Skip to content
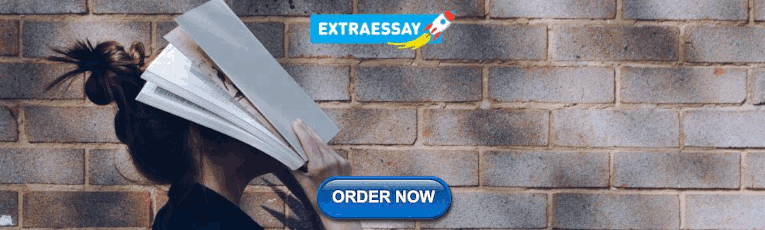
Georgia Institute of Technology
Search form.
- You are here:
- PhD Program
Fellowship & Award Opportunities
There are a variety of internal and external fellowship and award opportunities for graduate students. Note:
- Always check the official websites for the most accurate deadlines, eligibility and benefits , as they tend to change each year (sometimes can shift by months) and likely do not fully synchronize with information shown on this page. The deadlines show below are the typical deadlines from previous years.
- Some opportunities require coordination within Georgia Tech (e.g., 2 total nominees from GT). We highlight such opportunities' deadlines in yellow ; internal deadlines are typically one month earlier (e.g., suppose a fellowship's official deadline is 9/30 , assume 8/30 as its GT-internal deadline).
- This fellowship listing is centered on Computing students. Georgia Tech's Fellowships Office is available for ALL students.
For questions, updates and corrections, email us .
We thank Carnegie Mellon University's Computer Science Department for their list of fellowships that we have adapted, and the many Georiga Tech staff and faculty members who have contributed to the curation of this list.
Georgia Tech Resources
- Offices & Departments
- News Center
- Campus Calendar
- Special Events
- Institute Communications
Visitor Resources
- Campus Visits
- Directions to Campus
- Visitor Parking Information
- GTvisitor Wireless Network Information
- Georgia Tech Global Learning Center
- Georgia Tech Hotel & Conference Center
- Barnes & Noble at Georgia Tech
- Ferst Center for the Arts
- Robert C. Williams Paper Museum
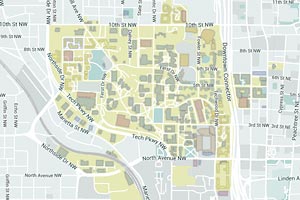
Georgia Institute of Technology North Avenue, Atlanta, GA 30332 Phone: 404-894-2000
Apple Scholars in AI/ML PhD Fellowship Program
The Apple Scholars in AI/ML PhD fellowship program recognizes the contributions of emerging leaders in computer science and engineering at the graduate and postgraduate level. The PhD fellowship in AI/ML was created as part of the Apple Scholars program to support the work of outstanding PhD students from around the world, who are pursuing cutting edge research in machine learning and artificial intelligence.
Duke may nominate up to (3) students. Universities may nominate a maximum of (1) student to any of the research areas (see below).
Guidelines will be released in June. The password to access the guidelines will be available by request. Duke students interested in this opportunity can contact [email protected] .
- Duke Internal Deadline: July 8, 2024
- Deadline for Nominations: Sep. 10, 2024
Each university may nominate up to (3) students. Universities may nominate a maximum of (1) student to any of the following research areas:
- Privacy Preserving Machine Learning
- Human Centered AI
- AI for Ethics and Fairness
- AI for Accessibility
- AI for Health and Wellness
- ML Algorithms and Architectures
- Embodied ML
- Speech and Natural Language
- Computer Vision
- Information Retrieval and Knowledge
- Data-Centric AI
Nominees must meet the following criteria to be considered:
• Nominee must be enrolled full time at the nominating university at the start of Fall 2025, and expect to be enrolled through the end of the 2025/2026 academic year
• Nominee should be entering their last 2-3 years of study as of Fall 2025
• Nominee must not hold another industry-sponsored full fellowship while they are an Apple Scholar in AI/ML (Fall 2025 to Summer 2027)
Owing to the sponsor's restriction on the number of applications that may be submitted from Duke, anyone wishing to pursue nomination should submit the following materials as one PDF:
• CV and publication list
• A one-page abstract describing your innovative research, your record as thought leaders and collaborators in your fields, and your unique commitment to take risks and push the envelope in machine learning and AI.
Please submit internal materials through My Research Proposal. (Code: ILN) https://www.grantinterface.com/sl/HQShLh
Instructions for creating an account (if needed) and submitting your materials: https://ctsi.duke.edu/about-myresearchproposal
Machine Learning - CMU
Phd program in machine learning.
Carnegie Mellon University's doctoral program in Machine Learning is designed to train students to become tomorrow's leaders through a combination of interdisciplinary coursework, hands-on applications, and cutting-edge research. Graduates of the Ph.D. program in Machine Learning will be uniquely positioned to pioneer new developments in the field, and to be leaders in both industry and academia.
Understanding the most effective ways of using the vast amounts of data that are now being stored is a significant challenge to society, and therefore to science and technology, as it seeks to obtain a return on the huge investment that is being made in computerization and data collection. Advances in the development of automated techniques for data analysis and decision making requires interdisciplinary work in areas such as machine learning algorithms and foundations, statistics, complexity theory, optimization, data mining, etc.
The Ph.D. Program in Machine Learning is for students who are interested in research in Machine Learning. For questions and concerns, please contact us .
The PhD program is a full-time in-person committment and is not offered on-line or part-time.
PhD Requirements
Requirements for the phd in machine learning.
- Completion of required courses , (6 Core Courses + 1 Elective)
- Mastery of proficiencies in Teaching and Presentation skills.
- Successful defense of a Ph.D. thesis.
Teaching Ph.D. students are required to serve as Teaching Assistants for two semesters in Machine Learning courses (10-xxx), beginning in their second year. This fulfills their Teaching Skills requirement.
Conference Presentation Skills During their second or third year, Ph.D. students must give a talk at least 30 minutes long, and invite members of the Speaking Skills committee to attend and evaluate it.
Research It is expected that all Ph.D. students engage in active research from their first semester. Moreover, advisor selection occurs in the first month of entering the Ph.D. program, with the option to change at a later time. Roughly half of a student's time should be allocated to research and lab work, and half to courses until these are completed.
Master of Science in Machine Learning Research - along the way to your PhD Degree.
Other Requirements In addition, students must follow all university policies and procedures .
Rules for the MLD PhD Thesis Committee (applicable to all ML PhDs): The committee should be assembled by the student and their advisor, and approved by the PhD Program Director(s). It must include:
- At least one MLD Core Faculty member
- At least one additional MLD Core or Affiliated Faculty member
- At least one External Member, usually meaning external to CMU
- A total of at least four members, including the advisor who is the committee chair
Financial Support
Application Information
For applicants applying in Fall 2023 for a start date of August 2024 in the Machine Learning PhD program, GRE Scores are REQUIRED. The committee uses GRE scores to gauge quantitative skills, and to a lesser extent, also verbal skills.
Proof of English Language Proficiency If you will be studying on an F-1 or J-1 visa, and English is not a native language for you (native language…meaning spoken at home and from birth), we are required to formally evaluate your English proficiency. We require applicants who will be studying on an F-1 or J-1 visa, and for whom English is not a native language, to demonstrate English proficiency via one of these standardized tests: TOEFL (preferred), IELTS, or Duolingo. We discourage the use of the "TOEFL ITP Plus for China," since speaking is not scored. We do not issue waivers for non-native speakers of English. In particular, we do not issue waivers based on previous study at a U.S. high school, college, or university. We also do not issue waivers based on previous study at an English-language high school, college, or university outside of the United States. No amount of educational experience in English, regardless of which country it occurred in, will result in a test waiver.
Submit valid, recent scores: If as described above you are required to submit proof of English proficiency, your TOEFL, IELTS or Duolingo test scores will be considered valid as follows: If you have not received a bachelor’s degree in the U.S., you will need to submit an English proficiency score no older than two years. (scores from exams taken before Sept. 1, 2021, will not be accepted.) If you are currently working on or have received a bachelor's and/or a master's degree in the U.S., you may submit an expired test score up to five years old. (scores from exams taken before Sept. 1, 2018, will not be accepted.)
Graduate Online Application
- Early Application Deadline – November 29, 2023 (3:00 p.m. EST)
- Final Application Deadline - December 13, 2023 (3:00 p.m. EST)
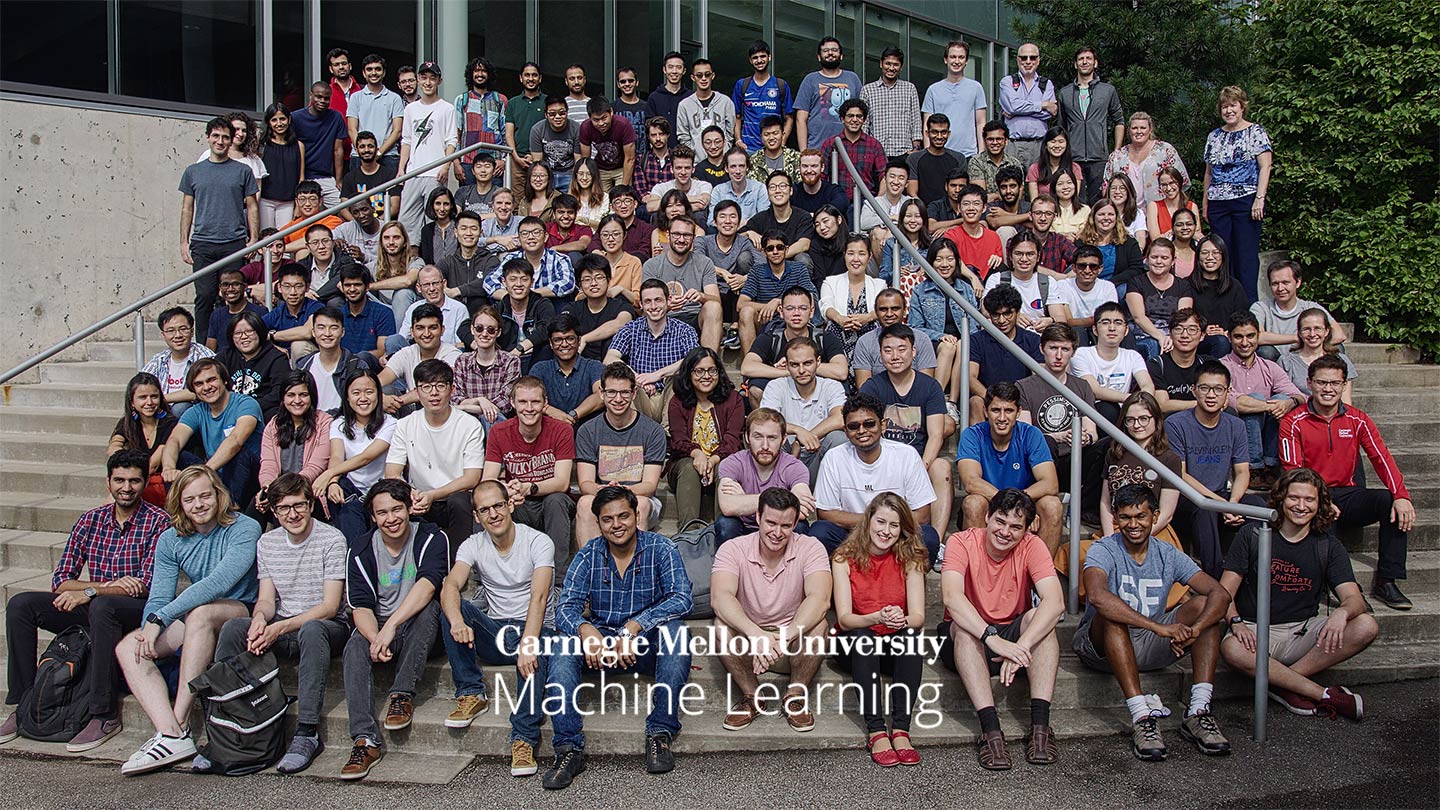
Computer Science & Engineering

DeepMind Fellowships
Computer science and engineering is proud to offer two deepmind fellowships for fall 2023 (two masters fellowships and one phd fellowship to be awarded for ay23-24).
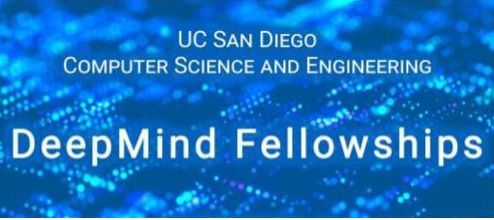
These fellowships were made possible by a recent generous gift from DeepMind , a London-based leader in artificial intelligence (AI) research and how it’s applied in the real world. UC San Diego is currently one of just three universities in the United States, and one of five in North America, selected to participate in this program.
Masters Fellowship
The Master's Fellowships are designed to support machine learning (ML) masters students. Students from cultural, racial, linguistic, geographic and socioeconomic backgrounds and genders who are underrepresented in ML graduate education are encouraged to apply. DeepMind Fellows receive two-year fellowships that cover tuition along with a stipend, a travel grant, funding to use towards housing, and access to a DeepMind mentor.
See current DeepMind Fellows here .
Who’s eligible: Admitted MS students specializing in Machine Learning who have demonstrated meaningful contributions to diversity and inclusion will be considered for DeepMind Fellowships.
How to apply: To be considered for a DeepMind Fellowship, applicants must (1) submit an MS application by the application deadline, (2) Complete the “ Additional Educational Experiences ” section of the application (highlighting how the applicant overcame barriers, and/or demonstrating a commitment to diversity) , and (3) Select AI/Machine Learning as an Interest Area.
The Fall 2023 MS Application Deadline is December 21, 2022. Fellowship awardees will be selected and contacted by March or early April.
DeepMind Fellowship Details (Masters):
- The award provides full tuition and fees (including nonresident supplemental tuition, if applicable) and funding to use towards housing for five quarters.
- Additional funding for living expenses, required books, equipment, and transportation costs recommended by the university
- A one-time travel grant of $2,250 to be used for relevant conference attendance
Contact [email protected]
PhD Fellowship
The Fall 2023 PhD Application Deadline is April 30, 2023. The fellowship awardee will be selected and contacted in Spring 2023.
This one-year fellowship is designed to support a machine learning (ML) PhD student. Students from cultural, racial, linguistic, geographic and socioeconomic backgrounds and genders who are underrepresented in ML graduate education are encouraged to apply. The DeepMind PhD Fellow will receive a one-year fellowships that covers partial tuition along with a travel grant and funding to use towards housing.
Who’s eligible: Admitted PhD students specializing in Machine Learning who have demonstrated meaningful contributions to diversity and inclusion will be considered.
How to apply: Please have your advisor submit your application packet here: https://forms.gle/wp6d99UJUnNnTtFh8 no later than April 30, 2023 . The application packet should include the following:
- A copy of your CV
- A 2-page statement of research that also addresses any ways your candidacy fulfills the EDI preference for this fellowship
- Two professional letters of recommendation
DeepMind Fellowship Details (PhD):
- The one-year fellowship provides funding towards tuition, fees and equipment, and funding to use towards housing.
Contact [email protected] or [email protected]

- Undergraduate Students
- Doctoral Students
- Master’s Students
- Engineering Master’s Students
- Faculty & Staff
- Parents & Families
- Asian / Pacific Islander
- Black/African American
- First Generation/Low Income
- Hispanic/Latinx
- International
- Native American/Indigenous
- Neurodiverse
- Student Athletes
- Students with Disabilities
- Undocumented
- What is a Career Community?
- Business, Finance & Consulting
- Data, Technology & Engineering
- Discovery & Exploration
- Education, Government, Nonprofit & Policy
- Energy, Environment & Sustainability
- Entertainment, Media & Arts
- Healthcare & Biomedical Sciences
- Innovation, Entrepreneurship & Design
- Know Yourself
- Explore Options
- Focus & Prepare
- Take Action
- Evaluate & Refine
- Featured Opportunities
- Career Readiness Resources
- Personalize Your Hub
- For Employers
- Share This: Share Apple Scholars in AIML PhD Fellowship on Facebook Share Apple Scholars in AIML PhD Fellowship on LinkedIn Share Apple Scholars in AIML PhD Fellowship on X

The Apple Scholars in AI/ML PhD fellowship program recognizes the contributions of emerging leaders in computer science and engineering at the graduate and postgraduate level. The PhD fellowship in AI/ML was created as part of the Apple Scholars program to support the work of outstanding PhD students from around the world, who are pursuing cutting edge research in machine learning and artificial intelligence.
Duke may nominate up to (3) students. Universities may nominate a maximum of (1) student to any of the research areas (see below).
Guidelines will be released in June. The password to access the guidelines will be available by request. Duke students interested in this opportunity can contact [email protected] .
- Duke Internal Deadline: July 8, 2024
- Deadline for Nominations: Sep. 10, 2024
Areas of Interest
Each university may nominate up to (3) students. Universities may nominate a maximum of (1) student to any of the following research areas:
- Privacy Preserving Machine Learning
- Human Centered AI
- AI for Ethics and Fairness
- AI for Accessibility
- AI for Health and Wellness
- ML Algorithms and Architectures
- Embodied ML
- Speech and Natural Language
- Computer Vision
- Information Retrieval and Knowledge
- Data-Centric AI
Eligibility Requirements
Nominees must meet the following criteria to be considered:
• Nominee must be enrolled full time at the nominating university at the start of Fall 2025, and expect to be enrolled through the end of the 2025/2026 academic year
• Nominee should be entering their last 2-3 years of study as of Fall 2025
• Nominee must not hold another industry-sponsored full fellowship while they are an Apple Scholar in AI/ML (Fall 2025 to Summer 2027)
Internal Nomination
Owing to the sponsor’s restriction on the number of applications that may be submitted from Duke, anyone wishing to pursue nomination should submit the following materials as one PDF:
• CV and publication list
• A one-page abstract describing your innovative research, your record as thought leaders and collaborators in your fields, and your unique commitment to take risks and push the envelope in machine learning and AI.
Please submit internal materials through My Research Proposal. (Code: ILN) https://www.grantinterface.com/sl/HQShLh
Instructions for creating an account (if needed) and submitting your materials: https://ctsi.duke.edu/about-myresearchproposal

Google PhD Fellowship recipients
Previous years:, algorithms, optimizations and markets.
Brice Huang, Massachusetts Institute of Technology
Debajyoti Kar, Indian Institute of Science
Jamie Tucker-Foltz, Harvard University
Joakim Blikstad, KTH Royal Institute of Technology
Mahdieh Labani, Macquarie University
Rehema Hamis Mwawado, University of Rwanda
Uddalok Sarkar, Indian Statistical Institute Kolkata
Computational Neural and Cognitive Sciences
Gizem Özdil, École polytechnique fédérale de Lausanne
Sreejan Kumar, Princeton University
Bridget Chak, University of Chicago
Li-Wen Chiu, National Yang Ming Chiao Tung University
Md. Saiful Islam, University of Rochester
Rutendo Jakachira, Brown University
Tsai-Min Chen, National Taiwan University
Wenhao Gao, Massachusetts Institute of Technology
Human Computer Interaction
Brianna Wimer, University of Notre Dame
Emily Kuang, Rochester Institute of Technology
Eunkyung Jo, University of California - Irvine
Georgianna Lin, University of Toronto
Gustavo Pacheco Santiago, Universidad Nacional Autónoma de México
Marcelo Marques da Rocha, Universidade Federal Fluminense
Yulia Goldenberg, Ben Gurion University
Zixiong Su, The University of Tokyo
Machine Learning
Berivan Isik, Stanford University
Blake Bordelon, Harvard University
Cristhian Delgado Fajardo, University of Otago
Denish Azamuke, Makerere University
Fuzhao Xue, National University of Singapore
Heinrich Pieter van Deventer, University of Pretoria
Imane Araf, Mohammed VI Polytechnic University
Itamar Franco Salazar Reque, Pontificia Universidad Católica del Perú
Jihoon Tack, Korea Advanced Institute of Science and Technology
Julliet Chepngeno Kirui, Strathmore University
Krystal Dacey, Charles Sturt University
Laura Smith, University of California - Berkeley
Marcos Paulo Silva Gôlo, Universidade de São Paulo
Melisa Yael Vinograd, Universidad de Buenos Aires
Miriam Rateike, Saarland University
Mitchell Wortsman, University of Washington
Natalia Gil Canto, Universidade Estadual de Campinas
Nicolás Esteban Valenzuela Figueroa, Universidad de Chile
Omprakash Chakraborty, Indian Institute of Technology Kharagpur
S. Durga, Indian Institute of Technology Bombay
Strato Angsoteng Bayitaa, C.K. Tedam University of Technology and Applied Sciences
Yiding Jiang, Carnegie Mellon University
Yifan Zhang, National University of Singapore
Machine Perception, Speech Technology and Computer Vision
Antoine Yang, National Institute for Research in Digital Science and Technology (Inria)
Astitva Srivastava, International Institute of Information Technology Hyderabad
Chen Yu, National University of Singapore
Ethan Tseng, Princeton University
Matheus Viana da Silva, Universidade Federal de São Carlos
Sunghwan Hong, Korea University
Sungyeon Kim, Pohang University of Science and Technology
Vincent Milimo Masilokwa Punabantu, University of Cape Town
Yanxi Li, The University of Sydney
Yosef Gandelsman, University of California - Berkeley
Ziqi Huang, Nanyang Technological University
Mobile Computing
Ke Sun, University of California - San Diego
Kyungjin Lee, Seoul National University
Natural Language Processing
Allahsera Auguste Tapo, Rochester Institute of Technology
Cheng-Han Chiang, National Taiwan University
Liunian Li, University of California - Los Angeles
Sarah Masud, Indraprastha Institute of Information Technology Delhi
Sumanth Doddapaneni, Indian Institute of Technology Madras
Zhiqing Sun, Carnegie Mellon University
Privacy and Security
Jiayuan Ye, National University of Singapore
Miranda Wei, University of Washington
Neha Jawalkar, Indian Institute of Science
Yihui Zeng, Arizona State University
Programming Technology and Software Engineering
Aaditya Naik, University of Pennsylvania
Thanh Le-Cong, The University of Melbourne
Quantum Computing
Diego Hernando Useche Reyes, Universidad Nacional de Colombia
Elies Gil-Fuster, Free University of Berlin
Juan David Nieto García, Universidade Estadual de Campinas
Lia Yeh, University of Oxford
Structured Data and Database Management
Zezhou Huang, Columbia University
Systems and Networking
Jennifer Switzer, University of California - San Diego
Jiaxin Lin, University of Texas at Austin
Jinhyung Koo, Daegu Gyeongbuk Institute of Science & Technology
Maurine Chepkoech, University of Cape Town
Qinghao Hu, Nanyang Technological University
Anjali Gupta, Indian Institute of Technology Delhi
Shunhua Jiang, Columbia University
Shyam Sivasathya Narayanan, Massachusetts Institute of Technology
Venkata Naga Sreenivasulu Karnati, Indian Institute of Science
Yang P. Liu, Stanford University
Aditi Jha, Princeton University
Klavdia Zemlianova, New York University
Devon Jarvis, University of the Witwatersrand
Emily Schwenger, Albert Einstein College of Medicine
Reihaneh Torkzadehmahani, TU Munich
Xin Liu, University of Washington
Qian Niu, Kyoto University
Karthik Mahadevan, University of Toronto
Meena Muralikumar, University of Washington
Nika Nour, University of California - Irvine
Pang Suwanaposee, University of Canterbury
Ryan Louie, Northwestern University
Tiffany Li, University of Illinois - Urbana-Champaign
Zhongyi Zhou, The University of Tokyo
Eunji Kim, Seoul National University
Hayeon Lee, Korea Advanced Institute of Science and Technology
Julius von Kügelgen, Max Planck Institute for Intelligent Systems
Kaloma Usman Majikumna, Euromed University of Fes, Morocco
Lily Xu, Harvard University
Maksym Andriushchenko, EPFL
Pierre Marion, Fondation Sciences Mathématiques de Paris
Shashank Rajput, University of Wisconsin - Madison
Sheheryar Zaidi, University of Oxford
Sindy Löwe, University of Amsterdam
Tan Wang, Nanyang Technological University
Xiaobo Xia, University of Sydney
Yixin Liu, Monash University
Efthymios Tzinis, University of Illinois - Urbana-Champaign
Elizabeth Ndunge Mutua, Strathmore University
Haipeng Xiong, National University of Singapore
Jianyuan Guo, University of Sydney
Jiawei Ren, Nanyang Technological University
Juhong Min, Pohang University of Science and Technology
Liliane Momeni, University of Oxford
Qianqian Wang, Cornell University
Shuo Yang, University of Technology Sydney
Tahir Javed, Indian Institute of Technology Madras
Wei-Ting Chen, National Taiwan University
Yuming Jiang. Nanyang Technological University
Yu-Ying Yeh, University of California - San Diego
Binbin Xie, University of Massachusetts - Amherst
Clara Isabel Meister, ETH Zurich
Julia Mendelsohn, University of Michigan
Sachin Kumar, Carnegie Mellon University
Saley Vishal Vivek, Indian Institute of Technology Delhi
Swarnadeep Saha, University of North Carolina - Chapel Hill
Shuyi Wang, The University of Queensland
Thong Nguyen, National University of Singapore
Ussen Kimanuka, Pan African University Institute For Basic Sciences, Technology and Innovation
Amy Elizabeth Gooden, University Kwazulu-Natal
Promise Ricardo Agbedanu, University of Rwanda
Alexander Bienstock, New York University
Daniel De Almeida Braga, Universite Rennes 1
Gaurang Bansal, National University of Singapore
Nicolas Huaman Groschopf, Leibniz University of Hanover
Simon Spies, Max Planck Institute for Software Systems
Ilkwon Byun, Seoul National University
Margaret Fortman, University of Wisconsin - Madison
Oscar Higgott, University College London
Sam Gunn, University of California - Berkeley
Recommender Systems
Jessie J. Smith, University of Colorado - Boulder
Wenjie Wang, National University of Singapore
Nikolaos Tziavelis, Northeastern University
Humphrey Owuor Otieno, University of Cape Town
Jiarong Xing, Rice University
Shweta Pandey, Indian Institute of Science
Sunil Kumar, Indraprastha Institute of Information Technology Delhi
Yang Zhou, Harvard University
Yujeong Choi, Korea Advanced Institute of Science and Technology
Daniel Mutembesa, Makerere University
Kevin Tian, Stanford University
Prerona Chatterjee, Tata Institute of Fundamental Research
Sampson Wong, The University of Sydney
Santhoshini Velusamy, Harvard University
Sruthi Gorantla, Indian Institute of Science
Wenshuo Guo, University of California, Berkeley
Malvern Madondo, Emory University
Steffen Schneider, University of Tübingen
Nalini Singh, Massachusetts Institute of Technology
Roman Koshkin, Okinawa Institute of Science and Technology
Vishwali Mhasawade, New York University
Anupriya Tuli, Indraprastha Institute of Information Technology - Delhi
Chia-Hsing Chiu, National Taiwan University of Science and Technology
Dennis Makafui Dogbey, University of Cape Town
George Hope Chidziwisano, Michigan State University
Harmanpreet Kaur, University of Michigan
Srishti Palani, University of California, San Diego
Amir-Hossein Karimi, Max Planck Institute for Intelligent Systems
Anastasia Koloskova, EPFL, Lausanne
Anirudh Goyal, University of Montreal
Daniel Kang, Stanford University
Elena Fillola, University of Bristol
Emmanuel Chinyere Echeonwu, Nnamdi Azikiwe University, Nigeria
Gal Yona, Weizmann Institute of Science
Hae Beom Lee, KAIST
Jaekyeom Kim, Seoul National University
Logan Engstrom, Massachusetts Institute of Technology
Piyushi Manupriya, Indian Institute of Technology - Hyderabad
Qinbin Li, National University of Singapore
Shen Li, National University of Singapore
Shubhada Agrawal, Tata Institute of Fundamental Research
Theekshana Dissanayake, Queensland University of Technology
Tianyuan Jin, National University of Singapore
Yun Li, The University of New South Wales
Andrea Burns, Boston University
Fangzhou Hong, Nanyang Technological University
Hai-Bin Wu, National Taiwan University
Jogendra Nath Kundu, Indian Institute of Science
Kelvin C.K. Chan, Nanyang Technological University
Sanghyun Woo, KAIST
Sara El-Ateif, National School For Computer Science (ENSIAS)
Soo Ye Kim, KAIST
Tewodros Amberbir Habtegebrial, Technical University of Kaiserslautern
Xinlong Wang, The University of Adelaide
Xueting Li, University of California, Merced
Zhiqin Chen, Simon Fraser University
Byungjin Jun, Northwestern University
Soundarya Ramesh, National University of Singapore
Derguene Mbaye, Universite Cheikh Anta Diop
Eya Hammami, LARODEC
Haoyue Shi, Toyota Technological Institute at Chicago
Kalpesh Krishna, University of Massachusetts Amherst
Peter Hase, University of North Carolina at Chapel Hill
Rochelle Choenni, University of Amsterdam
Chandan Kumar, Indian Institute of Technology - Kharagpur
Kevin Loughlin, University of Michigan
Teodora Baluta, National University of Singapore
Yuqing Zhu, University of California, Santa Barbara
Aishwarya Sivaraman, University of California, Los Angeles
Jenna Wise, Carnegie Mellon University
Alicja Dutkiewicz, Leiden University
Hsin-Yuan Huang, California Institute of Technology
Mykyta Onizhuk, The University of Chicago
Sayantan Chakraborty, Tata Institute of Fundamental Research
Brian Kundinger, Duke University
Yiru Chen, Columbia University
Yu Meng, University of Illinois at Urbana-Champaign
Zheng Wang, Nanyang Technological University
Aishwariya Chakraborty, Indian Institute of Technology - Kharagpur
Alireza Farshin, KTH Royal Institute of Technology
Erika Hunhoff, University of Colorado Boulder
S. VenkataKeerthy, Indian Institute of Technology - Hyderabad
Soroush Ghodrati, University of California, San Diego
Yejin Lee, Seoul National University
Jan van den Brand, KTH Royal Institute of Technology
Mahsa Derakhshan, University of Maryland, College Park
Sidhanth Mohanty, University of California, Berkeley
Computational Neuroscience
Connor Brennan, University of Pennsylvania
Abdelkareem Bedri, Carnegie Mellon University
Brendan David-John, University of Florida
Hiromu Yakura, University of Tsukuba
Manaswi Saha, University of Washington
Muratcan Cicek, University of California, Santa Cruz
Prashan Madumal, University of Melbourne
Alon Brutzkus, Tel Aviv University
Chin-Wei Huang, Universite de Montreal
Eli Sherman, Johns Hopkins University
Esther Rolf, University of California, Berkeley
Imke Mayer, Fondation Sciences Mathématique de Paris
Jean Michel Sarr, Cheikh Anta Diop University
Lei Bai, University of New South Wales
Nontawat Charoenphakdee, The University of Tokyo
Preetum Nakkiran, Harvard University
Sravanti Addepalli, Indian Institute of Science
Taesik Gong, Korea Advanced Institute of Science and Technology
Vihari Piratla, Indian Institute of Technology - Bombay
Vishakha Patil, Indian Institute of Science
Wilson Tsakane Mongwe, University of Johannesburg
Xinshi Chen, Georgia Institute of Technology
Yadan Luo, University of Queensland
Benjamin van Niekerk, University of Stellenbosch
Eric Heiden, University of Southern California
Gyeongsik Moon, Seoul National University
Hou-Ning Hu, National Tsing Hua University
Nan Wu, New York University
Shaoshuai Shi, The Chinese University of Hong Kong
Yifan Liu, University of Adelaide
Yu Wu, University of Technology Sydney
Zhengqi Li, Cornell University
Xiaofan Zhang, University of Illinois at Urbana-Champaign
Anjalie Field, Carnegie Mellon University
Mingda Chen, Toyota Technological Institute at Chicago
Shang-Yu Su, National Taiwan University
Yanai Elazar, Bar-Ilan
Julien Gamba, Universidad Carlos III de Madrid
Shuwen Deng, Yale University
Yunusa Simpa Abdulsalm, Mohammed VI Polytechnic University
Adriana Sejfia, University of Southern California
John Cyphert, University of Wisconsin-Madison
Amira Abbas, University of KwaZulu-Natal
Mozafari Ghoraba Fereshte, EPFL
Yanqing Peng, University of Utah
Huynh Nguyen Van, University of Technology Sydney
Michael Sammler, Saarland University, MPI-SWS
Sihang Liu, University of Virginia
Yun-Zhan Cai, National Cheng Kung University
Aidasadat Mousavifar, EPFL Ecole Polytechnique Fédérale de Lausanne
Peilin Zhong, Columbia University
Siddharth Bhandari, Tata Institute of Fundamental Research
Soheil Behnezhad, University of Maryland at College Park
Zhe Feng, Harvard University
Caroline Haimerl, New York University
Mai Gamal, German University in Cairo
Catalin Voss, Stanford university
Hua Hua, Australian National University
Zhanna Sarsenbayeva, University of Melbourne
Abdulsalam Ometere Latifat, African University of Science and Technology Abuja
Adji Bousso Dieng, Columbia University
Anshul Mittal, IIT Delhi
Blake Woodworth, Toyota Technological Institute at Chicago
Diana Cai, Princeton University
Francesco Locatello, ETH Zurich
Ihsane Gryech, International University Of Rabat, Morocco
Jaemin Yoo, Seoul National University
Maruan Al-Shedivat, Carnegie Mellon University
Ousseynou Mbaye, Alioune Diop University of Bambey
Rendani Mbuvha, University of Johannesburg
Shibani Santurkar, Massachusetts Institute of Technology
Takashi Ishida, University of Tokyo
Chenxi Liu, Johns Hopkins University
Kayode Kolawole Olaleye, Stellenbosch University
Ruohan Gao, The University of Texas at Austin
Tiancheng Sun, University of California San Diego
Xuanyi Dong, University of Technology Sydney
Yu Liu, Chinese University of Hong Kong
Zhi Tian, University of Adelaide
Naoki Kimura, University of Tokyo
Abigail See, Stanford University
Ananya Sai B, IIT Madras
Byeongchang Kim, Seoul National University
Daniel Patrick Fried, UC Berkeley
Hao Peng, University of Washington
Reinald Kim Amplayo, University of Edinburgh
Sungjoon Park, Korea Advanced Institute of Science and Technology
Ajith Suresh, Indian Institute of Science
Itsaka Rakotonirina, Inria Nancy
Milad Nasr, University of Massachusetts Amherst
Sarah Ann Scheffler, Boston University
Caroline Lemieux, UC Berkeley
Conrad Watt, University of Cambridge
Umang Mathur, University of Illinois at Urbana-Champaign
Amy Greene, Massachusetts Institute of Technology
Leonard Wossnig, University College London
Yuan Su, University of Maryland at College Park
Amir Gilad, Tel Aviv University
Nofar Carmeli, Technion
Zhuoyue Zhao, University of Utah
Chinmay Kulkarni, University of Utah
Nicolai Oswald, University of Edinburgh
Saksham Agarwal, Cornell University
Emmanouil Zampetakis, Massachusetts Institute of Technology
Manuela Fischer, ETH Zurich
Pranjal Dutta, Chennai Mathematical Institute
Thodoris Lykouris, Cornell University
Yuan Deng, Duke University
Ella Batty, Columbia University
Neha Spenta Wadia, University of California - Berkeley
Reuben Feinman, New York University
Human-Computer Interaction
Gierad Laput, Carnegie Mellon University
Mike Schaekermann, University of Waterloo
Minsuk (Brian) Kahng, Georgia Institute of Technology
Niels van Berkel, The University of Melbourne
Siqi Wu, Australian National University
Xiang Zhang, The University of New South Wales
Abhijeet Awasthi, Indian Institute of Technology - Bombay
Aditi Raghunathan, Stanford University
Futoshi Futami, University of Tokyo
Lin Chen, Yale University
Qian Yu, University of Southern California
Ravid Shwartz-Ziv, Hebrew University
Shuai Li, Chinese University of Hong Kong
Shuang Liu, University of California - San Diego
Stephen Tu, University of California - Berkeley
Steven James, University of the Witwatersrand
Xinchen Yan, University of Michigan
Zelda Mariet, Massachusetts Institute of Technology
Machine Perception, Speech Technology, and Computer Vision
Antoine Miech, INRIA
Arsha Nagrani, University of Oxford
Arulkumar S, Indian Institute of Technology - Madras
Joseph Redmon, University of Washington
Raymond Yeh, University of Illinois - Urbana-Champaign
Shanmukha Ramakrishna Vedantam, Georgia Institute of Technology
Lili Wei, Hong Kong University of Science & Technology
Rizanne Elbakly, Egypt-Japan University of Science and Technology
Shilin Zhu, University of California - San Diego
Anne Cocos, University of Pennsylvania
Hongwei Wang, Shanghai Jiao Tong University
Jonathan Herzig, Tel Aviv University
Rotem Dror, Technion - Israel Institute of Technology
Shikhar Vashishth, Indian Institute of Science - Bangalore
Yang Liu, University of Edinburgh
Yoon Kim, Harvard University
Zhehuai Chen, Shanghai Jiao Tong University
Imane khaouja, Université Internationale de Rabat
Aayush Jain, University of California - Los Angeles
Gowtham Kaki, Purdue University
Joseph Benedict Nyansiro, University of Dar es Salaam
Reyhaneh Jabbarvand, University of California - Irvine
Victor Lanvin, Fondation Sciences Mathématiques de Paris
Erika Ye, California Institute of Technology
Lingjiao Chen, University of Wisconsin - Madison
Andrea Lattuada, ETH Zurich
Chen Sun, Tsinghua University
Lana Josipovic, EPFL
Michael Schaarschmidt, University of Cambridge
Rachee Singh, University of Massachusetts - Amherst
Stephen Mallon, The University of Sydney
Chiu Wai Sam Wong, University of California, Berkeley
Eric Balkanski, Harvard University
Haifeng Xu, University of Southern California
Motahhare Eslami, University of Illinois, Urbana-Champaign
Sarah D'Angelo, Northwestern University
Sarah Mcroberts, University of Minnesota - Twin Cities
Sarah Webber, The University of Melbourne
Aude Genevay, Fondation Sciences Mathématiques de Paris
Dustin Tran, Columbia University
Jamie Hayes, University College London
Jin-Hwa Kim, Seoul National University
Ling Luo, The University of Sydney
Martin Arjovsky, New York University
Sayak Ray Chowdhury, Indian Institute of Science
Song Zuo, Tsinghua University
Taco Cohen, University of Amsterdam
Yuhuai Wu, University of Toronto
Yunhe Wang, Peking University
Yunye Gong, Cornell University
Avijit Dasgupta, International Institute of Information Technology - Hyderabad
Franziska Müller, Saarland University - Saarbrücken GSCS and Max Planck Institute for Informatics
George Trigeorgis, Imperial College London
Iro Armeni, Stanford University
Saining Xie, University of California, San Diego
Yu-Chuan Su, University of Texas, Austin
Sangeun Oh, Korea Advanced Institute of Science and Technology
Shuo Yang, Shanghai Jiao Tong University
Bidisha Samanta, Indian Institute of Technology Kharagpur
Ekaterina Vylomova, The University of Melbourne
Jianpeng Cheng, The University of Edinburgh
Kevin Clark, Stanford University
Meng Zhang, Tsinghua University
Preksha Nama, Indian Institute of Technology Madras
Tim Rocktaschel, University College London
Romain Gay, ENS - École Normale Supérieure
Xi He, Duke University
Yupeng Zhang, University of Maryland, College Park
Programming Languages, Algorithms and Software Engineering
Christoffer Quist Adamsen, Aarhus University
Muhammad Ali Gulzar, University of California, Los Angeles
Oded Padon, Tel-Aviv University
Amir Shaikhha, EPFL CS
Jingbo Shang, University of Illinois, Urbana-Champaign
Ahmed M. Said Mohamed Tawfik Issa, Georgia Institute of Technology
Khanh Nguyen, University of California, Irvine
Radhika Mittal, University of California, Berkeley
Ryan Beckett, Princeton University
Samaneh Movassaghi, Australian National University
Google Australia PhD Fellowships
Chitra Javali, Security, The University of New South Wales
Dana McKay, Human Computer Interaction, The University of Melbourne
Kwan Hui Lim, Machine Learning, The University of Melbourne
Weitao Xu, Machine Perception, The University of Queensland
Google East Asia PhD Fellowships
Chungkuk YOO, Mobile Computing, Korea Advanced Institute of Science and Technology
Hong ZHANG, Systems and Networking, The Hong Kong University of Science and Technology
Quanming YAO, Machine Learning, The Hong Kong University of Science and Technology
Tian TAN, Speech Technology, Shanghai Jiao Tong University
Woosang LIM, Machine Learning, Korea Advanced Institute of Science and Technology
Ying CHEN, Systems and Networking, Tsinghua University
Google India PhD Fellowships
Arpita Biswas, Algorithms, Indian Institute of Science
Aniruddha Singh Kushwaha, Networking, Indian Institute of Technology Bombay
Anirban Santara, Machine Learning, Indian Institute of Technology Kharagpur
Gurunath Reddy, Speech Technology, Indian Institute of Technology Kharagpur
Google North America, Europe and the Middle East PhD Fellowships
Cameron, Po-Hsuan Chen, Computational Neuroscience, Princeton University
Grace Lindsay, Computational Neuroscience, Columbia University
Martino Sorbaro Sindaci, Computational Neuroscience, The University of Edinburgh
Koki Nagano, Human-Computer Interaction, University of Southern California
Arvind Satyanarayan, Human-Computer Interaction, Stanford University
Amy Xian Zhang, Human-Computer Interaction, Massachusetts Institute of Technology
Olivier Bachem, Machine Learning, Swiss Federal Institute of Technology Zurich
Tianqi Chen, Machine Learning, University of Washington
Emily Denton, Machine Learning, New York University
Yves-Laurent Kom Samo, Machine Learning, University of Oxford
Daniel Jaymin Mankowitz, Machine Learning, Technion - Israel Institute of Technology
Lucas Maystre , Machine Learning, École Polytechnique Fédérale de Lausanne
Arvind Neelakantan, Machine Learning, University of Massachusetts, Amherst
Ludwig Schmidt, Machine Learning, Massachusetts Institute of Technology
Shandian Zhe, Machine Learning, Purdue University, West Lafayette
Eugen Beck, Machine Perception, RWTH Aachen University
Yu-Wei Chao, Machine Perception, University of Michigan, Ann Arbor
Wei Liu, Machine Perception, University of North Carolina at Chapel Hill
Aron Monszpart, Machine Perception, University College London
Thomas Schoeps, Machine Perception, Swiss Federal Institute of Technology Zurich
Chia-Yin Tsai, Machine Perception, Carnegie Mellon University
Hossein Esfandiari, Market Algorithms, University of Maryland, College Park
Sandy Heydrich, Market Algorithms, Saarland University - Saarbrucken GSCS
Rad Niazadeh, Market Algorithms, Cornell University
Sadra Yazdanbod, Market Algorithms, Georgia Institute of Technology
Lei Kang, Mobile Computing, University of Wisconsin
Tauhidur Rahman, Mobile Computing, Cornell University
Yuhao Zhu, Mobile Computing, University of Texas, Austin
Tamer Alkhouli, Natural Language Processing, RWTH Aachen University
Jose Camacho Collados, Natural Language Processing, Sapienza - Università di Roma
Kartik Nayak, Privacy and Security, University of Maryland, College Park
Nicolas Papernot, Privacy and Security, Pennsylvania State University
Damian Vizar, Privacy and Security, École Polytechnique Fédérale de Lausanne
Xi Wu, Privacy and Security, University of Wisconsin
Marcelo Sousa, Programming Languages and Software Engineering, University of Oxford
Xiang Ren, Structured Data and Database Management, University of Illinois, Urbana-Champaign
Andrew Crotty, Systems and Networking, Brown University
Ilias Marinos, Systems and Networking, University of Cambridge
Kay Ousterhout, Systems and Networking, University of California, Berkeley
Bahar Salehi, Natural Language Processing, University of Melbourne
Siqi Liu, Computational Neuroscience, University of Sydney
Qian Ge, Systems, University of New South Wales
Bo Xin, Artificial Intelligence, Peking University
Xingyu Zeng, Computer Vision, The Chinese University of Hong Kong
Suining He, Mobile Computing, The Hong Kong University of Science and Technology
Zhenzhe Zheng, Mobile Networking, Shanghai Jiao Tong University
Jinpeng Wang, Natural Language Processing, Peking University
Zijia Lin, Search and Information Retrieval, Tsinghua University
Shinae Woo, Networking and Distributed Systems, Korea Advanced Institute of Science and Technology
Jungdam Won, Robotics, Seoul National University
Palash Dey, Algorithms, Indian Institute of Science
Avisek Lahiri, Machine Perception, Indian Institute of Technology Kharagpur
Malavika Samak, Programming Languages and Software Engineering, Indian Institute of Science
Google Europe and the Middle East PhD Fellowships
Heike Adel, Natural Language Processing, University of Munich
Thang Bui, Speech Technology, University of Cambridge
Victoria Caparrós Cabezas, Distributed Systems, Swiss Federal Institute of Technology Zurich
Nadav Cohen, Machine Learning, The Hebrew University of Jerusalem
Josip Djolonga, Probabilistic Inference, Swiss Federal Institute of Technology Zurich
Jakob Julian Engel, Computer Vision, Technische Universität München
Nikola Gvozdiev, Computer Networking, University College London
Felix Hill, Language Understanding, University of Cambridge
Durk Kingma, Deep Learning, University of Amsterdam
Massimo Nicosia, Statistical Natural Language Processing, University of Trento
George Prekas, Operating Systems, École Polytechnique Fédérale de Lausanne
Roman Prutkin, Graph Algorithms, Karlsruhe Institute of Technology
Siva Reddy, Multilingual Semantic Parsing, The University of Edinburgh
Immanuel Trummer, Structured Data Analysis, École Polytechnique Fédérale de Lausanne
Margarita Vald, Security, Tel Aviv University
Google United States/Canada PhD Fellowships
Waleed Ammar, Natural Language Processing, Carnegie Mellon University
Justin Meza, Systems Reliability, Carnegie Mellon University
Nick Arnosti, Market Algorithms, Stanford University
Osbert Bastani, Programming Languages, Stanford University
Saurabh Gupta, Computer Vision, University of California, Berkeley
Masoud Moshref Javadi, Computer Networking, University of Southern California
Muhammad Naveed, Security, University of Illinois at Urbana-Champaign
Aaron Parks, Mobile Networking, University of Washington
Kyle Rector, Human Computer Interaction, University of Washington
Riley Spahn, Privacy, Columbia University
Yun Teng, Computer Graphics, University of California, Santa Barbara
Carl Vondrick, Machine Perception,, Massachusetts Institute of Technology
Xiaolan Wang, Structured Data, University of Massachusetts Amherst
Tan Zhang, Mobile Systems, University of Wisconsin-Madison
Wojciech Zaremba, Machine Learning, New York University
Guosheng Lin, Machine Perception, University of Adelaide
Kellie Webster, Natural Language Processing, University of Sydney
- Internal wiki
PhD Programme in Advanced Machine Learning
The Cambridge Machine Learning Group (MLG) runs a PhD programme in Advanced Machine Learning. The supervisors are Jose Miguel Hernandez-Lobato , Carl Rasmussen , Richard E. Turner , Adrian Weller , Hong Ge and David Krueger . Zoubin Ghahramani is currently on academic leave and not accepting new students at this time.
We encourage applications from outstanding candidates with academic backgrounds in Mathematics, Physics, Computer Science, Engineering and related fields, and a keen interest in doing basic research in machine learning and its scientific applications. There are no additional restrictions on the topic of the PhD, but for further information on our current research areas, please consult our webpages at http://mlg.eng.cam.ac.uk .
The typical duration of the PhD will be four years.
Applicants must formally apply through the Applicant Portal at the University of Cambridge by the deadline, indicating “PhD in Engineering” as the course (supervisor Hernandez-Lobato, Rasmussen, Turner, Weller, Ge and/or Krueger). Applicants who want to apply for University funding need to reply ‘Yes’ to the question ‘Apply for Cambridge Scholarships’. See http://www.admin.cam.ac.uk/students/gradadmissions/prospec/apply/deadlines.html for details. Note that applications will not be complete until all the required material has been uploaded (including reference letters), and we will not be able to see any applications until that happens.
Gates funding applicants (US or other overseas) need to fill out the dedicated Gates Cambridge Scholarships section later on the form which is sent on to the administrators of Gates funding.
Deadline for PhD Application: noon 5 December, 2023
Applications from outstanding individuals may be considered after this time, but applying later may adversely impact your chances for both admission and funding.
FURTHER INFORMATION ABOUT COMPLETING THE ADMISSIONS FORMS:
The Machine Learning Group is based in the Department of Engineering, not Computer Science.
We will assess your application on three criteria:
1 Academic performance (ensure evidence for strong academic achievement, e.g. position in year, awards, etc.) 2 references (clearly your references will need to be strong; they should also mention evidence of excellence as quotes will be drawn from them) 3 research (detail your research experience, especially that which relates to machine learning)
You will also need to put together a research proposal. We do not offer individual support for this. It is part of the application assessment, i.e. ascertaining whether you can write about a research area in a sensible way and pose interesting questions. It is not a commitment to what you will work on during your PhD. Most often PhD topics crystallise over the first year. The research proposal should be about 2 pages long and can be attached to your application (you can indicate that your proposal is attached in the 1500 character count Research Summary box). This aspect of the application does not carry a huge amount of weight so do not spend a large amount of time on it. Please also attach a recent CV to your application too.
INFORMATION ABOUT THE CAMBRIDGE-TUEBINGEN PROGRAMME:
We also offer a small number of PhDs on the Cambridge-Tuebingen programme. This stream is for specific candidates whose research interests are well-matched to both the machine learning group in Cambridge and the MPI for Intelligent Systems in Tuebingen. For more information about the Cambridge-Tuebingen programme and how to apply see here . IMPORTANT: remember to download your application form before you submit so that you can send a copy to the administrators in Tuebingen directly . Note that the application deadline for the Cambridge-Tuebingen programme is noon, 5th December, 2023, CET.
What background do I need?
An ideal background is a top undergraduate or Masters degree in Mathematics, Physics, Computer Science, or Electrical Engineering. You should be both very strong mathematically and have an intuitive and practical grasp of computation. Successful applicants often have research experience in statistical machine learning. Shortlisted applicants are interviewed.
Do you have funding?
There are a number of funding sources at Cambridge University for PhD students, including for international students. All our students receive partial or full funding for the full three years of the PhD. We do not give preference to “self-funded” students. To be eligible for funding it is important to apply early (see https://www.graduate.study.cam.ac.uk/finance/funding – current deadlines are 10 October for US students, and 1 December for others). Also make sure you tick the box on the application saying you wish to be considered for funding!
If you are applying to the Cambridge-Tuebingen programme, note that this source of funding will not be listed as one of the official funding sources, but if you apply to this programme, please tick the other possible sources of funding if you want to maximise your chances of getting funding from Cambridge.
What is my likelihood of being admitted?
Because we receive so many applications, unfortunately we can’t admit many excellent candidates, even some who have funding. Successful applicants tend to be among the very top students at their institution, have very strong mathematics backgrounds, and references, and have some research experience in statistical machine learning.
Do I have to contact one of the faculty members first or can I apply formally directly?
It is not necessary, but if you have doubts about whether your background is suitable for the programme, or if you have questions about the group, you are welcome to contact one of the faculty members directly. Due to their high email volume you may not receive an immediate response but they will endeavour to get back to you as quickly as possible. It is important to make your official application to Graduate Admissions at Cambridge before the funding deadlines, even if you don’t hear back from us; otherwise we may not be able to consider you.
Do you take Masters students, or part-time PhD students?
We generally don’t admit students for a part-time PhD. We also don’t usually admit students just for a pure-research Masters in machine learning , except for specific programs such as the Churchill and Marshall scholarships. However, please do note that we run a one-year taught Master’s Programme: The MPhil in Machine Learning, and Machine Intelligence . You are welcome to apply directly to this.
What Department / course should I indicate on my application form?
This machine learning group is in the Department of Engineering. The degree you would be applying for is a PhD in Engineering (not Computer Science or Statistics).
How long does a PhD take?
A typical PhD from our group takes 3-4 years. The first year requires students to pass some courses and submit a first-year research report. Students must submit their PhD before the 4th year.
What research topics do you have projects on?
We don’t generally pre-specify projects for students. We prefer to find a research area that suits the student. For a sample of our research, you can check group members’ personal pages or our research publications page.
What are the career prospects for PhD students from your group?
Students and postdocs from the group have moved on to excellent positions both in academia and industry. Have a look at our list of recent alumni on the Machine Learning group webpage . Research expertise in machine learning is in very high demand these days.
187 phd-machine-learning-fully-funded PhD scholarships
Filtered by.
- Scholarship
- phd-machine-learning-fully-funded
Refine Your Search
- Last-24-hours 1
- Last-3-days 6
- Last-7-days 6
- Last-30-days 38
- United Kingdom 81
- Netherlands 33
- Switzerland 10
- Luxembourg 8
- Australia 3
- Swansea University 11
- University of Nottingham 11
- ; Swansea University 8
- Technical University of Denmark 8
- University of Luxembourg 8
- University of Groningen 7
- ; University of East Anglia 6
- Ghent University 6
- ; University of Southampton 5
- University of Basel 5
- Amsterdam UMC 4
- Cranfield University 4
- Wageningen University & Research 4
- ; University of Nottingham 3
- ETH Zurich 3
- Eindhoven University of Technology (TU/e) 3
- University of Galway 3
- Wageningen University and Research Center 3
- ; The University of Edinburgh 2
- Chalmers University of Technology 2
- Eindhoven University of Technology 2
- University of Nicosia Medical School 2
- ; Cranfield University 1
- ; Durham University 1
- ; Leeds Beckett University 1
- ; Northeastern University London 1
- ; The University of Manchester 1
- ; University of Birmingham 1
- ; University of Dundee 1
- ; University of Exeter 1
- ; University of Hertfordshire 1
- ; University of Plymouth 1
- ; University of Sheffield 1
- ; University of Warwick 1
- Abertay University 1
- AcademicTransfer 1
- Berliner Hochschule für Technik 1
- Centre for Functional Ecology - Science for the People & the Planet 1
- Curtin University 1
- Deakin University 1
- Delft University of Technology 1
- Delft University of Technology (TU Delft) 1
- ETH Zürich 1
- Erasmus University Rotterdam 1
- Fondation Artanim 1
- Fraunhofer-Gesellschaft 1
- Harper Adams University 1
- IFP Energies nouvelles (IFPEN) 1
- INSA Rennes 1
- J. Heyrovský Institute of Physical Chemistry of the CAS, v. v. i. 1
- KTH Royal Institute of Technology 1
- KU Leuven 1
- Kristiania University College 1
- Leiden University 1
- Maastricht University (UM) 1
- Max Planck Institute for Biological Intelligence • 1
- Max Planck Institute for the Study of Societies • 1
- NTNU - Norwegian University of Science and Technology 1
- NTNU Norwegian University of Science and Technology 1
- RPTU University of Kaiserslautern-Landau • 1
- Radboud University Medical Center (Radboudumc) 1
- SciLifeLab 1
- Tallinn University of Technology 1
- Technical University of Munich 1
- Technische Universität Berlin • 1
- The University of Manchester 1
- The University of Newcastle 1
- Trinity College Dublin 1
- University of Agder 1
- University of Agder (UiA) 1
- University of Amsterdam 1
- University of Antwerp 1
- University of Iceland 1
- University of Oslo 1
- University of Sheffield 1
- University of Southern Denmark 1
- University of Strasbourg - ICUBE Laboratory (UMR7357) 1
- University of Twente (UT) 1
- University of Tübingen 1
- Vrije Universiteit Amsterdam (VU) 1
- Computer Science 120
- Engineering 10
- Mathematics 10
- Medical Sciences 9
- Economics 7
- Chemistry 6
- Environment 3
- Linguistics 3
- Materials Science 2
- Arts and Literature 1
- Earth Sciences 1
- Electrical Engineering 1
- Psychology 1
Fully Funded Swansea University PhD Scholarship: Enhancing Cardiomyocyte Dynamic Network Analysis with Machine Learning (ECIDNA-ML)
Funding providers: Swansea University's Faculty of Science and Engineering Subject areas: Computer Science ( Machine Learning /Pattern Recognition applied to molecular cardiology) Project start date
Computer Science Fully Funded Swansea University PhD Scholarship: Enhancing Cardiomyocyte Dynamic Network Analysis with Machine Learning (Ecidna-ML)
? Not funded by an EU programme Is the Job related to staff position within a Research Infrastructure? No Offer Description Computer Science Fully Funded Swansea University PhD Scholarship: Enhancing
PhD Studentship: Fully Funded PhD Scholarship: Forecasting Future Environmental Impacts of Photovoltaic (PV) Manufacture Using Machine Learning Techniques
to leverage innovative machine learning (ML) techniques adept at handling sparse information. The impact of data pre-processing techniques will be assessed with the aim of improving the accuracy of predictions
Science and Engineering Fully Funded PhD Scholarship: Forecasting Future Environmental Impacts of Photovoltaic (PV) Manufacture Using Machine Learning Techniques
Research Framework Programme? Not funded by an EU programme Is the Job related to staff position within a Research Infrastructure? No Offer Description Science and Engineering: Fully Funded PhD Scholarship
Fully Funded PhD Scholarship. Networks and Data Science for Advancing Healthcare Analytics: Machine Learning Applications to Model Health Outcomes in Social Care Settings
part of a major investment, Northeastern University London (NU London) has multiple, fully - funded PhD studentships available to accelerate its interdisciplinary research in the humanities, social
Civil Engineering: Fully Funded EPSRC and Swansea PhD Scholarship: Physics Informed Machine Learning in High-speed Railway infrastructure safety analysis
the development of machine learning , data-driven method has become a powerful tool for civil engineers. Especially, Physics Informed Machine Learning (PIMM) can be used as a comprehensive analysis considering both
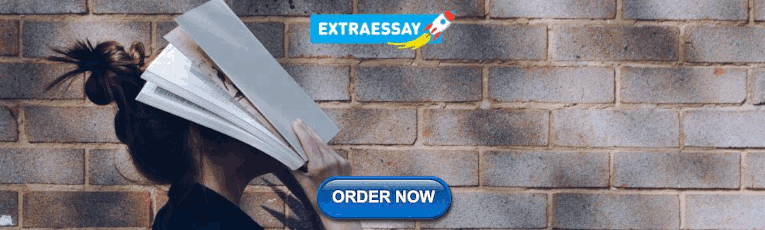
Fully Funded EPSRC and Swansea PhD Scholarship: Physics Informed Machine Learning in High-speed Railway Infrastructure Safety Analysis
learning , data-driven method has become a powerful tool for civil engineers. Especially, Physics Informed Machine Learning (PIMM) can be used as a comprehensive analysis considering both physics-based
PhD Studentship: Big Data and Artificial Intelligence for Urban Mobility
We are excited to offer a fully funded PhD position at the intersection of big data, artificial intelligence, and urban mobility. The rapid development of big data analytics, along with advances in
The Universities for Nottingham and the Centre for Sustainable Chemistry - PhD Scholarships available
the areas of: Sustainable/resilient chemical supply chains Artificial Intelligence / Machine Learning for the prediction of bulk properties in performance molecules and materials Synthetic electrochemical
PhD Studentship: Enhancing Adaptive Control System Performance Using Advanced Machine Learning Techniques
Overview Qualification type: PhD Subject area: Control and Machine Learning Location/Campus: College Lane, Hatfield Closing application date: 10 June 2024 Start date: July 2024 or as soon as
Searches related to phd machine learning fully funded
- machine learning phd
- machine learning
- computer science
- phd machine learning
- data science
- artificial intelligence phd
- computer vision
- data analytics phd
- big data phd
Unlocking Intelligence
We seek to understand the basis of intelligence in natural and artificial systems.
Deeper Learning
Our research blog highlights the newest research, findings and code from members of the Kempner community.
Our Community
We seek to build a community of scholars who work across boundaries and fields and are poised to solve some of the most compelling mysteries of our time. Coming together to learn, collaborate, and discover, Kempner community members are scientists who seek to fundamentally and radically advance our understanding of natural and artificial intelligence.
Our Mission
The Kempner's core mission is to innovate and advance the field of intelligence research and devise solutions to complex problems for the benefit of humanity.
Upcoming Events
Constructing spiking networks as a foundation for the theory of manifolds as computational substrate, transmission versus truth: ai models as cultural technologies and epistemic agents, recent news, a space for researchers to meet, and ai and natural intelligence to do the same, unlocking intelligence: the kempner institute.
Harvard Machine Learning Foundations Group
We are a research group focused on some of the foundational questions in modern machine learning. We are interested in both experimental and theoretical approaches that advance our understanding. Our group contains ML practitioners, theoretical computer scientists, statisticians, and neuroscientists, all sharing the goal of placing machine and natural learning on firmer foundations, and elucidating their fundamental capabilities and limitations.
Our group organizes the Kempner Seminar Series - a research seminar on the foundations of both natural and artificial learning. See mailing list , Google calendar , and list of talks .
Opportunities: We are looking for graduate students and postdocs . See opportunities section below. Announcements on positions will also be posted on social media .
Researchers
Gustaf ahdritz, phd student, alex atanasov, blake bordelon, chi-ning chou, ben edelman, lucas janson, samy jelassi, postdoctoral fellow, sham kakade, anat kleiman, depen morwani, costin-andrei oncescu, cengiz pehlevan, aayush karan, david brandfonbrener, yonadav shavit, nikhil vyas, sara kangaslahti, roy rinberg, natalie abreu, clara mohri, hanlin zhang, jonathan geuter, david alvarez melis, masters student, jacob zavatone-veth, runyu (cathy) zhang, finale doshi-velez, hima lakkaraju, michael mitzenmacher, morgane austern, preetum nakkiran, dimitris kalimeris, yamini bansal, sharon qian, tristan yang, undergraduate, recent publications.
By our group and its members.
(This list is not comprehensive. Also, we’re sometimes slow in updates—see individual homepages and the arXiv for the latest publications.)
Hidden Progress in Deep Learning: SGD Learns Parities Near the Computational Limit
NeurIPS 2022
Contrasting random and learned features in deep Bayesian linear regression
Manuscript 2022
Deconstructing Distributions: A Pointwise Framework of Learning
Depth induces scale-averaging in overparameterized linear bayesian neural networks.
55th Asilomar Conference 2021
Neural Networks as Kernel Learners: The Silent Alignment Effect
Inductive biases and variable creation in self-attention mechanisms, capacity of group-invariant linear readouts from equivariant representations: how many objects can be linearly classified under all possible views, revisiting model stitching to compare neural representations.
NeurIPS 2021
Learning Curves for SGD on Structured Features
Out-of-distribution generalization in kernel regression, asymptotics of representation learning in finite bayesian neural networks, for self-supervised learning, rationality implies generalization, provably, the deep bootstrap: good online learners are good offline generalizers, distributional generalization: a new kind of generalization.
Manuscript 2020.
Learning From Strategic Agents: Accuracy, Improvement, and Causality
Deep double descent: where bigger models and more data hurt, sgd on neural networks learns functions of increasing complexity.
NeurIPS 2019 spotlight talk (top 15% of accepted papers) .
More Data Can Hurt for Linear Regression: Sample-wise Double Descent
Manuscript. 2019.
Computational Limitations in Robust Classification and Win-Win Results
Minnorm training: an algorithm for training over-parameterized deep neural networks, adversarial robustness may be at odds with simplicity, on the information bottleneck theory of deep learning, recent & upcoming talks, brian depasquale, kim stachenfeld - predictive models for representation learning and simulation, stefano ermon - score entropy discrete diffusion models, andrea montanari - solving overparametrized systems of nonlinear equations, tom griffiths - using the tools of cognitive science to understand the behavior of large language models, larry abbott - modeling the navigational circuitry of the fly, rajesh rao - active predictive coding: a sensory-motor theory of the neocortex and a unifying framework for ai, noam brown - cicero: human-level performance in the game of diplomacy by combining language models with strategic reasoning, carsen stringer - unsupervised pretraining in biological neural networks, emmanuel abbe - logic reasoning and generalization on the unseen, denny zhou - teach language models to reason, tom goldstein - dataset security issues in generative ai, yann lecun - towards machines that can learn, reason, and plan., timothy lillicrap - model-based reinforcement learning and the future of language models, yejin choi - common sense: the dark matter of language and intelligence, seminar calendar.
Below is the calendar of events in the Kempner ML Foundations seminar. Join the mailing list for talk announcements.
Opportunities
We are looking for undergraduate researchers , graduate students and postdocs in the ML foundations group.
For undergraduate students , we are only able to work with students at Harvard or MIT (with preference to the former). If you are a Harvard or MIT student interested in collaborating, informally or formally, with us, please fill out the following google form . Students might also be interested in taking Boaz’s Spring 2023 seminar on the foundations of deep learning .
For graduate students we have openings in Computer Science , Electrical Engineering , applied mathematics or statistics degrees. New: Kempner Institute Graduate Fellowship: See more details here
If you are applying for graduate studies in CS and are interested in machine learning foundations, please mark both “Machine Learning” and “Theory of Computation” as areas of interest. Please also list the names of faculty you want to work with on your application. ML foundations group faculty include Demba Ba (Electrical Engineering and Bioengineering), David Alvarez-Melis , Boaz Barak , Sitan Chen , Jonathan Frankle , Sham Kakade (Computer Science), Cengiz Pehlevan (Applied Mathematics), and Lucas Janson (Statistics). There are also ML foundations affiliated faculty in all of the above departments and more. All of us are also open to the possibilities of co-advising students, including across different departments and schools.
Postdoc opportunities for 2024-2025 Academic year:
There are a number of opportunities at Harvard for postdoc positions. Applying to multiple positions is not just allowed but encouraged, and we urge you to apply to any of those that are of interest to you.
Kempner Institute Fellows - a three-year prestigious position for postdocs in AI/ML/related areas interested in “fundamentally advancing our understanding of natural and artificial intelligence.” Apply by October 9 2023
Computer science postdocs Postdocs in the ML foundations, Rabin Fellowship, Privacy Tools, Theory of Society. Coming soon.
Postdoctoral positions at Harvard Data Science initiative
The George F. Carrier Postdoctoral Fellowship in Applied Mathematics.
Postdoctoral fellow in theoretical and computational neuroscience at the Swartz Program
Center for Research on Computation and Society (CRCS) postdoc position
Postdoc positions at the Materials Intelligence Group
Follow us on social media for announcements of more opportunities.
PhD-positions
Part of the International Alliance of Research Universities (IARU), and among Europe’s top-ranking universities, the University of Copenhagen promotes research and teaching of the highest international standard. Rich in tradition and modern in outlook, the University gives students and staff the opportunity to cultivate their talent in an ambitious and informal environment. An effective organisation – with good working conditions and a collaborative work culture – creates the ideal framework for a successful academic career.
Search all vacancies
- Postdoc India
- Postdoc Abroad
- Postdoc (SS)
- RESEARCHERSJOB
- Post a position
- JRF/SRF/Project
- Science News
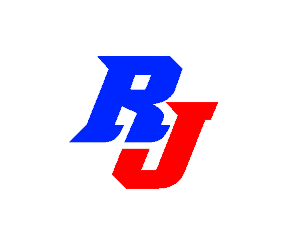
PhD Scholarship: Machine Learning at Luleå University, Sweden
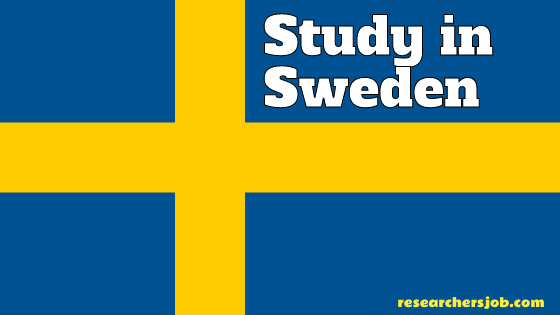
PhD Scholarship: Machine Learning: Luleå University of Technology invites applications for a PhD scholarship in Machine Learning, based in Luleå, Sweden. This opportunity is part of the university’s commitment to cutting-edge research and its contribution to the development of a sustainable society. The successful candidate will join the Machine Learning Research Laboratory, contributing to a project focused on document analysis using machine learning techniques.
PhD Scholarship Opportunity: Machine Learning Research at Luleå University
Summary Table:
Study Area: Machine Learning
Location: Luleå, Sweden
Eligibility/Qualification: We seek a highly motivated individual with a Master’s degree in computer science or a related field. Applicants should possess experience in machine learning, Deep Learning, and statistics. Proficiency in English, both spoken and written, is essential. The successful candidate should demonstrate the ability to work independently as well as in collaborative research environments.
Description: The selected PhD student will become part of a project focused on the application of machine learning methods for document analysis. This involves working with historical and modern documents, ranging from blueprints to circuit diagrams, and employing innovative techniques to enhance document recognition. Collaboration with regional and national archives in Sweden will enrich the research experience, providing opportunities for interdisciplinary engagement.
Duties: As a PhD student, responsibilities include conducting experimental and theoretical research, presenting findings at national and international conferences, and publishing in scientific journals. While the primary focus is on research, there may be opportunities for involvement in teaching activities.
How to Apply: Interested candidates are encouraged to apply using the provided application button. Applications must include a CV, a personal letter outlining research interests and motivations, and copies of verified diplomas from previous educational institutions. All materials must be submitted in English or Swedish and marked with the reference number: 2011-2024.
Last Date to Apply: August 30, 2024
For further inquiries, please contact Professor Elisa Barney at [email protected] or by phone at +46 920 49 2194.
Union representatives: SACO-S Kjell Johansson (+46)920-49 1529 [email protected] OFR-S Lars Frisk, (+46)920-49 1792 [email protected]
In case of discrepancies between the English and Swedish versions of this announcement, the Swedish version prevails.
RELATED ARTICLES MORE FROM AUTHOR
Phd position in chemistry: umeå university, sweden, phd positions commencing 2024/2025, la trobe university, melbourne, australia, fully-funded phd and temporary student: university of florida, usa, doctoral scholarship: machine learning, kth, sweden, fully funded phd position: single-cell transcriptomics, czech republic, phd student or postdoc: biomolecules, max planck institute for multidisciplinary sciences, göttingen, germany, leave a reply cancel reply.
Save my name, email, and website in this browser for the next time I comment.
Follow us on Instagram @researchersjob_rj
- Terms Of Service
- Privacy Policy
Postdoctoral Associate in DNA Damage, University of Oxford, UK

Apply Now: IRSA Postdoctoral Fellowship
The School of Statistics and the Institute for Research in Statistics and Its Applications (IRSA) at the University of Minnesota invites applications for the IRSA Faragher Distinguished Postdoctoral Fellowship . This endowed fellowship seeks to bring exceptional scientists of outstanding potential to the university. This two year fellowship, with no teaching or service obligations, is to begin on August 28, 2023. The Fellow will hold the rank of postdoctoral research scientist. A competitive annual salary will be supplemented with generous funding for relocation, conference travel, and research support.
Applications in all areas of statistics or related data sciences will be considered: the primary selection criterion will be the candidates’ exceptional promise to produce high quality and visible research. Fellows will be expected to pursue a vigorous research agenda and to participate actively in the intellectual life of the school.
The school, like the university itself, is an extraordinarily vibrant academic community. We are especially interested in candidates who will contribute to the diversity and excellence of the academic community. Women and minorities are especially encouraged to apply.
Applications must be submitted online. See links below for full details. You may also visit the University of Minnesota Office of Human Resources site and search for Job ID 351577.
Internal Candidates
See full job description for internal candidates .
External Candidates
See full job description for external candidates .
The University of Minnesota shall provide equal access to and opportunity in its programs, facilities, and employment without regard to race, color, creed, religion, national origin, gender, age, marital status, disability, public assistance status, veteran status, sexual orientation, gender identity, or gender expression.
Related News

Combining Academic and Industry Research Modes to Advance Machine Learning
Associate Professor Jie Ding partners U of M academics and Cisco industry professionals in a productive and evolving exploration of machine learning.
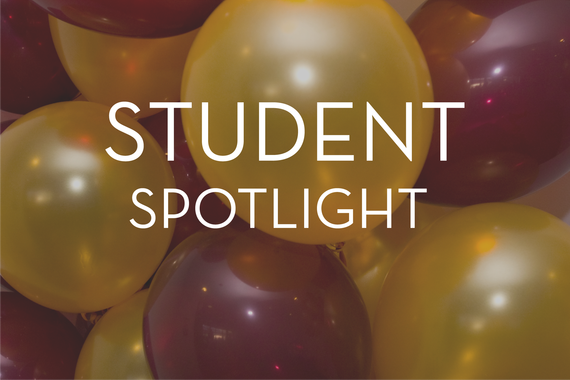
Spotlight on Recent PhD Graduates
See what some of our most recent PhD graduates are up to.
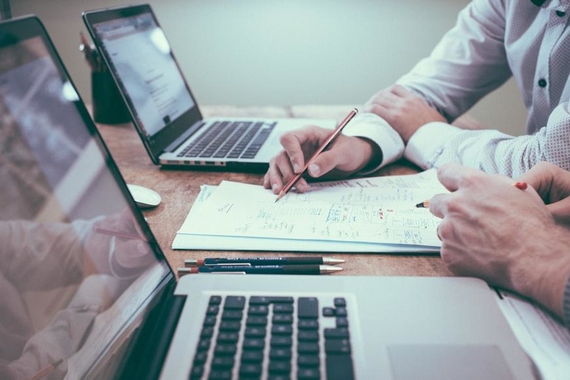
Statistics Support for University Researchers
IRSA is accepting applications for its 2024 Summer Statistical Consulting program
More Statistics News
PhD Proposal: Speeding up Density Functional Theory with Machine Learning
IRB IRB-4105
The Kohn-Sham (KS) equations are a nonlinear eigenvalue problem of the form $H[\rho]\Psi = E\Psi$, where $H$ is a real symmetric matrix called the \textit{Hamiltonian}, $\Psi$ is an eigenvector called the \textit{wave function}, $E$ is an eigenvalue called the \textit{energy}, and $\rho(\mathbf{r}) = \sum_i |\Psi_i(\mathbf{r})|^2$ is an real-valued field called the \textit{charge density}, which is unknown a priori. The KS equations are nonlinear in the sense that the matrix $H$ depends on the charge density $\rho$, which in turn depends on the eigenvectors $\Psi$ of $H$. Typically it is solved by fixed-point iteration, where an initial guess for $\rho$ is made and then a sequence of \textit{linear} eigenvalue problems are solved until convergence. The cost of the iteration is dominated by the eigenvalue problem. Consequently methods of reducing the number of requisite iterations are of great interest.
In this thesis, we investigate the use of machine-learning models for solving the KS equations. Our strategy is to develop machine-learning models which approximate numerical quantities in existing algorithms for electronic structure. In particular we use equivariant graph-neural-networks to predict the Kohn-Sham charge density. We show such methods obtain an average savings of $13\%$ in the number of iterations needed to reach convergence. Our methods are general, but we focus on learning data from catalysis, an application with potentially large environmental impact.
PhD Position Multivariate Dependence Modelling and Statistical Machine Learning Algorithms for Patient Risk Profiling

Job Information
Offer description.
The Applied Probability research group within the Delft Institute of Applied Mathematics at TU Delft (see https://www.tudelft.nl/ewi/over-de-faculteit/afdelingen/applied-mathema… ) is offering a full-time PhD position in the area of Multivariate dependence modelling and statistical machine learning algorithms for patient risk profiling.
In partnership with RECENTRE (see https://www.4tu.nl/recentre/ ), a project dedicated to helping people take charge of their health, we're working on mathematical approaches to quantify health-related risks. As a PhD researcher, you'll be at the forefront of this exciting journey, focusing on multivariate dependence models and statistical machine learning algorithms at its core to create personalized individual risk profiles. Along with developing novel methods and investigating their theoretical properties, you will also apply your methods and validate them using the latest available datasets.
The project will be co-supervised by Özge Şahin and Tina Nane in Applied Probability from TU Delft and Annemieke Witteveen in Biomedical Signals and Systems from the University of Twente.
Besides carrying out mathematical research and working on scientific articles, you will be expected to actively participate in seminars and discussions with colleagues from the department, as well as to present the findings at (inter)national conferences or workshops. Furthermore, you are supposed to carry out minor teaching tasks (at most 15% of the contract time). Teaching in English will be possible. Communication will be in English. If desired, there is the opportunity to attend Dutch language courses offered by the Language Center for Languages and Academic Skills at TU Delft, see https://www.tudelft.nl/en/tpm/itav/education/dutch-courses .
Requirements
- Curious Learner: You're eager to learn and explore, holding a Master's degree (MSc) in Statistics, Applied Mathematics, or a related field. You're passionate about using statistical, probabilistic, and mathematical principles to solve real-world problems in healthcare. While prior experience with copula methods is not required, it is a plus. A willingness to learn and apply new techniques in healthcare research is highly valued
- Analytical Thinker: With a strong background in statistics, probability theory, and mathematics, you excel at analyzing complex datasets to derive meaningful insights.
- Tech-Savvy: You're comfortable navigating through datasets, especially in healthcare, using your technical skills. You are proficient in programming languages in R or Python.
- Collaborative Team Player: You engage with stakeholders across academia, healthcare, and industry, fostering inclusive partnerships to drive innovation in healthcare.
Female candidates are strongly encouraged to apply for this position.
Doing a PhD at TU Delft requires English proficiency at a certain level to ensure that the candidate is able to communicate and interact well, participate in English-taught Doctoral Education courses, and write scientific articles and a final thesis. For more details please check the Graduate Schools Admission Requirements .
Additional Information
Doctoral candidates will be offered a 4-year period of employment in principle, but in the form of 2 employment contracts. An initial 1,5 year contract with an official go/no go progress assessment within 15 months. Followed by an additional contract for the remaining 2,5 years assuming everything goes well and performance requirements are met.
Salary and benefits are in accordance with the Collective Labour Agreement for Dutch Universities, increasing from € 2770 per month in the first year to € 3539 in the fourth year. As a PhD candidate you will be enrolled in the TU Delft Graduate School. The TU Delft Graduate School provides an inspiring research environment with an excellent team of supervisors, academic staff and a mentor. The Doctoral Education Programme is aimed at developing your transferable, discipline-related and research skills.
The TU Delft offers a customisable compensation package, discounts on health insurance, and a monthly work costs contribution. Flexible work schedules can be arranged.
For international applicants, TU Delft has the Coming to Delft Service . This service provides information for new international employees to help you prepare the relocation and to settle in the Netherlands. The Coming to Delft Service offers a Dual Career Programme for partners and they organise events to expand your (social) network.
Interested in this opportunity? To apply, click the application button and submit the following documents:
- Cover letter outlining your background and the motivation to pursue the PhD project (max. 1 page).
- A comprehesive Curriculum Vitae.
- Contact information of at least one academic referee.
- Transcripts and Master Degree / Diploma (if already available).
- Copy of Master thesis (or writing sample).
The position will remain open until June 23, and applications will be reviewed biweekly. The aim is to plan the interviews before the summer holidays (so until July 15th).
- A pre-employment screening can be part of the selection procedure.
- You can apply online. We will not process applications sent by email and/or post.
- Please do not contact us for unsolicited services.
For more information about this vacancy, please get in touch with Özge Şahin: [email protected] .
Work Location(s)
Where to apply.
Announcing the 2022 Apple Scholars in AIML
Apple is proud to announce the 2022 recipients of the Apple Scholars in AIML PhD fellowship.
We are committed to supporting the academic research community by amplifying emerging leaders in their field and their cutting-edge machine learning research.
The Apple Scholars in AIML PhD fellowship recognizes the contributions of emerging leaders in computer science and engineering at the graduate and postgraduate level. Each Scholar receives funding as they pursue their PhD, internship opportunities, and mentorship with an Apple researcher in their field. Apple Scholars in AIML are selected based on their innovative research, record as thought leaders and collaborators, and commitment to advance their respective fields.
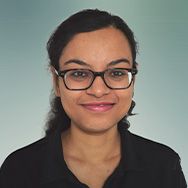
Sushmita Bhattacharya
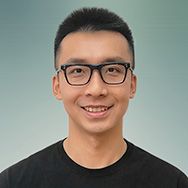
Yichen Jiang
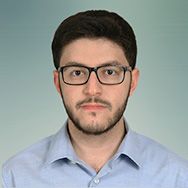
Omar Khattab
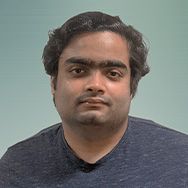
Aviral Kumar
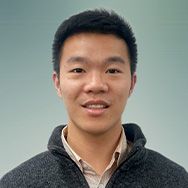
Venkatesh Potluri
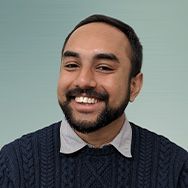
Aahlad Puli
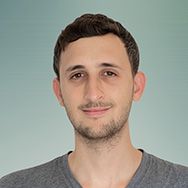
Elizabeth Salesky
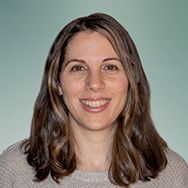
Ortal Senouf
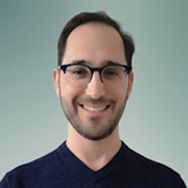
Moshe Shenfeld
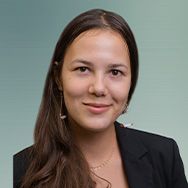
Prune Truong
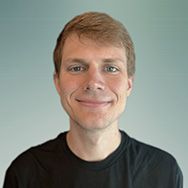
Eric Wallace
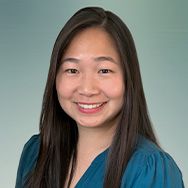
Serena Wang
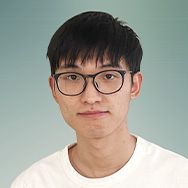
Guodong Zhang
The Apple Scholars in AIML PhD fellowship program is currently open to invited institutions. For more information, please email [email protected].
Learn more about the 2021 cohort of Apple Scholars in AIML
Learn more about the inaugural class of Apple Scholars in AIML
Related readings and updates.
Announcing the 2024 apple scholars in aiml.
Apple is proud to announce the 2024 recipients of the Apple Scholars in AIML PhD fellowship.
Announcing the 2023 Apple Scholars in AIML
Apple is proud to announce the 2023 recipients of the Apple Scholars in AIML PhD fellowship.
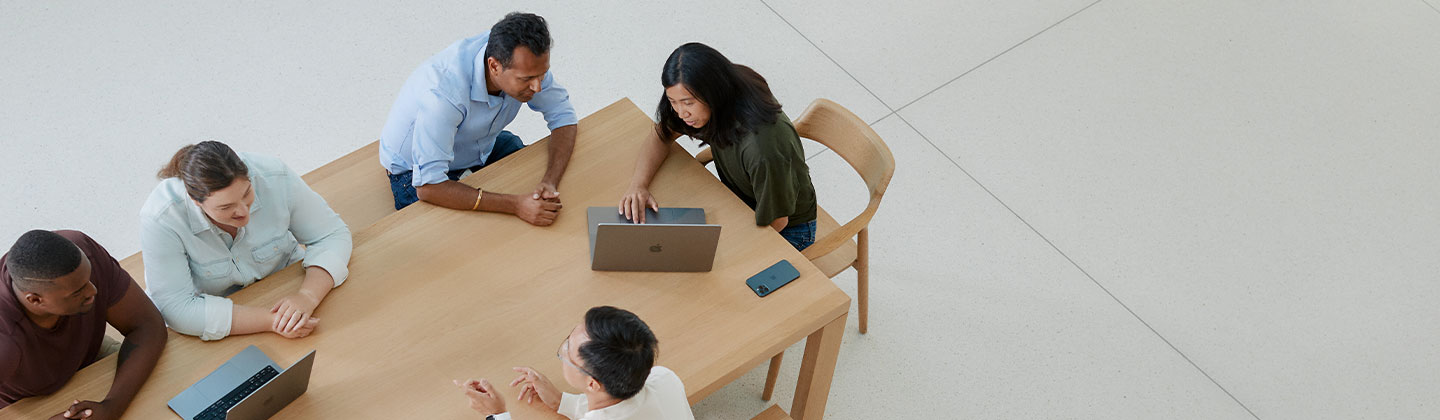
Discover opportunities in Machine Learning.
Our research in machine learning breaks new ground every day.
Work with us
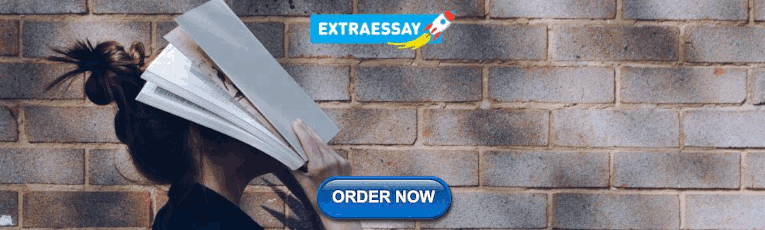
IMAGES
VIDEO
COMMENTS
For example, nominees that are enrolled in a four-year graduate program should have completed two years of their graduate program as of summer 2021 in order to benefit the most. 2-year fellowship. $60,000 in year one and $35,000 in year two. First or a second year of graduate student as of the application date.
The Google PhD Fellowship Program was created to recognize outstanding graduate students doing exceptional and innovative research in areas relevant to computer science and related fields. Fellowships support promising PhD candidates of all backgrounds who seek to influence the future of technology. Google's mission is to foster inclusive ...
Apple is proud to announce the 2021 recipients of the Apple Scholars in AIML PhD fellowship. Each Scholar receives two years of funding as they pursue their PhD, internship opportunities, and a two-year mentorship with an Apple researcher in their field. Nominated students were selected based on their innovative research, their record as ...
Apple is proud to announce the 2024 recipients of the Apple Scholars in AIML PhD fellowship. We are committed to supporting the academic research community by amplifying emerging leaders in their field and their cutting-edge machine learning research. The Apple Scholars in AIML PhD fellowship recognizes the contributions of researchers in ...
Apple Scholars is a program created to recognize the contributions of emerging leaders in computer science and engineering at the graduate and postgraduate level. As part of Apple Scholars, Apple is proud to announce the recipients of PhD fellowships in AIML. In recognition of these outstanding PhD students, each will receive support for their ...
The PhD fellowship in AI/ML was created as part of the Apple Scholars program to support the work of outstanding PhD students from around the world, who are pursuing cutting edge research in machine learning and artificial intelligence. Duke may nominate up to (3) students. Universities may nominate a maximum of (1) student to any of the ...
The Machine Learning (ML) Ph.D. program is a fully-funded doctoral program in machine learning (ML), designed to train students to become tomorrow's leaders through a combination of interdisciplinary coursework, and cutting-edge research. Graduates of the Ph.D. program in machine learning are uniquely positioned to pioneer new developments in the field, and to be leaders in both industry and ...
Harvard University, PhD in Computer Science includes Machine Learning. (Cambridge, Massachusetts): The financial aid program features guaranteed funding for the first five years to all Ph.D. students and a variety of funding options and fellowships for other students. This includes tuition, fees, and a cost-of-living stipend.
The fellowship awardee will be selected and contacted in Spring 2023. This one-year fellowship is designed to support a machine learning (ML) PhD student. Students from cultural, racial, linguistic, geographic and socioeconomic backgrounds and genders who are underrepresented in ML graduate education are encouraged to apply.
PhD Program. The machine learning (ML) Ph.D. program is a collaborative venture between Georgia Tech's colleges of Computing, Engineering, and Sciences. Approximately 25-30 students enter the program each year through nine different academic units.
The University of Cambridge Machine Learning Group and the Max Planck Institute for Intelligent Systems Empirical Inference Department in Tübingen are two of the world's leading centres for machine learning research. In 2014, we launched a new and exciting initiative whereby a small group of select PhD candidates are jointly supervised at ...
The Apple Scholars in AI/ML PhD fellowship program recognizes the contributions of emerging leaders in computer science and engineering at the graduate and postgraduate level.The PhD fellowship in AI/ML was created as part of the Apple Scholars program to support the work of outstanding PhD students from around the world, who are pursuing cutting edge research in machine learning and ...
The Google PhD Fellowship Program recognizes outstanding graduate students doing exceptional work in computer science, related disciplines, or promising research areas. ... Woosang LIM, Machine Learning, Korea Advanced Institute of Science and Technology. Ying CHEN, Systems and Networking, Tsinghua University.
The Cambridge Machine Learning Group (MLG) runs a PhD programme in Advanced Machine Learning. The supervisors are Jose Miguel Hernandez-Lobato, Carl Rasmussen, Richard E. Turner, Adrian Weller, Hong Ge and David Krueger. Zoubin Ghahramani is currently on academic leave and not accepting new students at this time.. We encourage applications from outstanding candidates with academic backgrounds ...
Global Scholarship. Merit-based. Read more about eligibility. Tulane University. New Orleans, United States. 1 of 46. Discover exclusive Machine Learning scholarships for PhD students. Unlock financial support for your Machine Learning studies with PhDportal.
Fully Funded EPSRC and Swansea PhD Scholarship: Physics Informed Machine Learning in High-speed Railway Infrastructure Safety Analysis. learning, data-driven method has become a powerful tool for civil engineers. Especially, Physics Informed Machine Learning (PIMM) can be used as a comprehensive analysis considering both physics-based.
We seek to build a community of scholars who work across boundaries and fields and are poised to solve some of the most compelling mysteries of our time. Coming together to learn, collaborate, and discover, Kempner community members are scientists who seek to fundamentally and radically advance our understanding of natural and artificial ...
The PhD fellowships in Machine Learning and AI were created to celebrate the contributions of students pursuing cutting-edge fundamental and applied machine learning research worldwide. ... As AI-based solutions spread across disciplines, the need for domain experts to understand machine learning and apply their expertise in ML settings grows ...
New: Kempner Institute Graduate Fellowship: See more details here. If you are applying for graduate studies in CS and are interested in machine learning foundations, please mark both "Machine Learning" and "Theory of Computation" as areas of interest. Please also list the names of faculty you want to work with on your application.
The Department of Computer Science (DIKU) at the University of Copenhagen invites applicants for a PhD fellowship in Machine Learning with a particular focus on Trustworthy Machine Learning or learning with imperfect data. Start date is (expected to be) on 1 November 2024, or as soon as possible thereafter.
This is a graduate course in a new branch of machine learning: federated learning (FL). In FL, machine learning models are trained on mobile devices with an explicit effort to preserve the privacy of users' data. FL combines supervised machine learning, privacy, distributed and edge computing, optimization, communication compression, and systems.
May 13, 2024. PhD Scholarship: Machine Learning: Luleå University of Technology invites applications for a PhD scholarship in Machine Learning, based in Luleå, Sweden. This opportunity is part of the university's commitment to cutting-edge research and its contribution to the development of a sustainable society.
January 31, 2023. The School of Statistics and the Institute for Research in Statistics and Its Applications (IRSA) at the University of Minnesota invites applications for the IRSA Faragher Distinguished Postdoctoral Fellowship. This endowed fellowship seeks to bring exceptional scientists of outstanding potential to the university.
In this thesis, we investigate the use of machine-learning models for solving the KS equations. Our strategy is to develop machine-learning models which approximate numerical quantities in existing algorithms for electronic structure. In particular we use equivariant graph-neural-networks to predict the Kohn-Sham charge density.
Apple is proud to announce the 2023 recipients of the Apple Scholars in AIML PhD fellowship. We are committed to supporting the academic research community by amplifying emerging leaders in their field and their cutting-edge machine learning research. The Apple Scholars in AIML PhD fellowship recognizes the contributions of researchers in ...
The Data-Driven Mechanics Laboratory is seeking a highly motivated doctoral student to study physics- and thermodynamics-informed machine learning (ML) for the mechanics of material failure. The project focuses on learning the effective behavior of materials of civil engineering interest (granular geomaterials, structural materials) arising from complex microstructural mechanisms involving ...
May 14, 2024. By Sean Yoder. Penn State graduate student Denzell Ivery earned a Boren Fellowship, which will provide him the opportunity to study the Turkish language and eventually travel to Baku, Azerbaijan. Ivery, of Philadelphia, is a veteran Marine enrolled in the Master of Professional of Studies in Community and Economic Development ...
As a PhD researcher, you'll be at the forefront of this exciting journey, focusing on multivariate dependence models and statistical machine learning algorithms at its core to create personalized individual risk profiles. Along with developing novel methods and investigating their theoretical properties, you will also apply your methods and ...
Apple is proud to announce the 2022 recipients of the Apple Scholars in AIML PhD fellowship. We are committed to supporting the academic research community by amplifying emerging leaders in their field and their cutting-edge machine learning research. The Apple Scholars in AIML PhD fellowship recognizes the contributions of emerging leaders in ...