Home — Essay Samples — Sociology — Fake News — Misinformation in Social Media

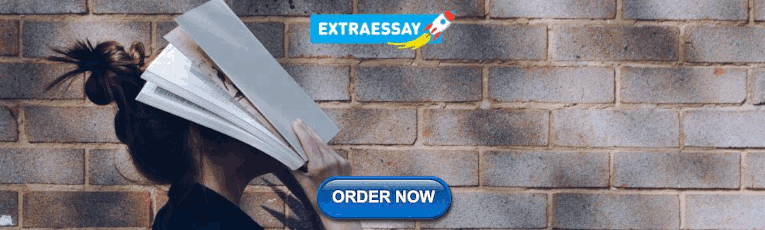
Misinformation in Social Media
- Categories: Fake News Social Media
About this sample

Words: 708 |
Published: Sep 1, 2023
Words: 708 | Pages: 2 | 4 min read
- Bakshy, E., Messing, S., & Adamic, L. A. (2015). Exposure to ideologically diverse news and opinion on Facebook. Science , 348(6239), 1130-1132.
- Johnson, B. K., & Grier, S. A. (2017). Framing public opinion in competitive democracies: How politicians respond to changes in the composition of their constituencies. The Journal of Politics , 79(2), 687-701.
- Khatoon, S., & Lamsal, S. (2020). Misinformation and media literacy: A study on media literacy among university students. Journal of Mass Communication & Journalism , 10(1), 1-6.
- Roozenbeek, J., & van der Linden, S. (2019). Fake news game confers psychological resistance against online misinformation. Palgrave Communications , 5(1), 96.
- Sunstein, C. R. (2017). #Republic: Divided democracy in the age of social media. Princeton University Press.
- Vosoughi, S., Roy, D., & Aral, S. (2018). The spread of true and false news online. Science , 359(6380), 1146-1151.
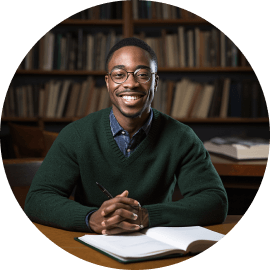
Cite this Essay
Let us write you an essay from scratch
- 450+ experts on 30 subjects ready to help
- Custom essay delivered in as few as 3 hours
Get high-quality help
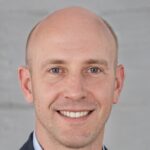
Prof. Kifaru
Verified writer
- Expert in: Sociology
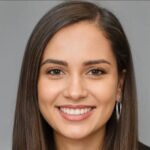
+ 120 experts online
By clicking “Check Writers’ Offers”, you agree to our terms of service and privacy policy . We’ll occasionally send you promo and account related email
No need to pay just yet!
Related Essays
1 pages / 448 words
5 pages / 2086 words
4 pages / 1882 words
1 pages / 496 words
Remember! This is just a sample.
You can get your custom paper by one of our expert writers.
121 writers online
Still can’t find what you need?
Browse our vast selection of original essay samples, each expertly formatted and styled
Related Essays on Fake News
A few decades ago people could only receive news from, what we call today, traditional media sources, such as newspapers, radios and television. These sources were and still are trusted by people due to strict regulations their [...]
Are you ready to dive into the intriguing world of fact-checking clown purge analysis? Imagine a scenario where rumors of a nationwide clown purge sweep the internet, causing panic and fear among the public. In this essay, we [...]
In today's information age, the ability to assess the credibility of evidence and resources is crucial for obtaining reliable and accurate information. The internet has made it easier than ever to access a vast amount of [...]
The era of social media has revolutionized how we connect, communicate, and share information. However, beneath the glossy filters and curated posts lies a pervasive issue - the prevalence of fake content. This essay delves into [...]
This morning started off like any other, I lit a cigarette, wrapped myself in my fuzzy blanket and started to scroll through Facebook. I saw pictures of my friend’s babies, what my grandma did at the casino last weekend, and [...]
The Daily Show with Jon Stewart is a comical news show that has been running since 1996. The Daily Show is known for its real world news, combined with a satirical edge. The show has grown more and more in popularity due to the [...]
Related Topics
By clicking “Send”, you agree to our Terms of service and Privacy statement . We will occasionally send you account related emails.
Where do you want us to send this sample?
By clicking “Continue”, you agree to our terms of service and privacy policy.
Be careful. This essay is not unique
This essay was donated by a student and is likely to have been used and submitted before
Download this Sample
Free samples may contain mistakes and not unique parts
Sorry, we could not paraphrase this essay. Our professional writers can rewrite it and get you a unique paper.
Please check your inbox.
We can write you a custom essay that will follow your exact instructions and meet the deadlines. Let's fix your grades together!
Get Your Personalized Essay in 3 Hours or Less!
We use cookies to personalyze your web-site experience. By continuing we’ll assume you board with our cookie policy .
- Instructions Followed To The Letter
- Deadlines Met At Every Stage
- Unique And Plagiarism Free
How misinformation spreads on social media—And what to do about it
Subscribe to the center for middle east policy newsletter, chris meserole chris meserole fellow - foreign policy , strobe talbott center for security, strategy, and technology @chrismeserole.
May 9, 2018
As widespread as misinformation online is, opportunities to glimpse it in action are fairly rare. Yet shortly after the recent attack in Toronto, a journalist unwittingly carried out a kind of natural experiment on Twitter. This piece originally appeared on Lawfare .
“We take misinformation seriously,” Facebook CEO Mark Zuckerberg wrote just weeks after the 2016 election. In the year since, the question of how to counteract the damage done by “fake news” has become a pressing issue both for technology companies and governments across the globe.
Yet as widespread as the problem is, opportunities to glimpse misinformation in action are fairly rare. Most users who generate misinformation do not share accurate information too, so it can be difficult to tease out the effect of misinformation itself. For example, when President Trump shares misinformation on Twitter , his tweets tend to go viral. But they may not be going viral because of the misinformation: All those retweets may instead owe to the popularity of Trump’s account, or the fact that he writes about politically charged subjects. Without a corresponding set of accurate tweets from Trump, there’s no way of knowing what role misinformation is playing.
For researchers, isolating the effect of misinformation is thus extremely challenging. It’s not often that a user will share both accurate and inaccurate information about the same event, and at nearly the same time.
Yet shortly after the recent attack in Toronto , that is exactly what a CBC journalist did. In the chaotic aftermath of the attack, Natasha Fatah published two competing eyewitness accounts: one (wrongly, as it turned out) identifying the attacker as “angry” and “Middle Eastern,” and another correctly identifying him as “white.”
Fatah’s tweets are by no means definitive, but they do represent a natural experiment of sorts. And the results show just how fast misinformation can travel. As the graphic below illustrates, the initial tweet—which wrongly identified the attacker as Middle Eastern—received far more engagement than the accurate one in the roughly five hours after the attack:
Worse, the tweet containing correct information did not perform much better over a longer time horizon, up to 24 hours after the attack:

(Data and code for the graphics above are available here .)
Taken together, Fatah’s tweets suggest that misinformation on social media genuinely is a problem. As such, they raise two questions: First, why did the incorrect tweet spread so much faster than the correct one? And second, what can be done to prevent the similar spread of misinformation in the future?
The Speed of Misinformation on Twitter
For most of Twitter’s history, its newsfeed was straightforward: The app showed tweets in reverse chronological order. That changed in 2015 with the introduction of Twitter’s an algorithmic newsfeed , which displayed tweets based on a calculation of “ relevance ” rather than recency.
Last year, the company’s engineering team revealed how its current algorithm works . As with Facebook and YouTube , Twitter now relies on a deep learning algorithm that has learned to prioritize content with greater prior engagement. By combing through Twitter’s data, the algorithm has taught itself that Twitter users are more likely to stick around if they see content that has already gotten a lot of retweets and mentions, compared with content that has fewer.
The flow of misinformation on Twitter is thus a function of both human and technical factors. Human biases play an important role : Since we’re more likely to react to content that taps into our existing grievances and beliefs, inflammatory tweets will generate quick engagement. It’s only after that engagement happens that the technical side kicks in: If a tweet is retweeted, favorited, or replied to by enough of its first viewers, the newsfeed algorithm will show it to more users, at which point it will tap into the biases of those users too—prompting even more engagement, and so on. At its worse, this cycle can turn social media into a kind of confirmation bias machine , one perfectly tailored for the spread of misinformation.
If you look at Fatah’s tweets, the process above plays out almost to a tee. A small subset of Fatah’s followers immediately engaged with the tweet reporting a bystander’s account of the attacker as “angry” and “Middle Eastern,” which set off a cycle in which greater engagement begat greater viewership and vice versa. By contrast, the tweet that accurately identified the attacker received little initial engagement, was flagged less by the newsfeed algorithm, and thus never really caught on. The result is the graph above, which shows an exponential increase in engagement for the inaccurate tweet, but only a modest increase for the accurate one.
What To Do About It
Just as the problem has both a human and technical side, so too does any potential solution.
Where Twitter’s algorithms are concerned, there is no shortage of low-hanging fruit. During an attack itself, Twitter could promote police or government accounts so that accurate information is disseminated as quickly as possible. Alternately, it could also display a warning at the top of its search and trending feeds about the unreliability of initial eyewitness accounts.
Even more, Twitter could update its “While You Were Away” and search features. In the case of the Toronto attack, Twitter could not have been expected to identify the truth faster than the Toronto police. But once the police had identified the attacker, Twitter should have had systems in place to restrict the visibility of Fatah’s tweet and other trending misinformation. For example, over ten days after the attack, the top two results for a search of the attacker were these :

(I conducted the above search while logged into my own Twitter account, but a search while logged out produced the same results.)
Unfortunately, these were not isolated tweets. Anyone using Twitter to follow and learn about the attack has been greeted with a wealth of misinformation and invective . This is something Twitter can combat: Either it can hire an editorial team to track and remove blatant misinformation from trending searches, or it can introduce a new reporting feature for users to flag misinformation as they come across it. Neither option is perfect, and the latter would not be trivial to implement. But the status quo is worse. How many Twitter users continue to think the Toronto attack was the work of Middle Eastern jihadists, and that Prime Minister Justin Trudeau’s immigration policies are to blame?
Ultimately, however, the solution to misinformation will also need to involve the users themselves. Not only do Twitter’s users need to better understand their own biases, but journalists in particular need to better understand how their mistakes can be exploited. In this case, the biggest errors were human ones: Fatah tweeted out an account without corroborating it, even though the eyewitness in question, a man named David Leonard, himself noted that “I can’t confirm or deny whether my observation is correct.”
To counter misinformation online, we can and should demand that newsfeed algorithms not amplify our worst instincts. But we can’t expect them to save us from ourselves.
Related Content
Chris Meserole
April 25, 2018
Media & Journalism
Foreign Policy
Center for Middle East Policy
Daniel S. Schiff, Kaylyn Jackson Schiff, Natália Bueno
May 30, 2024
Courtney C. Radsch
March 25, 2024
Valerie Wirtschafter
October 26, 2023
June 21, 2018
Biases Make People Vulnerable to Misinformation Spread by Social Media
Researchers have developed tools to study the cognitive, societal and algorithmic biases that help fake news spread
By Giovanni Luca Ciampaglia , Filippo Menczer & The Conversation US
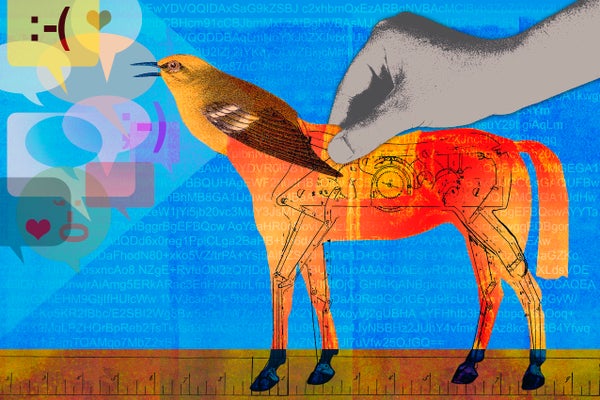
Roy Scott Getty Images
The following essay is reprinted with permission from The Conversation , an online publication covering the latest research.
Social media are among the primary sources of news in the U.S. and across the world. Yet users are exposed to content of questionable accuracy, including conspiracy theories , clickbait , hyperpartisan content , pseudo science and even fabricated “fake news” reports .
It’s not surprising that there’s so much disinformation published: Spam and online fraud are lucrative for criminals , and government and political propaganda yield both partisan and financial benefits . But the fact that low-credibility content spreads so quickly and easily suggests that people and the algorithms behind social media platforms are vulnerable to manipulation.
On supporting science journalism
If you're enjoying this article, consider supporting our award-winning journalism by subscribing . By purchasing a subscription you are helping to ensure the future of impactful stories about the discoveries and ideas shaping our world today.
Explaining the tools developed at the Observatory on Social Media.
Our research has identified three types of bias that make the social media ecosystem vulnerable to both intentional and accidental misinformation. That is why our Observatory on Social Media at Indiana University is building tools to help people become aware of these biases and protect themselves from outside influences designed to exploit them.
Bias in the brain
Cognitive biases originate in the way the brain processes the information that every person encounters every day. The brain can deal with only a finite amount of information, and too many incoming stimuli can cause information overload . That in itself has serious implications for the quality of information on social media. We have found that steep competition for users’ limited attention means that some ideas go viral despite their low quality —even when people prefer to share high-quality content.*
To avoid getting overwhelmed, the brain uses a number of tricks . These methods are usually effective, but may also become biases when applied in the wrong contexts.
One cognitive shortcut happens when a person is deciding whether to share a story that appears on their social media feed. People are very affected by the emotional connotations of a headline , even though that’s not a good indicator of an article’s accuracy. Much more important is who wrote the piece .
To counter this bias, and help people pay more attention to the source of a claim before sharing it, we developed Fakey , a mobile news literacy game (free on Android and iOS ) simulating a typical social media news feed, with a mix of news articles from mainstream and low-credibility sources. Players get more points for sharing news from reliable sources and flagging suspicious content for fact-checking. In the process, they learn to recognize signals of source credibility, such as hyperpartisan claims and emotionally charged headlines.
Bias in society
Another source of bias comes from society. When people connect directly with their peers, the social biases that guide their selection of friends come to influence the information they see.
In fact, in our research we have found that it is possible to determine the political leanings of a Twitter user by simply looking at the partisan preferences of their friends. Our analysis of the structure of these partisan communication networks found social networks are particularly efficient at disseminating information – accurate or not – when they are closely tied together and disconnected from other parts of society .
The tendency to evaluate information more favorably if it comes from within their own social circles creates “ echo chambers ” that are ripe for manipulation, either consciously or unintentionally. This helps explain why so many online conversations devolve into “us versus them” confrontations .
To study how the structure of online social networks makes users vulnerable to disinformation, we built Hoaxy , a system that tracks and visualizes the spread of content from low-credibility sources, and how it competes with fact-checking content. Our analysis of the data collected by Hoaxy during the 2016 U.S. presidential elections shows that Twitter accounts that shared misinformation were almost completely cut off from the corrections made by the fact-checkers.
When we drilled down on the misinformation-spreading accounts, we found a very dense core group of accounts retweeting each other almost exclusively – including several bots. The only times that fact-checking organizations were ever quoted or mentioned by the users in the misinformed group were when questioning their legitimacy or claiming the opposite of what they wrote.
Bias in the machine
The third group of biases arises directly from the algorithms used to determine what people see online. Both social media platforms and search engines employ them. These personalization technologies are designed to select only the most engaging and relevant content for each individual user. But in doing so, it may end up reinforcing the cognitive and social biases of users, thus making them even more vulnerable to manipulation.
For instance, the detailed advertising tools built into many social media platforms let disinformation campaigners exploit confirmation bias by tailoring messages to people who are already inclined to believe them.
Also, if a user often clicks on Facebook links from a particular news source, Facebook will tend to show that person more of that site’s content . This so-called “ filter bubble ” effect may isolate people from diverse perspectives, strengthening confirmation bias.
Our own research shows that social media platforms expose users to a less diverse set of sources than do non-social media sites like Wikipedia. Because this is at the level of a whole platform, not of a single user, we call this the homogeneity bias .
Another important ingredient of social media is information that is trending on the platform, according to what is getting the most clicks. We call this popularity bias , because we have found that an algorithm designed to promote popular content may negatively affect the overall quality of information on the platform. This also feeds into existing cognitive bias, reinforcing what appears to be popular irrespective of its quality.
All these algorithmic biases can be manipulated by social bots , computer programs that interact with humans through social media accounts. Most social bots, like Twitter’s Big Ben , are harmless. However, some conceal their real nature and are used for malicious intents, such as boosting disinformation or falsely creating the appearance of a grassroots movement , also called “astroturfing.” We found evidence of this type of manipulation in the run-up to the 2010 U.S. midterm election.
To study these manipulation strategies, we developed a tool to detect social bots called Botometer . Botometer uses machine learning to detect bot accounts, by inspecting thousands of different features of Twitter accounts, like the times of its posts, how often it tweets, and the accounts it follows and retweets. It is not perfect, but it has revealed that as many as 15 percent of Twitter accounts show signs of being bots .
Using Botometer in conjunction with Hoaxy, we analyzed the core of the misinformation network during the 2016 U.S. presidential campaign. We found many bots exploiting both the cognitive, confirmation and popularity biases of their victims and Twitter’s algorithmic biases.
These bots are able to construct filter bubbles around vulnerable users, feeding them false claims and misinformation. First, they can attract the attention of human users who support a particular candidate by tweeting that candidate’s hashtags or by mentioning and retweeting the person. Then the bots can amplify false claims smearing opponents by retweeting articles from low-credibility sources that match certain keywords. This activity also makes the algorithm highlight for other users false stories that are being shared widely.
Understanding complex vulnerabilities
Even as our research, and others’, shows how individuals, institutions and even entire societies can be manipulated on social media, there are many questions left to answer. It’s especially important to discover how these different biases interact with each other, potentially creating more complex vulnerabilities.
Tools like ours offer internet users more information about disinformation, and therefore some degree of protection from its harms. The solutions will not likely be only technological , though there will probably be some technical aspects to them. But they must take into account the cognitive and social aspects of the problem.
*Editor’s note: This article was updated on Jan. 10, 2019, to remove a link to a study that has been retracted. The text of the article is still accurate, and remains unchanged.
This article was originally published on The Conversation . Read the original article .
- Follow us on Facebook
- Follow us on Twitter
- Criminal Justice
- Environment
- Politics & Government
- Race & Gender
Expert Commentary
Fake news and the spread of misinformation: A research roundup
This collection of research offers insights into the impacts of fake news and other forms of misinformation, including fake Twitter images, and how people use the internet to spread rumors and misinformation.
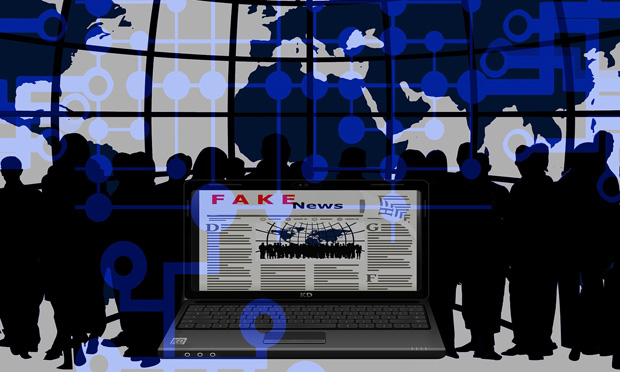
Republish this article

This work is licensed under a Creative Commons Attribution-NoDerivatives 4.0 International License .
by Denise-Marie Ordway, The Journalist's Resource September 1, 2017
This <a target="_blank" href="https://journalistsresource.org/politics-and-government/fake-news-conspiracy-theories-journalism-research/">article</a> first appeared on <a target="_blank" href="https://journalistsresource.org">The Journalist's Resource</a> and is republished here under a Creative Commons license.<img src="https://journalistsresource.org/wp-content/uploads/2020/11/cropped-jr-favicon-150x150.png" style="width:1em;height:1em;margin-left:10px;">
It’s too soon to say whether Google ’s and Facebook ’s attempts to clamp down on fake news will have a significant impact. But fabricated stories posing as serious journalism are not likely to go away as they have become a means for some writers to make money and potentially influence public opinion. Even as Americans recognize that fake news causes confusion about current issues and events, they continue to circulate it. A December 2016 survey by the Pew Research Center suggests that 23 percent of U.S. adults have shared fake news, knowingly or unknowingly, with friends and others.
“Fake news” is a term that can mean different things, depending on the context. News satire is often called fake news as are parodies such as the “Saturday Night Live” mock newscast Weekend Update. Much of the fake news that flooded the internet during the 2016 election season consisted of written pieces and recorded segments promoting false information or perpetuating conspiracy theories. Some news organizations published reports spotlighting examples of hoaxes, fake news and misinformation on Election Day 2016.
The news media has written a lot about fake news and other forms of misinformation, but scholars are still trying to understand it — for example, how it travels and why some people believe it and even seek it out. Below, Journalist’s Resource has pulled together academic studies to help newsrooms better understand the problem and its impacts. Two other resources that may be helpful are the Poynter Institute’s tips on debunking fake news stories and the First Draft Partner Network , a global collaboration of newsrooms, social media platforms and fact-checking organizations that was launched in September 2016 to battle fake news. In mid-2018, JR ‘s managing editor, Denise-Marie Ordway, wrote an article for Harvard Business Review explaining what researchers know to date about the amount of misinformation people consume, why they believe it and the best ways to fight it.
—————————
“The Science of Fake News” Lazer, David M. J.; et al. Science , March 2018. DOI: 10.1126/science.aao2998.
Summary: “The rise of fake news highlights the erosion of long-standing institutional bulwarks against misinformation in the internet age. Concern over the problem is global. However, much remains unknown regarding the vulnerabilities of individuals, institutions, and society to manipulations by malicious actors. A new system of safeguards is needed. Below, we discuss extant social and computer science research regarding belief in fake news and the mechanisms by which it spreads. Fake news has a long history, but we focus on unanswered scientific questions raised by the proliferation of its most recent, politically oriented incarnation. Beyond selected references in the text, suggested further reading can be found in the supplementary materials.”
“Who Falls for Fake News? The Roles of Bullshit Receptivity, Overclaiming, Familiarity, and Analytical Thinking” Pennycook, Gordon; Rand, David G. May 2018. Available at SSRN. DOI: 10.2139/ssrn.3023545.
Abstract: “Inaccurate beliefs pose a threat to democracy and fake news represents a particularly egregious and direct avenue by which inaccurate beliefs have been propagated via social media. Here we present three studies (MTurk, N = 1,606) investigating the cognitive psychological profile of individuals who fall prey to fake news. We find consistent evidence that the tendency to ascribe profundity to randomly generated sentences — pseudo-profound bullshit receptivity — correlates positively with perceptions of fake news accuracy, and negatively with the ability to differentiate between fake and real news (media truth discernment). Relatedly, individuals who overclaim regarding their level of knowledge (i.e. who produce bullshit) also perceive fake news as more accurate. Conversely, the tendency to ascribe profundity to prototypically profound (non-bullshit) quotations is not associated with media truth discernment; and both profundity measures are positively correlated with willingness to share both fake and real news on social media. We also replicate prior results regarding analytic thinking — which correlates negatively with perceived accuracy of fake news and positively with media truth discernment — and shed further light on this relationship by showing that it is not moderated by the presence versus absence of information about the new headline’s source (which has no effect on perceived accuracy), or by prior familiarity with the news headlines (which correlates positively with perceived accuracy of fake and real news). Our results suggest that belief in fake news has similar cognitive properties to other forms of bullshit receptivity, and reinforce the important role that analytic thinking plays in the recognition of misinformation.”
“Social Media and Fake News in the 2016 Election” Allcott, Hunt; Gentzkow, Matthew. Working paper for the National Bureau of Economic Research, No. 23089, 2017.
Abstract: “We present new evidence on the role of false stories circulated on social media prior to the 2016 U.S. presidential election. Drawing on audience data, archives of fact-checking websites, and results from a new online survey, we find: (i) social media was an important but not dominant source of news in the run-up to the election, with 14 percent of Americans calling social media their “most important” source of election news; (ii) of the known false news stories that appeared in the three months before the election, those favoring Trump were shared a total of 30 million times on Facebook, while those favoring Clinton were shared eight million times; (iii) the average American saw and remembered 0.92 pro-Trump fake news stories and 0.23 pro-Clinton fake news stories, with just over half of those who recalled seeing fake news stories believing them; (iv) for fake news to have changed the outcome of the election, a single fake article would need to have had the same persuasive effect as 36 television campaign ads.”
“Debunking: A Meta-Analysis of the Psychological Efficacy of Messages Countering Misinformation” Chan, Man-pui Sally; Jones, Christopher R.; Jamieson, Kathleen Hall; Albarracín, Dolores. Psychological Science , September 2017. DOI: 10.1177/0956797617714579.
Abstract: “This meta-analysis investigated the factors underlying effective messages to counter attitudes and beliefs based on misinformation. Because misinformation can lead to poor decisions about consequential matters and is persistent and difficult to correct, debunking it is an important scientific and public-policy goal. This meta-analysis (k = 52, N = 6,878) revealed large effects for presenting misinformation (ds = 2.41–3.08), debunking (ds = 1.14–1.33), and the persistence of misinformation in the face of debunking (ds = 0.75–1.06). Persistence was stronger and the debunking effect was weaker when audiences generated reasons in support of the initial misinformation. A detailed debunking message correlated positively with the debunking effect. Surprisingly, however, a detailed debunking message also correlated positively with the misinformation-persistence effect.”
“Displacing Misinformation about Events: An Experimental Test of Causal Corrections” Nyhan, Brendan; Reifler, Jason. Journal of Experimental Political Science , 2015. doi: 10.1017/XPS.2014.22.
Abstract: “Misinformation can be very difficult to correct and may have lasting effects even after it is discredited. One reason for this persistence is the manner in which people make causal inferences based on available information about a given event or outcome. As a result, false information may continue to influence beliefs and attitudes even after being debunked if it is not replaced by an alternate causal explanation. We test this hypothesis using an experimental paradigm adapted from the psychology literature on the continued influence effect and find that a causal explanation for an unexplained event is significantly more effective than a denial even when the denial is backed by unusually strong evidence. This result has significant implications for how to most effectively counter misinformation about controversial political events and outcomes.”
“Rumors and Health Care Reform: Experiments in Political Misinformation” Berinsky, Adam J. British Journal of Political Science , 2015. doi: 10.1017/S0007123415000186.
Abstract: “This article explores belief in political rumors surrounding the health care reforms enacted by Congress in 2010. Refuting rumors with statements from unlikely sources can, under certain circumstances, increase the willingness of citizens to reject rumors regardless of their own political predilections. Such source credibility effects, while well known in the political persuasion literature, have not been applied to the study of rumor. Though source credibility appears to be an effective tool for debunking political rumors, risks remain. Drawing upon research from psychology on ‘fluency’ — the ease of information recall — this article argues that rumors acquire power through familiarity. Attempting to quash rumors through direct refutation may facilitate their diffusion by increasing fluency. The empirical results find that merely repeating a rumor increases its power.”
“Rumors and Factitious Informational Blends: The Role of the Web in Speculative Politics” Rojecki, Andrew; Meraz, Sharon. New Media & Society , 2016. doi: 10.1177/1461444814535724.
Abstract: “The World Wide Web has changed the dynamics of information transmission and agenda-setting. Facts mingle with half-truths and untruths to create factitious informational blends (FIBs) that drive speculative politics. We specify an information environment that mirrors and contributes to a polarized political system and develop a methodology that measures the interaction of the two. We do so by examining the evolution of two comparable claims during the 2004 presidential campaign in three streams of data: (1) web pages, (2) Google searches, and (3) media coverage. We find that the web is not sufficient alone for spreading misinformation, but it leads the agenda for traditional media. We find no evidence for equality of influence in network actors.”
“Analyzing How People Orient to and Spread Rumors in Social Media by Looking at Conversational Threads” Zubiaga, Arkaitz; et al. PLOS ONE, 2016. doi: 10.1371/journal.pone.0150989.
Abstract: “As breaking news unfolds people increasingly rely on social media to stay abreast of the latest updates. The use of social media in such situations comes with the caveat that new information being released piecemeal may encourage rumors, many of which remain unverified long after their point of release. Little is known, however, about the dynamics of the life cycle of a social media rumor. In this paper we present a methodology that has enabled us to collect, identify and annotate a dataset of 330 rumor threads (4,842 tweets) associated with 9 newsworthy events. We analyze this dataset to understand how users spread, support, or deny rumors that are later proven true or false, by distinguishing two levels of status in a rumor life cycle i.e., before and after its veracity status is resolved. The identification of rumors associated with each event, as well as the tweet that resolved each rumor as true or false, was performed by journalist members of the research team who tracked the events in real time. Our study shows that rumors that are ultimately proven true tend to be resolved faster than those that turn out to be false. Whilst one can readily see users denying rumors once they have been debunked, users appear to be less capable of distinguishing true from false rumors when their veracity remains in question. In fact, we show that the prevalent tendency for users is to support every unverified rumor. We also analyze the role of different types of users, finding that highly reputable users such as news organizations endeavor to post well-grounded statements, which appear to be certain and accompanied by evidence. Nevertheless, these often prove to be unverified pieces of information that give rise to false rumors. Our study reinforces the need for developing robust machine learning techniques that can provide assistance in real time for assessing the veracity of rumors. The findings of our study provide useful insights for achieving this aim.”
“Miley, CNN and The Onion” Berkowitz, Dan; Schwartz, David Asa. Journalism Practice , 2016. doi: 10.1080/17512786.2015.1006933.
Abstract: “Following a twerk-heavy performance by Miley Cyrus on the Video Music Awards program, CNN featured the story on the top of its website. The Onion — a fake-news organization — then ran a satirical column purporting to be by CNN’s Web editor explaining this decision. Through textual analysis, this paper demonstrates how a Fifth Estate comprised of bloggers, columnists and fake news organizations worked to relocate mainstream journalism back to within its professional boundaries.”
“Emotions, Partisanship, and Misperceptions: How Anger and Anxiety Moderate the Effect of Partisan Bias on Susceptibility to Political Misinformation”
Weeks, Brian E. Journal of Communication , 2015. doi: 10.1111/jcom.12164.
Abstract: “Citizens are frequently misinformed about political issues and candidates but the circumstances under which inaccurate beliefs emerge are not fully understood. This experimental study demonstrates that the independent experience of two emotions, anger and anxiety, in part determines whether citizens consider misinformation in a partisan or open-minded fashion. Anger encourages partisan, motivated evaluation of uncorrected misinformation that results in beliefs consistent with the supported political party, while anxiety at times promotes initial beliefs based less on partisanship and more on the information environment. However, exposure to corrections improves belief accuracy, regardless of emotion or partisanship. The results indicate that the unique experience of anger and anxiety can affect the accuracy of political beliefs by strengthening or attenuating the influence of partisanship.”
“Deception Detection for News: Three Types of Fakes” Rubin, Victoria L.; Chen, Yimin; Conroy, Niall J. Proceedings of the Association for Information Science and Technology , 2015, Vol. 52. doi: 10.1002/pra2.2015.145052010083.
Abstract: “A fake news detection system aims to assist users in detecting and filtering out varieties of potentially deceptive news. The prediction of the chances that a particular news item is intentionally deceptive is based on the analysis of previously seen truthful and deceptive news. A scarcity of deceptive news, available as corpora for predictive modeling, is a major stumbling block in this field of natural language processing (NLP) and deception detection. This paper discusses three types of fake news, each in contrast to genuine serious reporting, and weighs their pros and cons as a corpus for text analytics and predictive modeling. Filtering, vetting, and verifying online information continues to be essential in library and information science (LIS), as the lines between traditional news and online information are blurring.”
“When Fake News Becomes Real: Combined Exposure to Multiple News Sources and Political Attitudes of Inefficacy, Alienation, and Cynicism” Balmas, Meital. Communication Research , 2014, Vol. 41. doi: 10.1177/0093650212453600.
Abstract: “This research assesses possible associations between viewing fake news (i.e., political satire) and attitudes of inefficacy, alienation, and cynicism toward political candidates. Using survey data collected during the 2006 Israeli election campaign, the study provides evidence for an indirect positive effect of fake news viewing in fostering the feelings of inefficacy, alienation, and cynicism, through the mediator variable of perceived realism of fake news. Within this process, hard news viewing serves as a moderator of the association between viewing fake news and their perceived realism. It was also demonstrated that perceived realism of fake news is stronger among individuals with high exposure to fake news and low exposure to hard news than among those with high exposure to both fake and hard news. Overall, this study contributes to the scientific knowledge regarding the influence of the interaction between various types of media use on political effects.”
“Faking Sandy: Characterizing and Identifying Fake Images on Twitter During Hurricane Sandy” Gupta, Aditi; Lamba, Hemank; Kumaraguru, Ponnurangam; Joshi, Anupam. Proceedings of the 22nd International Conference on World Wide Web , 2013. doi: 10.1145/2487788.2488033.
Abstract: “In today’s world, online social media plays a vital role during real world events, especially crisis events. There are both positive and negative effects of social media coverage of events. It can be used by authorities for effective disaster management or by malicious entities to spread rumors and fake news. The aim of this paper is to highlight the role of Twitter during Hurricane Sandy (2012) to spread fake images about the disaster. We identified 10,350 unique tweets containing fake images that were circulated on Twitter during Hurricane Sandy. We performed a characterization analysis, to understand the temporal, social reputation and influence patterns for the spread of fake images. Eighty-six percent of tweets spreading the fake images were retweets, hence very few were original tweets. Our results showed that the top 30 users out of 10,215 users (0.3 percent) resulted in 90 percent of the retweets of fake images; also network links such as follower relationships of Twitter, contributed very little (only 11 percent) to the spread of these fake photos URLs. Next, we used classification models, to distinguish fake images from real images of Hurricane Sandy. Best results were obtained from Decision Tree classifier, we got 97 percent accuracy in predicting fake images from real. Also, tweet-based features were very effective in distinguishing fake images tweets from real, while the performance of user-based features was very poor. Our results showed that automated techniques can be used in identifying real images from fake images posted on Twitter.”
“The Impact of Real News about ‘Fake News’: Intertextual Processes and Political Satire” Brewer, Paul R.; Young, Dannagal Goldthwaite; Morreale, Michelle. International Journal of Public Opinion Research , 2013. doi: 10.1093/ijpor/edt015.
Abstract: “This study builds on research about political humor, press meta-coverage, and intertextuality to examine the effects of news coverage about political satire on audience members. The analysis uses experimental data to test whether news coverage of Stephen Colbert’s Super PAC influenced knowledge and opinion regarding Citizens United, as well as political trust and internal political efficacy. It also tests whether such effects depended on previous exposure to The Colbert Report (Colbert’s satirical television show) and traditional news. Results indicate that exposure to news coverage of satire can influence knowledge, opinion, and political trust. Additionally, regular satire viewers may experience stronger effects on opinion, as well as increased internal efficacy, when consuming news coverage about issues previously highlighted in satire programming.”
“With Facebook, Blogs, and Fake News, Teens Reject Journalistic ‘Objectivity’” Marchi, Regina. Journal of Communication Inquiry , 2012. doi: 10.1177/0196859912458700.
Abstract: “This article examines the news behaviors and attitudes of teenagers, an understudied demographic in the research on youth and news media. Based on interviews with 61 racially diverse high school students, it discusses how adolescents become informed about current events and why they prefer certain news formats to others. The results reveal changing ways news information is being accessed, new attitudes about what it means to be informed, and a youth preference for opinionated rather than objective news. This does not indicate that young people disregard the basic ideals of professional journalism but, rather, that they desire more authentic renderings of them.”
Keywords: alt-right, credibility, truth discovery, post-truth era, fact checking, news sharing, news literacy, misinformation, disinformation
5 fascinating digital media studies from fall 2018
Facebook and the newsroom: 6 questions for Siva Vaidhyanathan
About The Author
Denise-Marie Ordway
Featured Articles
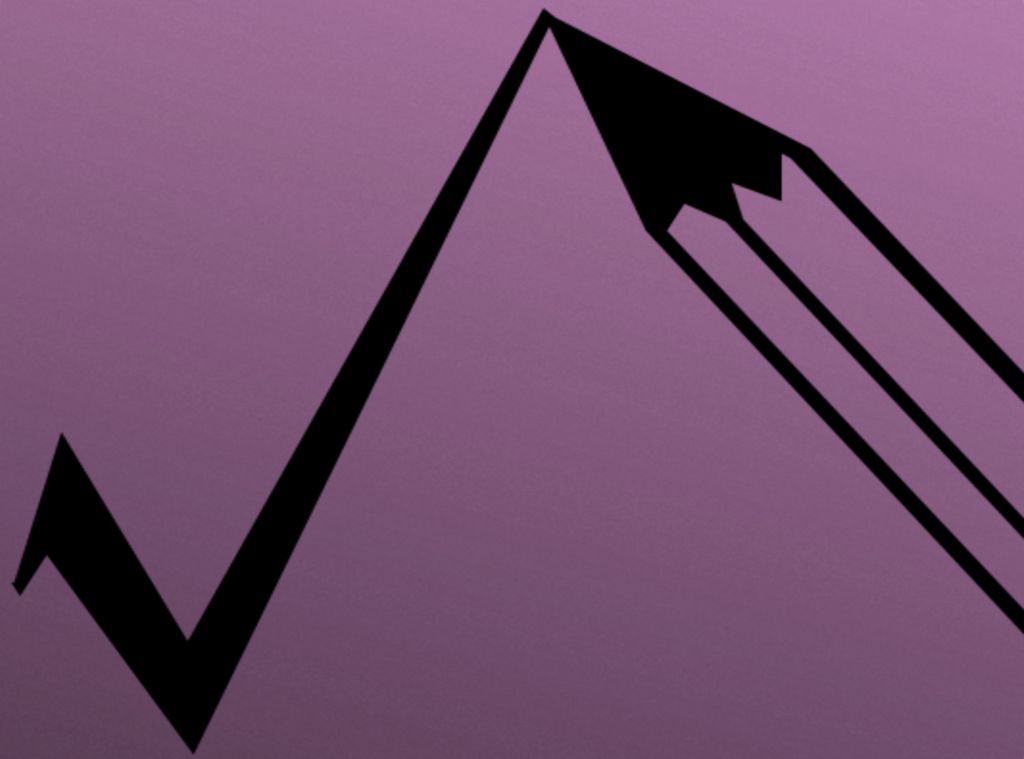
June 5, 2024
Who reports witnessing and performing corrections on social media in the United States, United Kingdom, Canada, and France?
Rongwei Tang, Emily K. Vraga, Leticia Bode and Shelley Boulianne
Observed corrections of misinformation on social media can encourage more accurate beliefs, but for these benefits to occur, corrections must happen. By exploring people’s perceptions of witnessing and performing corrections on social media, we find that many people say they observe and perform corrections across the United States, the United Kingdom, Canada, and France.
Read the Essay
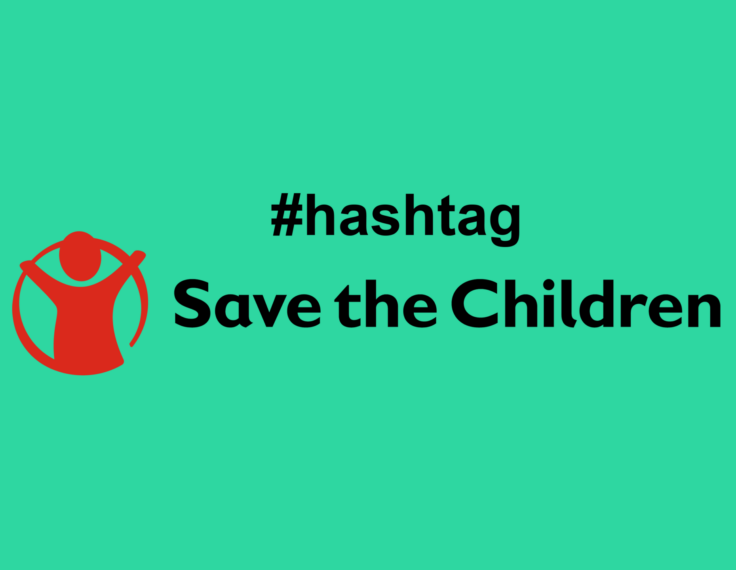
#SaveTheChildren: A pilot study of a social media movement co-opted by conspiracy theorists
Katherine M. FitzGerald and Timothy Graham
In a preliminary analysis of 121,984 posts from X (formerly known as Twitter) containing the hashtag #SaveTheChildren, we found that conspiratorial posts received more engagement than authentic hashtag activism between January 2022 and March 2023. Conspiratorial posts received twice the number of reposts as non-conspiratorial content.
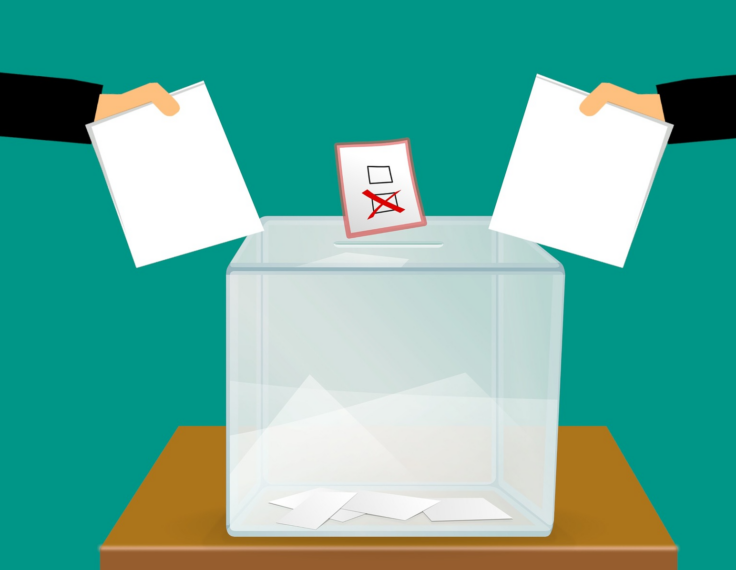
US-skepticism and transnational conspiracy in the 2024 Taiwanese presidential election
Ho-Chun Herbert Chang, Austin Horng-En Wang and Yu Sunny Fang
Taiwan has one of the highest freedom of speech indexes while it also encounters the largest amount of foreign interference due to its contentious history with China. Because of the large influx of misinformation, Taiwan has taken a public crowdsourcing approach to combatting misinformation, using both fact-checking ChatBots and public dataset called CoFacts.
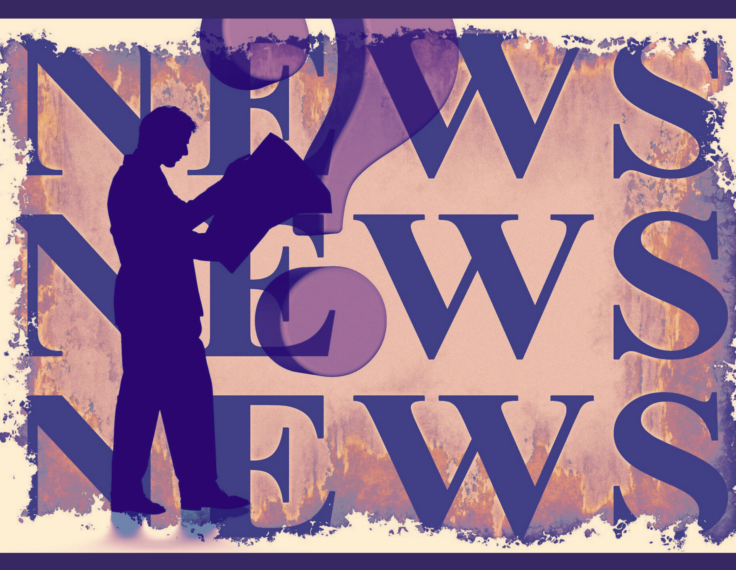
Misinformation perceived as a bigger informational threat than negativity: A cross-country survey on challenges of the news environment
Toni G. L. A. van der Meer and Michael Hameleers
This study integrates research on negativity bias and misinformation, as a comparison of how systematic (negativity) and incidental (misinformation) challenges to the news are perceived differently by audiences. Through a cross-country survey, we found that both challenges are perceived as highly salient and disruptive.
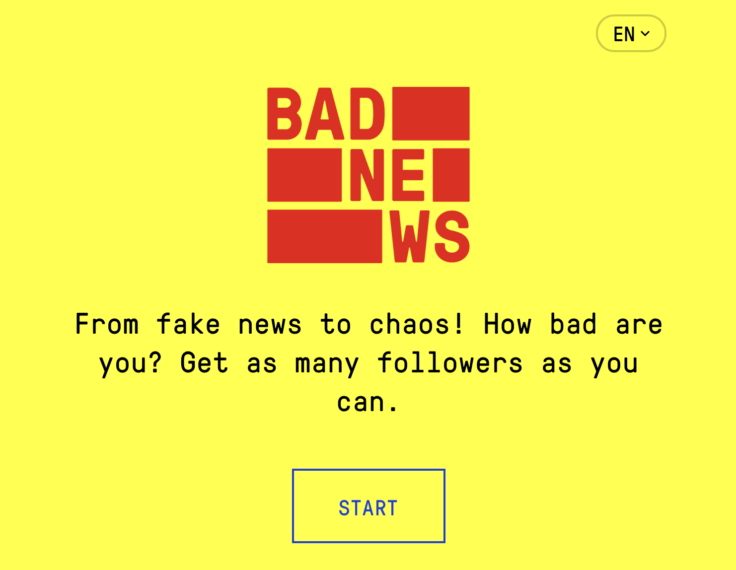
Gamified inoculation reduces susceptibility to misinformation from political ingroups
Cecilie Steenbuch Traberg, Jon Roozenbeek and Sander van der Linden
Psychological inoculation interventions, which seek to pre-emptively build resistance against unwanted persuasion attempts, have shown promise in reducing susceptibility to misinformation. However, as many people receive news from popular, mainstream ingroup sources (e.g., a left-wing person consuming left-wing media) which may host misleading or false content, and as ingroup sources may be more persuasive, the impact of source effects on inoculation interventions demands attention.
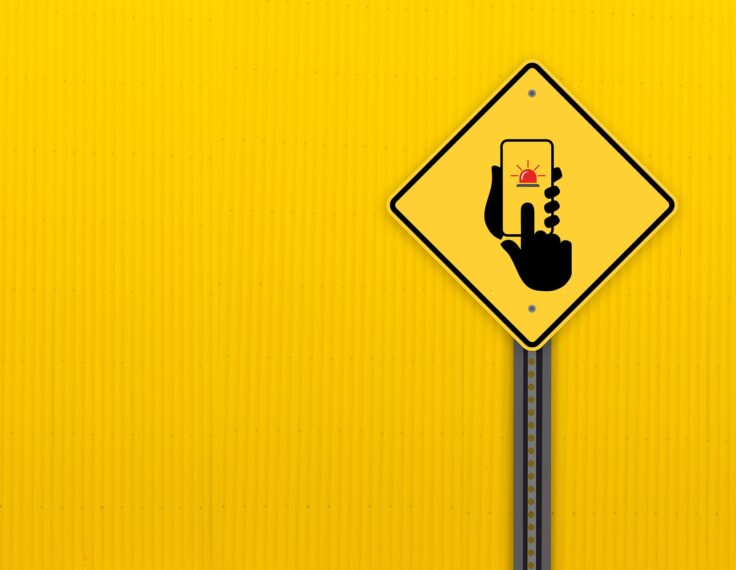
Journalistic interventions matter: Understanding how Americans perceive fact-checking labels
Chenyan Jia and Taeyoung Lee
While algorithms and crowdsourcing have been increasingly used to debunk or label misinformation on social media, such tasks might be most effective when performed by professional fact checkers or journalists. Drawing on a national survey (N = 1,003), we found that U.S. adults evaluated fact-checking labels created by professional fact checkers as more effective than labels by algorithms and other users. News
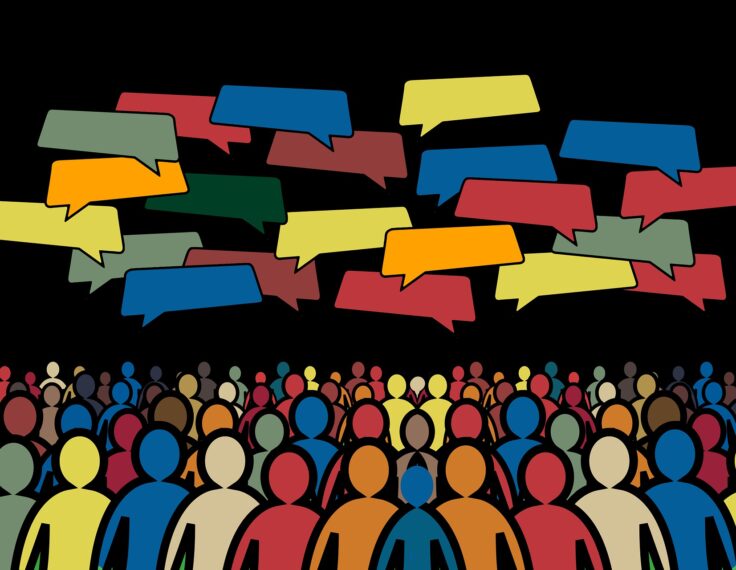
Brazilian Capitol attack: The interaction between Bolsonaro’s supporters’ content, WhatsApp, Twitter, and news media
Joao V. S. Ozawa, Josephine Lukito, Felipe Bailez and Luis G. P. Fakhouri
Bolsonaro’s supporters used social media to spread content during key events related to the Brasília attack. An unprecedented analysis of more than 15,000 public WhatsApp groups showed that these political actors tried to manufacture consensus in preparation for and after the attack. A cross-platform time series analysis showed that the spread of content on Twitter predicted the spread of content on WhatsApp.
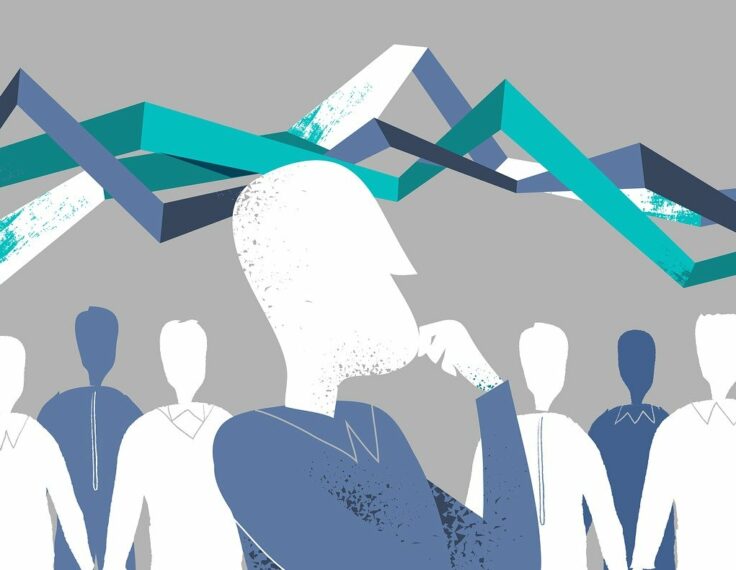
Fact-opinion differentiation
Matthew Mettler and Jeffery J. Mondak
Statements of fact can be proved or disproved with objective evidence, whereas statements of opinion depend on personal values and preferences. Distinguishing between these types of statements contributes to information competence. Conversely, failure at fact-opinion differentiation potentially brings resistance to corrections of misinformation and susceptibility to manipulation.
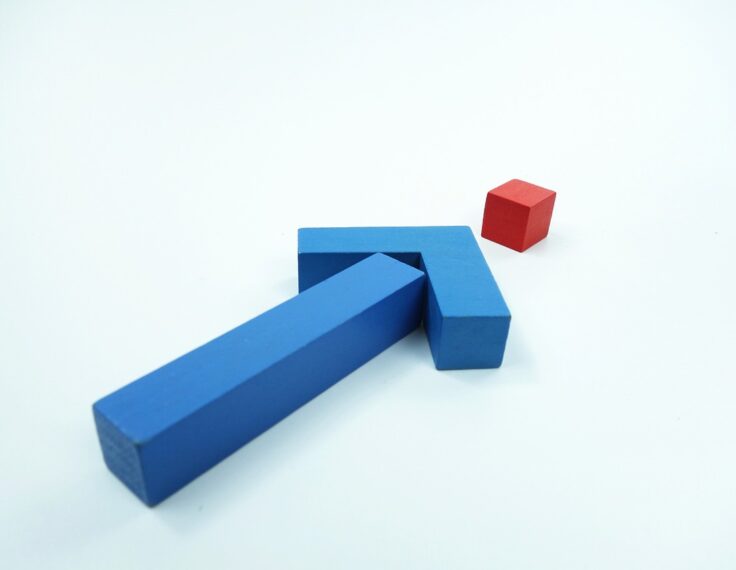
Debunking and exposing misinformation among fringe communities: Testing source exposure and debunking anti-Ukrainian misinformation among German fringe communities
Johannes Christiern Santos Okholm, Amir Ebrahimi Fard and Marijn ten Thij
Through an online field experiment, we test traditional and novel counter-misinformation strategies among fringe communities. Though generally effective, traditional strategies have not been tested in fringe communities, and do not address the online infrastructure of misinformation sources supporting such consumption. Instead, we propose to activate source criticism by exposing sources’ unreliability.
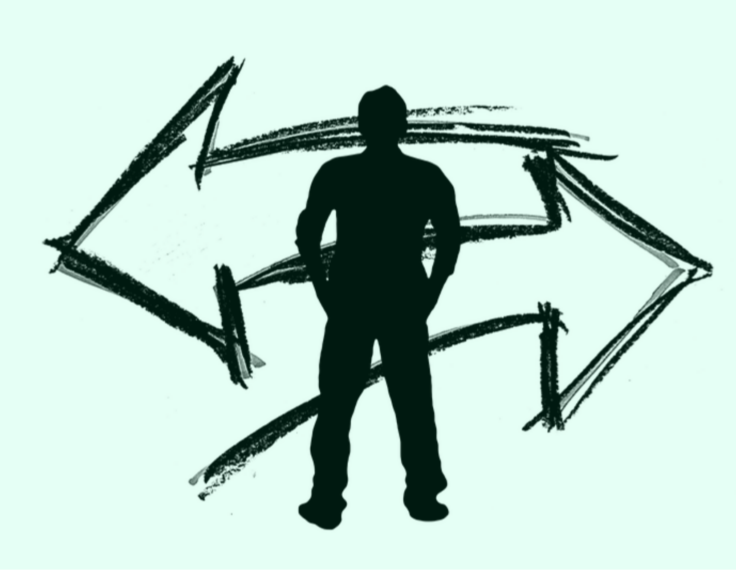
Seeing lies and laying blame: Partisanship and U.S. public perceptions about disinformation
Kaitlin Peach, Joseph Ripberger, Kuhika Gupta, Andrew Fox, Hank Jenkins-Smith and Carol Silva
Using data from a nationally representative survey of 2,036 U.S. adults, we analyze partisan perceptions of the risk disinformation poses to the U.S. government and society, as well as the actors viewed as responsible for and harmed by disinformation. Our findings indicate relatively high concern about disinformation across a variety of societal issues, with broad bipartisan agreement that disinformation poses significant risks and causes harms to several groups.
- Share full article
Advertisement
How Social Media Amplifies Misinformation More Than Information
A new analysis found that algorithms and some features of social media sites help false posts go viral.

By Steven Lee Myers
- Oct. 13, 2022
It is well known that social media amplifies misinformation and other harmful content. The Integrity Institute, an advocacy group, is now trying to measure exactly how much — and on Thursday it began publishing results that it plans to update each week through the midterm elections on Nov. 8.
The institute’s initial report, posted online , found that a “well-crafted lie” will get more engagements than typical, truthful content and that some features of social media sites and their algorithms contribute to the spread of misinformation.
Twitter, the analysis showed, has what the institute called the great misinformation amplification factor, in large part because of its feature allowing people to share, or “retweet,” posts easily. It was followed by TikTok, the Chinese-owned video site, which uses machine-learning models to predict engagement and make recommendations to users.
“We see a difference for each platform because each platform has different mechanisms for virality on it,” said Jeff Allen, a former integrity officer at Facebook and a founder and the chief research officer at the Integrity Institute. “The more mechanisms there are for virality on the platform, the more we see misinformation getting additional distribution.”
The institute calculated its findings by comparing posts that members of the International Fact-Checking Network have identified as false with the engagement of previous posts that were not flagged from the same accounts. It analyzed nearly 600 fact-checked posts in September on a variety of subjects, including the Covid-19 pandemic, the war in Ukraine and the upcoming elections.
Facebook, according to the sample that the institute has studied so far, had the most instances of misinformation but amplified such claims to a lesser degree, in part because sharing posts requires more steps. But some of its newer features are more prone to amplify misinformation, the institute found.
Facebook’s amplification factor of video content alone is closer to TikTok’s, the institute found. That’s because the platform’s Reels and Facebook Watch, which are video features, “both rely heavily on algorithmic content recommendations” based on engagements, according to the institute’s calculations.
Instagram, which like Facebook is owned by Meta, had the lowest amplification rate. There was not yet sufficient data to make a statistically significant estimate for YouTube, according to the institute.
The institute plans to update its findings to track how the amplification fluctuates, especially as the midterm elections near. Misinformation, the institute’s report said, is much more likely to be shared than merely factual content.
“Amplification of misinformation can rise around critical events if misinformation narratives take hold,” the report said. “It can also fall, if platforms implement design changes around the event that reduce the spread of misinformation.”
Steven Lee Myers covers misinformation for The Times. He has worked in Washington, Moscow, Baghdad and Beijing, where he contributed to the articles that won the Pulitzer Prize for public service in 2021. He is also the author of “The New Tsar: The Rise and Reign of Vladimir Putin.” More about Steven Lee Myers

- Expert Advisory Panel
- Our partners
- Become a partner
- Advice for parents and carers
- Advice for professionals
- Connecting Safely Online
- Fostering Digital Skills
- UKCIS Vulnerable Users Working Group
- Online hate
- Online grooming
- Fake news and misinformation
- Screen time
- Inappropriate content
- Cyberbullying
- Online reputation
- Online Pornography
- Radicalisation
- Privacy and identity theft
- Report issue
- Pre-school (0-5)
- Young Children (6-10)
- Pre-teen (11-13)
- Teens ( 14+)
- Social media privacy guides
- Gaming platforms and devices
- Smartphones and other devices
- Broadband & mobile networks
- Entertainment & search engines
- Get smart about smartphones
- My Family’s Digital Toolkit
- Navigating teens’ online relationships
- Online gaming advice hub
- Social media advice hub
- Press Start for PlayStation Safety
- Guide to apps
- Digital resilience toolkit
- Online money management guide
- The dangers of digital piracy
- Guide to buying tech
- UKCIS Digital Passport
- Online safety leaflets & resources
- Digital wellbeing research programme
- Parent Stories
- Expert opinion
- Press releases
- Our expert panel
- Free digital stories and lessons
- Early years
- Primary school
- Secondary school
- Connect school to home
- Professional guidance
- Online safety issues
- Fake news and misinformation advice hub
- What is misinformation and fake news?
What is misinformation?
Learn about fake news its impact on children.
With so many sources of information online, some children might struggle to make sense of what is true.
In this guide, learn about misinformation, what it looks like and how it impacts children’s wellbeing and safety online.
Fake news can be found embedded in traditional news social media or fake news sites and has no basis in fact but is presented as being factually accurate. This has allowed hackers controls over even politicians to use the net to spread disinformation online.
Our children can struggle to separate fact from fiction thanks to the spread of fake news. Here are some basic strategies to help them develop critical digital literacy:
- talk to them: children rely more on their family than social media for their news so talk to them about what is going on; - read: many people share stories who don't actually read. Encourage your kids to read beyond the headline; - check: teach children quick and easy ways to check the reliability of information like considering the source, doing a search to double-check the author's credibility, seeing if the information is available on reputable sites and using credible fact-checking websites to get more information; - get involved: digital literacy is about participation. Teach your kids to be honest, vigilant and creative digital citizens.
4 quick things to know about misinformation
Fake news is not the preferred term.
‘Fake news’ refers to false information and news online. However, it’s more appropriate to use ‘misinformation’ and ‘disinformation’.
Misinformation is false information spread by people who think it’s true.
Disinformation is false information spread by people who know it’s false .
Mis/disinformation is an online harm
Misinformation can impact children’s:
- mental health
- physical wellbeing
- future finances
- views towards other people.
It can also lead to mistrust and confusion related to the information they come across online.
Misinformation comes in different forms
Mis/disinformation and fake news might look like:
- social media hoaxes
- phishing emails
- popular videos
- sponsored posts
Misinformation is hard to spot for children who might not yet have the skills to fact-check. It can spread on social media, through satire news websites, via parody videos and other spaces.
Learn more about the forms it can take.
Insights from Ofcom
- 32% of 8-17-year-olds believe that all or most of what they see on social media is true.
- 70% of 12-17s said they were confident they could judge whether something was real or fake.
- Nearly a quarter of those children were unable to do so in practise.
This mismatch between confidence and ability could leave these children exposed to harm.
On a more positive point, of those who said they were confident, 48% were also able.
See Ofcom’s 2023 research .
Quick guide to tackling misinformation
Help children develop their digital literacy and critical thinking online.
Misinformation is false information that is spread by people who think it's true. This is different from 'fake news' and disinformation.
Fake news refers to websites that share mis or disinformation. This might be via satire sites like The Onion, but it also refers to those pretending to be trustworthy news sources.
Sometimes, people use the term ‘fake news’ to discredit true information. As such, it’s better to use more general terms such as ‘misinformation’ and ‘disinformation’.
Disinformation is false information that someone or a group spreads online while knowing it’s false. Generally, they do this for a specific intention, usually for the purpose of influencing others to believe their point of view.
7 types of mis and disinformation
UNICEF identifies 7 main types of mis and disinformation, all of which can impact children.

Types of misinformation and fake news
Satirical content and parodies can spread misinformation.
This is misleading information that is not intended to harm. Creators of the content know the information is false, but share it for humour. However, if people misunderstand the intent, they might spread it as true.
Clickbait for views can mislead users
This is content where the headline, visuals or captions don’t match the actual content. This is often clickbait to get more views on a video, visits to a page or engagement on social media.
Intentionally misleading content can create anger
People might share information in misleading way to frame an event, issue or person in a particular way. An example is when an old photo is used on a recent social media post. It might spread outrage or fear until the photo receives the right context.
Giving fake context can cause unnecessary outrage
Fake context is when information is shared with incorrect background information.
A lighthearted example is a popular photo of young director Steven Spielberg posing and smiling with a large dead animal. Many people felt outrage for his hunting of an endangered animal. However, the correct context was that he was on set of Jurassic Park and posing with a prop triceratops.
Usually, someone spreading disinformation will ‘alter’ the context of information. The intention is to convince people of their belief or viewpoint.
Impersonation can cause harm in many ways
This is when a person, group or organisation pretends they are another person or source. Imposter content can trick people into:
- sending money
- sharing personal information
- further spreading misinformation.
Manipulated content
True information that’s altered is hard to notice
Manipulated content is real information, images or videos that are altered or changed in some way to deceive others. Some deepfakes are an example of such content.
Completely false information can lead to harm
Fabricated content is disinformation created without any connection to truth. Its overall intention is to deceive and harm. Fabricated content can quickly become misinformation.
How does misinformation spread online?
From social media to news, misinformation can spread all over the world in an instant.
For children, misinformation and disinformation often looks very convincing. This is especially true with the popularity of generative AI and the ability to create deepfakes.
Learn more about using artificial intelligence tools safely.
Artificial intelligence can help scammers create convincing ads and content that tricks people. Unfortunately, unless reported (and sometimes even when reported), these ads can reach millions of people quickly.
While misinformation is nothing new, the internet means it can spread a lot quicker and reach many more people.
How social media spreads false information
From sock puppet accounts to scam ads, social media can help spread misinformation to thousands if not millions of people at once. Unfortunately, social media algorithms make it so any interaction helps the content reach more people.
Angry reactions on Facebook or comments calling a post out as false only helps the poster reach more people. This is because the algorithm only understands whether something is popular or not. It can’t tell if information is false; that’s why users must report false information rather than engage with it.
How echo chambers spread misinformation
‘Echo chambers’ is a term used to describe the experience of only seeing one type of content. Essentially, the more someone engages with the content, the more likely they are to see similar content.
So, if a child interacts with an influencer spreading misogyny, they will see more similar content. If they interact with that content, then they see more, and so on. This continues until all they see is content around misogyny.
When an algorithm creates an echo chamber, it means the user will only see content that supports the user’s view. As such, it’s really difficult to hear others’ perspectives and widen their worldview. This means, when challenged, they become more defensive and are likely to spread hate.
Learn more about algorithms and echo chambers.
How design impacts the way misinformation spreads
In a Risky-by-Design case study from the 5Rights Foundation , the following design features also contributed to misinformation spreading online.
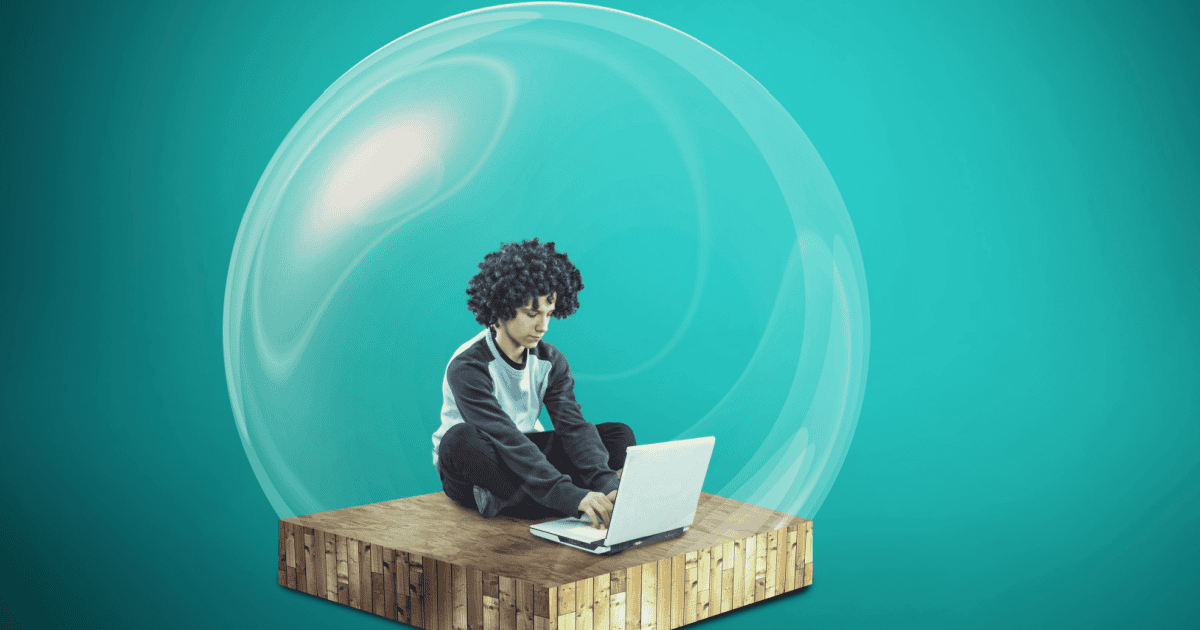
Manage algorithms and echo chambers
Recommendations favour popular creators.
Content creators who have a large following and spread misinformation have a wider reach. This is largely due to algorithms designed for the platform.
Many platforms are overrun with bots
Bots and fake profiles (or sock puppet accounts) may spread misinformation as their sole purpose. These can also manipulate information or make the source of disinformation harder to trace. It’s also often quite difficult as a user to successfully report fake or hacked accounts.
Recommendations can create echo chambers
Algorithms can create echo chambers or “a narrowing cycle of similar posts to read, videos to watch or groups to join.” Additionally, some content creators that spread misinformation also have interests in less harmful content. So, the algorithm might recommend this harmless content to users like children. Children then watch these new content creators, eventually seeing the misinformation.
For example, self-described misogynist Andrew Tate also shared content relating to finance and flashy cars. This content might appeal to a group of people who don’t agree with misogyny. For instance, our research shows that boys are more likely than girls to see content from Andrew Tate on social media. However, both girls and boys are similarly likely to see content about Andrew Tate on social media.
Not all content labels are clear
Subtle content label design — such as for identifying something as an ad or joke — are often easy to miss. More obvious labels could help children accurately navigate potential misinformation online.
Autoplay makes accidental viewing easy
When videos or audio that a child chooses finishes, many apps automatically start playing a new one by design. As such they might accidentally engage with misinformation that then feeds into the algorithm.
Most platforms allow you to turn off this feature.
Apps that hide content can support misinformation
Content that gets shared and then quickly removed is harder to fact-check. It spreads misinformation because it doesn’t give viewers the chance to check if it’s true. Children might engage with this type of content on apps like Snapchat where disappearing messages are the norm.
Algorithms cannot assess trending content
Algorithms can identify which hashtags or topics are most popular, sharing them with more users. However, these algorithms can’t tell if it relates to misinformation. So, it’s up to the user to make this decision, which many children might struggle with.
Misinformation can easily reach many
When sharing content directly, many apps and platforms suggest a ready-made list of people. This makes it easy to share misinformation with a large group of people at once.
What impact can fake news have on young people?
Nearly all children are now online, but many of them do not yet have the skills to assess information online.
Half of the children surveyed by the National Literacy Trust admitted to worrying about fake news. Additionally, teachers in the same survey noted an increase in issues of anxiety, self-esteem and a general skewing of world views.
Misinformation can impact children in a number of ways. These could include:
- Scams : falling for scams could lead to data breaches, financial loss, impacts on credit score and more.
- Harmful belief systems : if children watch content that spreads hate, this can become a part of their worldview. This could lead to mistreatment of people different from them or even lead to radicalisation and extremism.
- Dangerous challenges or hacks : some videos online might promote dangerous challenges or ‘life hacks’ that can cause serious harm. These hacks are common in videos from content farms .
- Confusion and distrust : If a child becomes a victim of dis or misinformation, they might struggle with new information. This can lead to distrust, confusion and maybe anxiety, depending on the extent of the misinformation.
Research into misinformation and fake news
Below are some figures into how misinformation can affect children and young people.
According to Ofcom, 79% of 12-15-year-olds feel that news they hear from family is ‘always’ or ‘mostly’ true.
28% of children aged 12-15 use TikTok as a news source (Ofcom).
6 in 10 parents worry about their child ‘being scammed/defrauded/lied to/impersonated’ by someone they didn’t know.
Around 4 in 10 children aged 9-16 said they experienced the feeling of ‘being unsure about whether what I see is true’. This was the second most common experience after ‘spending too much time online’.
NewsWise from The National Literacy Trust helped children develop their media literacy skills. Over that time, the children able to accurately assess news as false or true increased from 49.2% to 68%. This demonstrates the importance of teaching media literacy.
Resources to tackle misinformation
Help children become critical thinkers and avoid harm from misinformation with these resources.
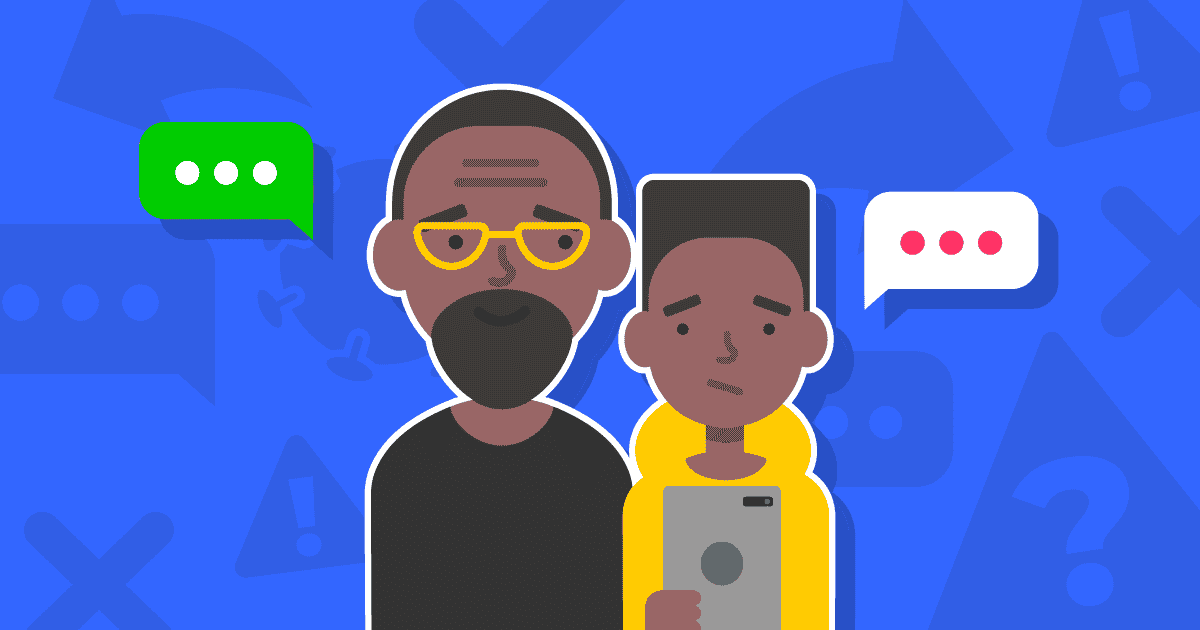
How to prevent misinformation

Dealing with misinformation online
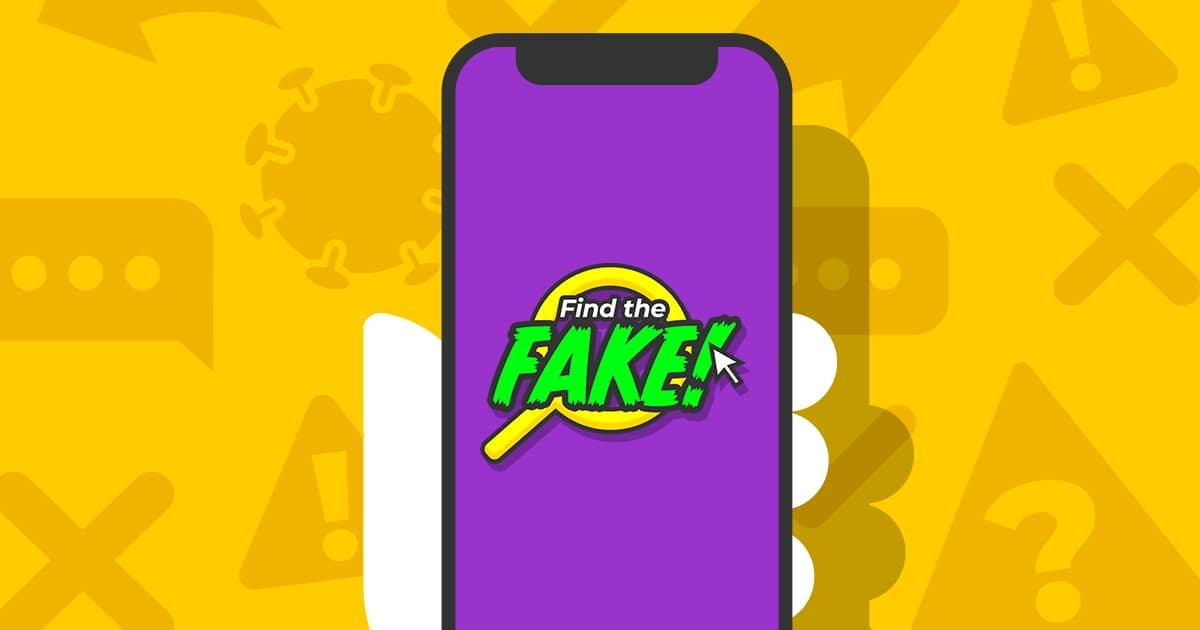
Help children identify 'fake news' online
Download workbook.
- To receive personalised online safety guidance in the future, we’d like to ask for your name and email. Simply fill your details below. You can choose to skip, if you prefer.
- First name *
- Last name *
- Email Address *
- I am a * Parent/Carer Teacher Professional
- Organisation name
- Skip and download
- Comments This field is for validation purposes and should be left unchanged.

An official website of the United States government
The .gov means it’s official. Federal government websites often end in .gov or .mil. Before sharing sensitive information, make sure you’re on a federal government site.
The site is secure. The https:// ensures that you are connecting to the official website and that any information you provide is encrypted and transmitted securely.
- Publications
- Account settings
Preview improvements coming to the PMC website in October 2024. Learn More or Try it out now .
- Advanced Search
- Journal List
- J Med Internet Res
- v.23(1); 2021 Jan

Prevalence of Health Misinformation on Social Media: Systematic Review
Victor suarez-lledo.
1 Department of Biomedicine, Biotechnology and Public Health, University of Cadiz, Cadiz, Spain
2 Computational Social Science DataLab, University Research Institute on Social Sciences, University of Cadiz, Jerez de la Frontera, Cadiz, Spain
Javier Alvarez-Galvez
Associated data.
Search terms and results from the search query.
Data extraction sheet.
Summary of quality scores.
Summary table with objectives and conclusions about misinformation prevalence in social media.
Although at present there is broad agreement among researchers, health professionals, and policy makers on the need to control and combat health misinformation, the magnitude of this problem is still unknown. Consequently, it is fundamental to discover both the most prevalent health topics and the social media platforms from which these topics are initially framed and subsequently disseminated.
This systematic review aimed to identify the main health misinformation topics and their prevalence on different social media platforms, focusing on methodological quality and the diverse solutions that are being implemented to address this public health concern.
We searched PubMed, MEDLINE, Scopus, and Web of Science for articles published in English before March 2019, with a focus on the study of health misinformation in social media. We defined health misinformation as a health-related claim that is based on anecdotal evidence, false, or misleading owing to the lack of existing scientific knowledge. We included (1) articles that focused on health misinformation in social media, including those in which the authors discussed the consequences or purposes of health misinformation and (2) studies that described empirical findings regarding the measurement of health misinformation on these platforms.
A total of 69 studies were identified as eligible, and they covered a wide range of health topics and social media platforms. The topics were articulated around the following six principal categories: vaccines (32%), drugs or smoking (22%), noncommunicable diseases (19%), pandemics (10%), eating disorders (9%), and medical treatments (7%). Studies were mainly based on the following five methodological approaches: social network analysis (28%), evaluating content (26%), evaluating quality (24%), content/text analysis (16%), and sentiment analysis (6%). Health misinformation was most prevalent in studies related to smoking products and drugs such as opioids and marijuana. Posts with misinformation reached 87% in some studies. Health misinformation about vaccines was also very common (43%), with the human papilloma virus vaccine being the most affected. Health misinformation related to diets or pro–eating disorder arguments were moderate in comparison to the aforementioned topics (36%). Studies focused on diseases (ie, noncommunicable diseases and pandemics) also reported moderate misinformation rates (40%), especially in the case of cancer. Finally, the lowest levels of health misinformation were related to medical treatments (30%).
Conclusions
The prevalence of health misinformation was the highest on Twitter and on issues related to smoking products and drugs. However, misinformation on major public health issues, such as vaccines and diseases, was also high. Our study offers a comprehensive characterization of the dominant health misinformation topics and a comprehensive description of their prevalence on different social media platforms, which can guide future studies and help in the development of evidence-based digital policy action plans.
Introduction
Over the last two decades, internet users have been increasingly using social media to seek and share health information [ 1 ]. These social platforms have gained wider participation among health information consumers from all social groups regardless of gender or age [ 2 ]. Health professionals and organizations are also using this medium to disseminate health-related knowledge on healthy habits and medical information for disease prevention, as it represents an unprecedented opportunity to increase health literacy, self-efficacy, and treatment adherence among populations [ 3 - 9 ]. However, these public tools have also opened the door to unprecedented social and health risks [ 10 , 11 ]. Although these platforms have demonstrated usefulness for health promotion [ 7 , 12 ], recent studies have suggested that false or misleading health information may spread more easily than scientific knowledge through social media [ 13 , 14 ]. Therefore, it is necessary to understand how health misinformation spreads and how it can affect decision-making and health behaviors [ 15 ].
Although the term “health misinformation” is increasingly present in our societies, its definition is becoming increasingly elusive owing to the inherent dynamism of the social media ecosystem and the broad range of health topics [ 16 ]. Using a broad term that can include the wide variety of definitions in scientific literature, we here define health misinformation as a health-related claim that is based on anecdotal evidence, false, or misleading owing to the lack of existing scientific knowledge [ 1 ]. This general definition would consider, on the one hand, information that is false but not created with the intention of causing harm (ie, misinformation) and, on the other, information that is false or based on reality but deliberately created to harm a particular person, social group, institution, or country (ie, disinformation and malinformation).
The fundamental role of health misinformation on social media has been recently highlighted by the COVID-19 pandemic, as well as the need for quality and veracity of health messages in order to manage the present public health crisis and the subsequent infodemic. In fact, at present, the propagation of health misinformation through social media has become a major public health concern [ 17 ]. The lack of control over health information on social media is used as evidence for the current demand to regulate the quality and public availability of online information [ 18 ]. In fact, although today there is broad agreement among health professionals and policy makers on the need to control health misinformation, there is still little evidence about the effects that the dissemination of false or misleading health messages through social media could have on public health in the near future. Although recent studies are exploring innovative ways to effectively combat health misinformation online [ 19 - 22 ], additional research is needed to characterize and capture this complex social phenomenon [ 23 ].
More specifically, four knowledge gaps have been detected from the field of public health [ 1 ]. First, we have to identify the dominant health misinformation trends and specifically assess their prevalence on different social platforms. Second, we need to understand the interactive mechanisms and factors that make it possible to progressively spread health misinformation through social media (eg, vaccination myths, miracle diets, alternative treatments based on anecdotal evidence, and misleading advertisements on health products). Factors, such as the sources of misinformation, structure and dynamics of online communities, idiosyncrasies of social media channels, motivation and profile of people seeking health information, content and framing of health messages, and context in which misinformation is shared, are critical to understanding the dynamics of health misinformation through these platforms. For instance, although the role of social bots in spreading misinformation through social media platforms during political campaigns and election periods is widely recognized, health debates on social media are also affected by social bots [ 24 ]. At present, social bots are used to promote certain products in order to increase company profits, as well as to benefit certain ideological positions or contradict health evidence (eg, in the case of vaccines) [ 25 ]. Third, a key challenge in epidemiology and public health research is to determine not only the effective impact of these tools in the dissemination of health misinformation but also their impact on the development and reproduction of unhealthy or dangerous behaviors. Finally, regarding health interventions, we need to know which strategies are the best in fighting and reducing the negative impact of health misinformation without reducing the inherent communicative potential to propagate health information with these same tools.
In line with the abovementioned gaps, a recent report represents one of the first steps forward in the comparative study of health misinformation on social media [ 16 ]. Through a systematic review of the literature, this study offers a general characterization of the main topics, areas of research, methods, and techniques used for the study of health misinformation. However, despite the commendable effort made to compose a comprehensible image of this highly complex phenomenon, the lack of objective indicators that make it possible to measure the problem of health misinformation is still evident today.
Taking into account this wide set of considerations, this systematic review aimed to specifically address the knowledge gap. In order to guide future studies in this field of knowledge, our objective was to identify and compare the prevalence of health misinformation topics on social media platforms, with specific attention paid to the methodological quality of the studies and the diverse analytical techniques that are being implemented to address this public health concern.
This systematic review was conducted according to the Preferred Reporting Items for Systematic Reviews and Meta-Analyses (PRISMA) guidelines [ 26 ].
Inclusion Criteria
Studies were included if (1) the objectives were to address the study of health misinformation on social media, search systematically for health misinformation, and explicitly discuss the impact, consequences, or purposes of misinformation; (2) the results were based on empirical results and the study used quantitative, qualitative, and computational methods; and (3) the research was specifically focused on social media platforms (eg, Twitter, Facebook, Instagram, Flickr, Sina Weibo, VK, YouTube, Reddit, Myspace, Pinterest, and WhatsApp). For comparability, we included studies written in English that were published after 2000 until March 2019.
Exclusion Criteria
Articles were excluded if they addressed health information quality in general or if they partially mentioned the existence of health misinformation without providing empirical findings. We did not include studies that dealt with content posted on other social media platforms. During the screening process, papers with a lack of methodological quality were also excluded.
Search Strategy
We searched MEDLINE and PREMEDLINE in March 2019 using the PubMed search engine. Based on previous findings [ 16 ], the query searched for MeSH terms and keywords (in the entire body of the manuscript) related to the following three basic analytical dimensions that articulated our research objective: (1) social media, (2) health, and (3) misinformation. The MeSH terms were social media AND health (ie, this term included health behaviors) AND (misinformation OR information seeking behavior OR communication OR health knowledge, attitudes, practice). Based on the results obtained through this initial search, we added some keywords that (having been extracted from the articles that met the inclusion criteria) were specifically focused on the issue of health misinformation on social media. The search using MeSH terms was supplemented with the following keywords: social media (eg, “Twitter” OR “Facebook” OR “Instagram” OR “Flickr” OR “Sina Weibo” OR “YouTube” OR “Pinterest”) AND health AND misinformation (eg, “inaccurate information” OR “poor quality information” OR “misleading information” OR “seeking information” OR “rumor” OR “gossip” OR “hoax” OR “urban legend” OR “myth” OR “fallacy” OR “conspiracy theory”). This initial search retrieved 1693 records. Additionally, this search strategy was adapted for its use in Scopus (3969 records) and Web of Science (1541 records). A full description of the search terms can be found in Multimedia Appendix 1 .
Study Selection
In total, we collected 5018 research articles. After removing duplicates, we screened 3563 articles and retrieved 226 potentially eligible articles. In the next stage, we independently carried out a full-text selection process for inclusion (k=0.89). Discrepancies were shared and resolved by mutual agreement. Finally, a total of 69 articles were included in this systematic review ( Figure 1 ).

Preferred Reporting Items for Systematic Reviews and Meta-Analyses flow chart.
Data Extraction
In the first phase, the data were extracted by VSL and then checked by VSL and JAG. In order to evaluate the quality of the selected studies and given the wide variety of methodologies and approaches found in the articles, we composed an extraction form based on previous work [ 27 - 29 ]. Each extraction form contained 62 items, most of which were closed questions that could be answered using predefined forms (yes/good, no/poor, partially/fair, etc). Following this coding scheme, we extracted the following four different fields of information: (1) descriptive information (27 items), (2) search strategy evaluation (eight items), (3) information evaluation (six items), and (4) the quality and rigor of methodology and reporting (15 items) for either quantitative or qualitative studies ( Multimedia Appendix 1 ). Questions in field 2, which have been used in previous studies [ 27 ], assessed the quality of information provided to demonstrate how well reported, systematic, and comprehensive the search strategy was (S score). The items in field 3 measured how rigorous the evaluation was (E score) for health-related misinformation [ 27 ]. Field 4 contained items designed for the general evaluation of quality in the research process, whether quantitative [ 28 ] or qualitative [ 29 ]. This Q-score approach takes into account general aspects of the research and reporting, such as the study, methodology, and quality of the discussion. For each of the information fields, we calculated the raw score as the sum of each of the items by equating “yes” or “good” as 1 point, “fair” as 0.5 points, and “no” or “poor” as 0 points ( Multimedia Appendix 2 ). The purpose of these questions is to guarantee the quality of the selected studies.
Furthermore, in order to be able to compare the methods used in the selected studies, the studies were classified into several categories. The studies classified as “content/text analysis” used methods related to textual and content analysis, emphasizing the word/topic frequency, linguistic inquiry word count, n-grams, etc. The second category “evaluating content” grouped together studies whose methods were focused on the evaluation of content and information. In general, these studies analyzed different dimensions of the information published on social media. The third category “evaluating quality” included studies that analyzed the quality of the information offered in a global way. This category considered other dimensions in addition to content, such as readability, accuracy, usefulness, and sources of information. The fourth category “sentiment analysis” included studies whose methods were focused on sentiment analysis techniques (ie, methods measuring the reactions and the general tone of the conversation on social media). Finally, the “social network analysis” category included those studies whose methods were based on social network analysis techniques. These studies focused on measuring how misinformation spreads on social media, the relationship between the quality of information and its popularity on these social platforms, the relationship between users and opinions, echochambers effects, and opinion formation.
Of the 226 studies available for full-text review, 157 were excluded for various reasons, including research topics that were not focused on health misinformation (n=133). We also excluded articles whose research was based on websites rather than social media platforms (n=16), studies that did not assess the quality of health information (n=6) or evaluated institutional communication (n=5), nonempirical studies (n=2), and research protocols (n=1). In addition, two papers were excluded because of a lack of quality requirements (Q score <50%). Finally, the protocol of this review was registered at the International Prospective Register of Systematic Reviews (PROSPERO CRD42019136694).
Prevalence of Health Misinformation
Ultimately, 69 studies were identified as eligible, and they covered a wide range of health topics and social media platforms, with the most common data source being Twitter (29/69, 43%), followed by YouTube (25/69, 37%) and Facebook (6/69, 9%). The less common sources were Instagram, MySpace, Pinterest, Tumblr, WhatsApp, and VK or a combination of these. Overall, 90% (61/69) of the studies were published in health science journals, and only 7% (5/69) of the studies were published in communication journals. The vast majority of articles analyzed posts written exclusively in one language (63/69, 91%). Only a small percentage assessed posts in more than one language (6/69, 10%).
Table 1 classifies the studies by topic and social media platform [ 30 - 97 ]. It also includes the prevalence of health misinformation posts. The topics were articulated around the following six principal categories: vaccines (22/69, 32%), drugs or smoking issues (16/69, 22%), noncommunicable diseases (13/69, 19%), pandemics (7/69, 10%), eating disorders (6/69, 9%), and medical treatments (5/69, 7%). The quality assessment results for the S score, E score, and Q score are reported in Multimedia Appendix 3 .
Summary of the prevalence of misinformation by topic and social media platform.
Authors | Year | Topic | Social media platform | Prevalence of health misinformation posts |
Abukaraky et al [ ] | 2018 | Treatments | YouTube | 30% |
Ahmed et al [ ] | 2019 | Pandemics | N/A | |
Al Khaja et al [ ] | 2018 | Drugs | 27% | |
Allem et al [ ] | 2017 | Drugs | 59% | |
Allem et al [ ] | 2017 | Drugs | N/A | |
Arseniev-Koehler et al [ ] | 2016 | EDs | 36% | |
Basch et al [ ] | 2017 | Vaccines | YouTube | 65% |
Becker et al [ ] | 2016 | Vaccines | 1% | |
Biggs et al [ ] | 2013 | NCDs | YouTube | 39% |
Blankenship et al [ ] | 2018 | Vaccines | 24% | |
Bora et al [ ] | 2018 | Pandemics | YouTube | 23% |
Branley et al [ ] | 2017 | EDs | Twitter and Tumblr | 25% |
Briones et al [ ] | 2012 | Vaccines | YouTube | 51% |
Broniatowski et al [ ] | 2018 | Vaccines | 35% | |
Buchanan et al [ ] | 2014 | Vaccines | 43% | |
Butler et al [ ] | 2013 | Treatments | YouTube | N/A |
Cavazos-Rehg et al [ ] | 2018 | Drugs | 75% | |
Chary et al [ ] | 2017 | Drugs | 0% | |
Chew et al [ ] | 2010 | Pandemics | 4% | |
Covolo et al [ ] | 2017 | Vaccines | YouTube | 23% |
Dunn et al [ ] | 2015 | Vaccines | 25% | |
Dunn et al [ ] | 2017 | Vaccines | N/A | |
Ekram et al [ ] | 2018 | Vaccines | YouTube | 57% |
Erdem et al [ ] | 2018 | Treatments | YouTube | 0% |
Faasse et al [ ] | 2016 | Vaccines | N/A | |
Fullwood et al [ ] | 2016 | Drugs | YouTube | 34% |
Garg et al [ ] | 2015 | Vaccines | YouTube | 11% |
Gimenez-Perez et al [ ] | 2018 | NCDs | YouTube | 50% |
Goobie et al [ ] | 2019 | NCDs | YouTube | N/A |
Guidry et al [ ] | 2017 | Pandemics | Twitter and Instagram | N/A |
Guidry et al [ ] | 2016 | Drugs | 97% | |
Guidry et al [ ] | 2015 | Vaccines | 74% | |
Hanson et al [ ] | 2013 | Drugs | 0% | |
Harris et al [ ] | 2018 | EDs | N/A | |
Haymes et al [ ] | 2016 | NCDs | YouTube | 47% |
Helmi et al [ ] | 2018 | NCDs | Different sources | N/A |
Kang et al [ ] | 2017 | Vaccines | 42% | |
Katsuki et al [ ] | 2015 | Drugs | 6% | |
Keelan et al [ ] | 2010 | Vaccines | MySpace | 43% |
Keim-Malpass et al [ ] | 2017 | Vaccines | 43% | |
Kim et al [ ] | 2017 | NCDs | YouTube | 22% |
Krauss et al [ ] | 2017 | Drugs | 50% | |
Krauss et al [ ] | 2015 | Drugs | 87% | |
Kumar et al [ ] | 2014 | NCDs | YouTube | 33% |
Laestadius et al [ ] | 2016 | Drugs | N/A | |
Leong et al [ ] | 2018 | NCDs | YouTube | 33% |
Lewis et al [ ] | 2015 | Treatments | YouTube | N/A |
Loeb et al [ ] | 2018 | NCDs | YouTube | 77% |
Love et al [ ] | 2013 | Vaccines | 13% | |
Martinez et al [ ] | 2018 | Drugs | 67% | |
Massey et al [ ] | 2016 | Vaccines | 25% | |
McNeil et al [ ] | 2012 | NCDs | 41% | |
Menon et al [ ] | 2017 | Treatments | YouTube | 2% |
Merianos et al [ ] | 2016 | Drugs | YouTube | 65% |
Meylakhs et al [ ] | 2014 | NCDs | VK | N/A |
Morin et al [ ] | 2018 | Pandemics | N/A | |
Mueller et al [ ] | 2019 | NCDs | YouTube | 66% |
Porat et al [ ] | 2019 | Pandemics | 0% | |
Radzikowski et al [ ] | 2016 | Vaccines | N/A | |
Schmidt et al [ ] | 2018 | Vaccines | 4% | |
Seltzer et al [ ] | 2017 | Pandemics | 60% | |
Seymour et al [ ] | 2015 | NCDs | N/A | |
Syed-Abdul et al [ ] | 2013 | EDs | YouTube | 29% |
Teufel et al [ ] | 2013 | EDs | 22% | |
Tiggermann et al [ ] | 2018 | EDs | 29% | |
Tuells et al [ ] | 2015 | Vaccines | YouTube | 12% |
van der Tempel et al [ ] | 2016 | Drugs | N/A | |
Waszak et al [ ] | 2018 | NCDs | 40% | |
Yang et al [ ] | 2018 | Drugs | YouTube | 98% |
a N/A: not applicable.
b EDs: eating disorders.
c NCDs: noncommunicable diseases.
Figure 2 shows the prevalence of health misinformation grouped by different topics and social media typology. Studies are ordered according to the percentage of health misinformation posts found in the studies selected. These works were also classified according to the type of social media under study. In this way, papers focused on Twitter, Tumblr, or Myspace were categorized as “microblogging.” Additionally, papers focused on YouTube, Pinterest, or Instagram were classified within “media sharing” platforms. Moreover, papers focused on Facebook, VK, or WhatsApp were included within the group of “social network” platforms. While all topics were present on all the different social media platforms, we found some differences in their prevalence. On one hand, vaccines, drugs, and pandemics were more prevalent topics on microblogging platforms (ie, Twitter or MySpace). On the other hand, on media sharing platforms (ie, YouTube, Instagram, or Pinterest) and social network platforms (ie, Facebook, VK, or WhatsApp), noncommunicable diseases and treatments were the most prevalent topics. More specifically, Twitter was the most used source for work on vaccines (10/69), drugs or smoking products (10/69), pandemics (4/69), and eating disorders (3/69). For studies on noncommunicable diseases (9/69) or treatments (5/69), YouTube was the most used social media platform.

Prevalence of health misinformation grouped by different topics and social media type.
Overall, health misinformation was most prevalent in studies related to smoking products, such as hookah and water pipes [ 33 , 59 , 71 ], e-cigarettes, and drugs, such as opioids and marijuana [ 45 , 70 , 97 ]. Health misinformation about vaccines was also very common. However, studies reported different levels of health misinformation depending on the type of vaccine studied, with the human papilloma virus (HPV) vaccine being the most affected [ 67 , 68 ]. Health misinformation related to diets or pro–eating disorder arguments were moderate in comparison to the aforementioned topics [ 35 , 93 ]. Studies focused on diseases (ie, noncommunicable diseases and pandemics) also reported moderate misinformation rates [ 56 , 85 ], especially in the case of cancer [ 76 , 96 ]. Finally, the lowest levels of health misinformation were observed in studies evaluating the presence of health misinformation regarding medical treatments. Although first-aid information on burns or information on dental implants was limited in quantity and quality, the prevalence of misinformation for these topics was low. Surgical treatment misinformation was the least prevalent. This was due to the fact that the content related to surgical treatments mainly came from official accounts, which made the online information complete and reliable.
Regarding the methods used in the different studies, there were some differences between the diverse social media platforms. We classified the studies based on the methods applied into the following five categories: social network analysis (19/69), evaluating content (18/69), evaluating quality (16/69), content/text analysis (12/69), and sentiment analysis (4/69). Figure 3 shows the different methods applied in the studies classified by the type of social media platform and ordered by the percentage of misinformation posts. Among platforms, such as YouTube and Instagram, methods focused on the evaluation of health information quality and content were common, representing 22% (15/69) and 12% (8/69), respectively. On microblogging platforms, such as Twitter and Tumblr, social network analysis was the method most used by 19% (13/69) of the studies. Finally, on social media platforms, such as Facebook, VK, and WhatsApp, studies whose methods were related to social network analysis represented 3% (2/69) of the included studies and those focused on the evaluation of content represented 4% (3/69) of the included studies.

Prevalence of health misinformation grouped by methods and social media type.
Misinformation Topics and Methods
Overall, 32% (22/69) of the studies focused on vaccines or vaccination decision-making–related topics. Additionally, 14% (10/69) of the selected articles focused on social media discussion regarding the potential side effects of vaccination [ 23 , 36 , 48 , 53 , 55 , 60 , 65 , 77 , 87 , 88 ], 12% (8/69) were centered on the debate around the HPV vaccine [ 42 , 49 - 51 , 67 , 68 , 79 , 94 ], and 3% (2/69) were centered on the antivaccine movement [ 39 , 43 ]. According to social media platforms, 9% (6/69) of the studies were focused on the debate and narratives about vaccines in general on Twitter, and 6% (4/69) specifically analyzed the HPV debate on this platform. Papers focused on YouTube also followed a similar trend, and they were centered on the HPV debate and on the public discussion on vaccine side effects and risks for specific population groups (eg, autism in children). Regarding Facebook, all studies were particularly focused on vaccination decision-making.
Most authors studied differences in language use, the effect of a heterogeneous community structure in the propagation of health misinformation, and the role played by fake profiles or bots in the spread of poor quality, doubtful, or ambiguous health content. In line with these concerns, authors pointed out the need to further study the circumstances surrounding those who adopt these arguments [ 49 ], and whether alternative strategies to education could improve the fight against antivaccine content [ 51 ]. Authors also recommended paying close attention to social media as these tools are assumed to play a fundamental role in the propagation of misinformation. For instance, the role played by the echochamber or the heterogeneous community structure on Twitter has been shown to skew the information to which users are exposed in relation to HPV vaccines [ 49 ]. In this sense, it is widely acknowledged that health professionals should pay more attention to antivaccine arguments on social media, so that they can better respond to patients’ concerns [ 36 , 43 , 65 , 77 ]. Furthermore, governmental organizations could also use social media platforms to reach a greater number of people [ 39 , 55 ].
Drugs and Smoking
Several studies (16/69, 22%) covered misuse and misinformation about e-cigarettes, marijuana, opioid consumption, and prescription drug abuse. Studies covering the promotion of e-cigarette use and other forms of smoking, such as hookah (ie, water pipes or narghiles) represented 7% (5/69) of the articles analyzed. The rest (16%, 11/69) were focused on the analysis of drug misinformation.
According to topic, regarding drug and opioid use, studies investigated the dissemination of misinformation through social media platforms [ 32 , 45 , 46 , 70 , 97 ], the consumption of misinformation related to these products, drug abuse, and the sale of online medical products [ 61 , 66 ]. These studies highlighted the risk, especially for young people, caused by the high rate of misinformation related to the dissemination of drug practice and misuse (predominantly marijuana and opioids) [ 45 ]. In addition, social media platforms were identified as a potential source of illegal promotion of the sale of controlled substances directly to consumers [ 66 ]. Most drug-related messages on social media were potentially misleading or false claims that lacked credible evidence to support them [ 32 ]. Other studies pointed to social media as a potential source of information that illegally promotes the sale of controlled prescription drugs directly to consumers [ 66 ]. In the case of cannabinoids, there was often content that described, encouraged, promoted [ 54 ], or even normalized the consumption of illicit substances [ 70 ].
Unlike drug studies, most of the papers analyzed how e-cigarettes and hookah [ 33 , 34 , 59 , 71 , 73 , 78 , 82 , 95 ] are portrayed on social media and/or the role of bots in promoting e-cigarettes. Regarding e-cigarettes, studies pointed out the high prevalence of misinformation denying health damage [ 95 ]. In this sense, it is worth noting the importance of sources of misinformation. While in the case of vaccines, the source of health misinformation was mainly individuals or groups of people with a particular interest (eg, antivaccine movement), social media was found to be frequently contaminated by misinformation from bots (ie, software applications that autonomously run tasks such as spreading positive discourse about e-cigarettes and other tobacco products) [ 78 ]. In fact, these fake accounts may influence the online conversation in favor of e-cigarettes given the scientific appearance of profiles [ 78 ]. Some of the claims found in this study denied the harmfulness of e-cigarettes. In line with these findings, other studies pointed to the high percentage of messages favoring e-cigarettes as an aid to quitting smoking [ 95 ].
We found that 10% (7/69) of the studies used methods focused on evaluating the content of the posts. These studies aimed to explore the misperceptions of drug abuse or alternative forms of tobacco consumption. Along these lines, another study (1/69, 1%) focused on evaluating the quality of content. The authors evaluated the truthfulness of claims about drugs. In particular, we found that 7% (5/69) of the studies used social network analysis techniques. These studies analyzed the popularity of messages based on whether they promoted illegal access to drugs online and the interaction of users with this content. Other studies (3/69, 3%) used content analysis techniques. These studies evaluated the prevalence of misinformation on platforms and geographically, as a kind of “toxicosurveillance” system [ 34 , 46 ].
Noncommunicable Diseases
A relevant proportion (13/69, 19%) of studies assessed noncommunicable diseases, such as cancer, diabetes, and epilepsy. Most of the studies focused on the objective evaluation of information quality on YouTube [ 38 , 56 , 57 , 69 , 72 , 74 , 76 , 80 , 85 ]. Overall, 13% (9/69) of these studies used methods to assess the quality of the information. The authors analyzed the usefulness and accuracy of the information. Moreover, 4% (3/69) of the studies used methods related to content assessment. The main objective of these studies was to analyze which are the most common misinformation topics. Furthermore, 3% (2/69) used social network analysis, and the main objective of the analysis was to study the information dissemination patterns or the social spread of scientifically inaccurate health information.
Some studies evaluated the potential of this platform as a source of information specially for health students or self-directed education among the general public. Unfortunately, the general tone of research findings was that YouTube is not an advisable source for health professionals or health information seekers. Regarding diabetes, the probability of finding misleading videos was high [ 56 ]. Misleading videos promoted cures for diabetes, negated scientific arguments, or provided treatments with no scientific basis. Furthermore, misleading videos related to diabetes were found to be more popular than those with evidence-based health information [ 74 ], which increased the probability of consuming low-quality health content. The same misinformation pattern was detected for other chronic diseases such as hypertension [ 72 ], prostate cancer [ 76 ], and epilepsy [ 80 ].
Pandemics and Communicable Diseases
Results indicated that 10% (7/69) of the studies covered misinformation related to pandemics and communicable diseases such as H1N1 [ 31 , 47 ], Zika [ 40 , 89 ], Ebola [ 58 , 84 ], and diphtheria [ 86 ]. All these studies analyzed how online platforms were used by both health information seekers and health and governmental authorities during the pandemic period.
We found that 14% (10/69) of the studies on this topic evaluated the quality of the information. To achieve this, most of the studies used external instruments such as DISCERN and AAD7 Self-Care Behaviors. Overall, 9% (6/69) of the papers evaluated the content of the information. These studies were focused on analysis of the issues of misinformation. Another 4% (3/69) used social media analysis to observe the propagation of misinformation. Finally, 3% (2/69) used textual analysis as the main method. These studies focused on the study of the prevalence of health misinformation.
These studies identified social media as a public forum for free discussion and indicated that this freedom might lead to rumors on anecdotal evidence and misunderstandings regarding pandemics. Consequently, although social media was described as a forum for sharing health-related knowledge, these tools are also recognized by researchers and health professionals as a source of misinformation that needs to be controlled by health experts [ 83 , 84 ]. Therefore, while social media serves as a place where people commonly share their experiences and concerns, these platforms can be potentially used by health professionals to fight against false beliefs on communicable diseases (eg, as it is happening today during the COVID-19 pandemic). Accordingly, social media platforms have been found to be powerful tools for health promotion among governmental institutions and health-related workers, and new instruments that, for instance, are being used to increase health surveillance and intervention against false beliefs and misinformation [ 31 , 89 ]. In fact, different authors agreed that governmental/health institutions should increase their presence on social media platforms during pandemic crises [ 47 , 58 , 84 , 86 ].
Diet/Eating Disorders
Studies focusing on diet and eating disorders represented 9% (6/69) of the included studies. This set of studies identified pro–eating disorder groups and discourses within social media [ 35 ], and how pro–eating disorder information was shared and spread on these platforms [ 91 ]. Anorexia was the most studied eating disorder along with bulimia. Furthermore, discourses promoting fitness or recovery after an eating disorder were often compared with those issued by pro–eating disorder groups [ 41 , 62 , 92 , 93 ]. In general, the authors agreed on the relevance of pro–eating disorder online groups, the mutual support among members, and the way they reinforce their opinions and health behaviors [ 35 ].
Overall, 4% (3/69) of the studies used social network analysis techniques. The authors focused on analyzing the existing connections between individuals in the pro–eating disorder community and their engagement, or comparing the cohesion of these communities with other communities, such as the fitness community, that promote healthier habits. Moreover, 3% (2/69) of the studies evaluated the quality of the content and particularly focused on informative analysis of the videos, that is, the content was classified as informative when it described the health consequences of anorexia or proana if, on the contrary, anorexia was presented as a fashion or a source of beauty. Furthermore, only one study used content analysis techniques. The authors classified the posts according to the following categories: proana, antiana, and prorecovery. Pro–eating disorder pages tended to identify themselves with body-associated pictures owing to the importance they attributed to motivational aspects of pro–eating disorder communities [ 92 ]. The pro–eating disorder claims contained practices about weight loss, wanting a certain body type or characteristic of a body part, eating disorders, binge eating, and purging [ 62 ]. Pro–eating disorder conversations also had a high content of social support in the form of tips and tricks (eg, “Crunch on some ice chips if you are feeling a hunger craving. This will help you feel as if you are eating something substantial” and “How do you all feel about laxatives?”) [ 92 ].
Regarding eating disorders on social media, paying attention to community structure is important according to authors. Although it is widely acknowledged that communities can be positive by providing social support, such as recovery and well-being, certain groups on social media may also reaffirm the pro–eating disorder identity [ 35 ]. In fact, polarized pro/anti–eating disorder communities can become closed echochambers where community members are selectively exposed to the content they are looking for and therefore only hear the arguments they want to hear. In this case, the echochamber effect might explain why information campaigns are limited in scope and often encourage polarization of opinion, and can even reinforce existing divides in pro–eating disorder opinions [ 88 ].
Treatments and Medical Interventions
Finally, we found that 7% (5/69) of the studies assessed the quality of health information regarding different medical treatments or therapies recommended through social media [ 63 , 81 ]. According to method, 6% (4/69) of the studies evaluated the quality of information related to the proposed treatments and therapies. In this sense, the fundamental goal of these studies was aimed at assessing the quality and accuracy of the information.
As in the case of noncommunicable diseases, professionals scanned social networks, especially YouTube, and evaluated the quality of online health content as an adequate instrument for self-care or for health student training. There were specific cases where information was particularly limited in quality and quantity, such as dental implants and first-aid information on burns [ 30 , 44 ]. However, most surgical treatments or tools were found to have a sufficient level of quality information on YouTube [ 52 , 81 ]. In relation to this topic, it is worth pointing out the source of the misinformation. In this particular case, most of the posts were published by private companies. They used the platforms to promote their medical products. Therefore, the amount of misinformation was considerably low compared with other topics, such as eating disorders and vaccines, that are closely linked to the general public. In general, the videos were accurate, were well presented, and framed treatments in a useful way for both health workers and health information seekers.
A full description of the objectives and main conclusions of the reviewed articles is presented in Multimedia Appendix 4 .
Main Findings
This work represents, to our knowledge, the first effort aimed at finding objective and comparable measures to quantify the extent of health misinformation in the social media ecosystem. Our study offers an initial characterization of dominant health misinformation topics and specifically assesses their prevalence on different social platforms. Therefore, our systematic review provides new insights on the following unanswered question that has been recurrently highlighted in studies of health misinformation on social media: How prevalent is health misinformation for different topics on different social platform types (ie, microblogging, media sharing, and social networks)?
We found that health misinformation on social media is generally linked to the following six topical domains: (1) vaccines, (2) diets and eating disorders, (3) drugs and new tobacco products, (4) pandemics and communicable diseases, (5) noncommunicable diseases, and (6) medical treatments and health interventions.
With regard to vaccines, we found some interesting results throughout the different studies. Although antivaccine ideas have been traditionally linked to emotional discourse against the rationality of the scientific and expert community, we curiously observed that in certain online discussions, antivaccine groups tend to incorporate scientific language in their own discourse with logically structured statements and/or with less usage of emotional expressions [ 53 ]. Thus, the assimilation of the scientific presentation and its combination with anecdotal evidence can rapidly spread along these platforms through a progressive increment of visits and “likes” that can make antivaccine arguments particularly convincing for health information seekers [ 53 , 55 ]. Furthermore, we found that the complex and heterogeneous community structure of these online groups must be taken into account. For instance, those more exposed to antivaccine information tend to spread more negative concerns about vaccines (ie, misinformation or opinions related to vaccine hesitancy) than users exposed to positive or neutral opinions [ 49 ]. Therefore, negative/positive opinions are reinforced through the network structure of particular social media platforms. Moreover, fake profiles tend to amplify the debate and discussion, thereby undermining the possible public consensus on the effectiveness and safety of vaccines, especially in the case of HPV; measles, mumps, and rubella (MMR); and influenza [ 23 ].
As observed in our review, health topics were omnipresent over all social media platforms included in our study; however, the health misinformation prevalence for each topic varied depending on platform characteristics. Therefore, the potential effect on population health was ambivalent, that is, we found both positive and negative effects depending on the topic and on the group of health information seekers. For instance, content related to eating disorders was frequently hidden or not so evident to the general public, since pro–eating disorder communities use their own codes to reach specific audiences (eg, younger groups) [ 98 ]. To provide a simple example, it is worth mentioning the usage of nicknames, such as proana for proanorexia and promia for probulimia, as a way to reach people with these health conditions and make it easier for people to talk openly about their eating disorders. More positively, these tools have been useful in prevention campaigns during health crises. For example, during the H1N1, Ebola, and Zika pandemics, and, even more recently, with the ongoing COVID-19 pandemic, platforms, such as Twitter, have been valuable instruments for spreading evidence-based health knowledge, expert recommendations, and educative content aimed at avoiding the propagation of rumors, risk behaviors, and diseases [ 31 , 89 ].
Throughout our review, we found different types of misinformation claims depending on the topic. Concerning vaccines, misinformation was often framed with a scientific appearance against scientific evidence [ 53 ]. Drug-related misinformation promoted the consumption and abuse of these substances [ 66 ]. However, these statements lacked scientific evidence to support them [ 32 ]. As with vaccines, false accounts that influenced the online conversation did so with a scientific appearance in favor of e-cigarettes [ 82 ]. In this sense, most accounts tended to promote the use and abuse of these items. With beauty as the final goal, misinformation about eating disorders promoted changes in the eating habits of social media users [ 91 ]. Furthermore, we found that social media facilitated the development of pro–eating disorder online communities [ 35 ]. In general, the results indicated that this type of content promoted unhealthy practices while normalizing eating disorders. In contrast, epidemic/pandemic-related misinformation was not directly malicious. Misinformation on this topic involved rumors, misunderstandings, and doubts arising from a lack of scientific knowledge [ 31 ]. The statements were within the framework of the health emergency arising from the pandemic. In line with these findings, we noted findings related to noncommunicable diseases. Messages that focused on this topic promoted cures for chronic diseases or for conditions with no cure through fallacies or urban legends [ 85 ].
In this study, we focused on analysis of the results obtained and the conclusions of the authors. Some of our findings are in line with those obtained in recent works [ 16 ]. The reviewed studies indicate, on one hand, the difficulty in characterizing and evaluating the quality of health information on social media [ 1 ] and, on the other, the conceptual fuzziness that can result from the convergence of multiple disciplines trying to apprehend the multidisciplinary and complex phenomenon of health misinformation on social media. This research field is being studied by health and social scientists [ 70 , 73 ], as well as by researchers from the fields of computer science, mathematics, sociophysics, etc [ 99 , 100 ]. Therefore, we must understand that the inherent multidisciplinary and methodological diversity of studies and the highly dynamic world of social media are a perfect match for making it more difficult to identify comprehensive and transversal solutions to the problem of health misinformation. In fact, as we have found, misinformation on vaccines, drugs, and new smoking products is more prevalent on media-sharing platforms (eg, YouTube) and microblogging applications (eg, Twitter), while misinformation on noncommunicable diseases is particularly prevalent on media sharing platforms where users can widely describe disease symptoms, medical treatments, and therapies [ 76 , 85 ]. Platforms, such as YouTube, owing to their characteristics, allow more space for users to share this type of information, while the natural dynamism of Twitter makes it an ideal medium for discussion among online communities with different political or ideological orientations (eg, pro/antivaccination communities).
Finally, we should mention that the current results are limited to the availability and quality of social media data. Although the digitalization of social life offers researchers an unprecedented amount of health and social information that can be used to understand human behaviors and health outcomes, accessing this online data is becoming increasingly difficult, and some measures have to be taken to mitigate bias [ 40 , 43 , 67 , 79 ]. Over the last few years, new concerns around privacy have emerged and led governments to tighten regulations around data access and storage [ 101 , 102 ]. Consequently, in response to these new directives, as well as scandals involving data sharing and data breaches such as the Cambridge Analytica case, social media companies are developing new controls and barriers to data in their platforms. This is why free access to application programming interfaces (APIs) is becoming increasingly difficult and the range of social data accessible via APIs is gradually decreasing. These difficulties in accessing data are also determining which platforms are most frequently used by researchers, which are not used, and which will be used in the near future.
Limitations and Strengths
The present study has some limitations. First, the conceptual definition of health misinformation is one limitation. In any case, taking into account that we were facing a new field of study, we considered a broad definition in order to be more inclusive and operative in the selection of studies. Therefore, we included as many papers as possible for the review in order to perform an analysis of the largest number of possible topics. Second, from a methodological perspective, our findings are limited to research published in English language journals and do not cover all the social media platforms that exist. Besides, we discovered some technical limitations when conducting this systematic review. Owing to the newness of this research topic, our study revealed difficulties in comparing different research studies characterized by specific theoretical approaches, working definitions, methodologies, data collection processes, and analytical techniques. Some studies selected involved observational designs (using survey methods and textual analysis), whereas others were based on the application of automatic or semiautomatic computational procedures with the aim of classifying and analyzing health misinformation on social media. Finally, taking into account the particular features of each type of social media (ie, microblogging service, video sharing service, or social network) and the progressive barriers in accessing social media data, we need to consider the information and selection bias when studying health misinformation on these platforms. According to these biases, we should ponder which users are behind these tools and how we can extrapolate specific findings (ie, applied to certain groups and social media platforms) to a broader social context.
Despite the limitations described above, it is necessary to mention the strengths of our work. First, we believe that this study represents one of the first steps in advancing research involving health misinformation on social media. Unlike previous work, we offer some measures that can serve as guidance and a comparative baseline for subsequent studies. In addition, our study highlights the need to redirect future research toward social media platforms, which, perhaps due to the difficulties of automatic data collection, are currently being neglected by researchers. Our study also highlights the need for both researchers and health professionals to explore the possibility of using these digital tools for health promotion and the need for them to progressively colonize the social media ecosystem with the ultimate goal of combating the waves of health misinformation that recurrently flood our societies.
Health misinformation was most common on Twitter and on issues related to smoking products and drugs. Although we should be aware of the difficulties inherent in the dynamic magnitude of online opinion flows, our systematic review offers a comprehensive comparative framework that identifies subsequent action areas in the study of health misinformation on social media. Despite the abovementioned limitations, our research presents some advances when compared with previous studies. Our study provides (1) an overview of the prevalence of health misinformation identified on different social media platforms; (2) a methodological characterization of studies focused on health misinformation; and (3) a comprehensive description of the current research lines and knowledge gaps in this research field.
According to the studies reviewed, the greatest challenge lies in the difficulty of characterizing and evaluating the quality of the information on social media. Knowing the prevalence of health misinformation and the methods used for its study, as well as the present knowledge gaps in this field will help us to guide future studies and, specifically, to develop evidence-based digital policy action plans aimed at combating this public health problem through different social media platforms.
Acknowledgments
We would like to acknowledge the support of the University Research Institute on Social Sciences (INDESS, University of Cadiz) and the Ramon & Cajal Program. JAG was subsidized by the Ramon & Cajal Program operated by the Ministry of Economy and Business (RYC-2016-19353) and the European Social Fund.
Abbreviations
API | application programming interface |
HPV | human papilloma virus |
Multimedia Appendix 1
Multimedia appendix 2, multimedia appendix 3, multimedia appendix 4.
Conflicts of Interest: None declared.
What are you looking for?
The researchers sought to understand how the reward structure of social media sites drives users to develop habits of posting misinformation on social media. (Photo/AdobeStock)
USC study reveals the key reason why fake news spreads on social media
The USC-led study of more than 2,400 Facebook users suggests that platforms — more than individual users — have a larger role to play in stopping the spread of misinformation online.
USC researchers may have found the biggest influencer in the spread of fake news: social platforms’ structure of rewarding users for habitually sharing information.
The team’s findings, published Monday by Proceedings of the National Academy of Sciences , upend popular misconceptions that misinformation spreads because users lack the critical thinking skills necessary for discerning truth from falsehood or because their strong political beliefs skew their judgment.
Just 15% of the most habitual news sharers in the research were responsible for spreading about 30% to 40% of the fake news.
The research team from the USC Marshall School of Business and the USC Dornsife College of Letters, Arts and Sciences wondered: What motivates these users? As it turns out, much like any video game, social media has a rewards system that encourages users to stay on their accounts and keep posting and sharing. Users who post and share frequently, especially sensational, eye-catching information, are likely to attract attention.
“Due to the reward-based learning systems on social media, users form habits of sharing information that gets recognition from others,” the researchers wrote. “Once habits form, information sharing is automatically activated by cues on the platform without users considering critical response outcomes, such as spreading misinformation.”
Posting, sharing and engaging with others on social media can, therefore, become a habit.
“[Misinformation is] really a function of the structure of the social media sites themselves.” — Wendy Wood , USC expert on habits
“Our findings show that misinformation isn’t spread through a deficit of users. It’s really a function of the structure of the social media sites themselves,” said Wendy Wood , an expert on habits and USC emerita Provost Professor of psychology and business.
“The habits of social media users are a bigger driver of misinformation spread than individual attributes. We know from prior research that some people don’t process information critically, and others form opinions based on political biases, which also affects their ability to recognize false stories online,” said Gizem Ceylan, who led the study during her doctorate at USC Marshall and is now a postdoctoral researcher at the Yale School of Management . “However, we show that the reward structure of social media platforms plays a bigger role when it comes to misinformation spread.”
In a novel approach, Ceylan and her co-authors sought to understand how the reward structure of social media sites drives users to develop habits of posting misinformation on social media.
Why fake news spreads: behind the social network
Overall, the study involved 2,476 active Facebook users ranging in age from 18 to 89 who volunteered in response to online advertising to participate. They were compensated to complete a “decision-making” survey approximately seven minutes long.
Surprisingly, the researchers found that users’ social media habits doubled and, in some cases, tripled the amount of fake news they shared. Their habits were more influential in sharing fake news than other factors, including political beliefs and lack of critical reasoning.
Frequent, habitual users forwarded six times more fake news than occasional or new users.
“This type of behavior has been rewarded in the past by algorithms that prioritize engagement when selecting which posts users see in their news feed, and by the structure and design of the sites themselves,” said second author Ian A. Anderson , a behavioral scientist and doctoral candidate at USC Dornsife. “Understanding the dynamics behind misinformation spread is important given its political, health and social consequences.”
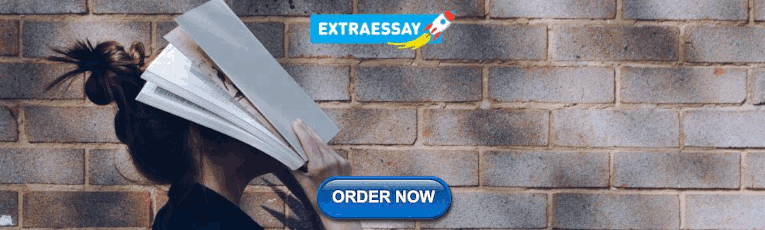
Experimenting with different scenarios to see why fake news spreads
In the first experiment, the researchers found that habitual users of social media share both true and fake news.
In another experiment, the researchers found that habitual sharing of misinformation is part of a broader pattern of insensitivity to the information being shared. In fact, habitual users shared politically discordant news — news that challenged their political beliefs — as much as concordant news that they endorsed.
Lastly, the team tested whether social media reward structures could be devised to promote sharing of true over false information. They showed that incentives for accuracy rather than popularity (as is currently the case on social media sites) doubled the amount of accurate news that users share on social platforms.
The study’s conclusions:
- Habitual sharing of misinformation is not inevitable.
- Users could be incentivized to build sharing habits that make them more sensitive to sharing truthful content.
- Effectively reducing misinformation would require restructuring the online environments that promote and support its sharing.
These findings suggest that social media platforms can take a more active step than moderating what information is posted and instead pursue structural changes in their reward structure to limit the spread of misinformation.
About the study: The research was supported and funded by the USC Dornsife College of Letters, Arts and Sciences Department of Psychology, the USC Marshall School of Business and the Yale University School of Management.
Related Articles
Molecules derived from sea sponge show promising effects in cancer, mitochondrial function, usc viterbi faculty member tackles climate and air quality challenges, how the usc stem cell master’s program changed the lives of 10 alumni.
Misinformation in Social Media
Subject: | Entertainment & Media |
---|---|
Pages: | 1 |
Words: | 304 |
Reading time: | 2 min |
Study level: | College |
In modern society, the internet and social media have replaced real-life communication for many people. Social networking sites have become an integral part of fulfilling lives of every member of society. People spend most of their free time on social networking sites contacting friends and family, finding the inspiration for work, and being engaged in different social activities. At the times of emerging technologies, it is essential to find a balance between networking and real life. The effects of social media on human life can be both: positive and negative.
On the one hand, people have a convenient way to communicate, which saves time significantly. Today, we can contact people worldwide and keep in touch with distant relatives and friends. The working communication is also alleviated thanks to social media. Various services help people to satisfy all their communicational and information-gaining needs. On the other hand, the temptation to procrastinate is evolving, which leads to inefficient time management. Moreover, the main problem of the widespread of social media is the so-called networking manipulation (Carley et al., 2019). The human brain cannot comprehend large volumes of different information. As a result, people are lost because they cannot determine whether the data is truthful. This can cause such psychological problems as anxiety and incredulity.
Thus, social media have both positive and negative impacts on human life. Thanks to the internet and social networking sites, people have access to information and easy communication. However, the easier some process, the less value people see in it. As a result, social media can cause irrelevant time management problems. Another problem is the misleading information in social media, which can be used for malicious aims. People should use these technological opportunities for their personal development and avoid degradation and laziness, which can be negative implications of social media active usage.
Carley, K., Kiu, H., Morstatter, F., & Wu, L. (2019). Misinformation in social media: Definition, manipulation, and detection. ACM SIGKDD Explorations Newsletter, 21 (2), 80–90. Web.
The disaster of misinformation: a review of research in social media
- Published: 15 February 2022
- Volume 13 , pages 271–285, ( 2022 )
Cite this article
- Sadiq Muhammed T ORCID: orcid.org/0000-0002-4614-2333 1 &
- Saji K. Mathew ORCID: orcid.org/0000-0002-8551-8209 1
50k Accesses
57 Citations
550 Altmetric
69 Mentions
Explore all metrics
The spread of misinformation in social media has become a severe threat to public interests. For example, several incidents of public health concerns arose out of social media misinformation during the COVID-19 pandemic. Against the backdrop of the emerging IS research focus on social media and the impact of misinformation during recent events such as the COVID-19, Australian Bushfire, and the USA elections, we identified disaster, health, and politics as specific domains for a research review on social media misinformation. Following a systematic review process, we chose 28 articles, relevant to the three themes, for synthesis. We discuss the characteristics of misinformation in the three domains, the methodologies that have been used by researchers, and the theories used to study misinformation. We adapt an Antecedents-Misinformation-Outcomes (AMIO) framework for integrating key concepts from prior studies. Based on the AMIO framework, we further discuss the inter-relationships of concepts and the strategies to control the spread of misinformation on social media. Ours is one of the early reviews focusing on social media misinformation research, particularly on three socially sensitive domains; disaster, health, and politics. This review contributes to the emerging body of knowledge in Data Science and social media and informs strategies to combat social media misinformation.
Similar content being viewed by others
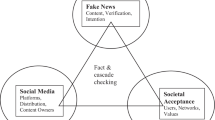
Fake news on Social Media: the Impact on Society
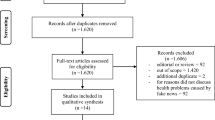
The impact of fake news on social media and its influence on health during the COVID-19 pandemic: a systematic review
Media and Stereotypes
Avoid common mistakes on your manuscript.
1 Introduction
1.1 information disorder in social media.
Rumors, misinformation, disinformation, and mal-information are common challenges confronting media of all types. It is, however, worse in the case of digital media, especially on social media platforms. Ease of access and use, speed of information diffusion, and difficulty in correcting false information make control of undesirable information a horrid task [ 1 ]. Alongside these challenges, social media has also been highly influential in spreading timely and useful information. For example, the recent #BlackLivesMatter movement was enabled by social media, which united concurring people's solidarity across the world when George Floyd was killed due to police brutality, and so are 2011 Arab spring in the Middle East and the 2017 #MeToo movement against sexual harassments and abuse [ 2 , 3 ]. Although, scholars have addressed information disorder in social media, a synthesis of the insights from these studies are rare.
The information which is fake or misleading and spreads unintentionally is known as misinformation [ 4 ]. Prior research on misinformation in social media has highlighted various characteristics of misinformation and interventions thereof in different contexts. The issue of misinformation has become dominant with the rise of social media, attracting scholarly attention, particularly after the 2016 USA Presidential election, when misinformation apparently influenced the election results [ 5 ]. The word 'misinformation' was listed as one of the global risks by the World Economic Forum [ 6 ]. A similar term that is popular and confusing along with misinformation is 'disinformation'. It is defined as the information that is fake or misleading, and unlike misinformation, spreads intentionally. Disinformation campaigns are often seen in a political context where state actors create them for political gains. In India, during the initial stage of COVID-19, there was reportedly a surge in fake news linking the virus outbreak to a particular religious group. This disinformation spread gained media attention as it was widely shared on social media platforms. As a result of the targeting, it eventually translated into physical violence and discriminatory treatment against members of the community in some of the Indian states [ 7 ]. 'Rumors' and 'fake news' are similar terms related to misinformation. 'Rumors' are unverified information or statements circulated with uncertainty, and 'fake news' is the misinformation that is distributed in an official news format. Source ambiguity, personal involvement, confirmation bias, and social ties are some of the rumor-causing factors. Yet another related term, mal-information, is accurate information that is used in different contexts to spread hatred or abuse of a person or a particular group. Our review focuses on misinformation that is spread through social media platforms. The words 'rumor', and 'misinformation' are used interchangeably in this paper. Further, we identify factors that cause misinformation based on a systematic review of prior studies.
Ours is one of the early attempts to review social media research on misinformation. This review focuses on three sensitive domains of disaster, health, and politics, setting three objectives: (a) to analyze previous studies to understand the impact of misinformation on the three domains (b) to identify theoretical perspectives used to examine the spread of misinformation on social media and (c) to develop a framework to study key concepts and their inter-relationships emerging from prior studies. We identified these specific areas as the impact of misinformation with regards to both speed of spread and scale of influence are high and detrimental to the public and governments. To the best of our knowledge, the review of the literature on social media misinformation themes are relatively scanty. This review contributes to an emerging body of knowledge in Data Science and informs the efforts to combat social media misinformation. Data Science is an interdisciplinary area which incorporates different areas like statistics, management, and sociology to study the data and create knowledge out of data [ 8 ]. This review will also inform future studies that aim to evaluate and compare patterns of misinformation on sensitive themes of social relevance, such as disaster, health, and politics.
The paper is structured as follows. The first section introduces misinformation in social media context. In Sect. 2 , we provide a brief overview of prior research works on misinformation and social media. Section 3 describes the research methodology, which includes details of the literature search and selection process. Section 4 discusses the analysis of spread of misinformation on social media based on three themes- disaster, health, and politics and the review findings. This includes current state of research, theoretical foundations, determinants of misinformation in social media platforms, and strategies to control the spread of misinformation. Section 5 concludes with the implications and limitations of the paper.
2 Social media and spread of misinformation
Misinformation arises in uncertain contexts when people are confronted with a scarcity of information they need. During unforeseen circumstances, the affected individual or community experiences nervousness or anxiety. Anxiety is one of the primary reasons behind the spread of misinformation. To overcome this tension, people tend to gather information from sources such as mainstream media and official government social media handles to verify the information they have received. When they fail to receive information from official sources, they collect related information from their peer circles or other informal sources, which would help them to control social tension [ 9 ]. Furthermore, in an emergency context, misinformation helps community members to reach a common understanding of the uncertain situation.
2.1 The echo chamber of social media
Social media has increasingly grown in power and influence and has acted as a medium to accelerate sociopolitical movements. Network effects enhance participation in social media platforms which in turn spread information (good or bad) at a faster pace compared to traditional media. Furthermore, due to a massive surge in online content consumption primarily through social media both business organizations and political parties have begun to share content that are ambiguous or fake to influence online users and their decisions for financial and political gains [ 9 , 10 ]. On the other hand, people often approach social media with a hedonic mindset, which reduces their tendency to verify the information they receive [ 9 ]. Repetitive exposure to contents that coincides with their pre-existing beliefs, increases believability and shareability of content. This process known as the echo-chamber effect [ 11 ] is fueled by confirmation bias. Confirmation bias is the tendency of the person to support information that reinforces pre-existing beliefs and neglect opposing perspectives and viewpoints other than their own.
Platforms’ structure and algorithms also have an essential role in spreading misinformation. Tiwana et al. [ 12 ] have defined platform architecture as ‘a conceptual blueprint that describes how the ecosystem is partitioned into a relatively stable platform and a complementary set of modules that are encouraged to vary, and the design rules binding on both’. Business models of these platforms are based upon maximizing user engagement. For example, in the case of Facebook or Twitter, user feed is based on their existing belief or preferences. User feeds provide users with similar content that matches their existing beliefs, thus contributing to the echo chamber effect.
Platform architecture makes the transmission and retransmission of misinformation easier [ 12 , 13 ]. For instance, WhatsApp has a one-touch forward option that enables users to forward messages simultaneously to multiple users. Earlier, a WhatsApp user could forward a message to 250 groups or users at a time, which as a measure for controlling the spread of misinformation was limited to five members in 2019. WhatsApp claimed that globally this restriction reduced message forwarding by 25% [ 14 ]. Apart from platform politics, users also have an essential role in creating or distributing misinformation. In a disaster context, people tend to share misinformation based on their subjective feeling [ 15 ].
Misinformation has the power to influence the decisions of its audience. It can change a citizen's approach toward a topic or a subject. The anti-vaccine movement on Twitter during the 2015 measles (highly communicable disease) outbreak in Disneyland, California, serves as a good example. The movement created conspiracy theories and mistrust on the State, which increased vaccine refusal rate [ 16 ]. Misinformation could even influence election of governments by manipulating citizens’ political attitudes as seen in the 2016 USA and 2017 French elections [ 17 ]. Of late, people rely heavily on Twitter and Facebook to collect the latest happenings from mainstream media [ 18 ].
Combating misinformation in social media has been a challenging task for governments in several countries. When social media influences elections [ 17 ] and health campaigns (like vaccination), governments and international agencies demand social media owners to take necessary actions to combat misinformation [ 13 , 15 ]. Platforms began to regulate bots that were used to spread misinformation. Facebook announced the filtering of their algorithms to combat misinformation, down-ranking the post flagged by their fact-checkers which will reduce the popularity of the post or page. [ 17 ]. However, misinformation has become a complicated issue due to the growth of new users and the emergence of new social media platforms. Jang et al. [ 19 ] have suggested two approaches other than governmental regulation to control misinformation literary and corrective. The literary approach proposes educating users to increase their cognitive ability to differentiate misinformation from the information. The corrective approach provides more fact-checking facilities for users. Warnings would be provided against potentially fabricated content based on crowdsourcing. Both approaches have limitations; the literary approach attracted criticism as it transfers responsibility for the spread of misinformation to citizens. The corrective approach will only have a limited impact as the volume of fabricated content escalates [ 19 , 20 , 21 ].
An overview of the literature on misinformation reveals that most investigations focus on examining the methods to combat misinformation. Social media platforms are still discovering new tools and techniques to mitigate misinformation from their platforms, this calls for a research to understand their strategies.
3 Review method
This research followed a systematic literature review process. The study employed a structured approach based on Webster’s Guidelines [ 22 ] to identify relevant literature on the spread of misinformation. These guidelines helped in maintaining a quality standard while selecting the literature for review. The initial stage of the study involved exploring research papers from relevant databases to understand the volumes and availability of research articles. We extended the literature search to interdisciplinary databases too. We gathered articles from Web of Science, ACM digital library, AIS electronic library, EBSCO host business source premier, ScienceDirect, Scopus, and Springer link. Apart from this, a manual search was performed in Information Systems (IS) scholars' basket of journals [ 23 ] to ensure we did not miss any articles from these journals. We have also preferred articles that have Data Science and Information Systems background. The systematic review process began with keyword search using predefined keywords (Fig. 2 ). We identified related synonyms such as 'misinformation', 'rumors', 'spread', and 'social media' along with their combinations for the search process. The keyword search was on the title, abstract, and on the list of keywords. The literature search was conducted in the month of April 2020. Later, we revisited the literature in December 2021 to include latest publications from 2020 to 2021.
It was observed that scholarly discussion about ‘misinformation and social media’ began to appear in research after 2008. Later in 2010, the topic gained more attention when Twitter bots were used or spreading fake news on the replacement of a USA Senator [ 24 ]. Hate campaigns and fake follower activities were simultaneously growing during that period. As evident from Fig. 1 , showing number of articles published between 2005 and 2021 on misinformation in three databases: Scopus, S pringer, and EBSCO, academic engagement on misinformation seems to have gained more impetus after the 2016 US Presidential election, when social media platforms had apparently influenced the election [ 20 ].
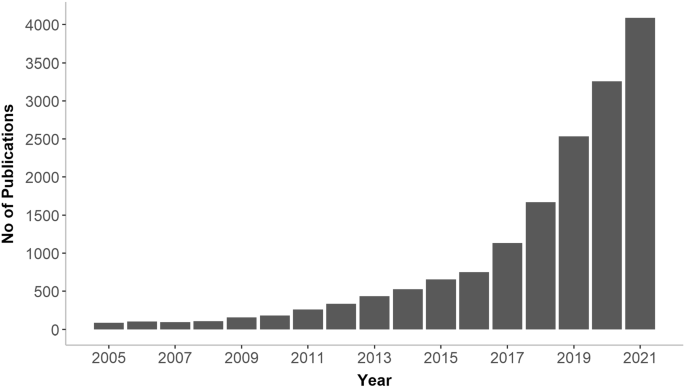
Articles published on misinformation during 2005–2021 (Databases; Scopus, Springer, and EBSCO)
As Data Science is an interdisciplinary field, the focus of our literature review goes beyond disciplinary boundaries. In particular, we focused on the three domains of disaster, health, and politics. This thematic focus of our review has two underlying reasons (a) the impact of misinformation through social media is sporadic and has the most damaging effects in these three domains and (b) our selection criteria in systematic review finally resulted in research papers that related to these three domains. This review has excluded platforms that are designed for professional and business users such as LinkedIn and Behance. A rational for the choice of these themes are discussed in the next section.
3.1 Inclusion–exclusion criteria
Figure 2 depicts the systematic review process followed in this study. In our preliminary search, 2148 records were retrieved from databases—all those articles were gathered onto a spreadsheet, which was manually cross-checked with the journals linked to the articles. Studies published during 2005–2021, studies published in English language, articles published from peer-reviewed journals, journals rating and papers relevant to misinformation were used as the inclusion criteria. We have excluded reviews, thesis, dissertations, and editorials; and articles on misinformation that are not akin to social media. To fetch the best from these articles, we selected articles that were from top journals, rated above three according to ABS rating and A*, A, and B according to ABDC rating. This process, while ensuring the quality of papers, also effectively shortened purview of study to 643 articles of acceptable quality. We have not performed track-back and track-forward on references. During this process, duplicate records were also identified and removed. Further screening of articles based on the title, abstract, and full text (wherever necessary)—brought down the number to 207 articles.
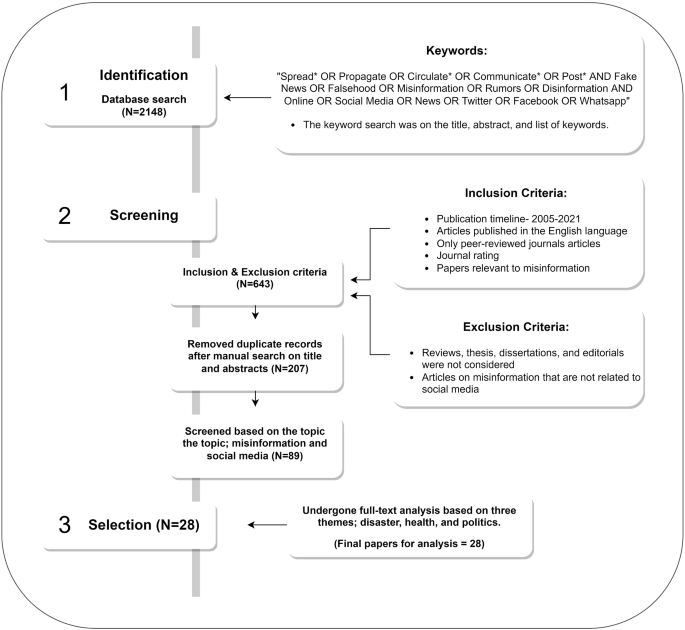
Systematic literature review process
Further screening based on the three themes reduced the focus to 89 articles. We conducted a full-text analysis of these 89 articles. We further excluded articles that had not considered misinformation as a central theme and finally arrived at 28 articles for detailed review (Table 1 ).
The selected studies used a variety of research methods to examine the misinformation on social media. Experimentation and text mining of tweets emerged as the most frequent research methods; there were 11 studies that used experimental methods, and eight used Twitter data analyses. Apart from these, there were three survey methods, two mixed methods, and case study methods each, and one opportunistic sampling and exploratory study each. The selected literature for review includes nine articles on disaster, eight on healthcare, and eleven from politics. We preferred papers for review based on three major social media platforms; Twitter, Facebook, and WhatsApp. These are the three social media owners with the highest transmission rates and most active users [ 25 ] and most likely platforms for misinformation propagation.
3.2 Coding procedure
Initially both the authors have manually coded the articles individually by reading full text of each article and then identified the three themes; disaster, health, and politics. We used an inductive coding approach to derive codes from the data. The intercoder reliability rate between the authors were 82.1%. Disagreement among authors related to deciding in which theme few papers fall under were discussed and a resolution was arrived at. Later we used NVIVO, a qualitative data analysis software, to analyze unstructured data to encode and categorize the themes from the articles. The codes emerged from the articles were categorized into sub-themes and later attached to the main themes; disaster, health, and politics. NVIVO produced a rank list of codes based on frequency of occurrence (“ Appendix ”). An intercoder reliability check was completed for the data by an external research scholar having a different areas of expertise to ensure reliability. The coder agreed upon 26 articles out of 28 (92.8%), which indicated a high level intercoder reliability [ 49 ]. The independent researcher’s disagreement about the code for two authors was discussed between the authors and the research scholar and a consensus was arrived at.
We initially reviewed articles separately from the categories of disaster, health, and politics. We first provide emergent issues that cut across these themes.
4.1 Social media misinformation research
Disaster, health, and politics emerged as the three domains (“ Appendix ”) where misinformation can cause severe harm, often leading to casualties or even irreversible effects. The mitigation of these effects can also demand substantial financial or human resources burden considering the scale of effect and risk of spreading negative information to the public altogether. All these areas are sensitive in nature. Further, disaster, health, and politics have gained the attention of researchers and governments as the challenges of misinformation confronting these domains are rampant. Besides sensitivity, misinformation in these areas has higher potential to exacerbate the existing crisis in society. During the 2020 Munich security conference, WHO’s Director-General noted: “We are not just fighting an epidemic; we are fighting an infodemic”, referring to the faster spread of COVID-19 misinformation than the virus [ 50 ].
More than 6000 people were hospitalized due to COVID-19 related misinformation in the first three months of 2020 [ 51 ]. As COVID-19 vaccination began, one of the popular myths was that Bill Gates wanted to use vaccines to embed microchips in people to track them and this created vaccine hesitancy among the citizens [ 52 ]. These reports show the severity of the spread of misinformation and how misinformation can aggravate a public health crisis.
4.2 Misinformation during disaster
In the context of emergency situations (unforeseen circumstances), the credibility of social media information has often been questioned [ 11 ]. When a crisis occurs, affected communities often experience a lack of localized information needed for them to make emergency decisions. This accelerates the spread of misinformation as people tend to fill this information gap with misinformation or 'improvised news' [ 9 , 24 , 25 ]. The broadcasting power of social media and re-sharing of misinformation could weaken and slow down rescue operations [ 24 , 25 ]. As the local people have more access to the disaster area, they become immediate reporters of a crisis through social media. Mainstream media comes into picture only later. However, recent incidents reveals that voluntary reporting of this kind has begun to affect rescue operations negatively as it often acts as a collective rumor mill [ 9 ], which propagates misinformation. During the 2018 floods in the South-Indian state of Kerala a fake video on Mullaperiyar Dam leakage created unnecessary panic among the citizens, thus negatively impacting the rescue operations [ 53 ]. Information from mainstream media is relatively more reliable as they have traditional gatekeepers such as peer reviewers and editors who cross-check the information source before publication. Chua et al. [ 28 ] found that a major chunk of corrective tweets were retweeted from mainstream news media, thus mainstream media is considered as a preferred rumor correction channel, where they attempt to correct misinformation with the right information.
4.2.1 Characterizing disaster misinformation
Oh et al. [ 9 ] studied citizen-driven information processing based on three social crises using rumor theory. The main characteristic of a crisis is the complexity of information processing and sharing [ 9 , 24 ]. A task is considered complex when characterized by increase in information load, information diversity or rate of information change [ 54 ]. Information overload and information dearth are the two grave concerns that interrupt the communication between the affected community and a rescue team. Information overload, where too many enquiries and fake news distract a response team, slows them down to recognize valid information [ 9 , 27 ]. According to Balan and Mathew [ 55 ] information overload occurs when volume of information such as complexity of words and multiple languages that exceeds and cannot be processed by a human being. Here information dearth in our context is the lack of localized information that is supposed to help the affected community to make emergency decisions. When the official government communication channels or mainstream media cannot fulfill citizen's needs, they resort to information from their social media peers [ 9 , 27 , 29 ].
In a social crisis context, Tamotsu Shibutani [ 56 ] defines rumoring as collective sharing and exchange of information, which helps the community members to reach a common understanding about the crisis situation [ 30 ]. This mechanism works in social media, which creates information dearth and information overload. Anxiety, information ambiguity (source ambiguity and content ambiguity), personal involvement, and social ties are the rumor-causing variables in a crisis context [ 9 , 27 ]. In general, anxiety is a negative feeling caused by distress or stressful situation, which fabricates or produces adverse outcomes [ 57 ]. In the context of a crisis or emergency, a community may experience anxiety in the absence of reliable information or in other cases when confronted with overload of information, making it difficult to take appropriate decisions. Under such circumstances, people may tend to rely on rumors as a primary source of information. The influence level of anxiety is higher during a community crisis than during a business crisis [ 9 ]. However, anxiety, as an attribute, varies based on the nature of platforms. For example, Oh et al. [ 9 ] found that the Twitter community do not fall into social pressure as like WhatsApp community [ 30 ]. Simon et al. [ 30 ] developed a model of rumor retransmission on social media and identified information ambiguity, anxiety and personal involvement as motives for rumormongering. Attractiveness is another rumor-causing variable. It occurs when aesthetically appealing visual aids or designs capture a receiver’s attention. Here believability matters more than the content’s reliability or the truth of the information received.
The second stage of the spread of misinformation is misinformation retransmission. Apart from the rumor-causing variables that are reported in Oh et al. [ 9 ], Liu et al. [ 13 ] found senders credibility and attractiveness as significant variables related to misinformation retransmission. Personal involvement and content ambiguity can also affect misinformation transmission [ 13 ]. Abdullah et al. [ 25 ] explored retweeter's motive on the Twitter platform to spread disaster information. Content relevance, early information [ 27 , 31 ], trustworthiness of the content, emotional influence [ 30 ], retweet count, pro-social behavior (altruistic behavior among the citizens during the crisis), and the need to inform their circle are the factors that drive users’ retweet [ 25 ]. Lee et al. [ 26 ] have also examined the impact of Twitter features on message diffusion based on the 2013 Boston marathon tragedy. The study reported that during crisis events (especially during disasters), a tweet that has less reaction time (time between the crisis and initial tweet) and had higher impact than other tweets. This shows that to an extent, misinformation can be controlled if officials could communicate at the early stage of a crisis [ 27 ]. Liu et al. [ 13 ] showed that tweets with hashtags influence spread of misinformation. Further, Lee et al. [ 26 ] found that tweets with no hashtags had more influence due to contextual differences. For instance, usage of hashtags for marketing or advertising has a positive impact, while in the case of disaster or emergency situations, usage of hashtags (as in case of Twitter) has a negative impact. Messages with no hashtag get widely diffused when compared to messages with the hashtag [ 26 ].
Oh et al. [ 15 ] explored the behavioral aspects of social media participants that led to retransmission and spread of misinformation. They found that when people believe a threatening piece of misinformation they received, they are more likely to spread it, and they take necessary safety measures (sometimes even extreme actions). Repetition of the same misinformation from different sources also makes it more believable [ 28 ]. However, when they realize the received information was false they were less likely to share it with others [ 13 , 26 ]. The characteristics of the platform used to deliver the misinformation also matters. For instance, numbers of likes and shares of the information increases the believability of the social media post [ 47 ].
In summary, we found that platform architecture also has an essential role in spreading and believability of misinformation. While conducting this systematic literature review, we observed that more studies on disaster and misinformation are based on the Twitter platform. The six papers out of nine that we reviewed on disaster area were based on the Twitter platform. When a message was delivered in video format, it had a higher impact compared to audio or text messages. If the message had a religious or cultural narrative, it led to behavioral action (danger control response) [ 15 ]. Users were more likely to spread misinformation through WhatsApp than Twitter. It was difficult to find the source of shared information on WhatsApp [ 30 ].
4.3 Misinformation related to healthcare
From our review, we found two systematic literature reviews that discusses health-related misinformation on social media. Yang et al. [ 58 ] explores the characteristics, impact and influences of health misinformation on social media. Wang et al. [ 59 ] addresses health misinformation related to vaccines and infectious diseases. This review shows that health-related misinformation, especially on M.M.R. vaccine and autism are largely spreading on social media and the government is unable to control it.
The spread of health misinformation is an emerging issue facing public health authorities. Health misinformation could delay proper treatment to patients, which could further add more casualties to the public health domain [ 28 , 59 , 60 ]. Often people tend to believe health-related information that is shared by their peers. Some of them tend to share their treatment experience or traditional remedies online. This information could be in a different context and may not be even accurate [ 33 , 34 ]. Compared to health-related websites, the language used to detail the health information shared on social media will be simple and may not include essential details [ 35 , 37 ]. Some studies reported that conspiracy theories and pseudoscience have escalated casualties [ 33 ]. Pseudoscience is the term referred to as the false claim, which pretends as if the shared misinformation has scientific evidence. The anti-vaccination movement on Twitter is one of the examples of pseudoscience [ 61 ]. Here the user might have shared the information due to the lack of scientific knowledge [ 35 ].
4.3.1 Characterizing healthcare misinformation
The attributes that characterize healthcare misinformation are distinctly different from other domains. Chua and Banerjee, [ 37 ] identified the characteristics of health misinformation as dread and wish. Dread is the rumor which creates more panic and unpleasant consequences. For example, in the wake of COVID-19, misinformation was widely shared on social media, which claimed that children 'died on the spot' after the mass COVID-19 vaccination program in Senegal, West Africa [ 61 ]. This message created panic among the citizens, as the misinformation was shared more than 7000 times on Facebook [ 61 ]. Wish is the type of rumor that gives hope to the receiver (e.g.,: rumor on free medicine distribution) [ 62 ]. Dread rumor looks more trustworthy and more likely to get viral. Dread rumor was the cause of violence against a minority group in India during COVID-19 [ 7 ]. Chua and Banerjee, [ 32 ] added pictorial and textual representations as the characteristics of health misinformation. The rumor that contains only text is textual rumor. Pictorial rumor on the other hand contains both text and images. However, Chua and Banerjee, [ 32 ] found that users prefer textual rumor than pictorial. Unlike rumors that are circulated during a natural disaster, health misinformation will be long-lasting, and it can spread cutting across boundaries. Personal involvement (the importance of information for both sender and receiver), rumor type and presence of counter rumor are some of the variables that can escalate users’ trusting and sharing behavior related to rumor [ 37 ]. The study of Madraki et al. [ 46 ] study on COVID-19 misinformation /disinformation reported that COVID-19 misinformation on social media differs significantly based on the languages, countries and their culture and beliefs. Acceptance of social media platforms as well as Governmental censorship also play an important role here.
Widespread misinformation could also change collective opinion [ 29 ]. Online users’ epistemic beliefs could control their sharing decisions. Chua and Banerjee, [ 32 ] argued that epistemologically naïve users (users who think knowledge can be acquired easily) are the type of users who accelerate the spread of misinformation on platforms. Those who read or share the misinformation are not likely to follow it [ 37 ]. Gu and Hong [ 34 ] examined health misinformation on mobile social media context. Mobile internet users are different from large screen users. The mobile phone user might have a more emotional attachment toward the gadget. It also motivates them to believe received misinformation. The corrective effort focused on large screen users may not work with mobile phone users or small screen users. Chua and Banerjee [ 32 ] suggested that simplified sharing options of platforms also motivate users to share the received misinformation before validating it. Shahi et al. [ 47 ] found that misinformation is also propagated or shared even by the verified Twitter handles. They become a part of misinformation transmission either by creating it or endorsing it by liking or sharing the information.
The focus of existing studies is heavily based on data from social networking sites such as Facebook and Twitter, although other platforms too escalate the spread of misinformation. Such a phenomenon was evident in the wake of COVID-19 as an intense trend of misinformation spread was reported on WhatsApp, TikTok, and Instagram.
4.4 Social media misinformation and politics
There have been several studies on the influence of misinformation on politics across the world [ 43 , 44 ]. Political misinformation has been predominantly used to influence the voters. The USA Presidential election of 2016, French election of 2017 and Indian elections in 2019 have been reported as examples where misinformation has influenced election process [ 15 , 17 , 45 ]. During the 2016 USA election, the partisan effect was a key challenge, where false information was presented as if it was from an authorized source [ 39 ]. Based on a user's prior behavior on the platform, algorithms can manipulate the user's feed [ 40 ]. In a political context, fake news can create more harm as it can influence the voters and the public. Although, fake news has less ‘life’, it's consequences may not be short living. Verification of fake news takes time and by the time verification results are shared, fake news could achieve its goal [ 43 , 48 , 63 ].
4.4.1 Characterizing misinformation in politics
Confirmation bias has a dominant role in social media misinformation related to politics. Readers are more likely to read and engage with the information that confirms their preexisting beliefs and political affiliations and reject information that challenges it [ 46 , 48 ]. For example, in the 2016 USA election, Pro-Trump fake news was accepted by Republicans [ 19 ]. Misinformation spreads quickly among people who have similar ideologies [ 19 ]. The nature of interface also could escalate the spread of misinformation. Kim and Dennis [ 36 ] investigated the influence of platforms' information presentation format and reported that social media platforms indirectly force users to accept certain information; they present information such that little importance is given to the source of information. This presentation is manipulative as people tend to believe information from a reputed source and are more likely to reject information that is from a less-known source [ 42 ].
Pennycook et al. [ 39 ], and Garrett and Poulsen [ 40 ] argued that warning tags (or flagging) on the headline can reduce the spread of misinformation. However, it is not practical to assign warning tags to all misinformation as it gets generated faster than valid information. The fact-checking process in social media also takes time. Hence, people tend to believe that the headlines which do not have warning tags are true and the idea of warning tags will thus not serve any purpose [ 39 ]. Furthermore, it could increase the reader's belief in warning tags and lead to misperception [ 39 ]. Readers tend to believe that all information is verified and consider untagged false information as more accurate. This phenomenon is known as the implied truth effect [ 39 ]. In this case, source reputation rating will influence the credibility of the information. The reader gives less importance to the source that has a low rating [ 17 , 50 ].
5 Theoretical perspectives of social media misinformation
We identified six theories among the articles we reviewed in relation to social media misinformation. We found rumor theory was used most frequently among all the studies chosen for our review; the theory was used in four articles as a theoretical foundation [ 9 , 11 , 13 , 37 , 43 ]. Oh et al. [ 9 ], studied citizen-driven information processing on Twitter using rumor theory in three social crises. This paper identified four key variables (source ambiguity, personal involvement, and anxiety) that spread misinformation. The authors further examined the acceptance of hate rumors and the aftermath of community crisis based on the Bangalore mass exodus of 2012. Liu et al. [ 13 ], examined the reason behind the retransmission of messages using rumor theory in disasters. Hazel Kwon and Raghav Rao [ 43 ] investigated how internet surveillance by the government impacts citizens’ involvement with cyber-rumors during a homeland security threat. Diffusion theory has also been used in IS research to discern the adoption of technological innovation. Researchers have used diffusion theory to study the retweeting behavior among Twitter users (tweet diffusion) during extreme events [ 26 ]. This research investigated information diffusion during extreme events based on four major elements of diffusion: innovation, time, communication channels and social systems. Kim et al. [ 36 ] examined the effect of rating news sources on users’ belief in social media articles based on three different rating mechanisms expert rating, user article rating and user source rating. Reputation theory was used to show how users would discern cognitive biases in expert ratings.
Murungi et al. [ 38 ] used rhetorical theory to argue that fact-checkers have less effectiveness on fake news that spreads on social media platforms. The study proposed a different approaches by focusing on underlying belief structure that accepts misinformation. The theory was used to identify fake news and socially constructed beliefs in the context of Alabama’s senatorial election in 2017. Using third person effect as the theoretical ground, the characteristics of rumor corrections on Twitter platform have also been examined in the context of death hoax of Singapore’s first prime minister Lee Kuan Yew [ 28 ]. This paper explored the motives behind collective rumor and identified the key characteristics of collective rumor correction. Using situational crisis communication theory (SCCT), Paek and Hove [ 44 ] examined how government could effectively respond to risk-related rumors during national-level crises in the context of food safety rumor. Refuting rumor, denying it and attacking the source of rumor are the three rumor response strategies suggested by the authors to counter rumor-mongering (Table 2 ).
5.1 Determinants of misinformation in social media platforms
Figure 3 depicts the concepts that emerged from our review using a framework of Antecedents-Misinformation-Outcomes (AMIO) framework, an approach we adapt from Smith HJ et al. [ 66 ]. Originally developed to study information privacy, the Antecedent-Privacy-Concerns-Outcomes (APCO) framework provided a nomological canvas to present determinants, mediators and outcome variables pertaining to information privacy. Following this canvas, we discuss the antecedents of misinformation, mediators of misinformation and misinformation outcomes, as they emerged from prior studies (Fig. 3 ).
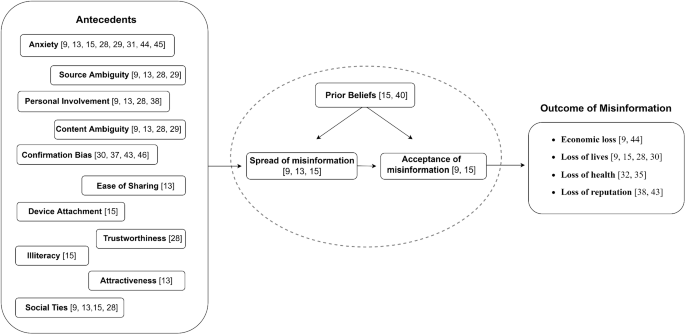
Determinants of misinformation
Anxiety, source ambiguity, trustworthiness, content ambiguity, personal involvement, social ties, confirmation bias, attractiveness, illiteracy, ease of sharing options and device attachment emerged as the variables determining misinformation in social media.
Anxiety is the emotional feeling of the person who sends or receives the information. If the person is anxious about the information received, he or she is more likely to share or spread misinformation [ 9 ]. Source ambiguity deals with the origin of the message. When the person is convinced of the source of information, it increases his trustworthiness and the person shares it. Content ambiguity addresses the content clarity of the information [ 9 , 13 ]. Personal involvement denotes how much the information is important for both the sender and receiver [ 9 ]. Social ties, information shared by a family member or social peers will influence the person to share the information [ 9 , 13 ]. From prior literature, it is understood that confirmation bias is one of the root causes of political misinformation. Research on attractiveness of the received information reveals that users tend to believe and share the information that is received on her or his personal device [ 34 ]. After receiving the misinformation from various sources, users accept it based on their existing beliefs, and social, cognitive factors and political factors. Oh et al. [ 15 ] observed that during crises, people by default have a tendency to believe unverified information especially when it helps them to make sense of the situation. Misinformation has significant effects on individuals and society. Loss of lives [ 9 , 15 , 28 , 30 ], economic loss [ 9 , 44 ], loss of health [ 32 , 35 ] and loss of reputation [ 38 , 43 ] are the major outcome of misinformation emerged from our review.
5.2 Strategies for controlling the spread of misinformation
Discourse on social media misinformation mitigation has resulted in prioritization of strategies such as early communication from the officials and use of scientific evidence [ 9 , 35 ]. When people realize that the received information or message is false, they are less likely to share that information with others [ 15 ]. Other strategies are 'rumor refutation—reducing citizens' intention to spread misinformation by real information which reduces their uncertainty and serves to control misinformation [ 44 ]. Rumor correction models for social media platforms also employ algorithms and crowdsourcing [ 28 ]. Majority of the papers that we have reviewed suggested fact-checking by experts, source rating of the received information, attaching warning tags to the headlines or entire news [ 36 ], and flagging content by the platform owners [ 40 ] as the strategies to control the spread of misinformation. Studies on controlling misinformation in the public health context showed that the government could also seek the help of public health professionals to mitigate misinformation [ 31 ].
However, the aforementioned strategies have been criticized for several limitations. Most papers mentioned confirmation bias as having a significant impact on the misinformation mitigation strategies, especially in the political context where people tend to believe the information that matches their prior belief. Garrett and Poulsen [ 40 ] argued that during an emergency situation, misinformation recipient may not be able to characterize the misinformation as true or false. Thus, providing alternative explanation or the real information to the users have more effect than providing fact-checking report. Studies by Garrett and Poulsen [ 40 ], and Pennycook et al. [ 39 ] reveal a drawback of attaching warning tags to news headlines. Once the flagging or tagging of the information is introduced, the information with the absence of tags will be considered as true or reliable information. This creates an implied truth effect. Further, it is also not always practical to evaluate all social media posts. Similarly, Kim and Dennis [ 36 ] studied fake news flagging and found that fake news flags did not influence users’ belief. However, they created cognitive dissonance and users were in search of the truthfulness of the headline. Later in 2017 Facebook discontinued the fake news flagging service owing to its limitations [ 45 ]
6 Key research gaps and future directions
Although, misinformation is a multi-sectoral issue, our systematic review observed that interdisciplinary research on social media misinformation is relatively scarce. ‘Confirmation bias’ is one of the most significant behavioral problem that motivates the spread of misinformation. However, lack of research on it reveals the scope for future interdisciplinary research across the fields of Data Science, Information Systems and Psychology in domains such as politics and health care. In the disaster context, there is a scope for study on the behavior of a first respondent and an emergency manager to understand their information exchange pattern with the public. Similarly, future researchers could analyze communication patterns between citizens and frontline workers in the public health context, which may be useful to design counter-misinformation campaigns and awareness interventions. Since information disorder is a multi-sectoral issue, researchers need to understand misinformation patterns among multiple government departments for coordinated counter-misinformation intervention.
There is a further dearth of studies on institutional responses to control misinformation. To fill the gap, future studies could concentrate on the analysis of governmental and organizational interventions to control misinformation at the level of policies, regulatory mechanisms, and communication strategies. For example, in India there is no specific law against misinformation but there are some provisions in the Information Technology Act (IT Act) and Disaster Management Act which can control misinformation and disinformation. An example of awareness intervention is an initiative named ‘Satyameva Jayate’ launched in Kannur district of Kerala, India which focused on sensitizing children at school to spot misinformation [ 67 ]. As noted earlier, within the research on Misinformation in the political context, there is a lack of research on strategies adopted by the state to counter misinformation. Therefore, building on cases like 'Satyameva Jayate' would further contribute to knowledge in this area.
Technology-based strategies adopted by social media to control the spread of misinformation emphasize the corrective algorithms, keywords and hashtags as a solution [ 32 , 37 , 43 ]. However, these corrective measures have their own limitations. Misinformation corrective algorithms are ineffective if not used immediately after the misinformation has been created. Related hashtags and keywords are used by researchers to find content shared on social media platforms to retrieve data. However, it may not be possible for researchers to cover all the keywords or hashtags employed by users. Further, algorithms may not decipher content shared in regional languages. Another limitation of algorithms employed by platforms is that they recommend and often display content based on user activities and interests which limits the users access to information from multiple perspectives, thus reinforcing their existing belief [ 29 ]. A reparative measure is to display corrective information as 'related stories' for misinformation. However, Facebook’s related stories algorithm only activates when an individual clicks on an outside link, which limits the number of people who will see the corrective information through the algorithm which turns out to be a challenge. Future research could investigate the impact of related stories as a corrective measure by analyzing the relation between misinformation and frequency of related stories posted vis a vis real information.
Our review also found a scarcity of research on the spread of misinformation on certain social media platforms while studies being skewed toward a few others. Of the studies reviewed, 15 articles were concentrated on misinformation spread on Twitter and Facebook. Although, from recent news reports it is evident that largely misinformation and disinformation are spread through popular messaging platforms like the 'WhatsApp', ‘Telegram’, ‘WeChat’, and ‘Line’, research using data from these platforms are, however, scanty. Especially in the Indian context, the magnitude of problems arising from misinformation through WhatsApp are overwhelming [ 68 ]. To address the lacunae of research on messaging platforms, we suggest future researchers to concentrate on investigating the patterns of misinformation spreading on platforms like WhatsApp. Moreover, message diffusion patterns are unique to each social media platform; therefore, it is useful to study the misinformation diffusion patterns on different social media platforms. Future studies could also address the differential roles, patterns and intensity of the spread of misinformation on various messaging and photo/ video-sharing social networking services.
Evident from our review, most research on misinformation is based on Euro-American context and the dominant models proposed for controlling misinformation may have limited applicability to other regions. Moreover, the popularity of social media platforms and usage patterns are diverse across the globe consequent to cultural differences and political regimes of the region, therefore necessitating researchers of social media to take cognizance of empirical experiences of ' left-over' regions.
7 Conclusion
To understand the spread of misinformation on social media platforms, we conducted a systematic literature review in three important domains where misinformation is rampant: disaster, health, and politics. We reviewed 28 articles relevant to the themes chosen for the study. This is one of the earliest reviews focusing on social media misinformation research, especially based on three sensitive domains. We have discussed how misinformation spreads in the three sectors, the methodologies that have been used by researchers, theoretical perspectives, Antecedents-Misinformation-Outcomes (AMIO) framework for understanding key concepts and their inter-relationships, and strategies to control the spread of misinformation.
Our review also identified major gaps in IS research on misinformation in social media. This includes the need for methodological innovations in addition to experimental methods which have been widely used. This study has some limitations that we acknowledge. We might not have identified all relevant papers on spread of misinformation on social media from existing literature as some authors might have used different keywords and also due to our strict inclusion and exclusion criteria. There might also have been relevant publications in languages other than English which were not covered in this review. Our focus on three domains also restricted the number of papers we reviewed.
Thai, M.T., Wu, W., Xiong, H.: Big Data in Complex and Social Networks, 1st edn. CRC Press, Boca Raton (2017)
Google Scholar
Peters, B.: How Social Media is Changing Law and Policy. Fair Observer (2020)
Granillo, G.: The Role of Social Media in Social Movements. Portland Monthly (2020)
Wu, L., Morstatter, F., Carley, K.M., Liu, H.: Misinformation in social media: definition, manipulation, and detection. ACM SIGKDD Explor. 21 (1), 80–90 (2019)
Article Google Scholar
Sam, L.: Mark Zuckerberg: I regret ridiculing fears over Facebook’s effect on election|technology|the guardian. Theguardian (2017)
WEF: Global Risks 2013—World Economic Forum (2013)
Scroll: Communalisation of Tablighi Jamaat Event. Scroll.in (2020)
Cao, L.: Data science: a comprehensive overview. ACM Comput. Surv. 50 (43), 1–42 (2017)
Oh, O., Agrawal, M., Rao, H.R.: Community intelligence and social media services: a rumor theoretic analysis of tweets during social crises. MIS Q. Manag. Inf. Syst. 37 (2), 407–426 (2013)
Mukherjee, A., Liu, B., Glance, N.: Spotting fake reviewer groups in consumer reviews. In: WWW’12—Proceedings of the 21st Annual Conference on World Wide Web, pp. 191–200 (2012)
Cerf, V.G.: Information and misinformation on the internet. Commun. ACM 60 (1), 9–9 (2016)
Tiwana, A., Konsynski, B., Bush, A.A.: Platform evolution: coevolution of platform architecture, governance, and environmental dynamics. Inf. Syst. Res. 21 (4), 675–687 (2010)
Liu, F., Burton-Jones, A., Xu, D.: Rumors on social media in disasters: extending transmission to retransmission. PACIS 2014
Hern, A.: WhatsApp to impose new limit on forwarding to fight fake news. The Guardian (2020)
Oh, O., Gupta, P., Agrawal, M., Raghav Rao, H.: ICT mediated rumor beliefs and resulting user actions during a community crisis. Gov. Inf. Q. 35 (2), 243–258 (2018)
Xiaoyi, A.T.C.Y.: Examining online vaccination discussion and communities in Twitter. In: SMSociety ’18: Proceedings of the 9th International Conference on Social Media and Society (2018)
Lazer, D.M.J., et al.: The science of fake news. Sciencemag.org (2018)
Peter, S.: More Americans are getting their news from social media. forbes.com (2019)
Jang, S.M., et al.: A computational approach for examining the roots and spreading patterns of fake news: evolution tree analysis. Comput. Hum. Behav. 84 , 103–113 (2018)
Mele, N., et al.: Combating Fake News: An Agenda for Research and Action (2017)
Bernhard, U., Dohle, M.: Corrective or confirmative actions? Political online participation as a consequence of presumed media influences in election campaigns. J. Inf. Technol. Polit. 12 (3), 285–302 (2015)
Webster, J., Watson, R.T.: Analyzing the past to prepare for the future: writing a literature review. MIS Q. 26 (2) (2002)
aisnet.org: Senior Scholars’ Basket of Journals|AIS. aisnet.org . [Online]. Available: https://aisnet.org/page/SeniorScholarBasket . Accessed: 16 Sept 2021
Torres, R.R., Gerhart, N., Negahban, A.: Epistemology in the era of fake news: an exploration of information verification behaviors among social networking site users. ACM 49 , 78–97 (2018)
Abdullah, N.A., Nishioka, D., Tanaka, Y., Murayama, Y.: Why I retweet? Exploring user’s perspective on decision-making of information spreading during disasters. In: Proceedings of the 50th Hawaii International Conference on System Sciences (2017)
Lee, J., Agrawal, M., Rao, H.R.: Message diffusion through social network service: the case of rumor and non-rumor related tweets during Boston bombing 2013. Inf. Syst. Front. 17 (5), 997–1005 (2015)
Mondal, T., Pramanik, P., Bhattacharya, I., Boral, N., Ghosh, S.: Analysis and early detection of rumors in a post disaster scenario. Inf. Syst. Front. 20 (5), 961–979 (2018)
Chua, A.Y.K., Cheah, S.-M., Goh, D.H., Lim, E.-P.: Collective rumor correction on the death hoax. In: PACIS 2016 Proceedings (2016)
Bode, L., Vraga, E.K.: In related news, that was wrong: the correction of misinformation through related stories functionality in social media. J. Commun. 65 (4), 619–638 (2015)
Simon, T., Goldberg, A., Leykin, D., Adini, B.: Kidnapping WhatsApp—rumors during the search and rescue operation of three kidnapped youth. Comput. Hum. Behav. 64 , 183–190 (2016)
Ghenai, A., Mejova, Y.: Fake cures: user-centric modeling of health misinformation in social media. In: Proceedings of ACM Human–Computer Interaction, vol. 2, no. CSCW, pp. 1–20 (2018)
Chua, A.Y.K., Banerjee, S.: To share or not to share: the role of epistemic belief in online health rumors. Int. J. Med. Inf. 108 , 36–41 (2017)
Kou, Y., Gui, X., Chen, Y., Pine, K.H.: Conspiracy talk on social media: collective sensemaking during a public health crisis. In: Proceedings of ACM Human–Computer Interaction, vol. 1, no. CSCW, pp. 1–21 (2017)
Gu, R., Hong, Y.K.: Addressing health misinformation dissemination on mobile social media. In: ICIS 2019 Proceedings (2019)
Bode, L., Vraga, E.K.: See something, say something: correction of global health misinformation on social media. Health Commun. 33 (9), 1131–1140 (2018)
Kim, A., Moravec, P.L., Dennis, A.R.: Combating fake news on social media with source ratings: the effects of user and expert reputation ratings. J. Manag. Inf. Syst. 36 (3), 931–968 (2019)
Chua, A.Y.K., Banerjee, S.: Intentions to trust and share online health rumors: an experiment with medical professionals. Comput. Hum. Behav. 87 , 1–9 (2018)
Murungi, D., Purao, S., Yates, D.: Beyond facts: a new spin on fake news in the age of social media. In: AMCIS 2018 Proceedings (2018)
Pennycook, G., Bear, A., Collins, E.T., Rand, D.G.: The implied truth effect: attaching warnings to a subset of fake news headlines increases perceived accuracy of headlines without warnings. Manag. Sci. (2020). https://doi.org/10.1287/mnsc.2019.3478
Garrett, R., Poulsen, S.: Flagging Facebook falsehoods: self identified humor warnings outperform fact checker and peer warnings. J. Comput. Commun. (2019). https://doi.org/10.1093/jcmc/zmz012
Shin, J., Thorson, K.: Partisan selective sharing: the biased diffusion of fact-checking messages on social media. J. Commun. 67 (2), 233–255 (2017)
Kim, A., Dennis, A.R.: Says who? The effects of presentation format and source rating on fake news in social media. MIS Q. (2019). https://doi.org/10.25300/MISQ/2019/15188
Hazel Kwon, K., Raghav Rao, H.: Cyber-rumor sharing under a homeland security threat in the context of government Internet surveillance: the case of South–North Korea conflict. Gov. Inf. Q. 34 (2), 307–316 (2017)
Paek, H.J., Hove, T.: Effective strategies for responding to rumors about risks: the case of radiation-contaminated food in South Korea. Public Relat. Rev. 45 (3), 101762 (2019)
Moravec, P.L., Minas, R.K., Dennis, A.R.: Fake news on social media: people believe what they want to believe when it makes no sense at all. MIS Q. (2019). https://doi.org/10.25300/MISQ/2019/15505
Madraki et al.: Characterizing and comparing COVID-19 misinformation across languages, countries and platforms. In: WWW ’21 Companion Proceedings of Web Conference (2021)
Shahi, G.K., Dirkson, A., Majchrzak, T.A.: An exploratory study of COVID-19 misinformation on Twitter. Public Heal. Emerg. COVID-19 Initiat. 22 , 100104 (2021)
Otala, M., et al.: Political polarization and platform migration: a study of Parler and Twitter usage by United States of America Congress Members. In: WWW ’21 Companion Proceedings of Web Conference (2021)
Paul, L.J.: Encyclopedia of Survey Research Methods. Sage Research Methods, Thousand Oaks (2008)
WHO Munich Security Conference: WHO.int. [Online]. Available: https://www.who.int/director-general/speeches/detail/munich-security-conference . Accessed 24 Sept 2021
Coleman, A.: Hundreds dead’ because of Covid-19 misinformation—BBC News. BBC News (2020)
Benenson, E.: Vaccine myths Facts vs fiction|VCU Health. vcuhealth.org , 2021. [Online]. Available: https://www.vcuhealth.org/news/covid-19/vaccine-myths-facts-vs-fiction . Accessed 24 Sept 2021
Pierpoint, G.: Kerala floods: fake news ‘creating unnecessary panic’—BBC News. BBC (2018)
Campbell, D.J.: Task complexity: a review and analysis. Acad. Manag. Rev. 13 (1), 40 (1988)
Balan, M.U., Mathew, S.K.: Personalize, summarize or let them read? A study on online word of mouth strategies and consumer decision process. Inf. Syst. Front. 23 , 1–21 (2020)
Shibutani, T.: Improvised News: A Sociological Study of Rumor. The Bobbs-Merrill Company Inc, Indianapolis (1966)
Pezzo MV, Beckstead JW (2006) A multilevel analysis of rumor transmission: effects of anxiety and belief in two field experiments Basic Appl. Soc. Psychol. https://doi.org/10.1207/s15324834basp2801_8
Li, Y.-J., Cheung, C.M.K. Shen, X.-L., Lee, M.K.O.: Health misinformation on social media: a literature review. In: Association for Information Systems (2019)
Wang, Y., McKee, M., Torbica, A., Stuckler, D.: Systematic literature review on the spread of health-related misinformation on social media. Soc. Sci. Med. 240 , 112552 (2019)
Pappa, D., Stergioulas, L.K.: Harnessing social media data for pharmacovigilance: a review of current state of the art, challenges and future directions. Int. J. Data Sci. Anal. 8 (2), 113–135 (2019)
BBC: Fighting Covid-19 fake news in Africa. BBC News (2020)
Chua, A.Y.K., Aricat, R., Goh, D.: Message content in the life of rumors: comparing three rumor types. In: 2017 12th International Conference on Digital Information Management, ICDIM 2017, vol. 2018, pp. 263–268
Lee, A.R., Son, S.-M., Kim, K.K.: Information and communication technology overload and social networking service fatigue: a stress perspective. Comput. Hum. Behav. 55 , 51–61 (2016)
Foss, K., Foss, S., Griffin, C.: Feminist rhetorical theories (1999). https://doi.org/10.1080/07491409.2000.10162571
Coombs, W., Holladay, S.J.: Reasoned action in crisis communication: an attribution theory-based approach to crisis management. Responding to Cris. A Rhetor. approach to Cris. Commun. (2004)
Smith, H.J., Dinev, T., Xu, H.: Information privacy research: an interdisciplinary review. MIS Q. 35 , 989–1015 (2011)
Ammu, C.: Kerala: Kannur district teaches school kids to spot fake news—the week. theweek.in (2018)
Ponniah, K.: WhatsApp: the ‘black hole’ of fake news in India’s election. BBC News (2019)
Download references
This research did not receive any specific Grant from funding agencies in the public, commercial, or not-for-profit sectors.
Author information
Authors and affiliations.
Department of Management Studies (DoMS), Indian Institute of Technology Madras, Chennai, Tamil Nadu, 600036, India
Sadiq Muhammed T & Saji K. Mathew
You can also search for this author in PubMed Google Scholar
Contributions
TMS: Conceptualization, Methodology, Investigation, Writing—Original Draft, SKM: Writing—Review & Editing, Supervision.
Corresponding author
Correspondence to Sadiq Muhammed T .
Ethics declarations
Conflict of interest.
On behalf of two authors, the corresponding author states that there is no conflict of interest in this research paper.
Additional information
Publisher's note.
Springer Nature remains neutral with regard to jurisdictional claims in published maps and institutional affiliations.
Code | Sub themes | Frequency | Themes |
---|---|---|---|
Social crisis situations | Situations | 43 | Disaster |
Uncertain situations | |||
Real community crisis situations | |||
Post-disaster situation | |||
Crisis situations | |||
Ambiguous situations | |||
Unpredictable crisis situations | |||
Uncertain crisis situations | |||
Emergency situations | |||
Disaster situations | |||
Emergency crisis communication | Crisis | 36 | |
Unexpected crisis events | |||
Crisis scenario | |||
Crisis management | |||
Addressing health misinformation dissemination | Health | 77 | Health |
Global health misinformation | |||
Online health misinformation | |||
Health communication | |||
Public health | |||
Health pandemic | |||
Health-related conspiracy theories | Conspiracy | 33 | |
Anti-government rumors | Rumor | 44 | Politics |
Political headlines | Headlines | 30 | |
Political situations | Situations | 25 | |
National threat situations | |||
Homeland threat situations | |||
Military conflict situations |
Rights and permissions
Reprints and permissions
About this article
Muhammed T, S., Mathew, S.K. The disaster of misinformation: a review of research in social media. Int J Data Sci Anal 13 , 271–285 (2022). https://doi.org/10.1007/s41060-022-00311-6
Download citation
Received : 31 May 2021
Accepted : 06 January 2022
Published : 15 February 2022
Issue Date : May 2022
DOI : https://doi.org/10.1007/s41060-022-00311-6
Share this article
Anyone you share the following link with will be able to read this content:
Sorry, a shareable link is not currently available for this article.
Provided by the Springer Nature SharedIt content-sharing initiative
- Misinformation
- Information disorder
- Social media
- Systematic literature review
- Find a journal
- Publish with us
- Track your research

Misinformation and disinformation

Misinformation is false or inaccurate information—getting the facts wrong. Disinformation is false information which is deliberately intended to mislead—intentionally misstating the facts.
The spread of misinformation and disinformation has affected our ability to improve public health, address climate change, maintain a stable democracy, and more. By providing valuable insight into how and why we are likely to believe misinformation and disinformation, psychological science can inform how we protect ourselves against its ill effects.
APA resolution

Combating misinformation and promoting psychological science literacy
Approved by APA Council of Representatives, February 2024

Using psychological science to understand and fight health misinformation
This report describes the best available psychological science on misinformation, particularly as it relates to health.
It offers eight specific recommendations to help scientists, policymakers, and health professionals respond to the ongoing threats posed by misinformation.

Is it safe to get health advice from influencers?

Eight specific ways to combat misinformation

Factors that make people believe misinformation

How and why does misinformation spread?
Magination Press children’s book

True or False? The Science of Perception, Misinformation, and Disinformation
Written for preteens and young teens in lively text accompanied by fun facts, this book explores what psychology tells us about development and persistence of false perceptions and beliefs and the difficulty of correcting them, plus ways to debunk misinformation and think critically and factually about the world around us.
Advice to stem misinformation

What employers can do to counter election misinformation in the workplace

Using psychological science to fight misinformation: A guide for journalists
More from APA

Psychology is leading the way on fighting misinformation

This election year, fighting misinformation is messier and more important than ever

Stopping the spread of misinformation

The anatomy of a misinformation attack
Webinars and presentations
Tackling Misinformation Ahead of Election Day
APA and the Civic Alliance collaborated to address the impact of mis- and disinformation on our democracy. APA experts discussed the psychology behind how mis- and disinformation occurs, and why we should care.
Building Back Trust in Science: Community-Centered Solutions
APA collaborated with American Public Health Association, National League of Cities, and Research!America to host a virtual national conversation about the psychology and impact of misinformation on public health.
Fighting Misinformation With Psychological Science
Psychological science is playing a key role in the global cooperative effort to combat misinformation and change the course on how we’re tackling critical societal issues.
Studying misinformation
Explore the latest psychological research on misinformation and disinformation
How long does gamified psychological inoculation protect people against misinformation?
Perceptions of fake news, misinformation, and disinformation amid the COVID-19 pandemic: A qualitative exploration
Quantifying the effects of fake news on behavior: Evidence from a study of COVID-19 misinformation
Countering misinformation and fake news through inoculation and prebunking
Who is susceptible to online health misinformation? A test of four psychosocial hypotheses
It might become true: How prefactual thinking licenses dishonesty
Federal resources
- Centers for Disease Control and Prevention How to address Covid -19 vaccine misinformation
- U.S. Surgeon General Health misinformation
Resources from other organizations
- AARP Teaching students how to spot misinformation
- American Public Health Association Podcast series: Confronting our disease of disinformation Part 1 | Part 2 | Part 3 | Part 4
- News Literacy Project Webinar: Your brain and misinformation: Why people believe lies and conspiracy theories
- NPC Journalism Institute Webinar: Disinformation, midterms & the mind: How psychology can help journalists fight misinformation
To find a researcher studying misinformation and disinformation, please contact our press office .
Thank you for visiting nature.com. You are using a browser version with limited support for CSS. To obtain the best experience, we recommend you use a more up to date browser (or turn off compatibility mode in Internet Explorer). In the meantime, to ensure continued support, we are displaying the site without styles and JavaScript.
- View all journals
- Explore content
- About the journal
- Publish with us
- Sign up for alerts
- Published: 05 June 2024
Post-January 6th deplatforming reduced the reach of misinformation on Twitter
- Stefan D. McCabe ORCID: orcid.org/0000-0002-7180-145X 1 na1 ,
- Diogo Ferrari ORCID: orcid.org/0000-0003-2454-0776 2 na1 ,
- Jon Green 3 ,
- David M. J. Lazer ORCID: orcid.org/0000-0002-7991-9110 4 , 5 &
- Kevin M. Esterling ORCID: orcid.org/0000-0002-5529-6422 2 , 6
Nature volume 630 , pages 132–140 ( 2024 ) Cite this article
1754 Accesses
193 Altmetric
Metrics details
The social media platforms of the twenty-first century have an enormous role in regulating speech in the USA and worldwide 1 . However, there has been little research on platform-wide interventions on speech 2 , 3 . Here we evaluate the effect of the decision by Twitter to suddenly deplatform 70,000 misinformation traffickers in response to the violence at the US Capitol on 6 January 2021 (a series of events commonly known as and referred to here as ‘January 6th’). Using a panel of more than 500,000 active Twitter users 4 , 5 and natural experimental designs 6 , 7 , we evaluate the effects of this intervention on the circulation of misinformation on Twitter. We show that the intervention reduced circulation of misinformation by the deplatformed users as well as by those who followed the deplatformed users, though we cannot identify the magnitude of the causal estimates owing to the co-occurrence of the deplatforming intervention with the events surrounding January 6th. We also find that many of the misinformation traffickers who were not deplatformed left Twitter following the intervention. The results inform the historical record surrounding the insurrection, a momentous event in US history, and indicate the capacity of social media platforms to control the circulation of misinformation, and more generally to regulate public discourse.
This is a preview of subscription content, access via your institution
Access options
Access Nature and 54 other Nature Portfolio journals
Get Nature+, our best-value online-access subscription
$29.99 / 30 days
cancel any time
Subscribe to this journal
Receive 51 print issues and online access
$199.00 per year
only $3.90 per issue
Buy this article
- Purchase on Springer Link
- Instant access to full article PDF
Prices may be subject to local taxes which are calculated during checkout
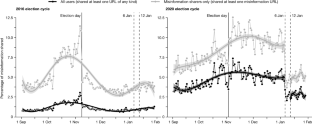
Similar content being viewed by others
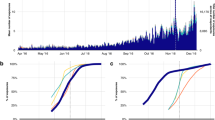
Exposure to the Russian Internet Research Agency foreign influence campaign on Twitter in the 2016 US election and its relationship to attitudes and voting behavior
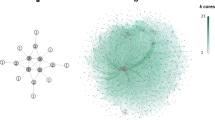
Measuring the scope of pro-Kremlin disinformation on Twitter
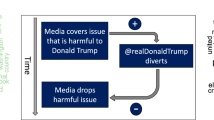
Using the president’s tweets to understand political diversion in the age of social media
Data availability.
Aggregate data used in the analysis are publicly available at the OSF project website ( https://doi.org/10.17605/OSF.IO/KU8Z4 ) to any researcher for purposes of reproducing or extending the analysis. The tweet-level data and specific user demographics cannot be publicly shared owing to privacy concerns arising from matching data to administrative records, data use agreements and platforms’ terms of service. Our replication materials include the code used to produce the aggregate data from the tweet-level data, and the tweet-level data can be accessed after signing a data-use agreement. For access requests, please contact D.M.J.L.
Code availability
All code necessary for reproduction of the results is available at the OSF project site https://doi.org/10.17605/OSF.IO/KU8Z4 .
Lazer, D. The rise of the social algorithm. Science 348 , 1090–1091 (2015).
Article ADS MathSciNet CAS PubMed Google Scholar
Jhaver, S., Boylston, C., Yang, D. & Bruckman, A. Evaluating the effectiveness of deplatforming as a moderation strategy on Twitter. Proc. ACM Hum.-Comput. Interact. 5 , 381 (2021).
Article Google Scholar
Broniatowski, D. A., Simons, J. R., Gu, J., Jamison, A. M. & Abroms, L. C. The efficacy of Facebook’s vaccine misinformation policies and architecture during the COVID-19 pandemic. Sci. Adv. 9 , eadh2132 (2023).
Article PubMed PubMed Central Google Scholar
Hughes, A. G. et al. Using administrative records and survey data to construct samples of tweeters and tweets. Public Opin. Q. 85 , 323–346 (2021).
Shugars, S. et al. Pandemics, protests, and publics: demographic activity and engagement on Twitter in 2020. J. Quant. Descr. Digit. Media https://doi.org/10.51685/jqd.2021.002 (2021).
Imbens, G. W., & Lemieux, T. Regression discontinuity designs: a guide to practice. J. Econom. 142 , 615–635 (2008).
Article MathSciNet Google Scholar
Gerber, A. S. & Green, D. P. Field Experiments: Design, Analysis, and Interpretation (W.W. Norton, 2012).
Grinberg, N., Joseph, K., Friedland, L., Swire-Thompson, B. & Lazer, D. Fake news on Twitter during the 2016 U.S. presidential election. Science 363 , 374–378 (2019).
Article ADS CAS PubMed Google Scholar
Munger, K. & Phillips, J. Right-wing YouTube: a supply and demand perspective. Int. J. Press Polit. 27 , 186–219 (2022).
Guess, et al. How do social media feed algorithms affect attitudes and behavior in an election campaign? Science 381 , 398–404 (2023).
Persily, N. in New Technologies of Communication and the First Amendment: The Internet, Social Media and Censorship (ed. Bollinger L. C. & Stone, G. R.) (Oxford Univ. Press, 2022).
Sevanian, A. M. Section 230 of the Communications Decency Act: a ‘good Samaritan’ law without the requirement of acting as a ‘good Samaritan’. UCLA Ent. L. Rev. https://doi.org/10.5070/LR8211027178 (2014).
Lazer, D. M. J. et al. The science of fake news. Science 359 , 1094–1096 (2018).
Suzor, N. Digital constitutionalism: using the rule of law to evaluate the legitimacy of governance by platforms. Soc. Media Soc. 4 , 2056305118787812 (2018).
Google Scholar
Napoli, P. M. Social Media and the Public Interest (Columbia Univ. Press, 2019).
DeNardis, L. & Hackl, A. M. Internet governance by social media platforms. Telecomm. Policy 39 , 761–770 (2015).
TwitterSafety. An update following the riots in Washington, DC. Twitter https://blog.x.com/en_us/topics/company/2021/protecting--the-conversation-following-the-riots-in-washington-- (2021).
Twitter. Civic Integrity Policy. Twitter https://help.twitter.com/en/rules-and-policies/election-integrity-policy (2021).
Promoting safety and expression. Facebook https://about.facebook.com/actions/promoting-safety-and-expression/ (2021).
Dwoskin, E. Trump is suspended from Facebook for 2 years and can’t return until ‘risk to public safety is receded’. The Washington Post https://www.washingtonpost.com/technology/2021/06/03/trump-facebook-oversight-board/ (4 June 2021).
Huszár, F. et al. Algorithmic amplification of politics on Twitter. Proc. Natl Acad. Sci. USA 119 , e2025334119 (2021).
Article PubMed Central Google Scholar
Guess, A. M., Nyhan, B. & Reifler, J. Exposure to untrustworthy websites in the 2016 US election. Nat. Hum. Behav. 4 , 472–480 (2020).
Sunstein, C. R. #Republic: Divided Democracy in the Age of Social Media (Princeton Univ. Press, 2017).
Timberg, C., Dwoskin, E. & Albergotti, R. Inside Facebook, Jan. 6 violence fueled anger, regret over missed warning signs. The Washington Post https://www.washingtonpost.com/technology/2021/10/22/jan-6-capitol-riot-facebook/ (22 October 2021).
Chandrasekharan, E. et al. You can’t stay here: the efficacy of Reddit’s 2015 ban examined through hate speech. Proc. ACM Hum. Comput. Interact. 1 , 31 (2017).
Matias, J. N. Preventing harassment and increasing group participation through social norms in 2,190 online science discussions. Proc. Natl Acad. Sci. USA 116 , 9785–9789 (2019).
Article ADS CAS PubMed PubMed Central Google Scholar
Yildirim, M. M., Nagler, J., Bonneau, R. & Tucker, J. A. Short of suspension: how suspension warnings can reduce hate speech on Twitter. Perspect. Politics 21 , 651–663 (2023).
Guess, A. M. et al. Reshares on social media amplify political news but do not detectably affect beliefs or opinions. Science 381 , 404–408 (2023).
Nyhan, B. et al. Like-minded sources on Facebook are prevalent but not polarizing. Nature 620 , 137–144 (2023).
Dang, S. Elon Musk’s X restructuring curtails disinformation research, spurs legal fears. Reuters https://www.reuters.com/technology/elon-musks-x-restructuring-curtails-disinformation-research-spurs-legal-fears-2023-11-06/ (6 November 2023).
Duffy, C. For misinformation peddlers on social media, it’s three strikes and you’re out. Or five. Maybe more. CNN Business https://edition.cnn.com/2021/09/01/tech/social-media-misinformation-strike-policies/index.html (1 September 2021).
Conger, K. Twitter removes Chinese disinformation campaign. The New York Times https://www.nytimes.com/2020/06/11/technology/twitter-chinese-misinformation.html (11 June 2020).
Timberg, C. & Mahtani, S. Facebook bans Myanmar’s military, citing threat of new violence after Feb. 1 coup. The Washington Post https://www.washingtonpost.com/technology/2021/02/24/facebook-myanmar-coup-genocide/ (24 February 2021).
Barry, D. & Frenkel, S. ‘Be there. Will be wild!’: Trump all but circled the date. The New York Times https://www.nytimes.com/2021/01/06/us/politics/capitol-mob-trump-supporters.html (6 January 2021).
Timberg, C. Twitter ban reveals that tech companies held keys to Trump’s power all along. The Washington Post https://www.washingtonpost.com/technology/2021/01/14/trump-twitter-megaphone/ (14 January 2021).
Dwoskin, E. & Tiku, N. How Twitter, on the front lines of history, finally decided to ban Trump. The Washington Post https://www.washingtonpost.com/technology/2021/01/16/how-twitter-banned-trump/ (16 January 2021).
Harwell, D. New video undercuts claim Twitter censored pro-Trump views before Jan. 6. The Washington Post https://www.washingtonpost.com/technology/2023/06/23/new-twitter-video-jan6/ (23 June 2023).
Romm, T. & Dwoskin, E. Twitter purged more than 70,000 accounts affiliated with QAnon following Capitol riot. The Washington Post https://www.washingtonpost.com/technology/2021/01/11/trump-twitter-ban/ (11 January 2021).
Denham, H. These are the platforms that have banned Trump and his allies. The Washington Post https://www.washingtonpost.com/technology/2021/01/11/trump-banned-social-media/ (13 January 2021).
Graphika Team. DisQualified: network impact of Twitter’s latest QAnon enforcement. Graphika Blog https://graphika.com/posts/disqualified-network-impact-of-twitters-latest-qanon-enforcement/ (2021).
Dwoskin, E. & Timberg, C. Misinformation dropped dramatically the week after Twitter banned Trump and some allies. The Washington Post https://www.washingtonpost.com/technology/2021/01/16/misinformation-trump-twitter/ (16 January 2021).
Harwell, D. & Dawsey, J. Trump is sliding toward online irrelevance. His new blog isn’t helping. The Washington Post https://www.washingtonpost.com/technology/2021/05/21/trump-online-traffic-plunge/ (21 May 2021).
Olteanu, A., Castillo, C., Boy, J. & Varshney, K. The effect of extremist violence on hateful speech online. In Proc. 12th International AAAI Conference on Web and Social Media https://doi.org/10.1609/icwsm.v12i1.15040 (ICWSM, 2018).
Lin, H. et al. High level of correspondence across different news domain quality rating sets. PNAS Nexus 2 , gad286 (2023).
Abilov, A., Hua, Y., Matatov, H., Amir, O., & Naaman, M. VoterFraud2020: a multi-modal dataset of election fraud claims on Twitter.” Proc. Int. AAAI Conf. Weblogs Soc. Media 15 , 901–912 (2021).
Calonico, S., Cattaneo, M. D. & Titiunik, R. Robust nonparametric confidence intervals for regression-discontinuity designs. Econometrica 82 , 2295–2326 (2014).
Jackson, S., Gorman, B. & Nakatsuka, M. QAnon on Twitter: An Overview (Institute for Data, Democracy and Politics, George Washington Univ. 2021).
Shearer, E. & Mitchell, A. News use across social media platforms in 2020. Pew Research Center https://www.pewresearch.org/journalism/2021/01/12/news-use-across-social-media-platforms-in-2020/ (2021).
McGregor, S. C. Social media as public opinion: How journalists use social media to represent public opinion. Journalism 20 , 1070–1086 (2019).
Hammond-Errey, M. Elon Musk’s Twitter is becoming a sewer of disinformation. Foreign Policy https://foreignpolicy.com/2023/07/15/elon-musk-twitter-blue-checks-verification-disinformation-propaganda-russia-china-trust-safety/ (15 July 2023).
Joseph, K. et al. (Mis)alignment between stance expressed in social media data and public opinion surveys. Proc. 2021 Conference on Empirical Methods in Natural Language Processing 312–324 (Association for Computational Linguistics, 2021).
Robertson, R. E. et al. Auditing partisan audience bias within Google search. Proc. ACM Hum. Comput. Interact. 2 , 148 (2018).
McCrary, J. Manipulation of the running variable in the regression discontinuity design: a density. Test 142 , 698–714 (2008).
MathSciNet Google Scholar
Roth, J., Sant’Anna, P. H. C., Bilinski, A. & Poe, J. What’s trending in difference-in-differences? A synthesis of the recent econometrics literature. J. Econom. 235 , 2218–2244 (2023).
Wing, C., Simon, K. & Bello-Gomez, R. A. Designing difference in difference studies: best practices for public health policy research. Annu. Rev. Public Health 39 , 453–469 (2018).
Article PubMed Google Scholar
Baker, A. C., Larcker, D. F. & Wang, C. C. Y. How much should we trust staggered difference-in-differences estimates? J. Financ. Econ. 144 , 370–395 (2022).
Callaway, B. & Sant’Anna, P. H. C. Difference-in-differences with multiple time periods. J. Econom. 225 , 200–230 (2021).
R Core Team. R: A Language and Environment for Statistical Computing, v.4.3.1. https://www.R-project.org/ (2023).
rdrobust: Robust data-driven statistical inference in regression-discontinuity designs. https://cran.r-project.org/package=rdrobust (2023).
Calonico, S., Cattaneo, M. D. & Titiunik, R. Optimal data-driven regression discontinuity plots. J. Am. Stat. Assoc. 110 , 1753–1769 (2015).
Article MathSciNet CAS Google Scholar
Calonico, S., Cattaneo, M. D. & Farrell, M. H. On the effect of bias estimation on coverage accuracy in nonparametric inference. J. Am. Stat. Assoc. 113 , 767–779 (2018).
Zeileis, A. & Hothorn, T. Diagnostic checking in regression relationships. R News 2 , 7–10 (2002).
Cameron, A. C., Gelbach, J. B. & Miller, D. L. Robust inference with multiway clustering. J. Bus. Econ. Stat. 29 , 238–249 (2011).
Zeileis, A. Econometric computing with HC and HAC covariance matrix estimators. J. Stat. Softw . https://doi.org/10.18637/jss.v011.i10 (2004).
Eckles, D., Karrer, B. & Johan, U. Design and analysis of experiments in networks: reducing bias from interference. J. Causal Inference https://doi.org/10.1515/jci-2015-0021 (2016).
Download references
Acknowledgements
The authors thank N. Grinberg, L. Friedland and K. Joseph for earlier technical work on the development of the Twitter dataset. Earlier versions of this paper were presented at the Social Media Analysis Workshop, UC Riverside, 26 August 2022; at the Annual Meeting of the American Political Science Association, 17 September 2022; and at the Center for Social Media and Politics, NYU, 23 April 2021. Special thanks go to A. Guess for suggesting the DID analysis. D.M.J.L. acknowledges support from the William & Flora Hewlett Foundation and the Volkswagen Foundation. S.D.M. was supported by the John S. and James L. Knight Foundation through a grant to the Institute for Data, Democracy & Politics at the George Washington University.
Author information
These authors contributed equally: Stefan D. McCabe, Diogo Ferrari
Authors and Affiliations
Institute for Data, Democracy & Politics, George Washington University, Washington, DC, USA
Stefan D. McCabe
Department of Political Science, University of California, Riverside, Riverside, CA, USA
Diogo Ferrari & Kevin M. Esterling
Department of Political Science, Duke University, Durham, NC, USA
Network Science Institute, Northeastern University, Boston, MA, USA
David M. J. Lazer
Institute for Quantitative Social Science, Harvard University, Cambridge, MA, USA
School of Public Policy, University of California, Riverside, Riverside, CA, USA
Kevin M. Esterling
You can also search for this author in PubMed Google Scholar
Contributions
The order of author listed here does not indicate level of contribution. Conceptualization of theory and research design: S.D.M., D.M.J.L., D.F., K.M.E. and J.G. Data curation: S.D.M. and J.G. Methodology: D.F. Visualization: D.F. Funding acquisition: D.M.J.L. Project administration: K.M.E., S.D.M. and D.M.J.L. Writing, original draft: K.M.E. and D.M.J.L. Writing, review and editing: K.M.E., D.F., S.D.M., D.M.J.L. and J.G.
Corresponding author
Correspondence to David M. J. Lazer .
Ethics declarations
Competing interests.
The authors declare no competing interests.
Peer review
Peer review information.
Nature thanks Jason Reifler and the other, anonymous, reviewer(s) for their contribution to the peer review of this work. Peer review reports are available.
Additional information
Publisher’s note Springer Nature remains neutral with regard to jurisdictional claims in published maps and institutional affiliations.
Extended data figures and tables
Extended data fig. 1 replication of the did results varying the number of deplatformed accounts..
DID estimates where the intervention depends on the number of deplatformed users that were followed by the not-deplatformed misinformation sharers. Results are two-way fixed effect point estimates (dots) and 95% confidence intervals (bars) of the difference-in-differences for all activity levels combined. Estimates use ordinary least squares with clustered standard errors at user-level. The Figure shows results including and excluding Trump followers (color code). The x-axis shows the minimum number of deplatformed accounts the user followed from at least one (1+) to at least ten (10+). Total sample sizes for each dosage level: Follow Trump (No): 1: 625,865; 2: 538,460; 3: 495,723; 4: 470,380; 5: 451,468; 6: 437,574; 7: 426,772; 8: 417,200; 9: 408,672; 10: 401,467; Follow Trump (Yes): 1: 688,174; 2: 570,637; 3: 514,352; 4: 481,684; 5: 460,676; 6: 444,656; 7: 432,659; 8: 421,924; 9: 413,241; 10: 405,766.
Extended Data Fig. 2 SRD results for total (bottom row) and average (top row) misinformation tweets and retweets, for deplatformed and not-deplatformed users.
Sample size includes 546 observations (days) on average across groups (x-axis), 404 before and 136 after. The effective number of observations is 64.31 days before and after on average. The estimation excludes data between Jan 6 (cutoff point) and 12 (included). January 6th is the score value 0, and January 12th the score value 1. Optimal bandwidth of 32.6 days with triangular kernel and order-one polynomial. Bars indicate 95% robust bias-corrected confidence intervals.
Extended Data Fig. 3 Time series of the daily mean of non-misinformation URL sharing.
Degree five polynomial regression (fitted line) before and after the deplatforming intervention, separated by subgroup (panel rows), for liberal-slant news (right column), and conservative-slant news (left column) sharing activity. Shaded area around the fitted line is the 95% confidence interval of the fitted values. As a placebo test we evaluate the effect of the intervention on sharing non-fake news for each of our subgroups. Since sharing non-misinformation does not violate Twitter’s Civic Integrity policy – irrespective of the ideological slant of the news – we do not expect the intervention to have an impact on this form of Twitter engagement; see SI for how we identify liberal and conservative slant of these domains from ref. 52 . Among the subgroups, users typically did not change their sharing of liberal or conservative non-fake news. Taking these results alongside those in Fig. 2 implies that these subgroups of users did not substitute non-misinformation conservative news sharing during and after the insurrection in place of misinformation.
Extended Data Fig. 4 Time series of misinformation tweets and retweets (panel columns), separately for high, medium and low activity users (panel rows).
Fitted straight lines describe a linear regression fitted using ordinary least squares of daily total misinformation retweeted standardized (y-axis) on days (x-axis) before January 6th and after January 12th. Shaded areas around the fitted line are 95% confidence intervals.
Extended Data Fig. 5 Replicates Fig. 5 but with adjustment covariates.
Corresponding regression tables are Supplementary Information Tables 1 to 3 . Two-way fixed effect point estimates (dots) and 95% confidence intervals (bars) of the difference-in-differences for high, moderate, and low activity users, as well as all these levels combined (x-axis). P-values (stars) are from two-sided t-tests based on ordinary least squares estimates with clustered standard errors at user-level. Estimates compare followers (treated group) and not-followers (reference group) of deplatformed users after January 12th (post-treatment period) and before January 6th (pre-treatment period). No multiple test correction was used. See Supplementary Information Tables 1 – 3 for exact values with all activity level users combined. Total sample sizes of not-followers (reference) and Trump-only followers: combined: 306,089, high: 53,962, moderate: 219,375, low: 32,003; Followers: combined: 662,216, high: 156,941, moderate: 449,560, low: 53,442; Followers (4+): combined: 463,176, high: 115,264, moderate: 302,907, low: 43,218.
Extended Data Fig. 6 Placebo test of SRD results for total (bottom row) and average (top row) shopping and sports tweets and retweets at the deplatforming intervention, among those not deplatformed.
Sample size includes 545 observations (days), 404 before the intervention and 141 after. Optimal bandwidth of 843.6 days with triangular kernel and order-one polynomial. Cutoff points on January 6th (score 0) and January 12th (score 1). Bars indicate 95% robust bias-corrected confidence intervals. These are placebo tests since tweets about sports and shoppings should not be affected by the insurrection or deplatforming.
Extended Data Fig. 7 Placebo test of SRD results for total (bottom row) and average (top row) misinformation tweets and retweets using December 20th as an arbitrary cutoff point.
Sample size includes 551 observations (days), 387 before the intervention and 164 after. Optimal bandwidth of 37.2 days with triangular kernel and order-one polynomial. Bars indicate 95% robust bias-corrected confidence intervals about the SRD coefficients. This is a placebo test of the intervention period.
Extended Data Fig. 8 Placebo test of SRD results for total (bottom row) and average (top row) misinformation tweets and retweets using January 18th as a cutoff point.
The parameters are very similar to Extended Data Fig. 7 .
Supplementary information
Supplementary information.
Supplementary Figs. 1–5 provide descriptive information about our subgroups, a replication of the panel data using the Decahose, and robustness analyses for the SRD. Supplementary Tables 1–5 show full parameter estimates for the DID models, summary statistics for follower type and activity level, and P values for the DID analyses under different multiple comparisons corrections.
Reporting Summary
Peer review file, rights and permissions.
Springer Nature or its licensor (e.g. a society or other partner) holds exclusive rights to this article under a publishing agreement with the author(s) or other rightsholder(s); author self-archiving of the accepted manuscript version of this article is solely governed by the terms of such publishing agreement and applicable law.
Reprints and permissions
About this article
Cite this article.
McCabe, S.D., Ferrari, D., Green, J. et al. Post-January 6th deplatforming reduced the reach of misinformation on Twitter. Nature 630 , 132–140 (2024). https://doi.org/10.1038/s41586-024-07524-8
Download citation
Received : 27 October 2023
Accepted : 06 May 2024
Published : 05 June 2024
Issue Date : 06 June 2024
DOI : https://doi.org/10.1038/s41586-024-07524-8
Share this article
Anyone you share the following link with will be able to read this content:
Sorry, a shareable link is not currently available for this article.
Provided by the Springer Nature SharedIt content-sharing initiative
By submitting a comment you agree to abide by our Terms and Community Guidelines . If you find something abusive or that does not comply with our terms or guidelines please flag it as inappropriate.
Quick links
- Explore articles by subject
- Guide to authors
- Editorial policies
Sign up for the Nature Briefing newsletter — what matters in science, free to your inbox daily.

Reexamining Misinformation: How Unflagged, Factual Content Drives Vaccine Hesitancy
Research from the Computational Social Science Lab finds that factual, vaccine-skeptical content on Facebook has a greater overall effect than “fake news,” discouraging millions from the COVID-19 shot.
By Ian Scheffler, Penn Engineering

What threatens public health more, a deliberately false Facebook post about tracking microchips in the COVID-19 vaccine that is flagged as misinformation, or an unflagged, factual article about the rare case of a young, healthy person who died after receiving the vaccine?
According to Duncan J. Watts, Stevens University Professor in Computer and Information Science at Penn Engineering and Director of the Computational Social Science (CSS) Lab , along with David G. Rand, Erwin H. Schell Professor at MIT Sloan School of Management, and Jennifer Allen, 2024 MIT Sloan School of Management Ph.D. graduate and incoming CSS postdoctoral fellow, the latter is much more damaging. “The misinformation flagged by fact-checkers was 46 times less impactful than the unflagged content that nonetheless encouraged vaccine skepticism,” they conclude in a new paper in Science.
Historically, research on “fake news” has focused almost exclusively on deliberately false or misleading content, on the theory that such content is much more likely to shape human behavior. But, as Allen points out, “When you actually look at the stories people encounter in their day-to-day information diets, fake news is a miniscule percentage. What people are seeing is either no news at all or mainstream media.”
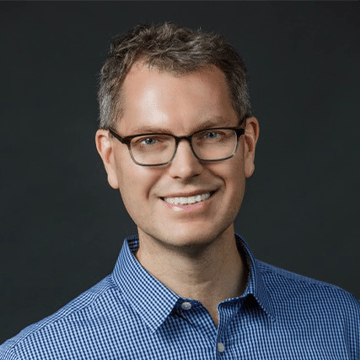
“Since the 2016 U.S. presidential election, many thousands of papers have been published about the dangers of false information propagating on social media,” says Watts. “But what this literature has almost universally overlooked is the related danger of information that is merely biased. That’s what we look at here in the context of COVID vaccines.”
In the study, Watts, one of the paper’s senior authors, and Allen, the paper’s first author, used thousands of survey results and AI to estimate the impact of more than 13,000 individual Facebook posts. “Our methodology allows us to estimate the effect of each piece of content on Facebook,” says Allen. “What makes our paper really unique is that it allows us to break open Facebook and actually understand what types of content are driving misinformed-ness.”
One of the paper’s key findings is that “fake news,” or articles flagged as misinformation by professional fact-checkers, has a much smaller overall effect on vaccine hesitancy than unflagged stories that the researchers describe as “vaccine-skeptical,” many of which focus on statistical anomalies that suggest that COVID-19 vaccines are dangerous.
“Obviously, people are misinformed,” says Allen, pointing to the low vaccination rates among U.S. adults, in particular for the COVID-19 booster vaccine, “but it doesn’t seem like fake news is doing it.” One of the most viewed URLs on Facebook during the time period covered by the study, at the height of the pandemic, for instance, was a true story in a reputable newspaper about a doctor who happened to die shortly after receiving the COVID-19 vaccine.
That story racked up tens of millions of views on the platform, multiples of the combined number of views of all COVID-19-related URLs that Facebook flagged as misinformation during the time period covered by the study. “Vaccine-skeptical content that’s not being flagged by Facebook is potentially lowering users’ intentions to get vaccinated by 2.3 percentage points,” Allen says. “A back-of-the-envelope estimate suggests that translates to approximately 3 million people who might have gotten vaccinated had they not seen this content.”
Despite the fact that, in the survey results, fake news identified by fact-checkers proved more persuasive on an individual basis, so many more users were exposed to the factual, vaccine-skeptical articles with clickbait-style headlines that the overall impact of the latter outstripped that of the former.
“Even though misinformation, when people see it, can be more persuasive than factual content in the context of vaccine hesitancy,” says Allen, “it is seen so little that these accurate, ‘vaccine-skeptical’ stories dwarf the impact of outright false claims.”
As the researchers point out, being able to quantify the impact of misleading but factual stories points to a fundamental tension between free expression and combating misinformation, as Facebook would be unlikely to shut down mainstream publications. “Deciding how to weigh these competing values is an extremely challenging normative question with no straightforward solution,” the authors write in the paper.
Allen points to content moderation that involves the user community as one possible means to address this challenge. “Crowdsourcing fact-checking and moderation works surprisingly well,” she says. “That’s a potential, more democratic solution.”
With the 2024 U.S. Presidential election on the horizon, Allen emphasizes the need for Americans to seriously consider these tradeoffs. “The most popular story on Facebook in the lead-up to the 2020 election was about military ballots found in the trash that were mostly votes for Donald Trump,” she notes. “That was a real story, but the headline did not mention that there were nine votes total, seven of them for Trump.”
This study was conducted at the University of Pennsylvania’s School of Engineering and Applied Science, the Annenberg School for Communication and the Wharton School, along with the Massachusetts Institute of Technology Sloan School of Management, and was supported by funding from Alain Rossmann.
This article originally appeared on the Penn Engineering Blog.
- Public Health
- Social Media
Research Areas
- Health Communication
- Science Communication
Related News
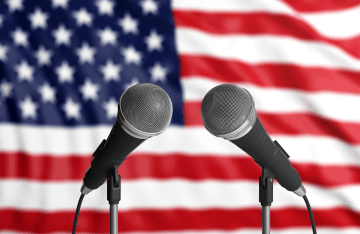
Recommendations of APPC Working Group May Be Implemented in 2024 Presidential Debates
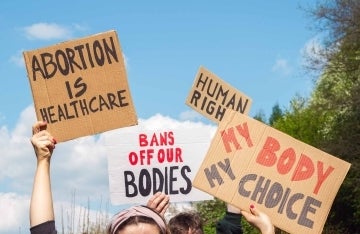
Abortion, Not Inflation, Directly Affected Congressional Voting in 2022

No Vacations, No Sleep, but Good Journalism: What It’s Like To Start a Nonprofit Newsroom

Debunking Viral Claims
FactCheck.org is one of several organizations working with Facebook to debunk misinformation shared on the social media network. We provide several resources for readers: a guide on how to flag suspicious stories on Facebook and a list of websites that have carried false or satirical articles, as well as a video and story on how to spot false stories.
Meme Spreads Unsupported Claim About Net Worth of Alvin Bragg
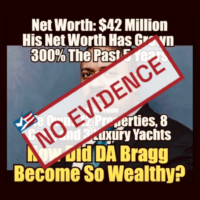
Manhattan District Attorney Alvin Bragg announced the conviction of former President Donald Trump on 34 felony counts of falsifying business records on May 30. Now Bragg has become the target of viral social media posts that claim, without evidence, that he has a net worth of $42 million or more and baselessly imply that Bragg is corrupt.
Antarctic Ice Loss Is Significant, Contrary to Claims
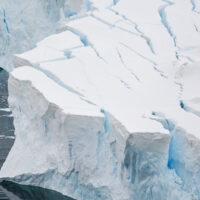
Antarctica is losing ice mass to the ocean, contributing to global sea level rise. But a popular video misrepresented work focused on Antarctic ice shelves — which float in the sea at the edges of the continent — to incorrectly suggest that “it is unclear if Antarctica is losing any ice on balance.”
Gaza Tunnel Photo Mislabeled on Social Media
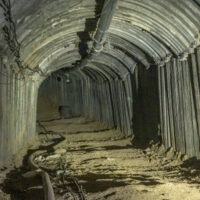
A photo taken in January shows a large tunnel under Gaza’s northern border with Israel, reportedly used in the Oct. 7 attack by Hamas. But recent social media posts falsely claim that the photo shows a tunnel connecting Egypt with the southern Gaza city of Rafah — where Palestinians displaced by the Israel-Hamas war have been sheltering.
Exaggerated Claims Circulate About Judge Merchan’s Family
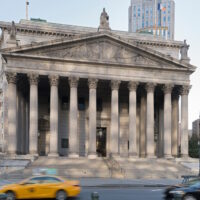
Social media posts seeking to discredit the judge who presided over former President Donald Trump’s criminal case in New York have been circulating online. Contrary to a popular meme, the judge’s wife works for a Republican district attorney, not the Democratic state attorney general, and his daughter was not personally paid by a high-profile Democrat.
Posts Misleadingly Link Town Clerk’s Case to 2020 Presidential Election
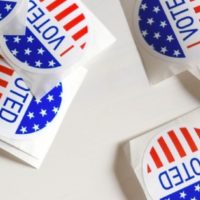
A Michigan town clerk pleaded no contest in 2023 to a charge of misconduct in office. Social media posts misleadingly highlight her case to push the false narrative that the 2020 presidential election was “rigged.” The clerk’s case was related to her local primary race, not the presidential election.
Trump, Allies Misrepresent FBI Order on Document Search at Mar-a-Lago
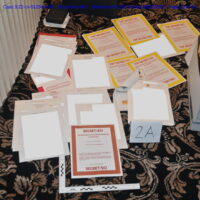
FBI agents who searched for classified documents held by former President Donald Trump at Mar-a-Lago in 2022 followed standard protocol. But Trump supporters and social media posts now falsely claim the raid was an “attempted assassination” of Trump. The claim is based on a misquote of FBI policy in a legal motion — and Trump wasn’t in Florida during the search.
Pearl Jam Singer’s Criticism of Harrison Butker Didn’t Affect Concert Schedule
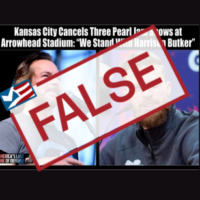
College commencement remarks by Kansas City Chiefs kicker Harrison Butker on the roles of women drew widespread criticism, including from Pearl Jam singer Eddie Vedder. Social media posts falsely claimed Arrowhead Stadium, where the Chiefs play, then canceled concerts by the band. A team spokesperson said Pearl Jam was “never scheduled to perform” at the venue.
Partisans Distort Proposed MOMS Act and Website for Pregnancy Resources
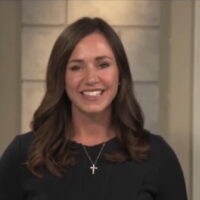
Republican Sen. Katie Britt has introduced a bill that would create a government website to help connect pregnant people with resources, excluding abortion services. Some Democrats and partisan websites have misleadingly claimed the proposed law would create a federal database of pregnant people. The bill doesn’t require users to provide any personal information.
Social Media Posts Circulate Altered Image of Donald Trump, Stormy Daniels
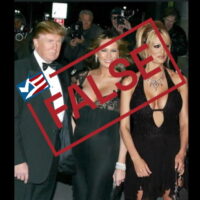
Adult film star Stormy Daniels recently testified at the criminal trial of former President Donald Trump, who is charged with falsifying records during his 2016 campaign to conceal an affair with Daniels. Social media posts falsely claim to show evidence of the affair by sharing a fake, digitally altered photo of Donald and Melania Trump with Daniels.
Posts Misrepresent Unfreezing of $16 Billion in Iranian Funds
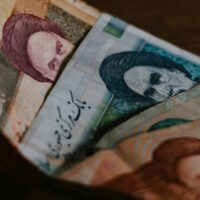
A recent deal involving a prisoner swap and the extension of a Trump-era waiver have freed $16 billion in previously frozen Iranian funds. Social media posts distort the sources of the money to falsely claim “Joe Biden gave 16 billion to Iran.” The Iranian money has been unfrozen with restrictions that it be used for humanitarian purposes.
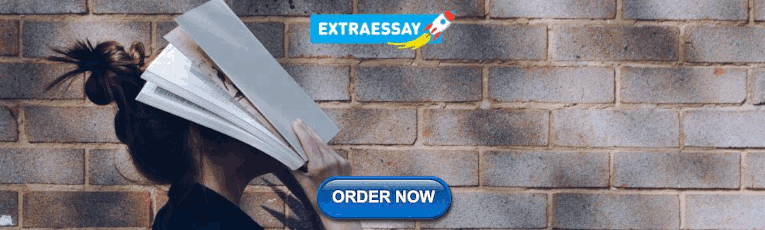
IMAGES
COMMENTS
Misinformation in Social Media. The rapid rise of social media has transformed the way information is disseminated and consumed, but it has also given birth to a growing concern - the proliferation of misinformation. Misinformation, defined as false or misleading information spread unintentionally, has become a pervasive issue in the digital age.
Social media outperformed television as the major news source for young people of the UK and the USA. 10 Moreover, as it is easier to generate and disseminate news online than with traditional media or face to face, large volumes of fake news are produced online for many reasons (Shu et al. 2017).Furthermore, it has been reported in a previous study about the spread of online news on Twitter ...
Social media is commonly assumed to be culpable for this growth, with 'the news' and current affairs deemed the epicentre of the battle for information credibility. This review begins by explaining the key definitions and discussions of the subject of fake news, and online misinformation and disinformation with the aid of each book in turn.
As widespread as misinformation online is, opportunities to glimpse it in action are fairly rare. Yet shortly after the recent attack in Toronto, a journalist unwittingly carried out a kind of ...
The following essay is reprinted with permission from The Conversation, an online publication covering the latest research.. Social media are among the primary sources of news in the U.S. and ...
The spread of misinformation in social media has become a severe threat to public interests. ... French election of 2017 and Indian elections in 2019 have been reported as examples where misinformation has influenced ... We might not have identified all relevant papers on spread of misinformation on social media from existing literature as some ...
Online social networks meet several of the criteria known by psychologists to make statements persuasive. For example, posts promoting unvetted claims can be endorsed and shared by friends and family. "Social media are practically built for spreading fake news," says Norbert Schwarz, PhD, a psychologist who studies misinformation.
Citing these absolute numbers may contribute to misunderstandings about how much of the content on social media is misinformation 59,60: for example, US citizens estimate that 65% of the news they ...
The hypotheses will be tested using a unique dataset that would include user consumption and production habits, as well as content exposure, and time spent on several social media platforms coupled with other information like an online survey and in-depth interviews of users who have been exposed to misinformation across social media platforms.
Summary: "The rise of fake news highlights the erosion of long-standing institutional bulwarks against misinformation in the internet age. Concern over the problem is global. However, much remains unknown regarding the vulnerabilities of individuals, institutions, and society to manipulations by malicious actors.
1. Introduction. Although the spread of misinformation is as old as human history, social media has changed the game by enabling people to generate misinformation easily and spread it rapidly in an anonymous and decentralized fashion (Del Vicario et al., 2016; Wu et al., 2016).The impact of misinformation can be destructive to various aspects of our lives, from public health and politics to ...
The widespread dissemination of misinformation in social media has recently received a lot of attention in academia. While the problem of misinformation in social media has been intensively studied, there are seemingly di erent def-initions for the same problem, and inconsistent results in di erent studies. In this survey, we aim to consolidate the
This essay argues that the recent scholarship on misinformation and fake news suffers from a lack of historical contextualization. The fact that misinformation scholarship has, by and large, failed to engage with the history of propaganda and with how propaganda has been studied by media and communication researchers is an empirical detriment to it, and
This study examines the prevalence and characteristics of synthetic media on social media platform X from December 2022 to September 2023. Read the Essay . ... While algorithms and crowdsourcing have been increasingly used to debunk or label misinformation on social media, such tasks might be most effective when performed by professional fact ...
For example, studies often test one-off exposures to a single message rather than persuasion as a function of repeated exposure to misinformation from diverse social and traditional media sources.
By Steven Lee Myers. Oct. 13, 2022. It is well known that social media amplifies misinformation and other harmful content. The Integrity Institute, an advocacy group, is now trying to measure ...
An example is when an old photo is used on a recent social media post. It might spread outrage or fear until the photo receives the right context. Fake context ... From social media to news, misinformation can spread all over the world in an instant. For children, misinformation and disinformation often looks very convincing. ...
Health misinformation was most prevalent in studies related to smoking products and drugs such as opioids and marijuana. Posts with misinformation reached 87% in some studies. Health misinformation about vaccines was also very common (43%), with the human papilloma virus vaccine being the most affected.
USC study reveals the key reason why fake news spreads on social media. The USC-led study of more than 2,400 Facebook users suggests that platforms — more than individual users — have a larger role to play in stopping the spread of misinformation online. January 17, 2023 By Pamela Madrid.
formation. In this essay, I will discuss the censorship on social media platforms related to COVID-19 and the problems it raises along with an alternative approach to counteract the spread of medical and scientific misinfor-mation. Censorship on social media platforms Censorship on major social media platforms, such as Facebook, Twitter and ...
The effects of social media on human life can be both: positive and negative. In only 3 hours we'll deliver a custom Misinformation in Social Media essay written 100% from scratch Learn more. On the one hand, people have a convenient way to communicate, which saves time significantly. Today, we can contact people worldwide and keep in touch ...
Main social media platforms have also actively fought against false information by filtering out or flagging content considered as misinformation. In this essay, I will discuss the censorship on social media platforms related to COVID‐19 and the problems it raises along with an alternative approach to counteract the spread of medical and ...
The spread of misinformation in social media has become a severe threat to public interests. For example, several incidents of public health concerns arose out of social media misinformation during the COVID-19 pandemic. Against the backdrop of the emerging IS research focus on social media and the impact of misinformation during recent events such as the COVID-19, Australian Bushfire, and the ...
Misinformation is false or inaccurate information—getting the facts wrong. Disinformation is false information which is deliberately intended to mislead—intentionally misstating the facts. The spread of misinformation and disinformation has affected our ability to improve public health, address climate change, maintain a stable democracy ...
The social media platforms of the twenty-first century have an enormous role in regulating speech in the USA and worldwide1. However, there has been little research on platform-wide interventions ...
"The misinformation flagged by fact-checkers was 46 times less impactful than the unflagged content that nonetheless encouraged vaccine skepticism," they conclude in a new paper in Science. ... many thousands of papers have been published about the dangers of false information propagating on social media," says Watts. "But what this ...
Allen et al. combine high-powered controlled experiments with machine learning and social media data on roughly 2.7 billion vaccine-related URL views on Facebook during the rollout of the first COVID-19 vaccine at the start of 2021.Contrary to claims that misinformation does not affect choices (), the causal estimates reported by Allen et al. suggest that exposure to a single piece of vaccine ...
May 24, 2024. A Michigan town clerk pleaded no contest in 2023 to a charge of misconduct in office. Social media posts misleadingly highlight her case to push the false narrative that the 2020 ...