Subscribe to the PwC Newsletter
Join the community, add a new evaluation result row, data visualization.
88 papers with code • 0 benchmarks • 2 datasets
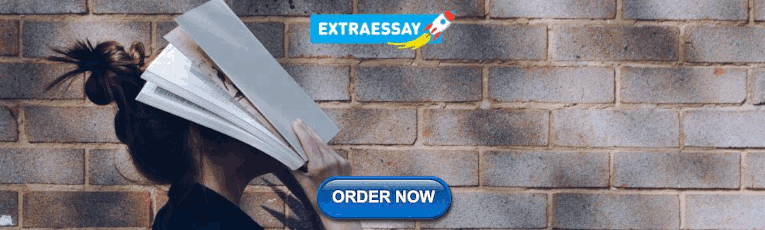
Benchmarks Add a Result

Latest papers
Boostlet.js: image processing plugins for the web via javascript injection.
mpsych/boostlet • 13 May 2024
Can web-based image processing and visualization tools easily integrate into existing websites without significant time and effort?
Cross-Care: Assessing the Healthcare Implications of Pre-training Data on Language Model Bias
Large language models (LLMs) are increasingly essential in processing natural languages, yet their application is frequently compromised by biases and inaccuracies originating in their training data.
CoSense3D: an Agent-based Efficient Learning Framework for Collective Perception

This framework not only provides an API for flexibly prototyping the data processing pipeline and defining the gradient calculation for each agent, but also provides the user interface for interactive training, testing and data visualization.
CBMAP: Clustering-based manifold approximation and projection for dimensionality reduction
Dimensionality reduction methods are employed to decrease data dimensionality, either to enhance machine learning performance or to facilitate data visualization in two or three-dimensional spaces.
Automated Data Visualization from Natural Language via Large Language Models: An Exploratory Study
In particular, we first explore the ways of transforming structured tabular data into sequential text prompts, as to feed them into LLMs and analyze which table content contributes most to the NL2Vis.
A visualization method for data domain changes in CNN networks and the optimization method for selecting thresholds in classification tasks
In recent years, Face Anti-Spoofing (FAS) has played a crucial role in preserving the security of face recognition technology.
Curvature Augmented Manifold Embedding and Learning
ymlasu/camel • 21 Mar 2024
A new dimensional reduction (DR) and data visualization method, Curvature-Augmented Manifold Embedding and Learning (CAMEL), is proposed.
From Pixels to Insights: A Survey on Automatic Chart Understanding in the Era of Large Foundation Models
khuangaf/awesome-chart-understanding • 18 Mar 2024
This survey paper serves as a comprehensive resource for researchers and practitioners in the fields of natural language processing, computer vision, and data analysis, providing valuable insights and directions for future research in chart understanding leveraging large foundation models.
ChartThinker: A Contextual Chain-of-Thought Approach to Optimized Chart Summarization

Data visualization serves as a critical means for presenting data and mining its valuable insights.
DiffRed: Dimensionality Reduction guided by stable rank
s3-lab-iit/diffred • 9 Mar 2024
We rigorously prove that DiffRed achieves a general upper bound of $O\left(\sqrt{\frac{1-p}{k_2}}\right)$ on Stress and $O\left(\frac{(1-p)}{\sqrt{k_2*\rho(A^{*})}}\right)$ on M1 where $p$ is the fraction of variance explained by the first $k_1$ principal components and $\rho(A^{*})$ is the stable rank of $A^{*}$.
- Survey paper
- Open access
- Published: 01 October 2015
Visualizing Big Data with augmented and virtual reality: challenges and research agenda
- Ekaterina Olshannikova 1 ,
- Aleksandr Ometov 1 ,
- Yevgeni Koucheryavy 1 &
- Thomas Olsson 2
Journal of Big Data volume 2 , Article number: 22 ( 2015 ) Cite this article
79k Accesses
179 Citations
83 Altmetric
Metrics details
This paper provides a multi-disciplinary overview of the research issues and achievements in the field of Big Data and its visualization techniques and tools. The main aim is to summarize challenges in visualization methods for existing Big Data, as well as to offer novel solutions for issues related to the current state of Big Data Visualization. This paper provides a classification of existing data types, analytical methods, visualization techniques and tools, with a particular emphasis placed on surveying the evolution of visualization methodology over the past years. Based on the results, we reveal disadvantages of existing visualization methods. Despite the technological development of the modern world, human involvement (interaction), judgment and logical thinking are necessary while working with Big Data. Therefore, the role of human perceptional limitations involving large amounts of information is evaluated. Based on the results, a non-traditional approach is proposed: we discuss how the capabilities of Augmented Reality and Virtual Reality could be applied to the field of Big Data Visualization. We discuss the promising utility of Mixed Reality technology integration with applications in Big Data Visualization. Placing the most essential data in the central area of the human visual field in Mixed Reality would allow one to obtain the presented information in a short period of time without significant data losses due to human perceptual issues. Furthermore, we discuss the impacts of new technologies, such as Virtual Reality displays and Augmented Reality helmets on the Big Data visualization as well as to the classification of the main challenges of integrating the technology.
Introduction
The whole history of humanity is an enormous accumulation of data. Information has been stored for thousands of years. Data has become an integral part of history, politics, science, economics and business structures, and now even social lives. This trend is clearly visible in social networks such as Facebook, Twitter and Instagram where users produce an enormous stream of different types of information daily (music, pictures, text, etc.) [ 1 ]. Now, government, scientific and technical laboratory data as well as space research information are available not only for review, but also for public use. For instance, there is the 1000 Genomes Project [ 2 , 3 ], which provide 260 terabytes of human genome data. More than 20 terabytes of data are publicly available at Internet Archive [ 4 , 5 ], ClueWeb09 [ 6 ], among others.
Lately, Big Data processing has become more affordable for companies from resource and cost points of view. Simply put, revenues generated from it are higher than the costs, so Big Data processing is becoming more and more widely used in industry and business [ 7 ]. According to International Data Corporation (IDC), data trading is forming a separate market [ 8 ]. Indeed, 70 % of large organizations already purchase external data, and it is expected to reach 100 % by the beginning of 2019.
Simultaneously, Big Data characteristics such as volume, velocity, variety [ 9 ], value and veracity [ 10 ] require quick decisions in implementation, as the information may become less up to date and can lose value fast. According to IDC [ 11 ], data volumes have grown exponentially, and by 2020 the number of digital bits will be comparable to the number of stars in the universe. As the size of bits geminates every two years, for the period from 2013 to 2020 worldwide data will increase from 4.4 to 44 zettabytes. Such fast data expansion may result in challenges related to human ability to manage the data, extract information and gain knowledge from it.
The complexity of Big Data analysis presents an undeniable challenge: visualization techniques and methods need to be improved. Many companies and open-source projects see the future of Big Data Analytics via Visualization, and are establishing new interactive platforms and supporting research in this area. Husain et al. [ 12 ] in their paper provide a wide list of contemporary and recently developed visualization platforms. There are commercial Big Data platforms such as International Business Machines (IBM) Software [ 13 ], Microsoft [ 14 ], Amazon [ 15 ] and Google [ 16 ]. There exists an open-source project, Socrata [ 17 ], which deals with dynamic data from public, government and private organizations. Another platform is a JavaScript library D3 [ 18 ] for dynamic data visualizations. This list can be extended with Cytoscape [ 19 ], Tableau [ 20 ], Data Wrangler [ 21 ] and others. Intel [ 22 ] and Statistical Analysis System (SAS) [ 23 ] are performing research in data visualization as well but more from a business perspective.
Organizations and social media generate enormous amounts of data every day and, traditionally, represent it in a format consistent with the poorly structured databases: web blogs, text documents, or machine code, such as geospatial data that may be collected in various stores even outside of a company/organization [ 24 ]. On the other hand, information stored in a multitude repository and the use of cloud storage or data centers is also widely common [ 25 ]. Furthermore, companies have the necessary tools to establish the relationship between data segments in addition to the process of making the basis for meaningful conclusions. As data processing rates are growing continuously, a situation may appear when traditional analytical methods would not be able to stay up to date, especially with the growing amount of constantly updated data, which ultimately opens the way for Big Data technologies [ 26 ].
This paper provides information about various types of existing data to which certain techniques are useful for the analysis. Recently, many visualization methods have been developed for a quick representation of data that is already preprocessed. There has been a step away from planar images towards multi-dimensional volumetric visualizations. However, Big Data visualization evolution cannot be considered as finished, inasmuch as new techniques generate new research challenges and solutions that will be discussed in the following paper.
Current activity in the field of Big Data visualization is focused on the invention of tools that allow a person to produce quick and effective results working with large amounts of data. Moreover, it would be possible to assess the analysis of the visualized information from all the angles in novel, scalable ways. Based on Big Data related literature, we identify the main visualization challenges and propose a novel technical approach to visualize Big Data based on the understandings of human perception and new Mixed Reality (MR) technologies. From our perspective, one of the more promising methods for improving upon current Big Data visualization techniques is in its correlation with Augmented Reality (AR) and Virtual Reality (VR) that are suitable for the limited perception capabilities of humans. We identify important steps for the research agenda to implement this approach.
This paper covers various issues and topics, but there are three main directions of this survey:
Human cognitive limitations in terms of Big Data Visualization.
Applying Augmented and Virtual reality opportunities towards Big Data Visualization.
Challenges and benefits of the proposed visualization approach.
The rest of paper is organized as follows: The first section provides a definition of Big Data and looks at currently used methods for Big Data processing and their specifications. Also it indicates the main challenges and issues in Big Data analysis. Next, in the section Visualization methods, the historical background of this field is given, modern visualization techniques for massive amounts of information are presented and the evolution of visualization methods is discussed. Further in the last section, Integration with Augmented and Virtual Reality, the history of AR and VR is detailed with respect to its influence on Big Data. These developmental processes are supported by the proposed oncoming Big Data visualization extension for VR and AR, which can solve actual perception and cognition challenges. Finally, important data visualization challenges and future research agenda are discussed.
Big Data: an overview
Today large data sources are ubiquitous throughout the world. Data used for processing may be obtained from measuring devices, radio frequency identifiers, social network message flows, meteorological data, remote sensing, location data streams of mobile subscribers and devices, and audio and video recordings. So, as Big Data is more and more used all over the world, a new and important research field is being established. The mass distribution of the technology and innovative models that utilize these different kinds of devices and services, appeared to be a starting point for the penetration of Big Data in almost all areas of human activity, including the commercial sector and public administration [ 27 ].
Nowadays, Big Data and the continuing dramatic increase in human and machine-generated data associated with it are quite evident. However, do we actually know what Big Data is, and how close are the various definitions put forward for this term? For instance, there was a article in Forbes in 2014 which is related to this controversial question [ 28 ]. It gave a brief history of the establishment of the term, and provided several existing explanations and descriptions of Big Data to improve the core understanding of the phenomenon. On the other hand, Berkeley School of Information published a list with more than 40 definitions of the term [ 29 ].
As Big Data covers various fields and sectors, the meaning of this term should be specifically defined in accordance with the activity of the specific organization/person. For instance, in contrast to industry-driven Big Data “V’s” definitions, Dr. Ivo Dinov for his research scope listed another data’s multi-dimensional characteristics [ 30 ] such as data size, incompleteness, incongruency, complex representation, multiscale nature and heterogeneity of its sources [ 31 , 32 ].
In this paper the modified Gartner Inc. definition [ 33 , 34 ] is used: Big Data is a technology to process high-volume, high-velocity, high-variety data or data-sets to extract intended data value and ensure high veracity of original data and obtained information that demand cost-effective, innovative forms of data and information processing (analytics) for enhanced insight, decision making, and processes control [ 35 ].
Big Data processing methods
Currently, there exist many different techniques for data analysis [ 36 ], mainly based on tools used in statistics and computer science. The most advanced techniques to analyze large amounts of data include: artificial neural networks [ 37 – 39 ]; models based on the principle of the organization and functioning of biological neural networks [ 40 , 41 ]; methods of predictive analysis [ 42 ]; statistics [ 43 , 44 ]; Natural Language Processing [ 45 ]; etc. Big Data processing methods embrace different disciplines including applied mathematics, statistics, computer science and economics. Those are the basis for data analysis techniques such as Data Mining [ 39 , 46 – 49 ], Neural Networks [ 41 , 50 – 52 ], Machine Learning [ 53 – 55 ], Signal Processing [ 56 – 58 ] and Visualization Methods [ 59 – 61 ]. Most of these methods are interconnected and used simultaneously during data processing, which increases system utilization tremendously (see Fig. 1 ).
Big Data processing methods interconnection. Applied mathematics, statistics, economics and computer science are foundation of the Big Data processing methods. Meanwhile, Data Mining, Signal Processing, Neural Networks, Visualization and Machine learning are strongly connected to each other
We would like to familiarize reader with the primary methods and techniques in Big Data processing. As this topic is not a focus of the paper, this list is not exhaustive. Nevertheless, the main interconnections between these methods are shown and application examples are given.
Optimization methods are mathematical tools for efficient data analysis. Optimization includes numerical analysis focused on problem solving in various Big Data challenges: volume, velocity, variety and veracity [ 62 ] that will be discussed in more detail later. Some widely used analytical techniques are genetic programming [ 63 – 65 ], evolutionary programming [ 66 ] and particle swarm optimization [ 67 , 68 ]. Optimization is focused on the search of the optimal set of actions needed to improve system performance. Notably, genetic algorithms are also a specific part of machine learning direction [ 69 ]. Moreover, statistical testing , predictive and simulation models are applied also as for Statistics methods [ 70 ].
Statistics methods are used to collect, organize and interpret data, as well as to outline interconnections between realized objectives. Data-driven statistical analysis concentrates on implementation of statistics algorithms [ 71 , 72 ]. A/B testing [ 73 ] technique is an example of a statistics method. In terms of Big Data there is a possibility to perform a variety of tests. The aim of A/B tests is to detect statistically important differences and regularities between groups of variables to reveal improvements. Besides, statistical techniques contain cluster analysis, data mining and predictive modelling methods. Some techniques in spatial analysis [ 74 ] originate from the field of statistics as well. It allows analysis of topological, geometric or geographic characteristics of data sets.
Data mining includes cluster analysis , classification , regression and association rule learning techniques. This method is aimed at identifying and extracting beneficial information from extensive data or datasets. Cluster analysis [ 75 , 76 ] is based on principles of similarities to classify objects. This technique belongs to unsupervised learning [ 77 , 78 ] where training data [ 79 ] is used. Classification [ 80 ] is a set of techniques which are aimed at recognizing categories with new data points. In contrast to cluster analysis, a classification technique uses training data sets to discover predictive relationships. Regression [ 81 ] is a set of a statistical techniques that are aimed at determining changes between dependent and independent variables. This technique is mostly used for prediction or forecasting. Association rule learning [ 82 , 83 ] is set of techniques designed to detect valuable relationships or association rules among variables in databases.
Machine Learning is a significant area in computer science which aims to create algorithms and protocols. The main goal of this method is to improve computers’ behaviors on the basis of empirical data. Its implementation allows recognition of complicated patterns and automatic application of intelligent decision-making based on. Pattern recognition, natural language processing, ensemble learning and sentiment analysis are examples of machine learning techniques. Pattern recognition [ 84 , 85 ] is a set of techniques that use a certain algorithm to associate an output value with a given input value. Classification technique is an example of this. Natural language processing [ 86 ] takes its origins from computer science within the fields of artificial intelligence and linguistics. This set of techniques performs analysis of human language. Sometimes it uses a sentiment analysis [ 87 ] that is able to identify and extract specific information from text materials evaluating words, degree and strength of a sentiment. Ensemble learning [ 88 , 89 ] in automated decision-making systems is a useful technique for diminishing variance and increase accuracy. It aims to solve diverse machine learning issues such as confidence estimation, missing feature and error correction, etc.
Signal processing consists of various techniques that are part of electrical engineering and applied mathematics. The key aspect of this method is the analysis of discrete and continuous signals. In other words, it enables the analog representation of physical quantities (e.g. radio signals or sounds, etc.). Signal detection theory [ 90 ] is applied to evaluate the capacity for distinguishing between signal and noise in some techniques. A time series analysis [ 91 , 92 ] includes techniques from both statistics and signal processing. Primarily, it is designed to analyze sequences of data points with a demonstration of data values at consistent times. This technique is useful to predict future data values based on knowledge of past ones. Signal processing techniques can be applied to implement some types of data fusion [ 93 ]. Data fusion combines multiple sources to obtain improved information that is more relevant or less expensive and has higher quality [ 94 ].
Visualization methods concern the design of graphical representation, i.e. to visualize the innumerate amount of the analytical results as diagrams, tables and images. Visualization for Big Data differs from all of the previously mentioned processing methods and also from traditional visualization techniques. To visualize large-scale data, feature extraction and geometric modelling can be implemented. These processes are needed to decrease the data size before actual rendering [ 95 ]. Intuitively, visual representation is more likely to be accepted by a human in comparison with unstructured textual information. The era of Big Data has been rapidly promoting the data visualization market. According to Mordor Intelligence [ 96 ] the visualization market will increase at a compound annual growth rate (CAGR) of 9.21 % from $4.12 billions in 2014 to $6.40 billions by the end of 2019. SAS Institute provides results of an International Data Group (IDG) research study in the white paper [ 97 ]. The research is focused on how companies are performing Big Data analysis. It shows that 98 % of the most effective companies working with Big Data are presenting results of the analysis via visualization. Statistical data from this research provides evidence of the visualization benefits in terms of decision-making improvement, better ad-hoc data analysis, improved collaboration and information sharing inside/outside an organization.
Nowadays, different groups of people including designers, software developers and scientists are in the process of searching for new visualization tools and opportunities. For example, Amazon, Twitter, Apple, Facebook and Google are companies that utilize data visualization in order to make appropriate business decisions [ 98 ]. Visualization solutions can provide insights from different business perspectives. First of all, implementation of advanced visualization tools enables rapid exploration of all customers/users data to improve customer-company relationships. It allows marketers to create more precise customer segments based on data from purchasing history or life stage and other factors. Besides, correlation mapping may assist in the analysis of customer/user behavior to identify and analyze the most profitable of them. Secondly, visualization capabilities allow companies opportunities to reveal correlations between product, sales and customer profiles. Based on gathered metrics, organizations may provide novel special offers to their customers. Moreover, visualization enables tracking of revenue trends and can be useful for risk analysis. Thirdly, visualization as a tool provides better understanding of data. Higher efficiency is reached by obtaining relevant, consistent and accurate information. So, visualized data could assist organizations to find different effective marketing solutions. In this section we familiarized the reader with the main techniques of data analysis and described their strong correlation to each other. Nevertheless, the Big Data era is still in the beginning stage of its evolution. Therefore, Big Data processing methods are evolving to solve the problems of Big Data and new solutions are continuously being developed. By this statement we mean that big world of Big Data requires multiple multidisciplinary methods and techniques that lead to better understanding of the complicated structures and interconnections between them.
Big Data challenges
Big Data has some inherent challenges and problems that can be primarily divided into three groups according to Akerkar et al. [ 36 ]: (1) data, (2) processing and (3) management challenges (see Fig. 2 ). While dealing with large amounts of information we face such challenges as volume , variety , velocity and veracity that are also known as 5 V of Big Data. As those Big Data characteristics are well examined in scientific literature [ 99 – 101 ] we will only discuss them briefly. Volume refers to the large amount of data, especially, machine-generated. This characteristic defines a size of the data set that makes its storage and analysis problematic utilizing conventional database technology. Variety is related to different types and forms of data sources: structured (e.g. financial data) and unstructured (social media conversations, photos, videos, voice recordings and others). Multiplicity of the various data results in the issue of its handling. Velocity refers to the speed of new data generation and distribution. This characteristic requires the implementation of real-time processing for the streaming data analysis (e.g. on social media, different types of transactions or trading systems, etc.). Veracity refers to the complexity of data which may lead to a lack of quality and accuracy. This characteristic reveals several challenges: uncertainty, imprecision, missing values, misstatement and data availability. There is also a challenge regarding data discovery that is related to the search of high quality data in data sets.
Big Data challenges.The picture illustrates three main categories of Big Data challenges that are associated with data, its management and processing issues
The second branch of Big Data challenges is called processing challenges . It includes data collection, resolving similarities found in different sources, modification data to a type acceptable for the analysis, the analysis itself and output representation, i.e. the results visualization in a form most suitable for human perception.
The last type of challenge offered by this classification is related to data management . Management challenges usually refer to secured data storage, its processing and collection. Here the main focuses of study are: data privacy, its security, governance and ethical issues. Most of them are controlled based on policies and rules provided by information security institutes on state or international levels.
Over past generations, the results of analyzed data were represented as visualized plots and graphs. It is evident that collections of complex figures are sometimes hard to perceive, even by well-trained minds. Nowadays, the main factors causing difficulties in data visualization continue to be the limitations of human perception and new issues related to display sizes and resolutions. This question is studied in detail further in the section “Integration with Augmented and Virtual Reality”. Preparatory to the visualization, the main interaction problem is in the extraction of the useful portion of information from massive volumes. Extracted data is not always accurate and mostly overloaded with excrescent information. Visualization technique is useful for simplifying information and transforming it into a more accessible form for human perception.
In the near future, petascale data may cause analysis failures because of traditional approaches in usage, i.e. when the data is stored on a memory disk continuously waiting for further analysis. Hence, the conservative approach of data compressing may become ineffective in visualization methods. To solve this issue, developers should create a flexible tool for the practice of data collection and analysis. Increases in data size make the multilevel hierarchy approach incapable in data scalability. Hierarchy becomes complex and intensive, making navigation difficult for user perception. In this case, a combination of analytics and Data Visualization may enable more accessible data exploration and interaction, which would allow improving insights, outcomes and decision-making.
Contemporary methods, techniques and tools for data analysis are still not flexible enough to discover valuable information in the most efficient way. The question of data perception and presentation remains open. Scientists face the task of uniting the abstract world of data and the physical world through visual representation. Meanwhile, visualization-based tools should fulfill three requirements [ 102 , 103 ]: expressiveness (demonstrate exactly the information contained in the data), effectiveness (related to cognitive capabilities of human visual system) and appropriateness (cost-value ratio for visualization benefit assessment). Experience of previously used techniques can be repurposed to achieve more beneficial and novel goals in Big Data perception and representation.
Visualization methods
Historically, the primary areas of visualization were Science Visualization and Information Visualization. However, during recent decades, the field of Visual Analytics was actively developing.
As a separate discipline, visualization emerged in 1980 [ 104 ] as a reaction to the increasing amount of data generated by computer calculations. It was named Science Visualization [ 105 – 107 ], as it displays data from scientific experiments related to physical processes. This is primarily a realistic three-dimensional visualization, which has been used in architecture, medicine, biology, meteorology, etc. This visualization is also known as Spatial Data visualization, which focuses on the visualization of volumes and surfaces.
Information Visualization [ 108 – 111 ] emerged as a branch of the Human-Computer Interaction field in the end of 1980s. It utilizes graphics to assist people in comprehending and interpreting data. As it helps to form mental models of the data, for humans it is easier to reveal specific features and patterns of the obtained information.
Visual Analytics [ 112 – 114 ] combines visualization and data analysis. It has absorbed features of Information Visualization as well as Science Visualization. The main difference from other fields is the development and provision of visualization technologies and tools.
Efficient visualization tools should consider cognitive and perceptual properties of the human brain. Visualization aims to improve the clarity and aesthetic appeal of the displayed information and allows a person to understand large amount of data and interact with it. Significant purposes of Big Data visual representation are: to identify hidden patterns or anomalies in data; to increase flexibility while searching of certain values; to compare various units in order to obtain relative difference in quantities; to enable real-time human interaction (touring, scaling, etc.).
Visualization methods have evolved much over the last decades (see Fig. 3 ), the only limit for novel techniques being human imagination. To anticipate the next steps of data visualization development, it is necessary to take into account the successes of the past. It is considered that quantitative data visualization appeared in the field of statistics and analytics quite recently. However, the main precursors were cartography and statistical graphics, created before the 19th century for the expansion of statistical thinking, business planning and other purposes [ 115 ]. The evolution in the knowledge of visualization techniques resulted in mathematical and statistical advances as well as in drawing and reproducing images.
The evolution of visualization methodology. Development of visualization methods originates from 18th century and it is rapidly improving today due to technical sophistication
By the 16th century, tools for accurate observation and measurement were developed. Precisely, in those days the first steps were done in the development of data visualization. The 17th century was swept by the problem of space, time and distance measurements. Furthermore, the study of the world’s population and economic data had started.
The 18th century was marked by the expansion of statistical theory, ideas of data graphical representation and the advent of new graphic forms. At the end of the century thematic maps displaying geological, medical and economic data was used for the first time. For example, Charles de Fourcroy used geometric figures and cartograms to compare areas or demographic quantities [ 116 ]. Johann Lambert (1728–1777) was a revolutionary person, who used different types of tables and line graphs to display variable data [ 117 ]. The first methods were performed as simple plots followed by one-dimensional histograms [ 118 ]. Still, those examples are useful only for small amounts of data. By introducing more information, this type of diagram would reach a point of worthlessness.
At the turn of 20–21st centuries, steps were taken in the development of interactive statistical computing [ 119 ] and new paradigms for data analysis [ 120 ]. Technological progress was certainly a significant prerequisite for the rapid development of visualization techniques, methods and tools. More precisely, large-scale statistical and graphics software engineering was invented, and computer processing speed and capacity vastly increased [ 121 ].
However, the next step presenting a system with the addition of a time dimension appeared as a significant breakthrough. In the beginning of the present century few dimensional visualization methods were in use as a part 2D/3D node-link diagram [ 122 ]. Already at this level of abstraction, any user may classify the goal and specify further analytical steps for the research, but unfortunately, data scaling became an essential issue.
Moreover, currently used technologies for data visualization are already causing enormous resource demands which include high memory requirements and extremely high deployment cost. However, the currently existing environment faces a new limitation based on the large amounts of data to be visualized in contrast to past imagination issue. Modern effective methods are focused on representation in specified rooms equipped with widescreen monitors or projectors [ 123 ].
Nowadays, there are a fairly large number of data visualization tools offering different possibilities. These tools can be classified based on three factors: by the data type, by visualization technique type and by the interoperability. The first refers to the different types of data to be visualized [ 124 ]:
Univariate data One dimensional arrays, time series, etc.
Two-dimensional data Point two-dimensional graphs, geographical coordinates, etc.
Multidimensional data Financial indicators, results of experiments, etc.
Texts and hypertexts Newspaper articles, web documents, etc.
Hierarchical and links The structure subordination in the organization, e-mails, documents and hyperlinks, etc.
Algorithms and programs Information flows, debug operations, etc.
The second factor is based on visualization techniques and samples to represent different types of data . Visualization techniques can be both elementary (line graphs, charts, bar charts) and complex (based on the mathematical apparatus). Furthermore, visualization can be performed as a combination of various methods. However, visualized representation of data is abstract and extremely limited by one’s perception capabilities and requests (see Fig. 4 ).
Human perception capability issue. Human perceptional capabilities are not sufficient to embrace large amount of data
Types of visualization techniques are listed below:
2D/3D standard figure [ 125 ]. May be implemented as bars, line graphs, various charts, etc. (see Fig. 5 ). The main drawback of this type is the complexity of the acceptable visualization for complicated data structures;
Geometric transformations [ 126 ]. This technique represents information as scatter diagram (see Fig. 6 ). This type is geared towards a multi-dimensional data set’s transformation in order to display it in Cartesian and non-Cartesian geometric spaces. This class includes methods of mathematical statistics;
Display icons [ 127 ]. Ruled shapes (needle icons) and star icons. Basically, this type displays the values of elements of multidimensional data in properties of images (see Fig. 7 ). Such images may include human faces, arrows, stars, etc. Images can be grouped together for holistic analysis. The result of the visualization is a texture pattern, which varies according to the specific characteristics of the data;
Methods focused on the pixels [ 128 ]. Recursive templates and cyclic segments. The main idea is to display the values in each dimension into the colored pixel and to merge some of them according to specific measurements (see Fig. 8 ). Since one pixel is used to display a single value, therefore visualization of large amounts of data can be reachable with this methodology;
Hierarchical images [ 129 ]. Tree maps and overlay measurements (see Fig. 9 ). These type methods are used with the hierarchical structured data.
The third factor is related to the interoperability with visual imagery and techniques for better data analysis. The application used for the visualization should present visual forms that capture the essence of data itself. However, it is not always enough for a complete analysis. Data representation should be constructed in order to allow a user to have different visual points of view. Thus, the appropriate compatibility should be performed:
Dynamic projection [ 130 ]. Non-static change of projections in multidimensional data sets is used. An example of the dynamic projection in two-dimensional plane of multidimensional data in a scatter plots. It is necessary to note that the number of possible projections increases exponentially with the number of measurements and, thus, perception suffers more.
Interactive filtering [ 131 ]. In the investigation of large amounts of data there is a need to share data sets and highlight significant subsets in order to filter images. Significantly, that there should be an opportunity to have a visual representation in real time. A subset can be chosen either directly from a list or by determining a subset of the properties of interest;
Scaling images [ 132 ]. Scaling is a well-known method of interaction used in many applications. Especially for Big Data processing, this method is very useful due to the ability to represent data in a compressed form. It provides the ability to simultaneously display any part of an image in a more detailed form. Nevertheless, a lower level entity may be represented by a pixel at a higher level, a certain visual image or an accompanying text label;
Interactive distortion [ 133 ] supports the research process data using distortion scale with partial detail. The basic idea of this method is that a part of the fine granularity displayed data is shown in addition to one with a low level of details. The most popular methods are hyperbolic and spherical distortion;
Interactive combination [ 134 , 135 ] brings together a combination of different visualization techniques to overcome specific deficiencies by their conjugation. For example, different points of the dynamic projection can be combined with the techniques of coloring.
To summarize, any visualization method can be classified by data type, visualization technique and interoperability. Each method can support different types of data, various images and varied methods for interaction.
An example of the 2D/3D standard figures visualization techniques. a The simple line graph and b example of a bar chart
An example of the geometric transformations visualization techniques. a Example of a parallel coordinates and b the scatter plot
An example of the display icons visualization techniques. Picture demonstrates the visualization of various social connections in Australia
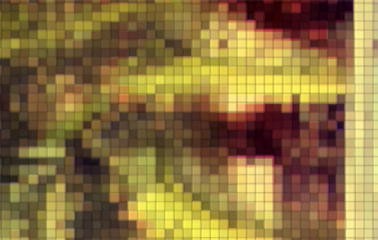
An example of the methods focused on the pixels. Picture demonstrates an amount of data visualized in pixels. Each color has its specific meaning
An example of the hierarchical images. Picture illustrates a tree map of data
A visual representation of Big Data analysis is crucial for its interpretation. As it was already mentioned, it is evident that human perception is limited. The main purpose of modern data representation methods is related to improvement in forms of images, diagrams or animation. Examples of well known techniques for data visualization are presented below [ 136 ]:
Tag cloud [ 137 ] is used in text analysis, with a weighting value dependent on the frequency of use (citation) of a particular word or phrase (see Fig. 10 ). It consists of an accumulation of lexical items (words, symbols or combination of the two). This technique is commonly integrated with web sources to quickly familiarize visitors with the content via key words.
Clustergram [ 138 ] is an imaging technique used in cluster analysis by means of representing the relation of individual elements of the data as they change their number (see Fig. 11 ). Choosing the optimal number of clusters is also an important component of cluster analysis.
Motion charts allow effective exploration of large and multivariate data and interact with it utilizing dynamic 2D bubble charts (see Fig. 12 ). The blobs (bubbles—central objects of this technique) can be controlled due to variable mapping for which it is designed. For instance, motion charts graphical data tools are provided by Google [ 139 ], amCharts [ 140 ] and IBM Many Eyes [ 141 ].
Dashboard [ 142 ] enables the display of log files of various formats and filter data based on chosen data ranges (see Fig. 13 ). Traditionally, dashboard consists of three layers [ 143 ]: data (raw data), analysis (includes formulas and imported data from data layer to tables) and presentation (graphical representation based on the analysis layer)
Nowadays, there are many publicly available tools to create meaningful and attractive visualizations. For instance, there is a chart of open visualization tools for data visualization and analysis published by Sharon Machils [ 144 ]. The author provides a list, which contains more than 30 tools from easiest to most difficult: Zoho Reports, Weave, Infogr.am, Datawrapper and others.
An example of the tag cloud. This picture illustrates visualization of the paper abstract
An example of the clustergram. This picture illustrates different state of data in several clusters
An example of the motion chart. This picture illustrates the data in forms of bubbles that have various meaning based on color and size
An example of the dashboard. This picture illustrates pie chart, visualization of data in pixels, line graph and bar chart
All of these modern methods and tools follow fundamental cognitive psychology principles and use the essential criteria of data successful representation [ 145 ] such as manipulation of size, color and connections between visual objects (see Fig. 14 ). In terms of human cognition, the Gestalt Principles [ 146 ] are relevant. The basis of Gestalt psychology is a study of visual perception. It suggests that people tend to perceive the world in a form of holistic ordered configuration rather than constituent fragments (e.g. at first, person perceives forest and after that can identify single trees as part of the whole). Moreover, our mind fills in the gaps, seeks to avoid uncertainty and easily recognizes similarities and differences. The main Gestalt principles such as law of proximity (collection of objects forming a group), law of similarity (objects are grouped perceptually if they are similar to each other), symmetry (people tend to perceive object as symmetrical shapes), closure (our mind tends to close up objects that are not complete) and figure-ground law (prominent and recessed roles of visual objects) should be taken into account in Big Data Visualization.
Fundamental cognitive psychology principles. Color is used to catch significant differences in the data sets by view; manipulation of visual object sizes may assist persons to identify the most important elements of the information; representation of connections improves patterns identifications and aims to facilitate data analysis; Grouping objects using similarity principle decreases cognitive load
To this end, the most effective visualization method is the one that uses multiple criteria in the optimal manner. Otherwise, too many colors, shapes, and interconnections may cause difficulties in the comprehension of data, or some visual elements may be too complex to recognize.
After observation and discussion about existing visualization methods and tools for Big Data, we can clarify and outline its important disadvantages that are sufficiently discussed by specialists from different fields [ 147 – 149 ]. Various ways of data interpretation make them meaningful. It is easy to distort valuable information in its visualization, because a picture convinces people more effectively than textual content. Existing visualization tools aim to create as simple and abstract images as possible, which can lead to a problem when significant data can be interpreted as disordered information and important connections between data units will be hidden from the user. It is a problem of visibility loss, which also refers to display resolution, where the quality of represented data depends on number of pixels and their density. A solution may be in the use of larger screens [ 150 ]. However, this concept brings a problem of human brain cognitive-perceptual limitations, as will be discussed in detail in the section Integration with Augmented and Virtual Reality.
Using visual and automated methods in Big Data processing gives a possibility to use human knowledge and intuition. Moreover, it becomes possible to discover novel solutions for complex data visualization [ 151 ]. Vast amounts of information motivate researchers and developers to create new tools for quick and accurate analysis. As an example, the rapid development of visualization techniques may be concerned. In the world of interconnected research areas, developers need to combine existing basic, effective visualization methods with new technological opportunities to solve the central problems and challenges of Big Data analysis.
Integration with augmented and virtual reality
It is well known that the vision perception capabilities of the human brain are limited [ 152 ]. Furthermore, handling a visualization process on currently used screens requires high costs in both time and health. This leads to the need of its proper usage in the case of image interpretation. Nevertheless, the market is in the process of being flooded with countless numbers of wearable devices [ 153 , 154 ] as well as various display devices [ 155 , 156 ].
The term Augmented Reality was invented by Tom Caudell and David Mizel in 1992 and meant to describe data produced by a computer that is superimposed to the real world [ 157 ]. Nevertheless, Ivan Sutherland created the first AR/VR system already in 1968. He developed the optical see-through head-mounted display that can reveal simple three-dimensional models in real time [ 158 ]. This invention was a predecessor to the modern VR displays and AR helmets [ 159 ] that seem to be an established research and industrial area for the coming decade [ 160 ]. Applications for use have already been found in military [ 161 ], education [ 162 ], healthcare [ 163 ], industry [ 164 ] and gaming fields [ 165 ]. At the moment, the Oculus Rift [ 166 ] helmet gives many opportunities for AR practice. Concretely, it will make it possible to embed virtual content into the physical world. William Steptoe has already done research in this field. The use of it in the visualization area might solve many issues from narrow visual angle, navigation, scaling, etc. For example, offering a way to have a complete 360-degrees view with a helmet can solve an angle problem. On the other hand, a solution can be obtained with help of specific widescreen rooms, which by definition involves enormous budgets. Focusing on the combination of dynamic projection and interactive filtering visualization methods, AR devices in combination with motion recognition tools might solve a significant scaling problem especially for multidimensional representations that comes to this area from the field of Architecture. Speaking more precisely, designers (specialized in 3D-visualization) work with flat projections in order to produce a visual model [ 167 ]. However, the only option to present a final image is in moving around it and thus navigation inside the model seems to be another influential issue [ 168 ].
From the Big Data visualization point of view, scaling is a significant issue mainly caused by multidimensional systems where a need to delve into a branch of information in order to obtain some specific value or knowledge takes its place. Unfortunately, it cannot be solved from a static point of view. Likewise, integration with motion detection wearables [ 169 ] would highly increase such visualization system usability. For example, the additional use of an MYO armband [ 170 ] may be a key to the interaction with visualized data in the most native way. Similar comparison may be given as a pencil-case in which one tries to find a sharpener and spreads stationery with his/her fingers.
However, the use of AR displays and helmets is also limited by specific characteristics of the human eye (visual system), such as field of view and/or diseases like scotoma [ 171 ] and blind spots [ 172 ]. Central vision [ 173 ] is most significant and necessary for human activities such as reading or driving. Additionally, it is responsible for accurate vision in the pointed direction and takes most of the visual cortex in the brain but its retinal size is less than 1 % [ 174 ]. Furthermore, it captures only two degrees of the vision field, which stays the most considerable for text and object recognition. Nevertheless, it is supported with Peripheral vision which is responsible for events outside the center of gaze. Many researchers around the world are currently working with virtual and AR to train young professionals [ 175 – 177 ], develop new areas [ 178 , 179 ] and analyze the patient’s behavior [ 180 ].
Despite the well known topics like colorblindness, natural field of view and other physiological abnormalities, recent research by Israel Abramov et al. [ 181 ] is overviewing physiological gender and age differences based on the cerebral cortex and its large number of testosterone receptors [ 182 ], as a basis for the variety in perception procedures. The study was mainly about the focused image onto the retina at the back of the eyeball and its visual system processing. We overview the main reasons for those differences, starting from prehistoric times, when African habitats in forest regions had limited distance for object detection and identification, thus obtained higher acuity for males may be explained. Also, sex differences might be related to different roles in the survival commune. So that males were mainly hunting (hunter-gatherer hypothesis)—they had to detect enemies and predators much faster [ 183 ]. Moreover, there are significant gender differences for far- and near-vision: males have their advantage in a far-space [ 184 ]. On the other hand, females are much more susceptible for brightness and color changes in addition to static objects in near-space [ 185 ]. However, we can conclude that male/female differences in the sensory capacities are adaptive but should be considered in order to optimize represented and visualized data for end-uses. Additionally, there exists a research area focusing on the human eye movement patterns during the perception of scenes and objects. It can be based on different factors starting from particular culture peculiar properties [ 186 ] and up to specific search tasks [ 187 ] being in high demand for Big Data visualization purposes.
Further studies shall be focused on the usage of ophthalmology and neurology for the development of the new visualization tools. Basically, such cross-discipline collaboration would support decision making for the image position selection, which is mainly related to the problem of the significant information losses due to the vision angle extension. Moreover, it is highly important to take in account current hardware quality and screens resolution in addition to the software part. Nevertheless, there is a need of the improvement for multicore GPU processors besides the address bus throughput refinement between CPU and GPU or even replacement for wireless transfer computations on cluster systems. Never the less, it is significant to discuss current visualization challenges to support future research.
Future research agenda and data visualization challenges
Visualized data can significantly improve the understanding of the preselected information for an average user. In fact, people start to explore the world using visual abilities since birth. Images are often more easier to perceive in comparison to text. In the modern world, we can see clear evolution towards visual data representation and imagery experience. Moreover, visualization software becomes ubiquitous and publicly available for ordinary user. As a result, visual objects are widely distributed—from social media to scientific papers and, thus, the role of visualization while working with large amount of data should be reconsidered. In this section, we overview important challenges and possible solutions related to future agenda for Big Data visualization with AR and VR usage:
Application development integration In order to operate with visualized objects, it is necessary to create a new interactive system for the user. It should support such actions as: scaling; navigating in visualized 3D space; selecting sub-spaces, objects, groups of visual elements (flow/path elements) and views; manipulating and placing; planning routes of view; generating, extracting and collecting data (based on the reviewed visualized data). A novel system should allow multimodal control by voice and/or gestures in order to make it more intuitive for users as it is shown in [ 188 – 190 ] and [ 191 ]. Nevertheless, one of the main issues regarding this direction of development is the fact that implementing effective gestural and voice interaction is not a trivial matter. There is a need to develop a machine learning system and to define basic intuitive gestures that are currently in research for general [ 192 – 194 ] and more specific (medical) purposes [ 195 ].
Equipment and virtual interface It is necessary to apply certain equipment for the implementation of such an interactive system in practice. Currently, there are optical and video see-trough head-mounted displays (HMD) [ 196 ] that merge virtual objects into the real scene view. Both have the following issues: distortion and resolution of the real scene; delay of a system; viewpoint matching; engineering and cost factors . As for the interaction issue, for an appropriate haptic feedback in an MR environment there is a need to create a framework that would allow an interaction with intuitive gesture . As it is revealed in the section Integration with Augmented and Virtual Reality, glove-based systems [ 197 ] are mainly used for virtual object manipulation. The disadvantage of hand-tracking input is so that there is no tactile feedback. In summary, the interface should be redesigned or reinvented in order to simplify user interaction. Software engineers should create new approaches, principles and methods in User Interface Design to make all instruments easily accessible and intuitive to use.
Tracking and recognition system Objects and tools have to be tracked in virtual space . The position and orientation values of virtual items are dynamic and have to be re-estimated during presentation. Tracking head movement is another significant challenge. It aims to avoid mismatch of the real view scene and computer generated objects. This challenge may be solved by using more flexible software platforms.
Perception and cognition Actually, the level of computer operation is high but still not sufficiently effective in comparison to human brain performance even in cases of neural networks. As was mentioned earlier in the section Integration with Augmented and Virtual Reality, human perception and cognition have their own characteristics and features, and the consideration of this issue by developers during hardware and interface design for AR is vital. In addition, the user’s ability to recognize and understand the data is a central issue. Tasks such as browsing and searching require a certain cognitive activity. Also, there can be issues related to different users’ reactions with regard to visualized objects depending on their personal and cultural backgrounds. In this sense, simplicity in information visualization has to be achieved in order to avoid misperceptions and cognitive overload [ 198 ]. Psychophysical studies would provide answers to questions regarding perception and would give the opportunity to improve performance by motion prediction.
Virtual and physical objects mismatch In an Augmented Reality environment, virtual images integrate with real world scenery at the static distance in the display while the distance to real objects varies. Consequently, a mismatch of virtual and physical distances is irreversible and it may result in incorrect focus, contrast and brightness of virtual objects in comparison to real ones. The human eye is capable of recognizing many levels of brightness, saturation and contrast [ 199 ], but most contemporary optical technologies cannot display all levels appropriately. Moreover, potential optical illusions arise from conflicts between computer-generated and real environment objects. Using modern equipment would be a solution for this challenge.
Screen limitations With the current technology development level, visualized information is presented mainly on screens. Even a VR helmet is equipped with two displays. Unfortunately, and because of the close-to-the-eye proximity, users can experience lack of comfort while working with it. It is mainly based on a low display resolution and high graininess and, thus, manufacturers should take it into consideration for further improvement.
Education As this concept is relatively new, there is a need to specify the value of the data visualization and its contribution to the users’ work. The value cannot be so obvious; that is why compelling showcase examples and publicly available tutorials can reveal AR and VR potential in visual analytics. Moreover, users need to be educated and trained for the oncoming interaction with this evolving technology. The visual literacy skill should be improved in order to have high performance while working with visualized objects. A preferable guideline can be chosen as Visual Information-Seeking Mantra: overview first, zoom and filter, then details on demand [ 200 ].
Despite all the challenges, the main benefit from the implementation of MR approach is human experience improvement. At the same time, such visualization allows convenient access to huge amounts of data and provides a view from different angles. The navigation is smooth and natural via tangible and verbal interaction. It also minimizes perceptional inaccuracy in data analysis and makes visualization powerful at conveying knowledge to the end user. Furthermore, it ensures actionable insights that improves decision making.
In conclusion, challenges of data visualization for AR and VR are associated not only with current technology development but also with human-centric issues. Interestingly, some researchers are already working on the conjugation of such complex fields as massive data analysis, its visualization and complex control of the visualized environment [ 201 ]. It is worthwhile to note that those factors should be taken into account simultaneously in order to achieve the best outcome for the established industrial field.
In practice, there are a lot of challenges for Big Data processing and analysis. As all the data is currently visualized by computers, it leads to difficulties in the extraction of data, followed by its perception and cognition. Those tasks are time-consuming and do not always provide correct or acceptable results.
In this paper we have obtained relevant Big Data Visualization methods classification and have suggested the modern tendency towards visualization-based tools for business support and other significant fields. Past and current states of data visualization were described and supported by analysis of advantages and disadvantages. The approach of utilizing VR, AR and MR for Big Data Visualization is presented and the advantages, disadvantages and possible optimization strategies of those are discussed.
For visualization problems discussed in this work, it is critical to understand the issues related to human perception and limited cognition. Only after that, the field of design can provide more efficient and useful ways to utilize Big Data. It can be concluded that data visualization methodology may be improved by considering fundamental cognitive psychological principles and by implementing most natural interaction with visualized virtual objects. Moreover, extending it with functions to exclude blind spots and decreased vision sectors would highly improve recognition time for people with such a disease. Furthermore, a step towards wireless solutions would extend device battery life in addition to computation and quality improvements.
Manyika J, Chui M, Brown B, Bughin J, Dobbs R, Roxburgh C, Byers AH. Big Data: the next frontier for innovation, competition, and productivity. June Progress Report. McKinsey Global Institute; 2011.
1000 Genomes: a Deep Catalog of Human Genetic Variation. 2015. http://www.1000genomes.org/ .
Via M, Gignoux C, Burchard EG. The 1000 Genomes Project: new opportunities for research and social challenges. Genome Med 2010;2(3)
Internet Archive: Internet Archive Wayback Machine. 2015. http://archive.org/web/web.php .
Nielsen J. Comparing content in web archives: differences between the Danish archive Netarkivet and Internet Archive. In: Two-day Conference at Aarhus University, Denmark. 2015
The Lemur Project: The ClueWeb09 Dataset. 2015. http://lemurproject.org/clueweb09.php/ .
Russom P. Managing Big Data. TDWI Best Practices Report, TDWI Research; 2013.
Gantz J, Reinsel D. The digital universe in 2020: Big data, bigger digital shadows, and biggest growth in the far east. IDC iView IDC Anal Future. 2012;2007:1–16.
Google Scholar
Beyer MA, Laney D. The importance of “Big Data”: a definition. Stamford: Gartner; 2012.
Demchenko Y, Ngo C, Membrey P. Architecture framework and components for the big data ecosystem. J Syst Netw Eng 2013;1–31 [SNE technical report SNE-UVA-2013-02] .
Turner V, Reinsel D, Gantz JF, Minton S. The Digital Universe of Opportunities: Rich Data and the Increasing Value of the Internet of Things. IDC Analyze the Future 2014.
Husain SS, Kalinin A, Truong A, Dinov ID. SOCR data dashboard: an integrated Big Data archive mashing medicare, labor, census and econometric information. J Big Data. 2015;2(1):1–18.
Article Google Scholar
Keahey TA. Using visualization to understand Big Data. IBM Business Analytics Advanced Visualisation; 2013.
Microsoft Corporation: Power BI—Microsoft. 2015. https://powerbi.microsoft.com/ .
Amazon.com, Inc. Amazone Web Services. 2015. https://aws.amazon.com/ .
Google, Inc. Google Cloud Platform. 2015. https://cloud.google.com/ .
Socrata. Data to the People. 2015. http://www.socrata.com .
D3.js: D3 Data-Draven Documents. 2015. http://d3js.org .
The Cytoscape Consortium: Network Data Integration, Analysis, and Visualization in Box. 2015. http://www.cytoscape.org .
Tableau—Business Intelligence and Analytics. http://tableau.com/
Kandel S, Paepcke A., Hellerstein J, Heer J. Wrangler: interactive visual specification of data transformation scripts. In: Proceedings of the SIGCHI Conference on Human Factors in Computing Systems, ACM; 2011. pp 3363–72.
Schaefer D, Chandramouly A, Carmack B, Kesavamurthy K. Delivering Self-Service BI, Data Visualization, and Big Data Analytics. Intel IT: Business Intelligence; 2013.
Choy J, Chawla V, Whitman L. Data Visualization Techniques: From Basics to Big Data with SAS Visual Analytics. SAS: White Paper; 2013.
Ganore P. Need to know what Big Data is? ESDS—Enabling Futurability. 2012.
Agrawal D, Das S, El Abbadi A. Big Data and cloud computing: current state and future opportunities. In: Proceedings of the 14th International Conference on Extending Database Technology, ACM; 2011. pp 530–3 (2011).
Kaur M. Challanges and issues during visualization of Big Data. Int J Technol Res Eng. 2013;1:174–6.
Childs H, Geveci B, Schroeder W, Meredith J, Moreland K, Sewell C, Kuhlen T, Bethel EW. Research challenges for visualization software. Computer. 2013;46:34–42.
Press G. 12 Big Data definitions: what’s yours? Forbes; 2014.
Dutcher J. What is Big Data? Berkley School of Information; 2014.
Bashour N. The Big Data Blog, Part V: Interview with Dr. Ivo Dinov. 2014. http://www.aaas.org/news/big-data-blog-part-v-interview-dr-ivo-dinov .
Komodakis N, Pesquet JC. Playing with duality: an overview of recent primal-dual approaches for solving large-scale optimization problems. arXiv preprint. 2014. http://arXiv:1406.5429 .
Manicassamy J, Kumar SS, Rangan M, Ananth V, Vengattaraman T, Dhavachelvan P. Gene suppressor: an added phase towards solving large scale optimization problems in genetic algorithm. Appl Soft Comp; 2015.
Gartner — IT Glossary. Big Data defintion. http://www.gartner.com/it-glossary/big-data/ .
Sicular S. Gartner’s Big Data Definition Consists of Three Parts, Not to Be Confused with Three “V”s, Gartner, Inc. Forbes; 2013.
Demchenko Y, De Laat C, Membrey P. Defining architecture components of the Big Data Ecosystem. In: Proceedings of International Conference on Collaboration Technologies and Systems (CTS), IEEE; 2014. pp 104–12 .
Akerkar R. Big Data computing. Boca Raton, FL: CRC Press, Taylor & Francis Group; 2013.
Book Google Scholar
Sethi IK, Jain AK. Artificial neural networks and statistical pattern recognition: old and new connections, vol. 1. New York: Elsevier; 2014.
Araghinejad S. Artificial neural networks. In: Data-driven modeling: using MATLAB in water resources and environmental engineering. Netherlands: Springer; 2014. pp 139–94.
Larose DT. Discovering knowledge in data: an introduction to data mining. Hoboken, NJ: John Wiley & Sons; 2014.
Maren AJ, Harston CT, Pap RM. Handbook of Neural Computing Applications. Academic Press; 2014.
Schmidhuber J. Deep learning in neural networks: an overview. Neural Netw. 2015;61:85–117.
McCue C. Data mining and predictive analysis: intelligence gathering and crime analysis. Butterworth-Heinemann; 2014.
Rudin C, Dunson D, Irizarry R, Ji H. Laber E, Leek J, McCormick T, Rose S, Schafer C, van der Laan M et al. Discovery with data: leveraging statistics with computer science to transform science and society. 2014.
Cressie N. Statistics for spatial data. Hoboken, NJ: John Wiley & Sons; 2015.
Lehnert WG, Ringle MH. Strategies for natural language processing. Hove, United Kingdom: Psychology Press; 2014.
Chu WW, editor. Data mining and knowledge discovery for Big Data. Studies in Big Data, vol. 1. Heidelberg: Springer; 2014.
Berry MJ, Linoff G. Data mining techniques: for marketing, sales, and customer support. New York: John Wiley & Sons; 1997.
PhridviRaj M, GuruRao C. Data mining-past, present and future-a typical survey on data streams. Procedia Technol. 2014;12:255–63.
Zaki MJ, Meira W Jr. Data mining and analysis: fundamental concepts and algorithms. Cambridge: Cambridge University Press; 2014.
Sutskever I, Vinyals O, Le QV. Sequence to sequence learning with neural networks. In: Advances in Neural Information Processing Systems; 2014. 3104–12.
Rojas R, Feldman J. Neural networks: a systematic introduction. Springer; 2013.
Gurney K. An introduction to neural networks. Taylor and Francis; 2003.
Mohri M, Rostamizadeh A, Talwalkar A. Foundations of Machine Learning. Adaptive computation and machine learning series: MIT Press; 2012.
Murphy KP. Machine learning: a probabilistic perspective. Adaptive computation and machine learning series. MIT Press; 2012.
Alpaydin E. Introduction to machine learning. Adaptive Computation and Machine Learning Series. MIT Press; 2014.
Vetterli M, Kovačević J, Goyal VK. Foundations of signal processing. Cambridge University Press; 2014.
Xhafa F, Barolli L, Barolli A, Papajorgji P. Modeling and Processing for Next-Generation Big-Data Technologies: With Applications and Case Studies. Modeling and Optimization in Science and Technologies: Springer; 2014.
Giannakis GB, Bach F, Cendrillon R, Mahoney M, Neville J. Signal processing for Big Data. Signal Process Mag IEEE. 2014;31(5):15–6.
Shneiderman B. The big picture for Big Data: visualization. Science. 2014;343:730.
Marr B. Big Data: using SMART Big Data. Analytics and Metrics To Make Better Decisions and Improve Performance: Wiley; 2015.
Minelli M, Chambers M, Dhiraj A. Big Data, big analytics: emerging business intelligence and analytic trends for today’s businesses. Wiley CIO: Wiley; 2012.
Puget JF. Optimization Is Ready For Big Data. IBM White Paper (2015)
Poli R, Rowe JE, Stephens CR, Wright AH. Allele diffusion in linear genetic programming and variable-length genetic algorithms with subtree crossover. Springer; 2002.
Langdon WB. Genetic programming and data structures: genetic programming + data structures = Automatic Programming!, vol. 1. Springer; 2012.
Poli R, Koza J. Genetic programming. Springer; 2014.
Kothari DP. Power system optimization. In: Proceedings of 2nd National Conference on Computational Intelligence and Signal Processing (CISP), IEEE; 2012; pp 18–21.
Moradi M, Abedini M. A combination of genetic algorithm and particle swarm optimization for optimal DG location and sizing in distribution systems. Int J Elect Power Energ Syst. 2012;34(1):66–74.
Engelbrecht A. Particle swarm optimization. In: Proceedings of the 2014 Conference Companion on Genetic and Evolutionary Computation Companion, ACM; 2014. pp 381–406.
Melanie M. An introduction to genetic algorithms. Cambridge, Massachusetts London, England, Fifth printing; 1999. p 3.
Kitchin R. The data revolution: big data, open data. Data infrastructures and their consequences. SAGE Publications; 2014.
Pébay P, Thompson D, Bennett J, Mascarenhas A. Design and performance of a scalable, parallel statistics toolkit. In: Proceedings of International Symposium on Parallel and Distributed Processing Workshops and Phd Forum (IPDPSW), IEEE; 2011. pp 1475–84.
Bennett J, Grout R, Pébay P, Roe D, Thompson D. Numerically stable, single-pass, parallel statistics algorithms. In: International Conference on Cluster Computing and Workshops, IEEE; 2009. pp 1–8.
Lake P, Drake R. Information systems management in the Big Data era. Advanced information and knowledge processing. Springer; 2015.
Anselin L, Getis A. Spatial statistical analysis and geographic information systems. In: Perspectives on Spatial Data Analysis, Springer; 2010. pp 35–47.
Kaufman L, Rousseeuw PJ. Finding groups in data: an introduction to cluster analysis, vol. 344. John Wiley and Sons; 2009.
Anderberg MR. Cluster analysis for applications: probability and mathematical statistics: a series of monographs and textbooks, vol 19, Academic press; 2014.
Hastie T, Tibshirani R, Friedman J. Unsupervised Learning. Springer; 2009.
Fisher DH, Pazzani MJ, Langley P. Concept formation: knowledge and experience in unsupervised learning. Morgan Kaufmann; 2014.
McKenzie M, Wong S. Subset selection of training data for machine learning: a situational awareness system case study. In: SPIE Sensing Technology + Applications. International Society for Optics and Photonics; 2015.
Aggarwal CC. Data classification: algorithms and applications. CRC Press; 2014.
Ryan TP. Modern regression methods. Wiley Series in Probability and Statistics. John Wiley & Sons; 2008.
Zhang C, Zhang S. Association rule mining: models and algorithms. Springer; 2002.
Cleophas TJ, Zwinderman AH. Machine learning in medicine: part two. Machine learning in medicine: Springer; 2013.
Bishop CM. Pattern recognition and machine learning. Springer; 2006.
Devroye L, Györfi L, Lugosi G. A probabilistic theory of pattern recognition, Vol. 31, Springer; 2013.
Powers DM, Turk CC. Machine learning of natural language. Springer; 2012.
Liu B, Zhang L. A survey of opinion mining and sentiment analysis. In: Mining Text Data, Springer; 2012. p. 415–63.
Polikar R. Ensemble learning. In: Ensemble Machine Learning, Springer; 2012. p. 1–34.
Zhang C, Ma Y. Ensemble machine learning. Springer; 2012.
Helstrom CW. Statistical theory of signal detection: international series of monographs in electronics and instrumentation, Vol. 9, Elsevier; 2013.
Shumway RH, Stoffer DS. Time series analysis and its applications. Springer; 2013.
Akaike H, Kitagawa G. The practice of time series analysis. Springer; 2012.
Viswanathan R. Data fusion. In: Computer Vision, Springer; 2014. p. 166–68.
Castanedo F. A review of data fusion techniques. Sci World J 2013. 2013.
Thompson D, Levine JA, Bennett JC, Bremer PT, Gyulassy A, Pascucci V, Pébay PP. Analysis of large-scale scalar data using hixels. In: Proceedings of Symposium on Large Data Analysis and Visualization (LDAV), IEEE; 2011. p. 23–30.
Report: Data Visualization Applications Market Future Of Decision Making Trends, Forecasts And The Challengers (2014–2019). Mordor Intelligence; 2014.
SAS: Data visualization: making big data approachable and valuable. Market Pulse: White Paper (2013)
Simon P. The visual organization: data visualization, Big Data, and the quest for better decisions. John Wiley & Sons; 2014.
Kaisler S, Armour F, Espinosa JA, Money W. Big Data: issues and challenges moving forward. In: Proceedings of 46th Hawaii International Conference on System Sciences (HICSS), IEEE; 2013. p. 995–1004.
Tole AA, et al. Big Data challenges. Database Syst J. 2013;4(3):31–40.
MathSciNet Google Scholar
Chen M, Mao S, Zhang Y, Leung VC. Big Data: related technologies. Challenges and future prospects: Springer; 2014.
Miksch S, Aigner W. A matter of time: applying a data-users-tasks design triangle to visual analytics of time-oriented data. Comp Graph. 2014;38:286–90.
MiilIer W, Schumann H. Visualization method for time-dependent data—an overview. In: Proceedings of the 2003 Winter Simulation Conference, vol. 1. IEEE; 2003.
Telea AC. Data visualization: principles and practice, Second Edition. Taylor and Francis; 2014.
Wright H. Introduction to scientific visualization. Springer; 2007.
Bonneau GP, Ertl T, Nielson G. Scientific Visualization: The Visual Extraction of Knowledge from Data. Mathematics and Visualization: Springer; 2006.
Rosenblum L, Rosenblum LJ. Scientific visualization: advances and challenges. Policy Series; 19. Academic; 1994.
Ware C. Information visualization: perception for design. Morgan Kaufmann; 2013.
Kerren A, Stasko J, Fekete JD. Information Visualization: Human-Centered Issues and Perspectives. LNCS sublibrary: Information systems and applications, incl. Internet/Web, and HCI. Springer; 2008.
Mazza R. Introduction to information visualization. Computer science: Springer; 2009.
Bederson BB, Shneiderman B. The Craft of Information Visualization: Readings and Reflections. Interactive Technologies: Elsevier Science; 2003.
Dill J, Earnshaw R, Kasik D, Vince J, Wong PC. Expanding the frontiers of visual analytics and visualization. SpringerLink: Bücher. Springer; 2012.
Simoff S, Böhlen MH, Mazeika A. Visual data mining: theory, techniques and tools for visual analytics. LNCS sublibrary: Information systems and applications, incl. Internet/Web, and HCI. Springer; 2008.
Zhang Q. Visual analytics and interactive technologies: data, text and web mining applications: data. Information Science Reference: Text and Web Mining Applications. Premier reference source; 2010.
Few S, EDGE P. Data visualization: past, present, and future. IBM Cognos Innovation Center; 2007.
Bertin J. La graphique. Communications. 1970;15:169–85.
Gray JJ. Johann Heinrich Lambert, mathematician and scientist, 1728–1777. Historia Mathematica. 1978;5:13–41.
Article MATH MathSciNet Google Scholar
Tufte ER. The visual display for quantitative information. Chelshire: Graphics Press; 1983.
Kehrer J, Boubela RN, Filzmoser P, Piringer H. A generic model for the integration of interactive visualization and statistical computing using R. In: Conference on Visual Analytics Science and Technology (VAST), IEEE; 2012. p. 233–34.
Härdle W, Klinke S, Turlach B. XploRe: an Interactive Statistical Computing Environment. Springer; 2012.
Friendly M. A brief history of data visualization. Springer; 2006.
Mering C. Traditional node-link diagram of a network of yeast protein-protein and protein-DNA interactions with over 3,000 nodes and 6,800 links. Nature. 2002;417:399–403.
Febretti A, Nishimoto A, Thigpen T, Talandis J, Long L, Pirtle J, Peterka T, Verlo A, Brown M, Plepys D et al. CAVE2: a hybrid reality environment for immersive simulation and information analysis. In: IS&T/SPIE Electronic Imaging (2013). International Society for Optics and Photonics
Friendly M. Milestones in the history of data visualization: a case study in statistical historiography. In: Classification—the Ubiquitous Challenge, Springer; 2005. p. 34–52.
Tory M, Kirkpatrick AE, Atkins MS, Moller T. Visualization task performance with 2D, 3D, and combination displays. IEEE Trans Visual Comp Graph. 2006;12(1):2–13.
Stanley R, Oliveria M, Zaiane OR. Geometric data transformation for privacy preserving clustering. Departament of Computing Science; 2003.
Healey CG, Enns JT. Large datasets at a glance: combining textures and colors in scientific visualization. IEEE Trans Visual Comp Graph. 1999;5(2):145–67.
Keim DA. Designing pixel-oriented visualization techniques: theory and applications. IEEE Trans Visual Comp Graph. 2000;6(1):59–78.
Kamel M, Camphilho A. Hierarchic Image Classification Visualization. In: Proceedings of Image Analysis and Recognition 10th International Conference, ICIAR; 2013.
Buja A, Cook D, Asimov D, Hurley C. Computational methods for high-dimensional rotations in data visualization. Handbook Stat Data Mining Data Visual. 2004;24:391–415.
Meijester A, Westenberg MA, Wilkinson MHF. Interactive shape preserving filtering and visualization of volumetric data. In: Proceedings of the Fourth IASTED International Conference; 2002. p. 640–43.
Borg I, Groenen P. Modern multidimensional scaling: theory and applications. J Educ Measure. 2003;40:277–80.
Bajaj C, Krishnamurthy B. Data visualization techniques, vol. 6. Wiley; 1999.
Plaisant C, Monroe M, Meyer T, Shneiderman B. Interactive visualization. CRC Press; 2014.
Janvrin DJ, Raschke RL, Dilla WN. Making sense of complex data using interactive data visualization. J Account Educ. 2014;32(4):31–48.
Manyika J, Chui M, Brown B, Bughin J, Dobbs R, Roxburgh C, Byers AH. Big data: the next frontier for innovation, competition, and productivity. McKinsey Global Institute; 2011.
Ebert A, Dix A, Gershon ND, Pohl M. Human Aspects of Visualization: Second IFIP WG 13.7 Workshop on Human-Computer Interaction and Visualization, HCIV (INTERACT), Uppsala, Sweden, August 24, 2009, Revised Selected Papers. LNCS sublibrary: Information systems and applications, incl. Internet/Web, and HCI. Springer; 2009. p 2011.
Schonlau M. Visualizing non-hierarchical and hierarchical cluster analyses with clustergrams. Comput Stat. 2004;19(1):95–111.
Google, Inc.: Google Visualization Guide. 2015. https://developers.google.com .
Amcharts.com: amCharts visualization. 2004–2015. http://www.amcharts.com/ .
Viégas F, Wattenberg M. IBM—Many Eyes Project. 2013. http://www-01.ibm.com/software/analytics/many-eyes/
Körner C. Data Visualization with D3 and AngularJS. Community experience distilled: Packt Publishing; 2015.
Azzam T, Evergreen S. J-B PE Single Issue (Program) Evaluation, vol. pt. 1. Wiley\
Machlis S. Chart and image gallery: 30+ free tools for data visualization and analysis. 2015. http://www.computerworld.com/
Julie Steele NI. Beautiful visualization: looking at data through the eyes of experts. O’Reilly Media; 2010.
Guberman S. On Gestalt theory principles. GESTALT THEORY. 2015;37(1):25–44.
Chen C. Top 10 unsolved information visualization problems. Comp Graph Appl IEEE. 2005;25(4):12–6.
Johnson C. Top scientific visualization research problems. Comp Graph Applications IEEE. 2004;24(4):13–7.
Tory M, Möller T. Human factors in visualization research. Trans Visual Comp Graph. 2004;10(1):72–84.
Andrews C, Endert A, Yost B, North C. Information visualization on large, high-resolution displays: Issues, challenges, and opportunities. Information Visualization; 2011.
Suthaharan S. Big Data classification: problems and challenges in network intrusion prediction with machine learning. ACM SIGMETRICS. 2014;41:70–3.
Field DJ, Hayes A, Hess RF. Contour integration by the human visual system: evidence for a local “association field”. Vision Res. 1993;33:173–93.
Picard RW, Healy J. Affective Wearables. Vol 1, Springer. 1997; p. 231–40.
Mann S et al. Wearable technology. St James Ethics Centre; 2014.
Carmigniani J, Furht B, Anisetti M, Ceravolo P, Damiani E, Ivkovic M. Augmented reality technologies, systems and applications. 2010;51:341–77.
Papagiannakis G, Singh G, Magnenat-Thalmann N. A survey of mobile and wireless technologies for Augmented Reality systems. Comp Anim Virtual Worlds. 2008;19(1):3–22.
Caudell TP, Mizell DW. Augmented reality: an application of heads-up display technology to manual manufacturing processes. IEEE Syst Sci. 1992;2:659–69.
Sutherland I. A head-mounted three dimensional display. In: Proceedings of the Fall Joint Computer Conference; 1968. p. 757–64.
Chacos B. Shining light on virtual reality: Busting the 5 most inaccurate Oculus Rift myths. PCWorld; 2014.
Krevelen DWF, Poelman R. A survey of augmented reality technologies, applications and limitations. Int J Virtual Reality. 2010;9:1–20.
Stevens J, Eifert L. Augmented reality technology in US army training (WIP). In: Proceedings of the 2014 Summer Simulation Multiconference, Society for Computer Simulation International; 2014, p 62.
Bower M, Howe C, McCredie N, Robinson A, Grover D. Augmented Reality in education-cases, places and potentials. Educ Media Int. 2014;51(1):1–15.
Ma M, Jain LC, Anderson P. Future trends of virtual, augmented reality, and games for health. In: Virtual, Augmented Reality and Serious Games for Healthcare vol. 1, Springer; 2014. p. 1–6.
Mousavi M, Abdul Aziz F, Ismail N. Investigation of 3D Modelling and Virtual Reality Systems in Malaysian Automotive Industry. In: Proceedings of International Conference on Computer, Communications and Information Technology (2014). Atlantis Press; 2014.
Chung IC, Huang CY, Yeh SC, Chiang WC, Tseng MH. Developing Kinect Games Integrated with Virtual Reality on Activities of Daily Living for Children with Developmental Delay. In: Advanced Technologies, Embedded and Multimedia for Human-centric Computing, Springer, 2014. p. 1091–97.
Steptoe W. AR-Rift: stereo camera for the Rift and immersive AR showcase. Oculus Developer Forums; 2013.
Pina JL, Cerezo E, Seron F. Semantic visualization of 3D urban environments. Multimed Tools Appl. 2012;59:505–21.
Fonseca D, Villagrasa S, Marta N, Redondo E, Sanchez A. Visualization methods in architecture education using 3D virtual models and augmented reality in mobile and social networks. Procedia Soc Behav Sci. 2013;93:1337–43.
Varkey JP, Pompili D, Walls TA. Human motion recognition using a wireless sensor-based wearable system. Personal Ubiquitous Comp. 2011;16:897–910.
Nuwer R. Armband adds a twitch to gesture control. New Scientist. 2013;217(2906):21.
Timberlake GT, Mainster MA, Peli E, Augliere RA, Essock EA, Arend LE. Reading with a macular scotoma. I. Retinal location of scotoma and fixation area. Investig Ophthalmol Visual Sci. 1986;27(7):1137–47.
Foster PJ, Buhrmann R, Quigley HA, Johnson GJ. The definition and classification of glaucoma in prevalence surveys. Br J Ophthalmol. 2002;86:238–42.
Deering MF. The limits of human vision. In: Proceedings the 2nd International Immersive Projection Technology Workshop; 1998.
Krantz J. Experiencing sensation and perception. Pearson Education (Us); 2012.
Rajanbabu A, Drudi L, Lau S, Press JZ, Gotlieb WH. Virtual reality surgical simulators-a prerequisite for robotic surgery. Indian J Surg Oncol. 2014;1–3.
Moglia A, Ferrari V, Morelli L, Melfi F, Ferrari M, Mosca F, Cuschieri A. Distribution of innate ability for surgery amongst medical students assessed by an advanced virtual reality surgical simulator. Surg Endosc. 2014;28(6):1830–7.
Ahn W, Dargar S, Halic T, Lee J, Li B, Pan J, Sankaranarayanan G, Roberts K, De S. Development of a Virtual Reality Simulator for Natural Orifice Translumenal Endoscopic Surgery (NOTES) Cholecystectomy Procedure. Medicine Meets Virtual Reality 21: NextMed/MMVR21 196, 1(2014).
Ma M, Jain LC, Anderson P. Virtual, Augmented Reality and Serious Games for Healthcare 1. Springer; 2014.
Wright WG. Using virtual reality to augment perception, enhance sensorimotor adaptation, and change our minds. Front Syst Neurosci. 2014; 8.
Parsons TD, Trost Z. Virtual reality graded exposure therapy as treatment for pain-related fear and disability in chronic pain. In: Virtual, Augmented Reality and Serious Games for Healthcare 1, Springer; 2014. p. 523–46.
Abramov I, Gordon J, Feldman O, Chavarga A. Biology of sex differences, p. 1–14.
McFadden D. Masculinization effects in the auditory system. Archiv Sexual Behav. 2002;31(1):99–111.
Voyer D, Voyer S, Bryden MP. Magnitude of sex differences in spatial abilities: a meta-analysis and consideration of critical variables. Psychol Bull. 1995;117:250–70.
Stancey H, Turner M. Close women, distant men: line bisection reveals sex-dimorphic patterns of visuomotor performance in near and far space. Br J Psychol. 2010;101:293–309.
Rizzolatti G, Matelli M, Pavesi G. Deficits in attention and movement following the removal of postarcuate (area 6) and prearcuate (area 8) cortex in macaque monkeys. Brain. 1983;106:655–73.
Chua HF, Boland JE, Nisbett RE. Cultural variation in eye movements during scene perception. PNA. 2005;102(35):12629–33.
Zelinsky GJ, Adeli H, Peng Y, Samaras D. Modelling eye movements in a categorical search task. Phisiol Trans Royal Soc. 2013.
Piumsomboon T, Clark A, Billinghurst M, Cockburn A. user-defined gestures for augmented reality. In: Human-Computer Interaction–INTERACT 2013, Springer; 2013. p. 282–99.
Mistry P, Maes P, Chang L. WUW-wear Ur world: a wearable gestural interface. In: Extended Abstracts on Human Factors in Computing Systems, ACM; 2009. p. 4111–16.
Vanacken D, Beznosyk A, Coninx K. Help systems for gestural interfaces and their effect on collaboration and communication. In: Workshop on Gesture-based Interaction Design: Communication and Cognition (2014)
Mulling T, Lopes C, Cabreira A. Gestural interfaces touchscreen: thinking interactions beyond the Button from Interaction Design for Gmail Android App. In: Design, sser Experience, and Usability. User Experience Design for Diverse Interaction Platforms and Environments, Springer, 2014; p. 279–88.
Piumsomboon T, Clark A., Billinghurst M. [DEMO] G-SIAR: gesture-speech interface for augmented reality. In: Proceedings of International Symposium on Mixed and Augmented Reality (ISMAR), IEEE; 2014. p. 365–66.
Vafadar M, Behrad A. A vision based system for communicating in virtual reality environments by recognizing human hand gestures. Multi Tools Appl. 2014;1–21.
Roupé M, Bosch-Sijtsema P, Johansson M. Interactive navigation interface for Virtual Reality using the human body. Comp Environ Urban Syst. 2014;43:42–50.
Wen R, Tay WL, Nguyen BP, Chng CB, Chui CK. Hand gesture guided robot-assisted surgery based on a direct augmented reality interface. Comp Method Program Biomed. 2014.
Rolland JP, Fuchs H. Optical versus video see-through head-mounted displays in medical visualization. Presence Teleoperators Virtual Environ. 2000;9(3):287–309.
Silanon K, Suvonvorn N. Real time hand tracking as a user input device. Springer; 2011. p. 178–89.
Keim DA, Mansmann F, Schneidewind J, Ziegler H. Challenges in visual data analysis. In: Proceedings of 10th International Conference on Information Visualization, IEEE; 2006. p. 9–16.
Sonka M, Hlavac V, Boyle R. Image processing, analysis, and machine vision. Cengage Learning. 2014.
Shneiderman B. The eyes have it: a task by data type taxonomy for information visualizations. In: Proceedings of IEEE Symposium on Visual Languages; 1996. p. 336–43.
Coffey D, Malbraaten N, Le T, Borazjani I, Sotiropoulos F, Keefe DF. Slice WIM: a multi-surface, multi-touch interface for overview+ detail exploration of volume datasets in virtual reality. In: Proc. of Symposium on Interactive 3D Graphics and Games, ACM. 2011. p. 191–98.
Download references
Authors' contributions
EO performed the primary literature review and analysis for this work as well as designed illustrations. Manuscript was drafted by EO and AO. EK introduced this topic to other authors and coordinate the work process to complete the manuscript. EO, AO and TO worked together to develop the article’s framework and focus. All authors read and approved the final manuscript.
Compliance with ethical guidelines
Competing interests The authors EO, AO, EK and TO declare that they have no competing interests.
Author information
Authors and affiliations.
Department of Electronics and Communications Engineering, Tampere University of Technology, Korkeakoulunkatu 10, 33720, Tampere, Finland
Ekaterina Olshannikova, Aleksandr Ometov & Yevgeni Koucheryavy
Department of Pervasive Computing, Tampere University of Technology, Korkeakoulunkatu 10, 33720, Tampere, Finland
Thomas Olsson
You can also search for this author in PubMed Google Scholar
Corresponding author
Correspondence to Ekaterina Olshannikova .
Rights and permissions
Open Access This article is distributed under the terms of the Creative Commons Attribution 4.0 International License ( http://creativecommons.org/licenses/by/4.0/ ), which permits unrestricted use, distribution, and reproduction in any medium, provided you give appropriate credit to the original author(s) and the source, provide a link to the Creative Commons license, and indicate if changes were made.
Reprints and permissions
About this article
Cite this article.
Olshannikova, E., Ometov, A., Koucheryavy, Y. et al. Visualizing Big Data with augmented and virtual reality: challenges and research agenda. Journal of Big Data 2 , 22 (2015). https://doi.org/10.1186/s40537-015-0031-2
Download citation
Received : 24 May 2015
Accepted : 16 September 2015
Published : 01 October 2015
DOI : https://doi.org/10.1186/s40537-015-0031-2
Share this article
Anyone you share the following link with will be able to read this content:
Sorry, a shareable link is not currently available for this article.
Provided by the Springer Nature SharedIt content-sharing initiative
- Visualization
- Virtual reality
- Augmented reality
- Mixed reality
- Human interaction
IEEE Account
- Change Username/Password
- Update Address
Purchase Details
- Payment Options
- Order History
- View Purchased Documents
Profile Information
- Communications Preferences
- Profession and Education
- Technical Interests
- US & Canada: +1 800 678 4333
- Worldwide: +1 732 981 0060
- Contact & Support
- About IEEE Xplore
- Accessibility
- Terms of Use
- Nondiscrimination Policy
- Privacy & Opting Out of Cookies
A not-for-profit organization, IEEE is the world's largest technical professional organization dedicated to advancing technology for the benefit of humanity. © Copyright 2024 IEEE - All rights reserved. Use of this web site signifies your agreement to the terms and conditions.
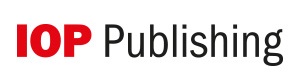
We apologize for the inconvenience...
To ensure we keep this website safe, please can you confirm you are a human by ticking the box below.
If you are unable to complete the above request please contact us using the below link, providing a screenshot of your experience.
https://ioppublishing.org/contacts/
- Original Article
- Open access
- Published: 29 October 2021
Examining data visualization pitfalls in scientific publications
- Vinh T Nguyen 1 ,
- Kwanghee Jung ORCID: orcid.org/0000-0002-7459-7407 2 &
- Vibhuti Gupta 3
Visual Computing for Industry, Biomedicine, and Art volume 4 , Article number: 27 ( 2021 ) Cite this article
10k Accesses
6 Citations
Metrics details
Data visualization blends art and science to convey stories from data via graphical representations. Considering different problems, applications, requirements, and design goals, it is challenging to combine these two components at their full force. While the art component involves creating visually appealing and easily interpreted graphics for users, the science component requires accurate representations of a large amount of input data. With a lack of the science component, visualization cannot serve its role of creating correct representations of the actual data, thus leading to wrong perception, interpretation, and decision. It might be even worse if incorrect visual representations were intentionally produced to deceive the viewers. To address common pitfalls in graphical representations, this paper focuses on identifying and understanding the root causes of misinformation in graphical representations. We reviewed the misleading data visualization examples in the scientific publications collected from indexing databases and then projected them onto the fundamental units of visual communication such as color, shape, size, and spatial orientation. Moreover, a text mining technique was applied to extract practical insights from common visualization pitfalls. Cochran’s Q test and McNemar’s test were conducted to examine if there is any difference in the proportions of common errors among color, shape, size, and spatial orientation. The findings showed that the pie chart is the most misused graphical representation, and size is the most critical issue. It was also observed that there were statistically significant differences in the proportion of errors among color, shape, size, and spatial orientation.
Introduction
With the advancement of the internet, data storage, and search methods, an increasing number of scientific publications and academic papers are being deposited online. This facilitates readers’ access to scientific knowledge and publications. To help in quick and efficient browsing, online resources allow full papers to be stored in several formats such as text, photographs, tables, and charts. This proprietary database helps the reader find it faster, while it raises some problems in understanding data. When tables and charts are positioned closest to their descriptions, the author’s intention will be delivered more clearly. However, it is not surprising that we see so many tables and charts that do not themselves contain information to convey. When a reader fails to read the description, the author loses the ability to communicate his or her information. As a result, the design, arrangement, and organization of data in tables and charts play an important role in information dissemination.
Visualization has long been used as an effective method for transcribing data into information and help people carry out data analysis tasks and make decisions. A number of visual encodings (such as color, shape, and size) can be used individually or together for representing various visualization tasks [ 1 , 2 , 3 ]. Misinformation in data visualization has become one of the main issues to convey knowledge more effectively [ 4 , 5 ]. As opposed to creating effective visualization, misinformation in data visualization receives less attention and becoming one of the major issues for conveying information [ 5 ]. Misinformation can be classified into two categories including intentional misinformation and unintentional misinformation [ 6 ]. Intentional misinformation [ 7 ] in data visualization refers to the use of charts, graphics to distort, hide, or fabricate data in an attempt to deceive users. On the other hand, unintentional misinformation [ 8 ] implies providing false and inaccurate information to the end-users because of human cognitive bias and carelessness in designing/selecting visual channels to encode the corresponded data. From the creator’s point of view, the former is controllable while the latter requires a lot of training. This work will be focusing more on the latter one as it is encouraged to build trust in visualization rather than lies [ 9 ].
A good visualization tool supports users to get insights (i.e., trends, patterns, and outliers) and extract meaningful information from data. Wilkinson [ 10 ] describes the building blocks for constructing a wide range of statistical graphics in his notable book “The Grammar of Graphics”. On the other hand, many articles on the web provide bad examples of visual designs leading to misinterpretation but they are usually viewed from different perspectives. In line with this research direction, Bresciani and Eppler [ 6 ] provided a comprehensive review on common errors in data visualization and classified these pitfalls into three categories: cognitive, emotional, and social. Their high-level abstract of the theoretically grounded classification can be used as a checklist for practitioners to examine the visualization for avoiding common drawbacks. However, it is challenging to go through the entire checklist due to the different human cognitive and one’s preference styles. As such, the authors suggested that a more rigorous study should be conducted to “rank the pitfalls according to how common or severe they are.” In response to these needs, in this paper, we try to compromise the most common errors into a structure based on the units of visualization. In another word, we address the following research questions: (R1) What are the most common effects resulting from visualization pitfalls? (R2) What are the most common errors in constructing a representation in terms of color, shape, size, and orientation? Or (R3) Is there any difference in the proportions of common errors among color, shape, size, and spatial orientation? We believe that going from these units would benefit practitioners, especially novice users when they are mostly working with these elements. We expect that the result of this study will be served as a reference for avoiding visualization pitfalls in general. Ultimately, in the long run, we will attempt to answer the questions such as what color [ 11 ] should we use to represent data? what visualization types [ 12 , 13 ] should be taking into account for analysis? what type of scale should we apply? As such, this is preliminary research work to accomplish the goals.
This study is a continuation of a previously published work at the symposium on visual information communication and interaction [ 14 ]. The new version was extended by expanding the existing database (Google Scholars) with Scopus, incorporating a text mining technique to extract practical insights from common visualization pitfalls, and examining if there is any difference in the proportions of common errors among color, shape, size, and spatial orientation with Cochran’s Q test and McNemar’s test.
The rest of this paper is structured as follows: First, a literature review relevant to our work was presented. Then, the instruments and processes to conduct the research were provided along with the analytics. After that, the results were presented. Finally, the findings were discussed and concluded.
Related work
Many recent works have addressed the issues of misinformation in data visualization. Huff [ 15 ] showed a variety of ways a designer can trick people, e.g., truncating certain portions of the graph. As such, the discrepancy is more obvious when seen as a whole. This goal can be accomplished by three common strategies [ 9 , 16 ]: (1) not revealing any data, (2) showing the data incorrectly, and (3) obfuscating the data.
Many authors attempted to provide designers with a boilerplate for creating a successful visualization, ranging from individual graph elements such as colors [ 17 , 18 , 19 ] and forms (or chart types) [ 20 ] to a detailed data visualization design [ 21 , 22 ]. However, bad visualization design persists in the presence of guidance, which leads to misinformation that can contribute to considerable problems, especially where individuals heavily rely on the data at hand when making business decisions. Study results raise concerns: what are the possible causes of disinformation visualization? This research is critical in developing a visualization platform that not only meets end-user needs but also mitigates the issues from misinformation and disinformation.
The pitfalls of visualization have been examined in a variety of fields such as information visualization [ 23 , 24 , 25 ] - psychological/aesthetic restrictions presented graphic format are highlighted, diagrammatic representations [ 13 , 26 , 27 , 28 ] using the diagram to understand concept or idea instead of algebraic means, human-computer interaction [ 21 , 29 ] - potential drawbacks of interactive visualizations, statistical graphic representations [ 22 , 30 ] - bad examples of representing data visually. The most comprehensive research was provided by Bresciani and Eppler [ 6 ], where the authors explored the potential drawbacks in graphic depictions. The authors compiled a list of 51 pitfalls of visualization drawbacks based on interviews with seven experts and divided them into three categories: cognitive, emotional, and social effects.
Unlike the previous research relying on high-level abstractions to avoid visualization errors, we focus on the basic elements that constitute visualization mapping. These elements are color, shape, size, and spatial orientation presented by Munzner [ 22 ]. According to Bresciani and Eppler’s research [ 6 ], there is a need to review a selection of images to assess the frequency and severity of the flaws. In this study, we aim to alleviate the problems of misinformation in data visualization.
Data sources
For the research, the preferred reporting items for systematic reviews and meta-analyses (PRISMA [ 31 ]) model served as a guideline. It is a bare-bones set of evidence-based features meant to help authors report on a wide range of systematic reviews and meta-analyses. Google Scholar and Scopus indexing databases were used to gather data for our study due to their popularity and credible results. The search terms were “misinformation visualization”, “misleading visualization”, “disinformation visualization”, “visualization pitfalls”, “bad visualization design”. Google Image search was used to collect representative images that illustrate visualization pitfalls to avoid direct criticism of previous studies. Table 1 provides the number of papers collected from the two indexing databases according to their keywords.
Data preprocessing
Search results from the database were consolidated into a single excel file format, and duplicated items were removed. The final list of items was filtered by the scientific articles’ abstracts and main contents. Here, we did not exclude items by their titles. Rather, we examined the details of abstracts and main contents to see where the keywords were positioned. It is noted that some of the collected papers contained keywords but were not relevant to our research goal, e.g., some papers did not contain figures to demonstrate the searching keywords. Such papers were excluded from our analysis. In the end, 178 papers were included in the study. Figure 1 depicts the flow of information through the different phases of the systematic review utilizing the PRISMA approach.
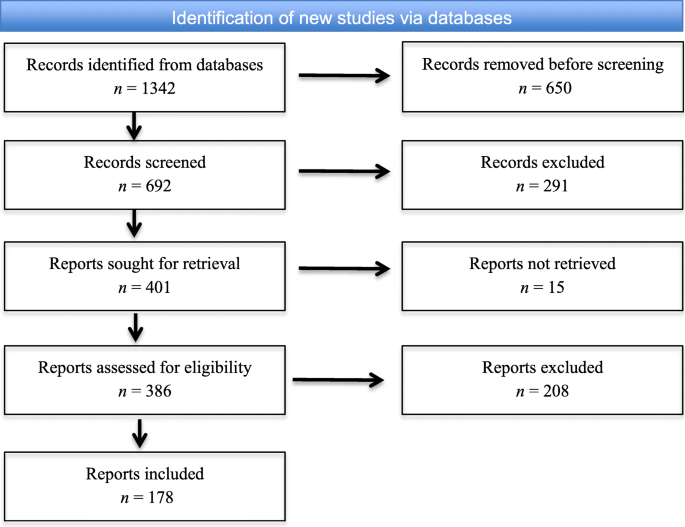
The PRISMA flow diagram
Data extraction
We extracted two types of data from the research papers: figures and their associated descriptions. Each figure is classified into one of four categories (i.e., color, shape, size, and spatial orientation) which indicates a type of violation in visualization. The relevant papers were screened through three phases: (1) checking the title, (2) reviewing the abstract, and (3) scrutinizing the full text of the paper.
Data analysis
For the first research question, we employed text mining techniques to gain practical insights from the collected data, including association rule mining to find out the relationships among the descriptions of figures. The inputs for this technique were taken from associated descriptions of each figure. We created a word cloud with the most often used words in related explanations, removing any stop words, non-ASCII characters, white space, and duplication. Word clouds are graphical representations of word frequency that highlight terms that appear regularly in a source document. The bigger the term in the visual, the more popular the word was in the text. This visualization method can help evaluators with exploratory textual research by highlighting terms that appear often in a series of interviews, records, or other languages. It may also be used to communicate the most important points or topics during the reporting stage. Association rule mining is a rule-based machine learning technique that seeks out interesting hidden relationships among variables in a dataset. Association rules have been used in a variety of applications, including opinion mining, intrusion prevention, continuous processing, and bioinformatics.
For the second research question, we grouped typical visualization pitfalls into four major categories denoted from M1 to M4 based on the basic elements (color, size, shape, and spatial orientation), as shown in Fig. 2 , where “red, blue, and green” represent the color, scaled squares represent the size, “circle, rectangle, and triangle” represent the shape, and scatter black dots represent the spatial orientation. As we move from left to right, misinformation for visual mapping can result from data itself, such as missing data or poor data, to cognitive perception, where color, shape, and scale are perfectly used but misinformation occurs due to cognitive mechanisms. Visual perception is the primary subject of this paper (for more information on data perception and cognitive perception, see Bresciani and Eppler’s study [ 6 ]).
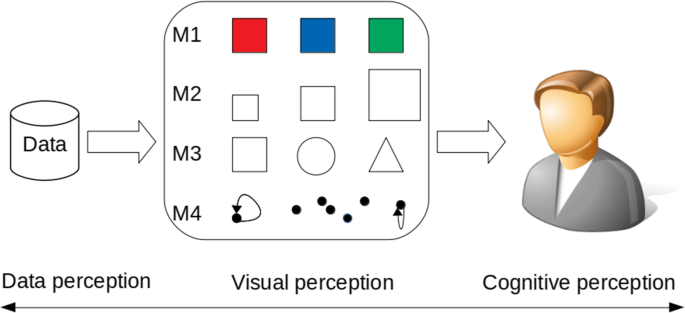
A visual approach for categorizing misinformation data visualization: M1 (color), M2 (size), M3 (shape), and M4 (spatial orientation)
For the third research question, we conducted Cochran’s Q test and McNemar’s test [ 32 ] to examine if there is any difference in the proportions of common errors among the fundamental units of color, shape, size, and spatial orientation. We examined each paper individually and extracted figures that were violated in terms of any of the four categories. It is noted that only figures generated from data by authors were included for the analysis. Figures taken from photos or other sources were excluded. We encoded “1” as being violated and “0” for not being violated. Thus, our data consists of four binary variables. Statistical analysis was performed with SPSS Version 25.
RQ1: What are the most common effects resulting from visualization pitfalls?
After removing special characters in the associated descriptions of figures, we had 363 items with 139 unique words. The items are the remaining words/keywords extracted from the descriptions after cleaning data. Figure 3 depicts the most frequently used terms for each input figure that encounter visualization pitfalls. Frequently used terms in Fig. 3 are shown in larger font sizes in the word cloud. In terms of dominant keywords, besides our proposed categories such as color (72), shape (36), size (132), a majority of misleading visualizations comes from pie chart (83) and bar chart (72), followed by using the wrong chart (63) that incur misinterpretation (21). In addition to the pie chart, donut (33) is another interesting finding that contributes to common errors, it seems that creators are interested in using circles to explain their messages. It is noticed that the terms “inform and axis” are also highlighted in our visual layout which indicates that creators and viewers have to take care of the axis while interpreting data to inform decision-making. We can see other terms that appear to be slightly different from others such as data, hard, and clutter, but they do not stand out apparently.
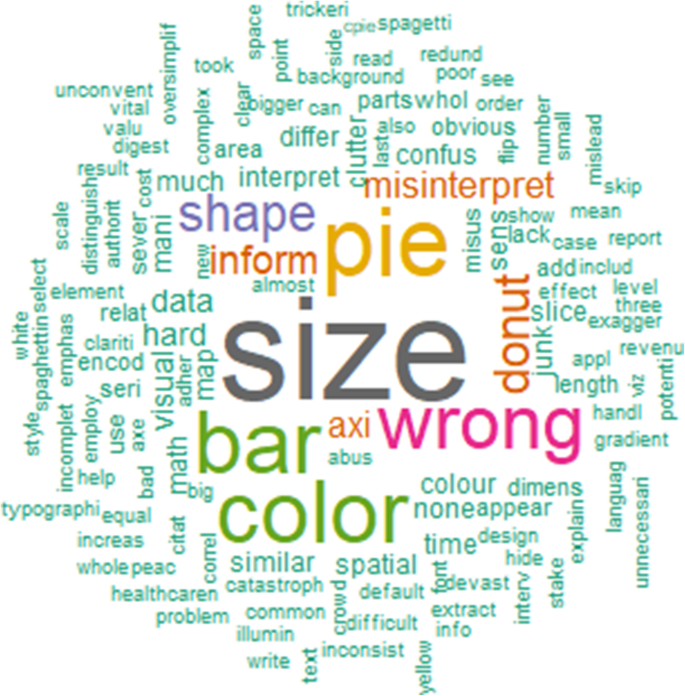
Word cloud constructed from associated descriptions about the figure that encounters visualization pitfalls
Figure 4 depicts the graphical layout of our transactions and items in the dataset, where black cells denote non-zero elements and empty cells represent zero elements. Rows (transactions) are associated description index of figures, while columns (items) are terms extracted from their corresponding descriptions. In total, we had 370 descriptions and 109 terms. The percentage of non-zero cells in this 370 × 109 matrix is shown by density. It is worth noting that a matrix is considered sparse if most of its elements are empty. On the other hand, if most of the elements are non-zero, the matrix is called dense. The sparsity of the matrix is defined as the number of zero-valued elements divided by the total number of elements. Our dataset showed a density of 0.0312 which means that on average, each description contains 3 to 4 keywords/terms. This is due to the data cleaning process, where all stop words were removed.
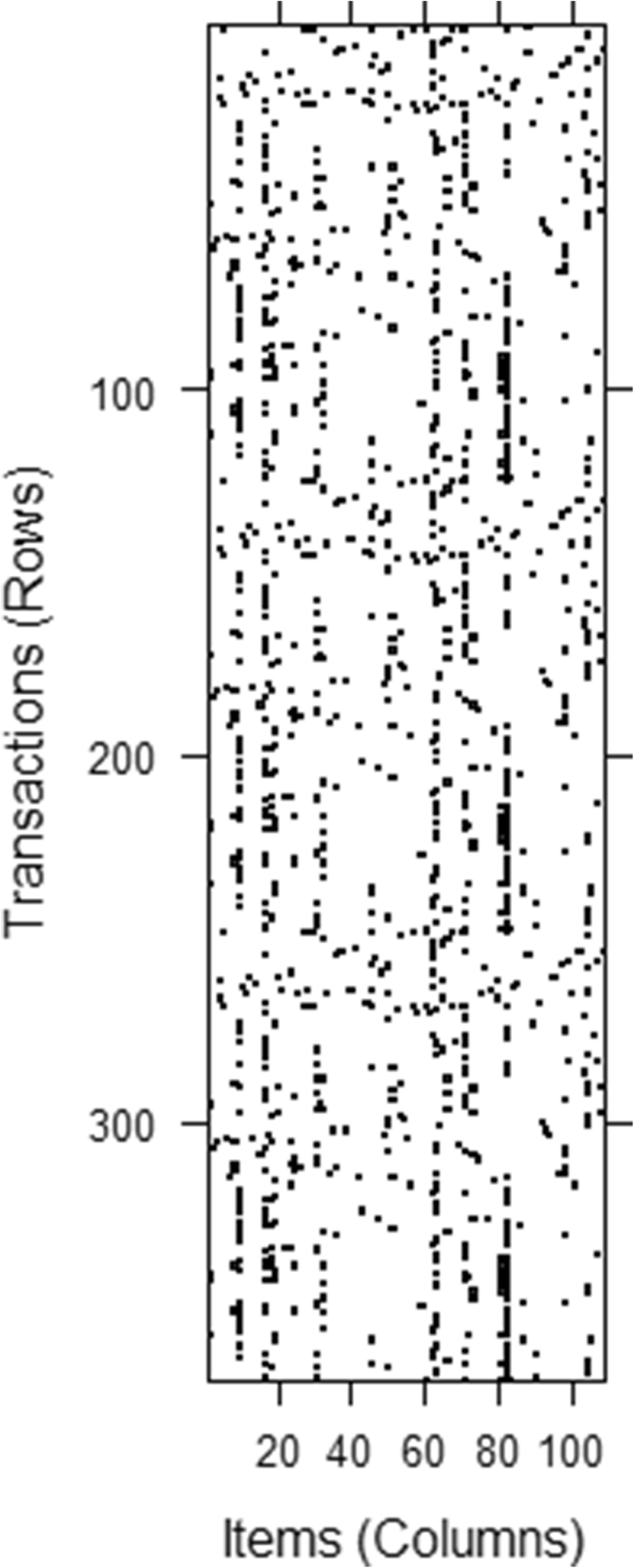
Transactions and items in a matrix
Table 2 showed a list of 14 rules generated from the A-Priori algorithm [ 33 ]. This algorithm necessitates both minimal support and a minimum confidence constraint. If no threshold value is defined, the function will consider the default values of support and confidence threshold as 0.1 and 0.8, respectively. Since the elements in the matrix are sparse, support and confidence are modified with lower values (support = 0.04 and confidence = 0.5) to mine the most potential rules rather than using default values. Support is the percentage of transactions (or figures) that contain both words in a rule and confidence is the strength of that rule. The A-Priori algorithm outputs the highest value of support (0.162), confidence (1), and lift (11.012) respectively. The lift value is an indicator of a rule’s significance. If the lift value is greater than 1, it indicates that the rule body (or Rhs) and the rule head (or Lhs) appear together more often than predicted, implying that the occurrence of the rule body has a positive impact on the occurrence of the rule head. On the other hand, if the lift value is less than 1, it implies that the occurrence of the Rhs has a negative effect on the occurrence of the Lhs. Table 2 shows that all lift values are greater than 1, indicating that items in the rules are positively correlated. It is noted that more than half of items (57.14 %) in the rule body involve ‘size’, which means that size is the most critical issue in visualization pitfalls. This statistical data is supported by the visual graph as depicted in Fig. 5 , where size is the center of the constructed network with a total of 10 connections from other rules. This network illustrates associations between items and rules. Bigger circles indicate higher support, while the red color implies higher lift. One interesting pattern revealed by the algorithm is when creators use size to express information in a bar chart, then misleading is likely to occur (rule 13, 14). In addition, incorrect usages of size can lead to confusion.
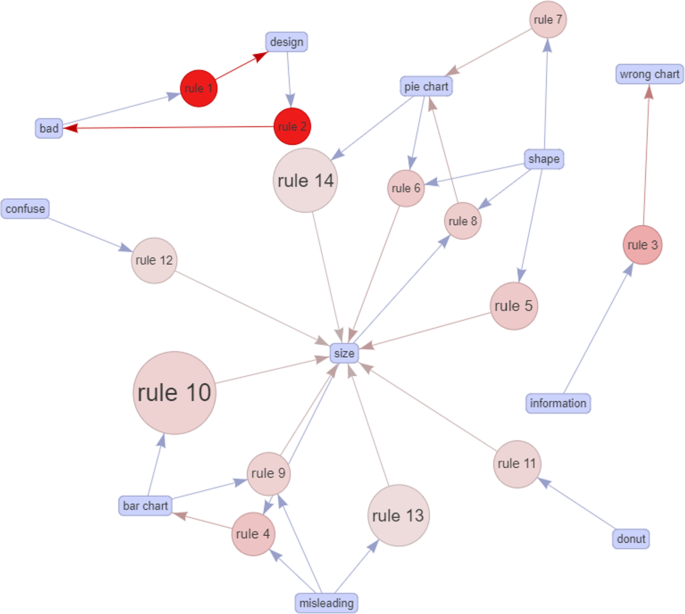
Graph-based visualization on the relationship between individual items in the ruleset
RQ2: What are the most common errors in constructing a representation in terms of color, shape, size, and orientation?
M1: misinformation due to color violation.
As shown in Fig. 6 , one of the most common visualization mistakes is the use of too many colors. Healey et al. [ 34 ] argued that the human eye can only process five to seven well-chosen colors pre-attentively, and qualitative color scales are thought to work best when three to five different colored categories are present. Choosing a color scheme is another critical issue when different categories are encoded by related color on a spectrum that makes it difficult to distinguish one from another as in Fig. 6 a - legend. In some cases, the use of continuous color even gets worse leading to misinterpretation of data [ 35 ], especially when using a non-monotonic scale or rainbow scale [ 11 ]. It can be seen from Fig. 6 d that the colors at the beginning and the end are almost equivalent (circulated color scales). Figure 6 b shows using the same color for different categorical data. Although each category can be annotated by a distinct shape, color is often misused to represent an additional dimension especially when the granularity of the new dimension is too low. It takes more time to locate the blue circle point of interest. Another common bad habit is using the color against the norm: it is often found in the practice that light color is usually for less density while darker color is for higher density or green for a healthy indicator vs. red for abnormal value. The example illustrated in Fig. 6 c misused red color business gains and green color for business losses. A first glance, this visualization misled us that the economy is flourishing.
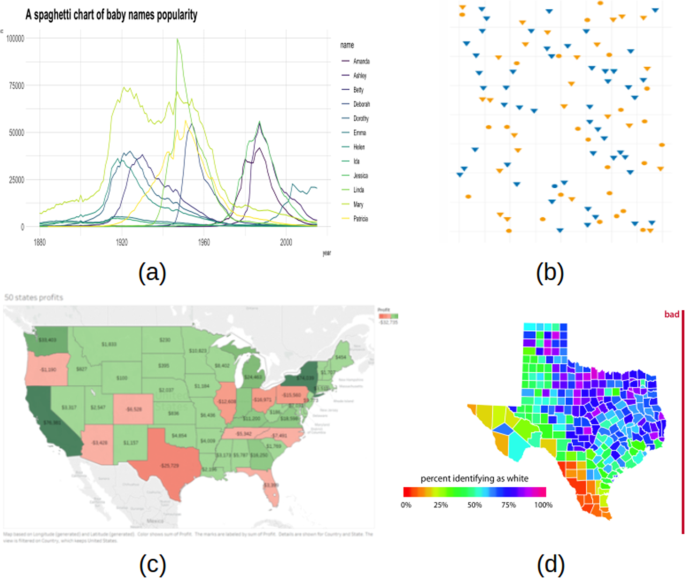
Misinformation due to color violation: a using too many colors [ 36 ], b unnecessary color usage [ 37 ], c color against the norm [ 38 ] and d rainbow color scale [ 39 ]
M2: Misinformation due to shape violation
The shape can be violated due to the use of inappropriate chart types. In fact, this is the most common misleading chart that we found in the literature. Particularly in the case of using a pie chart instead of a bar chart and vice versa. A pie chart is a type of graph in which a circle is broken down into segments (i.e., slices of pie) that each represent a proportion of the whole. Each slice of the pie chart is represented by a percentage value accumulating to 100%. Figure 7 a illustrates the improper use of the pie chart where each slice adds up to more than 100%. Even though the original intention was to compare values among different categories, this chart also violates color choices (so many colors, or similar colors for different categorical data). Common practice [ 37 ] suggested that the pie chart can be best used with less than seven categories (same magic number as in color) due to the difficulty for the eye to distinguish relativity of size between each segment. In this case, the bar chart should be a better choice. Figure 7 c shows an opposite case where the pie chart should be taken into consideration instead of using the bar chart for comparing proportional data as percentages add up to 100%.
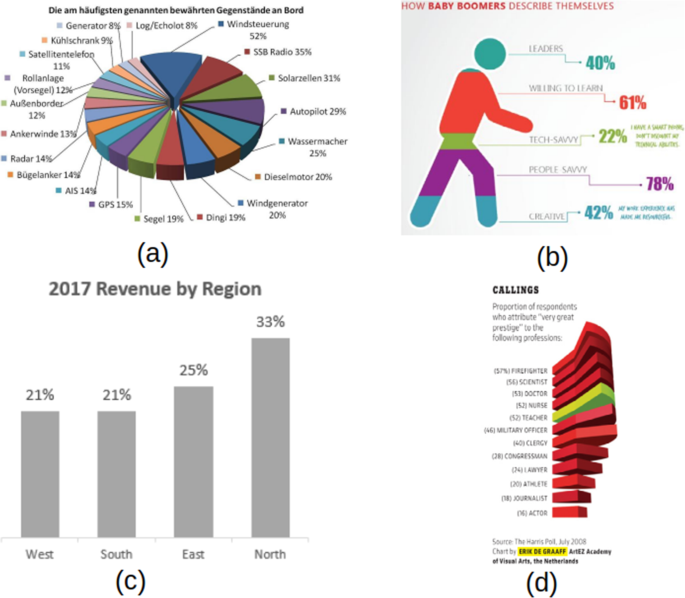
Misinformation due to shape violation: a using 3D pie chart for comparing proportion [ 40 ], b using wrong visualization chart type, c pie-chart should be used [ 41 ], and d bar chart should be used [ 42 ]
The second misleading information when performing visual mapping is to use a shape that does not reflect the information provided. It can be seen from Fig. 7 b that the body part misleads users from what it means. Like the case of the pie chart, proportionality does not add up to 100% or areas cannot be compared visually.
Another common misleading information is the use of a high-dimensional chart to represent lower-dimensional data. This is often due to the visual appeal of 3D charts or the priority of prioritizing design and technology over conveying information of the chart as it can be seen from Fig. 7 a and d where heights of each slice did not provide any additional information.
While doing visual mapping, the second deceptive information is to use a shape that does not represent the information presented. Figure 7 b demonstrates how the body part misleads people on what it means. Proportional, like the pie map, does not add up to 100%, or regions cannot be physically compared. Another example of deceptive evidence is the use of a high-dimensional map to display low-dimensional details. This is often attributed to the aesthetic appeal of 3D charts or the importance of prioritizing architecture and technology over conveying detail of the map, as seen in Fig. 7 a and d, where the heights of each slice provided no additional details.
M3: Misinformation due to size violation
Regarding size infringement, the most common problem is to use a single size dimension scale to express a two-dimensional value. For example, in Fig. 8 a, the diameter was used for the scale to display the proportion to GDP, but users often viewed the chart from the perspective of the region. In this case, the field expands exponentially (i.e., quadratic vs linear). Mathematically, 14.5 trillion is 2.56 times larger than 5.7 trillion, but the region seems to be 6.5 times larger.
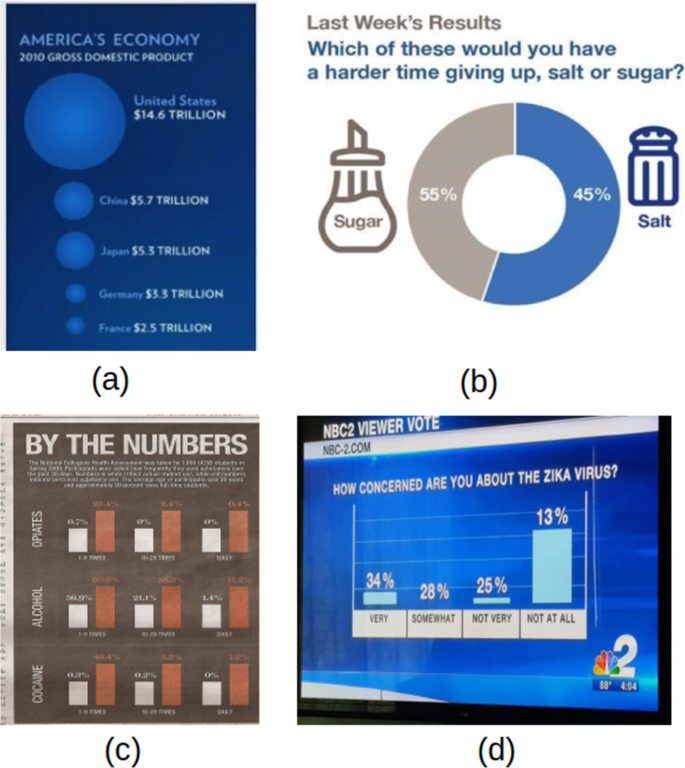
Misinformation due to size violation: a using wrong scale [ 43 ], b size is inverted [ 40 ], c all sizes are equal [ 44 ] and d size with no meaning [ 45 ]
Careless designers continue to apply incorrect labeling to visual mapping, especially where the chart is labeled manually without the assistance of automatic graphic software. Figure 8 b represented this scenario in which 55 % and 45 % could be swapped. Surprisingly, comparable findings in this form of pitfall are typically seen from news channel writers. Another method of softening the detrimental impact of a section is to use a light color, but we do not mention it in our study because it is considered an intention.
The use of equivalent scale shapes supplemented with numbers for contrast is the second common deceptive graph pitfall that belonged to this group, shown in Fig. 8 c. Similarly, to orange bars, all-white bars are the same height. Colors are often misused in three separate categories; however, the most serious flaw is the appearance of height where no meaning is present (0 % value of the white bars in the rightmost figure). These common pitfall patterns can also be seen in a pie chart of equal-sized parts.
Another pitfall is to use a graphic encoding that is unrelated to the values it contains, as seen in Fig. 8 d, where the height of each bar chart does not fit the value above it. Did the author use an inverted scale? Then 34 % should be the lowest, and where is the bar chart for the “SOMEWHAT” category? While this form of expression is uncommon in academia, social media has had many instances of it.
M4: Misinformation due to spatial orientation violation
In this study, we would use a subset of spatial features including spatial and temporal mapping which engages space and time, respectively.
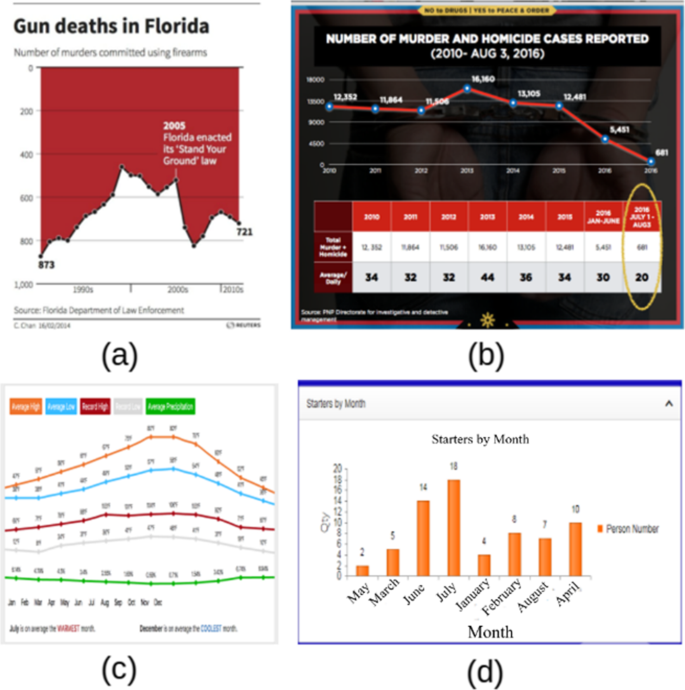
Misinformation due to spatial and temporal violation [ 46 ]: a inverted axis, b data split out, c missing axis to line up data, and d mixing time data
Inverting the vertical axis is a technique that allows viewers to see the flip side of the original data. In practice, the flip-side data is intentionally removed to convey other information. Figure 9 a is the most controversial chart being discussed whether it was created to deceive users [ 46 ]. For most viewers, this graph first gave the impression that gun deaths declined sharply after 2005 due to the illusion of the line chart, especially in the presence of black dots. Gun deaths increased. As indicated in ref. [ 46 ] “the artist does not appear to have intended the graph to be deceptive. Her view was that deaths are negative things and should be represented as such.” However, the focal point we want to address was that misleading information would make people feel that living in Florida get safer after the incident. A similar story can also be found in Fig. 9 b. Omitting data, truncating the axis (or non-zero-base axis), and over zooming to see the difference are popular techniques [ 9 , 16 ] to make a deceptive graph. From our point of view, in Fig. 9 b original data remains but misleading information still exists.
The most common problem with spatial orientation violation is reordering time series results. When creating a graph with a timestamp in one dimension, the order of data points at each time level is shown in Fig. 9 d, where months appear to be in a random position. The direction would be totally destroyed if the data are shuffled. Careless charting can also result in the lack of one dimension, shown in Fig. 9 c, where the author attempted to combine line graphs from different figures into one. We can see from the contradictory data unit and value location that does not match the upward trend. Misinformation due to data and visual interpretation is most often seen in the form of a table shown in Table 3 , where viewers must make at least two attempts to locate the absolute meaning (i.e., compare the length and compare the leftmost values). In this case, the order of magnitude in space is broken.
RQ3: Is there any difference in the proportions of common errors among color, shape, size, and spatial orientation?
Table 4 shows that the most frequently observed pitfalls were in size (69.4%). Color and shape showed a similar number of cases (39.7% and 37.2%, respectively). Spatial orientation had the least case (14.9%).
We conducted Cochran’s \(Q\) test and McNemar’s test [ 32 ] to examine if there is any difference in the proportions of common errors among color, shape, size, and spatial orientation. Cochran’s \(Q\) test determined that there was a statistically significant difference in the proportion of errors among the features of color, shape, size, and spatial orientation, \(Q\) (3) = 207.047, p < 0.001. This implies that at least two of the features had a different proportion of errors. Post hoc analysis with McNemar’s test was conducted. Table 5 shows the 2 × 2 classification tables for McNemar’s test. There were no significant differences between color and shape ( χ 2 = 0.547, p = 0.460). However, there was a statistically significant difference in proportions of errors between color and size ( χ 2 = 54.519, p < 0.001), color and spatial orientation ( χ 2 = 42.586, p < 0.001), shape and size ( χ 2 = 84.629, p < 0.001), and between size and spatial orientation ( χ 2 = 132.003, p < 0.001). The findings indicate that size is most frequent in the common pitfalls, which is statistically different in frequency from other features. Color and shape are the second most frequent common errors, which are statistically different from spatial orientation in terms of frequency. However, there is no significant difference between color and shape.
It is undeniable that visualization is becoming more common, especially in the age of the Internet of Things, where millions of data points are created every day. To succeed, we need to provide a big picture in a succinct graphic style to help customers digest details faster. When we screened the papers for the study, we discovered that the majority of the graphical layouts were created by hand or produced by a program using built-in features. Only a few studies used specialized software to present results. Part of the problem stems from developers’ lack of programming expertise, which forces them to rely on free software. In some cases, these tools have a fine, suggested interface (e.g., Google Form automatically generated charts based on survey data), but in others, the layout is much worse than if it were created manually (e.g., Google Form only generates one type of chart for numerical data without considering the number of categories). As a result, developers would use these preset visualizations without regard for visual interface clarity. Another risk that can trigger visualization pitfalls stems from the writing paper guidelines. Although there is a straightforward, concise description of how to write and section (e.g., abstract, introduction, methodology, and conclusion), there is no guidance on how to correctly present data in figures. As a result, authors place a greater emphasis on content rather than visual presentation. This problem is addressed in the area of data visualization, where instructions for presenting data can be found in the paper’s template. However, these recommendations are not systematic, making a boilerplate graphic suggestion for both inside and across fields challenging. We expect that artificial intelligence will be modified in the coming years to automatically screen and identify possible common errors based on research questions and the evidence presented. As a result, developers will be able to concentrate on mining the content rather than thinking about deceptive interfaces.
Conclusions
This paper dealt with typical visual representation pitfalls. We gathered data from two indexing databases, pooled it, and grouped it into four groups based on data representation units. The most popular visualization pitfalls from each segment were extracted, and data in each section was analyzed using both qualitative and quantitative methods. Word frequency from Word Cloud visualization shows that size is the most dominant keyword found in the descriptions of figures, followed by pie, bar charts, and color. Association rule mining reported that size is the center of the constructed network with a total of 10 connections from other rules and it plays a major concern in visualization pitfalls. Cochran’s Q test and McNemar’s test showed that there was a statistically significant difference in the proportion of errors among the fundamental units of color, shape, size, and spatial orientation. The size issue was most frequent in common pitfalls, followed by color and shape. Spatial orientation had the least case. This paper’s contribution can be thought of as a fine-grained (or subset) of general visualization pitfalls, with a focus on visual perception. We hoped that our findings would aid in the creation of a taxonomy of common errors at the information stage. User interface analysis on visual interpretation will be undertaken in the future with a more detailed test design, taking into account the user’s cognitive loads and cognitive style.
Availability of data and materials
The datasets used and/or analyzed during the current study are available from the corresponding author on reasonable request.
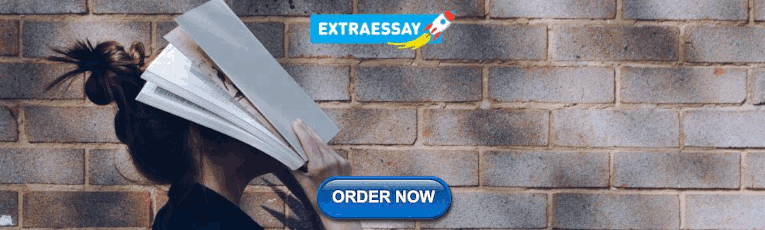
Abbreviations
Preferred reporting items for systematic reviews and meta-analyses
Ceneda D, Gschwandtner T, May T, Miksch S, Schulz HJ, Streit M et al (2016) Characterizing guidance in visual analytics. IEEE Trans Vis Comput Graph 23(1):111–120. https://doi.org/10.1109/TVCG.2016.2598468
Article Google Scholar
Nguyen VT, Namin AS, Dang T (2018) MalViz: an interactive visualization tool for tracing malware. In: Abstracts of the 27th ACM SIGSOFT international symposium on software testing and analysis, ACM, Amsterdam, 16–21 July 2018. https://doi.org/10.1145/3213846.3229501
Dang T, Nguyen VT (2018) ComModeler: topic modeling using community detection. In: Tominski C, von Landesberger T (eds) Eurovis workshop on visual analytics. The Eurographics Association, Brno, p 1–5
Google Scholar
Nguyen NVT, Nguyen VT, Dang T (2021) Color blind: can you sight? In: Abstracts of the 12th international conference on advances in information technology, Association for Computing Machinery, Bangkok, 29 June-1 July 2021. https://doi.org/10.1145/3468784.3471602
Bresciani S, Eppler MJ (2009) The risks of visualization: a classification of disadvantages associated with graphic representations of information. In: Schulz PJ, Hartung U, Keller S (eds) Identität und vielfalt der kommunikations-wissenschaft. UVK Verlagsgesellschaft mbH, Konstanz, p 165–178
Bresciani S, Eppler MJ (2015) The pitfalls of visual representations: a review and classification of common errors made while designing and interpreting visualizations. SAGE Open 5(4):2158244015611451. https://doi.org/10.1177/2158244015611451
Tufte ER (1983) The visual display of quantitative information. Graphics Press, Cheshire, p 200
Fishwick M (2004) Emotional design: why we love (or hate) everyday things. J Am Cult 27(2):234. https://doi.org/10.1111/j.1537-4726.2004.133_10.x
Cairo A (2015) Graphics lies, misleading visuals. In: Bihanic D (ed) New challenges for data design. Springer, London, p 103–116. https://doi.org/10.1007/978-1-4471-6596-5_5
Wilkinson L (2012) The grammar of graphics. In: Gentle JE, Härdle WK, Mori Y (eds) Handbook of computational statistics. Springer handbooks of computational statistics. Springer, Berlin, p 375–414. https://doi.org/10.1007/978-3-642-21551-3_13
Borland D, Taylor IIRM (2007) Rainbow color map (still) considered harmful. IEEE Comput Arch Lett 27(2):14–17. https://doi.org/10.1109/MCG.2007.323435
Acampora J (2018) When to use pie charts-best practices. https://www.excelcampus.com/charts/pie-charts-best-practices . Accessed 7 July 2019
Blackwell AF, Britton C, Cox A, Green TRG, Gurr C, Kadoda G et al (2001) Cognitive dimensions of notations: design tools for cognitive technology. In: Beynon M, Nehaniv CL, Dautenhahn K (eds) Cognitive technology: instruments of mind. 4th international conference, CT 2001, August 2001. Lecture notes in computer science, vol 2117. Springer, Berlin, Heidelberg, p 325–341. https://doi.org/10.1007/3-540-44617-6_31
Nguyen VT, Jung K, Dang T (2020) Revisiting common pitfalls in graphical representations utilizing a case-based learning approach. In: Abstracts of the 13th international symposium on visual information communication and interaction, ACM, Eindhoven, 8–10 December 2020. https://doi.org/10.1145/3430036.3430071
Huff D (1993) How to lie with statistics. W. W. Norton & Company, New York
Wainer H (2013) Visual revelations: graphical tales of fate and deception from napoleon bonaparte to ross perot. Psychology Press, Hove, p 56–61. https://doi.org/10.4324/9780203774793
Book Google Scholar
Silva S, Madeira J, Santos BS (2007) There is more to color scales than meets the eye: a review on the use of color in visualization. In: Abstracts of the 11th international conference information visualization, IEEE, Zurich, 4–6 July 2007. https://doi.org/10.1109/IV.2007.113
Wang LJ, Giesen J, McDonnell KT, Zolliker P, Mueller K (2008) Color design for illustrative visualization. IEEE Trans Vis Comput Graph 14(6):1739–1754. https://doi.org/10.1109/TVCG.2008.118
Stone M (2006) Choosing colors for data visualization. https://www.perceptualedge.com/articles/b-eye/choosing_colors.pdf . Accessed 22 July 2021.
Evergreen SDH (2019) Effective data visualization: the right chart for the right data, 2nd edn. SAGE Publications, Thousands Oaks, p 264
Nguyen HN, Nguyen VT, Dang T (2020) Interface design for HCI classroom: from learners’ perspective. In: Bebis G, Yin ZZ, Kim E, Bender J, Subr K, Kwon BC, et al (eds) Advances in visual computing. 15th international symposium, ISVC 2020, October 2020. Lecture notes in computer science, vol 12510. Springer, Cham, pp 545–557. https://doi.org/10.1007/978-3-030-64559-5_43 .
Munzner T (2014) Visualization analysis and design. CRC Press, Boca Ranton. https://doi.org/10.1201/b17511
Cawthon N (2007) Qualities of perceived aesthetic in data visualization. In: Abstracts of 2007 conference on designing for user eXperiences, ACM, Chicago, 5–7 November 2007. https://doi.org/10.1145/1389908.1389920
van Wijk JJ (2006) Views on visualization. IEEE Trans Vis Comput Graph 12(4):421–432. https://doi.org/10.1109/TVCG.2006.80
Kosslyn SM (2006) Graph design for the eye and mind. Oxford University Press, New York. https://doi.org/10.1093/acprof:oso/9780195311846.001.0001
Green TRG, Petre M (1996) Usability analysis of visual programming environments: a ‘cognitive dimensions’ framework. J Vis Lang Comput 7(2):131–174. https://doi.org/10.1006/jvlc.1996.0009
Crilly N, Blackwell AF, Clarkson PJ (2006) Graphic elicitation: using research diagrams as interview stimuli. Qual Res 6(3):341–366. https://doi.org/10.1177/1468794106065007
Nguyen QV, Zhang K, Simoff S (2015) Unlocking the complexity of port data with visualization. IEEE Trans Hum-Mach Syst 45(2):272–279. https://doi.org/10.1109/THMS.2014.2369375
Shneiderman B, Plaisant C, Cohen MS, Jacobs S, Elmqvist N, Diakopoulos N (2016) Designing the user interface: strategies for effective human-computer interaction. Pearson, Boston
Tufte ER (2006) Beautiful evidence. Graphics Press, Cheshire
Moher D, Liberati A, Tetzlaff J, Altman DG, PRISMA Group (2009) Preferred reporting items for systematic reviews and meta-analyses: the PRISMA statement. PLoS Med 6(7):e1000097. https://doi.org/10.1371/journal.pmed.1000097
Sheskin DJ (2007) Handbook of parametric and nonparametric statistical procedures. Chapman & Hall/CRC, Boca Raton
MATH Google Scholar
Agrawal R, Srikant R (1994) Fast algorithms for mining association rules in large databases. In: Abstracts of the 20th international conference on very large data bases, Morgan Kaufmann Publishers Inc., San Francisco, 12–15 September 1994
Healey CG, Booth KS, Enns JT (1995) Visualizing real-time multivariate data using preattentive processing. ACM Trans Model Comput Simul 5(3):190–221. https://doi.org/10.1145/217853.217855
Light A, Bartlein PJ (2004) The end of the rainbow? Color schemes for improved data graphics. Eos Trans Am Geophys Union 85(40):385–391. https://doi.org/10.1029/2004EO400002
Data toViz (2017) The spaghetti plot. https://www.data-to-viz.com/caveat/spaghetti.html . Accessed 22 July 2021
Healy K (2018) Data visualization: a practical introduction. Princeton University Press, Princeton
Shah S (2018) Fact or fiction: five common downfalls of data visualizations. https://www.business.com/articles/datavisualization-downfalls . Accessed 22 July 2021
Wilke CO (2019) Fundamentals of data visualization: a primer on making informative and compelling figures. O’Reilly Media, Sebastopol
Data toViz (2019) Calculation errors. https://www.data-to-viz.com/caveat/calculation_error.html . Accessed 22 July 2021.
Eckardt D (2019) Case study: how baby boomers describe themselves. https://vizfix.com/case-study-how-baby-boomers-describe-themselves/ . Accessed 22 July 2021.
Acampora J (2018) When to use pie charts - best practices. https://www.excelcampus.com/charts/pie-charts-best-practices/ . Accessed 22 July 2021
Meyer D (2011) [WCYDWT] obama botches SOTU infographic, stock market reels. https://blog.mrmeyer.com/2011/wcydwt-obama-botches-sotu-infographic-stock-market-reels . Accessed 22 July 2021
Hickey W (2013) The 27 worst charts of all time. https://www.businessinsider.com/the-27-worst-charts-of-all-time-2013-6 . Accessed 22 July 2021
Visualizations W (2016) How concerned are you about the Zika virus? https://viz.wtf/ . Accessed 22 July 2021
Visualizations W (2019) WTF visualizations. https://viz.wtf/ . Accessed 22 July 2021
Download references
Acknowledgements
Not applicable.
Author information
Authors and affiliations.
Department of Information Technology, TNU – University of Information and Communication Technology, Thai Nguyen, Vietnam
Vinh T Nguyen
Department of Educational Psychology, Leadership, and Counseling, Texas Tech University, Lubbock, TX, 79409, United States
Kwanghee Jung
Department of Computer Science and Data Science, Meharry Medical College, Nashville, TN, 37208, USA
Vibhuti Gupta
You can also search for this author in PubMed Google Scholar
Contributions
VTN and KJ provided conceptualization, formal analysis and investigation, methodology and visualization; KJ provided supervision; VTN, KJ and VG provided validation; VTN, KJ and VG wrote the paper. The authors read and approved the final manuscript.
Corresponding author
Correspondence to Kwanghee Jung .
Ethics declarations
Competing interests.
The authors declare that they have no competing interests.
Additional information
Publisher’s note.
Springer Nature remains neutral with regard to jurisdictional claims in published maps and institutional affiliations.
Rights and permissions
Open Access This article is licensed under a Creative Commons Attribution 4.0 International License, which permits use, sharing, adaptation, distribution and reproduction in any medium or format, as long as you give appropriate credit to the original author(s) and the source, provide a link to the Creative Commons licence, and indicate if changes were made. The images or other third party material in this article are included in the article's Creative Commons licence, unless indicated otherwise in a credit line to the material. If material is not included in the article's Creative Commons licence and your intended use is not permitted by statutory regulation or exceeds the permitted use, you will need to obtain permission directly from the copyright holder. To view a copy of this licence, visit http://creativecommons.org/licenses/by/4.0/ .
Reprints and permissions
About this article
Cite this article.
Nguyen, V.T., Jung, K. & Gupta, V. Examining data visualization pitfalls in scientific publications. Vis. Comput. Ind. Biomed. Art 4 , 27 (2021). https://doi.org/10.1186/s42492-021-00092-y
Download citation
Received : 03 May 2021
Accepted : 19 September 2021
Published : 29 October 2021
DOI : https://doi.org/10.1186/s42492-021-00092-y
Share this article
Anyone you share the following link with will be able to read this content:
Sorry, a shareable link is not currently available for this article.
Provided by the Springer Nature SharedIt content-sharing initiative
- Data visualization
- Graphical representations
- Misinformation
- Visual encodings
- Association rule mining
- Cochran’s Q test
- McNemar’s test

An official website of the United States government
The .gov means it’s official. Federal government websites often end in .gov or .mil. Before sharing sensitive information, make sure you’re on a federal government site.
The site is secure. The https:// ensures that you are connecting to the official website and that any information you provide is encrypted and transmitted securely.
- Publications
- Account settings
Preview improvements coming to the PMC website in October 2024. Learn More or Try it out now .
- Advanced Search
- Journal List
- Patterns (N Y)
- v.1(9); 2020 Dec 11

Principles of Effective Data Visualization
Stephen r. midway.
1 Department of Oceanography and Coastal Sciences, Louisiana State University, Baton Rouge, LA 70803, USA
We live in a contemporary society surrounded by visuals, which, along with software options and electronic distribution, has created an increased importance on effective scientific visuals. Unfortunately, across scientific disciplines, many figures incorrectly present information or, when not incorrect, still use suboptimal data visualization practices. Presented here are ten principles that serve as guidance for authors who seek to improve their visual message. Some principles are less technical, such as determining the message before starting the visual, while other principles are more technical, such as how different color combinations imply different information. Because figure making is often not formally taught and figure standards are not readily enforced in science, it is incumbent upon scientists to be aware of best practices in order to most effectively tell the story of their data.
The Bigger Picture
Visuals are an increasingly important form of science communication, yet many scientists are not well trained in design principles for effective messaging. Despite challenges, many visuals can be improved by taking some simple steps before, during, and after their creation. This article presents some sequential principles that are designed to improve visual messages created by scientists.
Many scientific visuals are not as effective as they could be because scientists often lack basic design principles. This article reviews the importance of effective data visualization and presents ten principles that scientists can use as guidance in developing effective visual messages.
Introduction
Visual learning is one of the primary forms of interpreting information, which has historically combined images such as charts and graphs (see Box 1 ) with reading text. 1 However, developments on learning styles have suggested splitting up the visual learning modality in order to recognize the distinction between text and images. 2 Technology has also enhanced visual presentation, in terms of the ability to quickly create complex visual information while also cheaply distributing it via digital means (compared with paper, ink, and physical distribution). Visual information has also increased in scientific literature. In addition to the fact that figures are commonplace in scientific publications, many journals now require graphical abstracts 3 or might tweet figures to advertise an article. Dating back to the 1970s when computer-generated graphics began, 4 papers represented by an image on the journal cover have been cited more frequently than papers without a cover image. 5
Regarding terminology, the terms graph , plot , chart , image , figure , and data visual(ization) are often used interchangeably, although they may have different meanings in different instances. Graph , plot , and chart often refer to the display of data, data summaries, and models, while image suggests a picture. Figure is a general term but is commonly used to refer to visual elements, such as plots, in a scientific work. A visual , or data visualization , is a newer and ostensibly more inclusive term to describe everything from figures to infographics. Here, I adopt common terminology, such as bar plot, while also attempting to use the terms figure and data visualization for general reference.
There are numerous advantages to quickly and effectively conveying scientific information; however, scientists often lack the design principles or technical skills to generate effective visuals. Going back several decades, Cleveland 6 found that 30% of graphs in the journal Science had at least one type of error. Several other studies have documented widespread errors or inefficiencies in scientific figures. 7 , 8 , 9 In fact, the increasing menu of visualization options can sometimes lead to poor fits between information and its presentation. These poor fits can even have the unintended consequence of confusing the readers and setting them back in their understanding of the material. While objective errors in graphs are hopefully in the minority of scientific works, what might be more common is suboptimal figure design, which takes place when a design element may not be objectively wrong but is ineffective to the point of limiting information transfer.
Effective figures suggest an understanding and interpretation of data; ineffective figures suggest the opposite. Although the field of data visualization has grown in recent years, the process of displaying information cannot—and perhaps should not—be fully mechanized. Much like statistical analyses often require expert opinions on top of best practices, figures also require choice despite well-documented recommendations. In other words, there may not be a singular best version of a given figure. Rather, there may be multiple effective versions of displaying a single piece of information, and it is the figure maker's job to weigh the advantages and disadvantages of each. Fortunately, there are numerous principles from which decisions can be made, and ultimately design is choice. 7
The data visualization literature includes many great resources. While several resources are targeted at developing design proficiency, such as the series of columns run by Nature Communications , 10 Wilkinson's The Grammar of Graphics 11 presents a unique technical interpretation of the structure of graphics. Wilkinson breaks down the notion of a graphic into its constituent parts—e.g., the data, scales, coordinates, geometries, aesthetics—much like conventional grammar breaks down a sentence into nouns, verbs, punctuation, and other elements of writing. The popularity and utility of this approach has been implemented in a number of software packages, including the popular ggplot2 package 12 currently available in R. 13 (Although the grammar of graphics approach is not explicitly adopted here, the term geometry is used consistently with Wilkinson to refer to different geometrical representations, whereas the term aesthetics is not used consistently with the grammar of graphics and is used simply to describe something that is visually appealing and effective.) By understanding basic visual design principles and their implementation, many figure authors may find new ways to emphasize and convey their information.
The Ten Principles
Principle #1 diagram first.
The first principle is perhaps the least technical but very important: before you make a visual, prioritize the information you want to share, envision it, and design it. Although this seems obvious, the larger point here is to focus on the information and message first, before you engage with software that in some way starts to limit or bias your visual tools. In other words, don't necessarily think of the geometries (dots, lines) you will eventually use, but think about the core information that needs to be conveyed and what about that information is going to make your point(s). Is your visual objective to show a comparison? A ranking? A composition? This step can be done mentally, or with a pen and paper for maximum freedom of thought. In parallel to this approach, it can be a good idea to save figures you come across in scientific literature that you identify as particularly effective. These are not just inspiration and evidence of what is possible, but will help you develop an eye for detail and technical skills that can be applied to your own figures.
Principle #2 Use the Right Software
Effective visuals typically require good command of one or more software. In other words, it might be unrealistic to expect complex, technical, and effective figures if you are using a simple spreadsheet program or some other software that is not designed to make complex, technical, and effective figures. Recognize that you might need to learn a new software—or expand your knowledge of a software you already know. While highly effective and aesthetically pleasing figures can be made quickly and simply, this may still represent a challenge to some. However, figure making is a method like anything else, and in order to do it, new methodologies may need to be learned. You would not expect to improve a field or lab method without changing something or learning something new. Data visualization is the same, with the added benefit that most software is readily available, inexpensive, or free, and many come with large online help resources. This article does not promote any specific software, and readers are encouraged to reference other work 14 for an overview of software resources.
Principle #3 Use an Effective Geometry and Show Data
Geometries are the shapes and features that are often synonymous with a type of figure; for example, the bar geometry creates a bar plot. While geometries might be the defining visual element of a figure, it can be tempting to jump directly from a dataset to pairing it with one of a small number of well-known geometries. Some of this thinking is likely to naturally happen. However, geometries are representations of the data in different forms, and often there may be more than one geometry to consider. Underlying all your decisions about geometries should be the data-ink ratio, 7 which is the ratio of ink used on data compared with overall ink used in a figure. High data-ink ratios are the best, and you might be surprised to find how much non-data-ink you use and how much of that can be removed.
Most geometries fall into categories: amounts (or comparisons), compositions (or proportions), distributions , or relationships . Although seemingly straightforward, one geometry may work in more than one category, in addition to the fact that one dataset may be visualized with more than one geometry (sometimes even in the same figure). Excellent resources exist on detailed approaches to selecting your geometry, 15 and this article only highlights some of the more common geometries and their applications.
Amounts or comparisons are often displayed with a bar plot ( Figure 1 A), although numerous other options exist, including Cleveland dot plots and even heatmaps ( Figure 1 F). Bar plots are among the most common geometry, along with lines, 9 although bar plots are noted for their very low data density 16 (i.e., low data-ink ratio). Geometries for amounts should only be used when the data do not have distributional information or uncertainty associated with them. A good use of a bar plot might be to show counts of something, while poor use of a bar plot might be to show group means. Numerous studies have discussed inappropriate uses of bar plots, 9 , 17 noting that “because the bars always start at zero, they can be misleading: for example, part of the range covered by the bar might have never been observed in the sample.” 17 Despite the numerous reports on incorrect usage, bar plots remain one of the most common problems in data visualization.

Examples of Visual Designs
(A) Clustered bar plots are effective at showing units within a group (A–C) when the data are amounts.
(B) Histograms are effective at showing the distribution of data, which in this case is a random draw of values from a Poisson distribution and which use a sequential color scheme that emphasizes the mean as red and values farther from the mean as yellow.
(C) Scatterplot where the black circles represent the data.
(D) Logistic regression where the blue line represents the fitted model, the gray shaded region represents the confidence interval for the fitted model, and the dark-gray dots represent the jittered data.
(E) Box plot showing (simulated) ages of respondents grouped by their answer to a question, with gray dots representing the raw data used in the box plot. The divergent colors emphasize the differences in values. For each box plot, the box represents the interquartile range (IQR), the thick black line represents the median value, and the whiskers extend to 1.5 times the IQR. Outliers are represented by the data.
(F) Heatmap of simulated visibility readings in four lakes over 5 months. The green colors represent lower visibility and the blue colors represent greater visibility. The white numbers in the cells are the average visibility measures (in meters).
(G) Density plot of simulated temperatures by season, where each season is presented as a small multiple within the larger figure.
For all figures the data were simulated, and any examples are fictitious.
Compositions or proportions may take a wide range of geometries. Although the traditional pie chart is one option, the pie geometry has fallen out of favor among some 18 due to the inherent difficulties in making visual comparisons. Although there may be some applications for a pie chart, stacked or clustered bar plots ( Figure 1 A), stacked density plots, mosaic plots, and treemaps offer alternatives.
Geometries for distributions are an often underused class of visuals that demonstrate high data density. The most common geometry for distributional information is the box plot 19 ( Figure 1 E), which shows five types of information in one object. Although more common in exploratory analyses than in final reports, the histogram ( Figure 1 B) is another robust geometry that can reveal information about data. Violin plots and density plots ( Figure 1 G) are other common distributional geometries, although many less-common options exist.
Relationships are the final category of visuals covered here, and they are often the workhorse of geometries because they include the popular scatterplot ( Figures 1 C and 1D) and other presentations of x - and y -coordinate data. The basic scatterplot remains very effective, and layering information by modifying point symbols, size, and color are good ways to highlight additional messages without taking away from the scatterplot. It is worth mentioning here that scatterplots often develop into line geometries ( Figure 1 D), and while this can be a good thing, presenting raw data and inferential statistical models are two different messages that need to be distinguished (see Data and Models Are Different Things ).
Finally, it is almost always recommended to show the data. 7 Even if a geometry might be the focus of the figure, data can usually be added and displayed in a way that does not detract from the geometry but instead provides the context for the geometry (e.g., Figures 1 D and 1E). The data are often at the core of the message, yet in figures the data are often ignored on account of their simplicity.
Principle #4 Colors Always Mean Something
The use of color in visualization can be incredibly powerful, and there is rarely a reason not to use color. Even if authors do not wish to pay for color figures in print, most journals still permit free color figures in digital formats. In a large study 20 of what makes visualizations memorable, colorful visualizations were reported as having a higher memorability score, and that seven or more colors are best. Although some of the visuals in this study were photographs, other studies 21 also document the effectiveness of colors.
In today's digital environment, color is cheap. This is overwhelmingly a good thing, but also comes with the risk of colors being applied without intention. Black-and-white visuals were more accepted decades ago when hard copies of papers were more common and color printing represented a large cost. Now, however, the vast majority of readers view scientific papers on an electronic screen where color is free. For those who still print documents, color printing can be done relatively cheaply in comparison with some years ago.
Color represents information, whether in a direct and obvious way, or in an indirect and subtle way. A direct example of using color may be in maps where water is blue and land is green or brown. However, the vast majority of (non-mapping) visualizations use color in one of three schemes: sequential , diverging , or qualitative . Sequential color schemes are those that range from light to dark typically in one or two (related) hues and are often applied to convey increasing values for increasing darkness ( Figures 1 B and 1F). Diverging color schemes are those that have two sequential schemes that represent two extremes, often with a white or neutral color in the middle ( Figure 1 E). A classic example of a diverging color scheme is the red to blue hues applied to jurisdictions in order to show voting preference in a two-party political system. Finally, qualitative color schemes are found when the intensity of the color is not of primary importance, but rather the objective is to use different and otherwise unrelated colors to convey qualitative group differences ( Figures 1 A and 1G).
While it is recommended to use color and capture the power that colors convey, there exist some technical recommendations. First, it is always recommended to design color figures that work effectively in both color and black-and-white formats ( Figures 1 B and 1F). In other words, whenever possible, use color that can be converted to an effective grayscale such that no information is lost in the conversion. Along with this approach, colors can be combined with symbols, line types, and other design elements to share the same information that the color was sharing. It is also good practice to use color schemes that are effective for colorblind readers ( Figures 1 A and 1E). Excellent resources, such as ColorBrewer, 22 exist to help in selecting color schemes based on colorblind criteria. Finally, color transparency is another powerful tool, much like a volume knob for color ( Figures 1 D and 1E). Not all colors have to be used at full value, and when not part of a sequential or diverging color scheme—and especially when a figure has more than one colored geometry—it can be very effective to increase the transparency such that the information of the color is retained but it is not visually overwhelming or outcompeting other design elements. Color will often be the first visual information a reader gets, and with this knowledge color should be strategically used to amplify your visual message.
Principle #5 Include Uncertainty
Not only is uncertainty an inherent part of understanding most systems, failure to include uncertainty in a visual can be misleading. There exist two primary challenges with including uncertainty in visuals: failure to include uncertainty and misrepresentation (or misinterpretation) of uncertainty.
Uncertainty is often not included in figures and, therefore, part of the statistical message is left out—possibly calling into question other parts of the statistical message, such as inference on the mean. Including uncertainty is typically easy in most software programs, and can take the form of common geometries such as error bars and shaded intervals (polygons), among other features. 15 Another way to approach visualizing uncertainty is whether it is included implicitly into the existing geometries, such as in a box plot ( Figure 1 E) or distribution ( Figures 1 B and 1G), or whether it is included explicitly as an additional geometry, such as an error bar or shaded region ( Figure 1 D).
Representing uncertainty is often a challenge. 23 Standard deviation, standard error, confidence intervals, and credible intervals are all common metrics of uncertainty, but each represents a different measure. Expressing uncertainty requires that readers be familiar with metrics of uncertainty and their interpretation; however, it is also the responsibility of the figure author to adopt the most appropriate measure of uncertainty. For instance, standard deviation is based on the spread of the data and therefore shares information about the entire population, including the range in which we might expect new values. On the other hand, standard error is a measure of the uncertainty in the mean (or some other estimate) and is strongly influenced by sample size—namely, standard error decreases with increasing sample size. Confidence intervals are primarily for displaying the reliability of a measurement. Credible intervals, almost exclusively associated with Bayesian methods, are typically built off distributions and have probabilistic interpretations.
Expressing uncertainty is important, but it is also important to interpret the correct message. Krzywinski and Altman 23 directly address a common misconception: “a gap between (error) bars does not ensure significance, nor does overlap rule it out—it depends on the type of bar.” This is a good reminder to be very clear not only in stating what type of uncertainty you are sharing, but what the interpretation is. Others 16 even go so far as to recommend that standard error not be used because it does not provide clear information about standard errors of differences among means. One recommendation to go along with expressing uncertainty is, if possible, to show the data (see Use an Effective Geometry and Show Data ). Particularly when the sample size is low, showing a reader where the data occur can help avoid misinterpretations of uncertainty.
Principle #6 Panel, when Possible (Small Multiples)
A particularly effective visual approach is to repeat a figure to highlight differences. This approach is often called small multiples , 7 and the technique may be referred to as paneling or faceting ( Figure 1 G). The strategy behind small multiples is that because many of the design elements are the same—for example, the axes, axes scales, and geometry are often the same—the differences in the data are easier to show. In other words, each panel represents a change in one variable, which is commonly a time step, a group, or some other factor. The objective of small multiples is to make the data inevitably comparable, 7 and effective small multiples always accomplish these comparisons.
Principle #7 Data and Models Are Different Things
Plotted information typically takes the form of raw data (e.g., scatterplot), summarized data (e.g., box plot), or an inferential statistic (e.g., fitted regression line; Figure 1 D). Raw data and summarized data are often relatively straightforward; however, a plotted model may require more explanation for a reader to be able to fully reproduce the work. Certainly any model in a study should be reported in a complete way that ensures reproducibility. However, any visual of a model should be explained in the figure caption or referenced elsewhere in the document so that a reader can find the complete details on what the model visual is representing. Although it happens, it is not acceptable practice to show a fitted model or other model results in a figure if the reader cannot backtrack the model details. Simply because a model geometry can be added to a figure does not mean that it should be.
Principle #8 Simple Visuals, Detailed Captions
As important as it is to use high data-ink ratios, it is equally important to have detailed captions that fully explain everything in the figure. A study of figures in the Journal of American Medicine 8 found that more than one-third of graphs were not self-explanatory. Captions should be standalone, which means that if the figure and caption were looked at independent from the rest of the study, the major point(s) could still be understood. Obviously not all figures can be completely standalone, as some statistical models and other procedures require more than a caption as explanation. However, the principle remains that captions should do all they can to explain the visualization and representations used. Captions should explain any geometries used; for instance, even in a simple scatterplot it should be stated that the black dots represent the data ( Figures 1 C–1E). Box plots also require descriptions of their geometry—it might be assumed what the features of a box plot are, yet not all box plot symbols are universal.
Principle #9 Consider an Infographic
It is unclear where a figure ends and an infographic begins; however, it is fair to say that figures tend to be focused on representing data and models, whereas infographics typically incorporate text, images, and other diagrammatic elements. Although it is not recommended to convert all figures to infographics, infographics were found 20 to have the highest memorability score and that diagrams outperformed points, bars, lines, and tables in terms of memorability. Scientists might improve their overall information transfer if they consider an infographic where blending different pieces of information could be effective. Also, an infographic of a study might be more effective outside of a peer-reviewed publication and in an oral or poster presentation where a visual needs to include more elements of the study but with less technical information.
Even if infographics are not adopted in most cases, technical visuals often still benefit from some text or other annotations. 16 Tufte's works 7 , 24 provide great examples of bringing together textual, visual, and quantitative information into effective visualizations. However, as figures move in the direction of infographics, it remains important to keep chart junk and other non-essential visual elements out of the design.
Principle #10 Get an Opinion
Although there may be principles and theories about effective data visualization, the reality is that the most effective visuals are the ones with which readers connect. Therefore, figure authors are encouraged to seek external reviews of their figures. So often when writing a study, the figures are quickly made, and even if thoughtfully made they are not subject to objective, outside review. Having one or more colleagues or people external to the study review figures will often provide useful feedback on what readers perceive, and therefore what is effective or ineffective in a visual. It is also recommended to have outside colleagues review only the figures. Not only might this please your colleague reviewers (because figure reviews require substantially less time than full document reviews), but it also allows them to provide feedback purely on the figures as they will not have the document text to fill in any uncertainties left by the visuals.
What About Tables?
Although often not included as data visualization, tables can be a powerful and effective way to show data. Like other visuals, tables are a type of hybrid visual—they typically only include alphanumeric information and no geometries (or other visual elements), so they are not classically a visual. However, tables are also not text in the same way a paragraph or description is text. Rather, tables are often summarized values or information, and are effective if the goal is to reference exact numbers. However, the interest in numerical results in the form of a study typically lies in comparisons and not absolute numbers. Gelman et al. 25 suggested that well-designed graphs were superior to tables. Similarly, Spence and Lewandowsky 26 compared pie charts, bar graphs, and tables and found a clear advantage for graphical displays over tabulations. Because tables are best suited for looking up specific information while graphs are better for perceiving trends and making comparisons and predictions, it is recommended that visuals are used before tables. Despite the reluctance to recommend tables, tables may benefit from digital formats. In other words, while tables may be less effective than figures in many cases, this does not mean tables are ineffective or do not share specific information that cannot always be displayed in a visual. Therefore, it is recommended to consider creating tables as supplementary or appendix information that does not go into the main document (alongside the figures), but which is still very easily accessed electronically for those interested in numerical specifics.
Conclusions
While many of the elements of peer-reviewed literature have remained constant over time, some elements are changing. For example, most articles now have more authors than in previous decades, and a much larger menu of journals creates a diversity of article lengths and other requirements. Despite these changes, the demand for visual representations of data and results remains high, as exemplified by graphical abstracts, overview figures, and infographics. Similarly, we now operate with more software than ever before, creating many choices and opportunities to customize scientific visualizations. However, as the demand for, and software to create, visualizations have both increased, there is not always adequate training among scientists and authors in terms of optimizing the visual for the message.
Figures are not just a scientific side dish but can be a critical point along the scientific process—a point at which the figure maker demonstrates their knowledge and communication of the data and results, and often one of the first stopping points for new readers of the information. The reality for the vast majority of figures is that you need to make your point in a few seconds. The longer someone looks at a figure and doesn't understand the message, the more likely they are to gain nothing from the figure and possibly even lose some understanding of your larger work. Following a set of guidelines and recommendations—summarized here and building on others—can help to build robust visuals that avoid many common pitfalls of ineffective figures ( Figure 2 ).

Overview of the Principles Presented in This Article
The two principles in yellow (bottom) are those that occur first, during the figure design phase. The six principles in green (middle) are generally considerations and decisions while making a figure. The two principles in blue (top) are final steps often considered after a figure has been drafted. While the general flow of the principles follows from bottom to top, there is no specific or required order, and the development of individual figures may require more or less consideration of different principles in a unique order.
All scientists seek to share their message as effectively as possible, and a better understanding of figure design and representation is undoubtedly a step toward better information dissemination and fewer errors in interpretation. Right now, much of the responsibility for effective figures lies with the authors, and learning best practices from literature, workshops, and other resources should be undertaken. Along with authors, journals play a gatekeeper role in figure quality. Journal editorial teams are in a position to adopt recommendations for more effective figures (and reject ineffective figures) and then translate those recommendations into submission requirements. However, due to the qualitative nature of design elements, it is difficult to imagine strict visual guidelines being enforced across scientific sectors. In the absence of such guidelines and with seemingly endless design choices available to figure authors, it remains important that a set of aesthetic criteria emerge to guide the efficient conveyance of visual information.
Acknowledgments
Thanks go to the numerous students with whom I have had fun, creative, and productive conversations about displaying information. Danielle DiIullo was extremely helpful in technical advice on software. Finally, Ron McKernan provided guidance on several principles.
Author Contributions
S.R.M. conceived the review topic, conducted the review, developed the principles, and wrote the manuscript.
Steve Midway is an assistant professor in the Department of Oceanography and Coastal Sciences at Louisiana State University. His work broadly lies in fisheries ecology and how sound science can be applied to management and conservation issues. He teaches a number of quantitative courses in ecology, all of which include data visualization.
Ohio State nav bar
The Ohio State University
- BuckeyeLink
- Find People
- Search Ohio State

- Research Commons
Connect. Collaborate. Contribute.
- Ohio State University Libraries
- Research Guides
Data Visualization
- Visualization best practices
- What is data visualization?
- Know Your Audience
Provide context and incorporate instructions
Add interactivity, apply visual best practices, choose an effective visual, test, test, test.
- Visualization Software, Programming Languages & Tools
Visualization Best Practices
Know your audience.
Think about the purpose of your visualization. Are you explaining or exploring a phenomenon? Will your audience better understand and respond to a simple respresentation of data, or something more complex.
The typical academic research paper provides a story arc, with introduction, background, literature review, methodology, discussion, and conclusion, which Stephanie Evergreen effectively points out in Effective Data Visualization , may not be appropriate for all audiences. In "fast-paced, decision-making contexts," she writes, "I don't think we actually want a story. We want an interpretation." This nuance is especially important when visualizing data. If your audience needs to reach a decision using available data, help your audience interpret the data you have visualized. Use graph titles and other visualization techniques to help audience members focus their attention towards key takeaways in your data.
Add interactivity to your visualization if possible, using tools like R Shiny or Tableau. This can allow anyone using your visualization to apply filters or seek aditional context for a data point.

Choosing an effective visual is one of the most difficult and rewarding aspects of visualizing data. Fortunately, a small number of graphs, when skillfully formatted and presented, fulfill most data visualization needs.
If you wish to display a relationship, comparison, composition, or distribution, Abela's Chart Chooser directs you to various charts based on the number of dimensions -- or categories -- and/or the number of measures -- or variables --- you have to visualize.

Quantitative/Qualitative Chart Chooser
If you are thinking about how to influence action using the data you've collected, Stephanie Evergreen's Quantitative Chart Chooser , which is published on the inside front cover of her book Effective Data Visualization, encourages you to consider what "you need the audience to do when viewing the data." If you need your audience to focus on a single number, try a large call-out number or icon array. If you need to show how a number changes over time, try a line chart or slope graph.
If you are struggling to find an effective way to share qualitative data, Evergreen's Qualitative Chart Chooser , which is published on the inside back cover, helps to connect the story in your data with effective visualization types. (See Chapter 8: "When Words Have Meaning: Visualizing Qualitative Data."
What chart to choose depends on your audience, your message - or what you're trying to show - and the dimensions and measures in your dataset.
Ask a colleague or friend to critique your visual. Try visualizing your data in two or three different ways to determine which presentation best suits your audience.
- << Previous: What is data visualization?
- Next: Visualization Software, Programming Languages & Tools >>

© The Ohio State University - University Libraries
1858 Neil Avenue Mall, Columbus, OH 43210
Phone: (614) 292-OSUL (6785)
Request an alternate format of this page | Accessibility | Privacy Policy | Contact Us
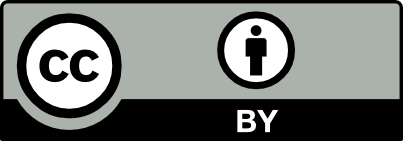
Copyright Information | Details and Exceptions
Visual narrative for data journalism based on user experience
- Regular Paper
- Published: 27 June 2024
Cite this article
- Shixiong Cao ORCID: orcid.org/0009-0002-9640-7771 1 ,
- Qing Chen 1 &
- Nan Cao 1
As data journalism continues to rise, narrative visualization has emerged as an essential method for conveying information. To improve the user experience of narrative visualization projects for data journalism, this study introduces an innovative approach for narrative visualization design centered on user experience. Firstly, through an in-depth analysis of existing research, we constructed a comprehensive user-experience-based narrative visualization model, considering the designers’ design process and the multiple levels of the user experience process. Then, through case analysis and user interviews, we identified the key elements that influence the user experience. Through the analysis of multiple cases, this study presents a practical narrative visualization design methodology comprising eight dimensions, aimed at enhancing user experience. The primary contribution of this research lies in the proposal of a practical narrative visualization model and the clear definition of key design elements, providing a comprehensive reference framework for designers and researchers to effectively optimize the user experience of narrative visualization. Moreover, our research findings unveil the inherent correlation between user experience and design elements, offering valuable insights for future research and practical applications.
Graphical Abstract
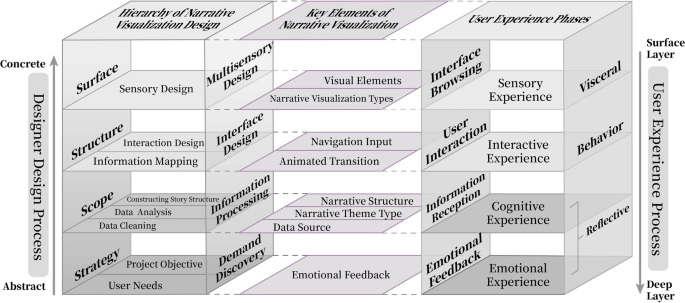
This is a preview of subscription content, log in via an institution to check access.
Access this article
Price includes VAT (Russian Federation)
Instant access to the full article PDF.
Rent this article via DeepDyve
Institutional subscriptions
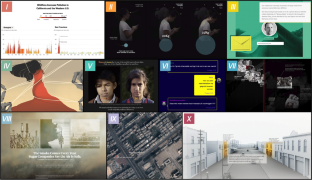
Al Jazeera Media Network 101 East, AJLabs (2021) This is Myanmar’s state of fear. https://sigmaawards.org/this-is-myanmars-state-of-fear/ . Accessed 04 May 2023
Anderson CW, Bell E, Shirky C (2015) Post-industrial journalism: adapting to the present. Geopolit Hist Int Relat 7(2):32
Google Scholar
Arab Reporters for Investigative Journalism (ARIJ) (2021) Lanes of death in east Cairo. https://sigmaawards.org/lanes-of-death-in-east-cairo/ . Accessed 04 05 2023
Arjun S, Drucker Steven M, Alex E, John S (2018) Augmenting visualizations with interactive data facts to facilitate interpretation and communication. IEEE Trans Vis Comput Graph 25(1):672–681
Bach B, Wang Z, Farinella M, Murray-Rust D, Henry Riche N (2018) Design patterns for data comics. In: Proceedings of the 2018 CHI conference on human factors in computing systems, pp 1–12
Borkin MA, Bylinskii Z, Kim NW, Bainbridge CM, Yeh CS, Borkin D, Pfister H, Oliva A (2015) Beyond memorability: visualization recognition and recall. IEEE Trans Vis Comput Graph 22(1):519–528
Article Google Scholar
Bradshaw P (2011) The inverted pyramid of data journalism. http://onlinejournalismblog.com/2011/07/07/the-inverted-pyramid-of-data-journalism/ . Accessed 04 May 2023
Brooks D (2013) What data can’t do The New York Times , vol 2
Cai X, Zhu J (2010) Concerning the expression of visual elements in information visualization. Creativ Des 6:29–31
Chen Q (2020) Research on the presentation of blockchain information based on cognitive experience. Master thesis, Hunan University of Technology, Zhuzhou, China
Chen Q, Cao S, Wang J, Cao N (2023) How does automation shape the process of narrative visualization: a survey of tools. IEEE Trans Vis Comput Graph. https://doi.org/10.1109/TVCG.2023.3261320
Book Google Scholar
Chu M, Tian S (2016) Research on the design methods of knowledge visualization. Sci Technol Vis 15:267–268
Forlizzi J, Battarbee K (2004) Understanding experience in interactive systems. In: Proceedings of the 5th conference on Designing interactive systems: processes, practices, methods, and techniques, pp 261–268
Google (2014) Material design. https://m2.material.io/design/motion/the-motion-system.html . Accessed 02 Mar 2023
Harrison L, Reinecke K, Chang R (2015) Infographic aesthetics: designing for the first impression. In Proceedings of the 33rd annual ACM conference on human factors in computing systems, pp 1187–1190
Hasan MT, Wolff A, Knutas A, Pässilä A, Kantola L (2022) Playing games through interactive data comics to explore water quality in a lake: a case study exploring the use of a data-driven storytelling method in co-design. In: CHI conference on human factors in computing systems extended abstracts, pp 1–7
Hassenzahl M, Tractinsky N (2006) User experience—a research agenda. Behav Inf Technol 25(2):91–97
HKYoyo (2020) Pagination versus infinite scroll. https://www.hkyoyo.com/blog/pagination-vs-infinite-scroll . Accessed 24 Jun 2023
Hua P (2021) Metaphor in visualization: research on narrative information visualization design methods. Art Obs 03:168–169
Hullman J, Drucker S, Riche NH, Lee B, Fisher D, Adar E (2013) A deeper understanding of sequence in narrative visualization. IEEE Trans Vis Comput Graph 19(12):2406–2415
Jesse James Garrett (2022) The elements of user experience. MyNavi Publishing, Tokyo, Japan
Jiang Z, Wang W, Tan BCY, Jie Yu (2016) The determinants and impacts of aesthetics in users’ first interaction with websites. J Manag Inf Syst 33(1):229–259
Jiang L, Wan L, Yan Yu (2017) Research on the user experience model in utilizing digital learning resources. Modern Educ Technol 27(03):85–92
Kennedy H, Hill RL (2018) The feeling of numbers: emotions in everyday engagements with data and their visualisation. Sociology 52(4):830–848
Kloop (2020) I would have killed her anyway”. Kloop’s investigation of femicide in kyrgyzstan. https://kloop.kg/blog/2021/01/28/femicide-in-kyrgyzstan/ . Accessed 04 May 2023
Kosara R, Mackinlay J (2013) Storytelling: the next step for visualization. Computer 46(5):44–50
Lan X, Shi Y, Zhang Y, Cao N (2021) Smile or scowl? Looking at infographic design through the affective lens. IEEE Trans Vis Comput Graph 27(6):2796–2807
Lan X, Wu Y, Shi Y, Chen Q, Cao N (2022) Negative emotions, positive outcomes? Exploring the communication of negativity in serious data stories. In: Proceedings of the 2022 CHI conference on human factors in computing systems, pp 1–14
Lan X, Xu X, Cao N (2021) Understanding narrative linearity for telling expressive time-oriented stories. In: Proceedings of the 2021 CHI conference on human factors in computing systems, pp. 1–13
Lin H, Moritz D, Heer J (2020) Dziban: balancing agency and automation in visualization design via anchored recommendations. In: Proceedings of the 2020 CHI conference on human factors in computing systems, pp 1–12
Lu J, Liu Y, Zhang X, Zhang J, Huang Q, Meng L (2016) Data visualization model based on the user experience. Packag Eng 37(2):52–56
Luo S, Zhu S, Sun S (2002) Human–computer interface design. China Machine Press, Beijing
McKenna S, Henry Riche N, Lee B, Boy J, Meyer M (2017) Visual narrative flow: exploring factors shaping data visualization story reading experiences. Comput Graph Forum 36:377–387
Munzner T (2009) A nested model for visualization design and validation. IEEE Trans Vis Comput Graph 15(6):921–928
Na N (2018) Research on data visualisation winning works of the data journalism awards. Master’s thesis, Lanzhou University, Lanzhou, China
Norman DA (1988) The psychology of everyday things. Basic Books, New York
Pointer (KRO-NCRV) (2020) Danish scam. https://sigmaawards.org/best-visualization-small-newsrooms-2020/ . Accessed 04 May 2023
Pointer (KRO-NCRV) (2021) The digital army. https://sigmaawards.org/the-digital-army/ . Accessed 04 May 2023
ProPublica The Palm Beach Post (2021) Black snow: Big sugar’s burning problem. https://sigmaawards.org/black-snow-big-sugars-burning-problem/ . Accessed 04 May 2023
Sacha D, Stoffel A, Stoffel F, Kwon BC, Ellis G, Keim DA (2014) Knowledge generation model for visual analytics. IEEE Trans Vis Comput Graph 20(12):1604–1613
Saket B, Endert A, Stasko J (2016) Beyond usability and performance: a review of user experience-focused evaluations in visualization. In: Proceedings of the 6th workshop on beyond time and errors on novel evaluation methods for visualization, pp 133–142
Sallam S, Sakamoto Y, Leboe-McGowan J, Latulipe C, Irani P (2022) Towards design guidelines for effective health-related data videos: an empirical investigation of affect, personality, and video content. In: Proceedings of the 2022 CHI conference on human factors in computing systems, pp 1–22
Schmitt B (1999) Experiential marketing. J Market Manag 15(1–3):53–67
Segel E, Heer J (2010) Narrative visualization: telling stories with data. IEEE Trans Vis Comput Graph 16(6):1139–1148
Shihu X, Fang S (2011) Information visualization design based on visual thinking. Packag Eng 32(16):11–14
Skelton CHAD (2014) How to crowd source a data journalism course. Media 1:21
South China Morning Post (2020) Why your smartphone is causing you ‘text neck’ syndrome. https://sigmaawards.org/best-visualization-large-newsrooms-2020-2/ . Accessed 04 May 2023
The New York Times (2020) See how the world’s most polluted air compares with your city’s. https://sigmaawards.org/best-visualization-large-newsrooms-2020/ . Accessed 04 May 2023
The New York Times (2020) Who gets to breathe clean air in new Delhi? https://sigmaawards.org/who-gets-to-breathe-clean-air-in-new-delhi/ . Accessed 04 May 2023
The New York Times (2021) What the 1921 Tulsa race massacre destroyed. https://sigmaawards.org/what-the-1921-tulsa-race-massacre-destroyed/ . Accessed 04 May 2023
Thudt A, Lee B, Choe EK, Carpendale S (2017) Expanding research methods for a realistic understanding of personal visualization. IEEE Comput Graph Appl 37(2):12–18
Tong C, Roberts R, Borgo R, Walton S, Laramee RS, Wegba K, Aidong L, Wang Y, Huamin Q, Luo Q et al (2018) Storytelling and visualization: an extended survey. Information 9(3):65
Tyagi A, Zhao J, Patel P, Khurana S, Klaus M (2021) User-centric semi-automated infographics authoring and recommendation. arXiv:2108.11914
Tynan C, McKechnie S (2009) Experience marketing: a review and reassessment. J Market Manag 25(5–6):501–517
Wang Y (2018) Research on data visualization design in smart city application scenarios. Master thesis, Shenyang Jianzhu University, Shenyang, China
Wang Z, Wang S, Farinella M, Murray-Rust D, Henry Riche N, Bach B (2019) Comparing effectiveness and engagement of data comics and infographics. In: Proceedings of the 2019 CHI conference on human factors in computing systems, pp 1–12
Wu B (2016) The research on designing user-oriented data visualization. Master’s thesis, Wuhan University of Technology, Wuhan, China
Yin H (2018) Research on information visualization design based on cognitive experience. Master thesis, China Central Academy of Fine Arts, Beijing, China
Zhu G, Guo L, Yin G, Yongli X (2010) Map design and compilation. Wuhan University Press, Wuhan
Download references
Acknowledgements
This work was supported in part by the NSFC 62002267, 62372327, 62072338, NSF Shanghai 23ZR1464700, Shanghai Education Development Foundation “Chen-Guang Project” (21CGA75), and China Postdoctoral Science Foundation (2023M732674). We would like to thank anonymous reviewers for their constructive feedback.
Author information
Authors and affiliations.
Intelligent Big Data Visualization Lab, Tongji University, Shanghai, China
Shixiong Cao, Qing Chen & Nan Cao
You can also search for this author in PubMed Google Scholar
Corresponding author
Correspondence to Qing Chen .
Additional information
Publisher's note.
Springer Nature remains neutral with regard to jurisdictional claims in published maps and institutional affiliations.
Rights and permissions
Springer Nature or its licensor (e.g. a society or other partner) holds exclusive rights to this article under a publishing agreement with the author(s) or other rightsholder(s); author self-archiving of the accepted manuscript version of this article is solely governed by the terms of such publishing agreement and applicable law.
Reprints and permissions
About this article
Cao, S., Chen, Q. & Cao, N. Visual narrative for data journalism based on user experience. J Vis (2024). https://doi.org/10.1007/s12650-024-01005-w
Download citation
Received : 23 November 2023
Revised : 23 November 2023
Accepted : 10 April 2024
Published : 27 June 2024
DOI : https://doi.org/10.1007/s12650-024-01005-w
Share this article
Anyone you share the following link with will be able to read this content:
Sorry, a shareable link is not currently available for this article.
Provided by the Springer Nature SharedIt content-sharing initiative
- User experience
- Narrative visualization
- Data journalism
- Design elements
- Find a journal
- Publish with us
- Track your research
Analysis of the Causes of Car Accidents in the United States of America in 2023: Gauge People Understanding of Data Visualisation
- Alhazmi, Hamoud
- Morales, Marcelo
- Jiang, Jiachen
- Zhou, Jinxin
This paper presents a comprehensive examination of interactive data visualization tools and their efficacy in the context of United States car accident data for the year 2023. We developed interactive heatmaps, histograms, and pie charts to enhance the understanding of accident severity distribution over time and location. Our research included the creation and distribution of an online survey, consisting of nine questions designed to test participants comprehension of the presented data. Fifteen respondents were recruited to complete the survey, with the intent of assessing the effectiveness of both static and interactive versions of each visualization tool. The results indicated that participants using interactive heatmaps showed a greater understanding of the data, as compared to those using histograms and pie charts. In contrast, no notable difference in comprehension was observed between users of static and interactive histograms. Unexpectedly, static pie charts were found to be slightly more effective than their interactive counterparts. These findings suggest that while interactive visualizations can be powerful, their utility may vary depending on the type and complexity of the data presented. Future research is recommended to explore the influence of socioeconomic factors on the understanding of car accident data, potentially leading to more tailored and effective visualization strategies. This could provide deeper insights into the patterns and causes of car accidents, facilitating better-informed decision-making for stakeholders. Visit our website to explore our interactive plots and engage directly with the data for a more comprehensive understanding of our findings.
- Computer Science - Human-Computer Interaction
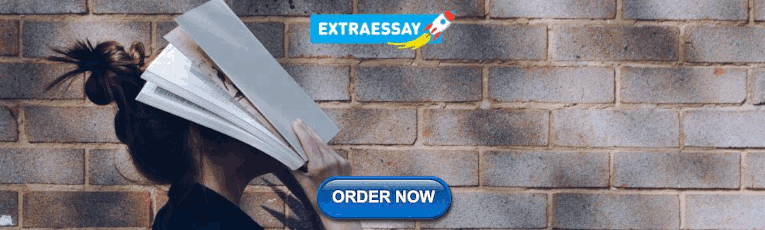
IMAGES
VIDEO
COMMENTS
Dating back to the 1970s when computer-generated graphics began, 4 papers represented by an image on the journal cover have been cited more frequently than papers ... such as plots, in a scientific work. A visual, or data visualization, is a newer and ostensibly more inclusive term to describe everything from figures to infographics. Here, I ...
The paper concludes by discussing how academic research might proceed in investigating the efficacy of interactive data visualization tools for fraud detection. View Show abstract
A data visualization based around the median is the box plot, pioneered by Spear (1952) and enhanced into its current form by Tukey (1977). For a dated visualization, the box plot remains extremely effective in conveying a large amount of information about the underlying data. Yet modern improvements have been made.
Understanding a visualization can depend on a graph schema: a knowledge structure that includes default expectations, rules, and associations that a viewer uses to extract conceptual information from a data visualization. Figure 16 serves as an example of why a graph schema is often needed to interpret a data visualization. It depicts the GDP ...
Data visualization [1] [2] [3] is a powerful tool for. enhancing understanding and co mmun ication of complex. data. It involves representing data in a graphical or pictorial. form, mak ing it ...
Other visualization methods involve dimensionality reduction techniques, which can lead to losing important information and reducing the interpretability of the data. In this paper, the Class ...
2.2.1 Introduction to Data Visualization. Before introducing the data visualization project and assigning teams, a PowerPoint presentation which defines data visualization and explains the principles of graphic design based on work by Alberto Cairo (Citation 2015) and principles of analytical design provided by Edward Tufte (Citation 1982), is shared with the class (see Table 1).
Like visualisations, underlying data sources have been examined through a number of studies. In the UK mainstream news media (N = 106), institutional sources, particularly government agencies, are the most used data sources; press releases by research institutes are often referred to in stories on social issues and health (Knight Citation 2015).
This statement emphasizes that data visualization as scientific research relies on computing technology and its utilization for the process of information. For this research, it is necessary to initially define the concept of data visualization in order to explore and identify the key forms and characteristics for designing a theoretical ...
From Pixels to Insights: A Survey on Automatic Chart Understanding in the Era of Large Foundation Models. khuangaf/awesome-chart-understanding • 18 Mar 2024 This survey paper serves as a comprehensive resource for researchers and practitioners in the fields of natural language processing, computer vision, and data analysis, providing valuable insights and directions for future research in ...
This paper provides a multi-disciplinary overview of the research issues and achievements in the field of Big Data and its visualization techniques and tools. The main aim is to summarize challenges in visualization methods for existing Big Data, as well as to offer novel solutions for issues related to the current state of Big Data Visualization. This paper provides a classification of ...
This paper provides an in-depth study and analysis of big-data-driven data visualization and visual communication design models. The characteristics of new media and the definition of traditional media are analyzed; the importance of the new media environment is derived through comparison; and the successful cases of new media integration today are analyzed.
Data visualization is a tool of data literacy: "Data visualization is the graphical representation of information and data. By using visual elements like charts, graphs, and maps, data visualization tools provide an accessible way to see and understand trends, outliers, and patterns in data" ( Tableau.com 2019, online).
Data visualization is a general term that describes any effort to help people understand the significance of data by placing it in a visual context. Patterns, trends and correlations that might go undetected in text-based data can be exposed and recognized easier with data visualization software. Data visualization is the presentation of quantitative information in a graphical form. In other ...
Paper • The following article is Open access. Research on Python Data Visualization Technology. Shengjia Cao 1, Yunhan ... researchers at home and abroad have accumulated a lot of experience in the research of data visualization technology, and they have played animportant role in scientific discovery, medical diagnosis, business decision ...
Visualization Tips for Exploratory Phase 1. List variables 2. List data types & relationships of interest 3. Produce many, quick visualizations programming very helpful here 32 Your Turn! Use your own research topic Common Charts Histograms Boxplots Scatter Plots Bar Charts Line Charts Data Types Continuous Ordinal Discrete Charts in R
Use of the programming language R (R Core Team, 2021) for data processing and statistical analysis by researchers is increasingly common; there was an average yearly growth of 87% in the number of citations of the R Core Team between 2006 and 2018 (Barrett, 2019).In addition to benefiting reproducibility and transparency, one of the advantages of using R is that researchers have a much larger ...
Data visualization tools have the potential to support decision-making for public health professionals. This review summarizes the science and evidence regarding data visualization and its impact ...
Data visualization blends art and science to convey stories from data via graphical representations. Considering different problems, applications, requirements, and design goals, it is challenging to combine these two components at their full force. While the art component involves creating visually appealing and easily interpreted graphics for users, the science component requires accurate ...
Dating back to the 1970s when computer-generated graphics began, 4 papers represented by an image on the journal cover have been cited more frequently than papers ... such as plots, in a scientific work. A visual, or data visualization, is a newer and ostensibly more inclusive term to describe everything from figures to infographics. Here, I ...
The paper analyzes 25 studies across disciplines. The findings suggest there is little agreement on the best way to visualize complex data for lay audiences, but some ... practice. Currently, data visualization research is dispersed across a range of fields and disciplines, which makes it difficult to build a coherent body of research. For
The typical academic research paper provides a story arc, with introduction, background, literature review ... New from Stephanie Evergreen! The Data Visualization Sketchbook provides advice on getting started with sketching and offers tips, guidance, and completed sample sketches for a number of reporting formats. Bundle Effective Data ...
As data journalism continues to rise, narrative visualization has emerged as an essential method for conveying information. To improve the user experience of narrative visualization projects for data journalism, this study introduces an innovative approach for narrative visualization design centered on user experience. Firstly, through an in-depth analysis of existing research, we constructed ...
using data visualization tools and techniques to display beautiful and attractive data in the pictorial format. Same is the case with educational institutions. We can use visual feature s like ...
This paper presents a comprehensive examination of interactive data visualization tools and their efficacy in the context of United States car accident data for the year 2023. We developed interactive heatmaps, histograms, and pie charts to enhance the understanding of accident severity distribution over time and location. Our research included the creation and distribution of an online survey ...