Have a language expert improve your writing
Run a free plagiarism check in 10 minutes, generate accurate citations for free.
- Knowledge Base
- Null and Alternative Hypotheses | Definitions & Examples
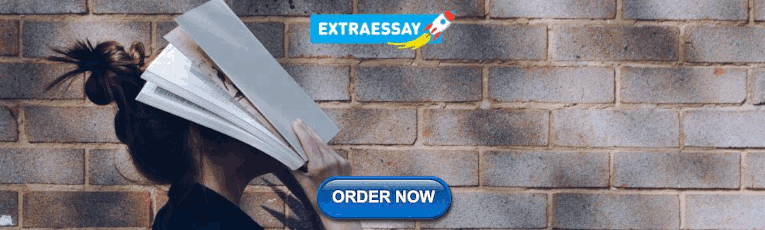
Null & Alternative Hypotheses | Definitions, Templates & Examples
Published on May 6, 2022 by Shaun Turney . Revised on June 22, 2023.
The null and alternative hypotheses are two competing claims that researchers weigh evidence for and against using a statistical test :
- Null hypothesis ( H 0 ): There’s no effect in the population .
- Alternative hypothesis ( H a or H 1 ) : There’s an effect in the population.
Table of contents
Answering your research question with hypotheses, what is a null hypothesis, what is an alternative hypothesis, similarities and differences between null and alternative hypotheses, how to write null and alternative hypotheses, other interesting articles, frequently asked questions.
The null and alternative hypotheses offer competing answers to your research question . When the research question asks “Does the independent variable affect the dependent variable?”:
- The null hypothesis ( H 0 ) answers “No, there’s no effect in the population.”
- The alternative hypothesis ( H a ) answers “Yes, there is an effect in the population.”
The null and alternative are always claims about the population. That’s because the goal of hypothesis testing is to make inferences about a population based on a sample . Often, we infer whether there’s an effect in the population by looking at differences between groups or relationships between variables in the sample. It’s critical for your research to write strong hypotheses .
You can use a statistical test to decide whether the evidence favors the null or alternative hypothesis. Each type of statistical test comes with a specific way of phrasing the null and alternative hypothesis. However, the hypotheses can also be phrased in a general way that applies to any test.
Prevent plagiarism. Run a free check.
The null hypothesis is the claim that there’s no effect in the population.
If the sample provides enough evidence against the claim that there’s no effect in the population ( p ≤ α), then we can reject the null hypothesis . Otherwise, we fail to reject the null hypothesis.
Although “fail to reject” may sound awkward, it’s the only wording that statisticians accept . Be careful not to say you “prove” or “accept” the null hypothesis.
Null hypotheses often include phrases such as “no effect,” “no difference,” or “no relationship.” When written in mathematical terms, they always include an equality (usually =, but sometimes ≥ or ≤).
You can never know with complete certainty whether there is an effect in the population. Some percentage of the time, your inference about the population will be incorrect. When you incorrectly reject the null hypothesis, it’s called a type I error . When you incorrectly fail to reject it, it’s a type II error.
Examples of null hypotheses
The table below gives examples of research questions and null hypotheses. There’s always more than one way to answer a research question, but these null hypotheses can help you get started.
( ) | ||
Does tooth flossing affect the number of cavities? | Tooth flossing has on the number of cavities. | test: The mean number of cavities per person does not differ between the flossing group (µ ) and the non-flossing group (µ ) in the population; µ = µ . |
Does the amount of text highlighted in the textbook affect exam scores? | The amount of text highlighted in the textbook has on exam scores. | : There is no relationship between the amount of text highlighted and exam scores in the population; β = 0. |
Does daily meditation decrease the incidence of depression? | Daily meditation the incidence of depression.* | test: The proportion of people with depression in the daily-meditation group ( ) is greater than or equal to the no-meditation group ( ) in the population; ≥ . |
*Note that some researchers prefer to always write the null hypothesis in terms of “no effect” and “=”. It would be fine to say that daily meditation has no effect on the incidence of depression and p 1 = p 2 .
The alternative hypothesis ( H a ) is the other answer to your research question . It claims that there’s an effect in the population.
Often, your alternative hypothesis is the same as your research hypothesis. In other words, it’s the claim that you expect or hope will be true.
The alternative hypothesis is the complement to the null hypothesis. Null and alternative hypotheses are exhaustive, meaning that together they cover every possible outcome. They are also mutually exclusive, meaning that only one can be true at a time.
Alternative hypotheses often include phrases such as “an effect,” “a difference,” or “a relationship.” When alternative hypotheses are written in mathematical terms, they always include an inequality (usually ≠, but sometimes < or >). As with null hypotheses, there are many acceptable ways to phrase an alternative hypothesis.
Examples of alternative hypotheses
The table below gives examples of research questions and alternative hypotheses to help you get started with formulating your own.
Does tooth flossing affect the number of cavities? | Tooth flossing has an on the number of cavities. | test: The mean number of cavities per person differs between the flossing group (µ ) and the non-flossing group (µ ) in the population; µ ≠ µ . |
Does the amount of text highlighted in a textbook affect exam scores? | The amount of text highlighted in the textbook has an on exam scores. | : There is a relationship between the amount of text highlighted and exam scores in the population; β ≠ 0. |
Does daily meditation decrease the incidence of depression? | Daily meditation the incidence of depression. | test: The proportion of people with depression in the daily-meditation group ( ) is less than the no-meditation group ( ) in the population; < . |
Null and alternative hypotheses are similar in some ways:
- They’re both answers to the research question.
- They both make claims about the population.
- They’re both evaluated by statistical tests.
However, there are important differences between the two types of hypotheses, summarized in the following table.
A claim that there is in the population. | A claim that there is in the population. | |
| ||
Equality symbol (=, ≥, or ≤) | Inequality symbol (≠, <, or >) | |
Rejected | Supported | |
Failed to reject | Not supported |
To help you write your hypotheses, you can use the template sentences below. If you know which statistical test you’re going to use, you can use the test-specific template sentences. Otherwise, you can use the general template sentences.
General template sentences
The only thing you need to know to use these general template sentences are your dependent and independent variables. To write your research question, null hypothesis, and alternative hypothesis, fill in the following sentences with your variables:
Does independent variable affect dependent variable ?
- Null hypothesis ( H 0 ): Independent variable does not affect dependent variable.
- Alternative hypothesis ( H a ): Independent variable affects dependent variable.
Test-specific template sentences
Once you know the statistical test you’ll be using, you can write your hypotheses in a more precise and mathematical way specific to the test you chose. The table below provides template sentences for common statistical tests.
( ) | ||
test
with two groups | The mean dependent variable does not differ between group 1 (µ ) and group 2 (µ ) in the population; µ = µ . | The mean dependent variable differs between group 1 (µ ) and group 2 (µ ) in the population; µ ≠ µ . |
with three groups | The mean dependent variable does not differ between group 1 (µ ), group 2 (µ ), and group 3 (µ ) in the population; µ = µ = µ . | The mean dependent variable of group 1 (µ ), group 2 (µ ), and group 3 (µ ) are not all equal in the population. |
There is no correlation between independent variable and dependent variable in the population; ρ = 0. | There is a correlation between independent variable and dependent variable in the population; ρ ≠ 0. | |
There is no relationship between independent variable and dependent variable in the population; β = 0. | There is a relationship between independent variable and dependent variable in the population; β ≠ 0. | |
Two-proportions test | The dependent variable expressed as a proportion does not differ between group 1 ( ) and group 2 ( ) in the population; = . | The dependent variable expressed as a proportion differs between group 1 ( ) and group 2 ( ) in the population; ≠ . |
Note: The template sentences above assume that you’re performing one-tailed tests . One-tailed tests are appropriate for most studies.
If you want to know more about statistics , methodology , or research bias , make sure to check out some of our other articles with explanations and examples.
- Normal distribution
- Descriptive statistics
- Measures of central tendency
- Correlation coefficient
Methodology
- Cluster sampling
- Stratified sampling
- Types of interviews
- Cohort study
- Thematic analysis
Research bias
- Implicit bias
- Cognitive bias
- Survivorship bias
- Availability heuristic
- Nonresponse bias
- Regression to the mean
Hypothesis testing is a formal procedure for investigating our ideas about the world using statistics. It is used by scientists to test specific predictions, called hypotheses , by calculating how likely it is that a pattern or relationship between variables could have arisen by chance.
Null and alternative hypotheses are used in statistical hypothesis testing . The null hypothesis of a test always predicts no effect or no relationship between variables, while the alternative hypothesis states your research prediction of an effect or relationship.
The null hypothesis is often abbreviated as H 0 . When the null hypothesis is written using mathematical symbols, it always includes an equality symbol (usually =, but sometimes ≥ or ≤).
The alternative hypothesis is often abbreviated as H a or H 1 . When the alternative hypothesis is written using mathematical symbols, it always includes an inequality symbol (usually ≠, but sometimes < or >).
A research hypothesis is your proposed answer to your research question. The research hypothesis usually includes an explanation (“ x affects y because …”).
A statistical hypothesis, on the other hand, is a mathematical statement about a population parameter. Statistical hypotheses always come in pairs: the null and alternative hypotheses . In a well-designed study , the statistical hypotheses correspond logically to the research hypothesis.
Cite this Scribbr article
If you want to cite this source, you can copy and paste the citation or click the “Cite this Scribbr article” button to automatically add the citation to our free Citation Generator.
Turney, S. (2023, June 22). Null & Alternative Hypotheses | Definitions, Templates & Examples. Scribbr. Retrieved August 27, 2024, from https://www.scribbr.com/statistics/null-and-alternative-hypotheses/
Is this article helpful?
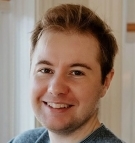
Shaun Turney
Other students also liked, inferential statistics | an easy introduction & examples, hypothesis testing | a step-by-step guide with easy examples, type i & type ii errors | differences, examples, visualizations, what is your plagiarism score.
- Skip to secondary menu
- Skip to main content
- Skip to primary sidebar
Statistics By Jim
Making statistics intuitive
Null Hypothesis: Definition, Rejecting & Examples
By Jim Frost 6 Comments
What is a Null Hypothesis?
The null hypothesis in statistics states that there is no difference between groups or no relationship between variables. It is one of two mutually exclusive hypotheses about a population in a hypothesis test.

- Null Hypothesis H 0 : No effect exists in the population.
- Alternative Hypothesis H A : The effect exists in the population.
In every study or experiment, researchers assess an effect or relationship. This effect can be the effectiveness of a new drug, building material, or other intervention that has benefits. There is a benefit or connection that the researchers hope to identify. Unfortunately, no effect may exist. In statistics, we call this lack of an effect the null hypothesis. Researchers assume that this notion of no effect is correct until they have enough evidence to suggest otherwise, similar to how a trial presumes innocence.
In this context, the analysts don’t necessarily believe the null hypothesis is correct. In fact, they typically want to reject it because that leads to more exciting finds about an effect or relationship. The new vaccine works!
You can think of it as the default theory that requires sufficiently strong evidence to reject. Like a prosecutor, researchers must collect sufficient evidence to overturn the presumption of no effect. Investigators must work hard to set up a study and a data collection system to obtain evidence that can reject the null hypothesis.
Related post : What is an Effect in Statistics?
Null Hypothesis Examples
Null hypotheses start as research questions that the investigator rephrases as a statement indicating there is no effect or relationship.
Does the vaccine prevent infections? | The vaccine does not affect the infection rate. |
Does the new additive increase product strength? | The additive does not affect mean product strength. |
Does the exercise intervention increase bone mineral density? | The intervention does not affect bone mineral density. |
As screen time increases, does test performance decrease? | There is no relationship between screen time and test performance. |
After reading these examples, you might think they’re a bit boring and pointless. However, the key is to remember that the null hypothesis defines the condition that the researchers need to discredit before suggesting an effect exists.
Let’s see how you reject the null hypothesis and get to those more exciting findings!
When to Reject the Null Hypothesis
So, you want to reject the null hypothesis, but how and when can you do that? To start, you’ll need to perform a statistical test on your data. The following is an overview of performing a study that uses a hypothesis test.
The first step is to devise a research question and the appropriate null hypothesis. After that, the investigators need to formulate an experimental design and data collection procedures that will allow them to gather data that can answer the research question. Then they collect the data. For more information about designing a scientific study that uses statistics, read my post 5 Steps for Conducting Studies with Statistics .
After data collection is complete, statistics and hypothesis testing enter the picture. Hypothesis testing takes your sample data and evaluates how consistent they are with the null hypothesis. The p-value is a crucial part of the statistical results because it quantifies how strongly the sample data contradict the null hypothesis.
When the sample data provide sufficient evidence, you can reject the null hypothesis. In a hypothesis test, this process involves comparing the p-value to your significance level .
Rejecting the Null Hypothesis
Reject the null hypothesis when the p-value is less than or equal to your significance level. Your sample data favor the alternative hypothesis, which suggests that the effect exists in the population. For a mnemonic device, remember—when the p-value is low, the null must go!
When you can reject the null hypothesis, your results are statistically significant. Learn more about Statistical Significance: Definition & Meaning .
Failing to Reject the Null Hypothesis
Conversely, when the p-value is greater than your significance level, you fail to reject the null hypothesis. The sample data provides insufficient data to conclude that the effect exists in the population. When the p-value is high, the null must fly!
Note that failing to reject the null is not the same as proving it. For more information about the difference, read my post about Failing to Reject the Null .
That’s a very general look at the process. But I hope you can see how the path to more exciting findings depends on being able to rule out the less exciting null hypothesis that states there’s nothing to see here!
Let’s move on to learning how to write the null hypothesis for different types of effects, relationships, and tests.
Related posts : How Hypothesis Tests Work and Interpreting P-values
How to Write a Null Hypothesis
The null hypothesis varies by the type of statistic and hypothesis test. Remember that inferential statistics use samples to draw conclusions about populations. Consequently, when you write a null hypothesis, it must make a claim about the relevant population parameter . Further, that claim usually indicates that the effect does not exist in the population. Below are typical examples of writing a null hypothesis for various parameters and hypothesis tests.
Related posts : Descriptive vs. Inferential Statistics and Populations, Parameters, and Samples in Inferential Statistics
Group Means
T-tests and ANOVA assess the differences between group means. For these tests, the null hypothesis states that there is no difference between group means in the population. In other words, the experimental conditions that define the groups do not affect the mean outcome. Mu (µ) is the population parameter for the mean, and you’ll need to include it in the statement for this type of study.
For example, an experiment compares the mean bone density changes for a new osteoporosis medication. The control group does not receive the medicine, while the treatment group does. The null states that the mean bone density changes for the control and treatment groups are equal.
- Null Hypothesis H 0 : Group means are equal in the population: µ 1 = µ 2 , or µ 1 – µ 2 = 0
- Alternative Hypothesis H A : Group means are not equal in the population: µ 1 ≠ µ 2 , or µ 1 – µ 2 ≠ 0.
Group Proportions
Proportions tests assess the differences between group proportions. For these tests, the null hypothesis states that there is no difference between group proportions. Again, the experimental conditions did not affect the proportion of events in the groups. P is the population proportion parameter that you’ll need to include.
For example, a vaccine experiment compares the infection rate in the treatment group to the control group. The treatment group receives the vaccine, while the control group does not. The null states that the infection rates for the control and treatment groups are equal.
- Null Hypothesis H 0 : Group proportions are equal in the population: p 1 = p 2 .
- Alternative Hypothesis H A : Group proportions are not equal in the population: p 1 ≠ p 2 .
Correlation and Regression Coefficients
Some studies assess the relationship between two continuous variables rather than differences between groups.
In these studies, analysts often use either correlation or regression analysis . For these tests, the null states that there is no relationship between the variables. Specifically, it says that the correlation or regression coefficient is zero. As one variable increases, there is no tendency for the other variable to increase or decrease. Rho (ρ) is the population correlation parameter and beta (β) is the regression coefficient parameter.
For example, a study assesses the relationship between screen time and test performance. The null states that there is no correlation between this pair of variables. As screen time increases, test performance does not tend to increase or decrease.
- Null Hypothesis H 0 : The correlation in the population is zero: ρ = 0.
- Alternative Hypothesis H A : The correlation in the population is not zero: ρ ≠ 0.
For all these cases, the analysts define the hypotheses before the study. After collecting the data, they perform a hypothesis test to determine whether they can reject the null hypothesis.
The preceding examples are all for two-tailed hypothesis tests. To learn about one-tailed tests and how to write a null hypothesis for them, read my post One-Tailed vs. Two-Tailed Tests .
Related post : Understanding Correlation
Neyman, J; Pearson, E. S. (January 1, 1933). On the Problem of the most Efficient Tests of Statistical Hypotheses . Philosophical Transactions of the Royal Society A . 231 (694–706): 289–337.
Share this:

Reader Interactions
January 11, 2024 at 2:57 pm
Thanks for the reply.
January 10, 2024 at 1:23 pm
Hi Jim, In your comment you state that equivalence test null and alternate hypotheses are reversed. For hypothesis tests of data fits to a probability distribution, the null hypothesis is that the probability distribution fits the data. Is this correct?
January 10, 2024 at 2:15 pm
Those two separate things, equivalence testing and normality tests. But, yes, you’re correct for both.
Hypotheses are switched for equivalence testing. You need to “work” (i.e., collect a large sample of good quality data) to be able to reject the null that the groups are different to be able to conclude they’re the same.
With typical hypothesis tests, if you have low quality data and a low sample size, you’ll fail to reject the null that they’re the same, concluding they’re equivalent. But that’s more a statement about the low quality and small sample size than anything to do with the groups being equal.
So, equivalence testing make you work to obtain a finding that the groups are the same (at least within some amount you define as a trivial difference).
For normality testing, and other distribution tests, the null states that the data follow the distribution (normal or whatever). If you reject the null, you have sufficient evidence to conclude that your sample data don’t follow the probability distribution. That’s a rare case where you hope to fail to reject the null. And it suffers from the problem I describe above where you might fail to reject the null simply because you have a small sample size. In that case, you’d conclude the data follow the probability distribution but it’s more that you don’t have enough data for the test to register the deviation. In this scenario, if you had a larger sample size, you’d reject the null and conclude it doesn’t follow that distribution.
I don’t know of any equivalence testing type approach for distribution fit tests where you’d need to work to show the data follow a distribution, although I haven’t looked for one either!
February 20, 2022 at 9:26 pm
Is a null hypothesis regularly (always) stated in the negative? “there is no” or “does not”
February 23, 2022 at 9:21 pm
Typically, the null hypothesis includes an equal sign. The null hypothesis states that the population parameter equals a particular value. That value is usually one that represents no effect. In the case of a one-sided hypothesis test, the null still contains an equal sign but it’s “greater than or equal to” or “less than or equal to.” If you wanted to translate the null hypothesis from its native mathematical expression, you could use the expression “there is no effect.” But the mathematical form more specifically states what it’s testing.
It’s the alternative hypothesis that typically contains does not equal.
There are some exceptions. For example, in an equivalence test where the researchers want to show that two things are equal, the null hypothesis states that they’re not equal.
In short, the null hypothesis states the condition that the researchers hope to reject. They need to work hard to set up an experiment and data collection that’ll gather enough evidence to be able to reject the null condition.
February 15, 2022 at 9:32 am
Dear sir I always read your notes on Research methods.. Kindly tell is there any available Book on all these..wonderfull Urgent
Comments and Questions Cancel reply
Have a thesis expert improve your writing
Check your thesis for plagiarism in 10 minutes, generate your apa citations for free.
- Knowledge Base
- Null and Alternative Hypotheses | Definitions & Examples
Null and Alternative Hypotheses | Definitions & Examples
Published on 5 October 2022 by Shaun Turney . Revised on 6 December 2022.
The null and alternative hypotheses are two competing claims that researchers weigh evidence for and against using a statistical test :
- Null hypothesis (H 0 ): There’s no effect in the population .
- Alternative hypothesis (H A ): There’s an effect in the population.
The effect is usually the effect of the independent variable on the dependent variable .
Table of contents
Answering your research question with hypotheses, what is a null hypothesis, what is an alternative hypothesis, differences between null and alternative hypotheses, how to write null and alternative hypotheses, frequently asked questions about null and alternative hypotheses.
The null and alternative hypotheses offer competing answers to your research question . When the research question asks “Does the independent variable affect the dependent variable?”, the null hypothesis (H 0 ) answers “No, there’s no effect in the population.” On the other hand, the alternative hypothesis (H A ) answers “Yes, there is an effect in the population.”
The null and alternative are always claims about the population. That’s because the goal of hypothesis testing is to make inferences about a population based on a sample . Often, we infer whether there’s an effect in the population by looking at differences between groups or relationships between variables in the sample.
You can use a statistical test to decide whether the evidence favors the null or alternative hypothesis. Each type of statistical test comes with a specific way of phrasing the null and alternative hypothesis. However, the hypotheses can also be phrased in a general way that applies to any test.
The null hypothesis is the claim that there’s no effect in the population.
If the sample provides enough evidence against the claim that there’s no effect in the population ( p ≤ α), then we can reject the null hypothesis . Otherwise, we fail to reject the null hypothesis.
Although “fail to reject” may sound awkward, it’s the only wording that statisticians accept. Be careful not to say you “prove” or “accept” the null hypothesis.
Null hypotheses often include phrases such as “no effect”, “no difference”, or “no relationship”. When written in mathematical terms, they always include an equality (usually =, but sometimes ≥ or ≤).
Examples of null hypotheses
The table below gives examples of research questions and null hypotheses. There’s always more than one way to answer a research question, but these null hypotheses can help you get started.
( ) | ||
Does tooth flossing affect the number of cavities? | Tooth flossing has on the number of cavities. | test: The mean number of cavities per person does not differ between the flossing group (µ ) and the non-flossing group (µ ) in the population; µ = µ . |
Does the amount of text highlighted in the textbook affect exam scores? | The amount of text highlighted in the textbook has on exam scores. | : There is no relationship between the amount of text highlighted and exam scores in the population; β = 0. |
Does daily meditation decrease the incidence of depression? | Daily meditation the incidence of depression.* | test: The proportion of people with depression in the daily-meditation group ( ) is greater than or equal to the no-meditation group ( ) in the population; ≥ . |
*Note that some researchers prefer to always write the null hypothesis in terms of “no effect” and “=”. It would be fine to say that daily meditation has no effect on the incidence of depression and p 1 = p 2 .
The alternative hypothesis (H A ) is the other answer to your research question . It claims that there’s an effect in the population.
Often, your alternative hypothesis is the same as your research hypothesis. In other words, it’s the claim that you expect or hope will be true.
The alternative hypothesis is the complement to the null hypothesis. Null and alternative hypotheses are exhaustive, meaning that together they cover every possible outcome. They are also mutually exclusive, meaning that only one can be true at a time.
Alternative hypotheses often include phrases such as “an effect”, “a difference”, or “a relationship”. When alternative hypotheses are written in mathematical terms, they always include an inequality (usually ≠, but sometimes > or <). As with null hypotheses, there are many acceptable ways to phrase an alternative hypothesis.
Examples of alternative hypotheses
The table below gives examples of research questions and alternative hypotheses to help you get started with formulating your own.
Does tooth flossing affect the number of cavities? | Tooth flossing has an on the number of cavities. | test: The mean number of cavities per person differs between the flossing group (µ ) and the non-flossing group (µ ) in the population; µ ≠ µ . |
Does the amount of text highlighted in a textbook affect exam scores? | The amount of text highlighted in the textbook has an on exam scores. | : There is a relationship between the amount of text highlighted and exam scores in the population; β ≠ 0. |
Does daily meditation decrease the incidence of depression? | Daily meditation the incidence of depression. | test: The proportion of people with depression in the daily-meditation group ( ) is less than the no-meditation group ( ) in the population; < . |
Null and alternative hypotheses are similar in some ways:
- They’re both answers to the research question
- They both make claims about the population
- They’re both evaluated by statistical tests.
However, there are important differences between the two types of hypotheses, summarized in the following table.
A claim that there is in the population. | A claim that there is in the population. | |
| ||
Equality symbol (=, ≥, or ≤) | Inequality symbol (≠, <, or >) | |
Rejected | Supported | |
Failed to reject | Not supported |
To help you write your hypotheses, you can use the template sentences below. If you know which statistical test you’re going to use, you can use the test-specific template sentences. Otherwise, you can use the general template sentences.
The only thing you need to know to use these general template sentences are your dependent and independent variables. To write your research question, null hypothesis, and alternative hypothesis, fill in the following sentences with your variables:
Does independent variable affect dependent variable ?
- Null hypothesis (H 0 ): Independent variable does not affect dependent variable .
- Alternative hypothesis (H A ): Independent variable affects dependent variable .
Test-specific
Once you know the statistical test you’ll be using, you can write your hypotheses in a more precise and mathematical way specific to the test you chose. The table below provides template sentences for common statistical tests.
( ) | ||
test
with two groups | The mean dependent variable does not differ between group 1 (µ ) and group 2 (µ ) in the population; µ = µ . | The mean dependent variable differs between group 1 (µ ) and group 2 (µ ) in the population; µ ≠ µ . |
with three groups | The mean dependent variable does not differ between group 1 (µ ), group 2 (µ ), and group 3 (µ ) in the population; µ = µ = µ . | The mean dependent variable of group 1 (µ ), group 2 (µ ), and group 3 (µ ) are not all equal in the population. |
There is no correlation between independent variable and dependent variable in the population; ρ = 0. | There is a correlation between independent variable and dependent variable in the population; ρ ≠ 0. | |
There is no relationship between independent variable and dependent variable in the population; β = 0. | There is a relationship between independent variable and dependent variable in the population; β ≠ 0. | |
Two-proportions test | The dependent variable expressed as a proportion does not differ between group 1 ( ) and group 2 ( ) in the population; = . | The dependent variable expressed as a proportion differs between group 1 ( ) and group 2 ( ) in the population; ≠ . |
Note: The template sentences above assume that you’re performing one-tailed tests . One-tailed tests are appropriate for most studies.
The null hypothesis is often abbreviated as H 0 . When the null hypothesis is written using mathematical symbols, it always includes an equality symbol (usually =, but sometimes ≥ or ≤).
The alternative hypothesis is often abbreviated as H a or H 1 . When the alternative hypothesis is written using mathematical symbols, it always includes an inequality symbol (usually ≠, but sometimes < or >).
A research hypothesis is your proposed answer to your research question. The research hypothesis usually includes an explanation (‘ x affects y because …’).
A statistical hypothesis, on the other hand, is a mathematical statement about a population parameter. Statistical hypotheses always come in pairs: the null and alternative hypotheses. In a well-designed study , the statistical hypotheses correspond logically to the research hypothesis.
Cite this Scribbr article
If you want to cite this source, you can copy and paste the citation or click the ‘Cite this Scribbr article’ button to automatically add the citation to our free Reference Generator.
Turney, S. (2022, December 06). Null and Alternative Hypotheses | Definitions & Examples. Scribbr. Retrieved 26 August 2024, from https://www.scribbr.co.uk/stats/null-and-alternative-hypothesis/
Is this article helpful?
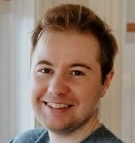
Shaun Turney
Other students also liked, levels of measurement: nominal, ordinal, interval, ratio, the standard normal distribution | calculator, examples & uses, types of variables in research | definitions & examples.
Null Hypothesis Examples
ThoughtCo / Hilary Allison
- Scientific Method
- Chemical Laws
- Periodic Table
- Projects & Experiments
- Biochemistry
- Physical Chemistry
- Medical Chemistry
- Chemistry In Everyday Life
- Famous Chemists
- Activities for Kids
- Abbreviations & Acronyms
- Weather & Climate
- Ph.D., Biomedical Sciences, University of Tennessee at Knoxville
- B.A., Physics and Mathematics, Hastings College
In statistical analysis, the null hypothesis assumes there is no meaningful relationship between two variables. Testing the null hypothesis can tell you whether your results are due to the effect of manipulating a dependent variable or due to chance. It's often used in conjunction with an alternative hypothesis, which assumes there is, in fact, a relationship between two variables.
The null hypothesis is among the easiest hypothesis to test using statistical analysis, making it perhaps the most valuable hypothesis for the scientific method. By evaluating a null hypothesis in addition to another hypothesis, researchers can support their conclusions with a higher level of confidence. Below are examples of how you might formulate a null hypothesis to fit certain questions.
What Is the Null Hypothesis?
The null hypothesis states there is no relationship between the measured phenomenon (the dependent variable ) and the independent variable , which is the variable an experimenter typically controls or changes. You do not need to believe that the null hypothesis is true to test it. On the contrary, you will likely suspect there is a relationship between a set of variables. One way to prove that this is the case is to reject the null hypothesis. Rejecting a hypothesis does not mean an experiment was "bad" or that it didn't produce results. In fact, it is often one of the first steps toward further inquiry.
To distinguish it from other hypotheses , the null hypothesis is written as H 0 (which is read as “H-nought,” "H-null," or "H-zero"). A significance test is used to determine the likelihood that the results supporting the null hypothesis are not due to chance. A confidence level of 95% or 99% is common. Keep in mind, even if the confidence level is high, there is still a small chance the null hypothesis is not true, perhaps because the experimenter did not account for a critical factor or because of chance. This is one reason why it's important to repeat experiments.
Examples of the Null Hypothesis
To write a null hypothesis, first start by asking a question. Rephrase that question in a form that assumes no relationship between the variables. In other words, assume a treatment has no effect. Write your hypothesis in a way that reflects this.
Are teens better at math than adults? | Age has no effect on mathematical ability. |
Does taking aspirin every day reduce the chance of having a heart attack? | Taking aspirin daily does not affect heart attack risk. |
Do teens use cell phones to access the internet more than adults? | Age has no effect on how cell phones are used for internet access. |
Do cats care about the color of their food? | Cats express no food preference based on color. |
Does chewing willow bark relieve pain? | There is no difference in pain relief after chewing willow bark versus taking a placebo. |
Other Types of Hypotheses
In addition to the null hypothesis, the alternative hypothesis is also a staple in traditional significance tests . It's essentially the opposite of the null hypothesis because it assumes the claim in question is true. For the first item in the table above, for example, an alternative hypothesis might be "Age does have an effect on mathematical ability."
Key Takeaways
- In hypothesis testing, the null hypothesis assumes no relationship between two variables, providing a baseline for statistical analysis.
- Rejecting the null hypothesis suggests there is evidence of a relationship between variables.
- By formulating a null hypothesis, researchers can systematically test assumptions and draw more reliable conclusions from their experiments.
- What Are Examples of a Hypothesis?
- Random Error vs. Systematic Error
- Six Steps of the Scientific Method
- What Is a Hypothesis? (Science)
- Scientific Method Flow Chart
- What Are the Elements of a Good Hypothesis?
- Scientific Method Vocabulary Terms
- Understanding Simple vs Controlled Experiments
- The Role of a Controlled Variable in an Experiment
- What Is an Experimental Constant?
- What Is a Testable Hypothesis?
- Scientific Hypothesis Examples
- What Is the Difference Between a Control Variable and Control Group?
- DRY MIX Experiment Variables Acronym
- What Is a Controlled Experiment?
- Scientific Variable
- PRO Courses Guides New Tech Help Pro Expert Videos About wikiHow Pro Upgrade Sign In
- EDIT Edit this Article
- EXPLORE Tech Help Pro About Us Random Article Quizzes Request a New Article Community Dashboard This Or That Game Happiness Hub Popular Categories Arts and Entertainment Artwork Books Movies Computers and Electronics Computers Phone Skills Technology Hacks Health Men's Health Mental Health Women's Health Relationships Dating Love Relationship Issues Hobbies and Crafts Crafts Drawing Games Education & Communication Communication Skills Personal Development Studying Personal Care and Style Fashion Hair Care Personal Hygiene Youth Personal Care School Stuff Dating All Categories Arts and Entertainment Finance and Business Home and Garden Relationship Quizzes Cars & Other Vehicles Food and Entertaining Personal Care and Style Sports and Fitness Computers and Electronics Health Pets and Animals Travel Education & Communication Hobbies and Crafts Philosophy and Religion Work World Family Life Holidays and Traditions Relationships Youth
- Browse Articles
- Learn Something New
- Quizzes Hot
- Happiness Hub
- This Or That Game
- Train Your Brain
- Explore More
- Support wikiHow
- About wikiHow
- Log in / Sign up
- Education and Communications
- College University and Postgraduate
- Academic Writing
Writing Null Hypotheses in Research and Statistics
Last Updated: January 17, 2024 Fact Checked
This article was co-authored by Joseph Quinones and by wikiHow staff writer, Jennifer Mueller, JD . Joseph Quinones is a High School Physics Teacher working at South Bronx Community Charter High School. Joseph specializes in astronomy and astrophysics and is interested in science education and science outreach, currently practicing ways to make physics accessible to more students with the goal of bringing more students of color into the STEM fields. He has experience working on Astrophysics research projects at the Museum of Natural History (AMNH). Joseph recieved his Bachelor's degree in Physics from Lehman College and his Masters in Physics Education from City College of New York (CCNY). He is also a member of a network called New York City Men Teach. There are 7 references cited in this article, which can be found at the bottom of the page. This article has been fact-checked, ensuring the accuracy of any cited facts and confirming the authority of its sources. This article has been viewed 29,054 times.
Are you working on a research project and struggling with how to write a null hypothesis? Well, you've come to the right place! Start by recognizing that the basic definition of "null" is "none" or "zero"—that's your biggest clue as to what a null hypothesis should say. Keep reading to learn everything you need to know about the null hypothesis, including how it relates to your research question and your alternative hypothesis as well as how to use it in different types of studies.
Things You Should Know
- Write a research null hypothesis as a statement that the studied variables have no relationship to each other, or that there's no difference between 2 groups.
- Adjust the format of your null hypothesis to match the statistical method you used to test it, such as using "mean" if you're comparing the mean between 2 groups.
What is a null hypothesis?

- Research hypothesis: States in plain language that there's no relationship between the 2 variables or there's no difference between the 2 groups being studied.
- Statistical hypothesis: States the predicted outcome of statistical analysis through a mathematical equation related to the statistical method you're using.
Examples of Null Hypotheses

Null Hypothesis vs. Alternative Hypothesis

- For example, your alternative hypothesis could state a positive correlation between 2 variables while your null hypothesis states there's no relationship. If there's a negative correlation, then both hypotheses are false.

- You need additional data or evidence to show that your alternative hypothesis is correct—proving the null hypothesis false is just the first step.
- In smaller studies, sometimes it's enough to show that there's some relationship and your hypothesis could be correct—you can leave the additional proof as an open question for other researchers to tackle.
How do I test a null hypothesis?

- Group means: Compare the mean of the variable in your sample with the mean of the variable in the general population. [6] X Research source
- Group proportions: Compare the proportion of the variable in your sample with the proportion of the variable in the general population. [7] X Research source
- Correlation: Correlation analysis looks at the relationship between 2 variables—specifically, whether they tend to happen together. [8] X Research source
- Regression: Regression analysis reveals the correlation between 2 variables while also controlling for the effect of other, interrelated variables. [9] X Research source
Templates for Null Hypotheses

- Research null hypothesis: There is no difference in the mean [dependent variable] between [group 1] and [group 2].
- Research null hypothesis: The proportion of [dependent variable] in [group 1] and [group 2] is the same.
- Research null hypothesis: There is no correlation between [independent variable] and [dependent variable] in the population.
- Research null hypothesis: There is no relationship between [independent variable] and [dependent variable] in the population.
Expert Q&A

You Might Also Like

Expert Interview

Thanks for reading our article! If you’d like to learn more about physics, check out our in-depth interview with Joseph Quinones .
- ↑ https://online.stat.psu.edu/stat100/lesson/10/10.1
- ↑ https://online.stat.psu.edu/stat501/lesson/2/2.12
- ↑ https://support.minitab.com/en-us/minitab/21/help-and-how-to/statistics/basic-statistics/supporting-topics/basics/null-and-alternative-hypotheses/
- ↑ https://www.ncbi.nlm.nih.gov/pmc/articles/PMC5635437/
- ↑ https://online.stat.psu.edu/statprogram/reviews/statistical-concepts/hypothesis-testing
- ↑ https://education.arcus.chop.edu/null-hypothesis-testing/
- ↑ https://sphweb.bumc.bu.edu/otlt/mph-modules/bs/bs704_hypothesistest-means-proportions/bs704_hypothesistest-means-proportions_print.html
About This Article

- Send fan mail to authors
Reader Success Stories

Dec 3, 2022
Did this article help you?

Featured Articles

Trending Articles

Watch Articles

- Terms of Use
- Privacy Policy
- Do Not Sell or Share My Info
- Not Selling Info
Don’t miss out! Sign up for
wikiHow’s newsletter
Module 12: Linear Regression and Correlation
Hypothesis test for correlation, learning outcomes.
- Conduct a linear regression t-test using p-values and critical values and interpret the conclusion in context
The correlation coefficient, r , tells us about the strength and direction of the linear relationship between x and y . However, the reliability of the linear model also depends on how many observed data points are in the sample. We need to look at both the value of the correlation coefficient r and the sample size n , together.
We perform a hypothesis test of the “ significance of the correlation coefficient ” to decide whether the linear relationship in the sample data is strong enough to use to model the relationship in the population.
The sample data are used to compute r , the correlation coefficient for the sample. If we had data for the entire population, we could find the population correlation coefficient. But because we only have sample data, we cannot calculate the population correlation coefficient. The sample correlation coefficient, r , is our estimate of the unknown population correlation coefficient.
- The symbol for the population correlation coefficient is ρ , the Greek letter “rho.”
- ρ = population correlation coefficient (unknown)
- r = sample correlation coefficient (known; calculated from sample data)
The hypothesis test lets us decide whether the value of the population correlation coefficient ρ is “close to zero” or “significantly different from zero.” We decide this based on the sample correlation coefficient r and the sample size n .
If the test concludes that the correlation coefficient is significantly different from zero, we say that the correlation coefficient is “significant.”
- Conclusion: There is sufficient evidence to conclude that there is a significant linear relationship between x and y because the correlation coefficient is significantly different from zero.
- What the conclusion means: There is a significant linear relationship between x and y . We can use the regression line to model the linear relationship between x and y in the population.
If the test concludes that the correlation coefficient is not significantly different from zero (it is close to zero), we say that the correlation coefficient is “not significant.”
- Conclusion: “There is insufficient evidence to conclude that there is a significant linear relationship between x and y because the correlation coefficient is not significantly different from zero.”
- What the conclusion means: There is not a significant linear relationship between x and y . Therefore, we CANNOT use the regression line to model a linear relationship between x and y in the population.
- If r is significant and the scatter plot shows a linear trend, the line can be used to predict the value of y for values of x that are within the domain of observed x values.
- If r is not significant OR if the scatter plot does not show a linear trend, the line should not be used for prediction.
- If r is significant and if the scatter plot shows a linear trend, the line may NOT be appropriate or reliable for prediction OUTSIDE the domain of observed x values in the data.
Performing the Hypothesis Test
- Null Hypothesis: H 0 : ρ = 0
- Alternate Hypothesis: H a : ρ ≠ 0
What the Hypotheses Mean in Words
- Null Hypothesis H 0 : The population correlation coefficient IS NOT significantly different from zero. There IS NOT a significant linear relationship (correlation) between x and y in the population.
- Alternate Hypothesis H a : The population correlation coefficient IS significantly DIFFERENT FROM zero. There IS A SIGNIFICANT LINEAR RELATIONSHIP (correlation) between x and y in the population.
Drawing a Conclusion
There are two methods of making the decision. The two methods are equivalent and give the same result.
- Method 1: Using the p -value
- Method 2: Using a table of critical values
In this chapter of this textbook, we will always use a significance level of 5%, α = 0.05
Using the p -value method, you could choose any appropriate significance level you want; you are not limited to using α = 0.05. But the table of critical values provided in this textbook assumes that we are using a significance level of 5%, α = 0.05. (If we wanted to use a different significance level than 5% with the critical value method, we would need different tables of critical values that are not provided in this textbook).
Method 1: Using a p -value to make a decision
Using the ti-83, 83+, 84, 84+ calculator.
To calculate the p -value using LinRegTTEST:
- On the LinRegTTEST input screen, on the line prompt for β or ρ , highlight “≠ 0”
- The output screen shows the p-value on the line that reads “p =”.
- (Most computer statistical software can calculate the p -value).
If the p -value is less than the significance level ( α = 0.05)
- Decision: Reject the null hypothesis.
- Conclusion: “There is sufficient evidence to conclude that there is a significant linear relationship between x and y because the correlation coefficient is significantly different from zero.”
If the p -value is NOT less than the significance level ( α = 0.05)
- Decision: DO NOT REJECT the null hypothesis.
- Conclusion: “There is insufficient evidence to conclude that there is a significant linear relationship between x and y because the correlation coefficient is NOT significantly different from zero.”
Calculation Notes:
- You will use technology to calculate the p -value. The following describes the calculations to compute the test statistics and the p -value:
- The p -value is calculated using a t -distribution with n – 2 degrees of freedom.
- The formula for the test statistic is [latex]\displaystyle{t}=\dfrac{{{r}\sqrt{{{n}-{2}}}}}{\sqrt{{{1}-{r}^{{2}}}}}[/latex]. The value of the test statistic, t , is shown in the computer or calculator output along with the p -value. The test statistic t has the same sign as the correlation coefficient r .
- The p -value is the combined area in both tails.
Recall: ORDER OF OPERATIONS
parentheses | exponents | multiplication | division | addition | subtraction |
[latex]( \ )[/latex] | [latex]x^2[/latex] | [latex]\times \ \mathrm{or} \ \div[/latex] | [latex]+ \ \mathrm{or} \ -[/latex] |
1st find the numerator:
Step 1: Find [latex]n-2[/latex], and then take the square root.
Step 2: Multiply the value in Step 1 by [latex]r[/latex].
2nd find the denominator:
Step 3: Find the square of [latex]r[/latex], which is [latex]r[/latex] multiplied by [latex]r[/latex].
Step 4: Subtract this value from 1, [latex]1 -r^2[/latex].
Step 5: Find the square root of Step 4.
3rd take the numerator and divide by the denominator.
An alternative way to calculate the p -value (p) given by LinRegTTest is the command 2*tcdf(abs(t),10^99, n-2) in 2nd DISTR.
THIRD-EXAM vs FINAL-EXAM EXAM: p- value method
- Consider the third exam/final exam example (example 2).
- The line of best fit is: [latex]\hat{y}[/latex] = -173.51 + 4.83 x with r = 0.6631 and there are n = 11 data points.
- Can the regression line be used for prediction? Given a third exam score ( x value), can we use the line to predict the final exam score (predicted y value)?
- H 0 : ρ = 0
- H a : ρ ≠ 0
- The p -value is 0.026 (from LinRegTTest on your calculator or from computer software).
- The p -value, 0.026, is less than the significance level of α = 0.05.
- Decision: Reject the Null Hypothesis H 0
- Conclusion: There is sufficient evidence to conclude that there is a significant linear relationship between the third exam score ( x ) and the final exam score ( y ) because the correlation coefficient is significantly different from zero.
Because r is significant and the scatter plot shows a linear trend, the regression line can be used to predict final exam scores.
Method 2: Using a table of Critical Values to make a decision
The 95% Critical Values of the Sample Correlation Coefficient Table can be used to give you a good idea of whether the computed value of r is significant or not . Compare r to the appropriate critical value in the table. If r is not between the positive and negative critical values, then the correlation coefficient is significant. If r is significant, then you may want to use the line for prediction.
Suppose you computed r = 0.801 using n = 10 data points. df = n – 2 = 10 – 2 = 8. The critical values associated with df = 8 are -0.632 and + 0.632. If r < negative critical value or r > positive critical value, then r is significant. Since r = 0.801 and 0.801 > 0.632, r is significant and the line may be used for prediction. If you view this example on a number line, it will help you.

r is not significant between -0.632 and +0.632. r = 0.801 > +0.632. Therefore, r is significant.
For a given line of best fit, you computed that r = 0.6501 using n = 12 data points and the critical value is 0.576. Can the line be used for prediction? Why or why not?
If the scatter plot looks linear then, yes, the line can be used for prediction, because r > the positive critical value.
Suppose you computed r = –0.624 with 14 data points. df = 14 – 2 = 12. The critical values are –0.532 and 0.532. Since –0.624 < –0.532, r is significant and the line can be used for prediction

r = –0.624-0.532. Therefore, r is significant.
For a given line of best fit, you compute that r = 0.5204 using n = 9 data points, and the critical value is 0.666. Can the line be used for prediction? Why or why not?
No, the line cannot be used for prediction, because r < the positive critical value.
Suppose you computed r = 0.776 and n = 6. df = 6 – 2 = 4. The critical values are –0.811 and 0.811. Since –0.811 < 0.776 < 0.811, r is not significant, and the line should not be used for prediction.

–0.811 < r = 0.776 < 0.811. Therefore, r is not significant.
For a given line of best fit, you compute that r = –0.7204 using n = 8 data points, and the critical value is = 0.707. Can the line be used for prediction? Why or why not?
Yes, the line can be used for prediction, because r < the negative critical value.
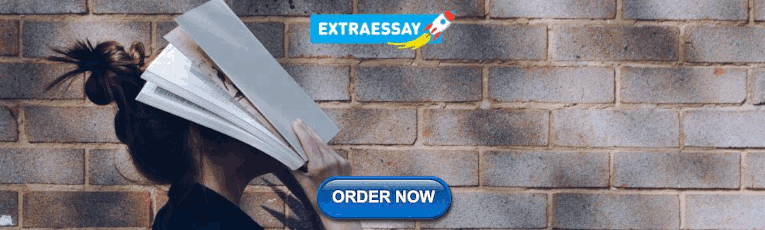
THIRD-EXAM vs FINAL-EXAM EXAMPLE: critical value method
Consider the third exam/final exam example again. The line of best fit is: [latex]\hat{y}[/latex] = –173.51+4.83 x with r = 0.6631 and there are n = 11 data points. Can the regression line be used for prediction? Given a third-exam score ( x value), can we use the line to predict the final exam score (predicted y value)?
- Use the “95% Critical Value” table for r with df = n – 2 = 11 – 2 = 9.
- The critical values are –0.602 and +0.602
- Since 0.6631 > 0.602, r is significant.
Suppose you computed the following correlation coefficients. Using the table at the end of the chapter, determine if r is significant and the line of best fit associated with each r can be used to predict a y value. If it helps, draw a number line.
- r = –0.567 and the sample size, n , is 19. The df = n – 2 = 17. The critical value is –0.456. –0.567 < –0.456 so r is significant.
- r = 0.708 and the sample size, n , is nine. The df = n – 2 = 7. The critical value is 0.666. 0.708 > 0.666 so r is significant.
- r = 0.134 and the sample size, n , is 14. The df = 14 – 2 = 12. The critical value is 0.532. 0.134 is between –0.532 and 0.532 so r is not significant.
- r = 0 and the sample size, n , is five. No matter what the dfs are, r = 0 is between the two critical values so r is not significant.
For a given line of best fit, you compute that r = 0 using n = 100 data points. Can the line be used for prediction? Why or why not?
No, the line cannot be used for prediction no matter what the sample size is.
Assumptions in Testing the Significance of the Correlation Coefficient
Testing the significance of the correlation coefficient requires that certain assumptions about the data are satisfied. The premise of this test is that the data are a sample of observed points taken from a larger population. We have not examined the entire population because it is not possible or feasible to do so. We are examining the sample to draw a conclusion about whether the linear relationship that we see between x and y in the sample data provides strong enough evidence so that we can conclude that there is a linear relationship between x and y in the population.
The regression line equation that we calculate from the sample data gives the best-fit line for our particular sample. We want to use this best-fit line for the sample as an estimate of the best-fit line for the population. Examining the scatterplot and testing the significance of the correlation coefficient helps us determine if it is appropriate to do this.
The assumptions underlying the test of significance are:
- There is a linear relationship in the population that models the average value of y for varying values of x . In other words, the expected value of y for each particular value lies on a straight line in the population. (We do not know the equation for the line for the population. Our regression line from the sample is our best estimate of this line in the population).
- The y values for any particular x value are normally distributed about the line. This implies that there are more y values scattered closer to the line than are scattered farther away. Assumption (1) implies that these normal distributions are centered on the line: the means of these normal distributions of y values lie on the line.
- The standard deviations of the population y values about the line are equal for each value of x . In other words, each of these normal distributions of y values has the same shape and spread about the line.
- The residual errors are mutually independent (no pattern).
- The data are produced from a well-designed, random sample or randomized experiment.
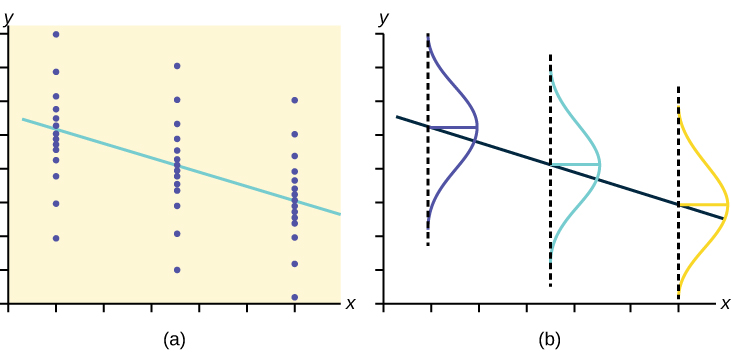
The y values for each x value are normally distributed about the line with the same standard deviation. For each x value, the mean of the y values lies on the regression line. More y values lie near the line than are scattered further away from the line.
- Provided by : Lumen Learning. License : CC BY: Attribution
- Testing the Significance of the Correlation Coefficient. Provided by : OpenStax. Located at : https://openstax.org/books/introductory-statistics/pages/12-4-testing-the-significance-of-the-correlation-coefficient . License : CC BY: Attribution . License Terms : Access for free at https://openstax.org/books/introductory-statistics/pages/1-introduction
- Introductory Statistics. Authored by : Barbara Illowsky, Susan Dean. Provided by : OpenStax. Located at : https://openstax.org/books/introductory-statistics/pages/1-introduction . License : CC BY: Attribution . License Terms : Access for free at https://openstax.org/books/introductory-statistics/pages/1-introduction
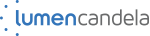
Privacy Policy
Learning Materials
- Business Studies
- Combined Science
- Computer Science
- Engineering
- English Literature
- Environmental Science
- Human Geography
- Macroeconomics
- Microeconomics
- Hypothesis Test for Correlation
Let's look at the hypothesis test for correlation, including the hypothesis test for correlation coefficient, the hypothesis test for negative correlation and the null hypothesis for correlation test.
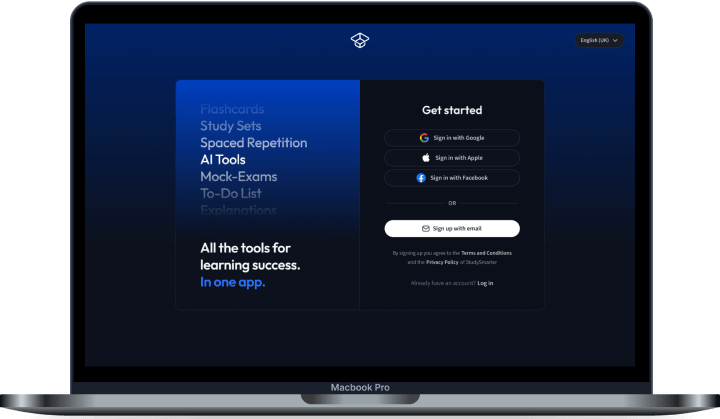
Create learning materials about Hypothesis Test for Correlation with our free learning app!
- Instand access to millions of learning materials
- Flashcards, notes, mock-exams and more
- Everything you need to ace your exams
Millions of flashcards designed to help you ace your studies
- Cell Biology
What does a PMCC, or r coefficient of 1 signify?
What does a PMCC, or r coefficient of 0 signify?
What does a PMCC, or r coefficient of -0.986 signify?
What does the null hypothesis state?
What is bivariate data?
What is the critical region?
What is the difference between a one-tailed and a two-tailed test?
Are hypotheses written in words or symbols?
What does a significance of 5% indicate?
Review generated flashcards
to start learning or create your own AI flashcards
Start learning or create your own AI flashcards
- Applied Mathematics
- Decision Maths
- Discrete Mathematics
- Logic and Functions
- Mechanics Maths
- Probability and Statistics
- Bayesian Statistics
- Bias in Experiments
- Binomial Distribution
- Binomial Hypothesis Test
- Biostatistics
- Bivariate Data
- Categorical Data Analysis
- Categorical Variables
- Causal Inference
- Central Limit Theorem
- Chi Square Test for Goodness of Fit
- Chi Square Test for Homogeneity
- Chi Square Test for Independence
- Chi-Square Distribution
- Cluster Analysis
- Combining Random Variables
- Comparing Data
- Comparing Two Means Hypothesis Testing
- Conditional Probability
- Conducting A Study
- Conducting a Survey
- Conducting an Experiment
- Confidence Interval for Population Mean
- Confidence Interval for Population Proportion
- Confidence Interval for Slope of Regression Line
- Confidence Interval for the Difference of Two Means
- Confidence Intervals
- Correlation Math
- Cox Regression
- Cumulative Distribution Function
- Cumulative Frequency
- Data Analysis
- Data Interpretation
- Decision Theory
- Degrees of Freedom
- Discrete Random Variable
- Discriminant Analysis
- Distributions
- Empirical Bayes Methods
- Empirical Rule
- Errors In Hypothesis Testing
- Estimation Theory
- Estimator Bias
- Events (Probability)
- Experimental Design
- Factor Analysis
- Frequency Polygons
- Generalization and Conclusions
- Geometric Distribution
- Geostatistics
- Hierarchical Modeling
- Hypothesis Test for Regression Slope
- Hypothesis Test of Two Population Proportions
- Hypothesis Testing
- Inference For Distributions Of Categorical Data
- Inferences in Statistics
- Item Response Theory
- Kaplan-Meier Estimate
- Kernel Density Estimation
- Large Data Set
- Lasso Regression
- Latent Variable Models
- Least Squares Linear Regression
- Linear Interpolation
- Linear Regression
- Logistic Regression
- Machine Learning
- Mann-Whitney Test
- Markov Chains
- Mean and Variance of Poisson Distributions
- Measures of Central Tendency
- Methods of Data Collection
- Mixed Models
- Multilevel Modeling
- Multivariate Analysis
- Neyman-Pearson Lemma
- Non-parametric Methods
- Normal Distribution
- Normal Distribution Hypothesis Test
- Normal Distribution Percentile
- Ordinal Regression
- Paired T-Test
- Parametric Methods
- Path Analysis
- Point Estimation
- Poisson Regression
- Principle Components Analysis
- Probability
- Probability Calculations
- Probability Density Function
- Probability Distribution
- Probability Generating Function
- Product Moment Correlation Coefficient
- Quantile Regression
- Quantitative Variables
- Random Effects Model
- Random Variables
- Randomized Block Design
- Regression Analysis
- Residual Sum of Squares
- Robust Statistics
- Sample Mean
- Sample Proportion
- Sampling Distribution
- Sampling Theory
- Scatter Graphs
- Sequential Analysis
- Single Variable Data
- Spearman's Rank Correlation
- Spearman's Rank Correlation Coefficient
- Standard Deviation
- Standard Error
- Standard Normal Distribution
- Statistical Graphs
- Statistical Inference
- Statistical Measures
- Stem and Leaf Graph
- Stochastic Processes
- Structural Equation Modeling
- Sum of Independent Random Variables
- Survey Bias
- Survival Analysis
- Survivor Function
- T-distribution
- The Power Function
- Time Series Analysis
- Transforming Random Variables
- Tree Diagram
- Two Categorical Variables
- Two Quantitative Variables
- Type I Error
- Type II Error
- Types of Data in Statistics
- Variance for Binomial Distribution
- Venn Diagrams
- Wilcoxon Test
- Zero-Inflated Models
- Theoretical and Mathematical Physics
What is the hypothesis test for correlation coefficient?
When given a sample of bivariate data (data which include two variables), it is possible to calculate how linearly correlated the data are, using a correlation coefficient.
The product moment correlation coefficient (PMCC) describes the extent to which one variable correlates with another. In other words, the strength of the correlation between two variables. The PMCC for a sample of data is denoted by r , while the PMCC for a population is denoted by ρ.
The PMCC is limited to values between -1 and 1 (included).
If r = 1 , there is a perfect positive linear correlation. All points lie on a straight line with a positive gradient, and the higher one of the variables is, the higher the other.
If r = 0 , there is no linear correlation between the variables.
If r = - 1 , there is a perfect negative linear correlation. All points lie on a straight line with a negative gradient, and the higher one of the variables is, the lower the other.
Correlation is not equivalent to causation, but a PMCC close to 1 or -1 can indicate that there is a higher likelihood that two variables are related.

The PMCC should be able to be calculated using a graphics calculator by finding the regression line of y on x, and hence finding r (this value is automatically calculated by the calculator), or by using the formula r = S x y S x x S y y , which is in the formula booklet. The closer r is to 1 or -1, the stronger the correlation between the variables, and hence the more closely associated the variables are. You need to be able to carry out hypothesis tests on a sample of bivariate data to determine if we can establish a linear relationship for an entire population. By calculating the PMCC, and comparing it to a critical value, it is possible to determine the likelihood of a linear relationship existing.
What is the hypothesis test for negative correlation?
To conduct a hypothesis test, a number of keywords must be understood:
Null hypothesis ( H 0 ) : the hypothesis assumed to be correct until proven otherwise
Alternative hypothesis ( H 1 ) : the conclusion made if H 0 is rejected.
Hypothesis test: a mathematical procedure to examine a value of a population parameter proposed by the null hypothesis compared to the alternative hypothesis.
Test statistic: is calculated from the sample and tested in cumulative probability tables or with the normal distribution as the last part of the significance test.
Critical region: the range of values that lead to the rejection of the null hypothesis.
Significance level: the actual significance level is the probability of rejecting H 0 when it is in fact true.
The null hypothesis is also known as the 'working hypothesis'. It is what we assume to be true for the purpose of the test, or until proven otherwise.
The alternative hypothesis is what is concluded if the null hypothesis is rejected. It also determines whether the test is one-tailed or two-tailed.
A one-tailed test allows for the possibility of an effect in one direction, while two-tailed tests allow for the possibility of an effect in two directions, in other words, both in the positive and the negative directions. Method: A series of steps must be followed to determine the existence of a linear relationship between 2 variables. 1 . Write down the null and alternative hypotheses ( H 0 a n d H 1 ). The null hypothesis is always ρ = 0 , while the alternative hypothesis depends on what is asked in the question. Both hypotheses must be stated in symbols only (not in words).
2 . Using a calculator, work out the value of the PMCC of the sample data, r .
3 . Use the significance level and sample size to figure out the critical value. This can be found in the PMCC table in the formula booklet.
4 . Take the absolute value of the PMCC and r , and compare these to the critical value. If the absolute value is greater than the critical value, the null hypothesis should be rejected. Otherwise, the null hypothesis should be accepted.
5 . Write a full conclusion in the context of the question. The conclusion should be stated in full: both in statistical language and in words reflecting the context of the question. A negative correlation signifies that the alternative hypothesis is rejected: the lack of one variable correlates with a stronger presence of the other variable, whereas, when there is a positive correlation, the presence of one variable correlates with the presence of the other.
How to interpret results based on the null hypothesis
From the observed results (test statistic), a decision must be made, determining whether to reject the null hypothesis or not.

Both the one-tailed and two-tailed tests are shown at the 5% level of significance. However, the 5% is distributed in both the positive and negative side in the two-tailed test, and solely on the positive side in the one-tailed test.
From the null hypothesis, the result could lie anywhere on the graph. If the observed result lies in the shaded area, the test statistic is significant at 5%, in other words, we reject H 0 . Therefore, H 0 could actually be true but it is still rejected. Hence, the significance level, 5%, is the probability that H 0 is rejected even though it is true, in other words, the probability that H 0 is incorrectly rejected. When H 0 is rejected, H 1 (the alternative hypothesis) is used to write the conclusion.
We can define the null and alternative hypotheses for one-tailed and two-tailed tests:
For a one-tailed test:
- H 0 : ρ = 0 : H 1 ρ > 0 o r
- H 0 : ρ = 0 : H 1 ρ < 0
For a two-tailed test:
- H 0 : ρ = 0 : H 1 ρ ≠ 0
Let us look at an example of testing for correlation.
12 students sat two biology tests: one was theoretical and the other was practical. The results are shown in the table.
a) Find the product moment correlation coefficient for this data, to 3 significant figures.
b) A teacher claims that students who do well in the theoretical test tend to do well in the practical test. Test this claim at the 0.05 level of significance, clearly stating your hypotheses.
a) Using a calculator, we find the PMCC (enter the data into two lists and calculate the regression line. the PMCC will appear). r = 0.935 to 3 sign. figures
b) We are testing for a positive correlation, since the claim is that a higher score in the theoretical test is associated with a higher score in the practical test. We will now use the five steps we previously looked at.
1. State the null and alternative hypotheses. H 0 : ρ = 0 and H 1 : ρ > 0
2. Calculate the PMCC. From part a), r = 0.935
3. Figure out the critical value from the sample size and significance level. The sample size, n , is 12. The significance level is 5%. The hypothesis is one-tailed since we are only testing for positive correlation. Using the table from the formula booklet, the critical value is shown to be cv = 0.4973
4. The absolute value of the PMCC is 0.935, which is larger than 0.4973. Since the PMCC is larger than the critical value at the 5% level of significance, we can reach a conclusion.
5. Since the PMCC is larger than the critical value, we choose to reject the null hypothesis. We can conclude that there is significant evidence to support the claim that students who do well in the theoretical biology test also tend to do well in the practical biology test.
Let us look at a second example.
A tetrahedral die (four faces) is rolled 40 times and 6 'ones' are observed. Is there any evidence at the 10% level that the probability of a score of 1 is less than a quarter?
The expected mean is 10 = 40 × 1 4 . The question asks whether the observed result (test statistic 6 is unusually low.
We now follow the same series of steps.
1. State the null and alternative hypotheses. H 0 : ρ = 0 and H 1 : ρ <0.25
2. We cannot calculate the PMCC since we are only given data for the frequency of 'ones'.
3. A one-tailed test is required ( ρ < 0.25) at the 10% significance level. We can convert this to a binomial distribution in which X is the number of 'ones' so X ~ B ( 40 , 0 . 25 ) , we then use the cumulative binomial tables. The observed value is X = 6. To P ( X ≤ 6 ' o n e s ' i n 40 r o l l s ) = 0 . 0962 .
4. Since 0.0962, or 9.62% <10%, the observed result lies in the critical region.
5. We reject and accept the alternative hypothesis. We conclude that there is evidence to show that the probability of rolling a 'one' is less than 1 4
Hypothesis Test for Correlation - Key takeaways
- The Product Moment Correlation Coefficient (PMCC), or r , is a measure of how strongly related 2 variables are. It ranges between -1 and 1, indicating the strength of a correlation.
- The closer r is to 1 or -1 the stronger the (positive or negative) correlation between two variables.
- The null hypothesis is the hypothesis that is assumed to be correct until proven otherwise. It states that there is no correlation between the variables.
- The alternative hypothesis is that which is accepted when the null hypothesis is rejected. It can be either one-tailed (looking at one outcome) or two-tailed (looking at both outcomes – positive and negative).
- If the significance level is 5%, this means that there is a 5% chance that the null hypothesis is incorrectly rejected.
Images One-tailed test: https://en.wikipedia.org/w/index.php?curid=35569621
Flashcards in Hypothesis Test for Correlation 9
There is a perfect positive linear correlation between 2 variables
There is no correlation between 2 variables
There is a strong negative linear correlation between the 2 variables
p = 0 (there is no correlation between the variables)
Data which includes 2 variables
The range of values which lead to the rejection of the null hypothesis
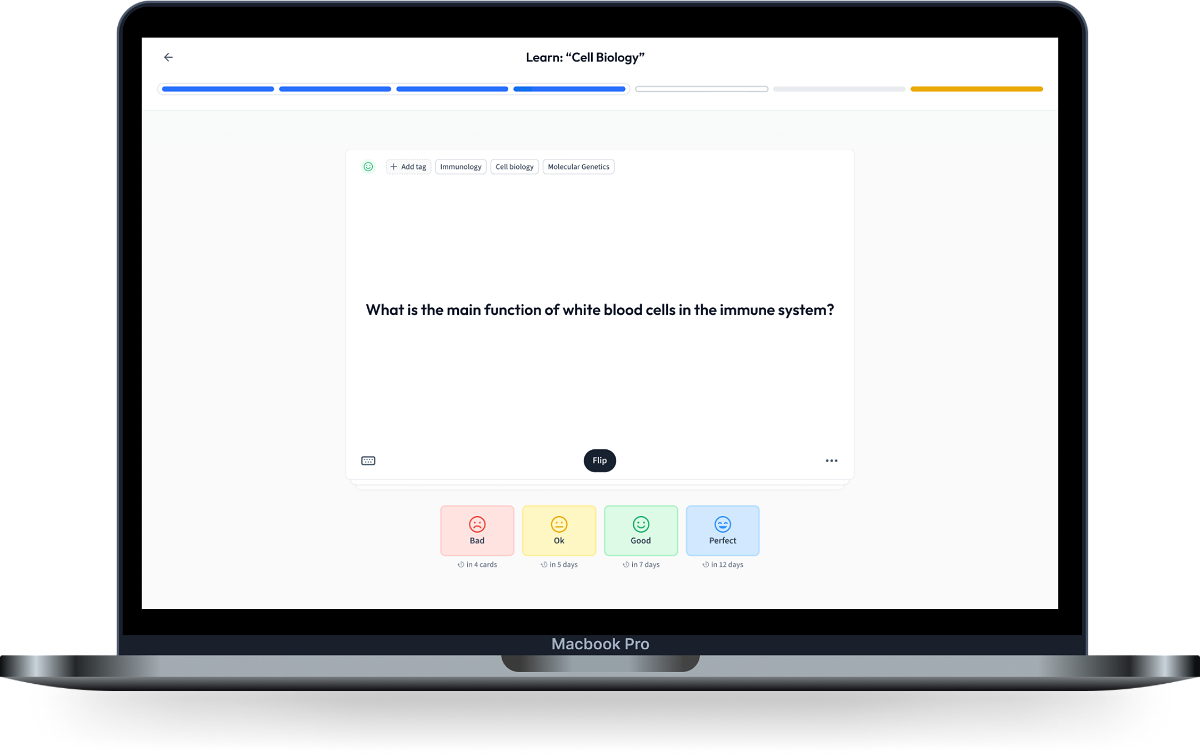
Learn with 9 Hypothesis Test for Correlation flashcards in the free StudySmarter app
We have 14,000 flashcards about Dynamic Landscapes.
Already have an account? Log in
Frequently Asked Questions about Hypothesis Test for Correlation
Is the Pearson correlation a hypothesis test?
Yes. The Pearson correlation produces a PMCC value, or r value, which indicates the strength of the relationship between two variables.
Can we test a hypothesis with correlation?
Yes. Correlation is not equivalent to causation, however we can test hypotheses to determine whether a correlation (or association) exists between two variables.
How do you set up the hypothesis test for correlation?
You need a null (p = 0) and alternative hypothesis. The PMCC, or r value must be calculated, based on the sample data. Based on the significance level and sample size, the critical value can be worked out from a table of values in the formula booklet. Finally the r value and critical value can be compared to determine which hypothesis is accepted.
Discover learning materials with the free StudySmarter app
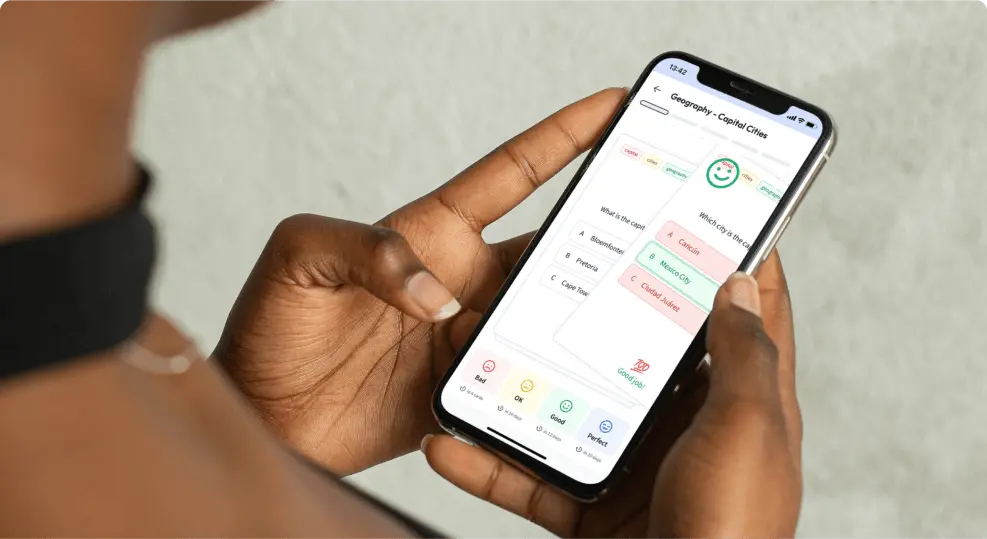
About StudySmarter
StudySmarter is a globally recognized educational technology company, offering a holistic learning platform designed for students of all ages and educational levels. Our platform provides learning support for a wide range of subjects, including STEM, Social Sciences, and Languages and also helps students to successfully master various tests and exams worldwide, such as GCSE, A Level, SAT, ACT, Abitur, and more. We offer an extensive library of learning materials, including interactive flashcards, comprehensive textbook solutions, and detailed explanations. The cutting-edge technology and tools we provide help students create their own learning materials. StudySmarter’s content is not only expert-verified but also regularly updated to ensure accuracy and relevance.
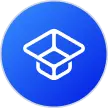
StudySmarter Editorial Team
Team Math Teachers
- 9 minutes reading time
- Checked by StudySmarter Editorial Team
Study anywhere. Anytime.Across all devices.
Create a free account to save this explanation..
Save explanations to your personalised space and access them anytime, anywhere!
By signing up, you agree to the Terms and Conditions and the Privacy Policy of StudySmarter.
Sign up to highlight and take notes. It’s 100% free.
Join over 22 million students in learning with our StudySmarter App
The first learning app that truly has everything you need to ace your exams in one place
- Flashcards & Quizzes
- AI Study Assistant
- Study Planner
- Smart Note-Taking
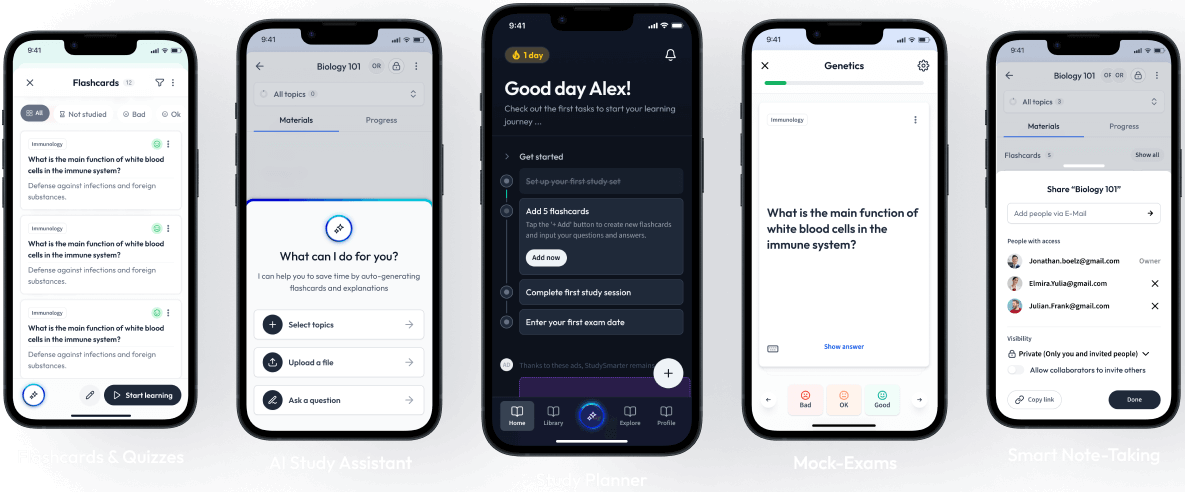
9.1 Null and Alternative Hypotheses
The actual test begins by considering two hypotheses . They are called the null hypothesis and the alternative hypothesis . These hypotheses contain opposing viewpoints.
H 0 , the — null hypothesis: a statement of no difference between sample means or proportions or no difference between a sample mean or proportion and a population mean or proportion. In other words, the difference equals 0.
H a —, the alternative hypothesis: a claim about the population that is contradictory to H 0 and what we conclude when we reject H 0 .
Since the null and alternative hypotheses are contradictory, you must examine evidence to decide if you have enough evidence to reject the null hypothesis or not. The evidence is in the form of sample data.
After you have determined which hypothesis the sample supports, you make a decision. There are two options for a decision. They are reject H 0 if the sample information favors the alternative hypothesis or do not reject H 0 or decline to reject H 0 if the sample information is insufficient to reject the null hypothesis.
Mathematical Symbols Used in H 0 and H a :
equal (=) | not equal (≠) greater than (>) less than (<) |
greater than or equal to (≥) | less than (<) |
less than or equal to (≤) | more than (>) |
H 0 always has a symbol with an equal in it. H a never has a symbol with an equal in it. The choice of symbol depends on the wording of the hypothesis test. However, be aware that many researchers use = in the null hypothesis, even with > or < as the symbol in the alternative hypothesis. This practice is acceptable because we only make the decision to reject or not reject the null hypothesis.
Example 9.1
H 0 : No more than 30 percent of the registered voters in Santa Clara County voted in the primary election. p ≤ 30 H a : More than 30 percent of the registered voters in Santa Clara County voted in the primary election. p > 30
A medical trial is conducted to test whether or not a new medicine reduces cholesterol by 25 percent. State the null and alternative hypotheses.
Example 9.2
We want to test whether the mean GPA of students in American colleges is different from 2.0 (out of 4.0). The null and alternative hypotheses are the following: H 0 : μ = 2.0 H a : μ ≠ 2.0
We want to test whether the mean height of eighth graders is 66 inches. State the null and alternative hypotheses. Fill in the correct symbol (=, ≠, ≥, <, ≤, >) for the null and alternative hypotheses.
- H 0 : μ __ 66
- H a : μ __ 66
Example 9.3
We want to test if college students take fewer than five years to graduate from college, on the average. The null and alternative hypotheses are the following: H 0 : μ ≥ 5 H a : μ < 5
We want to test if it takes fewer than 45 minutes to teach a lesson plan. State the null and alternative hypotheses. Fill in the correct symbol ( =, ≠, ≥, <, ≤, >) for the null and alternative hypotheses.
- H 0 : μ __ 45
- H a : μ __ 45
Example 9.4
An article on school standards stated that about half of all students in France, Germany, and Israel take advanced placement exams and a third of the students pass. The same article stated that 6.6 percent of U.S. students take advanced placement exams and 4.4 percent pass. Test if the percentage of U.S. students who take advanced placement exams is more than 6.6 percent. State the null and alternative hypotheses. H 0 : p ≤ 0.066 H a : p > 0.066
On a state driver’s test, about 40 percent pass the test on the first try. We want to test if more than 40 percent pass on the first try. Fill in the correct symbol (=, ≠, ≥, <, ≤, >) for the null and alternative hypotheses.
- H 0 : p __ 0.40
- H a : p __ 0.40
Collaborative Exercise
Bring to class a newspaper, some news magazines, and some internet articles. In groups, find articles from which your group can write null and alternative hypotheses. Discuss your hypotheses with the rest of the class.
This book may not be used in the training of large language models or otherwise be ingested into large language models or generative AI offerings without OpenStax's permission.
Want to cite, share, or modify this book? This book uses the Creative Commons Attribution License and you must attribute Texas Education Agency (TEA). The original material is available at: https://www.texasgateway.org/book/tea-statistics . Changes were made to the original material, including updates to art, structure, and other content updates.
Access for free at https://openstax.org/books/statistics/pages/1-introduction
- Authors: Barbara Illowsky, Susan Dean
- Publisher/website: OpenStax
- Book title: Statistics
- Publication date: Mar 27, 2020
- Location: Houston, Texas
- Book URL: https://openstax.org/books/statistics/pages/1-introduction
- Section URL: https://openstax.org/books/statistics/pages/9-1-null-and-alternative-hypotheses
© Apr 16, 2024 Texas Education Agency (TEA). The OpenStax name, OpenStax logo, OpenStax book covers, OpenStax CNX name, and OpenStax CNX logo are not subject to the Creative Commons license and may not be reproduced without the prior and express written consent of Rice University.
Stack Exchange Network
Stack Exchange network consists of 183 Q&A communities including Stack Overflow , the largest, most trusted online community for developers to learn, share their knowledge, and build their careers.
Q&A for work
Connect and share knowledge within a single location that is structured and easy to search.
Hypothesis testing for a correlation that is zero or negative
I would like to test for a correlation that is zero or negative using the following hypothesis test: $H_0: p>0$ (Null hypothesis: the correlation is positive) $H_A: p\le0$ (Alternative hypothesis: the correlation is zero or negative)
Since this is different from the usual correlation statistical hypothesis tests, $(H_0:p=0, H_A:p\ne0)$, I am having difficulty in figuring out the formulas.
How would I perform the calculations for this hypothesis test? (Of course the calculations for $r,\ r^2,\ SS_x,\ SS_y,\ n,$ etc. are all known.) Thank you in advance for your time!
- hypothesis-testing
- correlation

- 2 $\begingroup$ The difficulty is having the equality in the alternative. How is one to find the distribution of the test statistic under the null? (Can you explain more about the underlying situation?) $\endgroup$ – Glen_b Commented Feb 10, 2014 at 21:26
- $\begingroup$ Would it be possible to inverse it and calculate and report the p-value for the TypeII error? H0: p<=0 (Null hypothesis: the correlation is zero or negative) HA: p>0 (Alternative hypothesis: the correlation is positive) $\endgroup$ – user39947 Commented Feb 10, 2014 at 21:34
- $\begingroup$ The situation is to demonstrate that a certain action (treatment) is not providing any benefit (not a positive correlation). For the moment we don't care to distiguish if is has no effect or a negative effect. $\endgroup$ – user39947 Commented Feb 10, 2014 at 21:39
- 1 $\begingroup$ I don't understand what you mean by the phrase "report the p-value for the TypeII error". The usual approach to the problem you have would be to test against the alternative that it does have a positive effect; failure to reject doesn't prove 'no positive effect', but it does in does show an absence of evidence for it. $\endgroup$ – Glen_b Commented Feb 10, 2014 at 21:56
- 3 $\begingroup$ It might be better to simply look at a confidence interval for the effect (even two-sided), and to discuss the conclusions in the light of that interval. You may get less tangled up in the hypothesis-testing logic. An alternative would be to be to begin reviewing the way hypothesis tests actually work (I don't mean the hand-wavy explanations, I mean the mathematics). $\endgroup$ – Glen_b Commented Feb 10, 2014 at 22:13
3 Answers 3
Strange that no direct answer to the original question has been given (even though @Nick Stauner and @Glen_b nicely elaborated on possibly superior alternatives). The wikipedia article discusses various methods, including the following, which is probably the most direct answer.
A one-sided hypothesis test on a correlation can be performed via t as a test statistic. Here,
t $= r\sqrt\frac{{n-2}}{1-r^2}$
with the critical value found via $t_{\alpha,n-2}$ (in the more common two-sided case, only $\alpha$ is changed). So for your $H_0$ that r is smaller than 0, the test rejects if the t resulting from plugging your n and r into the above formula is smaller than the critical value determined by your n and desired $\alpha$. (Admittedly, even this does not precisely answer the question in the sense that a correlation of exactly 0 is filed on the wrong side.)
Alternatively, a permutation test can be performed (see the wiki article).
- 1 $\begingroup$ I was just about to edit my answer to include this following @whuber's comment :) IIRC, the fact that the zero was "filed on the wrong side" threw me off initially...However, I see the OP wasn't all that adamant about designing the hypotheses that way . $\endgroup$ – Nick Stauner Commented Jul 21, 2014 at 18:36
You might achieve what you're really after (if it's not exactly what you've asked, which is interesting in its own right; +1 and welcome to CV!) rather simply by fitting a confidence interval (CI) around the correlation (I see @Glen_b suggested this in a comment too). If your correlation is significantly negative, a 95% CI would exclude positive values (and zero) with 95% confidence, which is usually enough for many statistical applications (e.g., in the social sciences, from whence I come brandishing a PhD). See also: When are confidence intervals useful?
I don't know if it's legit to just keep increasing (or decreasing) your confidence levels until your upper bound exceeds zero, but I'm curious enough myself that I'll offer this idea, risk a little rep, and eagerly await any critical comments the community might have for us. I.e., I don't see why you couldn't just take the confidence level at which your correlation estimate's CI touches zero as your estimate of $1-p$ for a test of whether your estimate is on the proper side of zero, but also below the other, more extreme bound ...which means I still haven't answered your question exactly. Still, even if your estimate is above zero, you could calculate the level of confidence with which you can say future samples from the same distribution would exhibit correlations that are also above zero and below the upper bound of your CI ...
This idea is due in part to my general preference for CIs over significance tests, which itself is due partly to a recent book (Cumming, 2012) I haven't actually read, to be honest—I've heard some pretty credible praise from those who have though—enough to recommend it myself, whether that's wise or otherwise. Speaking of "credible", if you like the CI idea, you might also consider calculating credible intervals —the Bayesian approach to estimating the probability given the fixed data of a random population parameter value being within the interval, as opposed to the CI's probability of the random data given a fixed population parameter...but I'm no Bayesian (yet), so I can't speak to that, or even be certain that I've described the credible interval interpretation with precise accuracy. You may prefer to see these questions:
- Possible dupe of ^: What does a confidence interval (vs. a credible interval) actually express?
- Interpreting a confidence interval.
- Confidence intervals when using Bayes' theorem
- What's the difference between a confidence interval and a credible interval?
- Should I report credible intervals instead of confidence intervals?
- Are there any examples where Bayesian credible intervals are obviously inferior to frequentist confidence intervals
As you can see, there's a lot of confusion about these matters, and many ways of explaining them.
Cumming, G. (2012). Understanding the new statistics: Effect sizes, confidence intervals, and meta-analysis . New York: Routledge.
- 1 $\begingroup$ These are good remarks, but they seem never to address the question itself, which comes down to making a one-tailed versus a two-tailed test. $\endgroup$ – whuber ♦ Commented Jul 21, 2014 at 15:10
- 1 $\begingroup$ Yeah, it wasn't an answer regarding how to perform a one-sided NHST; it's about how to use a CI to (potentially) conclude something similar but upper-bounded as well. I didn't know the direct answer off the top of my head, and given the enthusiasm for confidence intervals that I expressed here, I hadn't felt the need to look up a direct answer. I suppose it was easy enough though, and @jona just filled it in for us. $\endgroup$ – Nick Stauner Commented Jul 21, 2014 at 18:38
The simplest way to do so (for Pearson correlation) is to use Fisher's z-transformation.
Let r be the correlation in question.
Let n be the sample size used to acquire the correlation. tanh is the hyperbolic tangent atanh or $\tanh^{-1}$ is the inverse hyperbolic tangent .
Let z = atanh(r) , then z is normally distributed with variance $\frac{1}{n-3}$`
Using this, you can construct a confidence interval
$ C.I.(\rho) = \tanh\left(\tanh^{-1}(\rho) \pm q \cdot \frac{1}{\sqrt{n-3}}\right) $, where $q$ is the value that describes the level of confidence you want (i.e., the value you would read from a normal distribution table (e.g., 1.96 for 95% confidence)),
If zero is in the confidence interval, then you would fail to reject the null hypothesis that the correlation is zero. Also, note that you cannot use this for correlations of $\pm 1$ because if they are one for data that is truly continuous, then you only need 3 data points to determine that.
For one-sided values, simply use the z-score you'd use for a 1-sided p-value for it, and then transform it back and see if your correlation is within the range of that interval.
Edit: You can use a 1-sided test using the same values. Also, I changed sample values $r$ to theoretical values $\rho$, since that's a more appropriate use of confidence intervals.
Source: http://en.wikipedia.org/wiki/Fisher_transformation
- 3 $\begingroup$ Although this is a good (standard) approach, you describe a two-tailed test rather than the one-tailed test requested in the question. $\endgroup$ – whuber ♦ Commented Jul 21, 2014 at 19:58
- $\begingroup$ I believe you can use the z-score and compute the p-value from $-\infty$, but yes, you are correct. $\endgroup$ – Max Candocia Commented Jul 21, 2014 at 21:52
- 1 $\begingroup$ Re the edit: unsophisticated readers may get the wrong idea. I think it's important to be clear and precise about how the z-score is related to the desired confidence. (For a given level of confidence, the z-scores for the one-sided interval are based on different quantiles than the z-scores for the two-sided interval.) An explicit account of that will reveal the difference between the two-sided test and the one-sided test sought by the O.P. $\endgroup$ – whuber ♦ Commented Jul 21, 2014 at 22:03
Your Answer
Sign up or log in, post as a guest.
Required, but never shown
By clicking “Post Your Answer”, you agree to our terms of service and acknowledge you have read our privacy policy .
Not the answer you're looking for? Browse other questions tagged hypothesis-testing correlation or ask your own question .
- Featured on Meta
- We've made changes to our Terms of Service & Privacy Policy - July 2024
- Bringing clarity to status tag usage on meta sites
Hot Network Questions
- Are there any theoretical reasons why we cannot measure the position of a particle with zero error?
- How do I safely remove a mystery cast iron pipe in my basement?
- What can I do when someone else is literally duplicating my PhD work?
- My visit is for two weeks but my host bought insurance for two months is it okay
- Is this explanation of chassis grounding correct?
- Can a rope thrower act as a propulsion method for land based craft?
- What is the significance of bringing the door to Nippur in the Epic of Gilgamesh?
- Do metal objects attract lightning?
- My school wants me to download an SSL certificate to connect to WiFi. Can I just avoid doing anything private while on the WiFi?
- What is the difference between using a resistor or a capacitor as current limiter?
- Recovering data from a phone that won't boot
- Why are most big lakes in North America aligned?
- Personal Bakashot in Shema Koleinu or Elokai
- What is this strengthening dent called in a sheet metal part?
- Is it possible to create a board position where White must make the move that leads to stalemating Black to avoid Black stalemating White?
- Why do National Geographic and Discovery Channel broadcast fake or pseudoscientific programs?
- What is the spiritual difference between hungering and thirsting? (Matthew 5:6)
- How can I draw water level in a cylinder like this?
- Can a 2-sphere be squashed flat?
- Is it advisable to contact faculty members at U.S. universities prior to submitting a PhD application?
- Are quantum states like the W, Bell, GHZ, and Dicke state actually used in quantum computing research?
- Routing radiused edges between nodes in TikZ
- Is "using active record pattern" a reason to inherit from standard container (eg:vector)?
- What did the Ancient Greeks think the stars were?
Thank you for visiting nature.com. You are using a browser version with limited support for CSS. To obtain the best experience, we recommend you use a more up to date browser (or turn off compatibility mode in Internet Explorer). In the meantime, to ensure continued support, we are displaying the site without styles and JavaScript.
- View all journals
- Explore content
- About the journal
- Publish with us
- Sign up for alerts
- Published: 26 August 2024
Neural populations in the language network differ in the size of their temporal receptive windows
- Tamar I. Regev ORCID: orcid.org/0000-0003-0639-0890 1 , 2 na1 ,
- Colton Casto ORCID: orcid.org/0000-0001-6966-1470 1 , 2 , 3 , 4 na1 ,
- Eghbal A. Hosseini 1 , 2 ,
- Markus Adamek ORCID: orcid.org/0000-0001-8519-9212 5 , 6 ,
- Anthony L. Ritaccio 7 ,
- Jon T. Willie ORCID: orcid.org/0000-0001-9565-4338 5 , 6 ,
- Peter Brunner ORCID: orcid.org/0000-0002-2588-2754 5 , 6 , 8 &
- Evelina Fedorenko ORCID: orcid.org/0000-0003-3823-514X 1 , 2 , 3
Nature Human Behaviour ( 2024 ) Cite this article
186 Accesses
49 Altmetric
Metrics details
Despite long knowing what brain areas support language comprehension, our knowledge of the neural computations that these frontal and temporal regions implement remains limited. One important unresolved question concerns functional differences among the neural populations that comprise the language network. Here we leveraged the high spatiotemporal resolution of human intracranial recordings ( n = 22) to examine responses to sentences and linguistically degraded conditions. We discovered three response profiles that differ in their temporal dynamics. These profiles appear to reflect different temporal receptive windows, with average windows of about 1, 4 and 6 words, respectively. Neural populations exhibiting these profiles are interleaved across the language network, which suggests that all language regions have direct access to distinct, multiscale representations of linguistic input—a property that may be critical for the efficiency and robustness of language processing.
This is a preview of subscription content, access via your institution
Access options
Access Nature and 54 other Nature Portfolio journals
Get Nature+, our best-value online-access subscription
24,99 € / 30 days
cancel any time
Subscribe to this journal
Receive 12 digital issues and online access to articles
111,21 € per year
only 9,27 € per issue
Buy this article
- Purchase on SpringerLink
- Instant access to full article PDF
Prices may be subject to local taxes which are calculated during checkout
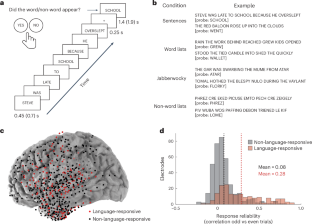
Similar content being viewed by others
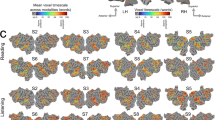
The cortical representation of language timescales is shared between reading and listening
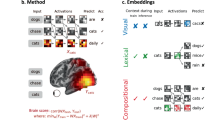
Brains and algorithms partially converge in natural language processing
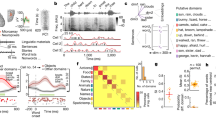
Semantic encoding during language comprehension at single-cell resolution
Data availability.
Preprocessed data, all stimuli and statistical results, as well as selected additional analyses are available on OSF at https://osf.io/xfbr8/ (ref. 37 ). Raw data may be provided upon request to the corresponding authors and institutional approval of a data-sharing agreement.
Code availability
Code used to conduct analyses and generate figures from the preprocessed data is available publicly on GitHub at https://github.com/coltoncasto/ecog_clustering_PUBLIC (ref. 93 ). The VERA software suite used to perform electrode localization can also be found on GitHub at https://github.com/neurotechcenter/VERA (ref. 82 ).
Fedorenko, E., Hsieh, P. J., Nieto-Castañón, A., Whitfield-Gabrieli, S. & Kanwisher, N. New method for fMRI investigations of language: defining ROIs functionally in individual subjects. J. Neurophysiol. 104 , 1177–1194 (2010).
Article PubMed PubMed Central Google Scholar
Pallier, C., Devauchelle, A. D. & Dehaene, S. Cortical representation of the constituent structure of sentences. Proc. Natl Acad. Sci. USA 108 , 2522–2527 (2011).
Article CAS PubMed PubMed Central Google Scholar
Regev, M., Honey, C. J., Simony, E. & Hasson, U. Selective and invariant neural responses to spoken and written narratives. J. Neurosci. 33 , 15978–15988 (2013).
Scott, T. L., Gallée, J. & Fedorenko, E. A new fun and robust version of an fMRI localizer for the frontotemporal language system. Cogn. Neurosci. 8 , 167–176 (2017).
Article PubMed Google Scholar
Diachek, E., Blank, I., Siegelman, M., Affourtit, J. & Fedorenko, E. The domain-general multiple demand (MD) network does not support core aspects of language comprehension: a large-scale fMRI investigation. J. Neurosci. 40 , 4536–4550 (2020).
Malik-Moraleda, S. et al. An investigation across 45 languages and 12 language families reveals a universal language network. Nat. Neurosci. 25 , 1014–1019 (2022).
Fedorenko, E., Behr, M. K. & Kanwisher, N. Functional specificity for high-level linguistic processing in the human brain. Proc. Natl Acad. Sci. USA 108 , 16428–16433 (2011).
Monti, M. M., Parsons, L. M. & Osherson, D. N. Thought beyond language: neural dissociation of algebra and natural language. Psychol. Sci. 23 , 914–922 (2012).
Deen, B., Koldewyn, K., Kanwisher, N. & Saxe, R. Functional organization of social perception and cognition in the superior temporal sulcus. Cereb. Cortex 25 , 4596–4609 (2015).
Ivanova, A. A. et al. The language network is recruited but not required for nonverbal event semantics. Neurobiol. Lang. 2 , 176–201 (2021).
Article Google Scholar
Chen, X. et al. The human language system, including its inferior frontal component in “Broca’s area,” does not support music perception. Cereb. Cortex 33 , 7904–7929 (2023).
Fedorenko, E., Ivanova, A. A. & Regev, T. I. The language network as a natural kind within the broader landscape of the human brain. Nat. Rev. Neurosci. 25 , 289–312 (2024).
Article CAS PubMed Google Scholar
Okada, K. & Hickok, G. Identification of lexical-phonological networks in the superior temporal sulcus using functional magnetic resonance imaging. Neuroreport 17 , 1293–1296 (2006).
Graves, W. W., Grabowski, T. J., Mehta, S. & Gupta, P. The left posterior superior temporal gyrus participates specifically in accessing lexical phonology. J. Cogn. Neurosci. 20 , 1698–1710 (2008).
DeWitt, I. & Rauschecker, J. P. Phoneme and word recognition in the auditory ventral stream. Proc. Natl Acad. Sci. USA 109 , E505–E514 (2012).
Price, C. J., Moore, C. J., Humphreys, G. W. & Wise, R. J. S. Segregating semantic from phonological processes during reading. J. Cogn. Neurosci. 9 , 727–733 (1997).
Mesulam, M. M. et al. Words and objects at the tip of the left temporal lobe in primary progressive aphasia. Brain 136 , 601–618 (2013).
Friederici, A. D. The brain basis of language processing: from structure to function. Physiol. Rev. 91 , 1357–1392 (2011).
Hagoort, P. On Broca, brain, and binding: a new framework. Trends Cogn. Sci. 9 , 416–423 (2005).
Grodzinsky, Y. & Santi, A. The battle for Broca’s region. Trends Cogn. Sci. 12 , 474–480 (2008).
Matchin, W. & Hickok, G. The cortical organization of syntax. Cereb. Cortex 30 , 1481–1498 (2020).
Fedorenko, E., Blank, I. A., Siegelman, M. & Mineroff, Z. Lack of selectivity for syntax relative to word meanings throughout the language network. Cognition 203 , 104348 (2020).
Bautista, A. & Wilson, S. M. Neural responses to grammatically and lexically degraded speech. Lang. Cogn. Neurosci. 31 , 567–574 (2016).
Anderson, A. J. et al. Deep artificial neural networks reveal a distributed cortical network encoding propositional sentence-level meaning. J. Neurosci. 41 , 4100–4119 (2021).
Regev, T. I. et al. High-level language brain regions process sublexical regularities. Cereb. Cortex 34 , bhae077 (2024).
Mukamel, R. & Fried, I. Human intracranial recordings and cognitive neuroscience. Annu. Rev. Psychol. 63 , 511–537 (2011).
Fedorenko, E. et al. Neural correlate of the construction of sentence meaning. Proc. Natl Acad. Sci. USA 113 , E6256–E6262 (2016).
Nelson, M. J. et al. Neurophysiological dynamics of phrase-structure building during sentence processing. Proc. Natl Acad. Sci. USA 114 , E3669–E3678 (2017).
Woolnough, O. et al. Spatiotemporally distributed frontotemporal networks for sentence reading. Proc. Natl Acad. Sci. USA 120 , e2300252120 (2023).
Desbordes, T. et al. Dimensionality and ramping: signatures of sentence integration in the dynamics of brains and deep language models. J. Neurosci. 43 , 5350–5364 (2023).
Goldstein, A. et al. Shared computational principles for language processing in humans and deep language models. Nat. Neurosci. 25 , 369–380 (2022).
Lerner, Y., Honey, C. J., Silbert, L. J. & Hasson, U. Topographic mapping of a hierarchy of temporal receptive windows using a narrated story. J. Neurosci. 31 , 2906–2915 (2011).
Blank, I. A. & Fedorenko, E. No evidence for differences among language regions in their temporal receptive windows. Neuroimage 219 , 116925 (2020).
Jain, S. et al. Interpretable multi-timescale models for predicting fMRI responses to continuous natural speech. In NeurIPS Proc. Advances in Neural Information Processing Systems 33 (NeurIPS 2020) (eds Larochelle, H. et al.) 1–12 (NeurIPS, 2020).
Fedorenko, E., Nieto-Castañon, A. & Kanwisher, N. Lexical and syntactic representations in the brain: an fMRI investigation with multi-voxel pattern analyses. Neuropsychologia 50 , 499–513 (2012).
Shain, C. et al. Distributed sensitivity to syntax and semantics throughout the human language network. J. Cogn. Neurosci. 36 , 1427–1471 (2024).
Regev, T. I., Casto, C. & Fedorenko, E. Neural populations in the language network differ in the size of their temporal receptive windows. OSF osf.io/xfbr8 (2024).
Stelzer, J., Chen, Y. & Turner, R. Statistical inference and multiple testing correction in classification-based multi-voxel pattern analysis (MVPA): random permutations and cluster size control. Neuroimage 65 , 69–82 (2013).
Maris, E. & Oostenveld, R. Nonparametric statistical testing of EEG- and MEG-data. J. Neurosci. Methods 164 , 177–190 (2007).
Hasson, U., Yang, E., Vallines, I., Heeger, D. J. & Rubin, N. A hierarchy of temporal receptive windows in human cortex. J. Neurosci. 28 , 2539–2550 (2008).
Norman-Haignere, S. V. et al. Multiscale temporal integration organizes hierarchical computation in human auditory cortex. Nat. Hum. Behav. 6 , 455–469 (2022).
Overath, T., McDermott, J. H., Zarate, J. M. & Poeppel, D. The cortical analysis of speech-specific temporal structure revealed by responses to sound quilts. Nat. Neurosci. 18 , 903–911 (2015).
Keshishian, M. et al. Joint, distributed and hierarchically organized encoding of linguistic features in the human auditory cortex. Nat. Hum. Behav. 7 , 740–753 (2023).
Braga, R. M., DiNicola, L. M., Becker, H. C. & Buckner, R. L. Situating the left-lateralized language network in the broader organization of multiple specialized large-scale distributed networks. J. Neurophysiol. 124 , 1415–1448 (2020).
Fedorenko, E. & Blank, I. A. Broca’s area is not a natural kind. Trends Cogn. Sci. 24 , 270–284 (2020).
Dick, F. et al. Language deficits, localization, and grammar: evidence for a distributive model of language breakdown in aphasic patients and neurologically intact individuals. Psychol. Rev. 108 , 759–788 (2001).
Runyan, C. A., Piasini, E., Panzeri, S. & Harvey, C. D. Distinct timescales of population coding across cortex. Nature 548 , 92–96 (2017).
Murray, J. D. et al. A hierarchy of intrinsic timescales across primate cortex. Nat. Neurosci. 17 , 1661–1663 (2014).
Chien, H. S. & Honey, C. J. Constructing and forgetting temporal context in the human cerebral cortex. Neuron 106 , 675–686 (2020).
Jacoby, N. & Fedorenko, E. Discourse-level comprehension engages medial frontal Theory of Mind brain regions even for expository texts. Lang. Cogn. Neurosci. 35 , 780–796 (2018).
Caucheteux, C., Gramfort, A. & King, J. R. Evidence of a predictive coding hierarchy in the human brain listening to speech. Nat. Hum. Behav. 7 , 430–441 (2023).
Chang, C. H. C., Nastase, S. A. & Hasson, U. Information flow across the cortical timescale hierarchy during narrative construction. Proc. Natl Acad. Sci. USA 119 , e2209307119 (2022).
Bozic, M., Tyler, L. K., Ives, D. T., Randall, B. & Marslen-Wilson, W. D. Bihemispheric foundations for human speech comprehension. Proc. Natl Acad. Sci. USA 107 , 17439–17444 (2010).
Paulk, A. C. et al. Large-scale neural recordings with single neuron resolution using Neuropixels probes in human cortex. Nat. Neurosci. 25 , 252–263 (2022).
Leonard, M. K. et al. Large-scale single-neuron speech sound encoding across the depth of human cortex. Nature 626 , 593–602 (2024).
Evans, N. & Levinson, S. C. The myth of language universals: language diversity and its importance for cognitive science. Behav. Brain Sci. 32 , 429–448 (2009).
Shannon, C. E. Communication in the presence of noise. Proc. IRE 37 , 10–21 (1949).
Levy, R. Expectation-based syntactic comprehension. Cognition 106 , 1126–1177 (2008).
Levy, R. A noisy-channel model of human sentence comprehension under uncertain input. In Proc. 2008 Conference on Empirical Methods in Natural Language Processing (eds Lapata, M. & Ng, H. T.) 234–243 (Association for Computational Linguistics, 2008).
Gibson, E., Bergen, L. & Piantadosi, S. T. Rational integration of noisy evidence and prior semantic expectations in sentence interpretation. Proc. Natl Acad. Sci. USA 110 , 8051–8056 (2013).
Keshev, M. & Meltzer-Asscher, A. Noisy is better than rare: comprehenders compromise subject–verb agreement to form more probable linguistic structures. Cogn. Psychol. 124 , 101359 (2021).
Gibson, E. et al. How efficiency shapes human language. Trends Cogn. Sci. 23 , 389–407 (2019).
Tuckute, G., Kanwisher, N. & Fedorenko, E. Language in brains, minds, and machines. Annu. Rev. Neurosci. https://doi.org/10.1146/annurev-neuro-120623-101142 (2024).
Norman-Haignere, S., Kanwisher, N. G. & McDermott, J. H. Distinct cortical pathways for music and speech revealed by hypothesis-free voxel decomposition. Neuron 88 , 1281–1296 (2015).
Baker, C. I. et al. Visual word processing and experiential origins of functional selectivity in human extrastriate cortex. Proc. Natl Acad. Sci. USA 104 , 9087–9092 (2007).
Buckner, R. L. & DiNicola, L. M. The brain’s default network: updated anatomy, physiology and evolving insights. Nat. Rev. Neurosci. 20 , 593–608 (2019).
Saxe, R., Brett, M. & Kanwisher, N. Divide and conquer: a defense of functional localizers. Neuroimage 30 , 1088–1096 (2006).
Baldassano, C. et al. Discovering event structure in continuous narrative perception and memory. Neuron 95 , 709–721 (2017).
Wilson, S. M. et al. Recovery from aphasia in the first year after stroke. Brain 146 , 1021–1039 (2023).
Piantadosi, S. T., Tily, H. & Gibson, E. Word lengths are optimized for efficient communication. Proc. Natl Acad. Sci. USA 108 , 3526–3529 (2011).
Shain, C., Blank, I. A., Fedorenko, E., Gibson, E. & Schuler, W. Robust effects of working memory demand during naturalistic language comprehension in language-selective cortex. J. Neurosci. 42 , 7412–7430 (2022).
Schrimpf, M. et al. The neural architecture of language: integrative modeling converges on predictive processing. Proc. Natl Acad. Sci. USA 118 , e2105646118 (2021).
Tuckute, G. et al. Driving and suppressing the human language network using large language models. Nat. Hum. Behav. 8 , 544–561 (2024).
Mollica, F. & Piantadosi, S. T. Humans store about 1.5 megabytes of information during language acquisition. R. Soc. Open Sci. 6 , 181393 (2019).
Skrill, D. & Norman-Haignere, S. V. Large language models transition from integrating across position-yoked, exponential windows to structure-yoked, power-law windows. Adv. Neural Inf. Process. Syst. 36 , 638–654 (2023).
Giglio, L., Ostarek, M., Weber, K. & Hagoort, P. Commonalities and asymmetries in the neurobiological infrastructure for language production and comprehension. Cereb. Cortex 32 , 1405–1418 (2022).
Hu, J. et al. Precision fMRI reveals that the language-selective network supports both phrase-structure building and lexical access during language production. Cereb. Cortex 33 , 4384–4404 (2023).
Lee, E. K., Brown-Schmidt, S. & Watson, D. G. Ways of looking ahead: hierarchical planning in language production. Cognition 129 , 544–562 (2013).
Wechsler, D. Wechsler abbreviated scale of intelligence (WASI) [Database record]. APA PsycTests https://psycnet.apa.org/doi/10.1037/t15170-000 (APA PsycNet, 1999).
Schalk, G., McFarland, D. J., Hinterberger, T., Birbaumer, N. & Wolpaw, J. R. BCI2000: a general-purpose brain-computer interface (BCI) system. IEEE Trans. Biomed. Eng. 51 , 1034–1043 (2004).
Adamek, M., Swift, J. R. & Brunner, P. VERA - Versatile Electrode Localization Framework. Zenodo https://doi.org/10.5281/zenodo.7486842 (2022).
Adamek, M., Swift, J. R. & Brunner, P. VERA - A Versatile Electrode Localization Framework (Version 1.0.0). GitHub https://github.com/neurotechcenter/VERA (2022).
Avants, B. B., Epstein, C. L., Grossman, M. & Gee, J. C. Symmetric diffeomorphic image registration with cross-correlation: evaluating automated labeling of elderly and neurodegenerative brain. Med. Image Anal. 12 , 26–41 (2008).
Janca, R. et al. Detection of interictal epileptiform discharges using signal envelope distribution modelling: application to epileptic and non-epileptic intracranial recordings. Brain Topogr. 28 , 172–183 (2015).
Dichter, B. K., Breshears, J. D., Leonard, M. K. & Chang, E. F. The control of vocal pitch in human laryngeal motor cortex. Cell 174 , 21–31 (2018).
Ray, S., Crone, N. E., Niebur, E., Franaszczuk, P. J. & Hsiao, S. S. Neural correlates of high-gamma oscillations (60–200 Hz) in macaque local field potentials and their potential implications in electrocorticography. J. Neurosci. 28 , 11526–11536 (2008).
Lipkin, B. et al. Probabilistic atlas for the language network based on precision fMRI data from >800 individuals. Sci. Data 9 , 529 (2022).
Kučera, H. Computational Analysis of Present-day American English (Univ. Pr. of New England, 1967).
Kaufman, L. & Rousseeuw, P. J. in Finding Groups in Data: An Introduction to Cluster Analysis (eds L. Kaufman, L. & Rousseeuw, P. J.) Ch. 2 (Wiley, 1990).
Rokach, L. & Maimon, O. in The Data Mining and Knowledge Discovery Handbook (eds Maimon, O. & Rokach, L.) 321–352 (Springer, 2005).
Wilkinson, G.N. & Rogers, C.E. Symbolic description of factorial models for analysis of variance. J. R. Stat. Soc., C: Appl.Stat. 22 , 392–399 (1973).
Google Scholar
Luke, S. G. Evaluating significance in linear mixed-effects models in R. Behav. Res. Methods 49 , 1494–1502 (2017).
Regev, T. I. et al. Neural populations in the language network differ in the size of their temporal receptive windows. GitHub https://github.com/coltoncasto/ecog_clustering_PUBLIC (2024).
Download references
Acknowledgements
We thank the participants for agreeing to take part in our study, as well as N. Kanwisher, former and current EvLab members, especially C. Shain and A. Ivanova, and the audience at the Neurobiology of Language conference (2022, Philadelphia) for helpful discussions and comments on the analyses and manuscript. T.I.R. was supported by the Zuckerman-CHE STEM Leadership Program and by the Poitras Center for Psychiatric Disorders Research. C.C. was supported by the Kempner Institute for the Study of Natural and Artificial Intelligence at Harvard University. A.L.R. was supported by NIH award U01-NS108916. J.T.W. was supported by NIH awards R01-MH120194 and P41-EB018783, and the American Epilepsy Society Research and Training Fellowship for clinicians. P.B. was supported by NIH awards R01-EB026439, U24-NS109103, U01-NS108916, U01-NS128612 and P41-EB018783, the McDonnell Center for Systems Neuroscience, and Fondazione Neurone. E.F. was supported by NIH awards R01-DC016607, R01-DC016950 and U01-NS121471, and research funds from the McGovern Institute for Brain Research, Brain and Cognitive Sciences Department, and the Simons Center for the Social Brain. The funders had no role in study design, data collection and analysis, decision to publish or preparation of the manuscript.
Author information
These authors contributed equally: Tamar I. Regev, Colton Casto.
Authors and Affiliations
Brain and Cognitive Sciences Department, Massachusetts Institute of Technology, Cambridge, MA, USA
Tamar I. Regev, Colton Casto, Eghbal A. Hosseini & Evelina Fedorenko
McGovern Institute for Brain Research, Massachusetts Institute of Technology, Cambridge, MA, USA
Program in Speech and Hearing Bioscience and Technology (SHBT), Harvard University, Boston, MA, USA
Colton Casto & Evelina Fedorenko
Kempner Institute for the Study of Natural and Artificial Intelligence, Harvard University, Allston, MA, USA
Colton Casto
National Center for Adaptive Neurotechnologies, Albany, NY, USA
Markus Adamek, Jon T. Willie & Peter Brunner
Department of Neurosurgery, Washington University School of Medicine, St Louis, MO, USA
Department of Neurology, Mayo Clinic, Jacksonville, FL, USA
Anthony L. Ritaccio
Department of Neurology, Albany Medical College, Albany, NY, USA
Peter Brunner
You can also search for this author in PubMed Google Scholar
Contributions
T.I.R. and C.C. equally contributed to study conception and design, data analysis and interpretation of results, and manuscript writing. E.A.H. contributed to data analysis and manuscript editing; M.A. to data collection and analysis; A.L.R., J.T.W. and P.B. to data collection and manuscript editing. E.F. contributed to study conception and design, supervision, interpretation of results and manuscript writing.
Corresponding authors
Correspondence to Tamar I. Regev , Colton Casto or Evelina Fedorenko .
Ethics declarations
Competing interests.
The authors declare no competing interests.
Peer review
Peer review information.
Nature Human Behaviour thanks Nima Mesgarani, Jonathan Venezia and the other, anonymous, reviewer(s) for their contribution to the peer review of this work. Peer reviewer reports are available.
Additional information
Publisher’s note Springer Nature remains neutral with regard to jurisdictional claims in published maps and institutional affiliations.
Extended data
Extended data fig. 1 dataset 1 k-medoids (k = 3) cluster assignments by participant..
Average cluster responses as in Fig. 2e grouped by participant. Shaded areas around the signal reflect a 99% confidence interval over electrodes. The number of electrodes constructing the average (n) is denoted above each signal in parenthesis. Prototypical responses for each of the three clusters were found in nearly all participants individually. However, for participants with only a few electrodes assigned to a given cluster (for example, P5 Cluster 3), the responses were more variable.
Extended Data Fig. 2 Dataset 1 k-medoids clustering with k = 10.
a) Clustering mean electrode responses (S + W + J + N) using k-medoids with k = 10 and a correlation-based distance. Shading of the data matrix reflects normalized high-gamma power (70–150 Hz). b) Electrode responses visualized on their first two principal components, colored by cluster. c) Timecourses of best representative electrodes (‘medoids’) selected by the algorithm from each of the ten clusters. d) Timecourses averaged across all electrodes in each cluster. Shaded areas around the signal reflect a 99% confidence interval over electrodes. Correlation with the k = 3 cluster averages are shown to the right of the timecourses. Many clusters exhibited high correlations with the k = 3 response profiles from Fig. 2 .
Extended Data Fig. 3 All Dataset 1 responses.
a-c) All Dataset 1 electrode responses. The timecourses (concatenated across the four conditions, ordered: sentences, word lists, Jabberwocky sentences, non-word lists) of all electrodes in Dataset 1 sorted by their correlation to the cluster medoid (medoid shown at the bottom of each cluster). Colors reflect the reliability of the measured neural signal, computed by correlating responses to odd and even trials (Fig. 1d ). The estimated temporal receptive window (TRW) using the toy model from Fig. 4 is displayed to the left, and the participant who contributed the electrode is displayed to the right. There was strong consistency in the responses from individual electrodes within a cluster (with more variability in the less reliable electrodes), and electrodes with responses that were more similar to the cluster medoid tended to be more reliable (more pink). Note that there were two reliable response profiles (relatively pink) that showed a pattern that was distinct from the three prototypical response profiles: One electrode in Cluster 2 (the 10th electrode from the top in panel B) responded only to the onset of the first word/nonword in each trial; and one electrode in Cluster 3 (the 4th electrode from the top in panel C) was highly locked to all onsets except the first word/nonword. These profiles indicate that although the prototypical clusters explain a substantial amount of the functional heterogeneity of responses in the language network, they were not the only observed responses.
Extended Data Fig. 4 Partial correlations of individual response profiles with each of the cluster medoids.
a) Pearson correlations of all response profiles with each of the cluster medoids, grouped by cluster assignment. b) Partial correlations ( Methods ) of all response profiles with each of the cluster medoids, controlling for the other two cluster medoids, grouped by cluster assignment. c) Response profiles from electrodes assigned to Cluster 1 that had a high partial correlation ( > 0.2, arbitrarily defined threshold) with the Cluster 2 medoid (and split-half reliability>0.3). Top: Average over all electrodes that met these criteria (n = 18, black). The Cluster 1 medoid is shown in red, and the Cluster 2 medoid is shown in green. Bottom: Four sample electrodes (black). d) Response profiles assigned to Cluster 2 that had a high partial correlation ( > 0.2, arbitrarily defined threshold) with the Cluster 1 medoid (and split-half reliability>0.3). Top: Average over all electrodes that meet these criteria (n = 12, black). The Cluster 1 medoid is shown in red, and the Cluster 2 medoid is shown in green. Bottom: Four sample electrodes (black; see osf.io/xfbr8/ for all mixed response profiles with split-half reliability>0.3). e) Anatomical distribution of electrodes in Dataset 1 colored by their partial correlation with a given cluster medoid (controlling for the other two medoids). Cluster-1- and Cluster-2-like responses were present throughout frontal and temporal areas (with Cluster 1 responses having a slightly higher concentration in the temporal pole and Cluster 2 responses having a slightly higher concentration in the superior temporal gyrus (STG)), whereas Cluster-3-like responses were localized to the posterior STG.
Extended Data Fig. 5 N-gram frequencies of sentences and word lists diverge with n-gram length.
N-gram frequencies were extracted from the Google n-gram online platform ( https://books.google.com/ngrams/ ), averaging across Google books corpora between the years 2010 and 2020. For each individual word, the n-gram frequency for n = 1 was the frequency of that word in the corpus; for n = 2 it was the frequency of the bigram (sequence of 2 words) ending in that word; for n = 3 it was the frequency of the trigram (sequence of 3 words) ending in that word; and so on. Sequences that were not found in the corpus were assigned a value of 0. Results are only presented until n = 4 because for n > 4 most of the string sequences, both from the Sentence and Word-list conditions, were not found in the corpora. The plot shows that the difference between the log n-gram values for the sentences and word lists in our stimulus set grows as a function of N. Error bars represent the standard error of the mean across all n-grams extracted from the stimuli used (640, 560, 480, 399 n-grams for n-gram length = 1, 2, 3, and 4, respectively).
Extended Data Fig. 6 Temporal receptive window (TRW) estimates with kernels of different shapes.
The toy TRW model from Fig. 4 was applied using five different kernel shapes: cosine ( a ), ‘wide’ Gaussian (Gaussian curves with a standard deviation of σ /2 that were truncated at +/− 1 standard deviation, as used in Fig. 4 ; b ), ‘narrow’ Gaussian (Gaussian curves with a standard deviation of σ /16 that were truncated at +/− 8 standard deviations; c ), a square (that is, boxcar) function (1 for the entire window; d ) and a linear asymmetric function (linear function with a value of 0 initially and a value of 1 at the end of the window; e ). For each kernel ( a-e ), the plots represent (left to right, all details are identical to Fig. 4 in the manuscript): 1) The kernel shapes for TRW = 1, 2, 3, 4, 6 and 8 words, superimposed on the simplified stimulus train; 2) The simulated neural signals for each of those TRWs; 3) violin plots of best fitted TRW values across electrodes (each dot represents an electrode, horizontal black lines are means across the electrodes, white dots are medians, vertical thin box represents lower and upper quartiles and ‘x’ marks indicate outliers; more than 1.5 interquartile ranges above the upper quartile or less than 1.5 interquartile ranges below the lower quartile) for all electrodes (black), or electrodes from only Clusters 1 (red) 2 (green) or 3 (blue); and 4) Estimated TRW as a function of goodness of fit. Each dot is an electrode, its size represents the reliability of its neural response, computed via correlation between the mean signals when using only odd vs. only even trials, x-axis is the electrode’s best fitted TRW, y-axis is the goodness of fit, computed via correlation between the neural signal and the closest simulated signal. For all kernels the TRWs showed a decreasing trend from Cluster 1 to 3.
Extended Data Fig. 7 Dataset 1 k-medoids clustering results with only S and N conditions.
a) Search for optimal k using the ‘elbow method’. Top: variance (sum of the distances of all electrodes to their assigned cluster centre) normalized by the variance when k = 1 as a function of k (normalized variance (NV)). Bottom: change in NV as a function of k (NV(k + 1) – NV(k)). After k = 3 the change in variance became more moderate, suggesting that 3 clusters appropriately described Dataset 1 when using only the responses to sentences and non-words (as was the case when all four conditions were used). b) Clustering mean electrode responses (only S and N, importantly) using k-medoids (k = 3) with a correlation-based distance. Shading of the data matrix reflects normalized high-gamma power (70–150 Hz). c) Average timecourse by cluster. Shaded areas around the signal reflect a 99% confidence interval over electrodes (n = 99, n = 61, and n = 17 electrodes for Cluster 1, 2, and 3, respectively). Clusters 1-3 showed a strong similarity to the clusters reported in Fig. 2 . d) Mean condition responses by cluster. Error bars reflect standard error of the mean over electrodes. e) Electrode responses visualized on their first two principal components, colored by cluster. f) Anatomical distribution of clusters across all participants (n = 6). g) Robustness of clusters to electrode omission (random subsets of electrodes were removed in increments of 5). Stars reflect significant similarity with the full dataset (with a p threshold of 0.05; evaluated with a one-sided permutation test, n = 1000 permutations; Methods ). Shaded regions reflect standard error of the mean over randomly sampled subsets of electrodes. Relative to when all conditions were used, Cluster 2 was less robust to electrode omission (although still more robust than Cluster 3), suggesting that responses to word lists and Jabberwocky sentences (both not present here) are particularly important for distinguishing Cluster 2 electrodes from Cluster 1 and 3 electrodes.
Extended Data Fig. 8 Dataset 2 electrode assignment to most correlated Dataset 1 cluster under ‘winner-take-all’ (WTA) approach.
a) Assigning electrodes from Dataset 2 to the most correlated cluster from Dataset 1. Assignment was performed using the correlation with the Dataset 1 cluster average, not the cluster medoid. Shading of the data matrix reflects normalized high-gamma power (70–150 Hz). b) Average timecourse by group. Shaded areas around the signal reflect a 99% confidence interval over electrodes (n = 142, n = 95, and n = 125 electrodes for groups 1, 2, and 3, respectively). c) Mean condition responses by group. Error bars reflect standard error of the mean over electrodes (n = 142, n = 95, and n = 125 electrodes for groups 1, 2, and 3, respectively, as in b ). d) Electrode responses visualized on their first two principal components, colored by group. e) Anatomical distribution of groups across all participants (n = 16). f-g) Comparison of cluster assignment of electrodes from Dataset 2 using clustering vs. winner-take-all (WTA) approach. f) The numbers in the matrix correspond to the number of electrodes assigned to cluster y during clustering (y-axis) versus the number electrodes assigned to group x during the WTA approach (x-axis). For instance, there were 44 electrodes that were assigned to Cluster 1 during clustering but were ‘pulled out’ to Group 2 (the analog of Cluster 2) during the WTA approach. The total number of electrodes assigned to each cluster during the clustering approach are shown to the right of each row. The total number of electrodes assigned to each group during the WTA approach are shown at the top of each column. N = 362 is the total number of electrodes in Dataset 2. g) Similar to F , but here the average timecourse across all electrodes assigned to the corresponding cluster/group during both procedures is presented. Shaded areas around the signals reflect a 99% confidence interval over electrodes.
Extended Data Fig. 9 Anatomical distribution of the clusters in Dataset 2.
a) Anatomical distribution of language-responsive electrodes in Dataset 2 across all subjects in MNI space, colored by cluster. Only Clusters 1 and 3 (those from Dataset 1 that replicate to Dataset 2) are shown. b) Anatomical distribution of language-responsive electrodes in subject-specific space for eight sample participants. c-h) Violin plots of MNI coordinate values for Clusters 1 and 3 in the left and right hemisphere ( c-e and f-h , respectively), where plotted points (n = 16 participants) represent the mean of all coordinate values for a given participant and cluster. The mean across participants is plotted with a black horizontal line, and the median is shown with a white circle. Vertical thin black boxes within violins plots represent the upper and lower quartiles. Significance is evaluated with a LME model ( Methods , Supplementary Tables 3 and 4 ). The Cluster 3 posterior bias from Dataset 1 was weakly present but not statistically reliable.
Extended Data Fig. 10 Estimation of temporal receptive window (TRW) sizes for electrodes in Dataset 2.
As in Fig. 4 but for electrodes in Dataset 2. a) Best TRW fit (using the toy model from Fig. 4 ) for all electrodes, colored by cluster (when k-medoids clustering with k = 3 was applied, Fig. 6 ) and sized by the reliability of the neural signal as estimated by correlating responses to odd and even trials (Fig. 6c ). The ‘goodness of fit’, or correlation between the simulated and observed neural signal (Sentence condition only), is shown on the y-axis. b) Estimated TRW sizes across all electrodes (grey) and per cluster (red, green, and blue). Black vertical lines correspond to the mean window size and the white dots correspond to the median. ‘x’ marks indicate outliers (more than 1.5 interquartile ranges above the upper quartile or less than 1.5 interquartile ranges below the lower quartile). Significance values were calculated using a linear mixed-effects model (comparing estimate values, two-sided ANOVA for LME, Methods , see Supplementary Table 8 for exact p-values). c-d) Same as A and B , respectively, except that clusters were assigned by highest correlation with Dataset 1 clusters (Extended Data Fig. 8 ). Under this procedure, Cluster 2 reliably separated from Cluster 3 in terms of its TRW (all ps<0.001, evaluated with a LME model, Methods , see Supplementary Table 9 for exact p-values).
Supplementary information
Supplementary information.
Supplementary Tables 1–11.
Reporting Summary
Peer review file, rights and permissions.
Springer Nature or its licensor (e.g. a society or other partner) holds exclusive rights to this article under a publishing agreement with the author(s) or other rightsholder(s); author self-archiving of the accepted manuscript version of this article is solely governed by the terms of such publishing agreement and applicable law.
Reprints and permissions
About this article
Cite this article.
Regev, T.I., Casto, C., Hosseini, E.A. et al. Neural populations in the language network differ in the size of their temporal receptive windows. Nat Hum Behav (2024). https://doi.org/10.1038/s41562-024-01944-2
Download citation
Received : 16 March 2023
Accepted : 03 July 2024
Published : 26 August 2024
DOI : https://doi.org/10.1038/s41562-024-01944-2
Share this article
Anyone you share the following link with will be able to read this content:
Sorry, a shareable link is not currently available for this article.
Provided by the Springer Nature SharedIt content-sharing initiative
Quick links
- Explore articles by subject
- Guide to authors
- Editorial policies
Sign up for the Nature Briefing newsletter — what matters in science, free to your inbox daily.

User Preferences
Content preview.
Arcu felis bibendum ut tristique et egestas quis:
- Ut enim ad minim veniam, quis nostrud exercitation ullamco laboris
- Duis aute irure dolor in reprehenderit in voluptate
- Excepteur sint occaecat cupidatat non proident
Keyboard Shortcuts
6.3 - testing for partial correlation.
When discussing ordinary correlations we looked at tests for the null hypothesis that the ordinary correlation is equal to zero, against the alternative that it is not equal to zero. If that null hypothesis is rejected, then we look at confidence intervals for the ordinary correlation. Similar objectives can be considered for the partial correlation.
First, consider testing the null hypothesis that a partial correlation is equal to zero against the alternative that it is not equal to zero. This is expressed below:
\(H_0\colon \rho_{jk\textbf{.x}}=0\) against \(H_a\colon \rho_{jk\textbf{.x}}\ne 0\)
Here we will use a test statistic that is similar to the one we used for an ordinary correlation. This test statistic is shown below:
\(t = r_{jk\textbf{.x}}\sqrt{\frac{n-2-c}{1-r^2_{jk\textbf{.x}}}}\) \(\dot{\sim}\) \(t_{n-2-c}\)
The only difference between this and the previous one is what appears in the numerator of the radical. Before we just took n - 2. Here we take n - 2 - c , where c is the number of variables upon which we are conditioning. In our Adult Intelligence data, we conditioned on two variables so c would be equal to 2 in this case.
Under the null hypothesis, this test statistic will be approximately t -distributed, also with n - 2 - c degrees of freedom.
We would reject \(H_{o}\colon\) if the absolute value of the test statistic exceeded the critical value from the t -table evaluated at \(\alpha\) over 2:
\(|t| > t_{n-2-c, \alpha/2}\)
Example 6-3: Wechsler Adult Intelligence Data Section
For the Wechsler Adult Intelligence Data, we found a partial correlation of 0.711879, which we enter into the expression for the test statistic as shown below:
\(t = 0.711879 \sqrt{\dfrac{37-2-2}{1-0.711879^2}}=5.82\)
The sample size is 37, along with the 2 variables upon which we are conditioning is also substituted in. Carry out the math and we get a test statistic of 5.82 as shown above.
Here we want to compare this value to a t -distribution with 33 degrees of freedom for an \(\alpha\) = 0.01 level test. Therefore, we are going to look at the critical value for 0.005 in the table (because 33 does not appear to use the closest df that does not exceed 33 which is 30). In this case it is 2.75, meaning that \(t _ { ( d f , 1 - \alpha / 2 ) } = t _ { ( 33,0.995 ) } \) is 2.75.
Because \(5.82 > 2.75 = t _ { ( 33,0.995 ) }\), we can reject the null hypothesis, \(H_{o}\) at the \(\alpha = 0.01\) level and conclude that there is a significant partial correlation between these two variables. In particular, we would include that this partial correlation is positive indicating that even after taking into account Arithmetic and Picture Completion, there is a positive association between Information and Similarities.
Confidence Interval for the partial correlation, \(\rho_{jk\textbf{.x}}\) Section
The procedure here is very similar to the procedure we used for ordinary correlation.
Compute Fisher's transformation of the partial correlation using the same formula as before.
\(z_{jk} = \dfrac{1}{2}\log \left( \dfrac{1+r_{jk\textbf{.X}}}{1-r_{jk\textbf{.X}}}\right) \)
In this case, for a large n , this Fisher transform variable will be possibly normally distributed. The mean is equal to the Fisher transform for the population value for this partial correlation, and the variance is equal to 1 over n-3-c .
\(z_{jk}\) \(\dot{\sim}\) \(N \left( \dfrac{1}{2}\log \dfrac{1+\rho_{jk\textbf{.X}}}{1-\rho_{jk\textbf{.X}}}, \dfrac{1}{n-3-c}\right)\)
Compute a \((1 - \alpha) × 100\%\) confidence interval for the Fisher transform correlation. This expression is shown below:
\( \dfrac{1}{2}\log \dfrac{1+\rho_{jk\textbf{.X}}}{1-\rho_{jk\textbf{.X}}}\)
This yields the bounds \(Z_{l}\) and \(Z_{u}\) as before.
\(\left(\underset{Z_l}{\underbrace{Z_{jk}-\dfrac{Z_{\alpha/2}}{\sqrt{n-3-c}}}}, \underset{Z_U}{\underbrace{Z_{jk}+\dfrac{Z_{\alpha/2}}{\sqrt{n-3-c}}}}\right)\)
Back transform to obtain the desired confidence interval for the partial correlation - \(\rho_{jk\textbf{.X}}\)
\(\left(\dfrac{e^{2Z_l}-1}{e^{2Z_l}+1}, \dfrac{e^{2Z_U}-1}{e^{2Z_U}+1}\right)\)
Example 6-3: Wechsler Adult Intelligence Data (Steps Shown) Section
The confidence interval is calculated by substituting the results from the Wechsler Adult Intelligence Data into the appropriate steps below:
Step 1 : Compute the Fisher transform:
\begin{align} Z_{12} &= \dfrac{1}{2}\log \frac{1+r_{12.34}}{1-r_{12.34}}\\[5pt] &= \dfrac{1}{2} \log \frac{1+0.711879}{1-0.711879}\\[5pt] &= 0.89098 \end{align}
Step 2 : Compute the 95% confidence interval for \( \frac{1}{2}\log \frac{1+\rho_{12.34}}{1-\rho_{12.34}}\) :
\begin{align} Z_l &= Z_{12}-Z_{0.025}/\sqrt{n-3-c}\\[5pt] & = 0.89098 - \dfrac{1.96}{\sqrt{37-3-2}}\\[5pt] &= 0.5445 \end{align}
\begin{align} Z_U &= Z_{12}+Z_{0.025}/\sqrt{n-3-c}\\[5pt] &= 0.89098 + \dfrac{1.96}{\sqrt{37-3-2}} \\[5pt] &= 1.2375 \end{align}
Step 3 : Back-transform to obtain the 95% confidence interval for \(\rho_{12.34}\) :
\(\left(\dfrac{\exp\{2Z_l\}-1}{\exp\{2Z_l\}+1}, \dfrac{\exp\{2Z_U\}-1}{\exp\{2Z_U\}+1}\right)\)
\(\left(\dfrac{\exp\{2\times 0.5445\}-1}{\exp\{2\times 0.5445\}+1}, \dfrac{\exp\{2\times 1.2375\}-1}{\exp\{2\times 1.2375\}+1}\right)\)
\((0.4964, 0.8447)\)
Based on this result, we can conclude that we are 95% confident that the interval (0.4964, 0.8447) contains the partial correlation between Information and Similarities scores given scores on Arithmetic and Picture Completion.
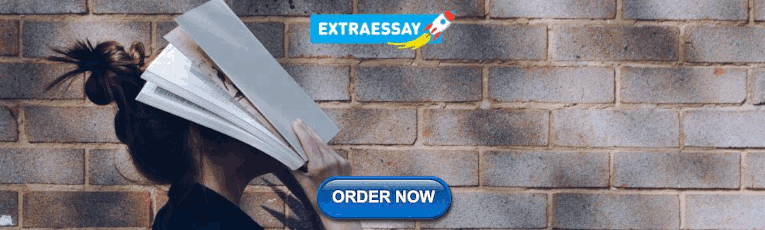
IMAGES
COMMENTS
11.2: Correlation Hypothesis Test. The correlation coefficient, , tells us about the strength and direction of the linear relationship between and . However, the reliability of the linear model also depends on how many observed data points are in the sample.
Example: Deciding whether to reject the null hypothesis For the correlation between weight and height in a sample of 10 newborns, the t value is less than the critical value of t. Therefore, we don't reject the null hypothesis that the Pearson correlation coefficient of the population ( ρ ) is 0.
The null-hypothesis of a two-tailed test states that there is no correlation (there is not a linear relation) between \(x\) and \(y\). The alternative-hypothesis states that there is a significant correlation (there is a linear relation) between \(x\) and \(y\). ... Figure 12-8: Scatterplot for spurious correlation example. With \(r = 0.9586 ...
A null hypothesis claims that there is no effect in the population, while an alternative hypothesis claims that there is an effect. ... Be careful not to say you "prove" or "accept" the null hypothesis. Example: ... There is a correlation between independent variable and dependent variable in the population; ρ ≠ 0.
Below are typical examples of writing a null hypothesis for various parameters and hypothesis tests. Related posts: Descriptive vs. Inferential Statistics and Populations, Parameters, and Samples in Inferential Statistics. ... Null Hypothesis H 0: The correlation in the population is zero: ρ = 0. Alternative Hypothesis H A: ...
Let's perform the hypothesis test on the husband's age and wife's age data in which the sample correlation based on n = 170 couples is r = 0.939. To test H 0: ρ = 0 against the alternative H A: ρ ≠ 0, we obtain the following test statistic: t ∗ = r n − 2 1 − R 2 = 0.939 170 − 2 1 − 0.939 2 = 35.39. To obtain the P -value, we need ...
Under the null hypothesis and with above assumptions, the test statistic, \(t^*\), found by: ... For the sales and advertising example, conduct a test for correlation with a significance level of 5% with Minitab. ... (\alpha=0.05\), we can reject the hypothesis that the population correlation coefficient is 0 and conclude that it is nonzero, i ...
PERFORMING THE HYPOTHESIS TEST. Null Hypothesis: H 0: ρ = 0 Alternate Hypothesis: H a: ρ ≠ 0 WHAT THE HYPOTHESES MEAN IN WORDS: Null Hypothesis H 0: The population correlation coefficient IS NOT significantly different from zero. There IS NOT a significant linear relationship (correlation) between x and y in the population.; Alternate Hypothesis H a: The population correlation coefficient ...
5.3 - Inferences for Correlations. Let us consider testing the null hypothesis that there is zero correlation between two variables X j and X k. Mathematically we write this as shown below: H 0: ρ j k = 0 against H a: ρ j k ≠ 0. Recall that the correlation is estimated by sample correlation r j k given in the expression below: r j k = s j k ...
The null and alternative hypotheses are two competing claims that researchers weigh evidence for and against using a statistical test: Null hypothesis (H0): There's no effect in the population. Alternative hypothesis (HA): There's an effect in the population. The effect is usually the effect of the independent variable on the dependent ...
To distinguish it from other hypotheses, the null hypothesis is written as H 0 (which is read as "H-nought," "H-null," or "H-zero"). A significance test is used to determine the likelihood that the results supporting the null hypothesis are not due to chance. A confidence level of 95% or 99% is common. Keep in mind, even if the confidence level is high, there is still a small chance the ...
Write a statistical null hypothesis as a mathematical equation, such as. μ 1 = μ 2 {\displaystyle \mu _ {1}=\mu _ {2}} if you're comparing group means. Adjust the format of your null hypothesis to match the statistical method you used to test it, such as using "mean" if you're comparing the mean between 2 groups.
The hypothesis test lets us decide whether the value of the population correlation coefficient ρ is "close to zero" or "significantly different from zero.". We decide this based on the sample correlation coefficient r and the sample size n. If the test concludes that the correlation coefficient is significantly different from zero, we ...
Hypothesis Test for Correlation - Key takeaways. The Product Moment Correlation Coefficient (PMCC), or r, is a measure of how strongly related 2 variables are. It ranges between -1 and 1, indicating the strength of a correlation. The closer r is to 1 or -1 the stronger the (positive or negative) correlation between two variables.
The actual test begins by considering two hypotheses.They are called the null hypothesis and the alternative hypothesis.These hypotheses contain opposing viewpoints. H 0, the —null hypothesis: a statement of no difference between sample means or proportions or no difference between a sample mean or proportion and a population mean or proportion. In other words, the difference equals 0.
Here are some examples. Example 10.2: Hypotheses with One Sample of One Categorical Variable Section . About 10% of the human population is left-handed. ... Null Hypothesis: The correlation between the daily stock price change (\$) and the daily stock purchases by non-management employees (\$) = 0.
If you're seeing this message, it means we're having trouble loading external resources on our website. If you're behind a web filter, please make sure that the domains *.kastatic.org and *.kasandbox.org are unblocked.
H 0 (Null Hypothesis): Population parameter =, ≤, ≥ some value. H A (Alternative Hypothesis): Population parameter <, >, ≠ some value. Note that the null hypothesis always contains the equal sign. We interpret the hypotheses as follows: Null hypothesis: The sample data provides no evidence to support some claim being made by an individual.
5.2 - Writing Hypotheses. The first step in conducting a hypothesis test is to write the hypothesis statements that are going to be tested. For each test you will have a null hypothesis ( H 0) and an alternative hypothesis ( H a ). When writing hypotheses there are three things that we need to know: (1) the parameter that we are testing (2) the ...
The null hypothesis and the alternative hypothesis are types of conjectures used in statistical tests to make statistical inferences, which are formal methods of reaching conclusions and separating scientific claims from statistical noise.. The statement being tested in a test of statistical significance is called the null hypothesis. The test of significance is designed to assess the strength ...
The formula for the test statistic is t = r√n − 2 √1 − r2. t = r n − 2 √ 1 − r 2 √. The value of the test statistic, t, is shown in the computer or calculator output along with the p-value. The test statistic t has the same sign as the correlation coefficient r. The p-value is the combined area in both tails.
A one-sided hypothesis test on a correlation can be performed via t as a test statistic. Here, t = r n−2 1−r2− −−−√ = r n − 2 1 − r 2. with the critical value found via tα,n−2 t α, n − 2 (in the more common two-sided case, only α α is changed). So for your H0 H 0 that r is smaller than 0, the test rejects if the t ...
For example, ref. 27 reported ... Partial correlation of individual electrodes with each of the cluster medoids. ... Under the null hypothesis, no distinct response profiles should be detectable ...
First, consider testing the null hypothesis that a partial correlation is equal to zero against the alternative that it is not equal to zero. This is expressed below: H 0: ρ j k .x = 0 against H a: ρ j k .x ≠ 0. Here we will use a test statistic that is similar to the one we used for an ordinary correlation. This test statistic is shown below: