Masks Strongly Recommended but Not Required in Maryland, Starting Immediately
Due to the downward trend in respiratory viruses in Maryland, masking is no longer required but remains strongly recommended in Johns Hopkins Medicine clinical locations in Maryland. Read more .
- Vaccines
- Masking Guidelines
- Visitor Guidelines
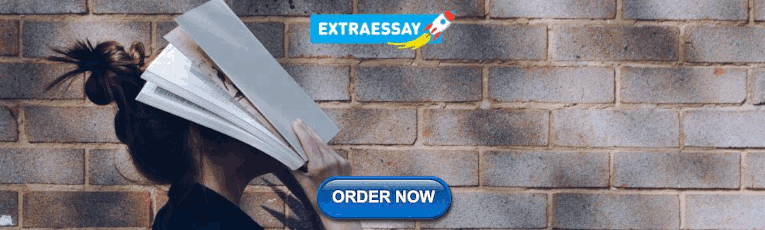
Institutional Review Board
Access to patient data for research: frequently asked questions.
September 2021
Introduction
Data Scientists and researchers from the Applied Physics Lab (APL), the Johns Hopkins Bloomberg School of Public Health (SPH), and other schools across Johns Hopkins University play an important role in many research projects involving Johns Hopkins Medicine (JHM) patient data. HIPAA and Common Rule regulations impact what data these Data Scientists and researchers can see and under what circumstances. This document answers questions related to collaboration with JHU researchers outside the JHM covered entity so that studies teams may structure their studies in a compliant manner.
What is the JHM Covered Entity?
A key concept in understanding healthcare regulations is that of the HIPAA covered entity, an entity that is “covered” by the HIPAA regulations. HIPAA regulations apply to health plans, health care providers that bill electronically for services, health care clearinghouses, Medicare Part D Pharmaceutical Providers, and Business Associates . See About HIPAA for information about the JHM covered entity. Note that the JHU Schools of Medicine (SOM) and Nursing (SON) are part of the JHM Covered Entity, while APL, SPH and the Homewood Schools (e.g. Whiting School of Engineering) are not.
Is APL able to access PHI in relation to the Precision Medicine infrastructure?
Due to its unique legal structure, a Business Associates Agreement (BAA) with APL allows APL workforce members to have access to Protected Health Information (PHI) to perform services on behalf of the JHM Covered Entity. Developing the Precision Medicine Analytics Platform (PMAP) infrastructure is an example of a service that is permitted under the BAA. Notably, conducting research is not considered a business service on behalf of the covered entity, and access to PHI for research purposes is not permitted by the BAA. The Institutional Review Board (IRB) must approve APL researcher access to JHM data for research purposes.
What is a Disclosure?
Any time an individual outside the Covered Entity accesses a patient’s facial identifiers (e.g. name, medical record number, address, etc. – see Definition of Limited data set to understand what identifiers must be removed for data to be considered a limited data set), it is considered a HIPAA disclosure. Therefore, sharing of PHI with facial identifiers with APL or SPH data scientists is considered a disclosure regardless of whether or not the data leaves the PMAP environment. Patients may authorize the sharing of their data with outside researchers in the consent and authorization that they sign to participate in a study. In the absence of a patient authorization, which is typically the case with large datasets, HIPAA mandates that disclosure of PHI for research purposes be the minimum necessary to conduct the research and it must be impractical to obtain patient authorization for the disclosure. The IRB requires a HIPAA Waiver of Authorization and reviews each data element shared with the outside organization to ensure there is a scientific justification for the disclosure. Such evaluations take time and may involve several questions from the IRB to the study team to establish whether sharing of direct identifiers is necessary to accomplish the research. In most cases such sharing is not required and the research may be accomplished by removing direct identifiers prior to sharing.
What data can JHU researchers outside the JHM covered entity access without patient authorization?
Research uses of data require IRB approval. In many cases, researchers outside the covered entity do not need access to direct identifiers included in the data; rather, they can use a subset of the data that consists of a limited dataset or a deidentified dataset for analysis. In the majority of cases, when data is shared outside of the covered entity without patient authorization, the IRB will require that the data to be shared be reduced to a limited data set in order to meet the minimum necessary standard.
HIPAA allows for the disclosure of limited datasets for IRB-approved research collaborations. To request a limited dataset for research, contact the CCDA.
Are JHU students able to access PHI for research purposes?
Research uses of data require IRB approval. Students from across JHU are able to access PHI for research purposes provided they complete Johns Hopkins HIPAA training courses and access data under the oversight of an SOM or SON faculty member serving as Principal Investigator (PI) of an IRB approved research protocol. Because the student is under the oversight of an employee within the JHM covered entity (the PI), they are considered a part of the JHM Covered Entity for HIPAA purposes. SOM and SON faculty providing oversight for student access to PHI take full responsibility for the student’s access and actions.
See Are faculty with Joint Appointments part of the JHM Covered Entity? for information regarding joint appointments). The IRB is responsible for approving study team members and their roles and will consider these factors. If the student has a role within the covered entity and a role outside the covered entity, the IRB will consider the role under which the student is participating in the research.
Are JHU faculty outside the JHM Covered Entity able to access PHI?
Faculty members outside the JHM Covered Entity are not under the oversight of a JHM Covered Entity employee and therefore require a HIPAA Waiver or signed HIPAA Authorization and approved IRB protocol to access PHI for research. Researchers should, to the maxim extent possible, design their studies to obtain prospective consent and authorization from research subjects for access to their PHI. In some cases where consent and authorization cannot be obtained prospectively, the IRB may determine that access to PHI by a JHU researcher outside the JHM covered entity is justifiable, in that the research cannot be practicably done without the access to PHI, and grant a HIPAA Waiver of Authorization.
In other cases, access to full PHI is not required for the researcher outside the JHM covered entity. The IRB may determine that work done by researchers outside the covered entity can be accomplished using a limited dataset. The protocol should explain the need for the limited data set as opposed to fully de-identified data.
How can JHU researchers outside the JHM covered entity participate in research projects using PMAP?
In cases where there is express patient authorization and consent, named members of the IRB research protocol may access a registry with PHI. In cases without patient authorization and consent, where the PMAP registry protocol has been approved by the IRB, a protocol for secondary data use may be submitted using an eformS ( see forms ) that establishes a projection of a subset of the PMAP registry, or even subsets of multiple registries, to answer specific research questions. The subset projected should be a limited dataset, which may include dates. APL and SPH data scientists and other researchers outside the JHM Covered Entity may be included as study team members on the protocol for secondary data use. JH Investigators outside the JHM covered entity receiving an LDS must include in their IRB application a data specification from the CCDA or a CCDA certified data manager that describes the data to be provisioned OR a document certifying the status of the dataset as a limited dataset provided by an individual certified in de-identification by the CCDA. If the team is a mix of JHM and JHU Non-JHM researchers, the specification should indicate that a limited data set is being shared with researchers outside the covered entity.
Do collaborations with researchers outside the JHM Covered Entity require Data Use Agreements?
A Data Use Agreement (DUA) establishes the terms under which data may be used by a third party collaborating on research involving patient data. The School of Medicine Office of Research Administration (ORA) negotiates and executes DUAs and other research agreements with data use terms for JHM PIs when research involves JHM patients or their data. A DUA or other agreement with terms for data use is required whenever JHM patient data is shared outside JH under a waiver of consent, even if the data is fully de-identified. JH Investigators outside the JHM covered entity receiving an LDS or full PHI pursuant to an IRB waiver of HIPAA authorization must complete the IRB requirements for study team members and agree to the Data Protection Attestation terms. APL Investigators receiving an LDS or full PHI require a DUA. The COEUS PD number for the DUA should be entered in section 36 of the IRB application. APL investigators receiving an LDS through PMAP may use the APL Master agreement; contact Suma Subbarao for more information.
Are faculty with Joint Appointments part of the JHM Covered Entity?
Faculty who have a joint appointment in the SOM or SON and who have clinical privileges are considered part of the JHM Covered Entity and do not need to request a DUA from ORA or JHURA to participate in research projects involving JHM data. The activity must be related to their SOM or SON role to be considered an activity within the JHM covered entity. The joint appointment does not need to be a dual primary appointment
Note that PHI used by such faculty must be stored and accessed in a HIPAA-compliant manner and described in the IRB-approved protocol. See Best Practices for Storage of Data for Research and Quality Improvement for details. Are there special considerations for PMAP Registries?
A Precision Medicine Center of Excellence (PMCOE) must submit an IRB application using an eForm R ( see forms ) for the creation of a PMAP registry supporting a PMCOE to the IRB. PMAP registries often have identifiers that enable joining of identified data across different datasets. The ability to join disparate datasets is one of the benefits of using the PMAP environment. Adding APL or SPH data scientists or other researchers outside the JHM Covered Entity to the protocol for the creation of a PMAP registry that includes such identifiers could constitute a disclosure and require the IRB to review the data elements shared to ensure that the minimum necessary for the research would be shared. For that reason, consider the following options.
- Leave faculty and staff outside the covered entity off the protocol for the creation of a registry that includes identifiers. These faculty and staff may offer guidance on the elements to be included in the registry, without looking at data from specific individuals.
- Bar access to PHI within the registry for study team members outside the covered entity. The PMAP team can separate out the PHI, making it accessible to a subset of the study team that excludes those outside the JHM covered entity.
- Create a secondary use protocol using an eform S that creates a derivative projection of the registry that is a limited dataset. Faculty and staff from outside the covered entity may be listed on the secondary use protocol and analyze the limited dataset. See How can JHU researchers outside the JHM covered entity participate in research projects using PMAP?
When is it appropriate for the HIPAA workforce member agreement to be used for personnel outside of the JHM covered entity who are working on a research registry?
The HIPAA workforce member agreement is appropriate when the personnel are performing a covered healthcare operations service for or on behalf of the covered entity. This would apply in cases where the personnel are designing broad, general infrastructure that might be used for many different research registries such as PMAP infrastructure.
When a person outside of the covered entity is performing registry specific work for a registry covered by an eForm R, a HIPAA workforce member agreement is not appropriate. Instead, the person should be listed on the protocol and the research team must seek a HIPAA waiver from the IRB or request access to a limited data set only and request a DUA if needed (see Do collaborations with researchers outside the JHM Covered Entity require Data Use Agreements?
- Research Article
- Open access
- Published: 07 May 2020
Generation and evaluation of synthetic patient data
- Andre Goncalves 1 ,
- Priyadip Ray 1 ,
- Braden Soper 1 ,
- Jennifer Stevens 2 ,
- Linda Coyle 2 &
- Ana Paula Sales 1
BMC Medical Research Methodology volume 20 , Article number: 108 ( 2020 ) Cite this article
29k Accesses
130 Citations
10 Altmetric
Metrics details
Machine learning (ML) has made a significant impact in medicine and cancer research; however, its impact in these areas has been undeniably slower and more limited than in other application domains. A major reason for this has been the lack of availability of patient data to the broader ML research community, in large part due to patient privacy protection concerns. High-quality, realistic, synthetic datasets can be leveraged to accelerate methodological developments in medicine. By and large, medical data is high dimensional and often categorical. These characteristics pose multiple modeling challenges.
In this paper, we evaluate three classes of synthetic data generation approaches; probabilistic models, classification-based imputation models, and generative adversarial neural networks. Metrics for evaluating the quality of the generated synthetic datasets are presented and discussed.
While the results and discussions are broadly applicable to medical data, for demonstration purposes we generate synthetic datasets for cancer based on the publicly available cancer registry data from the Surveillance Epidemiology and End Results (SEER) program. Specifically, our cohort consists of breast, respiratory, and non-solid cancer cases diagnosed between 2010 and 2015, which includes over 360,000 individual cases.
Conclusions
We discuss the trade-offs of the different methods and metrics, providing guidance on considerations for the generation and usage of medical synthetic data.
Peer Review reports
Increasingly, large amounts and types of patient data are being electronically collected by healthcare providers, governments, and private industry. While such datasets are potentially highly valuable resources for scientists, they are generally not accessible to the broader research community due to patient privacy concerns. Even when it is possible for a researcher to gain access to such data, ensuring proper data usage and protection is a lengthy process with strict legal requirements. This can severely delay the pace of research and, consequently, its translational benefits to patient care.
To make sensitive patient data available to others, data owners typically de-identify or anonymize the data in a number of ways, including removing identifiable features (e.g., names and addresses), perturbing them (e.g., adding noise to birth dates), or grouping variables into broader categories to ensure more than one individual in each category [ 1 ]. While the residual information contained in properly anonymized data alone may not be used to re-identify individuals, once linked to other datasets (e.g., social media platforms), they may contain enough information to identify specific individuals. Efforts to determine the efficacy of de-identification methods have been inconclusive, particularly in the context of large datasets [ 2 ]. As such, it remains extremely difficult to guarantee that re-identification of individual patients is not a possibility with current approaches.
Given the risks of re-identification of patient data and the delays inherent in making such data more widely available, synthetically generated data is a promising alternative or addition to standard anonymization procedures. Synthetic data generation has been researched for nearly three decades [ 3 ] and applied across a variety of domains [ 4 , 5 ], including patient data [ 6 ] and electronic health records (EHR) [ 7 , 8 ]. It can be a valuable tool when real data is expensive, scarce or simply unavailable. While in some applications it may not be possible, or advisable, to derive new knowledge directly from synthetic data, it can nevertheless be leveraged for a variety of secondary uses, such as educative or training purposes, software testing, and machine learning and statistical model development. Depending on one’s objective, synthetic data can either entirely replace real data, augment it, or be used as a reasonable proxy to accelerate research.
A number of synthetic patient data generation methods aim to minimize the use of actual patient data by combining simulation, public population-level statistics, and domain expert knowledge bases [ 7 – 10 ]. For example, in Dube and Gallagher [ 8 ] synthetic electronic health records are generated by leveraging publicly available health statistics, clinical practice guidelines, and medical coding and terminology standards. In a related approach, patient demographics (obtained from actual patient data) are combined with expert-curated, publicly available patient care patterns to generate synthetic electronic medical records [ 9 ]. While the emphasis on not accessing real patient data eliminates the issue of re-identification, this comes at the cost of a heavy reliance on domain-specific knowledge bases and manual curation. As such, these methods may not be readily deployable to new cohorts or sets of diseases. Entirely data-driven methods, in contrast, produce synthetic data by using patient data to learn parameters of generative models. Because there is no reliance on external information beyond the actual data of interest, these methods are generally disease or cohort agnostic, making them more readily transferable to new scenarios.
Synthetic patient data has the potential to have a real impact in patient care by enabling research on model development to move at a quicker pace. While there exists a wealth of methods for generating synthetic data, each of them uses different datasets and often different evaluation metrics. This makes a direct comparison of synthetic data generation methods surprisingly difficult. In this context, we find that there is a void in terms of guidelines or even discussions on how to compare and evaluate different methods in order to select the most appropriate one for a given application. Here, we have conducted a systematic study of several methods for generating synthetic patient data under different evaluation criteria. Each metric we use addresses one of three criteria of high-quality synthetic data: 1) Fidelity at the individual sample level (e.g., synthetic data should not include prostate cancer in a female patient), 2) Fidelity at the population level (e.g., marginal and joint distributions of features), and 3) privacy disclosure. The scope of the study is restricted to data-driven methods only, which, as per the above discussion, do not require manual curation or expert-knowledge and hence can be more readily deployed to new applications. While there is no single approach for generating synthetic data which is the best for all applications, or even a one-size-fits-all approach to evaluating synthetic data quality, we hope that the current discussion proves useful in guiding future researchers in identifying appropriate methodologies for their particular needs.
The paper is structured as follows. We start by providing a focused discussion on the relevant literature on data-driven methods for generation of synthetic data, specifically on categorical features, which is typical in medical data and presents a set of specific modeling challenges. Next, we describe the methods compared in the current study, along with a brief discussion of the advantages and drawbacks of each approach. We then describe the evaluation metrics, providing some intuition on the utility and limitation of each. The datasets used and our experimental setup are presented. Finally, we discuss our results followed by concluding remarks.
Related work
Synthetic data generation can roughly be categorized into two distinct classes: process-driven methods and data-driven methods. Process-driven methods derive synthetic data from computational or mathematical models of an underlying physical process. Examples include numerical simulations, Monte Carlo simulations, agent-based modeling, and discrete-event simulations. Data-driven methods, on the other hand, derive synthetic data from generative models that have been trained on observed data. Because this paper is mainly concerned with data-driven methods, we briefly review the state-of-the-art methods in this class of synthetic data generation techniques. We consider three main types of data-driven methods: Imputation based methods, full joint probability distribution methods, and function approximation methods.
Imputation based methods for synthetic data generation were first introduced by Rubin [ 3 ] and Little [ 11 ] in the context of Statistical Disclosure Control (SDC), or Statistical Disclosure Limitation (SDL) [ 4 ]. SDC and SDL methodologies are primarily concerned with reducing the risk of disclosing sensitive data when performing statistical analyses. A general survey paper on data privacy methods related to SDL is Matthews and Harel [ 12 ]. Standard techniques are based on multiple imputation [ 13 ], treating sensitive data as missing data and then releasing randomly sampled imputed values in place of the sensitive data. These methods were later extended to the fully synthetic case by Raghunathan, Reiter and Rubin [ 14 ]. Early methods focused on continuous data with extensions to categorical data following [ 15 ]. Generalized linear regression models are typically used, but non-linear methods (such as Random Forest and neural networks) can and have been used [ 16 ]. Remedies for some of the shortcomings with multiple imputation for generating synthetic data are offered in Loong and Rubin [ 17 ]. An empirical study of releasing synthetic data under the methods proposed in Raghunathan, Reiter and Rubin [ 14 ] is presented in Reiter and Drechsler [ 18 ]. Most of the SDC/SDL literature focuses on survey data from the social sciences and demography. The generation of synthetic electronic health records has been addressed in Dube and Gallagher [ 8 ].
Multiple imputation has been the de facto method for generating synthetic data in the context of SDC and SDL. While imputation based methods are fully probabilistic, there is no guarantee that the resulting generative model is an estimate of the full joint probability distribution of the sampled population. In some applications, it may be of interest to model this probability distribution directly, for example if parameter interpretability is important. In this case, any statistical modeling procedure that learns a joint probability distribution is capable of generating fully synthetic data.
In the case of generating synthetic electronic health care records, one must be able to handle multivariate categorical data. This is a challenging problem, particularly in high dimensions. It is often necessary to impose some sort of dependence structure on the data [ 19 ]. For example, Bayesian networks, which approximate a joint distribution using a first-order dependence tree, have been proposed in Zhang et al. [ 20 ] as a method for generating synthetic data with privacy constraints. More flexible non-parametric methods need not impose such dependence structures on the distributions. Examples of Bayesian non-parametric methods for multidimensional categorical data include latent Gaussian process methods [ 21 ] and Dirichlet mixture models [ 22 ].
Synthetic data has recently attracted attention from the machine learning (ML) and data science communities for reasons other than data privacy. Many state-of-the-art ML algorithms are based on function approximation methods such as deep neural networks (DNN). These models typically have a large number of parameters and require large amounts of data to train. When labeled data sets are impossible or expensive to obtain, it has been proposed that synthetically generated training data can complement scarce real data [ 23 ]. Similarly, transfer learning from synthetic data to real data to improve ML algorithms has also been explored [ 24 , 25 ]. Thus data augmentation methods from the ML literature are a class of synthetic data generation techniques that can be used in the bio-medical domain.
Generative Adversarial Networks (GANs) are a popular class of DNNs for unsupervised learning tasks [ 26 ]. In particular, they produce two jointly-trained networks; one which generates synthetic data intended to be similar to the training data, and one which tries to discriminate the synthetic data from the true training data. They have proven to be very adept at learning high-dimensional, continuous data such as images [ 26 , 27 ]. More recently GANs for categorical data have been proposed in Camino, Hammerschmidt and State [ 28 ] with specific applications to synthetic EHR data in Choi et al. [ 29 ].
Finally, we note that several open-source software packages exist for synthetic data generation. Recent examples include the R packages synthpop [ 30 ] and SimPop [ 31 ], the Python package DataSynthesizer [ 5 ], and the Java-based simulator Synthea [ 7 ].
Methods for synthetic data generation
In this paper we investigate various techniques for synthetic data generation. The techniques we investigate range from fully generative Bayesian models to neural network based adversarial models. We next provide brief descriptions of the synthetic data generation approaches considered.
Sampling from independent marginals
The Independent marginals (IM) method is based on sampling from the empirical marginal distributions of each variable. The empirical marginal distribution is estimated from the observed data. We next summarize the key advantages (+) and disadvantages (-) of this approach.
This approach is computationally efficient and the estimation of marginal distributions for different variables may be done in parallel.
IM does not capture statistical dependencies across variables, and hence the generated synthetic data may fail to capture the underlying structure of the data.
This method is included in our analysis solely as a simple baseline for other more complex approaches.
Bayesian network
Bayesian networks (BN) are probabilistic graphical models where each node represents a random variable, while the edges between the nodes represent probabilistic dependencies among the corresponding random variables. For synthetic data generation using a Bayesian network, the graph structure and the conditional probability distributions are inferred from the real data. In BN, the full joint distribution is factorized as:
where V is the set of random variables representing the categorical variables and x pa( v ) is the subset of parent variables of v , which is encoded in the directed acyclic graph.
The learning process consists of two steps: ( i ) learning a directed acyclic graph from the data, which expresses all the pairwise conditional (in)dependence among the variables, and ( ii ) estimating the conditional probability tables (CDP) for each variable via maximum likelihood. For the first step we use the Chow-Liu tree [ 19 ] method, which seeks a first-order dependency tree-based approximation with the smallest KL-divergence to the actual full joint probability distribution. The Chow-Liu algorithm provides an approximation and cannot represent higher-order dependencies. Nevertheless, it has been shown to provide good results for a wide range of practical problems.
The graph structure inferred from the real data encodes the conditional dependence among the variables. In addition, the inferred graph provides a visual representation of the variables’ relationships. Synthetic data may be generated by sampling from the inferred Bayesian network. We next summarize the key advantages and disadvantages of this approach.
BN is computationally efficient and scales well with the dimensionality of the dataset.
The directed acyclic graph can also be utilized for exploring the causal relationships across the variables.
Even though the full joint distribution’s factorization, as given by Eq. ( 1 ), is general enough to include any possible dependency structure, in practice, simplifying assumptions on the graphical structure are made to ease model inference. These assumptions may fail to represent higher-order dependencies.
The inference approach adopted in this paper is applicable only to discrete data. In addition, the Chow-Liu heuristic used here constructs the directed acyclic graph in a greedy manner. Therefore, an optimal first-order dependency tree is not guaranteed.
Mixture of product of multinomials
Any multivariate categorical data distribution can be expressed as a mixture of product of multinomials (MPoM) [ 22 ],
where x i =( x i 1 ,…, x ip ) represents a vector of p categorical variables, k is the number of mixture components, ν h is the weight associated with the h -th mixture component, and \(\psi _{hc_{j}}^{(j)} = Pr(x_{ij}= c_{j}|z_{i} = h)\) is the probability of x ij = c j given allocation of individual i to cluster h , where z i is a cluster indicator. Although any multivariate distribution may be expressed as in ( 2 ) for a sufficiently large k , proper choice of k is troublesome. To obtain k in a data-driven manner, Dunson and Xing [ 22 ] proposed a Dirichlet process mixture of product multinomials to model high-dimensional multivariate categorical data. We next summarize the key advantages and disadvantages of this approach.
Theoretical guarantees exist regarding the flexibility of mixture of product multinomials to model any multivariate categorical data.
The Dirichlet process mixture of product of multinomials is a fully conjugate model and efficient inference may be done via a Gibbs sampler.
Sampling based inference can be very slow in high dimensional problems.
While extending the model to mixed data types (such as continuous and categorical) is relatively straightforward, theoretical guarantees do not exist for mixed data types.
Categorical latent Gaussian process
The categorical latent Gaussian process (CLGP) is a generative model for multivariate categorical data [ 21 ]. CLGP uses a lower dimensional continuous latent space and non-linear transformations for mapping the points in the latent space to probabilities (via softmax) for generating categorical values. The authors employ standard Normal priors on the latent space and sparse Gaussian process (GPs) mappings to transform the latent space. For modeling clinical data related to cancer, the model assumes that each patient record (a data vector containing a set of categorical variables) has a continuous latent low-dimensional representation. The proposed model is not fully conjugate, but model inference may be performed via variational techniques.
The hierarchical CLGP model [ 21 ] is provided below:
for n ∈ [ N ] (the set of naturals between 1 and N), q ∈ [ Q ], d ∈ [ D ], k ∈ [ K ], m ∈ [ M ], covariance matrices K d , and where the Softmax distribution is defined as,
for k =0,..., K and with f 0 :=0. Each patient is represented in the latent space as x n . For each feature d , x n has a sequence of weights ( f n d 1 ,..., f ndK ), corresponding to each possible feature level k , that follows a Gaussian process. Softmax returns a feature value y nd based on these weights, resulting in the patient’s feature vector y n =( y n 1 ,..., y nD ). Note that CLGP does not explicitly model dependence across variables (features). However, the Gaussian process explicitly captures the dependence across patients and the shared low-dimensional latent space implicitly captures dependence across variables.
We next summarize the key advantages and disadvantages of this approach.
Like BN and MPoM, CLGP is a fully generative Bayesian model, but has richer latent non-linear mappings that allows for representation of very complex full joint distributions.
The inferred low-dimensional latent space in CLGP may be useful for data visualization and clustering.
Inference for CLGP is considerably more complex than other models due to its non-conjugacy. An approximate Bayesian inference method such as variational Bayes (VB) is required.
VB for CLGP requires several other approximations such as low-rank approximation for GPs as well as Monte Carlo integration. Hence, the inference for CLGP scales poorly with data size.
Generative adversarial networks
Generative adversarial networks (GANs) [ 26 ] have recently been shown to be remarkably successful for generating complex synthetic data, such as images and text [ 32 – 34 ]. In this approach, two neural networks are trained jointly in a competitive manner: the first network tries to generate realistic synthetic data, while the second one attempts to discriminate real and synthetic data generated by the first network. During the training each network pushes the other to perform better. A widely known limitation of GANs is that it is not directly applicable for generating categorical synthetic datasets, as it is not possible to compute the gradients on latent categorical variables that are required for training via backpropagation. As clinical patient data are often largely categorical, recent works like medGAN [ 29 ] have applied autoencoders to transform categorical data to a continuous space, after which GANs can be applied for generating synthetic electronic health records (EHR). However, medGAN is applicable to binary and count data, and not multi-categorical data. In this paper we adopt the multi-categorical extension of medGAN, called MC-MedGAN [ 28 ] to generate synthetic data related to cancer. We next summarize the key advantages and disadvantages of this approach.
Unlike POM, BN and CLGP, MC-MedGAN is a generative approach which does not require strict probabilistic model assumptions. Hence, it is more flexible compared to BN, CLGP and POM.
GANs-based models can be easily extended to deal with mixed data types, e.g., continuous and categorical variables.
MC-MedGAN is a deep model and has a very large number of parameters. Proper choice of multiple tuning parameters (hyper-parameters) is difficult and time consuming.
GANs are known to be difficult to train as the process of solving the associated min-max optimization problem can be very unstable. However, recently proposed variations of GAN such as Wasserstein GANs, and its variants, have significantly alleviated the problem of stability of training GANs [ 35 , 36 ].
Multiple imputation
Multiple imputation based methods have been very popular in the context of synthetic data generation, especially for applications where a part of the data is considered sensitive [ 4 ]. Among the existing imputation methods, the Multivariate Imputation by Chained Equations (MICE) [ 37 ] has emerged as a principled method for masking sensitive content in datasets with privacy constraints. The key idea is to treat sensitive data as missing data. One then imputes this “missing” data with randomly sampled values generated from models trained on the nonsensitive variables.
As discussed earlier, generating fully synthetic data often utilizes a generative model trained on an entire dataset. It is then possible to generate complete synthetic datasets from the trained model. This approach differs from standard multiple imputation methods such as MICE, which train on subsets of nonsensitive data to generate synthetic subsets of sensitive data. In this paper we use a variation of MICE for the task of fully synthetic data generation. Model inference proceeds as follows.
Define a topological ordering of the variables.
Compute the empirical marginal probability distribution for the first variable.
For each successive variables in the topological order, learn a probabilistic model for the conditional probability distribution on the current variable given the previous variables, that is, p ( x v | x : v ), which is done by regressing the v -th variable on all its predecessors as independent variables.
In the sampling phase, the first variable is sampled from the empirical distribution and the remaining variables are randomly sampled from the inferred conditional distributions following the topological ordering. While modeling the conditional distributions with generalized linear models is very popular, other non-linear techniques such as random forests and neural nets may be easily integrated in this framework.
For the MICE variation used here, the full joint probability distribution is factorized as follows:
where V is the set of random variables representing the variables to be generated, and p ( x v | x : v ) is the conditional probability distribution of the v -th random variable given all its predecessors. Clearly, the definition of the topological ordering plays a crucial role in the model construction. A common approach is to sort the variables by the number of levels either in ascending or descending order.
MICE is computationally fast and can scale to very large datasets, both in the number of variables and samples.
It can easily deal with continuous and categorical values by properly choosing either a Softmax or a Gaussian model for the conditional probability distribution for a given variable.
While MICE is probabilistic, there is no guarantee that the resulting generative model is a good estimate of the underlying joint distribution of the data.
MICE strongly relies on the flexibility of the model for the conditional probability distributions and also the topological ordering of the directed acyclic graph.
Evaluation metrics
To measure the quality of the synthetic data generators, we use a set of complementary metrics that can be divided into two groups: ( i ) data utility, and ( ii ) information disclosure. In the former, the metrics gauge the extent to which the statistical properties of the real (private) data are captured and transferred to the synthetic dataset. In the latter group, the metrics measure how much of the real data may be revealed (directly or indirectly) by the synthetic data. It has been well documented that increased generalization and suppression in anonymized data (or smoothing in synthetic data) for increased privacy protection can lead to a direct reduction in data utility [ 38 ]. In the context of this trade-off between data utility and privacy, evaluation of models for generating such data must take both opposing facets of synthetic data into consideration.
Data utility metrics
In this group, we consider the following metrics: Kullback-Leibler (KL) divergence , pairwise correlation difference , log-cluster , support coverage , and cross-classification .
The Kullback-Leibler (KL) divergence is computed over a pair of real and synthetic marginal probability mass functions (PMF) for a given variable, and it measures the similarity of the two PMFs. When both distributions are identical, the KL divergence is zero, while larger values of the KL divergence indicate a larger discrepancy between the two PMFs. Note that the KL divergence is computed for each variable independently; therefore, it does not measure dependencies among the variables. The KL divergence of two PMFs, P v and Q v for a given variable v , is computed as follows:
where | v | is the cardinality (number of levels) of the categorical variable v . Note that the KL divergence is defined at the variable level, not over the entire dataset.
The pairwise correlation difference (PCD) is intended to measure how much correlation among the variables the different methods were able to capture. PCD is defined as:
where X R and X S are the real and synthetic data matrices, respectively. PCD measures the difference in terms of Frobennius norm of the Pearson correlation matrices computed from real and synthetic datasets. The smaller the PCD, the closer the synthetic data is to the real data in terms of linear correlations across the variables. PCD is defined at the dataset level.
The log-cluster metric [ 39 ] is a measure of the similarity of the underlying latent structure of the real and synthetic datasets in terms of clustering. To compute this metric, first, the real and synthetic datasets are merged into one single dataset. Second, we perform a cluster analysis on the merged dataset with a fixed number of clusters G using the k -means algorithm. Finally, we calculate the metric as follows:
where n j is the number of samples in the j -th cluster, \(n_{j}^{R}\) is the number of samples from the real dataset in the j -th cluster, and c = n R /( n R + n S ). Large values of U c indicate disparities in the cluster memberships, suggesting differences in the distribution of real and synthetic data. In our experiments, the number of clusters was set to 20. The log-cluster metric is defined at the dataset level.
The support coverage metric measures how much of the variables support in the real data is covered in the synthetic data. The metric considers the ratio of the cardinalities of a variable’s support (number of levels) in the real and synthetic data. Mathematically, the metric is defined as the average of such ratios over all variables:
where \(\mathcal {R}^{v}\) and \(\mathcal {S}^{v}\) are the support of the v -th variable in the real and synthetic data, respectively. At its maximum (in the case of perfect support coverage), this metric is equal to 1. This metric penalizes synthetic datasets if less frequent categories are not well represented. It is defined at the dataset level.
The cross-classification metric is another measure of how well a synthetic dataset captures the statistical dependence structures existing in the real data. Unlike PCD, in which statistical dependence is measured by Pearson correlation, cross-classification measures dependence via predictions generated for one variable based on the other variables (via a classifier).
We consider two cross-classification metrics in this paper. The first cross-classification metric, referred to as CrCl-RS, involves training on the real data and testing on hold-out data from both the real and synthetic datasets. This metric is particularly useful for evaluating if the statistical properties of the real data are similar to those of the synthetic data. The second cross-classification metric, referred to as (CrCl-SR), involves training on the synthetic data and testing on hold-out data from both real and synthetic data. This metric is particularly useful in determining if scientific conclusions drawn from statistical/machine learning models trained on synthetic datasets can safely be applied to real datasets. We next provide additional details regarding the cross-classification metric CrCl-RS. The cross-classification metric CrCl-SR is computed in a similar manner.
The available real data is split into training and test sets. A classifier is trained on the training set (real) and applied to both test set (hold out real) and the synthetic data . Classification performance metrics are computed on both sets. CrCl-RS is defined as the ratio between the performance on synthetic data and on the held out real data. Figure 1 presents a schematic representation of the cross classification computation. Clearly, the classification performance is dependent on the chosen classifier. Here, we consider a decision tree as the classifier due to the discrete nature of the dataset. To perform the classification, one of the variables is used as a target, while the remaining are used as predictors. This procedure is repeated for each variable as target, and the average value is reported. In general, for both cross-classification metrics, a value close to 1 is ideal.
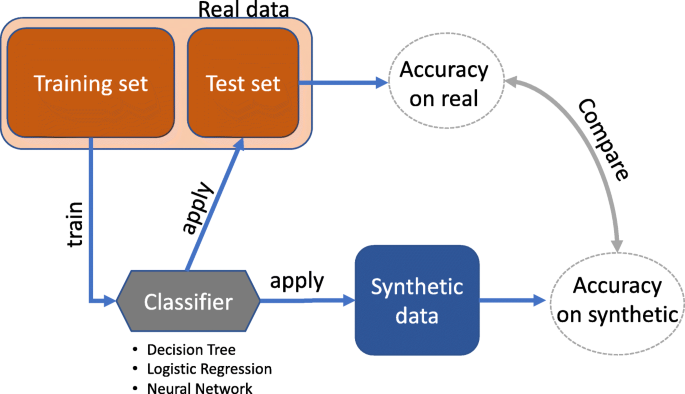
Schematic view of the cross-classification metric computation. It consists of the following steps: (1) real data is split into training and test sets; (2) classifier is trained on the training set; (3) classifier is applied on both test set (real) and synthetic data; and (4) the ratio of the classification performances is calculated
Disclosure metrics
There are two broad classes of privacy disclosure risks: identity disclosure and attribute disclosure . Identity or membership disclosure refers to the risk of an intruder correctly identifying an individual as being included in the confidential dataset. This attack is possible when the attacker has access to a complete set of patient records. In the fully synthetic case, the attacker wants to know whether a private record the attacker has access to was used for training the generative model that produced the publicly available synthetic data. Attribute disclosure refers to the risk of an intruder correctly guessing the original value of the synthesized attributes of an individual whose information is contained in the confidential dataset. In the “ Experimental analysis on SEER’s research dataset ” section, we will show results for both privacy disclosure metrics. Next, we provide details on how these metrics are computed.
In membership disclosure [ 29 ], one claims that a patient record x was present in the training set if there is at least one synthetic data sample within a certain distance (for example, in this paper we have considered Hamming distance) to the record x . Otherwise, it is claimed not to be present in the training set. To compute the membership disclosure of a given method m , we select a set of r patient records used to train the generative model and another set of r patient records that were not used for training, referred to as test records . With the possession of these 2 r patient records and a synthetic dataset generated by the method m , we compute the claim outcome for each patient record by calculating its Hamming distance to each sample from the synthetic dataset, and then determining if there is a synthetic data sample within a prescribed Hamming distance. For each claim outcome there are four possible scenarios: true positive (attacker correctly claims their targeted record is in the training set), false positive (attacker incorrectly claims their targeted record is in the training set), true negative (attacker correctly claims their targeted record is not in the training set), or false negative (attacker incorrectly claims their targeted record is not in the training set). Finally, we compute the precision and recall of the above claim outcomes. In our experiments, we set r =1000 records and used the entire set of synthetic data available.
Attribute disclosure [ 29 ] refers to the risk of an attacker correctly inferring sensitive attributes of a patient record (e.g., results of medical tests, medications, and diagnoses) based on a subset of attributes known to the attacker. For example, in the fully synthetic data case, an attacker can first extract the k nearest neighboring patient records of the synthetic dataset based on the known attributes, and then infer the unknown attributes via a majority voting rule. The chance of unveiling the private information is expected to be low if the synthetic generation method has not memorized the private dataset. The number of known attributes, the size of the synthetic dataset, and the number of k nearest neighbors used by the attacker affect the chance of revealing the unknown attributes. In our experiments we investigate the chance that an attacker can reveal all the unknown attributes, given different numbers of known attributes and several choices of k .
In addition to membership and attribute attacks, the framework of differential privacy has garnered a lot of interest [ 40 – 42 ]. The key idea is to protect the information of every individual in the database against an adversary with complete knowledge of the rest of the dataset. This is achieved by ensuring that the synthetic data does not depend too much on the information from any one individual. A significant amount of research has been devoted on designing α -differential or ( α , δ )-differential algorithms [ 43 , 44 ]. An interesting direction of research has been in converting popular machine learning algorithms, such as deep learning algorithms, to differentially private algorithms via techniques such as gradient clipping and noise addition [ 45 , 46 ]. In this paper, we have not considered differential privacy as a metric. While the algorithms discussed in this paper such as MC-MedGAN or MPoM may be modified to introduce differential privacy, that is beyond the scope of this paper.
Experimental analysis on SEER’s research dataset
In this section we describe the data used in our experimental analysis. We considered the methods previously discussed, namely Independent Marginals (IM), Bayesian Network (BN), Mixture of Product of Multinomials (MPoM), CLGP, MC-MedGAN, and MICE. Three variants of MICE were considered: MICE with Logistic Regression (LR) as classifier and variables ordered by the number of categories in an ascending manner (MICE-LR), MICE with LR and ordered in a descending manner (MICE-LR-DESC), and MICE with Decision Tree as classifier (MICE-DT) in ascending order. MICE-DT with descending and ascending order produced similar results and only one is reported in this paper for brevity.
Dataset variable selection
A subset of variables from the public research SEER’s dataset Footnote 1 was used in this experiment. The variables were selected after taking into account the characteristics of the variables and their temporal availability, as some variables were more recently introduced as compared to others. Two sets of variables were created: ( i ) a set of 8 variables with a small number of categories ( small-set ); and ( ii ) a larger set with ∼ 40 variables ( large-set ) that includes variables with a large number (hundreds) of categories. We want to see the relative performances of the different synthetic data generation approaches on a relatively easy dataset ( small-set ) and on a more challenging dataset ( large-set ).
The SEER’s research dataset is composed of sub-datasets, where each sub-dataset contains diagnosed cases of a specific cancer type collected from 1973 to 2015. For this analysis we considered the sub-datasets from patients diagnosed with breast cancer (BREAST), respiratory cancer (RESPIR), and lymphoma of all sites and leukemia (LYMYLEUK). We used data from cases diagnosed between 2010 and 2015 due to the nonexistence of some of variables prior to this period. The number of patient records in the BREAST, RESPIR, and LYMYLEUK datasets are 169,801; 112,698; and 84,132; respectively. We analyze the performance of the methods on each dataset separately. Table 1 presents the variables selected. A pre-processing step in some cases involves splitting a more complex variable into two variables, as some variables originally contained both categorical and integer (count) values.
The number of levels (categories) in each variable is diverse. In the small-set feature set the number of categories ranges from 1 to 14, while for the large-set it ranges from 1 to 257. The number of levels for each variable in presented Tables 2 and 3 .
Figure 2 depicts the histogram of some variables in the BREAST small-set dataset. Noticeably, the levels’ distributions are imbalanced and many levels are underrepresented in the real dataset. For example, variable DX_CONF mostly contains records with the same level, and LATERAL only has records with 2 out of 5 possible levels. This imbalance may inadvertently lead to disclosure of information in the synthetic dataset, as the methods are more prone to overfit when the data has a smaller number of possible record configurations.
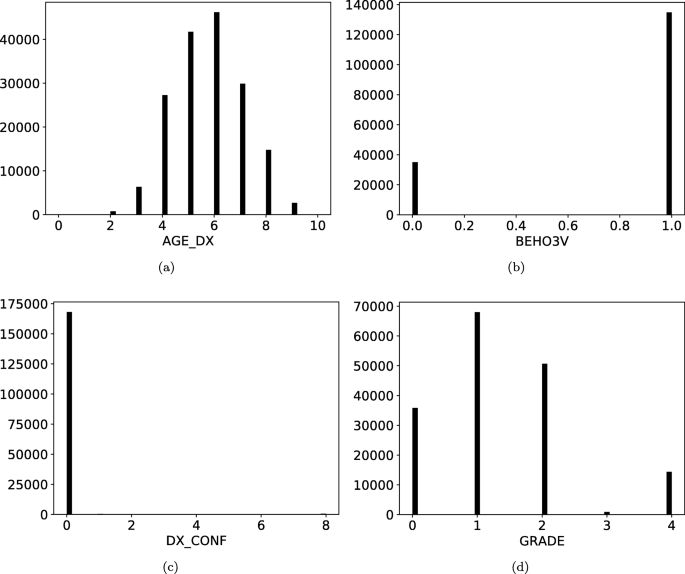
Histogram of four BREAST small-set variables from the real dataset. Levels’ distributions are clearly imbalanced
Implementation details and hyper-parameter selection
When available we used the code developed by the authors of the paper proposing the synthetic data generation method. For CLGP, we used the code from the authors’ GitHub repository [ 47 ]. For MC-MedGAN, we also utilized the code from the authors [ 48 ]. For the Bayesian networks, we used two Python packages: pomegranate [ 49 ] and libpgm [ 50 ]. All other methods were implemented by ourselves. The hyper-parameter values used for all methods were selected via grid-search. The selected values were those which provided the best performance for the log-cluster utility metric. This metric was used as it is the only metric, in our pool of utility metrics, that measures the similarity of the full real and synthetic data distributions, and not only the marginal distributions or only the relationship across variables. The range of hyper-parameter values explored for all methods is described below.
Hyper-parameter values
To select the best hyper-parameter values for each method, we performed a grid-search over a set of candidate values. Below we present the set of values tested. Bayesian networks and Independent Marginals did not have hyper-parameters to be selected. MPoM: The truncated Dirichlet process prior uses 30 clusters ( k =30), concentration parameter α =10, and 10,000 Gibbs sampling steps with 1,000 burn-in steps, for both small-set and large-set . For the grid-search selection, we tested k =[5,10,20,30,50], and k =30 led to the best log-cluster performance. CLGP: We used 100 inducing points and 5-dimensional latent space for small-set ; and 100 inducing points and 10-dimensional latent space for the large-set . Increasing the number of inducing points usually leads to a better utility performance, but the computational cost increases substantially. Empirically, we found that 100 inducing points provides an adequate balance between utility performance and computational cost. For the small-set , the values tested for the latent space size was [2, 3, 4, 5, 6] dimensions; and for the large-set [5, 10, 15] dimensions. MC-MedGAN: We tested two variations of model configuration used by the authors in the original paper. The variations were a smaller model (Model 1) and a bigger model (Model 2), in terms of number of parameters (See Table 4 ). The bigger model is more flexible and in theory can capture highly non-linear relations among the attributes, and provide better continuous representation of the discrete data, via an autoencoder. On the other hand, the smaller model is less prone to overfit the private data. We also noticed that a proper definition for the learning rate, both the pre-trained autoenconder and GAN, is crucial for the model performance. We tested both models with learning rate of [1e-2, 1e-3, 1e-4]. We then selected the best performing model for each feature set considering the log-cluster utility metric. “Model 1" performed better for small-set and “Model 2" for large-set . The best value for learning rate found was 1e-3. MICE-DT: The decision tree uses Gini split criterion, unlimited tree growth, minimum number of samples for node splitting is 2, and minimum number of samples in a leaf node is 1.
We evaluated the methods described in Section ‘Methods’ on the subsets of the SEER’s research dataset. To conserve space we only discuss results for the BREAST cancer dataset. Tables and figures for LYMYLEUK and RESPIR are shown at the end of the corresponding sections. From our empirical investigations, the conclusions drawn from the breast cancer dataset can be extended to the LYMYLEUK and RESPIR datasets. Unless stated otherwise, in all the following experiments, the number of synthetic samples generated is identical to the number of samples in the real dataset: BREAST = 169,801; RESPIR = 112,698; and LYMYLEUK = 84,132.
On the small-set
From Table 5 , we observe that many methods succeeded in capturing the statistical dependence among the variables, particularly MPoM, MICE-LR, MICE-LR-DESC, and MICE-DT. Synthetic data generated by these methods produced correlation matrices nearly identical to the one computed from real data (low PCD). Data distribution difference measured by log-cluster is also low. All methods showed a high support coverage. As seen in Fig. 2 , BREAST small-set variables have only a few levels dominating the existing records in the real dataset, while the remaining levels are underrepresented or even nonexistent. This level imbalance reduces the sampling space making the methods more likely to overfit and, consequently, exposes more real patient’s information.
Figure 3 shows the distribution of some of the utility metrics for all variables. KL divergences, shown in Fig. 3 c, are low for the majority of the methods, implying that the marginal distributions of real and synthetic datasets are equivalent. KL divergences for MC-MedGAN is reasonably larger compared to the other methods, particularly due to the variable AGE_DX (Fig. 4 c).
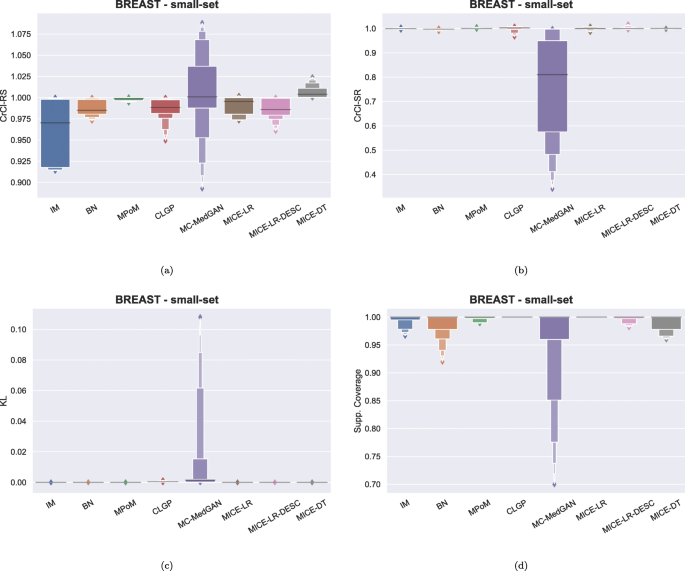
Data utility performance over all variables presented as boxplots on BREAST small-set . a CrCl-RS, b CrCl-SR, c KL divergence for each attribute, and d support coverage
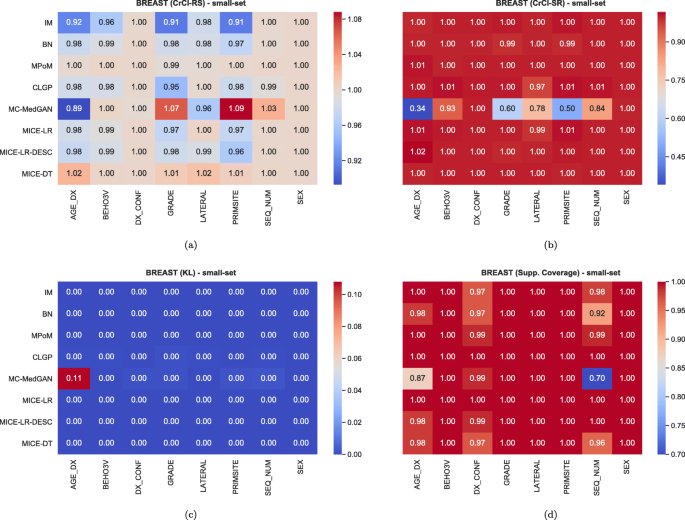
Heatmaps displaying CrCl-RS, CrCl-SR, KL divergence, and support coverage average computed over 10 independently generated synthetic BREAST small-set
Regarding CrCl-RS in Fig. 3 a, we observe that all methods are capable of learning and transferring variable dependencies from the real to the synthetic data. MPoM presented the lowest variance while MC-MedGAN has the largest, implying that MC-MedGAN is unable to capture the dependence of some of the variables. From Fig. 4 a, we identify AGE_DX, PRIMSITE, and GRADE as the most challenging variables for MC-MedGAN. AGE_DX and PRIMSITE are two of the variables with the largest set of levels, with 11 and 9, respectively. It suggests that MC-MedGAN potentially faces difficulties on datasets containing variables with a large number of categories.
From Fig. 3 b, we clearly note that the synthetic data generated by MC-MedGAN does not mimic variable dependencies from the real dataset, while all other methods succeeded in this task. Looking at the difference between CrCl-RS and CrCl-SR, one can infer how close the real and synthetic data distributions are. Performing well on CrCl-RS but not on CrCl-SR indicates that MC-MedGAN only generated data from a subspace of the real data distribution that can be attributed to partial modal collapse, which is a known issue for GANs [ 51 , 52 ]. This hypothesis is corroborated by the support coverage value of MC-MedGAN that is the lowest among all methods.
Figure 5 shows the attribute disclosure metric computed on BREAST cancer data with the small-set list of attributes, assuming the attacker tries to infer four (top) and three (bottom) unknown attributes, out of eight possible, of a given patient record. Different numbers of nearest neighbors are used to infer the unknown attributes, k =[1, 10, 100]. From the results, we notice that the larger the number of the nearest neighbors k , the lower the chance of an attacker successfully uncover the unknown attributes. Using only the closest synthetic record ( k =1) produced a more reliable guess for the attacker. When 4 attributes are unknown by the attacker, he/she could reveal about 70% of the cases, while this rate jumps to almost 100% when 3 attributes are unknown. Notice that IM consistently produced one of the best (lowest) attribute disclosures across all cases, as it does not model the dependence across the variables. MC-MedGAN shows significantly low attribute disclosure for k =1 and when the attacker knows 4 attributes, but it is not consistent across other experiments with BREAST data. MC-MedGAN produced the highest value for scenarios with k =10 and k =100.
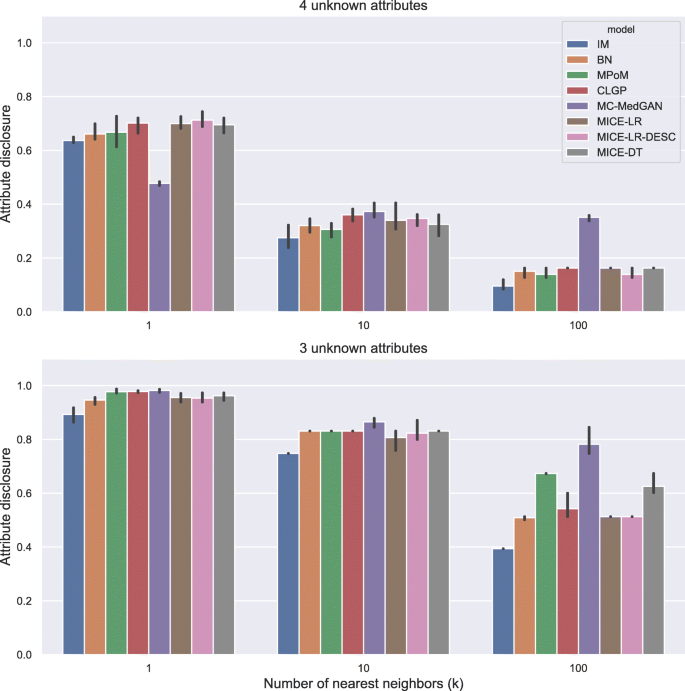
Attribute disclosure for distinct numbers of nearest neighbors (k). BREAST small-set . Top plot shows results for the scenario that an attacker tries to infer 4 unknown attributes out of 8 attributes in the dataset. Bottom plot presents the results for 3 unknown attributes
Membership disclosure results provided in Fig. 6 for BREAST small-set shows a precision around 0.5 for all methods across the entire range of Hamming distances. This means that among the set of patient records that the attacker claimed to be in the training set, based on the attacker’s analysis of the available synthetic data, only 50% of them are actually in the training set. Regarding the recall, all the methods except MC-MedGAN showed a recall around 0.9 for the smallest prescribed Hamming distances, indicating that the attacker could identify 90% of the patient records actually used for training. MC-MedGAN presented much lower recall in these scenarios, therefore it is more effective in protecting private patient records. For larger Hamming distances, as expected, all methods obtain a recall of one as there will be a higher chance of having at least one synthetic sample within the larger neighborhood (in terms of Hamming distance). Therefore, the attacker claims that all patient records are in the training set.
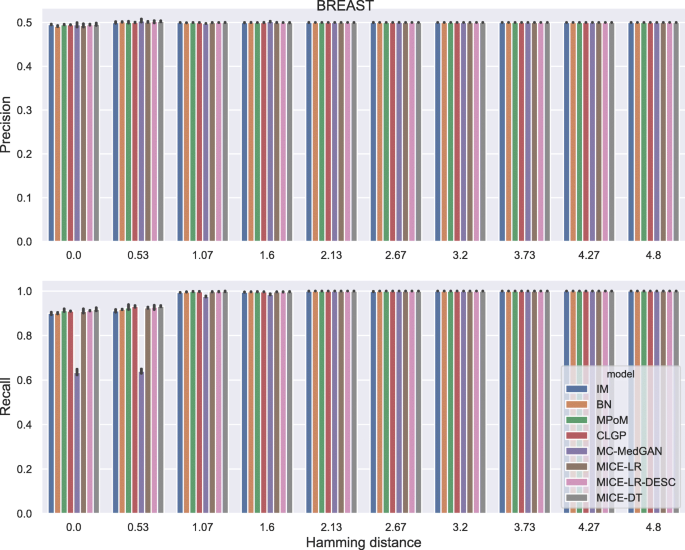
Precision and recall of membership disclosure for all methods. BREAST small-set . MC-MedGAN presents the best performance
Similarly to the analysis performed for the BREAST dataset, Tables 6 and 7 reports performance of the methods on LYMYLEUK and RESPIR datasets using the small-set selection of variables. Figures 7 , 8 , 9 , 10 , 11 , 12 , 13 , and 14 present utility and privacy methods’ performance plots for the LYMYLEUK and RESPIR datasets.
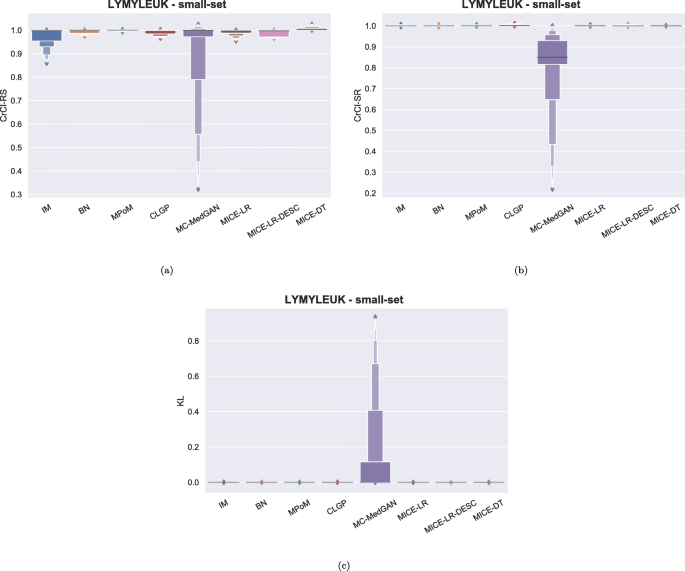
Data utility metrics performance distribution over all variables shown as boxplots on LYMYLEUK small-set
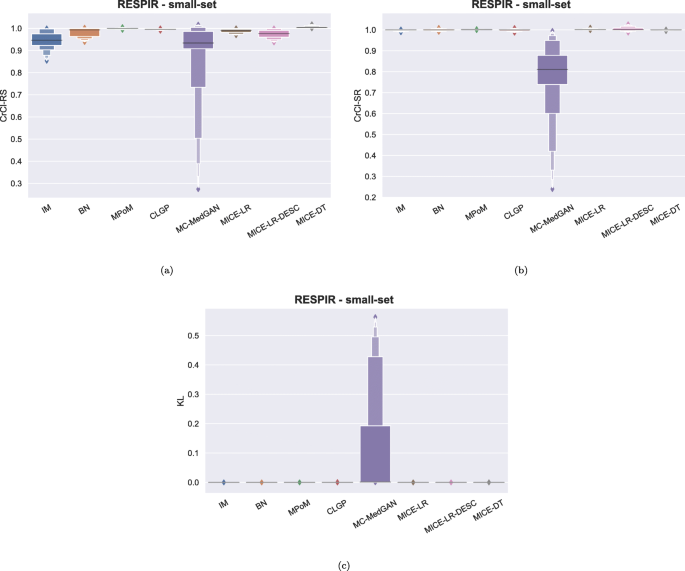
Metrics performance distribution over all variables shown as boxplots on RESPIR small-set
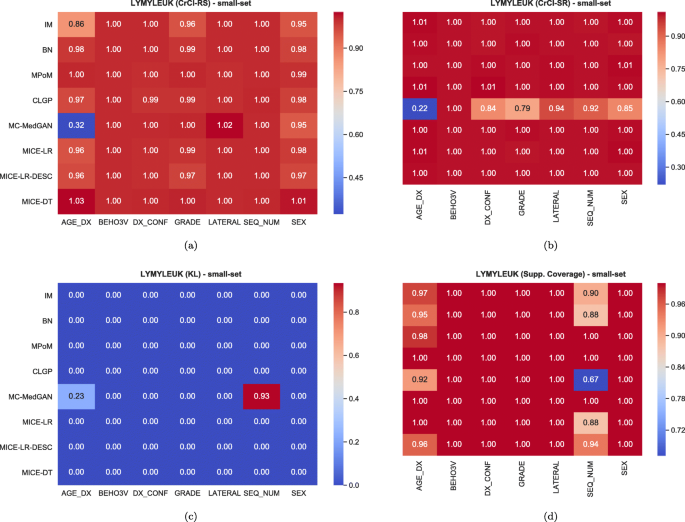
Heatmaps displaying the average over 10 independently generate synthetic datasets of ( a ) CrCl-RS, ( b ) CrCl-SR, ( c ) KL divergence, and ( d ) support coverage, at a variable level. LYMYLEUK small-set
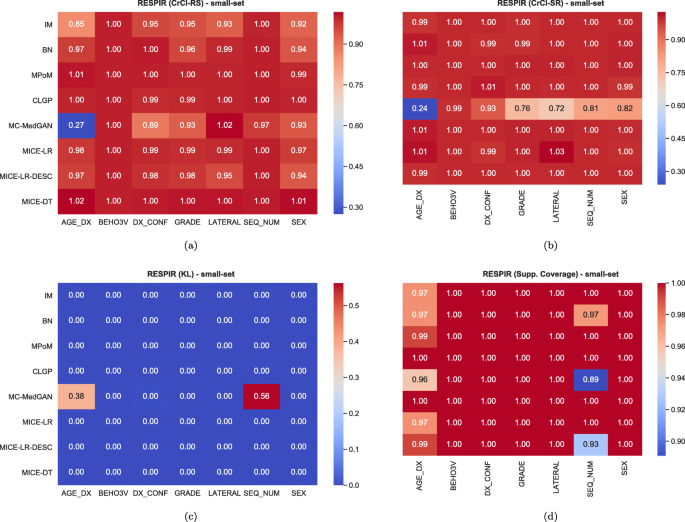
Heatmaps displaying the average over 10 independently generate synthetic datasets of ( a ) CrCl-RS, ( b ) CrCl-SR, ( c ) KL divergence, and ( d ) support coverage, at a variable level. RESPIR small-set
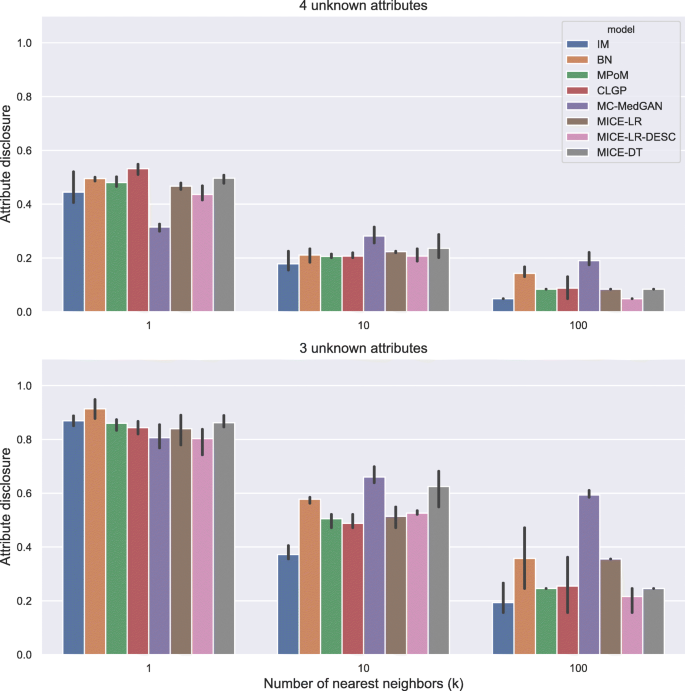
Attribute disclosure for LYMYLEUK small-set
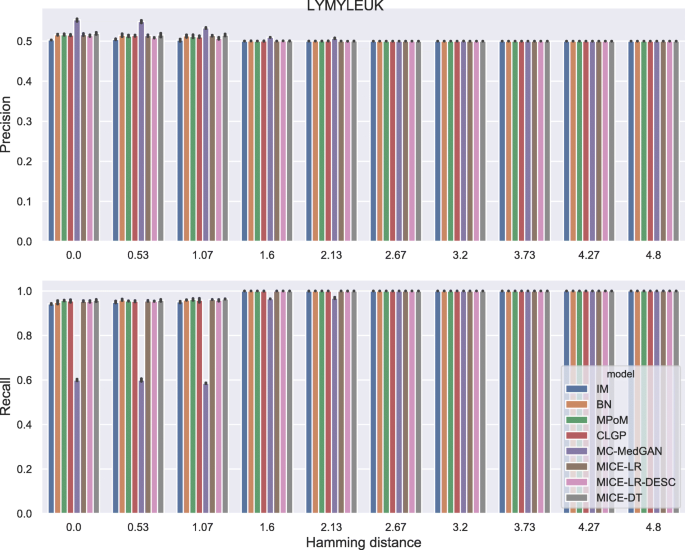
Precision and recall for membership disclosure for LYMYLEUK small-set
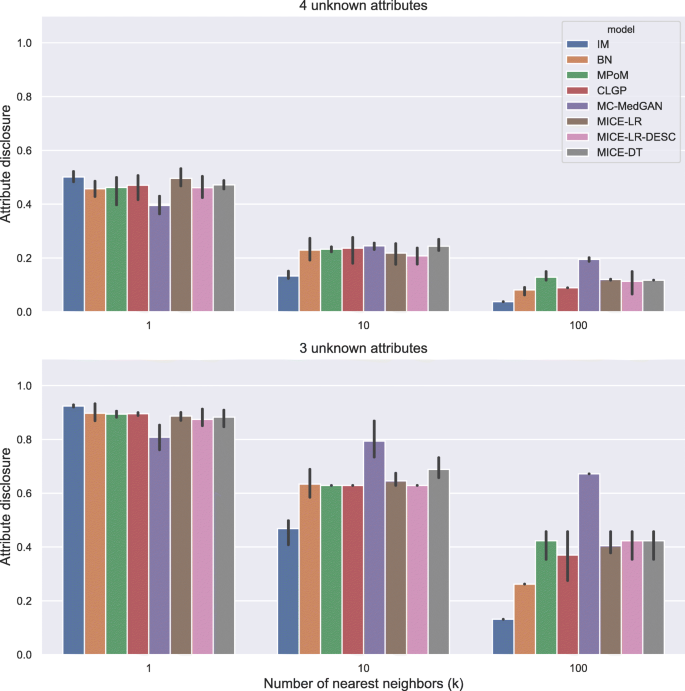
Attribute disclosure for RESPIR small-set
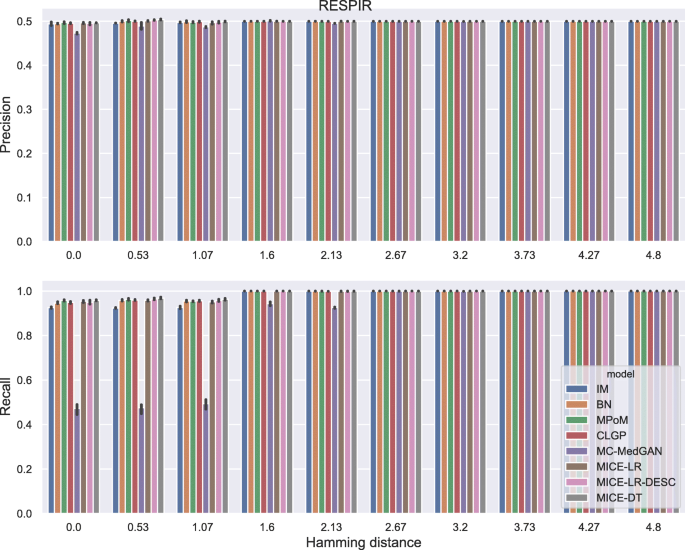
Precision and recall for membership disclosure for RESPIR small-set
On the large-set
The large-set imposes additional challenges to the synthetic data generation task, both in terms of the number of the variables and the inclusion of variables with a large number of levels. Modeling variables with too many levels requires an extended amount of training samples to properly cover all possible categories. Moreover, we noticed that in the real data a large portion of the categories are rarely observed, making the task even more challenging.
From Table 8 we observe that MICE-DT obtained significantly superior data utility performance compared to the competing models. As MICE-DT uses a flexible decision tree as the classifier, it is more likely to extract intricate attribute relationships that are consequently passed to the synthetic data. Conversely, MICE-DT is more susceptible to memorizing the private dataset (overfitting). Even though overfitting can be alleviated by changing the hyper-parameter values of the model, such as the maximum depth of the tree and the minimum number of samples at leaf nodes, this tuning process is required for each dataset which can be very time consuming. Using a MICE method with a less flexible classifier, such as MICE-LR, can be a viable alternative.
It is also worth mentioning that the order of the variables in MICE-LR has a significant impact, particularly in capturing the correlation of the variables measured by PCD. MICE-LR with ascending order produced a closer correlation matrix to the one computed in the real dataset, when compared to MICE-LR with attributes ordered in a descending manner. Our hypothesis is that by positioning the attributes with a smaller number of levels first, the initial classification problems are easier to solve and will possibly better capture the dependence among the attributes, and this improved performance will be carried over to the subsequent attributes. This is similar to the idea of curriculum learning [ 53 ].
Overall, CLGP presents the best data utility performance on the larget-set , consistently capturing dependence among variables (low PCD and CrCls close to one), and producing synthetic data that matches the distribution of the real data (low log-cluster). CLGP also has the best support coverage, meaning that all the existent categories in the real data also appear in the synthetic data. On the other extreme, MC-MedGAN was clearly unable to extract the statistical properties from the real data. As expected, IM also showed poor performance due to its lack of variables’ dependence modeling.
As observed in the small-set variable selection, MC-MedGAN performed poorly on CrCl-SR metric compared to CrCl-RS (Fig. 15 ) and only covered a small part of the variables’ support in the real dataset. From Fig. 16 a and b we note that a subset of variables are responsible for MC-MedGAN’s poor performance on CrCl-SR and CrCl-RS. Figure 16 b also indicates that MICE-LR-based generators struggled to properly generate synthetic data for some variables. We also highlight the surprisingly good results obtained by BN on CrCl-RS and CrCl-SR metrics, considering the fact that BN approximates the joint distribution using a simple first-order dependency tree.
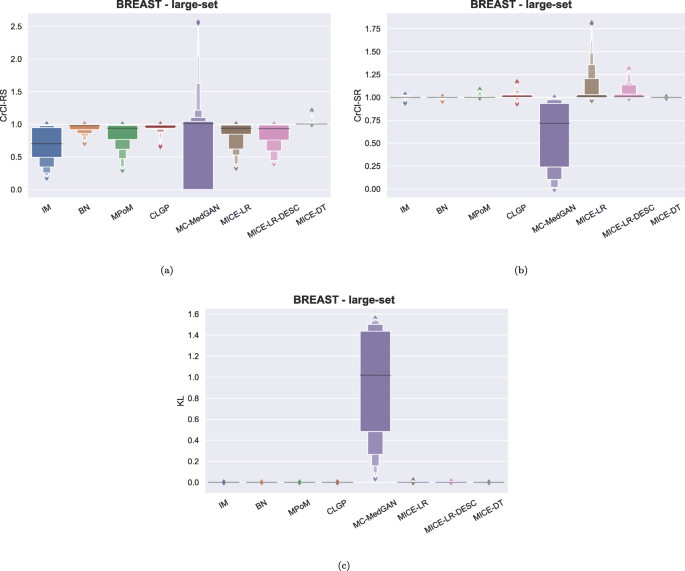
Data utility performance shown as boxplots on BREAST large-set
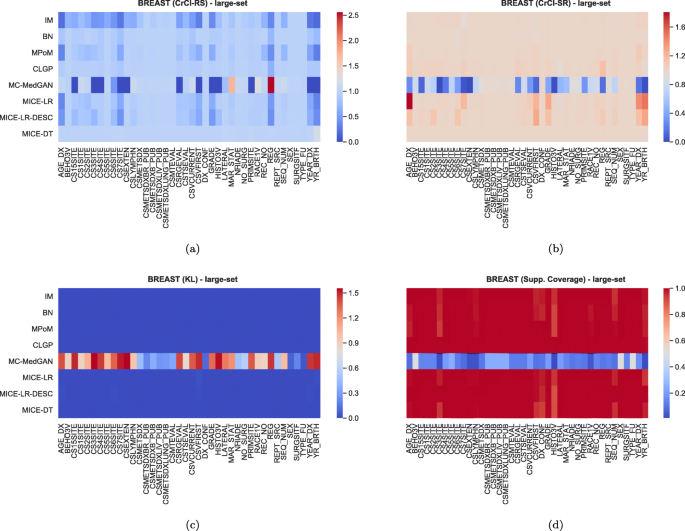
Heatmaps displaying the average over 10 independently generate synthetic datasets of ( a ) CrCl-RS, ( b ) CrCl-SR, ( c ) KL divergence, and ( d ) support coverage, at a variable level on BREAST large-set
Figure 17 shows the attribute disclosure for the BREAST large-set dataset for several numbers of nearest neighbors ( k ) and three different scenarios: when the attacker seeks to uncover 10, 6, and 3 unknown attributes, assuming she/he has access to the remaining attributes in the dataset. Overall, all methods but MC-MedGAN revealed almost 100% of the cases for values of k =1, when 3 attributes are unknown, but decrease to about 50% when 10 attributes are unknown. Clearly, MC-MedGAN has the best attribute disclosure as a low percentage of the unknown attributes of the real records are revealed. However, MC-MedGAN produces synthetic data with poor data utility performance, indicating that the synthetically generated data does not carry the statistical properties of the real dataset. MC-MedGAN relies on continuous embeddings of categorical data obtained via an autoencoder. We believe that the complexity and noisiness of the SEER data makes learning continuous embeddings of the categorical variables (while preserving their statistical relationships) very difficult. In fact, recent work [ 54 ] has shown that autoencoders can induce a barrier to the learning process, as the GAN will completely rely on the embeddings learned by the autoencoder. Additionally, works such as [ 55 ] have reported that while GANs often produce high quality synthetic data (for example realistic looking synthetic images), with respect to utility metrics such as classification accuracy they often underperform compared to likelihood based models. IM has the second best attribute disclosure, more pronounced for k >1, but as already seen, also fails to capture the variables’ dependencies. The best data utility performing methods (MICE-DT, MPoM, and CLGP) present a high attribute disclosure.
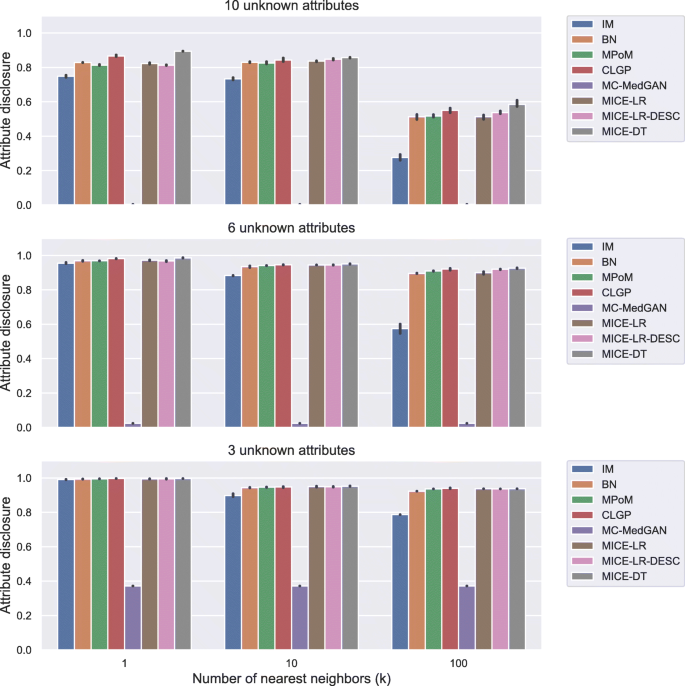
Attribute disclosure for several values of nearest neighbors (k). BREAST large-set . Results show attribute disclosure for the case an attacker seeks to infer 10, 6, and 3 unknown attributes, assuming she/he has access to the remaining attributes in the dataset
For membership disclosure, Fig. 18 , we notice that for exact match (Hamming distance 0), some of the methods have a high membership disclosure precision, indicating that from the set of patient records an attacker claimed to be present in the training set, a high percentage of them (around 90% for MICE-DT) were correct (high precision). However, there were many true records that the attacker inferred as negative (false negative). This can be seen by the lower recall values. A conservative attacker can be successful here for MICE-DT’s synthetic dataset. As discussed previously, MICE-DT is a more flexible model that provides a high data utility performance, but is more prone to release private information in the synthetic dataset. For Hamming distances larger than 6, the attacker claims true for all patient records, as the Hamming distance is large enough to always have at least one synthetic sample within the distance threshold. It is worth mentioning that it is hard for an attacker to easily identify the optimal Hamming distance to be used to maximize its utility, except if the attacker has a priori access to two sets of patients records, one of which is present in the training set and the other is absent from the training set.
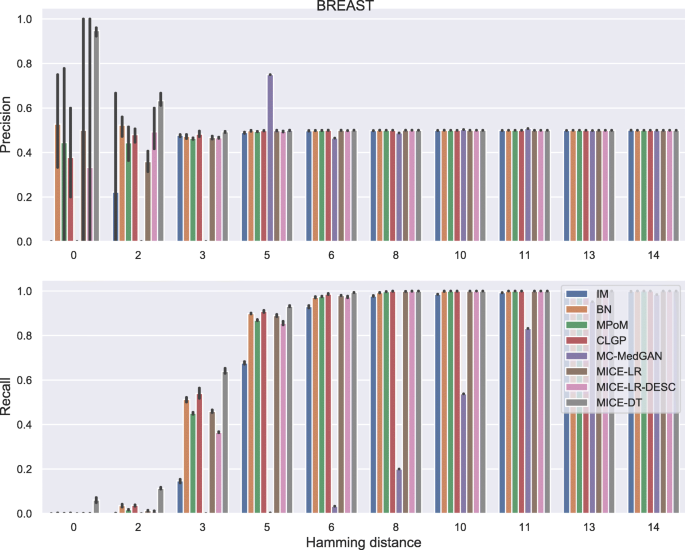
Precision and recall of membership disclosure for all methods varying the Hamming distance threshold. BREAST large-set
Tables 9 and 10 report performance of the methods on LYMYLEUK and RESPIR datasets using the large-set selection of variables. Figures 19 , 20 , 21 , 22 , 23 , 24 , 25 , and 26 present utility and privacy methods’ performance plots for the LYMYLEUK and RESPIR large-set datasets.
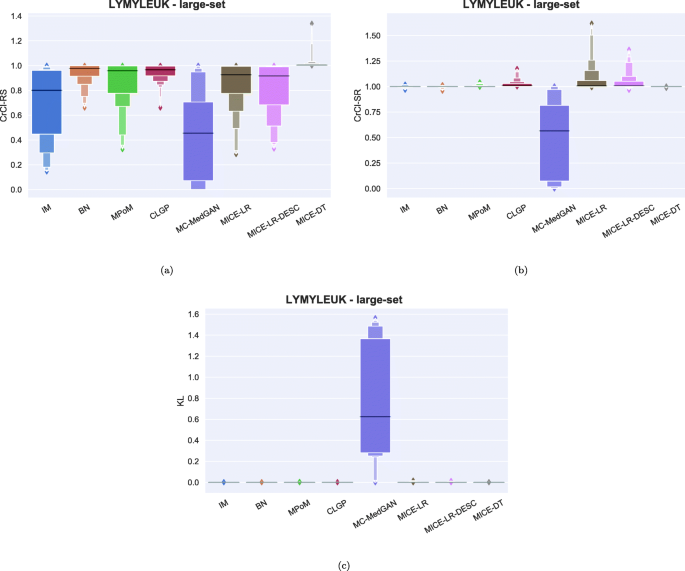
Data utility metrics shown as boxplots on LYMYLEUK large-set
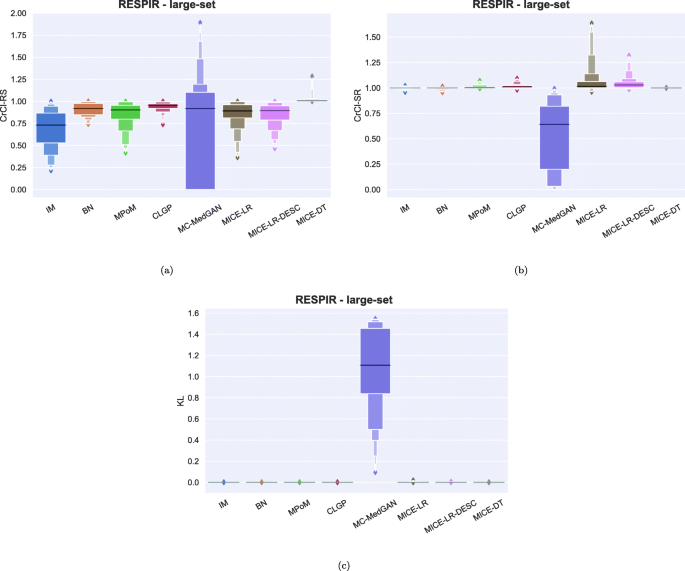
Data utility metrics shown as boxplots on RESPIR large-set
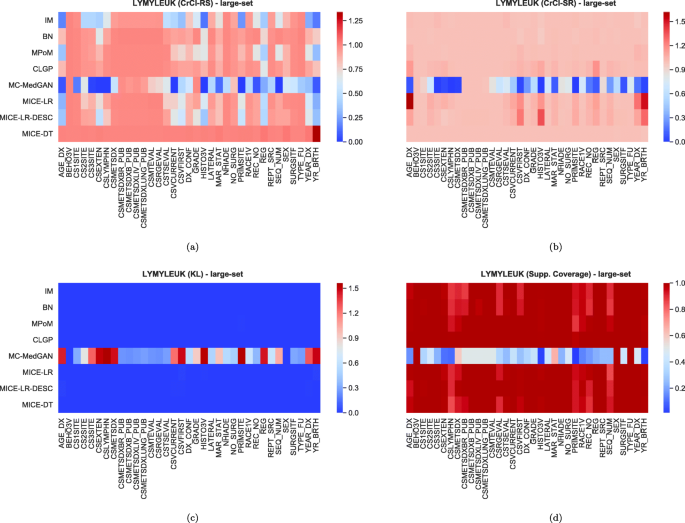
Heatmaps displaying ( a ) CrCl-RS, ( b ) CrCl-SR, ( c ) KL divergence, and ( d ) support coverage average over 10 independently generated synthetic datasets. LYMYLEUK large-set
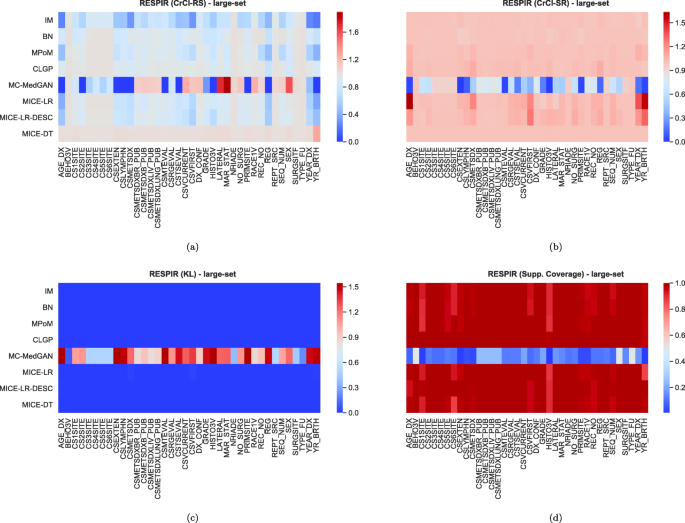
Heatmaps displaying ( a ) CrCl-RS, ( b ) CrCl-SR, ( c ) KL divergence, and ( d ) support coverage average over 10 independently generated synthetic datasets. RESPIR large-set
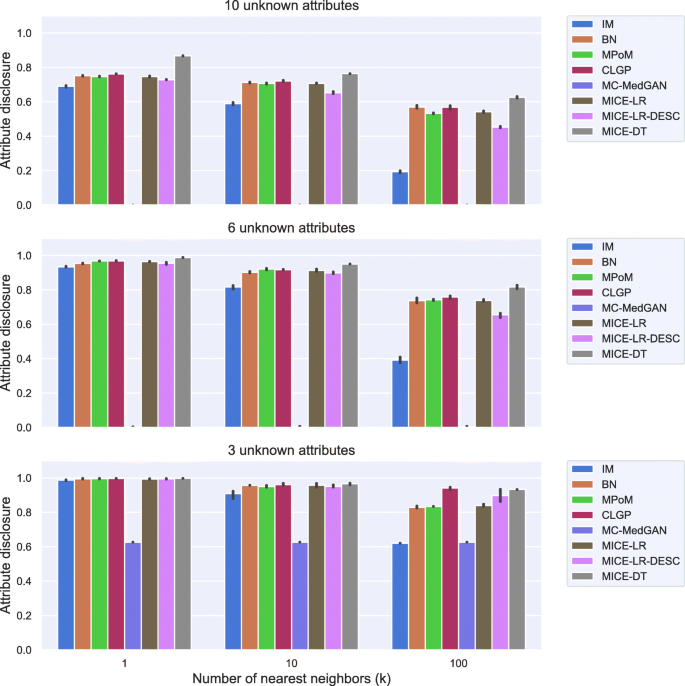
Attribute disclosure for LYMYLEUK large-set for the case 10, 6, and 3 attributes are unknown to the attacker
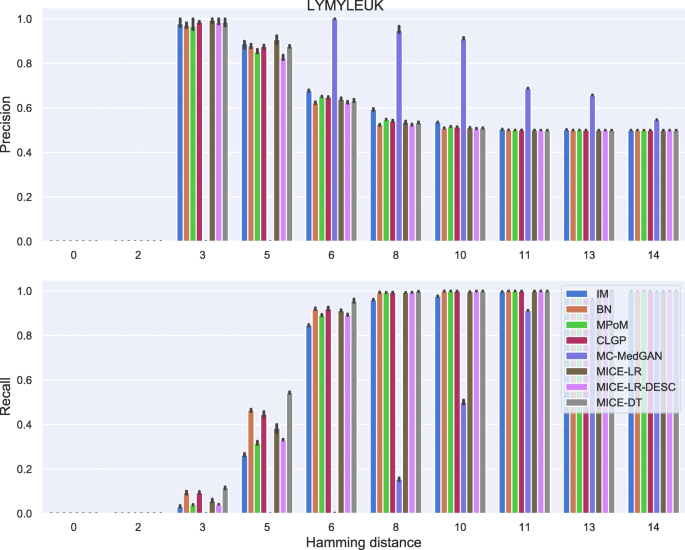
Precision and recall for membership disclosure for LYMYLEUK large-set
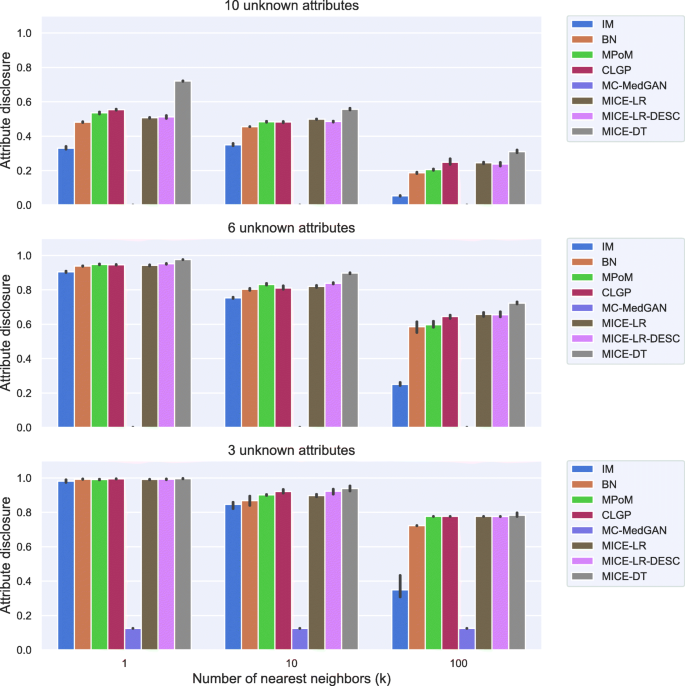
Attribute disclosure for RESPIR large-set for the case 10, 6, and 3 attributes are unknown to the attacker
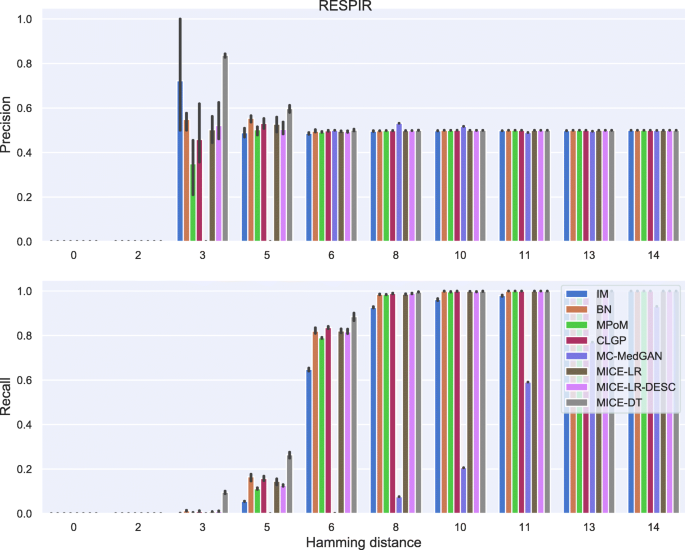
Precision and recall for membership disclosure for RESPIR large-set
Effect of varying synthetic data sample sizes on the evaluation metrics
The size of the synthetic dataset has an impact on the evaluation metrics, especially on the privacy metrics. For example, a membership attack may be more difficult if only a small synthetic sample size is provided. To assess the impact of the synthetic data sample size on the evaluation metrics, we performed experiments with different sample sizes of BREAST simulated data: 5,000; 10,000; and 20,000 samples. As a reference, the results provided so far have considered a synthetic sample dataset of the same size as the real dataset, which is approximately 170,000 samples for BREAST.
Table 11 presents the log-cluster, attribute disclosure, and membership disclosure performance metrics for varying sizes of synthetic BREAST small-set datasets. We observe an improvement (reduction) of the log-cluster performance with an increase in the size of the synthetic data. A significant reduction is seen for MPoM, BN, and all MICE variations. This is likely due to the fact that with an increase in the size of the synthetic dataset, a better estimate of the synthetic data distribution is obtained. Models with lower utility metrics, such as IM and MC-MedGAN, do not show large differences in performance over the range of 5,000 to 170,000 synthetic samples. Similar behavior to log-cluster was also observed for the other utility metrics, which are omitted for the sake of brevity.
The impact of sample size on the privacy metrics on the BREAST small-set are shown in Tables 12 , and 13 . For attribute disclosure (Table 11 ), we note that for the majority of the models a smaller impact on the privacy metric is observed when a larger k (number of nearest samples) is selected. For k =1, flexible models such as BN, MPoM and all MICE variations show a more than 10% increase in attribute disclosure over the range of 5000 to 170,000 synthetic samples. CLGP is more robust to the sample size, increasing only by 3%. In terms of membership disclosure (Table 13 ), precision is not affected by the synthetic sample size, while recall increases as more data is available. All models show an increase of 10% in recall over the range of 5,000 to 170,000 samples. This increase can be attributed to the higher probability of observing a similar real patient to a synthetic patient, as more patient samples are drawn from the synthetic data model.
We also ran similar experiments for the large-set with 40 attributes. The results are shown in Tables 14 , 15 , and 16 . Similar conclusions as those drawn for the small-set may be drawn for the large-set .
Running time and computational complexity
Figure 27 shows the training time for each method on the small-set and large-set of variables. For the range of models evaluated in this paper, the training times run from a few minutes to several days. This is primarily due to the diversity of the approaches and inferences considered in this paper. For MPoM, we performed fully Bayesian inference which involves running MCMC chains to obtain posterior samples, which is inherently costly. For CLGP, we performed approximate Bayesian inference (variational Bayes) which is computationally light compared to MCMC, however, inversion of the covariance matrix in Gaussian processes is the primary computational bottle-neck. The computation complexity of MC-MedGAN is primarily due to increased training time requirements for achieving convergence of the generator and the discriminator. The remaining approaches considered in this paper are primarily frequentist approaches based on optimization with no major computational bottle-necks. However, for the generation of synthetic datasets, the computational running time is not utterly important, since the models may be trained off-line on the real dataset for a considerable amount of time, and the final generated synthetic dataset can be distributed for public access. It is far more important that the synthetic dataset captures the structure and statistics of the real dataset, such that inferences obtained on the synthetic dataset closely reflects those obtained on the real dataset.
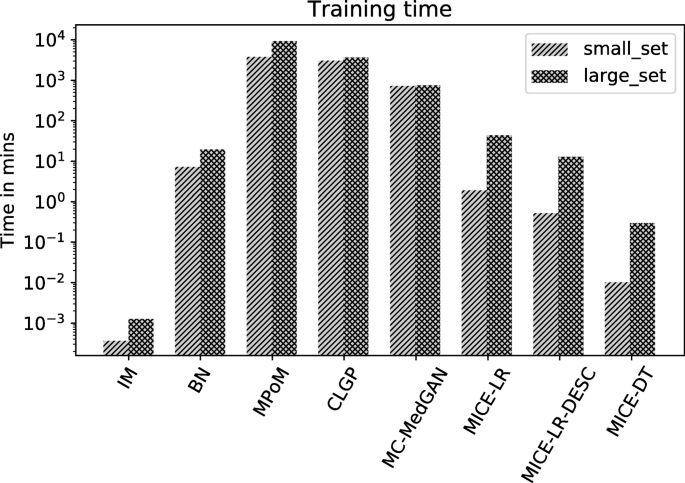
Training time in minutes for all methods on BREAST dataset considering both small-set and large-set
Edit checks
The SEER program developed a validation logic, known as “edits”, to test the quality of data fields. The SEER edits are executed as part of cancer data collection processes. Edits trigger manual reviews of unusual values and conflicting data items. The SEER edits are publicly available in a Java validation engine developed by Information Management Services, Inc. (software Footnote 2 ). All SEER data released to the public passes edits as well as several other quality measures.
There are approximately 1,400 SEER edits that check for inconsistencies in data items. The edit checks are basically if-then-else rules designed by data standard setters. Rules are implemented as small pieces of logic; each edit returns a Boolean value (true if the edit passes, false if it fails). For example, the edit that checks for inconsistent combinations of “Behavior" and “Diagnostic Confirmation" variables is represented as: “ If Behavior Code ICD-O-3[523] = 2 (in situ), Diagnostic Confirmation[490] must be 1,2 or 4 (microscopic confirmation) ".
Our purpose for using this software is to show that despite not explicitly encoding for these rules, they are implicit in the real data used to train the models (since that data passed these checks) and the models are able to generate data that for the most part does not conflict with these rules.
We ran the validation software on 10,000 synthetic BREAST samples and the percentage of records that failed in at least one of the 1400 edit checks are presented in Table 17 . All methods showed less than 1% of failures on the 10 variables set. As expected, IM has the largest number of failures, as it does not take variables dependence into account when sampling synthetic data. MICE-DT, MPoM, and BN performed best. On the larger set, 40 variables, MC-MedGAN and MICE-DT show less than 1% of failures. However, as previously discussed, these two methods provided samples with high disclosure probability, and also MC-MedGAN failed to capture statistical properties of the data. We also observe that BN presented less than 2% of failed samples. Results for LYMYLEUK and RESPIR are not presented in the paper, as some information required by the validation software is not available in the public (research) version of the SEER data.
It is also worth mentioning that, in practice, synthetically generated cancer cases that failed to pass at least one edit check may simply be excluded from the final list of cases to be released.
High quality synthetic data can be a valuable resource for, among other things, accelerating research. There are two opposing facets to high quality synthetic data. On one hand, the synthetic data must capture the relationships across the various features in the real population. On the other hand, the privacy of the subjects included in the real data must not be disclosed in the synthetic data. Here, we have presented a comparative study of different methods for generating categorical synthetic data, evaluating each of them under a variety of metrics that assess both aspects described above: data utility and privacy disclosure. Each metric evaluates a slightly different aspect of the data utility or disclosure. While there is some redundancy among them, we believe that in combination, they provide a more complete assessment of the quality of the synthetic data. For each method and each metric, we provided a brief discussion on their strengths and shortcomings, and hope that this discussion can be helpful in guiding researchers in identifying the most suitable approach for generating synthetic data for their specific application.
The experimental analysis was performed on data from the SEER research database on 1) breast, 2) lymphoma and leukemia, and 3) respiratory cancer cases diagnosed from 2010 to 2015. Additionally, we performed the same experiments on two sets of categorical variables in order to compare the methods under two challenge levels. Specifically, in the first set, 8 variables were included such that the maximum number of levels (i.e., number of unique possible values for the feature) was limited to 14. The larger feature set encompassed 40 features, including features with up to over 200 levels. Increasing the number of features and the number of levels per features results in a substantially larger parameter space to infer, which is aggravated by the absence or limited number of samples representing each possible combination.
From the experimental results on the two datasets of distinct complexity, small-set and large-set , we highlight the key differences:
The small-set records have fewer and less complex variables (in terms of the number of sub-categories per variable) than the large-set . Thus the learning problem is considerably easier and this is observed in the metric CrCl-RS provided in Tables 5 and 8 , where the small-set performs consistently better than the large-set across all datasets (BREAST, LYMYLEUK, and RESPIR).
SEER edit checks consist of a set of rules combined via various logical operators. For the large-set , the rules are significantly more complex and the chances of failure are higher. This is observed in Table 17 , where the percentage of failure is higher for the large-set compared to the small-set , across all methods.
As the dimensionality (as well as complexity, as some of the variables have a larger number of sub-categories) of the records in the large-set is considerably higher than the records in the small-set , in general, it is harder for an attacker to identify the real patient records used for model training. This is observed in Fig. 18 , where to achieve similar recall values for the membership attacks, the Hamming neighborhood has to be considerably larger for the large-set compared to the small-set .
The results showed that Bayesian Networks, Mixture of Product of Multinomials (MPoM) and CLGP were capable of capturing variables relationships, considering the data utility metrics used for comparison. Surprisingly, the generative adversarial network-based model MC-MedGAN failed to generate data with similar statistical characteristics to the real dataset.
In this paper, we presented a thorough comparison of existing methodologies to generate synthetic electronic health records (EHR). For each method, the process is as follows: given a set of private and real EHR samples, fit a model, and then generate new synthetic EHR samples from the learned model. By learning from real EHR samples, it is expected that the model is capable of extracting relevant statistical properties of the data.
From the performed experimental analysis, we observed that there is no single method that outperforms the others in all considered metrics. However, a few methods have shown the potential to be of great use in practice as they provide synthetic EHR samples with the following two characteristics: 1) statistical properties of the synthetic data are equivalent to the ones in the private real data, and 2) private information leakage from the model is not significant. In particular, we highlight the methods Mixture of Product of Multinomials (MPoM) and categorical latent Gaussian process (CLGP). Other methods, such as the Generative Adversarial Network (GAN), were not capable of generating realistic EHR samples.
Future research directions include handling variable types other than categorical, specifically continuous and ordinal. A more in-depth investigation of the limitations of GANs for medical synthetic data generation is also required.
Availability of data and materials
The SEER data is publicly available, and can be requested at https://seer.cancer.gov/data/access.html . Python code for the methods and metrics described here will be made available upon request.
https://seer.cancer.gov/data/
https://github.com/imsweb/validation
Abbreviations
Cross classification
Deep neural networks
Electronic health records
Generative adversarial network
Independent marginals
Kullback-Liebler
Multiple imputation by chained equations
Machine learning
Pairwise correlation difference
Statistical disclosure control
Statistical disclosure limitation
Surveillance, epidemiology, and end results program
Ursin G, Sen S, Mottu J-M, Nygård M. Protecting privacy in large datasets—first we assess the risk; then we fuzzy the data. Cancer Epidemiol Prev Biomark. 2017; 26(8):1219–24.
Article Google Scholar
El Emam K, Jonker E, Arbuckle L, Malin B. A systematic review of re-identification attacks on health data. PLoS ONE. 2011; 6(12):1–12. https://doi.org/10.1371/journal.pone.0028071 .
Article CAS Google Scholar
Rubin D. B.Discussion: Statistical disclosure limitation. J Off Stat. 1993; 9(2):461–8.
Google Scholar
Drechsler J.Synthetic Datasets for Statistical Disclosure Control: Theory and Implementation. Lecture notes in statistics, vol. 201. New York: Springer; 2011.
Book Google Scholar
Howe B, Stoyanovich J, Ping H, Herman B, Gee M. Synthetic Data for Social Good. In: Bloomberg Data for Good Exchange Conference: 2017. p. 1–8.
Kim J, Glide-Hurst C, Doemer A, Wen N, Movsas B, Chetty IJ. Implementation of a novel algorithm for generating synthetic ct images from magnetic resonance imaging data sets for prostate cancer radiation therapy. Int J Radiat Oncol Biol Phys. 2015; 91(1):39–47. https://doi.org/10.1016/j.ijrobp.2014.09.015 .
Walonoski J, Kramer M, Nichols J, Quina A, Moesel C, Hall D, Duffett C, Dube K, Gallagher T, McLachlan S. Synthea: An approach, method, and software mechanism for generating synthetic patients and the synthetic electronic health care record. J Am Med Inform Assoc. 2018; 25(3):230–8.
Dube K, Gallagher T. Approach and Method for Generating Realistic Synthetic Electronic Healthcare Records for Secondary Use. In: International Symposium on Foundations of Health Information Engineering and Systems. Springer: 2014. https://doi.org/10.1007/978-3-642-53956-5_6 .
Buczak AL, Babin S, Moniz L. Data-driven approach for creating synthetic electronic medical records. BMC Med Inform Decis Making. 2010; 10(1):59. https://doi.org/10.1186/1472-6947-10-59 .
Chen J, Chun D, Patel M, Chiang E, James J. The validity of synthetic clinical data: a validation study of a leading synthetic data generator (synthea) using clinical quality measures. BMC Med Inform Decis Making. 2019; 19(1):44.
Little RJA. Statistical analysis of masked data. J Off Stat. 1993; 9(2):407.
Matthews GJ, Harel O. Data confidentiality: A review of methods for statistical disclosure limitation and methods for assessing privacy. Stat Surv. 2011; 5(0):1–29.
Rubin DB. Multiple Imputation for Nonresponse in Surveys: Wiley; 1987. https://doi.org/10.1002/9780470316696 .
Raghunathan TE, Reiter JP, Rubin DB. Multiple imputation for statistical disclosure limitation. J Off Stat. 2003; 19:1–16.
Fienberg SE, Makov UE, Steele RJ. Disclosure Limitation Using Perturbation and Related Methods for Categorical Data. J Off Stat. 1998; 14(4):485–502.
Caiola G, Reiter JP. Random Forests for Generating Partially Synthetic, Categorical Data. Trans Data Priv. 2010; 3(1):27–42.
Loong B, Rubin DB. Multiply-Imputed Synthetic Data: Advice to the Imputer. J Off Stat. 2017; 33(4):1005–19.
Reiter JP, Drechsler J. Releasing Multiply-Imputed Synthetic Data Generated in Two Stages to Protect Confidentiality. Stat Sin. 2010; 20(1):405–21.
Chow C, Liu C. Approximating discrete probability distributions with dependence trees. IEEE Trans Inform Theory. 1968; 14(3):462–7.
Zhang J, Cormode G, Procopiuc CM, Srivastava D, Xiao X. PrivBayes: Private Data Release via Bayesian Networks. ACM Trans Database Syst. 2017; 42:1–41.
Gal Y, Chen Y, Ghahramani Z. Latent Gaussian processes for distribution estimation of multivariate categorical data. In: Int Conf Mach Learni: 2015. p. 645–54.
Dunson DB, Xing C. Nonparametric bayes modeling of multivariate categorical data. J Am Stat Assoc. 2009; 104(487):1042–51.
Perez L., Wang J.The effectiveness of data augmentation in image classification using deep learning. 2017:1–8. arXiv preprint arXiv:1712.04621.
Sankaranarayanan S, Balaji Y, Jain A, Nam Lim S, Chellappa R. Learning from synthetic data: Addressing domain shift for semantic segmentation. In: IEEE/CVF Conference on Computer Vision and Pattern Recognition. IEEE: 2018. https://doi.org/10.1109/cvpr.2018.00395 .
Tremblay J, Prakash A, Acuna D, Brophy M, Jampani V, Anil C, To T, Cameracci E, Boochoon S, Birchfield S. Training deep networks with synthetic data: Bridging the reality gap by domain randomization. In: 2018 IEEE/CVF Conference on Computer Vision and Pattern Recognition Workshops (CVPRW). IEEE: 2018. https://doi.org/10.1109/cvprw.2018.00143 .
Goodfellow I, Pouget-Abadie J, Mirza M, Xu B, Warde-Farley D, Ozair S, Courville A, Bengio Y. Generative adversarial nets. In: Neural Information Processing Systems: 2014. p. 2672–80.
Armanious K, Yang C, Fischer M, Kustner T, Nikolaou K, Gatidis S, Yang B. MedGAN: Medical Image Translation using GANs. CoRR. 2018; abs/1806.06397:1–16.
Camino R, Hammerschmidt C, State R. Generating multi-categorical samples with generative adversarial networks. In: ICML 2018 Workshop on Theoretical Foundations and Applications of Deep Generative Models: 2018. p. 1–7.
Choi E, Biswal S, Malin B, Duke J, Stewart WF, Sun J. Generating multi-label discrete patient records using generative adversarial networks. In: Machine Learning for Healthcare Conference: 2017. p. 286–305.
Nowok B, Raab G, Dibben C. synthpop: Bespoke Creation of Synthetic Data in R. J Stat Softw Artic. 2016; 74(11):1–26.
Templ M, Meindl B, Kowarik A, Dupriez O. Simulation of Synthetic Complex Data: The R Package simPop. J Stat Softw Artic. 2017; 79(10):1–38.
Mirza M, Osindero S. Conditional generative adversarial nets. 2014:1–7. arXiv preprint arXiv:1411.1784.
Reed S, Akata Z, Yan X, Logeswaran L, Schiele B, Lee H. Generative Adversarial Text to Image Synthesis In: Balcan MF, Weinberger KQ, editors. International Conference on Machine Learning, vol. 48: 2016. p. 1060–9.
Zhang Y, Gan Z, Fan K, Chen Z, Henao R, Shen D, Carin L. Adversarial feature matching for text generation. In: International Conference on Machine Learning: 2017. p. 4006–15.
Arjovsky M, Chintala S, Bottou L. Wasserstein gan. 2017. arXiv preprint arXiv:1701.07875.
Gulrajani I, Ahmed F, Arjovsky M, Dumoulin V, Courville AC. Improved training of wasserstein gans. In: Advances in Neural Information Processing Systems: 2017. p. 5767–77.
Azur MJ, Stuart EA, Frangakis C, Leaf PJ. Multiple imputation by chained equations: what is it and how does it work?. Int J Methods Psychiatr Res. 2011; 20(1):40–9.
Purdam K, Elliot MJ. A Case Study of the Impact of Statistical Disclosure Control on a Data Quality in the Individual UK Samples of Anonymised Records. Environ Plan A. 2007; 39(5):1101–18.
Woo M. -J., Reiter J. P., Oganian A., Karr A. F.Global Measures of Data Utility for Microdata Masked for Disclosure Limitation. J Priv Confidentiality. 2009; 1(1):111–24.
Dwork C., Roth A., et al. The algorithmic foundations of differential privacy. Found Trends Ⓡ Theor Comput Sci. 2014; 9(3–4):211–407.
McClure D, Reiter JP. Differential privacy and statistical disclosure risk measures: An investigation with binary synthetic data. Trans Data Priv. 2012; 5(3):535–52.
Charest A-S. How can we analyze differentially-private synthetic datasets?J Priv Confidentiality. 2011;2(2).
Xiao X, Wang G, Gehrke J. Differential privacy via wavelet transforms. IEEE Trans knowl Data Eng. 2010; 23(8):1200–14.
Dwork C, Rothblum GN, Vadhan S. Boosting and differential privacy. In: 2010 IEEE 51st Annual Symposium on Foundations of Computer Science. IEEE: 2010. p. 51–60.
Abadi M, Chu A, Goodfellow I, McMahan HB, Mironov I, Talwar K, Zhang L. Deep learning with differential privacy. In: Proceedings of the 2016 ACM SIGSAC Conference on Computer and Communications Security - CCS’16. ACM: 2016. p. 308–18. https://doi.org/10.1145/2976749.2978318 .
Xie L, Lin K, Wang S, Wang F, Zhou J. Differentially private generative adversarial network. arXiv preprint arXiv:1802.06739. 2018.
CLGP code. https://github.com/yaringal/CLGP . Accessed 12 Oct 2019.
MC-MedGAN code. https://github.com/rcamino/multi-categorical-gans . Accessed 12 Oct 2019.
pomegranate Python package. https://pomegranate.readthedocs.io/en/latest/ . Accessed 12 Oct 2019.
libpgm Python package. https://pythonhosted.org/libpgm/ . Accessed 12 Oct 2019.
Salimans T, Goodfellow I, Zaremba W, Cheung V, Radford A, Chen X. Improved techniques for training gans. In: Advances in Neural Information Processing Systems: 2016. p. 2234–42.
Metz L, Poole B, Pfau D, Sohl-Dickstein J. Unrolled generative adversarial networks. In: International Conference on Representation Learning: 2016. p. 1–25.
Bengio Y, Louradour J, Collobert R, Weston J. Curriculum learning. In: Proceedings of the 26th Annual International Conference on Machine Learning. ACM: 2009. p. 41–48.
Zhang Z, Yan C, Mesa DA, Sun J, Malin BA. Ensuring electronic medical record simulation through better training, modeling, and evaluation. J Am Med Inform Assoc. 2019; 27(1):99–108.
Ravuri S, Vinyals O. Classification accuracy score for conditional generative models. 2019. arXiv preprint arXiv:1905.10887.
Download references
Acknowledgements
This work is part of a larger effort. We thank our collaborators for their comments and suggestions along the way: Lynne Penberthy and the National Cancer Institute team, Gina Tourassi and the Oak Ridge National Laboratory team, and Tanmoy Bhattacharya and the Los Alamos National Laboratory team.
This work has been supported by the Joint Design of Advanced Computing Solutions for Cancer (JDACS4C) program established by the U.S. Department of Energy (DOE) and the National Cancer Institute (NCI) of the National Institutes of Health. This work was performed under the auspices of the U.S. Department of Energy by Argonne National Laboratory under Contract DE-AC02-06-CH11357, Lawrence Livermore National Laboratory under Contract DE-AC52-07NA27344, Los Alamos National Laboratory under Contract DE-AC5206NA25396, and Oak Ridge National Laboratory under Contract DE-AC05-00OR22725.
Our funding source had no impact on the design of the study, analysis, interpretation of data or in the writing of the manuscript.
Author information
Authors and affiliations.
Lawrence Livermore National Laboratory, 7000 East Ave, Livermore, CA, USA
Andre Goncalves, Priyadip Ray, Braden Soper & Ana Paula Sales
Information Management Systems, 1455 Research Blvd, Suite 315, Rockville, MD, USA
Jennifer Stevens & Linda Coyle
You can also search for this author in PubMed Google Scholar
Contributions
AS, PR, and BS conceptualized the study. JS and LC helped to prepare the data and provided guidance on the usage of the edit checks software. The experiments design was discussed by all authors. AG pre-processed the data, implemented the synthetic data generation methods, and performed all computational experiments. All authors contributed to the analysis of the results and the manuscript preparation. Final version was approved by all authors.
Corresponding author
Correspondence to Andre Goncalves .
Ethics declarations
Ethics approval and consent to participate.
Not applicable.
Consent for publication
Competing interests.
The authors declare that they have no competing interests.
Additional information
Publisher’s note.
Springer Nature remains neutral with regard to jurisdictional claims in published maps and institutional affiliations.
Rights and permissions
Open Access This article is licensed under a Creative Commons Attribution 4.0 International License, which permits use, sharing, adaptation, distribution and reproduction in any medium or format, as long as you give appropriate credit to the original author(s) and the source, provide a link to the Creative Commons licence, and indicate if changes were made. The images or other third party material in this article are included in the article’s Creative Commons licence, unless indicated otherwise in a credit line to the material. If material is not included in the article’s Creative Commons licence and your intended use is not permitted by statutory regulation or exceeds the permitted use, you will need to obtain permission directly from the copyright holder. To view a copy of this licence, visit http://creativecommons.org/licenses/by/4.0/ . The Creative Commons Public Domain Dedication waiver ( http://creativecommons.org/publicdomain/zero/1.0/ ) applies to the data made available in this article, unless otherwise stated in a credit line to the data.
Reprints and permissions
About this article
Cite this article.
Goncalves, A., Ray, P., Soper, B. et al. Generation and evaluation of synthetic patient data. BMC Med Res Methodol 20 , 108 (2020). https://doi.org/10.1186/s12874-020-00977-1
Download citation
Received : 29 July 2019
Accepted : 13 April 2020
Published : 07 May 2020
DOI : https://doi.org/10.1186/s12874-020-00977-1
Share this article
Anyone you share the following link with will be able to read this content:
Sorry, a shareable link is not currently available for this article.
Provided by the Springer Nature SharedIt content-sharing initiative
- Synthetic data generation
- Cancer patient data
- Information disclosure
- Generative models
BMC Medical Research Methodology
ISSN: 1471-2288
- General enquiries: [email protected]
- Open access
- Published: 15 December 2021
Secondary research use of personal medical data: patient attitudes towards data donation
- Gesine Richter 1 ,
- Christoph Borzikowsky 2 ,
- Bimba Franziska Hoyer 3 ,
- Matthias Laudes 4 &
- Michael Krawczak 2
BMC Medical Ethics volume 22 , Article number: 164 ( 2021 ) Cite this article
5246 Accesses
16 Citations
9 Altmetric
Metrics details
The SARS-CoV-2 pandemic has highlighted once more the great need for comprehensive access to, and uncomplicated use of, pre-existing patient data for medical research. Enabling secondary research-use of patient-data is a prerequisite for the efficient and sustainable promotion of translation and personalisation in medicine, and for the advancement of public-health. However, balancing the legitimate interests of scientists in broad and unrestricted data-access and the demand for individual autonomy, privacy and social justice is a great challenge for patient-based medical research.
We therefore conducted two questionnaire-based surveys among North-German outpatients (n = 650) to determine their attitude towards data-donation for medical research, implemented as an opt-out-process.
We observed a high level of acceptance (75.0%), the most powerful predictor of a positive attitude towards data-donation was the conviction that every citizen has a duty to contribute to the improvement of medical research (> 80% of participants approving data-donation). Interestingly, patients distinguished sharply between research inside and outside the EU, despite a general awareness that universities and public research institutions cooperate with commercial companies, willingness to allow use of donated data by the latter was very low (7.1% to 29.1%, depending upon location of company). The most popular measures among interviewees to counteract reservations against commercial data-use were regulation by law (61.4%), stipulating in the process that data are not sold or resold (84.6%). A majority requested control of both the use (46.8%) and the protection (41.5%) of the data by independent bodies.
Conclusions
In conclusion, data-donation for medical research, implemented as a combination of legal entitlement and easy-to-exercise-right to opt-out, was found to be widely supported by German patients and therefore warrants further consideration for a transposition into national law.
Peer Review reports
Personal medical data are generated and stored in a variety of contexts, from clinical routine and medical research, via government agencies and insurance companies, to health apps and social media. The secondary use of such pre-existing data can greatly improve the quality, fairness and efficiency of both clinical care and medical research. However, the current legal-ethical framework of the processing of personal data is more prohibitive than supportive in this regard in many countries, including several EU member states.
Undoubtedly, the ever-increasing efficiency of digital technologies bears great potential for abridging the way from primary data generation to secondary data use, but it also brings with it the responsibility to adapt these processes to an increasing demand for patient autonomy and privacy as well as for social justice. On the other hand, the SARS-CoV-2 pandemic has highlighted that enabling efficient access, integration and use of patient data is an indispensable prerequisite, not only for promoting translation and personalisation as the two major goals of medical research, but also for fostering public health with regard to disease monitoring, prevention and the evaluation of political measures. Balancing these concerns represents a great challenge for patient-based data-driven medical research.
Various programmes have been implemented in different countries to enable access to, and ensure the interoperability of, personal medical data for research (e.g. MyHealthRecord in Australia, www.myhealthrecord.gov.au ; FINDATA in Finland, www.findata.fi ). In most of these instances, data provision is legitimized by the prior informed consent of the data subjects. However, patient consent is mostly obtained in clinical care situations, which is problematic for a number of reasons.
Information and consent documents are usually handed out to patients during admission and alongside other documents relevant to their treatment. Moreover, for practicality reasons, information can realistically be given only in written form, with the help of other media, or orally by admission staff. However, even these options already entail a considerable demand of resources that is not easily met at large scale in routine care.
During the consent process, patients are usually awaiting a serious diagnosis or therapy. In such situations, weighing the pros and cons of the secondary research use of their data places a significant extra burden upon patients. Moreover, there is a risk that the temporal and spatial association of the consent process with clinical care measures leads to therapeutic [ 1 ] or diagnostic misconception [ 2 ].
It is inherently difficulty to create thorough understanding among patients of all aspects of the secondary research use of their medical data. At least in written form, this is rarely achievable within the time available, despite strong efforts to ensure readability and simple presentation [ 3 , 4 ].
There is generally great willingness among patients to contribute to medical research by way of sharing personal data, mainly out of altruism, solidarity and an idea of reciprocity [ 5 , 6 , 7 , 8 ]. At the same time, however, it is not easy for individuals to enable and control data access by researchers directly, which limits patient autonomy [ 9 ]. In view of these imbalances, the German Ethics Council recently brought into play the concept of ‘data donation’ to allow individuals to better facilitate research use of their medical data [ 10 , p. 266f]. Like others, the German Ethics Council understands data donation as consenting without temporal or factual restrictions provided that (a) the possible consequences of the data donation act are made sufficiently clear and (b) an appropriate infrastructure is in place to manage and protect the data.
First empirical studies have indicated that the great willingness among German patients to make personal data available for medical research may even extend to a model without explicit consent, if necessary. In fact, a representative survey of the German population revealed an approval rate of almost 80% to the donation of own medical data for medical research without attaching the donation act explicitly to prior consent [ 11 ].
The way in which decision-making for or against data donation is implemented (i.e. opt-in or opt-out) has major impact upon the scientific value of the data in question. With an opt-in model, participation rates can be expected to be rather low, leading to bias that may not only render the data useless for research but that may ultimately result in erroneous and, hence, potentially dangerous scientific conclusions. Moreover, some important types of patients could get lost to medical research in this scenario, including emergency cases as well as patients who are incapable of giving consent in the first place. Therefore, the German Federal Ministry of Health commissioned an expert opinion in 2019 on the current legal basis for data donation in Germany, designed so that it would exempt secondary data use for medical research from the requirement of informed prior consent and instead grants citizens an easy-to-exercise right to opt-out [ 12 ]. In their report to the Ministry, the authors concluded that such a framework would not only be compliant with the provisions of the EU General Data Protection Regulation (2016/679) but could also be made ethically acceptable through suitable outreach and trust-building measures.
To our knowledge, our study is the first in Germany to evaluate patient views of a mechanism of data donation that stipulates an opt-out scenario. In addition to assessing the level of acceptance of such a model, we also sought to explore further the negative attitude of patients towards the research use of their data by commercial companies, as identified in earlier studies [ 11 , 13 ], and how reservations against such use could be counteracted when implementing data donation in practice.
Materials and methods
Study participants.
Our study was conducted in the form of two questionnaire-based surveys, one to identify predictors of a positive attitude towards data donation (Survey 1), and one to verify and specify further these predictors (Survey 2) using a questionnaire built upon the results of Survey 1. The full texts of both questionnaires (in German) are provided in the Additional file 1 .
After approval by the local ethics committee, the two surveys were undertaken between May and November 2020, including 500 and 150 patients, respectively. Participants were approached in the waiting rooms of the Comprehensive Center for Inflammation Medicine (CCIM) and the joint Outpatient Center of the Departments of Internal Medicine and General Surgery (IMAC) at University Hospital Schleswig–Holstein (UKSH) Campus Kiel. The CCIM and IMAC patient populations adequately reflect the socio-demographic structure of all outpatients at UKSH Campus Kiel, and of the general population of the most northern part of Germany.
The questionnaire used in Survey 1 focussed upon the personal attitude of participants towards medical research (Section A) and data donation (Section B). Section A comprised 12 statement items addressing the understanding and personal views of medical research in general, including the potential duty to contribute to medical research and the role of commercial companies, among others. Answering options were formulated on either a three-graded Likert scale (1: “yes”, 2: “no”, 3: “unknown”) or a four-graded Likert scale (1: “fully agree”, 2: “rather agree”, 3: “rather disagree”, 4: “fully disagree”). Section B comprised (i) two four-graded Likert scale questions about the acceptance of data donation, (ii) five statement items concerning the possible implementation of data donation (site of data storage, rights-of-use, reservations against commercial data use, countermeasures against such reservations, context of consent decision-making), and (iii) two questions about whether the SARS-CoV-2 pandemic affected the participant’s attitude towards data donation and the research use of their data by commercial companies.
The questionnaire of Survey 2 was intended (i) to verify major results of Survey 1 and (ii) to address more specifically aspects of a potential practical implementation of data donation. It comprised two four-graded Likert scale questions about (i) the participant’s attitude towards legally permitting research use of pseudonymised medical data without prior consent (i.e. an opt-out implementation of data donation) and (ii) their agreement, or not, to a general civic duty to improve medical research (i.e. the main predictor of a positive attitude towards data donation identified in Survey 1). In addition, the two statement items in Survey 1 regarding storage site and rights-of-use were also included in Survey 2. However, the rights-of-use item was slightly refined so as to differentiate between use within and outside the EU (rather than within and outside Germany, as in Survey 1).
All statistical analyses were carried out with IBM SPSS Statistics for Windows [ 14 ]. The variables considered in our study were of categorical type (e.g., age class, agreement to a given statement) and were characterized by their absolute and relative class frequencies. Chi-squared (χ 2 ) or Fisher’s exact tests were used, as appropriate, to assess the statistically significance of frequency differences between groups of participants (e.g., proponents and opponents of data donation). Predictors of the approval, or otherwise, of data donation were identified by step-wise logistic regression analysis with forward selection, using a Wald test to ascertain non-zero regression coefficients. p values smaller than 0.05 were regarded as statistically significant.
Of the 500 questionnaires delivered in Survey 1, 376 (75.2%) were returned at a level of completeness sufficient for subsequent analysis. Of the 150 questionnaires delivered in Survey 2, 132 (88.0%) were returned in appropriate form. By and large, the two samples were representative of the general German population in terms of age and sex (Table 1 ), with one exception: The proportion of participants who did not rise above primary or secondary school level was significantly higher in Survey 2 (37.1%) than in Survey 1 (21.0%; χ 2 = 12.613, 1 df, p = 3.8 × 10 –4 ), with both percentages deviating by roughly the same amount from the 2019 nation-wide figure of 28.6% [ 15 ].
Acceptance of data donation
In Survey 1, the overall attitude towards data donation for medical research was ascertained by asking participants whether they would approve use of their data, free of charge and in compliance with pending data protection regulations, but without having to ask them for permission each time the data were used (question S1/Q5, Additional file 1 : Table S1). With this definition, the donation of medical data from own electronic health records (EHRs, e.g. examination results, anamneses, X-rays) was deemed acceptable by 296 participants (78.7%), who answered “Do fully agree” or “Do rather agree” to question S1/Q5 (henceforth referred to a s the ‘pro’ data donation subgroup), and was opposed by 80 participants (21.3%), who answered “Do rather disagree” or “Do fully disagree” (‘contra’ data donation; Fig. 1 a). With regard to the donation of self-acquired data, such as those generated by medical devices or mobile phones (S1/Q6), positive (n = 180, 47.8%) and negative attitudes (n = 196, 52.0%) were found to be balanced among participants (Fig. 1 b). Interestingly, a vast majority of participants reported that the SARS-CoV-2 pandemic had had no effect upon their attitude towards data donation (n = 289, 76.9%; S1/Q12).
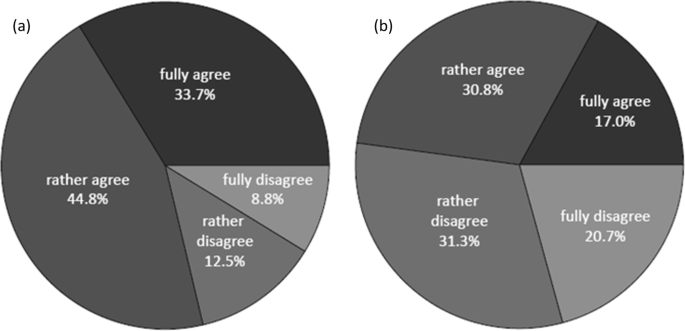
Attitude of participants in Survey 1 (n = 376) towards donation for medical research of a data from EHRs (S1/Q5) and b self-acquired medical data (S1/Q6). S1/Q5: “In the future, your personal health data will likely be stored in a digital health record. Would you agree that these data become available for medical research as a ‘data donation’, free of charge and in compliance with data protection laws, without asking for your permission prior to each use of the data?” S1/Q6: “Would you agree that data collected by yourself (e.g. via medical devices or mobile phones) are made available to medical research as a ‘data donation’, free of charge and in compliance with data protection regulations, without asking for your permission prior to each use of the data?”
For Survey 2, the question about the general attitude towards data donation (S2/Q3) was modified so as to address the strong demand expressed in Survey 1 for a legal regulation of the donation process (see below). The revised wording therefore implied that data donation comprised a legal entitlement of researchers to use patient data without prior consent, but that the entitlement was combined with a simple opt-out for patients. Even under these more liberal conditions, the rate of acceptance of data donation (n = 99, 75.0%; Fig. 2 , Additional file 1 : Table S2) was almost as high as in Survey 1. In both surveys, no correlation was observed between gender, age or educational level and the participants’ attitude towards data donation (Table 1 ).
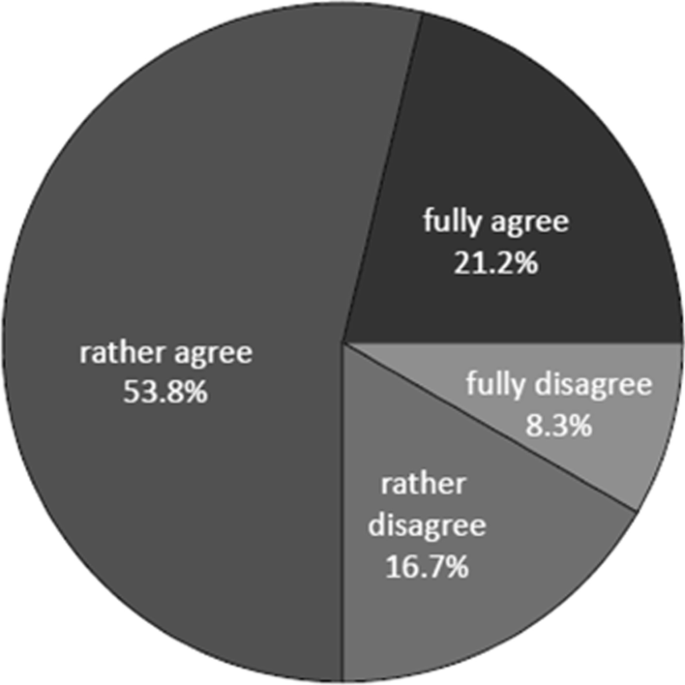
Attitude of participants in Survey 2 (n = 132) towards donation for medical research, implemented as an opt-out process (S2/Q3). S2/Q3: “There are currently considerations in Germany to legally allow medical research on pseudonymised data without the prior consent of patients, unless the patient objects to such use. The objection should be as simple as possible, e.g. possible to assert when visiting a doctor or a pharmacy. Would you agree to such a regulation?”
General understanding of medical research (Survey 1)
In Survey 1, participants were first asked about their general understanding of medical research (Additional file 1 : Table S1, S1/Q3). A vast majority agreed that data from routine clinical care can be useful for research (n = 322, 85.6%). When asked whether a legal entitlement, if any, to the research use of such data without prior consent of the patient was limited to the treating physicians, a majority rightly thought that this was the case (n = 209, 55.6%). Interestingly, such a ‘privilege of own research’ is a reality in almost all German federal states (‘Länder’), albeit not in Schleswig–Holstein where our study was carried out. A majority still was rightly aware that universities cooperate with commercial companies in medical research (n = 222, 59.0%), and that conclusions about individual patients cannot easily be drawn from scientific publications (n = 226, 60.1%). However, more than 20% of the participants found themselves unable to answer the latter two questions in the first place, with a somewhat higher level of indecisiveness noted in the ‘contra’ subgroup (32.5%, 31.3%) than in the ‘pro’ subgroup (29.1%, 20.3%). Even greater uncertainty prevailed about whether medical data that are used in research can be traced back to patients. Here, all three answering options (“yes”, “no”, “unknown”) were chosen with roughly equal frequency. Notably, a significantly higher proportion of participants in the ‘pro’ subgroup (n = 135, 45.6%) than in the ‘contra’ subgroup (n = 19, 23.8%; χ 2 = 11.555, 1 df, p = 6.8 × 10 –4 ) was aware of the fact that data from German patients may also be used for research abroad.
Personal attitude towards medical research
Most participants in both the ‘pro’ and ‘contra’ subgroup of Survey 1 took the so-called ‘reciprocity’ position that patients who benefit from medical research should contribute to research themselves (Additional file 1 : Table S1, S1/Q4; pro: n = 267, 90.2%; contra: n = 64, 80.0%; χ 2 = 5.292, 1 df, p = 0.0214). A highly significant difference became apparent, however, when participants were asked for whether every citizen (diseased, or not) has a duty to contribute to the improvement of medical research. While a vast majority of those supporting data donation shared this view (n = 247, 83.4%), just over half the opponents either “fully” or “rather” agreed to the respective statement (n = 45, 56.3%; χ 2 = 25.307, 1 df, p < 10 –5 ). While both subgroups were found in Survey 1 to be quite indifferent towards the possible entitlement to personal benefits of patients involved in medical research (pro: n = 155, 52.4%; contra: n = 33, 41.3%, non-significant), supporters of data donation were significantly more positive about the availability of patient data for medical research to commercial companies (n = 167, 54.4%) than those opposing data donation (n = 20, 25.0%; χ 2 = 23.628, 1 df, p < 10 –5 ). Notably, a majority in both subgroups rejected the idea that outsiders such as self-help groups, churches or charities should be involved in deciding about the research use of patient data, with a significantly less negative attitude in this regard prevailing in the ‘pro’ subgroup (n = 161, 54.4%) than in the ‘contra’ subgroup (n = 59, 73.8%; χ 2 = 8.941, 1 df, p = 0.0028). Finally, a vast majority of both subgroups shared a demand for more public information on individual research projects (pro: n = 274, 92.6%; contra: n = 73, 91.3%, non-significant).
That the conviction of a civic duty to advance medical research is a strong predictor of the attitude towards data donation was confirmed in Survey 2 (Additional file 1 : Table S2, S2/Q4). There, a vast majority of 90 participants in the ‘pro’ subgroup (90.9%) agreed to the supposition of such a duty, compared to only 13 participants in the contra subgroup (39.4%; χ 2 = 35.368, 1 df, p < 10 –5 ).
Framework of data donation
Site of data storage.
In both surveys, most participants from the ‘pro’ subgroup favoured storage of their donated data in a nation-wide centralized database (S1/Q7: n = 214, 72.3%, Additional file 1 : Table S1; S2/Q5: n = 75, 75.8%, Additional file 1 : Table S2). The second most favourite option was storage exclusively at the site of original data acquisition (Survey 1: n = 131, 44.3%; Survey 2: n = 37, 37.4%). Long-term storage at the research institutions that use the data was found to be acceptable only to a minority of participants (Survey 1: n = 110, 37.2%, Survey 2: n = 23, 23.2%).
Rights-of-use
In both surveys, participants were asked who should be allowed research use of their donated data (Fig. 3 ). In Survey 1, the respective question (S1/Q8) aimed at differentiating between public and commercial research institutions as well as between institutions within and outside Germany. In order to account more adequately for the legal framework of data donation in the EU and the current practise of data sharing in medical research in general, however, we slightly modified this question in Survey 2 so as to distinguish between institutions inside and outside the EU (S2/Q6). The vast majority of patients in the ‘pro’ subgroup was willing to allow data use by universities and public research institutions in Germany (Survey 1: n = 284, 95.9%) and in the EU (Survey 2, n = 91, 91.9%). When data usage abroad was specified as meaning ‘outside the EU’ in Survey 2, only a minority was still approving data access by universities and public research institutions there (n = 24, 24.2%). Drastically fewer participants were willing to accept data access by commercial companies, irrespective of whether they were located in Germany (Survey 1: n = 86, 29.1%), inside the EU (Survey 2: n = 14, 14.1%), or outside the EU (Survey 2: n = 7, 7.1%). All reductions in approval, compared to universities and public research institutions, were statistically highly significant (p < 10 –5 ).
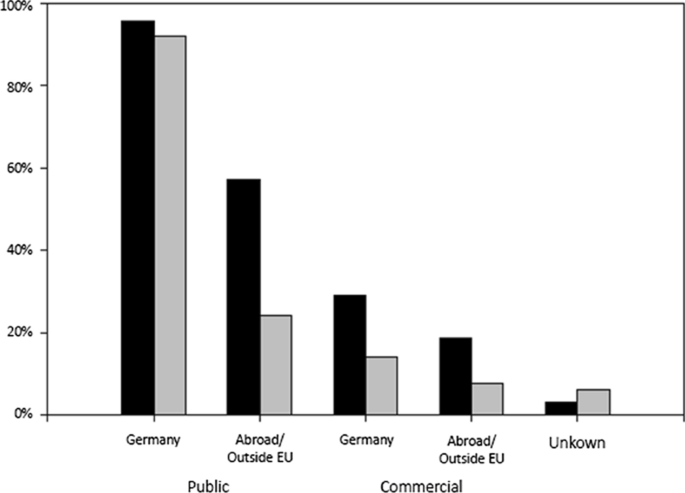
Rights-of-use granted by proponents of data donation in Survey 1 (S1/Q8; n = 296; black bars) and Survey 2 (S2/Q6; n = 99; grey bars). S1/Q8, S2/Q6: “Who should be allowed use of data donated for medical research (multiple answers possible)?”
Reservations against commercial data usage, and appropriate countermeasures
Previous research by ourselves [ 11 ] had unveiled reservations against the research use of personal medical data by commercial institutions. In Survey 1, we therefore asked patients who felt that they had such reservations in the first place, or who opposed data donation in general, for the precise nature of their reservations (n = 219, S1/Q9). The most frequently chosen items were fear of insufficient data protection by commercial companies (n = 150, 68.5%) and rejection of profit-making through the research use of patient data (n = 123, 56.2%). Doubt whether commercial companies would do research for the common good was less important (n = 86, 39.3%). This notwithstanding, there was an overwhelming acknowledgment among participants of the importance of commercial medical research, which was in fact questioned by only a small minority (n = 14, 6.4%).
Among the participants of Survey 1, the most popular measures to counteract potential reservations against the research use of patient data by commercial companies (S1/Q10) were an assurance that the data are not sold or resold (n = 318, 84.6%) and a legal regulation of the conditions for using the data (n = 231, 61.4%). A slightly smaller proportion of participants would have wished for research usage of the data to be controlled by an independent body (n = 176, 46.8%) and for the data protection measures taken by commercial companies to be monitored regularly and independently (n = 156, 41.5%). Other counter measures such as controlling data usage by state institutions (n = 127, 33.8%), the public availability of information on the data usage (n = 91, 24.2%), or a ban on data storage within the companies themselves (n = 88, 23.4%) were of minor importance.
Context of decision-making
The circumstances under which a patient is asked for their consent to the research use of their medical data is likely to impact upon both, the understanding of the information provided and the actual decision-making process. When participants of Survey 1 were asked about their preferences in this regard (S1/Q11), however, a majority answered that they would rather want to undergo the consent process as a patient in the clinic (n = 251, 66.8%). Only a minority wanted to make this decision outside the clinic (n = 50, 13.3%) or before they became ill at all (n = 56, 14.9%).
Predictors of attitude towards data donation
In Survey 1, logistic regression analysis with forward selection was carried out to formally identify predictors of a positive patient attitude towards data donation among the six possible responses to question S1/Q4 (“What is your personal attitude towards medical research?”; Additional file 1 : Table S1). The logistic regression analysis was adjusted for sex, age and highest level of education, but none of these covariates had a statistically significant influence upon the approval, or not, of data donation from EHRs (S1/Q5). In contrast, both an agreement to the civic duty to contribute to medical research (S1/Q4, item 2) and an approval of the research use of patient data by commercial companies (item 6) were significantly associated with a positive attitude (Table 2 ). The same predictors emerged for an approval of the donation of self-acquired medical data (S1/Q6), in addition to male sex which was interestingly found to be a positive predictor with an odds ratio of almost two (Table 2 ). Survey 2 was undertaken to determine more specifically the acceptance by patients of an opt-out implementation of data donation (S2/Q3), and the civic duty to support research (S2/Q4) was as an even stronger positive predictor here than in Survey 1 (Table 2 ). In contrast to Survey 1, however, agreement to the research use of donated data by commercial companies (implied by a positive response to item 3 or item 4, or both, of question S2/Q6) was not significantly associated with an approval of data donation.
Limitations
Influence of the sars-cov-2 pandemic.
The present study was undertaken between May and November 2020. Particularly during Survey 1, there was thus considerable uncertainty among patients about the pandemic-related conditions of their hospital stay. At the same time, medical research got more into the focus of public interest. Patients understood better that researchers in public and commercial institutions need to cooperate, both nationally and internationally, and the benefits of such cooperation for the public good became tangible by the rapid development of vaccines. Against this background, it cannot be excluded that the level of approval of data donation expressed in both surveys was influenced by the acute pandemic situation. However, when explicitly asked in Survey 1 for the possibility of a change of mind due to the pandemic (S1/Q11-Q12; Additional file 1 : Table S1), a majority of patients in both the ‘pro’ and the ‘contra’ subgroup claimed that the pandemic had had no significant influence upon their attitudes, neither towards data donation (n = 289, 76.9%) nor towards the research use of medical data by commercial research (n = 301, 80.1%). This notwithstanding, an unconscious influence cannot be completely ruled out.
Survey setting
Our study involved patients waiting for counselling or treatment in a UKSH outpatient unit. Therefore, it is possible that the answers given to question S1/Q11 about the preferred context of decision-making were biased by the setting of the survey itself. Indeed, to our surprise, a great majority in both subgroups (‘pro’ and ‘contra’) was found to be in favour of being asked for consent to the research use of their medical data in the clinic, and as a patient.
The SARS-CoV-2 pandemic has highlighted once more the great need for comprehensive access to, and uncomplicated use of, pre-existing patient data for medical research. Enabling the secondary use of such data is an indispensable prerequisite for the promotion of translation and personalisation in medicine and for the advancement of public health, none of which can be achieved by prospective studies alone. However, balancing the legitimate interests of scientists as well as current and future patients in broad and unrestricted data access for research on the one hand, and the demand for individual autonomy, privacy and social justice on the other, is a great challenge for patient-based medical research. Successfully meeting this task requires a broad understanding of benefits and risks that is both personal and societal [ 16 , p.122], a view that renders ‘data donation’, implemented as a legal entitlement to both, the use of data and the objection to such use, a potentially widely acceptable option in medical research.
Recent surveys undertaken against the backdrop of the SARS-CoV-2 pandemic revealed that a large majority of German citizens would be prepared to make personal health data available for research under the prevailing exceptional circumstances, including research by private companies [ 17 , 18 ]. Even before the pandemic, studies in various countries [ 19 , 20 , 21 ] demonstrated high willingness of patients and members of the general population to contribute personal data to medical research more generally. Not surprisingly then, initial considerations have gotten under way since then to create a legal basis in Germany for data donation in the manner described [ 12 , 22 , 23 ]. In fact, previous studies of ours [ 8 , 11 ] have suggested robust acceptance at the national level of data donation if understood as one of many possible forms of patient consent, but the envisaged legal and organizational concept was not differentiated in these surveys in much detail.
Our present study therefore aimed to determine the attitude of patients towards the specific design of data donation for medical research as an opt-out process. As a result, we observed a high level of acceptance, and by far the most powerful predictor of a positive attitude towards such a model was the conviction that every citizen (whether diseased or not) has a duty to contribute to the improvement of medical research. Notably, a recent study in the UK made very similar observations albeit for data donation as an opt-in process [ 24 ]. Nevertheless, the considerable agreement between the two studies suggests that the assumption, in Survey 2 of our study, of a legal stipulation did not particularly foster the view of participants who backed data donation that participation in the process were a civic duty.
Most patients included in our study were well aware that data from routine clinical care can be useful for medical research. Their high willingness to contribute such data to research was likely motivated by a so-called ‘reciprocity’ position which implies that patients who benefit from medical research should contribute to research themselves, an attitude encountered in many studies before [ 25 ]. The most prominent (potential) beneficiaries of such patient generosity were universities and public research institutions in Germany. Despite a general awareness that universities and public research institutions cooperate with commercial companies, willingness to allow use of the donated data by the latter was very low.
In view of this apparent contradiction, which agrees with results by other studies [ 26 , 27 , 28 , 29 , 30 ], we tried to identify the actual reasons for the observed reservations and to find out how they could be counteracted when designing a data donation process. According to Survey 1, the most frequent concerns are insufficient data protection by commercial companies and an objection of their profit-making through the use of the data. The view that commercial companies would not conduct research for the common good, as implied occasionally in the literature [ 28 , 31 ], played only a minor role in our study. Consequently, the most popular measure to counteract reservations on the side of patients was regulation of the commercial use of the data by law, stipulating in the process that the data are not sold or resold. Additionally, patients requested control of both the use and the protection of the data by independent bodies.
In their attitude towards data donation, patients distinguished sharply between research use of their data inside and outside the EU, particularly when commercial companies were involved. Since people in Germany have one of the highest levels in Europe of concern about data protection in general [ 32 ], this distinction most likely reflects greater trust in data protection measures taken inside than outside Europe, combined with the wide-spread mistrust in data protection by commercial institutions as alluded to above. This unwillingness may have been reinforced further by an inadequate perception of legal and administrative hurdles. In fact, the pros and cons of transferring personal data to outside the EU are likely unknown to most parts of the general population and, as was pointed out earlier, it is difficult to put an end to this ignorance under the conditions of current patient consent practice. Moreover, most people are likely unaware that commercial companies have good reason to work with patient data and are more than willing to benefit the original causes of data donation, for example, by transferring back their research results for use by others. In any case, if data donation is to be a success, it is the joint responsibility of politics and science to explain these aspects of sharing donated data better to citizens in order to reduce their potential fear of it.
A main motivation for contemplating, in Germany, data donation for medical research as a combination of legal entitlement and opt-out was the prospect of being able to separate the decision-making process of patients from a clinical care context [ 12 ]. This way, problems in comprehending the consent documents as well as possible therapeutic or diagnostic misconceptions could be avoided ‘by design’ through relaying the necessary information about data donation before the individuals concerned become ill and, hence, biased in terms of their personal perception and attitude. When preparing our study, we expected this prospective increase in fairness, associated with decoupling consent and medical treatment, to greatly appeal to participants. In contrast, however, a majority was found to wish to continue deciding about the research use of their data as patients in the clinic. Since it cannot be excluded that this observation reflects bias due to the survey context itself (see Limitations), additional studies outside the clinic seem well warranted in the future.
Our study strongly suggests that data donation for medical research, implemented as a combination of legal entitlement and an easy-to-exercise right to opt-out, is widely supported by German patients and therefore warrants further consideration for a transposition into national law. We observed that the most powerful predictor of a positive attitude towards data-donation is the conviction that every citizen is obliged to contribute to the improvement of medical research. Hence, endorsing and supporting such a sense of civic duty by way of better public outreach and stakeholder involvement may be a key for medical research to sustain the secondary use of patient data. Despite a general awareness that academic institutions cooperate with commercial companies, the willingness of participants in our study to allow use of their donated data by industry was found to be low. These reservations should be understood as a mandate to rethink the legal framework of the processing of personal medical data in research because it appears as if the reservation can be successfully counteracted by a strong legal ban on selling or reselling the data. In conclusion, we trust that our study will stimulate further the debate about the most efficient and, at the same time, ethically acceptable use of patient data for research purposes.
Availability of data and materials
Data supporting the results reported in the article can be found in the supplements added.
Appelbaum PS, Roth LH, Lidz CW, Benson P, Winslade W. False hopes and best data: consent to research and the therapeutic misconception. The Hastings Cen Rep. 1987;17(2):20–4.
Article Google Scholar
Nobile H, Vermeulen E, Thys K, Bergmann MM, Borry P. Why do participants enroll in population biobank studies? A systematic literature review. Expert Rev Mol Diagn. 2013;13(1):35–47.
D’Abramo F, Schildmann J, Vollmann J. Research participants’ perceptions and views on consent for biobank research: a review of empirical data and ethical analysis. BMC Med Ethics. 2015;16:60–70.
Richter G, Krawczak M, Lieb W, Wolff L, Buyx A. Broad consent for health care-embedded biobanking: understanding and reasons to donate in a large patient sample. Genet Med. 2018;20:76–82.
Aitken M, DeStJorre J, Pagiliari C, Jepson R, Cunningham-Burley S. Public responses to the sharing and linkage of health data for research purposes: a systematic review and thematic synthesis of qualitative studies. BMC Med Ethics. 2019;17(1):73.
Karampela M, Ouhbi S, Isomursu M. Connected health user willingness to share personal health data: questionnaire study. J Med Internet Res. 2019;21(11): e14537. https://doi.org/10.2196/14537 .
Nuffield Council on Bioethics. The collection, linking and use of data in biomedical research and health care: ethical issues, London 2015. https://www.nuffieldbioethics.org/publications/biological-and-health-data . Accessed 21 Dec 2020.
Richter G, Borzikowsky C, Lieb W, Schreiber S, Krawczak M, Buyx A. Patient views on research use of clinical data without consent: legal, but also acceptable? Eur J Hum Genet. 2019;27:841–7.
Krutzinna J, Floridi L. Ethical medical data donation: a pressing issue. In: Krutzinna J, Floridi L, editors. The ethics of medical data donation. Philosophical Studies Series. Springer, Cham; 2019, vol 137, 1:7.
German Ethics Council. Big Data and health—data sovereignty as informational freedom. November 2017.
Richter G, Borzikowsky C, Lesch W, Semler CC, Bunnik EM, Buyx A, Krawczak M. Secondary research use of personal medical data: Attitudes from patient and population surveys in The Netherlands and Germany. Eur J Hum Genet. 2021;29(3):495–502.
Strech D, von Kielmansegg S, Zenker S, Krawczak M, Semler SC, Wissenschaftliches Gutachten „Datenspende“ – Bedarf für die Forschung, ethische Bewertung, rechtliche, informationstechnologische und organisatorische Rahmenbedingungen. Berlin 2020; https://www.bundesgesundheitsministerium.de/fileadmin/Dateien/5_Publikationen/Ministerium/Berichte/Gutachten_Datenspende.pdf . Accessed 23 Mar 2021.
Barnes R, Votova K, Rahimzadeh V, Osman N, Penn AM, Zawati MH, Knoppers BM. Biobanking for genomic and personalized health research: participant perceptions and preferences. Biopreserv Biobank. 2020;18(3):204–12.
IBM [IBM SPSS Statistics, 2013]. Release 22.0.0.2 for windows, Armonk, NY: IBM.
Federal Statistical Office (Destatis), Educational attainment of the population - Results of the microcensus 2019, Edition 2020 (Statistisches Bundesamt (Destatis), Bildungsstand der Bevölkerung - Ergebnisse des Mikrozensus 2019, Ausgabe 2020), https://www.destatis.de/DE/Themen/Gesellschaft-Umwelt/Bildung-Forschung-Kultur/Bildungsstand/Publikationen/Downloads-Bildungsstand/bildungsstand-bevoelkerung-5210002197004.pdf?__blob=publicationFile . Accessed 23 Mar 2021.
Prainsack B, Buyx A. Solidarity in biomedicine and beyond. Cambridge,: Cambridge University Press; 2017.
Book Google Scholar
Bitkom Research im Auftrag des Digitalverbands Bitkom, Große Offenheit für Spende von Patientendaten, https://www.bitkom.org/Presse/Presseinformation/Grosse-Offenheit-fuer-Spende-von-Patientendaten , 03.07.2020. Accessed 21 Dec 2020.
Bethkenhagen D. Mehrheit zeigt Bereitschaft zur Datenspende, Civey-Umfrage im Auftrag von Tagesspiegel Background, veröffentlicht 04.09.2020, https://background.tagesspiegel.de/gesundheit/mehrheit-zeigt-bereitschaft-zur-datenspende . Accessed 21 Dec 2020.
Kim J, Kim H, Bell E, Bath T, Paul P, Pham A, Jiang X, et al. Patient perspectives about decisions to share medical data and biospecimens for research. JAMA Netw Open. 2019;2(8):e199550.
Kalkman S, van Delden J, Banerjee A, et al. Patients’ and public views and attitudes towards the sharing of health data for research: a narrative review of the empirical evidence. J Med Ethics. 2019. https://doi.org/10.1136/medethics-2019-105651 .
Boulos D, Morand E, Foo M, Trivedi JD, Lai R, Huntersmith R, et al. Acceptability of opt-out consent in a hospital patient population. Intern Med J. 2018;48(1):84–7.
Strategiepapier „Nutzbarmachung digitaler Daten für KI- und datengetriebene Gesundheitsforschung“ https://www.dlr.de/pt/Portaldata/45/Resources/Dokumente/GF/Strategiepapier_Nutzbarmachung_digitaler_Daten.pdf . Accessed 23 Mar 2021.
Communication from the Commission to the European Parliament, the Council, the European Economic and Social Committee and the Committee of the Regions, A European strategy for data, Brussels 19.02.2020, COM(2020) 66 final, https://eur-lex.europa.eu/legal-content/EN/TXT/PDF/?uri=CELEX:52020DC0066&from=DE , Accessed 23 Mar 2021.
Skatova A, Goulding J. Psychology of personal data donation. PLoS ONE. 2019;14(11): e0224240. https://doi.org/10.1371/journal.pone.0224240 .
Platt J, Raj M, Büyüktür AG, Trinidad MG, Olopade O, Ackerman MS, Kardia S. Willingness to Participate in Health Information Networks with Diverse Data Use: Evaluating Public Perspectives. EGEMS (Wash DC). 2019;7(1):33.
Google Scholar
Broekstra R, Aris-Meijer J, Maeckelberghe E, Stolk R, Otten S. Demographic and prosocial intrapersonal characteristics of biobank participants and refusers: the findings of a survey in the Netherlands. Eur J Hum Genet. 2021;29:11–9.
Liu FF, Tosoni S, Voruganti IS, Wong R, Virtanen C, Willison D, et al. The use of patient health information outside the circle of care: Consent preferences of patients from a large academic cancer centre. J Clin Oncol. 2020;38(15):e14122.
Mori I. The one-way mirror: public attitudes to commercial access to health data. Wellcome Trust. Journal contribution. 2017; https://doi.org/10.6084/m9.figshare.5616448.v1. Accessed 23 Mar 2021.
Shah N, Coathup V, Teare H, Forgie I, Giordano GN, Hansen TH, et al. Sharing data for future research—engaging participants’ views about data governance beyond the original project: a DIRECT Study. Genet Med. 2019;21:1131–8.
PwC Health Research Institute. The Covid-19 pandemic is influencing consumer health behaviour. Are the changes here to stay? 2020; https://www.pwc.com/us/en/library/covid-19/covid-19-consumer-behavior.html . Accessed 23 Ma 2021.
Aitken M, Porteous C, Creamer E, Cunningham-Burley S. Who benefits and how? Public expectations of public benefits from data-intensive health research. Big Data Soc. 2018;5:1–12.
Voigt TH, Holtz V, Niemiec E, Howard HC, Middleton A, Prainsack B. Willingness to donate genomic and other medical data: results from Germany. Eur J Hum Genet. 2020;28(8):1000–9.
Download references
Acknowledgements
The authors gratefully acknowledge the support by staff of the Comprehensive Centre for Inflammation Medicine (CCIM) and of the outpatient centre of Internal Medicine/General Surgery (IMAC), University Hospital Schleswig-Holstein Campus Kiel. Special thanks are due to all study participants.
Open Access funding enabled and organized by Projekt DEAL. This research received no specific grant from any funding agency in the public, commercial or not-for-profit sectors.
Author information
Authors and affiliations.
Institute of Experimental Medicine, Division of Biomedical Ethics, Kiel University, University Hospital Schleswig-Holstein, Niemannsweg 11, Haus 1, 24105, Kiel, Germany
Gesine Richter
Institute of Medical Informatics und Statistics, Kiel University, University Hospital Schleswig-Holstein, Kiel, Germany
Christoph Borzikowsky & Michael Krawczak
Department of Internal Medicine 1, University Hospital Schleswig-Holstein, Kiel, Germany
Bimba Franziska Hoyer
Division of Endocrinology, Diabetes and Clinical Nutrition, Department of Medicine 1, University Hospital Schleswig-Holstein, Kiel, Germany
Matthias Laudes
You can also search for this author in PubMed Google Scholar
Contributions
The study was planned by GR and MK. GR designed the questionnaire, with input from MK and CB. BH and ML facilitated the implementation of the survey. GR, MK and CB analysed and interpreted the data. GR and MK wrote a first draft of the manuscript. All authors read and approved the final manuscript.
Corresponding author
Correspondence to Gesine Richter .
Ethics declarations
Ethics approval and consent to participate.
This study was conducted with approval by the Ethics Committee of the Medical Faculty of Kiel University (Re. D438/20, 04.03.2020). We confirm that all research was performed in accordance with their specifications as well as other relevant guidelines and regulations. The ethics vote recognized that explicit consent to participate in the study was not required because consent was implied by the return of the completed questionnaire. All participants were fully informed beforehand about this fact as well as about the scope and purpose of the study, and that their data would be processed anonymously.
Consent for publication
Not applicable.
Competing interests
The authors declare no conflict of interest.
Additional information
Publisher's note.
Springer Nature remains neutral with regard to jurisdictional claims in published maps and institutional affiliations.
Supplementary Information
Additional file 1:.
Questionnaire 1. Questionnaire of Survey 1. Questionnaire 2. Questionnaire of Survey 2.
Rights and permissions
Open Access This article is licensed under a Creative Commons Attribution 4.0 International License, which permits use, sharing, adaptation, distribution and reproduction in any medium or format, as long as you give appropriate credit to the original author(s) and the source, provide a link to the Creative Commons licence, and indicate if changes were made. The images or other third party material in this article are included in the article's Creative Commons licence, unless indicated otherwise in a credit line to the material. If material is not included in the article's Creative Commons licence and your intended use is not permitted by statutory regulation or exceeds the permitted use, you will need to obtain permission directly from the copyright holder. To view a copy of this licence, visit http://creativecommons.org/licenses/by/4.0/ . The Creative Commons Public Domain Dedication waiver ( http://creativecommons.org/publicdomain/zero/1.0/ ) applies to the data made available in this article, unless otherwise stated in a credit line to the data.
Reprints and permissions
About this article
Cite this article.
Richter, G., Borzikowsky, C., Hoyer, B.F. et al. Secondary research use of personal medical data: patient attitudes towards data donation. BMC Med Ethics 22 , 164 (2021). https://doi.org/10.1186/s12910-021-00728-x
Download citation
Received : 05 May 2021
Accepted : 16 November 2021
Published : 15 December 2021
DOI : https://doi.org/10.1186/s12910-021-00728-x
Share this article
Anyone you share the following link with will be able to read this content:
Sorry, a shareable link is not currently available for this article.
Provided by the Springer Nature SharedIt content-sharing initiative
- Data donation
- Patient consent
- Medical research
- Secondary data use
- Precision medicine
- Public health
BMC Medical Ethics
ISSN: 1472-6939
- General enquiries: [email protected]
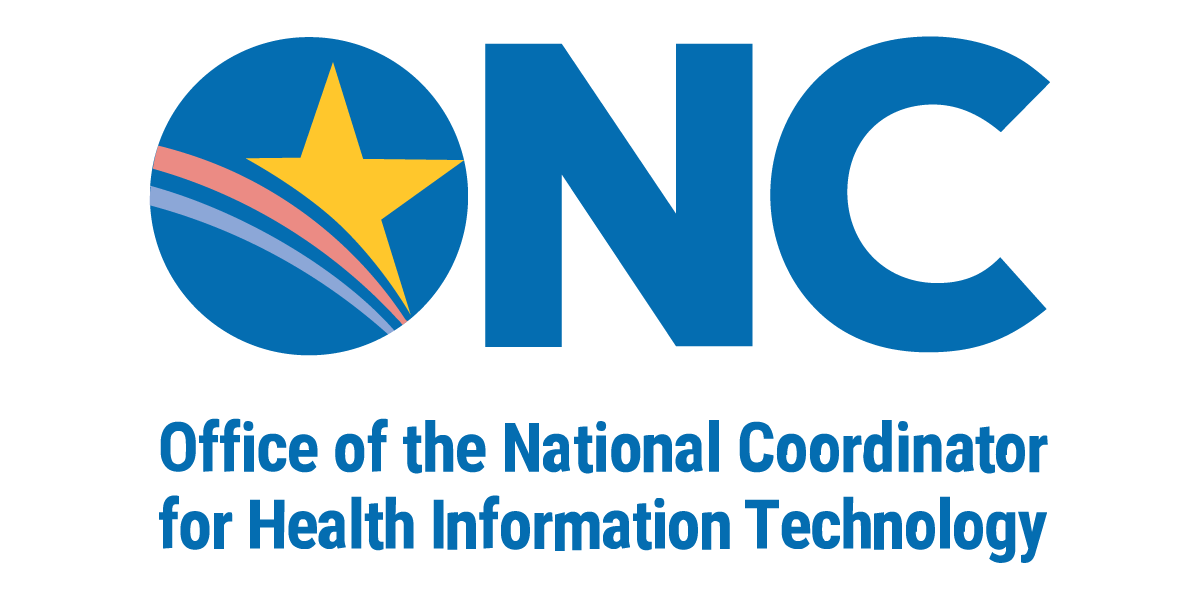
Certification of Health IT
Health information technology advisory committee (hitac), health equity, hti-1 final rule, information blocking, interoperability, patient access to health records, clinical quality and safety, health it and health information exchange basics, health it in health care settings, health it resources, laws, regulation, and policy, onc funding opportunities, onc hitech programs, privacy, security, and hipaa, scientific initiatives, standards & technology, usability and provider burden, patient-generated health data, what are patient-generated health data.
Patient-generated health data (PGHD) are health-related data created, recorded, or gathered by or from patients (or family members or other caregivers) to help address a health concern.
PGHD include, but are not limited to:
- health history
- treatment history
- biometric data
- lifestyle choices
Examples of PGHD include blood glucose monitoring or blood pressure readings using home health equipment, or exercise and diet tracking using a mobile app or wearable device.
Benefits of Patient-Generated Health Data
PGHD can supplement existing clinical data, filling in gaps in information and providing a more comprehensive picture of ongoing patient health. However, PGHD are distinct from data generated in clinical settings and through encounters with providers in two important ways:
- Patients, not providers, are primarily responsible for capturing or recording these data.
- Patients decide how to share or distribute these data to health care providers and others.
The use and sharing of PGHD in care delivery and research can:
- Gather important information about how patients are doing between medical visits.
- Provide information for use in shared decision-making about preventive and chronic care management.
- Offer potential cost savings and improvements in quality, care coordination, and patient safety.
Our Patient Engagement Playbook offers tips for providers incorporating PGHD use in medical practices.
Project Dates
This project began in 2015 and ended in 2018.
Project Goal
The goal of this project was to identify best practices, gaps, and opportunities to advance the collection and use of PGHD that may improve health outcomes and reduce costs while:
- Protecting the patient and the integrity of the patient record;
- Maximizing the provider-patient relationship;
- Building confidence among providers and researchers to use these data; and
- Encouraging individuals to donate their health data for research.
This project researched challenges and opportunities within seven PGHD topic areas:
- Patient recruitment for research studies and trials
- Collection and validation of data and tools
- Data donation
- Ability to combine PGHD with medical record data in multiple ways
- Data interoperability
- Big data analysis
- Regulatory overview
This project synthesized the lessons learned from two pilot demonstrations and the analysis of nearly 200 public comments on a draft white paper published in January 2017.
White Paper
To learn about the policy landscape, challenges and opportunities organized by stakeholder group, and considerations for a future policy framework that could further inform guidance in support of the capture, use, and sharing of PGHD, read the White Paper and download the infographic. *
Practical Guide
To find suggested practices and questions to consider for the implementation of the capture, use, and sharing of PGHD in clinical and research settings, read the Practical Guide and download the infographic. *
*Persons using assistive technology may not be able to fully access information in this file. For assistance, submit an issue on the Health IT Feedback Form .
Please contact [email protected] with questions about this project.
Open Survey
Data Resources in the Health Sciences
- Clinical Data
Introduction to Clinical Data
Electronic health record, administrative data, claims data, patient / disease registries, health surveys, clinical trials registries and databases, clinical research datasets.
- Scientific Data
- Statistics Sources: Health Sciences
- Preserve/Store Data
- Describe Data
- Analyze/Visualize Data
Defining Clinical Data Repositories
State of the Industry: Seven Characteristics of a Clinical Research Data Repository HIMSS
A Practical Guide to Clinical Data Warehousing Association for Clinical Data Management (ACDM)
Clinical data is a staple resource for most health and medical research. Clinical data is either collected during the course of ongoing patient care or as part of a formal clinical trial program. Clinical data falls into six major types:
- Electronic health records
- Administrative data
- Claims data
- Patient / Disease registries
- Health surveys
- Clinical trials data
See boxes below for examples of each major type.
The purest type of electronic clinical data which is obtained at the point of care at a medical facility, hospital, clinic or practice. Often referred to as the electronic medical record (EMR), the EMR is generally not available to outside researchers. The data collected includes administrative and demographic information, diagnosis, treatment, prescription drugs, laboratory tests, physiologic monitoring data, hospitalization, patient insurance, etc.
Individual organizations such as hospitals or health systems may provide access to internal staff. Larger collaborations, such as the NIH Collaboratory Distributed Research Network provides mediated or collaborative access to clinical data repositories by eligible researchers . Additionally, t he UW De-identified Clinical Data Repository (DCDR) and the Stanford Center for Clinical Informatics allow for initial cohort identification.
Often associated with electronic health records, these are primarily hospital discharge data reported to a government agency like AHRQ.
- Healthcare Cost & Utilization Project (H-CUP) HCUPnet is a free, on-line query system based on data from the Healthcare Cost and Utilization Project (HCUP). It provides access to health statistics and information on hospital inpatient and emergency department utilization. The project includes a number of datasets and sample studies listed under the information icon. Datasets are available for purchase. more... less... Nationwide Inpatient Sample Kids Inpatient Database State Inpatient Databases State Ambulatory Surgery Databases State Emergency Department Databases
Claims data describe the billable interactions (insurance claims) between insured patients and the healthcare delivery system. Claims data falls into four general categories: inpatient, outpatient, pharmacy, and enrollment. The sources of claims data can be obtained from the government (e.g., Medicare) and/or commercial health firms (e.g., United HealthCare).
- Basic Stand Alone (BSA) Medicare Claims Public Use Files (PUFs) This is the Basic Stand Alone (BSA) Public Use Files (PUF) for Medicare claims. This is a claim-level file in which each record is a claim incurred by a 5% sample of Medicare beneficiaries. Claims include inpatient/outpatient care, prescription drugs, DME, SNF, hospice, etc. There are some demographic and claim-related variables provided in every PUF.
- Medicare Provider Utilization and Payment Data Data that summarize utilization and payments for procedures, services, and prescription drugs provided to Medicare beneficiaries by specific inpatient and outpatient hospitals, physicians, and other suppliers.
- Medicaid Data Sources The Medicaid Analytic eXtract data contains state-submitted data on Medicaid eligibility, service utilization and payments. The CMS-64 provides data on Medicaid and SCHIP Budget and Expenditure Systems.
- Medicaid Statistical Information System MSIS is the basic source of state-submitted eligibility and claims data on the Medicaid population, their characteristics, utilization, and payments and is available by clicking on the link on the left-side column.
Disease registries are clinical information systems that track a narrow range of key data for certain chronic conditions such as Alzheimer's Disease, cancer, diabetes, heart disease, and asthma. Registries often provide critical information for managing patient conditions.
- Global Alzheimer's Association Interactive Network (GAAIN) The Global Alzheimer’s Association Interactive Network (GAAIN) is a collaborative project that will provide researchers around the globe with access to a vast repository of Alzheimer’s disease research data and the sophisticated analytical tools and computational power needed to work with that data.
- National Cardiovascular Data Registry (NCDR) The NCDR® is the American College of Cardiology’s worldwide suite of data registries helping hospitals and private practices measure and improve the quality of cardiovascular care they provide. The NCDR encompasses six hospital-based registries and one outpatient registry. There are currently more than 2,400 hospitals and nearly 1,000 outpatient providers participating in NCDR registries.
- National Program of Cancer Registries CDC provides support for states and territories to maintain registries that provide high-quality data. Data collected by local cancer registries enable public health professionals to understand and address the cancer burden more effectively.
- National Trauma Data Bank The National Trauma Data Bank® (NTDB) is the largest aggregation of trauma registry data ever assembled. The goal of the NTDB is to inform the medical community, the public, and decision makers about a wide variety of issues that characterize the current state of care for injured persons.
- Surveillance, Prevention, and Management of Diabetes Mellitus DataLink (SUPREME DM)
In order to provide an accurate evaluation of the population health, national surveys of the most common chronic conditions are generally conducted to provide prevalence estimates. National surveys are one of the few types of data collected specifically for research purposes, thus making it more widely accessible.
- Medicare Current Beneficiary Survey The Medicare Current Beneficiary Survey (MCBS) is a continuous, multipurpose survey of a nationally representative sample of the Medicare population. The central goals of MCBS are to determine expenditures and sources of payment for all services used by Medicare beneficiaries.
- National Health & Nutrition Examination Survey (NHANES) The National Health and Nutrition Examination Survey (NHANES) is a program of studies designed to assess the health and nutritional status of adults and children in the United States. The survey is unique in that it combines interviews and physical examinations.
- National Medical Expenditure Survey The Medical Expenditure Panel Survey (MEPS) is a set of large-scale surveys of families and individuals, their medical providers, and employers across the United States. MEPS is the most complete source of data on the cost and use of health care and health insurance coverage.
- National Center for Health Statistics A rich source of health data and statistics on a variety of topics.
- CMS Data Navigator Center for Medicare & Medicaid Services - Research, Statistics, Data & Systems
- National Health and Aging Trends Study (NHATS) NHATS is a study of Medicare beneficiaries age 65 years and older. The study is being conducted by the Johns Hopkins University Bloomberg School of Public Health, with data collection by Westat, and support from the National Institute on Aging. NHATS is intended to foster research that will guide efforts to reduce disability, maximize health and independent functioning, and enhance quality of life at older ages.
- ClinicalTrials.gov o Registry and results database hosted by the NIH. o Information on publicly and privately supported clinical studies from around the world.
- Cochrane Library o Trials database, CENTRAL, is component of Cochrane Library o Reports of randomized and quasi-randomized clinical trials taken from Medline, Embase, and elsewhere.
- WHO International Clinical Trials Registry Platform (ICTRP) o Clinical trial registration data from over 15 trial registries, including registries from the European Union, Africa, China, Japan, Brazil, and Australia. o Use "standard search" to look for NCT or ISRCTN numbers cited in articles.
- European Union Clinical Trials Database o Protocol and results information on interventional clinical trials conducted in the EU. o Good source of pediatric drug development trials.
- CenterWatch o Portal for actively recruiting pharmaceutical industry-sponsored clinical trials.
Clinical research data may be available through national or discipline-specific organizations. Level of access is likely restricted but available through proper channels.
Proprietary research data may also be available through individual agreements with private companies.
- Biologic Specimen and Data Repository Information Coordinating Center (NHLBI) Listing of studies with resources available for searching and request via BioLINCC.
- Biomedical Translational Research Information System (BTRIS) Research data available to the NIH intramural community only.
- Clinical Data Study Request Clinical trials data. Partners include Pharmaceutical companies.
- NIMH Clinical Trials - Limited Access Datasets Requirements for access at the bottom of the page.
- YODA (Yale Open Data Access) Access to participant-level clinical research data and/or comprehensive reports of clinical research. Partners include Medtronic and Johnson & Johnson.
- << Previous: Find Data
- Next: Scientific Data >>
- Last Updated: Feb 7, 2024 10:58 AM
- URL: https://guides.lib.uw.edu/hsl/data
Be boundless
1959 NE Pacific Street | T334 Health Sciences Building | Box 357155 | Seattle, WA 98195-7155 | 206-543-3390
© 2024 University of Washington | Seattle, WA

Featured Searches
- Clinical Trials
- Find a Doctor
- Cancer Care
- Heart Surgery
- Health Professional Schools
- UCH Leadership
- Diversity, Equity and Inclusion
- Mission, Vision & Values
UC researchers use health data to find actionable insights in record time
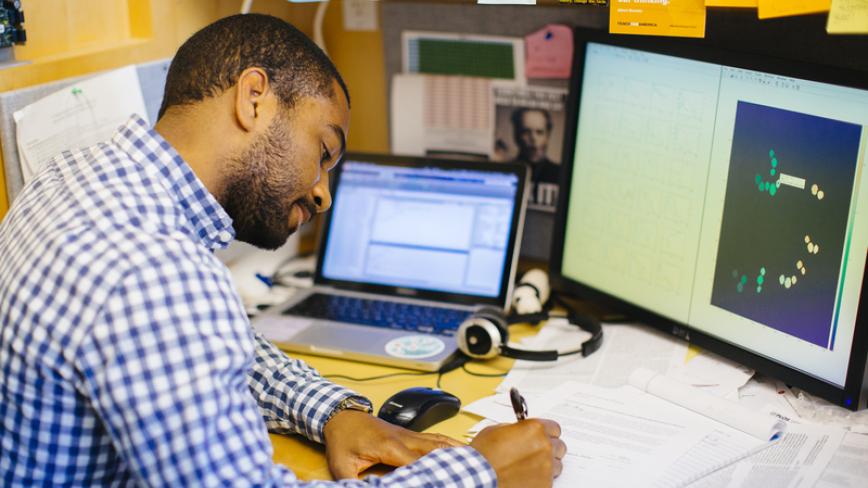
University of California researchers are making groundbreaking use of information from electronic health records with billions of data points to fast-track breakthrough insights in medical practice and treatment. This approach, enabled by use of data science methods, makes medical research more efficient by evolving traditional methodologies that have been the convention for collecting and analyzing clinical data.
Two recent publications demonstrate how data science in medical research has the potential to accelerate a national study of breast cancer detection methods and better understand COVID-19 breakthrough infection risk on-pace with the virus.
These studies have been made possible through UC’s Center for Data-driven Insights and Innovation (CDI2), which manages the UC Health Data Warehouse (UCHDW). The UCHDW is distinguished by its volume of information and the diversity represented in data from patients of UC’s six academic health centers—UC Davis Health, UCI Health, UCLA Health, UCR Health, UC San Diego Health and UCSF Health—going back more than 10 years. UCHDW also includes publicly available data from other sources, such as the California Department of Health Care Access and Information (HCAI).
“This kind of data science-driven research is truly a leap forward in our ability to bring precision medicine to more people and close the health equity gap, especially in California’s most vulnerable communities,” says Atul Butte, M.D., Ph.D., University of California Health’s chief data scientist and head of CDI2.
“In some cases, years and billions of dollars would be required for randomized clinical trials to arrive at these findings. What’s more, the diversity represented in this data allows us to study the impacts of care and treatment by age, gender, race and ethnicity, and more,” added Butte.
The UCHDW contains data for over 9 million patients, including more than 5.2 billion vital signs and test result measurements, as well as billions of procedures and medication orders and prescriptions, tens of thousands of sequenced cancer genomes and more than a billion diagnosis codes.
Better detection of breast cancer
The Women Informed to Screen Depending On Measures of risk ( WISDOM ) Study is investigating whether personalized screening based on a woman’s individual health and medical history is more effective than annual screening for breast cancer. Launched within the UC system in 2016, the study includes more than 55,000 patients (of whom 18,000 are UC patients) and has been extended to include women nationwide. Historically, collection of confirmed cancer diagnoses has relied on state and national cancer registries, where data validation can take months or longer to finalize, and patients electing to self-report.
Katherine Leggat-Barr, M.D., and colleagues at UCSF, led a study to explore whether the UCHDW could play a role in speeding the collection of confirmed diagnoses for the WISDOM Study.
Published in the American Society of Clinical Oncology’s Journal of Cancer Clinical Informatics , Leggat-Barr and her team show that using the real-world data in the UCHDW as well as self-reported data is more complete than self-reported information alone, although less complete than cancer registries once registry data is finalized after two years.
COVID-19 breakthrough infection insights at the pace of virus mutation
The University of California COVID Research Data Set (UC CORDS) was one of the nation’s first COVID-19-specific sets of real-time data from patient encounters, created in a matter of weeks at the start of the pandemic. UC CORDS has remained crucial to fighting the virus as well as understanding the risk and outcomes of illness for diverse populations.
Most recently, Michael Hogarth, M.D., UC San Diego, and a research team used UC CORDS to document the odds of breakthrough infection for a wide range of specific comorbidities.
The work was published in The American Journal of the Medical Sciences . Because patient data is continually added to the UCHDW, UC CORDS keeps pace with changes in clinical data in real time. Hogarth and team’s analysis provides a repeatable framework for researchers to use as the pandemic has evolved to become endemic.
Recognition for thought leadership
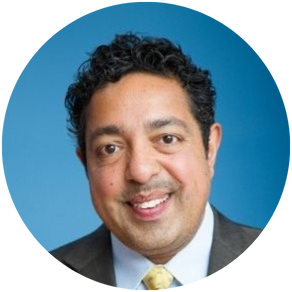
In recognition for his contribution to the transformation of health care through the use of health data, the American Medical Informatics Association has presented Butte with the 2023 William W. Stead Award for Thought Leadership in Informatics .
As a result of Butte’s leadership, data-driven research is expanding as intended, at the local level, and nationwide. At the local level, individual UC academic health centers are adopting the principles modeled by CDI2, including at UC San Diego, which has emulated the UC CORDS model in creating a data warehouse for all local electronic health records and establishing a team dedicated to facilitating researchers’ use of data. At the same time, UC-initiated research has expanded to include patients beyond the UC Health system, as in the case of the WISDOM Study. The research design is a model that can be replicated by other institutions and organizations that have an interest in advancing their own data-driven efforts.
About University of California Health
University of California Health comprises six academic health centers, 20 health professional schools, a Global Health Institute and systemwide services that improve the health of patients and the University’s students, faculty and employees. All of UC’s hospitals are ranked among the best in California and its medical schools and health professional schools are nationally ranked in their respective areas.
Hospitals are selling treasure troves of medical data — what could go wrong?
They don’t need patient consent to use de-identified data.
By Nicole Wetsman
Share this story
:format(webp)/cdn.vox-cdn.com/uploads/chorus_asset/file/19581743/VRG_ILLO_1777_AK_body_data.0.jpg)
Healthcare organizations and hospitals in the United States all sit on treasure troves: a stockpile of patient health data stored as electronic medical records. Those files show what people are sick with, how they were treated, and what happened next. Taken together, they’re hugely valuable resources for medical discovery.
Because of certain provisions of the Health Insurance Portability and Accountability Act (HIPAA), healthcare organizations are able to put that treasure trove to work. As long as they de-identify the records — removing information like patient names, locations, and phone numbers — they can give or sell the data to partners for research. They don’t need to get consent from patients to do it or even tell them about it.
More and more healthcare groups are taking advantage of those partnerships. The Mayo Clinic in Rochester, Minnesota, is working with startups to develop algorithms to diagnose and manage conditions based on health data. Fourteen US health systems formed a company to aggregate and sell de-identified data earlier this year. The healthcare company HCA announced a new data deal with Google in May.
There may be benefits to sharing this data — researchers can learn what types of treatments are best for people with certain medical conditions and develop tools to improve care. But there are risks to free-flowing data, says Eric Perakslis, chief science and digital officer at the Duke Clinical Research Institute. He outlined the ways the system could potentially harm patients in a recent New England Journal of Medicine article with Kenneth Mandl, director of the computational health informatics program at Boston Children’s Hospital.
“You don’t always understand the risks that come with the benefits until later”
“I’m a huge advocate for open data,” Perakslis says. “I think it’s very easy to get excited about the benefits. What we know with medical sciences, though, is that you don’t always understand the risks that come with the benefits until later.”
Perakslis talked to The Verge about what could go wrong and how to protect people from those risks.
This interview has been lightly edited for clarity.
When did healthcare organizations start taking advantage of their electronic medical records data in this way?
I want to say it was probably in 2017 or 2018. The thing that really pushed this into overdrive was the rise of privacy-preserving record linkage, which combines records from the same person without identifying them. The technologies are perfectly fine. But it almost makes a lot of people feel like, “Well, if I de-identified the data, I can almost do anything I want.”
Before those technologies, the only good way to do de-identification was to have a statistician do it. These technologies made it so almost anyone could do it. They’re not expensive. So the technology is ubiquitous, and it’s very easy to make deals and start marketing the data.
Who is using this data, and what’s it being used for?
If you look at ethical research, there are a lot of academic medical centers with great de-identified data sets and large research networks that have been well-monitored and well-designed. What’s happened beyond that is a place like an MRI center or a pharmacy has an agreement with a hospital, but that agreement doesn’t prohibit them from doing anything with that data. If you haven’t explicitly told them they can’t de-identify and sell data, they can. That’s the kind of thing we’d call a data leak.
So then there are these large data networks that are being formed, where people are putting their data in with other people’s, and then those big networks are trying to sell to pharma or government research labs or places like that. At the end of the day, everyone’s trying to sell to pharma because it’s so lucrative. In fairness, the pharma companies aren’t necessarily looking to do anything less than anything completely ethical, as far as getting data.
I think most healthcare institutions are interested in using data for profit and for research. I don’t think there’s anything wrong with that if you can actually say how you’re returning the benefit back to the core mission of the place.
So say I have my health record at a hospital that then decides to sell it to a private company that’s building a health database. It’s de-identified, so my name isn’t on it. In what ways does that put me at risk?
I’ve always kind of called de-identification a privacy placebo. It works about as well as the thermostat in a hotel room. There’s a lot of ways around it.
“I’ve always kind of called de-identification a privacy placebo.”
If it’s re-identified and the data is hacked or exposed, there are a few things that could go on. A lot of people will use solely medical data to make fraudulent medical claims, and then what happens is the victim of the identity theft gets all these bills. Your medical record contains financial information, so there’s the financial risk of that. The other thing that can go on is if you had a condition that you didn’t want your family to know about, or your employer or something like that, it could be exposed.
We’ve gotten really good at not fixing anything when this happens. Once the data is out, it’s out.
Those are the risks. But what are the benefits? How well can data from health records actually be used to solve health problems?
The usefulness of it is absolutely overblown. I think that, at the end of the day, electronic health records have been shown to be pretty good billing systems. While there’s great research done on them, that doesn’t mean the research is easy. It just means more people have taken it on. Good research requires really skilled people to do it. It’s really easy to underestimate the complexity of the problem. I get people calling me all the time with big studies, wondering why we couldn’t just do it using electronic health records data. We can do a lot of research that way, but it isn’t always high-quality research.
I think there are benefits, it just matters where you’re looking. There are great open-source data initiatives that have just really democratized the ability for smart people everywhere to get access to good-quality data for their ideas.
I’m sure people are going to do great things. But I’ve had long conversations with people in this market, and many of them genuinely believe that what they’re doing is going to help patients. But they’re naive, and there will be gaps in their methods that will invalidate the research. It’s the “move fast, break things” mentality, which is wonderful, but please don’t move fast and break things in my daughter’s medical records.
Are there other ways to do better research that also gives patients more protection in the process?
There’s also the traditional medical research establishment that gets patients’ consent and uses the same technology in that consented way. And they get IRB approval. [ Note: Institutional Review Boards, or IRBs, do ethics reviews of research that includes human subjects. ]
That’s done by the government and nonprofits and also pharma. There’s great stuff out there. So I guess the question is: why do we need this whole other thing? If pharma is de-identifying and sharing data where the patients consented for research and it’s overseen by an IRB, if all of that is working, why do we need this other, risky thing? The IRB also looks at the validity of the research. Nobody’s looking at the validity of the research of this off-the-grid stuff that’s going on.
Do you think health institutions or regulatory agencies will adjust anything to block some of these data leaks or prevent some of the risks to patients?
Something like this is like any other kind of medical harm. An adverse event could be a destroyed credit score. I think there are parts of healthcare that take this very seriously, but I don’t think it’s second nature yet.
That’s partly because the game is always being upped. I think it’s very difficult to stay on the curve, especially in medicine. On one side, you have these speed-of-light tech and cybercrime processes going on, and the other is smart people trying to take care of patients better. And they’re just mismatched.
But I think the industry could help itself a little bit, and be more open, and say that they’ll do more with consent. Or [regulators] could make re-identification of data illegal. Something that actually protects the people who are going to suffer from this. I actually don’t believe there’s anything wrong with the technologies. It’s really more a matter of saying, “If we’re going to do this type of research, how do we ensure we’re protecting the people that might be harmed by it?”
The MSI Claw is an embarrassment
New teslas might lose steam, the delta emulator is changing its logo after adobe threatened it, sugar’s big twist was more than a gimmick, behold ayaneo’s sophisticated takes on the game boy and game boy micro.

More from Science
:format(webp)/cdn.vox-cdn.com/uploads/chorus_asset/file/23935561/acastro_STK103__04.jpg)
Amazon — like SpaceX — claims the labor board is unconstitutional
:format(webp)/cdn.vox-cdn.com/uploads/chorus_asset/file/25288452/246992_AI_at_Work_REAL_COST_ECarter.png)
How much electricity does AI consume?
:format(webp)/cdn.vox-cdn.com/uploads/chorus_asset/file/25287681/1371856480.jpg)
A Big Tech-backed campaign to plant trees might have taken a wrong turn
:format(webp)/cdn.vox-cdn.com/uploads/chorus_asset/file/25287408/2003731596.jpg)
SpaceX successfully launches Odysseus in bid to return US to the lunar surface
- ODSC EUROPE
- AI+ Training
- Speak at ODSC

- Data Analytics
- Data Engineering
- Data Visualization
- Deep Learning
- Generative AI
- Machine Learning
- NLP and LLMs
- Business & Use Cases
- Career Advice
- Write for us
- ODSC Community Slack Channel
- Upcoming Webinars

15 Open Datasets for Healthcare
Featured Post Datasets Healthcare posted by Elizabeth Wallace, ODSC June 27, 2019 Elizabeth Wallace, ODSC
Machine Learning is exploding into the world of healthcare. When we talk about the ways ML will revolutionize certain fields, healthcare is always one of the top areas seeing huge strides, thanks to the processing and learning power of machines. There’s a good chance you either are or will soon be employed in the healthcare field. A while back, I wrote a list of 25 excellent open datasets for ML and included healthdata.gov and MIMIC Critical Care Database . Here are 15 more excellent datasets specifically for healthcare.
[Related Article: Major Applications of AI in Healthcare ]
General and Public Health:
WHO : Provides datasets based on global health priorities. The organization includes easy search and provides insights for topics along with the datasets.
CDC : Use this for US specific public health. The CDC maintains WONDER (Wide-ranging Online Data for Epidemiological Research) and sets are searchable by topic, state, and other factors.
data.gov : US focused healthcare data searchable by several different factors. Datasets are intended to improve the lives of people living in the US, but the information could be valuable for other training sets in research or other public health areas.
Scientific Research
Re3Data : Contains data from over 2000 research subjects defined across several broad categories. While not all datasets available are free, the structures are clearly marked and easily searchable based on fees, membership requirements, and copyright restrictions.
CHDS : Child Health and Development Studies datasets are intended to research how disease and health pass down through generation. It contains datasets for research into not just genomic expression but how social, environmental, and cultural factors play into disease and health.
Kent Ridge Biomedical Datasets : High-dimensional datasets in the biomedical field. It focuses on journal-published data (Nature, Science, and others).
Merck Molecular Health Activity Challenge : Datasets designed to foster the machine learning pursuit of drug discovery by simulating how molecule combinations could interact with each other.
SEER : Datasets arranged by demographic groups and provided by the US government. You can search based on age, race, and gender.
1000 Genomes Project : Sequencing from 2500 individuals and 26 different populations. It’s one of the biggest genome repositories you can access and is an international collaboration. It’s accessed through AWS. (Note, there are grants available for genome projects)
In-Person and Virtual Conference
September 5th to 6th, 2024 – London
Healthcare Services:
Medicare : Provides datasets based on services provided by Medicare accepting institutions. Datasets are well scrubbed for the most part and offer exciting insights into the service side of hospital care.
HCUP : Datasets from US hospitals. It includes emergency room stays, in-patient stays, and ambulance stats. It’s clean and illuminating into the services section of US healthcare.
OASIS : Open Access Series of Imaging makes neuroimages of the brain freely, hoping to foster research and new advances in both basic health and clinical neuroscience
OpenfMRI : Other imaging data sets from MRI machines to foster research, better diagnostics, and training. It includes 95 datasets from 3372 subjects with new material being added as researchers make their own data open to the public.
CT Medical Images : This one is a small dataset, but it’s specifically cancer-related. It contains labeled images with age, modality, and contrast tags. Again, high-quality images associated with training data may help speed breakthroughs.
Deep Lesion : One of the largest image sets currently available. CT images released from the NIH to help with better accuracy of lesion documentation and diagnosis. It includes over 32,000 lesions from 4000 unique patients.
Bonus! Dataset Aggregators
Kaggle : As always, an excellent resource for finding datasets pertaining not only to healthcare but other areas. If your healthcare explorations expand to a different subject or need other datasets for training, this is always a great resource.
Subreddit : It may take some doing, but you can find some serious gems within the subreddit discussions on open datasets. If you have a burning question that other public datasets can’t answer, this could be the solution.
Healthcare.ai : Not necessarily an aggregator but a full, opensource software and community dedicated to training, activism, and furthering the machine learning integration into all things healthcare.
[Related Article: Machine Learning and Compression Systems in Communications and Healthcare ]
ML in Healthcare
The world is living longer and needs new answers more than ever. If you’re a data scientist working with health organizations or conducting your own research into some of humanity’s most persistent questions, having free access to data is a critical part of that research. Get started with some of these datasets, and they could be a jumping off point for the answers you need.

Elizabeth Wallace, ODSC
Elizabeth is a Nashville-based freelance writer with a soft spot for startups. She spent 13 years teaching language in higher ed and now helps startups and other organizations explain - clearly - what it is they do. Connect with her on LinkedIn here: https://www.linkedin.com/in/elizabethawallace/

Breaking: OpenAI Disbands Team Focused on Long-Term AI Risk
AI and Data Science News posted by ODSC Team May 18, 2024 OpenAI has disbanded its team focused on the long-term risks of artificial intelligence just one year...

Senate Leaders Unveil $32 Billion Plan to Address AI Issues
AI and Data Science News posted by ODSC Team May 17, 2024 A bipartisan group of senators, spearheaded by Majority Leader Chuck Schumer, has released a highly anticipated...

OpenAI Introduces GPT-4o to the World
AI and Data Science News posted by ODSC Team May 17, 2024 In a blog, OpenAI announced the release of GPT-4o, a new GPT model that promises to...

- Português Br
- Journalist Pass
Mayo Clinic biostatisticians power every step of medical research
Kris Schanilec
Share this:
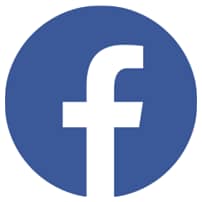
From groundbreaking discoveries in breast cancer treatment to advancements in genomics, biostatisticians bring a unique perspective and skill set to medical research. Last year, Mayo Clinic's Division of Clinical Trials and Biostatistics supported about 5,000 studies spanning discovery science, clinical trials, translational science and population health.
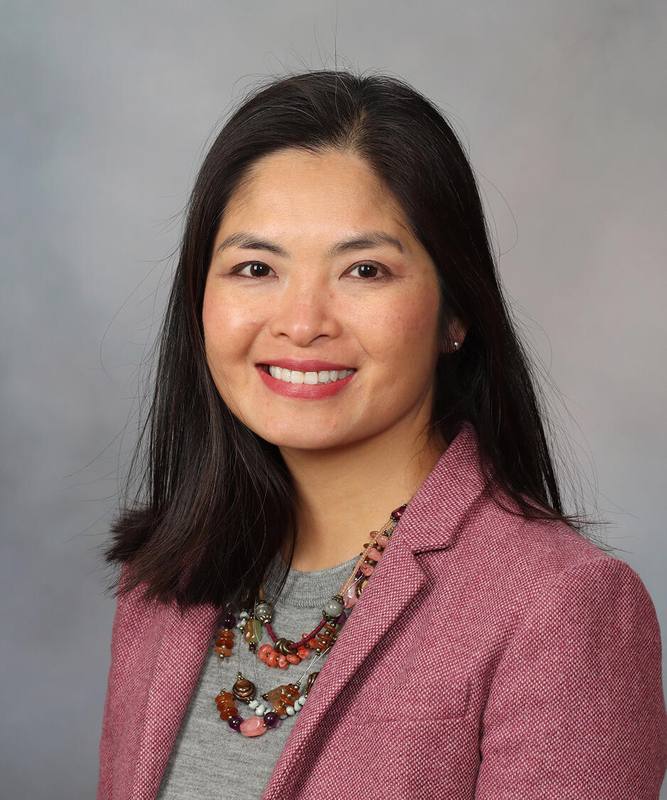
"As one of the largest biostatistics groups in the U.S., Mayo Clinic biostatisticians are integrated into research programs to ensure that the statistical methods we use are the most appropriate in the context of the research question," says Jennifer Le-Rademacher, Ph.D. , chair of the Division of Clinical Trials and Biostatistics.
Biostatistics staff support every step of a research study, starting with the research question. They advise on protocols and study designs. They build, curate, clean and analyze datasets. They report results, co-author papers and respond to statistical review comments.
The division has multiyear federal grants and serves as the statistics and data coordinating center for investigator-initiated, multicenter cancer research programs. Its staff members serve on National Institutes of Health committees and Food and Drug Administration (FDA) advisory panels, and they lead committees that guide national standards. They are faculty in national and international clinical research training programs.
Contributing to team science
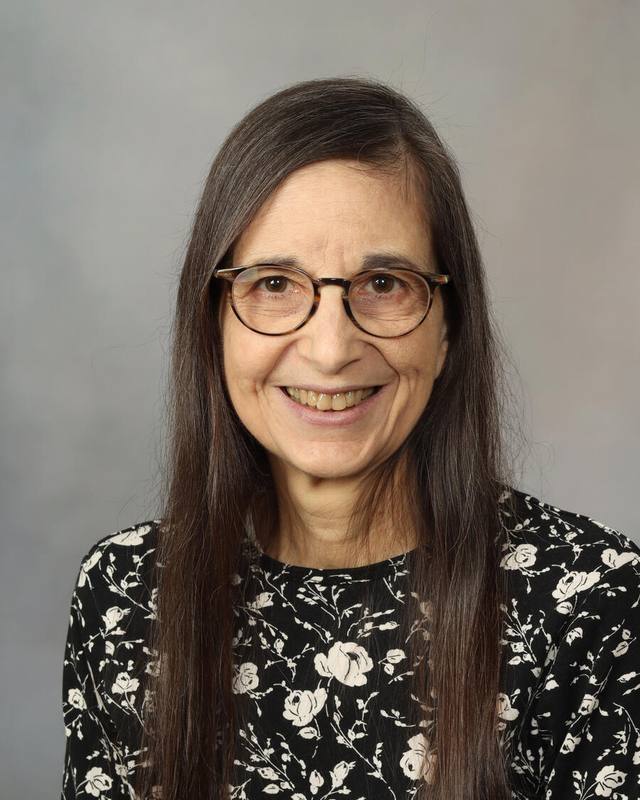
For most of her 33-year career, Mayo Clinic statistician Vera Suman, Ph.D. , has worked in the Mayo Clinic Comprehensive Cancer Center statistical unit, where she is the lead statistician for breast cancer and melanoma. She also directs the Biostatistics Core in Mayo Clinic's Breast Cancer Specialized Program of Research Excellence (SPORE).
She supported a major phase 3 clinical trial of the drug trastuzumab (Herceptin) with chemotherapy for breast cancer treatment. She was part of the team that decided the assay process for confirming participant eligibility, helping to ensure data integrity.
"I'm just one piece in this whole operation. We couldn't do the kinds of trials and the number of trials we do without everybody involved," Dr. Suman says. "This is team science. It is collaboration."
Finding the story in the data
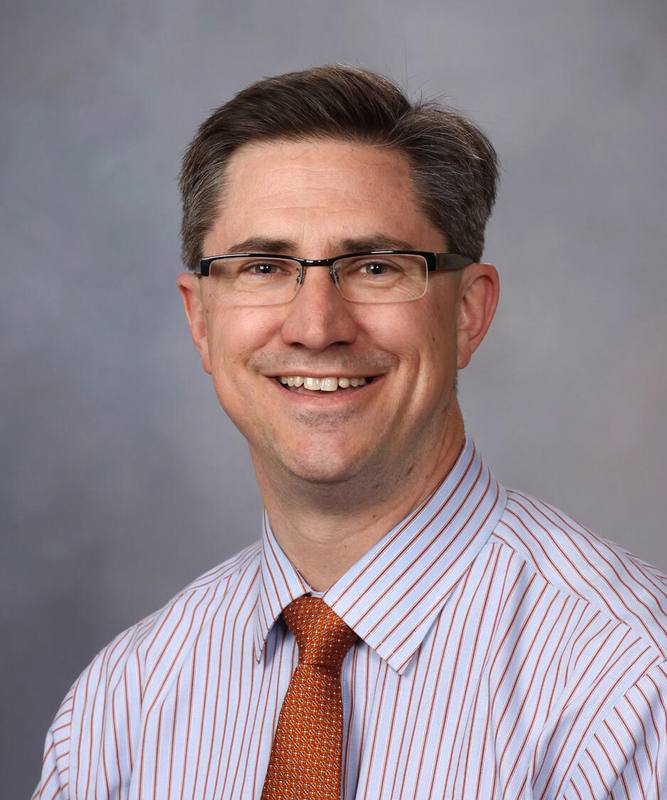
At any given time, principal biostatistician Ryan Lennon may be supporting 20 projects at various stages of development. He currently supports Mayo Clinic's Cardiac Catheterization Laboratory as well as the Gastroenterology and Rheumatology departments.
Lennon provided statistical expertise for a large pharmacogenetics clinical trial conducted at 40 centers worldwide. He helped plan the trial and played a key role throughout the seven-year study . The researchers found that genetic testing may be useful in selecting antiplatelet medications — a significant step forward in genetic-guided treatment.
For Lennon, it's about getting the best data for the research question. "I love getting to know the data and finding out what the story is inside it, and getting a number that helps people understand what the data is trying to tell us," he says.
Translating ideas to improve patient care
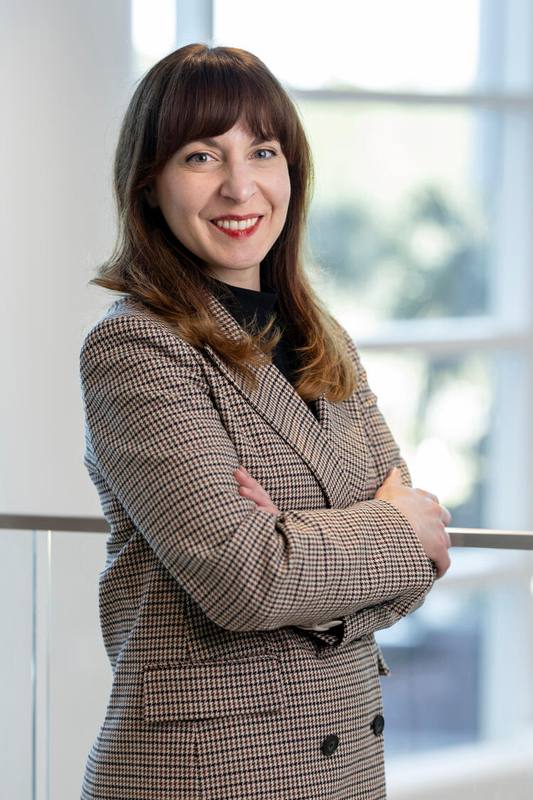
As a principal biostatistician at Mayo Clinic in Arizona, Katie L. Kunze, Ph.D., likens her work to that of a translator. "My goal is to understand where the investigators and study team are coming from, what is the background research and then build a study or an analysis, or create a body of work, to investigate those questions and support patient care," Dr. Kunze says.
She supported a study that found that 1 in 8 patients with cancer may have an inherited, cancer-related gene mutation that is clinically actionable — ushering in a new era of genetic screening and detection.
Dr. Kunze supports gastroenterology, Center for Individualized Medicine and other areas. "It brings me joy when I feel the work I'm doing is actually helping patients," she says.
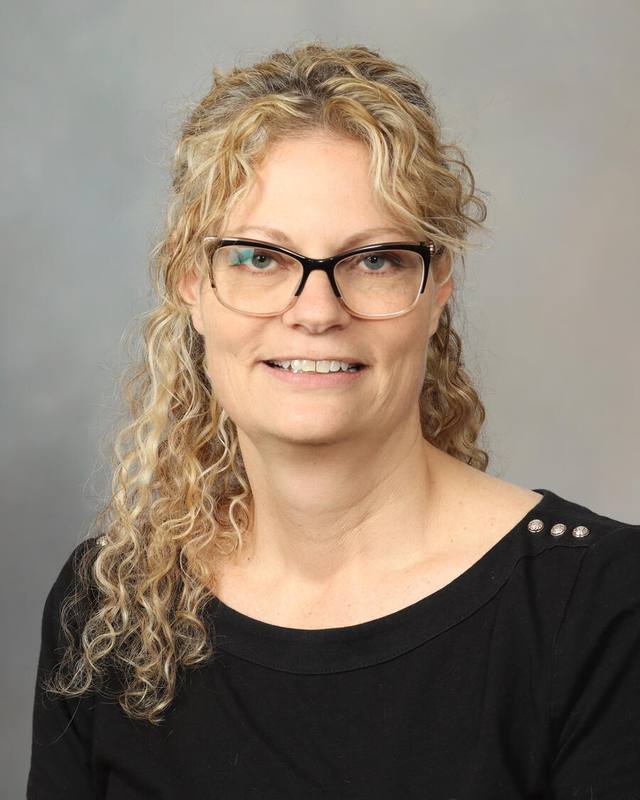
Stretching the limits of database design
Statistical programmers like Regina Herges ensure data quality and use various programming tools and techniques to support database design, data management, statistical analyses and results reporting.
Herges advises study teams on electronic systems to capture and house data. She also builds study databases and provides ongoing support. She has worked in many research areas and currently supports radiation oncology.
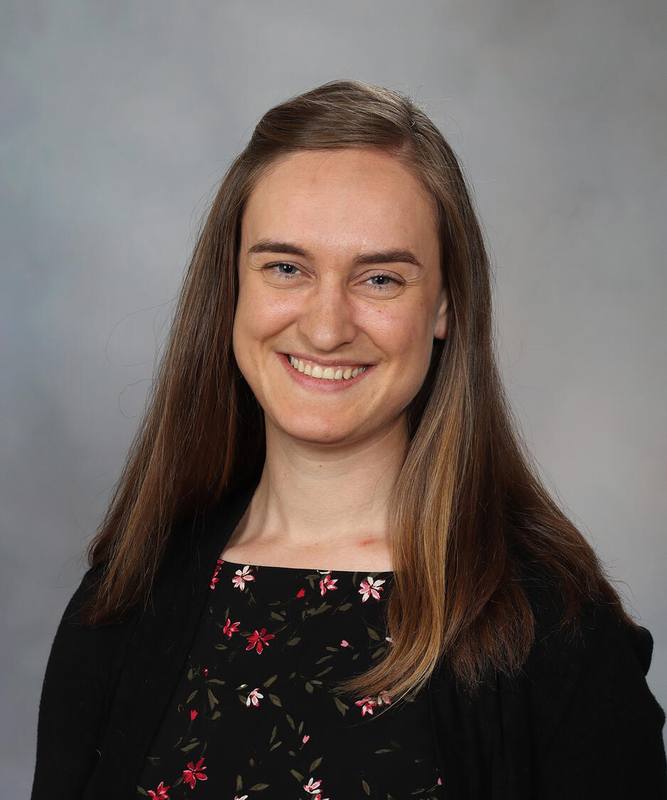
Shortly after Mayo Clinic launched a nationwide program in response to the COVID-19 pandemic, Herges and Laura A. Nelson were recruited to help solve an issue with a database serving more than 2,000 medical teams across the country. The Mayo team designed a single-system solution that could automatically link data on the back end when clinicians requested convalescent plasma for patients.
"It was a really unique challenge, and we helped come up with a great solution," Herges says.
Read more about Mayo Clinic's biostatisticians.
- Mayo Clinic to host 13th Annual Individualizing Medicine Conference Mayo Clinic Minute: Who should be screened for skin cancer?
Related Articles
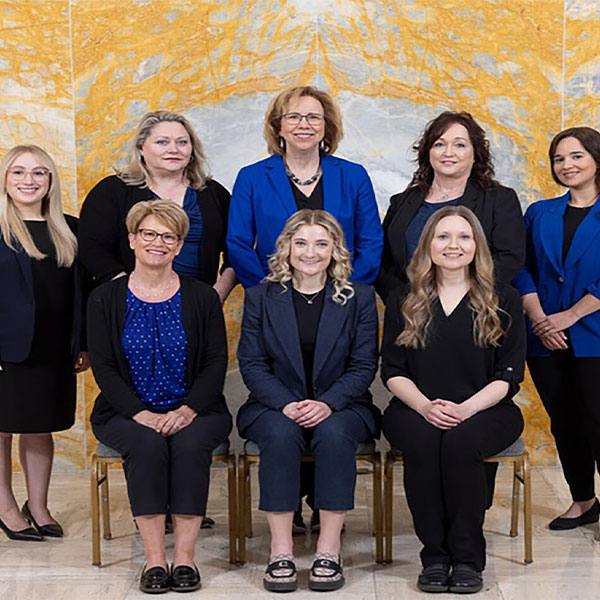

An official website of the United States government
The .gov means it’s official. Federal government websites often end in .gov or .mil. Before sharing sensitive information, make sure you’re on a federal government site.
The site is secure. The https:// ensures that you are connecting to the official website and that any information you provide is encrypted and transmitted securely.
- Publications
- Account settings
Preview improvements coming to the PMC website in October 2024. Learn More or Try it out now .
- Advanced Search
- Journal List
- Risk Manag Healthc Policy
The role of medical data in efficient patient care delivery: a review
Kasaw adane.
1 Unit of Quality Assurance and Laboratory Management, School of Biomedical and Laboratory Sciences, University of Gondar, Ethiopia, moc.liamg@enadawsk
Mucheye Gizachew
2 School of Biomedical and Laboratory Sciences, Department of Medical Microbiology, University of Gondar, Gondar, Ethiopia
Semalegne Kendie
3 School of Sociology and Social Work, Department of Social Work, University of Gondar, Gondar, Ethiopia
Implementing accurate data management systems ensure safe and efficient transfer of confidential health care data. However, health care professionals overlooked their important tasks of medical data processing. Hence, using high-quality electronic health record (EHR) applications in health care is important to minimize medical errors. Therefore, this review tries to indicate the roles of EHR in advancing quality health care service provisions.
The keywords identified were EHR, EMR, medical data processing, medical data retention, medical data destruction, health care, and patient care, and a few related terms with different combinations. PubMed (National Library of Medicine), Google Scholar, and Google search engine were used to search for articles from those databases. Searching was done using boolean words “AND”, “OR”, and “NOT” using all [All fields] and [MeSH Terms] searching strategies.
Articles were screened using the title, checked by their abstract, and the remaining related full-text materials were included or excluded by two individuals deciding its eligibility. Finally, 73 materials issued from 2013–2018 were used for qualitatively synthesizing and reconciling the idea to produce this review article.
Poor medical data processing systems are the key reasons for medical errors. Employing standardized data management systems reduce errors and associated sufferings. Therefore, using electronic tools in the health care institution ensures safe and efficient data management. Therefore, it is important to establish appropriate medical data management systems for efficient health care delivery.
The mission of health care institutions – restoring patient’s health – demands effective and efficient medical data for evidence-based intervention. 1 Installing an appropriate health care data management system with valid case definition enables efficient data extraction, 2 improves communication for clinical decision making in medical practice, 2 – 8 and clinical research, 9 , 10 and upgrades the quality of health care services. 11 Healthcare professionals are responsive to improve recording, distributing, monitoring, and implementing preventive measures to decrease morbidity. 12 This requires consistent, complete, comprehensive, and accurate information which attracts more attention in the health care industry. 3
The health care industry uses a paper-based record (PBR) and/or electronic health record (EHR) system to manage patient’s data. The EHR has become an integral part of medical care, 13 which transforms health care service quality 14 , 15 and improves clinicians’ satisfaction and facilitates patients’ decision. 8 , 16 Accurate information from EHR enables physicians’ order entry and measures clinical validity, which in turn upgrades the quality of patient care. 17 This functionality is crucial during diagnosis and therapy, 15 which benefits medical and legal practices too. 18
Decision-support embedded features – standardized checklists, alert signals, predictive tools, and guidelines 1 – motivate and encourage health care organization leaders and persuade physicians to better utilize best practice alerts (BPAs) in a more effective and efficient way. 19 In line with this, research reports backed up a position that health care practices are being transformed from PBR to EHR systems, 17 although a report revealed that, in the eye care practice, EHR is less versatile for recording. 20
Patient data were readily accessible and transferable 21 from the EHR system. This helps to make an accurate diagnosis and decision making 22 by reducing the access time and use. 1 , 2 Notification signal flags or BPAs prompt about “what content” and “with whom” to share 23 – 25 that trigger potential adverse events (AEs) using easily identifiable displays that alert patient records reviewers. 26 This enhances patient’s engagement in health care service provision 27 and decision-making processes, 28 as it builds trust 29 and confidence 30 that helps to identify specific and actionable adherence barriers. 31 In addition, automatic email text and telephone reminders can be sent to patients in order to motivate and maximize patients compliance. 32
Poor data management practices are the reasons for recurrent errors and associated injuries or death, 33 which is mostly happening due to illegible PBR 34 (mistakes in recording or transcribing). 35
The EHR application improves the process, 36 trustworthiness, safety, and efficiency of patient care delivery. 29 Hence, implementing standardized policies, processes, and procedures for an appropriate health care data management system that advances the quality of health services and efficiency, 34 , 37 avoids non-value adding activities, 34 and ensures major quality and safety improvement. 16 , 17 , 23 , 34 Therefore, this paper intends to indicate the roles of EHR in improving the quality of health care service provisions.
The keywords identified were EHR, EMR, electronic health record, electronic medical record, medical data recording, medical data processing, medical data retention, medical data destruction, health care, patient care, animal data, and plant data with different combinations. Searching was done using boolean words “AND”, “OR”, and “NOT”.
We used [((EHR OR EHR[MeSH terms]) OR EMR) OR (EMR[MeSH terms]) OR (electronic health record) OR (electronic health record[MeSH terms]) OR (electronic medical record) OR (medical data recording[MeSH terms]) OR (medical data processing) OR (medical data processing[MeSH terms]) OR (medical data retention) OR (medical data retention[MeSH terms]) OR (medical data destruction) OR (medical data destruction[MeSH terms])] AND [((health care) OR (health care)[MeSH terms]) OR (patient care) OR (patient care)[MeSH Terms])] to search articles from PubMed and Google Scholar databases and Google search engine. Information was extracted from downloaded materials and used for qualitative synthesis.
PubMed (National Library of Medicine [NLM]) databases and Google Scholar databases, as well as the Google search engine, were used for downloading published materials using EndNote ® Version X 5 for Window’s application. Published materials which were searched using the EndNote application were subsequently screened and checked for relevance using titles, abstracts, and full-text articles, which was done by two individuals, independently inspecting for its eligibility. From a total of 4,606 searched published materials, 73 full-text materials issued from 2013–2018 were used for the development of this review after passing the subsequent screening, selections, and checking processes. Information generated from referenced materials was qualitatively synthesized and the idea was reconciled to produce this review article. The overall study selection process is depicted in Figure 1 .

PRISMA flow diagram of article selection process.
Patient health care data management processes
Although the health care industry is an information enterprise, its data recording practices and its data protection laws vary considerably among hospitals and countries. 38 , 39 The overall health care data management policies must define confidentiality and prevent reconstruction after destruction controlled by security personnel.
The document destruction policy must define the medical data retention policy and its codes of practice that must file the advantages and disadvantages of destroying or maintaining medical data. 40
The benefits of EHR implementation
Implementing EHR increases the quality of services and ensures the safety of patients upon using decision-support tools result in error reduced services that increase clinicians and patient’s satisfaction, which in turn increases the health care seeking-behavior of clients.
Currently, about 1,000 EHR applications are published every month 42 for the purpose of increasing performance, 41 , 42 reducing fatigue, improving accessibility, ensuring compliance, fidelity, and satisfaction, 41 , 43 with acceptable safety gains. 44
The EHR tool was implemented in the United States and the United Kingdom, which own the largest private and public health care systems in the world, respectively, and succeeded in providing quality patient care. 45 It is an essential tool for the application of modern information technology that improves the quality of health care services 46 consistent with medico-legal considerations. 18
Accessing the EHR tool facilitates the health care delivery, 19 , 24 made more accurate decisions, 22 and contributes to the health care quality improvement and research output 47 , 48 at reduced cost. 49 , 50 The tool also ensures the safe transfer of health care data that meets the patient’s expectation, 51 supports the continuity of patient care, 11 and maintains the compliance with medication adherence. 52 , 53 Moreover, the tool helps diabetes goal achievement, while the service delivery process is assisted from non-physician workers. 54
The data generated from the EHR measure prevention, process, and outcome metric. 55 Implementing high-quality EHR improves epidemic surveillance, 56 decreases the length of patient stay, 40 achieves work efficiency 33 , 40 by reducing non-value adding activities, 34 achieves goals, 3 and helps to make for timely decisions at reduced cost. 49 , 57 The system reduces the nurses and the clerk’s time spent to access data to make timely interventions. 1 In its effectiveness, it ensures the quality of services at a reduced cost. 58 The potential benefits of EHR are improving quality, ensuring continuity of patient care, efficiency, and positive financial return on investment. 50
The effective use of EHR improves the patient’s safety, 48 trust, and their satisfaction on the health care system appeared orienting patients towards a health related information sources. 59 Patients usually want to control how and what details to be notified when their data are accessed. 23 The tools could be customized to notify and ensure the safe transfer of patient private confidential data, 33 and they need to get protected. 60
The interoperability of medical information among health care institutions increases the medical staff’s understanding of the disease, diagnosis, and decision-making processes. 61 The EHR allows automated disease surveillance, and helps in participation and promotion of safe and effective health care practices. 56
The challenges of implementing EHR
The EHR is perceived as a “double-edged sword” as it improves quality on the one hand and increases privacy and safety risks on the other hand. 34 These are important concerns of patients’ for transferring their health care data. 23 , 33
Although its adoption rate is currently rising, EHR is found at a low rate, particularly in developing countries. 46 , 62 , 63 Some of the factors for this low adoption rate include behavioral factors (lack of perceived benefits, 28 poor confidence, 64 dissatisfaction, 59 physicians’ resistance, 65 lack of stakeholders interest, 49 and ignorance on more advanced systems), 60 , 66 technical factors (interoperability, 64 lack of financial support or specific financial incentives, 49 and lack of technology infrastructure), 47 legal factors (lack of legal framework 64 and lack of comprehensive EHR national policy and strategy), 47 socio-demographic factors (age and education level of physicians), 59 practice related factors (high skill demand 28 and lack of training), 67 and knowledge related factors (poor awareness). 64
The Delphi study disclosed the barriers of medical practices to implement EHR, as hindered by a myriad of intrinsic (behavioral and cognitive) and extrinsic (economic and technological) barriers when faced with the initial decision to invest in an EMR system. 50
Healthcare service at a distance
Traditional telephone services were the milestones of modern telemedicine. Implementing electronic communication applications with high computational power enables the control of operations at a distance possible. Although reducing medical errors is an international agenda, physicians still commit different types of errors during manual medical data processing incurred during recording and/or fail to timely record health care data. 35 Errors associated with medical data are common and costly. However, the social, spiritual, psychological, and ethical scopes of the technology, as well as the technical feasibilities of the technology, must be considered, and all stakeholders must contribute while planning and implementing new health care technologies. The PBR systems are practically more error-prone, however, the mere replacement of the system with EHR could not ensure accuracy. 34 Hence, efficient processing, usage, and storage of medical data are important for both clinical and public health decisions.
The future perspectives
The promising EHR implementation systems, people, process, and product factors play an integral role in the fate of its implementation. 11 The stakeholder’s benefit from the systems which protect the patient’s need and ensure their privacy. 24 The access to accurate and complete clinical information is the main component of effective decision making. 69 This is facilitated by decision-support EHR tools - BPAs - and designed for behavioral health integration with the needs of health care institutions and the benefits of improving the patient experiences, 36 for instance, alcohol use. 30 The system can be used to update the current condition of a patient as input to obtain a corresponding recommendation for medical tests, possible diseases, and treatment plans. 69 Research indicated that the EHR “active choice” significantly increased influenza vaccination rates and ordering of colonoscopy and mammography screening services. 8
The successes or challenges of voice input application can be used to transcribe doctor’s dictation and facilitate the collection, indexation, storage, and retrieval processes of medical information. 17 According to a study, EHR promotes services but could not favor collaborative team’s culture and professionals. 70
The shift in the use of EHR by the health builds trusts and presents an opportunity to monitor admission, diagnosis, and outcome to inform public health policy and service provision. 58 The EHR vendors should be encouraged to incorporate social knowledge networking features into the systems. 71
Authors have also identified two issues demanding the researcher’s attention for more elaborated reasons for uncertainties. First, one national level research reported the adoption level of EHR as it was higher in rural practices than urban counterparts, reversing the earlier trends. 72 Another similar research also reported the necessity of considering the patient’s behavioral aspects while using the tool during patient rendering procedures so as to increase patient’s engagement level. 73 These issues may call for behavioral scientists to address this particular patient concern.
Medical data processing is one of the most basic tasks of the health care professionals. Computerized physician order entry applications having decision-support fields reduce avoidable medical errors using inbuilt memory aid. These automatic notification alert signals enable appropriate and timely intervention that ensures safer and efficient health care. The design policies of electronic technology must meet pre-stated standards and guidelines to ensure confidentiality. User-friendly technologies ensure the efficient and timely transfer of health care data for quality patient care meeting the needs of the patients and the organization.
Abbreviations
Author contributions
All authors contributed towards data analysis, drafting and critically revising the paper, gave final approval of the version to be published and agree to be accountable for all aspects of the work.
The authors report no conflicts of interest in this work.
Log in using your username and password
- Search More Search for this keyword Advanced search
- Latest content
- Current issue
- JME Commentaries
- BMJ Journals More You are viewing from: Google Indexer
You are here
- Online First
- Patient data for commercial companies? An ethical framework for sharing patients’ data with for-profit companies for research
- Article Text
- Article info
- Citation Tools
- Rapid Responses
- Article metrics

- http://orcid.org/0000-0001-7460-0154 Eva C Winkler 1 ,
- Martin Jungkunz 2 ,
- Adrian Thorogood 3 ,
- Vincent Lotz 1 ,
- Christoph Schickhardt 2
- 1 Section for Translational Medical Ethics, Department of Medical Oncology, National Center for Tumor Diseases , University Hospital Heidelberg , Heidelberg , Germany
- 2 Section for Translational Medical Ethics, National Center for Tumor Diseases , German Cancer Research Center , Heidelberg , Germany
- 3 Terry Fox Research Institute , Montréal , Québec , Canada
- Correspondence to Dr Eva C Winkler, Section for Translational Medical Ethics, Department of Medical Oncology, National Center for Tumor Diseases, University Hospital Heidelberg, Heidelberg 69120, Germany; eva.winkler{at}med.uni-heidelberg.de
Background Research using data from medical care promises to advance medical science and improve healthcare. Academia is not the only sector that expects such research to be of great benefit. The research-based health industry is also interested in so-called ‘real-world’ health data to develop new drugs, medical technologies or data-based health applications. While access to medical data is handled very differently in different countries, and some empirical data suggest people are uncomfortable with the idea of companies accessing health information, this paper aims to advance the ethical debate about secondary use of medical data generated in the public healthcare sector by for-profit companies for medical research (ReuseForPro).
Methods We first clarify some basic concepts and our ethical-normative approach, then discuss and ethically evaluate potential claims and interests of relevant stakeholders: patients as data subjects in the public healthcare system, for-profit companies, the public, and physicians and their healthcare institutions. Finally, we address the tensions between legitimate claims of different stakeholders in order to suggest conditions that might ensure ethically sound ReuseForPro.
Results We conclude that there are good reasons to grant for-profit companies access to medical data if they meet certain conditions: among others they need to respect patients’ informational rights and their actions need to be compatible with the public’s interest in health benefit from ReuseForPro.
- ethics- medical
- health care economics and organizations
- philosophy- medical
- public policy
- ethics- business
Data availability statement
Data sharing not applicable as no datasets generated and/or analysed for this study. Not applicable since no data were generated.
This is an open access article distributed in accordance with the Creative Commons Attribution Non Commercial (CC BY-NC 4.0) license, which permits others to distribute, remix, adapt, build upon this work non-commercially, and license their derivative works on different terms, provided the original work is properly cited, appropriate credit is given, any changes made indicated, and the use is non-commercial. See: http://creativecommons.org/licenses/by-nc/4.0/ .
https://doi.org/10.1136/jme-2022-108781
Statistics from Altmetric.com
Request permissions.
If you wish to reuse any or all of this article please use the link below which will take you to the Copyright Clearance Center’s RightsLink service. You will be able to get a quick price and instant permission to reuse the content in many different ways.
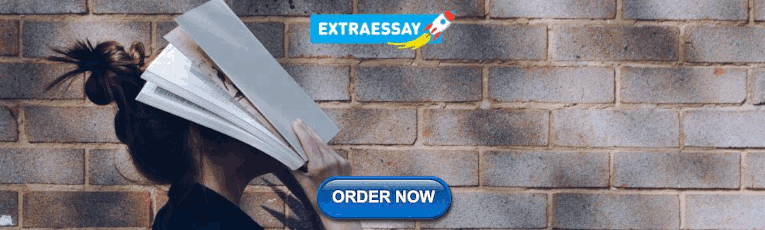
Introduction
Secondary use of medical data for research purposes is a promising approach to advance medical science and improve healthcare. Currently, many initiatives around the world are setting up infrastructures to enable systematic secondary research use of data generated in the healthcare system. 1 The development of such infrastructures has prompted ethical and legal debates about, for example, appropriate consent models, 2 approaches to privacy protection, and oversight of data access and use by academic researchers. 3 However, academia is not the only sector that expects secondary use of medical data to be of great benefit. The pharmaceutical and tech industry is interested in so-called ‘real-world’ health data not only for the launching and postmarketing surveillance of their products but increasingly also for research and development of new drugs and artificial intelligence (AI) solutions for healthcare. Medical data are expected to play an important role in informing research decisions about unmet needs, to serve as synthetic control arms in new trial designs, to optimise trial recruitment, and to understand safety and efficacy profiles. 4 5 They are the basis to develop, train and validate AI applications. 6 Hence, a crucial and obvious ethical question needs to be resolved about the reuse or secondary use of medical data generated in the public healthcare sector for medical research by for-profit companies (ReuseForPro): Should access be granted to for-profit companies to use medical data for research, and if so, how and under which conditions?
Some large-scale data initiatives that aim to enable reuse of patient data for research and data science allow access by for-profit companies in principle. Examples are the German Medicine Informatics Initiative that aims to collect clinical data from German university and affiliated hospitals 7 or the Mayo Clinic’s clinical data analytics platform. 8 Other initiatives like CancerLinQ—a platform for sharing clinical data from patients with cancer from healthcare IT systems all over the USA—only provide clinical data to ‘non-profit companies that can generate practical knowledge to shape the future of cancer care.’ 9
ReuseForPro is also a politically sensitive topic with the potential to cause public debate and concern as shown by two cases from the UK (for an overview, see Horn and Kerasidou 10 ). In 2013, the National Health System (NHS) announced its intention to collaborate with for-profit companies and made medical data collected from hospitals and general practitioners available for research by for-profit companies. 11–14 This initiative was publicly criticised for lack of transparency and public communication about the handling of patient records. Single hospitals also started sharing health records for ReuseForPro. The Royal Free Hospital in London did so in a cooperation with Google DeepMind which was publicly viewed as violation of patients’ privacy rights and data protection law. 15 16
Studies on patients’ willingness to share their medical data for ReuseForPro show a pattern of consistently lower approval rates for commercial research compared with academic research. One representative study with German participants shows a marked drop from 97% approval to supporting academic research with their data to 17%. 17 Other German studies found a similar, though less pronounced difference in acceptance of data use by academic versus commercial research. 18–20 In other countries, acceptance rates for ReuseForPro range between 50% and 60%, as has been shown for Australia 21 and the UK. 22 Moreover, in the UK study in question, 44% of those participants who were not willing to share their data with for-profit companies ‘earlier in the survey, agreed […] that research should be conducted by commercial organisations if, otherwise, the research would not take place.’ 22 Finally, some studies show that public benefit is a key factor moderating patients’ acceptance of ReuseForPro. 21
While we have seen some empirical data on patients’ willingness to provide their data for ReuseForPro, there is a need for a more comprehensive normative debate. Some authors are primarily concerned with the use of data collections held by private companies; they highlight the importance of public benefit 23 and improving quality of care 24 through research use of medical data. Graham presents four criteria that must be met for health data sharing with private companies to be trusted: transparency, accountability, representation and a clear social purpose. 25 Referring to the NHS of the UK, Horn and Kerasidou 10 argue for public–private partnerships for reuse of medical data, designed on the basis of solidarity and public benefit. However, the field still lacks a broader ethical framework that includes all relevant stakeholder perspectives on ReuseForPro. Hence, the main goal of this article is to start such an ethical conceptualisation of the debate on ReuseForPro. For this purpose, we first clarify some basic concepts and our ethical-normative perspective. We then discuss and ethically evaluate potential claims and interests of relevant stakeholders. Finally, we address the tensions between legitimate claims of different stakeholders to suggest conditions that might ensure ethically sound ReuseForPro.
Basic concepts and definitions
The focus of this paper is the reuse of medical data from the public healthcare sector for medical research by for-profit companies (ReuseForPro).
By 'medical data' we mean data of patients generated in and for the primary purpose of patient care. This includes data from preventive, diagnostic and treatment measures, but also data recorded for billing and reimbursement of medical services. In general, medical data are deidentified prior to any secondary use, that is, identifying characteristics such as names, date of birth are removed or replaced by a code. However, despite deidentification, there typically remains a residual risk of reidentification for patients. Thus, the data are usually treated as personal/person-related data falling under privacy and data protection norms. 3
We define the public healthcare sector by the source of funding of the infrastructure and of the healthcare delivery. Consequently, healthcare institutions in which infrastructure and healthcare are publicly financed are the ‘purest’ form of public healthcare. If the infrastructure is financed privately, as are, for example, private practices or hospitals, but the healthcare for patients is financed publicly, this represents a hybrid form according to our definition. For the sake of the argument, we will focus on the pure form of the public healthcare sector.
By ‘sharing medical data with companies’ we mean that companies receive access to the data and can use it for research purposes. Access can be operationalised in distinct ways. Traditionally, the hospital transfers the data directly to the companywhere the data analysis is performed. Alternatively, for greater security, the hospital may only provide the company with access to data that remains ‘in place’. 26 The company sends a computational analysis algorithm to the hospital, the hospital applies the algorithm to the relevant data set (ie, processes the data), and solely aggregated results are sent back to the company. In this way, patient data do not leave the walls of the hospital; only the aggregated results of the analyses are sent to the company. 27 28
Following the different forms of research indicated in several recitals of the EU, GDPR, 29 by medical research we mean research at all stages—basic, translational or applied research—in all fields of medicine and medical technologies, including IT and AI products for the healthcare sector, for generating scientific knowledge aiming at potential application in healthcare. We do not consider other forms of research using medical data like market research to fall under the rubric of medical research.
By for-profit companies we mean companies that participate in market competition, pursue the primary goal of profit making and are owned by private persons or holdings in private ownership. The specifics of the legal form are not relevant here.
Basic normative principles for ethical assessment of involvement of private companies
Before ethically analysing and assessing potential claims and duties of involved stakeholders, we need to roughly define our ethical approach, that is, the ethical-normative framework we rely on. The ethical analyses will be carried out from a position of liberalism inspired by John Rawls’ ‘A Theory of Justice’. Following Rawls’ interpretation, we take his theory as an approach that aims to transfer and apply the basic Kantian idea of respect for persons (as an end, not as means) to the area of political philosophy and social justice. According to this rights-based approach, all persons should be guaranteed an equal system of liberty rights. Several of these rights are of relevance for this article such as the right to privacy and informational self-determination and the right to academic freedom (freedom of research). However, according to the perspective of political liberalism, persons are not only entitled to liberty rights but also have certain duties to others and society. In Rawls’ thought experiment, people behind a veil of ignorance, that is, without knowledge of their position in society, would agree that everybody deserves support to really and substantially be able to use her liberty rights. Citizens, thus, have the duty to assist others to be able to use these liberties as well as the duty to support just institutions of the liberal-democratic society. From the liberal-egalitarian ideal of a democratic society ordered according to the rule of law by a constitutional state (Rechtsstaat), we also deduce general civic values and principles of relevance for our ethical analysis: the principles of transparency, accountability and liability as well as the principle of participation and representation in decision processes.
When defining the ethical framework of our investigation, the question of the moral status of companies arises and whether and to what degree they can be attributed duties, rights and responsibilities at all. Due to limited space, we cannot adequately portray the rich discussion on this topic across fields of social ontology, theory of collective agency, philosophy of law, social ethics and business ethics. Instead, we provide a pragmatic and basic account of ascribing rights, duties and responsibilities to companies, based on a few elementary considerations. First, companies have constitutional rights in western liberal and democratic states. For instance, companies have the basic right to freedom of scientific research according to the German constitutional law and to the EU Charter of Fundamental Rights. 30 One reason from legal theory and philosophy for granting determined constitutional rights to companies is to treat them as a placeholder of the rights of the individuals who own them and act through them. 31 Although the specific moral rights, obligations and responsibilities of companies are subject to diverging positions and debates i , there is a widespread basic consensus that ascribing rights, duties and responsibilities to corporations is meaningful, plausible and ethically justified. ii
Second, in terms of concrete rights and obligations, it is morally justified that privately owned businesses, including corporations, have the right to do business, to strive for profits on markets, and to carry out research and development. In compensation for their rights, they have moral obligations and responsibilities determined by legal obligations towards society, employees, customers or the environment. Again, there is a broadly accepted basic consensus that we should have an economic order that allows private business activities including privately owned corporations which compete on markets. iii This is the elementary normative approach for the ethical analysis of companies’ legitimate claims and obligations concerning ReuseForPro, which will ultimately be determined in reference to specific features and circumstances of the relevant context in the next section.
Morally relevant interests at stake and their ethical assessment
Based on this normative framework, we will now assess the potential claims and expectations of the respective stakeholders regarding ReuseForPro: patients, for-profit companies, the public and physicians and their healthcare institutions.
Patients as data subjects in the public healthcare system
When it comes to secondary use of their medical data, patients have several legitimate claims and expectations. Some of them apply to all types of secondary use of medical data, others are specific to ReuseForPro. We distinguish here between the current patients, whose data are to be used, versus the public and future patients (see ‘The public and future patients’), because the rights and interests of each group are affected in very different ways as the following aspects show.
Medical confidentiality
Patients have a legitimate expectation in a trusting relationship with their physicians. This trust is based on the quality of their physicians’ care, their commitment to the patients’ best interest as a priority goal, and to a relevant degree on the physician keeping personal information confidential. 32 , iv The patient’s right to confidentiality is firmly established in medical ethics and law. With ReuseForPro, medical confidentiality is affected if medical data that leave the protected realm of the patient–physician relationship is not adequately protected from unauthorised access and reidentification. In general, deidentification is a pivotal measure for protecting confidentiality. Technical safeguards including secure access mechanisms (see ‘Basic concepts and definitions ’) may add additional layers of protection against reidentification.
Informational self-determination
As referred to in the section ‘Basic normative principles for ethical assessment of involvement of private companies’, one of the relevant basic rights is the moral right to privacy and informational self-determination. We conceive the right to informational self-determination as follows: ‘The right to informational self-determination protects a person’s ability to freely decide whether and how personal data and information about her are collected, stored, multiplied, processed and transferred by third parties […]. In the following, we use the term informational self-determination instead of (informational) privacy for it better captures that the right is about actively determining what happens with one’s personal data and information.’ 33 This right becomes particularly important when it comes to highly sensitive medical data.
Representation and patient involvement
Out of a wish to govern the use of their data, patients might expect to be included in decision-making bodies of data initiatives. Such a form of participation is already being realised in some places through patient representatives on data access and use committees. This claim is substantiated by the principle of representation. Furthermore, involving patients has the potential to foster trust in the governance of data and improve the quality of oversight.
Interest in clinical benefit
In research activities such as phase III clinical trials where patients potentially gain a clinical benefit, the interest of participating patients in this clinical benefit is a valid moral claim to be factored into the overall ethical assessment of a study. In other research activities, it is highly unlikely that participating patients will benefit, so that appropriate information is required to avoid false hopes and therapeutic misconception. We assume that ReuseForPro is highly unlikely to generate clinical benefit for data donating patients due to the lengthy development and approval process for new therapies or medical products. Therefore, individual clinical benefit should play no role in the ethical assessment of ReuseForPro. The envisaged benefit of ReuseForPro is for future patients and the public (discussed in the section ‘The public an future patients’).
Share in profits
Patients might claim a right to a share in the profits of commercial success of products developed with their medical data. To make that claim, patients could refer either to the principle ‘to each person according to her effort’ or ‘to each person according to her contribution’ 34 as a principle of justice for the sharing of profit. However, we doubt that either claim can substantiate a case for patients’ right to have a share in profits from commercial success of products from ReuseForPro. As far as additional effort is concerned, medical data are traditionally generated by doctors and other specialists using the resources and infrastructure of the public health system as part of the individual care of patients. For patients, ReuseForPro creates little or no relevant extra effort, work, or investment other than the time during the information and consent process to decide whether or not to allow access to their diagnosis and treatment data when asked. The data are just a first level of effort in the highly complex, long and resource-intensive process of developing a successful medical product that requires significant efforts by the company.
The contribution principle emphasises that patients’ medical data are as a matter of fact—and completely independently from any effort, labour or investment—an indispensable contribution for the development of a profitable product based on ReuseForPro. Hence, patients can legitimately say that they contribute to data collection and that they want a share in the profits in proportion to their admittedly small contribution. Here, we would first point out that the data themselves and even new scientific insights do not typically generate profit directly. More importantly, we would argue along with other scholars 35–37 that these medical data should be a public resource, for the following reasons. The data themselves are generated in a publicly funded health system. The public and tax-payer investment in the healthcare infrastructure including the training and education of staff and the payment for diagnostics and therapies justify a claim on using them for public benefit. Hence, while medical data certainly fall under patients’ control required by the right to informational self-determination, patient ownership rights cannot necessarily be deduced.
Accountability and liability
Patients are entitled to clear accountability structures and compensation in the event of adverse consequences from ReuseForPro, a claim based on our principle of accountability and liability described above. Harm mitigation bodies have been suggested by some scholars for situations where data subjects experience harms arising from digital data use in the big data context. 38 While this suggestion is convincing in principle, the challenge lies in the implementation—that is, how exactly to demonstrate harm caused by ReuseForPro that may result from, for example, privacy breaches or negative ratings by predictive analytics. Provisions for either legal remedies or harm mitigation are, however, specific to neither the distinction between academic and for-profit data initiatives nor for healthcare or other economic sectors.
For-profit companies
Pharmaceutical and biotech companies make for a significant proportion of research and development in the healthcare sector. If for-profit companies invest in such research driven endeavours, they might make the following claims.
Freedom of research and right to access patient data
For-profit companies in the health sector are entitled to freedom of research. Freedom of research is primarily a defensive right that restricts the influence of state institutions on research activities, among other things, to the extent that researchers, and thus also private company researchers, are free to decide on their choice of research subject. Going beyond the defensive function, for-profit companies might additionally claim that the fundamental right to freedom of research constitutes a right to access patient data stored in publicly sponsored data sharing infrastructures. In fact, research-based pharmaceutical companies have repeatedly criticised that they are not given access rights to patient data, for example, under the Patient Data Protection Act in Germany. 39 However, it is not clear how freedom of research, as a defensive right, can substantiate a claim for access to medical records of patients stored in publicly funded infrastructures. For-profit companies might even have an interest in exclusively accessing real-world patient data for their research to keep competitors at arm’s length. However, exclusive access 40 would restrict rather than stimulate competition—which would tend to be in the interest of the company but not the interest of the greater public. What private companies can legitimately demand is a fair playing field so that there are equal and fair conditions of access for all companies alike. So, in principle, any data access provided to the private sector should be non-exclusive and non-discriminatory. v
Profitability
Certainly, private companies in the healthcare sector have a legitimate interest to generate profits and thrive on the market. The ideal way to achieve this goal is to develop high-quality products that generate real added value and address a need in an area with a high burden of disease. For these kinds of innovations societies are willing to pay high prices. While high market profits are in the company’s interest, two ethical debates are connected to the pricing strategies of pharmaceutical companies: first, whether it is legitimate to demand the highest possible price that the market allows if this puts the respective healthcare systems under great financial pressure. And second, whether pharmaceutical companies have ethical obligations to charge fair prices for essential medicines. These debates are particularly relevant for products developed with data from patients and from a publicly funded healthcare system. While companies have a legitimate right to pursue and realise profits, in the case of ReuseForPro particular restrictions to this right are justified if necessary to safeguard social benefit of ReuseForPro. 41
Corporate (social) responsibility
Corporate responsibility implies to take on social responsibility beyond the companies’ actual business purpose and is an expectation of society vis à vis private companies. At the same time, the formulation of a corporate social responsibility (CSR) strategy and the corresponding activities are generally also intended to improve the company’s image. In our context, companies working with patient data have an interest in CSR ideally out of a felt duty to protect patients’ privacy or generate value for society and certainly in order to build trust in their business model. This amalgamation of two goals—real assumption of responsibility and image cultivation—makes it necessary to check carefully whether companies live up to what they promise. Especially in the new field of AI development, concrete expectations towards corporate responsibilities of companies in developing new AI products are being formulated e.g., with a list of criteria for trustworthy AI of the European AI Alliance 42 or the Montreal declaration for responsible AI. 43
The public and future patients
There are two moral bases to justify claims by the public regarding ReuseForPro: for one, the public and those paying into health insurance finance the infrastructure and the healthcare personnel that generate clinical data; second, the state as legitimate representative and instrument of the people within a liberal-democratic order has the right and duty to ensure that all activities remain in compliance with the law and benefit the people; this holds in particular for activities with a strong public relevance and involvement such as the secondary use of medical data from the public healthcare system via a publicly funded data infrastructure.
Public benefit
The public and prospective patients have an interest in ReuseForPro as it has high potential to benefit society. 44 A strong orientation towards the public benefit (common good) is critical for public acceptance and trust concerning ReUseForPro, 10 and essential for its moral justification. Research with patient data generated through everyday healthcare encounters could promote drug safety for patients, make clinical trials more efficient, substitute placebo controls by so-called virtual control arms with existing patient data and increase the quality and cost-effectiveness of the health system with AI tools, for example, for decision support. Since pharmaceutical and biotech companies make for a significant proportion of research and development in the health sector, there are good reasons to assume that ReuseForPro will exploit the potential of medical data along a spectrum from high public benefit in post-market surveillance data to potential long-term benefits when used for research and development.
Return to the healthcare system
The public has a morally legitimate interest to receive a return from private entities for providing them with the opportunity to use patient data for secondary research purposes. This return can take many different forms that contribute to the maintenance and improvement of the healthcare system: increase of scientific medical knowledge; medical products that help improve the prevention, diagnosis or care of disease at a reasonable cost; or fees for data access that can be reinvested in healthcare. 45
Trust in the healthcare system
The public has an interest in ensuring that citizens and patients trust the public healthcare system and its institutions. Empirical studies show that the acceptance of the use of data by commercial users is significantly lower than acceptance of data use by academic users (see ‘Introduction’). ReuseForPro is a publicly and politically delicate endeavour and has the potential to seriously compromise public trust. 10
Effective competition
The public has a legitimate interest in ensuring that ReuseForPro is consistent with effective market competition and does not exacerbate existing imbalances in the market due to accumulated market power of individual companies. Effective competition including global competition involves preventing the formation of monopolies and oligopolies. Access to patients’ data for big players like Amazon or Alphabet (google) in ReuseForPro, as, for instance, in the case of NHS, could reinforce existing monopolies, and therefore, needs to be handled and regulated with particular attention and caution.
Promotion of national companies
If we conceive the public as the people of a state, the public has an interest in timely access to high-quality healthcare products, which is promoted by competition nationally and globally. The public might also have a special interest in promoting national companies or, in the case of EU members, at least companies of the EU if research and development take place ‘at home’ and taxes are paid within the state. National companies might also be perceived as more trustworthy in the public opinion and can be held accountable within the national jurisdiction. These reasons might serve as a weak argument for granting preferred access to national companies. However, data are not a limited resource since they are reproducible and such preferred access must not forestall effective competition.
Healthcare institutions and physicians
Hospitals and doctors’ offices are ultimately the places where patient data are generated. While public hospitals need to invest in information technology, infrastructure and finance personnel, physicians and nurses contribute a large and important part of the data through their documentation of patient treatment. For these reasons, healthcare institutions and physicians might make the following claims.
Ownership of health data
Physicians might claim that the data only exist because they collected and documented them, and therefore, they own their patients’ data. Likewise, hospital managers and the institution could claim that it is their stewardship of resources and governance that ensures functionality of the healthcare delivery in their institution and data collection is an important element of this and therefore the data belongs to the hospital. From these ownership claims, physicians and hospital managers could derive an entitlement to deny data use by private companies, or to condition such access on a share in the eventual profits. However, data ownership claims of physicians and hospitals are problematic. First and foremost, the concept of data ownership of second parties itself conflicts with patients’ right to informational self-determination. Second, medical data are not generated as an end in itself but as part of physicians’ primary task to provide state of the art healthcare. Third, actors such as technicians (eg, in connection with MRI) or laboratory staff also produce a relevant part of the data and would therefore also have a (partial) claim to data ownership. Fourth, with regard to the ownership claims of hospital management, most of the resources used for diagnostics and thus for data generation are financed with public money. The argument from the resources invested in data generation thus argues, if anything, for ownership claims on the part of society, with hospitals playing an important role as responsible stewards of this resource. However, to the extent to which ReuseForPro is associated with additional burdens, efforts and costs for hospitals or physicians, they have certain legitimate claims for compensation or reward for these additional efforts (see next section ‘Compensation for additional effort’).
Compensation for additional effort
Contrary to popular belief, the data are not just there as a treasure waiting to be exploited. To make the data usable, they must be documented in a uniform and structured manner and their context of origin must be annotated with sufficient accuracy in metadata. 46 This is associated with relevant additional data work, which does not necessarily have to be carried out by the physicians themselves. Especially since physicians today rightfully complain that they spend more and more time on documentation and data entry at the expense of time with the patient, it is important to see that additional standardisation and documentation are usually required to make the data usable for ReuseForPro. Hence, healthcare providers and individual physicians contributing data for ReuseForPro have a legitimate claim to be relieved from or compensated for any additional efforts for data procurement and documentation—meaning any extra effort beyond what they are obliged to do for ensuring quality patient care.
Interest in exclusive research with ‘own’ patient data
Healthcare institutions and physicians with academic affiliation might be interested in research with patient data themselves, especially if they have an academic mandate to do so. They might also team up with for-profit companies for joint research projects that may also lead to commercial outputs. Since data can be duplicated and are, therefore, a non-rivalrous resource for health research, access should be non-discriminatory meaning that academic researchers and private sector health researchers should have equal access to the data if a return to the healthcare system is secured and if patients have consented to ReuseForPro. If the data - requesting company pursues a research goal similar to that of an internal academic research project, there might be a situation of competition between the company on the one hand and the academic researchers or hospital on the other hand. Should this be the case, clear rules based on public value and patients’ consent to reuse data by academic and commercially oriented researchers are pivotal.
Table 1 maps potential claims of stakeholders, weighs them tentatively according to the ethical assessment and lists strategies for mitigating tensions between claims.
- View inline
Moral claims of stakeholders and mitigation strategies
Justification and requirements for the secondary use of patient data by private companies
In the following we discuss how potential tensions between the different stakeholders’ claims and interests explored above can be appropriately handled and mitigated in ReuseForPro. The most important areas of tension are for one, between the public interest and companies’ interest in profit maximisation and, second, between the individual’s right to informational self-determination and the public interest in maximising data utility.
The legitimate public interest in benefiting from research with patient data can go hand in hand with the company’s interest in generating profit with products based on this data. However, the public’s and the company’s interests can also come into conflict if the product does not convey any real added value, is overpriced or is not available on the domestic market at all. As stated above, it can be rightfully expected that ReuseForPro creates public benefit since the patient data are generated in the public healthcare system. This public interest standard is also underlined by the social responsibility of private companies and the principles of accountability. Hence, companies need to accept constraints on their liberty to pursue profit and the state must create framework conditions that ensure an appropriate return of benefits. First suggestions might be:
Limit ReuseForPro to research that aims to improve health or the healthcare system. 10 This would exclude, for example, uses for marketing, product placement or seeding trials. Within the limits of this goal, however, the private company is free to choose whatever research area it wants to develop and invest in.
Ensure and document a return from ReuseForPro that contributes to better health or healthcare, 25 for example, via health products themselves or payments for health data that can then be used to finance health programmes. The donating healthcare system should have preferential access to products developed with ReUseForPro. 10 Apart from an ex ante negotiation about potential returns, an ex post documentation and track record for each company about the products and benefits generated with patient data are ways to satisfy the principles of accountability and transparency. 25 Accountability implies setting up a governance system that gives a public account of the kinds of contracts governing secondary use; reasons and goals of usage; scientific quality, effects and outcomes on the project level; and more general information about a company’s mission, financial ties and contribution to the health sector.
If benefits are returned to the healthcare system, companies are free to generate profit especially with innovative products. The upper limit to pricing is dictated by the fair pricing argument that demands that products should not be overpriced and profits should be limited when high costs for vital products threaten the viability of the public healthcare system. One way to put this into practice would be to condition data access on fair commercialisation downstream.
Ideas on how to ensure this include obliging companies to register their research in advance in publicly accessible study registries to create scientific transparency and to create transparency about the level of public data support received by companies. Another idea is to clearly indicate in price negotiations to what extent their products were developed with patient data. If companies do not adhere to fair pricing, they can be excluded from further data use for a certain period of time. We advise against the introduction of legal sanctions as we believe that public moral pressure or fear of reputational damage is enough to motivate most companies to ensure fair marketing downstream.
A second area of tension is the patient’s right to informational self-determination and the public interest in the maximisation of the use of the data. While this conflict arises for data sharing generally, it is particularly acute for ReuseForPro, considering its relatively low acceptance rate. Hence, risks and benefits must be especially well explained. Since consent to ReuseForPro cannot be assumed, it is ethically necessary that patients get the option to either approve or reject ReuseForPro as an extra option separately from all other consent content. This includes complying with the above outlined principles of transparency and accountability as an indispensable condition for ReuseForPro.
Patients expect that their personal data is kept confidential. ReuseForPro governance should hold companies accountable for data security, protecting patients’ privacy and minimising risks. To this end, technical solutions like secure access mechanisms 10 (see ‘Basic concepts and definitions ’) wherever feasible are warranted, and different governance bodies may be needed depending on the character of ReuseForPro or the nature of the company—for example, whether it is a big tech company’s research and development department or a smaller start-up company that does basic research in AI solutions for the health sector.
The legitimate interests of parties affected should be represented in the process to set up and monitor data uses as underlined by the principle of representation and participation. 25 One important instrument in this regard are data access and use committees that may also include patient representatives.
In summary, even though private companies have rights to freedom of research or to pursue profit, these do not extend to a moral right to access and use medical data. There are, however, good reasons for patients and data access and use committees to grant private companies access if they meet certain conditions: above all companies need to respect patients’ informational rights and act in accordance with the public’s interest in health benefit from ReuseForPro.
The public has a legitimate interest in ReuseForPro for the sake of public benefit but has no morally justifiable claim obligating patients to provide their medical data for ReuseForPro. However, the public has an ethically justifiable claim towards the physicians and healthcare institutions of the public healthcare system to support ReuseForPro provided physicians and institutions are compensated for the additional effort required to provide the data. All things considered, secondary use of patient data by for-profit companies is not only justifiable but may even be mandated under certain conditions.
Ethics statements
Patient consent for publication.
Not applicable.
- Jungkunz M ,
- Köngeter A ,
- Mehlis K , et al
- Dagenais S ,
- Madsen A , et al
- Christie D , et al
- Hocking L ,
- Altenhofer M , et al
- Medical Informatics Initiative Germany (MI-I)
- ASCO CancerLinQ
- Kerasidou A
- Temperton J
- Laurie GT ,
- Dixon-Woods M
- Richter G ,
- Borzikowsky C ,
- Lesch W , et al
- Hoyer BF , et al
- Niemiec E , et al
- Schickhardt C ,
- Jungkunz M , et al
- Braunack-Mayer A ,
- Fabrianesi B ,
- Street J , et al
- Wilbanks JT ,
- Spector-Bagdady K
- Grossmann RL ,
- Maryellen LG ,
- Julie A , et al
- Isaeva J , et al
- GDPR. European Union
- Cornelius K ,
- Lockley E , et al
- Fleischer H ,
- Beauchamp TL ,
- Childress JF
- Ballantyne A ,
- Schaefer GO
- Ballantyne A
- McMahon A ,
- Prainsack B
- Deutsches Ärzteblatt
- Sengupta S ,
- Rossetti Née Collins S , et al
- Liddicoat J ,
- European AI Alliance
- Montreal Declaration Responsible AI
- Trinidad MG ,
- Ó Laoghaire T
- Platz J von
Contributors ECW set the subject matter, conceptualised the argument, wrote and revised the draft manuscript in close cooperation with MJ, CS and input from AT. MJ revised and updated the summary of the empirical and existing ethical literature and drafted several passages in the chapter on stakeholder interests. MJ contributed to the overall process of structuring and drafting the manuscript. AT contributed his expertise on governance and policy of large data initiatives, drafted the formulation of some passages, and contributed to initial and to the final comprehensive revision. VL was responsible for literature research of the empirical literature and summarised his findings in initial drafts of the presentation of the empirical literature in the introduction. CS drafted a first conceptual structure and contributed to all stages of the manuscript, in particular to the section about the normative framework. ECW is responsible for the overall content and the guarantor.
Funding The authors have not declared a specific grant for this research from any funding agency in the public, commercial or not-for-profit sectors.
Competing interests ECW and CS have been receiving grants by the German Ministry of Education and Research (BMBF) in the frame of the German Medical Informatics Initiative (MII) and have been involved in the MII Working Group "Consent" .
Provenance and peer review Not commissioned; externally peer reviewed.
↵ See for instance, Peter French’s prominent account of corporations as moral persons (that French subsequently revised). 47
↵ For accounts of corporative duties, see Ó Laoghaire. 48
↵ As one instance of this basic theoretical consensus, one might refer to a range of philosophical positions that includes libertarianism, 49 welfare state capitalism, property-owning democracy and liberal socialism (for private ownership of production measures in the two latter models according to Rawls, see von Platz 50
↵ Nuffield Report on Big Data: Medical confidentiality protects patients from harm in two ways: it both encourages them to disclose information essential to their treatment, so that they do not suffer the harm of untreated disease, and it provides assurance against any harm that may occur to them from a more general disclosure of the information. Over time, respecting confidence helps to foster trust . 32
↵ The Data Governance Act is a bit more nuanced for access to public sector data in that it admits the exceptional possibility of commercial exclusivity in specific cases, however this seems not directly transferable to the research scenario since research can be done in competition and is not based on exclusive data access. https://eur-lex.europa.eu/legal-content/EN/TXT/?uri=CELEX%3A32022R0868 . Recital 13
Read the full text or download the PDF:
Other content recommended for you.
- How should we think about clinical data ownership? Angela Ballantyne, Journal of Medical Ethics, 2020
- Multiple modes of data sharing can facilitate secondary use of sensitive health data for research Tsaone Tamuhla et al., BMJ Global Health, 2023
- Should free-text data in electronic medical records be shared for research? A citizens’ jury study in the UK Elizabeth Ford et al., Journal of Medical Ethics, 2020
- Patients’ and public views and attitudes towards the sharing of health data for research: a narrative review of the empirical evidence Shona Kalkman et al., Journal of Medical Ethics, 2019
- Glossary for public health surveillance in the age of data science Arnaud Chiolero et al., Journal of Epidemiology and Community Health, 2020
- The social licence for research: why care.data ran into trouble Pam Carter et al., Journal of Medical Ethics, 2015
- Building a house without foundations? A 24-country qualitative interview study on artificial intelligence in intensive care medicine Stuart McLennan et al., BMJ Health & Care Informatics, 2024
- Control, trust and the sharing of health information: the limits of trust Soren Holm et al., Journal of Medical Ethics, 2020
- Meta consent: a flexible and autonomous way of obtaining informed consent for secondary research Thomas Ploug et al., BMJ: British Medical Journal, 2015
- Safeguarding the future of independent, academic clinical cancer research in Europe for the benefit of patients Anastassia Negrouk et al., ESMO Open, 2017
- Alzheimer's disease & dementia
- Arthritis & Rheumatism
- Attention deficit disorders
- Autism spectrum disorders
- Biomedical technology
- Diseases, Conditions, Syndromes
- Endocrinology & Metabolism
- Gastroenterology
- Gerontology & Geriatrics
- Health informatics
- Inflammatory disorders
- Medical economics
- Medical research
- Medications
- Neuroscience
- Obstetrics & gynaecology
- Oncology & Cancer
- Ophthalmology
- Overweight & Obesity
- Parkinson's & Movement disorders
- Psychology & Psychiatry
- Radiology & Imaging
- Sleep disorders
- Sports medicine & Kinesiology
- Vaccination
- Breast cancer
- Cardiovascular disease
- Chronic obstructive pulmonary disease
- Colon cancer
- Coronary artery disease
- Heart attack
- Heart disease
- High blood pressure
- Kidney disease
- Lung cancer
- Multiple sclerosis
- Myocardial infarction
- Ovarian cancer
- Post traumatic stress disorder
- Rheumatoid arthritis
- Schizophrenia
- Skin cancer
- Type 2 diabetes
- Full List »
share this!
May 13, 2024
This article has been reviewed according to Science X's editorial process and policies . Editors have highlighted the following attributes while ensuring the content's credibility:
fact-checked
peer-reviewed publication
trusted source
Study shows ChatGPT can accurately analyze medical charts for clinical research, other applications
by UT Southwestern Medical Center

ChatGPT, the artificial intelligence (AI) chatbot designed to assist with language-based tasks, can effectively extract data for research purposes from physicians' clinical notes, UT Southwestern Medical Center researchers report in a new study.
Their findings , published in npj Digital Medicine , could significantly accelerate clinical research and lead to new innovations in computerized clinical decision-making aids.
"By transforming oceans of free-text health care data into structured knowledge, this work paves the way for leveraging artificial intelligence to derive insights, improve clinical decision-making, and ultimately enhance patient outcomes ," said study leader Yang Xie, Ph.D., Professor in the Peter O'Donnell Jr. School of Public Health and the Lyda Hill Department of Bioinformatics at UT Southwestern.
Dr. Xie is also Associate Dean of Data Sciences at UT Southwestern Medical School, Director of the Quantitative Biomedical Research Center, and a member of the Harold C. Simmons Comprehensive Cancer Center.
Much of the research in the Xie Lab focuses on developing and using data science and AI tools to improve biomedical research and health care. She and her colleagues wondered whether ChatGPT might speed the process of analyzing clinical notes—the memos physicians write to document patients' visits, diagnoses, and statuses as part of their medical record—to find relevant data for clinical research and other uses.
Clinical notes are a treasure trove of information, Dr. Xie explained; however, because they are written in free text, extracting structured data typically involves having a trained medical professional read and annotate them. This process requires a huge investment of time and often resources—and can also introduce human bias.
Existing programs that use natural language processing require extensive human annotation and model training. As a result, clinical notes are largely underused for research purposes.
To determine whether ChatGPT could convert clinical notes to structured data, Dr. Xie and her colleagues had it analyze more than 700 sets of pathology notes for lung cancer patients to find the major features of primary tumors, whether lymph nodes were involved, and the cancer stage and subtype.
Overall, Dr. Xie said, the average accuracy of ChatGPT to make these determinations was 89%, based on reviews by human readers.
Their analysis took several weeks of full-time work compared with the few days it took to fine-tune data extraction from the ChatGPT model. This accuracy was significantly better than other traditional natural language processing methods tested for this use.
To test whether this approach is applicable to other diseases, Dr. Xie and her colleagues used ChatGPT to extract information about cancer grade and margin status from 191 clinical notes on patients from Children's Health with osteosarcoma, the most common type of bone cancer in children and adolescents. Here, ChatGPT returned information with nearly 99% accuracy on grade and 100% accuracy on margin status.
Dr. Xie noted that the results were strongly influenced by what prompts ChatGPT was given to perform each task—a phenomenon called prompt engineering. Providing multiple options to choose from, giving examples of appropriate responses, and directing ChatGPT to rely on evidence to draw conclusions improved its performance.
She added that using ChatGPT or other large language models to extract structured data from clinical notes could not only speed clinical research but also help clinical trial enrollment by matching patients' information to clinical trial protocols. However, she said, ChatGPT won't replace the need for human physicians.
"Even though this technology is an extremely promising way to save time and effort, we should always use it with caution. Rigorous and continuous evaluation is very important," Dr. Xie said.
Explore further
Feedback to editors

Modular communicative leadless ICD found to be safe and exceeds performance expectations
12 hours ago

Creativity and humor shown to promote well-being in older adults via similar mechanisms

Sweet taste receptor affects how glucose is handled metabolically by humans
13 hours ago

Better medical record-keeping needed to fight antibiotic overuse, studies suggest
19 hours ago

Repeat COVID-19 vaccinations elicit antibodies that neutralize variants, other viruses

A long-term ketogenic diet accumulates aged cells in normal tissues, new study shows
May 17, 2024

Gut bacteria enhance cancer immunotherapy in mouse study

Research finds the protein VISTA directly blocks T cells from functioning in immunotherapy

Study opens the door to designing therapies to improve lung development in growth-restricted fetuses

Researchers make strides in microbiome-based cancer therapies via iron deprivation in the tumor microenvironment
Related stories.

ChatGPT found to display lower concern for child development 'warning signs' than physicians
May 3, 2024

Study: ChatGPT extracts data for ischemic stroke almost perfectly, is useful for thrombectomy data transfer
Apr 19, 2024

Good evidence confuses ChatGPT when used for health information, study finds
Apr 3, 2024

Study shows ChatGPT can produce medical record notes 10 times faster than doctors without compromising quality
Mar 26, 2024

ChatGPT shows poor performance in answering drug-related questions
Dec 12, 2023

Study shows ChatGPT can be helpful for Black women's self-education about HIV, PrEP
Recommended for you.

Artificial intelligence and the future of surgery

Research team develops new AI tool to help classify brain tumors

Global life expectancy projected to increase by nearly 5 years by 2050 despite various threats
May 16, 2024

AI may improve doctor–patient interactions for older adults with cancer
May 15, 2024

New tool can help surgeons quickly search videos and create interactive feedback

Artificial intelligence tool detects sex-related differences in brain structure
May 14, 2024
Let us know if there is a problem with our content
Use this form if you have come across a typo, inaccuracy or would like to send an edit request for the content on this page. For general inquiries, please use our contact form . For general feedback, use the public comments section below (please adhere to guidelines ).
Please select the most appropriate category to facilitate processing of your request
Thank you for taking time to provide your feedback to the editors.
Your feedback is important to us. However, we do not guarantee individual replies due to the high volume of messages.
E-mail the story
Your email address is used only to let the recipient know who sent the email. Neither your address nor the recipient's address will be used for any other purpose. The information you enter will appear in your e-mail message and is not retained by Medical Xpress in any form.
Newsletter sign up
Get weekly and/or daily updates delivered to your inbox. You can unsubscribe at any time and we'll never share your details to third parties.
More information Privacy policy
Donate and enjoy an ad-free experience
We keep our content available to everyone. Consider supporting Science X's mission by getting a premium account.
E-mail newsletter
Artificial intelligence is being used in healthcare for everything from answering patient questions to assisting with surgeries and developing new pharmaceuticals.
According to Statista , the artificial intelligence (AI) healthcare market, which is valued at $11 billion in 2021, is projected to be worth $187 billion in 2030. That massive increase means we will likely continue to see considerable changes in how medical providers, hospitals, pharmaceutical and biotechnology companies, and others in the healthcare industry operate.
Better machine learning (ML) algorithms, more access to data, cheaper hardware, and the availability of 5G have contributed to the increasing application of AI in the healthcare industry, accelerating the pace of change. AI and ML technologies can sift through enormous volumes of health data—from health records and clinical studies to genetic information—and analyze it much faster than humans.
Healthcare organizations are using AI to improve the efficiency of all kinds of processes, from back-office tasks to patient care. The following are some examples of how AI might be used to benefit staff and patients:
- Administrative workflow: Healthcare workers spend a lot of time doing paperwork and other administrative tasks. AI and automation can help perform many of those mundane tasks, freeing up employee time for other activities and giving them more face-to-face time with patients. For example, generative AI can help clinicians with note-taking and content summarization that can help keep medical records as thoroughly as possible. AI might also help with accurate coding and sharing of information between departments and billing.
- Virtual nursing assistants: One study found that 64% of patients are comfortable with the use of AI for around-the-clock access to answers that support nurses provide. AI virtual nurse assistants—which are AI-powered chatbots, apps, or other interfaces—can be used to help answer questions about medications, forward reports to doctors or surgeons and help patients schedule a visit with a physician. These sorts of routine tasks can help take work off the hands of clinical staff, who can then spend more time directly on patient care, where human judgment and interaction matter most.
- Dosage error reduction: AI can be used to help identify errors in how a patient self-administers medication. One example comes from a study in Nature Medicine , which found that up to 70% of patients don’t take insulin as prescribed. An AI-powered tool that sits in the patient’s background (much like a wifi router) might be used to flag errors in how the patient administers an insulin pen or inhaler.
- Less invasive surgeries: AI-enabled robots might be used to work around sensitive organs and tissues to help reduce blood loss, infection risk and post-surgery pain.
- Fraud prevention: Fraud in the healthcare industry is enormous, at $380 billion/year, and raises the cost of consumers’ medical premiums and out-of-pocket expenses. Implementing AI can help recognize unusual or suspicious patterns in insurance claims, such as billing for costly services or procedures that are not performed, unbundling (which is billing for the individual steps of a procedure as though they were separate procedures), and performing unnecessary tests to take advantage of insurance payments.
A recent study found that 83% of patients report poor communication as the worst part of their experience, demonstrating a strong need for clearer communication between patients and providers. AI technologies like natural language processing (NLP), predictive analytics, and speech recognition might help healthcare providers have more effective communication with patients. AI might, for instance, deliver more specific information about a patient’s treatment options, allowing the healthcare provider to have more meaningful conversations with the patient for shared decision-making.
According to Harvard’s School of Public Health , although it’s early days for this use, using AI to make diagnoses may reduce treatment costs by up to 50% and improve health outcomes by 40%.
One use case example is out of the University of Hawaii , where a research team found that deploying deep learning AI technology can improve breast cancer risk prediction. More research is needed, but the lead researcher pointed out that an AI algorithm can be trained on a much larger set of images than a radiologist—as many as a million or more radiology images. Also, that algorithm can be replicated at no cost except for hardware.
An MIT group developed an ML algorithm to determine when a human expert is needed. In some instances, such as identifying cardiomegaly in chest X-rays, they found that a hybrid human-AI model produced the best results.
Another published study found that AI recognized skin cancer better than experienced doctors. US, German and French researchers used deep learning on more than 100,000 images to identify skin cancer. Comparing the results of AI to those of 58 international dermatologists, they found AI did better.
As health and fitness monitors become more popular and more people use apps that track and analyze details about their health. They can share these real-time data sets with their doctors to monitor health issues and provide alerts in case of problems.
AI solutions—such as big data applications, machine learning algorithms and deep learning algorithms—might also be used to help humans analyze large data sets to help clinical and other decision-making. AI might also be used to help detect and track infectious diseases, such as COVID-19, tuberculosis, and malaria.
One benefit the use of AI brings to health systems is making gathering and sharing information easier. AI can help providers keep track of patient data more efficiently.
One example is diabetes. According to the Centers for Disease Control and Prevention , 10% of the US population has diabetes. Patients can now use wearable and other monitoring devices that provide feedback about their glucose levels to themselves and their medical team. AI can help providers gather that information, store, and analyze it, and provide data-driven insights from vast numbers of people. Using this information can help healthcare professionals determine how to better treat and manage diseases.
Organizations are also starting to use AI to help improve drug safety. The company SELTA SQUARE, for example, is innovating the pharmacovigilance (PV) process , a legally mandated discipline for detecting and reporting adverse effects from drugs, then assessing, understanding, and preventing those effects. PV demands significant effort and diligence from pharma producers because it’s performed from the clinical trials phase all the way through the drug’s lifetime availability. Selta Square uses a combination of AI and automation to make the PV process faster and more accurate, which helps make medicines safer for people worldwide.
Sometimes, AI might reduce the need to test potential drug compounds physically, which is an enormous cost-savings. High-fidelity molecular simulations can run on computers without incurring the high costs of traditional discovery methods.
AI also has the potential to help humans predict toxicity, bioactivity, and other characteristics of molecules or create previously unknown drug molecules from scratch.
As AI becomes more important in healthcare delivery and more AI medical applications are developed, ethical, and regulatory governance must be established. Issues that raise concern include the possibility of bias, lack of transparency, privacy concerns regarding data used for training AI models, and safety and liability issues.
“AI governance is necessary, especially for clinical applications of the technology,” said Laura Craft, VP Analyst at Gartner . “However, because new AI techniques are largely new territory for most [health delivery organizations], there is a lack of common rules, processes, and guidelines for eager entrepreneurs to follow as they design their pilots.”
The World Health Organization (WHO) spent 18 months deliberating with leading experts in ethics, digital technology, law, and human rights and various Ministries of Health members to produce a report that is called Ethics & Governance of Artificial Intelligence for Health . This report identifies ethical challenges to using AI in healthcare, identifies risks, and outlines six consensus principles to ensure AI works for the public’s benefit:
- Protecting autonomy
- Promoting human safety and well-being
- Ensuring transparency
- Fostering accountability
- Ensuring equity
- Promoting tools that are responsive and sustainable
The WHO report also provides recommendations that ensure governing AI for healthcare both maximizes the technology’s promise and holds healthcare workers accountable and responsive to the communities and people they work with.
AI provides opportunities to help reduce human error, assist medical professionals and staff, and provide patient services 24/7. As AI tools continue to develop, there is potential to use AI even more in reading medical images, X-rays and scans, diagnosing medical problems and creating treatment plans.
AI applications continue to help streamline various tasks, from answering phones to analyzing population health trends (and likely, applications yet to be considered). For instance, future AI tools may automate or augment more of the work of clinicians and staff members. That will free up humans to spend more time on more effective and compassionate face-to-face professional care.
When patients need help, they don’t want to (or can’t) wait on hold. Healthcare facilities’ resources are finite, so help isn’t always available instantaneously or 24/7—and even slight delays can create frustration and feelings of isolation or cause certain conditions to worsen.
IBM® watsonx Assistant™ AI healthcare chatbots can help providers do two things: keep their time focused where it needs to be and empower patients who call in to get quick answers to simple questions.
IBM watsonx Assistant is built on deep learning, machine learning and natural language processing (NLP) models to understand questions, search for the best answers and complete transactions by using conversational AI.
Get email updates about AI advancements, strategies, how-tos, expert perspective and more.
See IBM watsonx Assistant in action and request a demo
Get our newsletters and topic updates that deliver the latest thought leadership and insights on emerging trends.
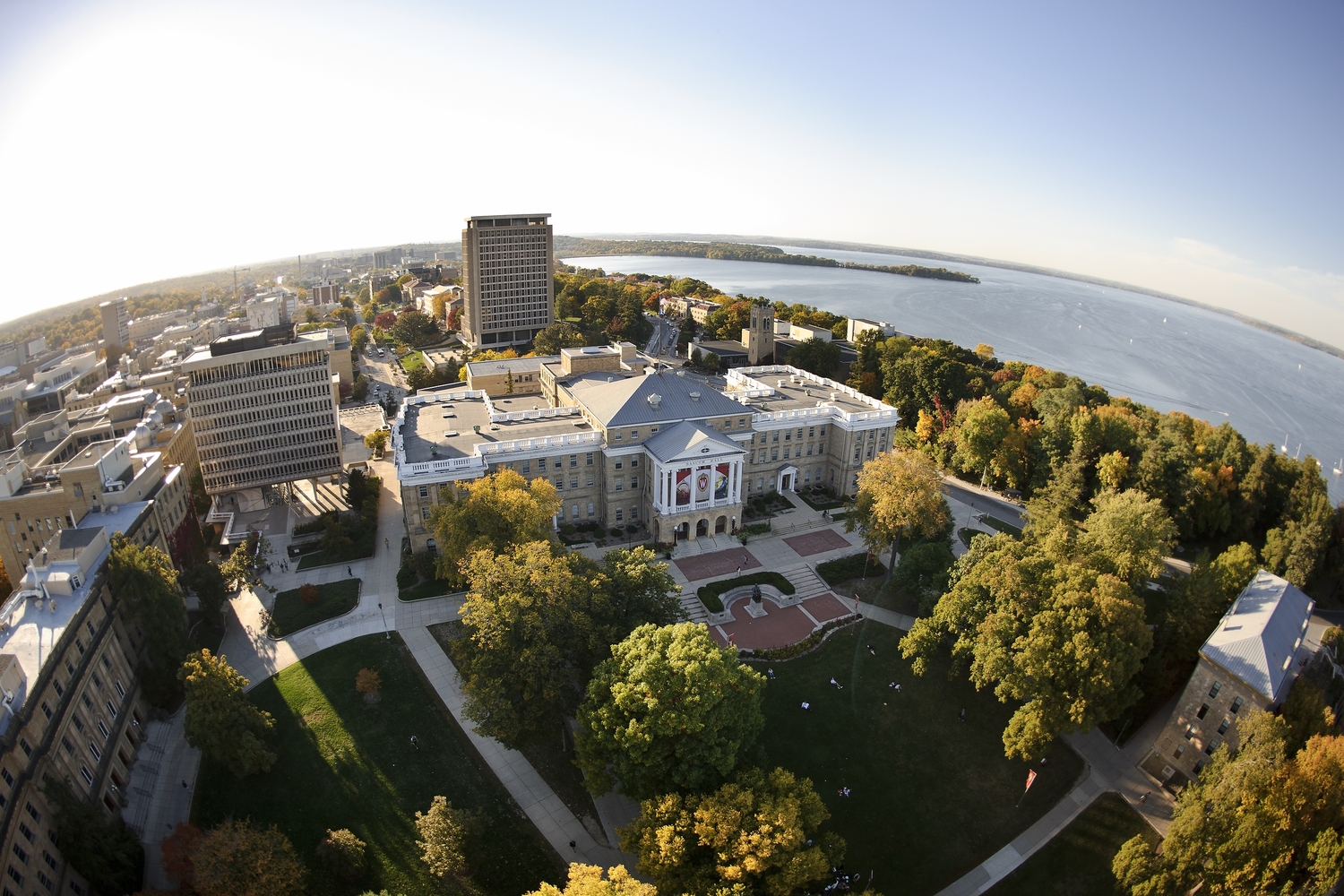
Research Specialist
- Madison, Wisconsin
- SCHOOL OF MEDICINE AND PUBLIC HEALTH/DEPARTMENT OF MEDICINE
- Partially Remote
- Staff-Full Time
- Staff-Part Time
- Opening at: May 15 2024 at 16:50 CDT
- Closing at: May 29 2024 at 23:55 CDT
Job Summary:
This position will support the research program of Dr. Michael Lucey. Dr. Lucey's research focuses on chronic liver disease, particularly alcohol-associated liver disease (ALD). Dr. Lucey leads a multi-disciplinary and cross-departmental group, of whom key members are Dr. John Rice of the Division of Gastroenterology and Hepatology and Dr. Randy Brown of the Department of Family Medicine and Director of Addiction Services of UW Health. Our research encompasses electronic medical record data analysis, qualitative research techniques (focus groups and patient engagement), and clinical/health systems interventions using implementation science. Dr. Lucey is the principal investigator on a prospective 7-year study (starting date August 1, 2023), funded by the NIH, investigating the selection for and outcome of liver transplantation in patients with ALD who have been drinking in the 6 months prior to selection. The successful candidate for this position will assist and support Dr. Lucey and his team in all aspects of their research. Specific duties include (but not limited to): 1. Work with Dr. Lucey and his co-investigators to conduct high quality research; maintaining knowledge of specific study guidelines to assist Dr. Lucey in ensuring compliance with study mandates and federal regulations, managing project data, compliance, and assuring data consistency/security, and maintaining data documentation. 2. Coordinate Drs. Lucey and Rice's research projects within UW and with outside partners. This mandate includes coordinating patient and staff engagement groups and multidisciplinary research team meetings, maintaining working group meeting notes and project website data. 3. Working with the Division of Gastroenterology and Hepatology research office on identifying candidates for subject recruitment, helping with recruitment, maintaining study databases, helping with regulatory aspects and annual reports, 4. Specifically relating to the R01 study: a. maintaining coordination and communication with sub-sites to ensure that we are getting complete data, tracking invoices, and other duties as assigned to support the needs of the project. b. acting as liaison with the data managing site (University of Southern California) to ensure the accurate and timely transmission of data. 5. Assist Dr. Lucey and Dr. Rice with research-related tasks such as preparation of grants, manuscripts, institutional review board (IRB) applications/documentation, data presentations and research reports, and maintaining fiscal and granting agency records. 6. Under the direction of Dr. Lucey and his co-investigators, coordinate local data abstraction from EHR or clinical encounter notes and medical records, maintain project management databases and records, prepare protocols, training manuals, abstraction tools, and supervising other abstractors. 7. Interface with other departments and organizations within the University of Wisconsin School of Medicine & Public Health and the University of Wisconsin Hospitals and Clinics as necessary to identify, collect and disseminate research protocol information and results. 8. Conduct medical and scientific reference bibliographic searches, including abstracting data from published sources, summarizing articles, and maintaining a bibliographic database. Candidates must be well organized and have strong written communication skills, with the ability to present material concisely. Proficiency with Microsoft Word, Excel & PowerPoint is essential. Must be able to work well on a team. A background in research is helpful. Interest in and willingness to learn about alcohol-associated liver disease is a plus.
Responsibilities:
- 20% Conducts research experiments according to established research protocols with moderate impact to the project(s). Collects data and monitors test results
- 5% Operates, cleans, and maintains organization of research equipment and research area. Tracks inventory levels and places replenishment orders
- 20% Reviews, analyzes, and interprets data and/or documents results for presentations and/or reporting to internal and external audiences
- 10% Participates in the development, interpretation, and implementation of research methodology and materials
- 15% Provides operational guidance on day-to-day activities of unit or program staff and/or student workers
- 10% Performs literature reviews and writes reports
- 10% Assists in identification of candidates for subject recruitment and directly supports subject recruitment as needed
- 10% Coordinates patient and staff engagement groups and multidisciplinary research team meetings, maintaining working group meeting notes and project website data
Institutional Statement on Diversity:
Diversity is a source of strength, creativity, and innovation for UW-Madison. We value the contributions of each person and respect the profound ways their identity, culture, background, experience, status, abilities, and opinion enrich the university community. We commit ourselves to the pursuit of excellence in teaching, research, outreach, and diversity as inextricably linked goals. The University of Wisconsin-Madison fulfills its public mission by creating a welcoming and inclusive community for people from every background - people who as students, faculty, and staff serve Wisconsin and the world. For more information on diversity and inclusion on campus, please visit: Diversity and Inclusion
Preferred Bachelor's Degree Biological or social sciences or health-related field preferred
Qualifications:
At least 1 year of experience in clinical, health or social research environment preferred. Applicants with relevant experience in a clinical or community/public health role with direct participant are also encouraged to apply. REDCap experience preferred. Experience in providing project management or staff support to multidisciplinary teams preferred.
Full or Part Time: 50% - 100% This position may require some work to be performed in-person, onsite, at a designated campus work location. Some work may be performed remotely, at an offsite, non-campus work location.
Appointment Type, Duration:
Ongoing/Renewable
Minimum $45,000 ANNUAL (12 months) Depending on Qualifications The expected salary range for this position is $45,000 up to $65,000 for highly experienced candidates. Actual pay will depend on experience and qualifications. Employees in this position can expect to receive benefits such as generous vacation, holidays, and sick leave; competitive insurances and savings accounts; retirement benefits. Benefits information can be found at ( https://hr.wisc.edu/benefits/ ).
Additional Information:
It is anticipated that this will be filled as a 50% FTE position, with higher FTE possible in the future depending upon program needs and funding availability. University sponsorship is not available for this position, including transfers of sponsorship. The selected applicant will be responsible for ensuring their continuous eligibility to work in the United States (i.e. a citizen or national of the United States, a lawful permanent resident, a foreign national authorized to work in the United States without the need of an employer sponsorship) on or before the effective date of appointment. This position is an ongoing position that will require continuous work eligibility. UW-Madison is not an E-Verify employer, and therefore, is not eligible to employ F1-OPT STEM Extension participants. If you are selected for this position you must provide proof of work authorization and eligibility to work. This position has been identified as a position of trust with access to vulnerable populations. The selected candidate will be required to pass an initial caregiver check to be eligible for employment under the Wisconsin Caregiver Law and every four years.
How to Apply:
To apply for this position, please click on the "Apply Now" button. You will be asked to upload a current resume/CV and a cover letter briefly describing your qualifications and experience. You will also be asked to provide contact information for three (3) references, including your current/most recent supervisor during the application process. References will not be contacted without prior notice.
Jacqueline Giese [email protected] 608-263-1326 Relay Access (WTRS): 7-1-1. See RELAY_SERVICE for further information.
Official Title:
Research Specialist(RE047)
Department(s):
A53-MEDICAL SCHOOL/MEDICINE/GASTROENT
Employment Class:
Academic Staff-Renewable
Job Number:
The university of wisconsin-madison is an equal opportunity and affirmative action employer..
You will be redirected to the application to launch your career momentarily. Thank you!
Frequently Asked Questions
Applicant Tutorial
Disability Accommodations
Pay Transparency Policy Statement
Refer a Friend
You've sent this job to a friend!
Website feedback, questions or accessibility issues: [email protected] .
Learn more about accessibility at UW–Madison .
© 2016–2024 Board of Regents of the University of Wisconsin System • Privacy Statement
- Introduction
- Conclusions
- Article Information
Data Sharing Statement
See More About
Sign up for emails based on your interests, select your interests.
Customize your JAMA Network experience by selecting one or more topics from the list below.
- Academic Medicine
- Acid Base, Electrolytes, Fluids
- Allergy and Clinical Immunology
- American Indian or Alaska Natives
- Anesthesiology
- Anticoagulation
- Art and Images in Psychiatry
- Artificial Intelligence
- Assisted Reproduction
- Bleeding and Transfusion
- Caring for the Critically Ill Patient
- Challenges in Clinical Electrocardiography
- Climate and Health
- Climate Change
- Clinical Challenge
- Clinical Decision Support
- Clinical Implications of Basic Neuroscience
- Clinical Pharmacy and Pharmacology
- Complementary and Alternative Medicine
- Consensus Statements
- Coronavirus (COVID-19)
- Critical Care Medicine
- Cultural Competency
- Dental Medicine
- Dermatology
- Diabetes and Endocrinology
- Diagnostic Test Interpretation
- Drug Development
- Electronic Health Records
- Emergency Medicine
- End of Life, Hospice, Palliative Care
- Environmental Health
- Equity, Diversity, and Inclusion
- Facial Plastic Surgery
- Gastroenterology and Hepatology
- Genetics and Genomics
- Genomics and Precision Health
- Global Health
- Guide to Statistics and Methods
- Hair Disorders
- Health Care Delivery Models
- Health Care Economics, Insurance, Payment
- Health Care Quality
- Health Care Reform
- Health Care Safety
- Health Care Workforce
- Health Disparities
- Health Inequities
- Health Policy
- Health Systems Science
- History of Medicine
- Hypertension
- Images in Neurology
- Implementation Science
- Infectious Diseases
- Innovations in Health Care Delivery
- JAMA Infographic
- Law and Medicine
- Leading Change
- Less is More
- LGBTQIA Medicine
- Lifestyle Behaviors
- Medical Coding
- Medical Devices and Equipment
- Medical Education
- Medical Education and Training
- Medical Journals and Publishing
- Mobile Health and Telemedicine
- Narrative Medicine
- Neuroscience and Psychiatry
- Notable Notes
- Nutrition, Obesity, Exercise
- Obstetrics and Gynecology
- Occupational Health
- Ophthalmology
- Orthopedics
- Otolaryngology
- Pain Medicine
- Palliative Care
- Pathology and Laboratory Medicine
- Patient Care
- Patient Information
- Performance Improvement
- Performance Measures
- Perioperative Care and Consultation
- Pharmacoeconomics
- Pharmacoepidemiology
- Pharmacogenetics
- Pharmacy and Clinical Pharmacology
- Physical Medicine and Rehabilitation
- Physical Therapy
- Physician Leadership
- Population Health
- Primary Care
- Professional Well-being
- Professionalism
- Psychiatry and Behavioral Health
- Public Health
- Pulmonary Medicine
- Regulatory Agencies
- Reproductive Health
- Research, Methods, Statistics
- Resuscitation
- Rheumatology
- Risk Management
- Scientific Discovery and the Future of Medicine
- Shared Decision Making and Communication
- Sleep Medicine
- Sports Medicine
- Stem Cell Transplantation
- Substance Use and Addiction Medicine
- Surgical Innovation
- Surgical Pearls
- Teachable Moment
- Technology and Finance
- The Art of JAMA
- The Arts and Medicine
- The Rational Clinical Examination
- Tobacco and e-Cigarettes
- Translational Medicine
- Trauma and Injury
- Treatment Adherence
- Ultrasonography
- Users' Guide to the Medical Literature
- Vaccination
- Venous Thromboembolism
- Veterans Health
- Women's Health
- Workflow and Process
- Wound Care, Infection, Healing
Get the latest research based on your areas of interest.
Others also liked.
- Download PDF
- X Facebook More LinkedIn
Luth EA , Brennan C , Hurley SL, et al. Hospice Readmission, Hospitalization, and Hospital Death Among Patients Discharged Alive from Hospice. JAMA Netw Open. 2024;7(5):e2411520. doi:10.1001/jamanetworkopen.2024.11520
Manage citations:
© 2024
- Permissions
Hospice Readmission, Hospitalization, and Hospital Death Among Patients Discharged Alive from Hospice
- 1 Rutgers University, New Brunswick, New Jersey
- 2 Care Dimensions, Danvers, Massachusetts
- 3 Weill Cornell Medicine, New York, New York
- 4 VNS Health, New York, New York
- 5 Emory University, Gainesville, Georgia
Question What factors are associated with burdensome transitions 2 days after live hospice discharge?
Findings This cohort study of 115 072 Medicare fee-for-service beneficiaries from 2014 to 2019 found that 9% of individuals discharged alive from hospice were hospitalized and readmitted to hospice and 3% were hospitalized and died in the hospital. Identifying as Black, having a short hospice stay, and receiving care from a for-profit hospice were associated with higher odds of burdensome transition.
Meaning These findings suggest that clinical practice and policy should attend to patients at greater risk for burdensome transitions after hospice live discharge, including systematic, incentivized discharge planning tailored to individual patient needs.
Importance Transitions in care settings following live discharge from hospice care are burdensome for patients and families. Factors contributing to risk of burdensome transitions following hospice discharge are understudied.
Objective To identify factors associated with 2 burdensome transitions following hospice live discharge, as defined by the Centers for Medicare & Medicaid Services.
Design, Setting, and Participants This population-based retrospective cohort study included a 20% random sample of Medicare fee-for-service beneficiaries using 2014 to 2019 Medicare claims data. Data were analyzed from April 22, 2023, to March 4, 2024.
Exposure Live hospice discharge.
Main Outcomes and Measures Multivariable logistic regression examined associations among patient, health care provision, and organizational characteristics with 2 burdensome transitions after live hospice discharge (outcomes): type 1, hospice discharge, hospitalization within 2 days, and hospice readmission within 2 days; and type 2, hospice discharge, hospitalization within 2 days, and hospital death.
Results This study included 115 072 Medicare beneficiaries discharged alive from hospice (mean [SD] age, 84.4 [6.6] years; 71892 [62.5%] female; 5462 [4.8%] Hispanic, 9822 [8.5%] non-Hispanic Black, and 96 115 [83.5%] non-Hispanic White). Overall, 10 381 individuals (9.0%) experienced a type 1 burdensome transition and 3144 individuals (2.7%) experienced a type 2 burdensome transition. In adjusted models, factors associated with higher odds of burdensome transitions included identifying as non-Hispanic Black (type 1: adjusted odds ratio [aOR], 1.47; 95% CI, 1.36-1.58; type 2: aOR, 1.70; 95% CI, 1.51-1.90), hospice stays of 7 days or fewer (type 1: aOR, 1.13; 95% CI, 1.06-1.21; type 2: aOR, 1.71; 95% CI, 1.53-1.90), and care from a for-profit hospice (type 1: aOR, 1.78; 95% CI, 1.62-1.96; type 2: aOR, 1.32; 95% CI, 1.15-1.52). Nursing home residence (type 1: aOR, 0.66; 95% CI, 0.61-0.72; type 2: aOR, 0.47; 95% CI, 0.40-0.54) and hospice stays of 180 days or longer (type 1: aOR, 0.63; 95% CI, 0.59-0.68; type 2: aOR, 0.60; 95% CI, 0.52-0.69) were associated with lower odds of burdensome transitions.
Conclusion and Relevance This retrospective cohort study of burdensome transitions following live hospice discharge found that non-Hispanic Black race, short hospice stays, and care from for-profit hospices were associated with higher odds of experiencing a burdensome transition. These findings suggest that changes to clinical practice and policy may reduce the risk of burdensome transitions, such as hospice discharge planning that is incentivized, systematically applied, and tailored to needs of patients at greater risk for burdensome transitions.
Live discharge from hospice—experienced by 15% of Medicare hospice users in 2020 1 —occurs when an individual leaves hospice before death. Reasons for live discharge include unplanned hospitalization, seeking curative treatment for a terminal condition, transferring hospice services, or condition stabilization that makes someone ineligible for hospice. Live discharge has policy, patient, and caregiver consequences. 2 - 4 It is typically disruptive, resulting in the loss of clinical and support services during the critical end-of-life period. 2 - 4 Nearly half of hospice patients (42%) die within 6 months of live discharge, 5 suggesting that uninterrupted hospice care may be appropriate for many individuals who were discharged alive.
The Centers for Medicare & Medicaid Services (CMS) are concerned about the number of hospice live discharges and potentially negative consequences for patient quality of life and death. In 2021, CMS added 4 measures related to hospice live discharge to their 10-item Hospice Care Index for hospice care quality. 6 These 4 measures include early (ie, ≤7 days of hospice enrollment) and late (ie, >180 days of hospice enrollment) live discharges and 2 types of posthospice burdensome discharge transition experiences. 6 Type 1 burdensome transitions focus on individuals who are admitted to a hospital within 2 days following hospice live discharge, and then readmitted to hospice within 2 days of hospital discharge. 6 Type 2 burdensome transitions identifies individuals who are hospitalized within 2 days after hospice live discharge and die while hospitalized. 6 Early and late live discharges are associated with racial and ethnic minoritized status, 7 - 11 younger age, 7 , 8 , 12 , 13 dual Medicare and Medicaid enrollment, 7 - 9 fewer comorbidities, 7 , 14 increased functional status, 7 , 15 and for-profit hospice status. 10 , 16 However, type 1 and 2 burdensome transitions have not been as well studied (a 2016 study by Prsic et al 16 is an exception), despite being potentially related to poor assessment of patient stability prior to live discharge 6 or nonsystematic approaches to live discharge planning 17 , 18 that may result in postdischarge care fragmentation. 19
In this study, we address the question of what individual patient, health care provision, and hospice organizational factors are associated with the 2 types of burdensome transitions following live discharge from hospice, as defined by CMS. Identifying factors associated with burdensome transitions is a necessary first step in identifying immediate and longer-term targets for intervention to improve end-of-life outcomes. Drawing from prior literature and a modified Holzemer framework, 20 we selected factors associated with increased risk of live discharge (eg, racial and ethnic minoritized status, dual enrollment, for-profit status) and factors believed to be associated with protection against live discharge (eg, older age, fewer comorbidities, less frailty, palliative care consultation). Patient sociodemographic and health characteristics could help identify which patients may require additional attention during live discharge if they have increased risk of postdischarge burdensome transitions. Aspects of hospice care are potentially modifiable in current clinical practice and future policies. Health care provision also includes ongoing processes, such as goals of care planning, that may impact postdischarge care trajectories. Organizational factors related to the hospice care setting could help identify potential targets for regulatory oversight.
This cohort study was approved by the Institutional Review Board at Weill Cornell Medicine with a waiver of consent because this study uses deidentified secondary claims data collected by CMS. We followed the Strengthening the Reporting of Observational Studies in Epidemiology ( STROBE ) reporting guideline.
We conducted a retrospective cohort study using a 20% random sample of 2014 to 2019 Medicare fee-for-service (FFS) beneficiaries. Medicare is the federally funded health insurance program in the US for individuals aged 65 years and older and for eligible individuals with end-stage kidney disease and disabilities. 21 - 23 We used Medicare hospice claims files to identify hospice live discharges using discharge status codes. 1 , 24 To exclude most hospice stays that might be readmissions following a hospice discharge in 2013, we implemented a washout period of the first 90 days of 2014 to only include patients who newly started their hospice benefits in the study period. 25 The analysis included 115 072 patients who were aged 65 years or older when admitted to hospice, continuously enrolled in Medicare Parts A and B for 12 months before hospice admission, and continuously enrolled in Medicare Parts A and B after hospice discharge until death or the end of the study period (December 31, 2019). In rare cases, if a patient had more than 1 hospice live discharge, we analyzed the first one.
We used Master Beneficiary Summary Files to extract patient demographics and enrollment information. We used hospice claims to identify service location, hospice services provided, principal diagnosis, and other hospice stay characteristics. We used Medicare Parts A (eg, inpatient hospital, skilled nursing facility, and home health 21 ) and B (eg, physician and outpatient services 21 ) files to identify claims related to hospital stays, patient health status, services prior to hospice admission, and burdensome transitions after hospice discharge. We extracted hospice ownership from CMS Provider of Services files 26 and hospice size data from CMS Medicare Post-Acute Care & Hospice 27 utilization and payment data. There were no missing data on any variables used for analysis.
We calculated 2 burdensome transition measures, as defined by CMS as part of the Hospice Care Index. Type 1 was defined as hospitalization within 2 days after hospice live discharge, followed by hospice readmission within 2 days of hospital discharge. Type 2 was defined as hospitalization within 2 days after hospice live discharge with in-hospital death. 24
We examined individual patient (sociodemographic and health), health care provision, and organizational characteristics that might be associated with burdensome transitions after hospice live discharge. Patient sociodemographic characteristics included age at hospice admission (65-74, 75-84, ≥85 years), sex (male, female), race and ethnicity as recorded in the Master Beneficiary Summary Files 28 (categorized as Hispanic, non-Hispanic Black, non-Hispanic White, and other [including American Indian or Alaska Native, Asian or Pacific Islander, or unknown]), dual enrollment status (Medicare-Medicaid, Medicare only), residence before hospice admission (urban/suburban, rural by zip code), 29 and long-term nursing home residence prior to hospice admission. 30 We used a categorical claims-based frailty index (not frail, prefrail, mildly frail, moderately to severely frail), 31 - 33 CMS Hierarchical Condition Category (HCC) score as a continuous variable, and principal diagnosis, including Alzheimer Disease and related dementias, the 5 most common cancers among Medicare beneficiaries (defined by the Chronic Conditions Data Warehouse: breast, colorectal, endometrial, lung, or prostate), cardiovascular disease (eg, heart failure, acute myocardial infarction, and ischemic heart disease), chronic kidney disease, and COPD (as these are the most common diagnoses among hospice patients), 34 or other to measure patient health status.
We examined several health care characteristics. The Medicare hospice benefit covers 4 levels of hospice care depending on patient and caregiver needs. 35 Routine home care, accounting for 99% of hospice days, 1 provides comfort and symptom management. It is delivered in the community or patient residence, assisted living, nursing home, inpatient hospital, hospice residence, or other settings. We measured location of hospice care as the location where most routine home care was delivered. Patients and caregivers can receive care in addition to routine home care, which may signal severe patient conditions. Therefore, we identified the use of continuous home care (management of acute medical symptoms, such as uncontrolled pain), inpatient respite care (short-term relief for family caregivers), and general inpatient care (GIP; short-term hospital care for symptom management) separately as dichotomous variables (yes or no). We also measured length of stay (short, ≤7 days; expected, 8-179 days; long, ≥180 days), and live discharge reason. Live discharge reasons included discharge home with cause, patient revocation of hospice benefits (eg, seeking curative treatment for a terminal condition), condition stabilization, patient unavailability, unplanned hospitalization, or transfer to another hospice. Goals of care planning were assessed using proxy measures, including advance care planning before hospice discharge (starting in 2016) (Current Procedural Terminology codes 99497 and 99498) and any palliative care encounter 6 months before hospice admission ( International Statistical Classification of Diseases and Related Health Problems, Tenth Revision [ ICD-10 ] code Z51.1).
For organizational setting characteristics, we examined hospice ownership (nonprofit, for-profit, government, other) and size (quintiles of number of total stays in each calendar year). We included hospice admission and discharge years as controls.
We compared individual, health care provision, and organizational factors by burdensome transition outcome status using χ 2 tests for categorical variables and t tests for continuous variables. For each burdensome transition outcome, we used multivariable logistic regression to examine factors associated with the likelihood of the outcome vs no burdensome transition. Individual, care, and organizational factors and admission year and discharge year fixed effects were included in the model. We used robust standard errors clustered by hospice organization to account for correlations of patients discharged from the same hospice.
In secondary analysis, we mapped patient residential zip codes into hospital referral regions (HRRs) and included them as fixed effects to account for regional variations in end-of-life health care preferences and hospice market characteristics. To facilitate comparisons across different categories of key factors associated with burdensome transitions (race and ethnicity, frailty, location and type of hospice care, hospice ownership), we calculated estimated probabilities, holding all other variables constant at their means.
P values were 2-sided, and statistical significance was set at P < .05. Analyses were conducted using Stata version 17 (StataCorp) from April 22, 2023, to March 4, 2024.
This study included 115 072 Medicare FFS beneficiaries discharged alive from hospice (mean [SD] age, 84.4 [6.6] years; 71 892 [62.5%] female; 5462 Hispanic individuals [4.8%], 9822 non-Hispanic Black individuals [8.5%], and 96 115 non-Hispanic White individuals [83.5%]). Burdensome transitions accounted for 11.7% of live discharges: 10 381 individuals (9.0%) experienced a type 1 transition (live discharge, hospitalization within 2 days, hospice readmission within 2 days) and 3144 individuals (2.7%) experienced a type 2 transition (live discharge, hospitalization within 2 days, hospital death). Individuals who experienced type 1 and 2 transitions differed from the general live discharge population for all sociodemographic, health, and hospice characteristics ( Table 1 ).
Factors associated with lower odds of experiencing a live discharge followed by hospitalization and subsequent hospice readmission included female sex (adjusted odds ratio [aOR], 0.95; 95% CI, 0.91-0.99; P = .01) dual Medicare and Medicaid enrollment (aOR, 0.91; 95% CI, 0.86-0.96; P = .001), nursing home residence (aOR, 0.66; 95% CI, 0.61-0.72; P < .001), hospice stay of 180 days or longer (aOR, 0.63; 95% CI, 0.59-0.68, P < .001), and receiving inpatient respite (aOR, 0.78; 95% CI, 0.70-0.87; P < .001) or GIP (aOR, 0.85; 95% CI, 0.75-0.97; P = .01) care. Factors associated with greater odds of experiencing a type 1 burdensome transition included identifying as non-Hispanic Black (aOR, 1.47; 95% CI, 1.36-1.58; P < .001), having any degree of frailty (eg, prefrail: aOR, 1.37; 95% CI, 1.24-1.52; P < .001), a cancer (aOR, 1.18; 95% CI, 1.10-1.27; P < .001) or COPD (aOR, 1.18; 95% CI, 1.10-1.26; P < .001) diagnosis, higher HCC score (aOR, 1.07; 95% CI, 1.05-1.08; P < .001), short hospice stay (aOR, 1.13; 95% CI, 1.06-1.21; P < .001), palliative care consultation (aOR, 1.08; 95% CI, 1.01-1.14; P = .02), and receiving care from a larger (eg, quintile 5: aOR, 1.36; 95% CI, 1.21-1.52; P < .001) or for-profit (aOR, 1.78; 95% CI, 1.62-1.96; P < .001) hospice ( Table 2 ).
Factors associated with lower odds of experiencing a type 2 burdensome transition included older age (eg, ≥85 years: aOR, 0.83; 95% CI, 0.75-0.92; P < .001), female sex (aOR, 0.86; 95% CI, 0.80-0.93; P < .001), long hospice stay (aOR, 0.60; 95% CI, 0.52-0.69; P < .001), nursing home residence (aOR, 0.47; 95% CI, 0.40-0.54; P < .001), and receiving hospice in assisted living (aOR, 0.67; 95% CI, 0.59-0.77; P < .001) or a hospice residence (aOR, 0.63; 95% CI, 0.45-0.88; P < .007). Factors associated with greater odds of experiencing a type 2 burdensome transition included identifying as any race or ethnicity but non-Hispanic White (Hispanic: aOR, 1.23; 95% CI, 1.05-1.45; P = .01; non-Hispanic Black: aOR, 1.70; 95% CI, 1.51-1.90; P < .001), a cancer (aOR, 1.45; 95% CI, 1.29-1.62; P < .001) or COPD (aOR, 1.30; 95% CI, 1.13-1.49; P < .001) diagnosis, higher HCC score (aOR, 1.09; 95% CI, 1.07-1.12; P < .001), short hospice stay (aOR, 1.71; 95% CI, 1.53-1.90; P < .001), palliative care consultation (aOR, 1.27; 95% CI, 1.15-1.39; P < .001), receiving care from a government hospice (aOR, 1.38; 95% CI, 1.01-1.87; P = .04), and receiving care in a nursing home (aOR, 1.16; 95% CI, 1.02-1.33; P = .03) or inpatient hospital (aOR, 1.49; 95% CI, 1.12-1.98; P = .007) ( Table 3 ).
Black individuals had a 2.8–percentage point higher probability of experiencing a type 1 transition and a 1.2–percentage-point higher probability of experiencing a type 2 transition, compared with non-Hispanic White individuals ( Table 4 ). Compared with care at nonprofit hospices, care at a for-profit hospice was associated with 3.5–percentage point higher probability of experiencing a type 1 transition and a 0.5–percentage-point higher probability of experiencing a type 2 transition. Palliative care consultations were associated with a 0.5–percentage point higher probability of type 2 transition. For experiencing type 1 transitions, any type of frailty was associated with 1.6– to 3.3–percentage point higher probability than nonfrailty. Receiving respite was associated with a 0.6–percentage point higher probability of experiencing a type 1 transition, and receiving GIP was associated with 0.9–percentage point lower probability of a type 1 transition ( Table 4 ).
In this cohort study of Medicare FFS beneficiaries who were discharged alive from hospice, we examined factors associated with burdensome transitions 2 days after live hospice discharge. Live discharge from hospice is burdensome for individuals who are seriously ill and their families. 2 , 36 Among the 15% of hospice patients discharged alive, 1 in 7 are either hospitalized within 2 days after discharge and readmitted to hospice or are hospitalized within 2 days after discharge and die while hospitalized. These transitions may signal problems with assessments of patient stability prior to discharge, 6 lack of systematic approaches to live discharge planning, or disincentives for hospices to provide certain types of costly care, such as GIP.
Some patient sociodemographic characteristics were associated with protection against experiencing a burdensome transition after live discharge. Older age and female sex were associated with lower odds of experiencing either burdensome transition, while dual Medicare and Medicaid enrollment was associated with protection against type 1 transitions only. Medicaid-enrolled individuals may be eligible for additional home- and community-based services, such as the 1915(c) waiver, 37 that were associated with protection against hospitalization and hospice readmission after hospice discharge. Residing in a nursing home prior to hospice admission was also associated with protection against burdensome transitions. Nursing homes are more familiar with end-of-life trajectories and may make more appropriate referrals to hospice. Patients remaining in the same care setting with staff familiar with their evolving care needs may benefit from this continuity and face fewer burdensome transitions after hospice discharge.
In contrast, identifying as Black was associated with higher odds of either type of burdensome transition; identifying as Hispanic was associated with higher odds of type 2 burdensome transition. Our findings identify another layer of hospice-related disparity and risk for individuals from racially and ethnically minoritized groups: Black and Hispanic individuals access hospice at lower rates than non-Hispanic White individuals, 34 experience live discharge at higher rates, 9 , 10 and are also at increased risk of burdensome transitions after live discharge. Consistent with lower rates of hospice enrollment, Hispanic individuals may be less likely to reenroll in hospice, including if they are hospitalized, which may explain the lack of association between Hispanic identity and type 1 burdensome transitions. Structural factors, such as inequitable distribution of and access to health care resources and institutionalized racism, are important contributing factors in observed racial and ethnic disparities in health outcomes. 38 , 39 In addition to addressing structural inequities, careful attention to the needs of individuals at increased risk for burdensome postdischarge transitions may help prevent them from occurring. 18
Factors related to health care provision were associated with burdensome transitions after live discharge. These are potentially modifiable, making them promising intervention targets. Longer hospice stays were associated with lower odds of burdensome transitions. Although discouraged by regulations, 40 , 41 longer stays may allow the hospice team to stabilize individuals who are seriously ill and establish care plans, which may be beneficial after hospice services cease. Inpatient respite and GIP were associated with lower odds of hospitalization and hospice readmission but not hospitalization and hospital death. These types of hospice care represent only 6.2% of hospice spending 34 due to restrictive eligibility criteria and limited availability. Our findings suggest they may be effective in supporting patients with complicated needs requiring temporary hospitalization. Increasing availability of inpatient respite and GIP within the hospice benefit may reduce burdensome transitions after live discharge. The lack of association between type of hospice care and type 2 transitions may relate to insufficient power to detect associations, as type 2 transitions, inpatient respite, and GIP occurred infrequently in our sample. Individuals receiving hospice in assisted living or a hospice residence had lower odds of hospitalization and hospital death but not hospitalization and hospice readmission. There may be support structures and professional medical care in these settings that prevent individuals from being hospitalized and dying in hospital after live discharge. Shorter hospice stays were associated with higher odds of burdensome transitions. Shorter stays likely reflect late referrals and do not allow the hospice team to put an effective care plan in place, potentially leading to additional transitions if live discharge occurs.
Although we could not assess the ongoing nature of goals-of-care planning, having a palliative care consultation in the months leading up to hospice admission was associated with higher odds of burdensome transitions. We would expect that palliative care would facilitate a timely transition into hospice 42 and be associated with lower likelihood of hospital death. 43 However, we found that palliative care encounters were associated with higher odds of burdensome transitions after live discharge. Possibly, palliative care consultations are sought for complex patients for whom hospice provides stability, but complications reoccur following live discharge, increasing risk for burdensome transition.
At the organizational level, individuals who received care from for-profit hospices had higher odds of a burdensome transition, possibly signaling a reverberating impact of poorer quality care documented in for-profit hospice agencies. 16 , 25 , 44 Financial incentives to discharge patients alive to reduce costs 25 may also contribute to postdischarge burdensome transitions. Hospices may discharge patients who require hospitalization and readmit them on hospital discharge to avoid paying for costly hospice care, such as GIP. Individuals receiving care at large hospices had higher odds of experiencing a hospitalization and hospice readmission. Larger hospice agencies may enroll patients with more complicated needs who require hospitalization for complex symptom management.
Our study has limitations. First, our results are only applicable to individuals receiving FFS Medicare and may not be generalizable to Medicare Advantage enrollees. However, Medicare Advantage did not have a hospice benefit during the study period, and prior studies have found lower rates of live discharge in Medicare Advantage populations compared with Medicare FFS. 45 Second, type 2 burdensome transitions (hospitalization followed by hospital death) were relatively uncommon: only 3000 individuals experienced this type of transition in a 6-year period, and so differences may not be detected for this group. Although hospitalization during a longer period after live discharge may be more common, we aligned our analysis with the CMS definition, given the policy relevance. Moreover, hospital admission within 2 days of live discharge is highly disruptive for patients and families and therefore important to consider. Third, we are unable to capture process-related measures, key in understanding and addressing adverse health outcomes. We used proxy measures to represent these processes (eg, advance care planning, palliative care consultations). Fourth, other factors not captured in claims data, such as family burden and resources and availability of paid and unpaid caregivers, may be protective against burdensome transitions. We have attempted to address potential bias by examining a comprehensive set of factors that may explain burdensome transitions.
This cohort study found that burdensome transitions following live discharge from hospice were associated with patient, health care provision, and organizational setting characteristics that require responses in clinical practice, policy, and research. In clinical practice, increased attention to the needs of persons from racially and ethnically minoritized groups and with more complicated care needs and frailty is required, as these patients may be susceptible to postdischarge burdensome transitions. Introduction of systemic discharge planning—in clinical practice and supported by policy—may alleviate burdensome transitions in individuals discharged alive from large, for-profit hospice agencies and receiving hospice in nursing homes. Policy facilitating patient access to and hospice-hospital partnership for GIP and inpatient respite services and adjusting hospice payment structures to disincentivize discharge among individuals needing this type of intensive care may reduce postdischarge hospitalization and readmission. Additional research is needed to understand the association between palliative care consultations and burdensome transitions after live hospice discharge, whether these consultations are a marker for patients with particularly complex needs that continue until death, and whether they may have an unintended negative long-term effect on individuals discharged alive from hospice.
Accepted for Publication: March 14, 2024.
Published: May 16, 2024. doi:10.1001/jamanetworkopen.2024.11520
Open Access: This is an open access article distributed under the terms of the CC-BY License . © 2024 Luth EA et al. JAMA Network Open .
Corresponding Author: Elizabeth A. Luth, PhD, Rutgers University, 112 Patterson Ave, New Brunswick, NJ 08901 ( [email protected] ).
Author Contributions: Dr Zhang had full access to all of the data in the study and takes responsibility for the integrity of the data and the accuracy of the data analysis.
Concept and design: Luth, Brennan, Prigerson, Zhang.
Acquisition, analysis, or interpretation of data: Luth, Hurley, Phongtankuel, Ryvicker, Shao, Zhang.
Drafting of the manuscript: Luth, Brennan, Zhang.
Critical review of the manuscript for important intellectual content: Brennan, Hurley, Phongtankuel, Prigerson, Ryvicker, Shao, Zhang.
Statistical analysis: Luth, Zhang.
Obtained funding: Zhang.
Administrative, technical, or material support: Luth, Shao, Zhang.
Supervision: Luth, Phongtankuel, Prigerson, Shao, Zhang.
Conflict of Interest Disclosures: Dr Zhang reported receiving grants from The Physicians Foundation during the conduct of the study and grants from The Novartis Foundation, Centers for Disease Control and Prevention, and US Department of Health & Human Services and personal fees from McDermott Will & Emery outside the submitted work. No other disclosures were reported.
Funding/Support: This study was supported by funding from the National Institutes on Aging (grant No. AG065624 [Dr Luth], AG064030 [Dr Zhang], and AG059997 [Dr Phongtankuel]) and the National Cancer Institute ( grant No. CA197730 [Dr Prigerson]).
Role of the Funder/Sponsor: The funders had no role in the design and conduct of the study; collection, management, analysis, and interpretation of the data; preparation, review, or approval of the manuscript; and decision to submit the manuscript for publication.
Data Sharing Statement: See the Supplement .
Additional Contributions: Manyao Zhang, MS (Department of Population Health Sciences, Weill Cornell Medicine) assisted in analyzing Medicare claims data. Ms Zhang was not compensated for this work.
- Register for email alerts with links to free full-text articles
- Access PDFs of free articles
- Manage your interests
- Save searches and receive search alerts
Patients love telehealth—physicians are not so sure
IRL or URL? Many physicians and patients used to see medical care as something best done in-person (in real life, or IRL). But the pandemic has spurred a massive transition to virtual (or URL) care. According to our recent surveys of consumers and physicians, opinions are split on what happens next (see sidebar, “Our methodology”). As the pandemic evolves, consumers still prefer the convenience of digital engagement and virtual-care options, according to our recent McKinsey Consumer Health Insights Survey. This preference could help more patients access care, while also helping providers to grow.
Our methodology
To help our clients understand responses to COVID-19, McKinsey launched a research effort to gather insights from physicians into how the pandemic is affecting their ability to provide care, their financial situation, and their level of stress, as well as what kind of support would interest them. Nationwide surveys were conducted online in 2020 from April 27–May 5 (538 respondents), July 22–27 (150 respondents), and September 22–27 (303 respondents), as well as from March 25–April 5, 2021 (379 respondents).
The participants were US physicians in a variety of practice types and sizes, and a range of employment types. The specialties included general practice and family practice; cardiology; orthopedics, sports medicine and musculoskeletal; dermatology; general surgery; obstetrics and gynecology; oncology; ophthalmology; otorhinolaryngology and ENT; pediatrics; plastic surgery; physical medicine and rehabilitation; psychiatry and behavioral health; emergency medicine; and urology. These surveys built on a prior one of 1,008 primary-care, cardiology, and orthopedic-surgery physicians in April 2019.
To provide timely insights on the reported behaviors, concerns, and desired support of adult consumers (18 years and older) in response to COVID-19, McKinsey launched consumer surveys in 2020 (March 16–17, March 27–29, April 11–13, April 25–27, May 15–18, June 4–8, July 11–14, September 5–7, October 22–26, and November 20–December 6) and 2021 (January 4–11, February 8–12, March 15–22, April 24–May 2, June 4–13, and August 13–23). These surveys represent the stated perspectives of consumers and are not meant to indicate or predict their actual future behavior. (In these surveys, we asked consumers about “Coronavirus/COVID-19,” given the general public’s colloquial use of coronavirus to refer to COVID-19.)
Many digital start-ups and tech and retail giants are rising to the occasion, but our most recent (2021) McKinsey Physician Survey indicates that physicians may prefer a return to pre-COVID-19 norms. In this article, we explore the trends creating disconnects between consumers and physicians and share ideas on how providers could offer digital services that work not only for them but also for patients. Bottom line: a seamless IRL/URL offering could retain patients while delivering high-quality care. Everybody benefits.
The rise of telehealth
These materials reflect general insight based on currently available information, which has not been independently verified and is inherently uncertain. Future results may differ materially from any statements of expectation, forecasts, or projections. These materials are not a guarantee of results and cannot be relied upon. These materials do not constitute legal, medical, policy, or other regulated advice and do not contain all the information needed to determine a future course of action.
At the onset of the COVID-19 pandemic, both physicians and patients embraced telehealth: in April 2020, the number of virtual visits was a stunning 78 times higher than it had been two months earlier, accounting for nearly one-third of outpatient visits. In May 2021, 88 percent of consumers said that they had used telehealth services at some point since the COVID-19 pandemic began. Physicians also felt dramatically more comfortable with virtual care. Eighty-three percent of those surveyed in the 2021 McKinsey Physician Survey offered virtual services, compared with only 13 percent in 2019. 1 See sidebar on methodology; McKinsey Physician Surveys conducted nationally in five waves between May 2019 and April 2021; May 1, 2019, n = 1,008; May 5, 2020, n = 500; July 2, 2020, n = 150; September 27, 2020, n = 500; April 5, 2021, n = 379.
However, as of mid-2021, consumers’ embrace of telehealth appeared to have dimmed a bit from its early COVID-19 peak: utilization was down to 38 times pre-COVID-19 levels. Also, more physicians were offering telehealth but recommending in-person care when possible in 2021, which could suggest that physicians are gravitating away from URL and would prefer a return to IRL care delivery (Exhibit 1).
Three trends from the late-stage pandemic
As COVID-19 continues, three emerging trends could set the stage for the next few years.
The number of virtual-first players keeps growing, and physicians struggle to keep up
The growth (and valuations) of virtual-first care providers suggest that demand by patients is persistent and growing. Teladoc increased the number of its visits by 156 percent in 2020, and its revenues jumped by 107 percent year over year. Amwell increased its supply of providers by 950 percent in 2020. 2 “Teladoc Health reports fourth-quarter and full-year 2020 results,” Teledoc Health, February 24, 2021; “Amwell announces results for the fourth quarter and full year 2020,” Amwell, March 24, 2021. By contrast, only 45 percent of physicians have been able to invest in telehealth during the pandemic, and only 16 percent have invested in other digital tools. Just 41 percent believe that they have the technology to deliver telehealth seamlessly. 3 McKinsey Physician Survey, April 5, 2021.
Some workflows, for example, require physicians to log into disparate systems that do not integrate seamlessly with an electronic health record (EHR). Audiovisual failures during virtual appointments continue to occur. To make these models work, providers may need to determine how to design operational workflows to make IRL/URL care as seamless as possible for both providers and patients. The workflows and care team models may need to vary, depending on the physician’s specialty and the amount of time they plan to devote to URL versus IRL care.
Patient–physician relationships are shifting
In McKinsey’s April 2021 Physician Survey, 58 percent of the respondents reported that they had lost patients to other physicians or to other health systems since the start of the COVID-19 pandemic. Corroborating those findings, our August 2021 survey of consumers showed that of those who had a primary-care physician (PCP), 15 percent had switched in the past year. Thirty-five percent of all consumers reported seeing a new healthcare provider who was not their regular PCP or specialist in the past year. Among consumers who had switched PCPs, 35 percent cited one or more reasons related to the patient experience—the desire for a PCP who better understood their needs (15 percent of respondents), a better experience (10 percent), or more convenient appointments (6 percent). Just half (50 percent) of consumers with a PCP say they are very satisfied. What’s more, Medicare regulations now give patients more ownership over their health data, and that could make it easier for them to switch physicians. 4 “Policies and technology for interoperability and burden reduction,” Centers for Medicare & Medicaid Services, December 9, 2021.
Physicians and patients see telehealth differently
Our surveys show that doctors and patients have starkly different opinions about telehealth and broader digital engagement (Exhibit 2). Take convenience: while two-thirds of physicians and 60 percent of patients said they agreed that virtual health is more convenient than in-person care for patients, only 36 percent of physicians find it more convenient for themselves.
This perception may be leading physicians to rethink telehealth. Most said they expect to return to a primarily in-person delivery model over the next year. Sixty-two percent said they recommend in-person over virtual care to patients. Physicians also expect telehealth to account for one-third less of their visits a year from now than it does today.
These physicians may be underestimating patient demand. Forty percent of patients in May 2021 said they believe they will continue to use telehealth in the pandemic’s aftermath. 5 McKinsey Consumer Health Insights Survey , May 7, 2021.
In November 2021, 55 percent of patients said they were more satisfied with telehealth/virtual care visits than with in-person appointments. 6 McKinsey Consumer Health Insights Survey , November 19, 2021. Thirty-five percent of consumers are currently using other digital services, such as ordering prescriptions online and home delivery. Of these, 42 percent started using these services during the pandemic and plan to keep using them, and an additional 15 percent are interested in starting digital services. 7 McKinsey Consumer Health Insights Survey , June 24, 2021.
Convenience is not the only concern. Physicians also worry about reimbursement. At the height of the COVID-19 pandemic in the United States, the Centers for Medicare & Medicaid Services (CMS) and several other payers switched to at-parity (equal) reimbursement for virtual and in-person visits. More than half of physician respondents said that if virtual rates were 15 percent lower than in-person rates, they would be less likely to offer telehealth. Telehealth takes investment: traditional providers may need time to transition their capital and operating expenses to deliver virtual care at a cost lower than that of IRL.
Four critical actions for providers to consider
Providers may want to define their IRL/URL care strategy to identify the appropriate places for various types of care—balancing clinical appropriateness with the preferences of physicians and patients.
Determine the most clinically appropriate setting
Clinical appropriateness may be the most crucial variable for deciding how and where to increase the utilization of telehealth. Almost half of physicians said they regard telehealth as appropriate for treatment of ongoing chronic conditions, and 38 percent said they believe it is appropriate when patients have an acute change in health—increases of 26 and 17 percentage points, respectively, since May 2019.
However, physicians remain conservative in their view of telehealth’s effectiveness compared with in-person care. Their opinions vary by visit type (Exhibit 3). Health systems may consider asking their frontline clinical-care delivery teams to determine the clinically appropriate setting for each type of care, taking into account whether physicians are confident that they can deliver equally high-quality care for both IRL and URL appointments.
Assess patient wants and needs in relevant markets and segments
Patient demand for telehealth remains high, but expectations appear to vary by age and income group, payer status, and type of care. Our survey shows that younger people (under the age of 55 ), people in higher income brackets (annual household income of $100,000 or more), and people with individual or employer-sponsored group insurance are more likely to use telehealth (Exhibit 4). Patient demand also is higher for virtual mental and behavioral health. Sixty-two percent of mental-health patients completed their most recent appointments virtually, but only 20 percent of patients logged in to see their primary-care provider, gynecologist, or pediatrician.
To meet market demand effectively, it may be crucial to base care delivery models on a deep understanding of the market, with a range of both IRL and URL options to meet the needs of multiple patient segments.
Partner with physicians to define a new operating model
Many physicians are turning away from the virtual operating model: 62 percent recommended in-person care in April 2021, up five percentage points since September 2020. As physicians evaluate their processes for 2022, 46 percent said they prefer to offer, at most, a couple of hours of virtual care each day. Twenty-nine percent would like to offer none at all—up ten percentage points from September 2020. Just 11 percent would dedicate one full day a week to telehealth, and almost none would want to offer virtual care full time (Exhibit 5).
To adapt to these views, care providers can try to meet the needs and the expectations of physicians. They could offer highly virtualized schedules to physicians who prefer telehealth, while allowing other physicians to remain in-person only. Matching the preferences of physicians may create the best experience both for them and for patients. Greater flexibility and greater control over decisions about when and how much virtual care to offer may also help address chronic physician burnout issues (Exhibit 6). Digital-first solutions (for example, online scheduling, digital registration, and virtual communications with providers) could also increase the reach of in-person-only care providers to the 60 percent of consumers interested in using these digital solutions after the pandemic abates.
Communicate clearly to patients and others
Physicians consistently emerge as the most trusted source of clinical information by patients: 90 percent consider providers trustworthy for healthcare-related issues. 8 McKinsey Consumer Survey, May 2020. Providers could play a pivotal role in counseling patients on the importance of continuity of care, as well as what can be done safely and effectively by IRL and URL, respectively. The goal is to help patients receive the care that they need in a timely manner and in the most clinically appropriate setting.
Potential benefits to providers
The strategic, purposeful design of a hybrid IRL/URL healthcare delivery model that respects the preferences of patients and physicians and offers virtual care when it is appropriate clinically may allow healthcare providers to participate in the near term, retain clinical talent, offer better value-based care, and differentiate themselves strategically for the future.
Telehealth and broader digital engagement tools have enjoyed persistent patient demand throughout the pandemic. That demand may persist well after it. Investment in digital health companies has grown rapidly—reaching $21.6 billion in 2020, a 103 percent year-over-year increase—which also suggests that this approach to medicine has staying power. 9 Q4 and annual 2020 digital health (healthcare IT) funding and M&A report , Executive Summary, Digital Health Funding and M&A, Mercom Capital Group.
That level of demand offers the potential for growth when physicians can meet it. If only new entrants fully meet consumer demand, traditional providers who do not offer URL options may risk losing market share over time as a result of patients’ initial visit and downstream care decisions. What’s more, as healthcare reimbursement continues to move toward value, virtual-delivery options could become a strategic differentiator that helps providers better manage costs. 10 Brian W. Powers, MD, et al., “Association between primary care payment model and telemedicine use for Medicare Advantage enrollees during the COVID-19 pandemic,” JAMA Network , July 16, 2021.
In all likelihood, one of the critical steps in the process will be engaging physicians in the design of new virtual-care models—for example, determining clinical appropriateness, how and where physicians prefer to deliver care, and the workflows that will maximize their productivity. This has the added benefit of potentially also addressing the problem of physician burnout by offering a range of options for how and where clinicians practice.
Most important, virtual care can offer an opportunity to improve outcomes for patients meaningfully by delivering timely care to those who might otherwise delay it or who live in areas with provider shortages. In addition, patients’ most trusted advisers on care decisions are physicians, so virtual care gives them a meaningful opportunity to help patients access the care they need in a way that both parties may find convenient and appropriate. 11 “Public & physician trust in the U.S. healthcare system,” ABIM Foundation, surveys conducted on December 29, 2020 and February 5, 2021.
Physicians are evaluating a variety of factors for delivering care to patients during and, eventually, after the COVID-19 pandemic. The strategic, purposeful design of a hybrid IRL/URL healthcare delivery model offers a triple unlock: improving the value of healthcare while better meeting consumer demand and improving physicians’ engagement. The full unlock is not easy—it requires deep engagement and cooperation between administrators, clinicians, and frontline staff, as well as focused investment. But it will yield dividends for patients and providers alike in the long run.
Jenny Cordina is a partner in McKinsey’s Detroit office, Jennifer Fowkes is a partner in the Washington, DC, office, Rupal Malani, MD , is a partner in the Cleveland office, and Laura Medford-Davis, MD , is an associate partner in the Houston office.
The article was edited by Elizabeth Newman, an executive editor in the Chicago office.
Explore a career with us
Related articles.

Physicians examine options in a post-COVID-19 era

Increased workforce turnover and pressures straining provider operations

Telehealth: A quarter-trillion-dollar post-COVID-19 reality?
Thank you for visiting nature.com. You are using a browser version with limited support for CSS. To obtain the best experience, we recommend you use a more up to date browser (or turn off compatibility mode in Internet Explorer). In the meantime, to ensure continued support, we are displaying the site without styles and JavaScript.
- View all journals
- My Account Login
- Explore content
- About the journal
- Publish with us
- Sign up for alerts
- Open access
- Published: 20 April 2020
Aggregating multiple real-world data sources using a patient-centered health-data-sharing platform
- Sanket S. Dhruva ORCID: orcid.org/0000-0003-0674-2032 1 ,
- Joseph S. Ross ORCID: orcid.org/0000-0002-9218-3320 2 , 3 , 4 ,
- Joseph G. Akar 4 , 5 ,
- Brittany Caldwell 6 ,
- Karla Childers 7 ,
- Wing Chow 7 ,
- Laura Ciaccio ORCID: orcid.org/0000-0002-9961-3151 4 ,
- Paul Coplan ORCID: orcid.org/0000-0002-7078-7780 7 ,
- Jun Dong 6 ,
- Hayley J. Dykhoff ORCID: orcid.org/0000-0003-1150-3351 8 ,
- Stephen Johnston 7 ,
- Todd Kellogg 9 ,
- Cynthia Long 6 ,
- Peter A. Noseworthy 10 , 11 ,
- Kurt Roberts 12 ,
- Anindita Saha 6 ,
- Andrew Yoo 7 &
- Nilay D. Shah 8 , 11
npj Digital Medicine volume 3 , Article number: 60 ( 2020 ) Cite this article
13k Accesses
41 Citations
22 Altmetric
Metrics details
- Data acquisition
- Data integration
Real-world data sources, including electronic health records (EHRs) and personal digital device data, are increasingly available, but are often siloed and cannot be easily integrated for clinical, research, or regulatory purposes. We conducted a prospective cohort study of 60 patients undergoing bariatric surgery or catheter-based atrial fibrillation ablation at two U.S. tertiary care hospitals, testing the feasibility of using a patient-centered health-data-sharing platform to obtain and aggregate health data from multiple sources. We successfully obtained EHR data for all patients at both hospitals, as well as from ten additional health systems, which were successfully aggregated with pharmacy data obtained for patients using CVS or Walgreens pharmacies; personal digital device data from activity monitors, digital weight scales, and single-lead ECGs, and patient-reported outcome measure data obtained through surveys to assess post-procedure recovery and disease-specific symptoms. A patient-centered health-data-sharing platform successfully aggregated data from multiple sources.
Similar content being viewed by others
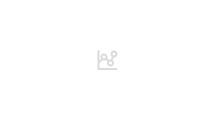
Secondary care provider attitudes towards patient generated health data from smartwatches
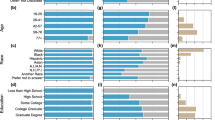
Assessment of ownership of smart devices and the acceptability of digital health data sharing
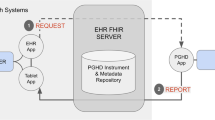
SMART Markers: collecting patient-generated health data as a standardized property of health information technology
Introduction.
Medical products, including drugs and devices, play an important role in clinical medicine, can provide substantial benefits to patients, and are regulated by the Food and Drug Administration (FDA) in the United States. In recent years, FDA increasingly moved towards a total product life cycle approach to medical product oversight, particularly for medical devices, with increasing use of evidence derived from real-world data collected in the postmarket setting as part of efforts to further evaluate medical product safety and effectiveness 1 , 2 . Real-world data used for this purpose can be derived from multiple sources, such as administrative claims, electronic health records (EHRs), disease and device registries, data gathered through personal digital devices, and patient-generated health data, such as patient-reported function or symptoms 2 , 3 , 4 ; however, aggregating data from multiple sources is often challenging.
Real-world data sources are most often siloed and represent only specific aspects of a patient’s experience. For example, one health system might have data from its EHR and some patient-generated health data, but because of lack of interoperability, is unlikely to have access to data from EHRs of other health systems where a given patient receives care. Similarly, registries extensively curate health data for patients, generally organized around patients having been diagnosed with a specific disease or receiving a specific device or procedure. But these data are typically limited to a point in time, focused on disease or device-specific data elements, and often have limited follow-up. When the Health Information Technology for Clinical and Economic Health (HITECH) Act was enacted in 2009, a key provision was for healthcare providers to provide people with digital access to their health records. Known as the Blue Button project, this effort enabled patients to access their personal health information as digital data through patient portals 5 , facilitating individual agency over their data, and has since been extended to administrative claims 6 . While portals provide patients access to their data, separate portals are often required if they receive care in different healthcare systems.
Mobile health (mHealth) technologies present an opportunity to obtain and aggregate multiple types of health data from multiple sources (e.g. EHR data from multiple healthcare systems), leveraging Blue Button technology or Application Programming Interfaces (APIs). Recent studies have shown that patients can be quickly enrolled into mHealth applications that allow them to contribute their personal digital data to research studies 7 . Additionally, mHealth technologies can enable people to answer questionnaires about their health and contribute patient-reported outcome measure (PROM) data; PROMs have been shown to correlate with digitally obtained physical activity levels 8 and clinician-assessed performance status 9 . However, to our knowledge, no study to date has aggregated multiple sources of patient health data, including personal digital device data, PROM data, EHR data, and data from pharmacies, devising an integrated approach that provides patients agency over their data while enabling pragmatic clinical research. Obtaining and rapidly aggregating these different real-world data sources for patients receiving therapeutic medical devices or procedures could help advance our understanding of medical device safety and effectiveness and provide a patient-centered approach to generating real-world evidence.
Accordingly, we conducted a study to test the feasibility of using a patient-centered health-data-sharing platform, Hugo (Hugo Health; Guilford, CT), to aggregate multiple real-world data sources as part of a prospective cohort study. Hugo facilitates patients’ access to their EHR, pharmacy, and patient-generated health data from personal digital devices (such as wearable and sensor-enabled devices) on any personal mobile device. Importantly, Hugo can aggregate data from multiple healthcare systems, wherever a patient receives care. Hugo can obtain EHR and pharmacy data in near real time, along with other data as often as it is synced. Patients can also elect to receive survey questionnaires to assess PROMs and other measures of patient status and symptom burden, responses from which are made available as soon as they are completed. Hugo allows patients to share these data with researchers through a permission-based system for research purposes. We conducted an 8-week cohort study to assess the ability of the Hugo mHealth platform to aggregate multiple sources of real-world and healthcare system data for patients undergoing two procedures that use medical devices: bariatric surgery (specifically, sleeve gastrectomy and gastric bypass) and catheter-based atrial fibrillation ablation.
Patient demographics and enrollment data
Study coordinators screened a total of 77 patients between January 26, 2018 and October 19, 2018; 11 declined enrollment, 6 could not be enrolled as they did not meet the eligibility criteria, and 60 patients (30 patients at each site and 15 patients for each procedure) consented and enrolled, of whom 59 underwent the planned procedure (one patient scheduled for bariatric surgery did not receive the procedure). The mean patient age was 55.2 years (standard deviation 13.5). Overall, 58.3% ( n = 35) of the 60 patients enrolled were female, including 76.7% ( n = 23) of the 30 bariatric surgery and 40.0% ( n = 12) of the 30 atrial fibrillation ablation patients. The median in-person enrollment time was 70 min (interquartile range (IQR), 58−100); 69 min (IQR, 46–76) for bariatric surgery patients and 73 min (IQR, 50–93) for atrial fibrillation ablation patients, with seven patients completing some enrollment steps remotely. The final patient completed follow-up on December 25, 2018.
EHR data: aggregation
EHR data were successfully aggregated from both the Yale-New Haven Hospital (YNHH) and Mayo Clinic EHRs for all patients who underwent their procedure. Among the 59 patients, 32 (54%) also received primary care services at YNHH or Mayo Clinic, in addition to the specialty care that led them to undergo either bariatric surgery or atrial fibrillation ablation at the hospital. Of the 27 patients who did not receive primary care at YNHH or Mayo Clinic, 10 (37%) used Hugo to link their EHR portals from other health systems. Of the 51 patients reached to complete close-out surveys, 18 (35%) reported receiving care at an institution other than YNHH or Mayo Clinic during the follow-up period; 6 (33%) of these 18 patients connected to outside EHR portals. In total, data from 13 EHRs were aggregated by Hugo for the 59 patients. During the study, the Hugo platform added new connections to three additional portals after patients identified receiving care at those health systems.
EHR data: validation
Among the 59 patients, there were 271 encounters identified in either the EHR data or Hugo, 221 (82%) were observed in both sources, 36 (13%) were observed only in the EHR data, and 14 (5%) were observed only in Hugo. For five patients (four at YNHH and one at Mayo Clinic), EHR data did not sync for the entire follow-up period, accounting for all 36 encounters observed in the EHR data but not in Hugo; the reasons for sync failure was that the portal account was locked after multiple incorrect password entries (by the patient, not by Hugo) and a major YNHH EHR upgrade. Among the 14 encounters observed only in Hugo, six (43%) were among YNHH patients and eight (57%) were among Mayo Clinic patients. These 14 encounters occurred at facilities or clinics within or affiliated with the health center, such that data representing these visits are included in the Continuity of Care document (CCD) and patient portal but are generally not populated within the health system EHRs because of patient privacy concerns (e.g, mental health encounters).
Of the 221 encounters observed in both the EHR data and in Hugo, the encounter date was identical for 215 (97%), the encounter type for 203 (92%), and the primary diagnosis for 204 (92%) (Table 1 ). One encounter date was missing (a mental health encounter) and one was a single day off. For encounter types with discrepancies, these were usually because the description was more specific in the EHR (e.g., “lab” or “imaging test”) than the description aggregated by Hugo (e.g., “outpatient” or “inpatient”). Two encounters were listed as “inpatient” within the EHR but “outpatient” in Hugo and one was listed as “outpatient” in the EHR but “inpatient” in Hugo. For diagnoses with discrepancies, nearly all occurred after a major YNHH Epic upgrade and were missing in Hugo; this upgrade required additional mapping from the Hugo platform to the YNHH patient portal. A single encounter diagnosis was listed as “paroxysmal atrial fibrillation” in the EHR, “cardiac arrhythmia, unspecified cardiac arrhythmia type” in Hugo.
Pharmacy data: aggregation
Among the 59 patients, 24 (41%) stated that they obtained at least some of their medications at either CVS or Walgreens pharmacies and connected their accounts to Hugo. Patients who did not use CVS or Walgreens either used Walmart, a large pharmacy that at the time of the study lacked an established API/patient portal, or smaller local pharmacies, mail order pharmacies, or grocery store pharmacies. Among the 15 patients with available CVS or Walgreens medication data, the mean number of prescription orders over the 8-week follow-up period was 4.5 (SD 4.3).
Personal digital devices: aggregation
For all 59 patients, weekly Fitbit TM syncs decreased from 47 patients who synced (80%) at week 1 to 34 patients (58%) at week 8; 64% overall for the study period (Fig. 1 ). A total of 17 bariatric surgery (59%) and 14 atrial fibrillation ablation (47%) patients synced their Fitbit TM across all 8 weeks, p = 0.85 for difference between groups. Of the 118 total Fitbit TM syncs at 1-, 4-, and 8 weeks post-procedure, step data were available for 114 (97%) and sleep data for 99 (84%). Among the 29 patients who underwent bariatric surgery, weekly Withings Body scale syncs decreased from 20 patients (69%) at week 1 to 14 patients (48%) at week 8; 56% overall for the study period. Eleven (38%) of the 29 bariatric surgery patients recorded a weight for all 8 weeks post-procedure. All 131 Withings syncs included data on patient weight. Among the 30 patients who underwent atrial fibrillation ablation, 20 (67%) synced their Kardia Mobile at both weeks 1 and 8; 70% overall for the study period, as the specific patients syncing changed over time. Fifteen (50%) of the ablation patients recorded a Kardia Mobile ECG for all 8 weeks. All 1340 Kardia Mobile syncs included data on reading duration, heart rate, and rhythm interpretation.
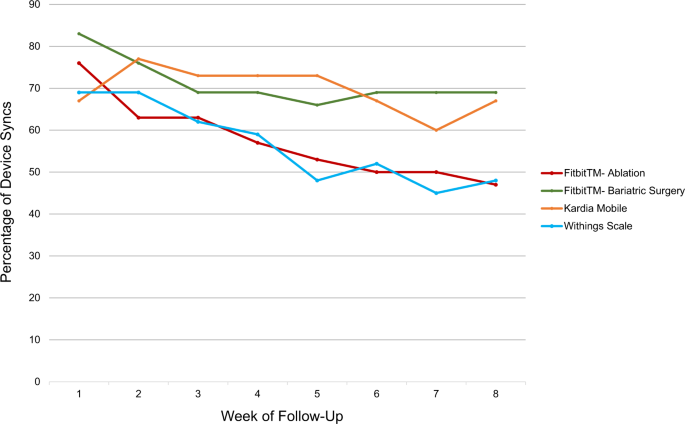
Data obtained over 8-week follow-up period for Fitbit Charge 2 TM (all patients), Kardia Mobile (ablation patients only), and Withings Body Scale (bariatric surgery patients only). Procedure occurred at week zero.
Examining weekly Fitbit TM syncs by age, 75%, 66%, and 52% of patients in the 18–44, 45–64, and 65+ age groups, respectively, synced their device, whereas for the second device (weight scale or Kardia Mobile), rates were 73%, 59%, and 61%, respectively. Examining weekly Fitbit TM syncs by sex, women completed 67% and men 60%. For the second device, these values were 58% and 69%, respectively.
Personal digital devices: patterns of recovery
Personal digital device data from patients’ Fitbits TM demonstrated that, on average, patients in both bariatric surgery and atrial fibrillation ablation groups increased their daily step counts in the first several days post-procedure and then plateaued (Figs. 2 and 3 ). Among the 22 bariatric surgery patients and 21 atrial fibrillation ablation patients with available pre-procedure data, 13 (59%) and 14 (66%), respectively, had higher post-procedure average step counts when compared to each individual’s pre-procedure step count. Personal digital device data from Withings scales demonstrated that among patients who recorded both a pre-procedure and week 8 weight, the median weight loss was 16.1 kg (IQR, 15–19) (Fig. 4 ). Data from Kardia Mobile showed that of 1340 readings by 30 patients, 834 (62%) detected normal sinus rhythm, 228 (17%) detected possible atrial fibrillation, 120 (9%) were an undetermined rhythm, and 158 (12%) had no definitive rhythm determination due to factors such as background noise or premature device disconnection. Average heart rate data indicate a small increase during the first 30 days and then a decrease (Fig. 5 ).
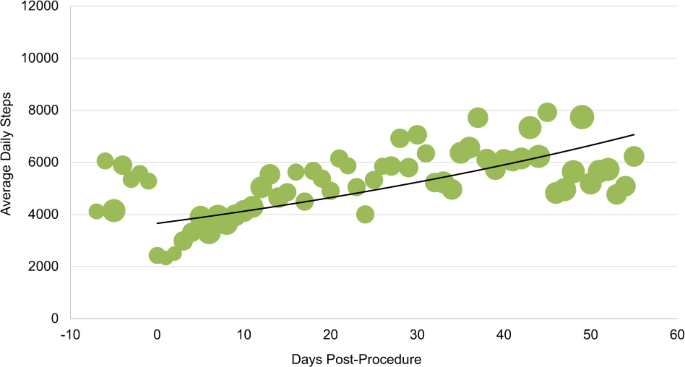
Bubble size corresponds to number of patients for whom data were synced. Procedure occurred on day 0. Negative days indicate pre-procedure data, when available. Data obtained via Fitbit Charge 2 TM .
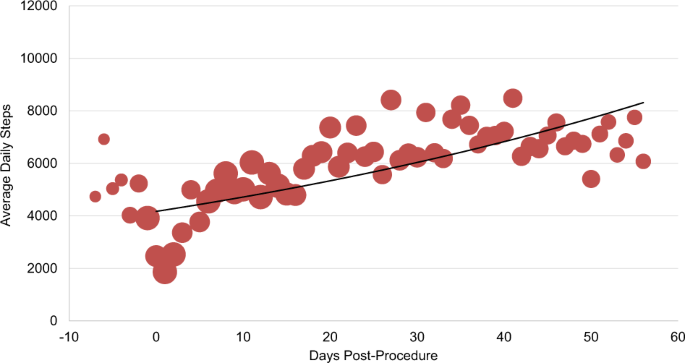
Bubble size corresponds to number of patients for whom data were synced. Procedure occurred on day 0. Data obtained via Withings Body Scale.
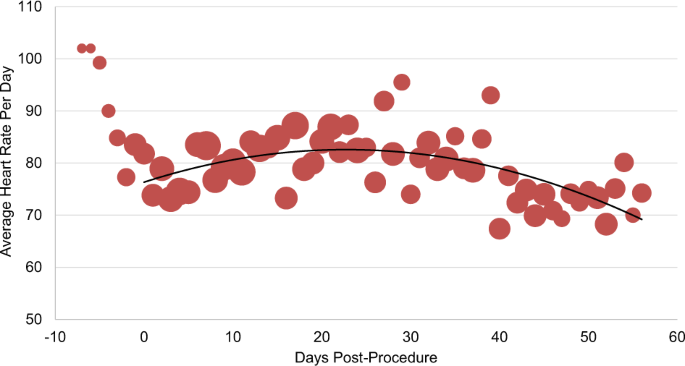
Bubble size corresponds to number of patients for whom data were synced. Procedure occurred on day 0. Negative days indicate pre-procedure data, when available. Data obtained via AliveCor Kardia Mobile.
PROMs: aggregation
Among the 59 patients, 440 of 539 (82%) post-procedure recovery PROMs were completed; 203 of 260 (78%) among the 29 bariatric surgery patients and 237 of 279 (85%) among the 30 atrial fibrillation ablation patients ( p = 0.04, Fig. 6 ). Rates of completion were 80%, 81%, and 84%, among patients in the 18–44, 45–64, and 65+ age groups, respectively, whereas women completed 83% and men 79%. On average, bariatric surgery patients completed 7.1 of 10 (71%) post-procedure recovery PROMs they received and atrial fibrillation ablation patients completed 7.7 of 10 (77%) post-procedure PROMs; 57 (97%) patients completed at least one post-procedure recovery PROM. The median time spent responding to post-procedure recovery PROMs per week was 20 s (IQR, 13–31), 14 s (IQR, 9–23) for bariatric surgery patients and 22 s (IQR, 17–41) for atrial fibrillation ablation patients.
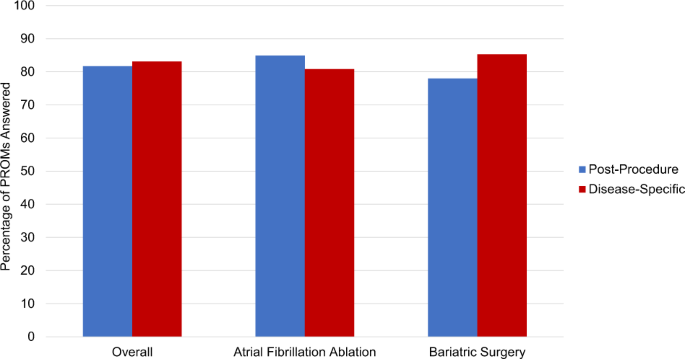
Post-procedure recovery questionnaires were obtained twice weekly for a total ten questionnaires post-procedure. Disease-specific questionnaires were obtained at 1, 4, and 8 weeks post-procedure. Data are stratified by patients who underwent atrial fibrillation ablation and bariatric surgery.
For disease-specific PROMs, 123 of 148 (83%) were completed; 64 of 75 (85%) among the bariatric surgery patients and 59 of 73 (81%) among the atrial fibrillation ablation patients ( p = 0.46, Fig. 6 ). Rates of completion were 86%, 85%, and 77%, among patients in the 18–44, 45–64, and 65+ age groups, respectively, whereas women completed 85% and men 81%. On average, bariatric surgery patients completed 3.2 of 4 (80%) disease-specific PROMs they received, atrial fibrillation ablation patients 2.7 of 4 (68%); 52 (88%) completed at least one disease-specific PROM. The median time spent responding to disease-specific PROMs was 8 min (IQR, 5–12), 5 min (4–7) for bariatric surgery patients and 11 min (8–16) for atrial fibrillation ablation patients. Of the 123 disease-specific PROMs completed, 46 (37%) were 100% complete and 118 (96%) included responses to at least 90% of the survey questions.
PROMs: patterns of recovery
PROM data reported by patients who underwent bariatric surgery demonstrated that appetite levels were initially low for the first week, and then increased and remained moderate and generally steady during the 5-week post-procedure period (Fig. 7 ) during which post-procedure recovery was assessed, whereas pain decreased over the first 1–2 weeks and then generally plateaued except for a slight increase at weeks 3–4. Similarly, PROM data reported by patients who underwent atrial fibrillation ablation demonstrated that palpitations decreased slightly over the first 2 weeks but then increased at week 3 (Fig. 8 ) and then decreased again, whereas pain levels remained low during the post-procedure period.
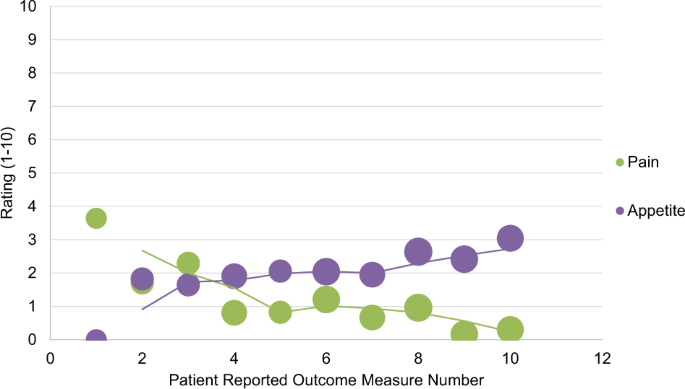
Post-procedure patient-reported outcome measure questionnaires were sent twice weekly, on Mondays and Thursdays, for a total of ten instances post-procedure. Regarding pain, patients were asked, “Do you have any pain (yes/no)?” and if they answered yes, were asked “How would you rate your pain?” (1 being mild, 10 being severe on a visual analog scale). Regarding appetite, patients were asked, “Do you have an appetite (yes/no)?” and if they answered yes, were asked “How strong is your appetite?” (1 being weak, 10 being strong on a visual analog scale). Bubble size corresponds to number of patients for whom patient-reported outcome measure questionnaire data were available.
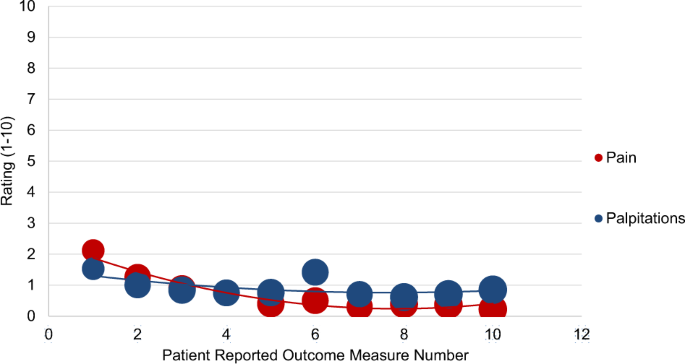
Post-procedure patient-reported outcome measure questionnaires were sent twice weekly, on Mondays and Thursdays, for a total of ten instances post-procedure. Regarding pain, patients were asked, “Do you have any pain (yes/no)?” and if they answered yes, were asked “How would you rate your pain?” (1 being mild, 10 being severe on a visual analog scale). Regarding palpitations, patients were asked, “Do you have any palpitations (yes/no)?” and if they answered yes, were asked “How would you rate your palpitations?” (1 being mild, 10 being severe, on a visual analog scale). Bubble size corresponds to number of patients for whom patient-reported outcome measure questionnaire data were available.
Our study demonstrates the feasibility of using the Hugo sync-for-science platform to obtain and aggregate patient data from multiple real-world data sources, including EHRs, pharmacies, PROMs, and personal digital devices, over an 8-week follow-up period in a near real-time, streaming fashion for patients who underwent atrial fibrillation ablation or bariatric surgery. These results suggest that there is great potential in using digital health technologies to enable aggregation of patient data across multiple sources for research and regulatory purposes. While our study was focused on procedures that use medical devices, the principles could be used for evaluating the safety and effectiveness of any medical product, as well as for better understanding patient recovery, function and symptoms after undergoing any medical procedure or surgery, behavioral intervention, or even a healthcare delivery redesign.
The FDA is increasingly using real-world evidence for medical product evaluations, including both medical devices and pharmaceuticals. The National Evaluation System for Health Technology (NEST) has been created to support this goal, with the mission of accelerating development and translation of new and safe health technologies by leveraging real-world evidence and innovative research 10 , 11 . Through NEST, a comprehensive and accurate framework can provide information about risks and benefits of devices 11 . Similarly, for pharmaceuticals, FDA recently released a Framework for its Real-World Evidence Program, pursuant to the 21st Century Cures Act, for the potential use of real-world evidence to support approval of a new indication for a drug on the market or to help support or satisfy post-approval requirements. These goals rely on obtaining and aggregating data from increasingly available real-world data sources, such as personal digital devices or digital PROMs. More importantly, it depends on removing the sequestration of data sources (including sequestration within a single source, such as EHRs lacking interoperability) and integrating them with other sources to provide a more comprehensive understanding of medical product performance and patient outcomes. Aggregation of multiple data sources allows data validation and overcomes the unreliability of a single data source 12 , 13 . Our study shows the potential to stream near real-time data during post-procedure follow-up from multiple digital sources and integrate them into a single dataset for research purposes, akin to a pragmatic clinical cohort study that required only upfront time and effort to enroll patients in the Hugo platform, but which otherwise relied on passive data collection without requiring patient visits with study personnel.
Continuous digital health technology advances are likely to help support the realization of this potential. For example, after initiation of our study, the Hugo platform made updates expected to improve response rates to PROMs and personal digital device data syncs, including text messaging reminders (this study used email reminders only) and text messaging PROMs with the goal of improving future workflows. For some patients, response rates may be higher through use of text messaging or app notifications compared to email. Data from patient APIs are also expected to increase over time, providing additional, detailed information. Further, the gradual, increasing penetration and individuals’ facility of digital technology, use of PROMs, personal digital devices, and additional sources are likely to increase patient engagement. In particular, personal digital devices, such as consumer wearables and sensors, are generating data previously unused in routine clinical care. Connecting these data to cloud-based sources will improve data aggregation and response rates, so that users will not be required to sync their devices to a connected interface to upload information. All of these advances will strengthen the potential for evaluations of patients being treated with devices, pharmaceuticals, and procedures through the use of multiple real-world data sources by improving understanding of patient recovery, function, and symptoms.
Our study is notable for its patient-centered nature: patients received access to their data and were then asked to share it with the research team to contribute to research and advance science. People continue to obtain greater access to their health data, such as through Medicare’s Blue Button 2.0, which enables over 50 million beneficiaries to obtain access to and share their claims data 10 . While there are limitations to the quantity of data made available through CCDs, health systems and pharmacies are increasingly making more health data available to patients, including clinician notes 14 ; as this occurs, patients will be able to share their own data for research purposes or use the personal health record available on their mobile device to provide additional information to their treating physicians for clinical purposes. Through aggregating multiple data sources and enabling patients to share their data, evaluations of medical products, patient status, adherence, and patient recovery can now be performed; thus far, such research has been difficult in the current limited data environment. This may be increasingly important as complex care is more frequently provided at tertiary medical centers, which may require patients to travel to obtain access to procedures. Preoperative lack of information along with postoperative loss to follow-up may bias outcomes research. One current limitation of using these data for medical device evaluations is that the unique device identifier, which allows identification of any medical device, has not been integrated into EHRs; accomplishing this integration and then providing the information to patients will strengthen the ability to study medical device safety and effectiveness 15 .
Our study should be considered in the context of important limitations. First, as this was a feasibility study, we enrolled a small number of patients (60) for a relatively short follow-up duration (8 weeks). However, there is no reason to believe that our findings would not scale to a larger number of diverse patients and for a longer follow-up duration. Further, many device-related events occur peri-procedurally and in the immediate post-procedure follow-up (particularly for atrial fibrillation ablation) 16 and, thus we would expect to capture them during this follow-up period. As no patient reported intentionally stopping data sharing to our knowledge, we expect longer follow-up durations would have similar success in obtaining data. Second, EHR data for five patients were not observable in Hugo due to sync failure after portals were locked out from multiple incorrect password entries or an EHR upgrade. This finding demonstrates the importance of “data stream checks” to ensure that data aggregation has not been interrupted; as a result, Hugo now monitors data connections and identifies those that are not functioning. Thus, sync failures due to account lockouts or EHR upgrades can be rapidly detected and addressed, which should ensure all information continues to be aggregated by Hugo. Third, we provided stipends to cover time and effort involved with study enrollment and participation and free personal digital devices, both of which may have enhanced engagement over the follow-up period. However, many patients reported being motivated to participate because they wanted to share their data with their clinicians; the opportunity to have their data integrated with clinical care is likely to be a major motivation, particularly given the association with improved outcomes 17 , 18 . Fourth, we did not examine the quality of data obtained. In contrast to clinical trials designed specifically to collect standardized, regulatory-grade data, the real-world data obtained in this study will require additional evaluation and validation to ensure that they reliably meet regulatory standards for medical product evaluations. In particular, strategies are necessary to handle missingness and artifact from personal digital devices as algorithms for step count metrics and rhythm determinations are refined over time.
In conclusion, our study demonstrated the feasibility of using a patient-centered health-data-sharing platform, Hugo, to aggregate multiple real-world data sources in near real time, thereby enabling evaluation of medical products, in this case medical devices, for both research and regulatory purposes and facilitating better understanding of patient recovery, function and symptoms.
Study population and enrollment
We enrolled a convenience sample of patients who were planning on undergoing either bariatric surgery (sleeve gastrectomy and gastric bypass) or catheter-based atrial fibrillation ablation at Yale-New Haven Hospital (YNHH) or the Mayo Clinic. We chose these two procedures because longitudinal follow-up is important to understanding the safety and effectiveness of the procedures. All patients had to be older than 18 years, English-speaking, have a compatible smartphone or tablet, and either have an email address or agree to create one.
Eligible patients were identified by their treating bariatric surgeon or cardiac electrophysiologist in advance of or following their final pre-procedural appointment. These patients were then contacted by phone and informed about the opportunity to participate in a digital health study to test the feasibility of using a mHealth application to enable integration of their data post-procedure. They were informed that this study would neither impact their peri-procedural care nor standard follow-up, as all data were collected only for research purposes to test the feasibility of the platform and were not related to the direction of their treatment. Study coordinators at both sites then met with patients to describe the study in full, obtain consent (from those who were willing), and enroll them into the mHealth platform, Hugo. Written informed consent was obtained for all patients enrolled in the study. Patients were offered a stipend to cover time and effort involved with study enrollment and participation.
Enrollment began on January 26, 2018 and continued until 15 patients were enrolled for each procedure at each site, for a total of 60 patients. Patients were followed for 8 weeks after their procedure. This study received Institutional Review Board approval at Yale University and Mayo Clinic. An IRB Authorization Agreement was signed by the FDA. This study was registered at Clinicaltrials.gov (NCT03436082) and posted on February 16, 2018.
We tested the feasibility of the Hugo platform to aggregate four different sources of data: EHRs, data from pharmacies, patient-generated health data from personal digital devices, and PROM data (Fig. 9 ).
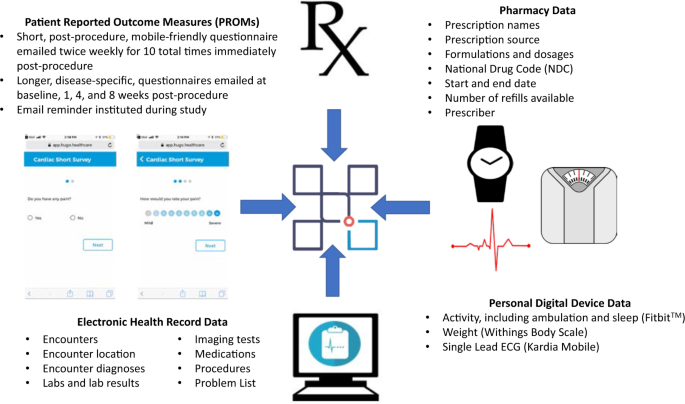
This figure demonstrates the 4 electronic health data sources aggregated in this study: patient reported outcome measures, electronic health record data, pharmacy data, and personal digital device data.
Electronic health records
Hugo aggregates EHR data by having patients link their health system patient portals. At the time of enrollment, Hugo was connected to more than 500 health systems using either an Epic or Cerner EHR system. Hugo can integrate with additional United States EHR vendors. Through this linkage, patients obtain access to their CCD from each of the health systems where they receive care. The content of CCDs varies by health system, but generally includes encounter dates, encounter types, encounter diagnoses, medications, problem list items, lab results, and imaging test results. Hugo enables patients to share the data within the CCD with researchers. The Hugo team then extracted data from the CCDs to provide a more user-friendly .csv format for our research team.
All study patients recruited from YNHH were asked to link their patient portals to the Hugo application by entering their patient portal credentials (username and password). When necessary, our study coordinators assisted patients with creating patient portal accounts. Additionally, patients were asked to link portals from any other health systems where they received care (i.e., outside of YNHH and Mayo Clinic). If a specific health system portal was unavailable within Hugo, this was documented by the study coordinator and shared with the Hugo team to determine whether that health system could be connected to the Hugo platform. Because Mayo Clinic was amidst a transition in EHR vendors during the time of the study, we did not use the standard Hugo mechanism of having patients connect their Mayo Clinic portals. Instead, for patients recruited from Mayo Clinic, every week each patient’s updated CCD as proxy EHR data was sent directly to Hugo, where it was uploaded and made available to patients through the Hugo application.
At the time our study was conducted, the only large pharmacy chains in Connecticut and Minnesota making their data available through patient portals were CVS and Walgreens. Walmart’s technical systems were in transition. Hugo aggregates pharmacy data from CVS and Walgreens by having patients link their pharmacy portal in a similar fashion as their patient portal. All patients recruited from either YNHH or Mayo Clinic who used either CVS or Walgreens as their primary pharmacy were asked to link their portals. When necessary, our study coordinators assisted patients in creating new pharmacy portal accounts. This linkage enabled Hugo to aggregate patients’ prescription medication names, dosages, instructions, national drug code (NDC), prescriber name, start date, and number of refills remaining.
Patient-generated health data: personal digital devices
Hugo connects to the public API for various personal digital devices. To collect personal digital device data over the course of the study, all patients received a Fitbit Charge 2 TM (Fitbit TM , San Francisco, California). Patients undergoing bariatric surgery also received a Withings digital weight scale (Withings, France), and patients undergoing atrial fibrillation ablation received a Kardia Mobile device (AliveCor, Mountain View, California). Study coordinators guided patients through device set-up at enrollment, including linking the devices to patients’ Hugo accounts, allowing Hugo to aggregate the information from each of the personal digital devices. After enrollment, patients received a follow-up email with user guides for the devices. Patients were asked to sync these personal digital devices at least once weekly during the 8-week post-procedure period. Beginning on July 6, 2018, approximately two-thirds through study completion, Hugo initiated a single weekly email reminder to remind all patients to utilize and sync their connected devices.
The data elements captured by a Fitbit Charge 2 TM included daily step counts and sleep duration. In some cases, physical activity, including the activity type and calories burned, was obtained automatically or entered by patients. Patients could also manually enter their height and weight. The sole data element captured by the Withings scale was weight, although patients were able to manually enter their heart rate. The data elements captured by the Kardia Mobile included reading duration, heart rate (usually averaged over 30 s), and a rhythm interpretation (atrial fibrillation, normal, or undetermined).
Patient-reported outcome measures (PROMs)
Hugo allows patients to elect to receive survey questionnaires to assess PROMs and other measures of patient status and symptom burden through emails or text messages (based on the individual’s preference) that generate a link that can be opened as a secure webpage on any device (e.g., smartphone, tablet, computer). For our study, two types of mobile-friendly PROMs were collected using email notification to all patients over the course of the study: post-procedure recovery PROMs and disease-specific PROMs. All responses were then aggregated and made available to researchers. Post-procedure recovery PROMs were emailed to patients twice weekly for a total of 5 weeks post-procedure. Bariatric surgery patients were asked two questions: (1) if they had pain (yes/no) and, if yes, to rate their pain on a scale of 1–10 and (2) if they had an appetite (yes/no) and, if yes, to rate their appetite on a scale of 1–10. Atrial fibrillation ablation patients were asked about pain, as well as whether they had palpitations and, if yes, to rate both symptoms on a scale of 1–10.
Disease-specific PROMs were emailed to patients at enrollment (pre-procedure) and 1, 4, and 8 weeks post-procedure and were tailored to patients depending on the procedure they received. For bariatric surgery patients, questions from the National Institute of Health’s (NIH) PROMIS® (Patient-Reported Outcomes Measurement Information System) relating to global health, pain, gastroesophageal reflux, nausea and vomiting, diarrhea, constipation, and sleep were adapted to a mobile-friendly format. For atrial fibrillation ablation patients, NIH PROMIS® questionnaires related to global health, dyspnea, and fatigue were adapted. Additionally, for these patients, we adapted the Cardiff Cardiac Ablation PROMS 1 and 2 to a mobile-friendly format 19 . Beginning on May 21, 2018, approximately halfway through study completion, Hugo initiated a single weekly email reminder to patients who had not completed their PROMs within 24 h.
For each PROM, the date and time that it was emailed to patients, the time it was initiated, and the time at which the final response was received were all available. Additionally, the content of response to each PROM question was obtained.
Data aggregation and validation of EHR data collected by Hugo
For each data source (EHR, pharmacy, digital device data, PROM), we determined the information made available via the Hugo platform. For EHR data, we validated the following components over 8 weeks follow-up: encounter date, encounter type, and encounter primary diagnosis. Specifically, we determined if encounters aggregated by Hugo matched encounters listed in each patient’s EHR (which were researcher-provisioned EHR views), and if there were any missing or discrepant encounters or diagnoses. We also examined reasons for discrepancies. Because of a complete change to the Mayo Clinic EHR partway through our study, our validations for Mayo Clinic patients were only performed in the new (Epic-based) EHR.
Statistical analysis
We conducted descriptive statistics with means and standard deviations or medians with interquartile range, as appropriate using Excel 2016 (Microsoft Corp., Redmond, WA). For all patients, we characterized age and sex based on EHR data. We calculated the in-person total enrollment time (from the time the study coordinator met the patient, including informed consent, set up of Hugo and other accounts, and completion of the first disease-specific PROM). While all patients received specialty care for bariatric surgery or atrial fibrillation ablation at YNHH or Mayo Clinic, we also identified those patients who also receive primary care at each health system.
For data collected via personal digital devices, we examined the frequency with which patients used and synced their personal digital devices as well as the data elements obtained from each device and also examined these data by age and sex. We considered a patient to have used a device and contributed data if there was a recording for steps or sleep for Fitbit TM , weight for the Withings Body Scale, and an ECG reading for the Kardia Mobile at least once every 7 days during 8 weeks post-procedure. For personal digital devices, we also examined changes over time in activity (Fitbit TM ), weight (Withings), and heart rate (Kardia Mobile). For patients with available pre- and post-procedure activity data, we compared each patient’s individual average pre- and post-procedure step count. For patients with available pre- and 8-week post-procedure weight data, we compared each patient’s individual average pre-procedure weight up to 7 days pre-procedure with the 8-week weight. For PROMs, we examined the completion rates, time to completion, as well as the proportion of survey items completed and completion rates by sex. For both personal digital device and post-procedure recovery PROM data, we examined trajectories in the content of data and responses over time.
Data from each personal digital device were plotted from 7 days pre-procedure (when available) up to 8 weeks (56 days) post-procedure by calculating the mean steps, weight, and heart rate per day and stratified by the patient cohort using these devices. A polynomial best fit line was fitted to the data starting from day 0. Similarly, we plotted data from post-procedure recovery PROMs, by averaging the reported pain and appetite (for bariatric surgery patients) and pain and palpitations (for atrial fibrillation ablation patients) for each of the ten surveys. For post-procedure recovery PROMs, the response score was counted as a “zero” when patients answered “no” to any of the questions.
We used Stata version 14.2 (StataCorp LP) to perform chi-square tests to compare syncing of the Fitbit TM device and response rate for the post-procedure recovery PROMs and disease-specific PROMs between patients in the bariatric surgery and atrial fibrillation ablation groups, using a p value < 0.05 to denote statistical significance.
Reporting summary
Further information on experimental design is available in the Nature Research Reporting Summary linked to this article.
Data availability
The dataset generated and analyzed for this study will not be made publicly available due to patient privacy and lack of informed consent to allow sharing of patient data outside of the research team.
Food and Drug Administration. CDRH Transparency: Total Product Life Cycle (TPLC) . (2020).
Food & Drug Administration. Use of real-world evidence to support regulatory decision-making for medical devices www.fda.gov/downloads/medicaldevices/deviceregulationandguidance/guidancedocuments/ucm513027.pdf (2017).
Sherman, R. E. et al. Real-world evidence—what is it and what can it tell us? N. Engl. J. Med. 375 , 2293–2297 (2016).
Article Google Scholar
Dhruva, S. S., Ross, J. S. & Desai, N. R. Real-world evidence: promise and peril for medical product evaluation. P. T. 43 , 464–472 (2018).
PubMed PubMed Central Google Scholar
Mohsen, M. O. & Aziz, H. A. The Blue Button Project: engaging patients in healthcare by a click of a button. Perspect. Health Inf. Manag. 12 , 1d (2015).
Centers for Medicare & Medicaid Services. Blue Button 2.0 https://bluebutton.cms.gov/ (2020).
McConnell, M. V. et al. Feasibility of obtaining measures of lifestyle from a smartphone app: the MyHeart Counts Cardiovascular Health Study. JAMA Cardiol. 2 , 67–76 (2017).
Speier, W. et al. Evaluating utility and compliance in a patient-based eHealth study using continuous-time heart rate and activity trackers. J. Am. Med. Inf. Assoc. 25 , 1386–1391 (2018).
Gresham, G. et al. Wearable activity monitors to assess performance status and predict clinical outcomes in advanced cancer patients. npj Digit. Med. https://doi.org/10.1038/s41746-018-0032-6 (2018).
NEST Coordinating Center. Strategic & Operational Planning: 2017−2022 https://nestcc.org/wp-content/uploads/2018/10/NESTcc-Strategic-and-Operational-Plan-September-2018.pdf (2018).
Shuren, J. & Califf, R. M. Need for a national evaluation system for health technology. JAMA 316 , 1153–1154 (2016).
Guimaraes, P. O. et al. Accuracy of medical claims for identifying cardiovascular and bleeding events after myocardial infarction: a secondary analysis of the TRANSLATE-ACS Study. JAMA Cardiol. 2 , 750–757 (2017).
Yasaitis, L. C., Berkman, L. F. & Chandra, A. Comparison of self-reported and Medicare claims-identified acute myocardial infarction. Circulation 131 , 1477–1485 (2015).
Delbanco, T. et al. Open notes: doctors and patients signing on. Ann. Intern. Med. 153 , 121–125 (2010).
Dhruva, S. S., Ross, J. S., Schulz, W. L. & Krumholz, H. M. Fulfilling the promise of unique device identifiers. Ann. Intern. Med. 169 , 183–185 (2018).
Abdur Rehman, K. et al. Life-threatening complications of atrial fibrillation ablation: 16-year experience in a large prospective tertiary care cohort. JACC Clin. Electrophysiol. 5 , 284–291 (2019).
Basch, E. Patient-reported outcomes—harnessing patients’ voices to improve clinical care. N. Engl. J. Med. 376 , 105–108 (2017).
Basch, E. et al. Overall survival results of a trial assessing patient-reported outcomes for symptom monitoring during routine cancer treatment. JAMA 318 , 197–198 (2017).
White, J. et al. Cardiff cardiac ablation patient-reported outcome measure (C-CAP): validation of a new questionnaire set for patients undergoing catheter ablation for cardiac arrhythmias in the UK. Qual. Life Res. 25 , 1571–1583 (2016).
Download references
Acknowledgements
This work was supported in part by a Center of Excellence in Regulatory Science and Innovation (CERSI) grant to Yale University and Mayo Clinic from the US Food & Drug Administration (U01FD005938) and by Johnson & Johnson. Its contents are solely the responsibility of the authors and do not necessarily represent the official views nor the endorsements of the Department of Health and Human Services, FDA, or Johnson & Johnson. Hugo Health, LLC is a private, for-profit company that developed the patient-centered data acquisition and integration platform used in this study. Neither Yale University nor the authors have any ownership interest nor receive compensation from Hugo Health. Fees were paid to support the use of the platform in the study. We gratefully acknowledge the National Evaluation System for Health Technology (NEST) designation of this work as a Real World Evidence Demonstration Project and the Hugo team for their assistance throughout this project. We acknowledge AliveCor for their generous donation of the Kardia Mobile devices used in this study. Most importantly, we gratefully acknowledge the contributions of patients to this study.
Author information
Authors and affiliations.
University of California, San Francisco School of Medicine, San Francisco, CA, USA
- Sanket S. Dhruva
Section of General Internal Medicine and the National Clinician Scholars Program, Yale School of Medicine, New Haven, CT, USA
Joseph S. Ross
Department of Health Policy and Management, Yale School of Public Health, New Haven, CT, USA
Center for Outcomes Research and Evaluation, Yale-New Haven Hospital, New Haven, CT, USA
Joseph S. Ross, Joseph G. Akar & Laura Ciaccio
Department of Internal Medicine, Cardiovascular Medicine, Yale School of Medicine, New Haven, CT, USA
Joseph G. Akar
Center for Devices and Radiological Health, U.S. Food and Drug Administration, White Oak, MD, USA
Brittany Caldwell, Jun Dong, Cynthia Long & Anindita Saha
Johnson & Johnson, New Brunswick, NJ, USA
Karla Childers, Wing Chow, Paul Coplan, Stephen Johnston & Andrew Yoo
Division of Health Care Policy and Research, Department of Health Sciences Research, Mayo Clinic, Rochester, MN, USA
Hayley J. Dykhoff & Nilay D. Shah
Division of Gastroenterologic and General Surgery, Mayo Clinic, Rochester, MN, USA
Todd Kellogg
Department of Cardiovascular Medicine, Mayo Clinic, Rochester, MN, USA
Peter A. Noseworthy
Robert D. and Patricia E. Kern Center for the Science of Health Care Delivery, Mayo Clinic, Rochester, MN, USA
Peter A. Noseworthy & Nilay D. Shah
Section of Gastrointestinal Surgery, Yale University School of Medicine, New Haven, CT, USA
Kurt Roberts
You can also search for this author in PubMed Google Scholar
Contributions
S.S.D., J.S.R., and N.D.S. contributed to the conceptualization and design of the study. J.G.A., B.C., K.C., W.C., L.C., H.J.D., J.D., S.J., T.K., C.L., P.A.N., K.R., A.S., A.Y., and N.D.S. contributed to the development of the study protocol. J.G.A., T.K., P.A.N., K.R., and cardiac electrophysiologists and bariatric surgeons at Mayo Clinic identified patients for enrollment. L.C. and H.J.D. oversaw patient recruitment and performed the data validation and analysis. All authors contributed to data interpretation. L.C. created the data visualization, figures, and table and L.C. and S.S.D. performed descriptive statistical analyses. S.S.D., J.S.R., L.C., H.J.D., and N.D.S. wrote the manuscript. All authors critically reviewed the manuscript.
Corresponding author
Correspondence to Joseph S. Ross .
Ethics declarations
Competing interests.
S.S.D. currently receives research support through the National Institute of Health (K12HL138046) and The Greenwall Foundation. J.S.R. formerly received research support through Yale University from Medtronic, Inc. and the Food and Drug Administration (FDA) to develop methods for postmarket surveillance of medical devices (U01FD004585), from the Centers of Medicare and Medicaid Services (CMS) to develop and maintain performance measures that are used for public reporting (HHSM-500-2013-13018I), and from the Blue Cross Blue Shield Association to better understand medical technology evaluation; J.S.R. currently receives research support through Yale University from Johnson and Johnson to develop methods of clinical trial data sharing, from the Medical Device Innovation Consortium as part of the National Evaluation System for Health Technology (NEST), from the Agency for Healthcare Research and Quality (R01HS022882), from the National Heart, Lung and Blood Institute of the National Institutes of Health (NIH) (R01HS025164), and from the Laura and John Arnold Foundation to establish the Good Pharma Scorecard at Bioethics International and to establish the Collaboration for Research Integrity and Transparency (CRIT) at Yale. In the past 36 months, N.D.S. has received research from the Centers of Medicare and Medicaid Innovation under the Transforming Clinical Practice Initiative (TCPI), from the Agency for Healthcare Research and Quality (R01HS025164; R01HS025402; 1U19HS024075; R03HS025517), from the National Heart, Lung and Blood Institute of the National Institutes of Health (NIH) (R56HL130496; R01HL131535), National Science Foundation, and from the Patient-Centered Outcomes Research Institute (PCORI) to develop a Clinical Data Research Network (LHSNet). P.C., A.Y., S.J., and K.C. are employees and stockholders of Johnson & Johnson; W.C. is an employee of Janssen Scientific Affairs, LLC, and a stockholder of Johnson & Johnson.
Additional information
Publisher’s note Springer Nature remains neutral with regard to jurisdictional claims in published maps and institutional affiliations.
Supplementary information
Reporting summary, rights and permissions.
Open Access This article is licensed under a Creative Commons Attribution 4.0 International License, which permits use, sharing, adaptation, distribution and reproduction in any medium or format, as long as you give appropriate credit to the original author(s) and the source, provide a link to the Creative Commons license, and indicate if changes were made. The images or other third party material in this article are included in the article’s Creative Commons license, unless indicated otherwise in a credit line to the material. If material is not included in the article’s Creative Commons license and your intended use is not permitted by statutory regulation or exceeds the permitted use, you will need to obtain permission directly from the copyright holder. To view a copy of this license, visit http://creativecommons.org/licenses/by/4.0/ .
Reprints and permissions
About this article
Cite this article.
Dhruva, S.S., Ross, J.S., Akar, J.G. et al. Aggregating multiple real-world data sources using a patient-centered health-data-sharing platform. npj Digit. Med. 3 , 60 (2020). https://doi.org/10.1038/s41746-020-0265-z
Download citation
Received : 07 November 2019
Accepted : 23 March 2020
Published : 20 April 2020
DOI : https://doi.org/10.1038/s41746-020-0265-z
Share this article
Anyone you share the following link with will be able to read this content:
Sorry, a shareable link is not currently available for this article.
Provided by the Springer Nature SharedIt content-sharing initiative
This article is cited by
Trauma systems in high socioeconomic index countries in 2050.
- Tobias Gauss
- Mariska de Jongh
- Pierre Bouzat
Critical Care (2024)
Characterization of multi-domain postoperative recovery trajectories after cardiac surgery using a digital platform
- Makoto Mori
- Harlan M. Krumholz
npj Digital Medicine (2022)
A Framework for Extension Studies Using Real-World Data to Examine Long-Term Safety and Effectiveness
- Mehmet Burcu
- Cyntia B. Manzano-Salgado
- Jennifer B. Christian
Therapeutic Innovation & Regulatory Science (2022)
Addressing misalignments to improve the US health care system by integrating patient-centred care, patient-centred real-world data, and knowledge-sharing: a review and approaches to system alignment
- Douglas S. Levine
- Douglas A. Drossman
Discover Health Systems (2022)
Feasibility and acceptability of electronic administration of patient reported outcomes using mHealth platform in emergency department patients with non-medical opioid use
- Kathryn Hawk
- Caitlin Malicki
- Arjun Venkatesh
Addiction Science & Clinical Practice (2021)
Quick links
- Explore articles by subject
- Guide to authors
- Editorial policies
Sign up for the Nature Briefing newsletter — what matters in science, free to your inbox daily.

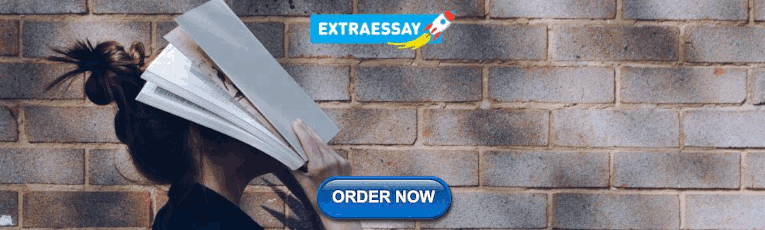
IMAGES
VIDEO
COMMENTS
In clinical research, the identification of patients to be included will be ad hoc, based on the specific research protocol, ... Additionally, access to private medical information by law enforcement agencies could be a risk for individuals and society. However, the current legal restriction on the joint use of health data imposed by the GDPR ...
Precision health calls for collecting and analyzing large amounts of data to capture an individual's unique behavior, lifestyle, genetics, and environmental context. The diffusion of digital tools has led to a significant growth of patient generated health data (PGHD), defined as health-related data created, gathered or inferred by or from ...
A Data Use Agreement (DUA) establishes the terms under which data may be used by a third party collaborating on research involving patient data. The School of Medicine Office of Research Administration (ORA) negotiates and executes DUAs and other research agreements with data use terms for JHM PIs when research involves JHM patients or their ...
Medical records can be tricky to access because of confidentiality and variability, but data-sharing efforts are helping to overcome these hurdles — without compromising patient privacy.
Background Machine learning (ML) has made a significant impact in medicine and cancer research; however, its impact in these areas has been undeniably slower and more limited than in other application domains. A major reason for this has been the lack of availability of patient data to the broader ML research community, in large part due to patient privacy protection concerns. High-quality ...
The SARS-CoV-2 pandemic has highlighted once more the great need for comprehensive access to, and uncomplicated use of, pre-existing patient data for medical research. Enabling secondary research-use of patient-data is a prerequisite for the efficient and sustainable promotion of translation and personalisation in medicine, and for the advancement of public-health.
Szarfman et al. discuss the importance of efficient, easy access to large quantities of health data to improve medical care and further medical research. They outline the issues currently ...
Hypocrisy Around Medical Patient Data: Issues of Access for Biomedical Research, Data Quality, Usefulness for the Purpose and Omics Data as Game Changer. Asian Bioethics Review 11 , 189-207 (2019).
The use and sharing of PGHD in care delivery and research can: Gather important information about how patients are doing between medical visits. Provide information for use in shared decision-making about preventive and chronic care management. Offer potential cost savings and improvements in quality, care coordination, and patient safety.
The first is the aforementioned move from a pay-for-service model, which financially rewards caregivers for performing procedures, to a value-based care model, which rewards them based on the health of their patient populations. Healthcare data analytics will enable the measurement and tracking of population health, thereby enabling this switch.
Clinical data is a staple resource for most health and medical research. Clinical data is either collected during the course of ongoing patient care or as part of a formal clinical trial program. Clinical data falls into six major types: Electronic health records; Administrative data; Claims data; Patient / Disease registries; Health surveys
Two recent publications demonstrate how data science in medical research has the potential to accelerate a national study of breast cancer detection methods and better understand COVID-19 breakthrough infection risk on-pace with the virus. ... The work was published in The American Journal of the Medical Sciences. Because patient data is ...
Jun 23, 2021, 11:22 AM PDT. Illustration by Ana Kova. Healthcare organizations and hospitals in the United States all sit on treasure troves: a stockpile of patient health data stored as ...
OpenfMRI: Other imaging data sets from MRI machines to foster research, better diagnostics, and training. It includes 95 datasets from 3372 subjects with new material being added as researchers make their own data open to the public. CT Medical Images: This one is a small dataset, but it's specifically cancer-related. It contains labeled ...
Biostatistics staff support every step of a research study, starting with the research question. They advise on protocols and study designs. They build, curate, clean and analyze datasets. They report results, co-author papers and respond to statistical review comments. The division has multiyear federal grants and serves as the statistics and ...
Background. The mission of health care institutions - restoring patient's health - demands effective and efficient medical data for evidence-based intervention. 1 Installing an appropriate health care data management system with valid case definition enables efficient data extraction, 2 improves communication for clinical decision making in medical practice, 2 - 8 and clinical research ...
data from IQVIA Medical Research Data. The detailed analysis conducted in real world data demonstrated: • Adjusted linear regression models compared the difference in mean change in HbA1c, BMI, and SBP after 12-months follow-up • The proportion of patients achieving HbA1c targets (<6.5%, <7.0%, <7.5%); HbA1c reduction >1%; and
Background Research using data from medical care promises to advance medical science and improve healthcare. Academia is not the only sector that expects such research to be of great benefit. The research-based health industry is also interested in so-called 'real-world' health data to develop new drugs, medical technologies or data-based health applications. While access to medical data ...
ChatGPT, the artificial intelligence (AI) chatbot designed to assist with language-based tasks, can effectively extract data for research purposes from physicians' clinical notes, UT Southwestern ...
Recent studies show that Medical Data Science (MDS) carries great potential to improve healthcare 1, 2, 3. Thereby, considering data from several medical areas and of different types, i.e. using ...
AI can help providers keep track of patient data more efficiently. One example is diabetes. According to the Centers for Disease Control and Prevention, 10% of the US population has diabetes. Patients can now use wearable and other monitoring devices that provide feedback about their glucose levels to themselves and their medical team.
The full-time faculty of more than 3,100 is responsible for groundbreaking medical advances and is committed to translating science-driven research quickly to new clinical treatments. UT Southwestern physicians provide care in more than 80 specialties to more than 120,000 hospitalized patients, more than 360,000 emergency room cases, and ...
Background: Patients with advanced cancer undergoing chemotherapy experience significant symptoms and declines in functional status, which are associated with poor outcomes. Remote monitoring of patient-reported outcomes (PROs; symptoms) and step counts (functional status) may proactively identify patients at risk of hospitalization or death.
The study found that in fall-winter 2023-2024, the risk of death in patients hospitalized for COVID-19 was greater than the risk of death in patients hospitalized for seasonal influenza. Compared with a study using the same database and methods, 3 the death rate at 30 days was 5.97% in 2022-2023 vs 5.70% in 2023-2024 for COVID-19 and 3.75% in ...
Location: Madison, Wisconsin. Department: SCHOOL OF MEDICINE AND PUBLIC HEALTH/DEPARTMENT OF MEDICINE. Category: Research. Employment Type: Partially Remote. Employment Type: Staff-Full Time. Employment Type: Staff-Part Time. Application Period Opens: May 15 2024 at 4:50 PM CDT. Apply By: May 29 2024 at 11:55 PM CDT. Job Number: 295718-AS.
Variables, variability, and variations research: implications for medical informatics. J Am Med Inform Assoc . 1995;2(3):183-190. doi: 10.1136/jamia.1995.95338871 PubMed Google Scholar Cross
To help our clients understand responses to COVID-19, McKinsey launched a research effort to gather insights from physicians into how the pandemic is affecting their ability to provide care, their financial situation, and their level of stress, as well as what kind of support would interest them. Nationwide surveys were conducted online in 2020 from April 27-May 5 (538 respondents), July 22 ...
Welcome! You can use this tool to find and compare different types of Medicare providers (like physicians, hospitals, nursing homes, and others). Use our maps and filters to help you identify providers that are right for you. Find Medicare-approved providers near you & compare care quality for nursing homes, doctors, hospitals, hospice centers ...
Patient demographics and enrollment data. Study coordinators screened a total of 77 patients between January 26, 2018 and October 19, 2018; 11 declined enrollment, 6 could not be enrolled as they ...