2021 ), with an additional “return history” category. The bottom level presents the algorithms described frequently, while some streamlining is required regarding the tools and approaches that seem the most common or most appropriate.
The schematic above (Fig. 7 ) is to be read as follows: In the context of E-Commerce + Artificial Intelligence (Layer 1), Consumer Return Forecasting (Layer 2) is an essential goal among six other goals. Layer 3 presents different purposes of analysis, which are the base for return forecasting. Realtime Basket Analysis is based on clickstream data and basket composition (browsing activities) to target interventions. Basket analysis benefits from customer and product information (dotted line). Graph-based approaches (Li et al. 2018 ; Zhu et al. 2018 ) are promising for real-time analysis due to their lower computing requirements, although cloud-based implementation of more complex algorithms or ensemble models might be feasible (Fuchs and Lutz 2021 ; Heilig et al. 2016 ; Hofmann et al. 2020 ). Customer Analysis and Product Analysis (e.g., Potdar and Rogers 2012 ) require adequate Data Preparation in the sense of input variable generation, extraction, and selection (Urbanke et al. 2015 , 2017 ). For these purposes, data regarding return history (e.g., Hofmann et al. 2020 ; Ketzenberg et al. 2020 ), purchase history (e.g., Cui et al. 2020 ; Fu et al. 2016 ), customer personal information (e.g., Heilig et al. 2016 ; Ketzenberg et al. 2020 ), clickstream data, and browsing activities are required as input (shown by cross-hatched circles). For each purpose, one or more possible algorithms are shown.
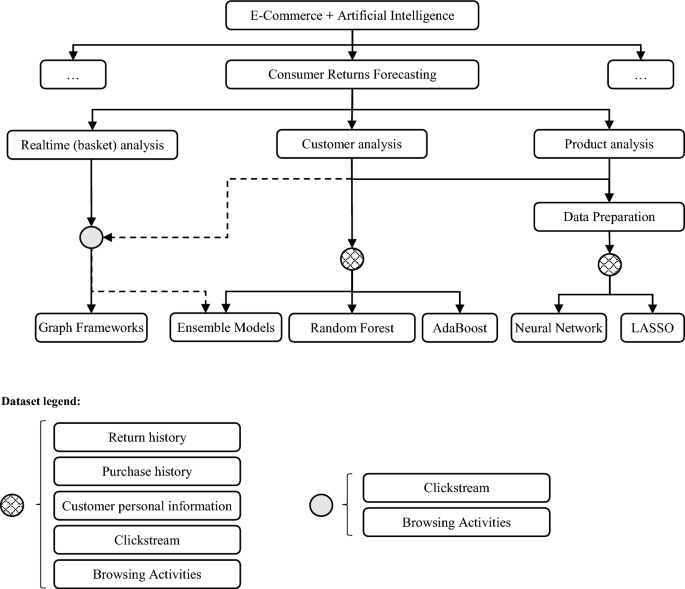
Proposed consumer returns forecasting extension to the E-commerce and Machine Learning techniques taxonomy of Micol Policarpo et al. ( 2021 , p. 13)
Compared to predicting purchase intention, return predictions seem to require more levels of data. Nevertheless, even simple rule-based interventions can promise benefits, e.g., selection orders that inevitably lead to a return shipment can be easily recognized (Hofmann et al. 2020 ; Sweidan et al. 2020 ). Different ML techniques are helpful for data preparation and input variable (feature) extraction and generation when considering more complex interrelations. NeuralNet is one example of an automatic selection of relevant features (Urbanke et al. 2017 ). These approaches are not only able to enhance forecasting accuracy (Rezaei et al. 2021 ) but can also render the many possible variables interpretable about their content.
5 Discussion
The analysis of the papers above revealed that research in this discipline seems heterogeneous and partly fragmented, and clear-cut research strands are still hard to identify. Thus, the existing literature calls for further publications to render this research field more comprehensive. Below, research opportunities are derived and embedded in a conceptual research framework derived from the results of the existing literature, also integrating the extension of the E-Commerce and Machine Learning taxonomy (Fig. 7 ). A conceptual framework improves the understanding of a complex topic by naming and explaining key concepts and their relationships important to a specific field (Jabareen 2009 ; Miles et al. 2020 ). Thus, this framework aims to organize problems and solutions discussed in the consumer returns forecasting literature and to embed and classify potential future research topics in the existing knowledge base (Ravitch and Riggan 2017 ). The subsections following the framework outline some potential research avenues (P1–P6) that have been touched on in the past but still leave considerable opportunities for further insights. These proposals should not be seen as comprehensive due to numerous other research opportunities in this field but rather as prioritization based on the current literature.
The framework derived (Fig. 8 ) underlines the interdisciplinary nature of this research field, integrating different perspectives (information systems research, marketing and operations perspective, and strategy and management perspective). From a managerial point of view, the literature included in this review is biased towards the information systems perspective. Thus, in contrast to the framework developed by Cirqueira et al. ( 2020 ) for purchase prediction, we do not take a process perspective but instead emphasize the interdependencies and interactions between research topics and highlight the managerial need to take a strategical perspective similar to the framework developed by Winklhofer et al. ( 1996 ). Consequently, a meta-layer on forecasting frameworks and practices includes the mainly technical development frameworks in this review but also accentuates the need for further research regarding actual organizational forecasting practices (e.g., P2, P5, P6). Around this meta-layer, some related research strands are linked in order to embed the topic of returns forecasting in the research landscape. E.g., in general, forecasting purchases and returns could be linked (P6), also effecting inventory decisions.
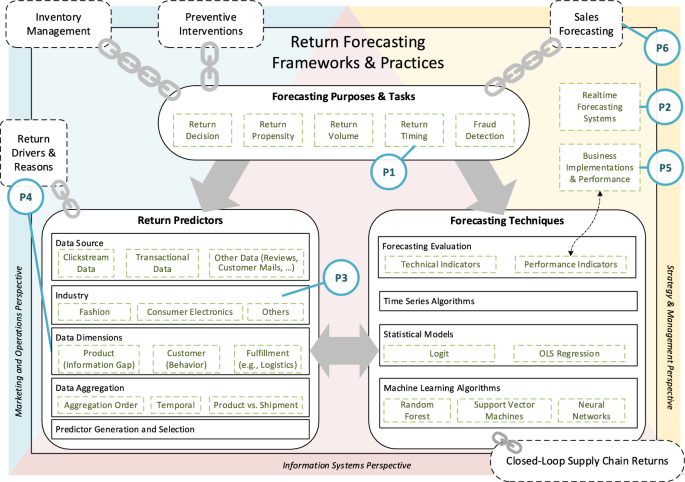
Conceptual Consumer Return Forecasting Framework
The center of the framework consists of three dimensions, namely purposes and tasks, predictors, and techniques. Depending on the strategical purpose, tasks are derived that determine (1) the data (predictors) needed and (2) the usable techniques to execute the forecasting. Different forecasting techniques require an individual set of predictors, whereas the availability of specific data allows and determines the use of more or less sophisticated algorithms.
In the literature, some forecasting purposes were more pronounced (return decisions or propensities), while others have gained less attention (return timing, P1). Regarding the data necessary for accurate forecasting, the return predictors discussed often were hardly comparable, as they originated from different data sources, different industries, were related to different dimensions, or were aggregated in another way. Systematically linking forecasting predictors and research on return drivers and reasons could contribute significant insights (P4) that, from a marketing perspective, may support the development of effective preventive instruments. Furthermore, the literature mainly refers to the fashion or consumer electronics industry, leaving room to validate the findings in the context of other industries (P3).
When (automatically) selecting or creating predictors, the boundaries between predictors and prediction techniques are blurred as machine learning algorithms prepare the input data before executing a forecasting model. Regarding forecasting techniques, time series forecasting was seldom used in recent publications. Machine learning algorithms were the most popular subject of investigation, with random forests, support vector machines, and neural networks as the most popular implementations. Classical statistical models like logit models for return decisions or OLS regression gained less research attention. Literature on end-of-life return forecasting could complement the research on techniques and their accuracy. Most publications used technical indicators for assessing the accuracy of forecasting models, which is the information systems perspective. From a managerial position, evaluating (monetary) performance outcomes (e.g., Ketzenberg et al. 2020 ) of forecasting systems should be more relevant.
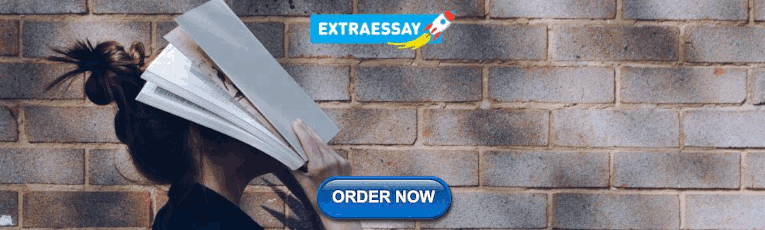
5.1 Research proposal P1: return timing for consumer returns
Toktay et al. ( 2004 ) encouraged the integrated forecasting of the return rate and the return time lag. In line with this, Shang et al. ( 2020 ) criticize the missing focus on the timing of return forecasts. The reviewed literature confirms that forecasting return propensities and decisions are more prominent than timing and volume forecasts. While the knowledge of when a return is expected is vital in managing end-of-life returns that occur over the years, for retail consumer returns, return periods are mostly 14–30 days. Thus, the variability of return timing seems limited compared to end-of-life returns in this context, which makes this forecasting purpose less critical. Nevertheless, some retailers offer up to 100 days of free returns (e.g., Zalando). Consequently, more studies about the importance of return timing forecasts in the e-commerce context from a business and planning perspective and their interdependence with return processing or warehousing issues could shed light on this topic and complement the current literature (Toktay et al. 2004 ; Shang et al. 2020 ).
5.2 Research proposal P2: realtime forecasting systems
Another research gap became apparent regarding the real-time use of forecasting systems and the associated activities and interventions, building on the initial research and the frameworks already published (e.g., Heilig et al. 2016 ; Urbanke et al. 2015 ). The generic framework developed by Fuchs and Lutz ( 2021 ) could serve as a launching pad for this stream of research.
The paper from Ketzenberg et al. ( 2020 ) could act as a stimulus and inspiration for a similar approach, not only focusing on return abuse as already examined but on return forecasting in general, the possible associated interventions for various consumer groups, and the resulting consequences for the retailer’s profit. Even the methodology of customer classification could be helpful for many retailers in targeting interventions.
Before real-time return forecasting is implemented, associated preventive return management instruments need to be designed and evaluated. Many of these measures are discussed (e.g., Urbanke et al. 2015 ; Walsh et al. 2014 ), but an overview of which preventive measures (for some examples, see Walsh and Möhring 2017 ) are effective in general (1) and how forecasting accuracy interdepends with their usefulness (2) is still missing, to substantially link the topics of forecasting and interventions. No answers could be found to the call by Urbanke et al. ( 2015 ) for field experiments to investigate such a link.
Thanks to cloud and parallelization technologies and the associated scalability of computing power (Bekkerman et al. 2011 ), algorithm runtimes are becoming less relevant. However, especially for real-time use, it should be evaluated which algorithms and underlying datasets exhibit an appropriate relationship between the targeted forecasting accuracy, the expected benefit, and the required computing power.
Recommendations concerning the algorithms and techniques can be derived (Urbanke et al. 2015 ), and a generic implementation framework was developed (Fuchs and Lutz 2021 ). However, from a business perspective, no contributions could be found regarding the actual implementation of real-time forecasting systems, the interventions involved, and their impact on consumer behavior or profit (also see proposal P5). In addition, the implementations of such systems need to be analyzed concerning the cost-effectiveness of the required investments.
5.3 Research proposal P3: cross-industry and multiple dataset studies
Many publications rely on a single data set from a specific industry or retailer. Only a few compare several retailers (e.g., Cui et al. 2020 ). Studies including and comparing different countries are missing, which is especially interesting since legal regulations for returns vary. For example, in contrast to the U.S., citizens within the EU are granted a 14-day right of withdrawal for distance selling purchases. Footnote 4 Although in most developed countries, liberal and broadly comparable returns policies are standard in practice due to competitive pressure, the generalizability of the results is frequently limited. One remedy for this problem is to use multiple data sets from different retailers (e.g., electronics vs. jewelry, Shang et al. 2020 ). Admittedly, it is challenging to simultaneously collaborate with several retailers and to combine different data sets, due to reasons of preserving corporate privacy and synchronizing various data sources. Nevertheless, research needs to draw conclusions from single data points, as well as logically replicate or falsify those results by integrating more data points to find patterns of similarities and differences, either within or cross-study (Hamermesh 2007 ). Therefore, we suggest that future studies acquire industry-related datasets from several retailers at once or replicate existing studies, which aligns with the aim and scope of Management Review Quarterly (Block and Kuckertz 2018 ). Cross-industry or cross-country manuscripts, which go beyond the mere assertion of an industry-agnostic approach (Hofmann et al. 2020 ) and jointly investigate data from several sectors, would promise an additional gain in knowledge and could be less challenging from a privacy perspective.
5.4 Research proposal P4: extended study of relevant predictors in forecasting applications
Although not the main focus of this review, predictors of consumer returns are especially interesting for marketing and e-commerce research, for example, regarding preventive measures for avoiding returns. In the past, many consumer return papers highlighted single aspects or a limited selection of return drivers or preventive measures employed but rarely attempted to model return behavior as comprehensively as possible. However, the latter is the very objective of returns forecasting, which is why the findings on influencing factors in articles with a forecasting focus tend to be more holistic, although not sufficiently complete (Hachimi et al. 2018 ). Some return reasons named in the literature (e.g., Stöcker et al. 2021 ) have not yet been included in forecasting approaches, and vice versa, only a part of the influencing factors investigated could be mapped to a return reason categorization. The reason categories assigned (Sect. 4.4 , Table 6 ) still contain some uncertainty. For example, a customer’s product return history may reflect the general returning behavior of a customer to some extent, while it can not be ruled out that repeated logistical problems caused the returns. Product attributes may reflect information gaps that consumers can only assess after physically inspecting the product, whereas product price–frequently cited and influential product attribute—is only related to information gaps when considering the price-performance ratio (Stöcker et al. 2021 ). Technical information about the web browser or device used by the customer is difficult to categorize, as it may reflect behavioral (impulse-driven mobile shopping) as well as informational (small display with few visible information) aspects. The payment method chosen by a customer, for example, could not be linked to one of the reason categories.
This reasoning should serve as a basis for linking forecasting predictors and return reasons more closely in the future. For example, the respective relative weighting of return drivers is more likely to be obtained considering as many factors involved as possible, minimizing the unexplained variation. From the reviewed literature, we extracted 18 different return predictor categories. For instance, seven papers (Cui et al. 2020 ; Fu et al. 2016 ; Ketzenberg et al. 2020 ; Li et al. 2018 , 2019 ; Urbanke et al. 2015 , 2017 ) integrated more than five predictor categories. But even though some papers integrate more than 5,000 features for automated feature selection (Ketzenberg et al. 2020 ), there are still combinations of input variable categories that have not been investigated and, more importantly, interpreted yet. Therefore, we call for more comprehensive research on return predictors and their interpretation, including associated preventive return measures, in the context of return forecasting.
5.5 Research proposal P5: descriptive case studies and business implementations surveys
This review identified a lack of publications regarding the actual benefit and the diffusion of consumer returns forecasting systems in different scopes and industries, building on the papers presenting return forecasting frameworks. In 2013, less than half of German retailers analyzed the likelihood of returns (Pur et al. 2013 ). Most of those who did were using naïve approaches that might be outperformed by the models presented in this review. Still, we do not know the status quo regarding the degree of adoption and implementation of forecasting systems for consumer returns in e-commerce firms (e.g., see Mentzer and Kahn 1995 for sales forecasting systems), country-specific and internationally.
Furthermore, the impact of return forecasting practices on company performance should be examined not only based on modeling, but on retrospective data (e.g., see Zotteri and Kalchschmidt 2007 for a similar study on demand forecasting practices in manufacturing). A possible hypothesis to examine might be that accuracy measures like RMSE or precision/recall and subsequently even the choice of the most accurate machine learning algorithm (e.g., see Asdecker and Karl 2018 ) are less relevant from a business perspective: (1) No algorithm clearly outperforms all other algorithms, and (2) the correlation between technical indicators and business value is unstable (Leitch and Tanner 1991 ). Methodologically, implementations of consumer returns forecasting in e-commerce should thus be surveyed and analyzed with multivariate statistical methods to examine critical factors and circumstances of return forecasting systems – similar to publications on reverse logistics performance (Agrawal and Singh 2020 ).
5.6 Research proposal P6: holistic forward and backward forecasting framework for e-tailers
Some publications present frameworks for forecasting returns (Fuchs and Lutz 2021 ). Nevertheless, in the past, forecasting in retail and especially e-commerce commonly focused more on demand (Micol Policarpo et al. 2021 ) than returns. Current approaches for demand forecasting try to predict individual purchase intentions based on click-stream data, online session attributes, and customer history (e.g., Esmeli et al. 2021 ). Our systematic approach could not identify any paper that connects and integrates both directions in e-commerce forecasting, neither conceptual (frameworks) nor with a quantitative or case-study-like approach. Nevertheless, first implementations of return predictions in inventory management are presented (e.g., Goedhart et al. 2023 ). Subsequently, similar to Goltsos et al. ( 2019 ), we call for research addressing both demand and return uncertainties by providing a holistic forecasting framework in the context of e-commerce.
6 Conclusion
To date, no systematic literature review has undertaken an in-depth exploration of the topic of forecasting consumer returns in the e-commerce context. Previous reviews have primarily focused on product returns forecasting within the broader context of reverse logistics or closed-loop supply chain management (Agrawal et al. 2015 ; Ambilkar et al. 2021 ; Hachimi et al. 2018 ). Regrettably, the interdisciplinary nature of this subject has often been overlooked, also neglecting the inclusion of results from information systems research.
The review first aims to provide an overview of the existing literature (Kraus et al. 2022 ) on forecasting consumer returns. The findings confirm that this once novel topic has significantly evolved in recent years. Consequently, this review is timely in examining current gaps and establishing a robust foundation for future research, which forms a second goal of systematic reviews (Kraus et al. 2022 ). The current body of work encompasses various aspects from different domains, including marketing, operations management/research, and information systems research, highlighting the interdisciplinary nature of e-commerce analytics and research. As a result, future studies can find suitable publication outlets in domain-specific as well as methodologically oriented journals and conferences.
Scientifically, the algorithms and predictors investigated in previous research serve as a foundational reference for subsequent publications and informed decisions regarding research design, ensuring that specific predictors and techniques are not overlooked. Researchers can utilize this review and the research framework developed as a structuring guide, e.g., regarding relevant publications on already examined algorithms or predictors.
Managerially, the extended taxonomy for machine learning in e-commerce (Micol Policarpo et al. 2021 ) can serve as a guideline for implementing forecasting systems for consumer returns. This review classifies possible prediction purposes, allowing businesses to apply them based on their respective challenges. Exploring the most frequently used predictors reveals the data that must be collected for the respective purposes. This review also offers valuable insights into data (pre-)processing and highlights popular algorithms. Furthermore, frameworks are outlined that support the design and implementation phase of such forecasting systems, supporting analytical purposes or enabling direct interventions during the online shopping process flow. As an exemplary and promising application, return policies could be personalized (Abbey et al. 2018 ) by identifying opportunistic or fraudulent basket compositions or high-returning customers, thereby reducing unwanted returns (Lantz and Hjort 2013 ).
Finally, a limitation of this review is the exclusion of forecasting algorithms for end-of-use returns, which could potentially be applicable to forecasting shorter-term retail consumer returns. However, the closed-loop supply chain and reverse logistics literature has been systematically excluded. Hence, future reviews could synthesize previous reviews on reverse logistics forecasting with the more detailed findings presented in this paper.
The use of Google Scholar for systematic scientific information search is controversely discussed (e.g., Halevi et al. 2017 ) due to the missing quality control and indexing guidelines, as well as limited advanced search options. But as an additional database for an initial search, the wide coverage of this search system can enrich the results.
External citations according to Google Scholar, which is preferable for citation tracking over controlled databases (Halevi et al. 2017 ).
Other literature also describes a counteracting effect of a reduced price due to lowered quality expectations or a higher perceived value of the “deal” itself (e.g., Sahoo et al. 2018 ).
It should be noted that the relevance of the forecasting topic depends on the maturity of the e-commerce sector. In most developing countries, B2C e-commerce is comparatively young and consumer returns are not yet a common phenomenon, which is why research on return forecasts is relatively insignificant for these countries.
References
Abbey JD, Ketzenberg ME, Metters R (2018) A more profitable approach to product returns. MIT Sloan Manag Rev 60(1):71–74
Google Scholar
Abdulla H, Ketzenberg ME, Abbey JD (2019) Taking stock of consumer returns: a review and classification of the literature. J Oper Manag 65(6):560–605. https://doi.org/10.1002/joom.1047
Article Google Scholar
Agrawal S, Singh RK (2020) Forecasting product returns and reverse logistics performance: structural equation modelling. MEQ 31(5):1223–1237. https://doi.org/10.1108/MEQ-05-2019-0109
Agrawal S, Singh RK, Murtaza Q (2015) A literature review and perspectives in reverse logistics. Resour Conserv Recycl 97:76–92. https://doi.org/10.1016/j.resconrec.2015.02.009
Ahmed F, Samorani M, Bellinger C, Zaiane OR (2016) Advantage of integration in big data: feature generation in multi-relational databases for imbalanced learning. In: Proceedings of the 4th IEEE international conference on big data, pp 532–539. https://doi.org/10.1109/BigData.2016.7840644
Ahsan K, Rahman S (2016) An investigation into critical service determinants of customer to business (C2B) type product returns in retail firms. Int Jnl Phys Dist Log Manage 46(6/7):606–633. https://doi.org/10.1108/IJPDLM-09-2015-0235
Akter S, Wamba SF (2016) Big data analytics in e-commerce: a systematic review and agenda for future research. Electron Markets 26(2):173–194. https://doi.org/10.1007/s12525-016-0219-0
Alfonso V, Boar C, Frost J, Gambacorta L, Liu J (2021) E-commerce in the pandemic and beyond. BIS Bulletin 36
Ambilkar P, Dohale V, Gunasekaran A, Bilolikar V (2021) Product returns management: a comprehensive review and future research agenda. Int J Prod Res. https://doi.org/10.1080/00207543.2021.1933645
Asdecker B (2015) Returning mail-order goods: analyzing the relationship between the rate of returns and the associated costs. Logist Res 8(1):1–12. https://doi.org/10.1007/s12159-015-0124-5
Asdecker B, Karl D (2018) Big data analytics in returns management–are complex techniques necessary to forecast consumer returns properly? In: Proceedings of the 2nd international conference on advanced research methods and analytics, Valencia, pp 39–46. https://doi.org/10.4995/CARMA2018.2018.8303
Asdecker B, Karl D, Sucky E (2017) Examining drivers of consumer returns in e-tailing with real shop data. In: Proceedings of the 50th Hawaii international conference on system sciences (HICSS). https://doi.org/10.24251/HICSS.2017.507
Bandara K, Shi P, Bergmeir C, Hewamalage H, Tran Q, Seaman B (2019) Sales Demand forecast in e-commerce using a long short-term memory neural network methodology. In: Gedeon T, Wong KW, Lee M (eds) Neural information processing: proceedings of the 26th international conference on neural information processing, 1st edn., vol 11955, pp 462–474. https://doi.org/10.1007/978-3-030-36718-3_39
Barbosa MW, La Vicente AdC, Ladeira MB, de Oliveira MPV (2018) Managing supply chain resources with big data analytics: a systematic review. Int J Log Res Appl 21(3):177–200. https://doi.org/10.1080/13675567.2017.1369501
Bekkerman R, Bilenko M, Langford J (2011) Scaling up machine learning. In: Proceedings of the 17th ACM SIGKDD international conference tutorials, p 1. https://doi.org/10.1145/2107736.2107740
Bernon M, Cullen J, Gorst J (2016) Online retail returns management. Int J Phys Distrib Logist Manag 46(6/7):584–605. https://doi.org/10.1108/IJPDLM-01-2015-0010
Block J, Kuckertz A (2018) Seven principles of effective replication studies: strengthening the evidence base of management research. Manag Rev Q 68(4):355–359. https://doi.org/10.1007/s11301-018-0149-3
Breiman L, Friedman JH, Olshen RA, Stone CJ (1984) Classification and regression trees. Wadsworth & Brooks/Cole Advanced Books & Software, Monterey, CA
Cirqueira D, Hofer M, Nedbal D, Helfert M, Bezbradica M (2020) Customer purchase behavior prediction in e-commerce: a conceptual framework and research Agenda. In: Ceci M, Loglisci C, Manco G, Masciari E, Raś Z (eds) New frontiers in mining complex patterns, vol 11948. Springer, Cham, pp 119–136. https://doi.org/10.1007/978-3-030-48861-1_8
Chapter Google Scholar
Clottey T, Benton WC (2014) Determining core acquisition quantities when products have long return lags. IIE Trans 46(9):880–893. https://doi.org/10.1080/0740817X.2014.882531
Cook SC, Yurchisin J (2017) Fast fashion environments: consumer’s heaven or retailer’s nightmare? Int J Retail Distrib Manag 45(2):143–157. https://doi.org/10.1108/IJRDM-03-2016-0027
Cui H, Rajagopalan S, Ward AR (2020) Predicting product return volume using machine learning methods. Eur J Oper Res 281(3):612–627. https://doi.org/10.1016/j.ejor.2019.05.046
Dalecke S, Karlsen R (2020) Designing dynamic and personalized nudges. In: Chbeir R, Manolopoulos Y, Akerkar R, Mizera-Pietraszko J (eds) Proceedings of the 10th international conference on web intelligence, mining and semantics. ACM, New York, pp 139–148. https://doi.org/10.1145/3405962.3405975
De P, Hu Y, Rahman MS (2013) Product-oriented web technologies and product returns: an exploratory study. Inf Syst Res 24(4):998–1010. https://doi.org/10.1287/isre.2013.0487
de Brito MP, Dekker R, Flapper SDP (2005) Reverse logistics: a review of case studies. In: Klose A, Fleischmann B (eds) Distribution logistics, vol 544. Springer. Berlin, Heidelberg, pp 243–281
Denyer D, Tranfield D (2009) Producing a systematic review. In: Buchanan DA, Bryman A (eds) The Sage handbook of organizational research methods. Sage, Thousand Oaks, CA, pp 671–689
Difrancesco RM, Huchzermeier A, Schröder D (2018) Optimizing the return window for online fashion retailers with closed-loop refurbishment. Omega 78:205–221. https://doi.org/10.1016/j.omega.2017.07.001
Diggins MA, Chen C, Chen J (2016) A review: customer returns in fashion retailing. In: Choi T-M (ed) Analytical modeling research in fashion business. Springer, Singapore, pp 31–48. https://doi.org/10.1007/978-981-10-1014-9_3
Ding Y, Xu H, Tan BCY (2016) Predicting product return rate with “tweets”. In: Proceedings of the 20th Pacific asia conference on information systems
Drechsler S, Lasch R (2015) Forecasting misused e-commerce consumer returns. In: Logistics management: proceedings of the 9th conference “Logistikmanagement”. Cham, pp 203–215.
Duong QH, Zhou L, Meng M, van Nguyen T, Ieromonachou P, Nguyen DT (2022) Understanding product returns: a systematic literature review using machine learning and bibliometric analysis. Int J Prod Econ 243:108340. https://doi.org/10.1016/j.ijpe.2021.108340
Esmeli R, Bader-El-Den M, Abdullahi H (2021) Towards early purchase intention prediction in online session based retailing systems. Electron Markets 31(3):697–715. https://doi.org/10.1007/s12525-020-00448-x
Fernández-Delgado M, Cernadas E, Barro S, Amorim D (2014) Do we need hundreds of classifiers to solve real world classification problems? J Mach Learn Res 15(1):3133–3181
Fisher RA (1936) The use of multiple measurements in taxonomic problems. Ann Eugen 7(2):179–188. https://doi.org/10.1111/j.1469-1809.1936.tb02137.x
Frei R, Jack L, Brown S (2020) Product returns: a growing problem for business, society and environment. IJOPM 40(10):1613–1621. https://doi.org/10.1108/IJOPM-02-2020-0083
Frei R, Jack L, Krzyzaniak S-A (2022) Mapping product returns processes in multichannel retailing: challenges and opportunities. Sustainability 14(3):1382. https://doi.org/10.3390/su14031382
Fu Y, Liu G, Papadimitriou S, Xiong H, Li X, Chen G (2016) Fused latent models for assessing product return propensity in online commerce. Decis Support Syst 91:77–88. https://doi.org/10.1016/j.dss.2016.08.002
Fuchs K, Lutz O (2021) A stitch in time saves nine–a meta-model for real-time prediction of product returns in ERP systems. In: Proceedings of the 29th european conference on information systems
Ge D, Pan Y, Shen Z-J, Di Wu, Yuan R, Zhang C (2019) Retail supply chain management: a review of theories and practices. J Data Manag 1:45–64. https://doi.org/10.1007/s42488-019-00004-z
Goedhart J, Haijema R, Akkerman R (2023) Modelling the influence of returns for an omni-channel retailer. Eur J Oper Res 306(3):1248–1263. https://doi.org/10.1016/j.ejor.2022.08.021
Goltsos TE, Ponte B, Wang SX, Liu Y, Naim MM, Syntetos AA (2019) The boomerang returns? Accounting for the impact of uncertainties on the dynamics of remanufacturing systems. Int J Prod Res 57(23):7361–7394. https://doi.org/10.1080/00207543.2018.1510191
Govindan K, Bouzon M (2018) From a literature review to a multi-perspective framework for reverse logistics barriers and drivers. J Clean Prod 187:318–337. https://doi.org/10.1016/j.jclepro.2018.03.040
Hachimi HEL, Oubrich M, Souissi O (2018) The optimization of reverse logistics activities: a literature review and future directions. In: Proceedings of the 5th IEEE international conference on technology management, operations and decisions, Piscataway, NJ, pp 18–24. https://doi.org/10.1109/ITMC.2018.8691285
Halevi G, Moed H, Bar-Ilan J (2017) Suitability of Google Scholar as a source of scientific information and as a source of data for scientific evaluation—review of the Literature. J Informet 11(3):823–834. https://doi.org/10.1016/j.joi.2017.06.005
Hamermesh DS (2007) Viewpoint: Replication in economics. Can J of Econ 40(3):715–733. https://doi.org/10.1111/j.1365-2966.2007.00428.x
Hastie T, Tibshirani R, Friedman JH (2017) The elements of statistical learning: data mining, inference, and prediction. Springer, New York, NY
Heilig L, Hofer J, Lessmann S, Voß S (2016) Data-driven product returns prediction: a cloud-based ensemble selection approach. In: Proceedings of the 24th european conference on information systems
Hess JD, Mayhew GE (1997) Modeling merchandise returns in direct marketing. J Direct Market 11(2):20–35. https://doi.org/10.1002/(SICI)1522-7138(199721)11:2<20:AID-DIR4>3.0.CO;2-#
Hevner A, March S, Park J, Ram S (2004) Design science in information systems research. MIS Q 28(1):75. https://doi.org/10.2307/25148625
Hofmann A, Gwinner F, Fuchs K, Winkelmann A (2020) An industry-agnostic approach for the prediction of return shipments. In: Proceedings of the 26th Americas conference on information systems, pp 1–10
Hong Y, Pavlou PA (2014) Product fit uncertainty in online markets: nature, effects, and antecedents. Inf Syst Res 25(2):328–344. https://doi.org/10.1287/isre.2014.0520
Imran AA, Amin MN (2020) Predicting the return of orders in the e-tail industry accompanying with model interpretation. Procedia Comput Sci 176:1170–1179. https://doi.org/10.1016/j.procs.2020.09.113
Jabareen Y (2009) Building a conceptual framework: philosophy, definitions, and procedure. Int J Qual Methods 8(4):49–62. https://doi.org/10.1177/160940690900800406
John S, Shah BJ, Kartha P (2020) Refund fraud analytics for an online retail purchases. J Bus Anal 3(1):56–66. https://doi.org/10.1080/2573234X.2020.1776164
Joshi T, Mukherjee A, Ippadi G (2018) One size does not fit all: predicting product returns in e-commerce platforms. In: Proceedings of the 10th IEEE/ACM international conference on advances in social networks analysis and mining, pp 926–927. https://doi.org/10.1109/ASONAM.2018.8508486
Kaiser D (2018) Individualized choices and digital nudging: multiple studies in digital retail channels. Karlsruher Institut für Technologie (KIT). https://doi.org/10.5445/IR/1000088341
Karl D, Asdecker B (2021) How does the Covid-19 pandemic affect consumer returns: an exploratory study. In: Proceedings of the 50th european marketing academy conference, vol 50
Karl D, Asdecker B, Feddersen-Arden C (2022) The impact of displaying quantity scarcity and relative discounts on sales and consumer returns in flash sale e-commerce. In: Proceedings of the 55th hawaii international conference on system sciences. https://doi.org/10.24251/HICSS.2022.556
Ketzenberg ME, Abbey JD, Heim GR, Kumar S (2020) Assessing customer return behaviors through data analytics. J Oper Manag 66(6):622–645. https://doi.org/10.1002/joom.1086
Kraus S, Breier M, Lim WM, Dabić M, Kumar S, Kanbach D, Mukherjee D, Corvello V, Piñeiro-Chousa J, Liguori E, Palacios-Marqués D, Schiavone F, Ferraris A, Fernandes C, Ferreira JJ (2022) Literature reviews as independent studies: guidelines for academic practice. Rev Manag Sci 16(8):2577–2595. https://doi.org/10.1007/s11846-022-00588-8
Lantz B, Hjort K (2013) Real e-customer behavioural responses to free delivery and free returns. Electron Commer Res 13(2):183–198. https://doi.org/10.1007/s10660-013-9125-0
Leitch G, Tanner JE (1991) Economic forecast evaluation: profits versus the conventional error measures. Am Econ Rev 81(3):580–590
Li X, Zhuang Y, Fu Y, He X (2019) A trust-aware random walk model for return propensity estimation and consumer anomaly scoring in online shopping. Sci China Inf Sci 62(5). https://doi.org/10.1007/s11432-018-9511-1
Li J, He J, Zhu Y (2018) E-tail product return prediction via hypergraph-based local graph cut. In: Proceedings of the 24th ACM sigkdd international conference on knowledge discovery & data mining, New York, NY, pp 519–527. https://doi.org/10.1145/3219819.3219829
Melacini M, Perotti S, Rasini M, Tappia E (2018) E-fulfilment and distribution in omni-channel retailing: a systematic literature review. Int Jnl Phys Dist Log Manage 48(4):391–414. https://doi.org/10.1108/IJPDLM-02-2017-0101
Mentzer JT, Kahn KB (1995) Forecasting technique familiarity, satisfaction, usage, and application. J Forecast 14(5):465–476. https://doi.org/10.1002/for.3980140506
Micol Policarpo L, da Silveira DE, da Rosa RR, Antunes Stoffel R, da Costa CA, Victória Barbosa JL, Scorsatto R, Arcot T (2021) Machine learning through the lens of e-commerce initiatives: an up-to-date systematic literature review. Comput Sci Rev 41:100414. https://doi.org/10.1016/j.cosrev.2021.100414
Miles MB, Huberman AM, Saldaña J (2020) Qualitative data analysis: A methods sourcebook. Sage, Los Angeles
National Retail Federation/Appriss Retail (2023) Consumer returns in the retail industry 2022. https://nrf.com/research/2022-consumer-returns-retail-industry . Accessed 23 May 2023
Ni J, Neslin SA, Sun B (2012) Database submission the ISMS durable goods data sets. Mark Sci 31(6):1008–1013. https://doi.org/10.1287/mksc.1120.0726
Page MJ, McKenzie JE, Bossuyt PM, Boutron I, Hoffmann TC, Mulrow CD, Shamseer L, Tetzlaff JM, Akl EA, Brennan SE, Chou R, Glanville J, Grimshaw JM, Hróbjartsson A, Lalu MM, Li T, Loder EW, Mayo-Wilson E, McDonald S, McGuinness LA, Stewart LA, Thomas J, Tricco AC, Welch VA, Whiting P, Moher D (2021) The PRISMA 2020 statement: an updated guideline for reporting systematic reviews. Syst Rev 10:89. https://doi.org/10.1186/s13643-021-01626-4
Pandya R, Pandya J (2015) C5.0 algorithm to improved decision tree with feature selection and reduced error pruning. IJCA 117(16):18–21. https://doi.org/10.5120/20639-3318
Petropoulos F, Apiletti D, Assimakopoulos V, Babai MZ, Barrow DK, Ben Taieb S, Bergmeir C, Bessa RJ, Bijak J, Boylan JE, Browell J, Carnevale C, Castle JL, Cirillo P, Clements MP, Cordeiro C, Cyrino Oliveira FL, de Baets S, Dokumentov A, Ellison J, Fiszeder P, Franses PH, Frazier DT, Gilliland M, Gönül MS, Goodwin P, Grossi L, Grushka-Cockayne Y, Guidolin M, Guidolin M, Gunter U, Guo X, Guseo R, Harvey N, Hendry DF, Hollyman R, Januschowski T, Jeon J, Jose VRR, Kang Y, Koehler AB, Kolassa S, Kourentzes N, Leva S, Li F, Litsiou K, Makridakis S, Martin GM, Martinez AB, Meeran S, Modis T, Nikolopoulos K, Önkal D, Paccagnini A, Panagiotelis A, Panapakidis I, Pavía JM, Pedio M, Pedregal DJ, Pinson P, Ramos P, Rapach DE, Reade JJ, Rostami-Tabar B, Rubaszek M, Sermpinis G, Shang HL, Spiliotis E, Syntetos AA, Talagala PD, Talagala TS, Tashman L, Thomakos D, Thorarinsdottir T, Todini E, Trapero Arenas JR, Wang X, Winkler RL, Yusupova A, Ziel F (2022) Forecasting: theory and practice. Int J Forecast 38(3):705–871. https://doi.org/10.1016/j.ijforecast.2021.11.001
Polikar R (2006) Ensemble based systems in decision making. IEEE Circuits Syst Mag 6(3):21–45. https://doi.org/10.1109/mcas.2006.1688199
Potdar A, Rogers J (2012) Reason-code based model to forecast product returns. Foresight 14(2):105–120. https://doi.org/10.1108/14636681211222393
Pur S, Stahl E, Wittmann M, Wittmann G, Weinfurtner S (2013) Retourenmanagement im Online-Handel–das Beste daraus machen: Daten, Fakten und Status quo. Ibi Research, Regensburg
Rajasekaran V, Priyadarshini R (2021) An e-commerce prototype for predicting the product return phenomenon using optimization and regression techniques. In: Singh M, Tyagi V, Gupta PK, Flusser J, Ören T, Sonawane VR (eds) Advances in computing and data sciences: proceedings of the 5th international conference on advances in computing and data sciences, 1st edn, vol 1441, pp 230–240. https://doi.org/10.1007/978-3-030-88244-0_22
Ravitch SM, Riggan M (2017) Reason and rigor: how conceptual frameworks guide research. Sage, Los Angeles, London, New Delhi, Singapore, Washington DC
Ren S, Chan H-L, Siqin T (2020) Demand forecasting in retail operations for fashionable products: methods, practices, and real case study. Ann Oper Res 291(1–2):761–777. https://doi.org/10.1007/s10479-019-03148-8
Rezaei M, Cribben I, Samorani M (2021) A clustering-based feature selection method for automatically generated relational attributes. Ann Oper Res 303(1–2):233–263. https://doi.org/10.1007/s10479-018-2830-2
Rogers DS, Lambert DM, Croxton KL, García-Dastugue SJ (2002) The returns management process. Int J Log Manag 13(2):1–18. https://doi.org/10.1108/09574090210806397
Röllecke FJ, Huchzermeier A, Schröder D (2018) Returning customers: the hidden strategic opportunity of returns management. Calif Manage Rev 60(2):176–203. https://doi.org/10.1177/0008125617741125
Sahoo N, Dellarocas C, Srinivasan S (2018) The impact of online product reviews on product returns. Inf Syst Res 29(3):723–738. https://doi.org/10.1287/isre.2017.0736
Samorani M, Ahmed F, Zaiane OR (2016) Automatic generation of relational attributes: an application to product returns. In: Proceedings of the 4th IEEE international conference on big data, pp 1454–1463
Santoro G, Fiano F, Bertoldi B, Ciampi F (2019) Big data for business management in the retail industry. MD 57(8):1980–1992. https://doi.org/10.1108/MD-07-2018-0829
Shaharudin MR, Zailani S, Tan KC (2015) Barriers to product returns and recovery management in a developing country: investigation using multiple methods. J Clean Prod 96:220–232. https://doi.org/10.1016/j.jclepro.2013.12.071
Shang G, McKie EC, Ferguson ME, Galbreth MR (2020) Using transactions data to improve consumer returns forecasting. J Oper Manag 66(3):326–348. https://doi.org/10.1002/joom.1071
Srivastava SK, Srivastava RK (2006) Managing product returns for reverse logistics. Int Jnl Phys Dist Log Manage 36(7):524–546. https://doi.org/10.1108/09600030610684962
Stock JR, Mulki JP (2009) Product returns processing: an examination of practices of manufacturers, wholesalers/distributors, and retailers. J Bus Logist 30(1):33–62. https://doi.org/10.1002/j.2158-1592.2009.tb00098.x
Stöcker B, Baier D, Brand BM (2021) New insights in online fashion retail returns from a customers’ perspective and their dynamics. J Bus Econ 91(8):1149–1187. https://doi.org/10.1007/s11573-021-01032-1
Sweidan D, Johansson U, Gidenstam A (2020) Predicting returns in men’s fashion. In: Proceedings of the 14th international fuzzy logic and intelligent technologies in nuclear science conference, pp 1506–1513. https://doi.org/10.1142/9789811223334_0180
Thaler RH, Sunstein CR (2009) Nudge: Improving decisions about health, wealth and happiness. Penguin
Tibben-Lembke RS, Rogers DS (2002) Differences between forward and reverse logistics in a retail environment. Supp Chain Mnagmnt 7(5):271–282. https://doi.org/10.1108/13598540210447719
Tibshirani R (1996) Regression shrinkage and selection via the lasso. J Roy Stat Soc: Ser B (Methodol) 58(1):267–288. https://doi.org/10.1111/j.2517-6161.1996.tb02080.x
Toktay LB, van der Laan EA, de Brito MP (2004) Managing product returns: the role of forecasting. In: Dekker R, Fleischmann M, Inderfurth K, van Wassenhove LN (eds) Reverse logistics. Springer, Berlin, Heidelberg, pp 45–64. https://doi.org/10.1007/978-3-540-24803-3_3
Toktay LB, Wein LM, Zenios SA (2000) Inventory management of remanufacturable products. Manage Sci 46(11):1412–142. https://doi.org/10.1287/mnsc.46.11.1412.12082
Tranfield D, Denyer D, Smart P (2003) Towards a methodology for developing evidence-informed management knowledge by means of systematic review. Br J Manag 14(3):207–222. https://doi.org/10.1111/1467-8551.00375
Uman LS (2011) Systematic reviews and meta-analyses. J Can Acad Child Adolesc Psychiatry 20(1):57–59
Urbanke P, Kranz J, Kolbe L (2015) Predicting product returns in e-commerce: the contribution of mahalanobis feature extraction. In: Proceedings of the 14th international conference on computer and information science
Urbanke P, Uhlig A, Kranz J (2017) A customized and interpretable deep neural network for high-dimensional business data–evidence from an e-commerce application. In: Proceedings of the 38th international conference on information systems
Vakulenko Y, Shams P, Hellström D, Hjort K (2019) Service innovation in e-commerce last mile delivery: mapping the e-customer journey. J Bus Res 101:461–468. https://doi.org/10.1016/j.jbusres.2019.01.016
vom Brocke J, Simons A, Niehaves B, Reimer K, Plattfaut R, Cleven A (2009) Reconstructing the giant: on the importance of rigour in documenting the literature search process. In: Proceedings of the 17 th european conference on information systems
von Zahn M, Bauer K, Mihale-Wilson C, Jagow J, Speicher M, Hinz O (2022) The smart green nudge: reducing product returns through enriched digital footprints and causal machine learning. SSRN J. https://doi.org/10.2139/ssrn.4262656
Walsh G, Möhring M (2017) Effectiveness of product return-prevention instruments: empirical evidence. Electron Mark 27(4):341–350. https://doi.org/10.1007/s12525-017-0259-0
Walsh G, Möhring M, Koot C, Schaarschmidt M (2014) Preventive product returns management systems–a review and model. In: Proceedings of the 22nd european conference on information systems
Webster J, Watson RT (2002) Analyzing the past to prepare for the future: writing a literature review. MIS Q 26(2):xiii–xxiii
Winklhofer H, Diamantopoulos A, Witt SF (1996) Forecasting practice: a review of the empirical literature and an agenda for future research. Int J Forecast 12(2):193–221. https://doi.org/10.1016/0169-2070(95)00647-8
Wirth R, Hipp J (2000) CRISP-DM: towards a standard process model for data mining. In: Proceedings of the 4th international conference on the practical applications of knowledge discovery and data mining, vol 1, pp 29–40
Zhao X, Hu S, Meng X (2020) Who should pay for return freight in the online retailing? Retailers or consumers. Electron Commer Res 20(2):427–452. https://doi.org/10.1007/s10660-019-09360-9
Zhu Y, Li J, He J, Quanz BL, Deshpande A (2018) A local algorithm for product return prediction in e-commerce. In: Proceedings of the 27th international joint conference on artificial intelligence, pp 3718–3724. https://doi.org/10.24963/ijcai.2018/517
Zotteri G, Kalchschmidt M (2007) Forecasting practices: empirical evidence and a framework for research. Int J Prod Econ 108(1–2):84–99. https://doi.org/10.1016/j.ijpe.2006.12.004
Download references
Open Access funding enabled and organized by Projekt DEAL. The authors have not disclosed any funding.
Author information
Authors and affiliations.
Chair of Operations Management and Logistics, University of Bamberg, Feldkirchenstr. 21, 96052, Bamberg, Germany
You can also search for this author in PubMed Google Scholar
Corresponding author
Correspondence to David Karl .
Ethics declarations
Conflict of interest.
The authors declare that no funds, grants, or other support were received during the preparation of this manuscript. The authors have no relevant financial or non-financial interests to disclose. The data that support the findings of this study are available from the corresponding author upon request.
Additional information
Publisher's note.
Springer Nature remains neutral with regard to jurisdictional claims in published maps and institutional affiliations.
Appendix: Author-centric content summary (with focus on forecasting issues)
1.1 journal publications.
Hess and Mayhew ( 1997 ) describe a forecasting approach, taking the example of a direct marketer for apparel with a lenient consumer return policy (free returns anytime). The analysis can plausibly be applied to a general retailer, although return time windows are somewhat different. A regression approach and a hazard model are compared. The regression approach itself is split into an OLS estimation of return timing (with poor fit) and a logit model of return propensities, which is in turn used for the split function of the box-cox-hazard approach for estimating the probability of a return over time. The accuracy was measured by fit statistics regarding the absolute deviation from the actual cumulative return proportion, with the split-hazard model outperforming the regression model. Besides price, the importance of fit of the respective product is used as a predictor.
Potdar and Rogers ( 2012 ) propose a method using reason codes combined with consumer behavior data for forecasting returns volume in the consumer electronics industry, aiming at the retailer stage as well as the preceding supply chain stages. The subject of their study is an offline retailer, which allows generalization for e-tailers due to a similar return policy (14 days free returns with no questions asked). In a multi-step approach, the authors are using essential statistical methods (moving averages, correlations, and linear regression), but use sophisticated domain and product knowledge like product features or price in relation to past return numbers, aiming to rank different competing products regarding their quality, and to predict the volume of returns for a given product for each given period of time.
Fu et al. ( 2016 ) derive a framework for the forecasting of product- and consumer-specific return propensities, i.e., the return propensity for individual purchases. Their study is directed at online shopping and is evaluated using the data from an online cosmetic retailer selling via Taobao.com. The predictors are categorized into inconsistencies in the buying and in the shipping phase of a transaction. A latent factor model is introduced for return propensities capturing differences between expectations and performance. This model is extended by product (e.g., warranty) and customer information (e.g., gender, credit score). The model is based on conditional probabilities, and an iterative expectation–maximization approach derives its parameters. MAE and RMSE, precision/recall, and AUC metrics assess the forecast accuracy. As benchmark models, two matrix factorization models and two memory-based models (historical consumer or product return rates) are compared, while the proposed model outperforms the references. Furthermore, this model allows identifying various return reasons, e.g., return abuse and fraud.
Building on the work of Fu et al. ( 2016 ), Li et al. ( 2019 ) investigate underlying reasons for consumer returns, taking the example and data of an online cosmetic retailer via Taobao.com. They examine the customers’ return propensity for product types, aiming at detecting abnormal returns suspecting abuse. Different from purchase decisions, they find customer profile data to be more important predictors for return decisions than product information or transaction details. The authors detect “selfish” or “fraud” consumers based on this rationale. For estimating return propensities for a given consumer and product, they calculate the return behavior depending on the return decision of similar consumers (“trust network”) and the amount of trust in these other consumers. MAE and precision-recall-measures are used to assess the prediction of different random walk models. The employed trust-based random walk model outperforms the other models on most indicators, building the basis for anomaly detection of consumers to cluster them into groups (honest/selfish/fraud) and individually address the return issues of these groups.
Although the paper from Cui et al. ( 2020 ) aims at product return forecasts from the perspective of the manufacturer, their case can be generalized for classic e-tailers, as the manufacturer is responsible for the return handling in their scenario—a task often performed by the retailer. They used a comprehensive data set from an automotive accessories manufacturer aiming to forecast return volume for sales channels and different products. The observed return rates lower than 1% are uncommonly low, and therefore the results must be interpreted with caution. First, a hierarchical OLS regression step-by-step incorporates up to 40 predictors regarding sales, time, product type, sales channel, and product details, including return history. The full model shows a significantly increased performance measured by a more than 50% decrease of MSE, which was used as the primary performance measure. Interestingly, relatively small differences in model quality (R 2 ) led to overproportional changes in the MSE. Using a machine-learning approach for predictor selection (“LASSO”), another MSE reduction of about 10% was achieved. Data Mining approaches (random forest, gradient boosting) could not outperform the LASSO approach. Forecasting performance was strongly dependent on the variation of the data. The two best predictors for return volume were past sales volume and lagged return statistics. The authors were wondering about the importance of lagged return information, failing to acknowledge that this predictor includes the consumer reaction to detailed product information, which has not been a significant predictor.
Ketzenberg et al. ( 2020 ) segment customers and target detecting the small number of abusive returners, as these are unprofitable for the retailer and generate significant losses over a long time. In general, high-returning customers are usually more profitable. The data used for this study is from a department store retailer with various product groups in the assortment. Predictors are transactional data and customer attributes. For classification, different algorithms like logit, Support Vector Machines (SVM), Random Forests (RF), Neural Networks (NN) are used in combination with different shrinkage methods like LASSO, ridge regression, and elastic net. Random Forests and especially Neural Networks outperform the other algorithms, assessed by sensitivity, precision, and AUC. In conclusion, a low rate of false positives could assure retailers of using abuse detection systems.
Shang et al. (Shang et al. 2020 ) developed a predict-aggregate (P-A) model adaptable both for retailers and manufacturers for forecasting return volume in a continuous timeframe, in contrast to commonly used aggregate-predict (A-P) models. Instead of aggregating data first (i.e., sales volume and returns volume), they first aggregate product-specific return probabilities and then aggregate the purchases by addition of the individual probabilities. As predictors, they only use timestamps and lagged return information. They tune and assess their models on two datasets from an offline electronics and an online jewelry retailer. ARIMA and lagged return models known from end-of-life forecasting (de Brito et al. 2005 ) are used as benchmarks, using RMSE as an assessment criterion. The authors show that even a basic version of their approach outperforms the benchmark models in almost all observed cases by up to 19%, though using only lagged returns and timestamps as input. Different extensions, e.g., including more predictor variables, can easily be integrated and are shown to further improve the forecasting performance.
John et al. ( 2020 ) try to predict the rare event of return fraud from customer representatives that make use of exactly knowing the e-commerce company’s return policy framework and buying and returning items fraudulently. Therefore, predictors range from transaction details to customer service agent attributes. A penalized likelihood logit model was chosen by the authors and was evaluated by precision and recall, focussing on maximizing recall and minimizing false negatives. The most important predictors were communication type and reason for interaction.
The paper by Rezaei et al. ( 2021 ) introduces a new algorithm to automatically select attributes from high-dimensional databases for forecasting purposes. As a demonstration sample, they use simulated data as well as the publicly available ISMS Durable Goods dataset (Ni et al. 2012 ) for consumer electronics. The results are assessed by AUC, precision, recall, and f1-score. They compare different configurations. For the simulated data, LASSO as shrinkage method generally works best, outperforming RF and BaggedTrees. For real-world data, based on a forecast with a logit model, they show that the proposed selection algorithm performs similar or better compared to LASSO, SVM, and RF, while the complexity of the chosen variables is lower.
1.2 Conference publications
Urbanke et al. ( 2015 ) describe a decision support system to better direct return-reducing interventions at e-commerce purchases with highly likely returns. They compare different approaches for extracting input variables for return propensity forecasting. They use a large dataset from a fashion e-tailer, aiming to reduce the input variables regarding consumer profile, product profile, and basket information from over 5,000 binary variables to 10 numeric variables by different algorithms (e.g., principal component analysis, non-negative matrix factorization, etc.). The results are then used to predict return propensities with a wide variety of state-of-the-art algorithms (AdaBoost, CART, ERT, GB, LDA, LR, RF, SVM), thus also revealing both feature selection and prediction performance. The proposed Mahalanobis feature extraction algorithm used as input for AdaBoost outperforms all other combinations presented, while interestingly, a logit model with all original inputs delivers relatively precise forecasts.
Building on some parts of this study, the paper of Urbanke et al. ( 2017 ) presents a return decision forecasting approach and aims at two targets, (1) high predictive accuracy and (2) interpretability of the model. Based on real-world data of a fashion and sports e-tailer, they first hand-craft 18 input variables and then use NN to extract more features and compare this approach to other feature extraction algorithms based on different forecasting algorithms. For assessment, they measure correlations between out-of-sample-predictions and class labels and AUC. The best performing classifier was AdaBoost, while the contribution of NN-based feature extraction shows interpretability as well as superior predictive performance.
Ahmed et al. ( 2016 ) focus on the automatic aggregation and integration of different data sources to generate input variables (features). They use return forecasting just as an exemplary classification problem for their data preparation approach, using various ML algorithms, e.g., RF, NN, DT-based algorithms, to detect returned purchases of an electronics retailer. Based on AUC measure, the results of their GARP-approach are superior to not using aggregations while generating an extensive amount of features with no pruning approach. In general, SVM and RF work best in combination with the proposed GARP approach. The data is based on the publicly available ISMS durable goods data sets (Ni et al. 2012 ).
A similar group of authors published another paper (Samorani et al. 2016 ), again using the aforementioned ISMS dataset as an example for data preparation and automatic attribute generation. Besides forecasting performance, in this paper, they want to generate knowledge about important return predictors; e.g., a higher price is associated with more returns, but only as long price levels are below a 1,500$ threshold. AUC is used to assess different levels of data integration, confirming that overfitting might happen when too many attributes are used.
Heilig et al. ( 2016 ) describe a Forecasting Support System (FSS) to predict return decisions in a real environment. First, they compare different forecasting approaches for data from a fashion e-tailer, assessed by AUC and accuracy metrics. The ensemble selection approach outperforms all other classifiers, with RF being the closest competitor. Computational times grow exponentially when using more data. Based on these results, they secondly describe a cloud framework for implementing such ensemble models for live use in a real shop environment.
Ding et al. ( 2016 ) present an approach to predict the daily return rate of an e-commerce company based on sentiment analysis of tweets regarding this company in the categories of news, experience, products, and service. Therefore, they use sophisticated text mining technologies, while the forecasting approach of an econometric vector autoregression is more or less common. The emotion of posts regarding different variables (news, product, service) impacts the returns rate negatively, while the emotion of purchasing experience impacts it positively, showing that the prediction accuracy enhances through classifying social network posts.
Drechsler and Lasch ( 2015 ) aim at forecasting the volume of fraudulent returns in e-commerce over several periods of time. They present different approaches multiplying the sales volume and the relative return rate, the first referring to Potdar and Rogers ( 2012 ), estimating the rate of misused returns directly based on time-lag-specific return rates. In a second approach referring to Toktay et al. ( 2000 ), they estimate the overall returns rate and multiply it by the time-specific ratio of fraudulent returns. The return rates were forecasted by moving averages and exponential smoothing techniques. Assessment criteria for performance comparison based on simulated data were MAE, MAPE, and TIC, showing the first approach to be superior, but both methods are not sufficiently robust. Therefore, the authors include further time-specific information (like promotions or special events, which could foster fraudulent returns) in a model using a Holt-Winters approach, showing superior performance. All of the models are highly dependent on low fluctuation in return rates, showing a shortcoming of these more or less naive forecasting techniques.
Asdecker and Karl ( 2018 ) compare the performance of different algorithms for forecasting binary return decisions: logit, linear discriminant analysis, neuronal networks, and a decision-tree-based algorithm (C5.0). Their analysis is based on the data of a fashion e-tailer, including price, consumer information, and shipment information (number of articles in shipment, delivery time). For the assessment of different algorithms, they use the total absolut error (TAE) and relative error. An ensemble learning approach performs best and similar to the C5.0 algorithm. Though, differences in performance are relatively small, while only about 68% of return decisions are forecasted correctly.
Li et al. ( 2018 ) propose a hypergraph representation of historical purchase and return information combined with a random-walk-based local graph cut algorithm to forecast return decisions on order (basket) level as well as on product level. By this, they aim to detect the underlying return causes. They use data from two omnichannel fashion e-tailers from the US and Europe to assess the performance of their approach, using precision/recall/F 0.5 /AUC metrics while arguing that precision is the most important indicator for targeted interventions. Three similarity-based approaches (e.g., a k-Nearest Neighbor model) are used as reference. The proposed approach performs best regarding AUC, precision, and F 0.5 metrics.
Zhu et al. ( 2018 ) developed a weighted hybrid graph algorithm representing historical customer behavior and customer/product similarity, combined with a random-walk-based algorithm for predicting customer/product combinations that will be returned. They report an experiment based on data from a European fashion e-tailer suffering from return rates as high as 50%. For assessment, they use precision, recall, and F 0.5 metrics. Their approach is superior to two reference competitors (similarity-based and a bipartite graph algorithm). As predictors, they use product similarities and historical return information, while their approach can be enriched with detailed customer attributes.
Joshi et al. ( 2018 ) model the return decisions based on the data of an Indian e-commerce company, especially dealing with returns for apparel due to fit issues. In a two-step approach, they first model return probabilities using concepts from network science based on a customer’s historical purchase and return decisions, and secondly use a SVM implementation with return probabilities as a single input to classify for the return decision. Assessed by F 1 /precision/recall scores, their approach is superior to a reference random-walk baseline model.
Imran and Amin ( 2020 ) compare different forecasting algorithms (XGBoost, CatBoost, LightGBM, TabNet) for return classification based on the data of a general e-commerce retailer from Bangladesh. As input variables, only order attributes, including payment method and order medium, are used. For evaluation, they use metrics like true negative rate, false-positive rate, false-negative rate, true positive rate, AUC, F 2 -score, precision, and accuracy. In the end, they chose TPR, AUC, and F 2 -score, claiming that misclassifying high return probability objects were the first thing to avoid. According to these metrics, TabNet as a deep learning algorithm outperforms the other models. The most important predictors were payment method, order location, and promotional orders.
As returns are most prominent in fashion e-commerce, most of the forecasting papers take this industry as an example, as forecasting models are more precise when returns are more frequent. Hofmann et al. ( 2020 ) develop a more generalized order-based return decision forecasting approach, appropriate for different industries and suitable also for low return rates. For their analysis, they use a dataset from a german technical wholesaler with a return rate as low as 5%. Input variables were just basket composition and return information. For assessment, they used precision and recall metrics. RF did not perform superior to a statistical baseline approach, nor with oversampling as data preparation, to deal with the group imbalance. The DART algorithm makes use of the group imbalance correction by random oversampling. In general, gradient boosting performs best with imbalanced groups, also without oversampling, but forecasting quality is lower than with more specialized forecasting approaches as described for fashion. Furthermore, results were more accurate on basket level than on single-item level.
Fuchs and Lutz ( 2021 ) use Design Science Research (DSR) principles to design a meta-model for the real-time prediction of returns. The goal is to influence consumer decisions by triggering a feedback system based on the basket composition and its return probability. For forecasting, which is not the primary focus of their paper, they build upon a gradient boosting model taken from existing research (Hofmann et al. 2020 ) and describe possible implementations into an ERP system regarding asynchronous communication requirements and possible architecture.
The paper by Sweidan et al. ( 2020 ) evaluates the forecasting performance of a random forest model for a shipment-based return decision, using real-world data of a fashion e-tailer. For their model, they use customer (e.g., lagged return rate) and order information as inputs. They find that predictions with high confidence are very precise (i.e., low false-positive rate). Thus, interventions can be targeted at such orders already when the items are in the consumers’ basket without risk of a misdirected intervention. For assessment, accuracy, AUC, precision, recall and specificity are used. Regarding the predictors, they note that selection orders (a product in different sizes) are the best predictor for order-based returns.
Rajasekaran and Priyadarshini ( 2021 ) develop a metaheuristic for forecasting the product-based return probabilities. In the first step, they determine return probabilities based on product feedback, time, and product attributes regarding manufacturer return statistics. Secondly, they compare different algorithms (OLS, RF, Gradient Boosting) by MAE, MSE, and RMSE metrics. Interestingly, linear regression performs best in all metrics, but no explanation and a misinterpretation regarding the best algorithm are given.
Rights and permissions
Open Access This article is licensed under a Creative Commons Attribution 4.0 International License, which permits use, sharing, adaptation, distribution and reproduction in any medium or format, as long as you give appropriate credit to the original author(s) and the source, provide a link to the Creative Commons licence, and indicate if changes were made. The images or other third party material in this article are included in the article's Creative Commons licence, unless indicated otherwise in a credit line to the material. If material is not included in the article's Creative Commons licence and your intended use is not permitted by statutory regulation or exceeds the permitted use, you will need to obtain permission directly from the copyright holder. To view a copy of this licence, visit http://creativecommons.org/licenses/by/4.0/ .
Reprints and permissions
About this article
Karl, D. Forecasting e-commerce consumer returns: a systematic literature review. Manag Rev Q (2024). https://doi.org/10.1007/s11301-024-00436-x
Download citation
Received : 24 August 2023
Accepted : 12 April 2024
Published : 21 May 2024
DOI : https://doi.org/10.1007/s11301-024-00436-x
Share this article
Anyone you share the following link with will be able to read this content:
Sorry, a shareable link is not currently available for this article.
Provided by the Springer Nature SharedIt content-sharing initiative
- Consumer returns
- Product returns
- Forecasting
- Literature review
JEL classification
- Find a journal
- Publish with us
- Track your research
- Account Menu
- Editorial Board
- Editorial Policy
- Statement of Ethics
- Statement of Open Access
- Copyright Policies
- Complaints Procedure
- Submit Manuscript Online
- Submission Guidelines
- Call For Papers
- Publication Fee
- About JBRMR
- Conferences
--> Journal of Business and Retail Management Research

About the journal
The Journal of Business and Retail Management Research (JBRMR) is proud to have a growing international reputation as a scholarly journal. It sets out to ensure that academics from across the world have a credible vehicle for disseminating their research papers. Those seeking fresh and original articles, particularly in relation to the retail sector will find the journal an invaluable resource. The JBRMR is published two times a year and is a journal of the Centre for Business & Economic Research (CBER). The Editors and Editorial Board of the JBRMR are committed to championing original academic papers that demonstrate method, academic rigor and integrity. Such a journal has a small, yet significant role in helping to enrich a wider understanding of a raft of issues of a macro and micro economic nature, and specialises in business and retail management. The JBRMR is already proving to be a respected platform for scholarly endeavour and as....
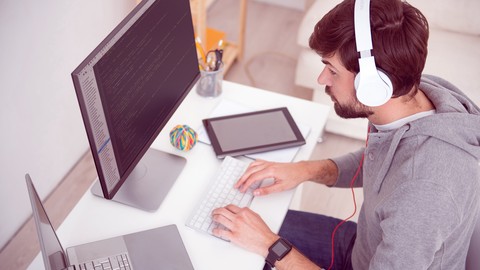
Submission Help
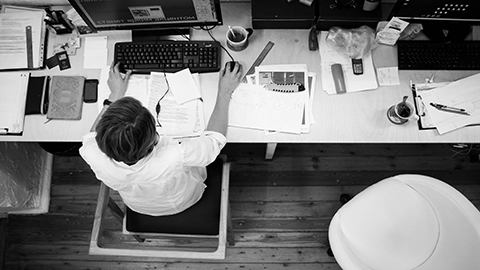
Step By Step procedure
- Submit Manuscript
- Review status of paper
- Camera ready submission
- Payment for Publication
- Publised Manuscript Online & Print
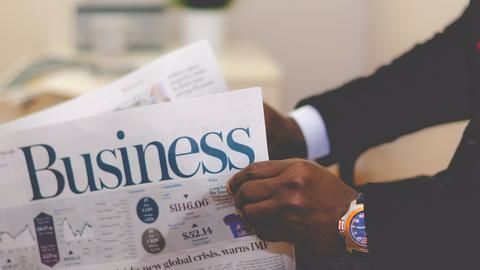
News and Headline
- 13th International Conference on Restructuring of the Global Economy (ROGE)-Promoting Sustainability
- 9th International Conference on Globalisation, Entrepreneurship, and Emerging Economies (ICGEEE)-2024
Latest articles
Indexed with.
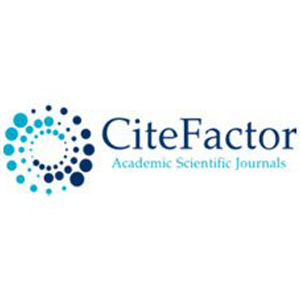
- Open Access
- Journal Indexing
- Copyright Policy
- Submission Guideline

© 2024 JBRMR : Journal of Business and Retail Management Research. All Rights Reserved.


An official website of the United States government
The .gov means it’s official. Federal government websites often end in .gov or .mil. Before sharing sensitive information, make sure you’re on a federal government site.
The site is secure. The https:// ensures that you are connecting to the official website and that any information you provide is encrypted and transmitted securely.
- Publications
- Account settings
Preview improvements coming to the PMC website in October 2024. Learn More or Try it out now .
- Advanced Search
- Journal List
- Elsevier - PMC COVID-19 Collection
- PMC10239906

Analysis of retail sector research evolution and trends during COVID-19
Brij b. gupta.
a International Center for AI and Cyber Security Research and Innovations & Department of Computer Science and Information Engineering, Asia University, Taiching, Taiwan
b Kyung Hee University, 26 Kyungheedae-ro, Dongdaemun-gu, Seoul, 02447, Korea
c Lebanese American University, Beirut, 1102, Lebanon
d Symbiosis Centre for Information Technology (SCIT), Symbiosis International University, Pune, India
e Center for Interdisciplinary Research at University of Petroleum and Energy Studies (UPES), Dehradun, India
Akshat Gaurav
f Ronin Institute, Montclair, NJ, USA
g University Center for Research & Development (UCRD), Chandigarh University, Chandigarh 140413, India
Prabin Kumar Panigrahi
h Indian Institute of Management Indore, India
Associated Data
Data will be made available on request.
The purpose of this study is to analysis the evolution of the retail sector during the COVID-19 period and to identify future research issues. Scopus databases were searched for articles published in English between 2020 and 2022 to discover current trends and concerns in the retail industry. A total of 1071 empirical and nonempirical studies were compiled as a result of the evaluation process. During the study period, the number of articles published in scientific journals increased exponentially, indicating that the research topic is still in the developmental phase. It also highlights the most important research trends, allowing numerous new research lines to be proposed via visual mapping of Thematic Maps. This study makes an important contribution to the field of the retail sector, providing a comprehensive overview of the field’s evolution and current status, as well as a comprehensive, synthesized, and organized summary of the various perspectives, definitions, and trends in the field.
1. Introduction
Societies and economies should take note of the COVID-19 virus as a warning, since both were caught off guard and now face serious consequences ( Lopes and Reis, 2021 , Chopra et al., 2022 , Srour et al., 2022 , Narayan et al., 2022 , Alowibdi et al., 2021 ). Globally, the current pandemic has had severe health, social, political, and economic consequences. To survive in this new reality, countries will need to implement new measures, policies, and habits ( Hall et al., 2021 , Bouncken et al., 2022 , Lee and Suh, 2022 ). As a result of increased food demand and the resulting strain on the supply chain, the retail sector has been forced to focus more on employee safety and health, improve hygiene in physical spaces, and better manage its workforce to ensure the well-being of the civilian population ( Trapp et al., 2022 , Rasmusen et al., 2022 , Vassiliades et al., 2022 ). In light of the fact that pandemics are likely to persist, this study aims is to determine the effects of the COVID-19 pandemic on the retail sector. It is also meant to serve as a pre-planning guide so that the industry can respond permanently to these scenarios and avert service closures. In this research, our main aim is to find the answer to the following questions:
- RQ1 What are the recent research themes, topics, and keywords related to the retail sector.
- RQ2 : Which countries are working to develop new theories and techniques for the retail sector.
- RQ3 : Who are the key researchers in the creation of novel approaches to the retail sector?
The rest of the paper is organized as follows: Section 2 documents the recent literature surveys. Section 3 explains the methodology; Section 4 analyzes the results. Section 5 presents the theoretical implications, and the conclusion is presented in Section 6 .
2. Literature review
This subsection discusses details about the latest survey conducted in the retail sector. Taking into account the proliferation of COVID-19 and the likely repercussions of its propagation, the authors in the review article ( Artemyeva et al., 2022 ) examine the process of e-commerce.
The author examines international online trade during COVID-19 ( Kofi Mensah and Simon Mwakapesa, 2021 ). In another review, the author says that the primary criterion for speeding up the performance of supply chains is ”Collaboration Efficiency”. In addition, ”Order Fulfillment” and ”Digital RSCs” are the most suitable resilient business methods to reduce long-term consequences ( Sharma et al., 2021 ).
The banking industry has long been seen as a leader in the use of new information and communication technologies in business operations. In this context, the authors of the paper ( Saprikis et al., 2022 ) try to answer the question “Why do people use mobile banking applications?” via an empirical investigation. For this study, the researchers looked at two groups of people: those who use m-banking applications, and those who don’t, and discover whether there are any differences or similarities in the variables that influence their decision to use or not use this sort of service.
According to our knowledge, none of the current evaluations have covered the retail sector under COVID-19 to its fullest extent. It is thus necessary for researchers to undertake a bibliometric analysis to evaluate the field’s state, identify the primary academic agents who are continually growing in this area, determine the important issues from present research as well as define future challenges for this field of study.
2.1. Latest business models for the retail sector
Recently many researchers proposed something different to improve the retail sector from the impact of COVID-19 ( Yu et al., 2020 , Tarhini et al., 2022 , Tan et al., 2021 , Chopra et al., 2022 ) using different techniques such as blockchain Martínez et al., 2022 , Stergiou et al., 2021 , Deveci et al., 2022 and machine learning ( Lv et al., 2022 , Gaurav et al., 2022 ). Some of the novel modes are as follows: The authors ( Vizuete-Luciano et al., 2022 ) propose a decision assistance system for grocery shop operators in Barcelona based on the Forgotten Effects Theory of the City’s 701 establishments. There are indirect impacts that can be determined by conducting an experiment like this one. Varied districts have different approaches to evaluating the study’s variables, as seen by these findings.
In recent years, there has been a surge in the use of the Omnichannel business model in recent years. In this investigation ( Piskunova, 2022 ) author analyze the omnichannel operations of merchants to propose a short-term omnichannel business strategy under the pressure of this economic crisis. Research shows that the COVID-19 situation has pushed the omnichannel retail paradigm forward. The researchers have outlined numerous step-by-step metrics in their study of the implementation process of the omnichannel strategy ( Mouawad et al., 2021 , Saab et al., 2021 ). The study includes retailers’ thoughts on new ways of doing business, which proposes how the concept of omnichannel development may be influenced by external influences, as well as offering opportunities for additional research in the development of the concept of omnichannel. This study provides a step-by-step strategy to implement an omnichannel business model for retail company managers.
Using FAHP and WASPAS methodologies, this research proposes a fuzzy MCDM-based solution to the problem ( Kao et al., 2022 ). We want to design a tool that may help a decision maker in a garment sector dealing with fuzzy environments choose a good supplier. In this case study, the suggested MCDM model is put into practice to illustrate its usefulness and viability. Due to the help of the model in achieving its desired results, an ideal garment industry supplier was found.
Cognitive similarity is a notion that the authors propose as the basis for the product recommendation system ( Krishnan and Nair, 2022 ). Collaborative filtering serves as the foundation for this hybrid recommendation approach. To ensure that the new approach is better than the old, it is compared to both the old and the new. In an additional study of research, the authors use a novel lifecycle data science approach. With the use of user behavior analytics and an RFM analysis technique, the suggested model predicts product sales and makes product suggestions to consumers ( Zhao and Keikhosrokiani, 2022 ). The suggested recommendation model gives excellent suggestions and sales combinations to increase sales and market response. New clients may also receive product recommendations from it. This study provide a highly practical and valuable case study of a company through a change in business model and provides guidance to others in a similar scenario.
Based on the constantly developing concept of “curbside pickup”, Ponis et al. Ponis et al. (2022) proposes a solution that is further strengthened using a Wi-Fi system and Augmented Reality. Retailers will be equipped with an easy-to-implement solution and will use one that will help them overcome the problems posed by COVID-19, a pandemic that has ravaged much of the world.
In another study, the authors address the issue of determining low-cost delivery routes in metropolitan regions for online purchases ( Cerrone et al., 2021 , Al Sobbahi and Tekli, 2022 ). The proposed system places the realistic 3D model and provides a virtual test experience using marker-based and marker-less methodologies ( Chavan et al., 2021 ). Despite the difficulties posed by COVID-19, companies will benefit from using this technology ( Koweyes et al., 2021 , Yang et al., 2022 ). Therefore, people will be able to keep their own space while yet interacting with one another via Augmented Reality (AR). The authors also proposed a similar concept in the proposed approach using cloud computing ( Van Ngoc and Xuan Canh, 2021 ).
2.2. Behavior analysis in retail sector
Many scholars employed state-of-the-art methodologies to examine consumer behavior with the aim of enhancing the retail industry. Several contemporary and inventive methods are currently available ( Paiva et al., 2022 , Mourad et al., 2020 , Haldma et al., 2021 ). Retailers may consider COVID-19 as an opportunity to build bike lanes and other forms of active transportation infrastructure to counteract the fact that fewer people are using public transit out of fear of infection.
The urban landscapes in London, Manchester, Newcastle and Liverpool are examined in this research article ( Cavada, 2022 ). This study uses case studies to investigate the effects of geographical isolation due to COVID-19. Some of the limitations of the metropolitan area, changes in sharing behavior, and gamification potential may be seen using this study’s data. The expected results will provide evidence-based scenarios for gamification systems.
3. Research methodology
A detailed literature study was conducted to determine the effect of COVID-19 on the retail sector. The PRISMA review method ( Page et al., 2021 ) was used to guide the review process. The following are the steps used in writing this paper: Selection of the database, modification of research criteria, coding of recovered material and evaluation of the information. Because the Web-of-Science database contains fewer indexed journals than Scopus ( Mongeon and Paul-Hus, 2014 ), this study uses the Scopus database as a sample to limit the chance of missing important articles throughout the search process.
3.1. Eligibility criteria
This research examined the evolution and trends in the retail industry during the COVID-19 pandemic. Publications published between 2019 and 2022 in English were included in the map to show the current state of research around the world.
3.2. Restrictions
Few papers were disqualified because they were irrelevant to the study’s fundamental objectives. For example, we restrict ourselves to researching articles written in English.
3.3. Data source
Using the Scopus bibliographic database, the data was gathered in December 2022. The following two keywords were included in the search strategy to answer the research question.
- • Retail
- • COVID-19
3.4. Search query selection
In order to get the information from Scopus database, we used the following query at different screening levels:
”(TITLE-ABS-KEY(retail) AND TITLE-ABS-KEY(”COVID-19”)) AND (LIMIT-TO (LANGUAGE,”English”))”
( TITLE-ABS-KEY ( retail ) AND TITLE-ABS-KEY (“COVID-19”)) AND (LIMIT-TO (LANGUAGE, “English”)) AND (LIMIT-TO (DOCTYPE, “cp”) OR LIMIT-TO (DOCTYPE, “ar”))
3.5. Tools used
R language was used to analyze the data. Science mapping research can be carried out using R language, which is based on the ( Cobo et al., 2011 ) science mapping analytic technique that allows longitudinal investigations. Another benefit of using this method is that it helps researchers discover connections and interactions between previously studied topics and new areas of study.
4. Results and discussion
Studies of an area’s bibliography may provide light on how the topic has developed and point to potential directions for future study. It provides a bird’s eye perspective of several facets of an area. This section is separated into two sub-sections for the sake of a more thorough study. An overview of scientific output over time is provided, as well as a breakdown by topic area and publication venue of the most widely cited publications, institutes, and authors. Furthermore, we examine the content findings to identify the most important trends in the growth of the retail sector.
The impact of COVID-19 on the retail industry is a significant field of study, as shown by Fig. 1 . The details of our database are represented in Fig. 2 . As represented in Fig. 2 , our dataset includes 1071 articles. Furthermore, more than 3735 unique authors published papers during this time; therefore, we can say that the impact of COVID-19 on the retail sector is an interesting topic because many authors were working on it.

Variation of Number of Papers.

Overview of Dataset.
In addition to the number of papers and authors, citations are also a good criteria for representing the productivity and importance of the topic. Since, this indicates that the research area is still fresh, and there is a scope of research in this area.
4.1. Analysis of source distribution
In this subsection, we give an analysis of the publication sources. To represent the productivity and impact of sources, we use the number of citations, the number of documents published, the h index, the g index and the m index as comparative variables. Therefore, the top 10 productive sources are represented in Table 1 . From Table 1 it is clear that the most productive source is JOURNAL OF RETAILING AND CONSUMER SERVICES with the highest number of citations. Other more popular and quantity-based journals are as follows: CANADIAN JOURNAL OF AGRICULTURAL ECONOMICS, INTERNATIONAL JOURNAL OF ENVIRONMENTAL RESEARCH AND PUBLIC HEALTH, JOURNAL OF BUSINESS RESEARCH, TIJDSCHRIFT VOOR ECONOMISCHE EN SOCIALE GEOGRAFIE, SUSTAINABILITY (SWITZERLAND), TRANSPORTATION RESEARCH INTERDISCIPLINARY PERSPECTIVES, PLOS ONE, SAFETY SCIENCE, JOURNAL OF SERVICE MANAGEMENT, JAMA NETWORK OPEN, and JOURNAL OF PUBLIC ECONOMICS .
Local source impact details.
Other than quality and quantity, the topic of interest is also a good criterion for the identification of the importance of a source. Fig. 3 represents this criterion using Sankey diagrams ( Riehmann et al., 2005 ). Visualizing the movement of energy or materials in networks and processes has historically been done with the use of Sankey diagrams. They depict quantitative information about flows, their interactions, and their transformations. Each node in a Sankey diagram is connected to other nodes through a weighted network, and each weighted node’s incoming weight is equal to its outgoing weights. The first, second map third row in Fig. 3 represents countries, keywords, and source, respectively. Therefore, this Sankey diagram representation can easily represent the core topics in which the respective source is publishing papers. From Fig. 3 , it is clear that sustainability is one of the most versatile journals because it covers a variety of topics. Fig. 4 represents the number of publications with respect to the source. Hence, it is clear from Fig. 4 that most of the articles related to the retail sectors are published in the MPDI journal followed by the LNCS springer.

Source and Keyword Plot.

Source variation according to total publication.
4.2. Analysis of authors and country distribution
In this subsection, we give statistical details about the authors who are actively working to study the impact of COVID-19 on the retail sector. There are many ways through which we can find the most productive authors in the respective field. One method is the classification of authors by the number of citations. The Fig. 5 represents the statics of the author. In Fig. 5(a) the authors are represented through the frequency of article factorization, and in Fig. 5(b) the authors most cited are represented. Therefore, from Fig. 5 , Fig. 5 it is clear that WANG J , KUMAR A , and LI Y , are the authors who actively work in the field of analyzing the effect of COVID-19 on the retail sector.

Authors Stastics.
Apart from the number of citations, frequency and reverence are also the variables via which the most renowned authors in a particular subject may be determined. This analysis is presented in Fig. 5 , Fig. 5 . Fig. 5(c) indicates a positive correlation between the progression of time and the level of interest in the respective research area. In 2020, only nine researchers were working in the field, but in 2021, more than 15 researchers started their research to analysis the effect of COVID-19 on the retail sector. This also shows that this research topic is still developing and there is a scope of research in this domain. Finally, Fig. 5(d) represents the working area of the authors, and this figure is constructed on the principles of Sankey diagrams. From Fig. 5(d) provides evidence that a majority of the authors have conducted research on various factors influencing the performance of the retail industry amidst the COVID-19 pandemic.
The distribution of researchers by nations is also a significant and beneficial component. This metric indicates the effectiveness of a country’s researchers. Fig. 6 represents the distribution of countries according to the total number of publications in the paper and the corresponding authors. From Fig. 6(a) the top countries according to the paper publication frequency the countries with the most published articles are:

Countries’ Scientific Production.
- • USA (913)
- • CHINA (297)
- • INDIA (279)
- • UK (270)
- • CANADA (171)
- • INDONESIA (113)
- • MALAYSIA (111)
- • ITALY (102)
Therefore, from Fig. 6(a) , we can say that USA researchers have been actively working in the field of the development of concepts for the retail sector for the past two years. The next important factor is the collaboration among the authors from different countries, which represents the productivity of a country. Fig. 6(b) represents the distribution of the corresponding authors and the nature of the paper (ie, single author (SCP) or multiauthor (MCP) paper).
4.3. Analysis of document distribution
In this subsection, we give details about the scientific distribution of the research papers. In the Scopus database, there are 1071 articles related to our study. However, not all the published papers are important and provide valuable information about the subject area. Therefore, to obtain the information of the article, we find highly cited articles related to the development of the retail sector during COVID-19 times. The details of this type of paper are presented in Table 2 . In Table 2 the papers are arranged according to total citations, average citations, and normalized citations.
Highly cited papers.
4.4. Analysis of trending research topics
We analyze the current research directions and trends in this subsection. First of all, we examine the distribution of the keywords of the paper. Keywords are a representation of the paper’s principal subject matter. Fig. 7(a) represents the frequency of keywords in our research database; In this figure, the frequency of the keyword dictates its size. According to the analysis, the most frequent keywords with occurrence frequency are

Trending Research Topic Identification.
- • COVID-19 (389)
- • human (213)
- • pandemic (191)
- • humans (182)
- • united states (126)
- • pandemics (110)
- • sales (110)
- • retailing (76)
- • epidemic (63)
- • electronic commerce (59)
- • workplace (58)
- • commerce (51)
- • public health (50)
These trending keywords give us an idea of the directions in which scholars are doing research. Therefore, we can say that ’ sales , retails stores ’ is the most common topic in the paper, and most researchers are doing research in this direction. IoT , sustainable development , AI , deep learning are some of the fields that are developing. This will help future researchers to find the direction of their research.
The next important technique for finding the trending topic is to group similar keywords using clustering and collaboration. Keyword collaboration is a technique for analyzing the relationship between different keywords. Fig. 7(b) represents the result of the clustering and collaboration among different keywords. Keyword collaboration is an important technique through which the relationship between different keywords can be analyzed. According to Fig. 7(b) there are four different clusters that contain the keywords:
- • Cluster 1: This cluster belongs to sales terms. These terms are: sales , commerce , decision making , and forecasting .
- • Cluster 2: This cluster belongs to the development in the retail sector. Important keywords in this cluster are: AI , COVID-19 , retail stores , deep learning , IoT , pandemic , supply chains , viruses , and sustainable development .
- • Cluster 3: This cluster represents the e-commerce industry. Important terms in this cluster are: e-commerce and COVID-19 pandemic
- • Cluster 4: This cluster represents the service sector terms. The important terms of this cluster are: service industry , consumer behavior , and digital transformation .
4.4.1. Thematic map of keywords
We used Co-word analyze to build the thematic diagrams for the keywords. Thematic map helps the user analyze the behavior of different topics in the research field ( Muñoz-Leiva et al., 2012 ). There are two parameters through which thematic maps are built ( Callon et al., 1991 ):
- • Centrality: This metric measures the degree of interconnectivity of a network. A concept’s centrality may be gauged by looking at how closely it is linked to other ideas. This number may be interpreted as an indicator of the relevance of a particular topic in the growth of the overall area of study under consideration. It is given by the following equations: C e n t r a l i t y ( c ) = 10 ∑ e h k (1) C e n t r a l i t y R a n k = r i c N (2) where, k a keyword belonging to the theme and h a keyword belonging to other themes. r is the r i c is the place of theme i in the theme cluster, and N is the total themes,
- • Density: It determines the inherent strength of the network. Density enables you to determine the degree to which all of the keywords in your subject are connected to one another. This variable may be used to track the theme’s progression. It is calculated by the following equations: D e n s i t y ( D ) = 100 ∑ e i j w (3) D e n s i t y R a n k = r i D N (4)
i and j denote the keyword of the theme, while w denotes the total number of keywords of the theme. r is the r i c is the place of the theme i in the theme group, and N is the total theme(see Fig. 7 ). The thematic diagram is created with the help of density rank and the centrality rank, as represented in Fig. 7(c) , Fig. 7(d) , and Fig. 7(e) . The thematic diagram is divided into four quadrants:
- • Motor Themes: In the thematic plot, these themes can be found in the upper right quadrant. All topics that are well established and vital to the organization of a study area are included in this quadrant.
- • Niche Themes: These themes are located in the thematic plot’s upper-left quadrant. All of these subjects have established internal connections but have insignificant outward connections and hence are of only minor relevance to the area.
- • Emerging Themes: The lower left quadrant of the diagram shows these themes. The themes of this quadrant have low density and low centrality, and they mostly reflect new or fading concepts.
- • Basic Themes: The lower right quadrant of the diagram shows these themes. Each and every one of the potential study topics that has been raised but has not yet been explored. As a result, this section focuses on universal and cross-cutting topics.
We break the time period into two subperiods to better understand the current trends and the progress of the study field. The details of the themes for each period are as follows:
- • First Period: In this period, there are three motor themes, two basic themes, and one emerging theme. The size of each circle depends on the citation values. From Fig. 7(d) , it is clear that in this period, sales is the main topic of research or in the development phase. Also, ’ service industry ’ and ’ IoT ’ are well-developed fields. Therefore, we can say that in the first phase, researchers are trying to develop new concepts to improve sales in the retail sector using the concepts of IoT and other smart devices.
- • Second Period: In this period, there are four basic themes, one motor theme, and two peripheral themes. It is represented by Fig. 7(e) . ’ Sales ’, ’ e-commerce ’, ’ behavioral analysis ’, and ’ sustainable development ’ are the basic themes. ’ COVID-19 ’ and ’ retail ’ are the motor themes with high centrality and density. Therefore, from the analysis of Fig. 7(e) , we can say that researchers are developing new concepts in the field of sales , e-commerce , sustainable development and behavioral analysis in the COVID-19 scenario for the retail sector.
- • Combine Analysis: Fig. 7(c) represents the general development of the research terms for the respective theme. There are three motor themes, three basic themes, and one niche theme. As can be seen in Fig. 7(c) , academics are using principles from artificial intelligence, deep learning, forecasting, the Internet of Things (IoT), and sustainable development to the study of e-Commerce, consumer behavior, the supply chain, and social networking.
In addition to the thematic map of keywords, research trends can also be analyzed by evaluating different keywords; Fig. 7(f) represents the keyword evaluation during the research period. The following facts can be determined from the evaluation of the keywords:
- • Researchers are using deep learning methods to address issues in the retail industry.
- • All of the anomaly-based approaches that were previously used to detect virus activity are now being used to analyze customer behavior.
- • Smart device ideas such as IoT are used in the e-commerce, retail, and sales sectors.
- • The retail business places a premium on the creation of ideas to increase sales.
5. Theoretical and practical implications
This section describes the observations made during the analysis. This paper’s primary objective is to document the research development in the retail sector due to COVID-19. Additionally, this research seeks to discover the most recent trends in the retail sector. We examine the work of experts from across the globe to assess the most recent research subjects and trends. From our research, we get the following observations:
- • The papers suggest that the pandemic has had a significant impact on online retail sales. Hwang et al. (2020) found that omnichannel retailers experienced an increase in online sales due to the closure of physical stores and the acquisition of new customers. Fihartini et al. (2021) found that consumers’ perceived health risk and positive perceptions of online retail ethics influenced their online shopping behavior during the pandemic. Dannenberg et al. (2020) found that the pandemic led to a general upswing in grocery trade, with disproportionately high growth in online grocery trade. Sayyida et al. (2021) found that webrooming and pure online shopping were the dominant shopping trends during the pandemic, with retail sales in physical stores exceeding 70% of total retail sales and retail e-commerce sales being less than 30% of total retail sales. Overall, the papers suggest that the pandemic has accelerated the shift towards online retail sales, but physical stores still play a significant role in retail sales.
- • The papers suggest that the pandemic has had a significant impact on retail employment. Kramer and Kramer (2020) discusses how the pandemic may change perceptions of the value and status of different occupations, resulting in changes in occupational supply and demand. Mack et al. (2021) found that workers in the transportation sector, which includes retail workers, were more likely to be unemployed due to the pandemic than workers in non-transportation industries. Izguttiyeva et al. (2021) notes that the pandemic has led to an increasing number of employers’ requirements for soft skills, which may affect the demand for retail workers. del Rio-Chanona et al. (2020) predicts that the pandemic will threaten a significant portion of the US economy’s GDP and jobs, with sectors such as entertainment, restaurants, and tourism facing large supply and demand shocks, which may affect retail employment.
- • The papers suggest that the pandemic has had a significant impact on the retail industry, with some sectors being affected more than others. Nanda et al. (2021) argues that the pandemic has accelerated the shift towards e-commerce and digitalization, which has led to changes in consumer behavior and the retail landscape. Lashgari and Shahab (2022) notes that the pandemic has intensified changes in customer preferences and the role of city centers, leading to more retailers adopting multichannel retailing and repositioning strategies. Sayyida et al. (2021) found that during the pandemic, consumers tended to use online channels to reduce face-to-face contact with marketers or other consumers, but physical stores still accounted for over 70% of total retail sales. Yang 2020 suggests that the pandemic has brought both challenges and opportunities to the retail industry. Overall, the papers suggest that the pandemic has accelerated changes in the retail industry, with e-commerce and digitalization becoming increasingly important, while physical stores are still relevant but may need to adapt to new consumer behaviors.
- • Based on the examination of scholarly articles, it can be posited that the global pandemic has exerted a substantial influence on consumer conduct. The study conducted by the authors ( Cox et al., 2020 ) revealed that households belonging to various income brackets reduced their expenditures during the initial months of the pandemic. However, it was observed that low-income households exhibited a more rapid recovery in their spending patterns. There were also large increases in liquid asset balances for households throughout the income distribution, with lower-income households contributing disproportionately to the aggregate increase in balances. Hesham et al. (2021) found that the pandemic increased the intention to buy among consumers of healthy foods, with women being more anxious about the pandemic than men and taking more precautions to avoid contamination. Finally, Baker et al. (2020) found that household consumption responded to the pandemic by radically altering typical spending across a number of major categories, with spending decreasing in the latter part of March except for food delivery and grocery spending.
- • It is also found that the pandemic has affected different demographics of consumers differently. Lee and Worthy (2021) found that age and race were related to changes in wellness-related behavior and lifestyle choices, with older respondents experiencing less change than younger respondents, and Whites and Asians reporting less change than Blacks or Hispanics. Crosta et al. (2021) found that changes in spending levels were associated with changes in consumer behavior, with necessities being predicted by anxiety and COVID-related fear, and non-necessities being predicted by depression. Bareja-Wawryszuk et al. (2022) found that there were clear differences in the nature of changes in consumer behavior between respondents from Turkey and Poland, with the purchasing habits of respondents in Turkey changing to a greater extent. Argyropoulou et al. (2023) found that the pandemic has impacted various aspects of consumer behavior in Greece, with online shopping and social media usage increasing during this period.
- • The papers suggest that the COVID-19 pandemic has had a significant impact on online shopping behavior. Alaimo et al. (2020) found that people who were already familiar with online grocery shopping and had a higher education level were more satisfied with the experience during the pandemic. Ecola et al. (2020) found that more Americans are shopping online and spending more money on online purchases, while those who lost employment during the pandemic spent less. Moon et al. (2021) analyzed the characteristics of consumers who used offline shopping channels during the pandemic and found that many aspects of daily life, including shopping, are now conducted online. Pollák and Konečný (2021) used Facebook data to analyze e-consumer behavior during the pandemic and found that the pandemic has caused changes in consumer behavior that are suitable for further research. Overall, the papers suggest that the pandemic has accelerated the shift towards online shopping and that this trend is likely to continue even after the pandemic ends.
- • According to the papers, the COVID-19 pandemic has exerted a substantial influence on the retail labor force. The research conducted by Khaled et al. (2020) revealed that the well-being of employees is positively associated with the performance of retailers. On the other hand, Alflayyeh et al. (2020) observed a significant surge in e-retailing during the pandemic, which has resulted in a change in customer preferences and purchasing patterns. Naseri et al. (2021) highlighted that the pandemic has caused a decrease in demand and business volume for the retail industry, leading to downsizing and a need for new approaches to shopping. Sayyida et al. (2021) found that consumers have shifted towards webrooming and pure online shopping during the pandemic, leading to changes in retail marketing strategies. Overall, the papers suggest that the pandemic has caused significant changes in the retail industry, with a shift towards online shopping and a need for retailers to prioritize employee well-being and adapt to new approaches to shopping.
6. Conclusion
Due to the COVID-19 pandemic, retail was in the midst of unprecedented change, largely driven by the effects of technology on customer behavior and retail processes. In this context, this paper aimed to analyze the change and development in the research field related to the retail sector. We statically compared and analyze different Scopus index papers. With the increase in the publication from 2020 to 2022 it is evident that many researchers are working to develop new technologies and theories to counter COVID-19 effects on the retail sector. The interest in retail is also visible in the contributions made by nations and institutions (the United States, China and India) of diverse origin. Additionally, the number of articles and citations has grown exponentially in the last two years, leading us to believe that retail research is a growing trend. Future research can include data from databases other than Scopus.
CRediT authorship contribution statement
Brij B. Gupta: Conceptualization, Formal analysis, writing, Supervision. Akshat Gaurav: Conceptualization, Formal analysis, Writing. Prabin Kumar Panigrahi: Conceptualization, writing, Supervision.
Biographies
Brij B. Gupta received the PhD degree from Indian Institute of Technology (IIT) Roorkee, India. In more than 16 years of his professional experience, he published over 400 papers in journals/conferences including 25 books and 09 Patents with over 15000citations. He has received numerous national and international awards including Canadian Commonwealth Scholarship (2009), Faculty Research Fellowship Award(2017), MeitY, GoI, IEEE GCCE outstanding and WIE paper awards and Best Faculty Award (2018 & 2019), NIT Kurukshetra, respectively. He is also selected in the 2021 and 2020 Stanford University’s ranking of the world’s top 2 also a visiting/adjunct professor with several universities worldwide. He is also an IEEE Senior Member (2017) and also selected as 2021 Distinguished Lecturer in IEEE CTSoc. Dr Gupta is also serving as Member-in-Large, Board of Governors, IEEE Consumer Technology Society (2022-204). Prof. Gupta is also leading IJSWIS, IJSSCI and IJCAC as Editor-in-Chief. Moreover, he is also serving as lead-editor of a Book Series with CRC and IET press. He also served as TPC members in more than 150 international conferences. Dr Gupta is also serving/served as Associate/Guest Editor of various journals and transactions. At present, Prof. Gupta is working as Director, International Center for AI and Cyber Security Research, Incubation and Innovations, and Full Professor with the Department of Computer Science and Information Engineering (CSIE), Asia University, Taiwan. His research interests include information security, Cyber physical systems, cloud computing, blockchain technologies, intrusion detection, AI, social media and networking.
Akshat Gaurav received the M.Tech degree in Computer Engineering (cyber security) from NIT Kurukshetra, India. His research interests include information security, cyber security, cloud computing, web security, intrusion detection, and computer network.
Prabin Kumar Panigrahi is a professor of Information Systems department at the Indian Institute of Management Indore, India. He earned his Ph.D. from Indian Institute of Technology, Kharagpur. His research interests include Emerging Technologies, Machine Learning, Text Mining of Vernacular Languages, Technology Adoption, e-Governance, e-Learning, e-Participation, Social Inclusion in Information Systems, and Business Value of Information Systems.
Data availability
- Abouk R., Heydari B. The immediate effect of COVID-19 policies on social-distancing behavior in the United States. Public Health Rep. 2021; 136 (2):245–252. doi: 10.1177/0033354920976575. [ PMC free article ] [ PubMed ] [ CrossRef ] [ Google Scholar ]
- Al Sobbahi R., Tekli J. Comparing deep learning models for low-light natural scene image enhancement and their impact on object detection and classification: overview, empirical evaluation, and challenges. Signal Process., Image Commun. 2022 [ Google Scholar ]
- Alaimo L.S., Fiore M., Galati A. How the Covid-19 pandemic is changing online food shopping human behaviour in Italy. Sustainability. 2020 [ Google Scholar ]
- Alflayyeh S., Haseebullah S., Belhaj F.A. The impact of coronavirus (COVID-19) pandemic on retail business in Saudi Arabia: A theoretical review. Eur. J. Mol. Clin. Med. 2020; 7 (1):3547–3554. [ Google Scholar ]
- Alowibdi J.S., Alshdadi A.A., Daud A., Dessouky M.M., Alhazmi E.A. Coronavirus pandemic (COVID-19): Emotional toll analysis on Twitter. Int. J. Semantic Web Inf. Syst. 2021; 17 (2):1–21. [ Google Scholar ]
- Argyropoulou M.D., Tzavara D., Argyropoulou R. Exploring how the Covid-19 pandemic has changed greek consumers’ habits. KnE Soc. Sci. 2023 [ Google Scholar ]
- Artemyeva M., Garina E., Kuznetsova S., Potashnik Y., Bezrukova N. Lecture Notes in Networks and Systems. 2022. Ecommerce surge as an element of a modern economy integration mechanism development; pp. 492–500. [ CrossRef ] [ Google Scholar ]
- Baker S.R., Farrokhnia R.A., Meyer S., Pagel M., Yannelis C. How does household spending respond to an epidemic? Consumption during the 2020 COVID-19 pandemic. Rev. Asset Pricing Stud. 2020 [ Google Scholar ]
- Bareja-Wawryszuk O., Pajewski T., Çakaröz K.M., Kavas B. Changes in consumer behavior during the COVID-19 pandemic: A comparative analysis between polish and turkish consumers. Sustainability. 2022 [ Google Scholar ]
- Bouncken R.B., Lapidus A., Qui Y. Organizational sustainability identity:‘new work’of home offices and coworking spaces as facilitators. Sustain. Technol. Entrepreneurship. 2022; 1 (2) [ Google Scholar ]
- Callon M., Courtial J.-P., Laville F. Co-word analysis as a tool for describing the network of interactions between basic and technological research: The case of polymer chemsitry. Scientometrics. 1991; 22 (1):155–205. [ Google Scholar ]
- Cavada M. Evaluate Space after Covid-19: Smart City Strategies for Gamification. Int. J. Hum.-Comput. Interaction. 2022 doi: 10.1080/10447318.2021.2012383. [ CrossRef ] [ Google Scholar ]
- Cerrone C., Cerulli R., Sciomachen A. Grocery distribution plans in urban networks with street crossing penalties. Networks. 2021; 78 (3):248–263. doi: 10.1002/net.22061. [ CrossRef ] [ Google Scholar ]
- Chavan V., Kamtankar S., Jagtap A., Patil B., Pakale S. 2021 IEEE 4th International Conference on Computing, Power and Communication Technologies, GUCON 2021. 2021. An Augmented Reality Application to Enhance the Shopping Experience of Electronic Appliances. [ CrossRef ] [ Google Scholar ]
- Chopra M., Singh S.K., Gupta A., Aggarwal K., Gupta B.B., Colace F. Analysis & prognosis of sustainable development goals using big data-based approach during COVID-19 pandemic. Sustain. Technol. Entrepreneurship. 2022; 1 (2) [ Google Scholar ]
- Cobo M.J., López-Herrera A.G., Herrera-Viedma E., Herrera F. An approach for detecting, quantifying, and visualizing the evolution of a research field: A practical application to the fuzzy sets theory field. J. Informetrics. 2011; 5 (1):146–166. [ Google Scholar ]
- Cox N., Ganong P., Noel P., Vavra J., Wong A., Farrell D., Greig F., Deadman E. Initial impacts of the pandemic on consumer behavior: Evidence from linked income, spending, and savings data. Brook. Pap. Econ. Act. 2020; 2020 :35–82. [ Google Scholar ]
- Cranfield J. Framing consumer food demand responses in a viral pandemic. Can. J. Agric. Econ. 2020; 68 (2):151–156. doi: 10.1111/cjag.12246. [ CrossRef ] [ Google Scholar ]
- Crosta A.D., Ceccato I., Marchetti D., Malva P.L., Maiella R., Cannito L., Cipi M., Mammarella N., Palumbo R., Verrocchio M.C., Palumbo R., Domenico A.D. Psychological factors and consumer behavior during the COVID-19 pandemic. PLoS ONE. 2021; 16 [ PMC free article ] [ PubMed ] [ Google Scholar ]
- Dannenberg P., Fuchs M., Riedler T., Wiedemann C. Digital transition by COVID-19 pandemic? The German food online retail. Tijdschrift Voor Economische En Soc. Geogr. 2020; 111 :543–560. [ PMC free article ] [ PubMed ] [ Google Scholar ]
- Deveci M., Pamucar D., Gokasar I., Köppen M., Gupta B.B. Personal mobility in metaverse with autonomous vehicles using Q-rung orthopair fuzzy sets based OPA-RAFSI model. IEEE Trans. Intell. Transp. Syst. 2022 [ Google Scholar ]
- Ecola L., Lu H., Rohr C. RAND; 2020. How Is COVID-19 Changing Americans’ Online Shopping Habits? [ Google Scholar ]
- Fihartini Y., Helmi A., Hassan M., Oesman Y.M. Perceived health risk, online retail ethics, and consumer behavior within online shopping during the COVID-19 pandemic. Innov. Mark. 2021 [ Google Scholar ]
- Forsythe E., Kahn L., Lange F., Wiczer D. Labor demand in the time of COVID-19: Evidence from vacancy postings and UI claims. J. Public Econ. 2020; 189 doi: 10.1016/j.jpubeco.2020.104238. [ PMC free article ] [ PubMed ] [ CrossRef ] [ Google Scholar ]
- Gaurav A., Gupta B.B., Panigrahi P.K. A comprehensive survey on machine learning approaches for malware detection in IoT-based enterprise information system. Enterp. Inf. Syst. 2022:1–25. [ Google Scholar ]
- Goddard E. The impact of COVID-19 on food retail and food service in Canada: Preliminary assessment. Can. J. Agricult. Econ. 2020; 68 (2):157–161. doi: 10.1111/cjag.12243. [ CrossRef ] [ Google Scholar ]
- Gray R. Agriculture, transportation, and the COVID-19 crisis. Can. J. Agricult. Econ. 2020; 68 (2):239–243. doi: 10.1111/cjag.12235. [ CrossRef ] [ Google Scholar ]
- Haischer M., Beilfuss R., Hart M., Opielinski L., Wrucke D., Zirgaitis G., Uhrich T., Hunter S. Who is wearing a mask? Gender-, age-, and location-related differences during the COVID-19 pandemic. PLoS ONE. 2020; 15 (10 October) doi: 10.1371/journal.pone.0240785. [ PMC free article ] [ PubMed ] [ CrossRef ] [ Google Scholar ]
- Haldma T., Dagiliene L., Varaniute V., Putter J., Laats K., Steinstrater M. 2021 IEEE International Conference on Technology and Entrepreneurship, ICTE 2021. 2021. Sustainability management in german and baltic retailing: communication patterns in Covid-19 circumstances. [ CrossRef ] [ Google Scholar ]
- Hall M., Prayag G., Fieger P., Dyason D. Beyond panic buying: consumption displacement and COVID-19. J. Serv. Manag. 2021; 32 (1):113–128. doi: 10.1108/JOSM-05-2020-0151. [ CrossRef ] [ Google Scholar ]
- Hesham F., Riadh H., Sihem N. What have we learned about the effects of the COVID-19 pandemic on consumer behavior? Sustainability. 2021; 13 :4304. [ Google Scholar ]
- Huynh T. Does culture matter social distancing under the COVID-19 pandemic? Saf. Sci. 2020; 130 doi: 10.1016/j.ssci.2020.104872. [ PMC free article ] [ PubMed ] [ CrossRef ] [ Google Scholar ]
- Hwang E.H., Nageswaran L., Cho S.-H. Impact of COVID-19 on omnichannel retail: Drivers of online sales during pandemic. SSRN Electron. J. 2020 [ Google Scholar ]
- Izguttiyeva K.Y., Tussupova L.A., Yeralina E. The impact of the pandemic on the labour market. Bull. “Turan” Univ. 2021 [ Google Scholar ]
- Kao J.-C., Wang C.-N., Nguyen V., Husain S. A fuzzy mcdm model of supplier selection in supply chain management. Intell. Autom. Soft Comput. 2022; 31 (3):1451–1466. doi: 10.32604/IASC.2022.021778. [ CrossRef ] [ Google Scholar ]
- Katrakazas C., Michelaraki E., Sekadakis M., Yannis G. A descriptive analysis of the effect of the COVID-19 pandemic on driving behavior and road safety. Transp. Res. Interdis. Perspect. 2020; 7 doi: 10.1016/j.trip.2020.100186. [ PMC free article ] [ PubMed ] [ CrossRef ] [ Google Scholar ]
- Khaled A.S.D., Alabsy N., Al-Homaidi E.A., Saeed A.M.M. The impact of the COVID-19 pandemic on retailer performance: empirical evidence from India. Innov. Mark. 2020 [ Google Scholar ]
- Kofi Mensah I., Simon Mwakapesa D. ACM International Conference Proceeding Series. 2021. Cross-Border E-Commerce Diffusion and Usage during the period of the COVID-19 Pandemic: A literature Review; pp. 59–65. [ CrossRef ] [ Google Scholar ]
- Koweyes J., Salloum T., Haidar S., Merhi G., Tokajian S. Covid-19 pandemic in lebanon: One year later, what have we learnt? Msystems. 2021; 6 (2):e00351–21. [ PMC free article ] [ PubMed ] [ Google Scholar ]
- Kramer A., Kramer K.Z. The potential impact of the Covid-19 pandemic on occupational status, work from home, and occupational mobility. J. Vocat. Behav. 2020; 119 :103442. [ PMC free article ] [ PubMed ] [ Google Scholar ]
- Kretchy I., Asiedu-Danso M., Kretchy J.-P. Medication management and adherence during the COVID-19 pandemic: Perspectives and experiences from low-and middle-income countries. Res. Soc. Admin. Pharmacy. 2021; 17 (1):2023–2026. doi: 10.1016/j.sapharm.2020.04.007. [ PMC free article ] [ PubMed ] [ CrossRef ] [ Google Scholar ]
- Krishnan R., Nair P. 2022. RFM-Based Customer Analysis and Product Recommendation System; pp. 159–164. [ CrossRef ] [ Google Scholar ]
- Laato S., Islam A., Farooq A., Dhir A. Unusual purchasing behavior during the early stages of the COVID-19 pandemic: The stimulus-organism-response approach. J. Retail. Consum. Serv. 2020; 57 doi: 10.1016/j.jretconser.2020.102224. [ CrossRef ] [ Google Scholar ]
- Lashgari Y.S., Shahab S. The impact of the COVID-19 pandemic on retail in city centres. Sustainability. 2022 [ Google Scholar ]
- Lee M.T., Suh I. Understanding the effects of environment, social, and governance conduct on financial performance: Arguments for a process and integrated modelling approach. Sustain. Technol. Entrepreneurship. 2022; 1 (1) [ Google Scholar ]
- Lee H., Worthy S.L. Changes in consumer wellness during the early weeks of the pandemic. J. Family Consum. Sci. 2021 [ Google Scholar ]
- Li J., Hallsworth A., Coca-Stefaniak J. Changing grocery shopping behaviours among Chinese consumers at the outset of the COVID-19 outbreak. Tijdschrift Voor Economische En Soc. Geogr. 2020; 111 (3):574–583. doi: 10.1111/tesg.12420. [ PMC free article ] [ PubMed ] [ CrossRef ] [ Google Scholar ]
- Lopes M., Reis J. 2021. the Influence of COVID-19 in Retail: A Systematic Literature Review; pp. 171–181. [ CrossRef ] [ Google Scholar ]
- Loske D. The impact of COVID-19 on transport volume and freight capacity dynamics: An empirical analysis in german food retail logistics. Transp. Res. Interdis. Perspect. 2020; 6 doi: 10.1016/j.trip.2020.100165. [ PMC free article ] [ PubMed ] [ CrossRef ] [ Google Scholar ]
- Lv L., Wu Z., Zhang L., Gupta B.B., Tian Z. An edge-AI based forecasting approach for improving smart microgrid efficiency. IEEE Trans. Ind. Inform. 2022; 18 (11):7946–7954. [ Google Scholar ]
- Mack E.A., Agrawal S., Wang S. The impacts of the COVID-19 pandemic on transportation employment: A comparative analysis. Transp. Res. Interdis. Perspect. 2021; 12 :100470. [ PMC free article ] [ PubMed ] [ Google Scholar ]
- Mahajan V., Cantelmo G., Antoniou C. Explaining demand patterns during COVID-19 using opportunistic data: a case study of the city of Munich. Eur. Transp. Res. Rev. 2021; 13 (1) doi: 10.1186/s12544-021-00485-3. [ CrossRef ] [ Google Scholar ]
- Martínez J.M.G., Carracedo P., Comas D.G., Siemens C.H. An analysis of the blockchain and COVID-19 research landscape using a bibliometric study. Sustain. Technol. Entrepreneurship. 2022; 1 (1) [ Google Scholar ]
- Mirza N., Rahat B., Naqvi B., Rizvi S. Impact of Covid-19 on corporate solvency and possible policy responses in the EU. Q. Rev. Econ. Finance. 2020 doi: 10.1016/j.qref.2020.09.002. [ PMC free article ] [ PubMed ] [ CrossRef ] [ Google Scholar ]
- Mongeon P., Paul-Hus A. The journal coverage of bibliometric databases: A comparison of Scopus and Web of Science. J. Cover. Web Sci. Scopus: A Comp. Anal. 2014; 10 Available Online. [ Google Scholar ]
- Moon J., Choe Y., Song H. Determinants of consumers’ online/offline shopping behaviours during the COVID-19 pandemic. Int. J. Environ. Res. Public Health. 2021; 18 [ PMC free article ] [ PubMed ] [ Google Scholar ]
- Mouawad P., Dubnov T., Dubnov S. Robust detection of COVID-19 in cough sounds. SN Comput. Sci. 2021; 2 (1):1–13. [ PMC free article ] [ PubMed ] [ Google Scholar ]
- Mourad A., Srour A., Harmanani H., Jenainati C., Arafeh M. Critical impact of social networks infodemic on defeating coronavirus COVID-19 pandemic: Twitter-based study and research directions. IEEE Trans. Netw. Serv. Manag. 2020; 17 (4):2145–2155. [ Google Scholar ]
- Muñoz-Leiva F., Viedma-del Jesús M.I., Sánchez-Fernández J., López-Herrera A.G. An application of co-word analysis and bibliometric maps for detecting the most highlighting themes in the consumer behaviour research from a longitudinal perspective. Qual. Quant. 2012; 46 (4):1077–1095. doi: 10.1007/s11135-011-9565-3. URL http://link.springer.com/10.1007/s11135-011-9565-3 . [ CrossRef ] [ Google Scholar ]
- Nanda A., Xu Y., Zhang F. How would the COVID-19 pandemic reshape retail real estate and high streets through acceleration of E-commerce and digitalization? J. Urban Manag. 2021 [ Google Scholar ]
- Narayan N., Jha R.K., Singh A. A differential epidemic model for information, misinformation, and disinformation in online social networks: Covid-19 vaccination. Int. J. Semantic Web Inform. Syst. 2022; 18 (1):1–20. [ Google Scholar ]
- Naseri R.N.N., et al. Issues and challenges of online shoppingactivities on the impact of corona pandemic: A study on Malaysia retail industry. Turk. J. Comput. Math. Educ. 2021; 12 (10):7682–7686. [ Google Scholar ]
- Page M.J., McKenzie J.E., Bossuyt P.M., Boutron I., Hoffmann T.C., Mulrow C.D., Shamseer L., Tetzlaff J.M., Akl E.A., Brennan S.E. The PRISMA 2020 statement: an updated guideline for reporting systematic reviews. Int. J. Surg. 2021; 88 Publisher: Elsevier. [ PubMed ] [ Google Scholar ]
- Paiva S., Corcoba V., Mourao F., Paneda X., Melendi D., Garcia R. Analysis of mobility changes caused by COVID-19 in a context of moderate restrictions using data collected by mobile devices. IEEE Access. 2022; 10 :8906–8915. doi: 10.1109/ACCESS.2022.3141083. [ CrossRef ] [ Google Scholar ]
- Pantano E., Pizzi G., Bilotta E., Pantano P. Shopping with(out) distancing: modelling the personal space to limit the spread of contagious disease among consumers in retail stores. J. Mark. Manag. 2021; 37 (17–18):1764–1782. doi: 10.1080/0267257X.2021.2003422. [ CrossRef ] [ Google Scholar ]
- Piskunova O. 2022. Fast-Growing ECommerce and Omnichannel Concept Development: Empirical Evidence from Russian Retail; pp. 493–505. [ CrossRef ] [ Google Scholar ]
- Pollák F., Konečný M. Analysis of E-consumer behaviour – selected findings from an analysis of czech E-shops and their customers during the global pandemic. SHS Web Conf. 2021 [ Google Scholar ]
- Ponis S., Aretoulaki E., Plakas G., Agalianos K., Maroutas T. Lecture Notes in Networks and Systems. 2022. Zero-touch customer order fulfillment to support the new normal of retail in the 21st century; pp. 1–10. [ CrossRef ] [ Google Scholar ]
- Rasmusen S.C., Penz M., Widauer S., Nako P., Kurteva A., Roa-Valverde A., Fensel A. Raising consent awareness with gamification and knowledge graphs: an automotive use case. Int. J. Semantic Web Inform. Syst. 2022; 18 (1):1–21. [ Google Scholar ]
- Richards T., Rickard B. COVID-19 impact on fruit and vegetable markets. Can. J. Agricult. Econ. 2020; 68 (2):189–194. doi: 10.1111/cjag.12231. [ CrossRef ] [ Google Scholar ]
- Riehmann P., Hanfler M., Froehlich B. Interactive Sankey diagrams. IEEE Symposium on Information Visualization, 2005; INFOVIS 2005; 2005. pp. 233–240. ISSN: 1522-404X. [ CrossRef ] [ Google Scholar ]
- del Rio-Chanona R.M., Mealy P., Pichler A., Lafond F., Farmer J.D. Supply and demand shocks in the COVID-19 pandemic: an industry and occupation perspective. Oxf. Rev. Econ. Policy. 2020 [ Google Scholar ]
- Saab S., Al Abbas M., Samaha R.N., Jaafar R., Saab K.K., Saab Jr. S.S. Setting the boundaries of COVID-19 lockdown relaxation measures. Library Hi Tech. 2021 [ Google Scholar ]
- Saprikis V., Avlogiaris G., Katarachia A. A comparative study of users versus non-users’ behavioral intention towards M-banking apps’ adoption. Information (Switzerland) 2022; 13 (1) doi: 10.3390/info13010030. [ CrossRef ] [ Google Scholar ]
- Sayyida S., Hartini S., Gunawan S., Husin S.N. The impact of the COVID-19 pandemic on retail consumer behavior. Aptisi Trans. Manag. 2021; 5 (1):79–88. [ Google Scholar ]
- Sharma M., Luthra S., Joshi S., Kumar A. Accelerating retail supply chain performance against pandemic disruption: adopting resilient strategies to mitigate the long-term effects. J. Enterp. Inf. Manag. 2021; 34 (6):1844–1873. doi: 10.1108/JEIM-07-2020-0286. [ CrossRef ] [ Google Scholar ]
- Siriwardhana Y., De Alwis C., Gur G., Ylianttila M., Liyanage M. The fight against the COVID-19 pandemic with 5G technologies. IEEE Eng. Manag. Rev. 2020; 48 (3):72–84. doi: 10.1109/EMR.2020.3017451. [ CrossRef ] [ Google Scholar ]
- Srour A., Ould-Slimane H., Mourad A., Harmanani H., Jenainati C. Joint theme and event based rating model for identifying relevant influencers on Twitter: COVID-19 case study. Online Soc. Netw. Media. 2022; 31 [ Google Scholar ]
- Stergiou C.L., Psannis K.E., Gupta B.B. Infemo: flexible big data management through a federated cloud system. ACM Trans. Internet Technol. 2021; 22 (2):1–22. [ Google Scholar ]
- Talwar M., Talwar S., Kaur P., Tripathy N., Dhir A. Has financial attitude impacted the trading activity of retail investors during the COVID-19 pandemic? J. Retail. Consum. Serv. 2021; 58 doi: 10.1016/j.jretconser.2020.102341. [ CrossRef ] [ Google Scholar ]
- Tan L., Yu K., Shi N., Yang C., Wei W., Lu H. Towards secure and privacy-preserving data sharing for covid-19 medical records: A blockchain-empowered approach. IEEE Trans. Netw. Sci. Eng. 2021 [ Google Scholar ]
- Tarhini A., Harfouche A., De Marco M. Artificial intelligence-based digital transformation for sustainable societies: The prevailing effect of COVID-19 crises. Pac. Asia J. Assoc. Inf. Syst. 2022; 14 (2):1. [ Google Scholar ]
- Trapp C.T., Kanbach D.K., Kraus S. Sector coupling and business models towards sustainability: The case of the hydrogen vehicle industry. Sustain. Technol. Entrepreneurship. 2022; 1 (2) [ Google Scholar ]
- Van Ngoc D., Xuan Canh C. Proceedings of 2021 International Conference on System Science and Engineering, ICSSE 2021. 2021. A scalable virtual try-on system based on cloud computing; pp. 128–132. [ CrossRef ] [ Google Scholar ]
- Vassiliades A., Bassiliades N., Patkos T., Vrakas D. An open-ended web knowledge retrieval framework for the household domain with explanation and learning through argumentation. Int. J. Semantic Web Inform. Syst. 2022; 18 (1):1–34. [ Google Scholar ]
- Vizuete-Luciano E., Boria-Reverter S., Solé-Moro M., Gil-Lafuente A. Lecture Notes in Networks and Systems. 2022. Adaptation of grocery stores to the post-Covid-19 environment. The case of Barcelona; pp. 288–300. [ CrossRef ] [ Google Scholar ]
- Yang H., Vijayakumar P., Shen J., Gupta B.B. A location-based privacy-preserving oblivious sharing scheme for indoor navigation. Future Gener. Comput. Syst. 2022; 137 :42–52. [ Google Scholar ]
- Yu K., Tan L., Shang X., Huang J., Srivastava G., Chatterjee P. Efficient and privacy-preserving medical research support platform against COVID-19: a blockchain-based approach. IEEE Consum. Electron. Mag. 2020; 10 (2):111–120. [ Google Scholar ]
- Zhao X., Keikhosrokiani P. Sales prediction and product recommendation model through user behavior analytics. Comput. Mater. Contin. 2022; 70 (2):3855–3874. doi: 10.32604/cmc.2022.019750. [ CrossRef ] [ Google Scholar ]
Academia.edu no longer supports Internet Explorer.
To browse Academia.edu and the wider internet faster and more securely, please take a few seconds to upgrade your browser .
- We're Hiring!
- Help Center
Retail Research
- Most Cited Papers
- Most Downloaded Papers
- Newest Papers
- Save to Library
- Last »
- Structure Equation Modeling Using AMOS Follow Following
- Factor analysis Follow Following
- Consumer Behavior Follow Following
- Structural Equation Modeling Follow Following
- Retail Design Follow Following
- Motivation of Customer to Shopping Mall Follow Following
- Research in Retail Design Follow Following
- Retailing Follow Following
- Financial Performance Follow Following
- Dissertations Follow Following
Enter the email address you signed up with and we'll email you a reset link.
- Academia.edu Publishing
- We're Hiring!
- Help Center
- Find new research papers in:
- Health Sciences
- Earth Sciences
- Cognitive Science
- Mathematics
- Computer Science
- Academia ©2024
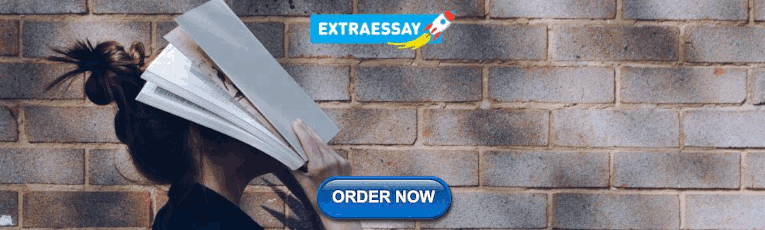
IMAGES
VIDEO
COMMENTS
The retail business places a premium on the creation of ideas to increase sales. 5. Theoretical and practical implications. This section describes the observations made during the analysis. This paper's primary objective is to document the research development in the retail sector due to COVID-19.
This research aims to investigate the multifaceted effects of the growing e-commerce sector on traditional retail businesses, considering economic, social, and policy dimensions.
1 INTRODUCTION TO RETAIL ANALYTICS: DEFINITION AND CLASSIFICATION. The retail industry is a major contributor to global economies (Mou et al., 2018), employing a substantial portion of the labor force in many nations.Given the importance of this industry to economic growth, Fisher et al. call for an infusion of "rocket science" into retail, citing the need for improved analytical decision ...
Retail. New research on the retail industry from Harvard Business School faculty on issues including online and brick-and-mortar strategies, consumer behavior, and use of technology. Page 1 of 188 Results →. 13 May 2024.
McKinsey on Consumer and Retail Podcast: Insights from experts on business and management. McKinsey experts discuss the latest trends and the most pressing challenges for leaders in the retail and consumer-goods industries. Hear about the immediate and longer-term moves that matter most for business success.
Abstract. In the digital age, retail store operations face a variety of novel challenges and complexities. We review 255 papers on retail store operations from 32 operations research, management science, retailing, and general management journals over the period 2008-2016. We assess the current state of research within the context of retail ...
That research has provided an initial discussion of how new electronic retail formats can create customer value through informational benefits such as search efficiency (Alba et al., 1997). Those studies also emphasized the need to consider features of the broader buying situation, such as product type or decision characteristics, to derive ...
This research responds to the call for further research into the types of retail technology and how they contribute to omnichannel experience as research into these relationships remains scarce (Alexander & Kent, 2022).Specifically, it addresses the need for integrated insights into the role of technology in omnichannel retailing from the perspectives of customers and retailers.
Rehan Iftikhar is a Research Fellow at the Innovation Value Institute, School of Business, Maynooth University, a member of Lero, the Science Foundation Ireland Research Centre for Software, and a part of the EU Innovative Training Network PERFORM (www.perform-network.eu). His main research interests are digital retail, digital transformation ...
Retail business has been rapidly evolving in the past decades with the boom of internet, mobile technologies and most importantly e-commerce. Supply chain management, as a core part of retail business, has also gone through significant changes with new business scenarios and more advanced technologies in both algorithm design and computation power. In this review, we focus on several core ...
Introduction. Online retailing is commonly framed as an intense disruptive force affecting the competitive nature of retailing, with structural implications for the reconfiguration of retail management processes (Doherty & Ellis‐Chadwick, Citation 2010), challenging traditional store-based retail business models and, by association, leaving town centres struggling amid gales of digital ...
The substantial growth of e-commerce during the last years has led to a surge in consumer returns. Recently, research interest in consumer returns has grown steadily. The availability of vast customer data and advancements in machine learning opened up new avenues for returns forecasting. However, existing reviews predominantly took a broader perspective, focussing on reverse logistics and ...
This paper is an outcome of a descriptive research on the retail industry in India and the application of customer analytics to shape the retail industry's business strategy. Read more ...
The aim of this paper is to make an investigation of retail sector and its business type. To gather the data, second resources has been used. A deeply scanning of literature has been performed.
Working papers are in draft form. This working paper is distributed for purposes of comment and discussion only. It may not be reproduced without permission of the copyright holder. Copies of working papers are available from the author. Funding for this research was provided in part by Harvard Business School. Research support was provided by the
The study theorises and tests a conceptual model with information quality, privacy concerns, perceived security, product variety, and product delivery as antecedents of customer satisfaction and loyalty towards retail websites. The research findings endeavor to improve the managerial implications of online retailing in the context of a modern ...
The Journal of Business and Retail Management Research (JBRMR) is proud to have a growing international reputation as a scholarly journal. It sets out to ensure that academics from across the world have a credible vehicle for disseminating their research papers. Those seeking fresh and original articles, particularly in relation to the retail ...
Research Papers. 1. Retail Marketing Strategies: ... The Indian retail business is inextricably linked . t o t he c o u n t ry 's e c o n om ic g r o wt h a n d . development. In India, consumer ...
Journal of Research in Business and Management Volume 4 ~ Issue 4 (2016) pp: 24-26 ISSN(Online) : 2347-3002 www.questjournals.org ... Research Paper Study on Retail Stores' Attributes and Increase in Sales By Influencing Consumers Sadaf Siddiqui Department Of Commerce, Faculty of Management Sciences, Shrivenkateshwara University, ...
The paper has shed light on the impact of effective product development for the MNCs or the Retail business. It has been evident that from the very beginning the world is flooded with a variety of businesses and the objectives are to appeal the customers with different products.
The purpose of this study is to analysis the evolution of the retail sector during the COVID-19 period and to identify future research issues. Scopus databases were searched for articles published in English between 2020 and 2022 to discover current trends and concerns in the retail industry. A total of 1071 empirical and nonempirical studies ...
Retailing in India has sever al outstanding achievements as well as i mpressive. growth during last few decades. Indian retail market is one of the top five retail. markets in the world b y ...
retail sector in various sectors. Research proposals are based on three different "sections" of retail; micro, meso and macro, from individual retail employees to societal changes are defined and research proposals created.One of the main implications is for retailers to adapt and build new business
View Retail Research Research Papers on Academia.edu for free. Skip to main content ... The Role of Human-Robot Interaction in Consumers' Acceptance of Humanoid Retail Service Robots, Journal of Business Research, 146, 489-503 [SSCI Impact factor 7.550; CiteScore 9.2]